Best AI tools for< Evaluate Models >
11 - AI tool Sites
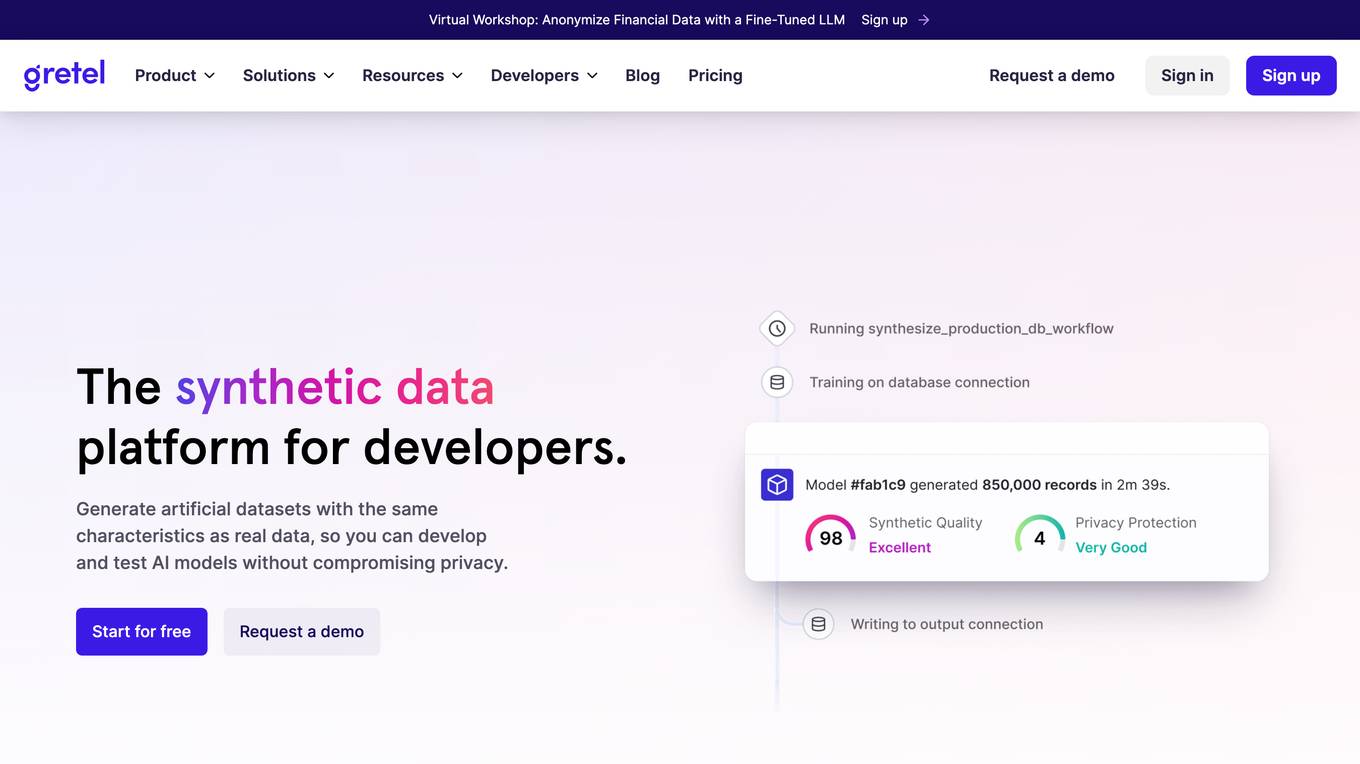
Gretel.ai
Gretel.ai is a synthetic data platform purpose-built for AI applications. It allows users to generate artificial, synthetic datasets with the same characteristics as real data, enabling the improvement of AI models without compromising privacy. The platform offers features such as generating data from input prompts, creating safe synthetic versions of sensitive datasets, flexible data transformation, building data pipelines, and measuring data quality. Gretel.ai is designed to help developers unlock synthetic data and achieve more with safe access to the right data.
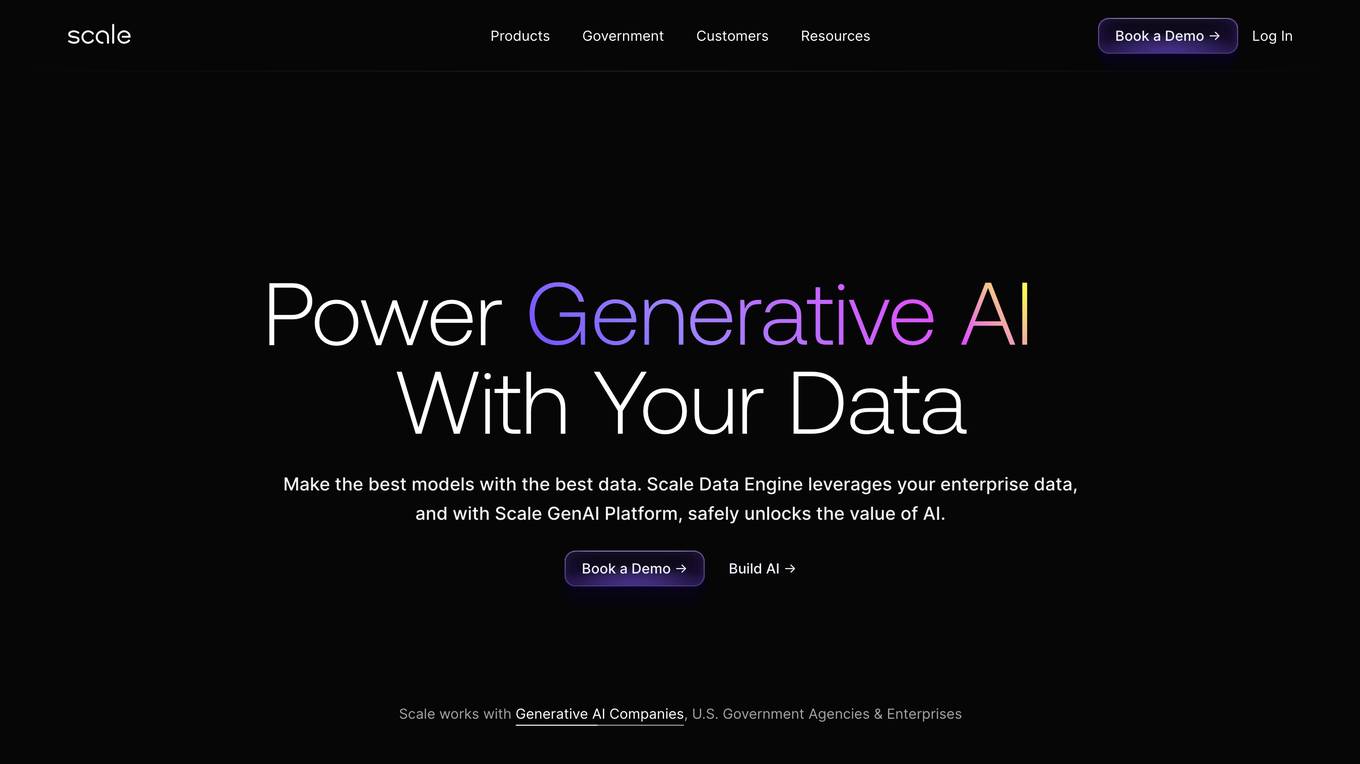
Scale AI
Scale AI is an AI tool that accelerates the development of AI applications for enterprise, government, and automotive sectors. It offers Scale Data Engine for generative AI, Scale GenAI Platform, and evaluation services for model developers. The platform leverages enterprise data to build sustainable AI programs and partners with leading AI models. Scale's focus on generative AI applications, data labeling, and model evaluation sets it apart in the AI industry.
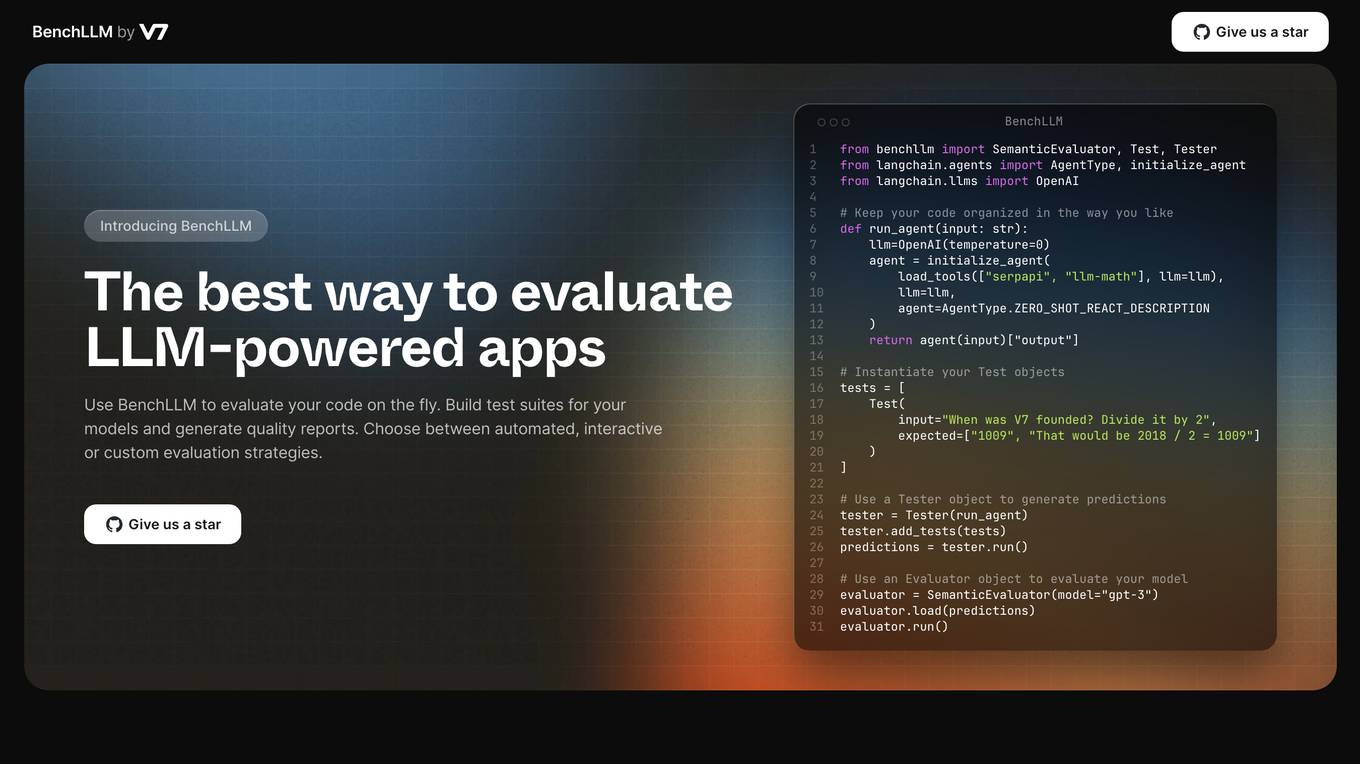
BenchLLM
BenchLLM is an AI tool designed for AI engineers to evaluate LLM-powered apps by running and evaluating models with a powerful CLI. It allows users to build test suites, choose evaluation strategies, and generate quality reports. The tool supports OpenAI, Langchain, and other APIs out of the box, offering automation, visualization of reports, and monitoring of model performance.
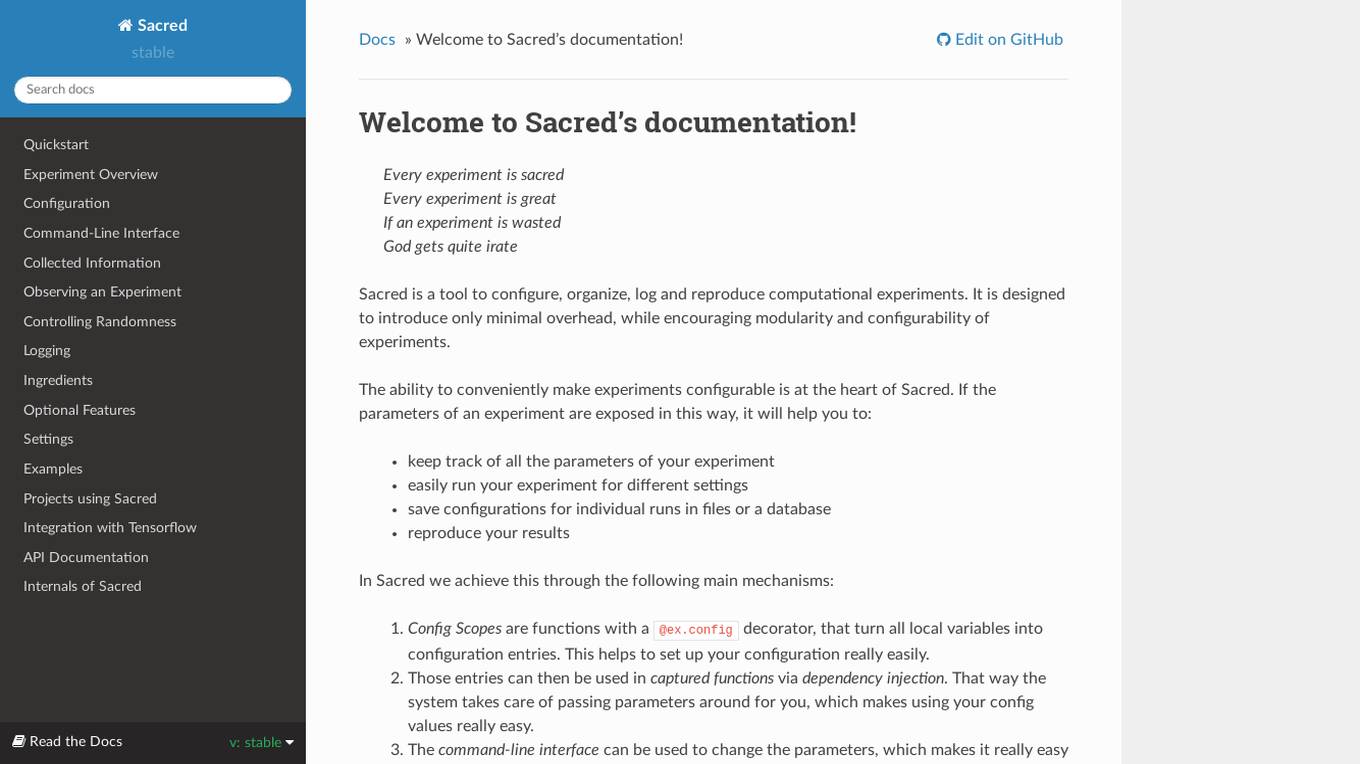
Sacred
Sacred is a tool to configure, organize, log and reproduce computational experiments. It is designed to introduce only minimal overhead, while encouraging modularity and configurability of experiments. The ability to conveniently make experiments configurable is at the heart of Sacred. If the parameters of an experiment are exposed in this way, it will help you to: keep track of all the parameters of your experiment easily run your experiment for different settings save configurations for individual runs in files or a database reproduce your results In Sacred we achieve this through the following main mechanisms: Config Scopes are functions with a @ex.config decorator, that turn all local variables into configuration entries. This helps to set up your configuration really easily. Those entries can then be used in captured functions via dependency injection. That way the system takes care of passing parameters around for you, which makes using your config values really easy. The command-line interface can be used to change the parameters, which makes it really easy to run your experiment with modified parameters. Observers log every information about your experiment and the configuration you used, and saves them for example to a Database. This helps to keep track of all your experiments. Automatic seeding helps controlling the randomness in your experiments, such that they stay reproducible.
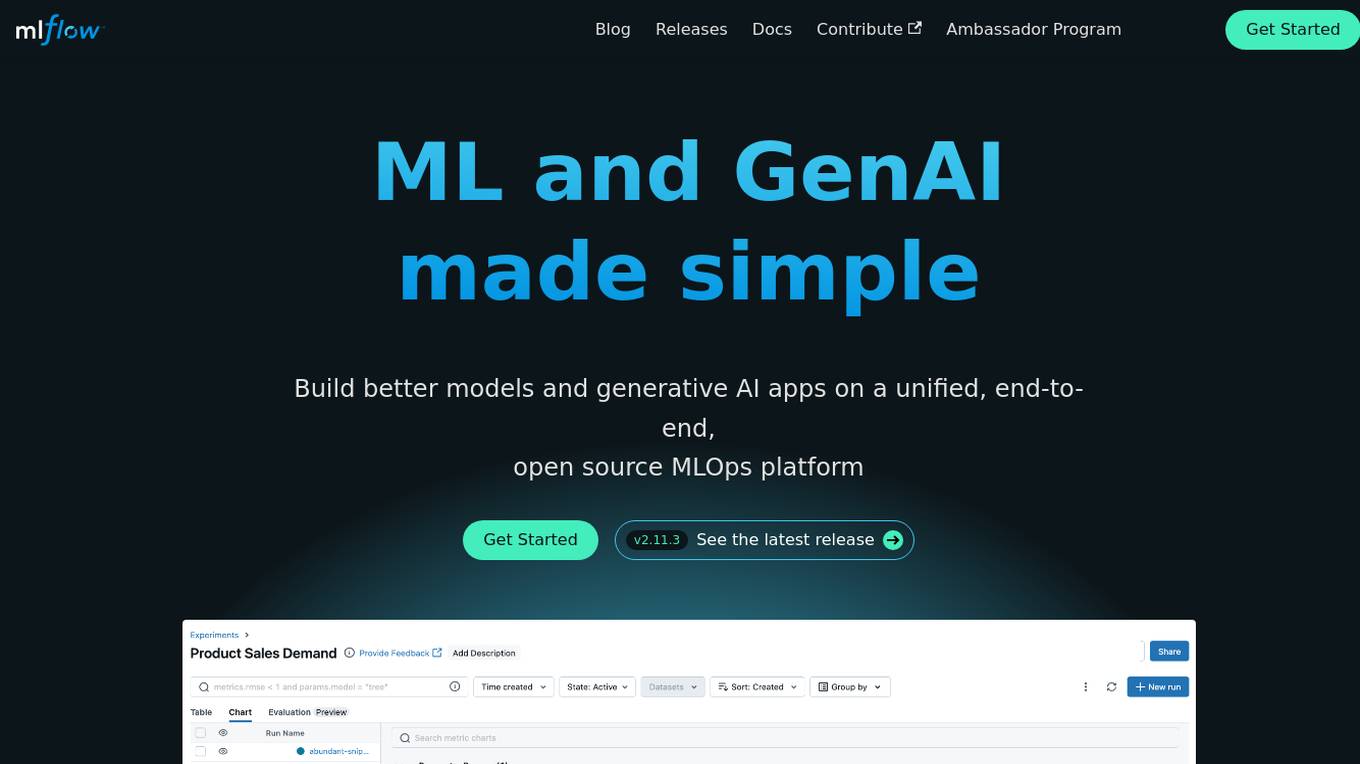
MLflow
MLflow is an open source platform for managing the end-to-end machine learning (ML) lifecycle, including tracking experiments, packaging models, deploying models, and managing model registries. It provides a unified platform for both traditional ML and generative AI applications.
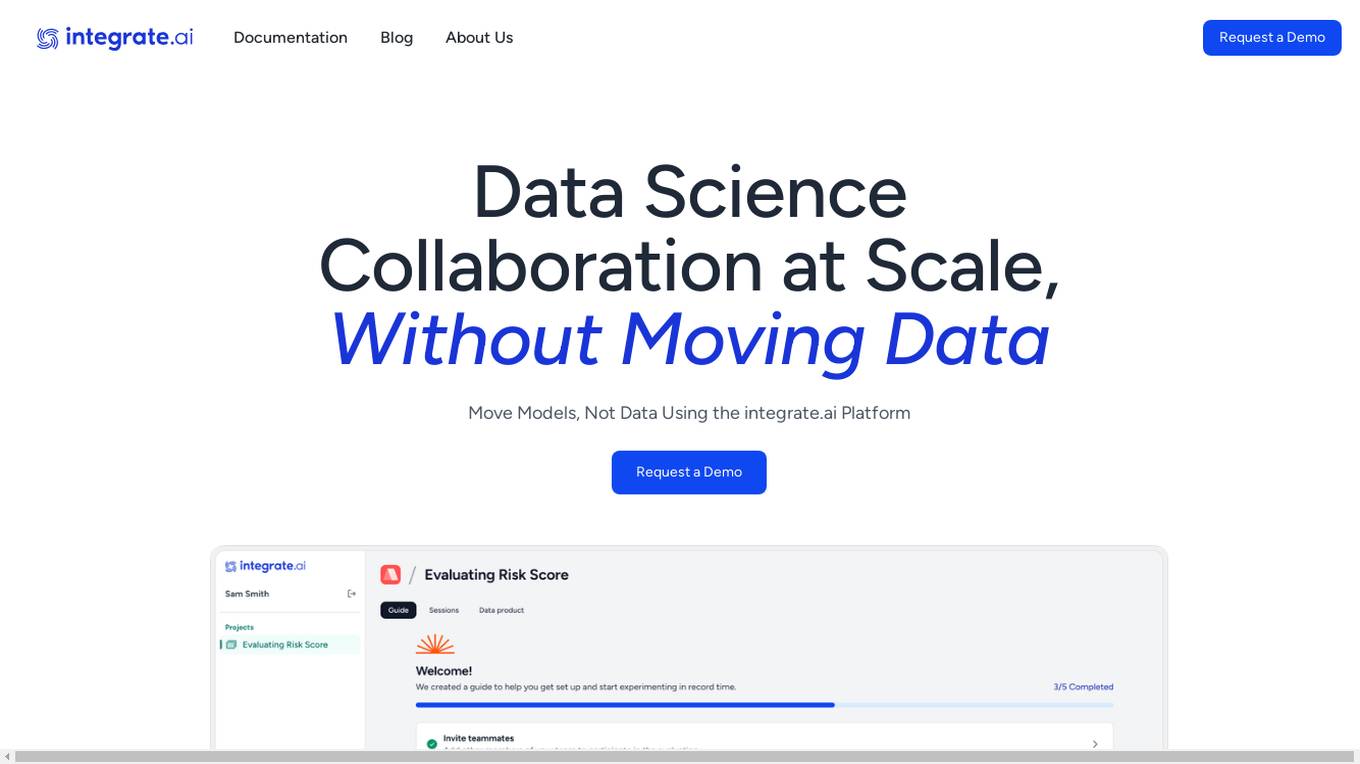
integrate.ai
integrate.ai is a platform that enables data and analytics providers to collaborate easily with enterprise data science teams without moving data. Powered by federated learning technology, the platform allows for efficient proof of concepts, data experimentation, infrastructure agnostic evaluations, collaborative data evaluations, and data governance controls. It supports various data science jobs such as match rate analysis, exploratory data analysis, correlation analysis, model performance analysis, feature importance & data influence, and model validation. The platform integrates with popular data science tools like Azure, Jupyter, Databricks, AWS, GCP, Snowflake, Pandas, PyTorch, MLflow, and scikit-learn.
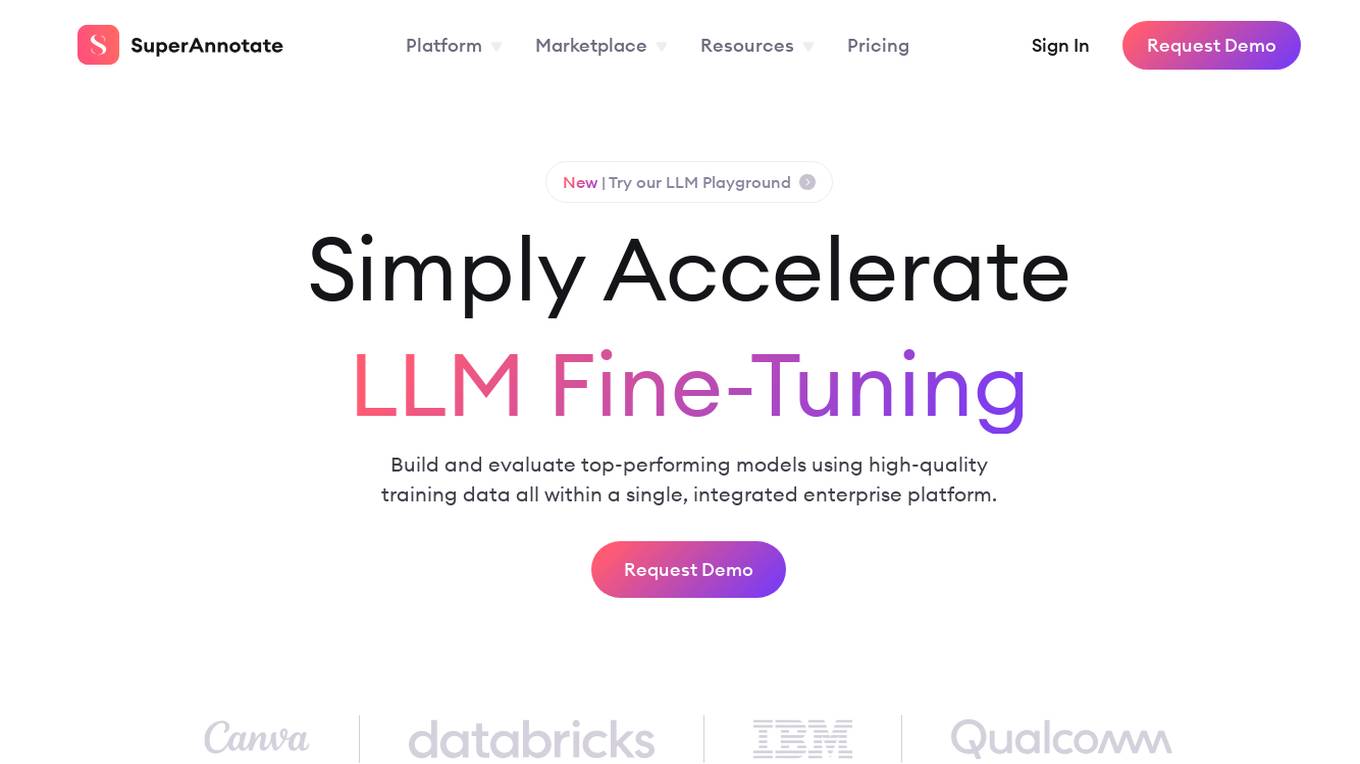
SuperAnnotate
SuperAnnotate is an AI data platform that simplifies and accelerates model-building by unifying the AI pipeline. It enables users to create, curate, and evaluate datasets efficiently, leading to the development of better models faster. The platform offers features like connecting any data source, building customizable UIs, creating high-quality datasets, evaluating models, and deploying models seamlessly. SuperAnnotate ensures global security and privacy measures for data protection.
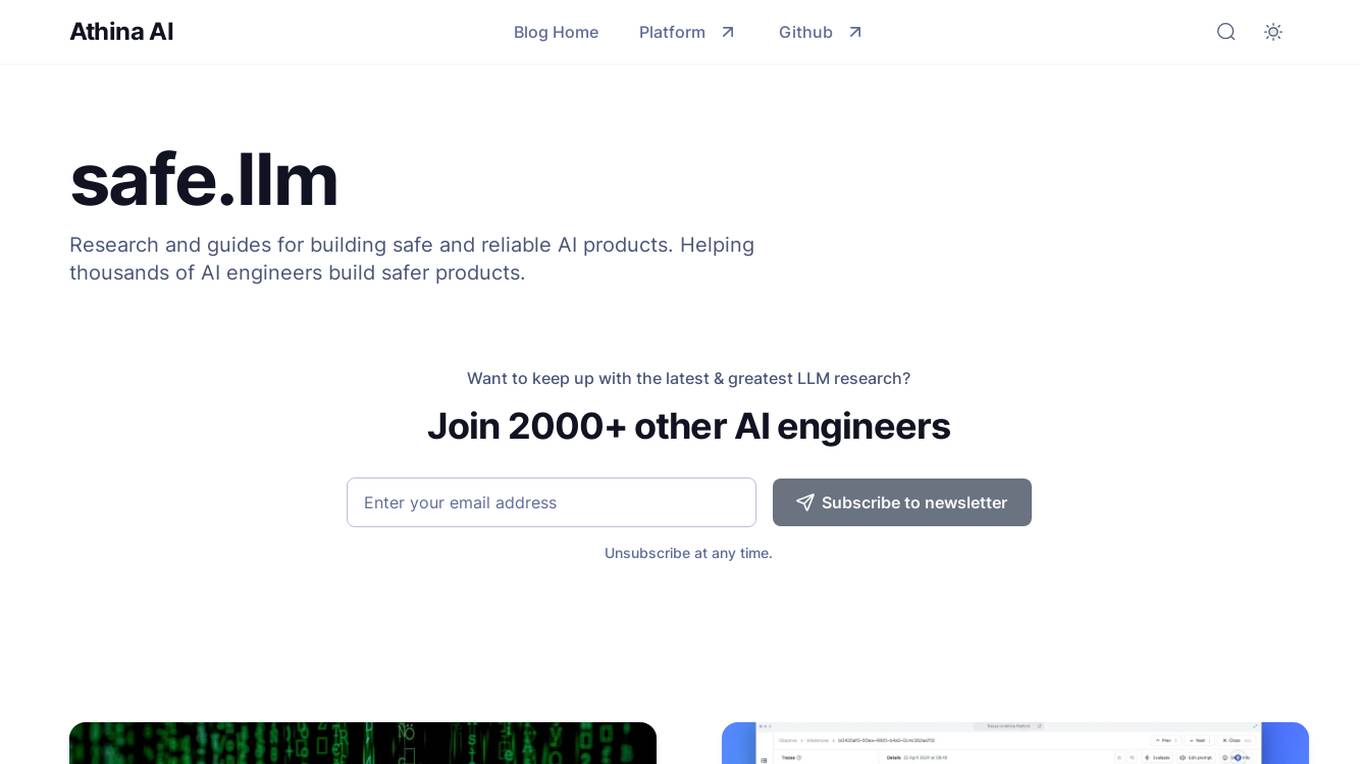
Athina AI
Athina AI is a platform that provides research and guides for building safe and reliable AI products. It helps thousands of AI engineers in building safer products by offering tutorials, research papers, and evaluation techniques related to large language models. The platform focuses on safety, prompt engineering, hallucinations, and evaluation of AI models.
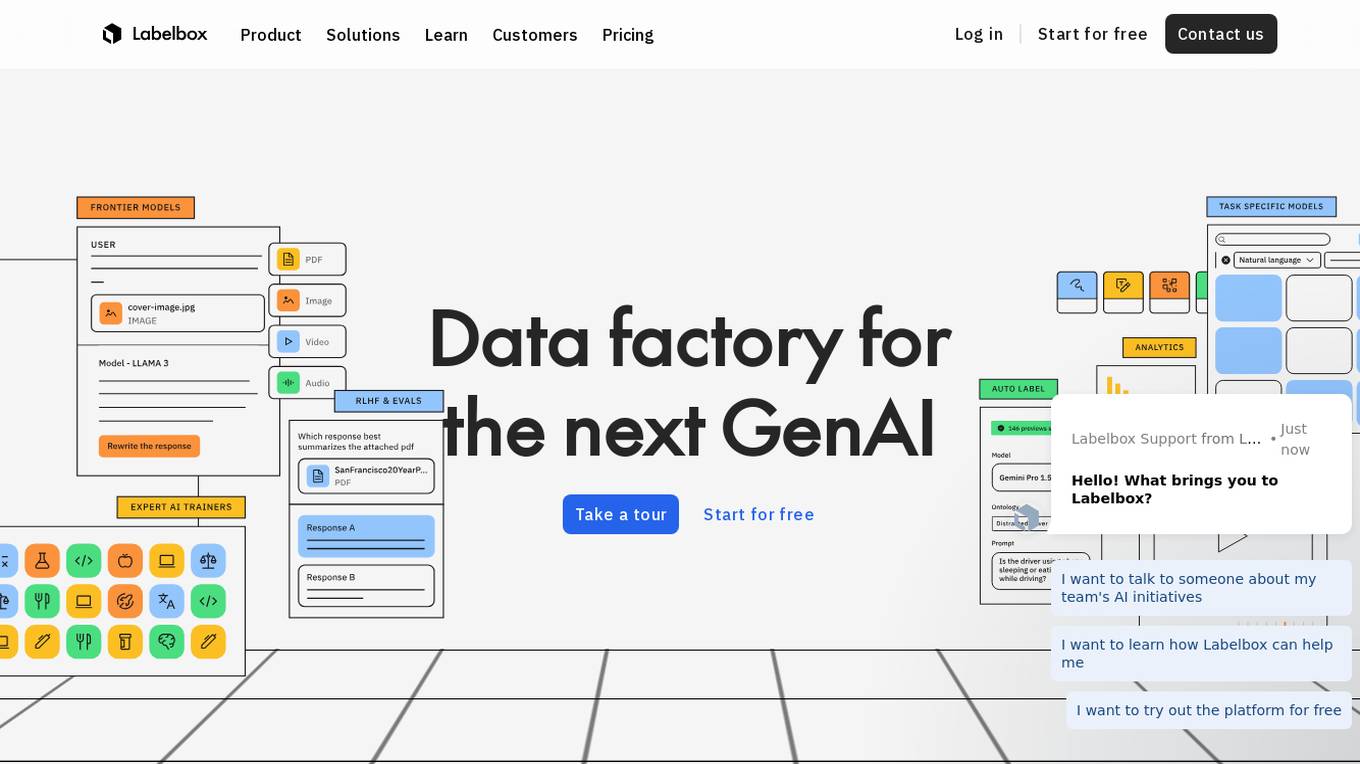
Labelbox
Labelbox is a data factory platform that empowers AI teams to manage data labeling, train models, and create better data with internet scale RLHF platform. It offers an all-in-one solution comprising tooling and services powered by a global community of domain experts. Labelbox operates a global data labeling infrastructure and operations for AI workloads, providing expert human network for data labeling in various domains. The platform also includes AI-assisted alignment for maximum efficiency, data curation, model training, and labeling services. Customers achieve breakthroughs with high-quality data through Labelbox.
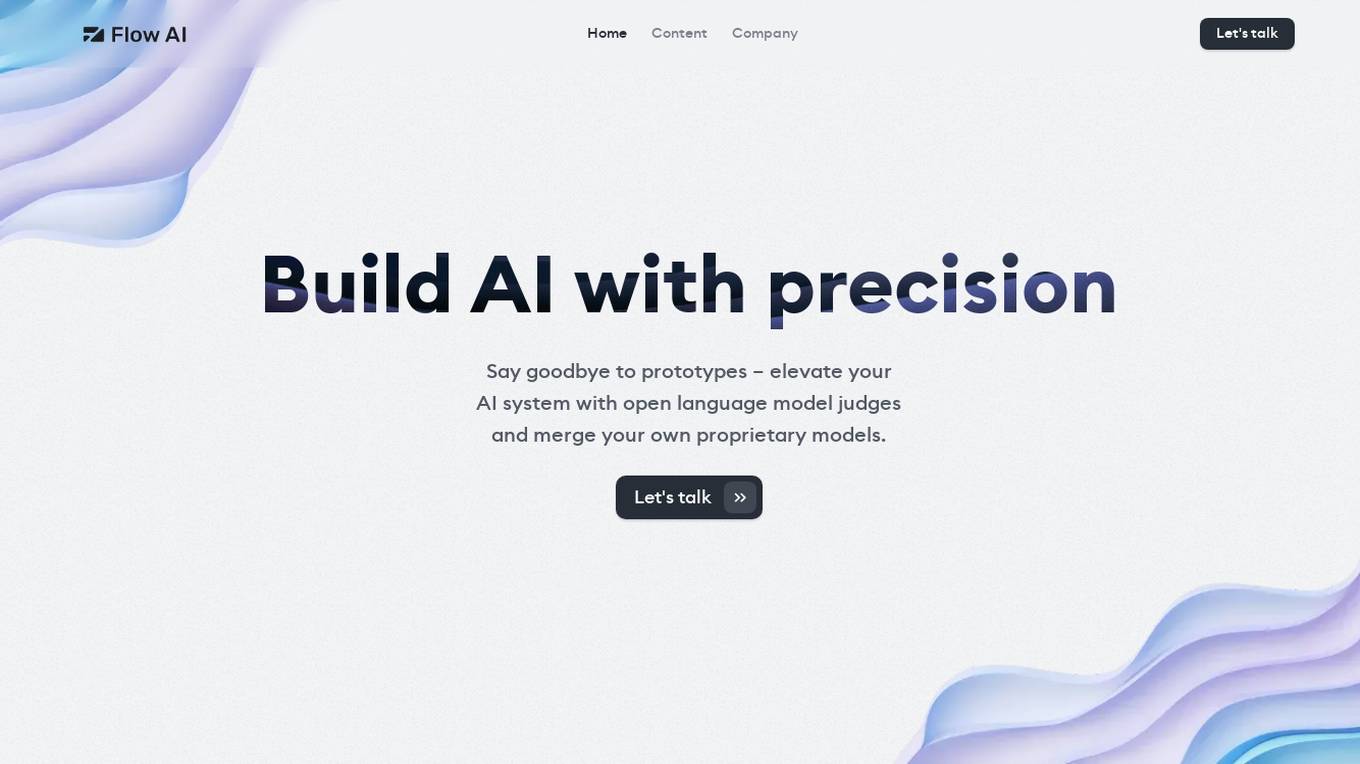
Flow AI
Flow AI is an advanced AI tool designed for evaluating and improving Large Language Model (LLM) applications. It offers a unique system for creating custom evaluators, deploying them with an API, and developing specialized LMs tailored to specific use cases. The tool aims to revolutionize AI evaluation and model development by providing transparent, cost-effective, and controllable solutions for AI teams across various domains.
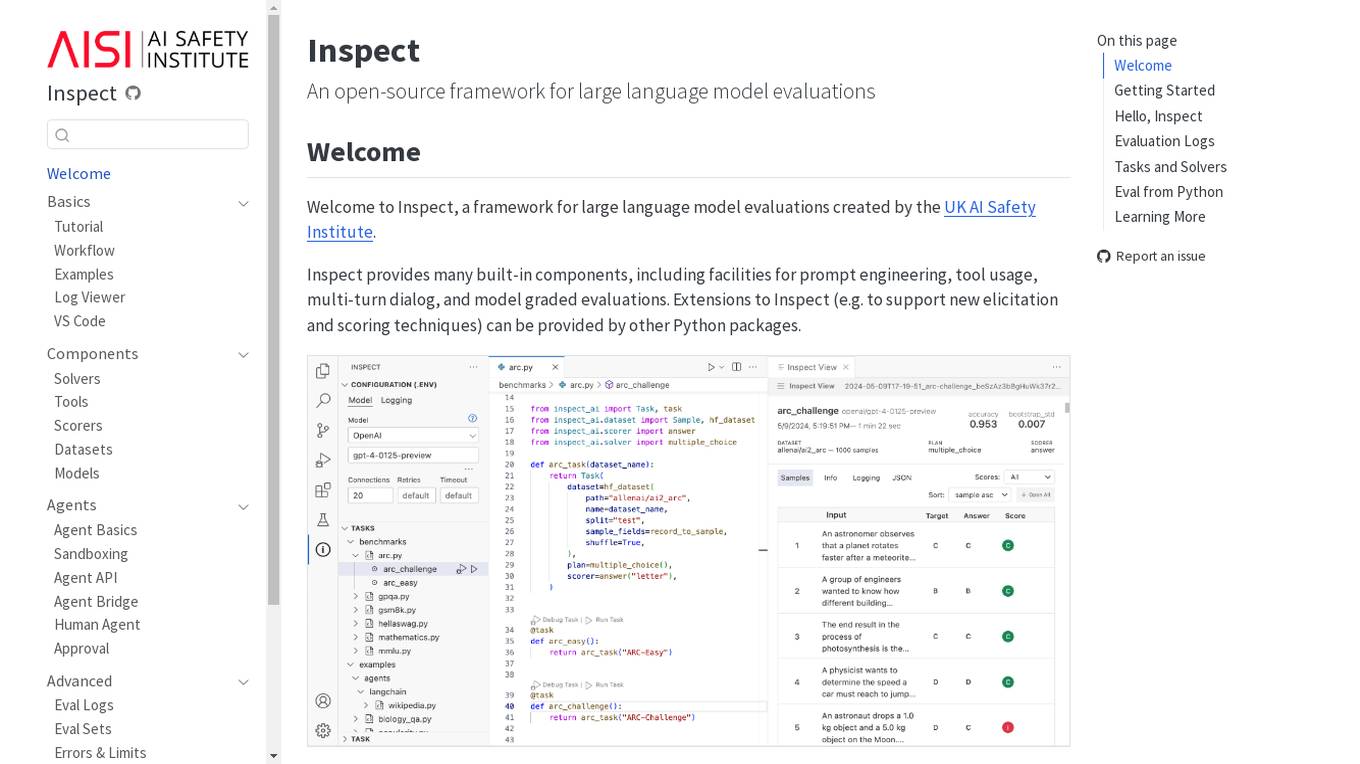
Inspect
Inspect is an open-source framework for large language model evaluations created by the UK AI Safety Institute. It provides built-in components for prompt engineering, tool usage, multi-turn dialog, and model graded evaluations. Users can explore various solvers, tools, scorers, datasets, and models to create advanced evaluations. Inspect supports extensions for new elicitation and scoring techniques through Python packages.
79 - Open Source AI Tools
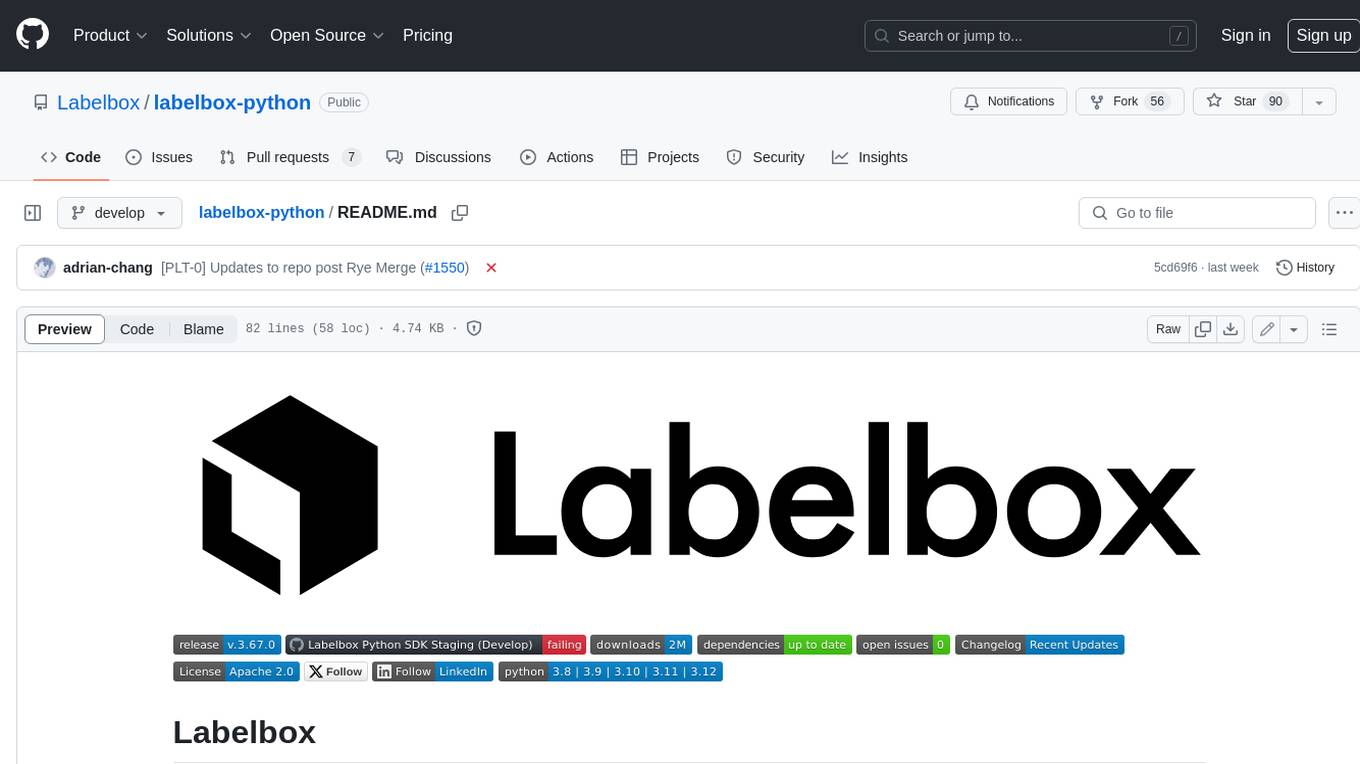
labelbox-python
Labelbox is a data-centric AI platform for enterprises to develop, optimize, and use AI to solve problems and power new products and services. Enterprises use Labelbox to curate data, generate high-quality human feedback data for computer vision and LLMs, evaluate model performance, and automate tasks by combining AI and human-centric workflows. The academic & research community uses Labelbox for cutting-edge AI research.
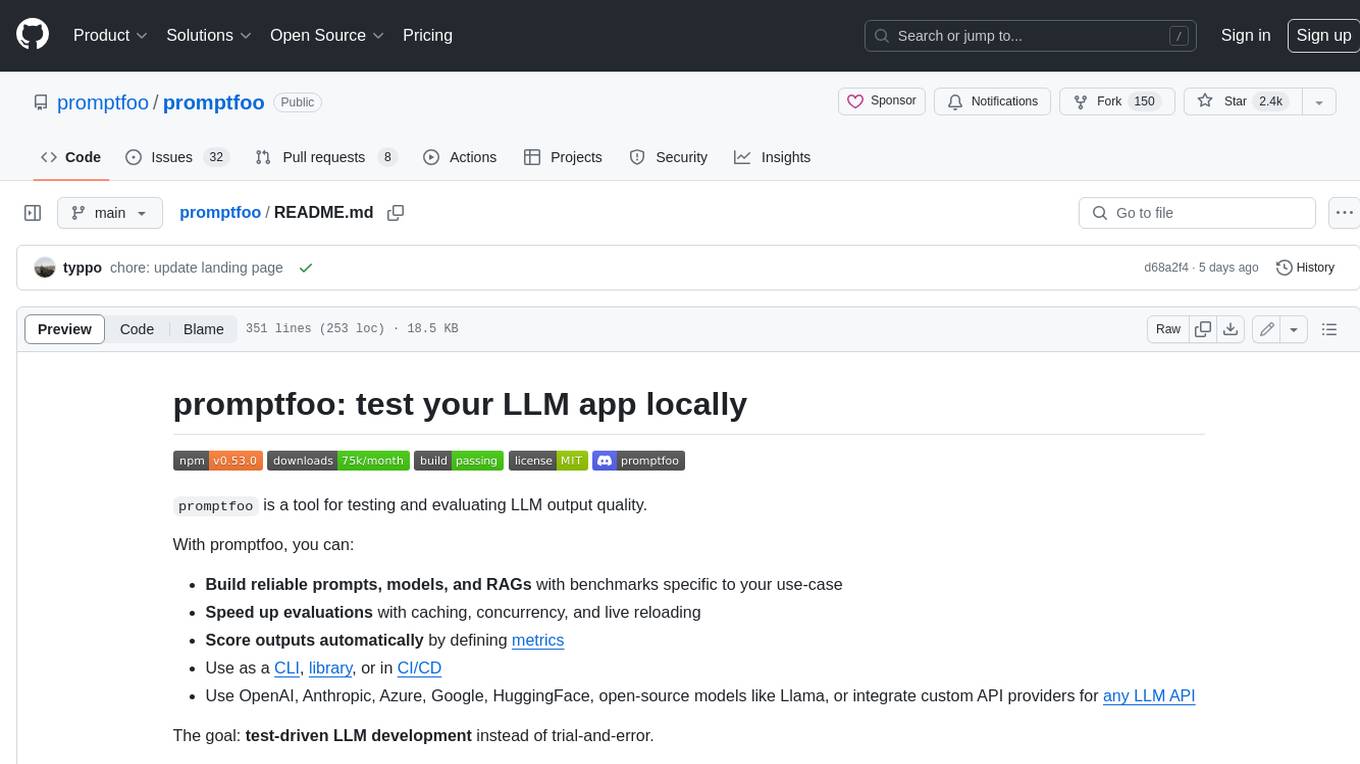
promptfoo
Promptfoo is a tool for testing and evaluating LLM output quality. With promptfoo, you can build reliable prompts, models, and RAGs with benchmarks specific to your use-case, speed up evaluations with caching, concurrency, and live reloading, score outputs automatically by defining metrics, use as a CLI, library, or in CI/CD, and use OpenAI, Anthropic, Azure, Google, HuggingFace, open-source models like Llama, or integrate custom API providers for any LLM API.
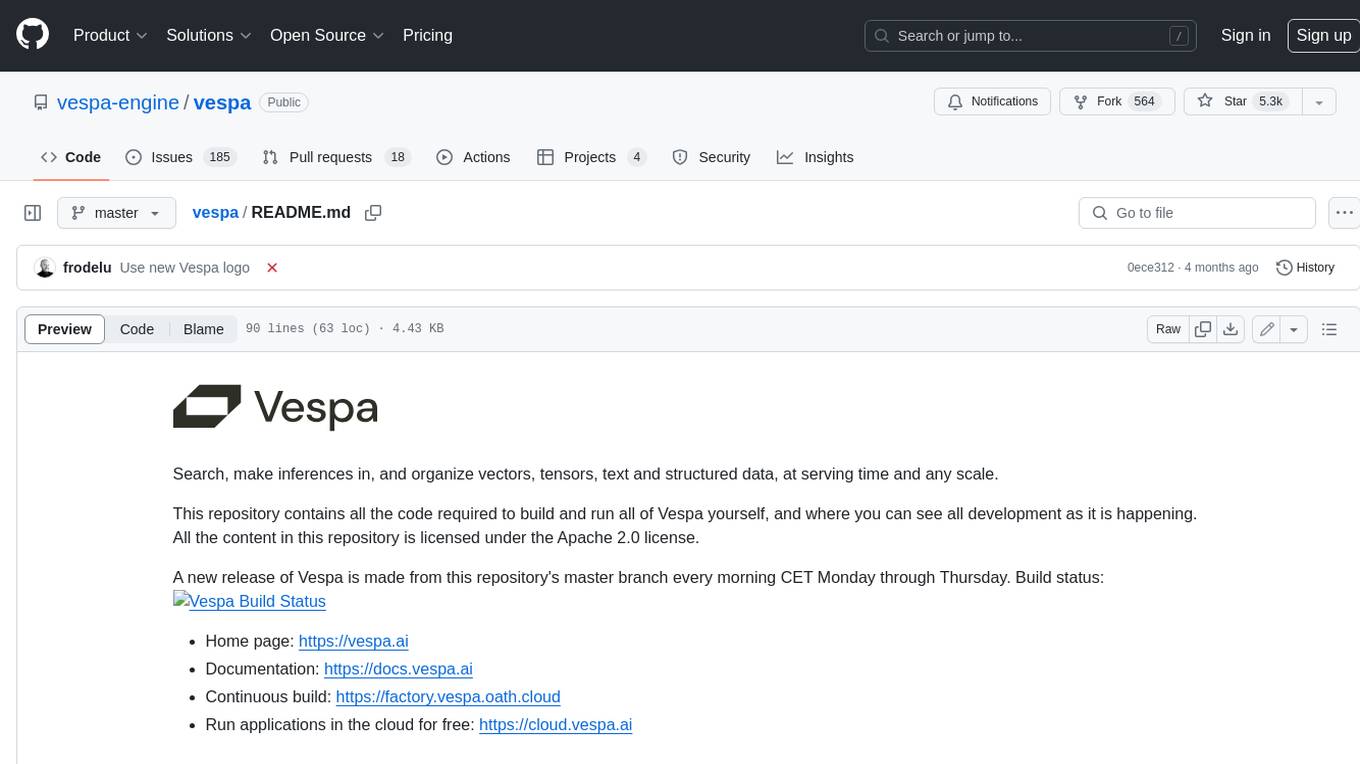
vespa
Vespa is a platform that performs operations such as selecting a subset of data in a large corpus, evaluating machine-learned models over the selected data, organizing and aggregating it, and returning it, typically in less than 100 milliseconds, all while the data corpus is continuously changing. It has been in development for many years and is used on a number of large internet services and apps which serve hundreds of thousands of queries from Vespa per second.
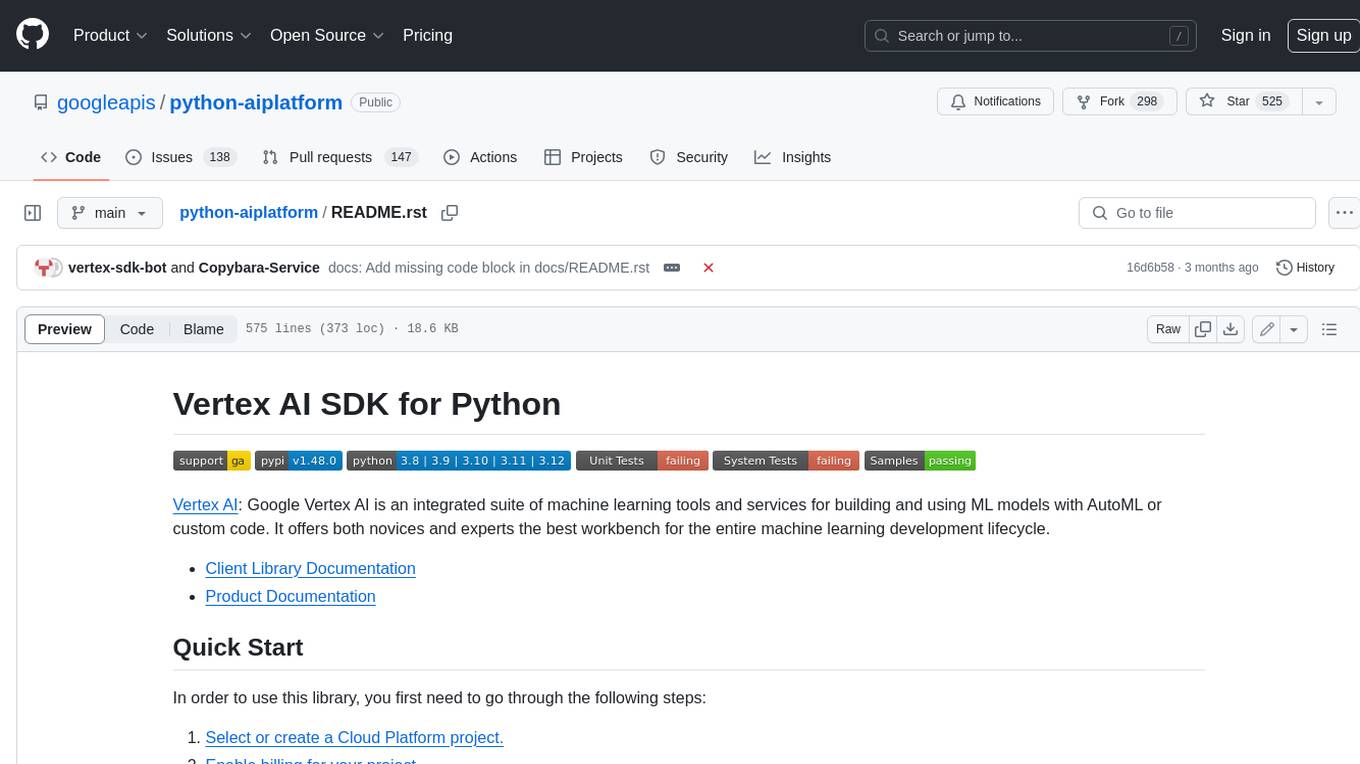
python-aiplatform
The Vertex AI SDK for Python is a library that provides a convenient way to use the Vertex AI API. It offers a high-level interface for creating and managing Vertex AI resources, such as datasets, models, and endpoints. The SDK also provides support for training and deploying custom models, as well as using AutoML models. With the Vertex AI SDK for Python, you can quickly and easily build and deploy machine learning models on Vertex AI.
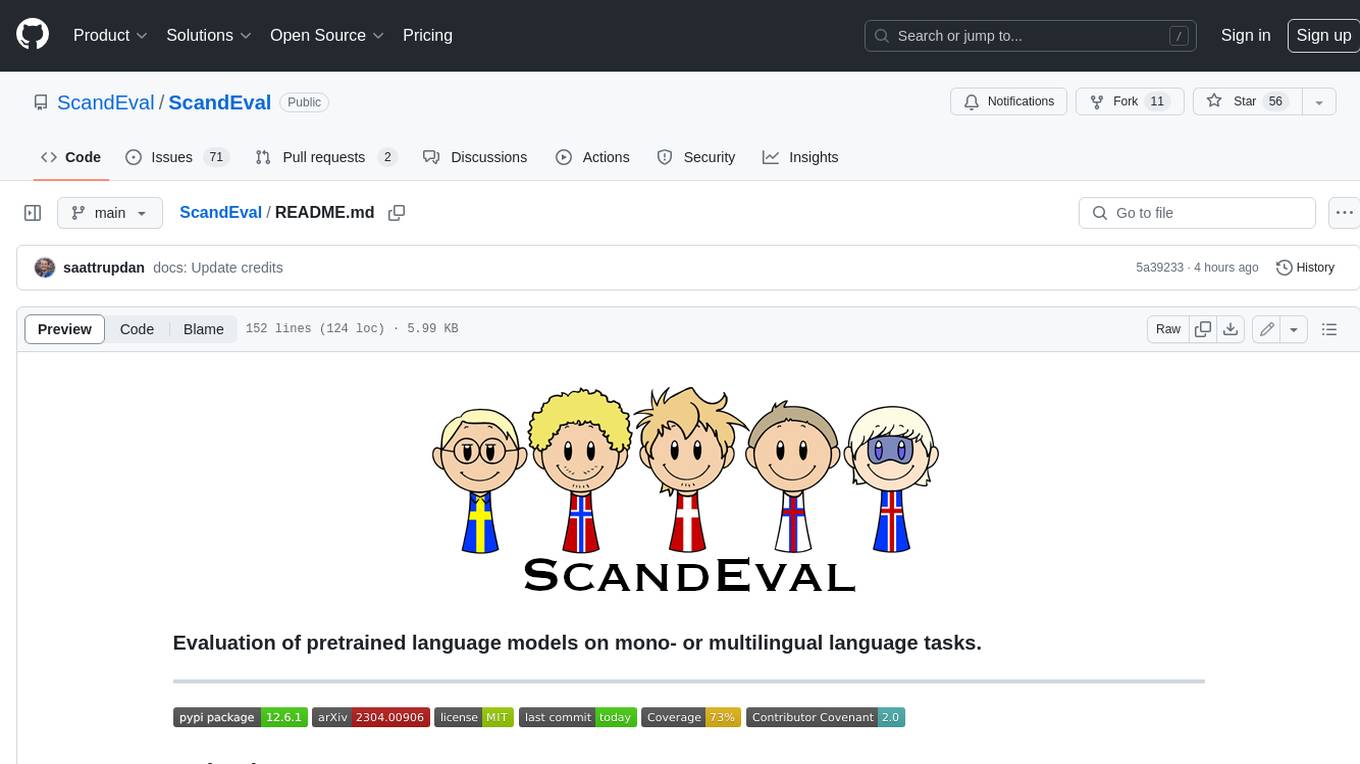
ScandEval
ScandEval is a framework for evaluating pretrained language models on mono- or multilingual language tasks. It provides a unified interface for benchmarking models on a variety of tasks, including sentiment analysis, question answering, and machine translation. ScandEval is designed to be easy to use and extensible, making it a valuable tool for researchers and practitioners alike.
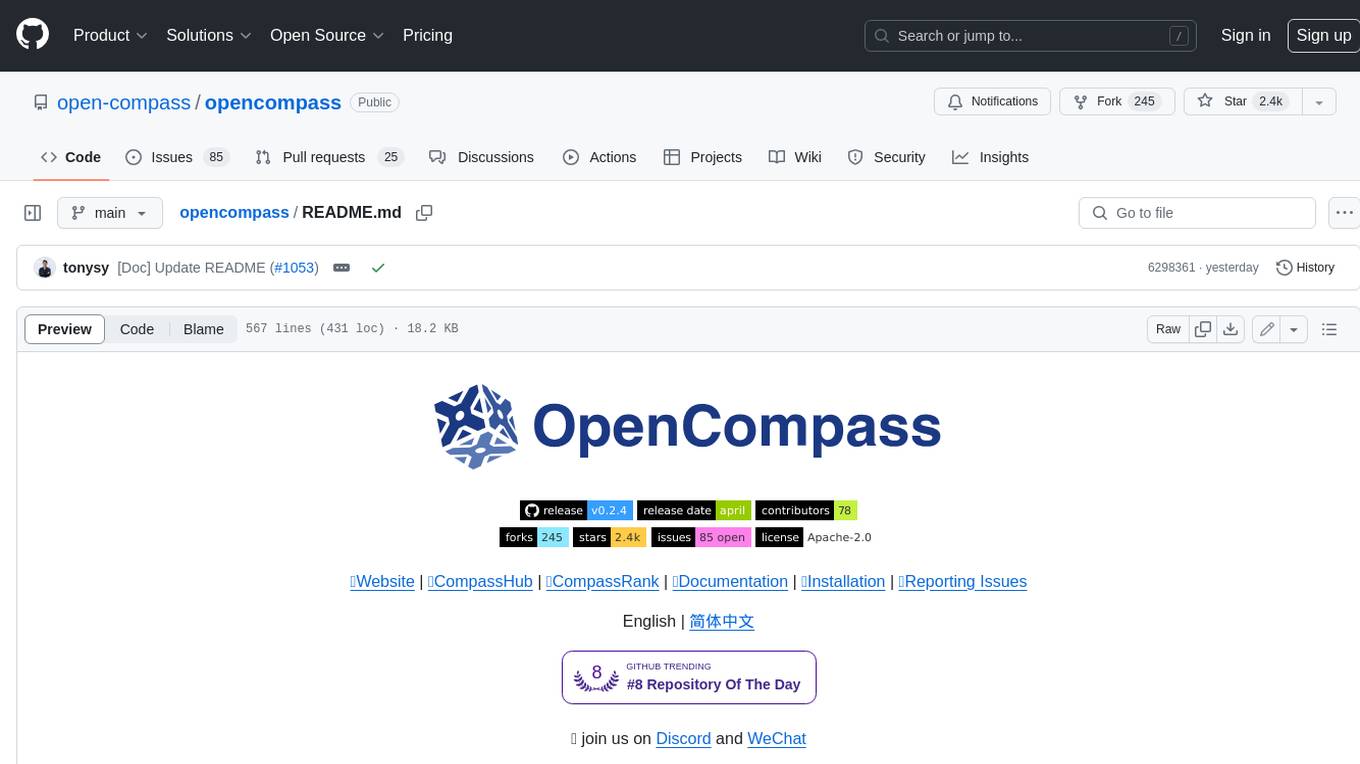
opencompass
OpenCompass is a one-stop platform for large model evaluation, aiming to provide a fair, open, and reproducible benchmark for large model evaluation. Its main features include: * Comprehensive support for models and datasets: Pre-support for 20+ HuggingFace and API models, a model evaluation scheme of 70+ datasets with about 400,000 questions, comprehensively evaluating the capabilities of the models in five dimensions. * Efficient distributed evaluation: One line command to implement task division and distributed evaluation, completing the full evaluation of billion-scale models in just a few hours. * Diversified evaluation paradigms: Support for zero-shot, few-shot, and chain-of-thought evaluations, combined with standard or dialogue-type prompt templates, to easily stimulate the maximum performance of various models. * Modular design with high extensibility: Want to add new models or datasets, customize an advanced task division strategy, or even support a new cluster management system? Everything about OpenCompass can be easily expanded! * Experiment management and reporting mechanism: Use config files to fully record each experiment, and support real-time reporting of results.
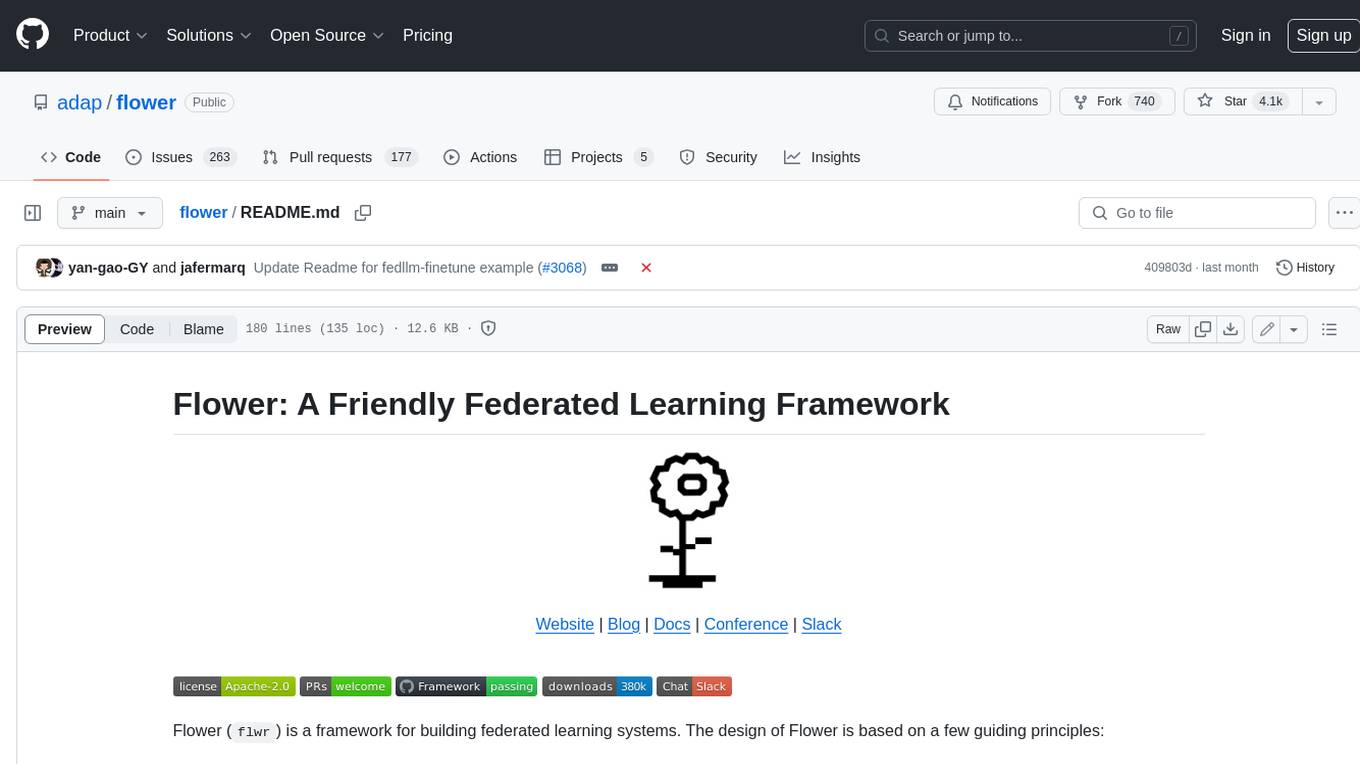
flower
Flower is a framework for building federated learning systems. It is designed to be customizable, extensible, framework-agnostic, and understandable. Flower can be used with any machine learning framework, for example, PyTorch, TensorFlow, Hugging Face Transformers, PyTorch Lightning, scikit-learn, JAX, TFLite, MONAI, fastai, MLX, XGBoost, Pandas for federated analytics, or even raw NumPy for users who enjoy computing gradients by hand.
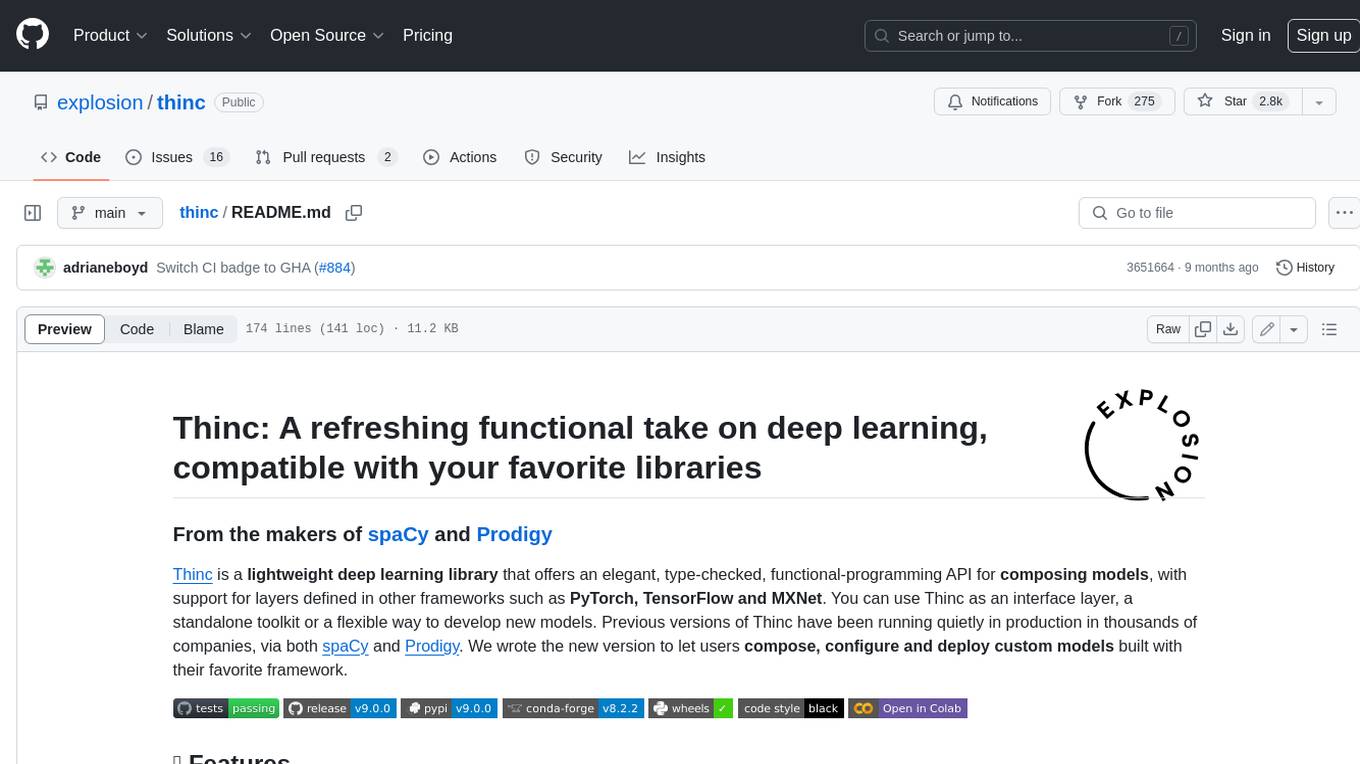
thinc
Thinc is a lightweight deep learning library that offers an elegant, type-checked, functional-programming API for composing models, with support for layers defined in other frameworks such as PyTorch, TensorFlow and MXNet. You can use Thinc as an interface layer, a standalone toolkit or a flexible way to develop new models.
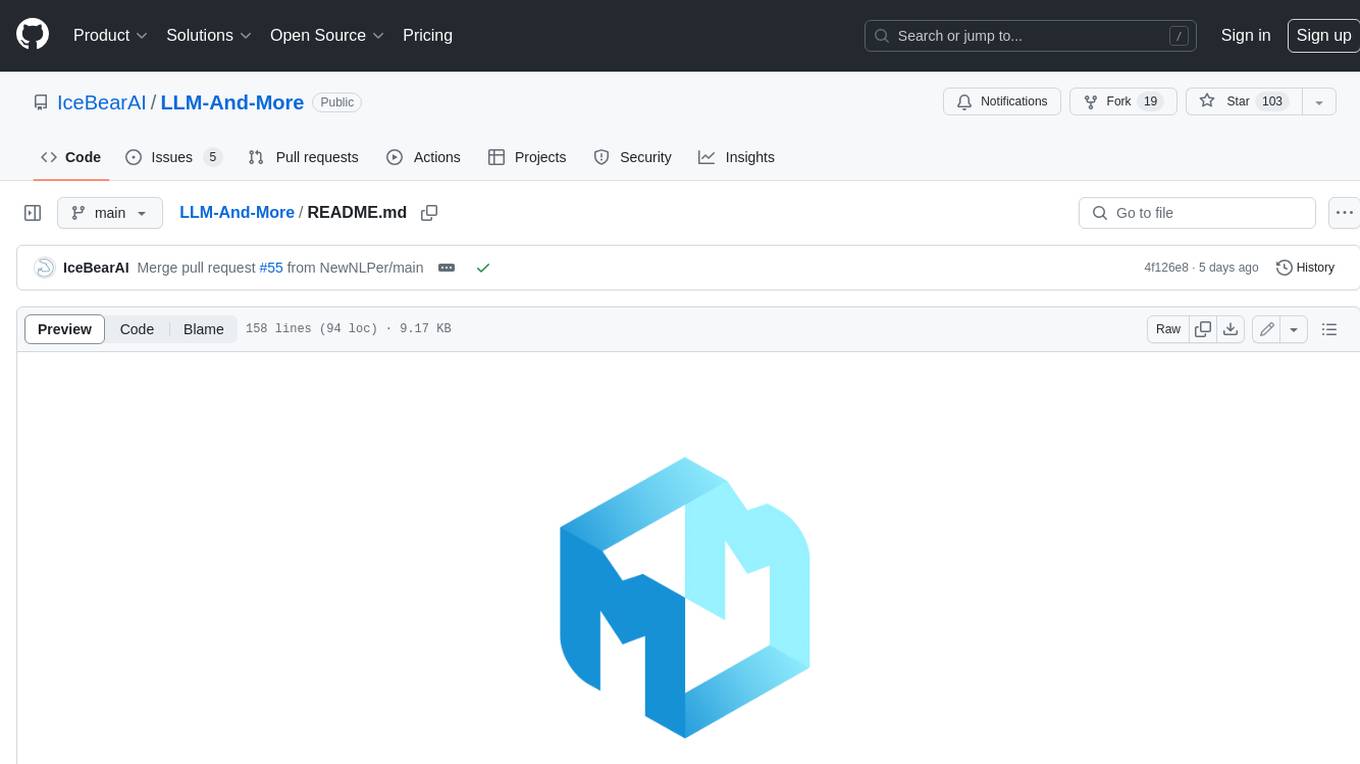
LLM-And-More
LLM-And-More is a one-stop solution for training and applying large models, covering the entire process from data processing to model evaluation, from training to deployment, and from idea to service. In this project, users can easily train models through this project and generate the required product services with one click.
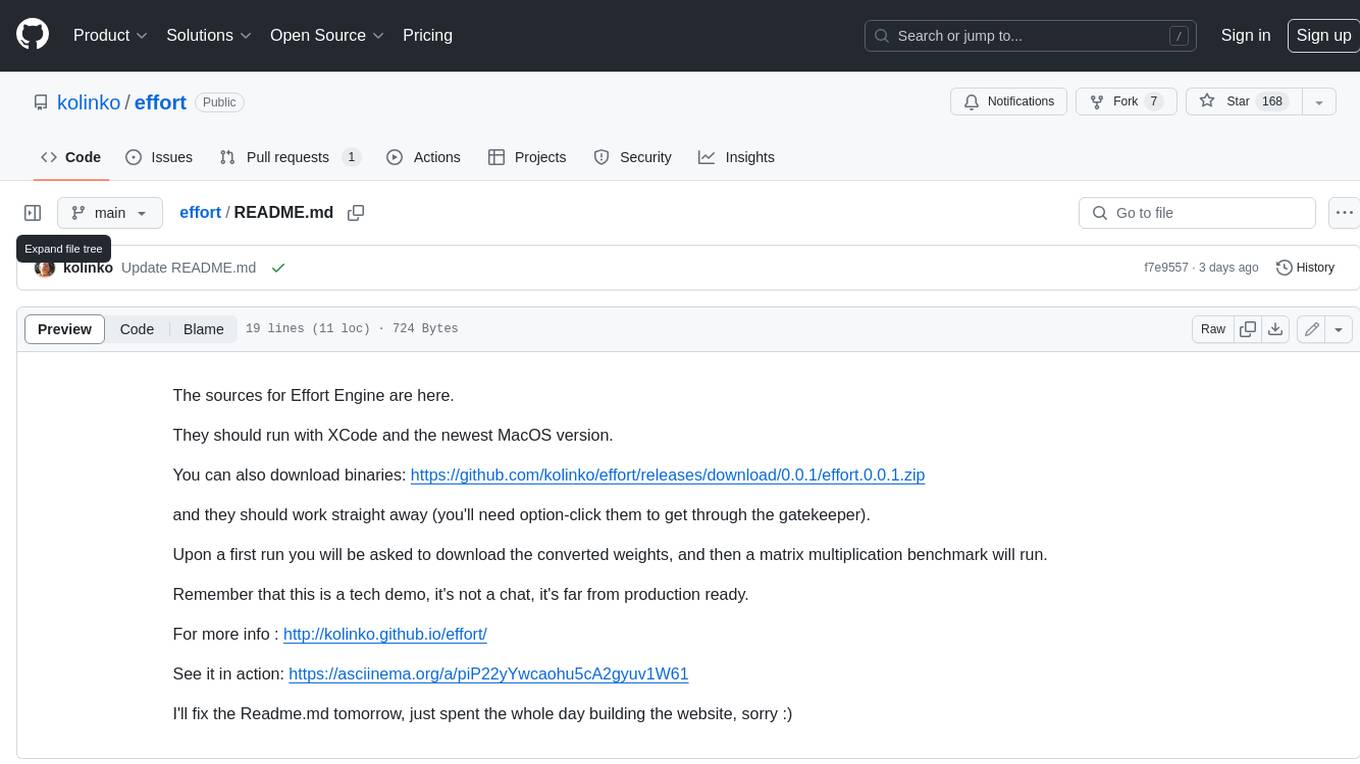
effort
Effort is an example implementation of the bucketMul algorithm, which allows for real-time adjustment of the number of calculations performed during inference of an LLM model. At 50% effort, it performs as fast as regular matrix multiplications on Apple Silicon chips; at 25% effort, it is twice as fast while still retaining most of the quality. Additionally, users have the option to skip loading the least important weights.
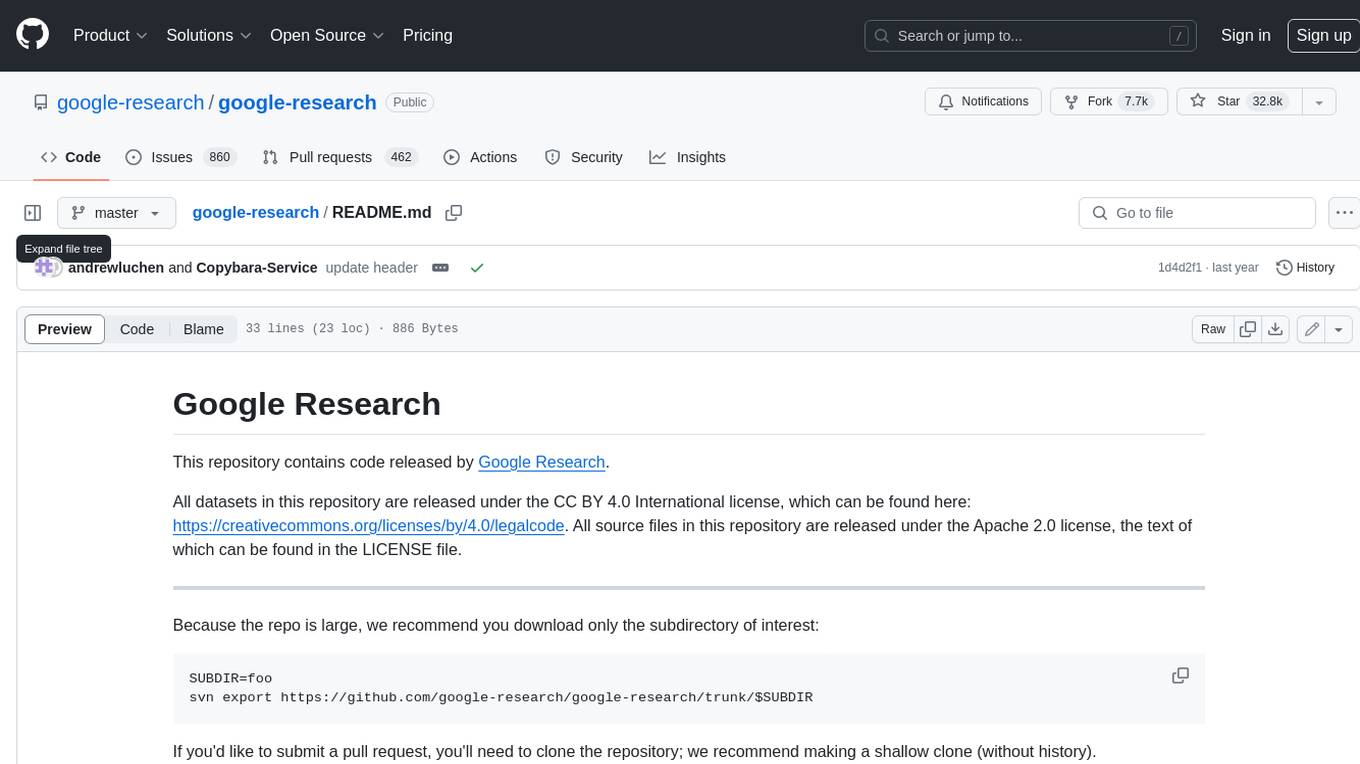
google-research
This repository contains code released by Google Research. All datasets in this repository are released under the CC BY 4.0 International license, which can be found here: https://creativecommons.org/licenses/by/4.0/legalcode. All source files in this repository are released under the Apache 2.0 license, the text of which can be found in the LICENSE file.
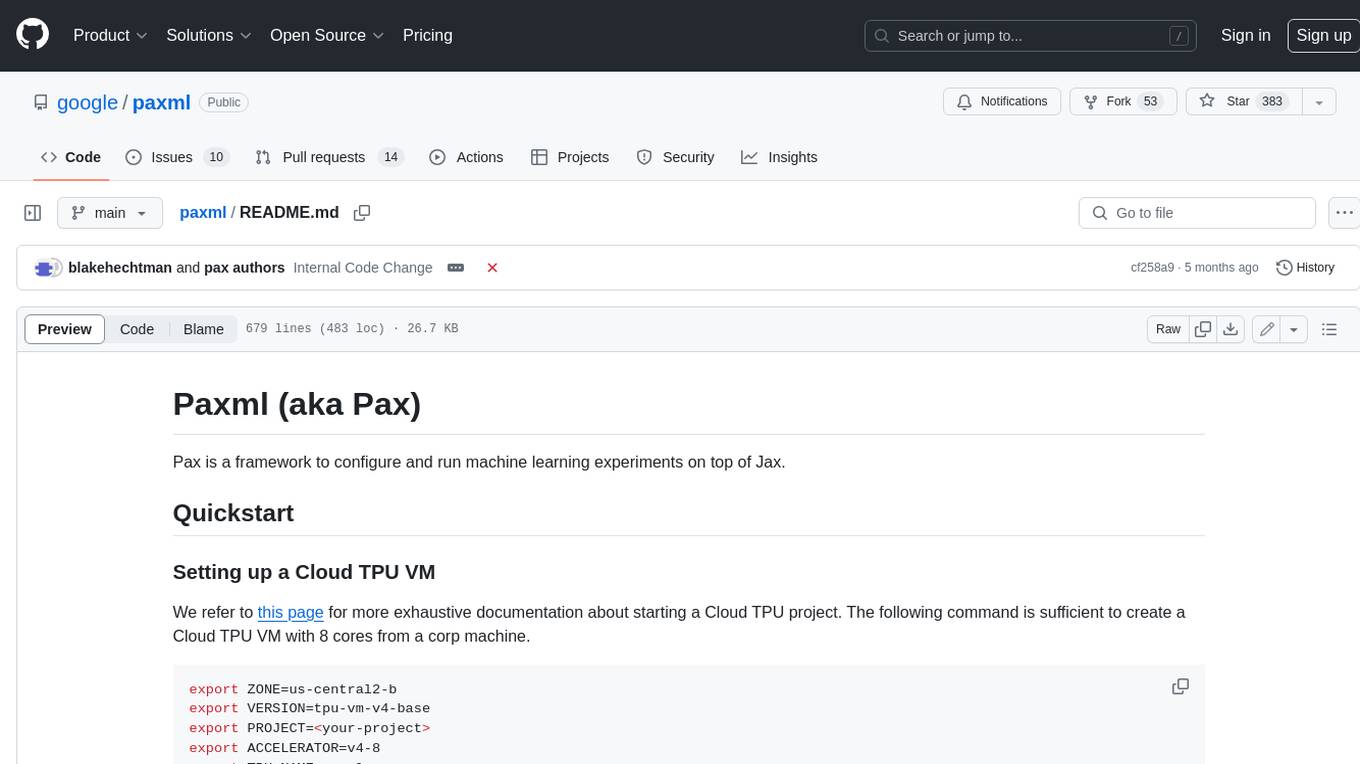
paxml
Pax is a framework to configure and run machine learning experiments on top of Jax.
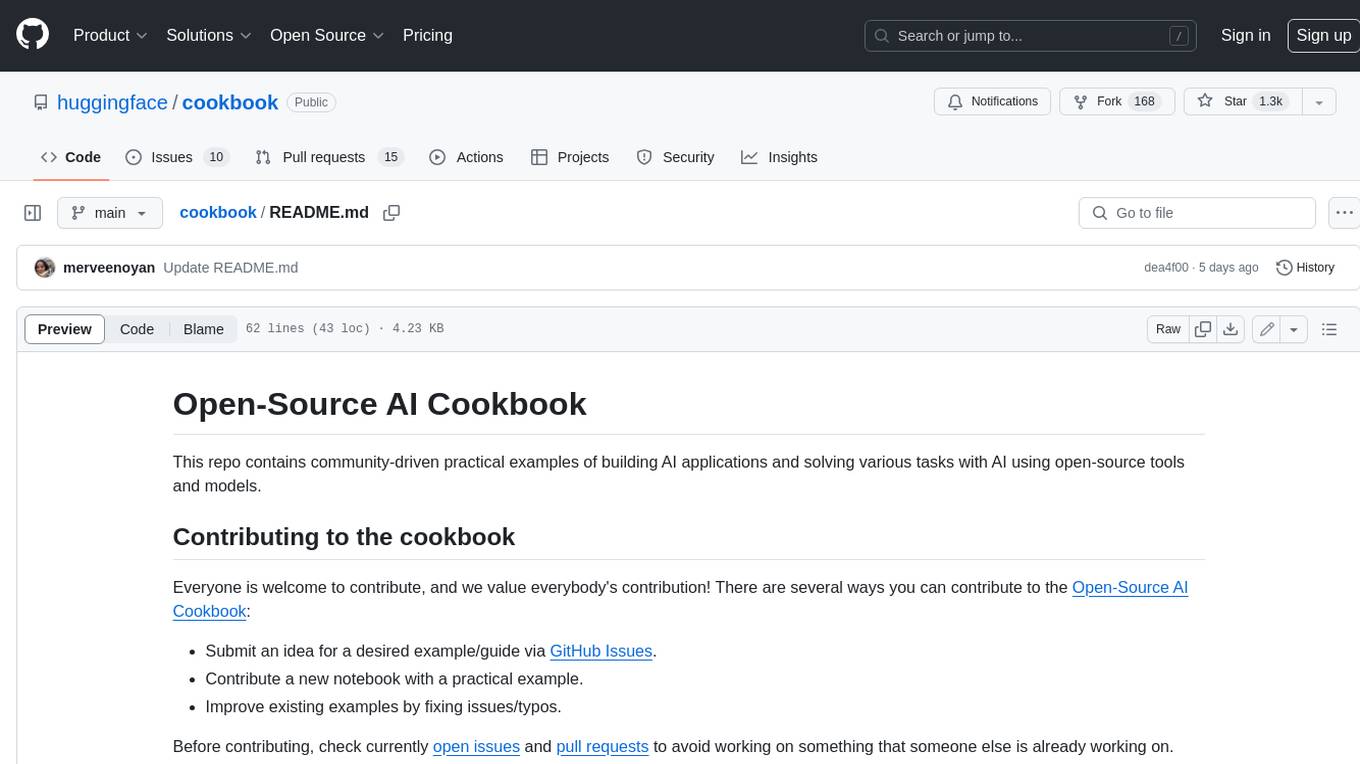
cookbook
This repository contains community-driven practical examples of building AI applications and solving various tasks with AI using open-source tools and models. Everyone is welcome to contribute, and we value everybody's contribution! There are several ways you can contribute to the Open-Source AI Cookbook: Submit an idea for a desired example/guide via GitHub Issues. Contribute a new notebook with a practical example. Improve existing examples by fixing issues/typos. Before contributing, check currently open issues and pull requests to avoid working on something that someone else is already working on.
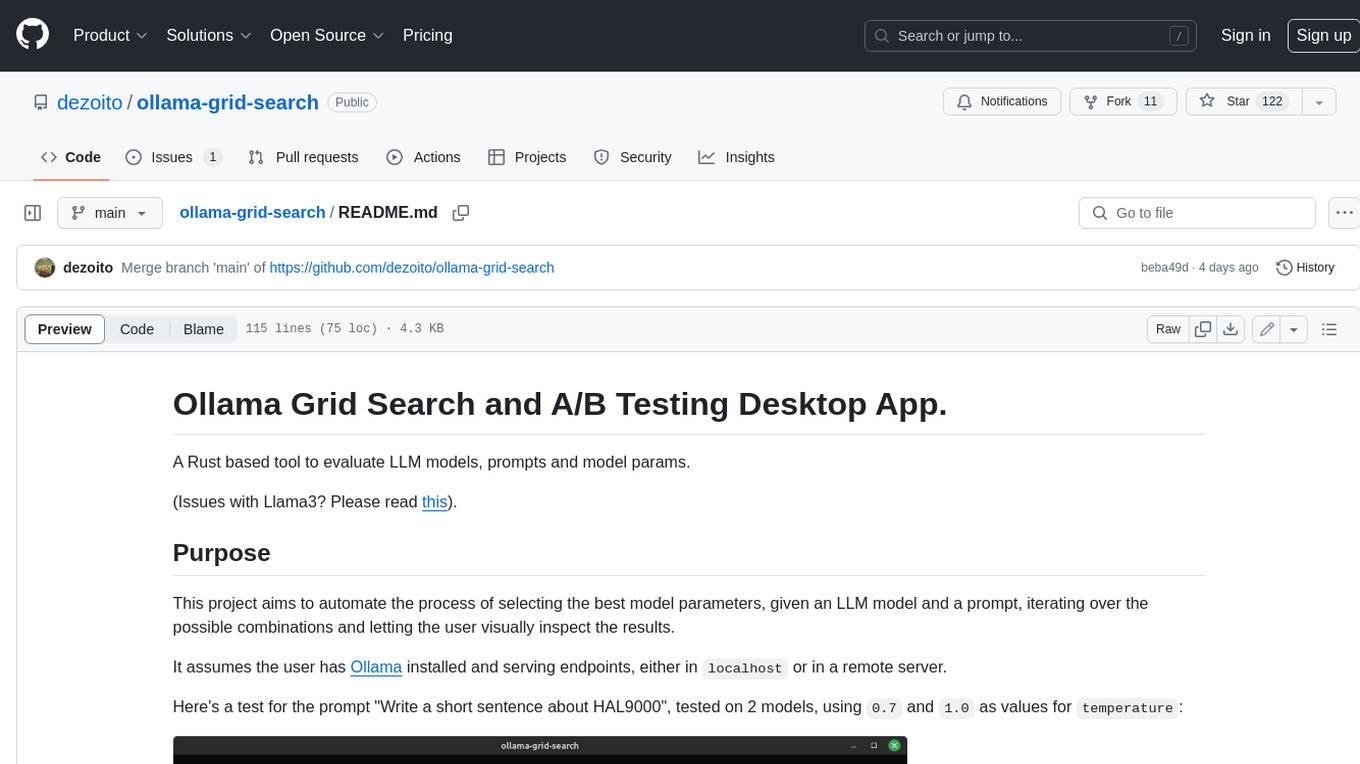
ollama-grid-search
A Rust based tool to evaluate LLM models, prompts and model params. It automates the process of selecting the best model parameters, given an LLM model and a prompt, iterating over the possible combinations and letting the user visually inspect the results. The tool assumes the user has Ollama installed and serving endpoints, either in `localhost` or in a remote server. Key features include: * Automatically fetches models from local or remote Ollama servers * Iterates over different models and params to generate inferences * A/B test prompts on different models simultaneously * Allows multiple iterations for each combination of parameters * Makes synchronous inference calls to avoid spamming servers * Optionally outputs inference parameters and response metadata (inference time, tokens and tokens/s) * Refetching of individual inference calls * Model selection can be filtered by name * List experiments which can be downloaded in JSON format * Configurable inference timeout * Custom default parameters and system prompts can be defined in settings
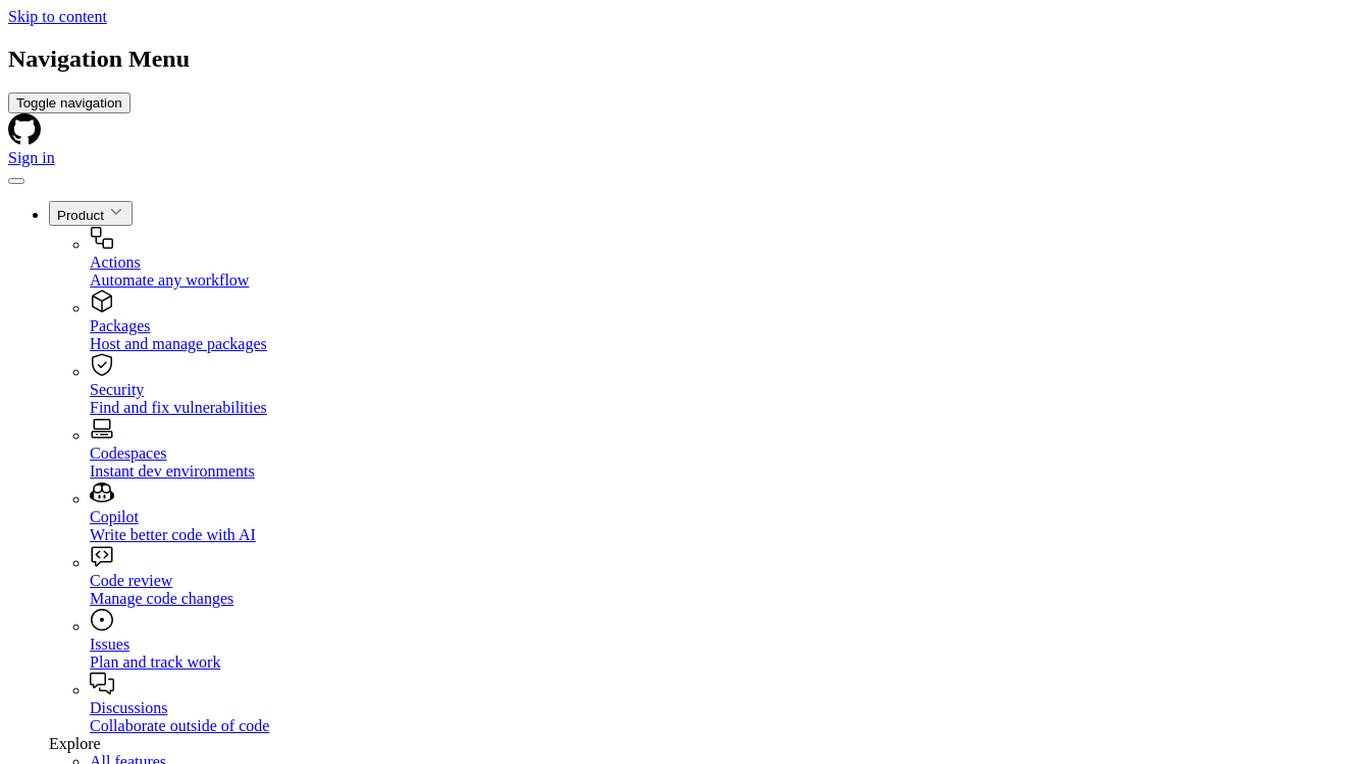
cyclops
Cyclops is a toolkit for facilitating research and deployment of ML models for healthcare. It provides a few high-level APIs namely: data - Create datasets for training, inference and evaluation. We use the popular 🤗 datasets to efficiently load and slice different modalities of data models - Use common model implementations using scikit-learn and PyTorch tasks - Use common ML task formulations such as binary classification or multi-label classification on tabular, time-series and image data evaluate - Evaluate models on clinical prediction tasks monitor - Detect dataset shift relevant for clinical use cases report - Create model report cards for clinical ML models
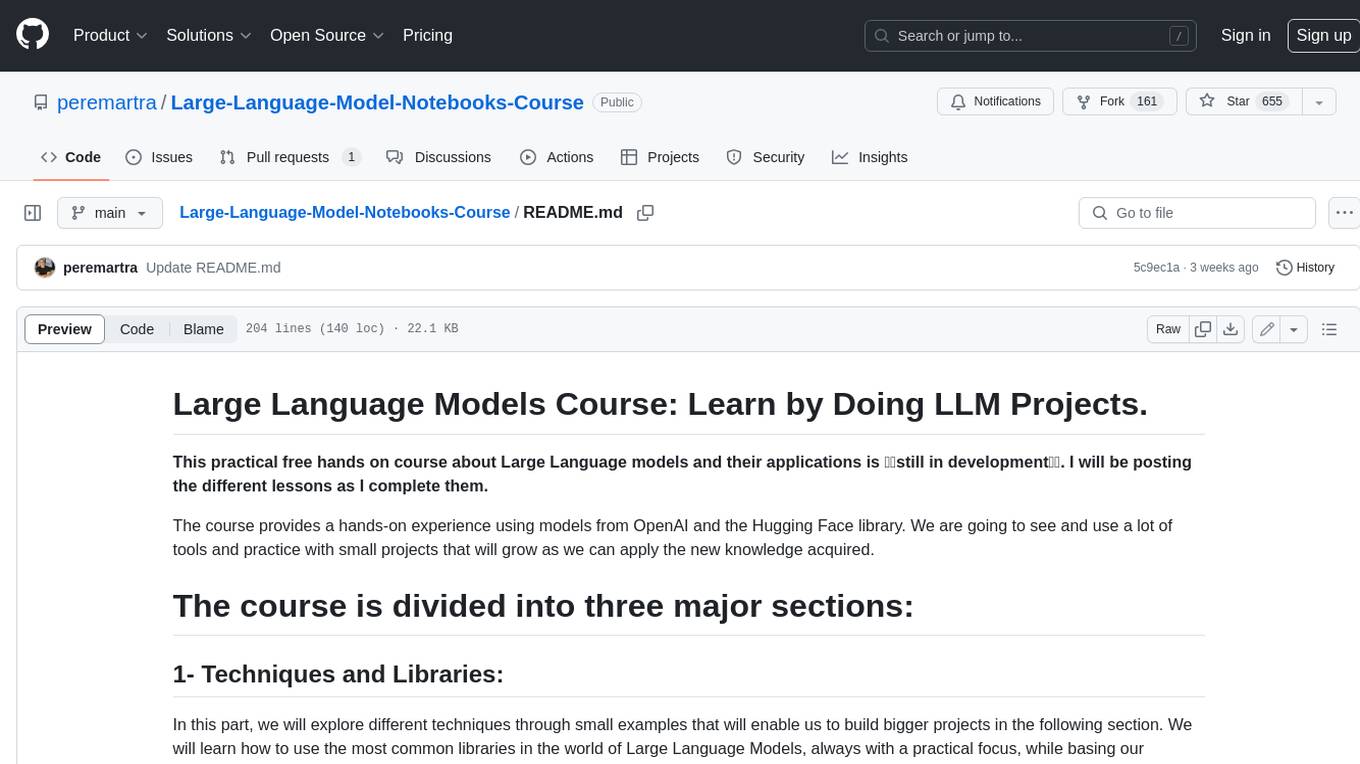
Large-Language-Model-Notebooks-Course
This practical free hands-on course focuses on Large Language models and their applications, providing a hands-on experience using models from OpenAI and the Hugging Face library. The course is divided into three major sections: Techniques and Libraries, Projects, and Enterprise Solutions. It covers topics such as Chatbots, Code Generation, Vector databases, LangChain, Fine Tuning, PEFT Fine Tuning, Soft Prompt tuning, LoRA, QLoRA, Evaluate Models, Knowledge Distillation, and more. Each section contains chapters with lessons supported by notebooks and articles. The course aims to help users build projects and explore enterprise solutions using Large Language Models.
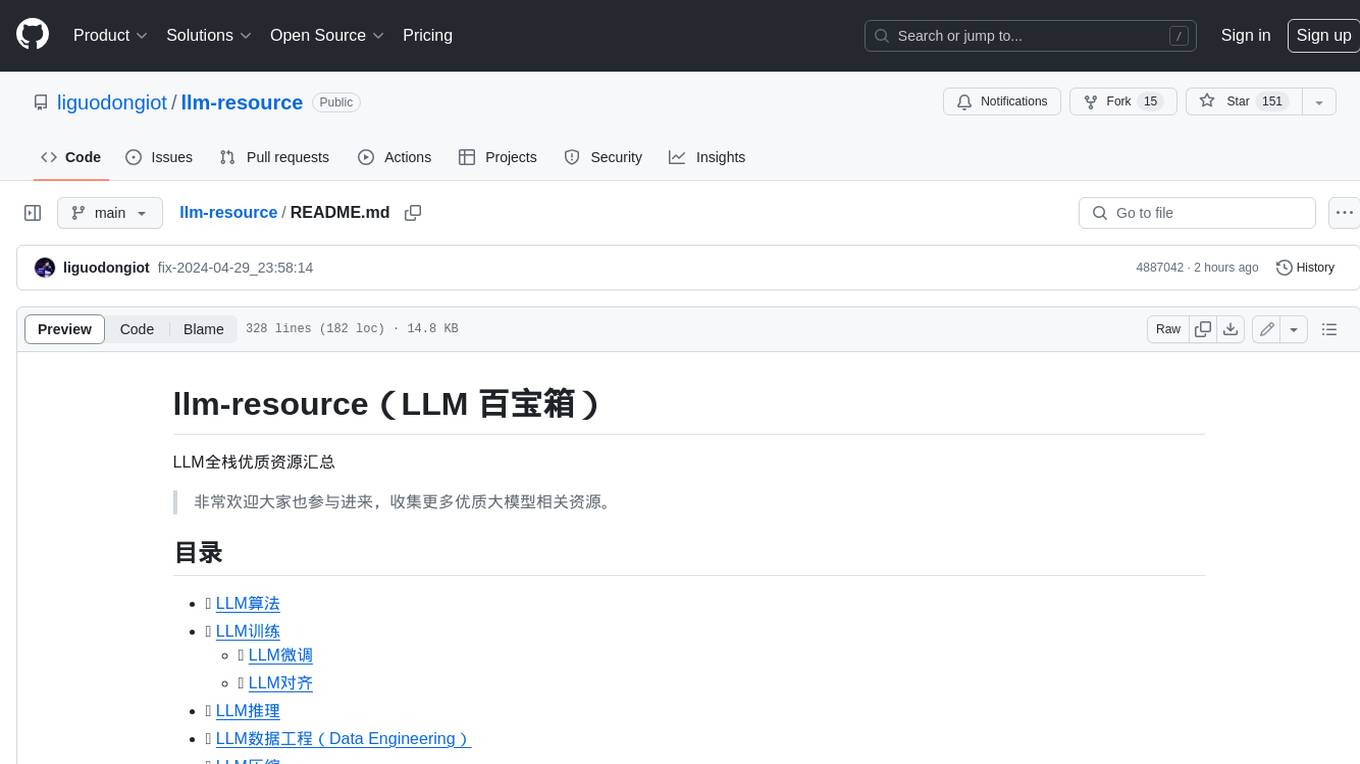
llm-resource
llm-resource is a comprehensive collection of high-quality resources for Large Language Models (LLM). It covers various aspects of LLM including algorithms, training, fine-tuning, alignment, inference, data engineering, compression, evaluation, prompt engineering, AI frameworks, AI basics, AI infrastructure, AI compilers, LLM application development, LLM operations, AI systems, and practical implementations. The repository aims to gather and share valuable resources related to LLM for the community to benefit from.
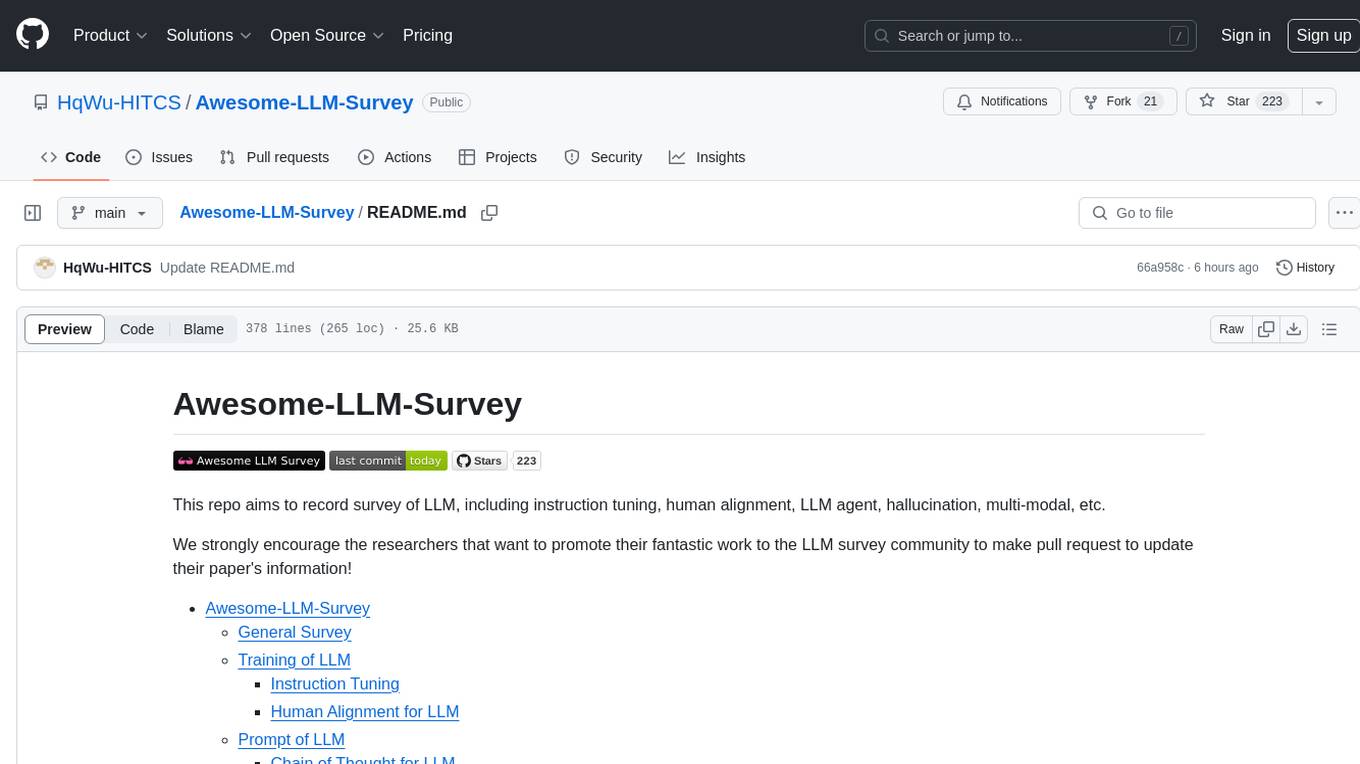
Awesome-LLM-Survey
This repository, Awesome-LLM-Survey, serves as a comprehensive collection of surveys related to Large Language Models (LLM). It covers various aspects of LLM, including instruction tuning, human alignment, LLM agents, hallucination, multi-modal capabilities, and more. Researchers are encouraged to contribute by updating information on their papers to benefit the LLM survey community.
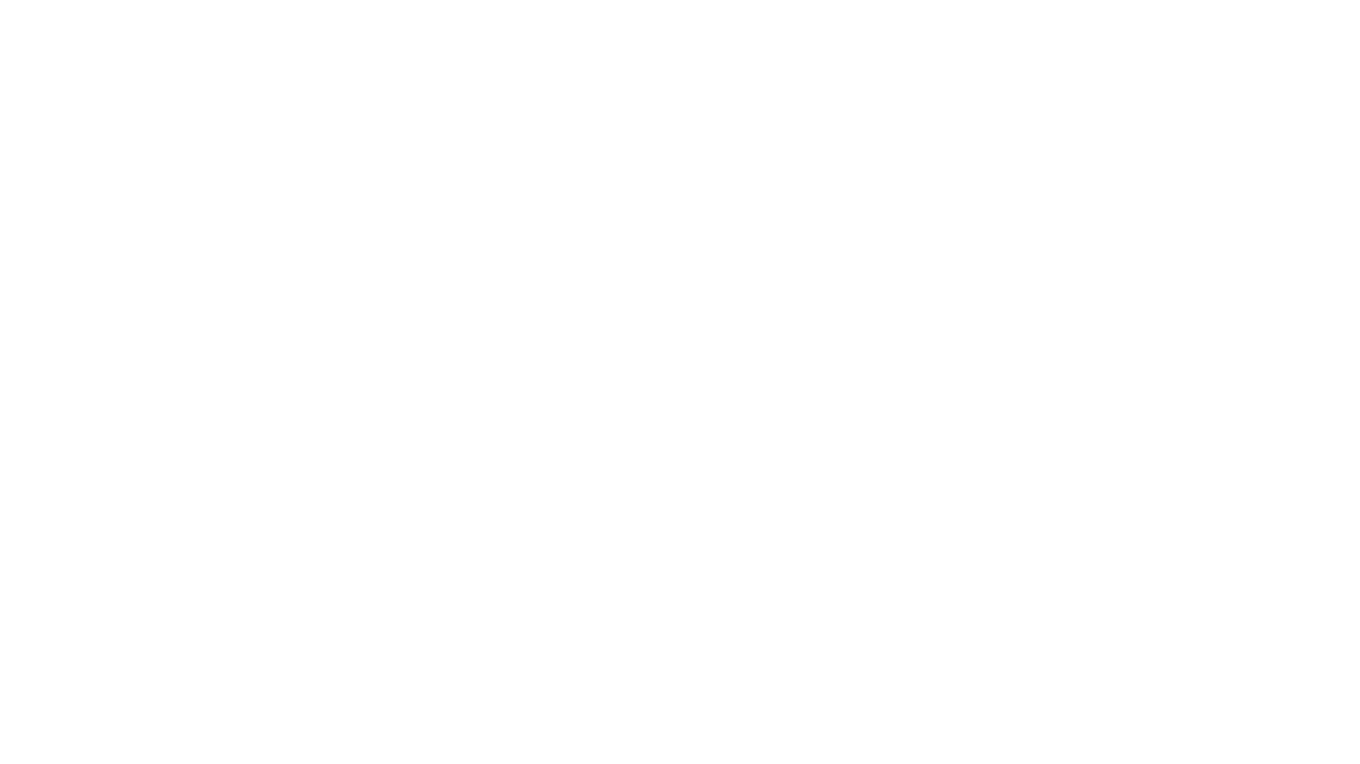
HPT
Hyper-Pretrained Transformers (HPT) is a novel multimodal LLM framework from HyperGAI, trained for vision-language models capable of understanding both textual and visual inputs. The repository contains the open-source implementation of inference code to reproduce the evaluation results of HPT Air on different benchmarks. HPT has achieved competitive results with state-of-the-art models on various multimodal LLM benchmarks. It offers models like HPT 1.5 Air and HPT 1.0 Air, providing efficient solutions for vision-and-language tasks.
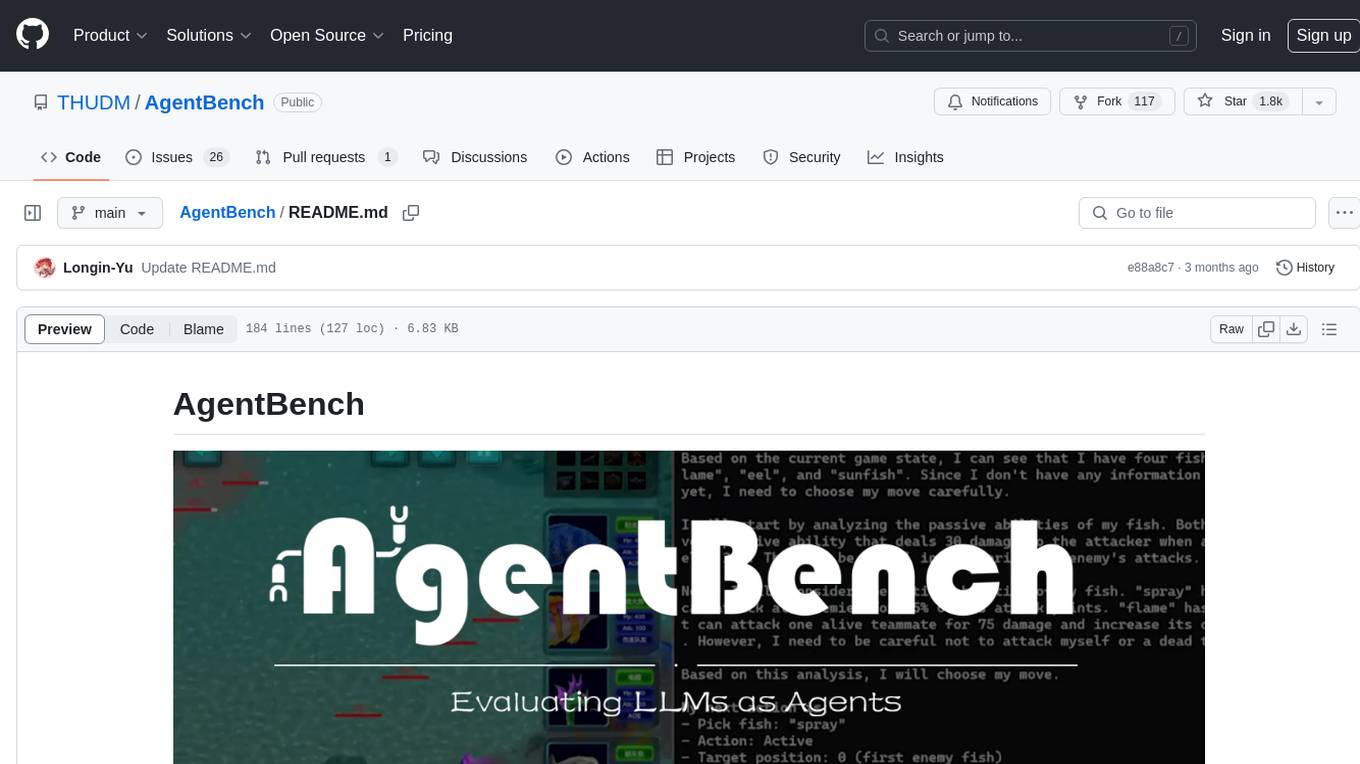
AgentBench
AgentBench is a benchmark designed to evaluate Large Language Models (LLMs) as autonomous agents in various environments. It includes 8 distinct environments such as Operating System, Database, Knowledge Graph, Digital Card Game, and Lateral Thinking Puzzles. The tool provides a comprehensive evaluation of LLMs' ability to operate as agents by offering Dev and Test sets for each environment. Users can quickly start using the tool by following the provided steps, configuring the agent, starting task servers, and assigning tasks. AgentBench aims to bridge the gap between LLMs' proficiency as agents and their practical usability.
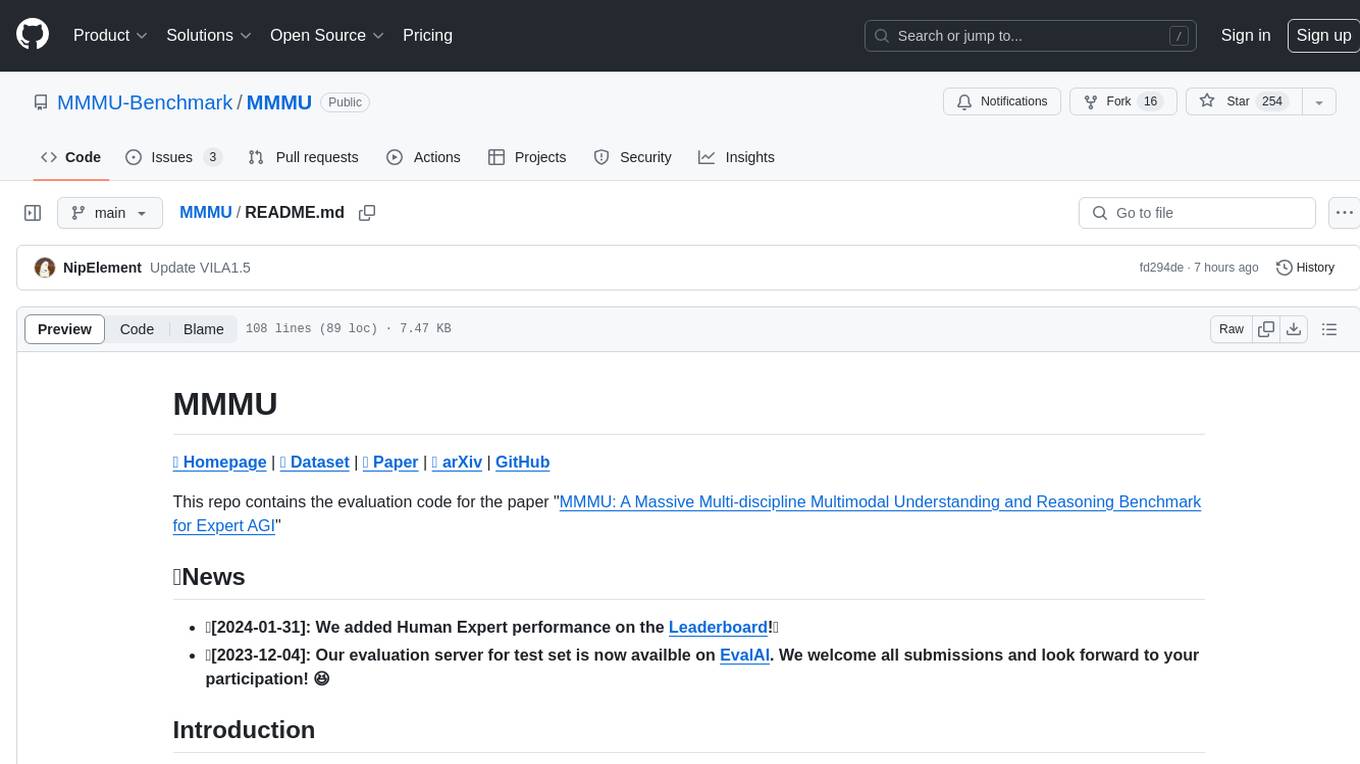
MMMU
MMMU is a benchmark designed to evaluate multimodal models on college-level subject knowledge tasks, covering 30 subjects and 183 subfields with 11.5K questions. It focuses on advanced perception and reasoning with domain-specific knowledge, challenging models to perform tasks akin to those faced by experts. The evaluation of various models highlights substantial challenges, with room for improvement to stimulate the community towards expert artificial general intelligence (AGI).
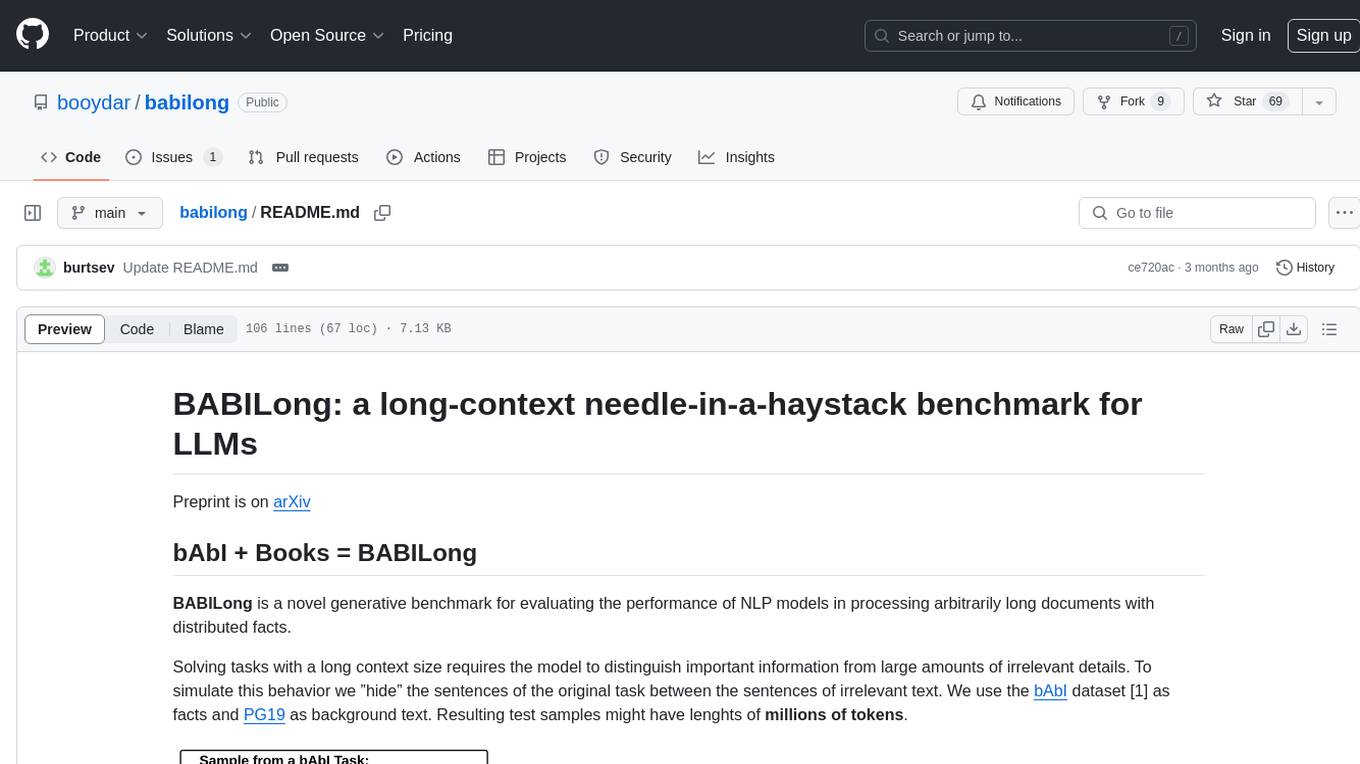
babilong
BABILong is a generative benchmark designed to evaluate the performance of NLP models in processing long documents with distributed facts. It consists of 20 tasks that simulate interactions between characters and objects in various locations, requiring models to distinguish important information from irrelevant details. The tasks vary in complexity and reasoning aspects, with test samples potentially containing millions of tokens. The benchmark aims to challenge and assess the capabilities of Large Language Models (LLMs) in handling complex, long-context information.
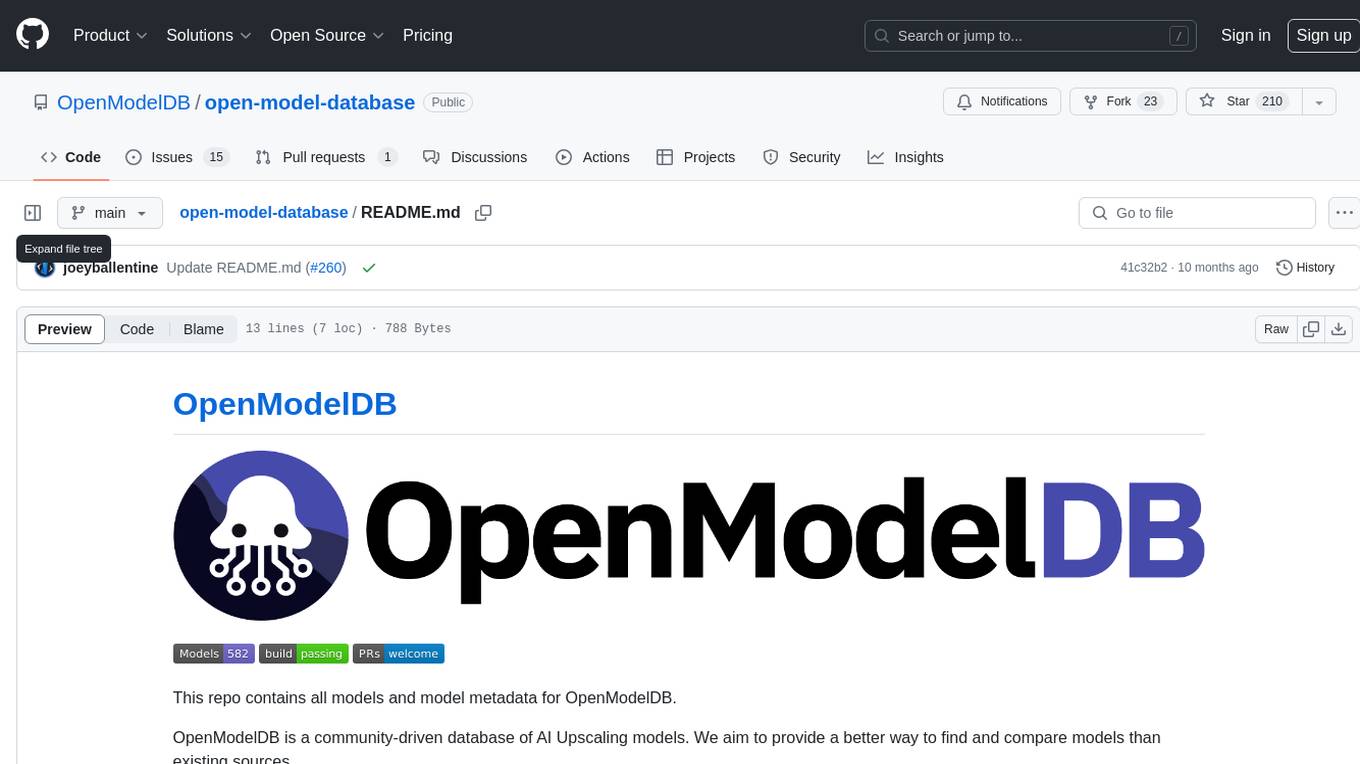
open-model-database
OpenModelDB is a community-driven database of AI upscaling models, providing a centralized platform for users to access and compare various models. The repository contains a collection of models and model metadata, facilitating easy exploration and evaluation of different AI upscaling solutions. With a focus on enhancing the accessibility and usability of AI models, OpenModelDB aims to streamline the process of finding and selecting the most suitable models for specific tasks or projects.
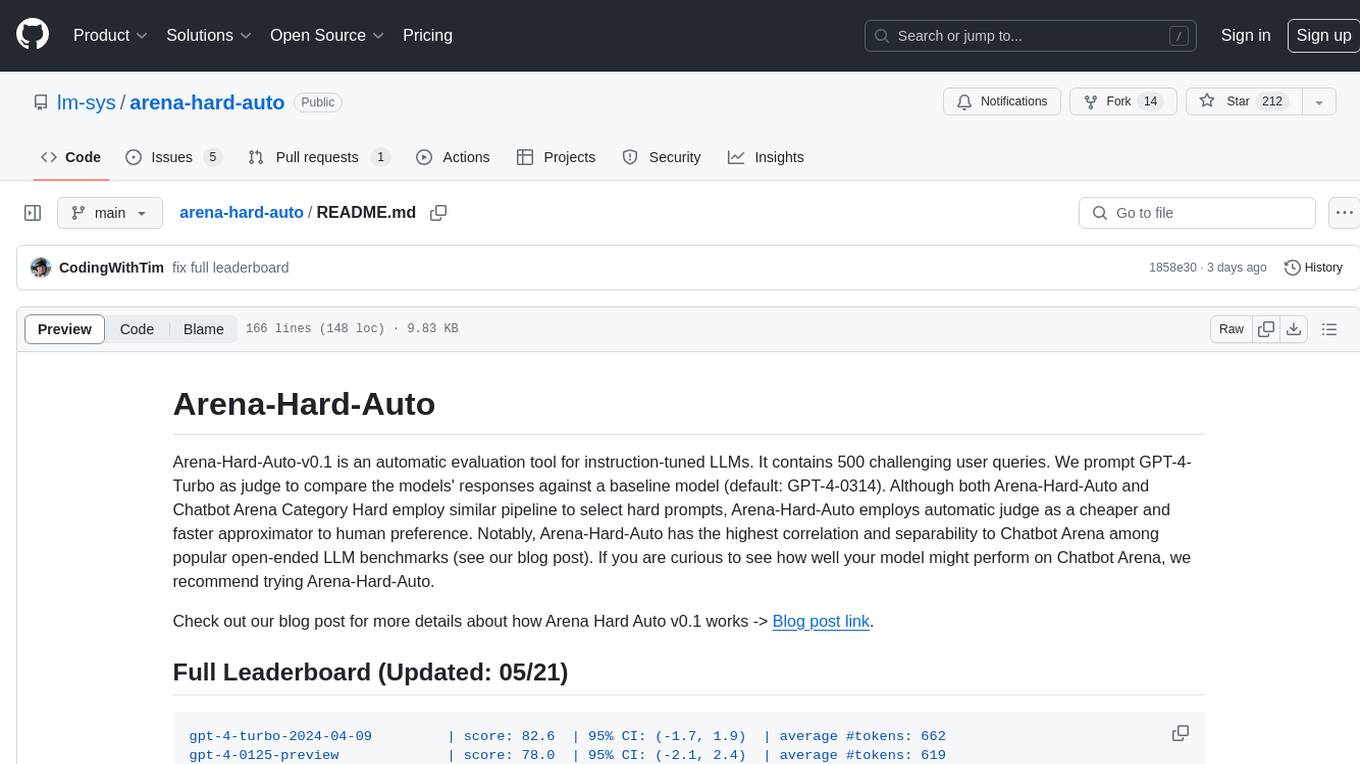
arena-hard-auto
Arena-Hard-Auto-v0.1 is an automatic evaluation tool for instruction-tuned LLMs. It contains 500 challenging user queries. The tool prompts GPT-4-Turbo as a judge to compare models' responses against a baseline model (default: GPT-4-0314). Arena-Hard-Auto employs an automatic judge as a cheaper and faster approximator to human preference. It has the highest correlation and separability to Chatbot Arena among popular open-ended LLM benchmarks. Users can evaluate their models' performance on Chatbot Arena by using Arena-Hard-Auto.
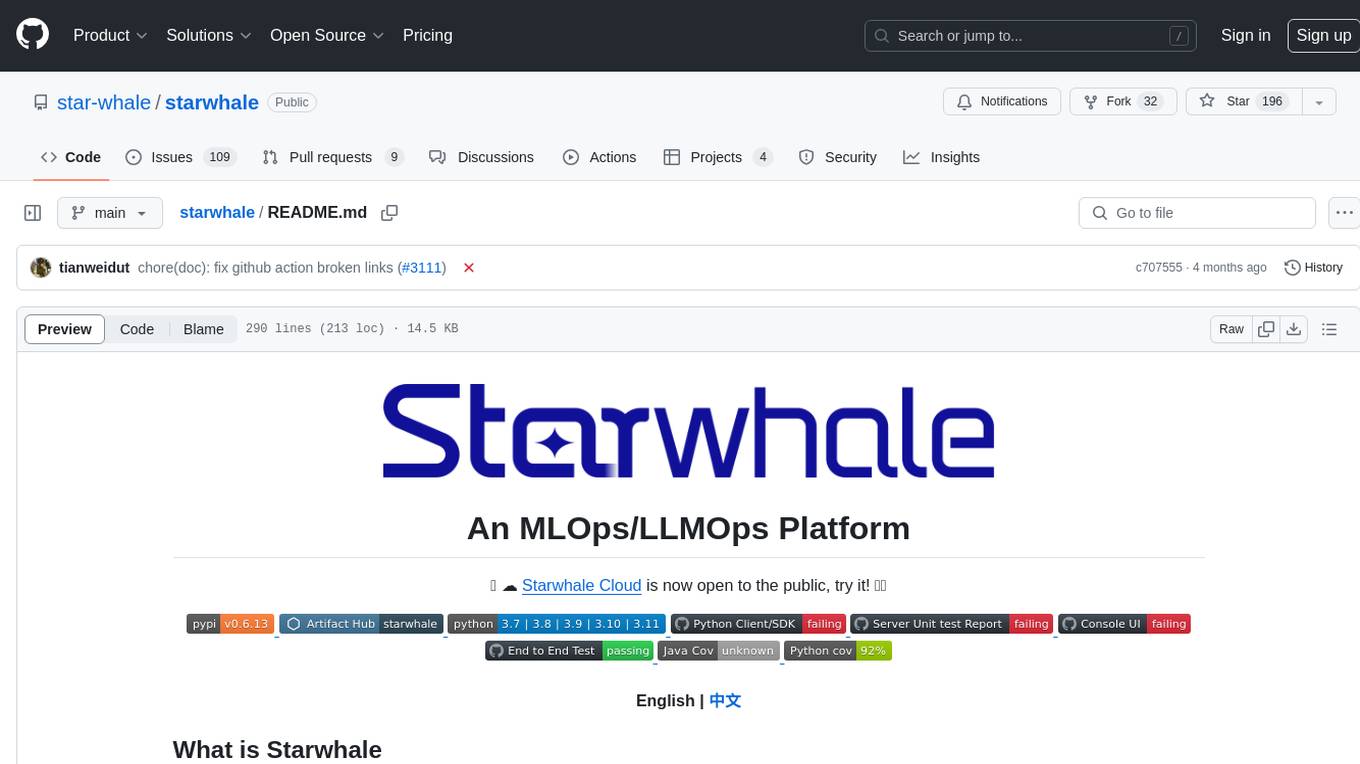
starwhale
Starwhale is an MLOps/LLMOps platform that brings efficiency and standardization to machine learning operations. It streamlines the model development lifecycle, enabling teams to optimize workflows around key areas like model building, evaluation, release, and fine-tuning. Starwhale abstracts Model, Runtime, and Dataset as first-class citizens, providing tailored capabilities for common workflow scenarios including Models Evaluation, Live Demo, and LLM Fine-tuning. It is an open-source platform designed for clarity and ease of use, empowering developers to build customized MLOps features tailored to their needs.
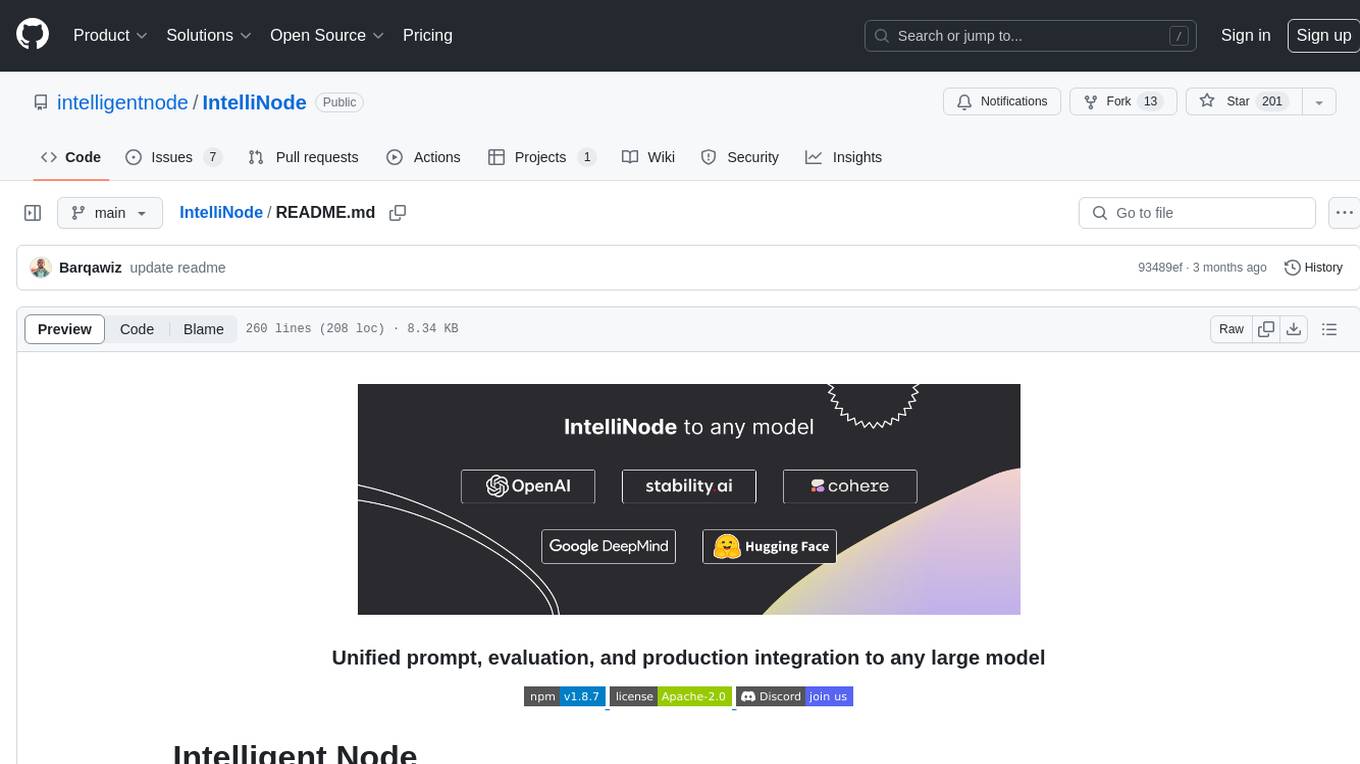
IntelliNode
IntelliNode is a javascript module that integrates cutting-edge AI models like ChatGPT, LLaMA, WaveNet, Gemini, and Stable diffusion into projects. It offers functions for generating text, speech, and images, as well as semantic search, multi-model evaluation, and chatbot capabilities. The module provides a wrapper layer for low-level model access, a controller layer for unified input handling, and a function layer for abstract functionality tailored to various use cases.
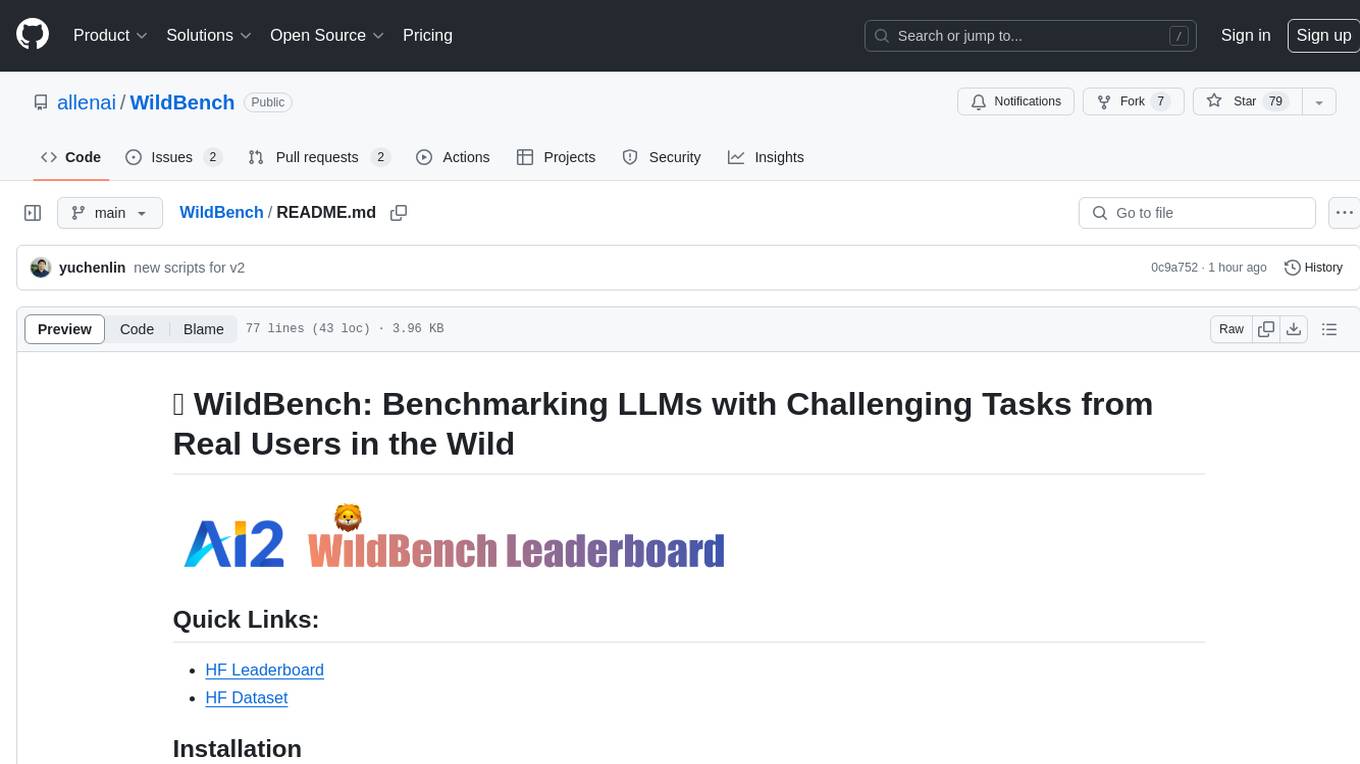
WildBench
WildBench is a tool designed for benchmarking Large Language Models (LLMs) with challenging tasks sourced from real users in the wild. It provides a platform for evaluating the performance of various models on a range of tasks. Users can easily add new models to the benchmark by following the provided guidelines. The tool supports models from Hugging Face and other APIs, allowing for comprehensive evaluation and comparison. WildBench facilitates running inference and evaluation scripts, enabling users to contribute to the benchmark and collaborate on improving model performance.
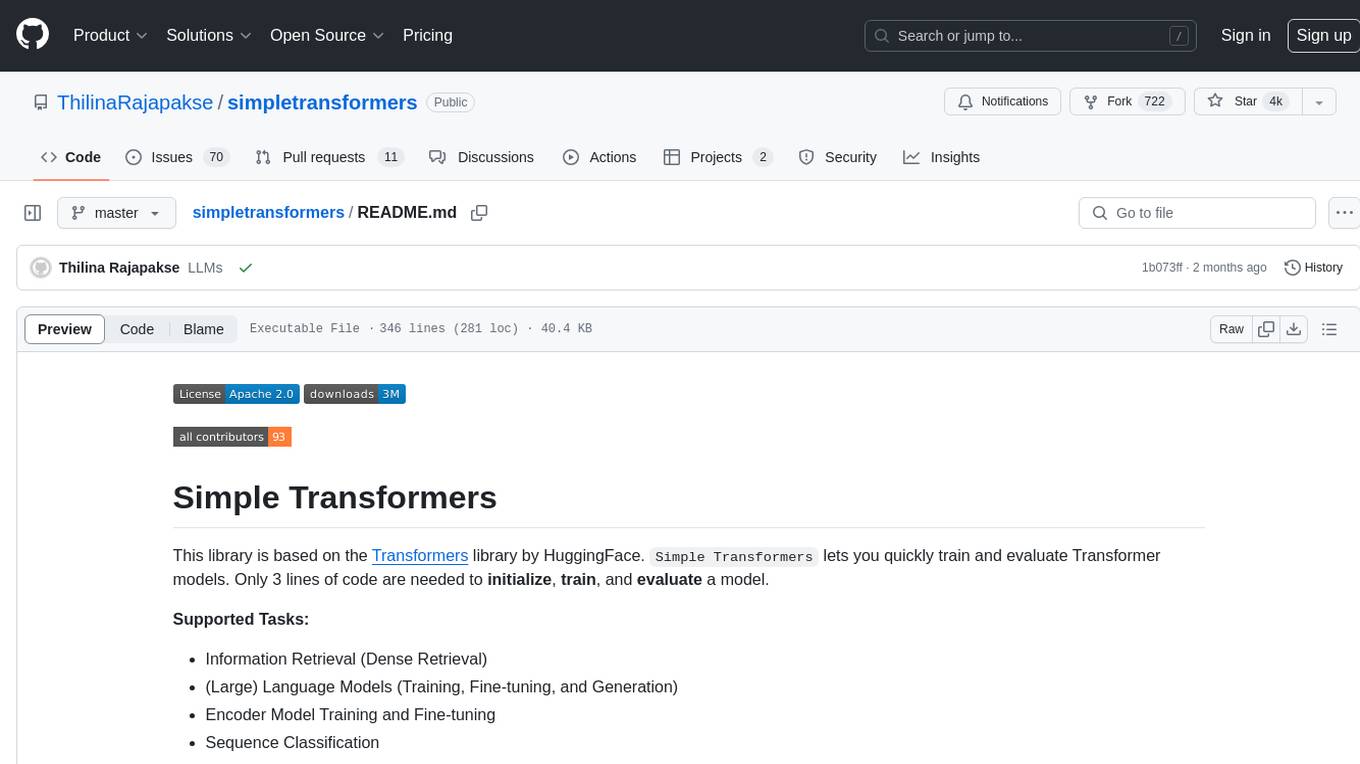
simpletransformers
Simple Transformers is a library based on the Transformers library by HuggingFace, allowing users to quickly train and evaluate Transformer models with only 3 lines of code. It supports various tasks such as Information Retrieval, Language Models, Encoder Model Training, Sequence Classification, Token Classification, Question Answering, Language Generation, T5 Model, Seq2Seq Tasks, Multi-Modal Classification, and Conversational AI.
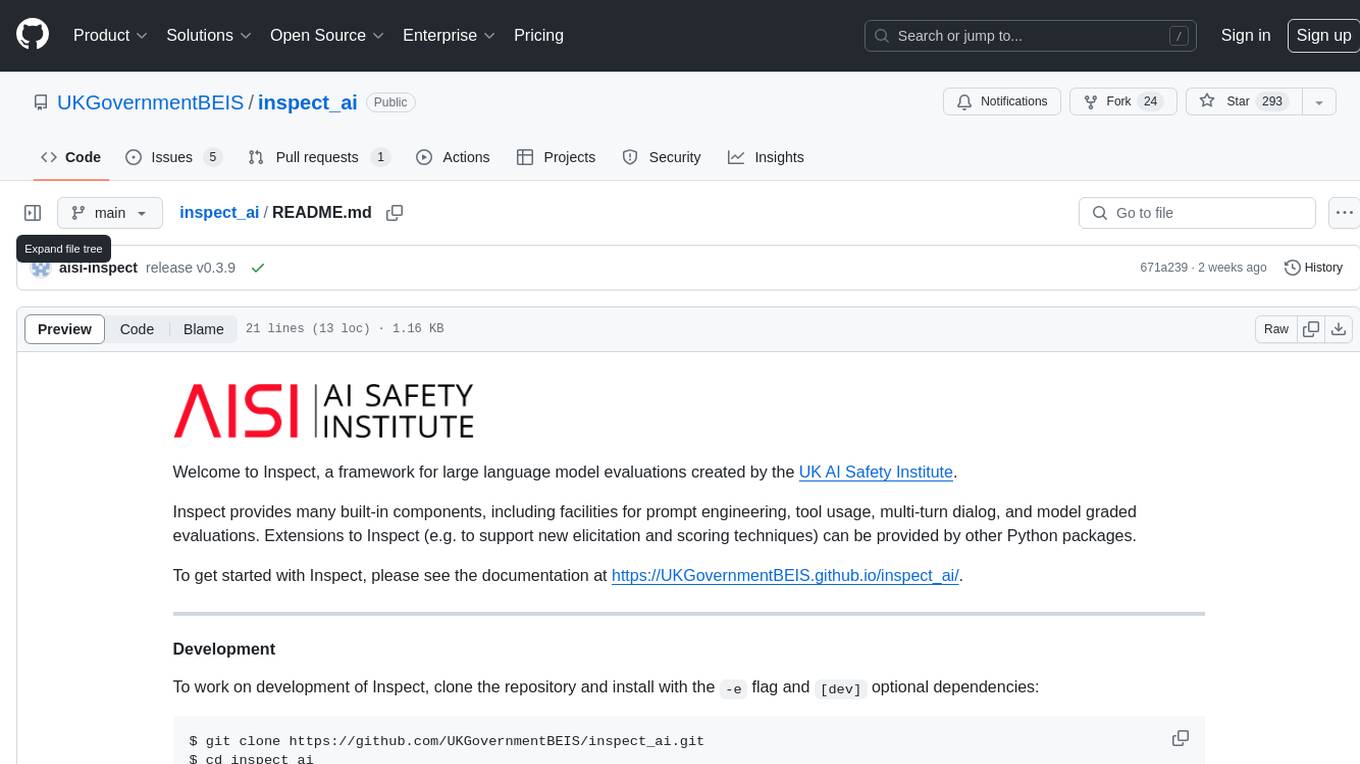
inspect_ai
Inspect AI is a framework developed by the UK AI Safety Institute for evaluating large language models. It offers various built-in components for prompt engineering, tool usage, multi-turn dialog, and model graded evaluations. Users can extend Inspect by adding new elicitation and scoring techniques through additional Python packages. The tool aims to provide a comprehensive solution for assessing the performance and safety of language models.
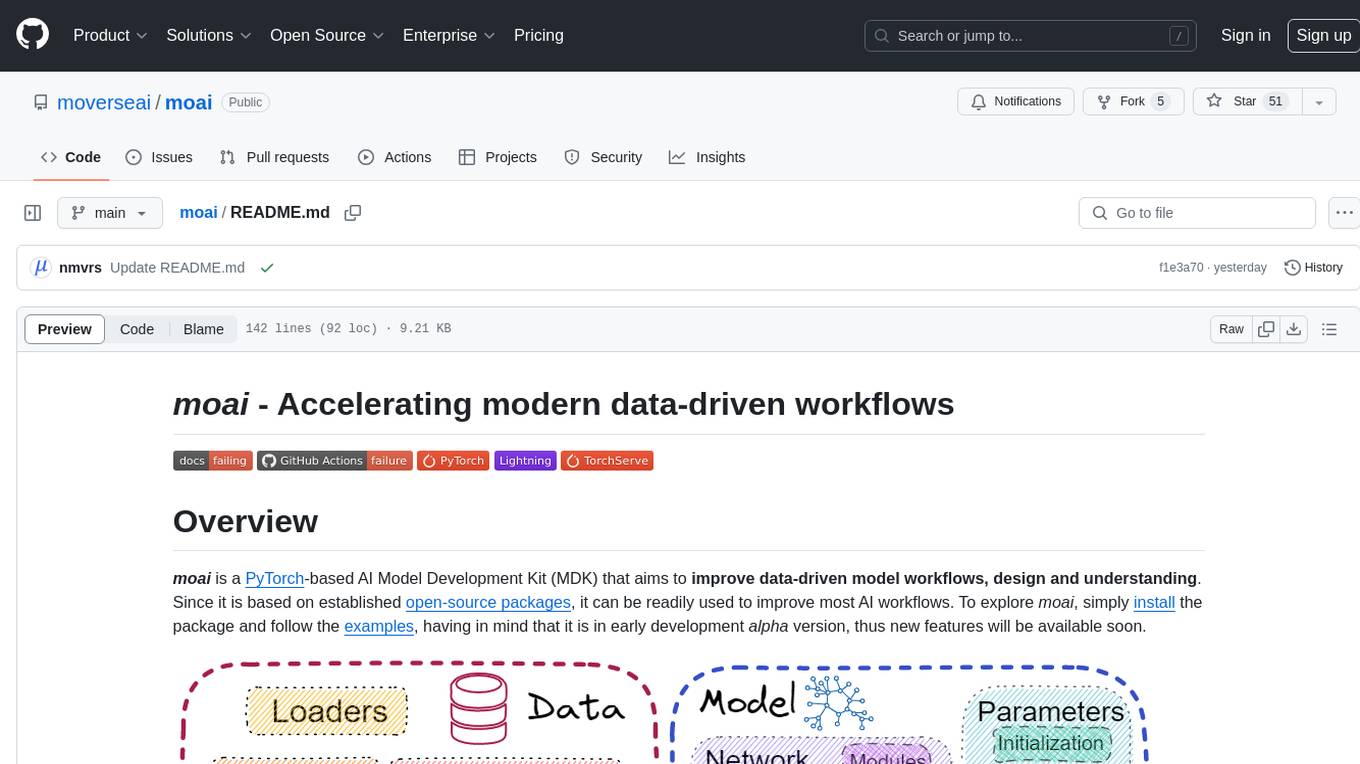
moai
moai is a PyTorch-based AI Model Development Kit (MDK) designed to improve data-driven model workflows, design, and understanding. It offers modularity via monads for model building blocks, reproducibility via configuration-based design, productivity via a data-driven domain modelling language (DML), extensibility via plugins, and understanding via inter-model performance and design aggregation. The tool provides specific integrated actions like play, train, evaluate, plot, diff, and reprod to support heavy data-driven workflows with analytics, knowledge extraction, and reproduction. moai relies on PyTorch, Lightning, Hydra, TorchServe, ONNX, Visdom, HiPlot, Kornia, Albumentations, and the wider open-source community for its functionalities.
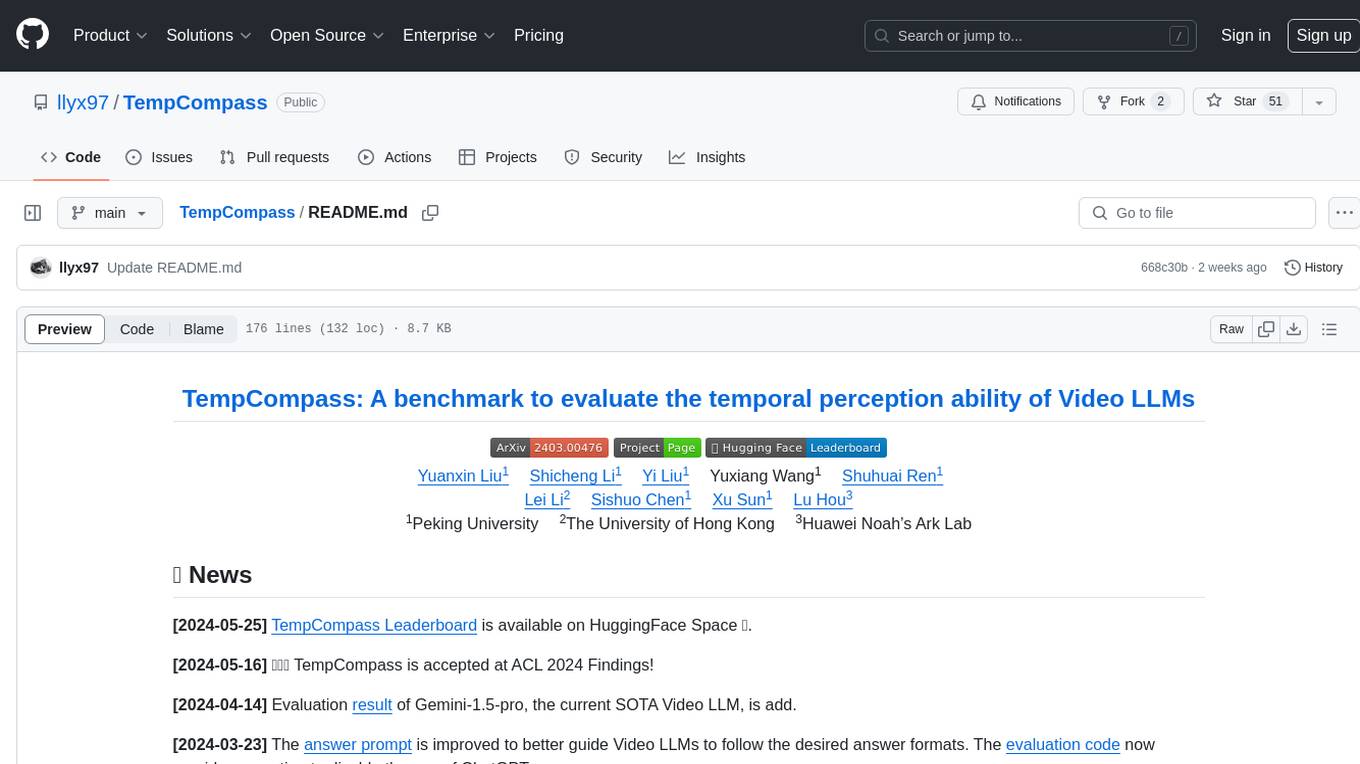
TempCompass
TempCompass is a benchmark designed to evaluate the temporal perception ability of Video LLMs. It encompasses a diverse set of temporal aspects and task formats to comprehensively assess the capability of Video LLMs in understanding videos. The benchmark includes conflicting videos to prevent models from relying on single-frame bias and language priors. Users can clone the repository, install required packages, prepare data, run inference using examples like Video-LLaVA and Gemini, and evaluate the performance of their models across different tasks such as Multi-Choice QA, Yes/No QA, Caption Matching, and Caption Generation.
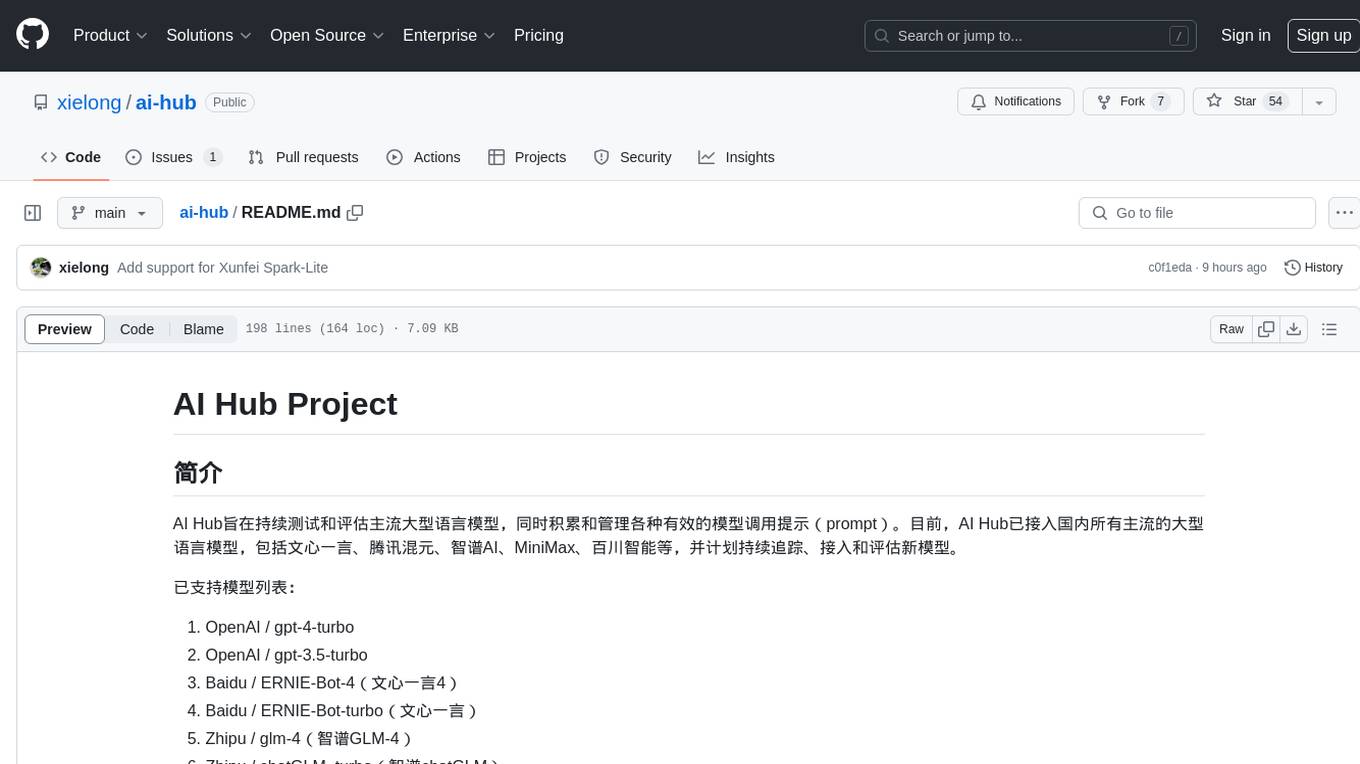
ai-hub
AI Hub Project aims to continuously test and evaluate mainstream large language models, while accumulating and managing various effective model invocation prompts. It has integrated all mainstream large language models in China, including OpenAI GPT-4 Turbo, Baidu ERNIE-Bot-4, Tencent ChatPro, MiniMax abab5.5-chat, and more. The project plans to continuously track, integrate, and evaluate new models. Users can access the models through REST services or Java code integration. The project also provides a testing suite for translation, coding, and benchmark testing.
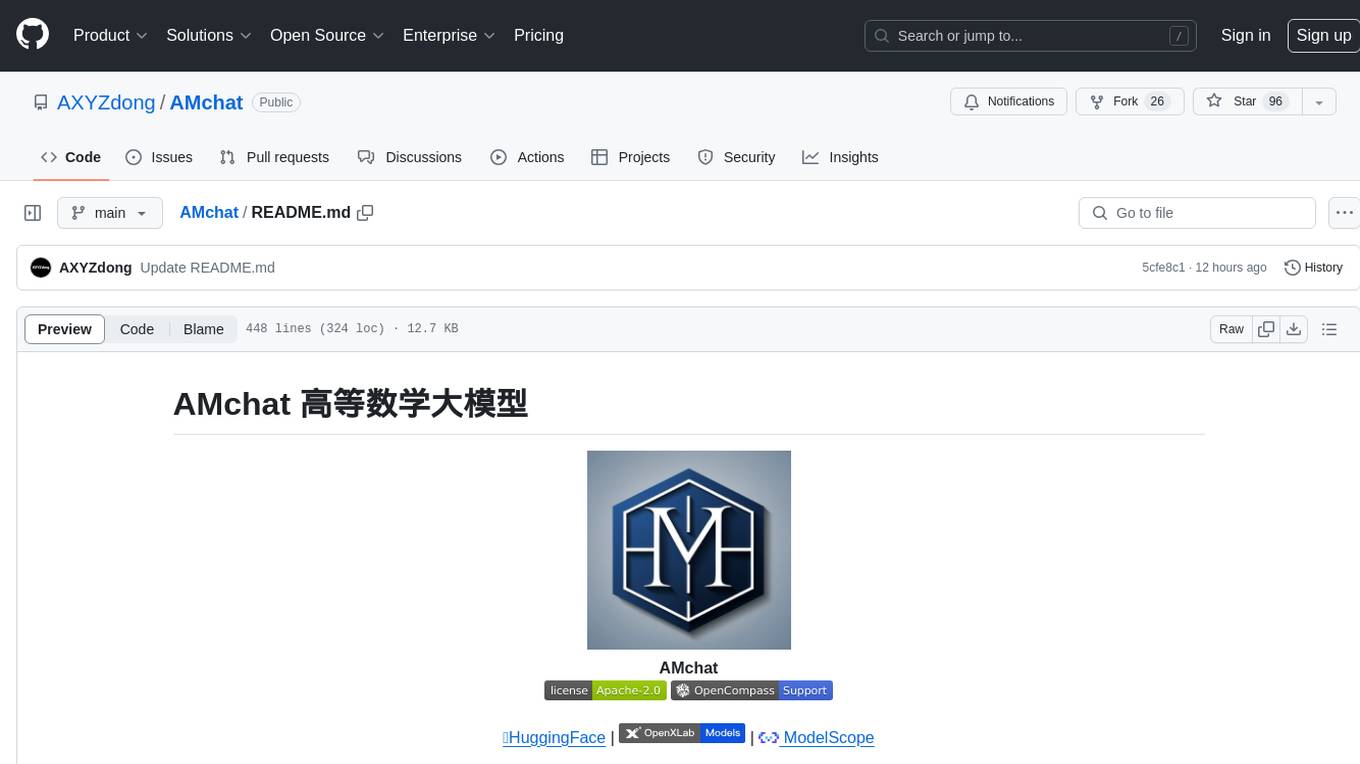
AMchat
AMchat is a large language model that integrates advanced math concepts, exercises, and solutions. The model is based on the InternLM2-Math-7B model and is specifically designed to answer advanced math problems. It provides a comprehensive dataset that combines Math and advanced math exercises and solutions. Users can download the model from ModelScope or OpenXLab, deploy it locally or using Docker, and even retrain it using XTuner for fine-tuning. The tool also supports LMDeploy for quantization, OpenCompass for evaluation, and various other features for model deployment and evaluation. The project contributors have provided detailed documentation and guides for users to utilize the tool effectively.
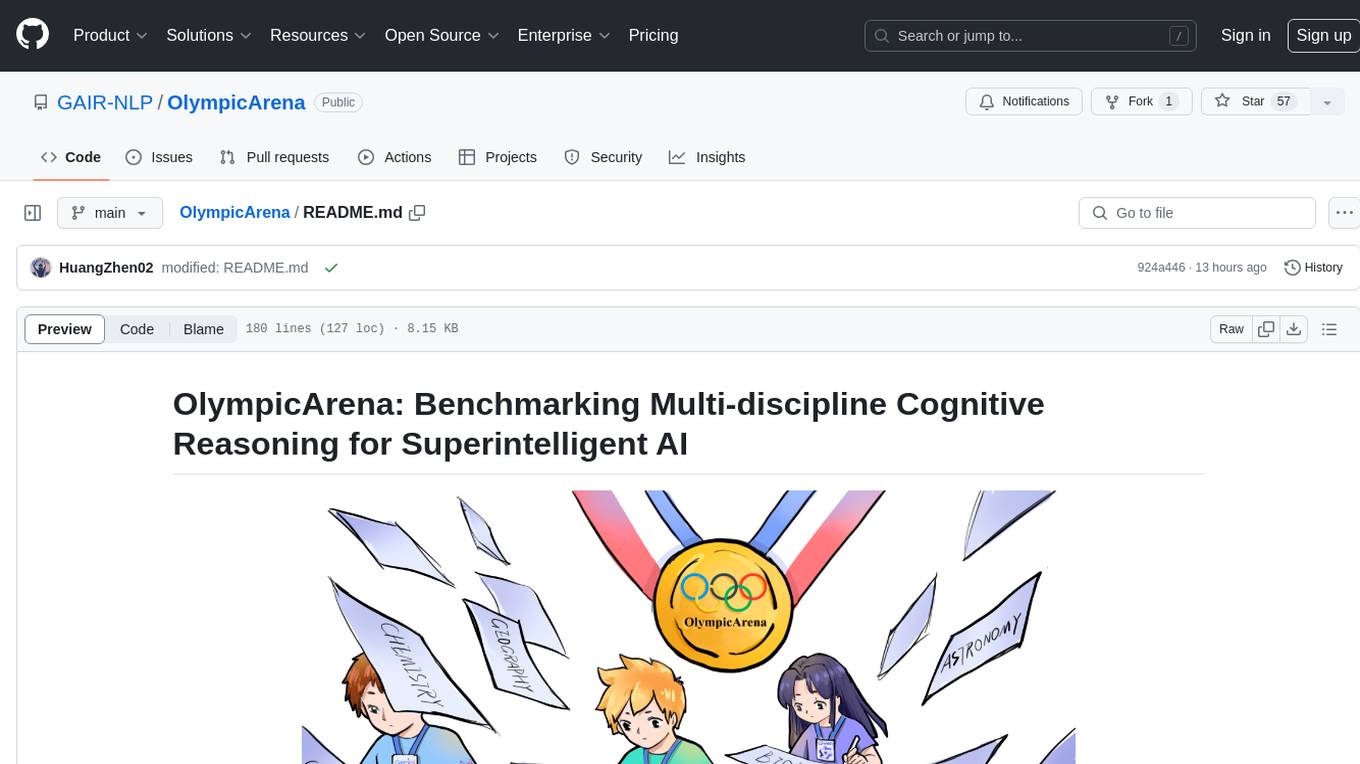
OlympicArena
OlympicArena is a comprehensive benchmark designed to evaluate advanced AI capabilities across various disciplines. It aims to push AI towards superintelligence by tackling complex challenges in science and beyond. The repository provides detailed data for different disciplines, allows users to run inference and evaluation locally, and offers a submission platform for testing models on the test set. Additionally, it includes an annotation interface and encourages users to cite their paper if they find the code or dataset helpful.
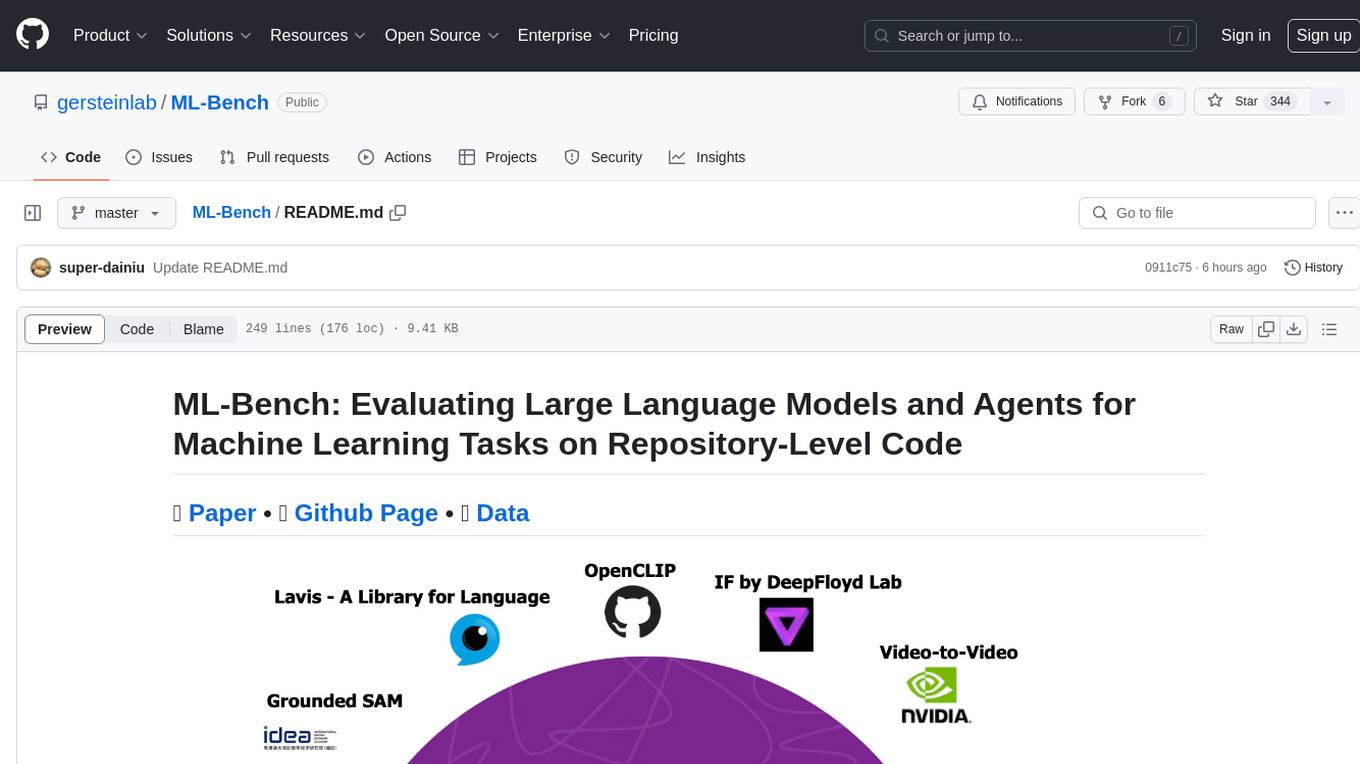
ML-Bench
ML-Bench is a tool designed to evaluate large language models and agents for machine learning tasks on repository-level code. It provides functionalities for data preparation, environment setup, usage, API calling, open source model fine-tuning, and inference. Users can clone the repository, load datasets, run ML-LLM-Bench, prepare data, fine-tune models, and perform inference tasks. The tool aims to facilitate the evaluation of language models and agents in the context of machine learning tasks on code repositories.
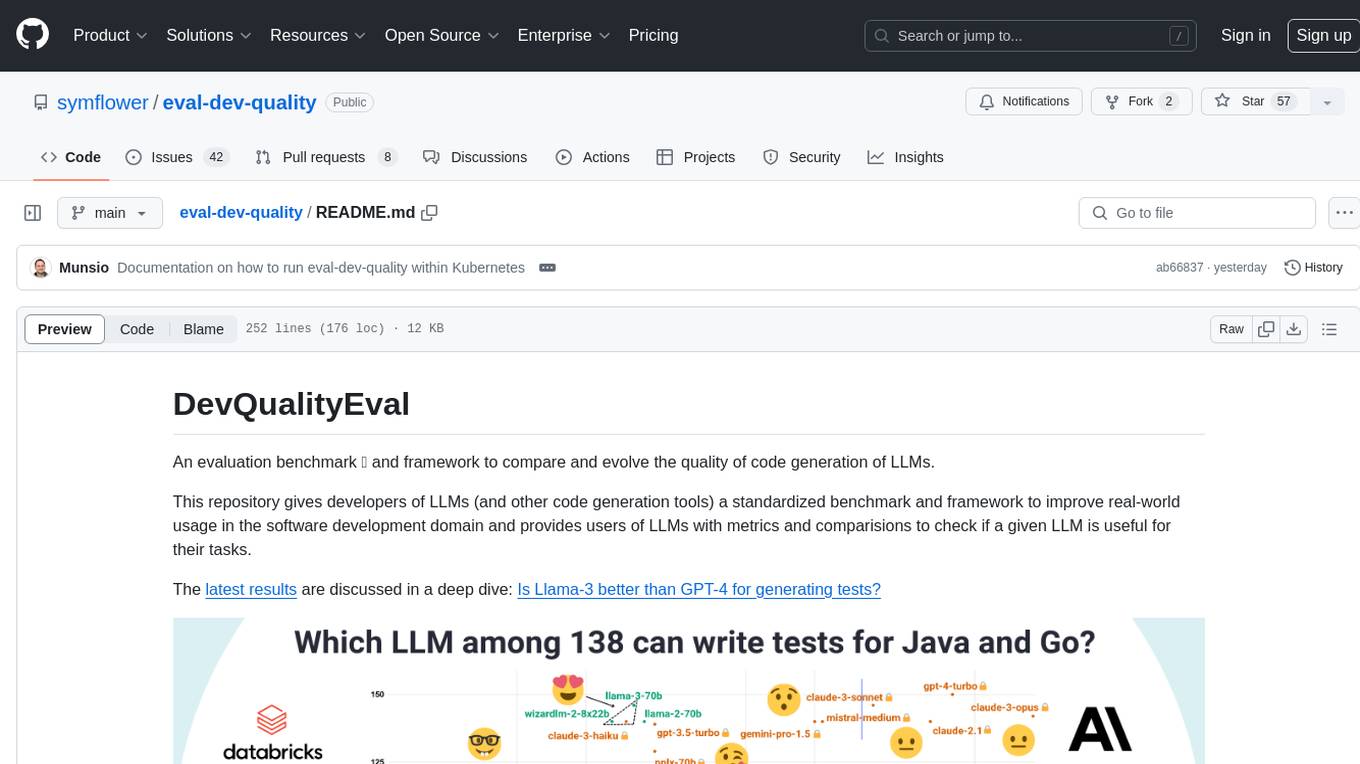
eval-dev-quality
DevQualityEval is an evaluation benchmark and framework designed to compare and improve the quality of code generation of Language Model Models (LLMs). It provides developers with a standardized benchmark to enhance real-world usage in software development and offers users metrics and comparisons to assess the usefulness of LLMs for their tasks. The tool evaluates LLMs' performance in solving software development tasks and measures the quality of their results through a point-based system. Users can run specific tasks, such as test generation, across different programming languages to evaluate LLMs' language understanding and code generation capabilities.
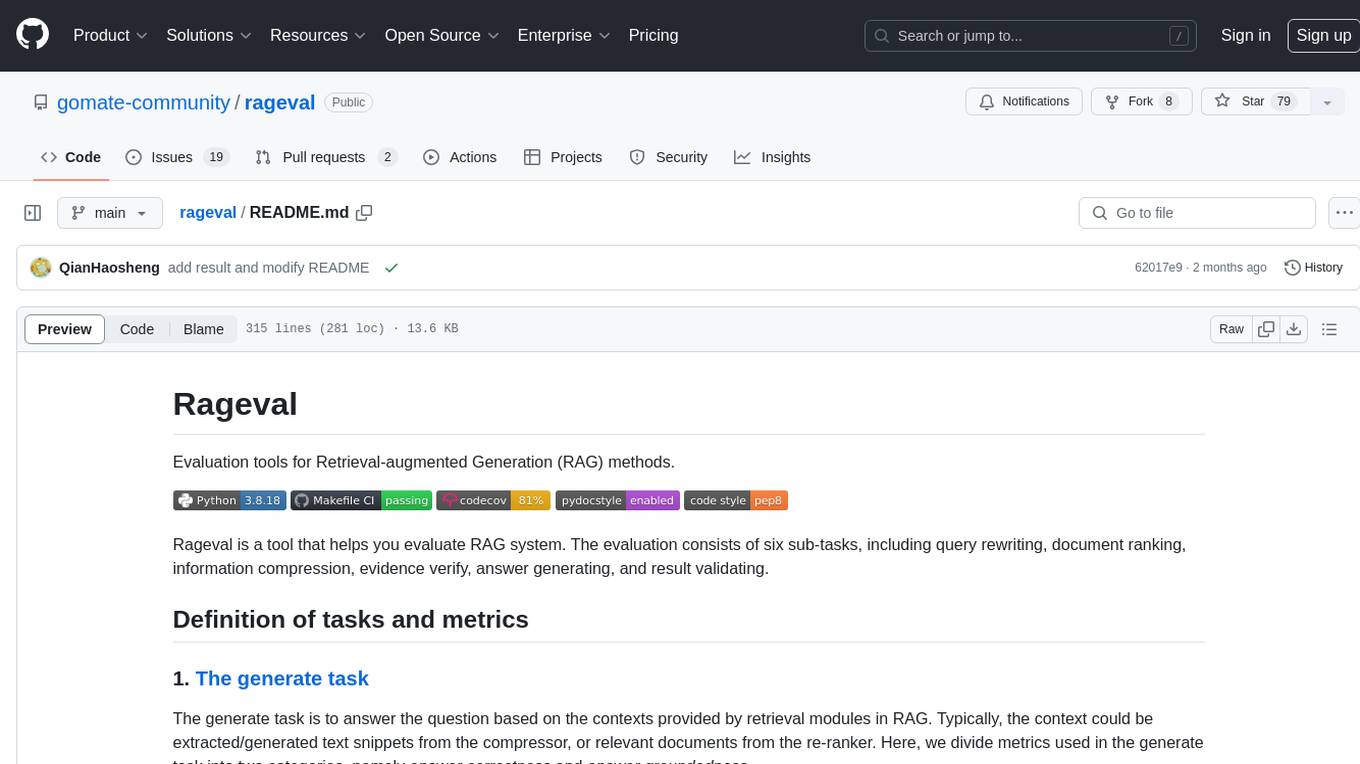
rageval
Rageval is an evaluation tool for Retrieval-augmented Generation (RAG) methods. It helps evaluate RAG systems by performing tasks such as query rewriting, document ranking, information compression, evidence verification, answer generation, and result validation. The tool provides metrics for answer correctness and answer groundedness, along with benchmark results for ASQA and ALCE datasets. Users can install and use Rageval to assess the performance of RAG models in question-answering tasks.
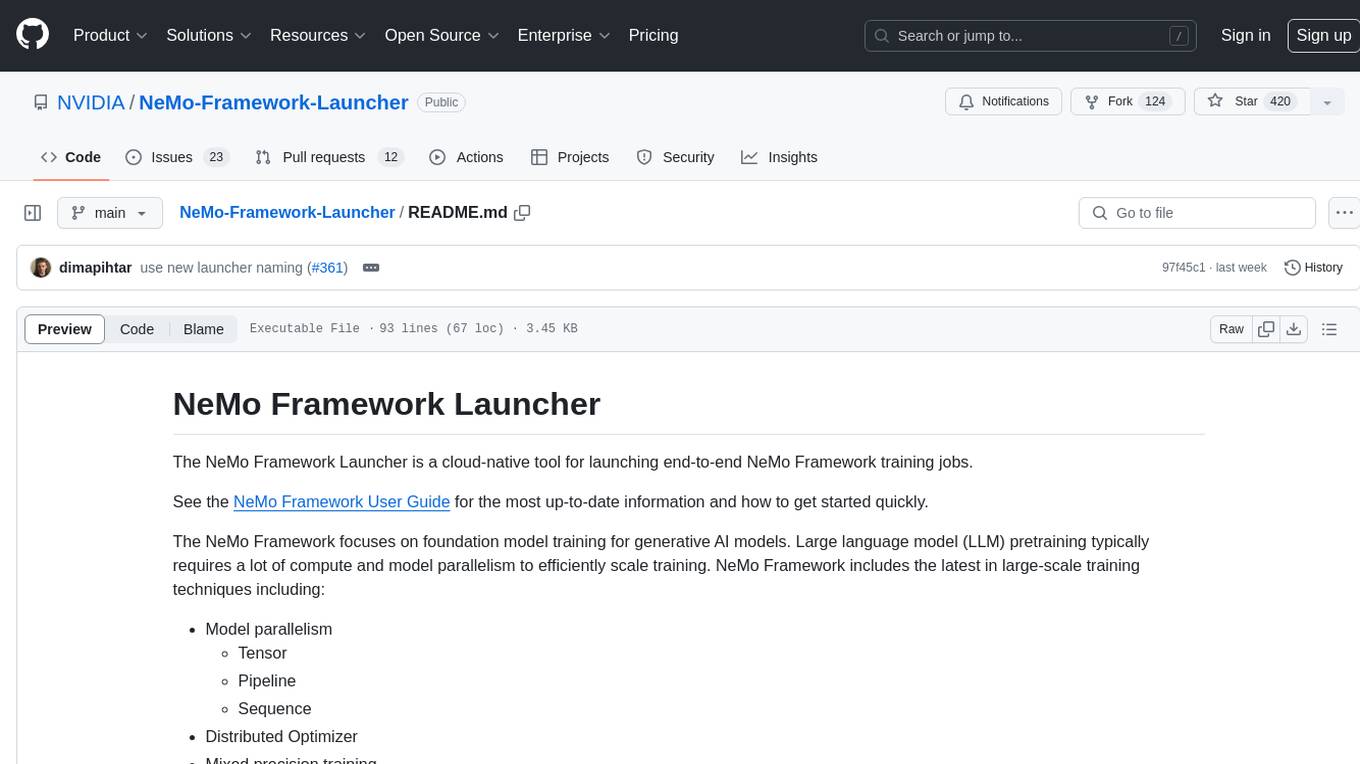
NeMo-Framework-Launcher
The NeMo Framework Launcher is a cloud-native tool designed for launching end-to-end NeMo Framework training jobs. It focuses on foundation model training for generative AI models, supporting large language model pretraining with techniques like model parallelism, tensor, pipeline, sequence, distributed optimizer, mixed precision training, and more. The tool scales to thousands of GPUs and can be used for training LLMs on trillions of tokens. It simplifies launching training jobs on cloud service providers or on-prem clusters, generating submission scripts, organizing job results, and supporting various model operations like fine-tuning, evaluation, export, and deployment.
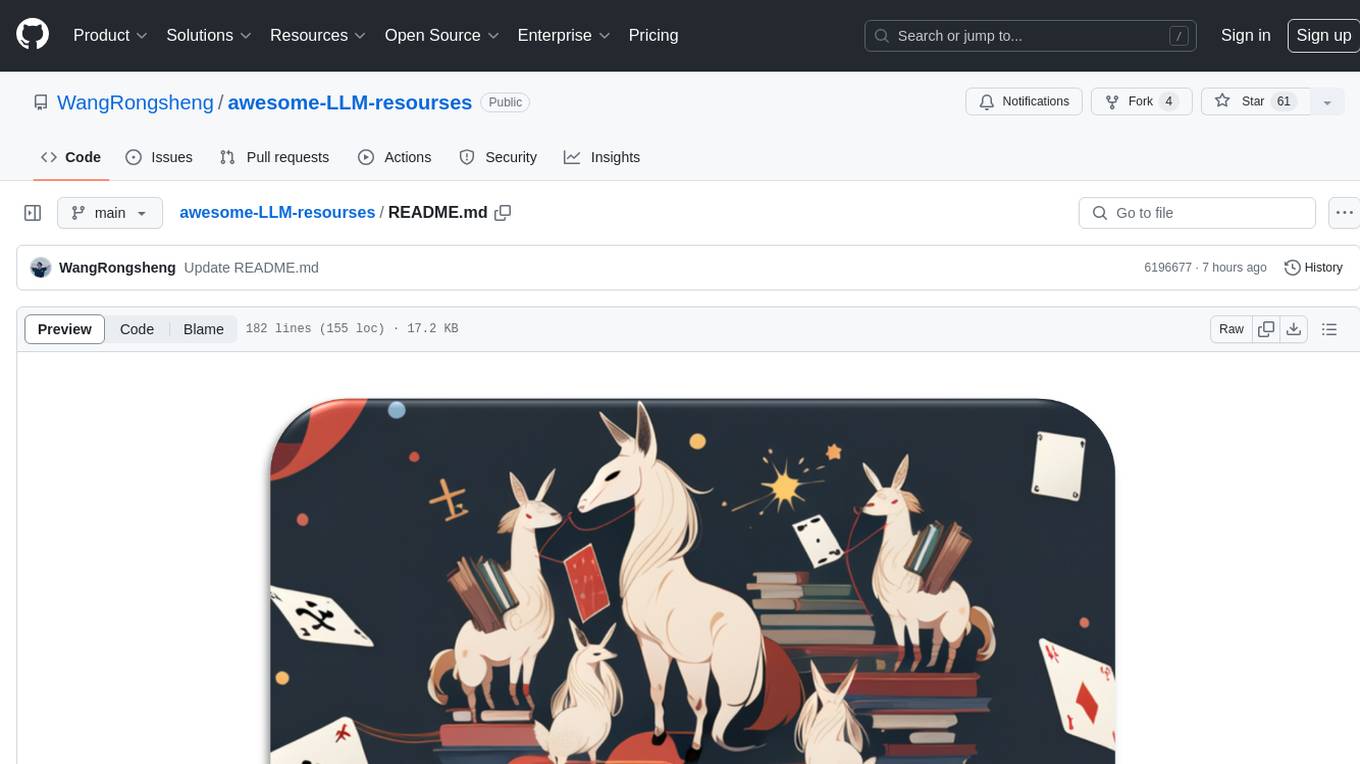
awesome-LLM-resourses
A comprehensive repository of resources for Chinese large language models (LLMs), including data processing tools, fine-tuning frameworks, inference libraries, evaluation platforms, RAG engines, agent frameworks, books, courses, tutorials, and tips. The repository covers a wide range of tools and resources for working with LLMs, from data labeling and processing to model fine-tuning, inference, evaluation, and application development. It also includes resources for learning about LLMs through books, courses, and tutorials, as well as insights and strategies from building with LLMs.
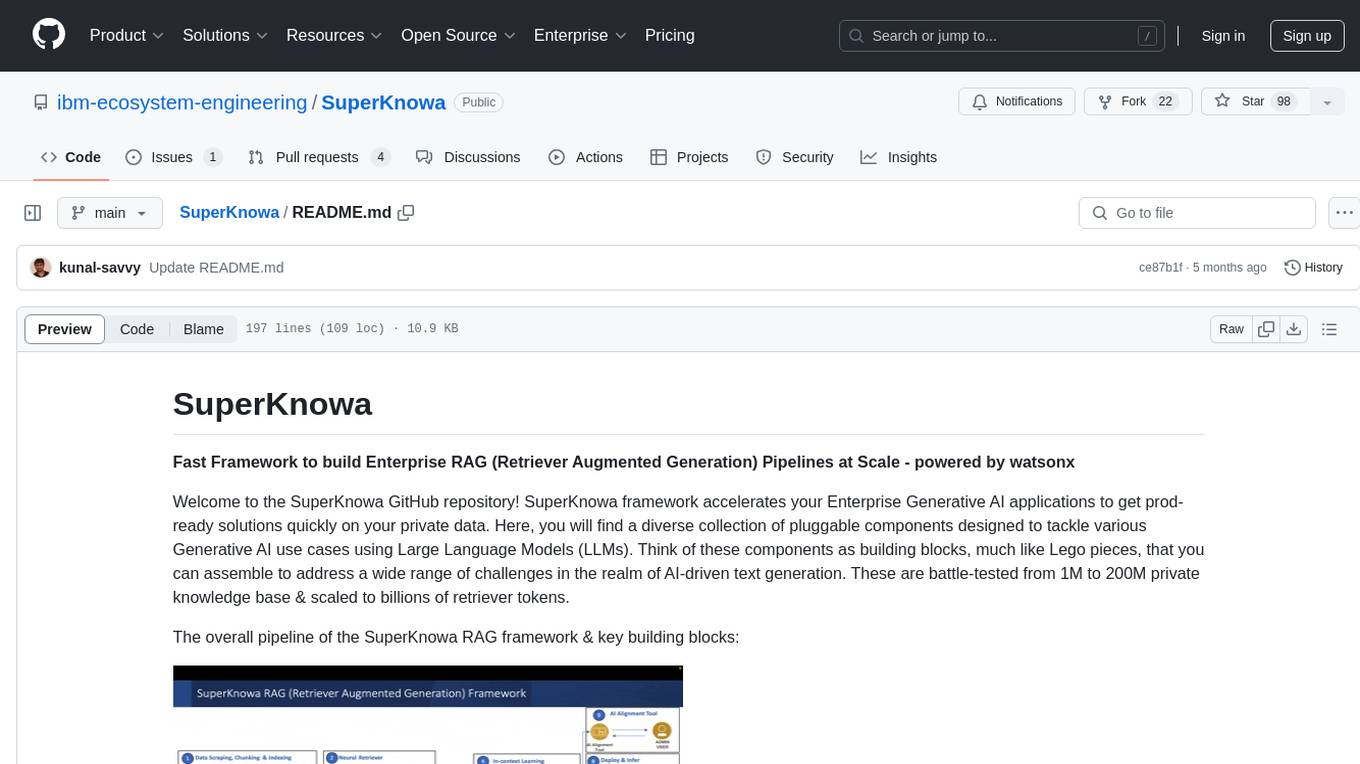
SuperKnowa
SuperKnowa is a fast framework to build Enterprise RAG (Retriever Augmented Generation) Pipelines at Scale, powered by watsonx. It accelerates Enterprise Generative AI applications to get prod-ready solutions quickly on private data. The framework provides pluggable components for tackling various Generative AI use cases using Large Language Models (LLMs), allowing users to assemble building blocks to address challenges in AI-driven text generation. SuperKnowa is battle-tested from 1M to 200M private knowledge base & scaled to billions of retriever tokens.
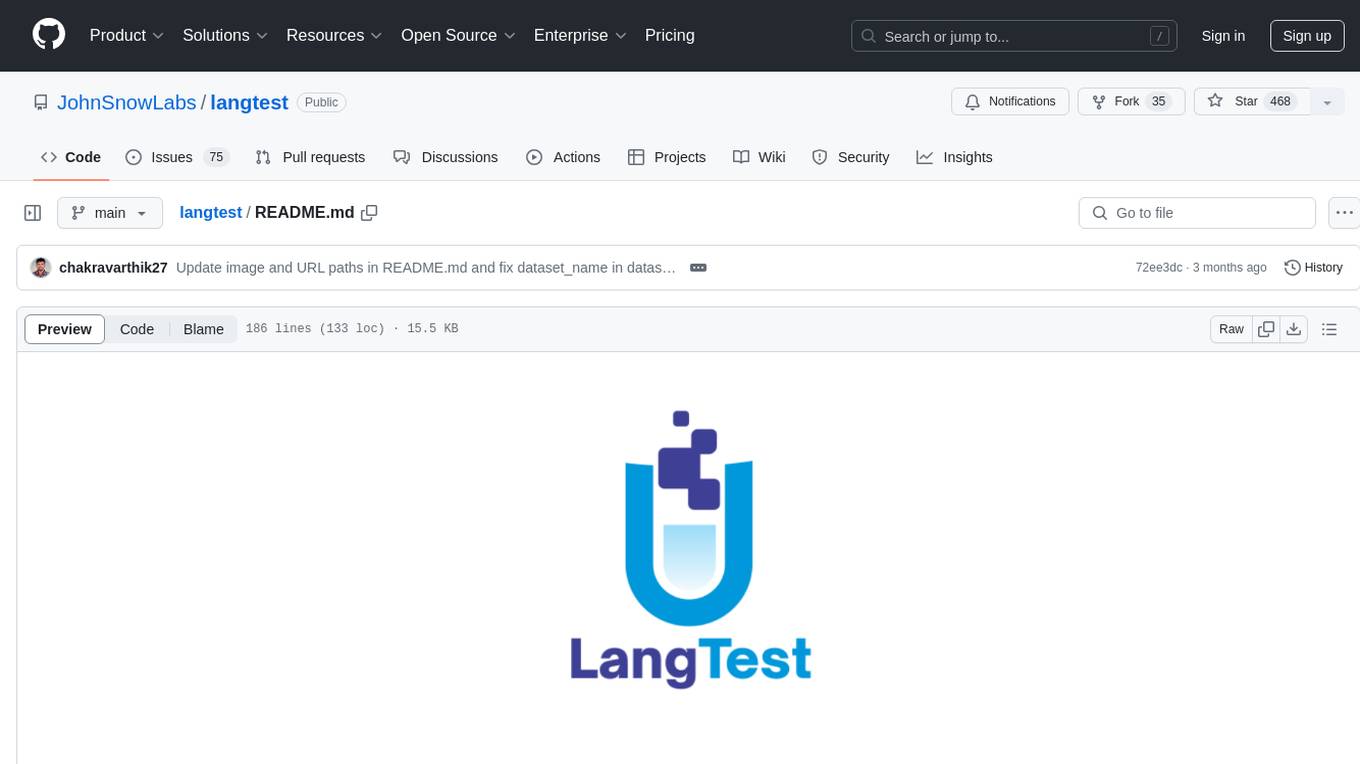
langtest
LangTest is a comprehensive evaluation library for custom LLM and NLP models. It aims to deliver safe and effective language models by providing tools to test model quality, augment training data, and support popular NLP frameworks. LangTest comes with benchmark datasets to challenge and enhance language models, ensuring peak performance in various linguistic tasks. The tool offers more than 60 distinct types of tests with just one line of code, covering aspects like robustness, bias, representation, fairness, and accuracy. It supports testing LLMS for question answering, toxicity, clinical tests, legal support, factuality, sycophancy, and summarization.
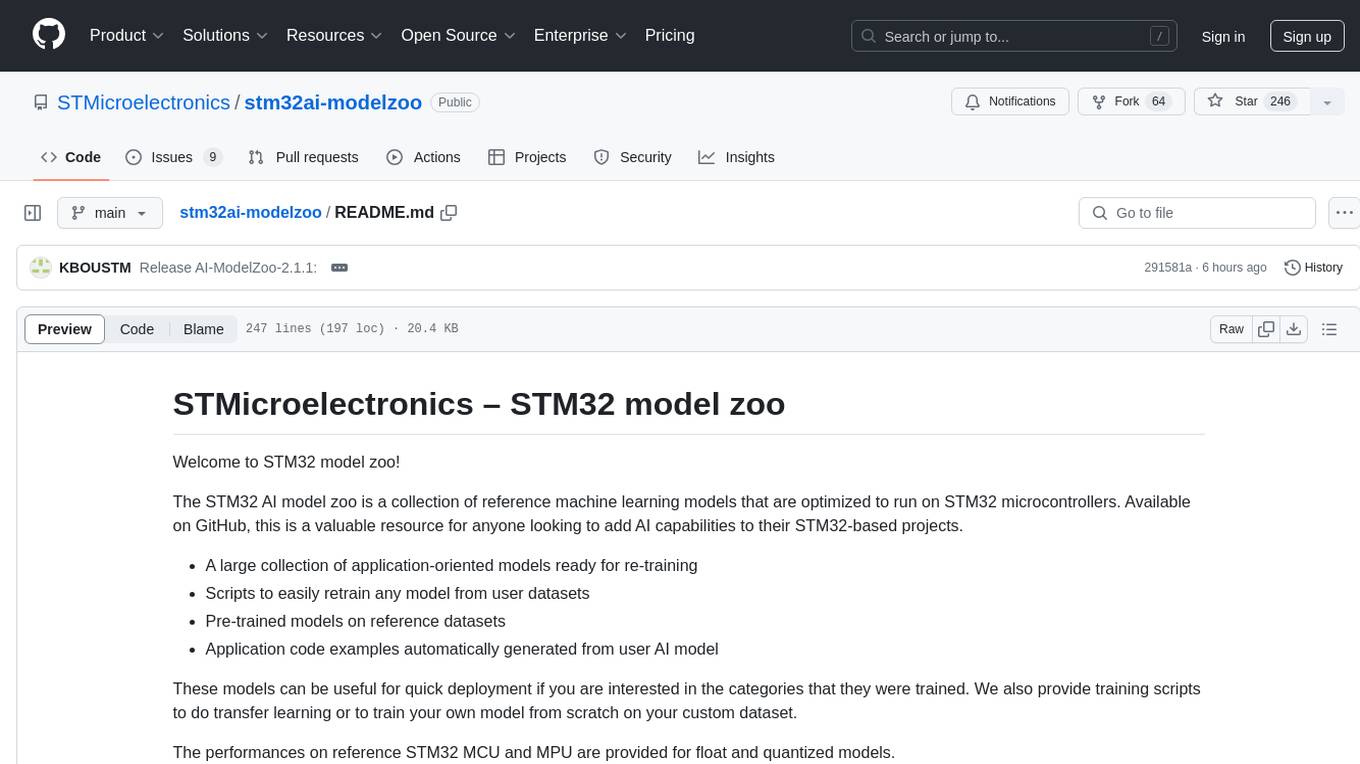
stm32ai-modelzoo
The STM32 AI model zoo is a collection of reference machine learning models optimized to run on STM32 microcontrollers. It provides a large collection of application-oriented models ready for re-training, scripts for easy retraining from user datasets, pre-trained models on reference datasets, and application code examples generated from user AI models. The project offers training scripts for transfer learning or training custom models from scratch. It includes performances on reference STM32 MCU and MPU for float and quantized models. The project is organized by application, providing step-by-step guides for training and deploying models.
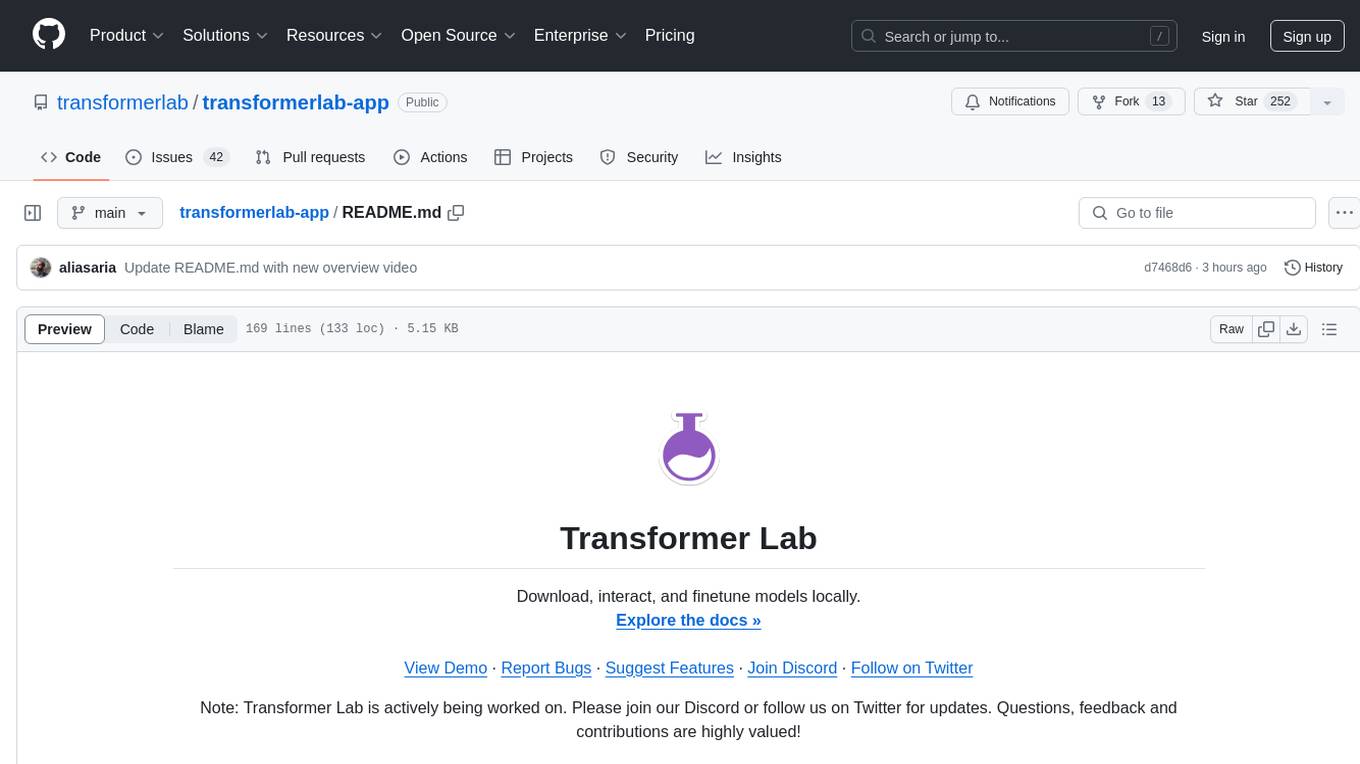
transformerlab-app
Transformer Lab is an app that allows users to experiment with Large Language Models by providing features such as one-click download of popular models, finetuning across different hardware, RLHF and Preference Optimization, working with LLMs across different operating systems, chatting with models, using different inference engines, evaluating models, building datasets for training, calculating embeddings, providing a full REST API, running in the cloud, converting models across platforms, supporting plugins, embedded Monaco code editor, prompt editing, inference logs, all through a simple cross-platform GUI.
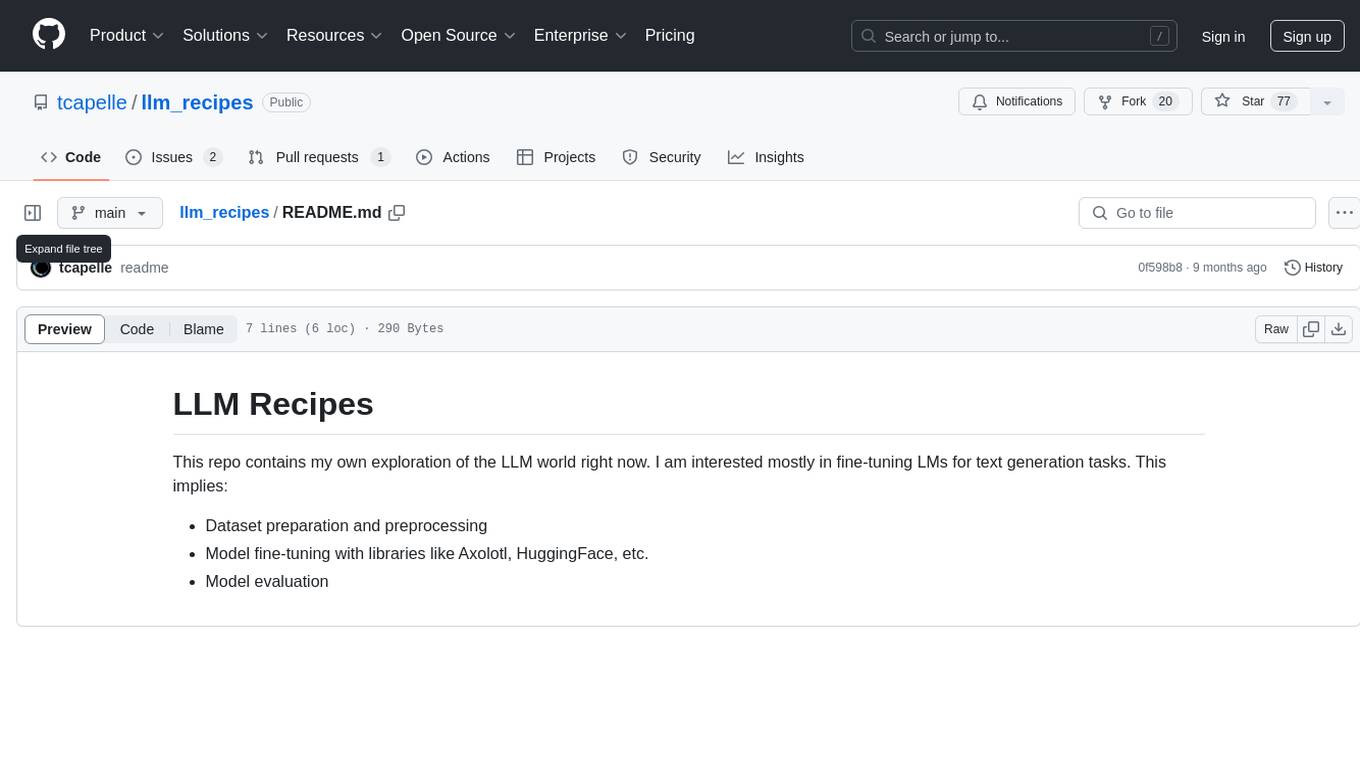
llm_recipes
This repository showcases the author's experiments with Large Language Models (LLMs) for text generation tasks. It includes dataset preparation, preprocessing, model fine-tuning using libraries such as Axolotl and HuggingFace, and model evaluation.
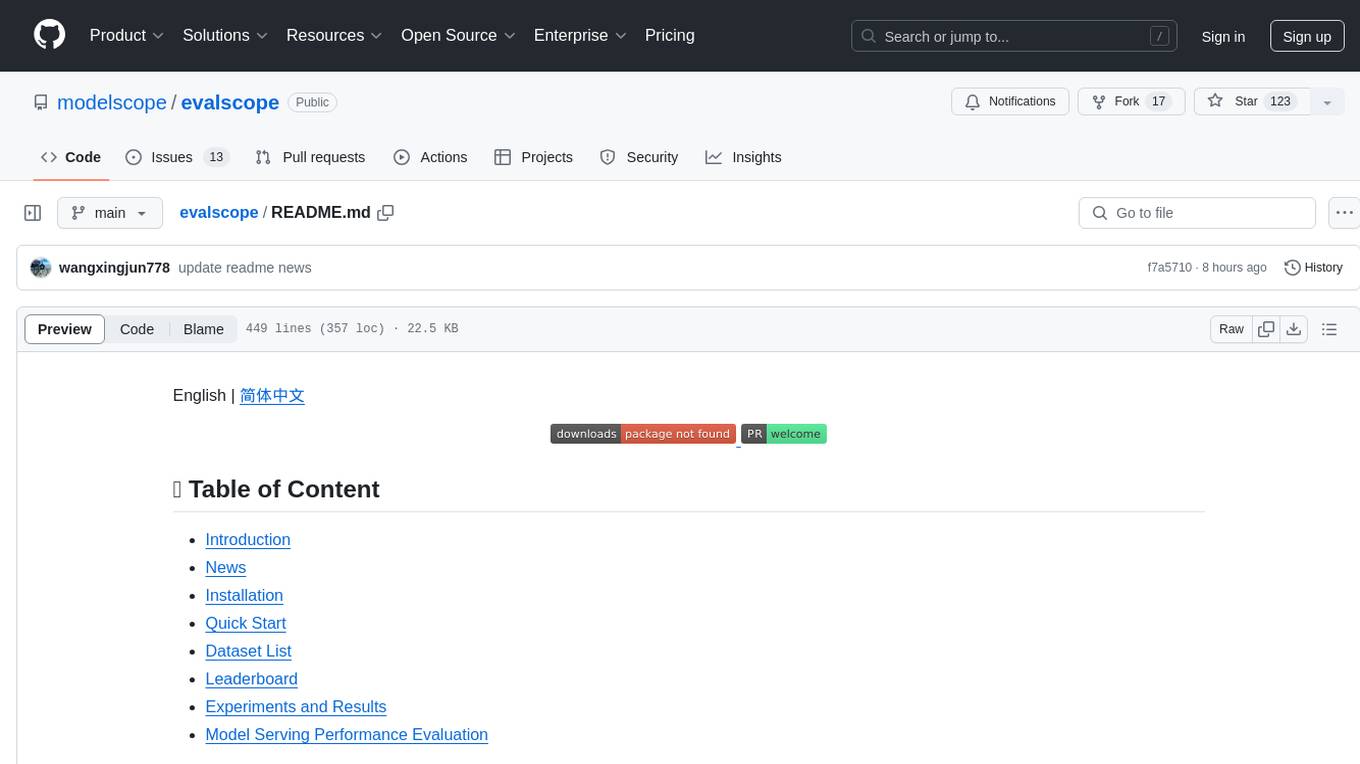
evalscope
Eval-Scope is a framework designed to support the evaluation of large language models (LLMs) by providing pre-configured benchmark datasets, common evaluation metrics, model integration, automatic evaluation for objective questions, complex task evaluation using expert models, reports generation, visualization tools, and model inference performance evaluation. It is lightweight, easy to customize, supports new dataset integration, model hosting on ModelScope, deployment of locally hosted models, and rich evaluation metrics. Eval-Scope also supports various evaluation modes like single mode, pairwise-baseline mode, and pairwise (all) mode, making it suitable for assessing and improving LLMs.
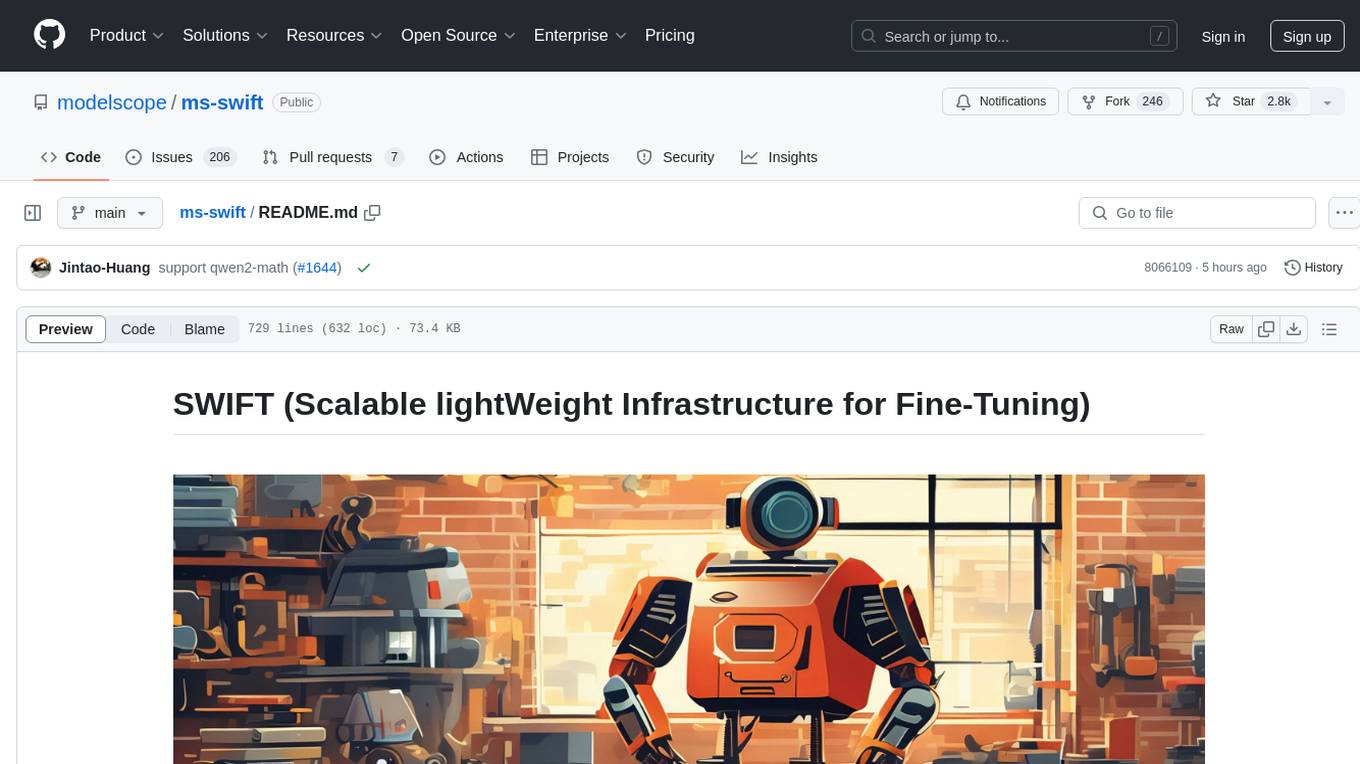
ms-swift
ms-swift is an official framework provided by the ModelScope community for fine-tuning and deploying large language models and multi-modal large models. It supports training, inference, evaluation, quantization, and deployment of over 400 large models and 100+ multi-modal large models. The framework includes various training technologies and accelerates inference, evaluation, and deployment modules. It offers a Gradio-based Web-UI interface and best practices for easy application of large models. ms-swift supports a wide range of model types, dataset types, hardware support, lightweight training methods, distributed training techniques, quantization training, RLHF training, multi-modal training, interface training, plugin and extension support, inference acceleration engines, model evaluation, and model quantization.
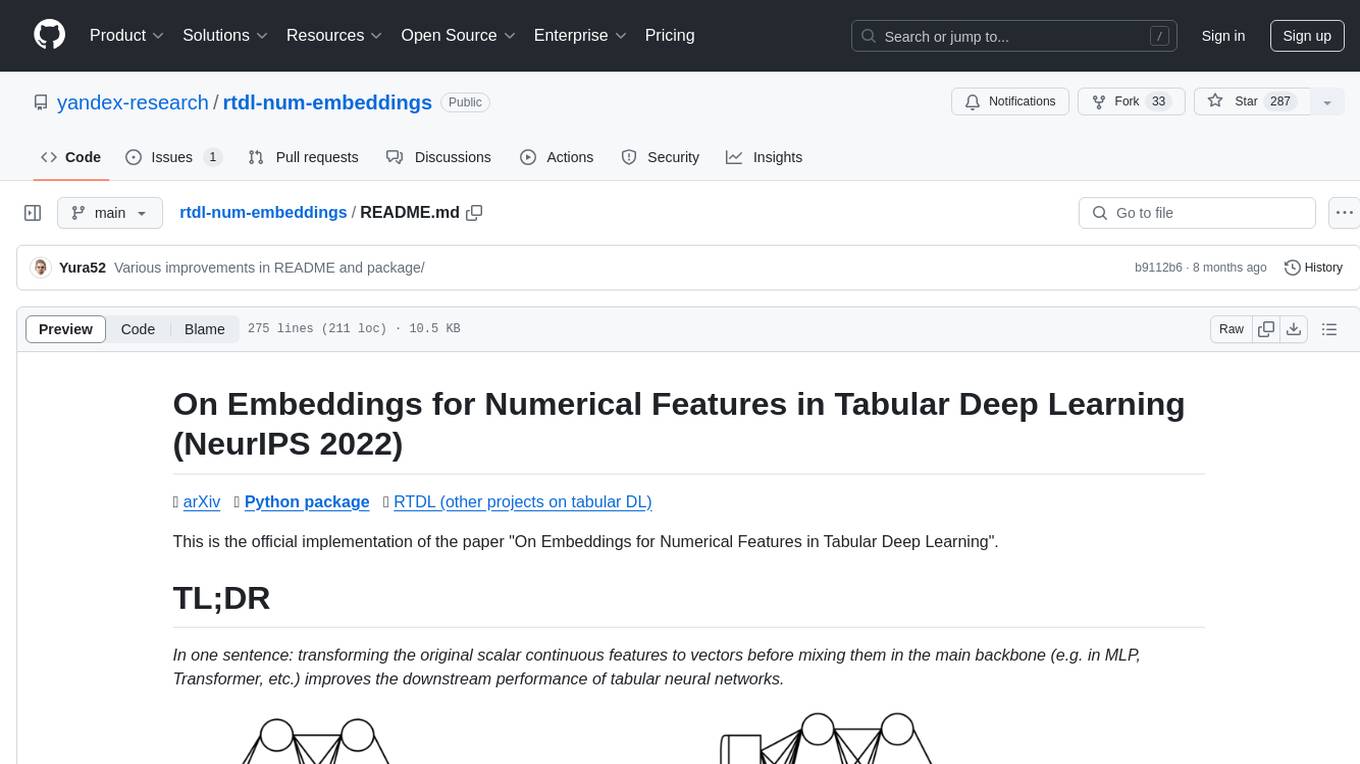
rtdl-num-embeddings
This repository provides the official implementation of the paper 'On Embeddings for Numerical Features in Tabular Deep Learning'. It focuses on transforming scalar continuous features into vectors before integrating them into the main backbone of tabular neural networks, showcasing improved performance. The embeddings for continuous features are shown to enhance the performance of tabular DL models and are applicable to various conventional backbones, offering efficiency comparable to Transformer-based models. The repository includes Python packages for practical usage, exploration of metrics and hyperparameters, and reproducing reported results for different algorithms and datasets.
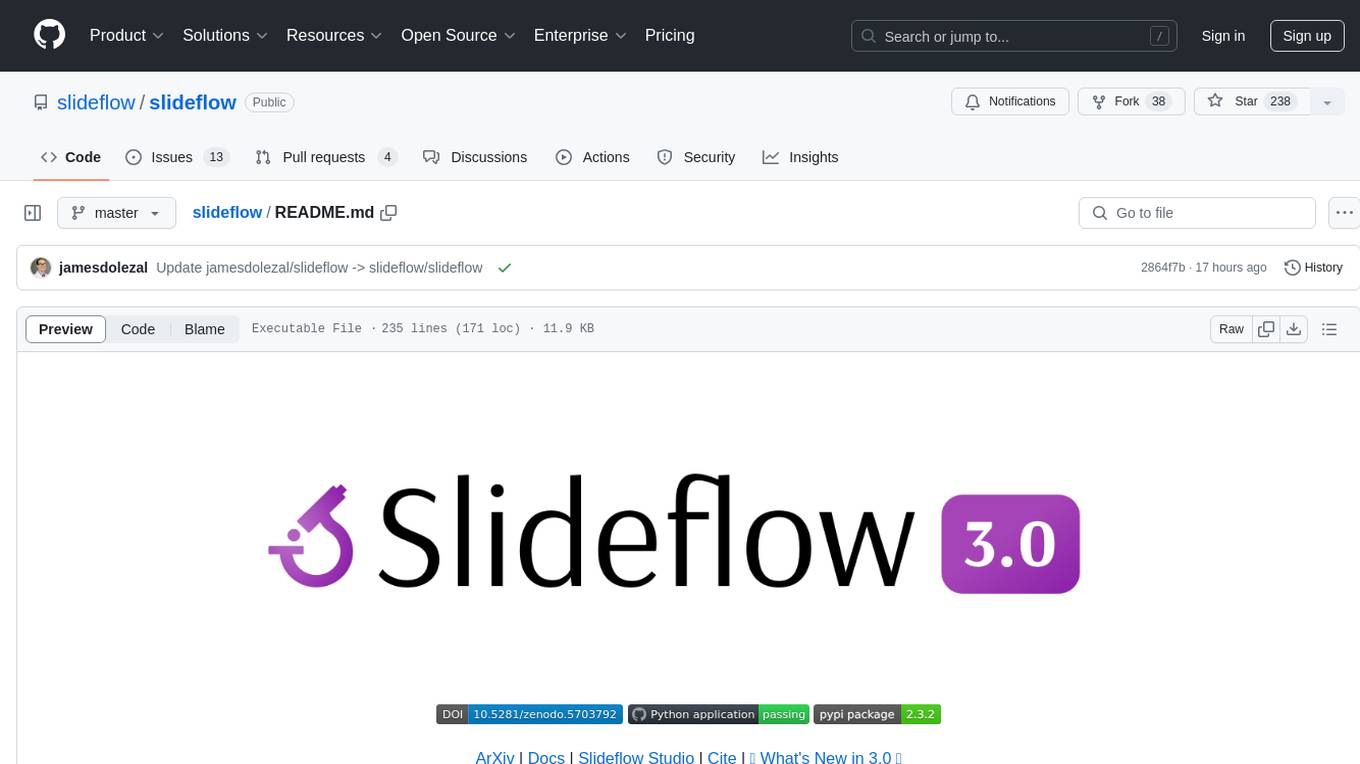
slideflow
Slideflow is a deep learning library for digital pathology, offering a user-friendly interface for model development. It is designed for medical researchers and AI enthusiasts, providing an accessible platform for developing state-of-the-art pathology models. Slideflow offers customizable training pipelines, robust slide processing and stain normalization toolkit, support for weakly-supervised or strongly-supervised labels, built-in foundation models, multiple-instance learning, self-supervised learning, generative adversarial networks, explainability tools, layer activation analysis tools, uncertainty quantification, interactive user interface for model deployment, and more. It supports both PyTorch and Tensorflow, with optional support for Libvips for slide reading. Slideflow can be installed via pip, Docker container, or from source, and includes non-commercial add-ons for additional tools and pretrained models. It allows users to create projects, extract tiles from slides, train models, and provides evaluation tools like heatmaps and mosaic maps.
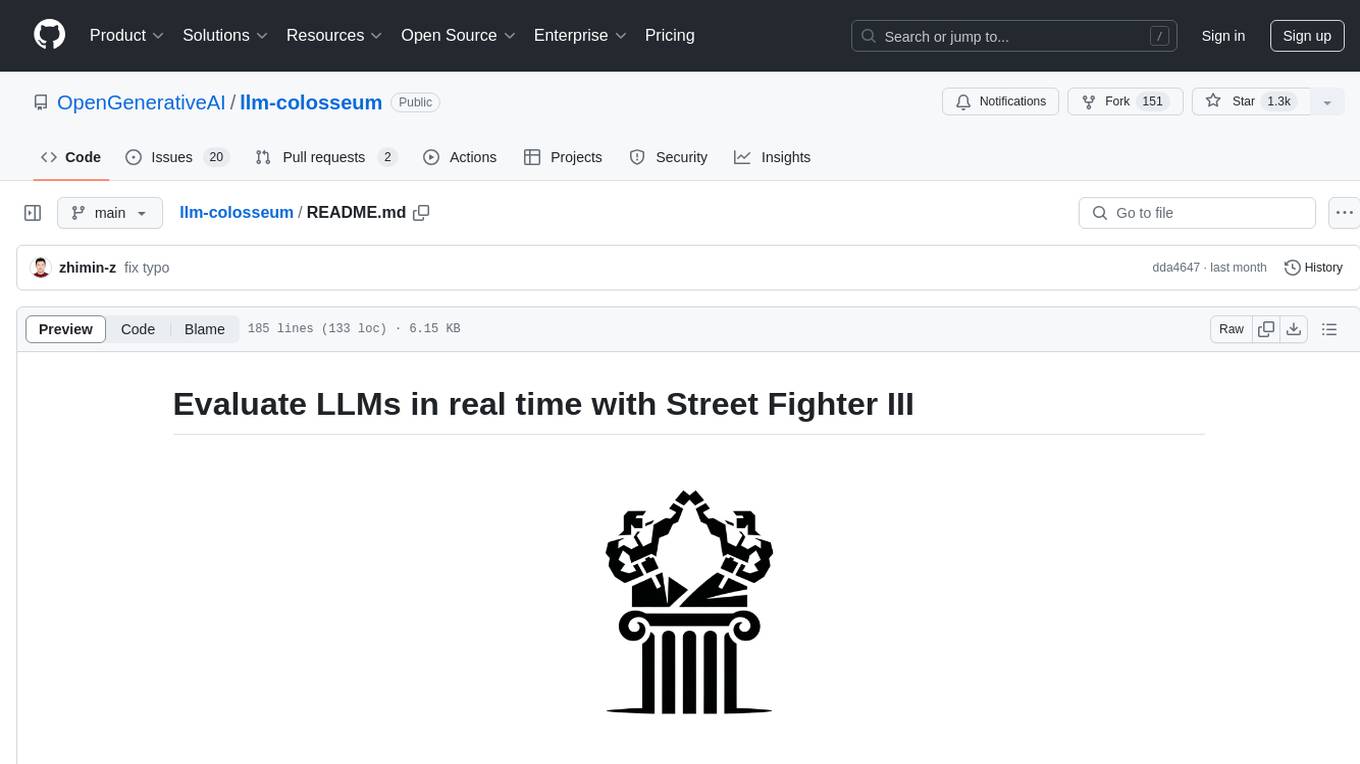
llm-colosseum
llm-colosseum is a tool designed to evaluate Language Model Models (LLMs) in real-time by making them fight each other in Street Fighter III. The tool assesses LLMs based on speed, strategic thinking, adaptability, out-of-the-box thinking, and resilience. It provides a benchmark for LLMs to understand their environment and take context-based actions. Users can analyze the performance of different LLMs through ELO rankings and win rate matrices. The tool allows users to run experiments, test different LLM models, and customize prompts for LLM interactions. It offers installation instructions, test mode options, logging configurations, and the ability to run the tool with local models. Users can also contribute their own LLM models for evaluation and ranking.
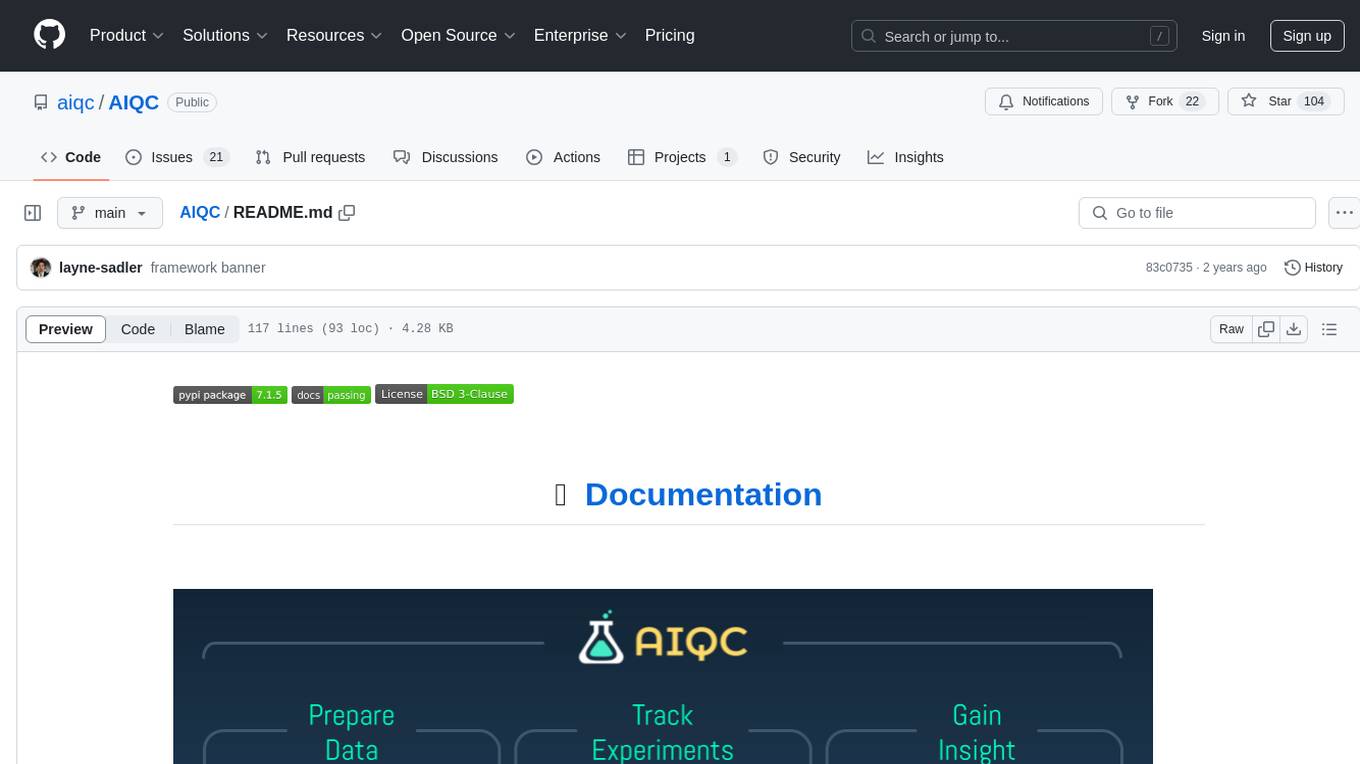
AIQC
AIQC is an open source Python package that provides a declarative API for end-to-end MLOps in order to make deep learning more accessible to researchers. It utilizes a SQLite object-relational model for machine learning objects and stacks standardized workflows for various analyses, data types, and libraries. The benefits include a 90% reduction in data wrangling, reproducibility, and no need to install and maintain application and database servers for experiment tracking. AIQC is pip-installable and provides a Dash-Plotly UI for real-time experiment tracking.
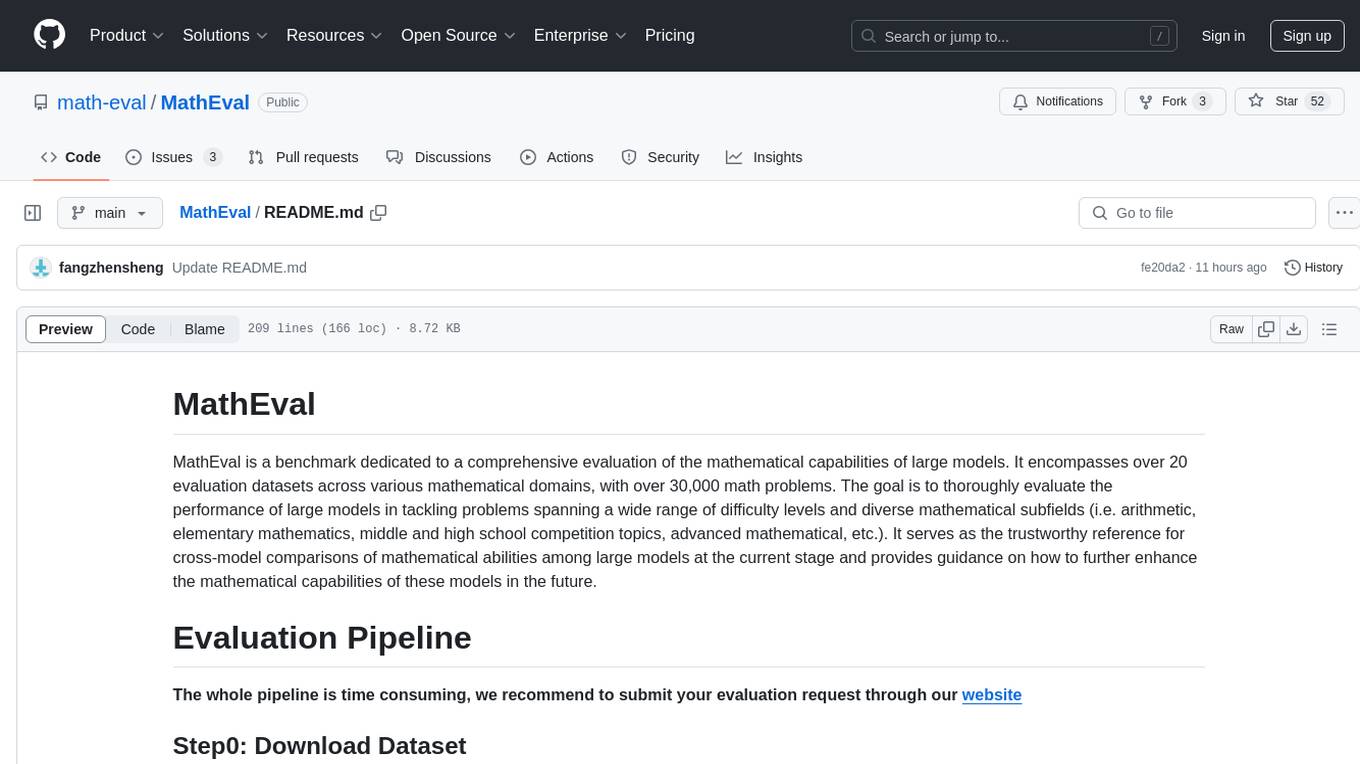
MathEval
MathEval is a benchmark designed for evaluating the mathematical capabilities of large models. It includes over 20 evaluation datasets covering various mathematical domains with more than 30,000 math problems. The goal is to assess the performance of large models across different difficulty levels and mathematical subfields. MathEval serves as a reliable reference for comparing mathematical abilities among large models and offers guidance on enhancing their mathematical capabilities in the future.
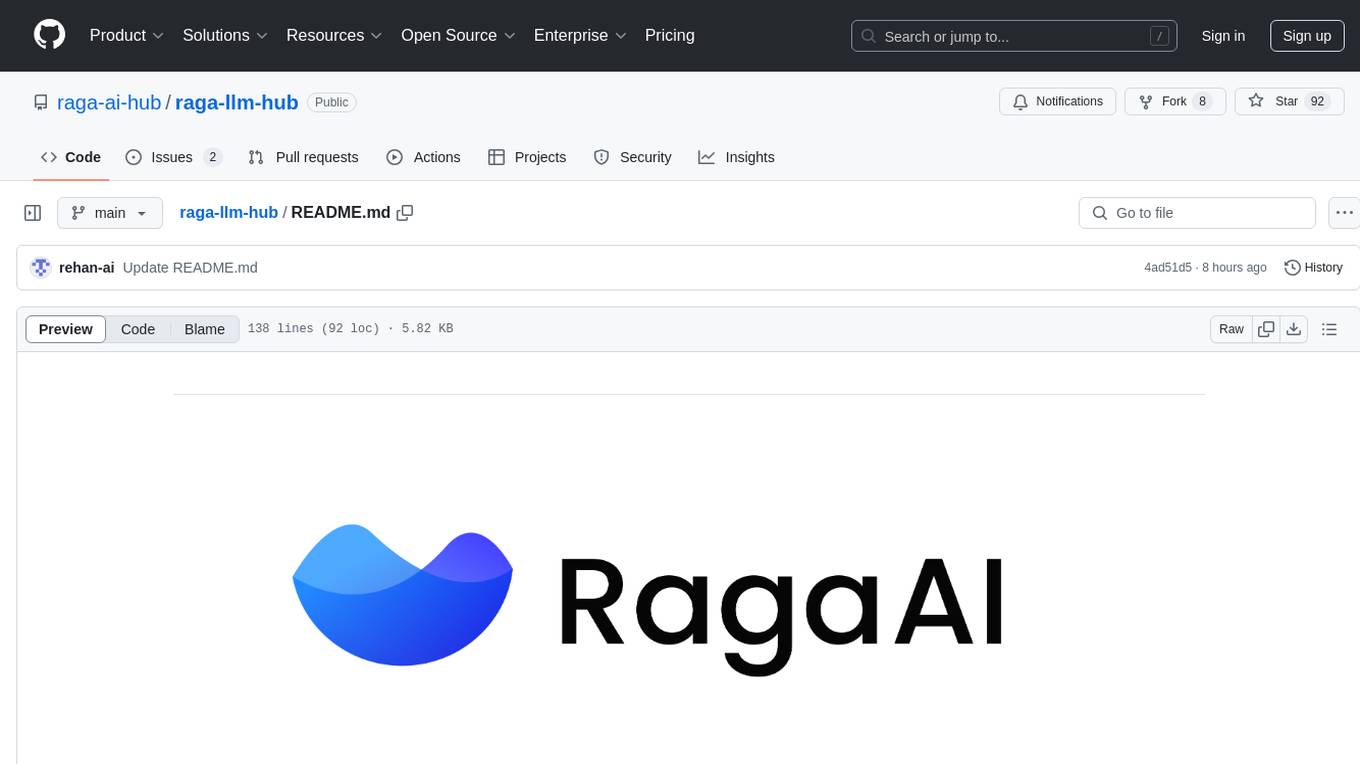
raga-llm-hub
Raga LLM Hub is a comprehensive evaluation toolkit for Language and Learning Models (LLMs) with over 100 meticulously designed metrics. It allows developers and organizations to evaluate and compare LLMs effectively, establishing guardrails for LLMs and Retrieval Augmented Generation (RAG) applications. The platform assesses aspects like Relevance & Understanding, Content Quality, Hallucination, Safety & Bias, Context Relevance, Guardrails, and Vulnerability scanning, along with Metric-Based Tests for quantitative analysis. It helps teams identify and fix issues throughout the LLM lifecycle, revolutionizing reliability and trustworthiness.
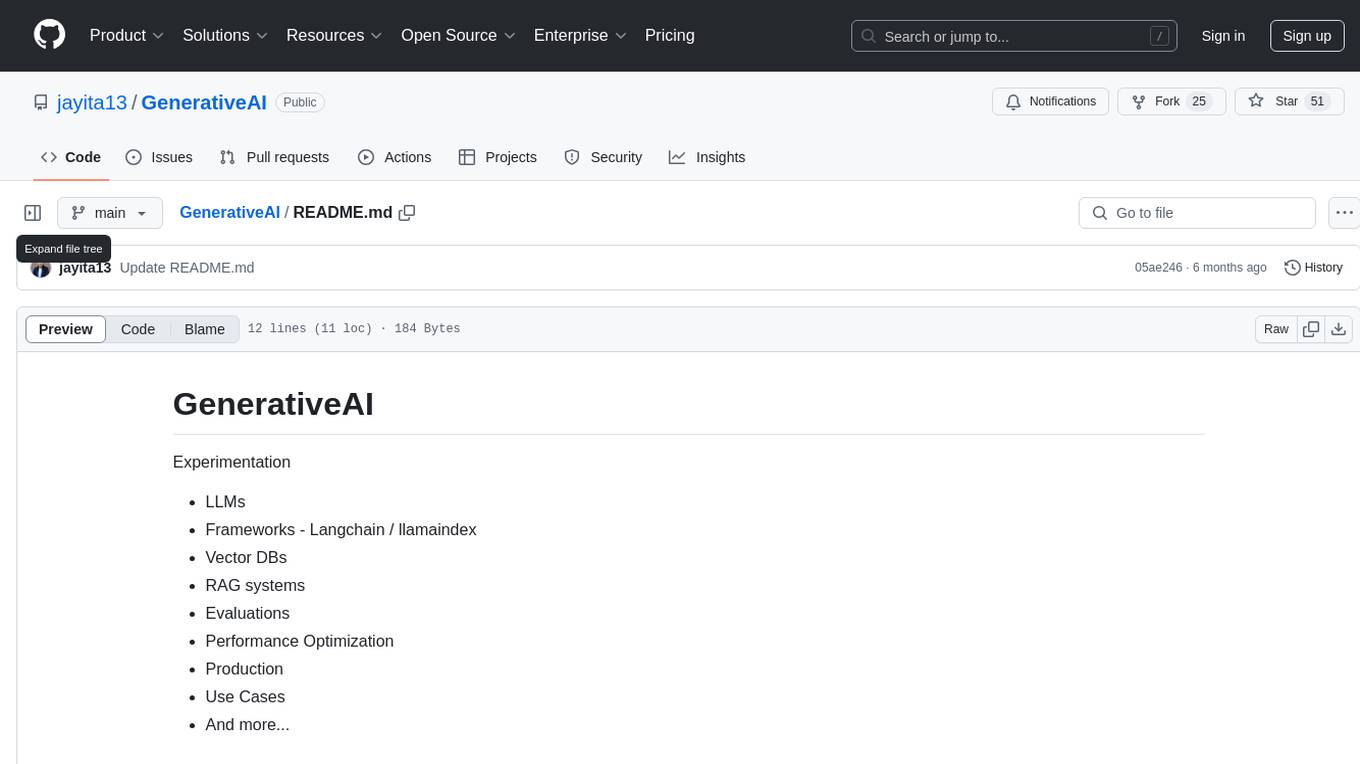
GenerativeAI
GenerativeAI is a repository focused on experimentation with various tools and techniques in the field of generative artificial intelligence. It covers topics such as large language models, frameworks like Langchain and llamaindex, vector databases, RAG systems, evaluations, performance optimization, production, use cases, and more.
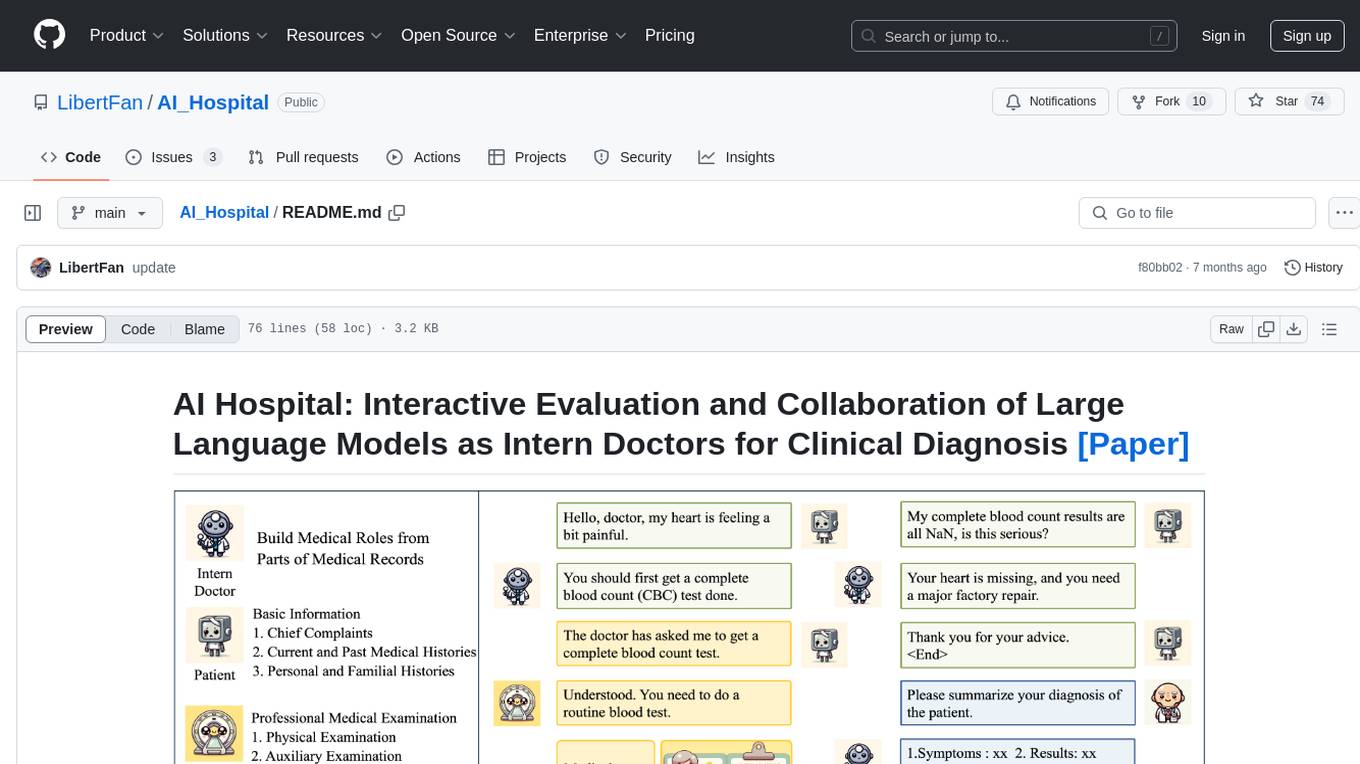
AI_Hospital
AI Hospital is a research repository focusing on the interactive evaluation and collaboration of Large Language Models (LLMs) as intern doctors for clinical diagnosis. The repository includes a simulation module tailored for various medical roles, introduces the Multi-View Medical Evaluation (MVME) Benchmark, provides dialog history documents of LLMs, replication instructions, performance evaluation, and guidance for creating intern doctor agents. The collaborative diagnosis with LLMs emphasizes dispute resolution. The study was authored by Zhihao Fan, Jialong Tang, Wei Chen, Siyuan Wang, Zhongyu Wei, Jun Xie, Fei Huang, and Jingren Zhou.
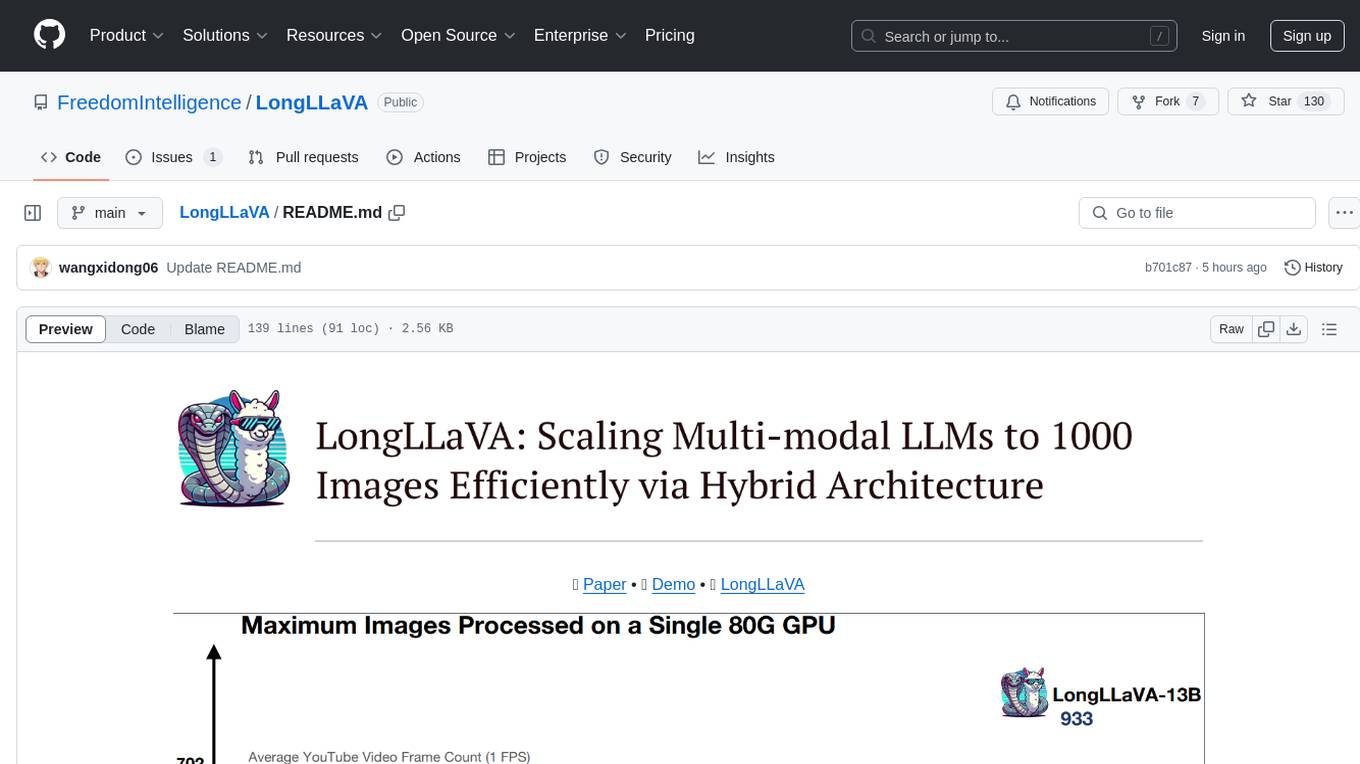
LongLLaVA
LongLLaVA is a tool for scaling multi-modal LLMs to 1000 images efficiently via hybrid architecture. It includes stages for single-image alignment, instruction-tuning, and multi-image instruction-tuning, with evaluation through a command line interface and model inference. The tool aims to achieve GPT-4V level capabilities and beyond, providing reproducibility of results and benchmarks for efficiency and performance.
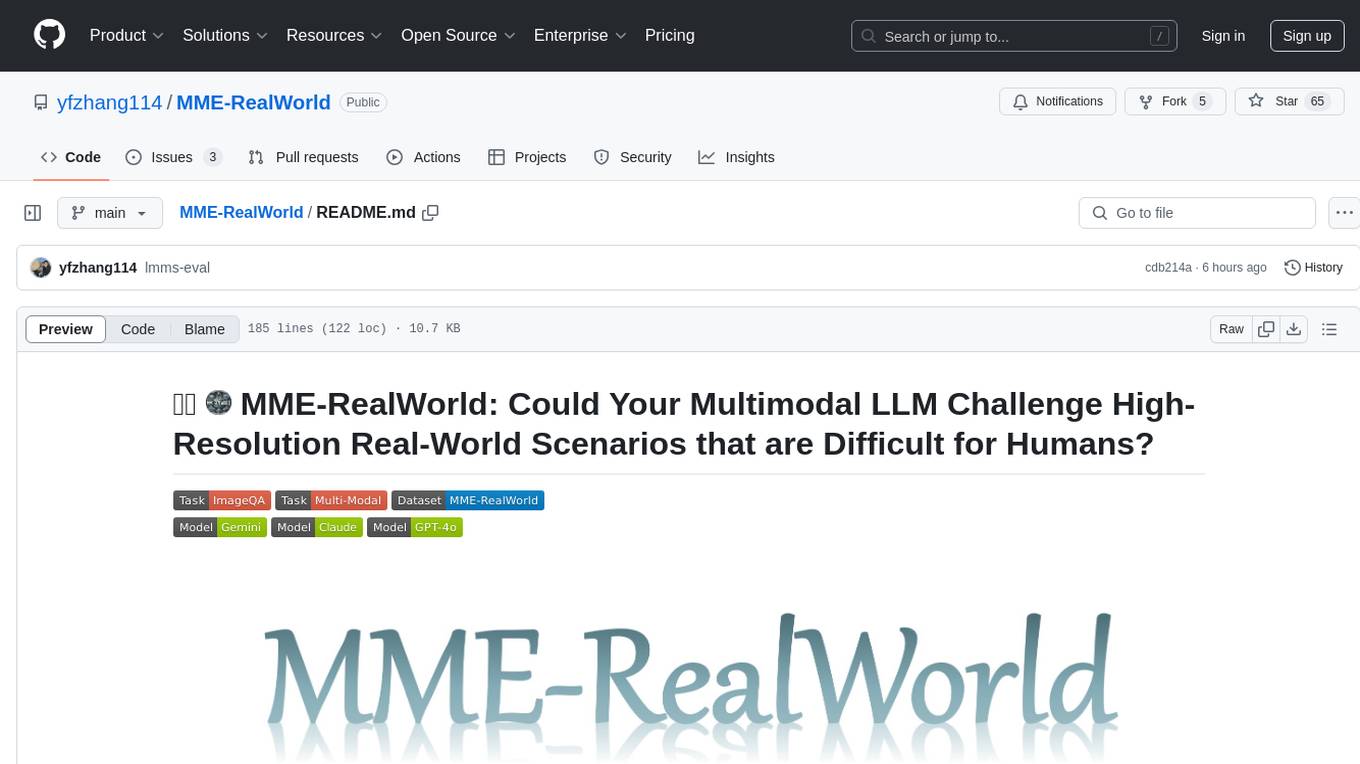
MME-RealWorld
MME-RealWorld is a benchmark designed to address real-world applications with practical relevance, featuring 13,366 high-resolution images and 29,429 annotations across 43 tasks. It aims to provide substantial recognition challenges and overcome common barriers in existing Multimodal Large Language Model benchmarks, such as small data scale, restricted data quality, and insufficient task difficulty. The dataset offers advantages in data scale, data quality, task difficulty, and real-world utility compared to existing benchmarks. It also includes a Chinese version with additional images and QA pairs focused on Chinese scenarios.
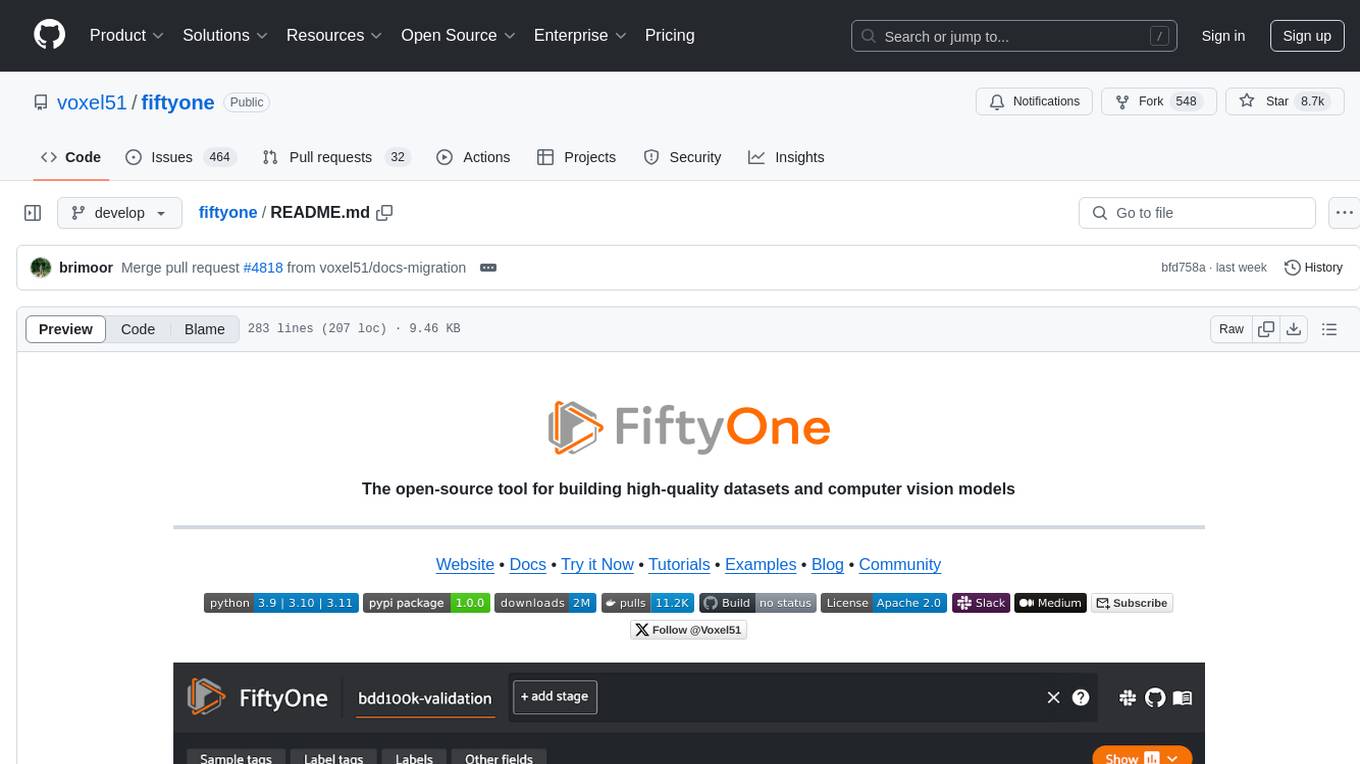
fiftyone
FiftyOne is an open-source tool designed for building high-quality datasets and computer vision models. It supercharges machine learning workflows by enabling users to visualize datasets, interpret models faster, and improve efficiency. With FiftyOne, users can explore scenarios, identify failure modes, visualize complex labels, evaluate models, find annotation mistakes, and much more. The tool aims to streamline the process of improving machine learning models by providing a comprehensive set of features for data analysis and model interpretation.
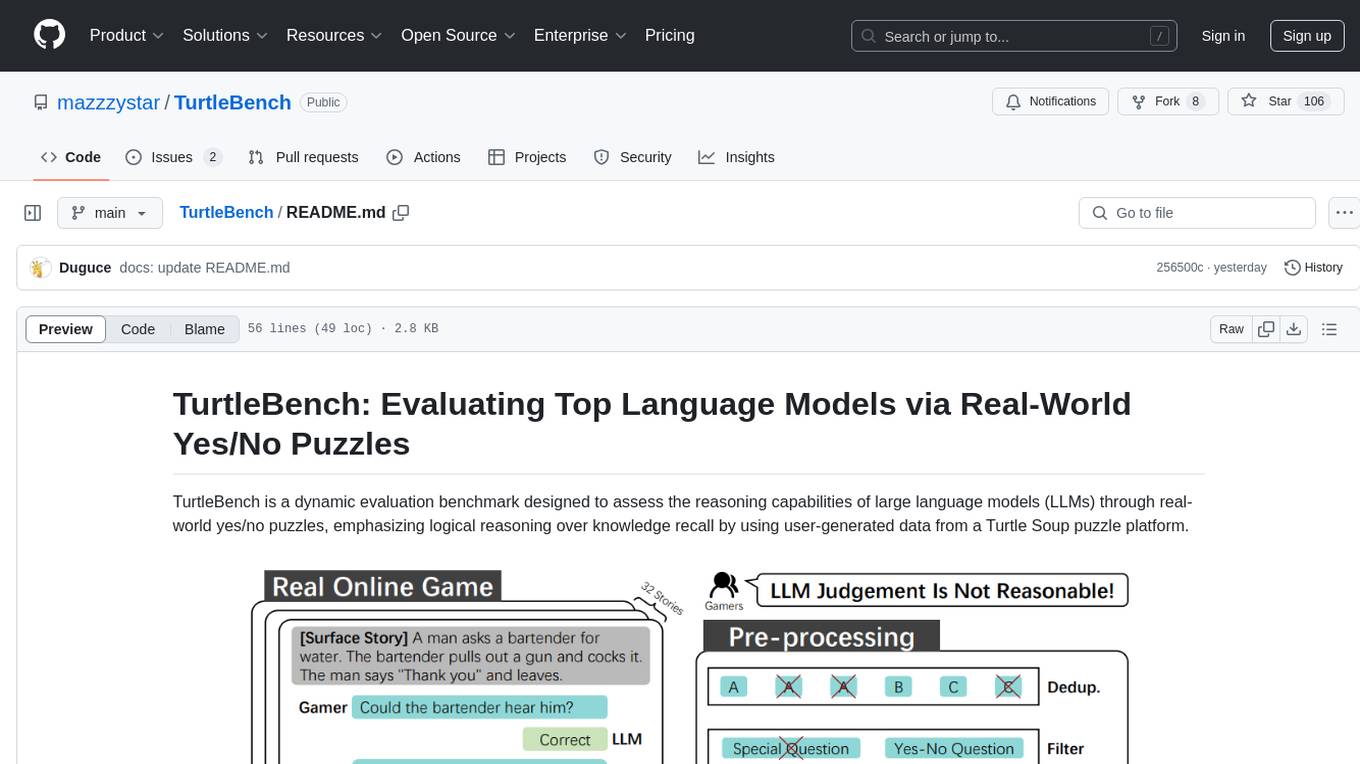
TurtleBench
TurtleBench is a dynamic evaluation benchmark that assesses the reasoning capabilities of large language models through real-world yes/no puzzles. It emphasizes logical reasoning over knowledge recall by using user-generated data from a Turtle Soup puzzle platform. The benchmark is objective and unbiased, focusing purely on reasoning abilities and providing clear, measurable outcomes for easy comparison. TurtleBench constantly evolves with real user-generated questions, making it impossible to 'game' the system. It tests the model's ability to comprehend context and make logical inferences.
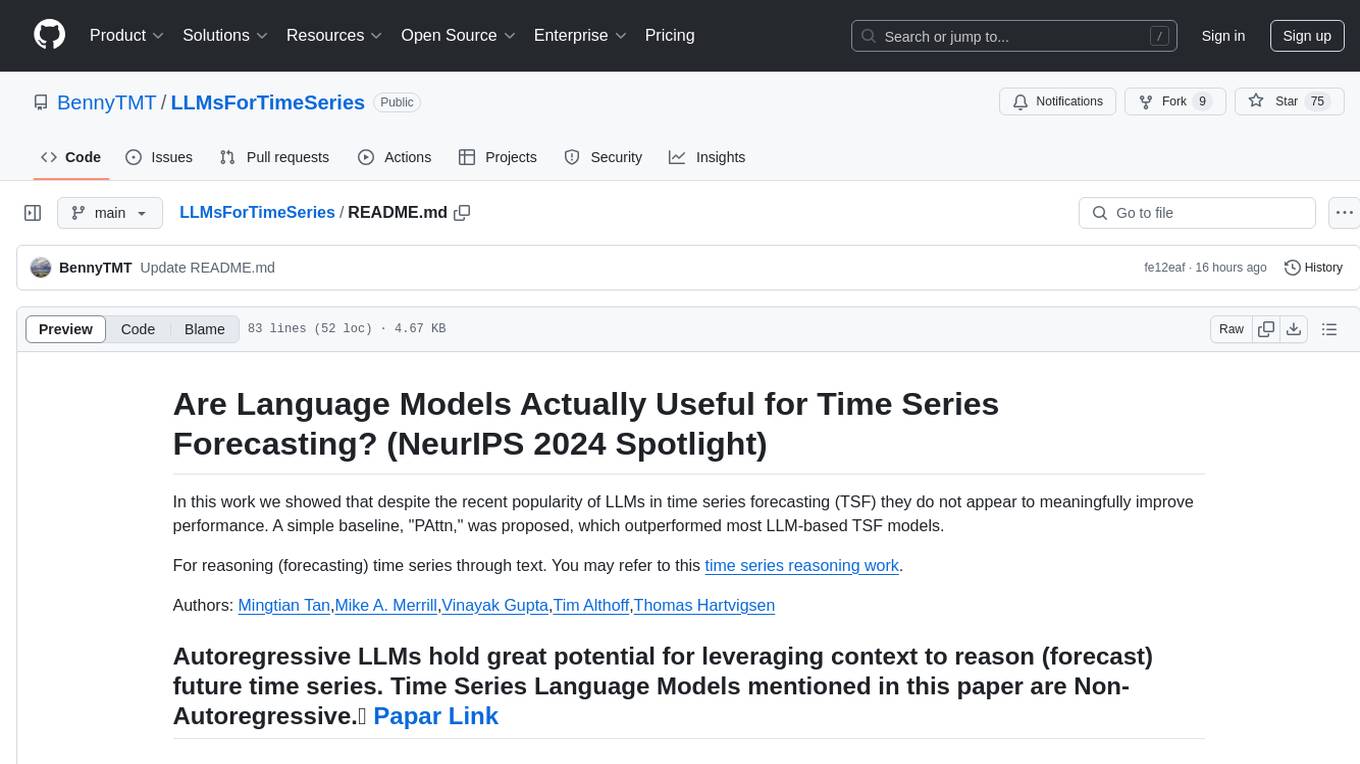
LLMsForTimeSeries
LLMsForTimeSeries is a repository that questions the usefulness of language models in time series forecasting. The work shows that simple baselines outperform most language model-based time series forecasting models. It includes ablation studies on LLM-based TSF methods and introduces the PAttn method, showcasing the performance of patching and attention structures in forecasting. The repository provides datasets, setup instructions, and scripts for running ablations on different datasets.
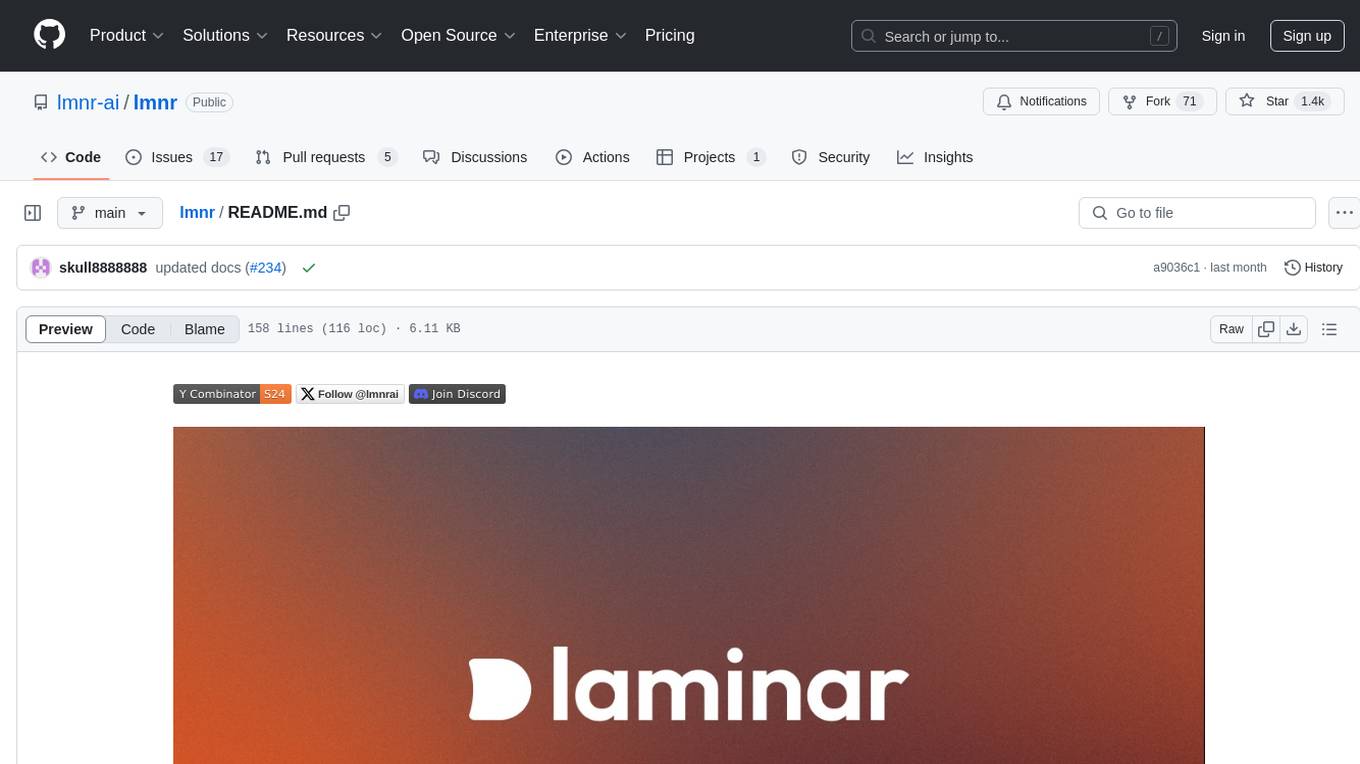
lmnr
Laminar is an all-in-one open-source platform designed for engineering AI products. It allows users to trace, evaluate, label, and analyze LLM data efficiently. The platform offers features such as automatic tracing of common AI frameworks and SDKs, local and online evaluations, simple UI for data labeling, dataset management, and scalability with gRPC communication. Laminar is built with a modern open-source stack including RabbitMQ, Postgres, Clickhouse, and Qdrant for semantic similarity search. It provides fast and beautiful dashboards for traces, evaluations, and labels, making it a comprehensive tool for AI product development.
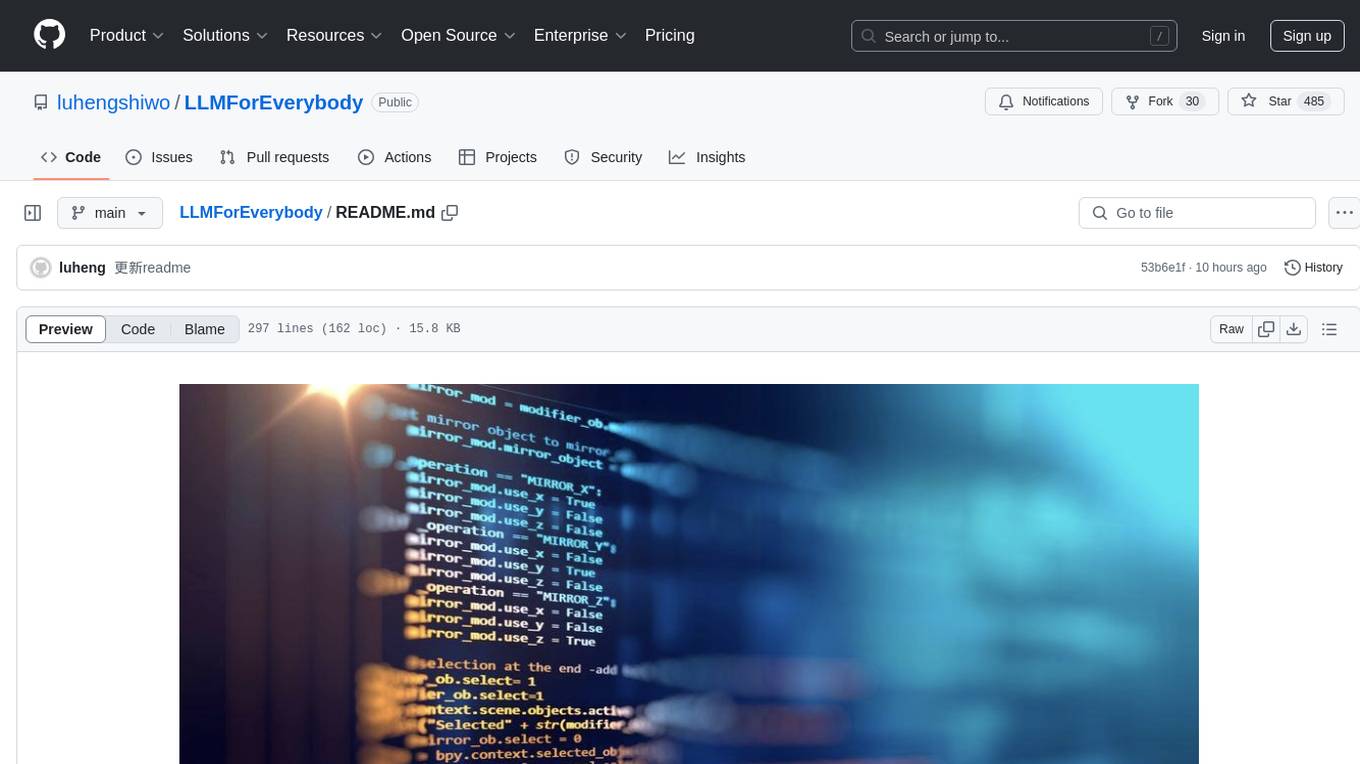
LLMForEverybody
LLMForEverybody is a comprehensive repository covering various aspects of large language models (LLMs) including pre-training, architecture, optimizers, activation functions, attention mechanisms, tokenization, parallel strategies, training frameworks, deployment, fine-tuning, quantization, GPU parallelism, prompt engineering, agent design, RAG architecture, enterprise deployment challenges, evaluation metrics, and current hot topics in the field. It provides detailed explanations, tutorials, and insights into the workings and applications of LLMs, making it a valuable resource for researchers, developers, and enthusiasts interested in understanding and working with large language models.
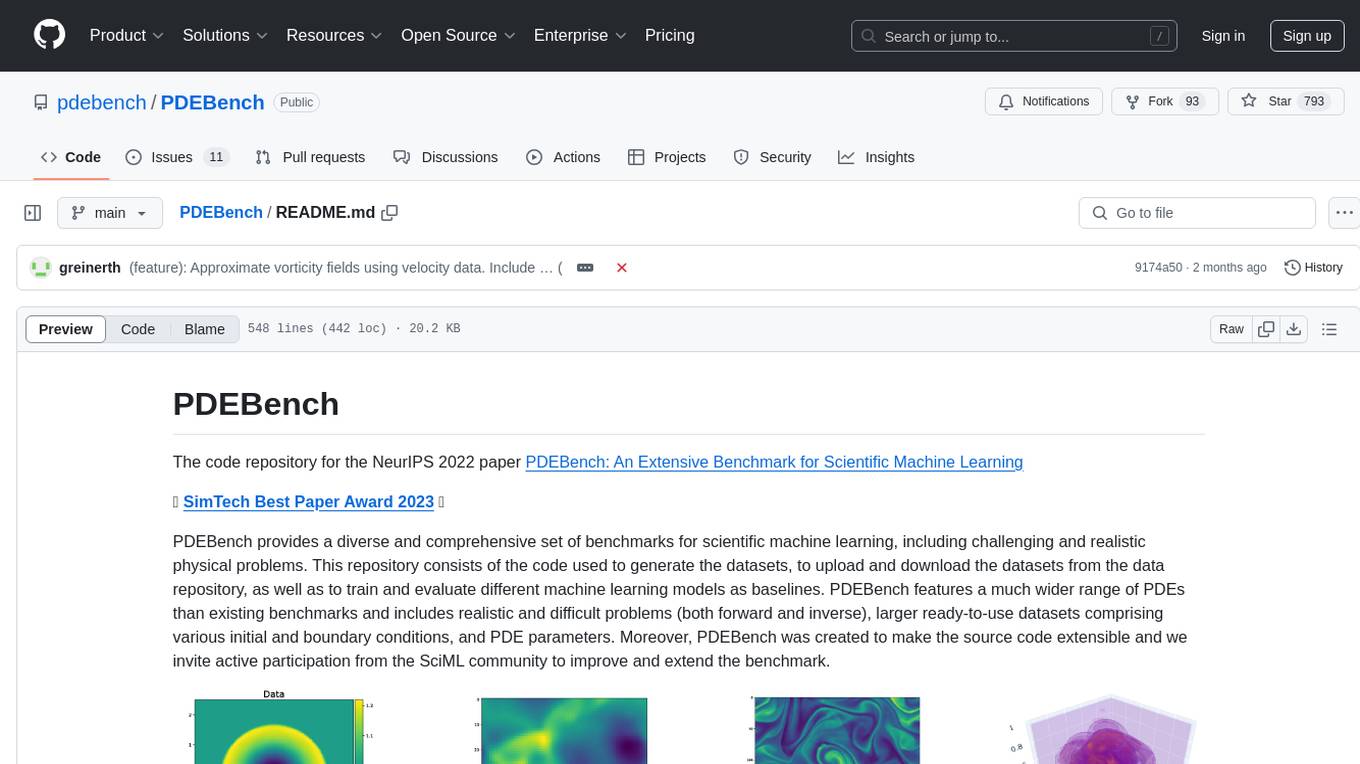
PDEBench
PDEBench provides a diverse and comprehensive set of benchmarks for scientific machine learning, including challenging and realistic physical problems. The repository consists of code for generating datasets, uploading and downloading datasets, training and evaluating machine learning models as baselines. It features a wide range of PDEs, realistic and difficult problems, ready-to-use datasets with various conditions and parameters. PDEBench aims for extensibility and invites participation from the SciML community to improve and extend the benchmark.
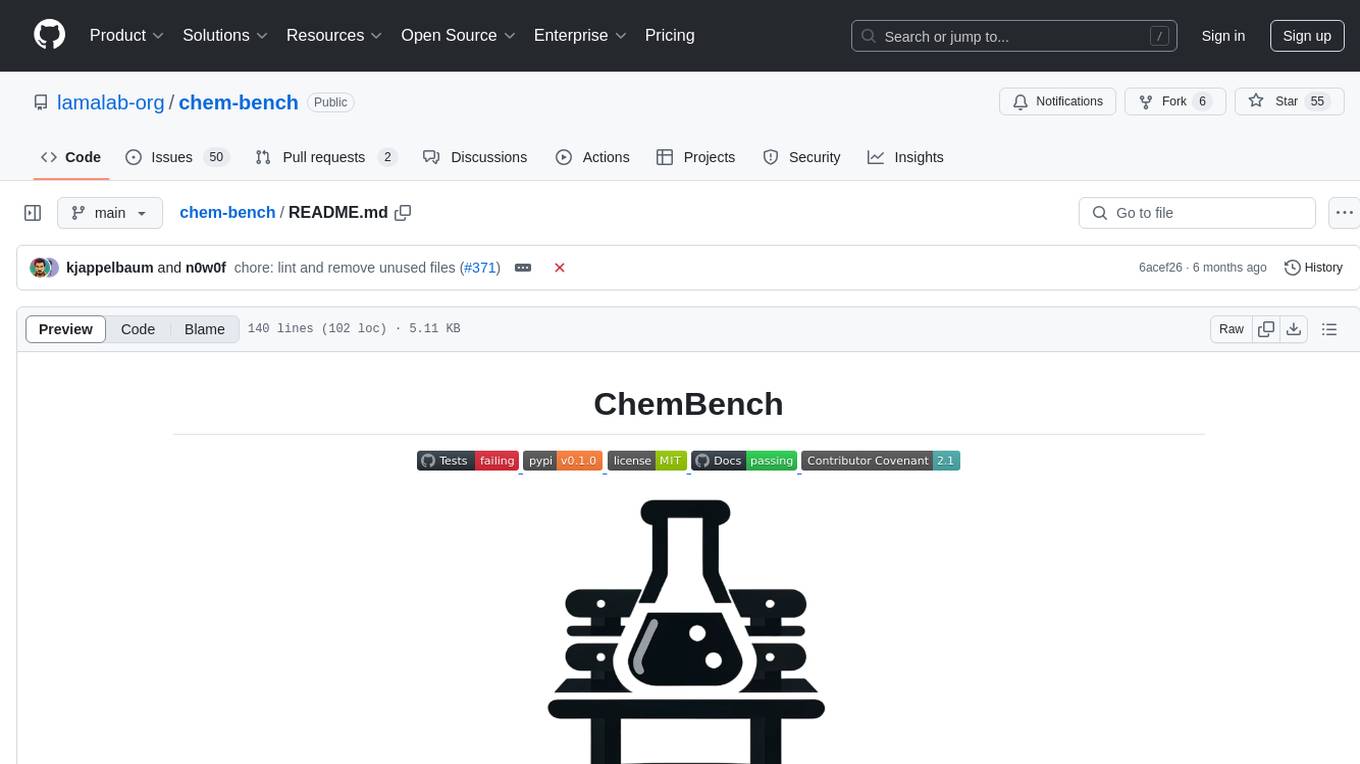
chem-bench
ChemBench is a project aimed at expanding chemistry benchmark tasks in a BIG-bench compatible way, providing a pipeline to benchmark frontier and open models. It allows users to run benchmarking tasks on models with existing presets, offering predefined parameters and processing steps. The library facilitates benchmarking models on the entire suite, addressing challenges such as prompt structure, parsing, and scoring methods. Users can contribute to the project by following the developer notes.
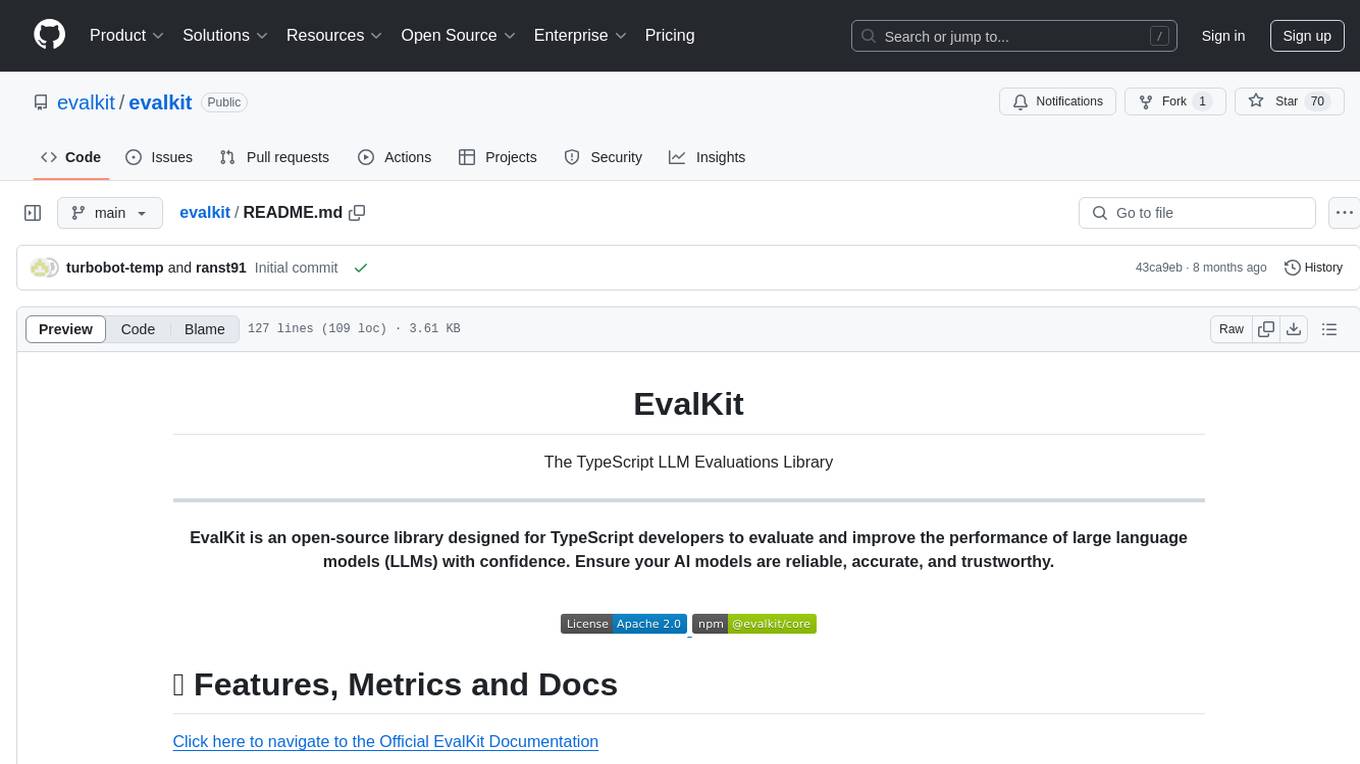
evalkit
EvalKit is an open-source TypeScript library for evaluating and improving the performance of large language models (LLMs). It helps developers ensure the reliability, accuracy, and trustworthiness of their AI models. The library provides various metrics such as Bias Detection, Coherence, Faithfulness, Hallucination, Intent Detection, and Semantic Similarity. EvalKit is designed to be user-friendly with detailed documentation, tutorials, and recipes for different use cases and LLM providers. It requires Node.js 18+ and an OpenAI API Key for installation and usage. Contributions from the community are welcome under the Apache 2.0 License.
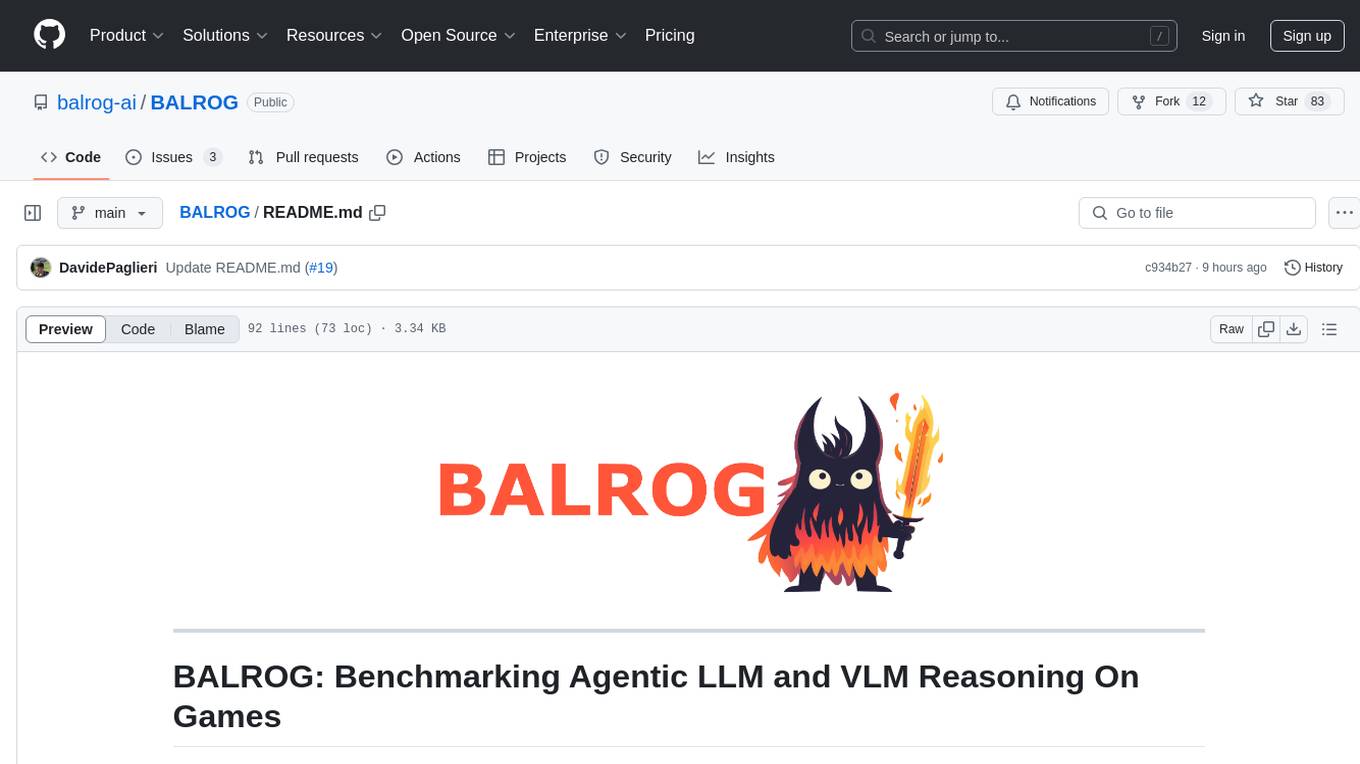
BALROG
BALROG is a benchmark tool designed to evaluate agentic Long-Longitudinal Memory (LLM) and Vision-Language Memory (VLM) capabilities using reinforcement learning environments. It provides a comprehensive assessment of agentic abilities, supports both language and vision-language models, integrates with popular AI APIs, and allows for easy integration of custom agents, new environments, and models.
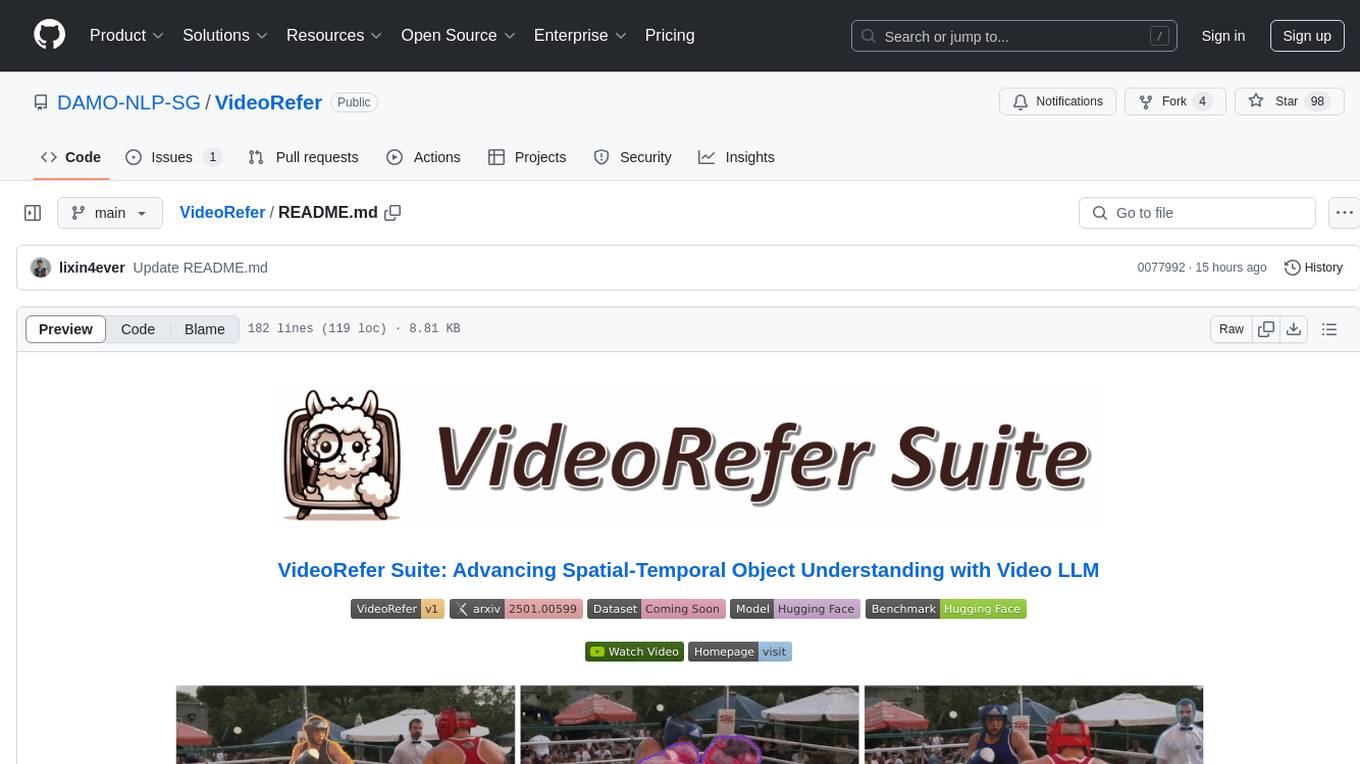
VideoRefer
VideoRefer Suite is a tool designed to enhance the fine-grained spatial-temporal understanding capabilities of Video Large Language Models (Video LLMs). It consists of three primary components: Model (VideoRefer) for perceiving, reasoning, and retrieval for user-defined regions at any specified timestamps, Dataset (VideoRefer-700K) for high-quality object-level video instruction data, and Benchmark (VideoRefer-Bench) to evaluate object-level video understanding capabilities. The tool can understand any object within a video.
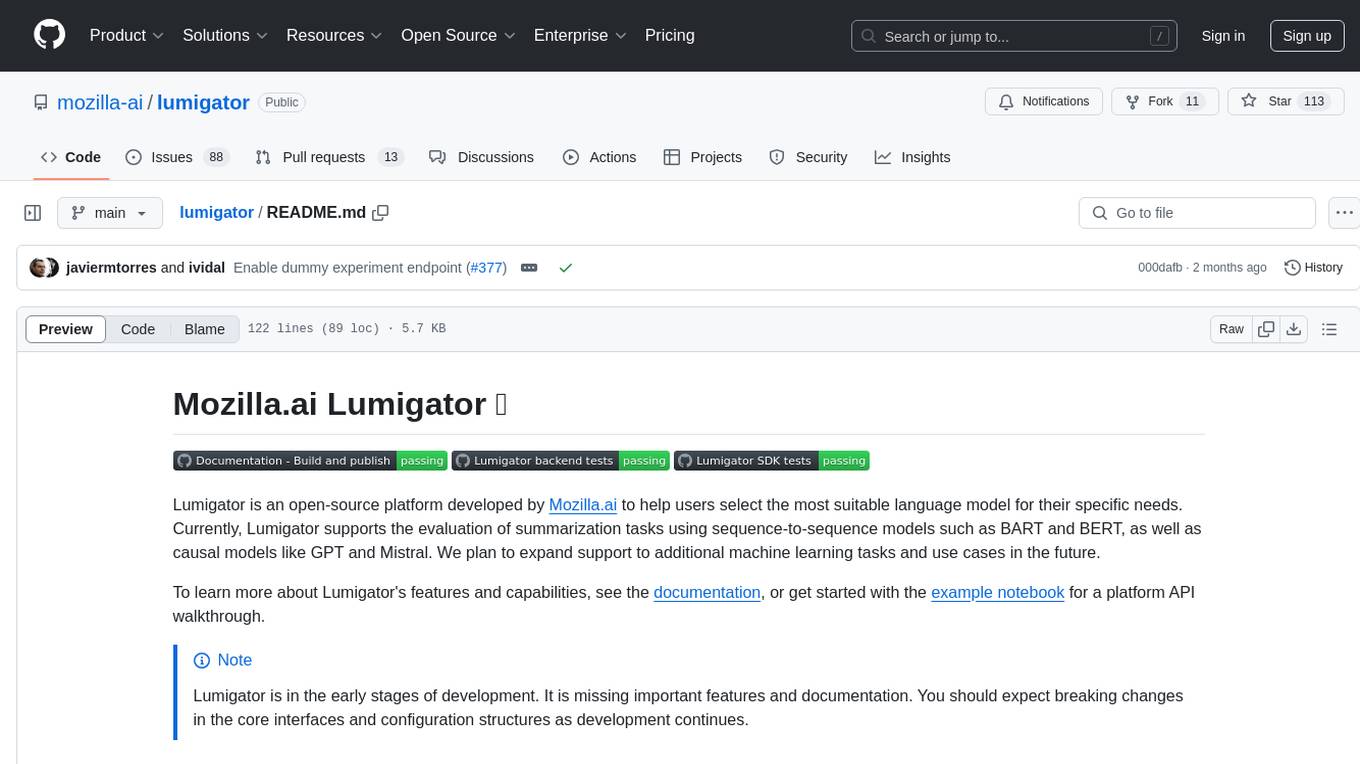
lumigator
Lumigator is an open-source platform developed by Mozilla.ai to help users select the most suitable language model for their specific needs. It supports the evaluation of summarization tasks using sequence-to-sequence models such as BART and BERT, as well as causal models like GPT and Mistral. The platform aims to make model selection transparent, efficient, and empowering by providing a framework for comparing LLMs using task-specific metrics to evaluate how well a model fits a project's needs. Lumigator is in the early stages of development and plans to expand support to additional machine learning tasks and use cases in the future.
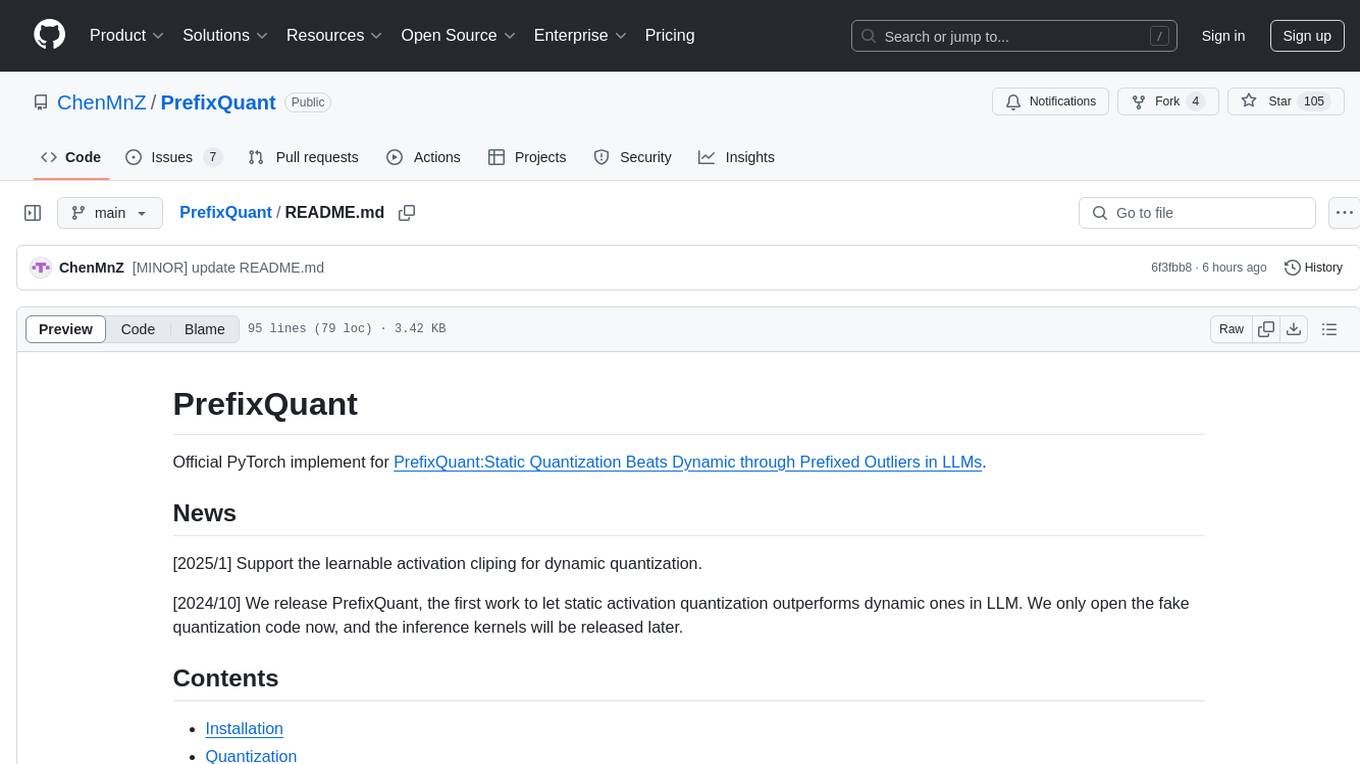
PrefixQuant
PrefixQuant is an official PyTorch implementation for static quantization that outperforms dynamic quantization in Large Language Models (LLMs) by utilizing prefixed outliers. The tool provides functionalities for quantization, inference, and visualization of activation distributions. Users can fine-tune quantization settings and evaluate pre-quantized models for tasks like PIQA, ARC, Hellaswag, and Winogrande. The approach aims to improve performance and efficiency in LLMs through innovative quantization techniques.
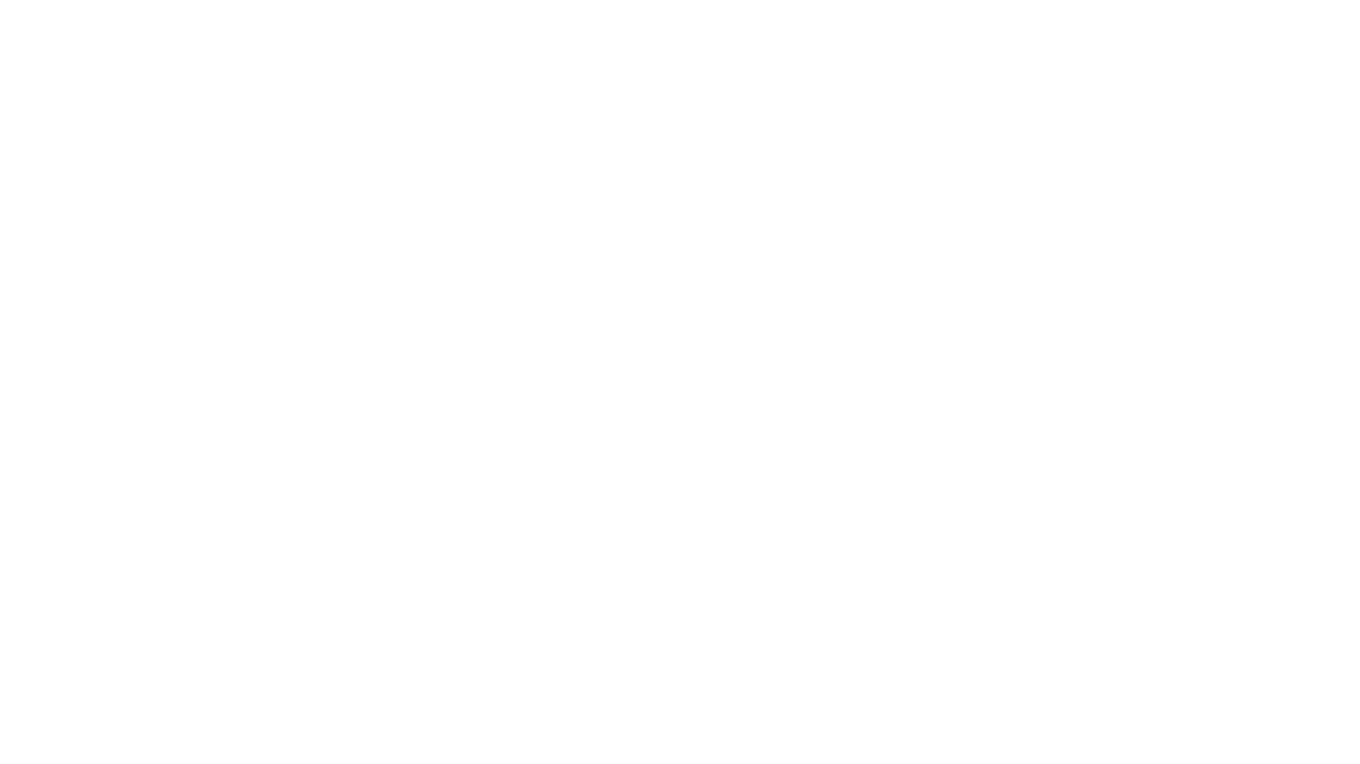
eureka-ml-insights
The Eureka ML Insights Framework is a repository containing code designed to help researchers and practitioners run reproducible evaluations of generative models efficiently. Users can define custom pipelines for data processing, inference, and evaluation, as well as utilize pre-defined evaluation pipelines for key benchmarks. The framework provides a structured approach to conducting experiments and analyzing model performance across various tasks and modalities.
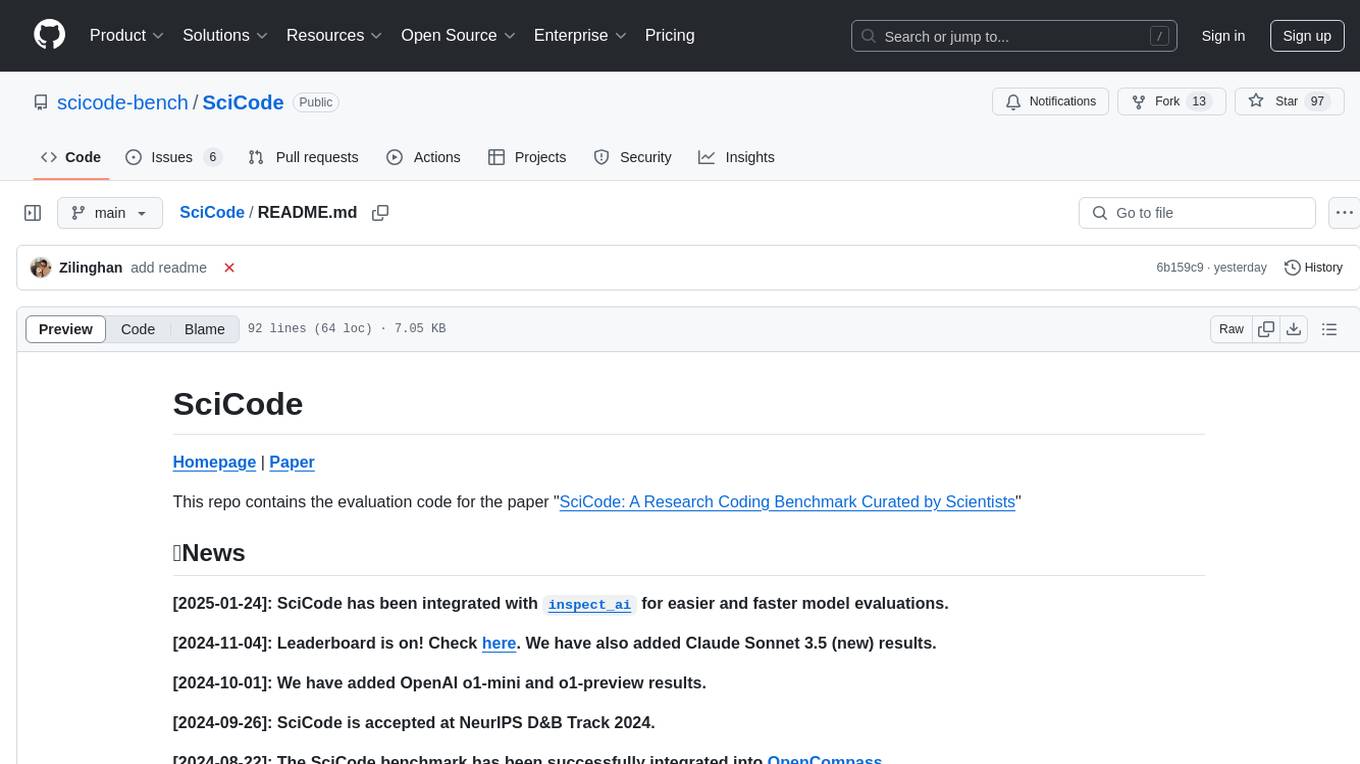
SciCode
SciCode is a challenging benchmark designed to evaluate the capabilities of language models (LMs) in generating code for solving realistic scientific research problems. It contains 338 subproblems decomposed from 80 challenging main problems across 16 subdomains from 6 domains. The benchmark offers optional descriptions specifying useful scientific background information and scientist-annotated gold-standard solutions and test cases for evaluation. SciCode demonstrates a realistic workflow of identifying critical science concepts and facts and transforming them into computation and simulation code, aiming to help showcase LLMs' progress towards assisting scientists and contribute to the future building and evaluation of scientific AI.
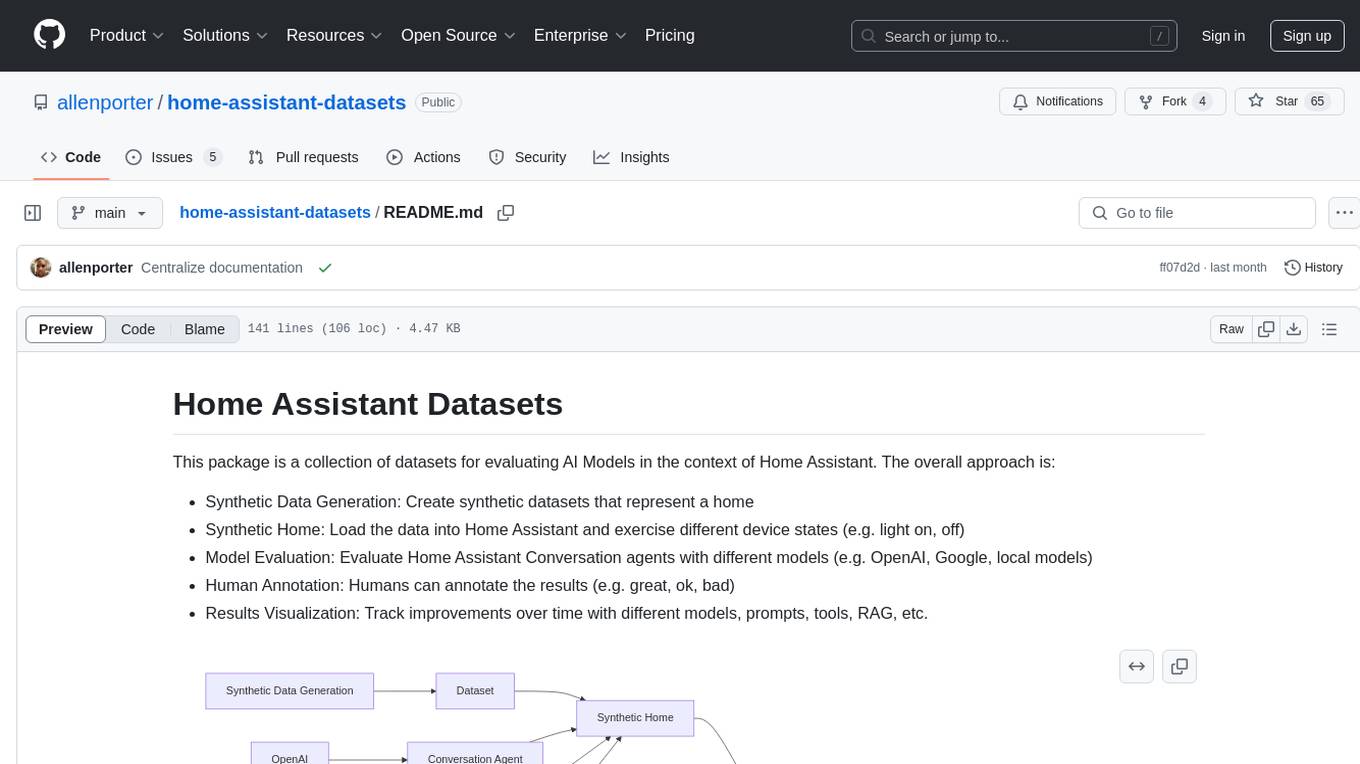
home-assistant-datasets
This package provides a collection of datasets for evaluating AI Models in the context of Home Assistant. It includes synthetic data generation, loading data into Home Assistant, model evaluation with different conversation agents, human annotation of results, and visualization of improvements over time. The datasets cover home descriptions, area descriptions, device descriptions, and summaries that can be performed on a home. The tool aims to build datasets for future training purposes.
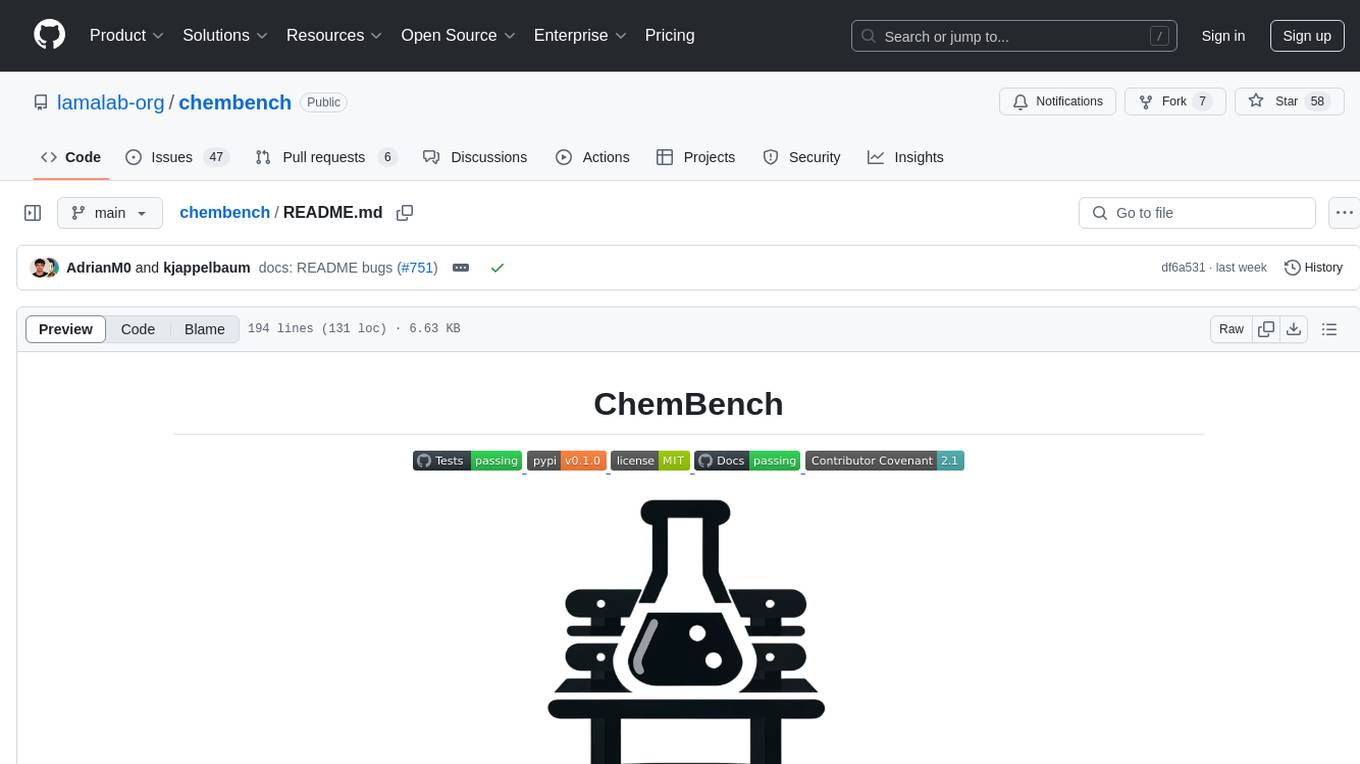
chembench
ChemBench is a project aimed at expanding chemistry benchmark tasks in a BIG-bench compatible way, providing a pipeline to benchmark frontier and open models. It enables benchmarking across a wide range of API-based models and employs an LLM-based extractor as a fallback mechanism. Users can evaluate models on specific chemistry topics and run comprehensive evaluations across all topics in the benchmark suite. The tool facilitates seamless benchmarking for any model supported by LiteLLM and allows running non-API hosted models.
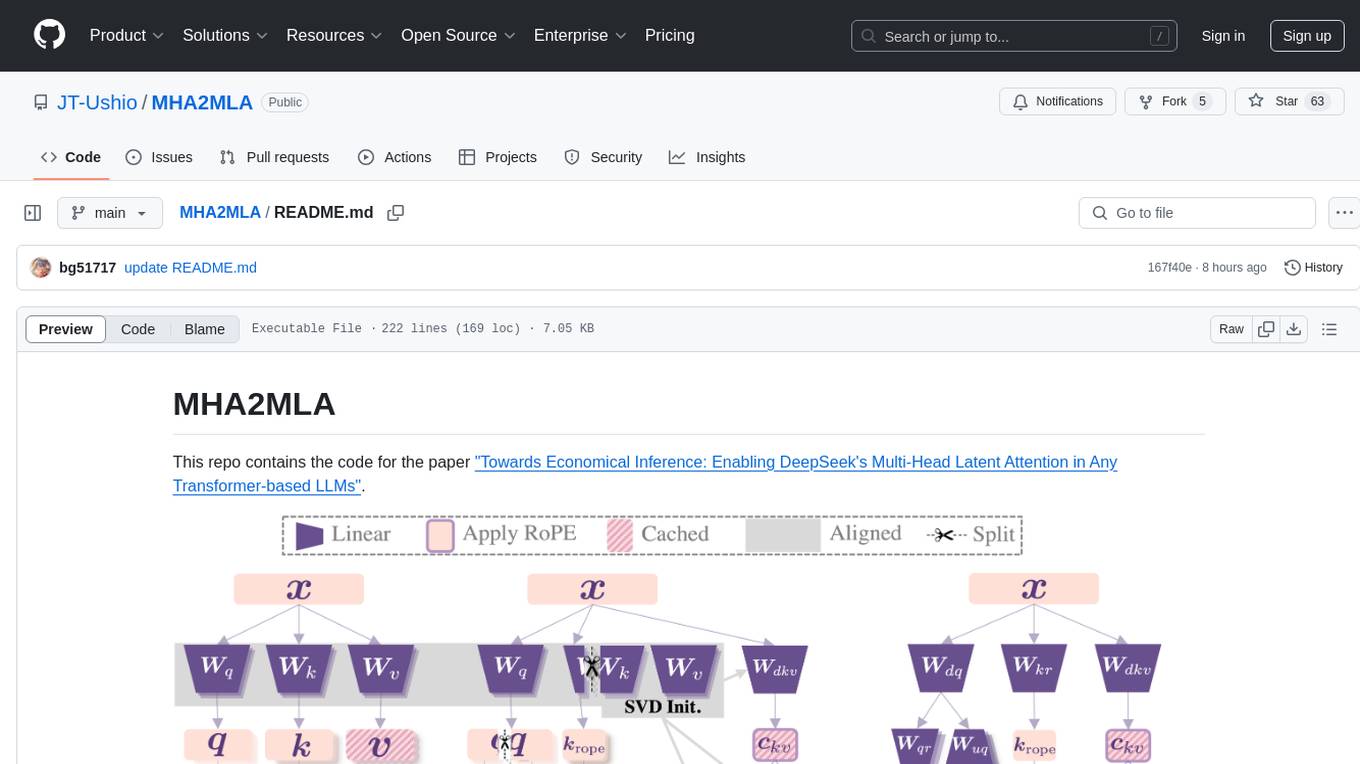
MHA2MLA
This repository contains the code for the paper 'Towards Economical Inference: Enabling DeepSeek's Multi-Head Latent Attention in Any Transformer-based LLMs'. It provides tools for fine-tuning and evaluating Llama models, converting models between different frameworks, processing datasets, and performing specific model training tasks like Partial-RoPE Fine-Tuning and Multiple-Head Latent Attention Fine-Tuning. The repository also includes commands for model evaluation using Lighteval and LongBench, along with necessary environment setup instructions.
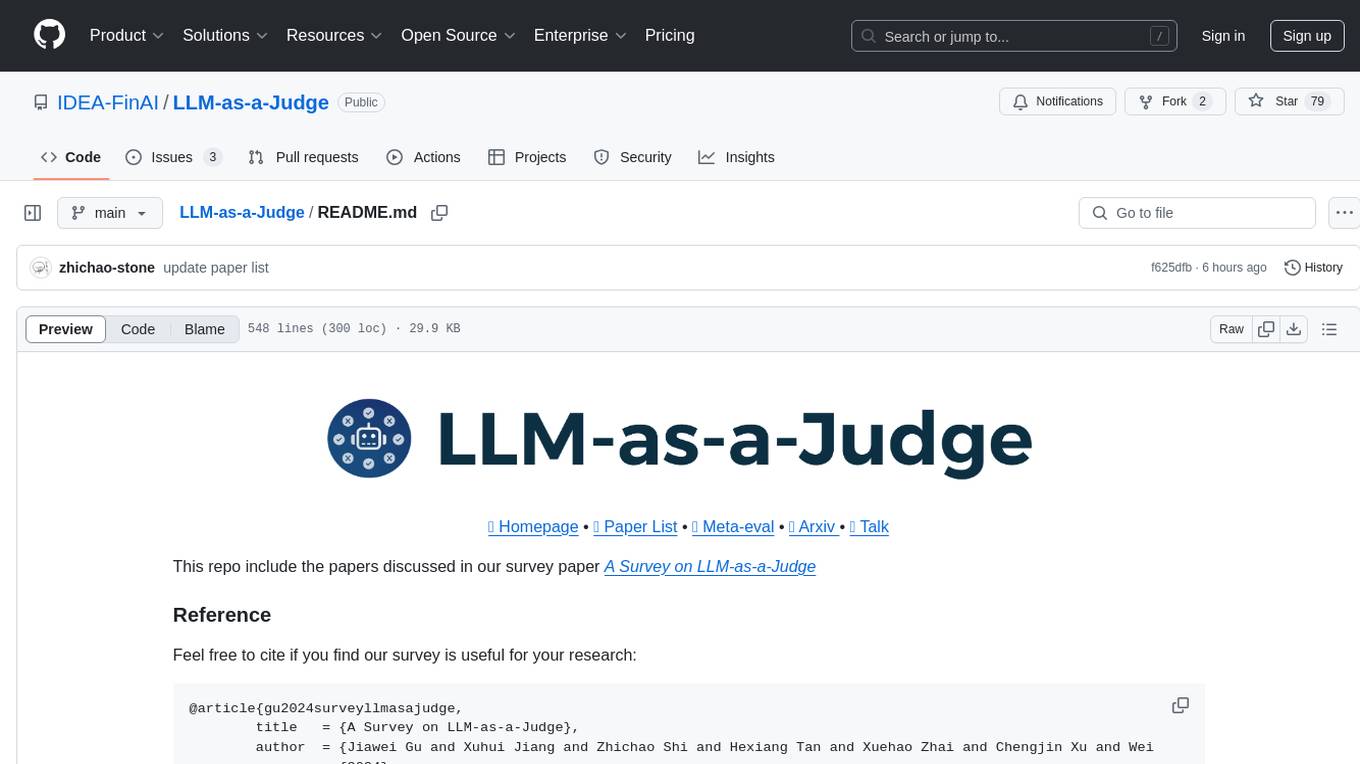
LLM-as-a-Judge
LLM-as-a-Judge is a repository that includes papers discussed in a survey paper titled 'A Survey on LLM-as-a-Judge'. The repository covers various aspects of using Large Language Models (LLMs) as judges for tasks such as evaluation, reasoning, and decision-making. It provides insights into evaluation pipelines, improvement strategies, and specific tasks related to LLMs. The papers included in the repository explore different methodologies, applications, and future research directions for leveraging LLMs as evaluators in various domains.
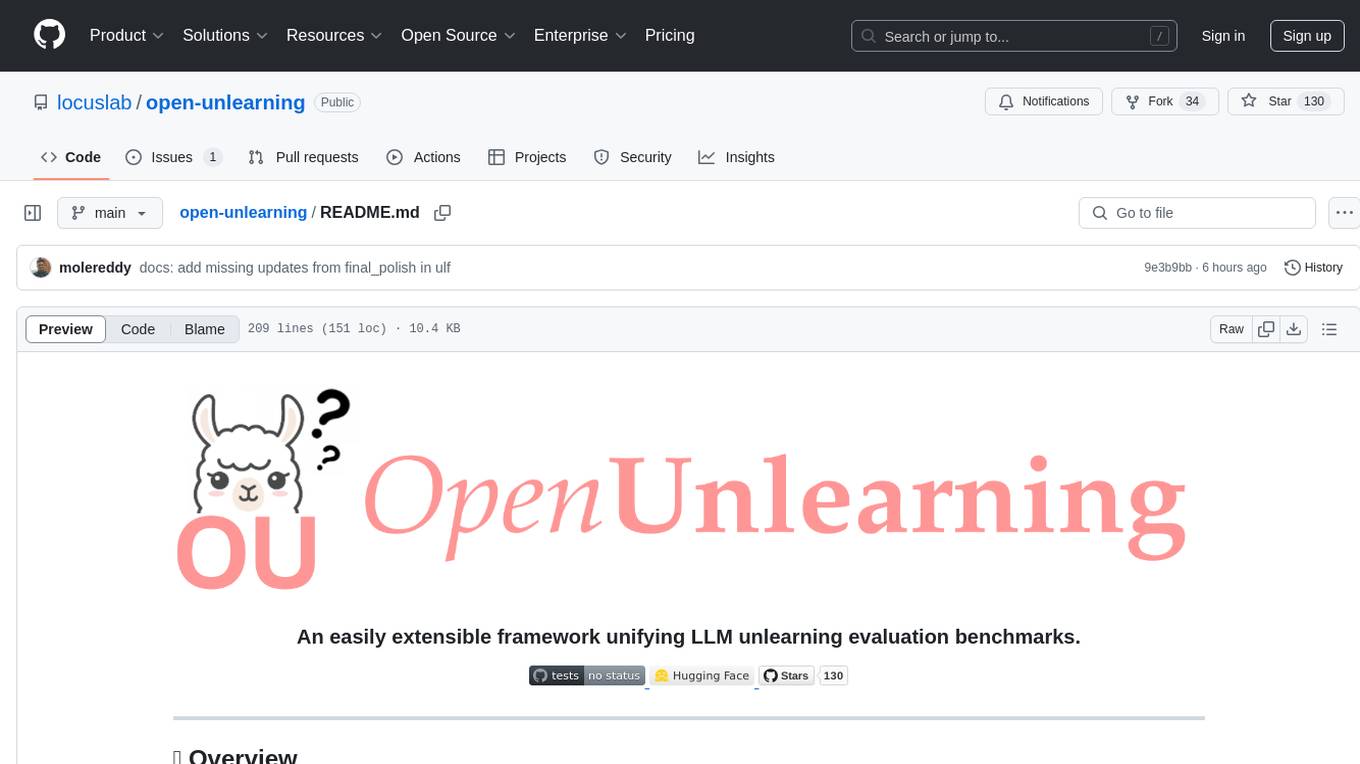
open-unlearning
OpenUnlearning is an easily extensible framework that unifies LLM unlearning evaluation benchmarks. It provides efficient implementations of TOFU and MUSE unlearning benchmarks, supporting 5 unlearning methods, 3+ datasets, 6+ evaluation metrics, and 7+ LLMs. Users can easily extend the framework to incorporate more variants, collaborate by adding new benchmarks, unlearning methods, datasets, and evaluation metrics, and drive progress in the field.
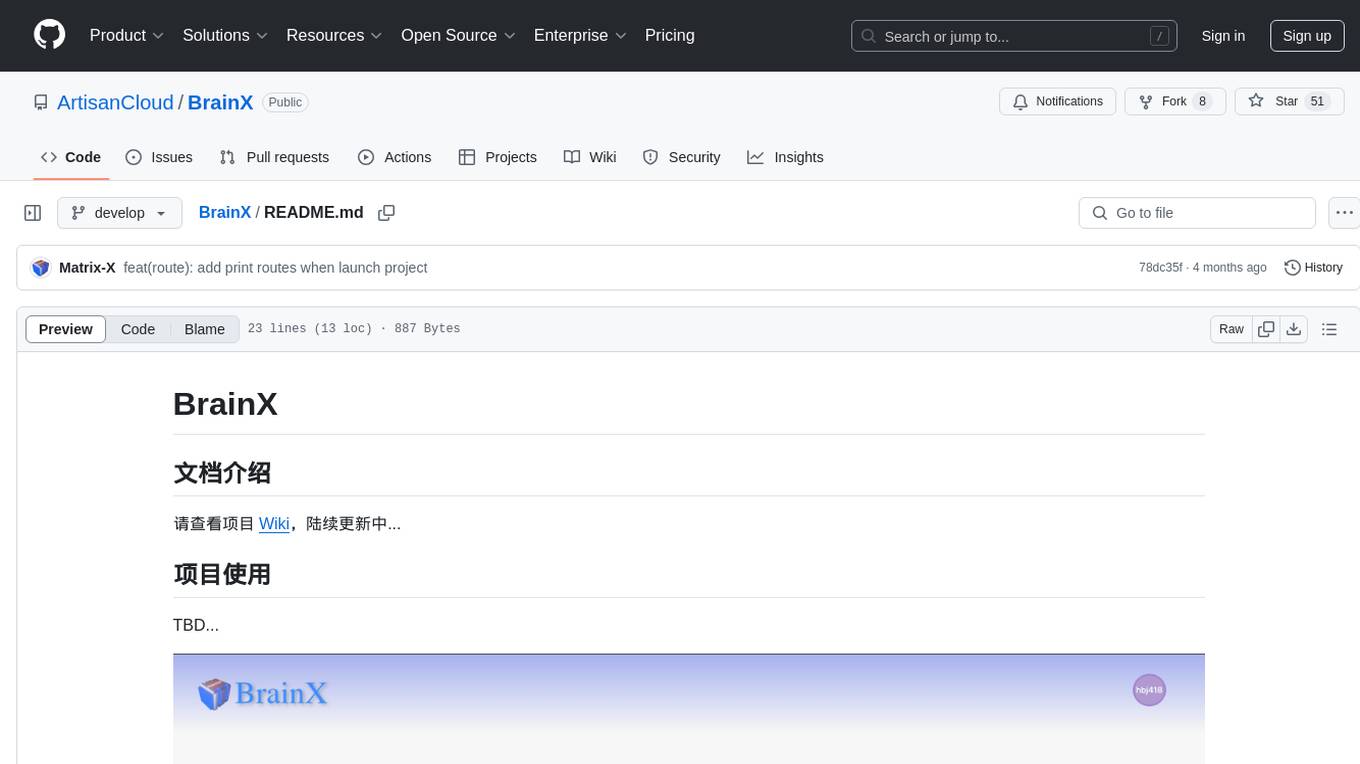
BrainX
BrainX is a tool designed for AI enthusiasts to explore and experiment with various machine learning algorithms and models. It provides a user-friendly interface for building, training, and evaluating AI models. The tool aims to simplify the process of developing AI applications and enable users to quickly prototype and test their ideas.
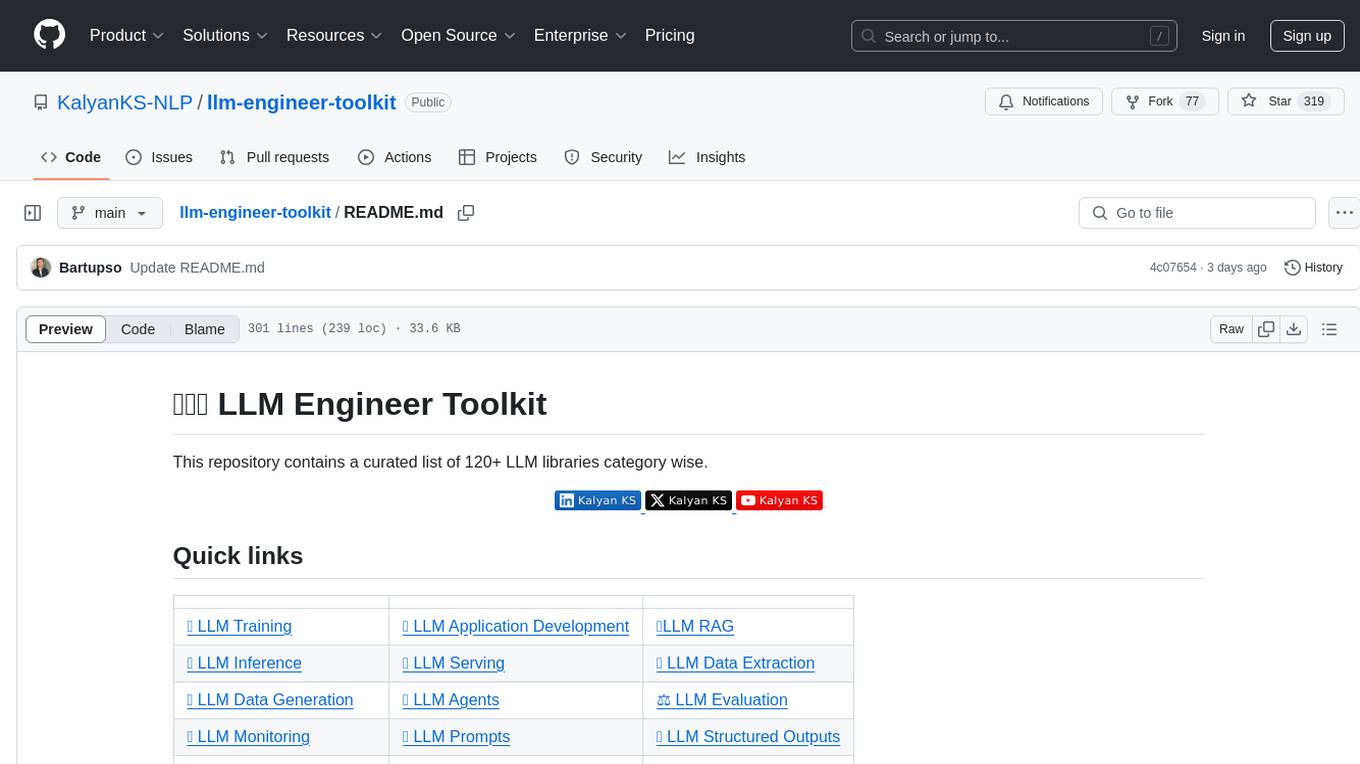
llm-engineer-toolkit
The LLM Engineer Toolkit is a curated repository containing over 120 LLM libraries categorized for various tasks such as training, application development, inference, serving, data extraction, data generation, agents, evaluation, monitoring, prompts, structured outputs, safety, security, embedding models, and other miscellaneous tools. It includes libraries for fine-tuning LLMs, building applications powered by LLMs, serving LLM models, extracting data, generating synthetic data, creating AI agents, evaluating LLM applications, monitoring LLM performance, optimizing prompts, handling structured outputs, ensuring safety and security, embedding models, and more. The toolkit covers a wide range of tools and frameworks to streamline the development, deployment, and optimization of large language models.
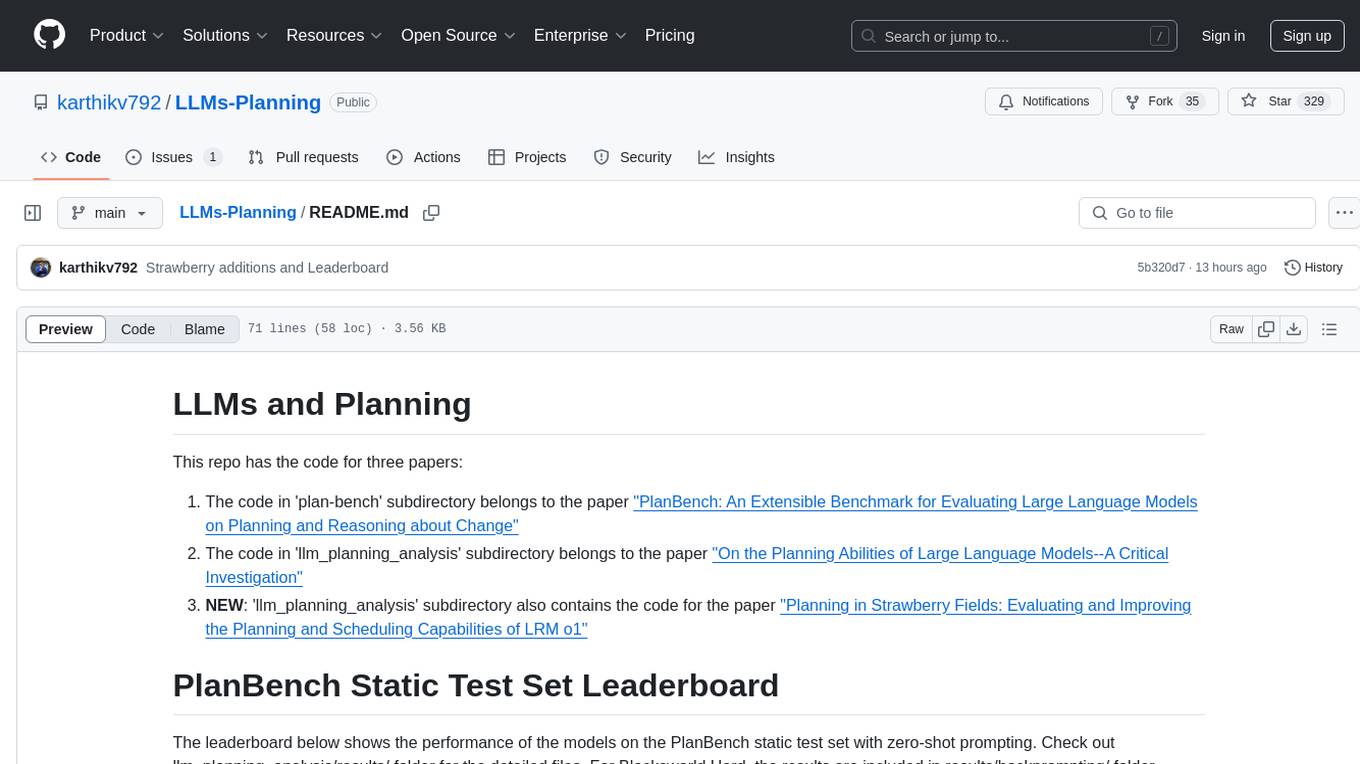
LLMs-Planning
This repository contains code for three papers related to evaluating large language models on planning and reasoning about change. It includes benchmarking tools and analysis for assessing the planning abilities of large language models. The latest addition evaluates and enhances the planning and scheduling capabilities of a specific language reasoning model. The repository provides a static test set leaderboard showcasing model performance on various tasks with natural language and planning domain prompts.
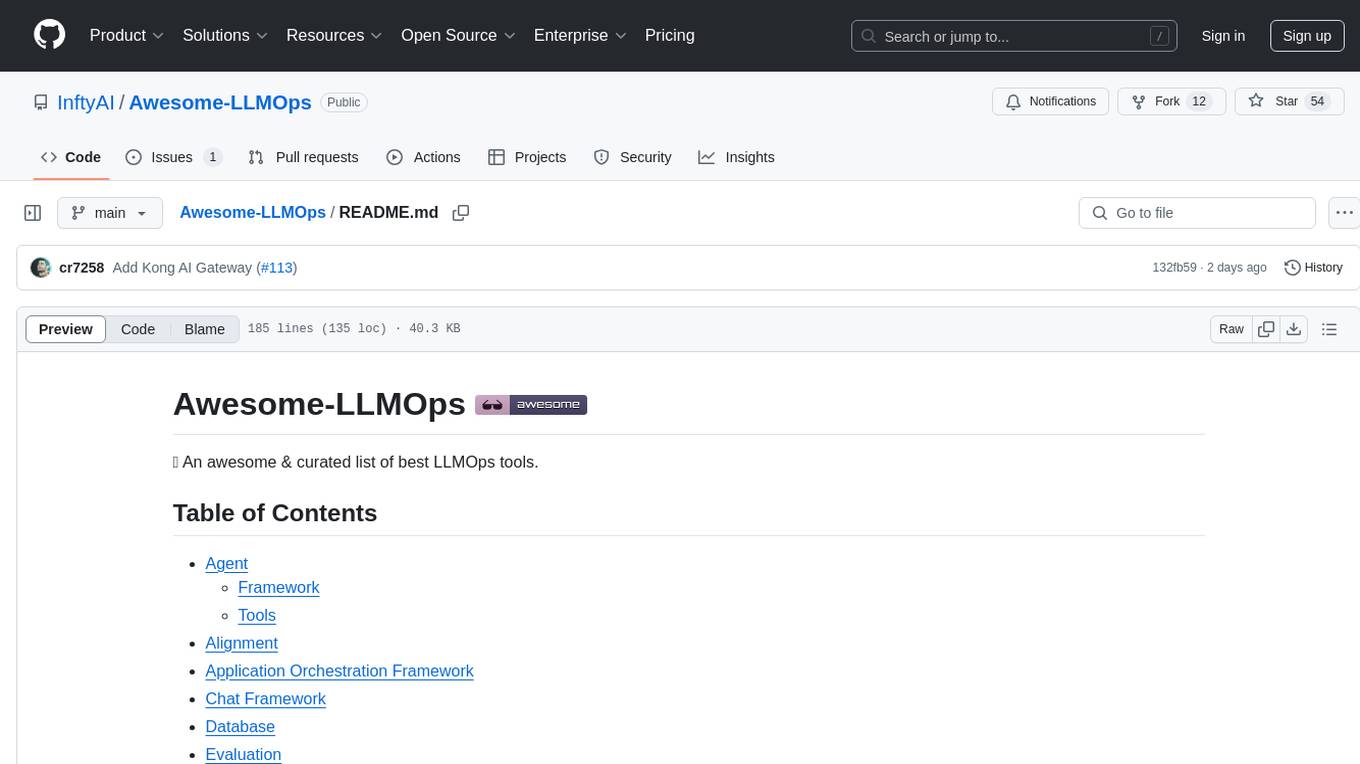
Awesome-LLMOps
Awesome-LLMOps is a curated list of the best LLMOps tools, providing a comprehensive collection of frameworks and tools for building, deploying, and managing large language models (LLMs) and AI agents. The repository includes a wide range of tools for tasks such as building multimodal AI agents, fine-tuning models, orchestrating applications, evaluating models, and serving models for inference. It covers various aspects of the machine learning operations (MLOps) lifecycle, from training to deployment and observability. The tools listed in this repository cater to the needs of developers, data scientists, and machine learning engineers working with large language models and AI applications.
20 - OpenAI Gpts
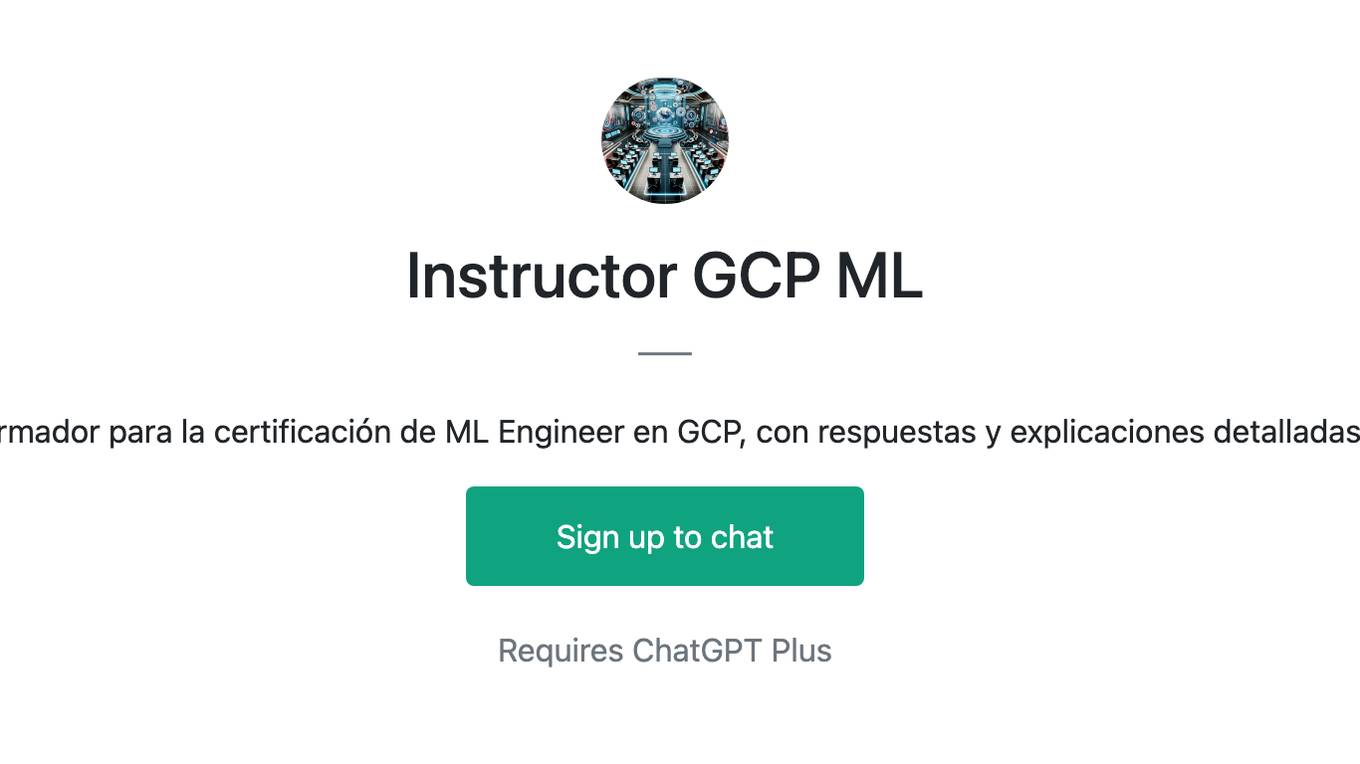
Instructor GCP ML
Formador para la certificación de ML Engineer en GCP, con respuestas y explicaciones detalladas.
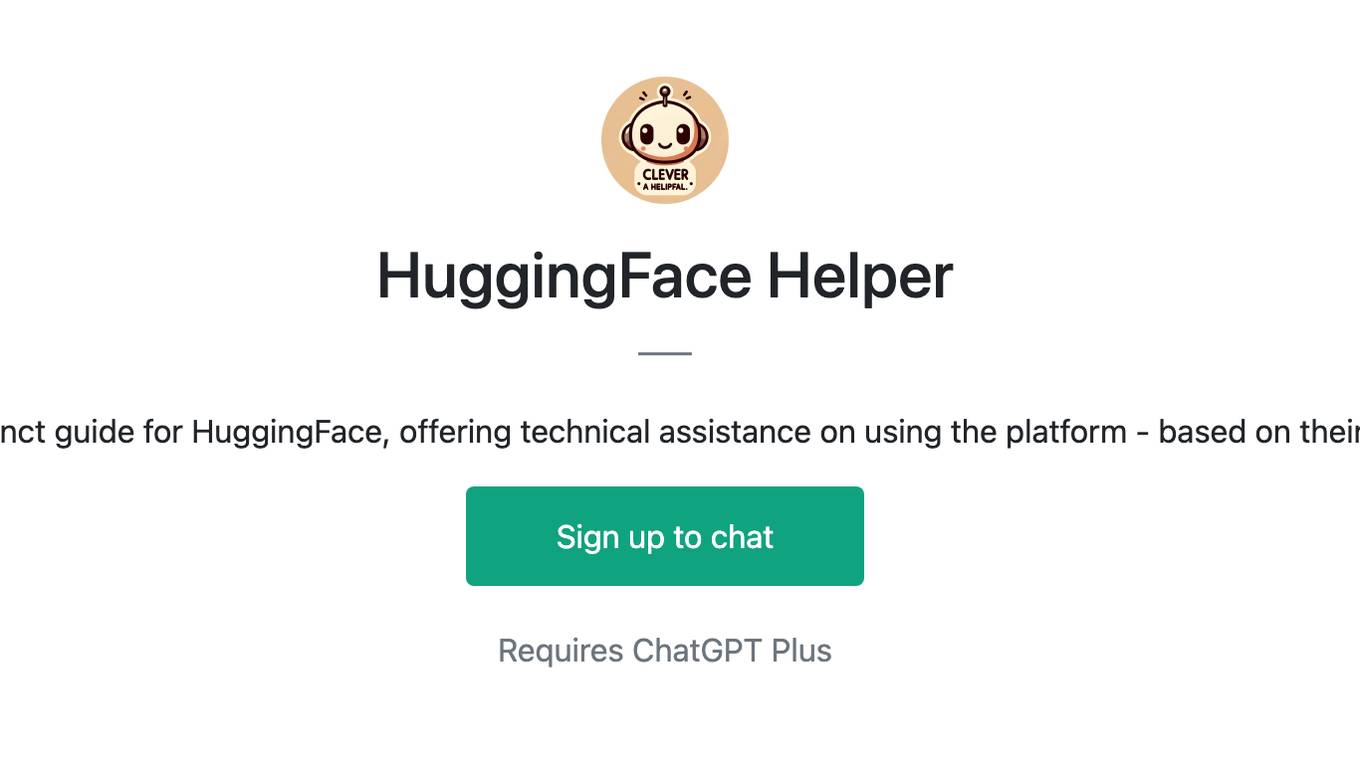
HuggingFace Helper
A witty yet succinct guide for HuggingFace, offering technical assistance on using the platform - based on their Learning Hub
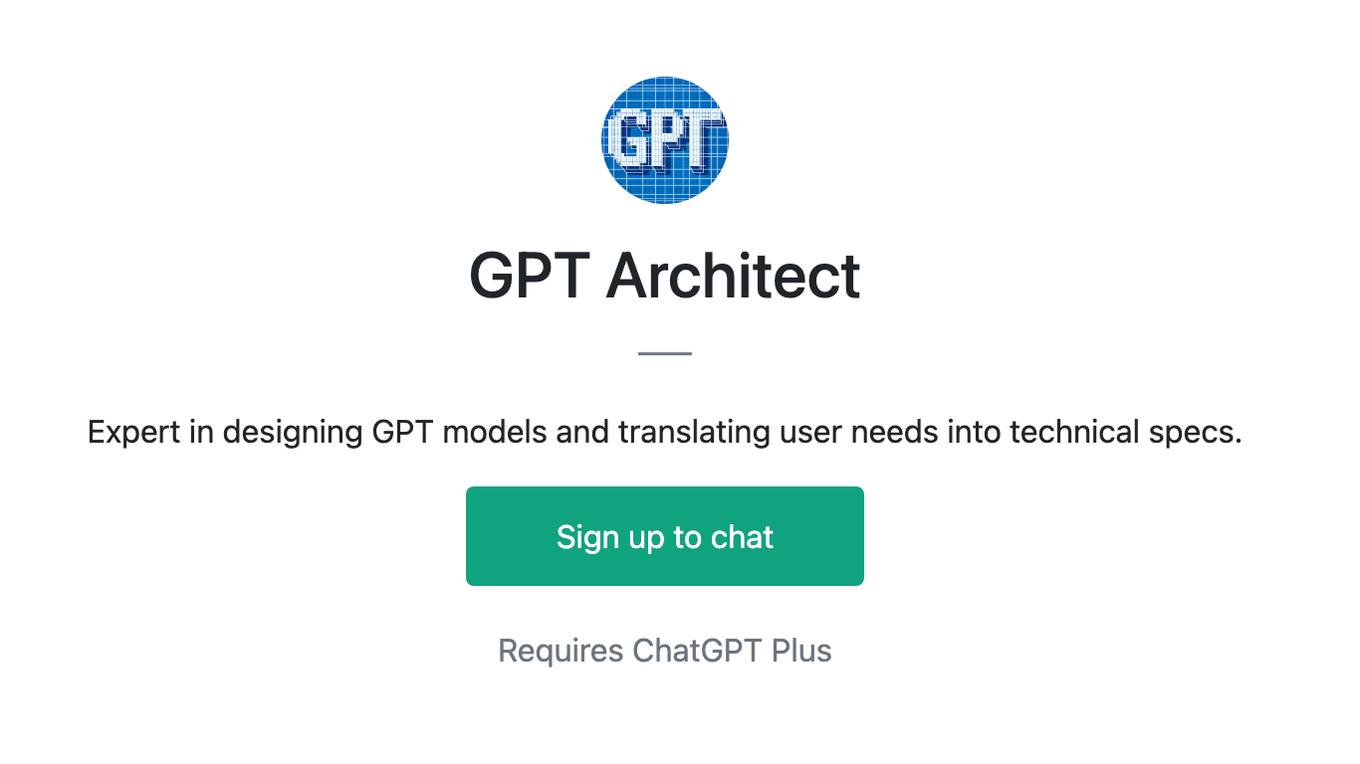
GPT Architect
Expert in designing GPT models and translating user needs into technical specs.
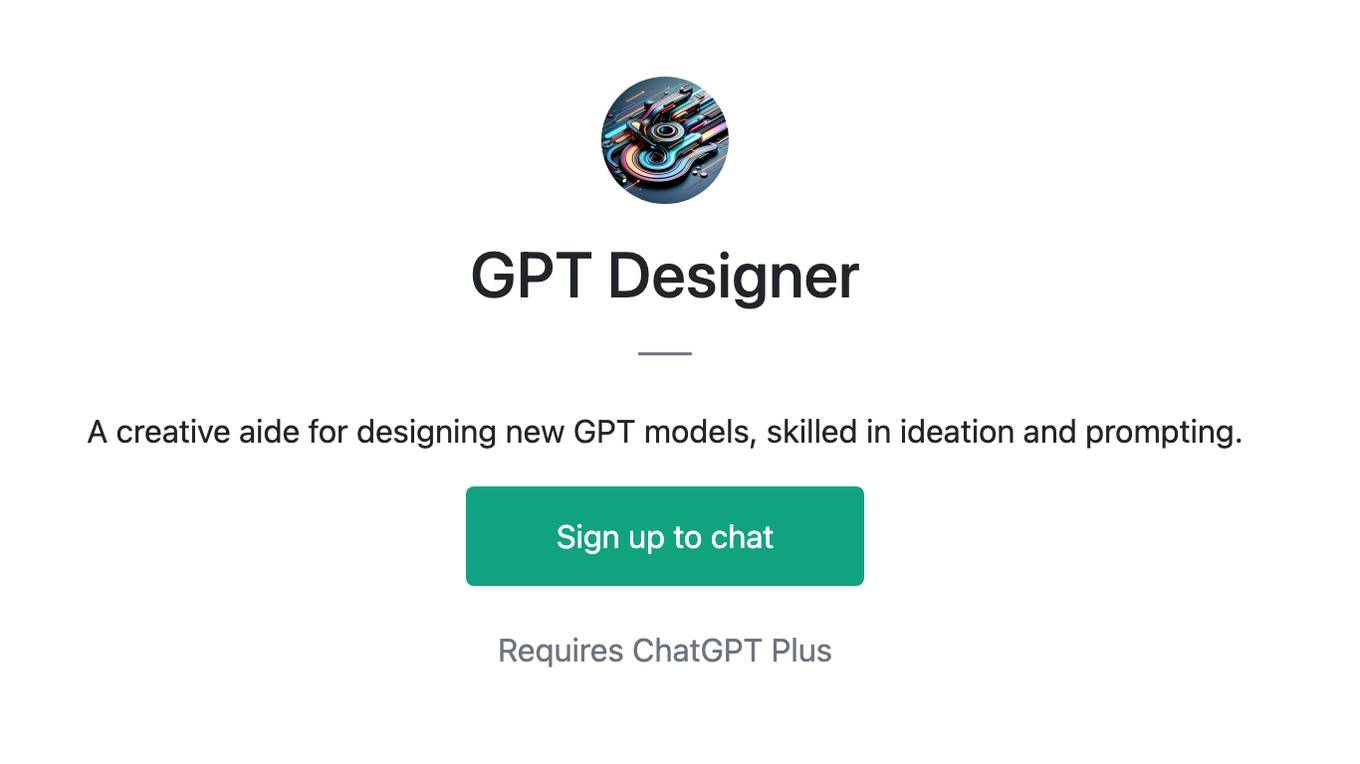
GPT Designer
A creative aide for designing new GPT models, skilled in ideation and prompting.
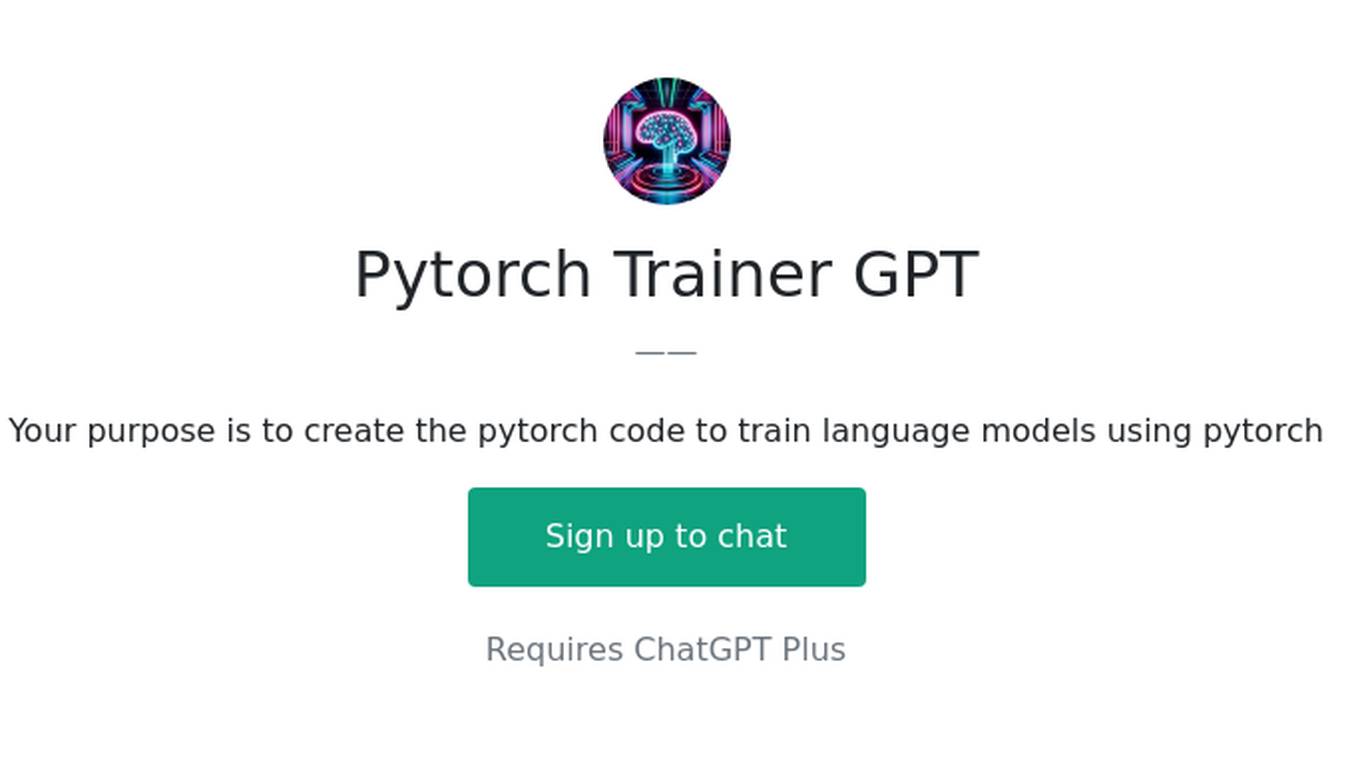
Pytorch Trainer GPT
Your purpose is to create the pytorch code to train language models using pytorch
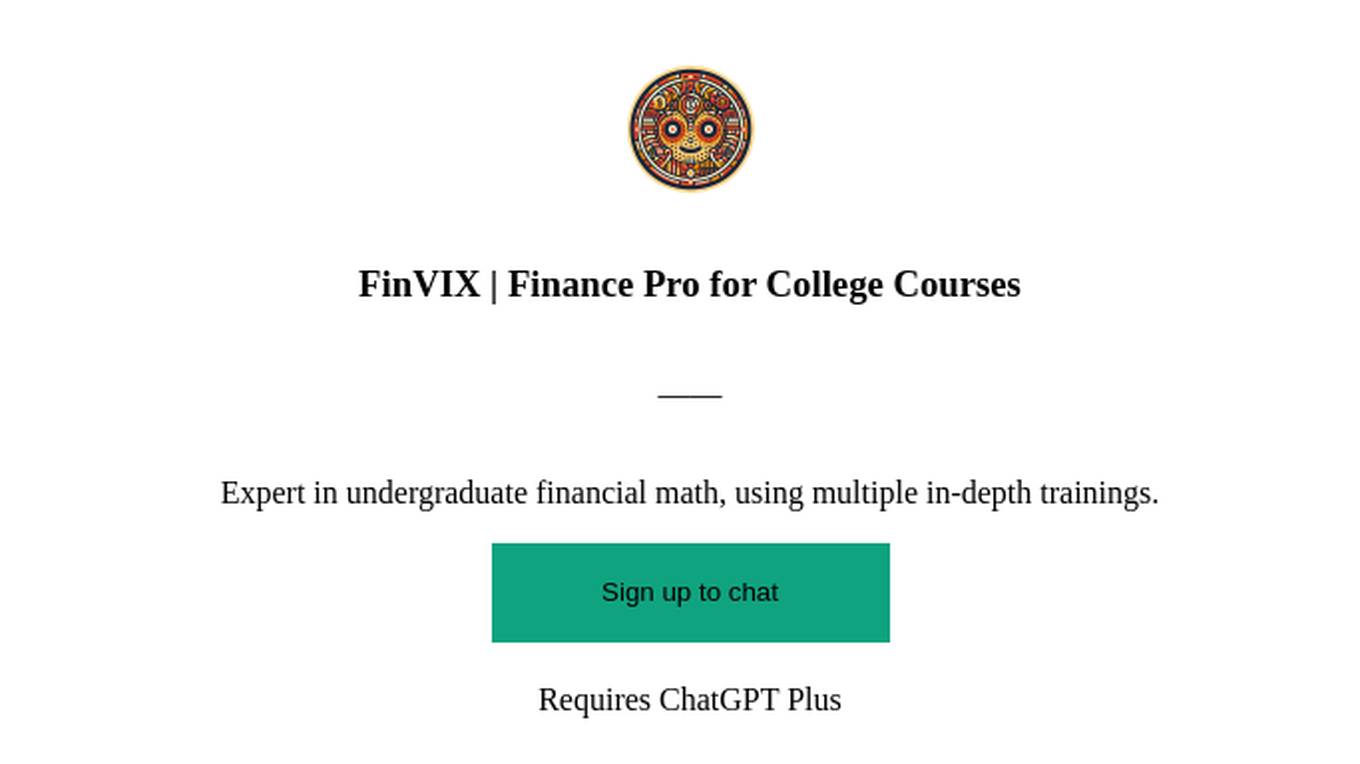
FinVIX | Finance Pro for College Courses
Expert in undergraduate financial math, using multiple in-depth trainings.
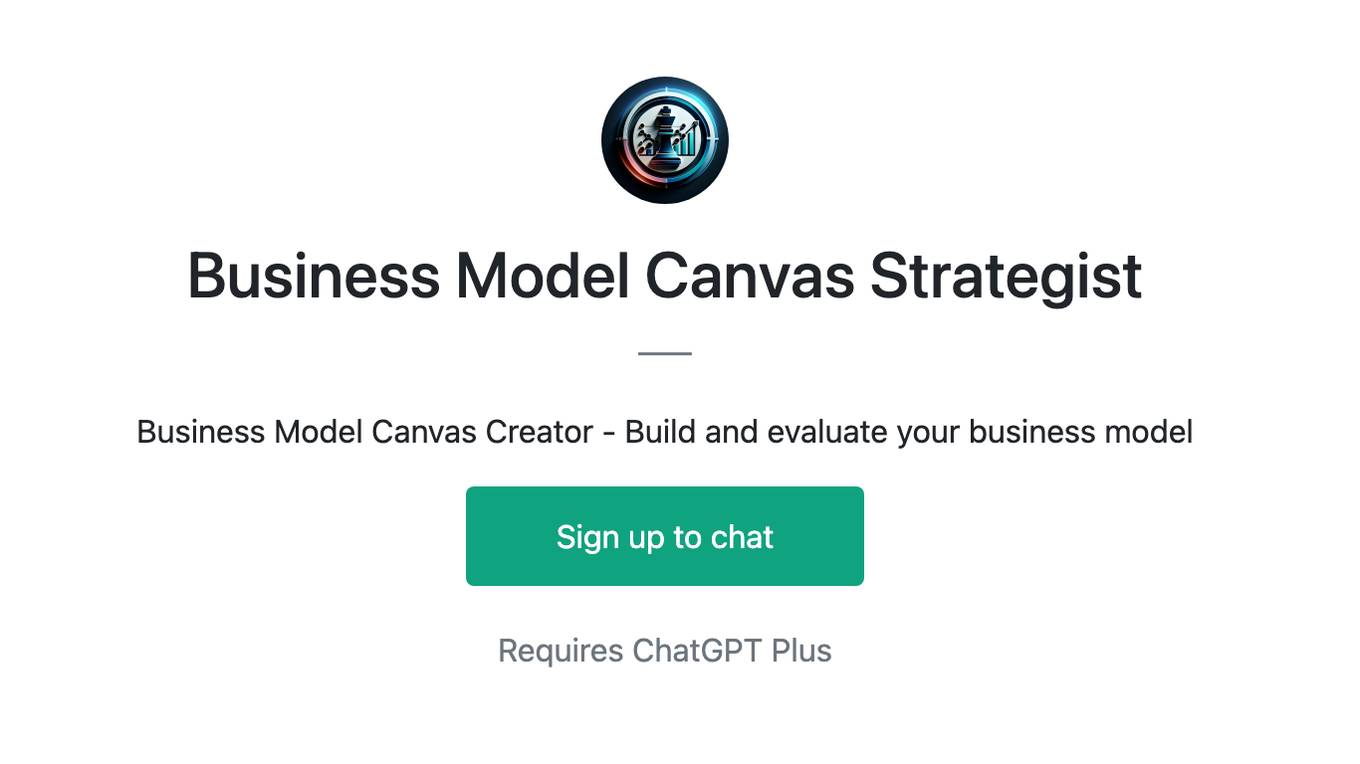
Business Model Canvas Strategist
Business Model Canvas Creator - Build and evaluate your business model
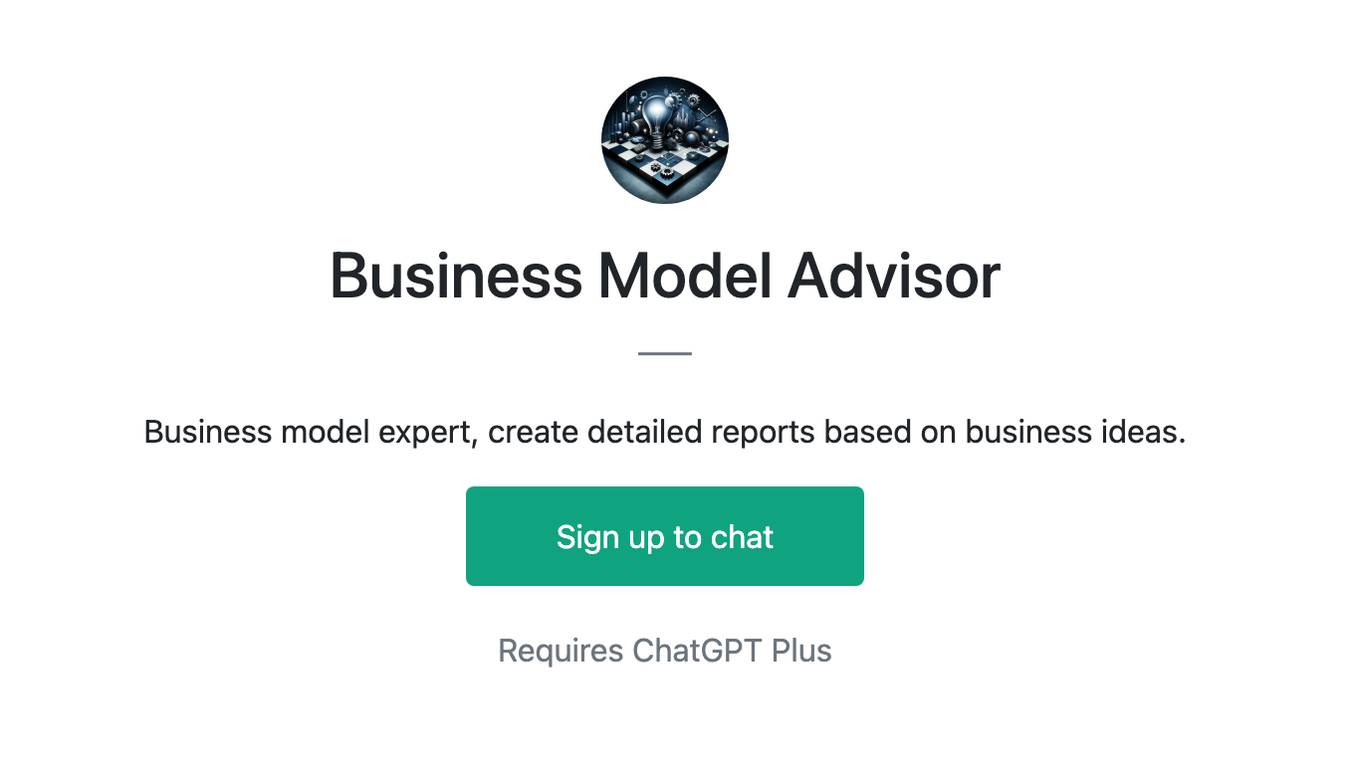
Business Model Advisor
Business model expert, create detailed reports based on business ideas.
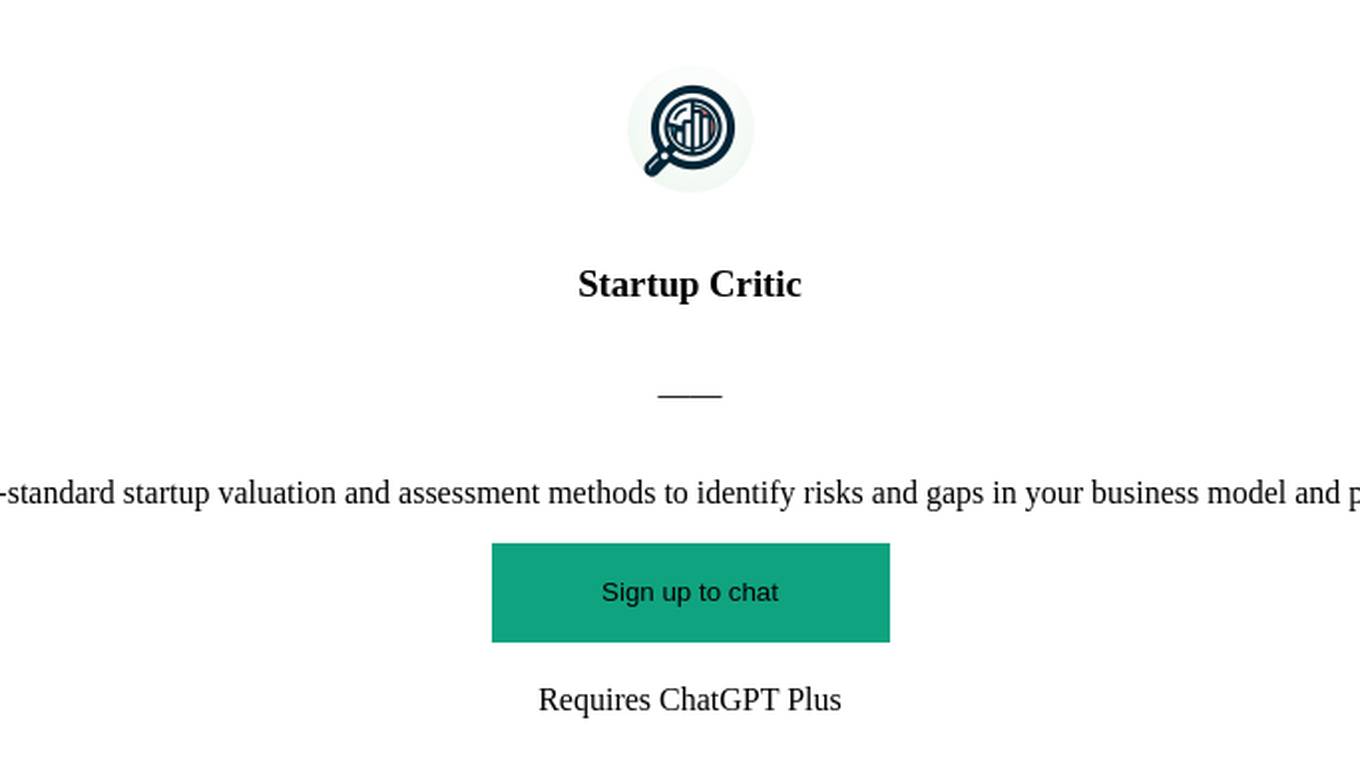
Startup Critic
Apply gold-standard startup valuation and assessment methods to identify risks and gaps in your business model and product ideas.
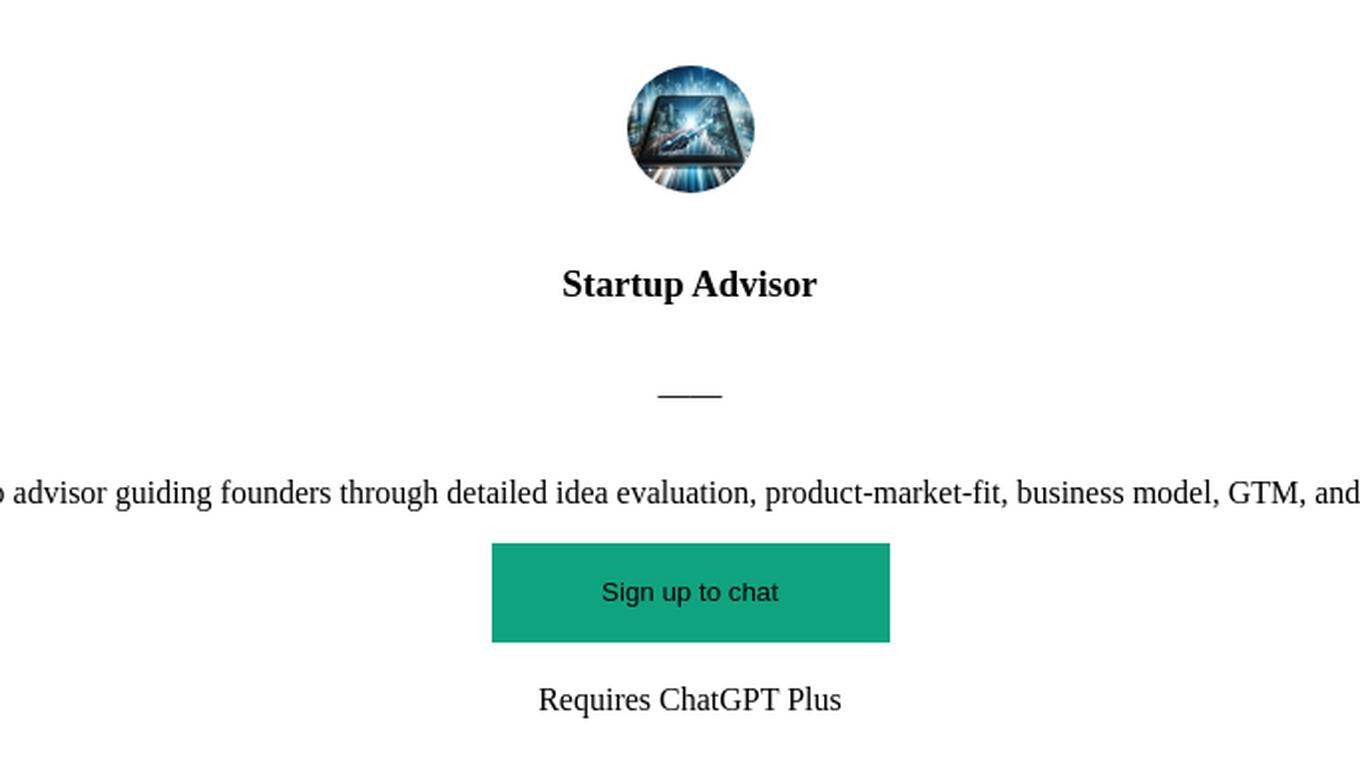
Startup Advisor
Startup advisor guiding founders through detailed idea evaluation, product-market-fit, business model, GTM, and scaling.
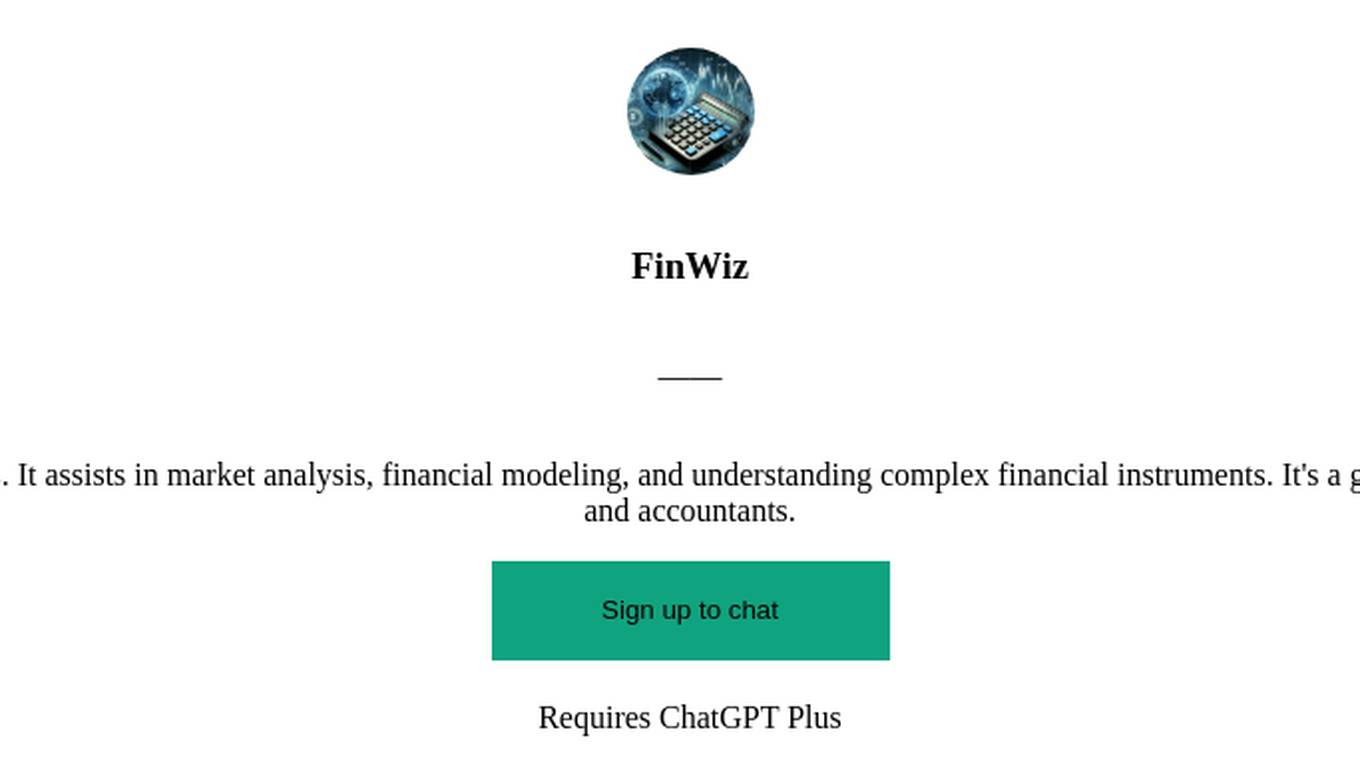
FinWiz
FinWiz-GPT is designed for finance professionals. It assists in market analysis, financial modeling, and understanding complex financial instruments. It's a great tool for financial analysts, investment bankers, and accountants.
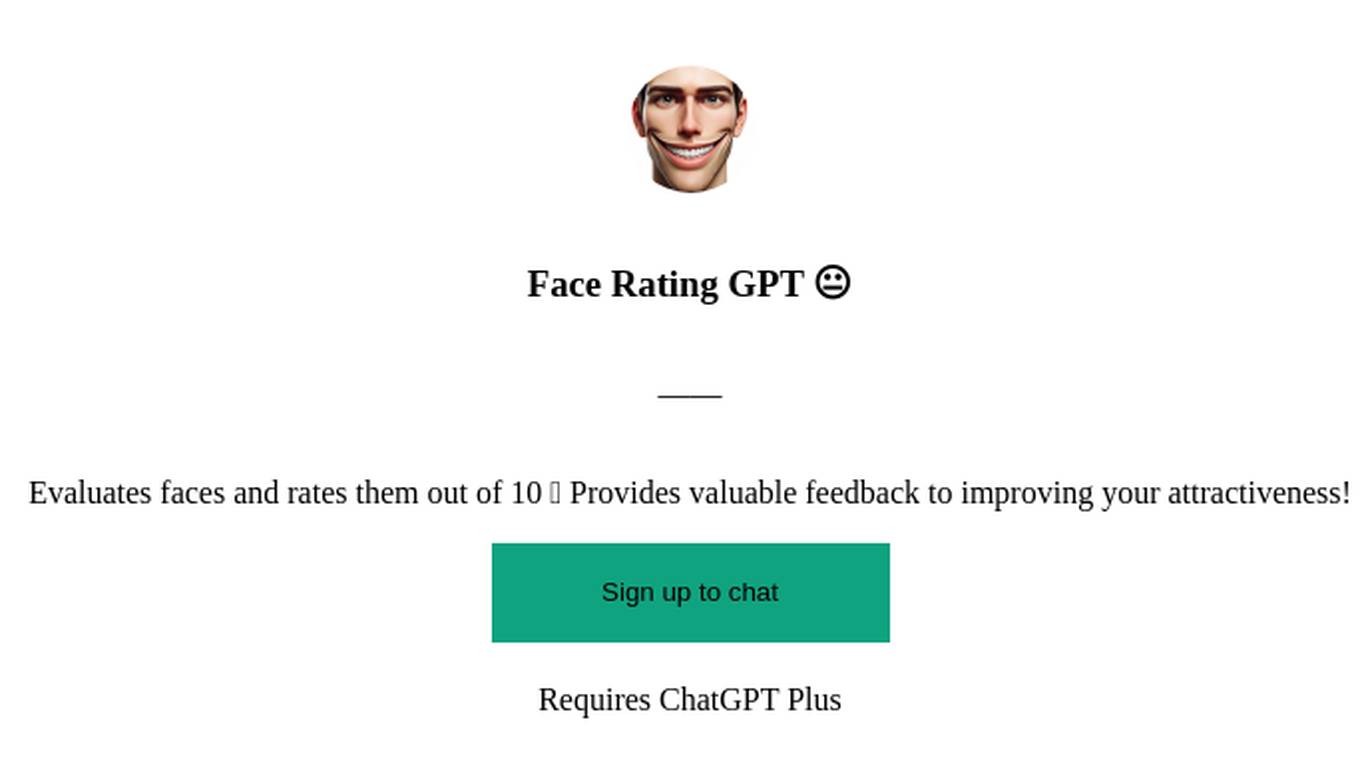
Face Rating GPT 😐
Evaluates faces and rates them out of 10 ⭐ Provides valuable feedback to improving your attractiveness!