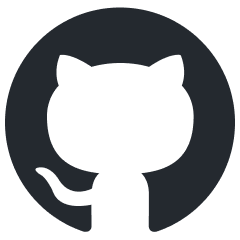
NeMo-Framework-Launcher
Provides end-to-end model development pipelines for LLMs and Multimodal models that can be launched on-prem or cloud-native.
Stars: 492
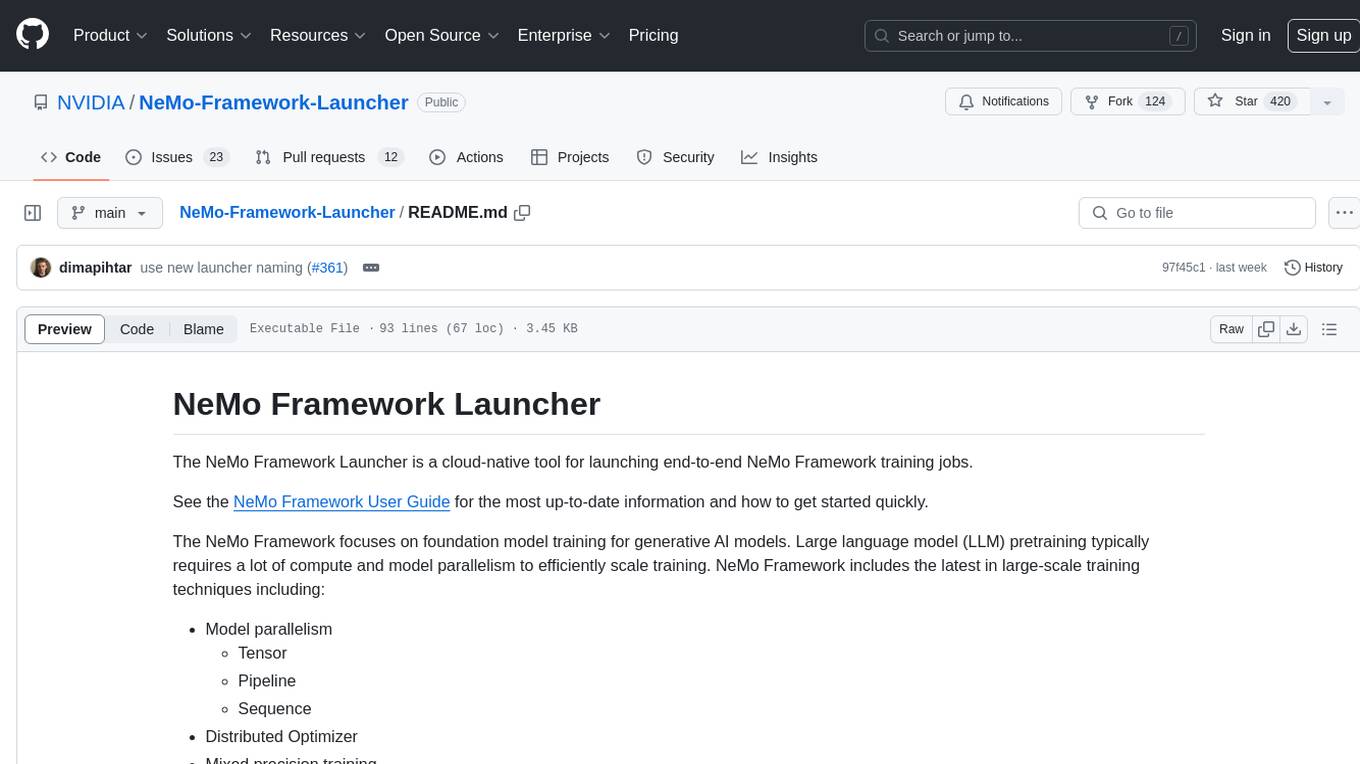
The NeMo Framework Launcher is a cloud-native tool designed for launching end-to-end NeMo Framework training jobs. It focuses on foundation model training for generative AI models, supporting large language model pretraining with techniques like model parallelism, tensor, pipeline, sequence, distributed optimizer, mixed precision training, and more. The tool scales to thousands of GPUs and can be used for training LLMs on trillions of tokens. It simplifies launching training jobs on cloud service providers or on-prem clusters, generating submission scripts, organizing job results, and supporting various model operations like fine-tuning, evaluation, export, and deployment.
README:
[!IMPORTANT]
NeMo Framework Launcher is compatible with NeMo version 1.0 only.
The NeMo Framework Launcher is a cloud-native tool for launching end-to-end NeMo Framework training jobs.
Please refer to the NeMo Launcher Guide for more information.
The NeMo Framework focuses on foundation model training for generative AI models. Large language model (LLM) pretraining typically requires a lot of compute and model parallelism to efficiently scale training. NeMo Framework includes the latest in large-scale training techniques including:
- Model parallelism
- Tensor
- Pipeline
- Sequence
- Distributed Optimizer
- Mixed precision training
- FP8
- BF16
- Distributed Checkpointing
- Community Models
- LLAMA-2
NeMo Framework model training scales to 1000's of GPUs and can be used for training LLMs on trillions of tokens.
The Launcher is designed to be a simple and easy to use tool for launching NeMo FW training jobs on CSPs or on-prem clusters. The launcher is typically used from a head node and only requires a minimal python installation.
The Launcher will generate and launch submission scripts for the cluster scheduler and will also organize and store jobs results. Tested configuration files are included with the launcher but anything in a configuration file can be easily modified by the user.
The NeMo FW Launcher is tested with the NeMo FW Container which can be applied for here. Access is automatic. Users may also easily configure the launcher to use any container image that they want to provide.
The NeMo FW launcher supports:
- Cluster setup and configuration
- Data downloading, curating, and processing
- Model parallel configuration
- Model training
- Model fine-tuning (SFT and PEFT)
- Model evaluation
- Model export and deployment
Some of the models that we support include:
- GPT
- Pretraining, Fine-tuning, SFT, PEFT
- BERT
- T5/MT5
- PEFT, MoE (non-expert)
See the Feature Matrix for more details.
The NeMo Framework Launcher should be installed on a head node or a local machine in a virtual python environment.
git clone https://github.com/NVIDIA/NeMo-Framework-Launcher.git
cd NeMo-Framework-Launcher
pip install -r requirements.txt
The best way to get started with the NeMo Framework Launcher is go through the NeMo Framework Playbooks
After everything is configured in the .yaml
files, the Launcher can be run with:
python main.py
Since the Launcher uses Hydra,
any configuration can be overridden directly in the .yaml
file or via the command line.
See Hydra's override grammar for more information.
Contributions are welcome!
To contribute to the NeMo Framework Launcher, simply create a pull request with the changes on GitHub. After the pull request is reviewed by a NeMo FW Developer, approved, and passes the unit and CI tests, then it will be merged.
The NeMo Framework Launcher is licensed under the Apache 2.0 License
For Tasks:
Click tags to check more tools for each tasksFor Jobs:
Alternative AI tools for NeMo-Framework-Launcher
Similar Open Source Tools
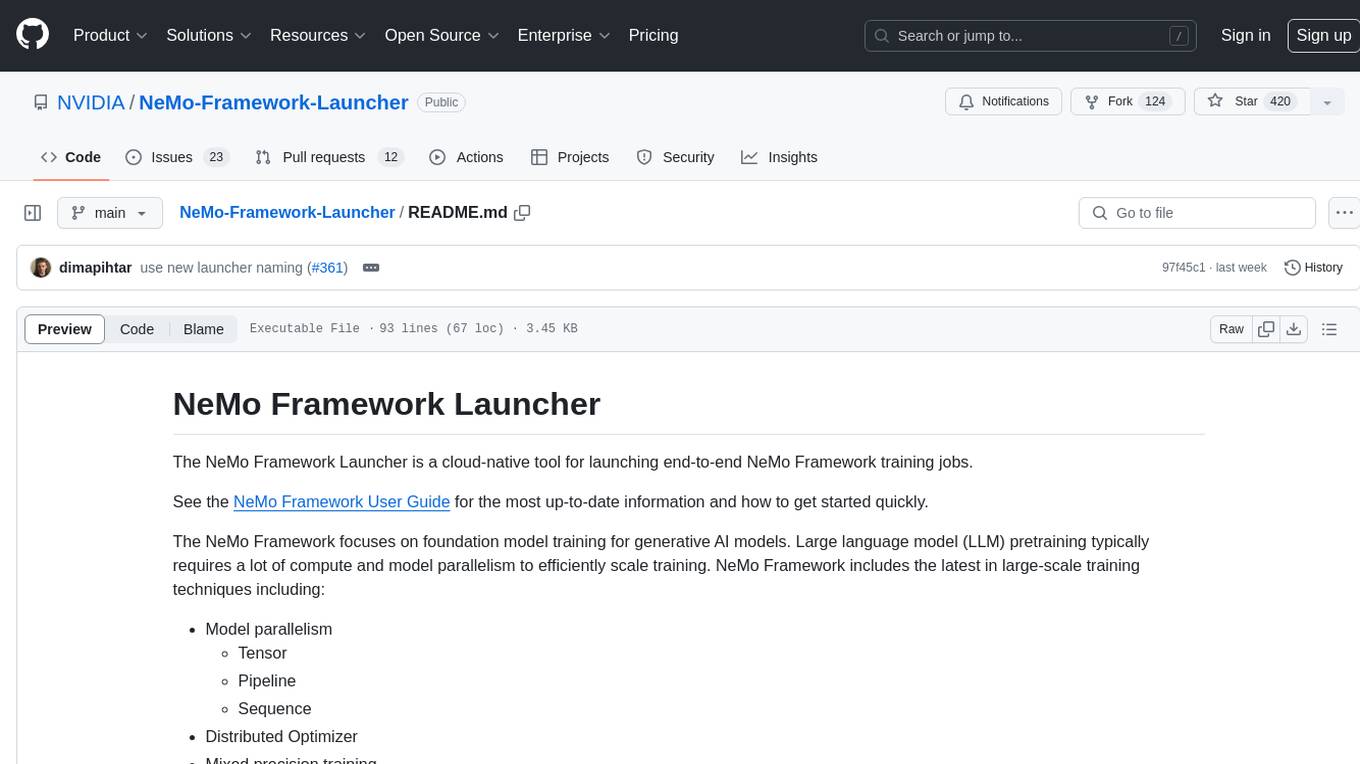
NeMo-Framework-Launcher
The NeMo Framework Launcher is a cloud-native tool designed for launching end-to-end NeMo Framework training jobs. It focuses on foundation model training for generative AI models, supporting large language model pretraining with techniques like model parallelism, tensor, pipeline, sequence, distributed optimizer, mixed precision training, and more. The tool scales to thousands of GPUs and can be used for training LLMs on trillions of tokens. It simplifies launching training jobs on cloud service providers or on-prem clusters, generating submission scripts, organizing job results, and supporting various model operations like fine-tuning, evaluation, export, and deployment.
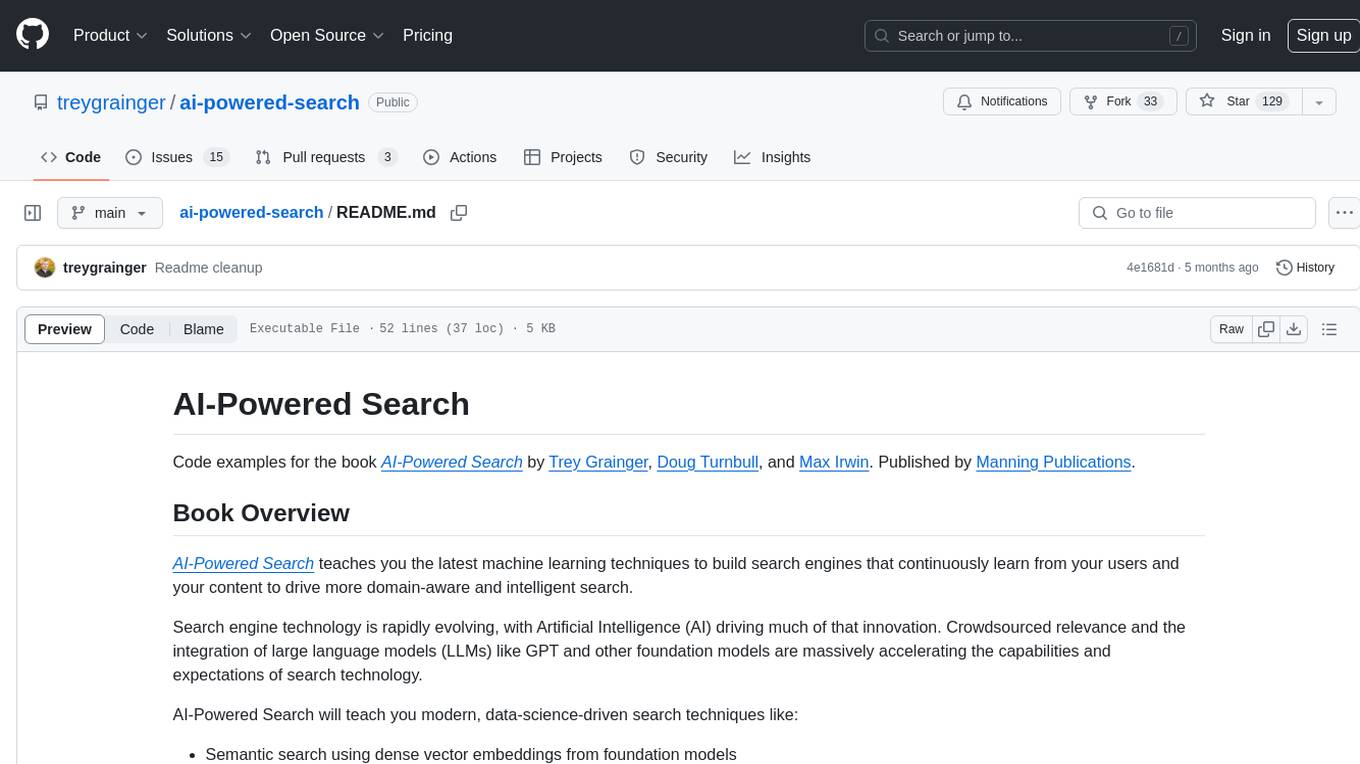
ai-powered-search
AI-Powered Search provides code examples for the book 'AI-Powered Search' by Trey Grainger, Doug Turnbull, and Max Irwin. The book teaches modern machine learning techniques for building search engines that continuously learn from users and content to deliver more intelligent and domain-aware search experiences. It covers semantic search, retrieval augmented generation, question answering, summarization, fine-tuning transformer-based models, personalized search, machine-learned ranking, click models, and more. The code examples are in Python, leveraging PySpark for data processing and Apache Solr as the default search engine. The repository is open source under the Apache License, Version 2.0.
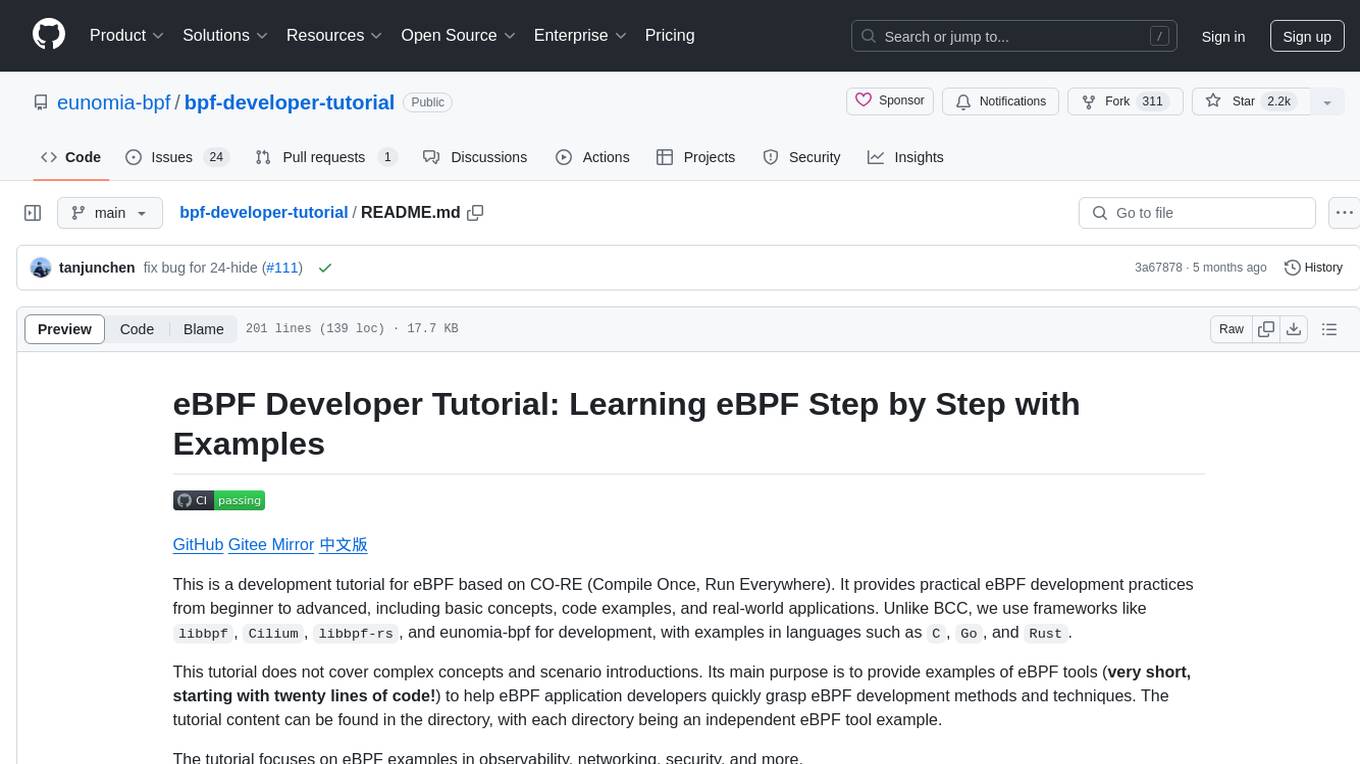
bpf-developer-tutorial
This is a development tutorial for eBPF based on CO-RE (Compile Once, Run Everywhere). It provides practical eBPF development practices from beginner to advanced, including basic concepts, code examples, and real-world applications. The tutorial focuses on eBPF examples in observability, networking, security, and more. It aims to help eBPF application developers quickly grasp eBPF development methods and techniques through examples in languages such as C, Go, and Rust. The tutorial is structured with independent eBPF tool examples in each directory, covering topics like kprobes, fentry, opensnoop, uprobe, sigsnoop, execsnoop, exitsnoop, runqlat, hardirqs, and more. The project is based on libbpf and frameworks like libbpf, Cilium, libbpf-rs, and eunomia-bpf for development.
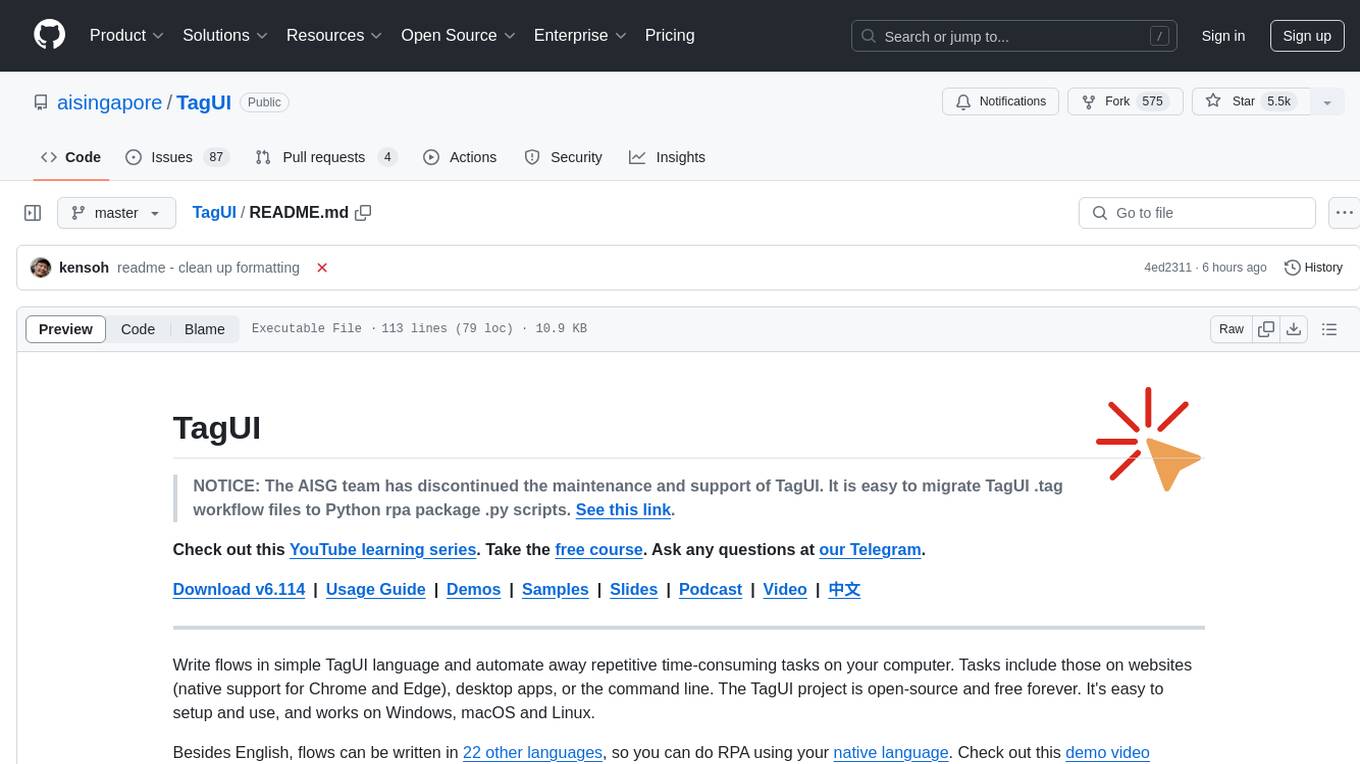
TagUI
TagUI is an open-source RPA tool that allows users to automate repetitive tasks on their computer, including tasks on websites, desktop apps, and the command line. It supports multiple languages and offers features like interacting with identifiers, automating data collection, moving data between TagUI and Excel, and sending Telegram notifications. Users can create RPA robots using MS Office Plug-ins or text editors, run TagUI on the cloud, and integrate with other RPA tools. TagUI prioritizes enterprise security by running on users' computers and not storing data. It offers detailed logs, enterprise installation guides, and support for centralised reporting.
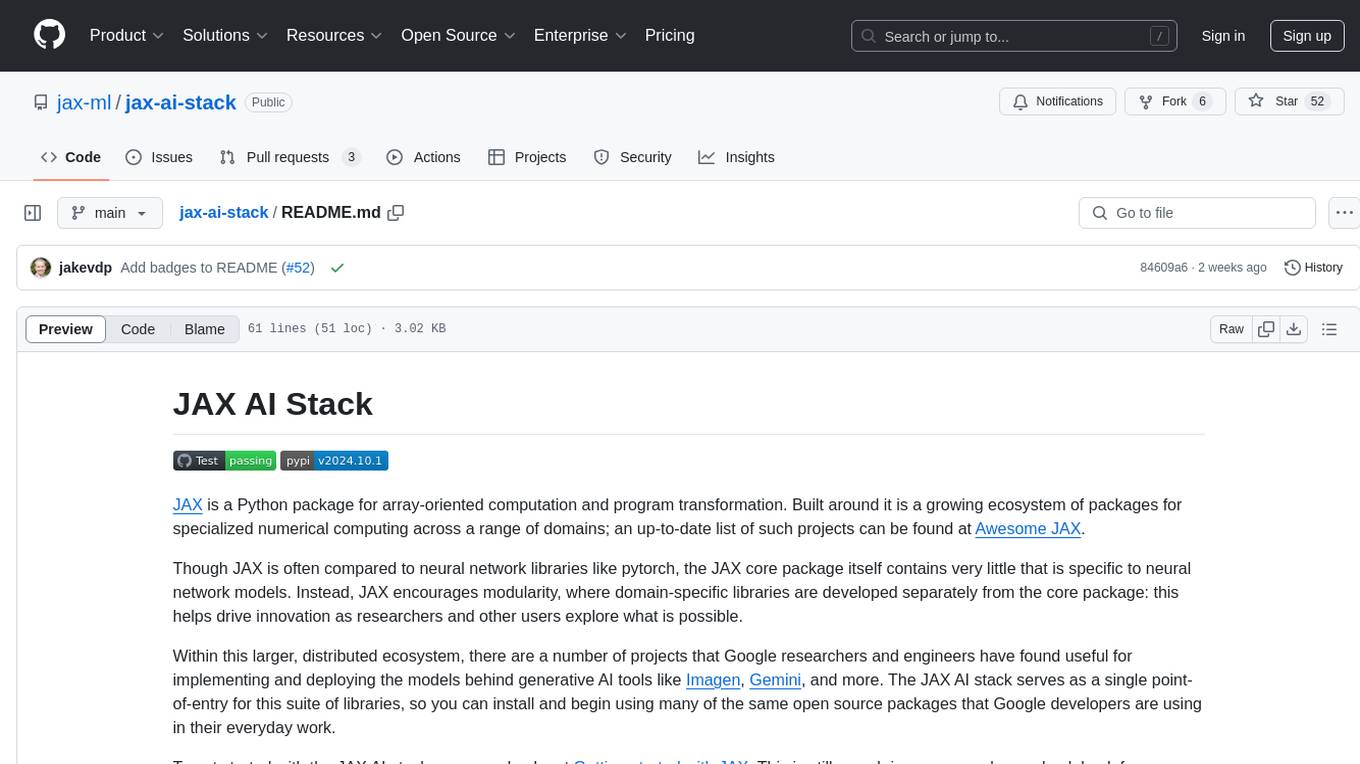
jax-ai-stack
JAX AI Stack is a suite of libraries built around the JAX Python package for array-oriented computation and program transformation. It provides a growing ecosystem of packages for specialized numerical computing across various domains, encouraging modularity and innovation in domain-specific libraries. The stack includes core packages like JAX, flax for building neural networks, ml_dtypes for NumPy dtype extensions, optax for gradient processing and optimization, and orbax for checkpointing and persistence utilities. Optional packages like grain data loader and tensorflow are also available for installation.
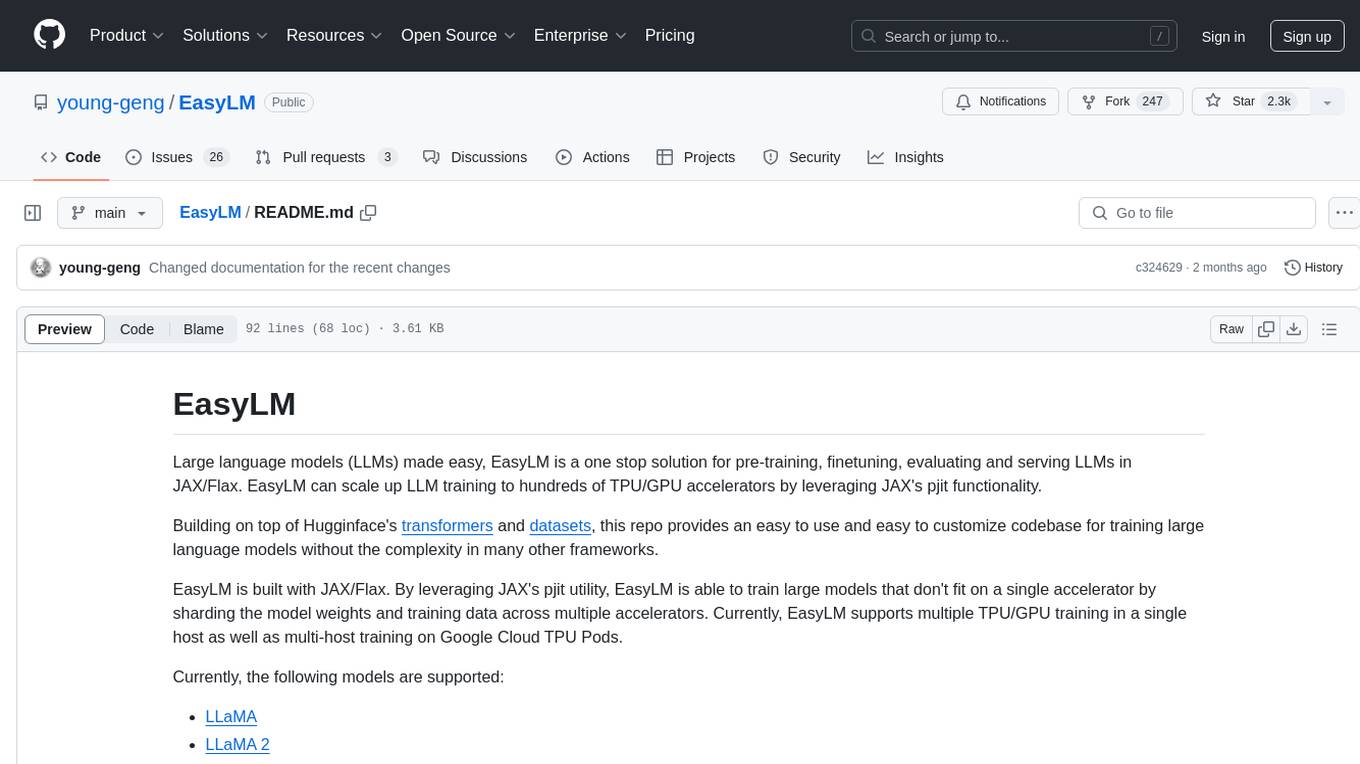
EasyLM
EasyLM is a one-stop solution for pre-training, fine-tuning, evaluating, and serving large language models in JAX/Flax. It simplifies the process by leveraging JAX's pjit functionality to scale up training to multiple TPU/GPU accelerators. Built on top of Huggingface's transformers and datasets, EasyLM offers an easy-to-use and customizable codebase for training large language models without the complexity found in other frameworks. It supports sharding model weights and training data across multiple accelerators, enabling multi-TPU/GPU training on a single host or across multiple hosts on Google Cloud TPU Pods. EasyLM currently supports models like LLaMA, LLaMA 2, and LLaMA 3.
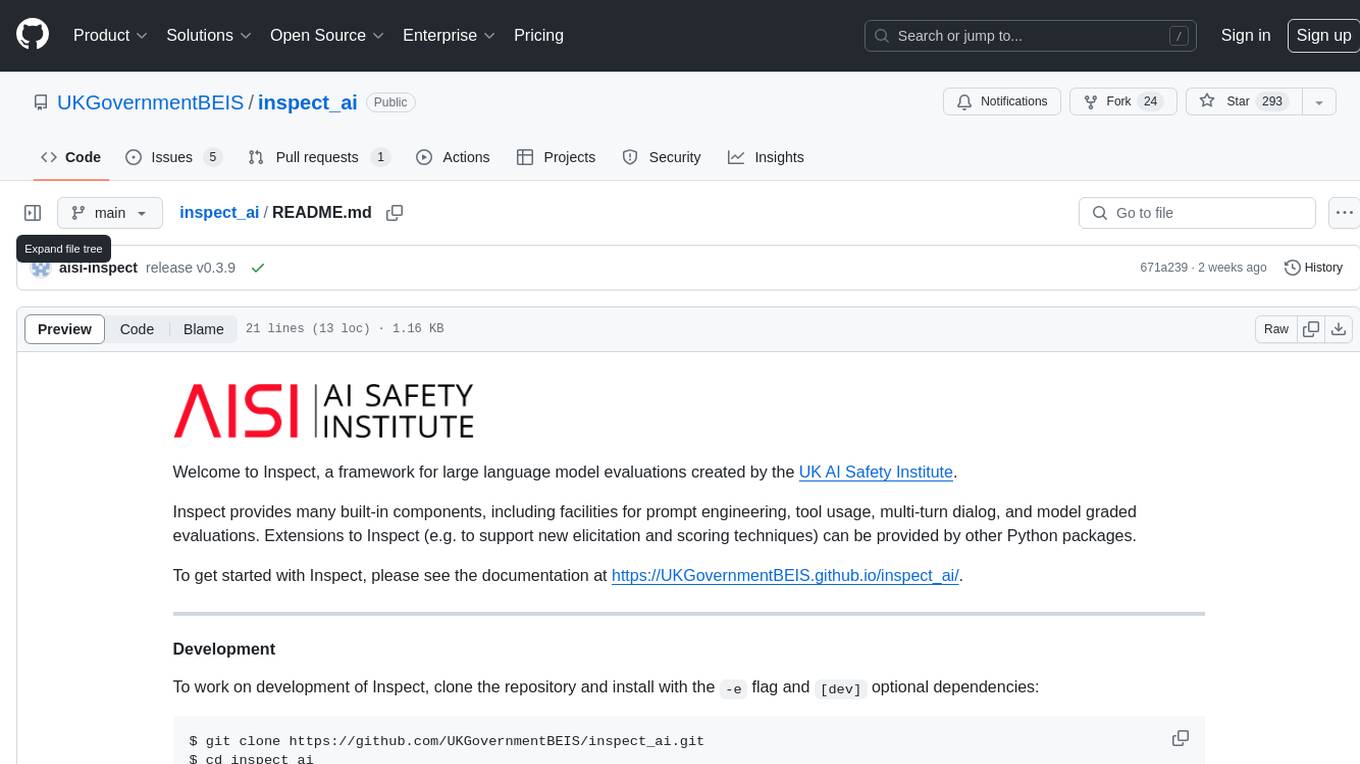
inspect_ai
Inspect AI is a framework developed by the UK AI Safety Institute for evaluating large language models. It offers various built-in components for prompt engineering, tool usage, multi-turn dialog, and model graded evaluations. Users can extend Inspect by adding new elicitation and scoring techniques through additional Python packages. The tool aims to provide a comprehensive solution for assessing the performance and safety of language models.
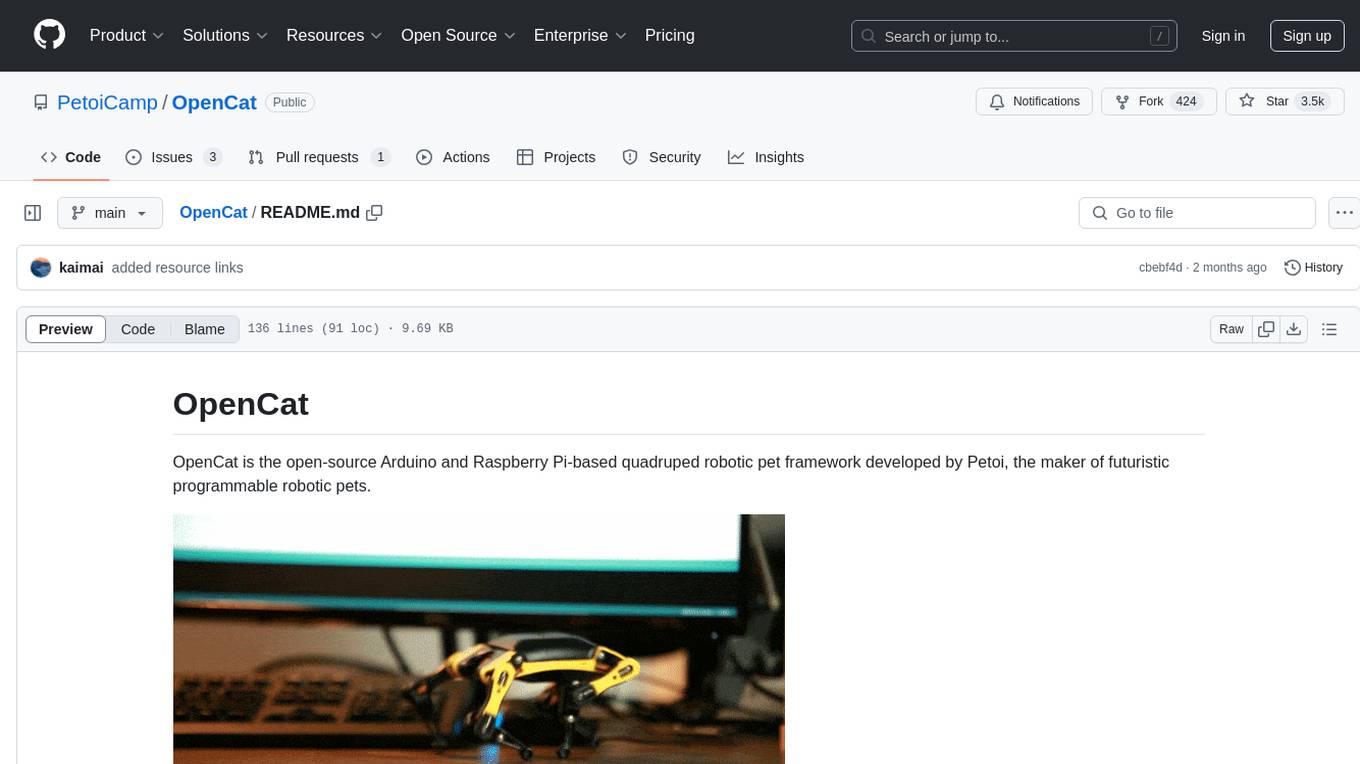
OpenCat
OpenCat is an open-source Arduino and Raspberry Pi-based quadruped robotic pet framework developed by Petoi. It aims to foster collaboration in quadruped robotics research, education, and engineering development of agile and affordable quadruped robot pets. The project provides a base open source platform for creating programmable gaits, locomotion, and deployment of inverse kinematics quadruped robots, enabling simulations to the real world via block-based coding/C/C++/Python programming languages. Users have deployed various robotics/AI/IoT applications and the project has successfully crowdfunded mini robot kits, shipped worldwide, and established a production line for affordable robotic kits and accessories.
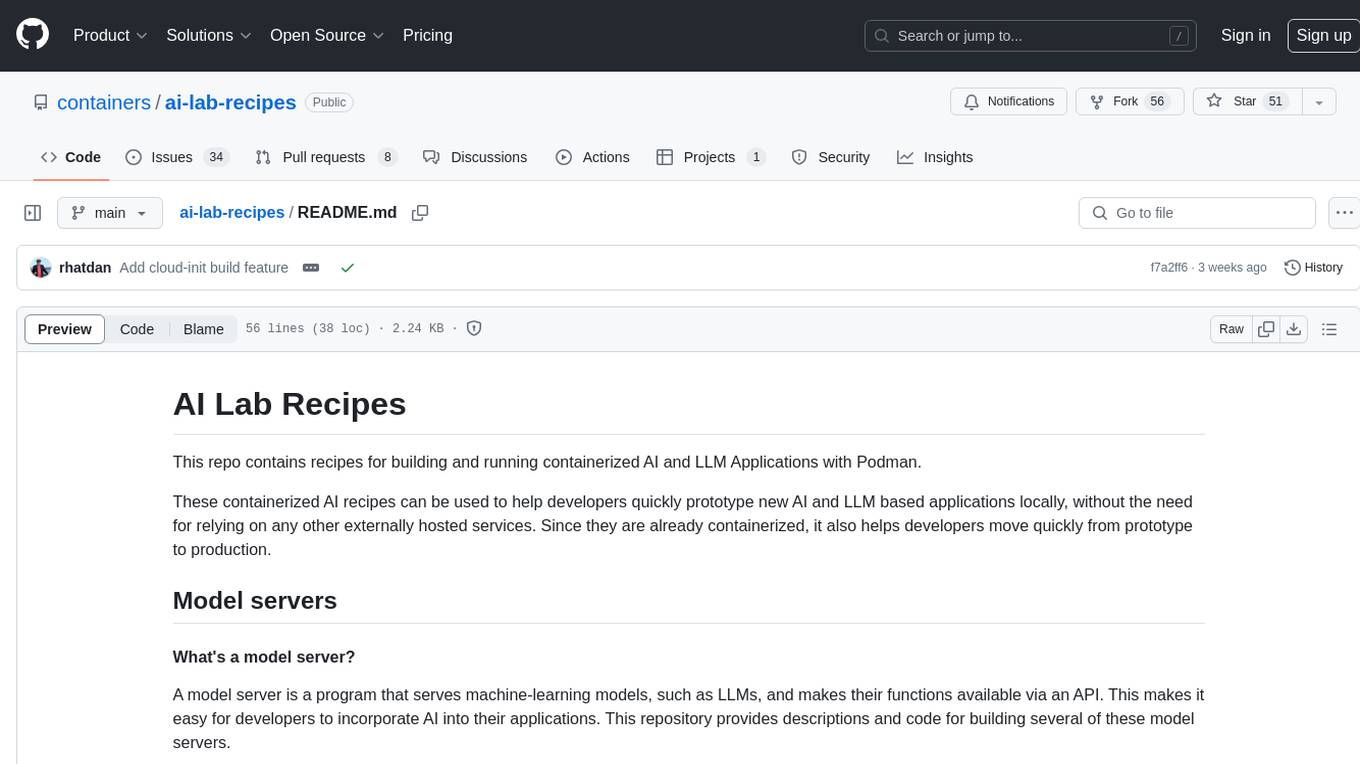
ai-lab-recipes
This repository contains recipes for building and running containerized AI and LLM applications with Podman. It provides model servers that serve machine-learning models via an API, allowing developers to quickly prototype new AI applications locally. The recipes include components like model servers and AI applications for tasks such as chat, summarization, object detection, etc. Images for sample applications and models are available in `quay.io`, and bootable containers for AI training on Linux OS are enabled.
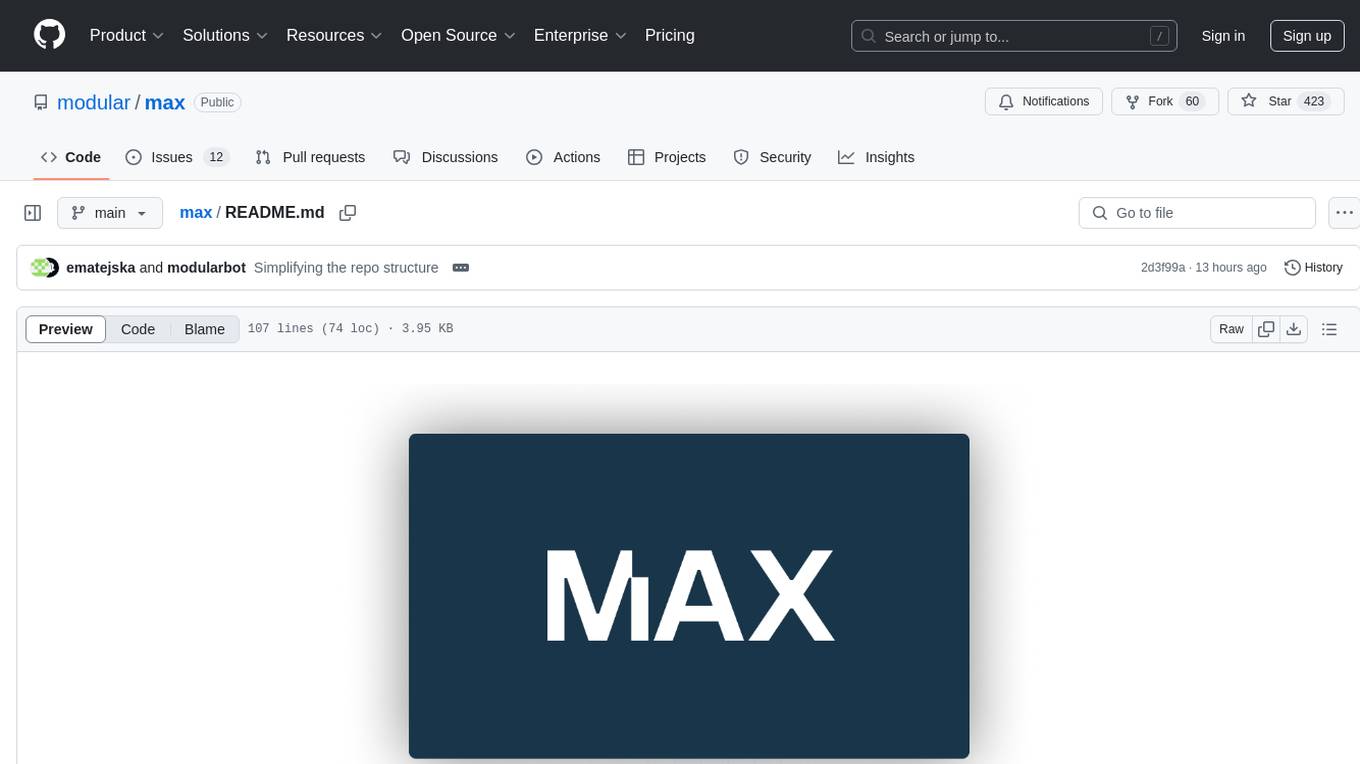
max
The Modular Accelerated Xecution (MAX) platform is an integrated suite of AI libraries, tools, and technologies that unifies commonly fragmented AI deployment workflows. MAX accelerates time to market for the latest innovations by giving AI developers a single toolchain that unlocks full programmability, unparalleled performance, and seamless hardware portability.
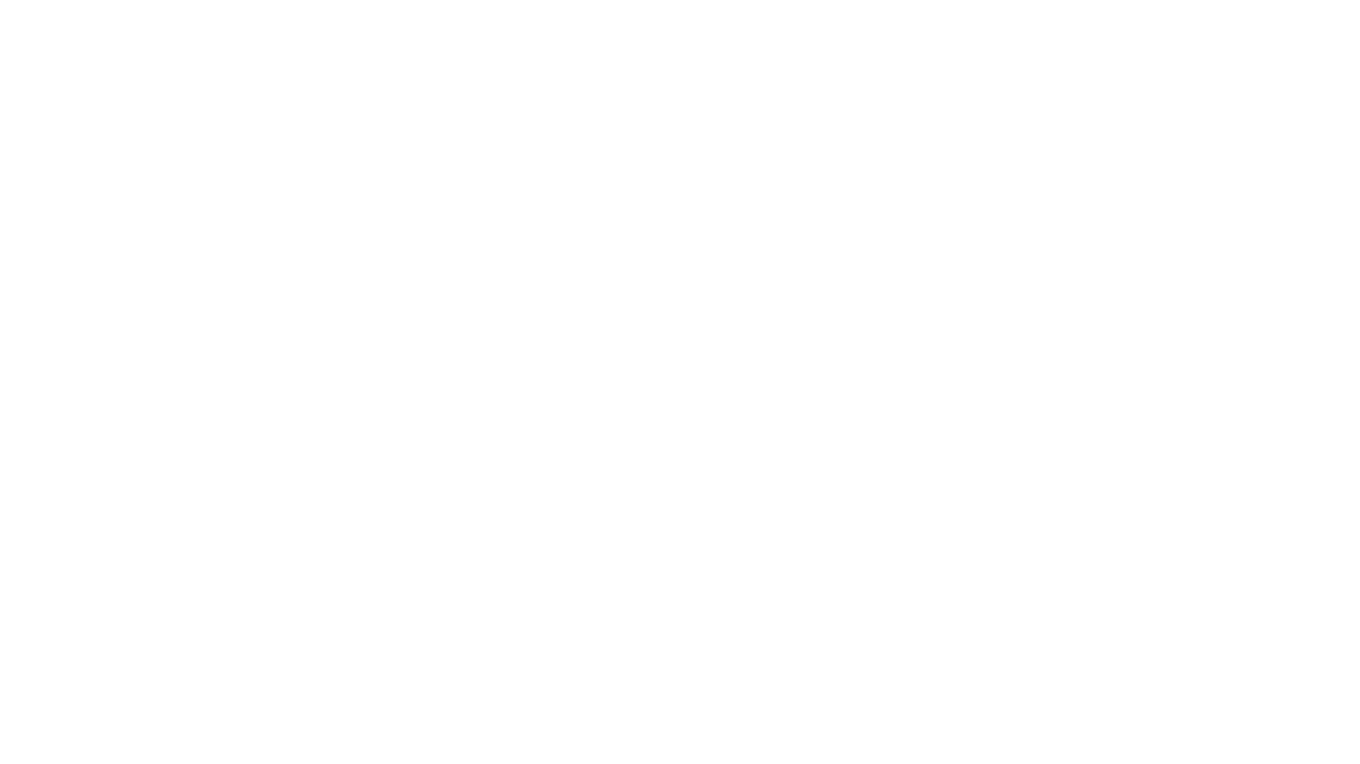
Chinese-Tiny-LLM
Chinese-Tiny-LLM is a repository containing procedures for cleaning Chinese web corpora and pre-training code. It introduces CT-LLM, a 2B parameter language model focused on the Chinese language. The model primarily uses Chinese data from a 1,200 billion token corpus, showing excellent performance in Chinese language tasks. The repository includes tools for filtering, deduplication, and pre-training, aiming to encourage further research and innovation in language model development.
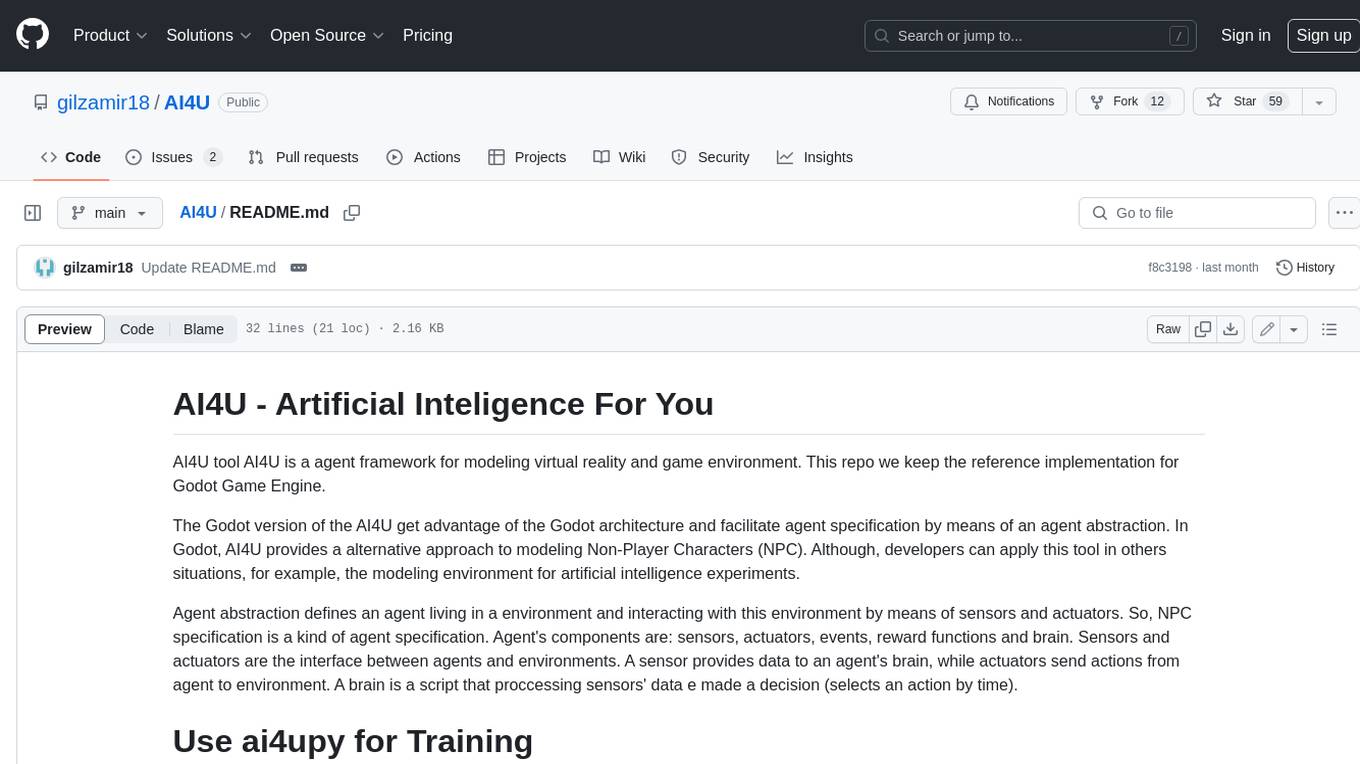
AI4U
AI4U is a tool that provides a framework for modeling virtual reality and game environments. It offers an alternative approach to modeling Non-Player Characters (NPCs) in Godot Game Engine. AI4U defines an agent living in an environment and interacting with it through sensors and actuators. Sensors provide data to the agent's brain, while actuators send actions from the agent to the environment. The brain processes the sensor data and makes decisions (selects an action by time). AI4U can also be used in other situations, such as modeling environments for artificial intelligence experiments.
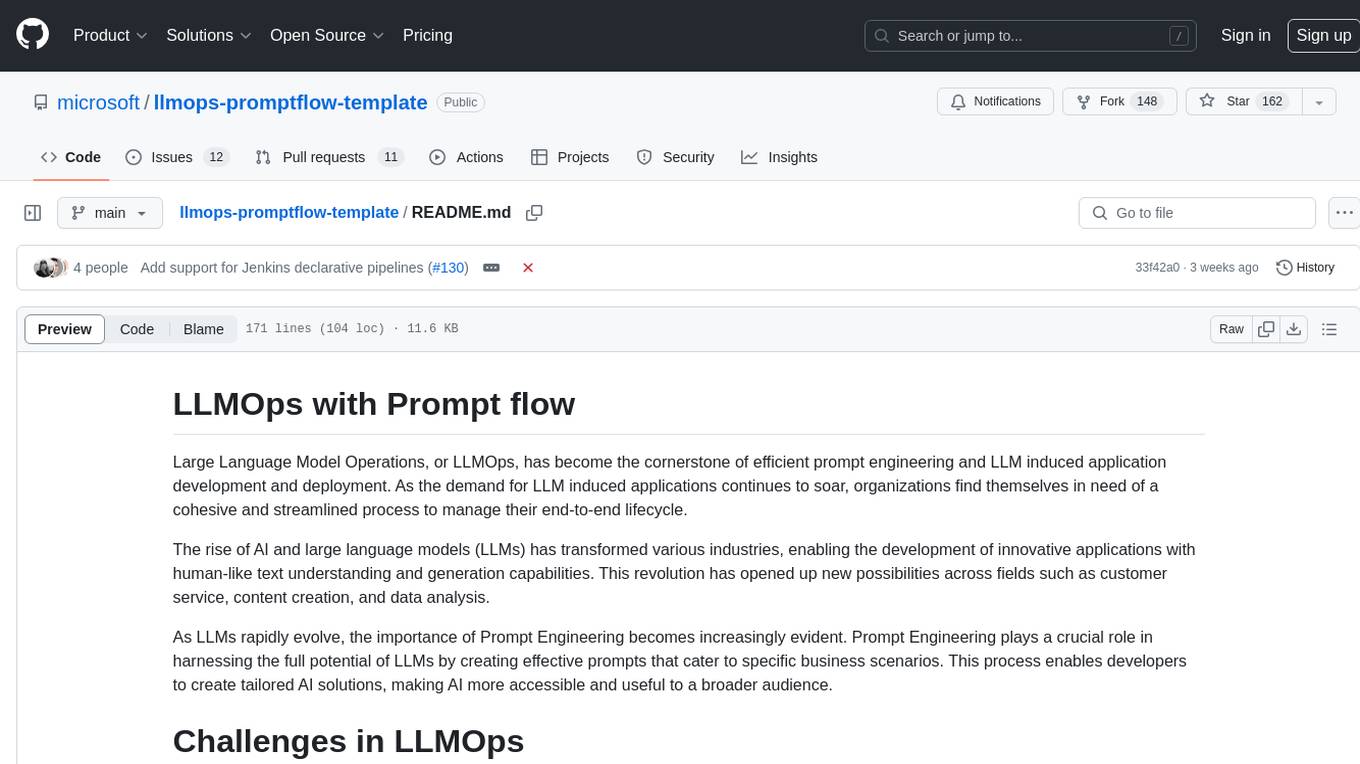
llmops-promptflow-template
LLMOps with Prompt flow is a template and guidance for building LLM-infused apps using Prompt flow. It provides centralized code hosting, lifecycle management, variant and hyperparameter experimentation, A/B deployment, many-to-many dataset/flow relationships, multiple deployment targets, comprehensive reporting, BYOF capabilities, configuration-based development, local prompt experimentation and evaluation, endpoint testing, and optional Human-in-loop validation. The tool is customizable to suit various application needs.
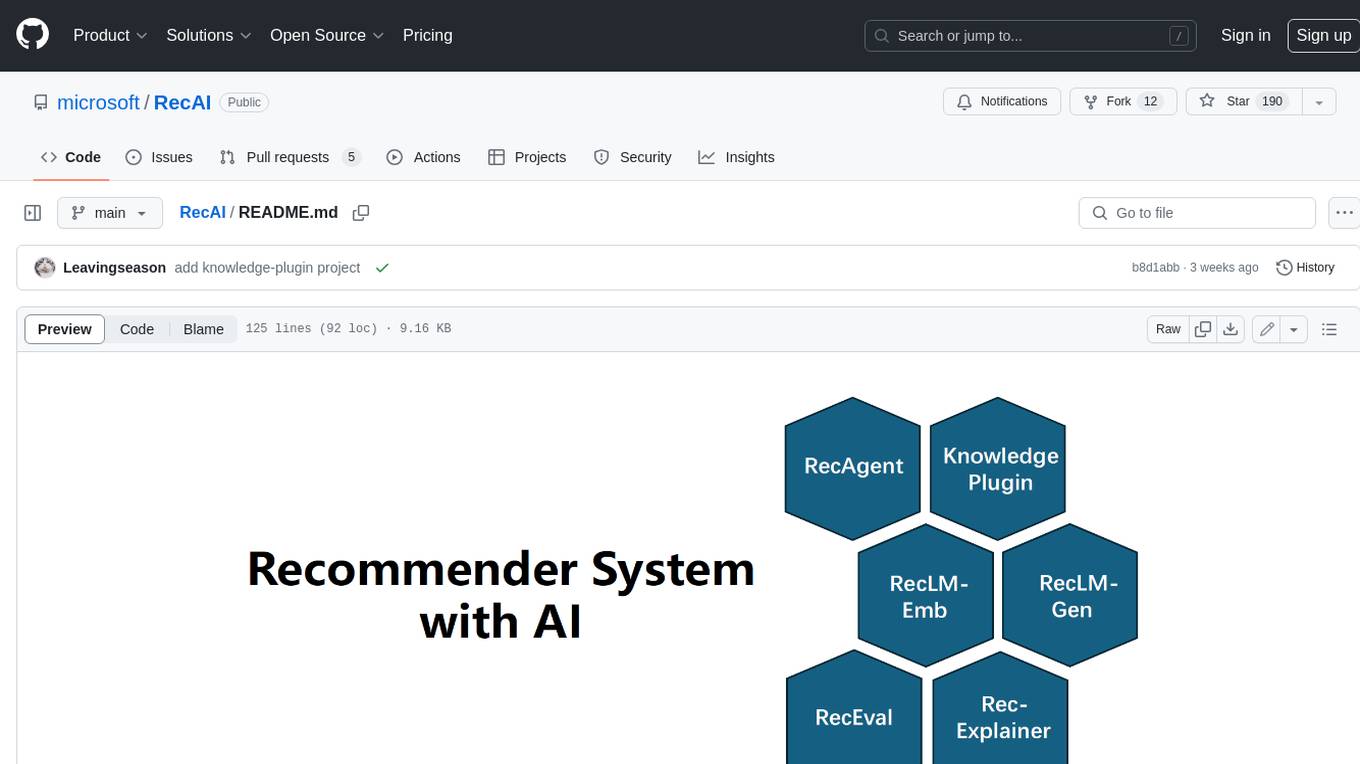
RecAI
RecAI is a project that explores the integration of Large Language Models (LLMs) into recommender systems, addressing the challenges of interactivity, explainability, and controllability. It aims to bridge the gap between general-purpose LLMs and domain-specific recommender systems, providing a holistic perspective on the practical requirements of LLM4Rec. The project investigates various techniques, including Recommender AI agents, selective knowledge injection, fine-tuning language models, evaluation, and LLMs as model explainers, to create more sophisticated, interactive, and user-centric recommender systems.
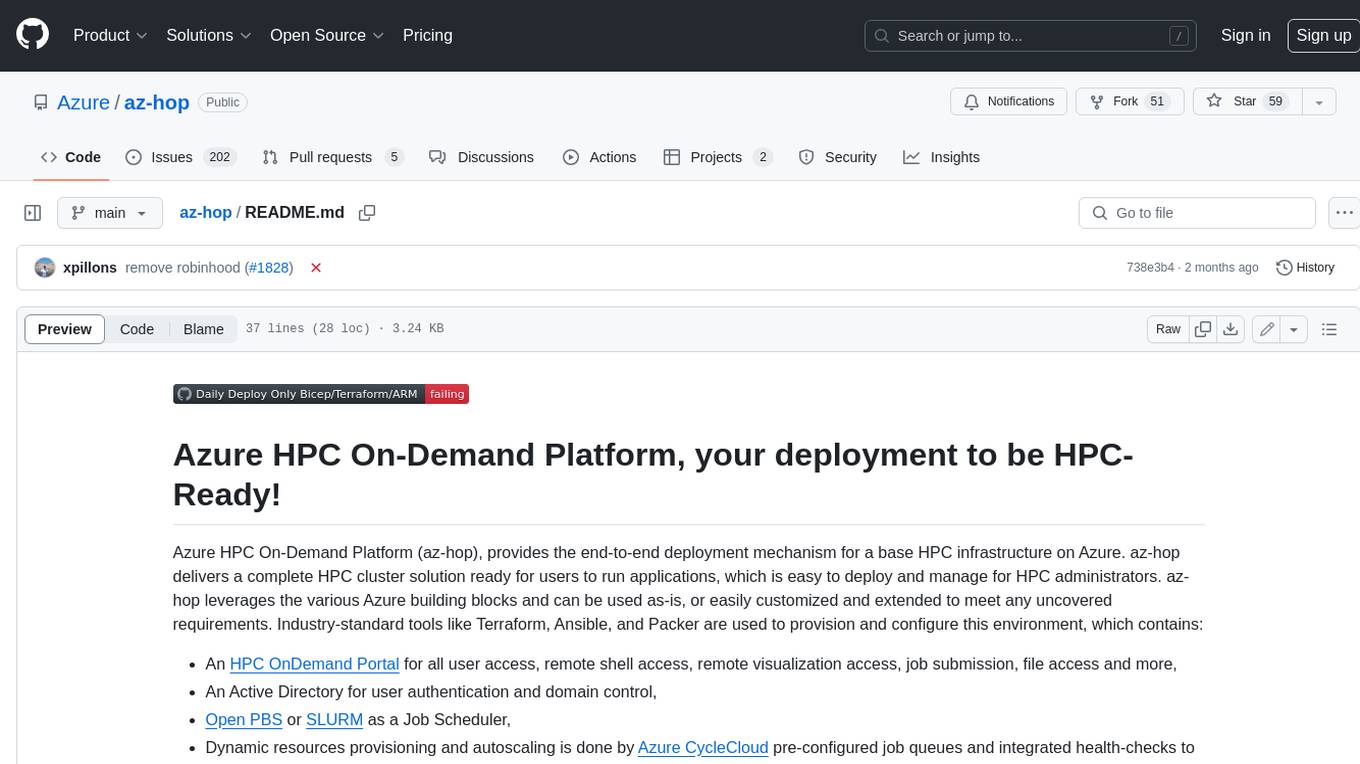
az-hop
Azure HPC On-Demand Platform (az-hop) provides an end-to-end deployment mechanism for a base HPC infrastructure on Azure. It delivers a complete HPC cluster solution ready for users to run applications, which is easy to deploy and manage for HPC administrators. az-hop leverages various Azure building blocks and can be used as-is or easily customized and extended to meet any uncovered requirements. Industry-standard tools like Terraform, Ansible, and Packer are used to provision and configure this environment, which contains: - An HPC OnDemand Portal for all user access, remote shell access, remote visualization access, job submission, file access, and more - An Active Directory for user authentication and domain control - Open PBS or SLURM as a Job Scheduler - Dynamic resources provisioning and autoscaling is done by Azure CycleCloud pre-configured job queues and integrated health-checks to quickly avoid non-optimal nodes - A Jumpbox to provide admin access - A common shared file system for home directory and applications is delivered by Azure Netapp Files - Grafana dashboards to monitor your cluster - Remote Visualization with noVNC and GPU acceleration with VirtualGL
For similar tasks
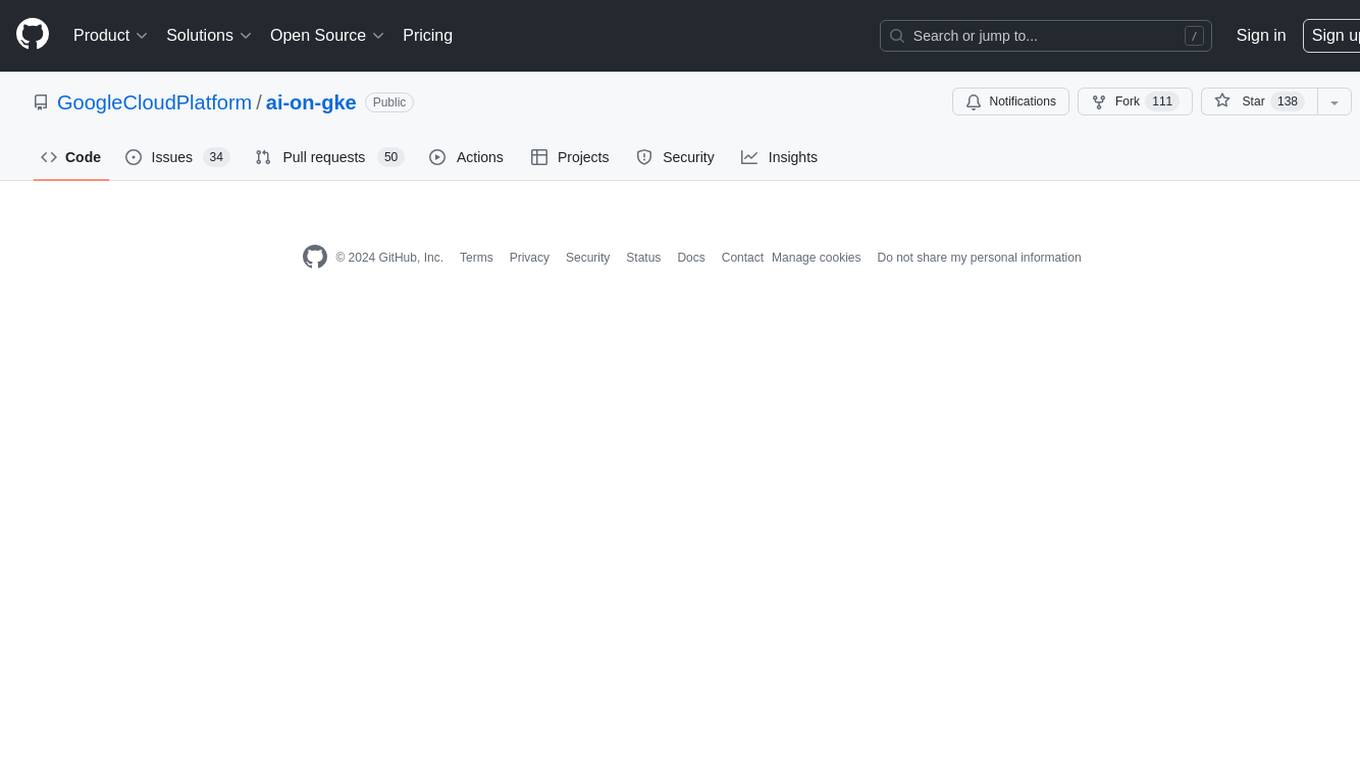
ai-on-gke
This repository contains assets related to AI/ML workloads on Google Kubernetes Engine (GKE). Run optimized AI/ML workloads with Google Kubernetes Engine (GKE) platform orchestration capabilities. A robust AI/ML platform considers the following layers: Infrastructure orchestration that support GPUs and TPUs for training and serving workloads at scale Flexible integration with distributed computing and data processing frameworks Support for multiple teams on the same infrastructure to maximize utilization of resources
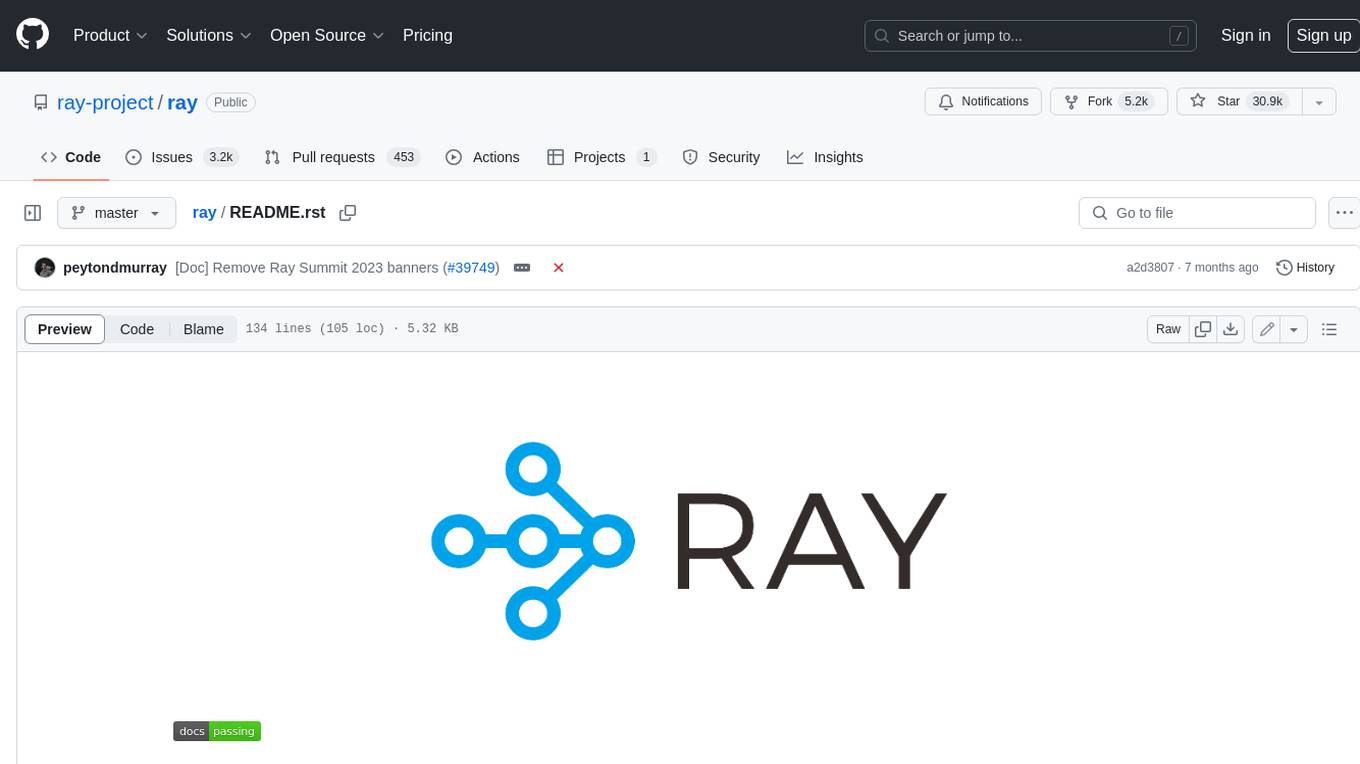
ray
Ray is a unified framework for scaling AI and Python applications. It consists of a core distributed runtime and a set of AI libraries for simplifying ML compute, including Data, Train, Tune, RLlib, and Serve. Ray runs on any machine, cluster, cloud provider, and Kubernetes, and features a growing ecosystem of community integrations. With Ray, you can seamlessly scale the same code from a laptop to a cluster, making it easy to meet the compute-intensive demands of modern ML workloads.
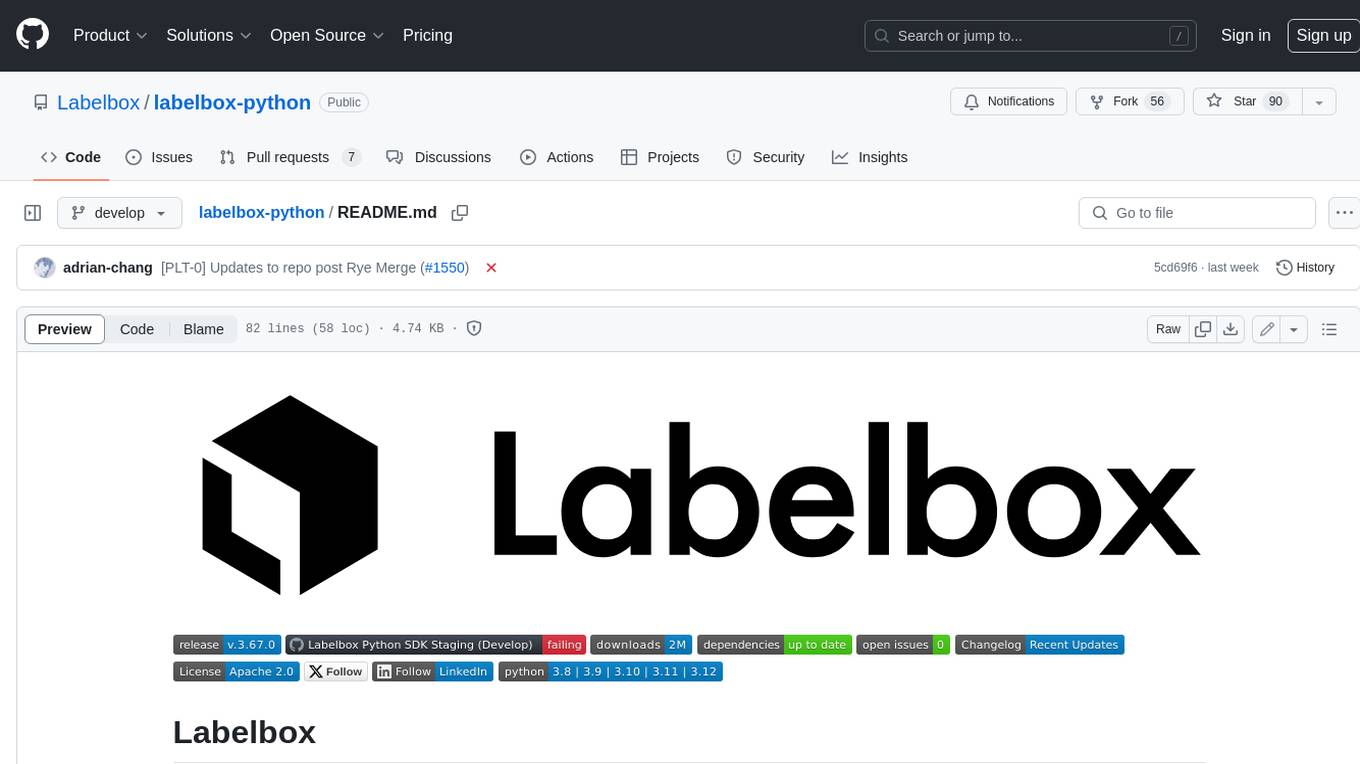
labelbox-python
Labelbox is a data-centric AI platform for enterprises to develop, optimize, and use AI to solve problems and power new products and services. Enterprises use Labelbox to curate data, generate high-quality human feedback data for computer vision and LLMs, evaluate model performance, and automate tasks by combining AI and human-centric workflows. The academic & research community uses Labelbox for cutting-edge AI research.
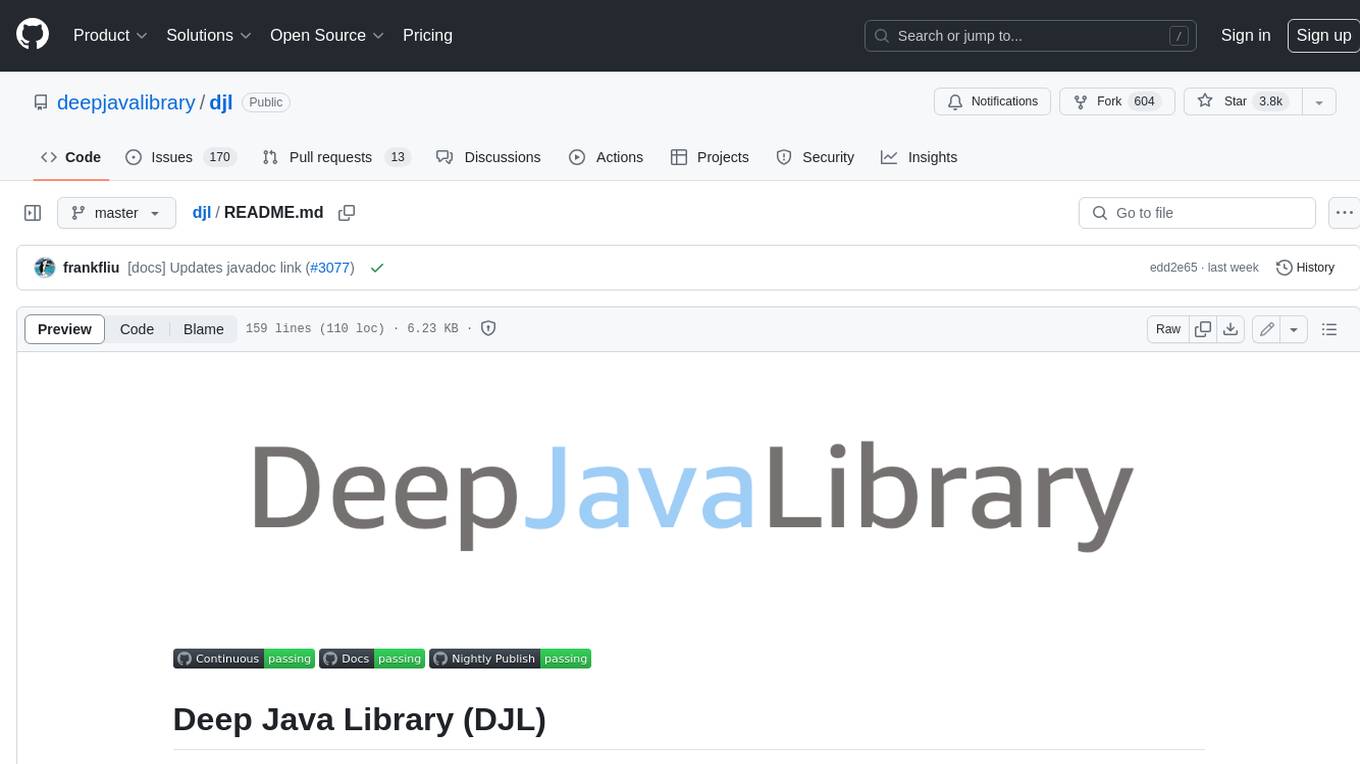
djl
Deep Java Library (DJL) is an open-source, high-level, engine-agnostic Java framework for deep learning. It is designed to be easy to get started with and simple to use for Java developers. DJL provides a native Java development experience and allows users to integrate machine learning and deep learning models with their Java applications. The framework is deep learning engine agnostic, enabling users to switch engines at any point for optimal performance. DJL's ergonomic API interface guides users with best practices to accomplish deep learning tasks, such as running inference and training neural networks.
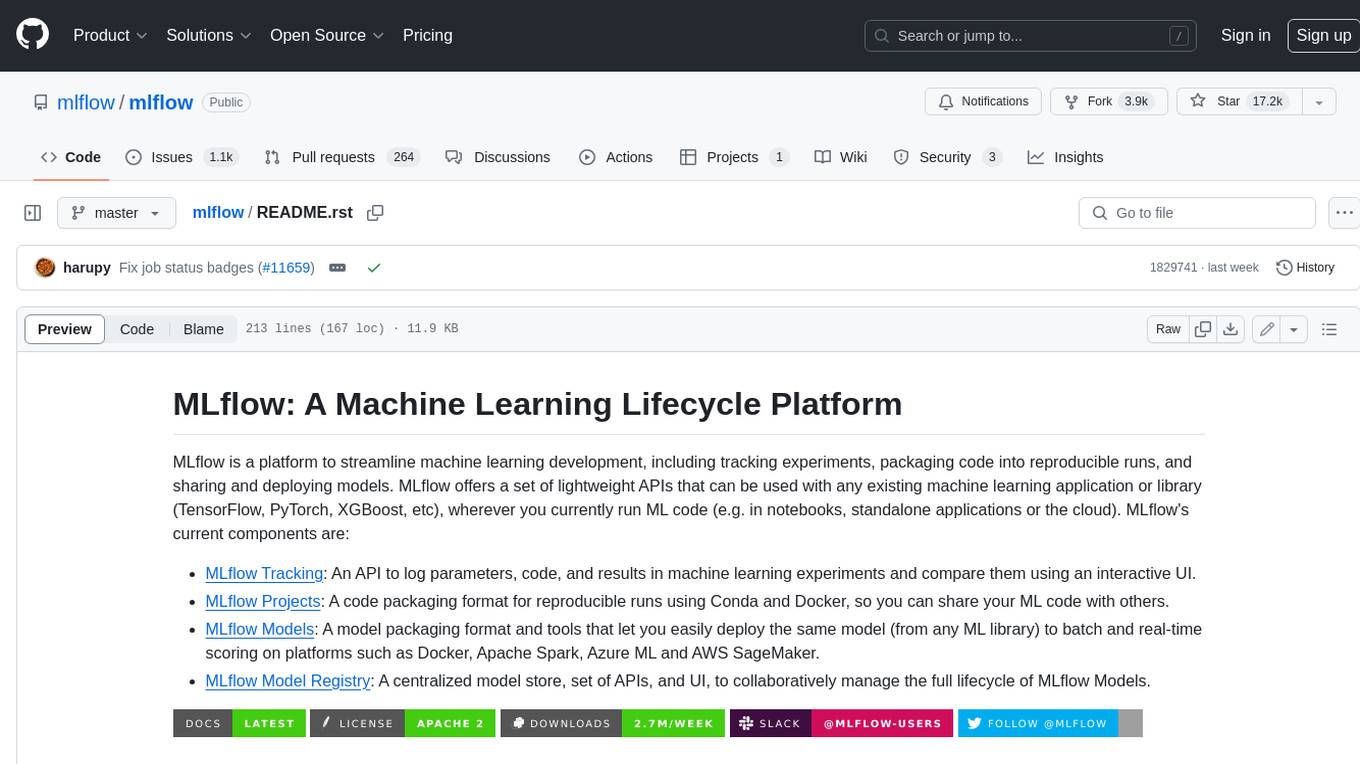
mlflow
MLflow is a platform to streamline machine learning development, including tracking experiments, packaging code into reproducible runs, and sharing and deploying models. MLflow offers a set of lightweight APIs that can be used with any existing machine learning application or library (TensorFlow, PyTorch, XGBoost, etc), wherever you currently run ML code (e.g. in notebooks, standalone applications or the cloud). MLflow's current components are:
* `MLflow Tracking
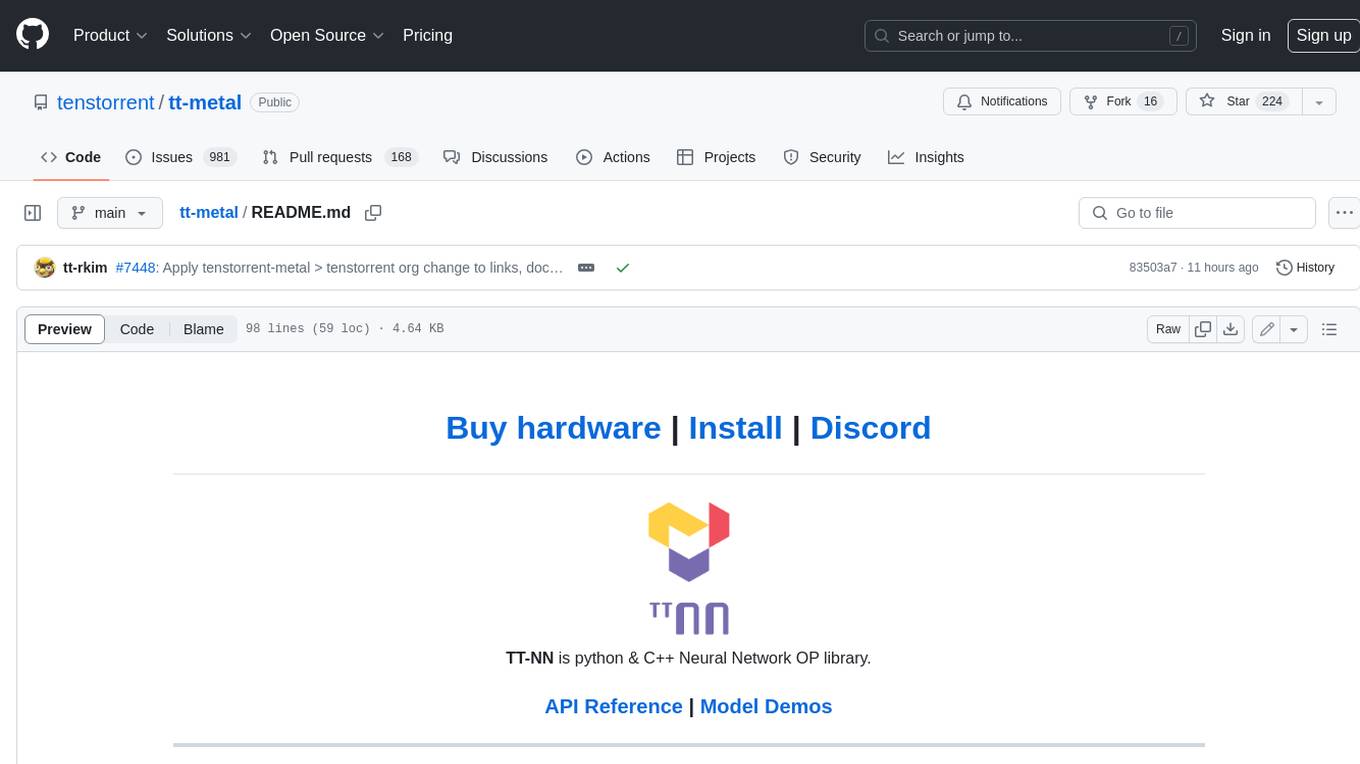
tt-metal
TT-NN is a python & C++ Neural Network OP library. It provides a low-level programming model, TT-Metalium, enabling kernel development for Tenstorrent hardware.
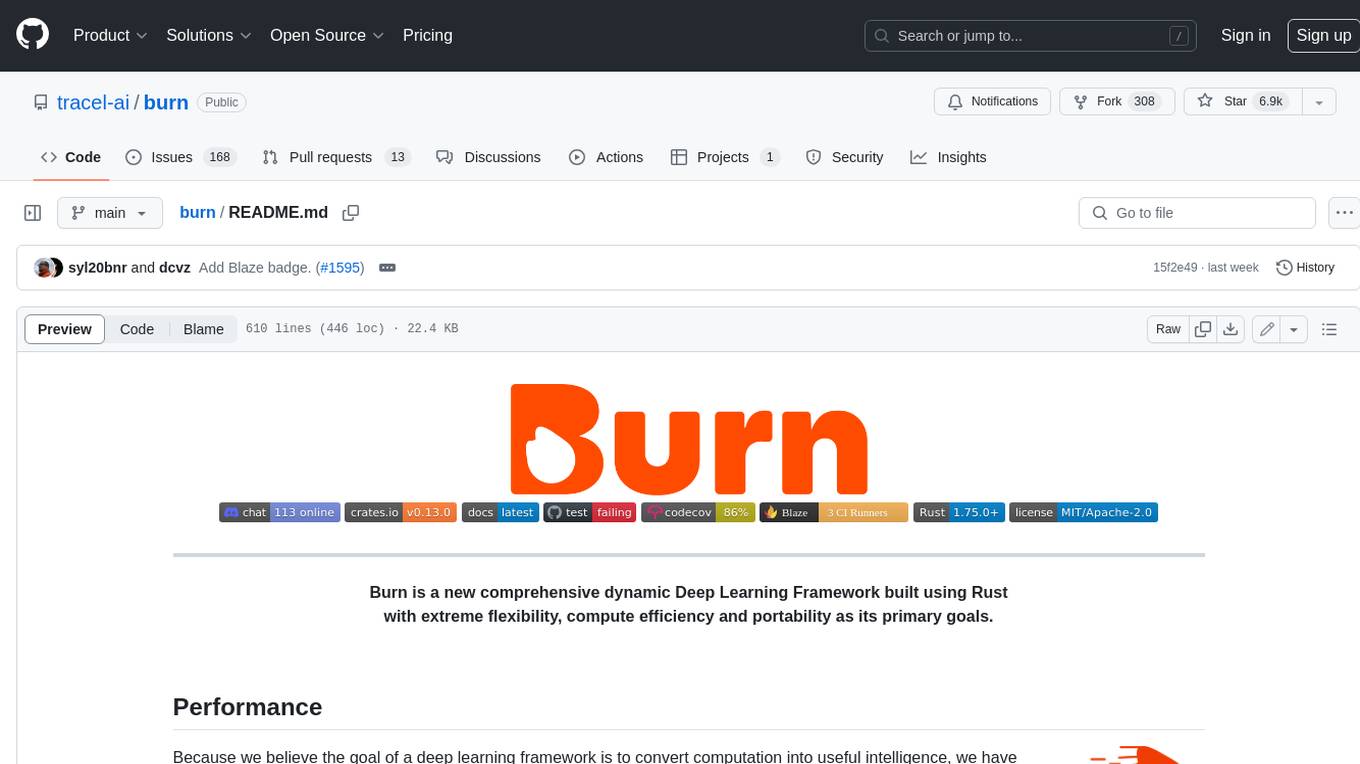
burn
Burn is a new comprehensive dynamic Deep Learning Framework built using Rust with extreme flexibility, compute efficiency and portability as its primary goals.
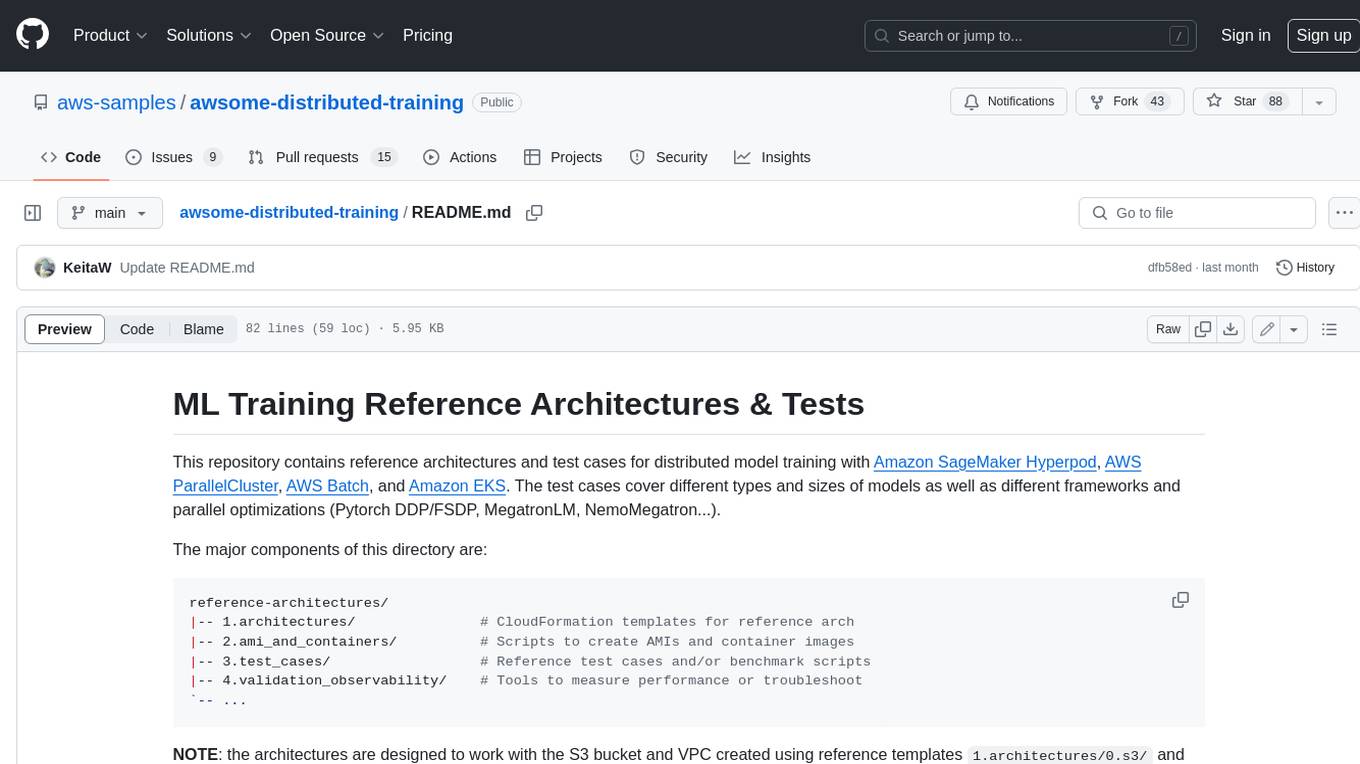
awsome-distributed-training
This repository contains reference architectures and test cases for distributed model training with Amazon SageMaker Hyperpod, AWS ParallelCluster, AWS Batch, and Amazon EKS. The test cases cover different types and sizes of models as well as different frameworks and parallel optimizations (Pytorch DDP/FSDP, MegatronLM, NemoMegatron...).
For similar jobs
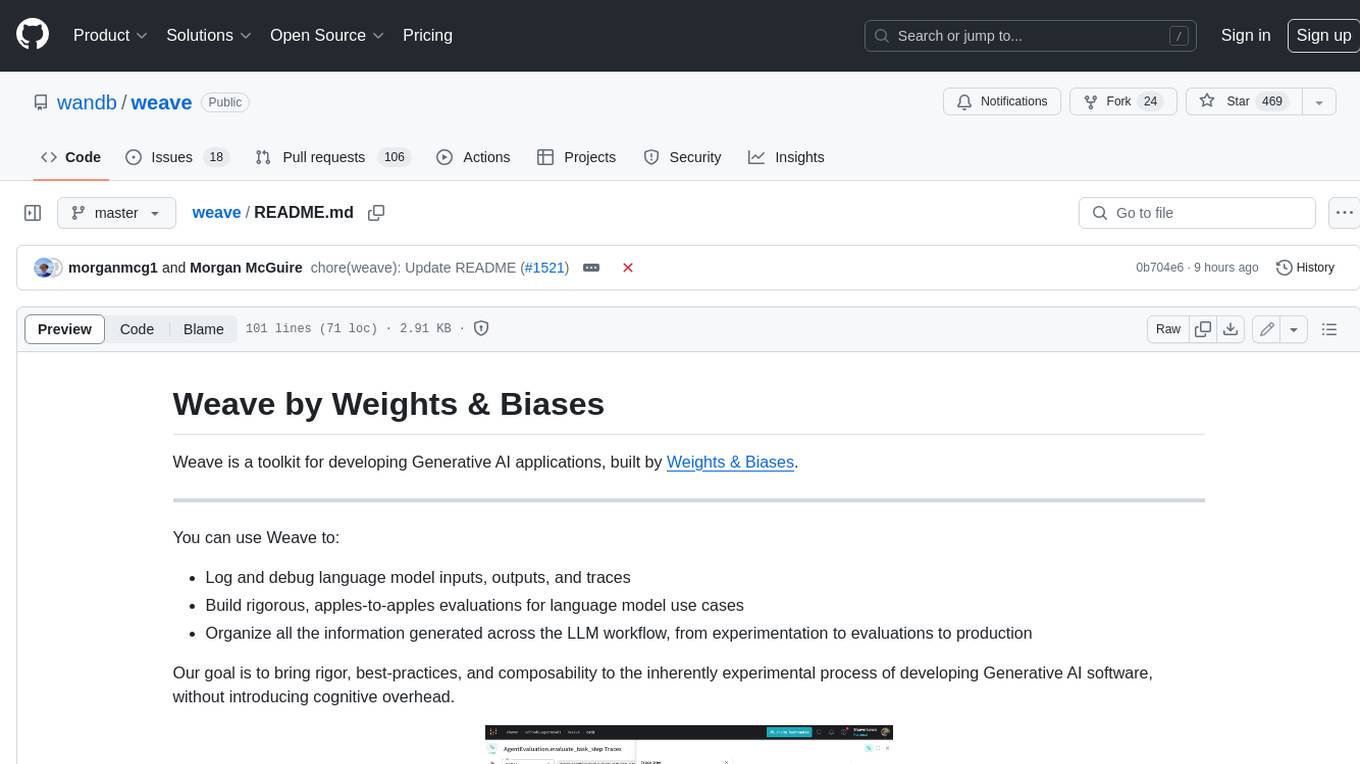
weave
Weave is a toolkit for developing Generative AI applications, built by Weights & Biases. With Weave, you can log and debug language model inputs, outputs, and traces; build rigorous, apples-to-apples evaluations for language model use cases; and organize all the information generated across the LLM workflow, from experimentation to evaluations to production. Weave aims to bring rigor, best-practices, and composability to the inherently experimental process of developing Generative AI software, without introducing cognitive overhead.
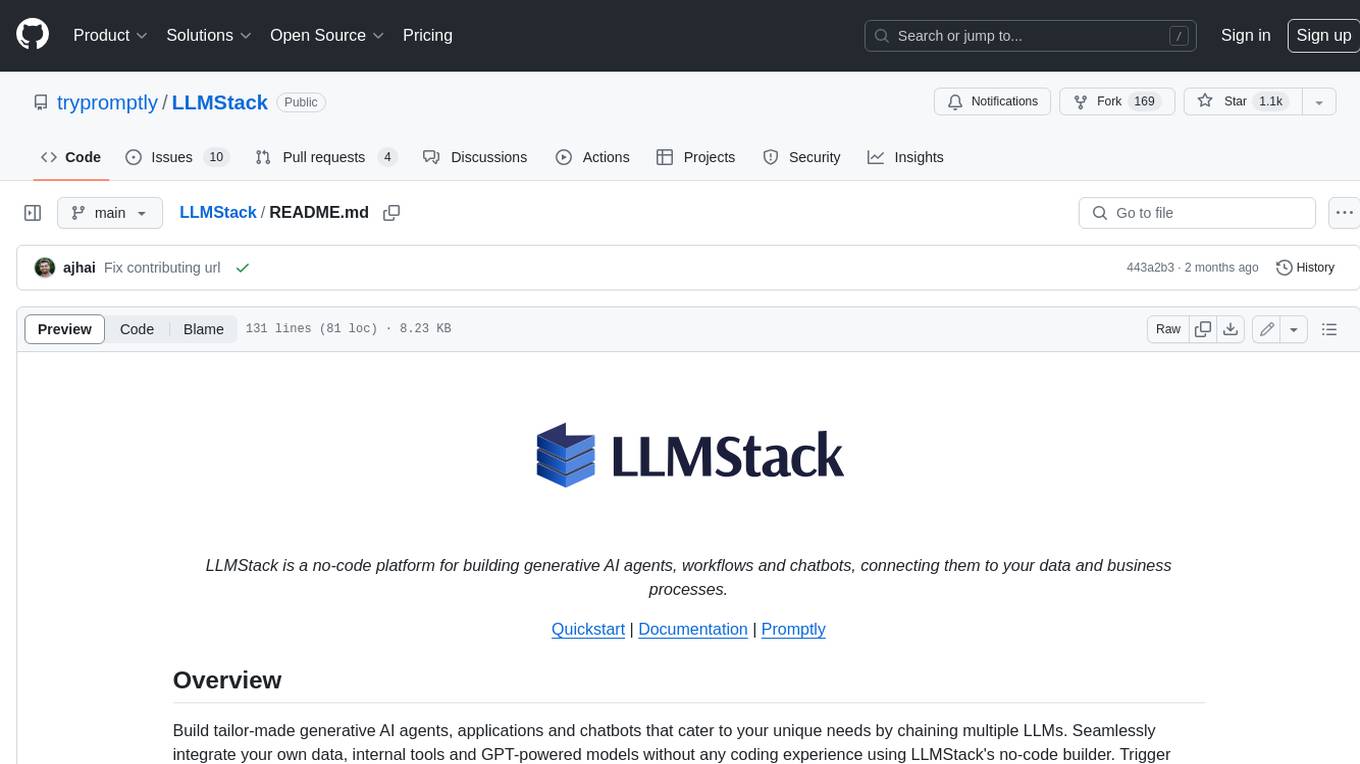
LLMStack
LLMStack is a no-code platform for building generative AI agents, workflows, and chatbots. It allows users to connect their own data, internal tools, and GPT-powered models without any coding experience. LLMStack can be deployed to the cloud or on-premise and can be accessed via HTTP API or triggered from Slack or Discord.
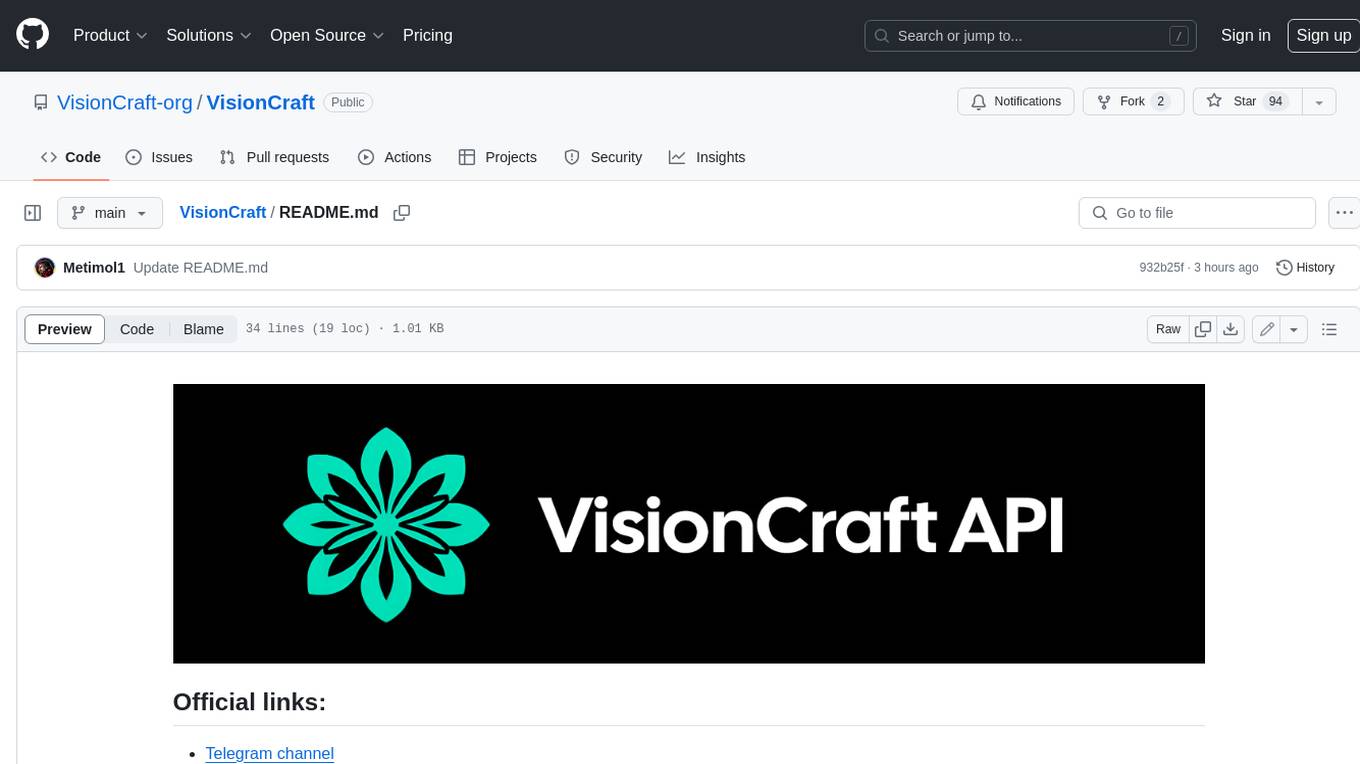
VisionCraft
The VisionCraft API is a free API for using over 100 different AI models. From images to sound.
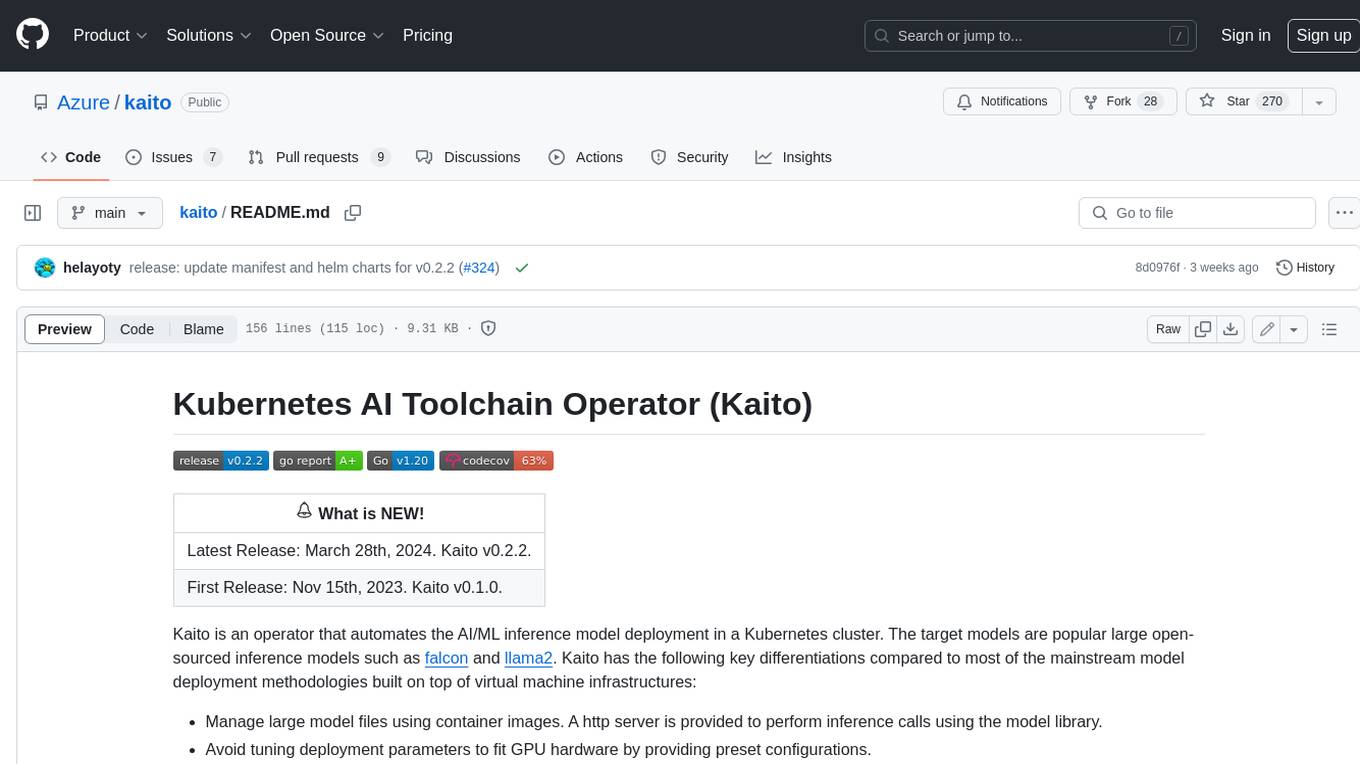
kaito
Kaito is an operator that automates the AI/ML inference model deployment in a Kubernetes cluster. It manages large model files using container images, avoids tuning deployment parameters to fit GPU hardware by providing preset configurations, auto-provisions GPU nodes based on model requirements, and hosts large model images in the public Microsoft Container Registry (MCR) if the license allows. Using Kaito, the workflow of onboarding large AI inference models in Kubernetes is largely simplified.
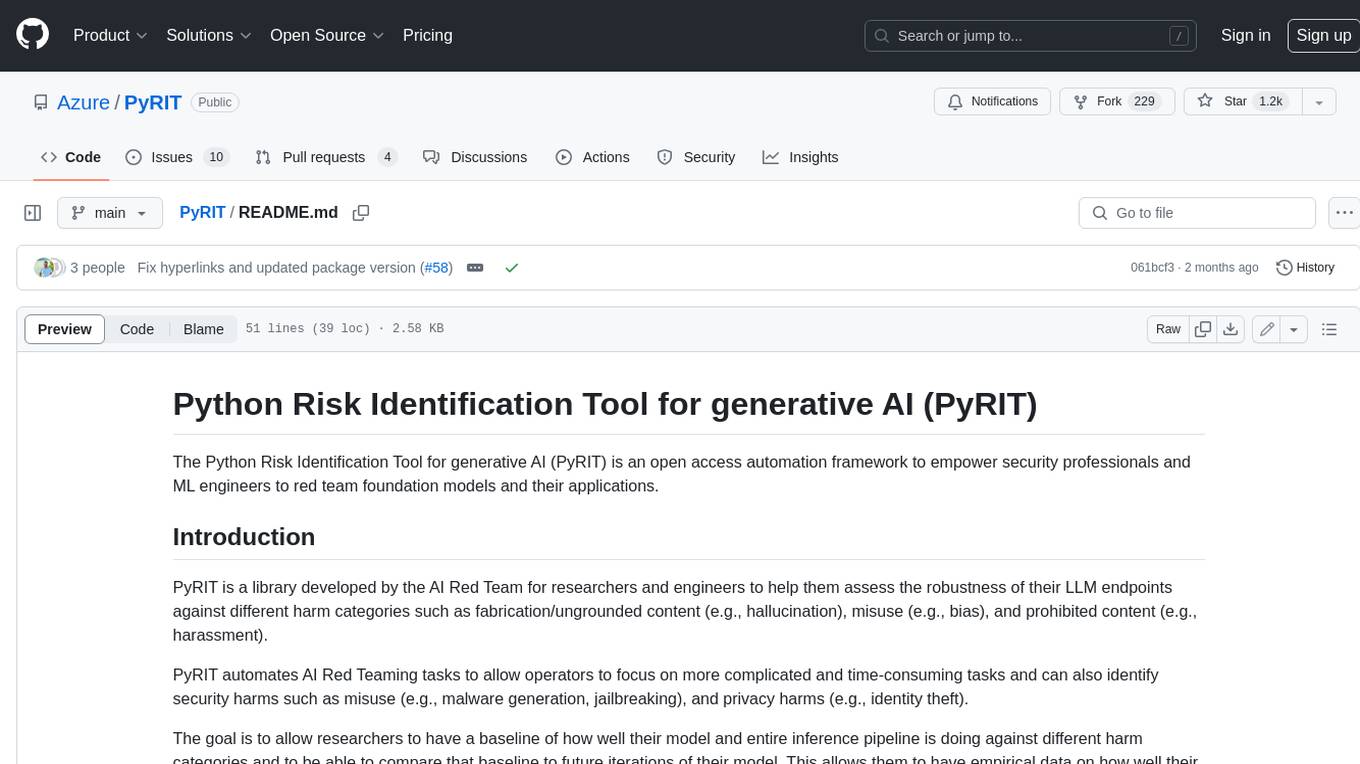
PyRIT
PyRIT is an open access automation framework designed to empower security professionals and ML engineers to red team foundation models and their applications. It automates AI Red Teaming tasks to allow operators to focus on more complicated and time-consuming tasks and can also identify security harms such as misuse (e.g., malware generation, jailbreaking), and privacy harms (e.g., identity theft). The goal is to allow researchers to have a baseline of how well their model and entire inference pipeline is doing against different harm categories and to be able to compare that baseline to future iterations of their model. This allows them to have empirical data on how well their model is doing today, and detect any degradation of performance based on future improvements.
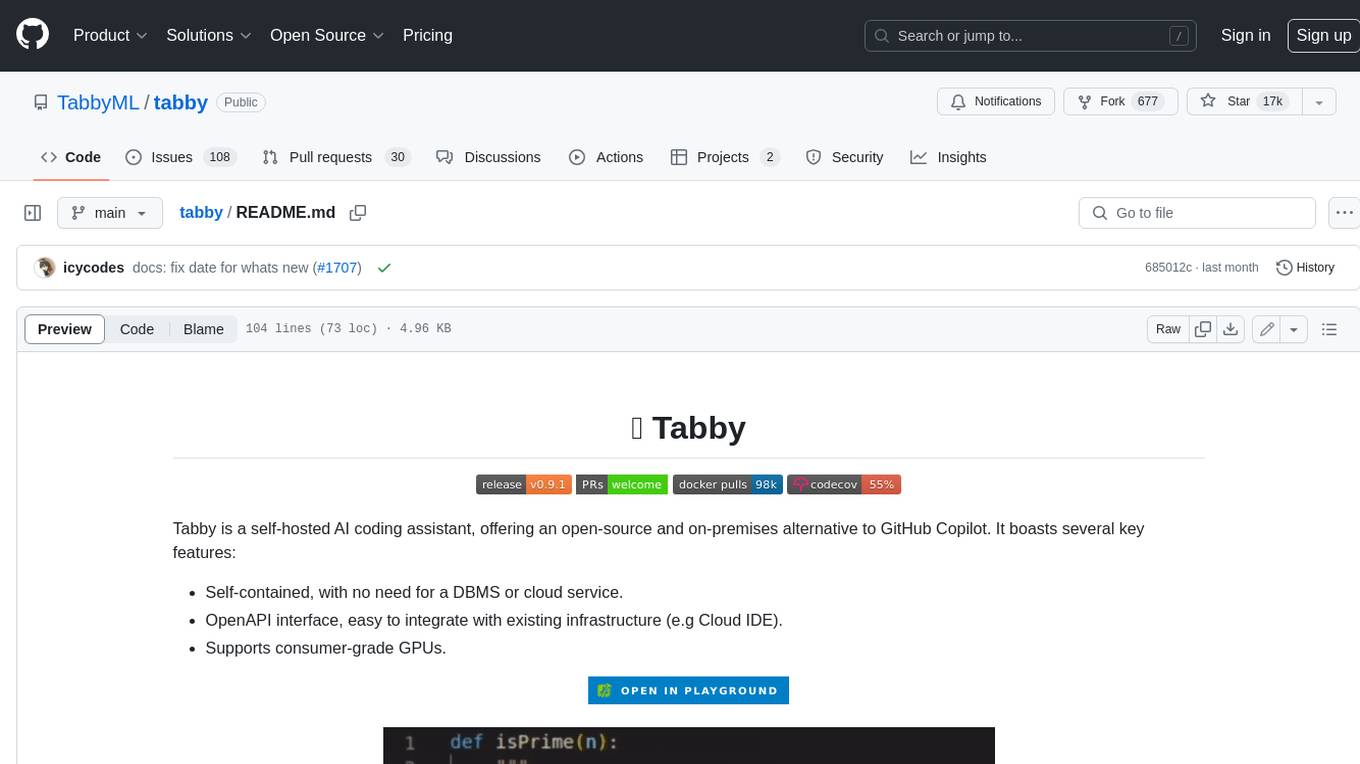
tabby
Tabby is a self-hosted AI coding assistant, offering an open-source and on-premises alternative to GitHub Copilot. It boasts several key features: * Self-contained, with no need for a DBMS or cloud service. * OpenAPI interface, easy to integrate with existing infrastructure (e.g Cloud IDE). * Supports consumer-grade GPUs.
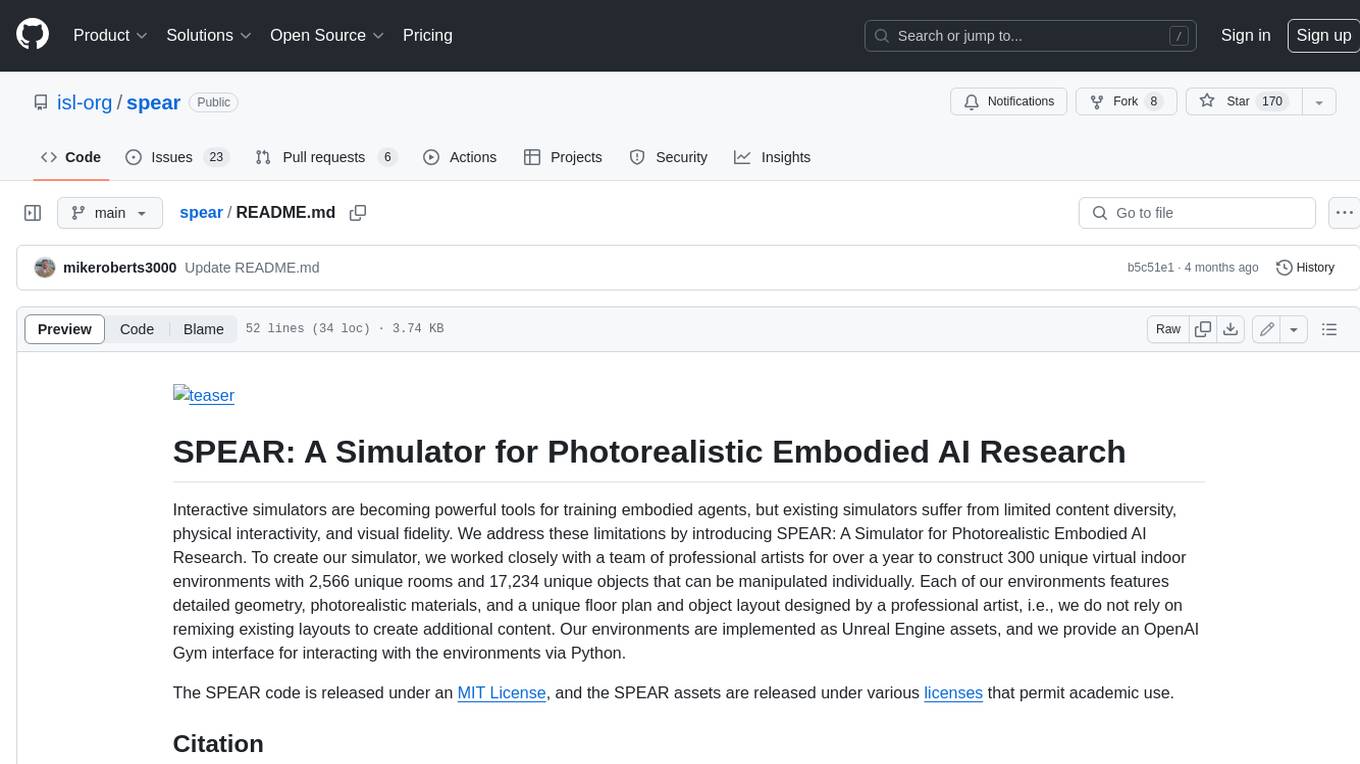
spear
SPEAR (Simulator for Photorealistic Embodied AI Research) is a powerful tool for training embodied agents. It features 300 unique virtual indoor environments with 2,566 unique rooms and 17,234 unique objects that can be manipulated individually. Each environment is designed by a professional artist and features detailed geometry, photorealistic materials, and a unique floor plan and object layout. SPEAR is implemented as Unreal Engine assets and provides an OpenAI Gym interface for interacting with the environments via Python.
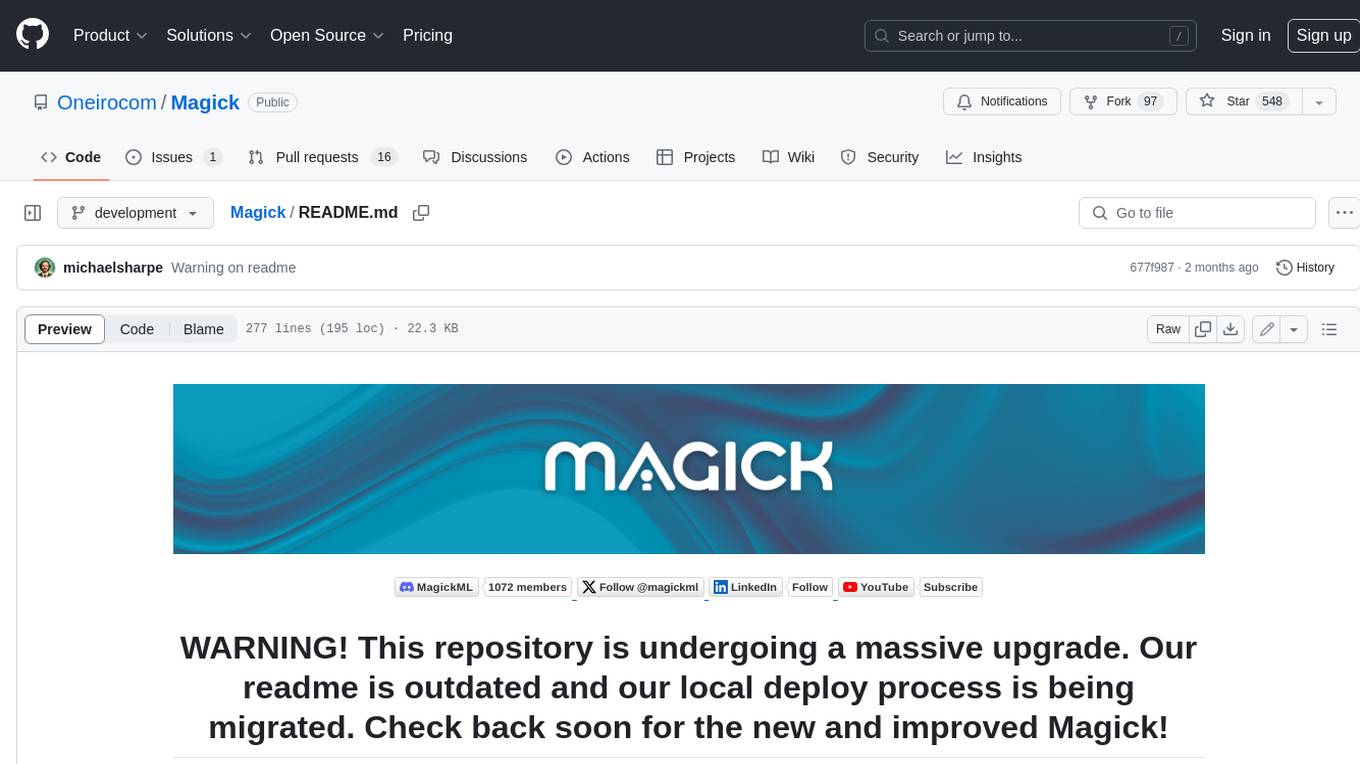
Magick
Magick is a groundbreaking visual AIDE (Artificial Intelligence Development Environment) for no-code data pipelines and multimodal agents. Magick can connect to other services and comes with nodes and templates well-suited for intelligent agents, chatbots, complex reasoning systems and realistic characters.