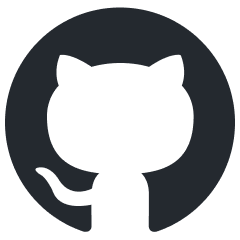
tt-metal
:metal: TT-NN operator library, and TT-Metalium low level kernel programming model.
Stars: 786
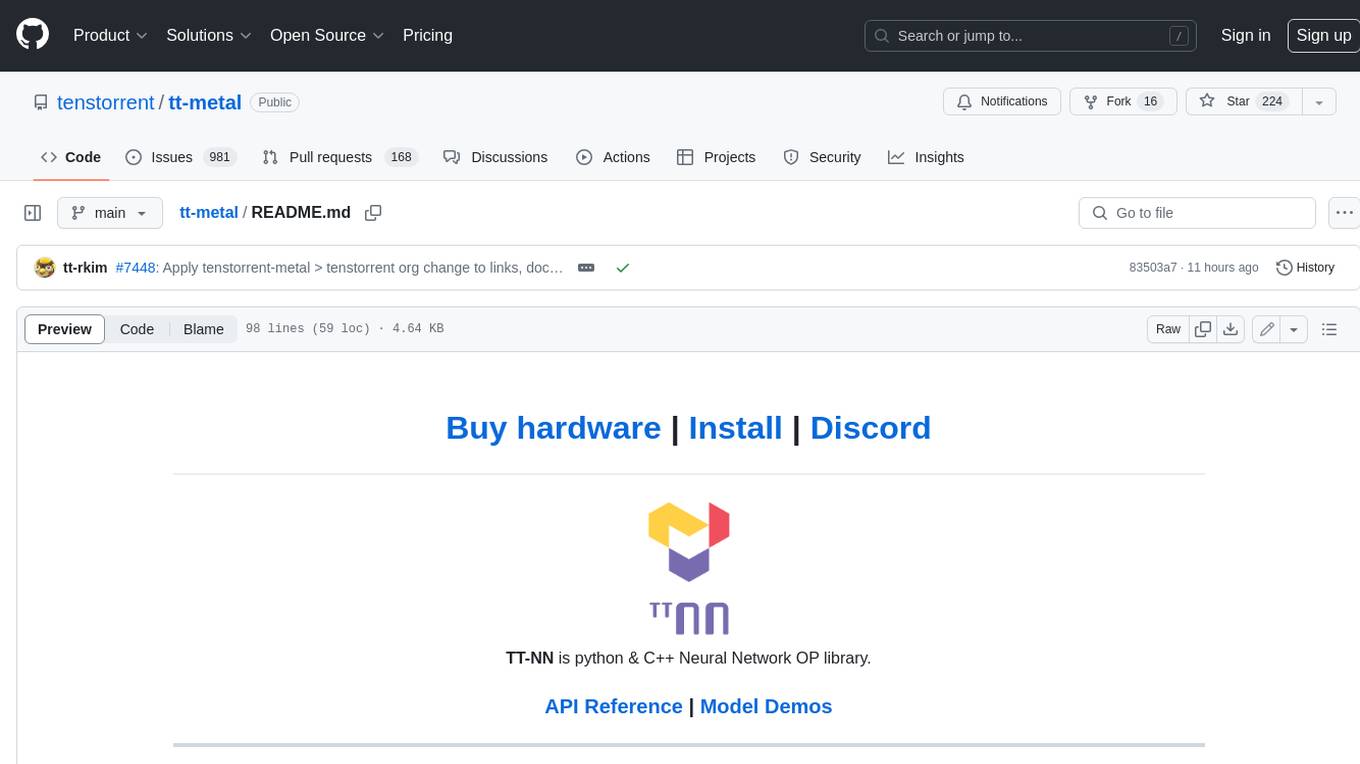
TT-NN is a python & C++ Neural Network OP library. It provides a low-level programming model, TT-Metalium, enabling kernel development for Tenstorrent hardware.
README:
Last Update: March 24, 2025
Notes:
- ttft = time to first token | t/s/u = tokens/second/user | t/s = tokens/second; where t/s = t/s/u * batch.
- TP = Tensor Parallel, DP = Data Parallel; Defines parallelization factors across multiple devices.
- The reported LLM performance is for an input sequence length (number of rows filled in the KV cache) of 128 for all models except Mamba (which can accept any sequence length).
- The t/s/u reported is the throughput of the first token generated after prefill, i.e. 1 / inter token latency.
Model | Batch | Hardware | ttft (ms) | t/s/u | Target t/s/u | t/s | TT-Metalium Release |
---|---|---|---|---|---|---|---|
Whisper (distil-large-v3) | 1 | n150 | 252 | 43.4 | 45 | 43.4 | v0.57.0-rc33 |
Model | Batch | Hardware | fps | Target fps | Release |
---|---|---|---|---|---|
ResNet-50 (224x224) | 16 | n150 | 4,700 | 7,000 | |
ResNet-50 (224x224) (DP=2) | 32 | n300 | 9,200 | 14,000 | |
ResNet-50 (224x224) (DP=8) | 128 | QuietBox | 35,800 | 56,000 | |
ResNet-50 (224x224) (DP=32) | 512 | Galaxy | 96,800 | 224,000 | |
ViT (224x224) | 8 | n150 | 1100 | 1,600 | |
Stable Diffusion 1.4 (512x512) | 1 | n150 | 0.167 | 0.3 | |
YOLOv4 (320x320) | 1 | n150 | 120 | 300 | |
YOLOv4 (640x640) | 1 | n150 | 50 | 100 | |
SegFormer Semantic Segmentation (512x512) | 1 | n150 | 90 | 300 | |
Stable Diffusion 3.5 medium (512x512) | 1 | n150 | 0.06 | 0.3 |
Model | Batch | Hardware | sen/sec | Target sen/sec | Release |
---|---|---|---|---|---|
BERT-Large | 8 | n150 | 270 | 400 |
For the latest model updates and features, please see MODEL_UPDATES.md
For information on initial model procedures, please see Model Bring-Up and Testing
- Advanced Performance Optimizations for Models (updated March 4th, 2025)
- Programming Mesh of Devices (updated Sept 9th, 2024)
- ViT Implementation in TT-NN on GS (updated Sept 22nd, 2024)
- LLMs Bring up in TT-NN (updated Oct 29th, 2024)
- YOLOv4 Implementation in TT-NN on WH (updated November 8th, 2024)
- CNN Bring up & Optimization in TT-NN (updated Jan 22nd, 2025)
- Matrix Multiply FLOPS on WH (updated November 13th, 2024)

TT-Metalium is our low-level programming model, enabling kernel development for Tenstorrent hardware.
Get started with simple kernels.
- Matrix Engine (updated Sept 6th, 2024)
- Data Formats (updated Sept 7th, 2024)
- Reconfiguring Data Formats (updated Oct 17th, 2024)
- Handling special floating-point numbers (updated Oct 5th, 2024)
- Allocator (Updated Dec 19th, 2024)
- Tensor Layouts (updated Sept 6th, 2024)
- Saturating DRAM Bandwidth (updated Sept 6th, 2024)
- Flash Attention on Wormhole (updated Sept 6th, 2024)
- CNNs on TT Architectures (updated Sept 6th, 2024)
- Ethernet and Multichip Basics (Updated Sept 20th, 2024)
- Collective Communication Library (CCL) (Updated Sept 20th, 2024)
- Blackhole Bring-Up Programming Guide (Updated Dec 18th, 2024)
- Sub-Devices (Updated Jan 7th, 2025)
- Matmul OP on a Single_core
- Matmul OP on Multi_core (Basic)
- Matmul Multi_core Reuse (Optimized)
- Matmul Multi_core Multi-Cast (Optimized)
This repo is a part of Tenstorrent’s bounty program. If you are interested in helping to improve tt-metal, please make sure to read the Tenstorrent Bounty Program Terms and Conditions before heading to the issues tab. Look for the issues that are tagged with both “bounty” and difficulty level!
For Tasks:
Click tags to check more tools for each tasksFor Jobs:
Alternative AI tools for tt-metal
Similar Open Source Tools
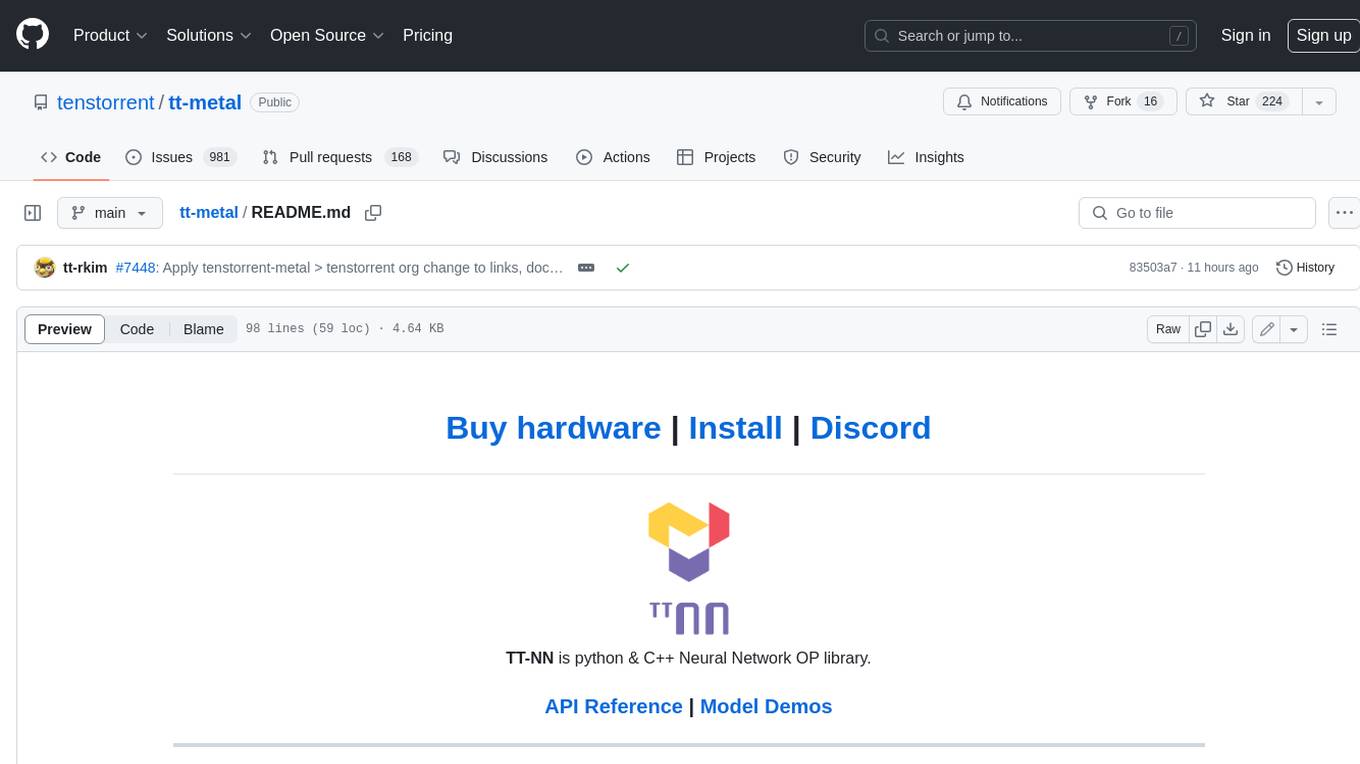
tt-metal
TT-NN is a python & C++ Neural Network OP library. It provides a low-level programming model, TT-Metalium, enabling kernel development for Tenstorrent hardware.
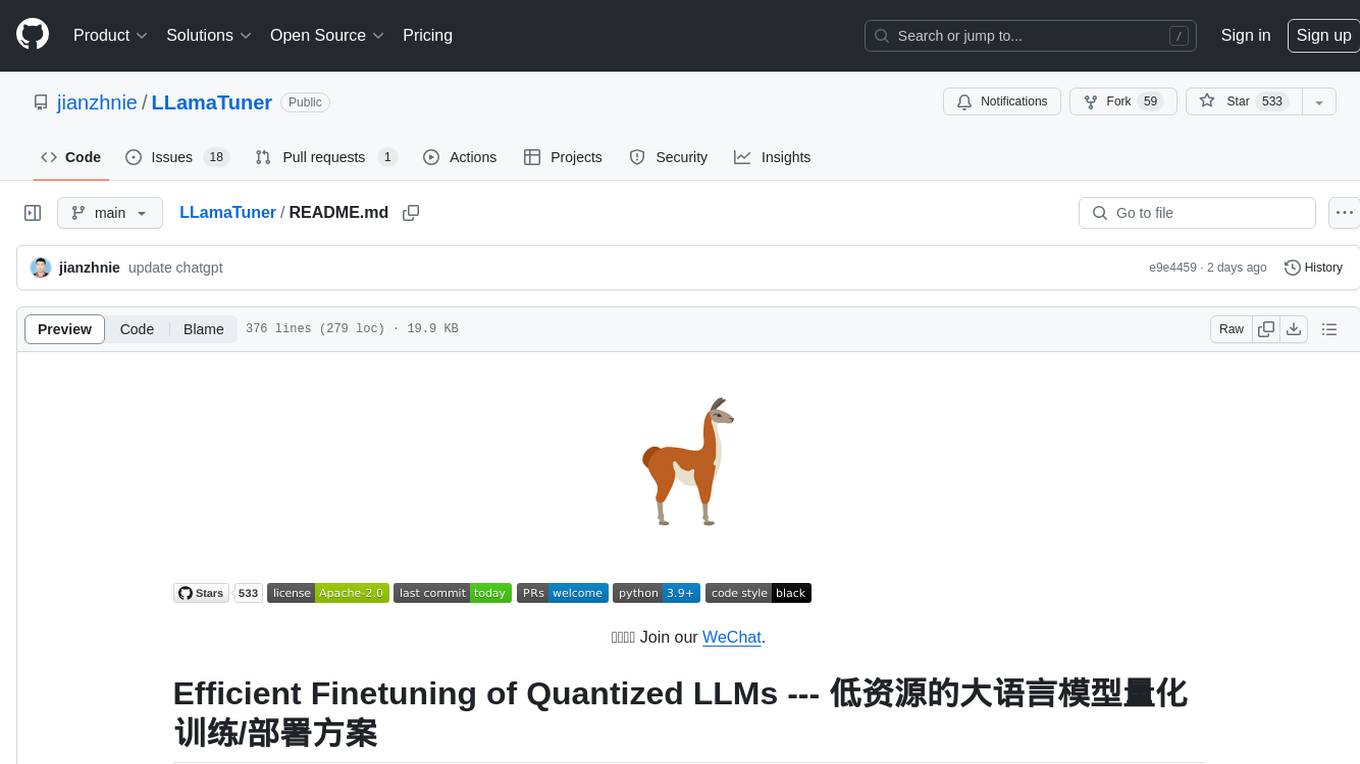
LLamaTuner
LLamaTuner is a repository for the Efficient Finetuning of Quantized LLMs project, focusing on building and sharing instruction-following Chinese baichuan-7b/LLaMA/Pythia/GLM model tuning methods. The project enables training on a single Nvidia RTX-2080TI and RTX-3090 for multi-round chatbot training. It utilizes bitsandbytes for quantization and is integrated with Huggingface's PEFT and transformers libraries. The repository supports various models, training approaches, and datasets for supervised fine-tuning, LoRA, QLoRA, and more. It also provides tools for data preprocessing and offers models in the Hugging Face model hub for inference and finetuning. The project is licensed under Apache 2.0 and acknowledges contributions from various open-source contributors.
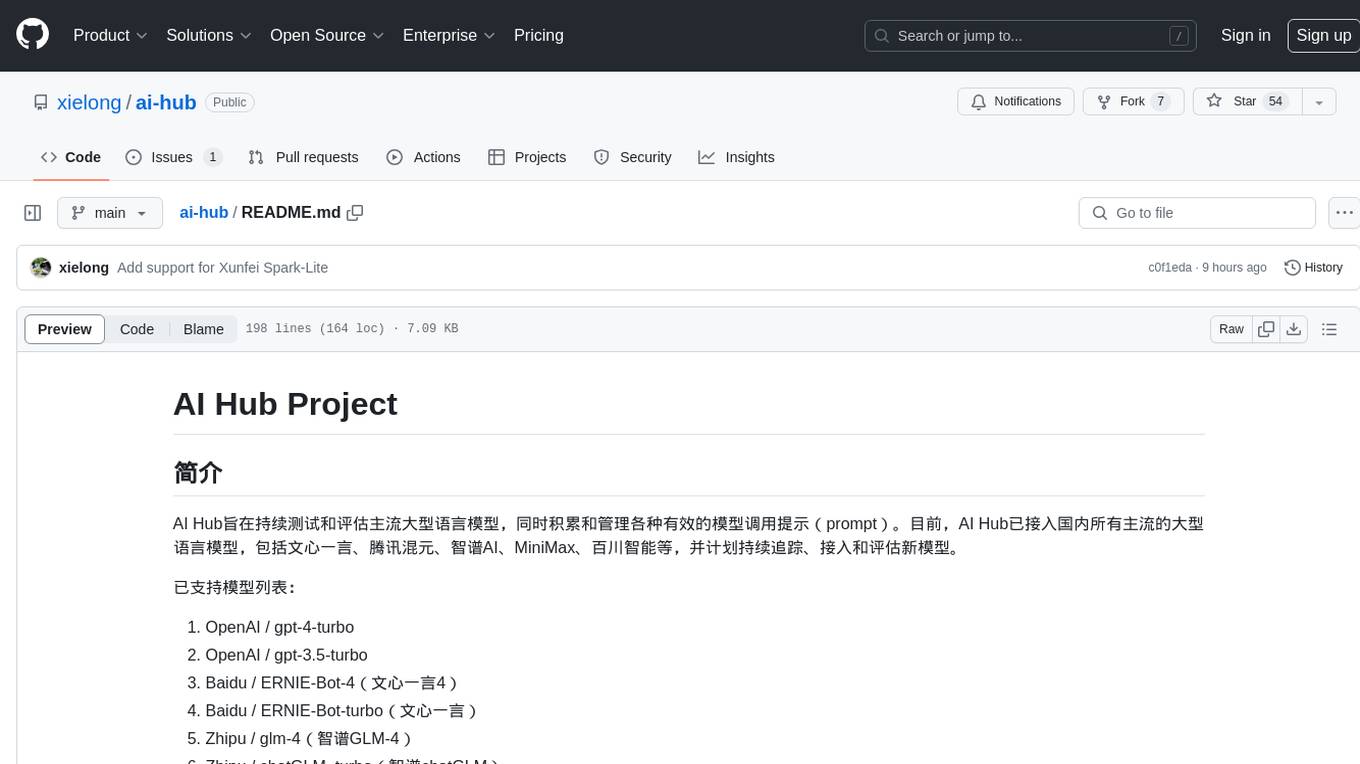
ai-hub
AI Hub Project aims to continuously test and evaluate mainstream large language models, while accumulating and managing various effective model invocation prompts. It has integrated all mainstream large language models in China, including OpenAI GPT-4 Turbo, Baidu ERNIE-Bot-4, Tencent ChatPro, MiniMax abab5.5-chat, and more. The project plans to continuously track, integrate, and evaluate new models. Users can access the models through REST services or Java code integration. The project also provides a testing suite for translation, coding, and benchmark testing.
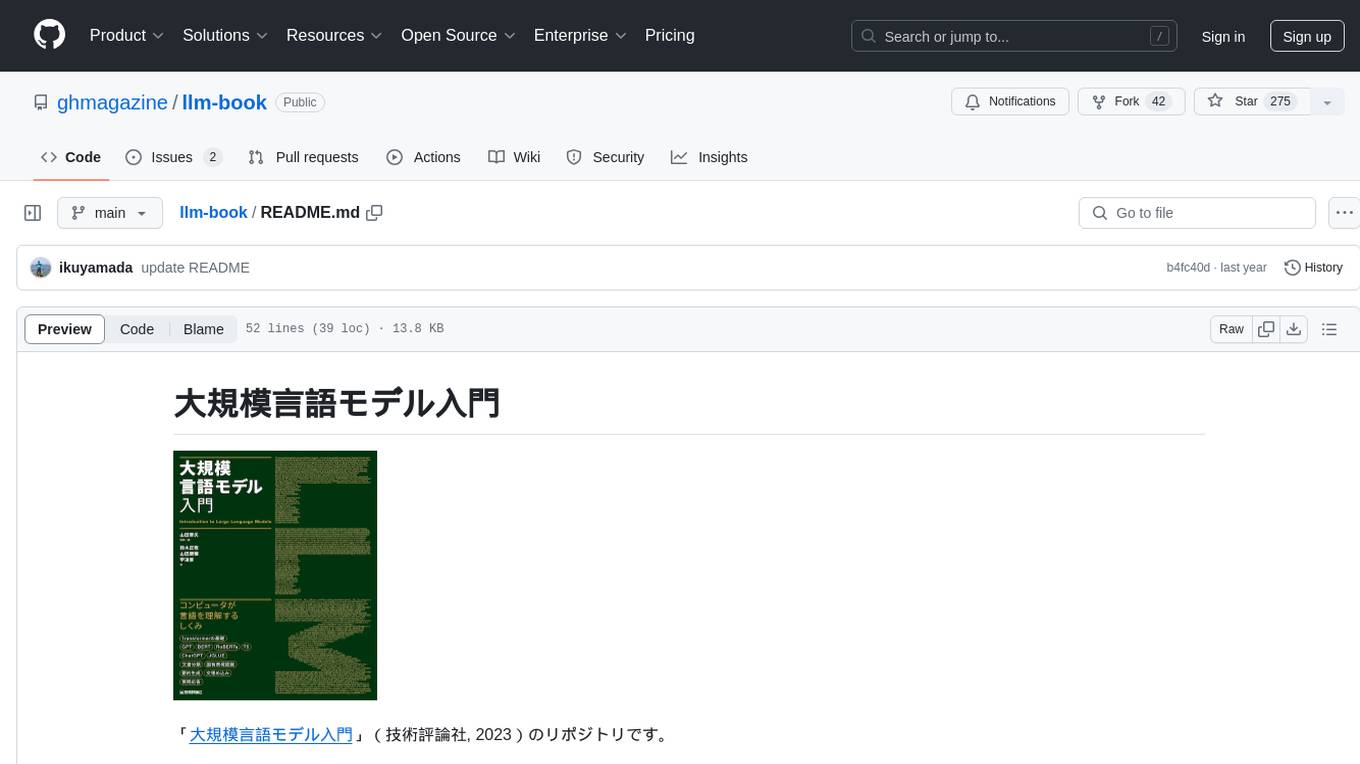
llm-book
The 'llm-book' repository is dedicated to the introduction of large-scale language models, focusing on natural language processing tasks. The code is designed to run on Google Colaboratory and utilizes datasets and models available on the Hugging Face Hub. Note that as of July 28, 2023, there are issues with the MARC-ja dataset links, but an alternative notebook using the WRIME Japanese sentiment analysis dataset has been added. The repository covers various chapters on topics such as Transformers, fine-tuning language models, entity recognition, summarization, document embedding, question answering, and more.
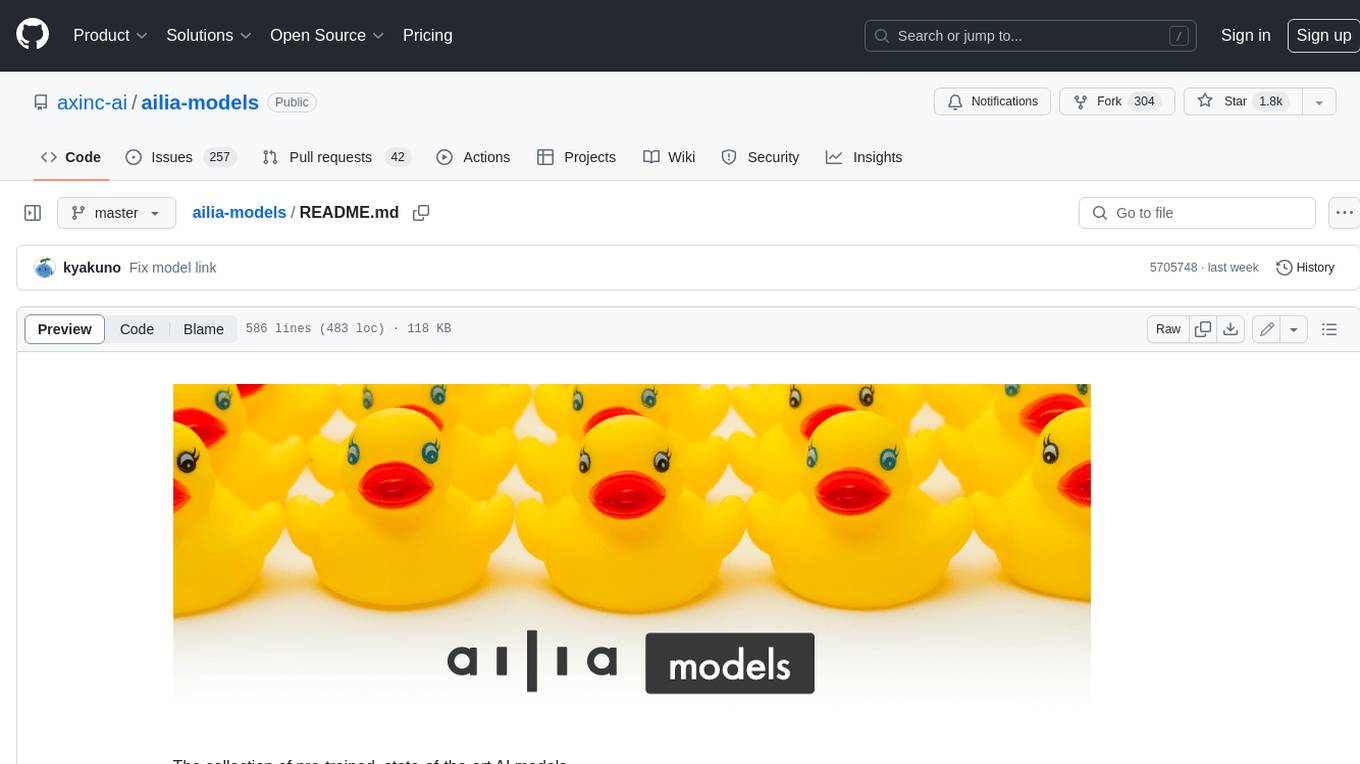
ailia-models
The collection of pre-trained, state-of-the-art AI models. ailia SDK is a self-contained, cross-platform, high-speed inference SDK for AI. The ailia SDK provides a consistent C++ API across Windows, Mac, Linux, iOS, Android, Jetson, and Raspberry Pi platforms. It also supports Unity (C#), Python, Rust, Flutter(Dart) and JNI for efficient AI implementation. The ailia SDK makes extensive use of the GPU through Vulkan and Metal to enable accelerated computing. # Supported models 323 models as of April 8th, 2024
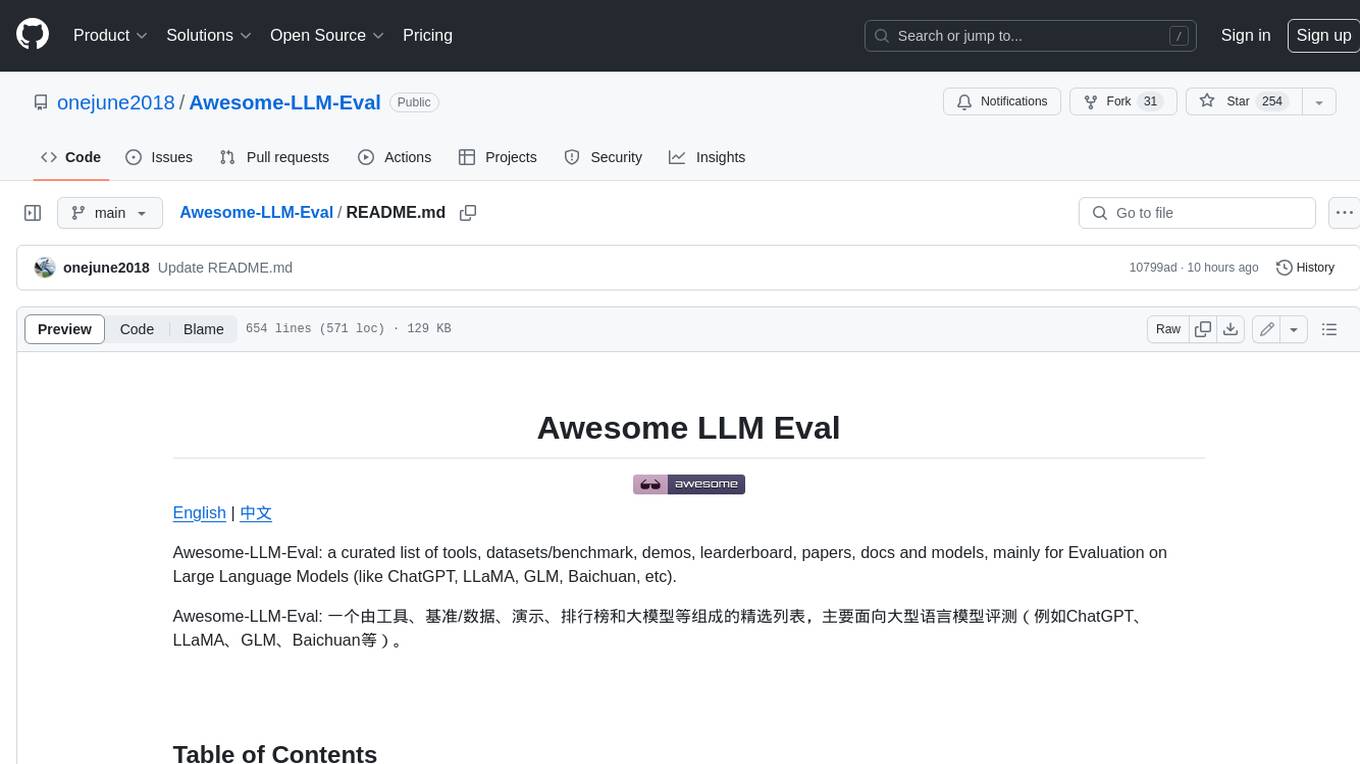
Awesome-LLM-Eval
Awesome-LLM-Eval: a curated list of tools, benchmarks, demos, papers for Large Language Models (like ChatGPT, LLaMA, GLM, Baichuan, etc) Evaluation on Language capabilities, Knowledge, Reasoning, Fairness and Safety.
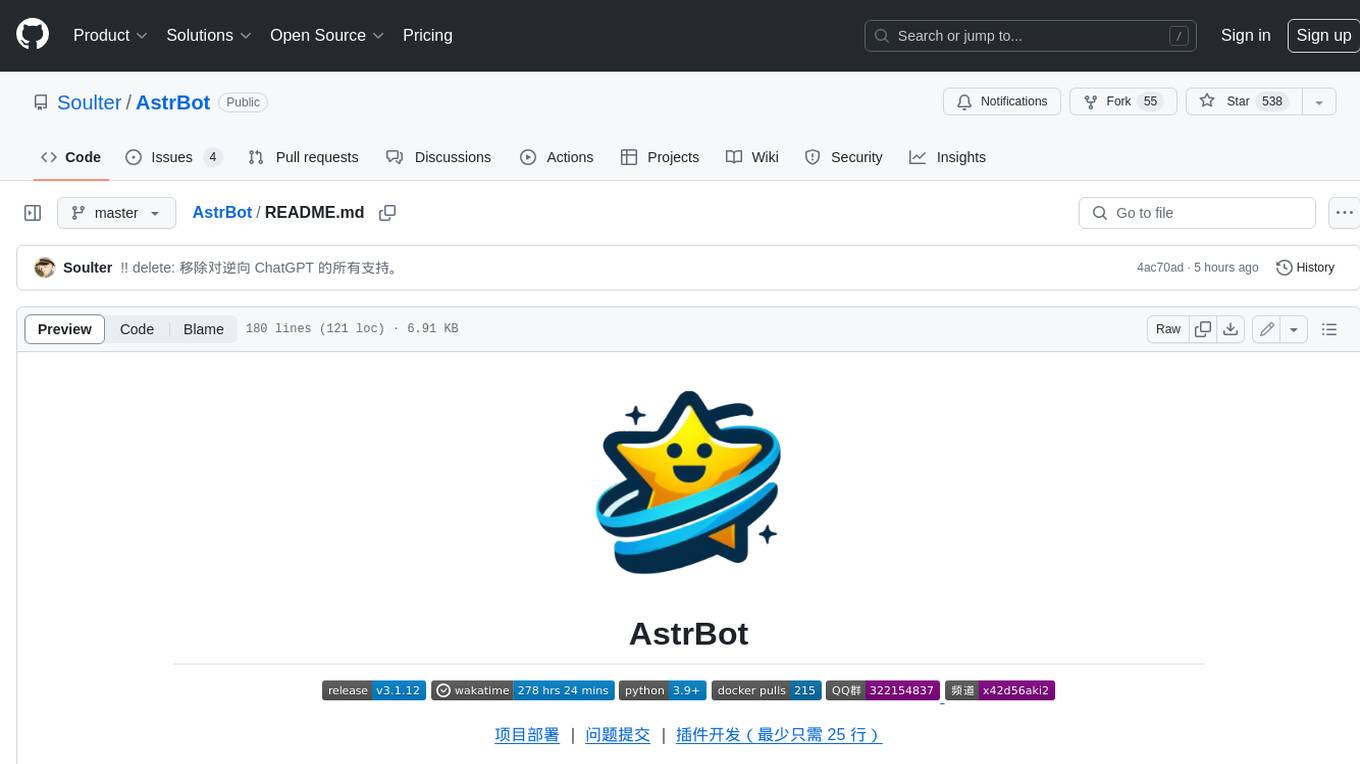
AstrBot
AstrBot is a powerful and versatile tool that leverages the capabilities of large language models (LLMs) like GPT-3, GPT-3.5, and GPT-4 to enhance communication and automate tasks. It seamlessly integrates with popular messaging platforms such as QQ, QQ Channel, and Telegram, enabling users to harness the power of AI within their daily conversations and workflows.
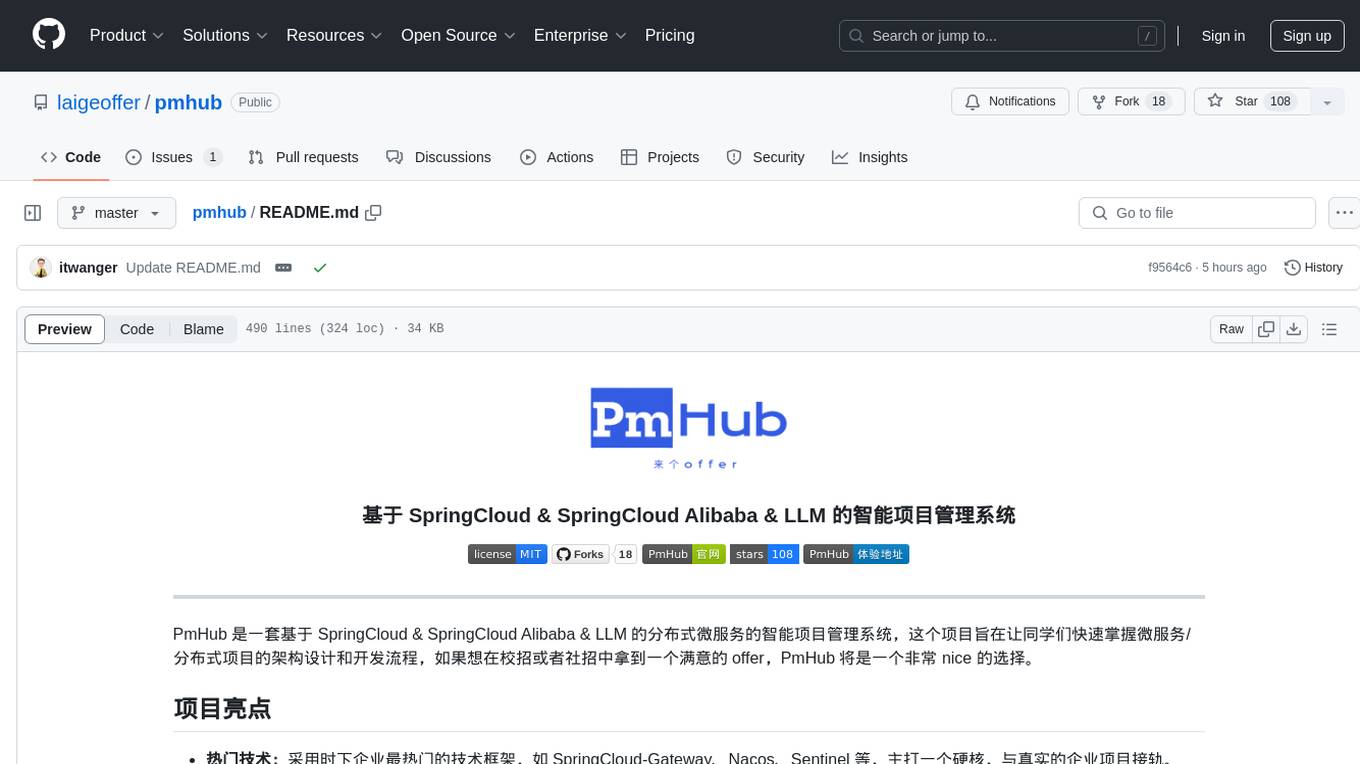
pmhub
PmHub is a smart project management system based on SpringCloud, SpringCloud Alibaba, and LLM. It aims to help students quickly grasp the architecture design and development process of microservices/distributed projects. PmHub provides a platform for students to experience the transformation from monolithic to microservices architecture, understand the pros and cons of both architectures, and prepare for job interviews. It offers popular technologies like SpringCloud-Gateway, Nacos, Sentinel, and provides high-quality code, continuous integration, product design documents, and an enterprise workflow system. PmHub is suitable for beginners and advanced learners who want to master core knowledge of microservices/distributed projects.
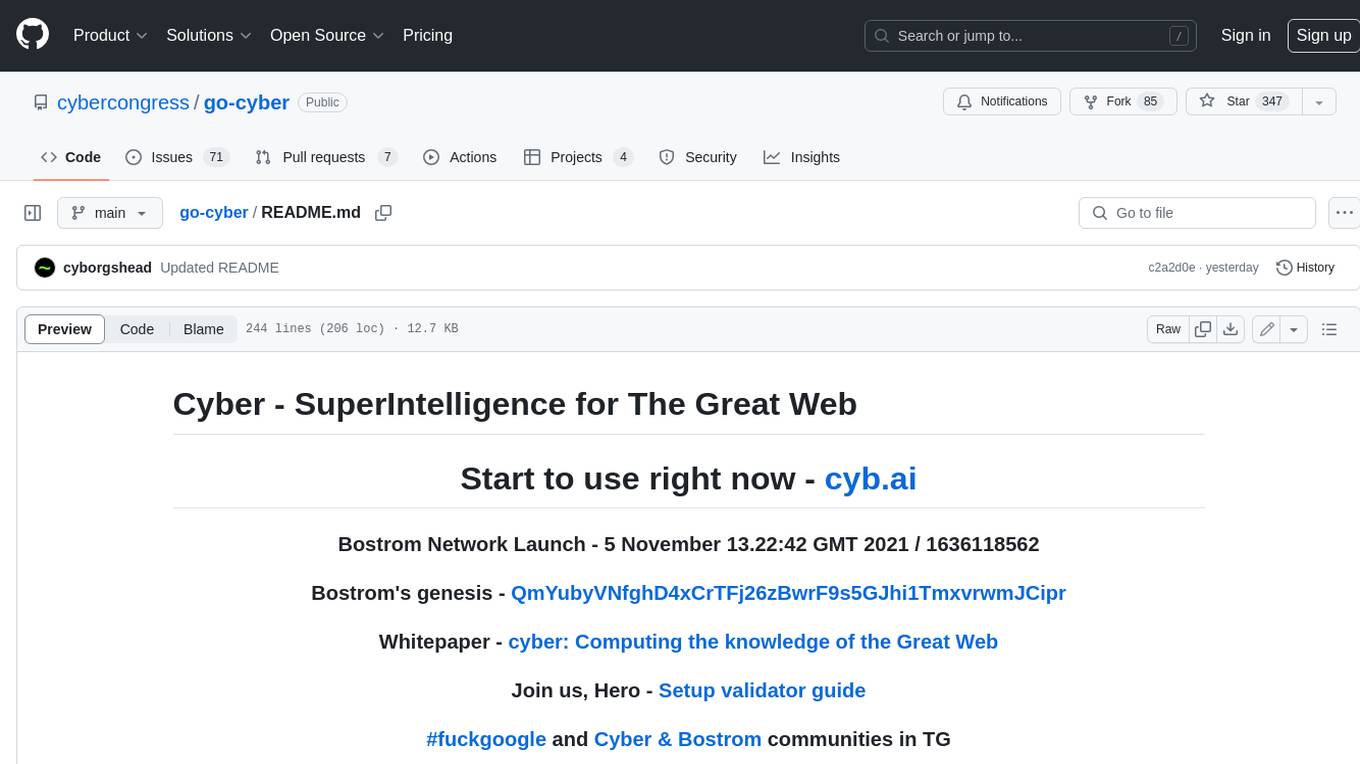
go-cyber
Cyber is a superintelligence protocol that aims to create a decentralized and censorship-resistant internet. It uses a novel consensus mechanism called CometBFT and a knowledge graph to store and process information. Cyber is designed to be scalable, secure, and efficient, and it has the potential to revolutionize the way we interact with the internet.
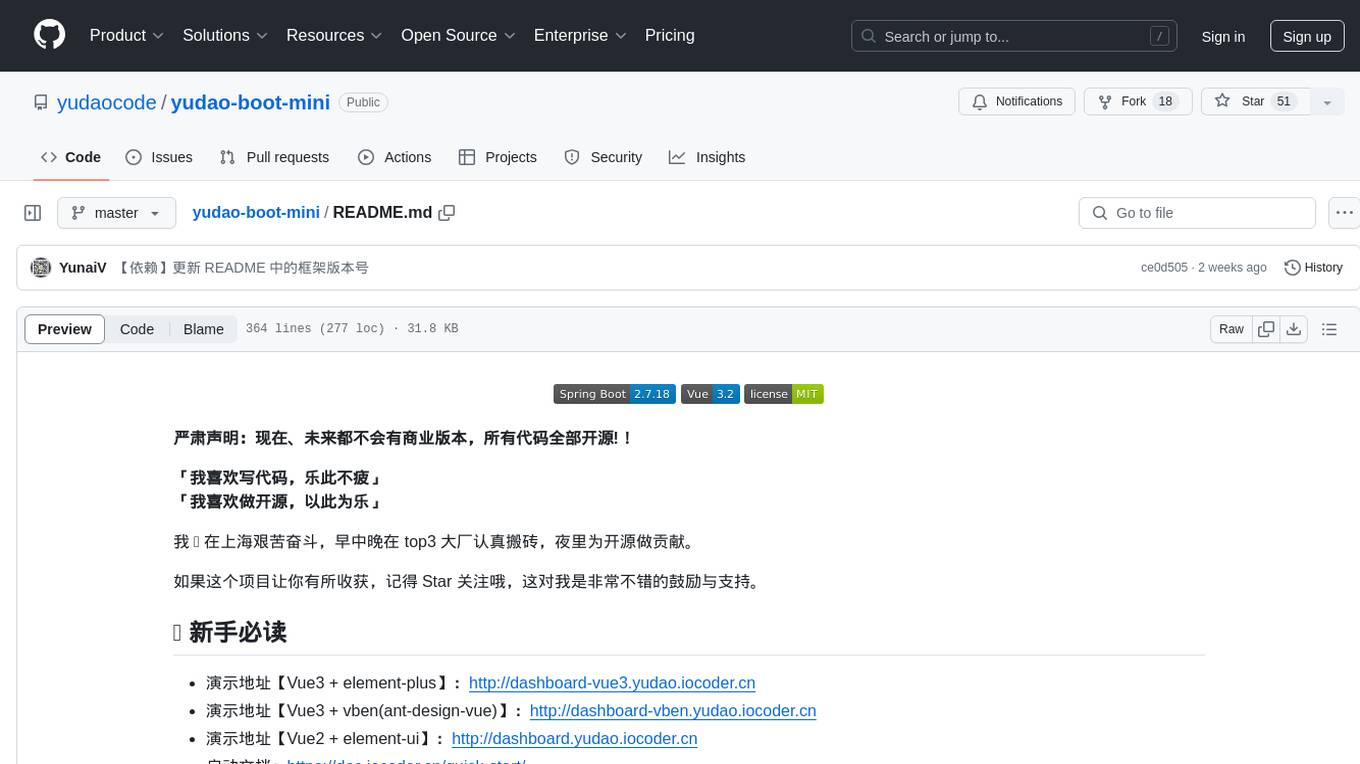
yudao-boot-mini
yudao-boot-mini is an open-source project focused on developing a rapid development platform for developers in China. It includes features like system functions, infrastructure, member center, data reports, workflow, mall system, WeChat official account, CRM, ERP, etc. The project is based on Spring Boot with Java backend and Vue for frontend. It offers various functionalities such as user management, role management, menu management, department management, workflow management, payment system, code generation, API documentation, database documentation, file service, WebSocket integration, message queue, Java monitoring, and more. The project is licensed under the MIT License, allowing both individuals and enterprises to use it freely without restrictions.
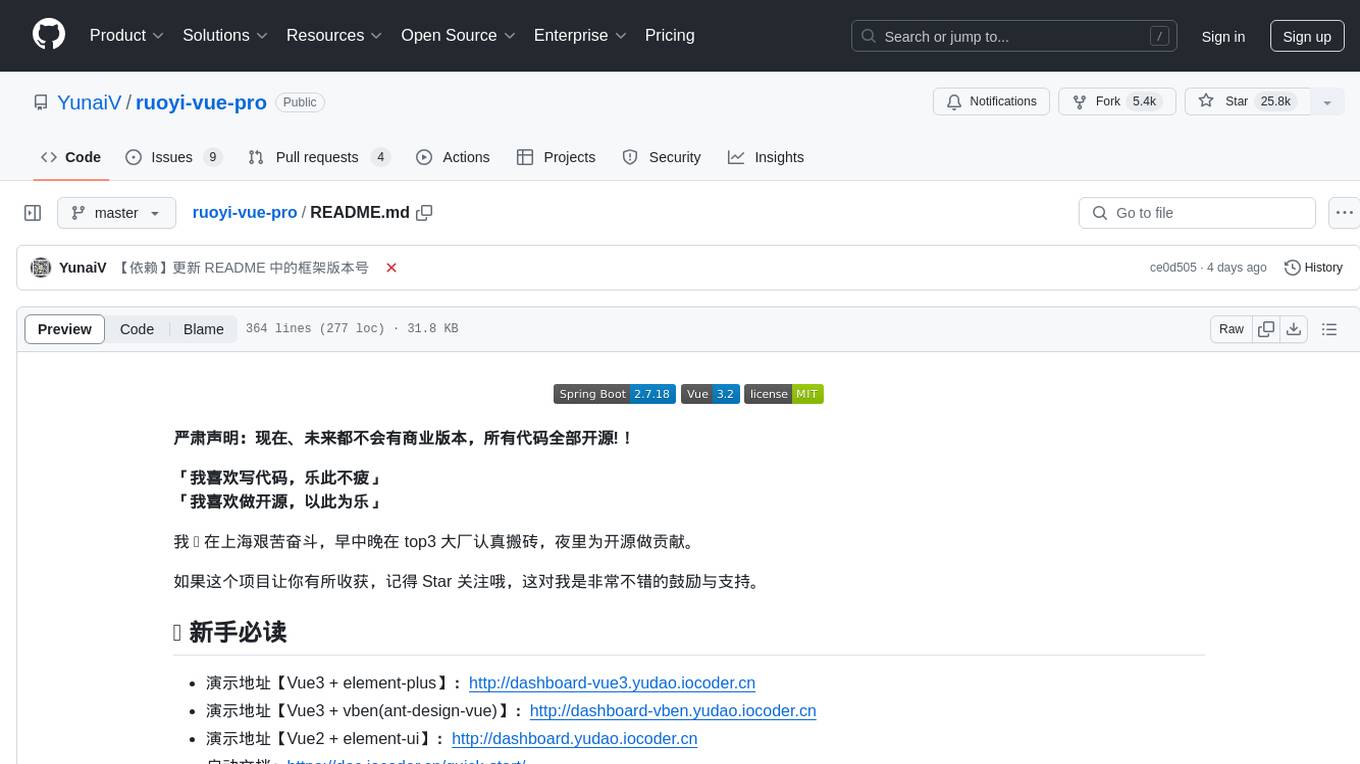
ruoyi-vue-pro
The ruoyi-vue-pro repository is an open-source project that provides a comprehensive development platform with various functionalities such as system features, infrastructure, member center, data reports, workflow, payment system, mall system, ERP system, CRM system, and AI big model. It is built using Java backend with Spring Boot framework and Vue frontend with different versions like Vue3 with element-plus, Vue3 with vben(ant-design-vue), and Vue2 with element-ui. The project aims to offer a fast development platform for developers and enterprises, supporting features like dynamic menu loading, button-level access control, SaaS multi-tenancy, code generator, real-time communication, integration with third-party services like WeChat, Alipay, and cloud services, and more.
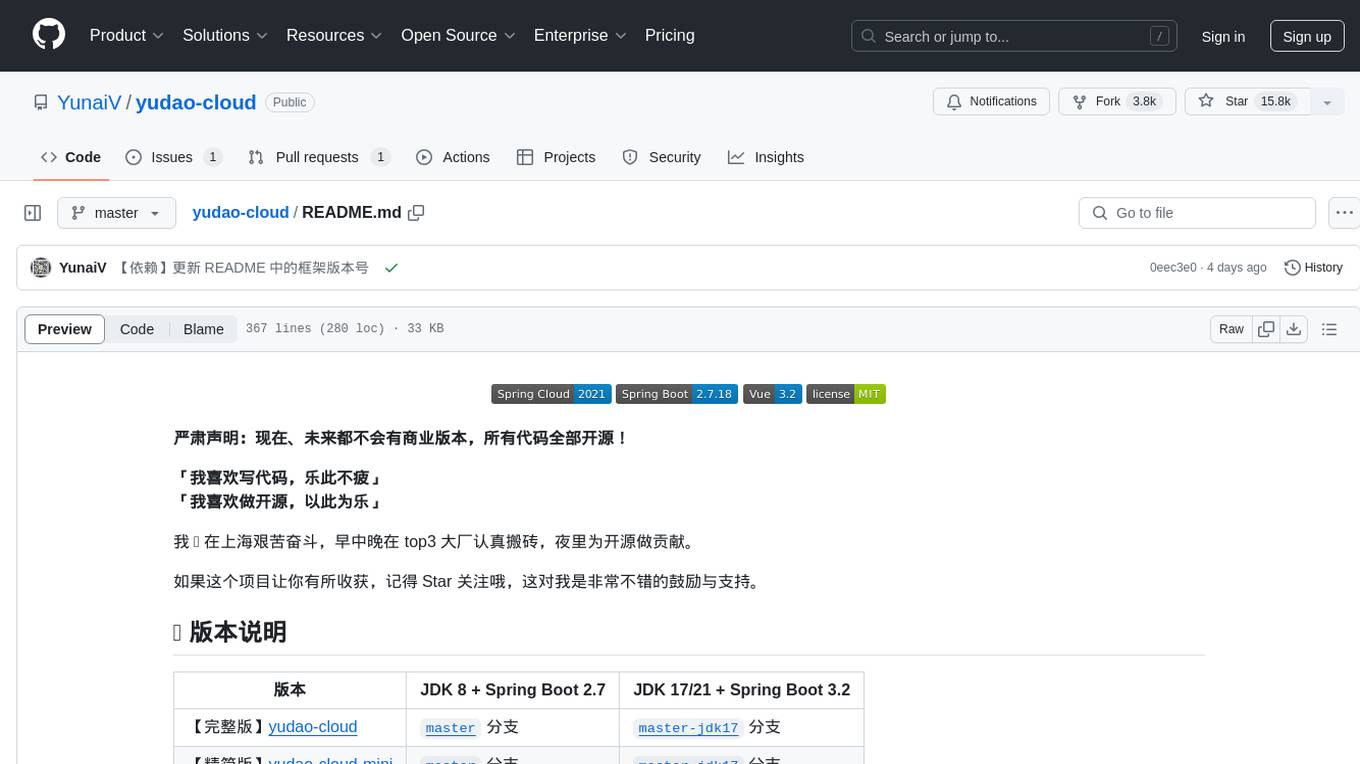
yudao-cloud
Yudao-cloud is an open-source project designed to provide a fast development platform for developers in China. It includes various system functions, infrastructure, member center, data reports, workflow, mall system, WeChat public account, CRM, ERP, etc. The project is based on Java backend with Spring Boot and Spring Cloud Alibaba microservices architecture. It supports multiple databases, message queues, authentication systems, dynamic menu loading, SaaS multi-tenant system, code generator, real-time communication, integration with third-party services like WeChat, Alipay, and more. The project is well-documented and follows the Alibaba Java development guidelines, ensuring clean code and architecture.
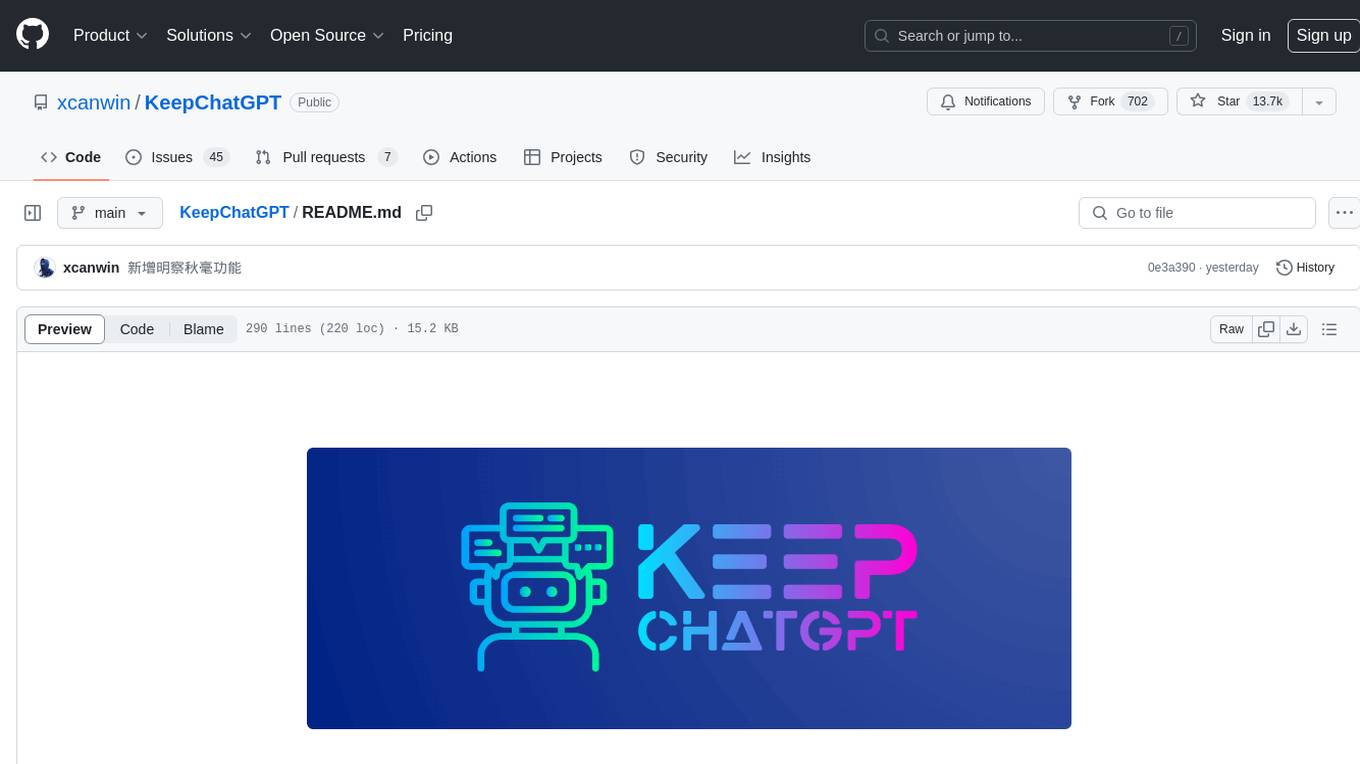
KeepChatGPT
KeepChatGPT is a plugin designed to enhance the data security capabilities and efficiency of ChatGPT. It aims to make your chat experience incredibly smooth, eliminating dozens or even hundreds of unnecessary steps, and permanently getting rid of various errors and warnings. It offers innovative features such as automatic refresh, activity maintenance, data security, audit cancellation, conversation cloning, endless conversations, page purification, large screen display, full screen display, tracking interception, rapid changes, and detailed insights. The plugin ensures that your AI experience is secure, smooth, efficient, concise, and seamless.
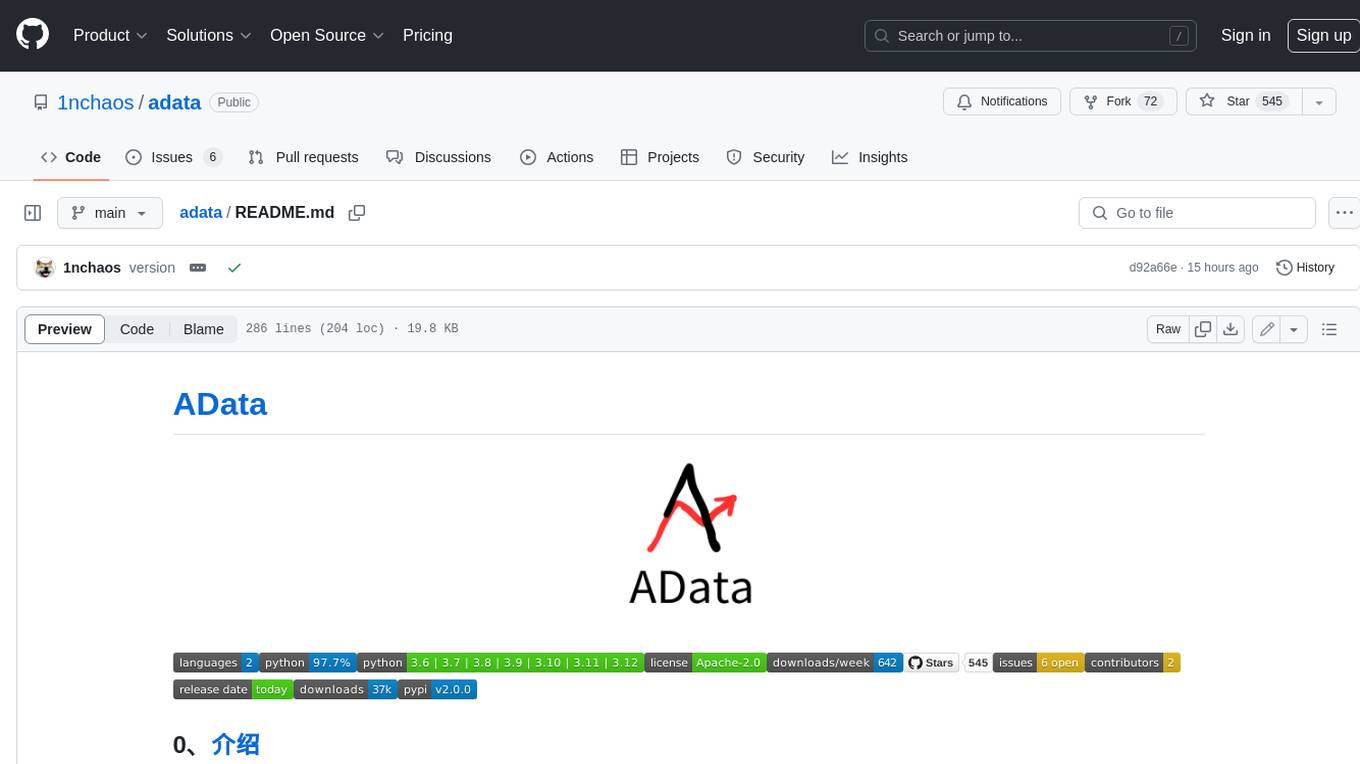
adata
AData is a free and open-source A-share database that focuses on transaction-related data. It provides comprehensive data on stocks, including basic information, market data, and sentiment analysis. AData is designed to be easy to use and integrate with other applications, making it a valuable tool for quantitative trading and AI training.
For similar tasks
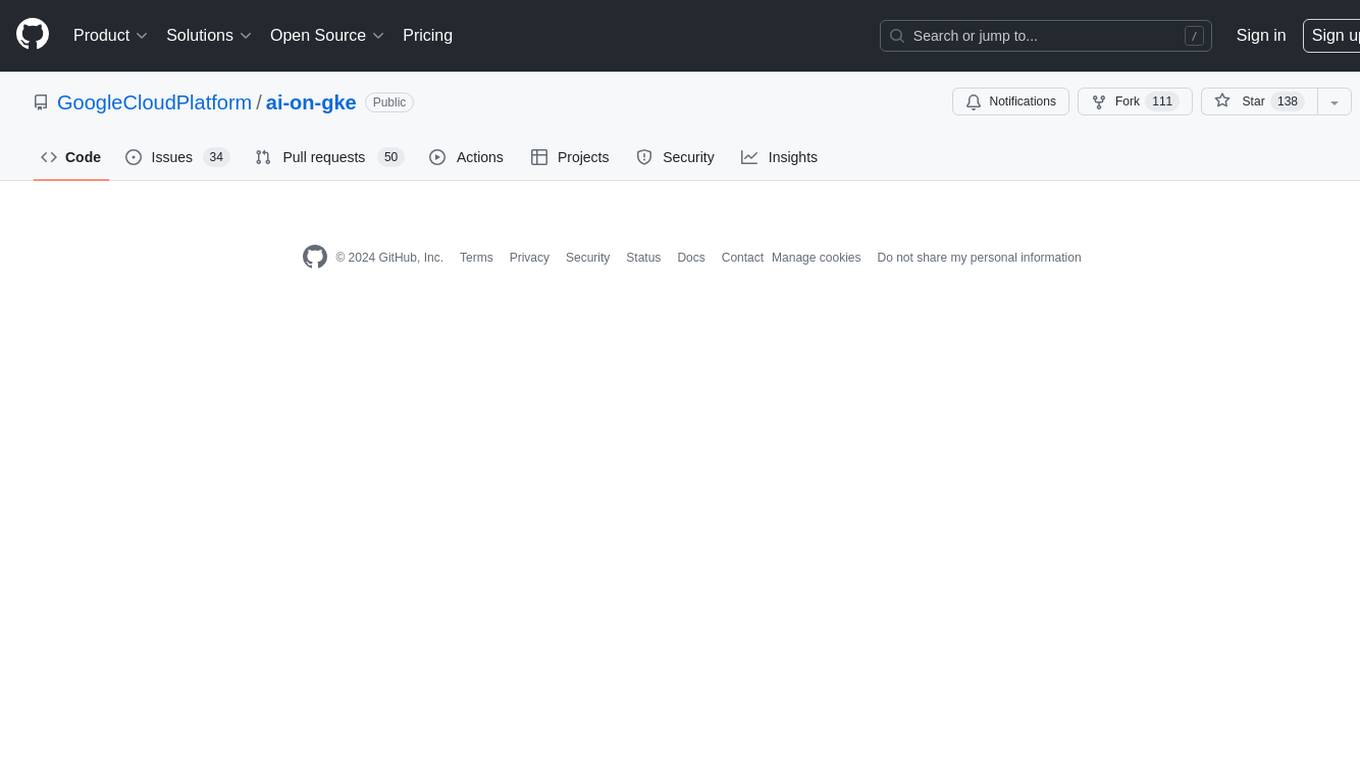
ai-on-gke
This repository contains assets related to AI/ML workloads on Google Kubernetes Engine (GKE). Run optimized AI/ML workloads with Google Kubernetes Engine (GKE) platform orchestration capabilities. A robust AI/ML platform considers the following layers: Infrastructure orchestration that support GPUs and TPUs for training and serving workloads at scale Flexible integration with distributed computing and data processing frameworks Support for multiple teams on the same infrastructure to maximize utilization of resources
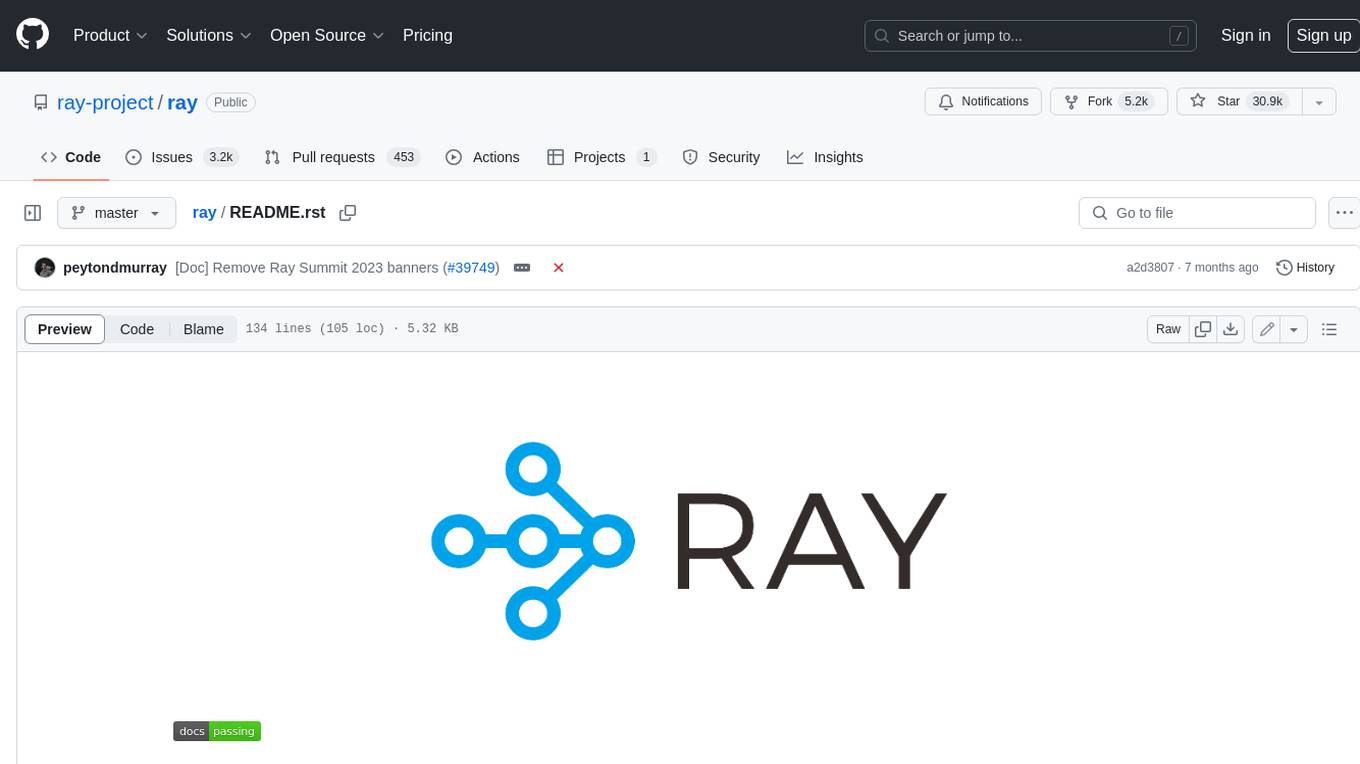
ray
Ray is a unified framework for scaling AI and Python applications. It consists of a core distributed runtime and a set of AI libraries for simplifying ML compute, including Data, Train, Tune, RLlib, and Serve. Ray runs on any machine, cluster, cloud provider, and Kubernetes, and features a growing ecosystem of community integrations. With Ray, you can seamlessly scale the same code from a laptop to a cluster, making it easy to meet the compute-intensive demands of modern ML workloads.
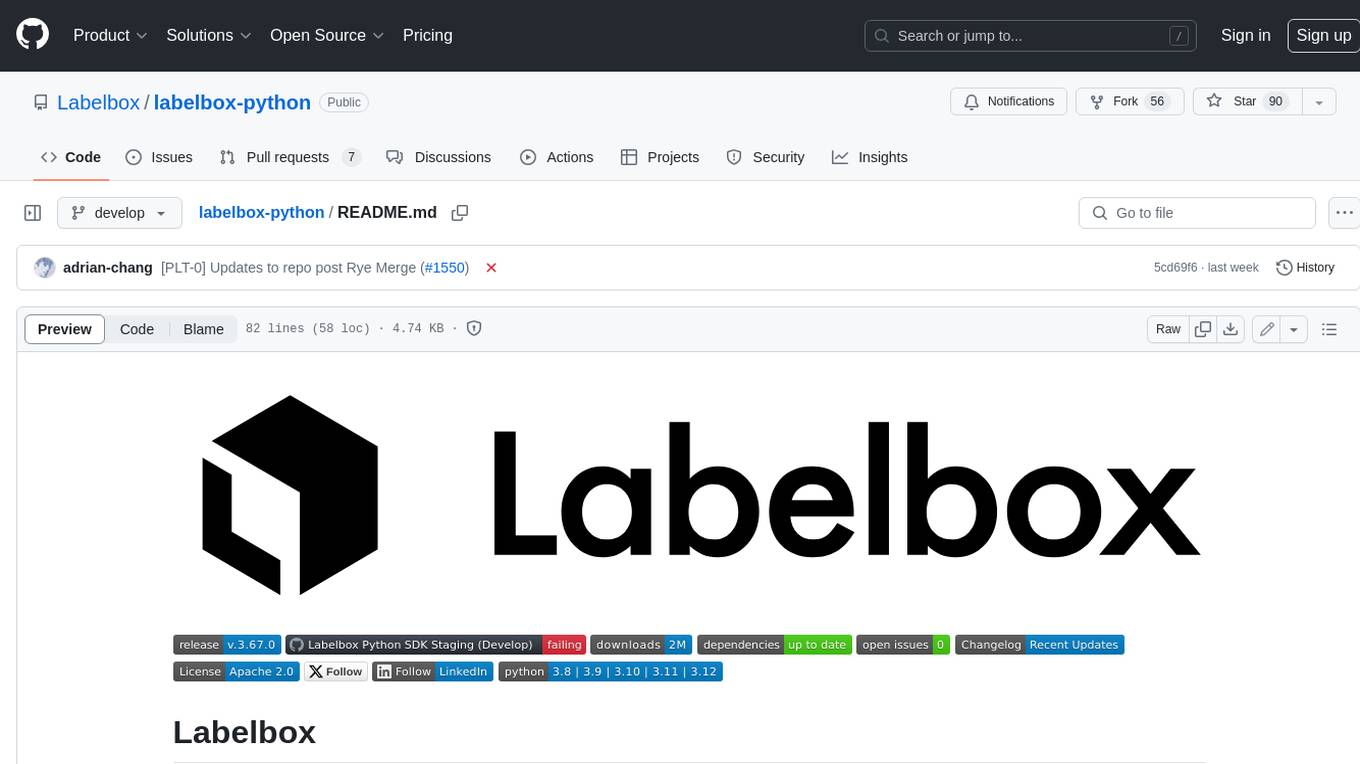
labelbox-python
Labelbox is a data-centric AI platform for enterprises to develop, optimize, and use AI to solve problems and power new products and services. Enterprises use Labelbox to curate data, generate high-quality human feedback data for computer vision and LLMs, evaluate model performance, and automate tasks by combining AI and human-centric workflows. The academic & research community uses Labelbox for cutting-edge AI research.
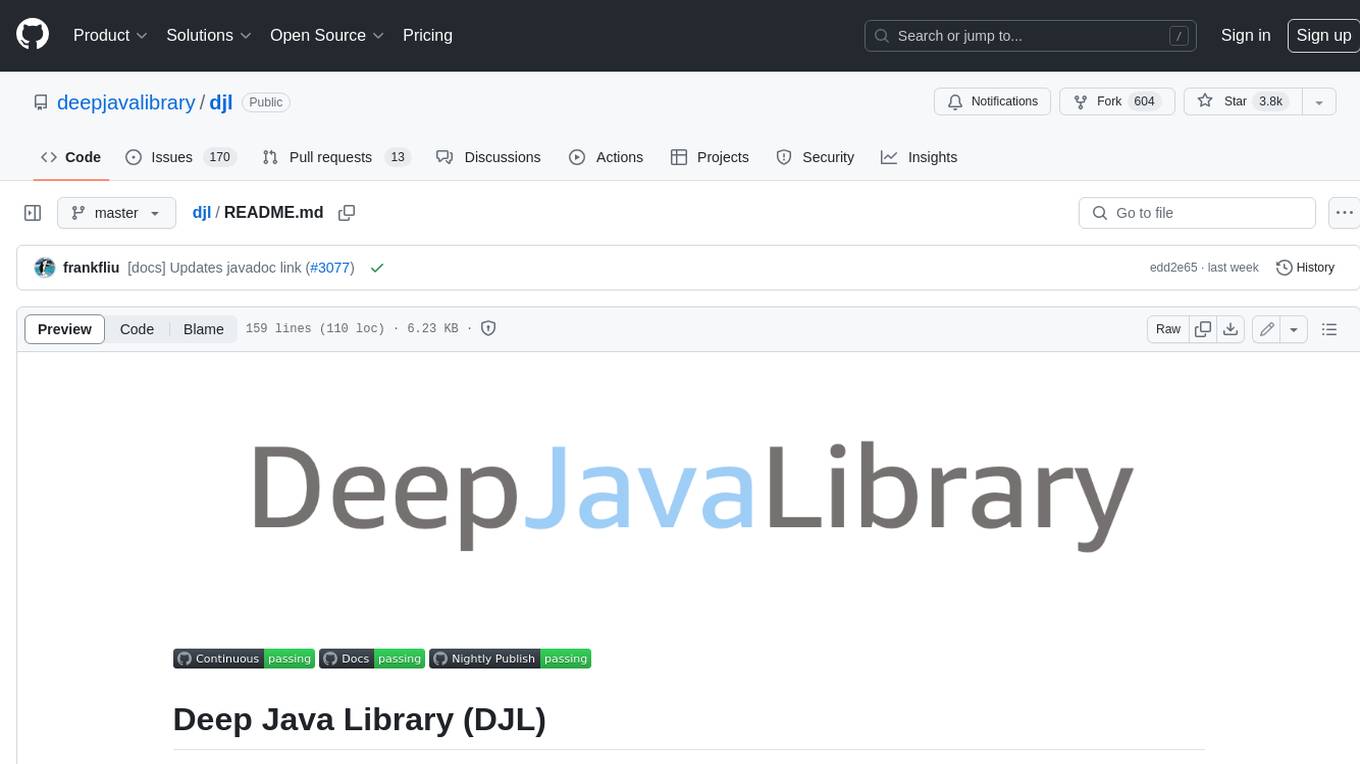
djl
Deep Java Library (DJL) is an open-source, high-level, engine-agnostic Java framework for deep learning. It is designed to be easy to get started with and simple to use for Java developers. DJL provides a native Java development experience and allows users to integrate machine learning and deep learning models with their Java applications. The framework is deep learning engine agnostic, enabling users to switch engines at any point for optimal performance. DJL's ergonomic API interface guides users with best practices to accomplish deep learning tasks, such as running inference and training neural networks.
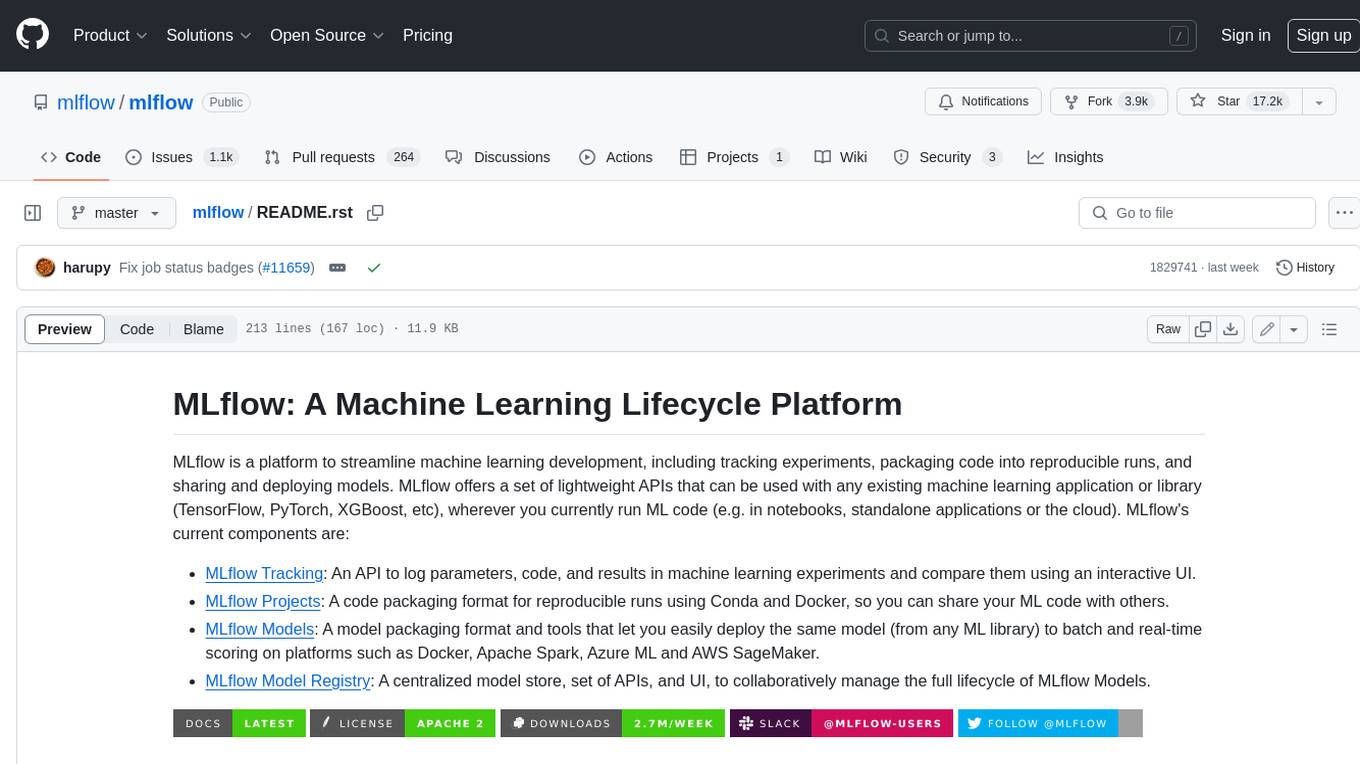
mlflow
MLflow is a platform to streamline machine learning development, including tracking experiments, packaging code into reproducible runs, and sharing and deploying models. MLflow offers a set of lightweight APIs that can be used with any existing machine learning application or library (TensorFlow, PyTorch, XGBoost, etc), wherever you currently run ML code (e.g. in notebooks, standalone applications or the cloud). MLflow's current components are:
* `MLflow Tracking
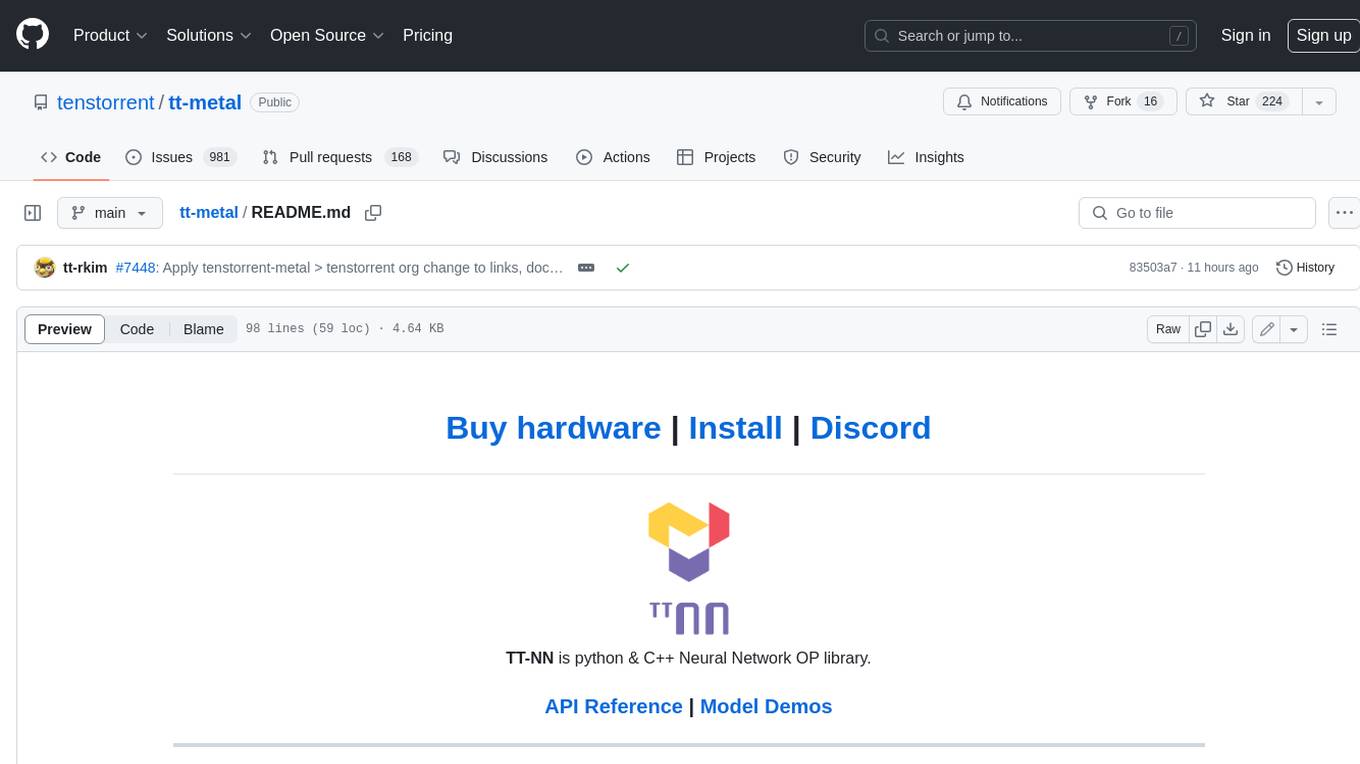
tt-metal
TT-NN is a python & C++ Neural Network OP library. It provides a low-level programming model, TT-Metalium, enabling kernel development for Tenstorrent hardware.
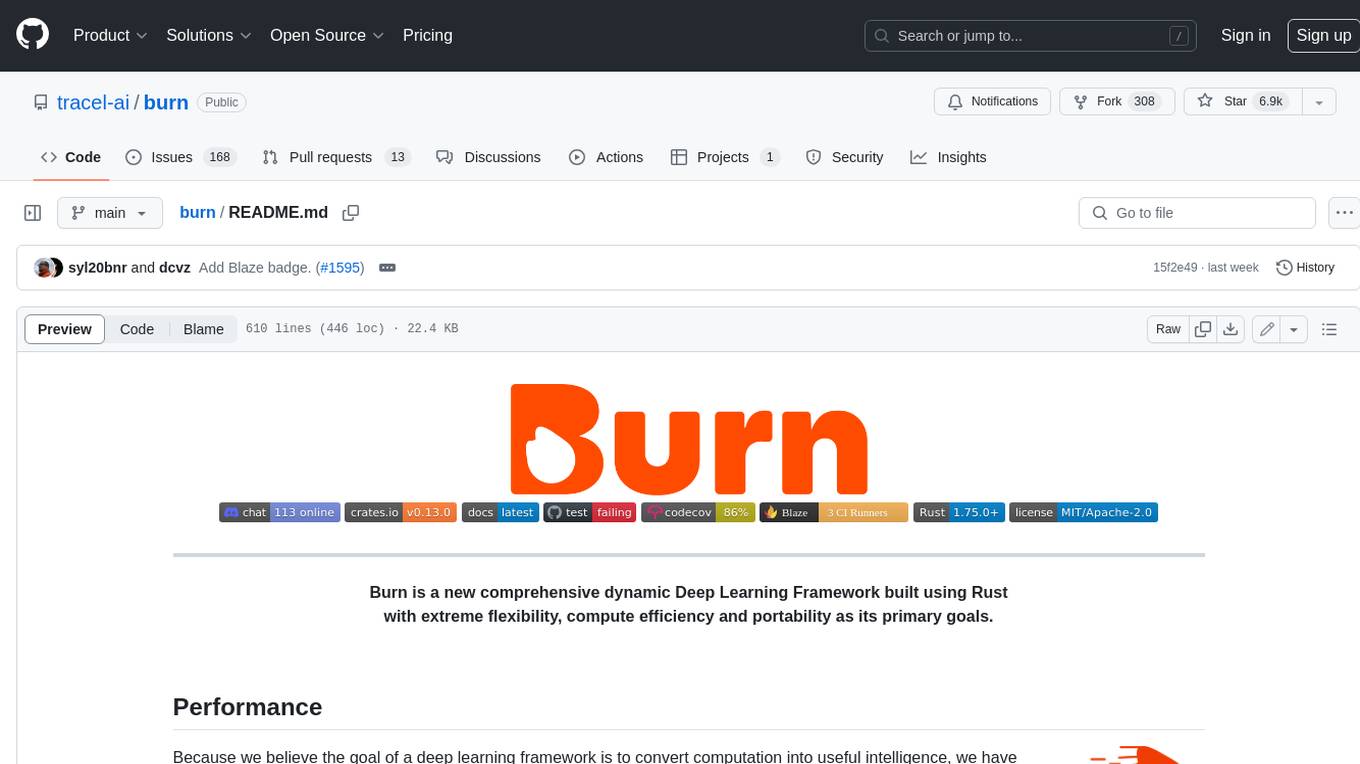
burn
Burn is a new comprehensive dynamic Deep Learning Framework built using Rust with extreme flexibility, compute efficiency and portability as its primary goals.
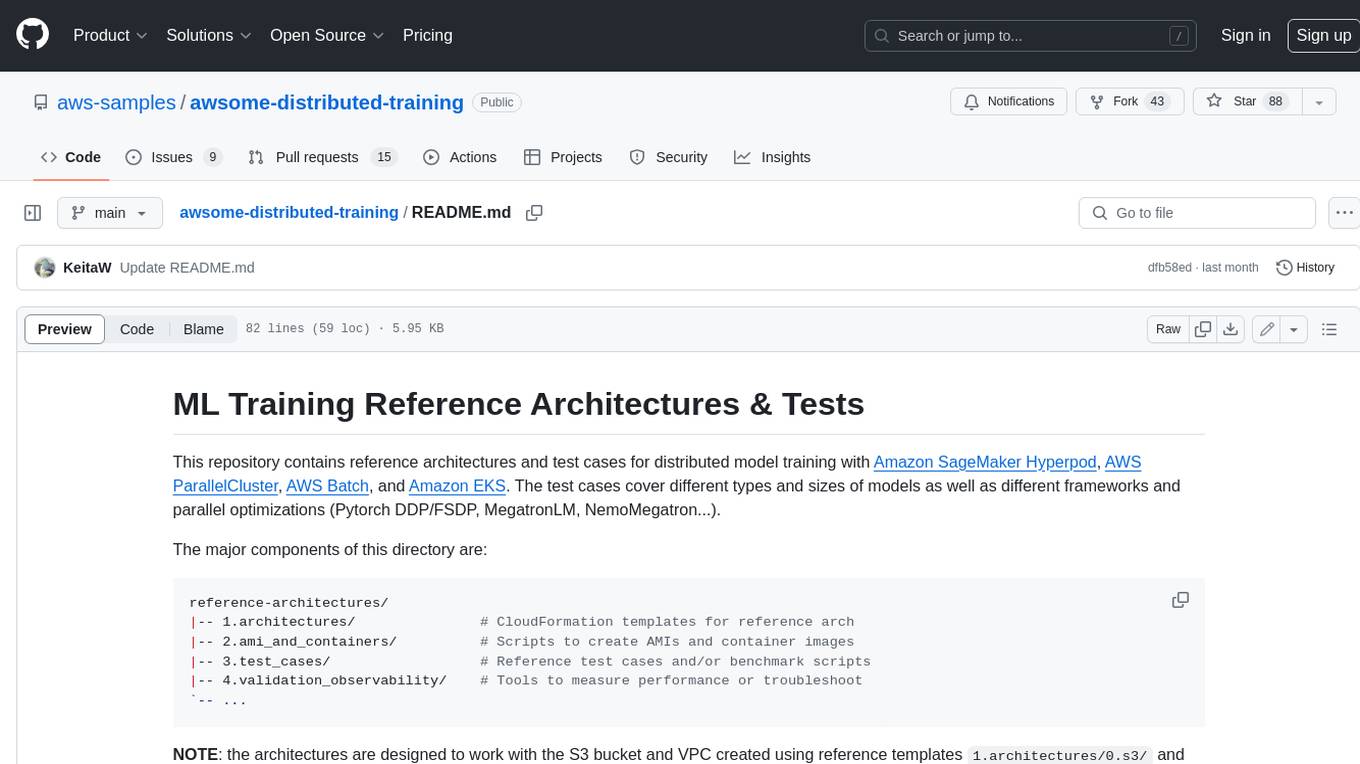
awsome-distributed-training
This repository contains reference architectures and test cases for distributed model training with Amazon SageMaker Hyperpod, AWS ParallelCluster, AWS Batch, and Amazon EKS. The test cases cover different types and sizes of models as well as different frameworks and parallel optimizations (Pytorch DDP/FSDP, MegatronLM, NemoMegatron...).
For similar jobs
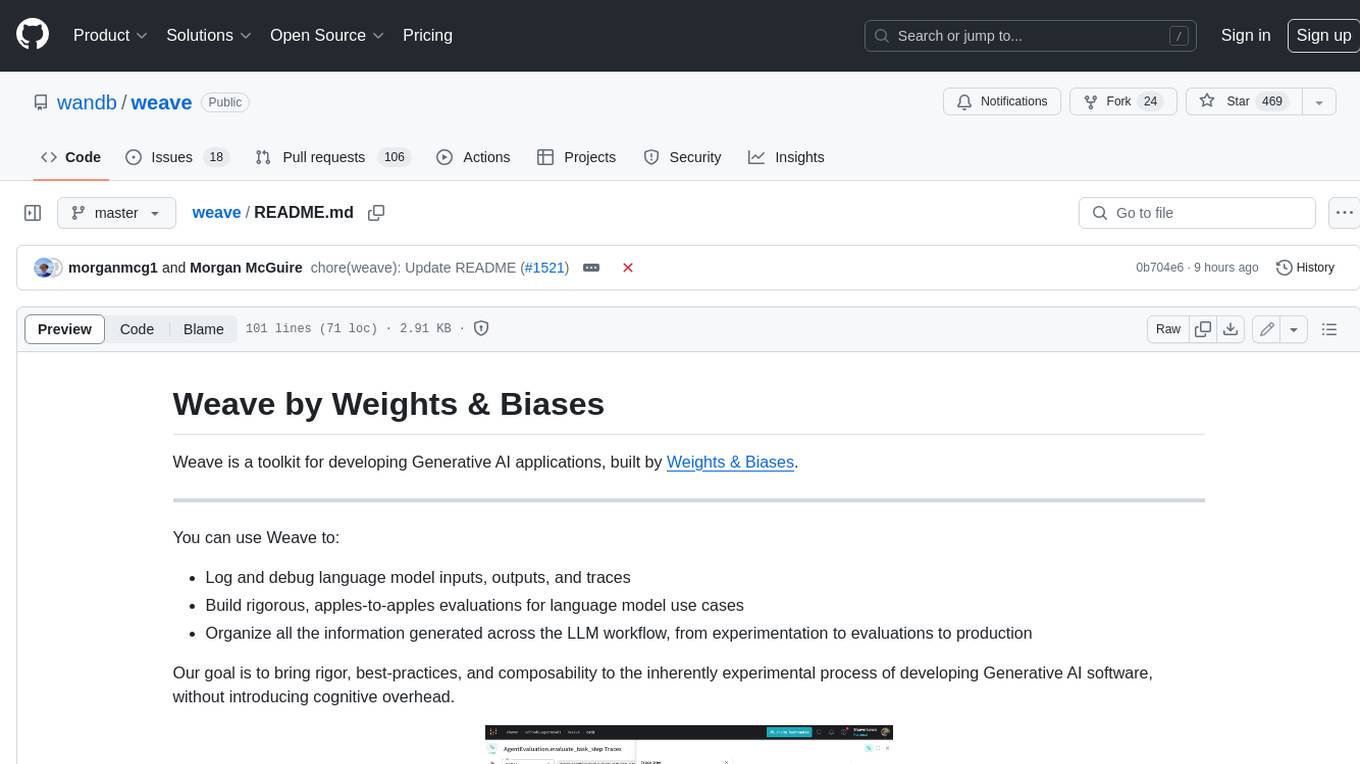
weave
Weave is a toolkit for developing Generative AI applications, built by Weights & Biases. With Weave, you can log and debug language model inputs, outputs, and traces; build rigorous, apples-to-apples evaluations for language model use cases; and organize all the information generated across the LLM workflow, from experimentation to evaluations to production. Weave aims to bring rigor, best-practices, and composability to the inherently experimental process of developing Generative AI software, without introducing cognitive overhead.
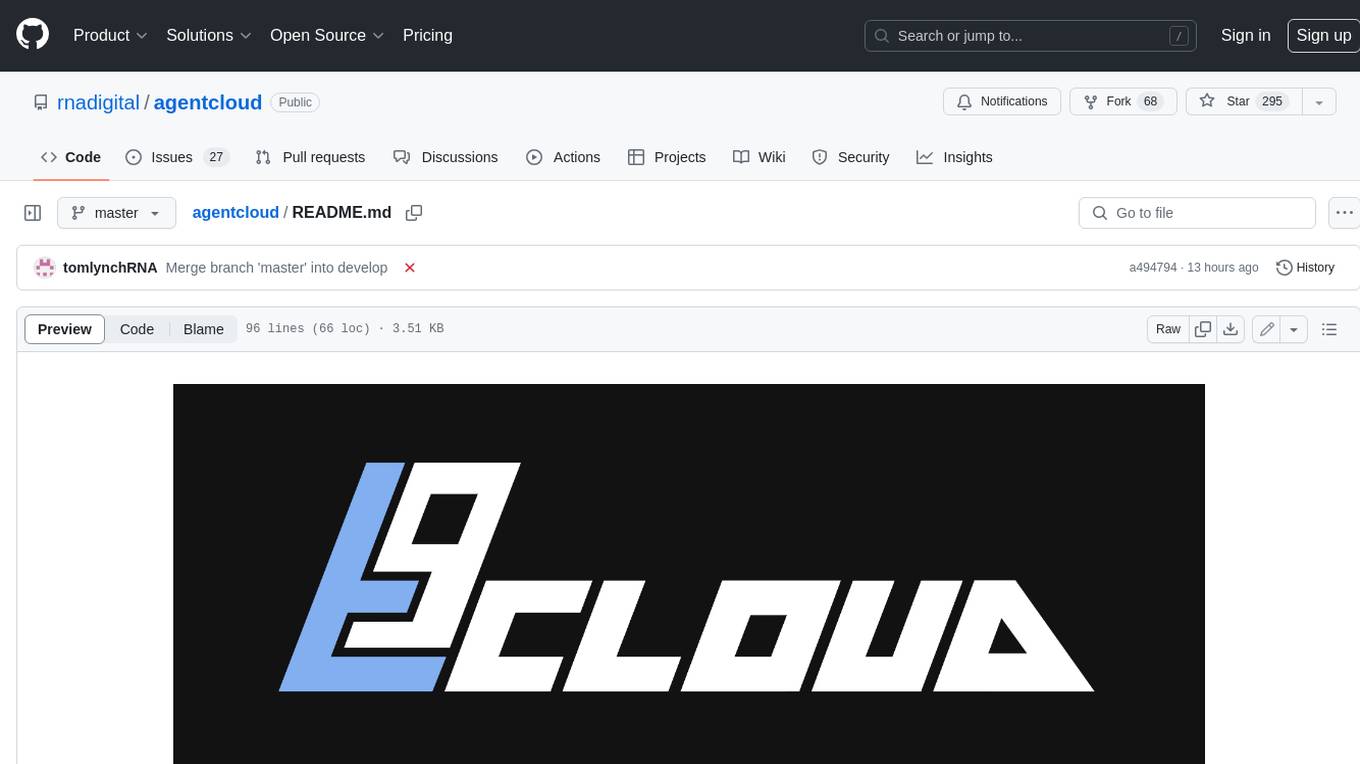
agentcloud
AgentCloud is an open-source platform that enables companies to build and deploy private LLM chat apps, empowering teams to securely interact with their data. It comprises three main components: Agent Backend, Webapp, and Vector Proxy. To run this project locally, clone the repository, install Docker, and start the services. The project is licensed under the GNU Affero General Public License, version 3 only. Contributions and feedback are welcome from the community.
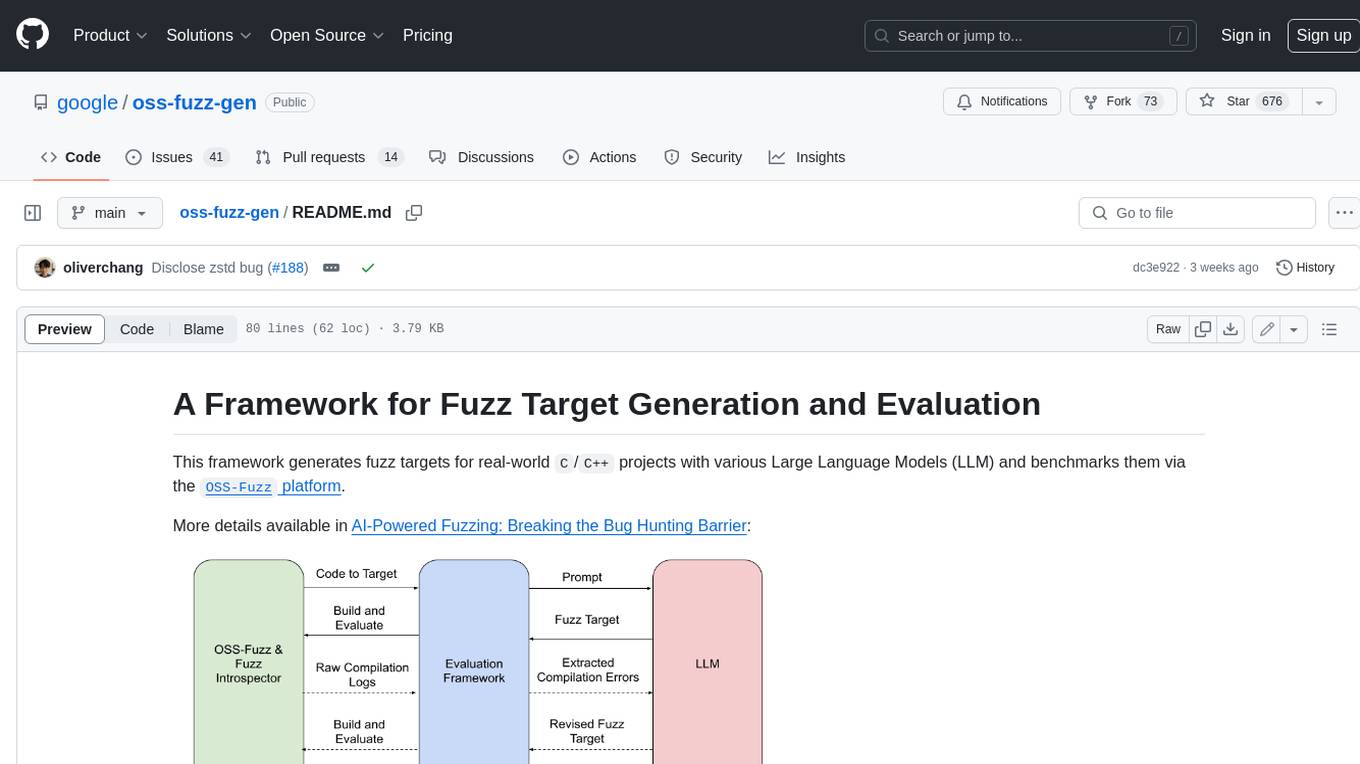
oss-fuzz-gen
This framework generates fuzz targets for real-world `C`/`C++` projects with various Large Language Models (LLM) and benchmarks them via the `OSS-Fuzz` platform. It manages to successfully leverage LLMs to generate valid fuzz targets (which generate non-zero coverage increase) for 160 C/C++ projects. The maximum line coverage increase is 29% from the existing human-written targets.
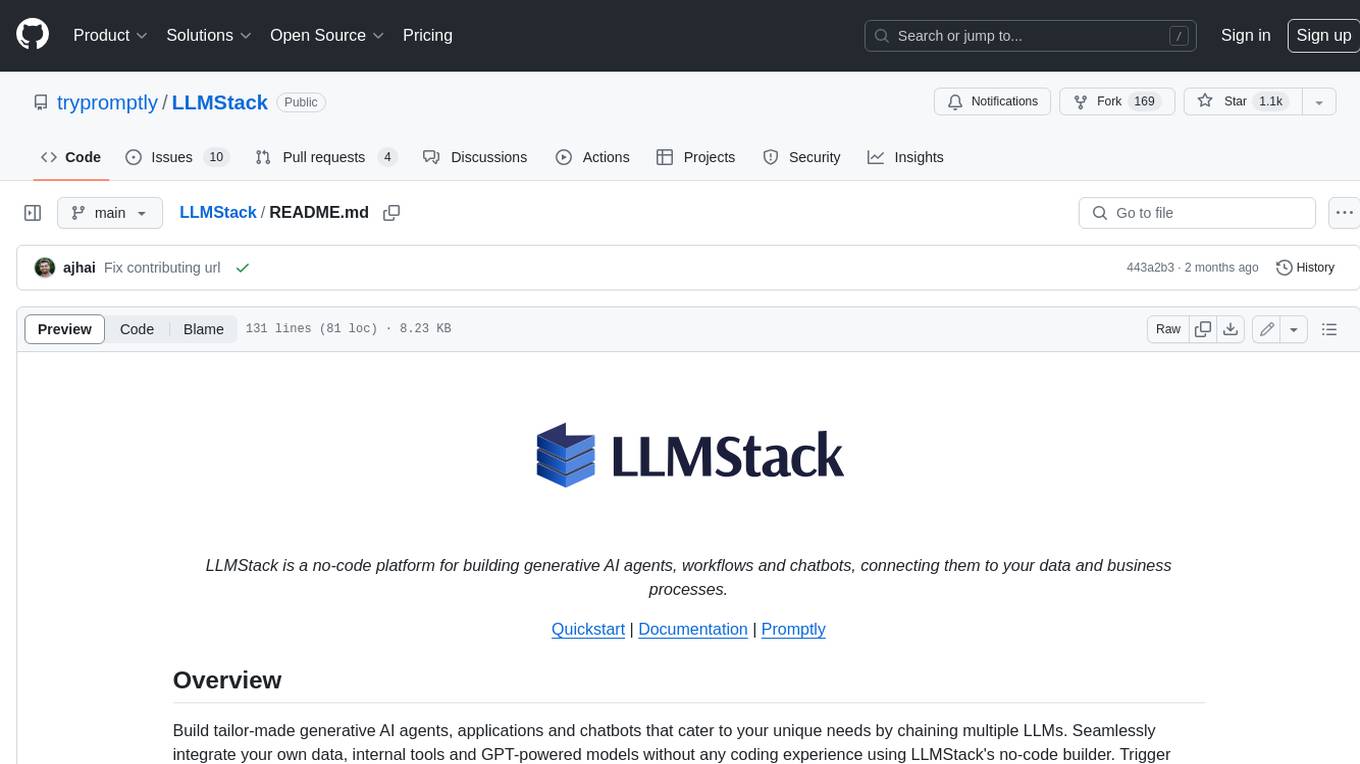
LLMStack
LLMStack is a no-code platform for building generative AI agents, workflows, and chatbots. It allows users to connect their own data, internal tools, and GPT-powered models without any coding experience. LLMStack can be deployed to the cloud or on-premise and can be accessed via HTTP API or triggered from Slack or Discord.
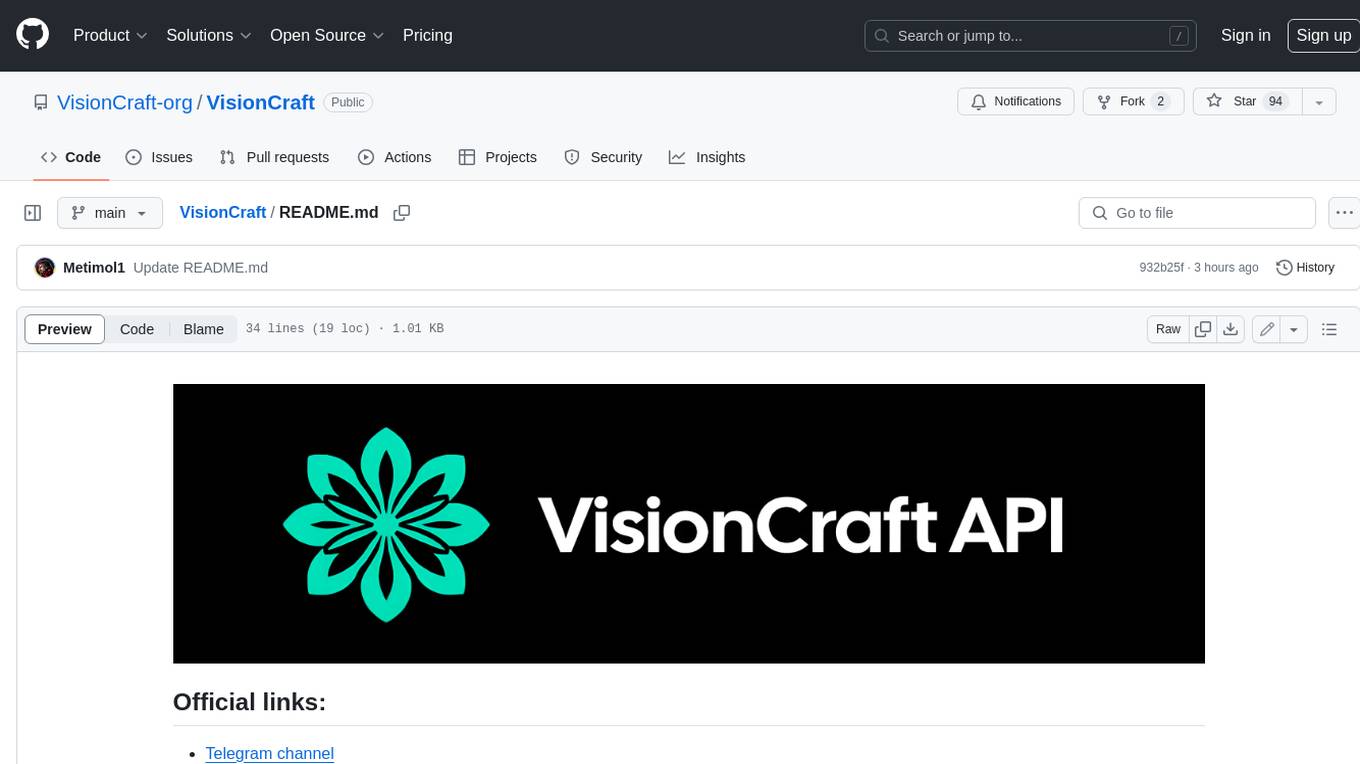
VisionCraft
The VisionCraft API is a free API for using over 100 different AI models. From images to sound.
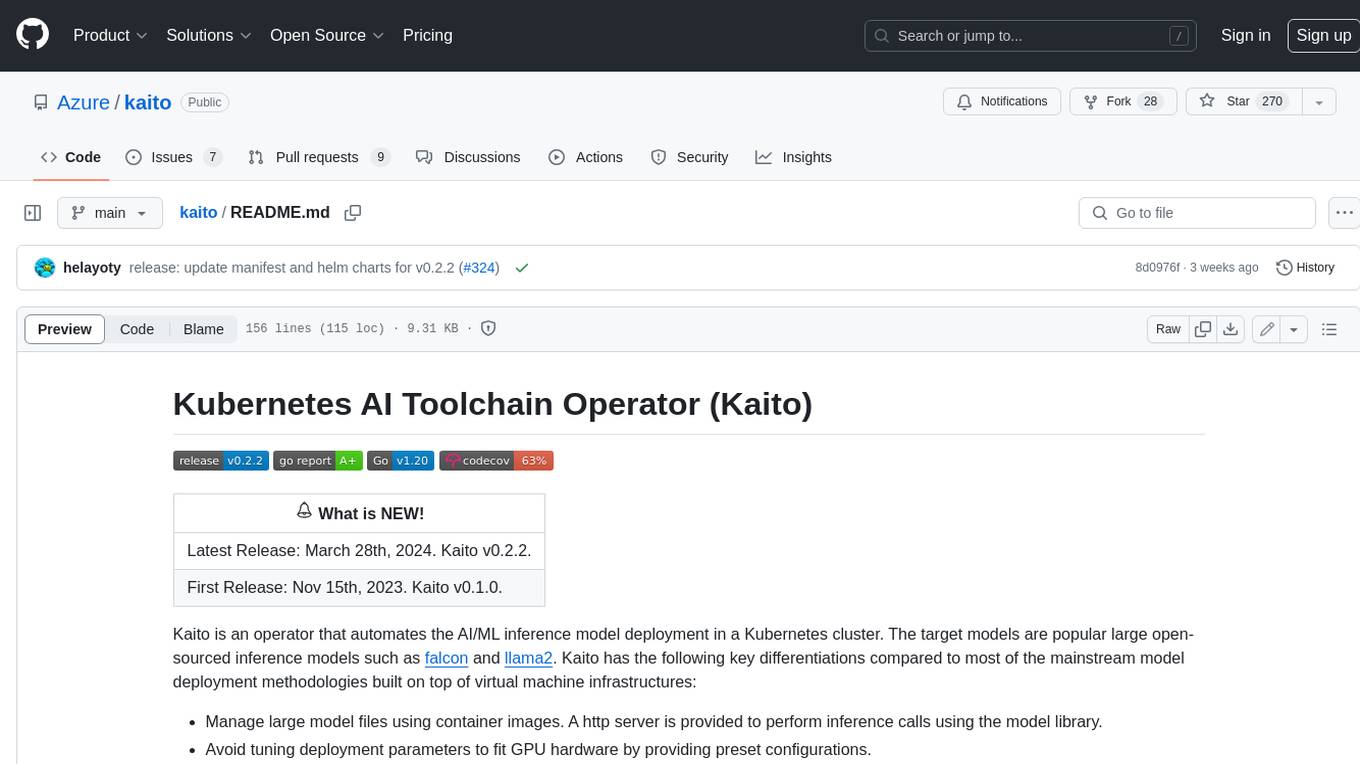
kaito
Kaito is an operator that automates the AI/ML inference model deployment in a Kubernetes cluster. It manages large model files using container images, avoids tuning deployment parameters to fit GPU hardware by providing preset configurations, auto-provisions GPU nodes based on model requirements, and hosts large model images in the public Microsoft Container Registry (MCR) if the license allows. Using Kaito, the workflow of onboarding large AI inference models in Kubernetes is largely simplified.
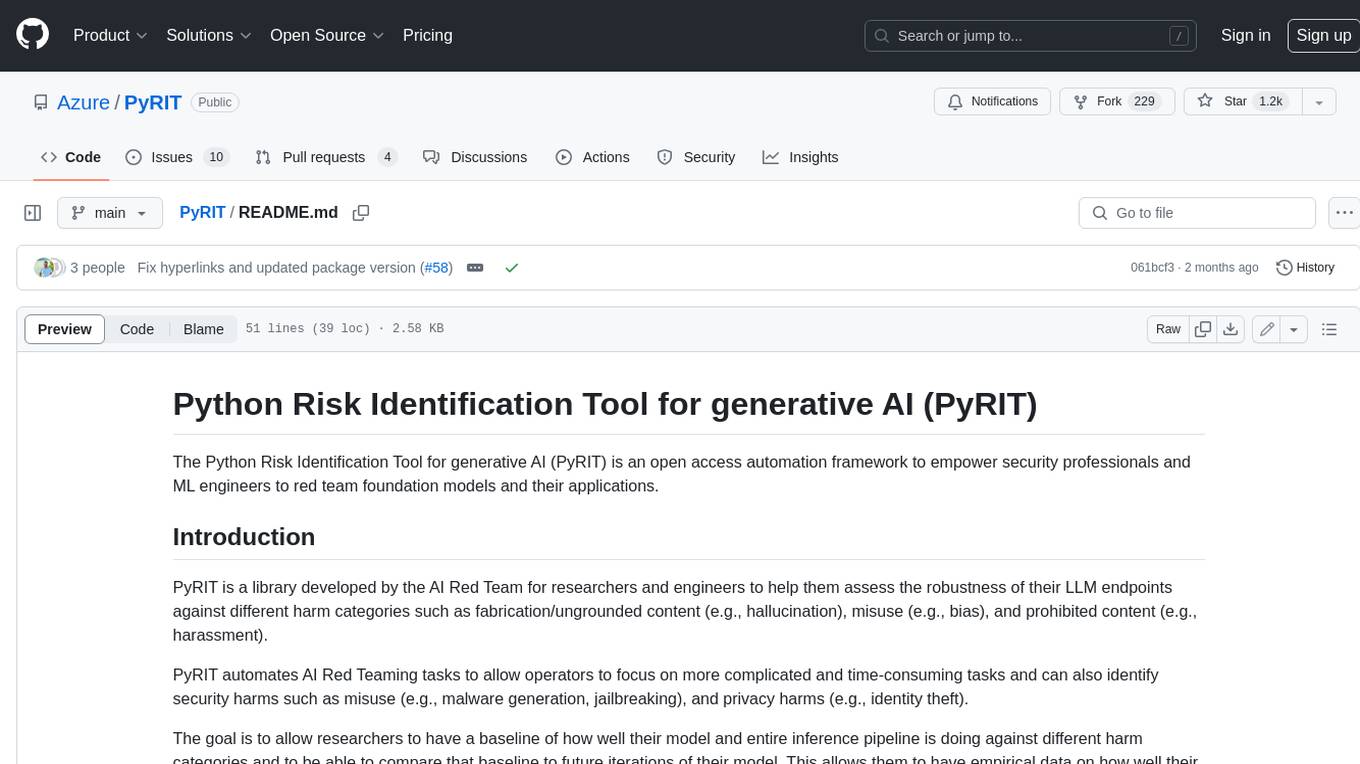
PyRIT
PyRIT is an open access automation framework designed to empower security professionals and ML engineers to red team foundation models and their applications. It automates AI Red Teaming tasks to allow operators to focus on more complicated and time-consuming tasks and can also identify security harms such as misuse (e.g., malware generation, jailbreaking), and privacy harms (e.g., identity theft). The goal is to allow researchers to have a baseline of how well their model and entire inference pipeline is doing against different harm categories and to be able to compare that baseline to future iterations of their model. This allows them to have empirical data on how well their model is doing today, and detect any degradation of performance based on future improvements.
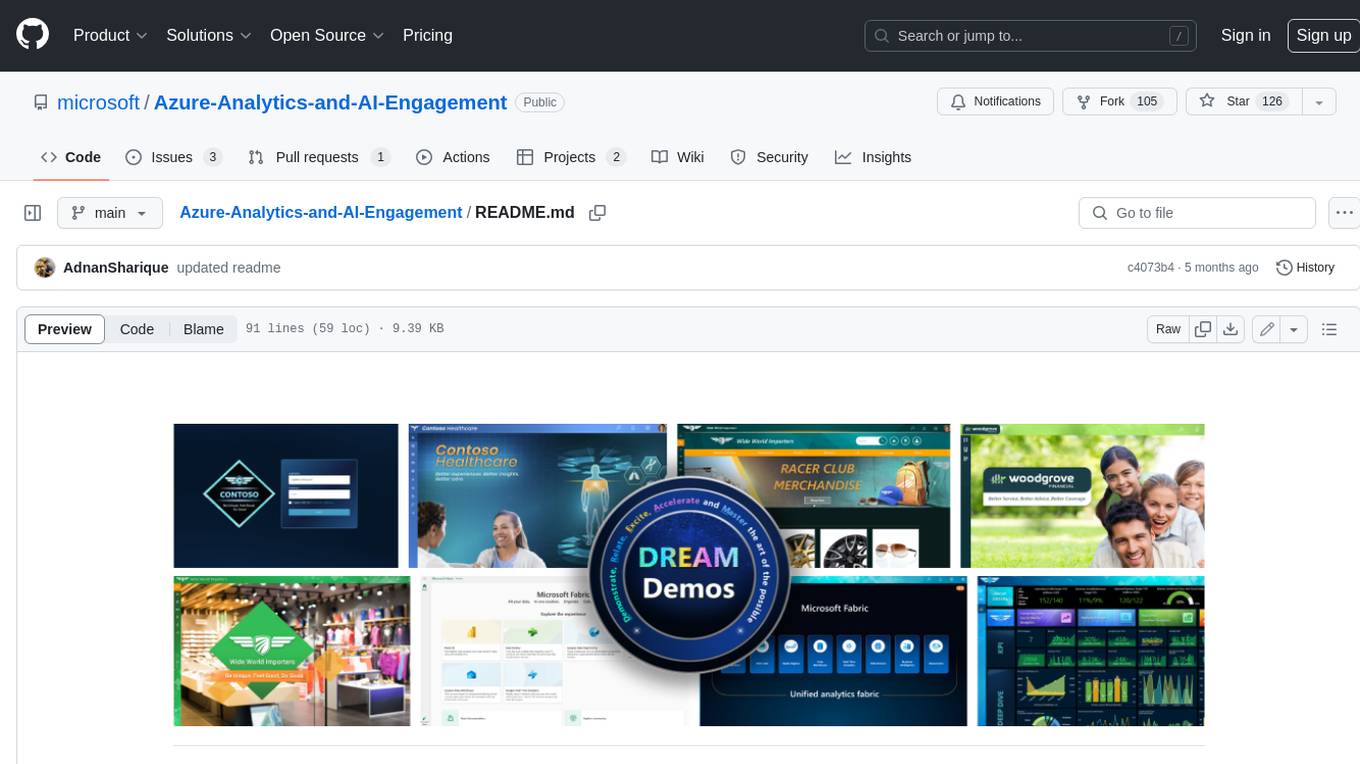
Azure-Analytics-and-AI-Engagement
The Azure-Analytics-and-AI-Engagement repository provides packaged Industry Scenario DREAM Demos with ARM templates (Containing a demo web application, Power BI reports, Synapse resources, AML Notebooks etc.) that can be deployed in a customer’s subscription using the CAPE tool within a matter of few hours. Partners can also deploy DREAM Demos in their own subscriptions using DPoC.