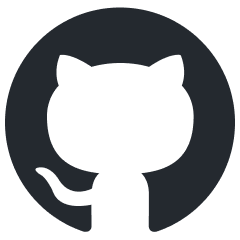
ColossalAI
Making large AI models cheaper, faster and more accessible
Stars: 38935
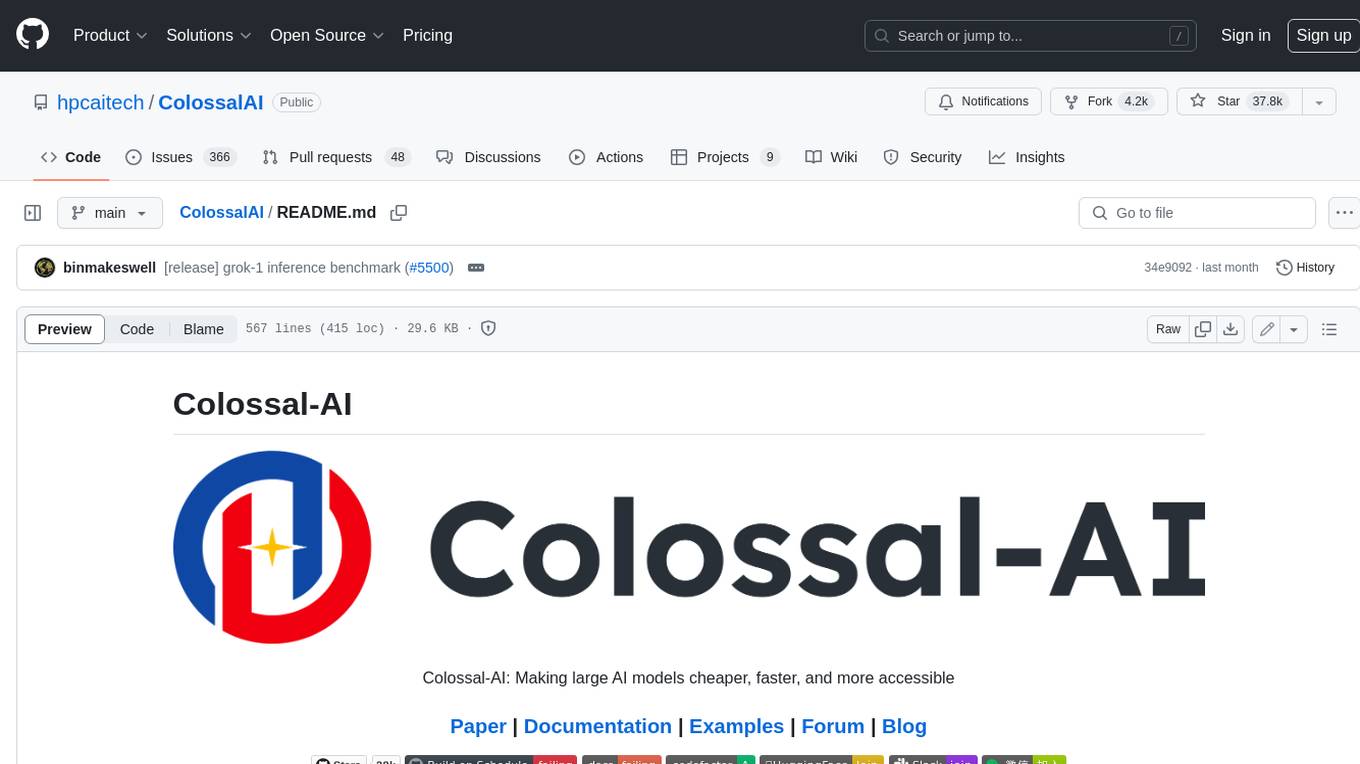
Colossal-AI is a deep learning system for large-scale parallel training. It provides a unified interface to scale sequential code of model training to distributed environments. Colossal-AI supports parallel training methods such as data, pipeline, tensor, and sequence parallelism and is integrated with heterogeneous training and zero redundancy optimizer.
README:
Colossal-AI: Making large AI models cheaper, faster, and more accessible
Paper | Documentation | Examples | Forum | GPU Cloud Playground | Blog
Access high-end, on-demand compute for your research instantly—no setup needed.
Sign up now and get $10 in credits!
Limited Academic Bonuses:
- Top up $1,000 and receive 300 credits
- Top up $500 and receive 100 credits
- [2024/12] The development cost of video generation models has saved by 50%! Open-source solutions are now available with H200 GPU vouchers [code] [vouchers]
- [2024/10] How to build a low-cost Sora-like app? Solutions for you
- [2024/09] Singapore Startup HPC-AI Tech Secures 50 Million USD in Series A Funding to Build the Video Generation AI Model and GPU Platform
- [2024/09] Reducing AI Large Model Training Costs by 30% Requires Just a Single Line of Code From FP8 Mixed Precision Training Upgrades
- [2024/06] Open-Sora Continues Open Source: Generate Any 16-Second 720p HD Video with One Click, Model Weights Ready to Use
- [2024/05] Large AI Models Inference Speed Doubled, Colossal-Inference Open Source Release
- [2024/04] Open-Sora Unveils Major Upgrade: Embracing Open Source with Single-Shot 16-Second Video Generation and 720p Resolution
- [2024/04] Most cost-effective solutions for inference, fine-tuning and pretraining, tailored to LLaMA3 series
- Why Colossal-AI
- Features
-
Colossal-AI for Real World Applications
- Open-Sora: Revealing Complete Model Parameters, Training Details, and Everything for Sora-like Video Generation Models
- Colossal-LLaMA-2: One Half-Day of Training Using a Few Hundred Dollars Yields Similar Results to Mainstream Large Models, Open-Source and Commercial-Free Domain-Specific Llm Solution
- ColossalChat: An Open-Source Solution for Cloning ChatGPT With a Complete RLHF Pipeline
- AIGC: Acceleration of Stable Diffusion
- Biomedicine: Acceleration of AlphaFold Protein Structure
- Parallel Training Demo
- Single GPU Training Demo
- Inference
- Installation
- Use Docker
- Community
- Contributing
- Cite Us
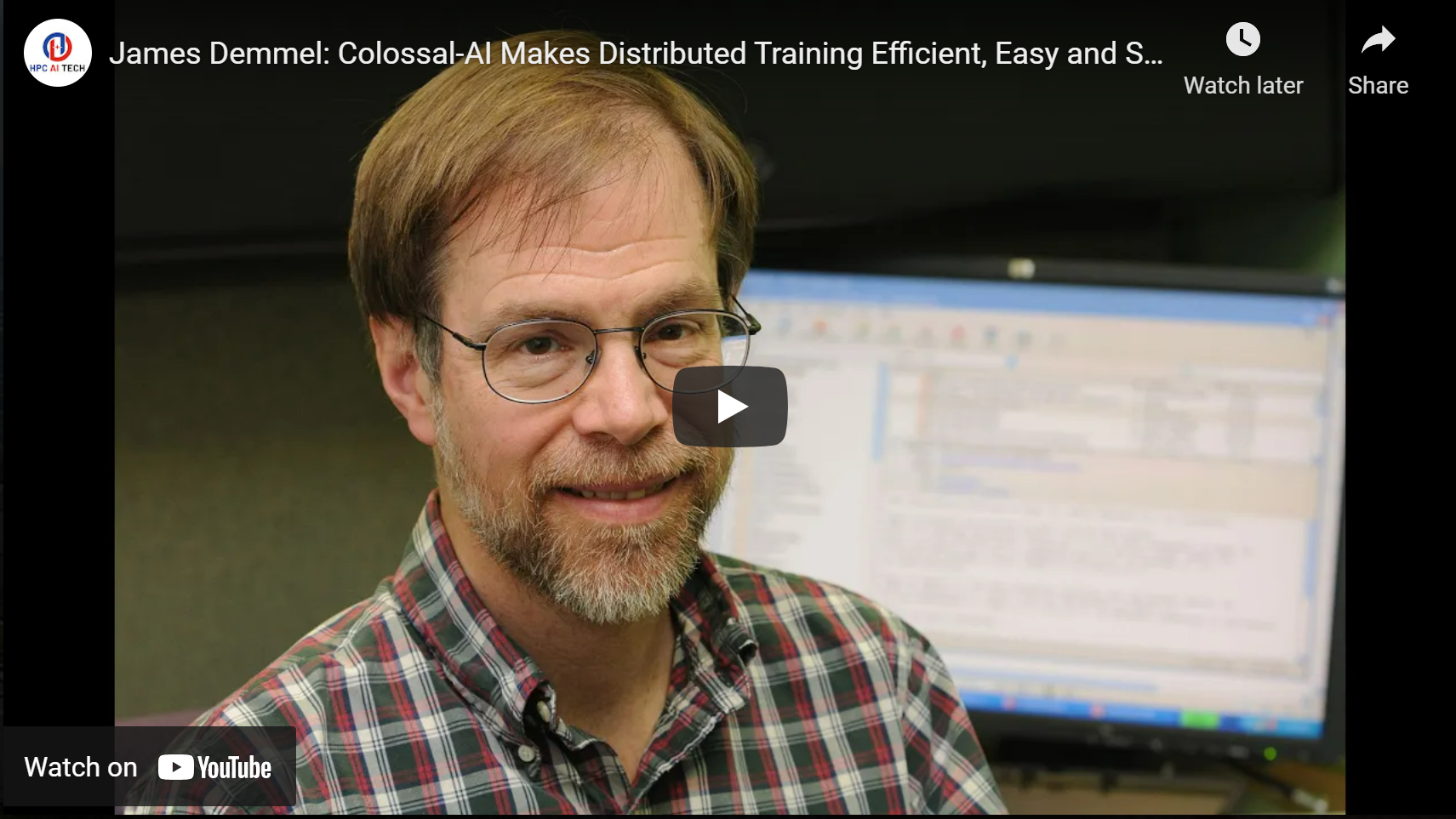
Prof. James Demmel (UC Berkeley): Colossal-AI makes training AI models efficient, easy, and scalable.
Colossal-AI provides a collection of parallel components for you. We aim to support you to write your distributed deep learning models just like how you write your model on your laptop. We provide user-friendly tools to kickstart distributed training and inference in a few lines.
-
Parallelism strategies
- Data Parallelism
- Pipeline Parallelism
- 1D, 2D, 2.5D, 3D Tensor Parallelism
- Sequence Parallelism
- Zero Redundancy Optimizer (ZeRO)
- Auto-Parallelism
-
Heterogeneous Memory Management
-
Friendly Usage
- Parallelism based on the configuration file
Open-Sora:Revealing Complete Model Parameters, Training Details, and Everything for Sora-like Video Generation Models [code] [blog] [Model weights] [Demo] [GPU Cloud Playground] [OpenSora Image]
[GPU Cloud Playground] [LLaMA3 Image]
-
7B: One half-day of training using a few hundred dollars yields similar results to mainstream large models, open-source and commercial-free domain-specific LLM solution. [code] [blog] [HuggingFace model weights] [Modelscope model weights]
-
13B: Construct refined 13B private model with just $5000 USD. [code] [blog] [HuggingFace model weights] [Modelscope model weights]
Model | Backbone | Tokens Consumed | MMLU (5-shot) | CMMLU (5-shot) | AGIEval (5-shot) | GAOKAO (0-shot) | CEval (5-shot) |
---|---|---|---|---|---|---|---|
Baichuan-7B | - | 1.2T | 42.32 (42.30) | 44.53 (44.02) | 38.72 | 36.74 | 42.80 |
Baichuan-13B-Base | - | 1.4T | 50.51 (51.60) | 55.73 (55.30) | 47.20 | 51.41 | 53.60 |
Baichuan2-7B-Base | - | 2.6T | 46.97 (54.16) | 57.67 (57.07) | 45.76 | 52.60 | 54.00 |
Baichuan2-13B-Base | - | 2.6T | 54.84 (59.17) | 62.62 (61.97) | 52.08 | 58.25 | 58.10 |
ChatGLM-6B | - | 1.0T | 39.67 (40.63) | 41.17 (-) | 40.10 | 36.53 | 38.90 |
ChatGLM2-6B | - | 1.4T | 44.74 (45.46) | 49.40 (-) | 46.36 | 45.49 | 51.70 |
InternLM-7B | - | 1.6T | 46.70 (51.00) | 52.00 (-) | 44.77 | 61.64 | 52.80 |
Qwen-7B | - | 2.2T | 54.29 (56.70) | 56.03 (58.80) | 52.47 | 56.42 | 59.60 |
Llama-2-7B | - | 2.0T | 44.47 (45.30) | 32.97 (-) | 32.60 | 25.46 | - |
Linly-AI/Chinese-LLaMA-2-7B-hf | Llama-2-7B | 1.0T | 37.43 | 29.92 | 32.00 | 27.57 | - |
wenge-research/yayi-7b-llama2 | Llama-2-7B | - | 38.56 | 31.52 | 30.99 | 25.95 | - |
ziqingyang/chinese-llama-2-7b | Llama-2-7B | - | 33.86 | 34.69 | 34.52 | 25.18 | 34.2 |
TigerResearch/tigerbot-7b-base | Llama-2-7B | 0.3T | 43.73 | 42.04 | 37.64 | 30.61 | - |
LinkSoul/Chinese-Llama-2-7b | Llama-2-7B | - | 48.41 | 38.31 | 38.45 | 27.72 | - |
FlagAlpha/Atom-7B | Llama-2-7B | 0.1T | 49.96 | 41.10 | 39.83 | 33.00 | - |
IDEA-CCNL/Ziya-LLaMA-13B-v1.1 | Llama-13B | 0.11T | 50.25 | 40.99 | 40.04 | 30.54 | - |
Colossal-LLaMA-2-7b-base | Llama-2-7B | 0.0085T | 53.06 | 49.89 | 51.48 | 58.82 | 50.2 |
Colossal-LLaMA-2-13b-base | Llama-2-13B | 0.025T | 56.42 | 61.80 | 54.69 | 69.53 | 60.3 |
ColossalChat: An open-source solution for cloning ChatGPT with a complete RLHF pipeline. [code] [blog] [demo] [tutorial]
- Up to 10 times faster for RLHF PPO Stage3 Training
- Up to 7.73 times faster for single server training and 1.42 times faster for single-GPU inference
- Up to 10.3x growth in model capacity on one GPU
- A mini demo training process requires only 1.62GB of GPU memory (any consumer-grade GPU)
- Increase the capacity of the fine-tuning model by up to 3.7 times on a single GPU
- Keep at a sufficiently high running speed
Acceleration of AIGC (AI-Generated Content) models such as Stable Diffusion v1 and Stable Diffusion v2.
- Training: Reduce Stable Diffusion memory consumption by up to 5.6x and hardware cost by up to 46x (from A100 to RTX3060).
- DreamBooth Fine-tuning: Personalize your model using just 3-5 images of the desired subject.
- Inference: Reduce inference GPU memory consumption by 2.5x.
Acceleration of AlphaFold Protein Structure
- FastFold: Accelerating training and inference on GPU Clusters, faster data processing, inference sequence containing more than 10000 residues.
- FastFold with Intel: 3x inference acceleration and 39% cost reduce.
- xTrimoMultimer: accelerating structure prediction of protein monomers and multimer by 11x.
- 70 billion parameter LLaMA3 model training accelerated by 18% [code] [GPU Cloud Playground] [LLaMA3 Image]
- Enhanced MoE parallelism, Open-source MoE model training can be 9 times more efficient [code] [blog]
- Save 50% GPU resources and 10.7% acceleration
- 11x lower GPU memory consumption, and superlinear scaling efficiency with Tensor Parallelism
- 24x larger model size on the same hardware
- over 3x acceleration
- 2x faster training, or 50% longer sequence length
- PaLM-colossalai: Scalable implementation of Google's Pathways Language Model (PaLM).
- Open Pretrained Transformer (OPT), a 175-Billion parameter AI language model released by Meta, which stimulates AI programmers to perform various downstream tasks and application deployments because of public pre-trained model weights.
- 45% speedup fine-tuning OPT at low cost in lines. [Example] [Online Serving]
Please visit our documentation and examples for more details.
- 14x larger batch size, and 5x faster training for Tensor Parallelism = 64
- Cached Embedding, utilize software cache to train larger embedding tables with a smaller GPU memory budget.
- 20x larger model size on the same hardware
- 120x larger model size on the same hardware (RTX 3080)
- 34x larger model size on the same hardware
- Large AI models inference speed doubled, compared to the offline inference performance of vLLM in some cases. [code] [blog] [GPU Cloud Playground] [LLaMA3 Image]
- 314 Billion Parameter Grok-1 Inference Accelerated by 3.8x, an easy-to-use Python + PyTorch + HuggingFace version for Inference.
[code] [blog] [HuggingFace Grok-1 PyTorch model weights] [ModelScope Grok-1 PyTorch model weights]
- SwiftInfer: Inference performance improved by 46%, open source solution breaks the length limit of LLM for multi-round conversations
Requirements:
- PyTorch >= 2.2
- Python >= 3.7
- CUDA >= 11.0
- NVIDIA GPU Compute Capability >= 7.0 (V100/RTX20 and higher)
- Linux OS
If you encounter any problem with installation, you may want to raise an issue in this repository.
You can easily install Colossal-AI with the following command. By default, we do not build PyTorch extensions during installation.
pip install colossalai
Note: only Linux is supported for now.
However, if you want to build the PyTorch extensions during installation, you can set BUILD_EXT=1
.
BUILD_EXT=1 pip install colossalai
Otherwise, CUDA kernels will be built during runtime when you actually need them.
We also keep releasing the nightly version to PyPI every week. This allows you to access the unreleased features and bug fixes in the main branch. Installation can be made via
pip install colossalai-nightly
The version of Colossal-AI will be in line with the main branch of the repository. Feel free to raise an issue if you encounter any problems. :)
git clone https://github.com/hpcaitech/ColossalAI.git
cd ColossalAI
# install colossalai
pip install .
By default, we do not compile CUDA/C++ kernels. ColossalAI will build them during runtime. If you want to install and enable CUDA kernel fusion (compulsory installation when using fused optimizer):
BUILD_EXT=1 pip install .
For Users with CUDA 10.2, you can still build ColossalAI from source. However, you need to manually download the cub library and copy it to the corresponding directory.
# clone the repository
git clone https://github.com/hpcaitech/ColossalAI.git
cd ColossalAI
# download the cub library
wget https://github.com/NVIDIA/cub/archive/refs/tags/1.8.0.zip
unzip 1.8.0.zip
cp -r cub-1.8.0/cub/ colossalai/kernel/cuda_native/csrc/kernels/include/
# install
BUILD_EXT=1 pip install .
You can directly pull the docker image from our DockerHub page. The image is automatically uploaded upon release.
Run the following command to build a docker image from Dockerfile provided.
Building Colossal-AI from scratch requires GPU support, you need to use Nvidia Docker Runtime as the default when doing
docker build
. More details can be found here. We recommend you install Colossal-AI from our project page directly.
cd ColossalAI
docker build -t colossalai ./docker
Run the following command to start the docker container in interactive mode.
docker run -ti --gpus all --rm --ipc=host colossalai bash
Join the Colossal-AI community on Forum, Slack, and WeChat(微信) to share your suggestions, feedback, and questions with our engineering team.
Referring to the successful attempts of BLOOM and Stable Diffusion, any and all developers and partners with computing powers, datasets, models are welcome to join and build the Colossal-AI community, making efforts towards the era of big AI models!
You may contact us or participate in the following ways:
- Leaving a Star ⭐ to show your like and support. Thanks!
- Posting an issue, or submitting a PR on GitHub follow the guideline in Contributing
- Send your official proposal to email [email protected]
Thanks so much to all of our amazing contributors!
We leverage the power of GitHub Actions to automate our development, release and deployment workflows. Please check out this documentation on how the automated workflows are operated.
This project is inspired by some related projects (some by our team and some by other organizations). We would like to credit these amazing projects as listed in the Reference List.
To cite this project, you can use the following BibTeX citation.
@inproceedings{10.1145/3605573.3605613,
author = {Li, Shenggui and Liu, Hongxin and Bian, Zhengda and Fang, Jiarui and Huang, Haichen and Liu, Yuliang and Wang, Boxiang and You, Yang},
title = {Colossal-AI: A Unified Deep Learning System For Large-Scale Parallel Training},
year = {2023},
isbn = {9798400708435},
publisher = {Association for Computing Machinery},
address = {New York, NY, USA},
url = {https://doi.org/10.1145/3605573.3605613},
doi = {10.1145/3605573.3605613},
abstract = {The success of Transformer models has pushed the deep learning model scale to billions of parameters, but the memory limitation of a single GPU has led to an urgent need for training on multi-GPU clusters. However, the best practice for choosing the optimal parallel strategy is still lacking, as it requires domain expertise in both deep learning and parallel computing. The Colossal-AI system addressed the above challenge by introducing a unified interface to scale your sequential code of model training to distributed environments. It supports parallel training methods such as data, pipeline, tensor, and sequence parallelism and is integrated with heterogeneous training and zero redundancy optimizer. Compared to the baseline system, Colossal-AI can achieve up to 2.76 times training speedup on large-scale models.},
booktitle = {Proceedings of the 52nd International Conference on Parallel Processing},
pages = {766–775},
numpages = {10},
keywords = {datasets, gaze detection, text tagging, neural networks},
location = {Salt Lake City, UT, USA},
series = {ICPP '23}
}
Colossal-AI has been accepted as official tutorial by top conferences NeurIPS, SC, AAAI, PPoPP, CVPR, ISC, NVIDIA GTC ,etc.
For Tasks:
Click tags to check more tools for each tasksFor Jobs:
Alternative AI tools for ColossalAI
Similar Open Source Tools
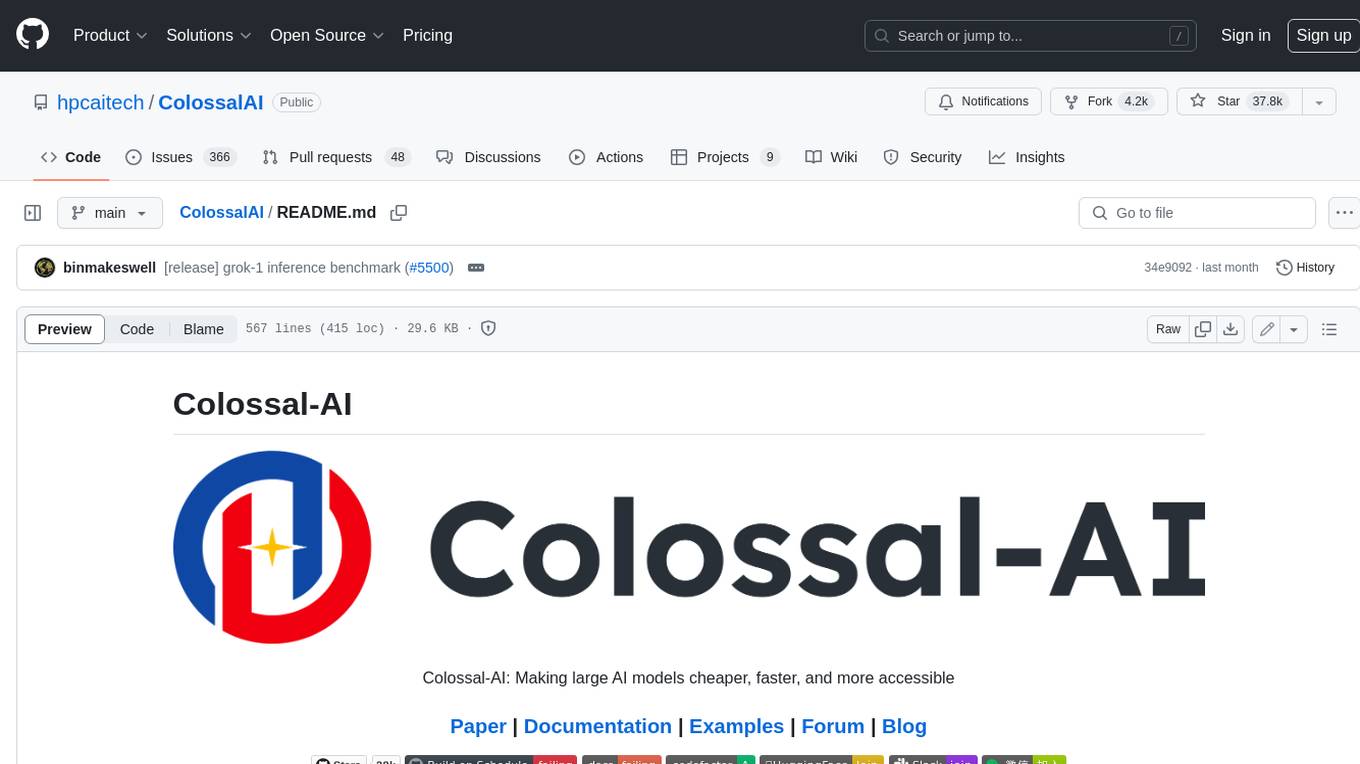
ColossalAI
Colossal-AI is a deep learning system for large-scale parallel training. It provides a unified interface to scale sequential code of model training to distributed environments. Colossal-AI supports parallel training methods such as data, pipeline, tensor, and sequence parallelism and is integrated with heterogeneous training and zero redundancy optimizer.
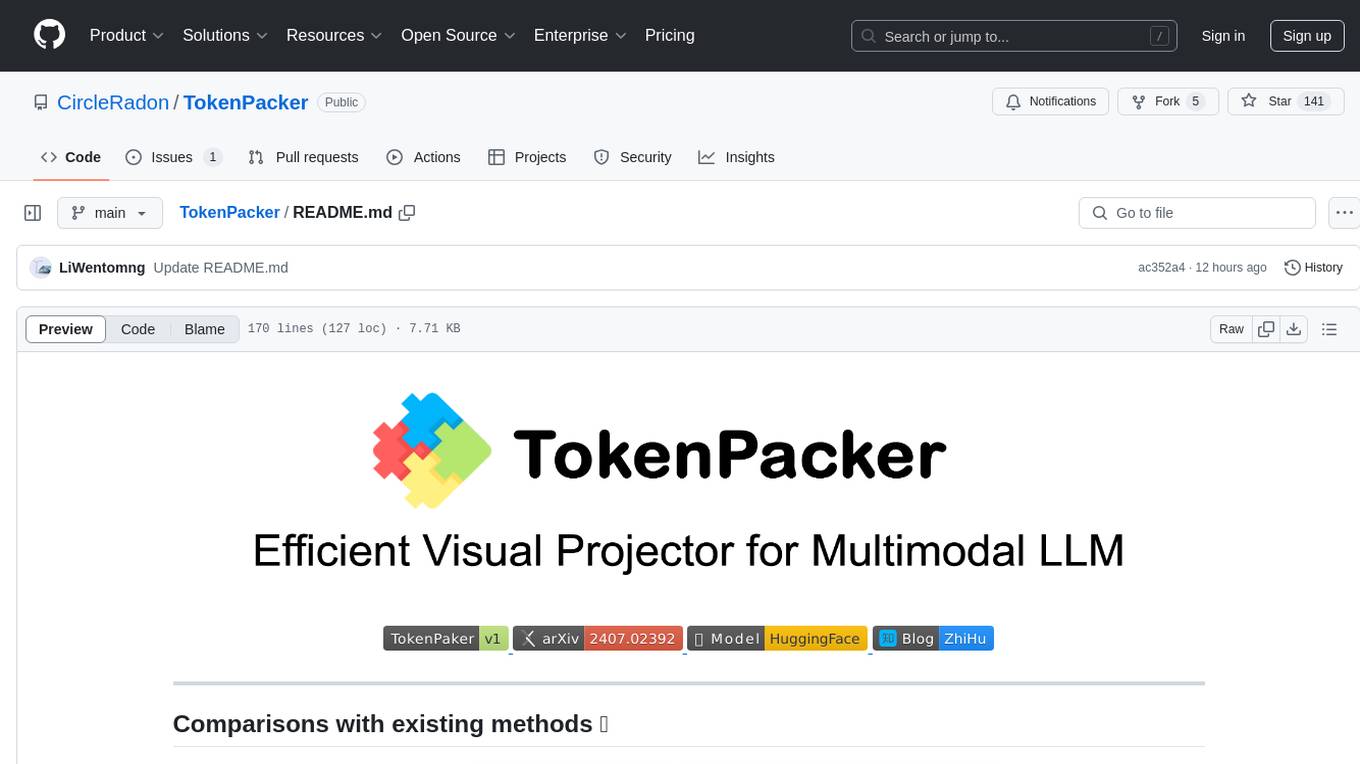
TokenPacker
TokenPacker is a novel visual projector that compresses visual tokens by 75%∼89% with high efficiency. It adopts a 'coarse-to-fine' scheme to generate condensed visual tokens, achieving comparable or better performance across diverse benchmarks. The tool includes TokenPacker for general use and TokenPacker-HD for high-resolution image understanding. It provides training scripts, checkpoints, and supports various compression ratios and patch numbers.
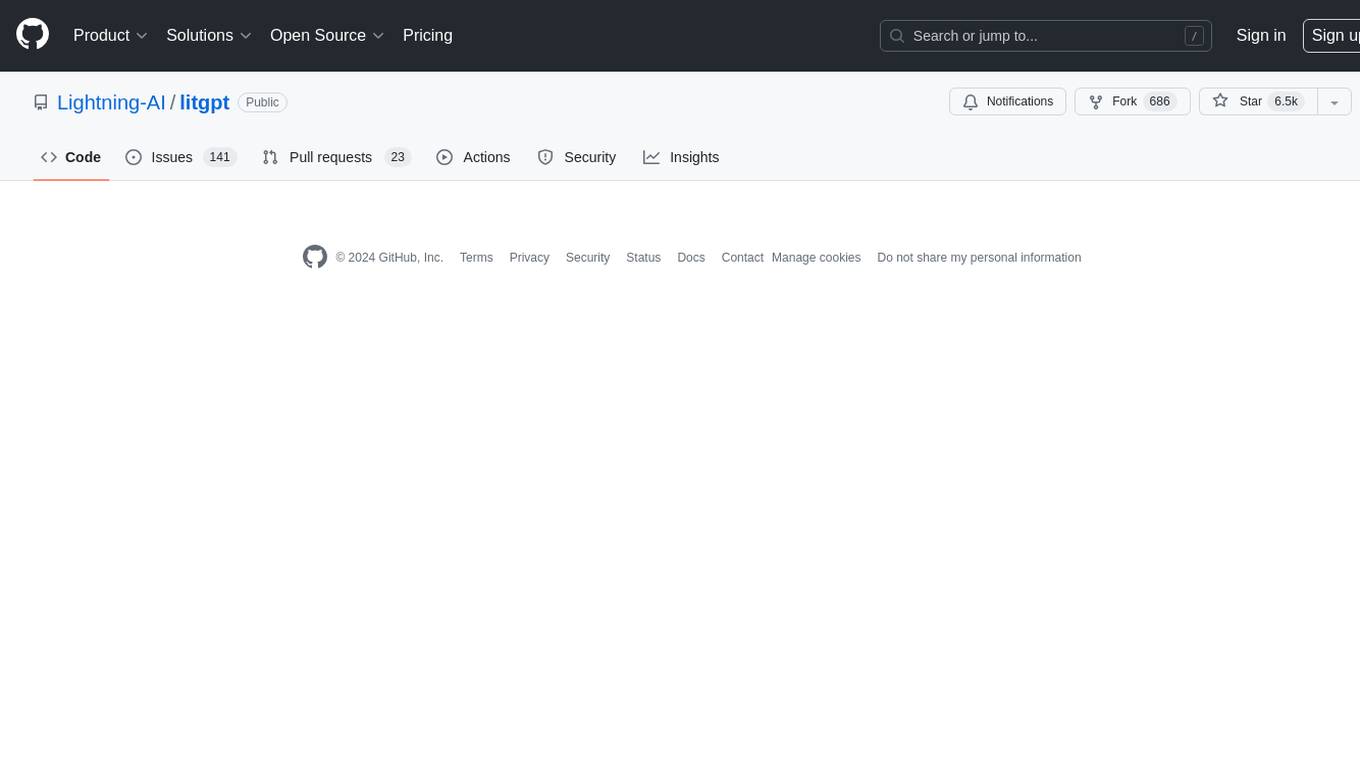
litgpt
LitGPT is a command-line tool designed to easily finetune, pretrain, evaluate, and deploy 20+ LLMs **on your own data**. It features highly-optimized training recipes for the world's most powerful open-source large-language-models (LLMs).
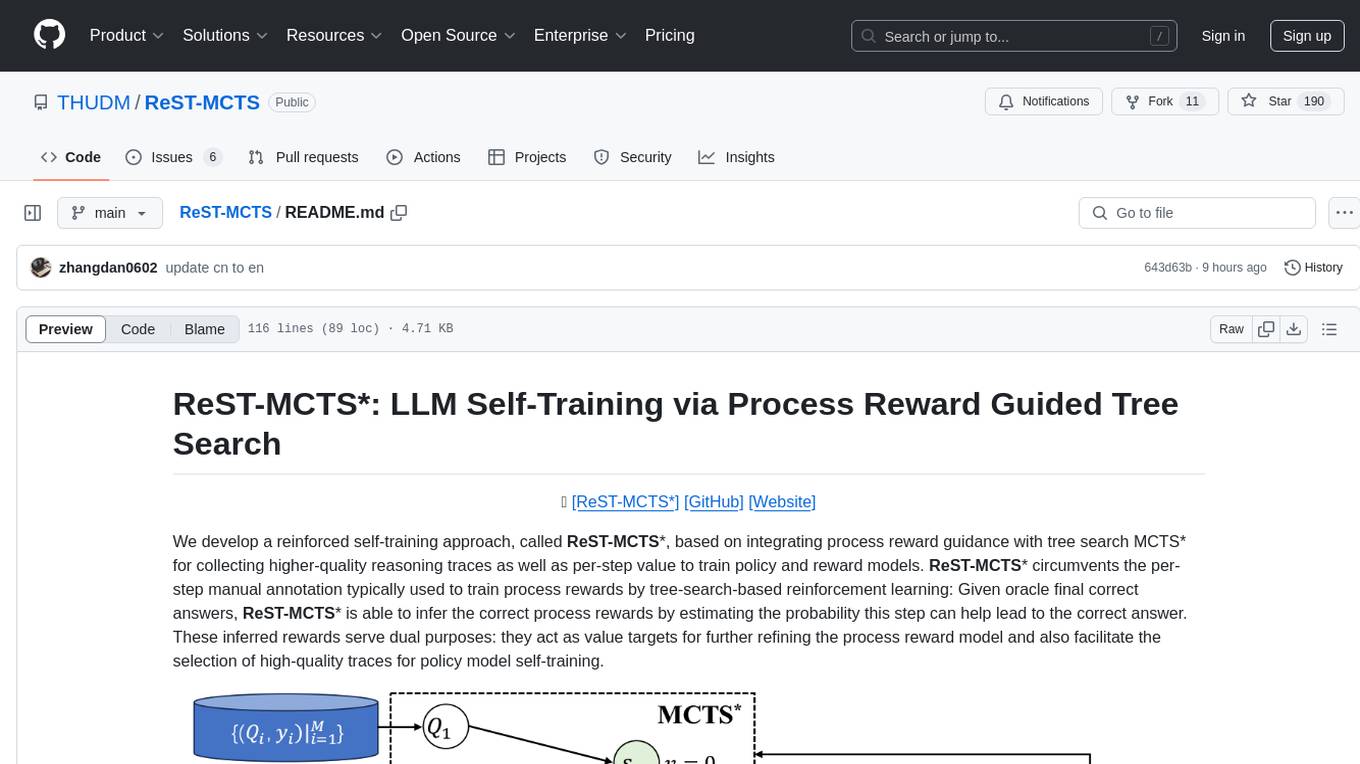
ReST-MCTS
ReST-MCTS is a reinforced self-training approach that integrates process reward guidance with tree search MCTS to collect higher-quality reasoning traces and per-step value for training policy and reward models. It eliminates the need for manual per-step annotation by estimating the probability of steps leading to correct answers. The inferred rewards refine the process reward model and aid in selecting high-quality traces for policy model self-training.
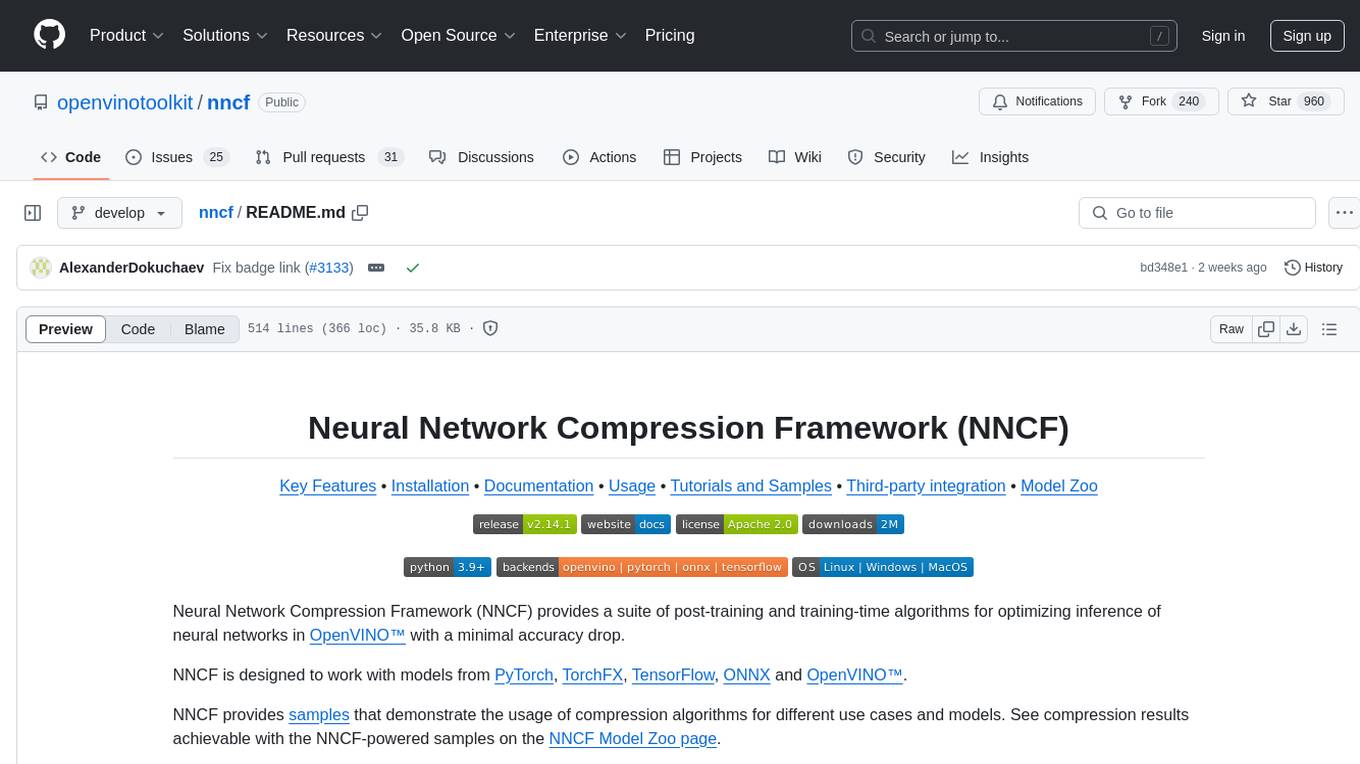
nncf
Neural Network Compression Framework (NNCF) provides a suite of post-training and training-time algorithms for optimizing inference of neural networks in OpenVINO™ with a minimal accuracy drop. It is designed to work with models from PyTorch, TorchFX, TensorFlow, ONNX, and OpenVINO™. NNCF offers samples demonstrating compression algorithms for various use cases and models, with the ability to add different compression algorithms easily. It supports GPU-accelerated layers, distributed training, and seamless combination of pruning, sparsity, and quantization algorithms. NNCF allows exporting compressed models to ONNX or TensorFlow formats for use with OpenVINO™ toolkit, and supports Accuracy-Aware model training pipelines via Adaptive Compression Level Training and Early Exit Training.
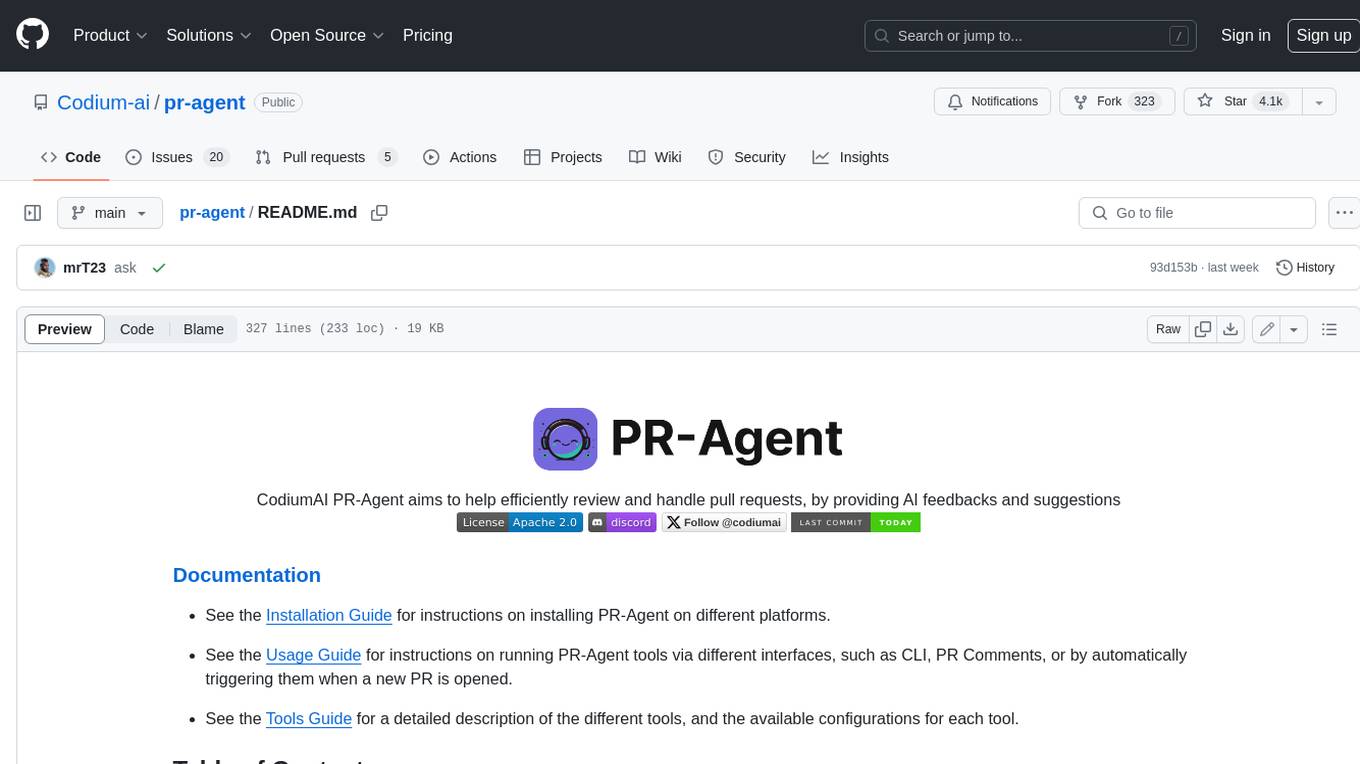
pr-agent
PR-Agent is a tool that helps to efficiently review and handle pull requests by providing AI feedbacks and suggestions. It supports various commands such as generating PR descriptions, providing code suggestions, answering questions about the PR, and updating the CHANGELOG.md file. PR-Agent can be used via CLI, GitHub Action, GitHub App, Docker, and supports multiple git providers and models. It emphasizes real-life practical usage, with each tool having a single GPT-4 call for quick and affordable responses. The PR Compression strategy enables effective handling of both short and long PRs, while the JSON prompting strategy allows for modular and customizable tools. PR-Agent Pro, the hosted version by CodiumAI, provides additional benefits such as full management, improved privacy, priority support, and extra features.
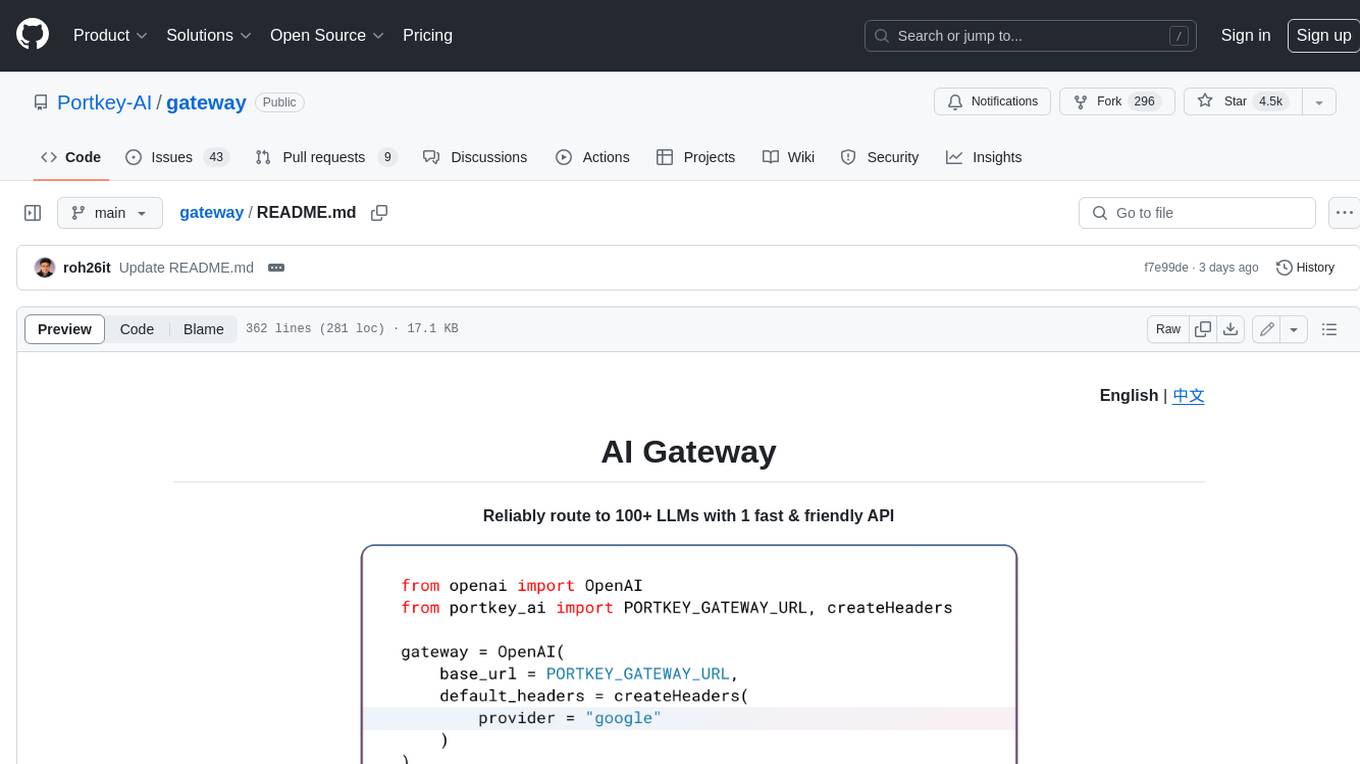
gateway
Gateway is a tool that streamlines requests to 100+ open & closed source models with a unified API. It is production-ready with support for caching, fallbacks, retries, timeouts, load balancing, and can be edge-deployed for minimum latency. It is blazing fast with a tiny footprint, supports load balancing across multiple models, providers, and keys, ensures app resilience with fallbacks, offers automatic retries with exponential fallbacks, allows configurable request timeouts, supports multimodal routing, and can be extended with plug-in middleware. It is battle-tested over 300B tokens and enterprise-ready for enhanced security, scale, and custom deployments.
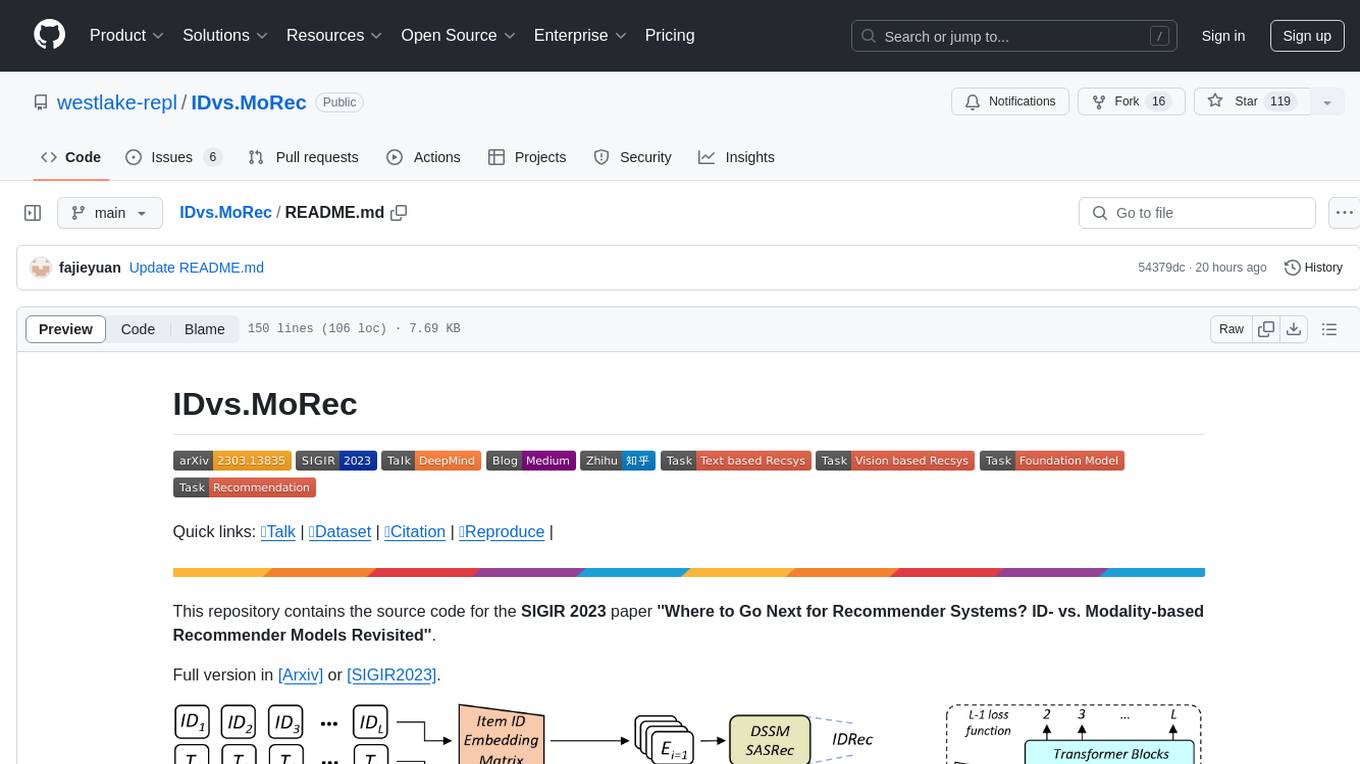
IDvs.MoRec
This repository contains the source code for the SIGIR 2023 paper 'Where to Go Next for Recommender Systems? ID- vs. Modality-based Recommender Models Revisited'. It provides resources for evaluating foundation, transferable, multi-modal, and LLM recommendation models, along with datasets, pre-trained models, and training strategies for IDRec and MoRec using in-batch debiased cross-entropy loss. The repository also offers large-scale datasets, code for SASRec with in-batch debias cross-entropy loss, and information on joining the lab for research opportunities.
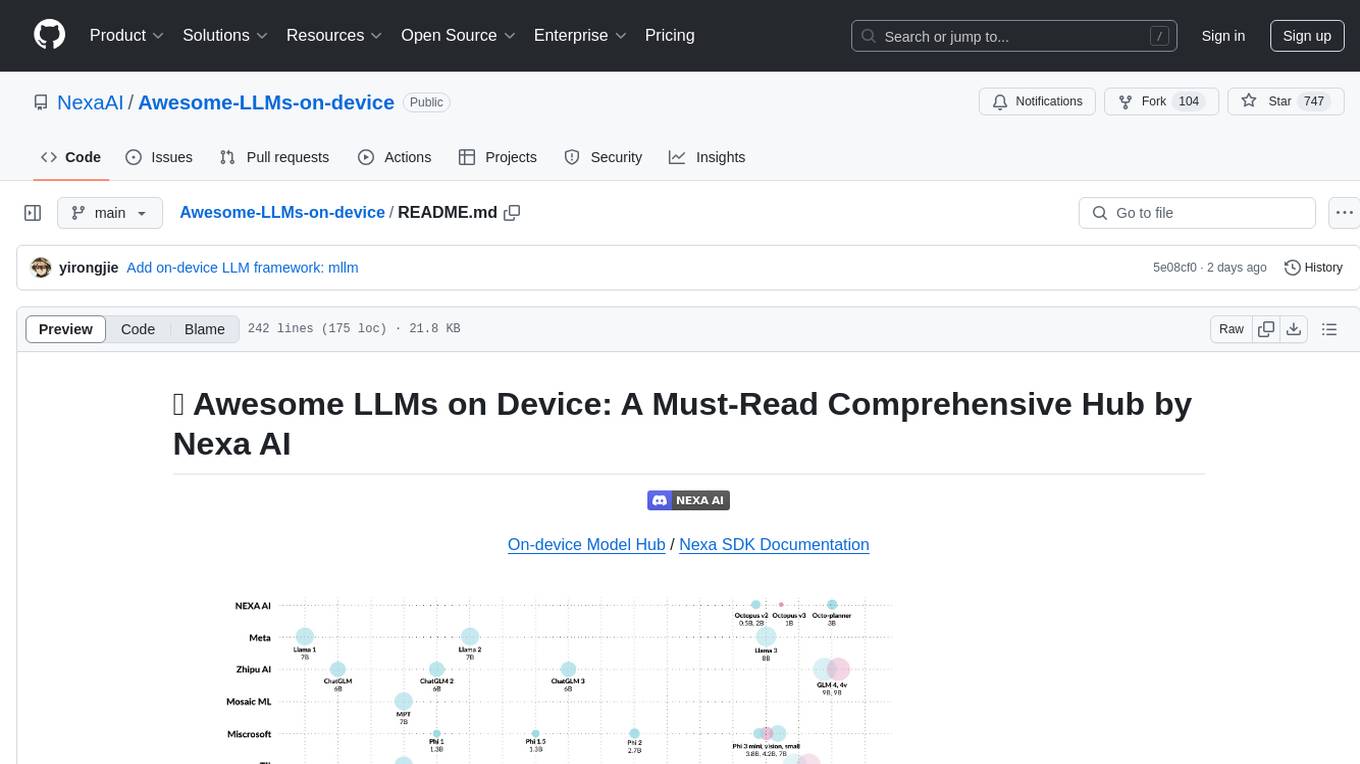
Awesome-LLMs-on-device
Welcome to the ultimate hub for on-device Large Language Models (LLMs)! This repository is your go-to resource for all things related to LLMs designed for on-device deployment. Whether you're a seasoned researcher, an innovative developer, or an enthusiastic learner, this comprehensive collection of cutting-edge knowledge is your gateway to understanding, leveraging, and contributing to the exciting world of on-device LLMs.
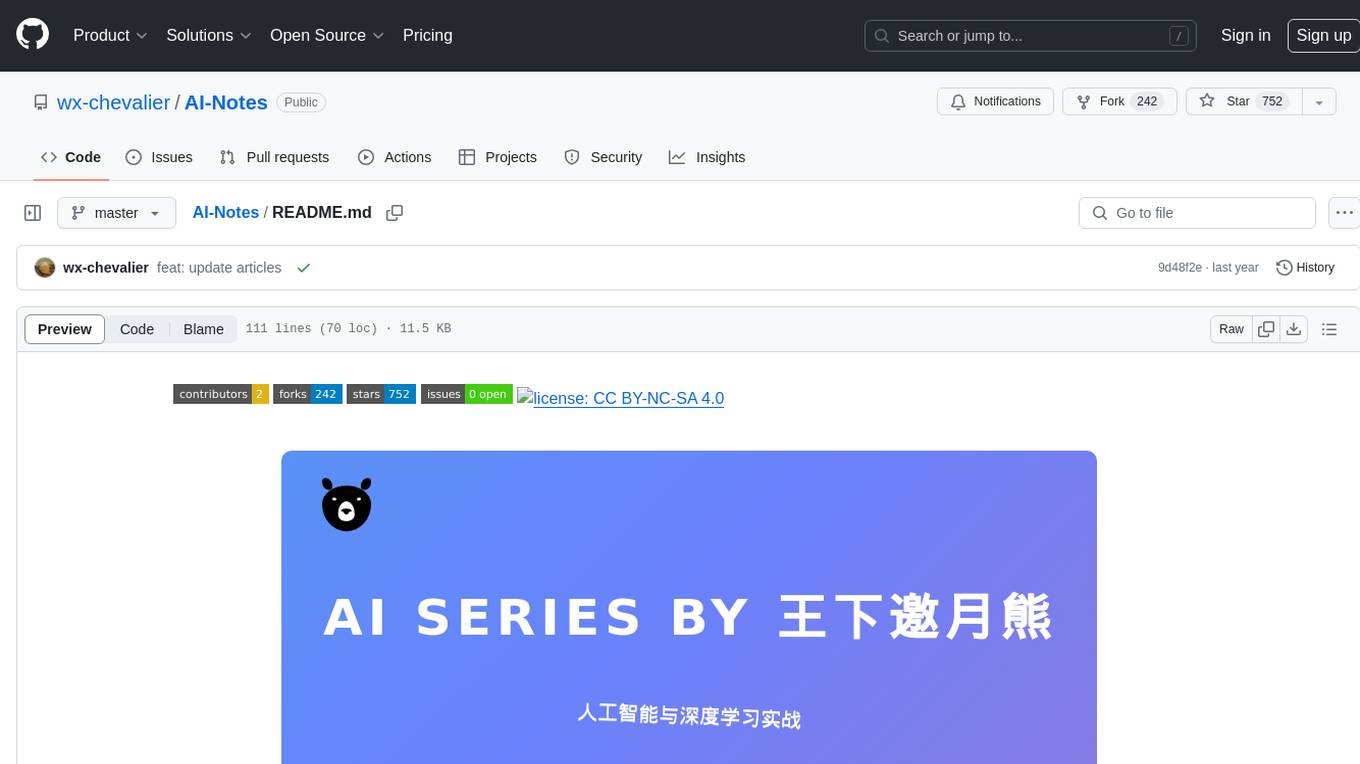
AI-Notes
AI-Notes is a repository dedicated to practical applications of artificial intelligence and deep learning. It covers concepts such as data mining, machine learning, natural language processing, and AI. The repository contains Jupyter Notebook examples for hands-on learning and experimentation. It explores the development stages of AI, from narrow artificial intelligence to general artificial intelligence and superintelligence. The content delves into machine learning algorithms, deep learning techniques, and the impact of AI on various industries like autonomous driving and healthcare. The repository aims to provide a comprehensive understanding of AI technologies and their real-world applications.
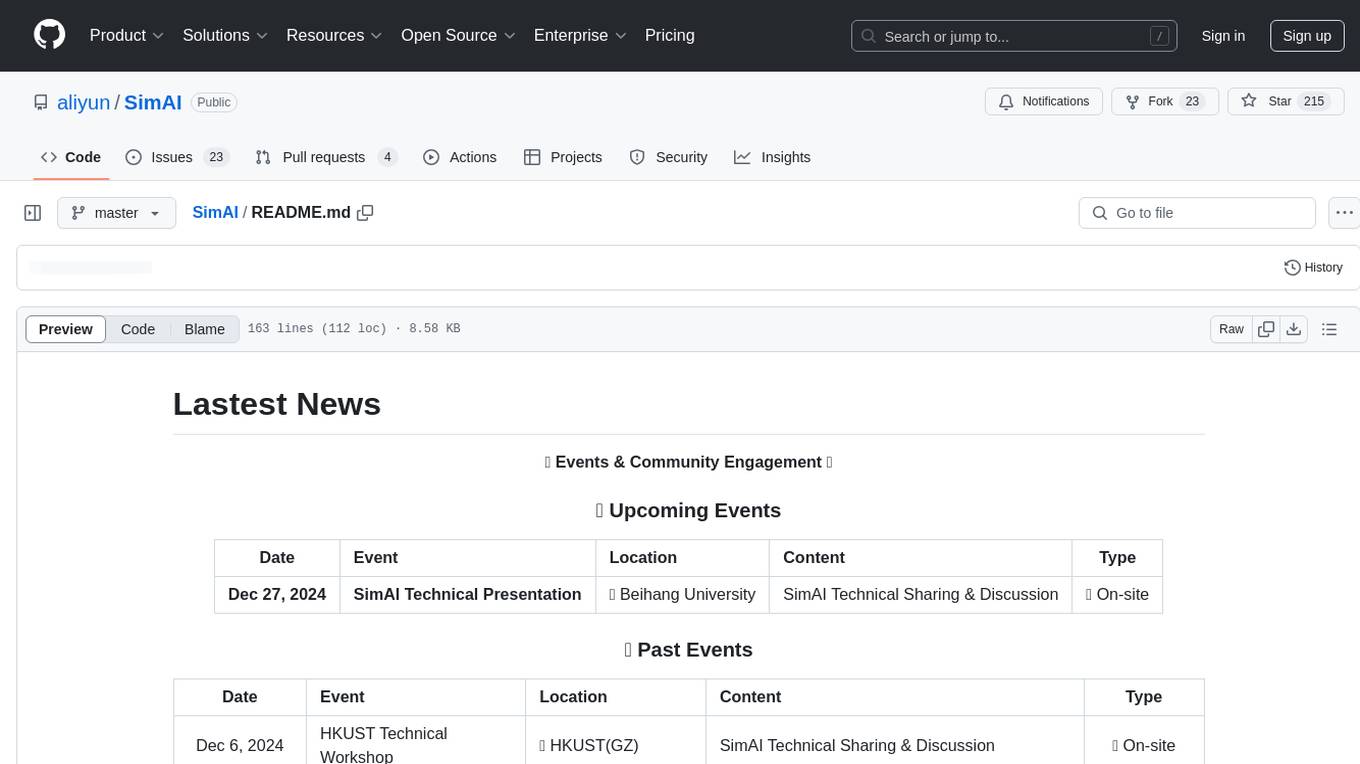
SimAI
SimAI is the industry's first full-stack, high-precision simulator for AI large-scale training. It provides detailed modeling and simulation of the entire LLM training process, encompassing framework, collective communication, network layers, and more. This comprehensive approach offers end-to-end performance data, enabling researchers to analyze training process details, evaluate time consumption of AI tasks under specific conditions, and assess performance gains from various algorithmic optimizations.
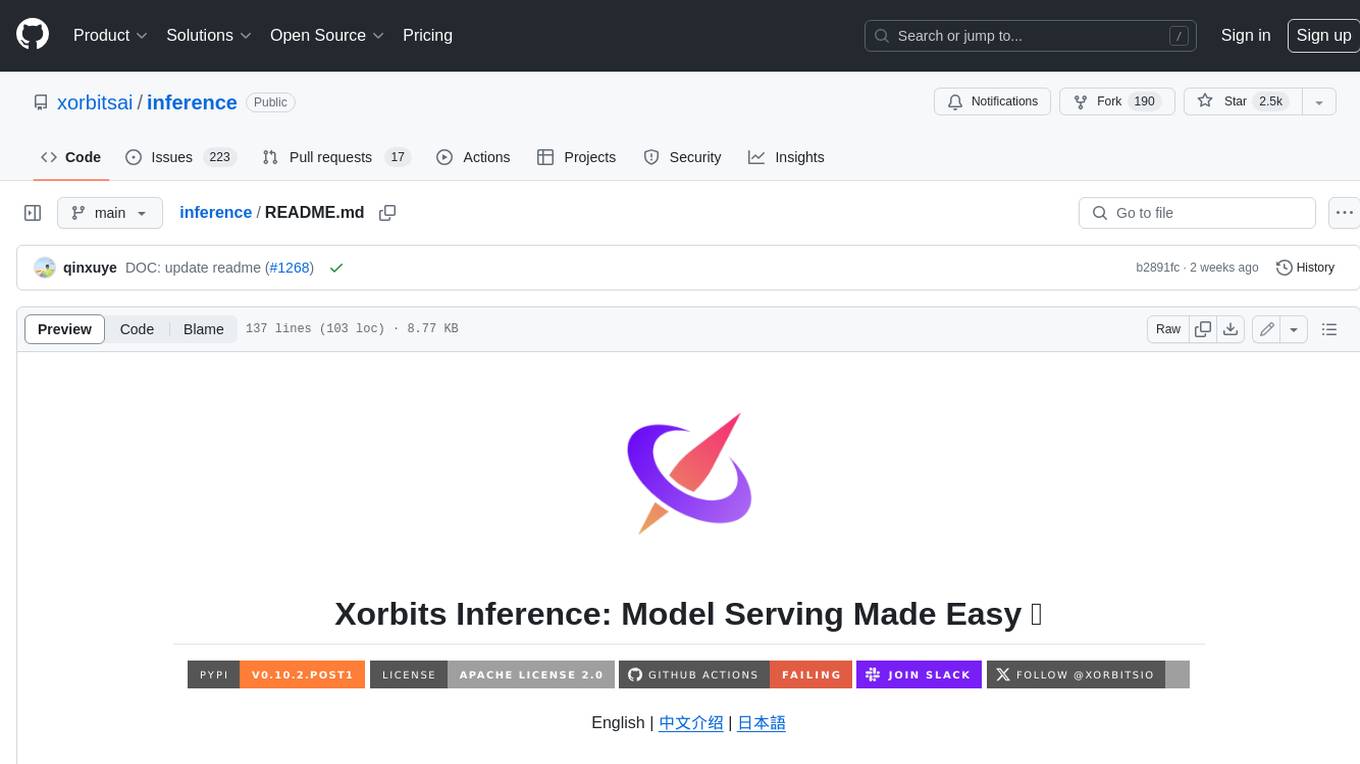
inference
Xorbits Inference (Xinference) is a powerful and versatile library designed to serve language, speech recognition, and multimodal models. With Xorbits Inference, you can effortlessly deploy and serve your or state-of-the-art built-in models using just a single command. Whether you are a researcher, developer, or data scientist, Xorbits Inference empowers you to unleash the full potential of cutting-edge AI models.
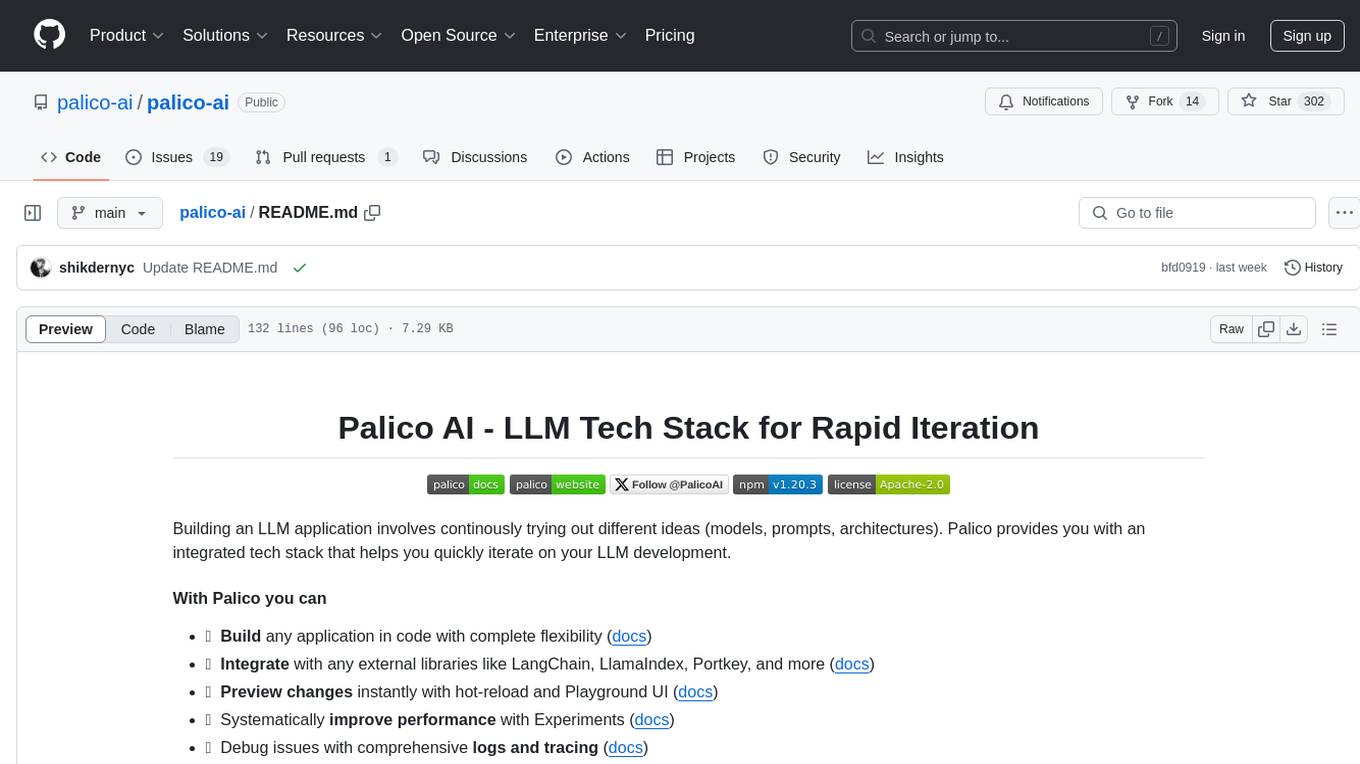
palico-ai
Palico AI is a tech stack designed for rapid iteration of LLM applications. It allows users to preview changes instantly, improve performance through experiments, debug issues with logs and tracing, deploy applications behind a REST API, and manage applications with a UI control panel. Users have complete flexibility in building their applications with Palico, integrating with various tools and libraries. The tool enables users to swap models, prompts, and logic easily using AppConfig. It also facilitates performance improvement through experiments and provides options for deploying applications to cloud providers or using managed hosting. Contributions to the project are welcomed, with easy ways to get involved by picking issues labeled as 'good first issue'.
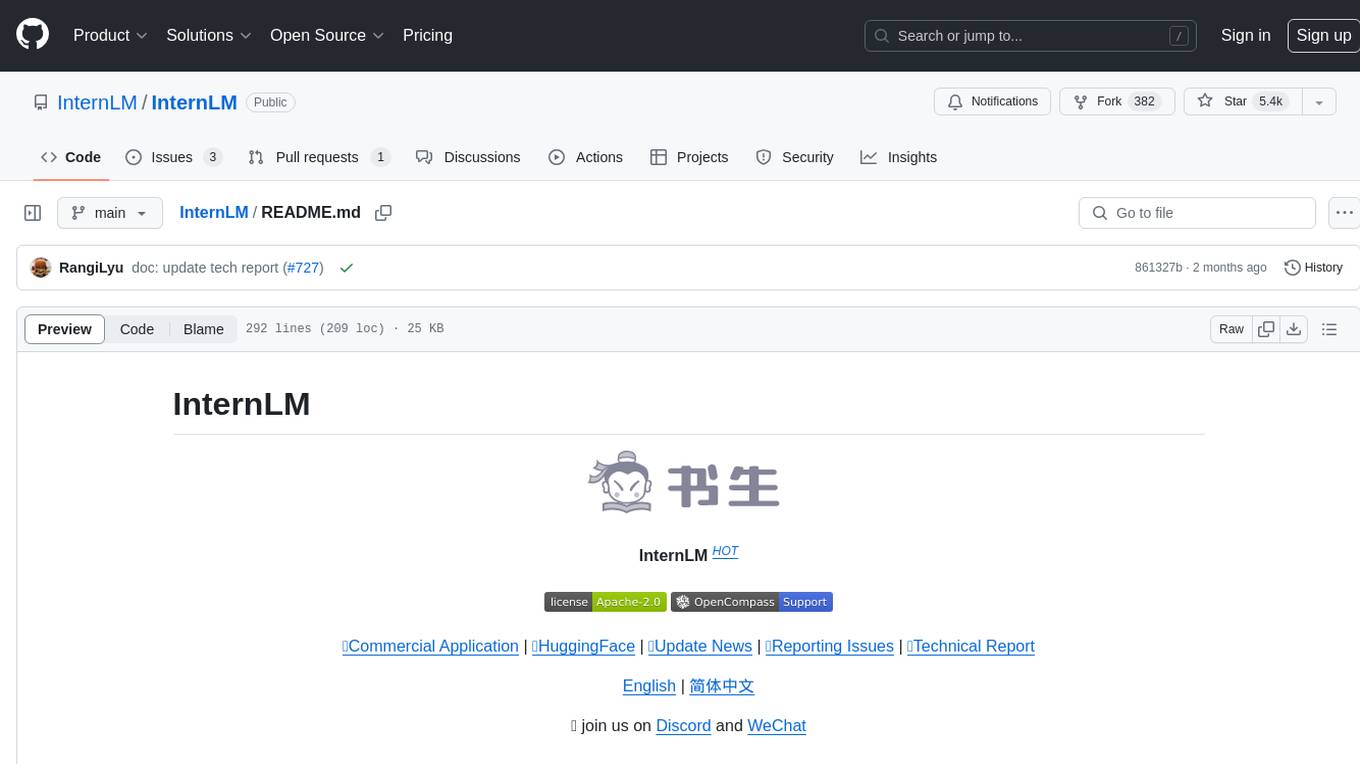
InternLM
InternLM is a powerful language model series with features such as 200K context window for long-context tasks, outstanding comprehensive performance in reasoning, math, code, chat experience, instruction following, and creative writing, code interpreter & data analysis capabilities, and stronger tool utilization capabilities. It offers models in sizes of 7B and 20B, suitable for research and complex scenarios. The models are recommended for various applications and exhibit better performance than previous generations. InternLM models may match or surpass other open-source models like ChatGPT. The tool has been evaluated on various datasets and has shown superior performance in multiple tasks. It requires Python >= 3.8, PyTorch >= 1.12.0, and Transformers >= 4.34 for usage. InternLM can be used for tasks like chat, agent applications, fine-tuning, deployment, and long-context inference.
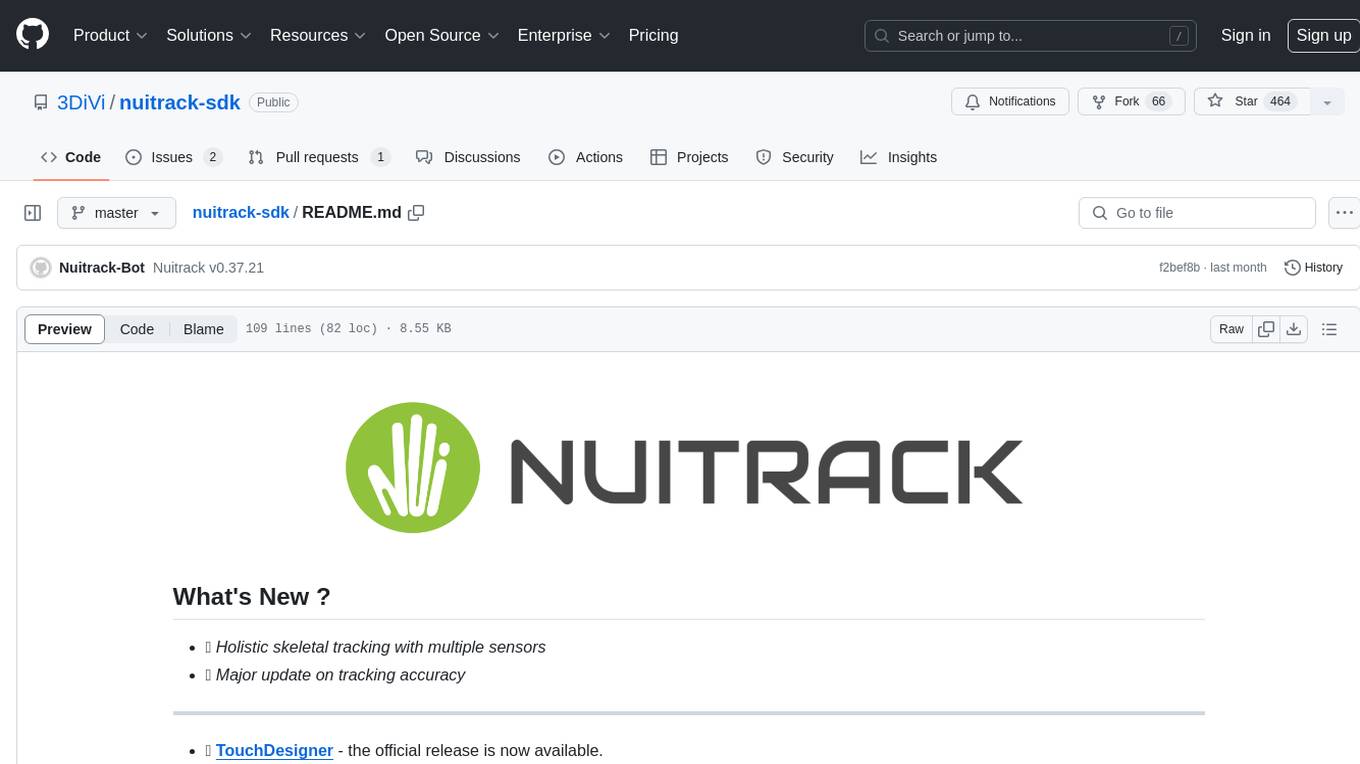
nuitrack-sdk
Nuitrack™ is an ultimate 3D body tracking solution developed by 3DiVi Inc. It enables body motion analytics applications for virtually any widespread depth sensors and hardware platforms, supporting a wide range of applications from real-time gesture recognition on embedded platforms to large-scale multisensor analytical systems. Nuitrack provides highly-sophisticated 3D skeletal tracking, basic facial analysis, hand tracking, and gesture recognition APIs for UI control. It offers two skeletal tracking engines: classical for embedded hardware and AI for complex poses, providing a human-centric spatial understanding tool for natural and intelligent user engagement.
For similar tasks
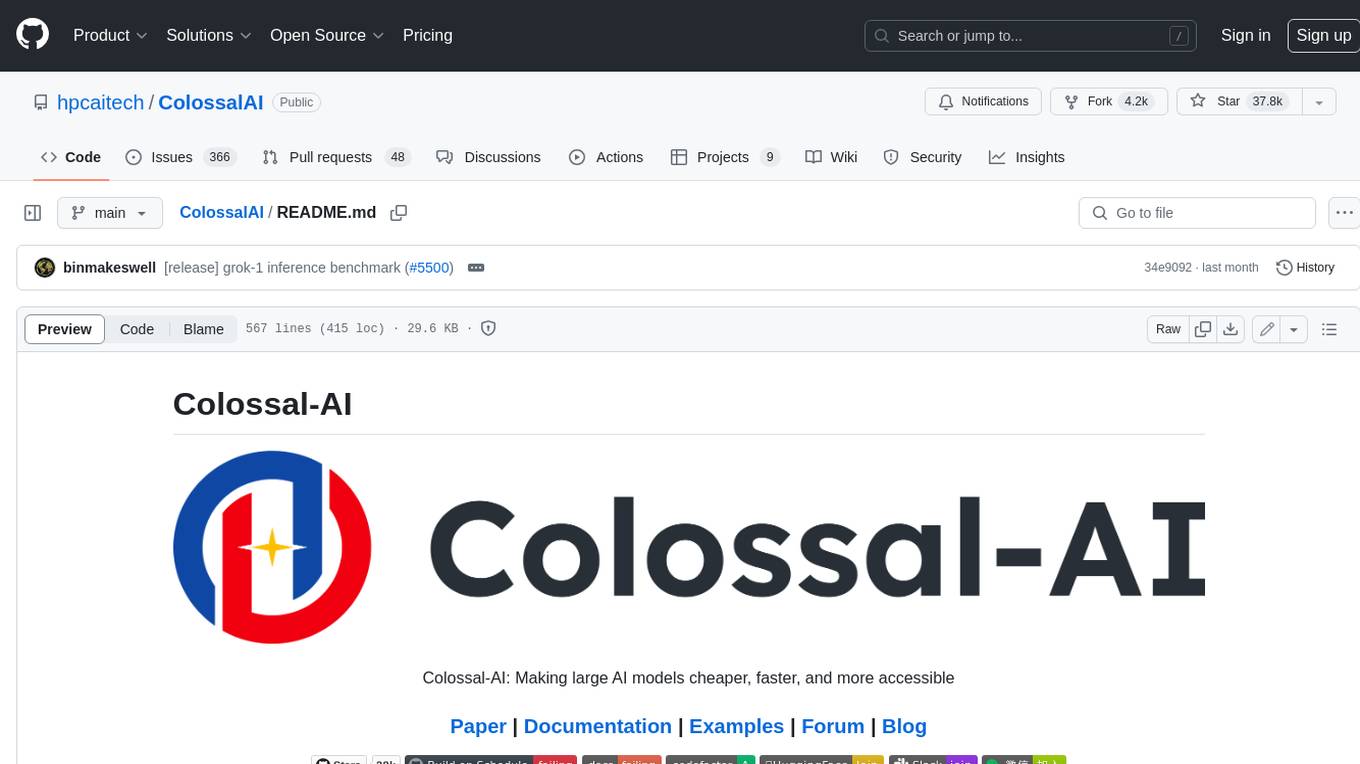
ColossalAI
Colossal-AI is a deep learning system for large-scale parallel training. It provides a unified interface to scale sequential code of model training to distributed environments. Colossal-AI supports parallel training methods such as data, pipeline, tensor, and sequence parallelism and is integrated with heterogeneous training and zero redundancy optimizer.
For similar jobs
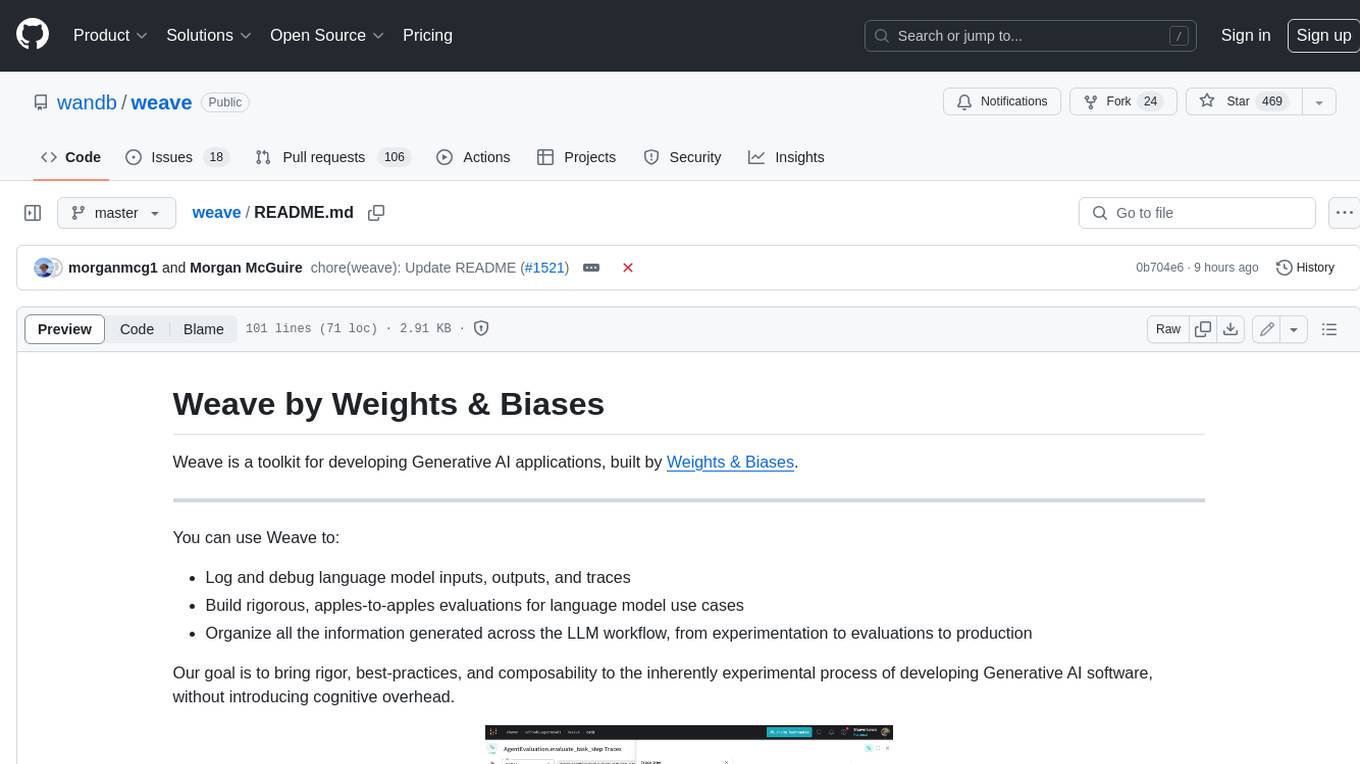
weave
Weave is a toolkit for developing Generative AI applications, built by Weights & Biases. With Weave, you can log and debug language model inputs, outputs, and traces; build rigorous, apples-to-apples evaluations for language model use cases; and organize all the information generated across the LLM workflow, from experimentation to evaluations to production. Weave aims to bring rigor, best-practices, and composability to the inherently experimental process of developing Generative AI software, without introducing cognitive overhead.
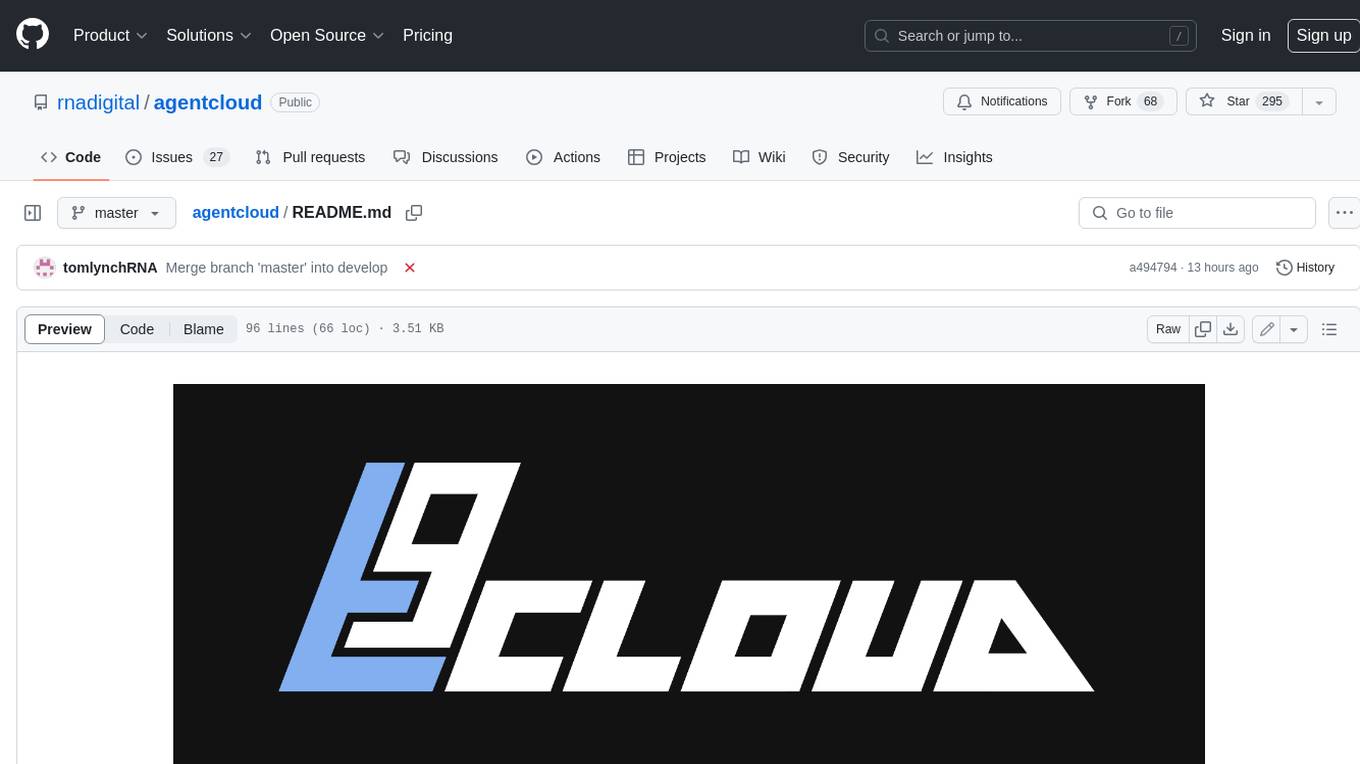
agentcloud
AgentCloud is an open-source platform that enables companies to build and deploy private LLM chat apps, empowering teams to securely interact with their data. It comprises three main components: Agent Backend, Webapp, and Vector Proxy. To run this project locally, clone the repository, install Docker, and start the services. The project is licensed under the GNU Affero General Public License, version 3 only. Contributions and feedback are welcome from the community.
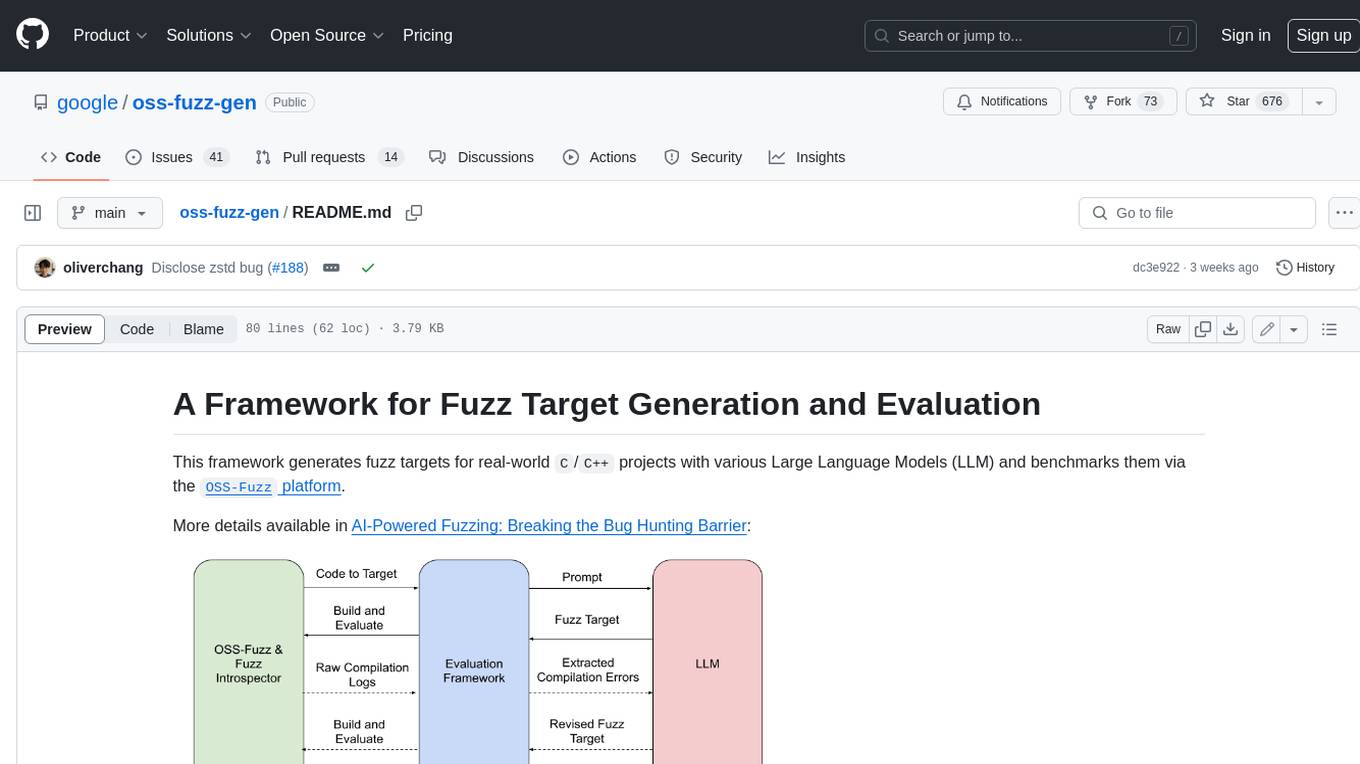
oss-fuzz-gen
This framework generates fuzz targets for real-world `C`/`C++` projects with various Large Language Models (LLM) and benchmarks them via the `OSS-Fuzz` platform. It manages to successfully leverage LLMs to generate valid fuzz targets (which generate non-zero coverage increase) for 160 C/C++ projects. The maximum line coverage increase is 29% from the existing human-written targets.
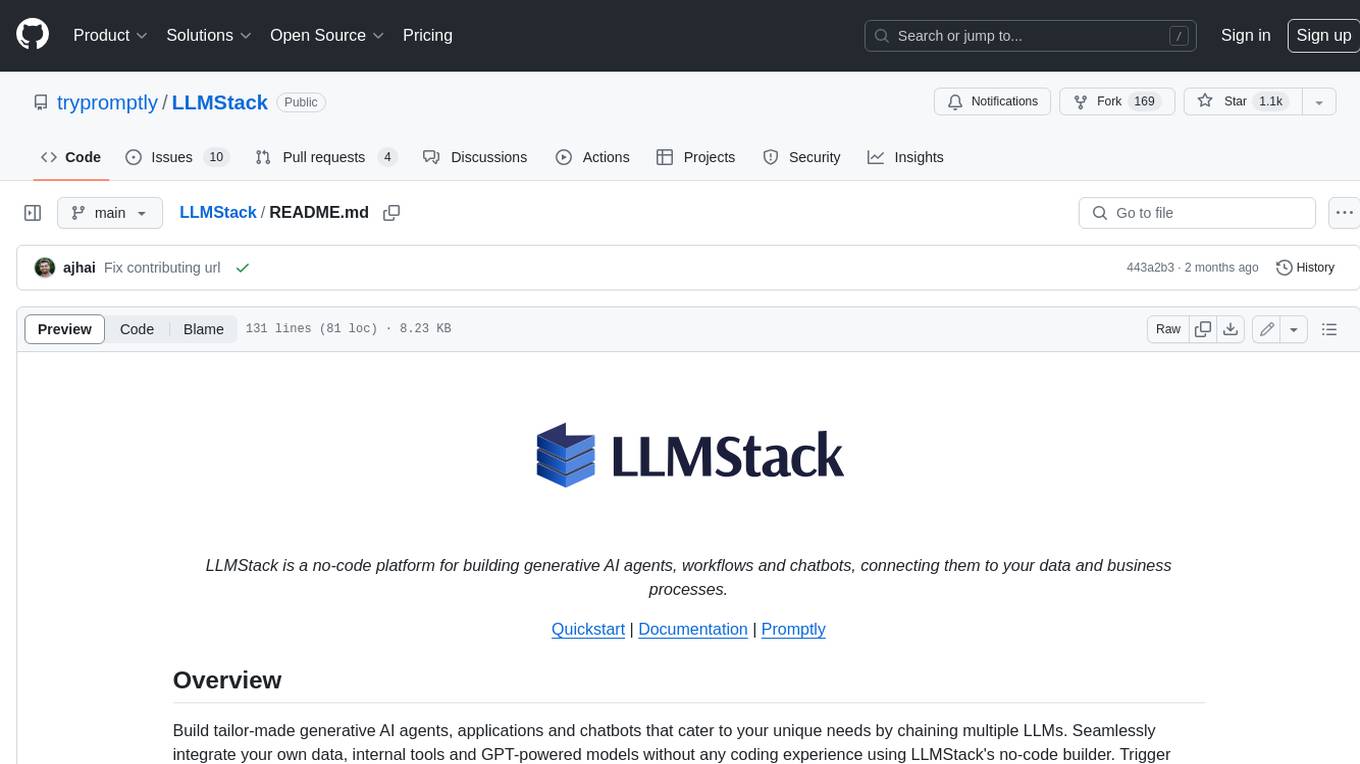
LLMStack
LLMStack is a no-code platform for building generative AI agents, workflows, and chatbots. It allows users to connect their own data, internal tools, and GPT-powered models without any coding experience. LLMStack can be deployed to the cloud or on-premise and can be accessed via HTTP API or triggered from Slack or Discord.
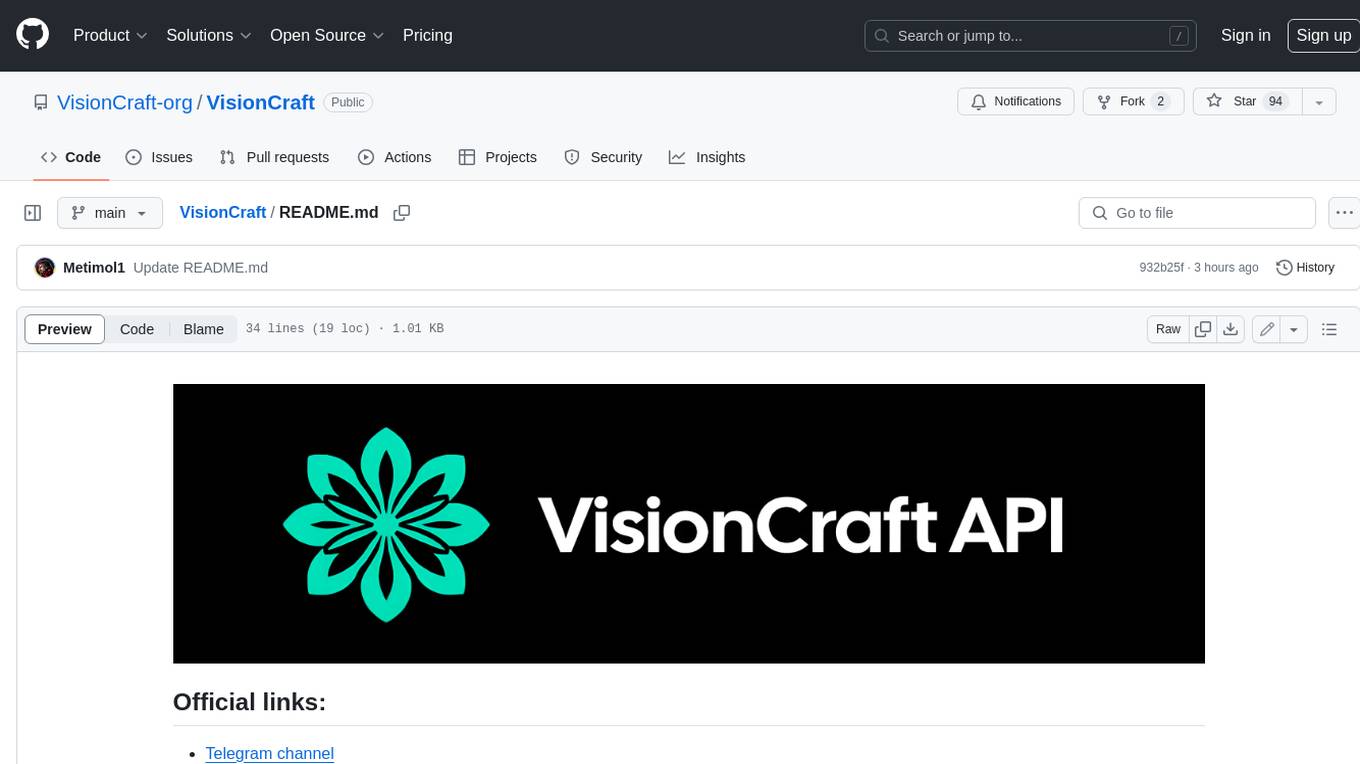
VisionCraft
The VisionCraft API is a free API for using over 100 different AI models. From images to sound.
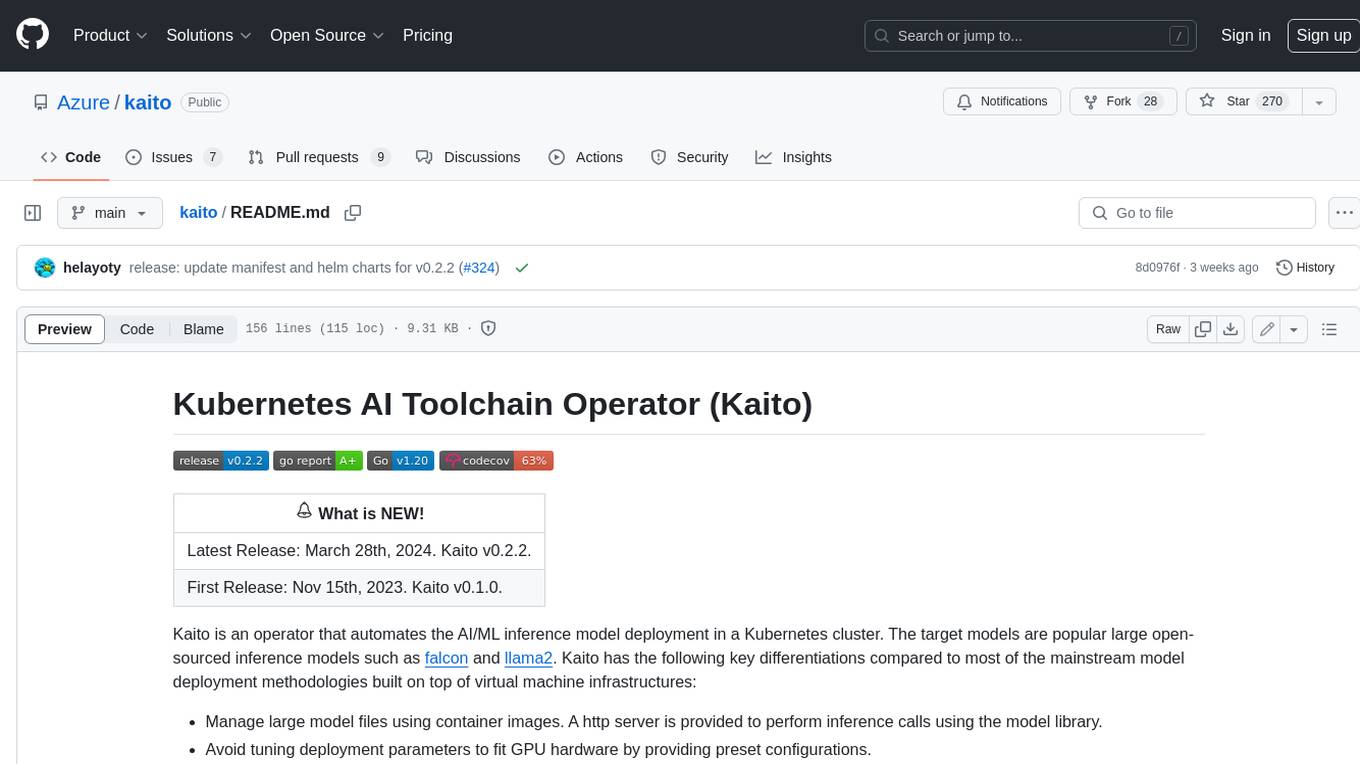
kaito
Kaito is an operator that automates the AI/ML inference model deployment in a Kubernetes cluster. It manages large model files using container images, avoids tuning deployment parameters to fit GPU hardware by providing preset configurations, auto-provisions GPU nodes based on model requirements, and hosts large model images in the public Microsoft Container Registry (MCR) if the license allows. Using Kaito, the workflow of onboarding large AI inference models in Kubernetes is largely simplified.
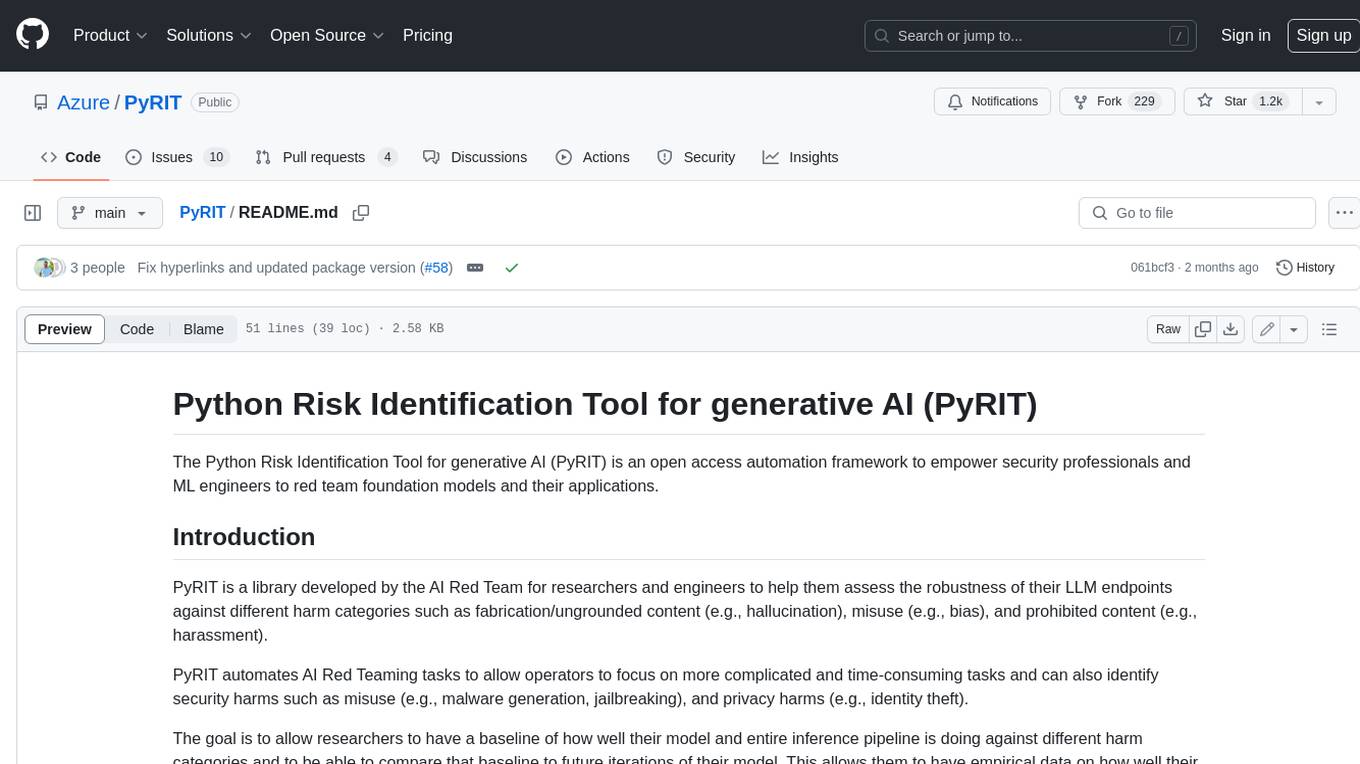
PyRIT
PyRIT is an open access automation framework designed to empower security professionals and ML engineers to red team foundation models and their applications. It automates AI Red Teaming tasks to allow operators to focus on more complicated and time-consuming tasks and can also identify security harms such as misuse (e.g., malware generation, jailbreaking), and privacy harms (e.g., identity theft). The goal is to allow researchers to have a baseline of how well their model and entire inference pipeline is doing against different harm categories and to be able to compare that baseline to future iterations of their model. This allows them to have empirical data on how well their model is doing today, and detect any degradation of performance based on future improvements.
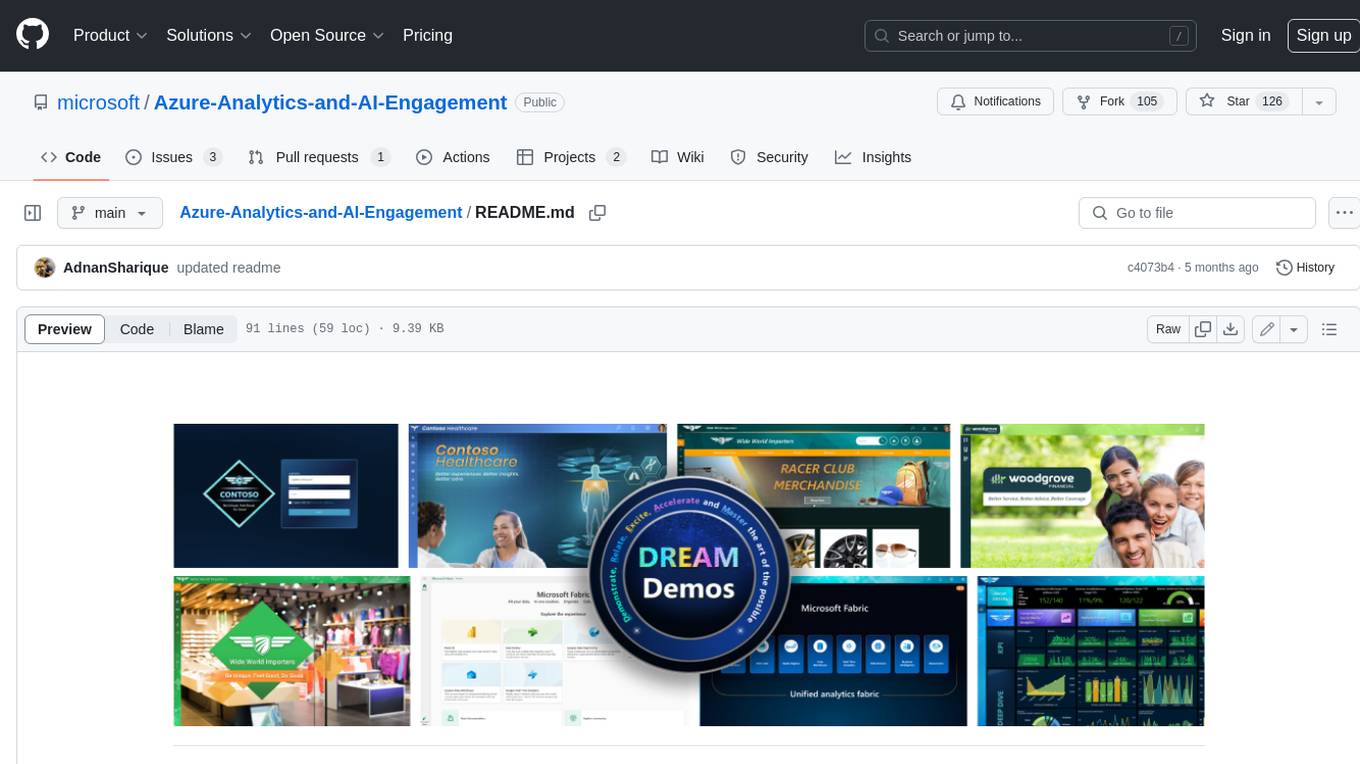
Azure-Analytics-and-AI-Engagement
The Azure-Analytics-and-AI-Engagement repository provides packaged Industry Scenario DREAM Demos with ARM templates (Containing a demo web application, Power BI reports, Synapse resources, AML Notebooks etc.) that can be deployed in a customer’s subscription using the CAPE tool within a matter of few hours. Partners can also deploy DREAM Demos in their own subscriptions using DPoC.