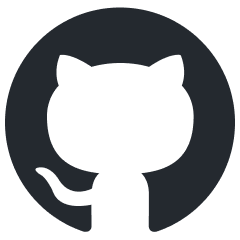
MaxKB
🚀 基于大语言模型和 RAG 的知识库问答系统。开箱即用、模型中立、灵活编排,支持快速嵌入到第三方业务系统。
Stars: 10861
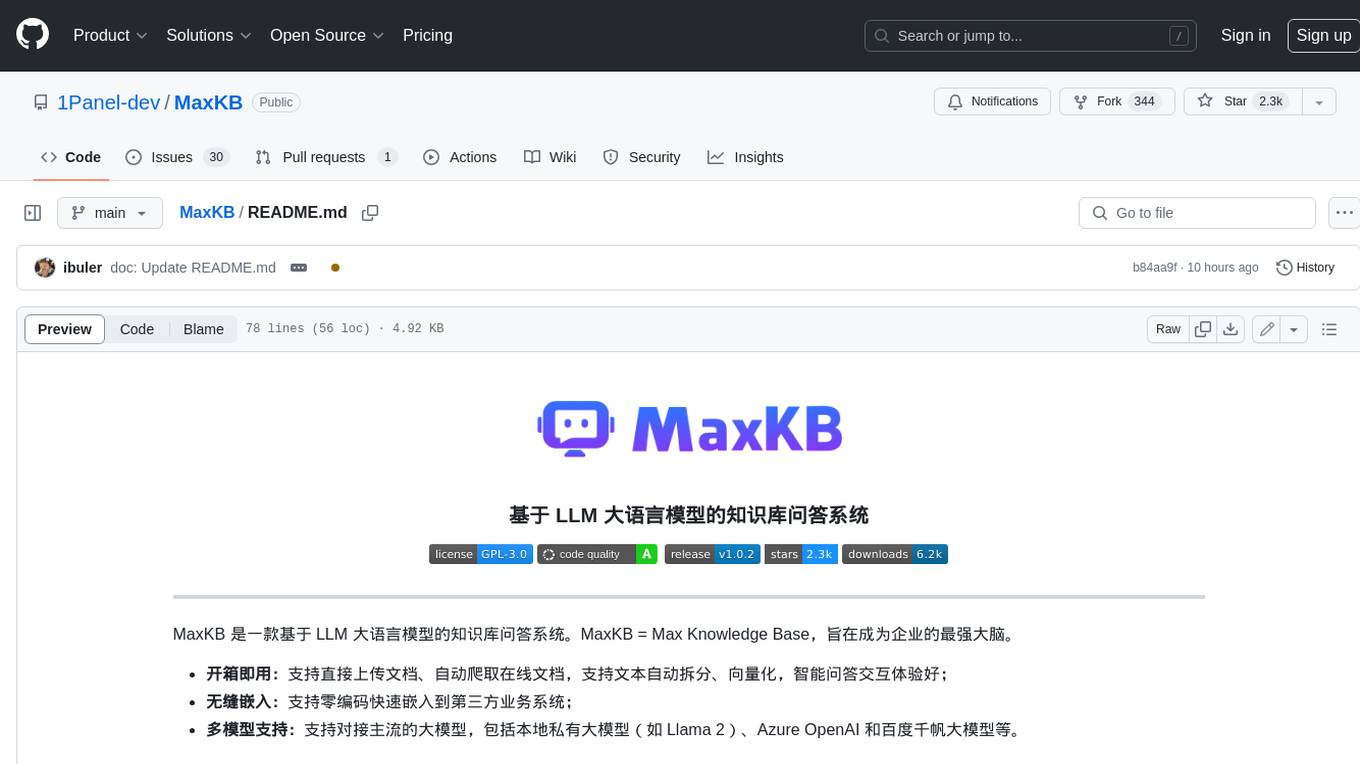
MaxKB is a knowledge base Q&A system based on the LLM large language model. MaxKB = Max Knowledge Base, which aims to become the most powerful brain of the enterprise.
README:
MaxKB = Max Knowledge Base,是一款基于大语言模型和 RAG 的开源知识库问答系统,广泛应用于企业内部知识库、客户服务、学术研究与教育等场景。
- 开箱即用:支持直接上传文档 / 自动爬取在线文档,支持文本自动拆分、向量化和 RAG(检索增强生成),有效减少大模型幻觉,智能问答交互体验好;
- 模型中立:支持对接各种大模型,包括本地私有大模型(Llama 3 / Qwen 2 等)、国内公共大模型(通义千问 / 腾讯混元 / 字节豆包 / 百度千帆 / 智谱 AI / Kimi 等)和国外公共大模型(OpenAI / Claude / Gemini 等);
- 灵活编排:内置强大的工作流引擎和函数库,支持编排 AI 工作过程,满足复杂业务场景下的需求;
- 无缝嵌入:支持零编码快速嵌入到第三方业务系统,让已有系统快速拥有智能问答能力,提高用户满意度。
docker run -d --name=maxkb --restart=always -p 8080:8080 -v ~/.maxkb:/var/lib/postgresql/data -v ~/.python-packages:/opt/maxkb/app/sandbox/python-packages cr2.fit2cloud.com/1panel/maxkb
# 用户名: admin
# 密码: MaxKB@123..
- 你也可以通过 1Panel 应用商店 快速部署 MaxKB + Ollama + Llama 3 / Qwen 2,快速上线基于本地大模型的 AI 知识库问答系统;
- 如果是内网环境,推荐使用 离线安装包 进行安装部署;
- 你也可以在线体验:DataEase 小助手,它是基于 MaxKB 搭建的智能 AI 问答系统,已经嵌入到 DataEase 产品及在线文档中;
- MaxKB 产品版本分为社区版和专业版,详情请参见:MaxKB 产品版本对比。
如你有更多问题,可以查看使用手册,或者通过论坛与我们交流。
- 前端:Vue.js
- 后端:Python / Django
- LangChain:LangChain
- 向量数据库:PostgreSQL / pgvector
- 大模型:各种本地私有或者公共大模型
- 1Panel - 现代化、开源的 Linux 服务器运维管理面板
- JumpServer - 广受欢迎的开源堡垒机
- DataEase - 人人可用的开源数据可视化分析工具
- MeterSphere - 新一代的开源持续测试工具
- Halo - 强大易用的开源建站工具
Copyright (c) 2014-2024 飞致云 FIT2CLOUD, All rights reserved.
Licensed under The GNU General Public License version 3 (GPLv3) (the "License"); you may not use this file except in compliance with the License. You may obtain a copy of the License at
https://www.gnu.org/licenses/gpl-3.0.html
Unless required by applicable law or agreed to in writing, software distributed under the License is distributed on an "AS IS" BASIS, WITHOUT WARRANTIES OR CONDITIONS OF ANY KIND, either express or implied. See the License for the specific language governing permissions and limitations under the License.
For Tasks:
Click tags to check more tools for each tasksFor Jobs:
Alternative AI tools for MaxKB
Similar Open Source Tools
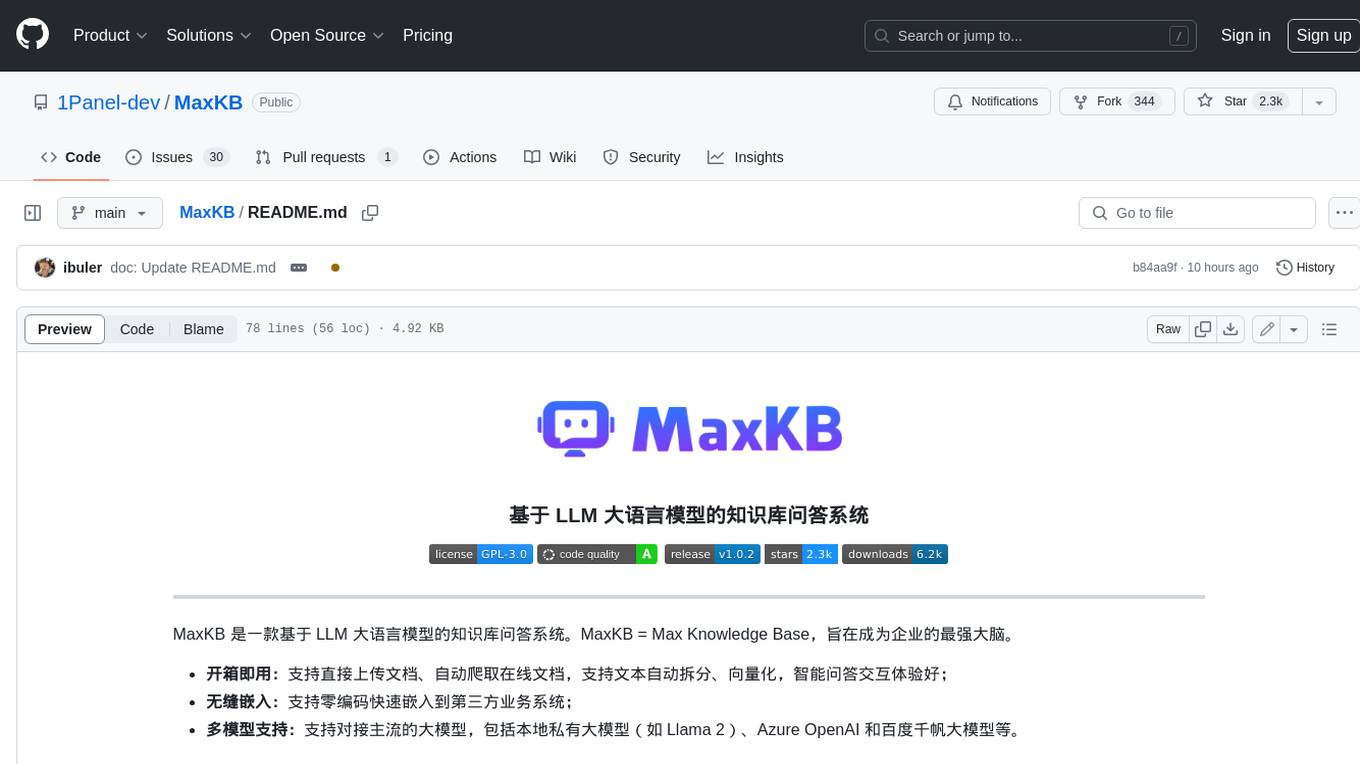
MaxKB
MaxKB is a knowledge base Q&A system based on the LLM large language model. MaxKB = Max Knowledge Base, which aims to become the most powerful brain of the enterprise.
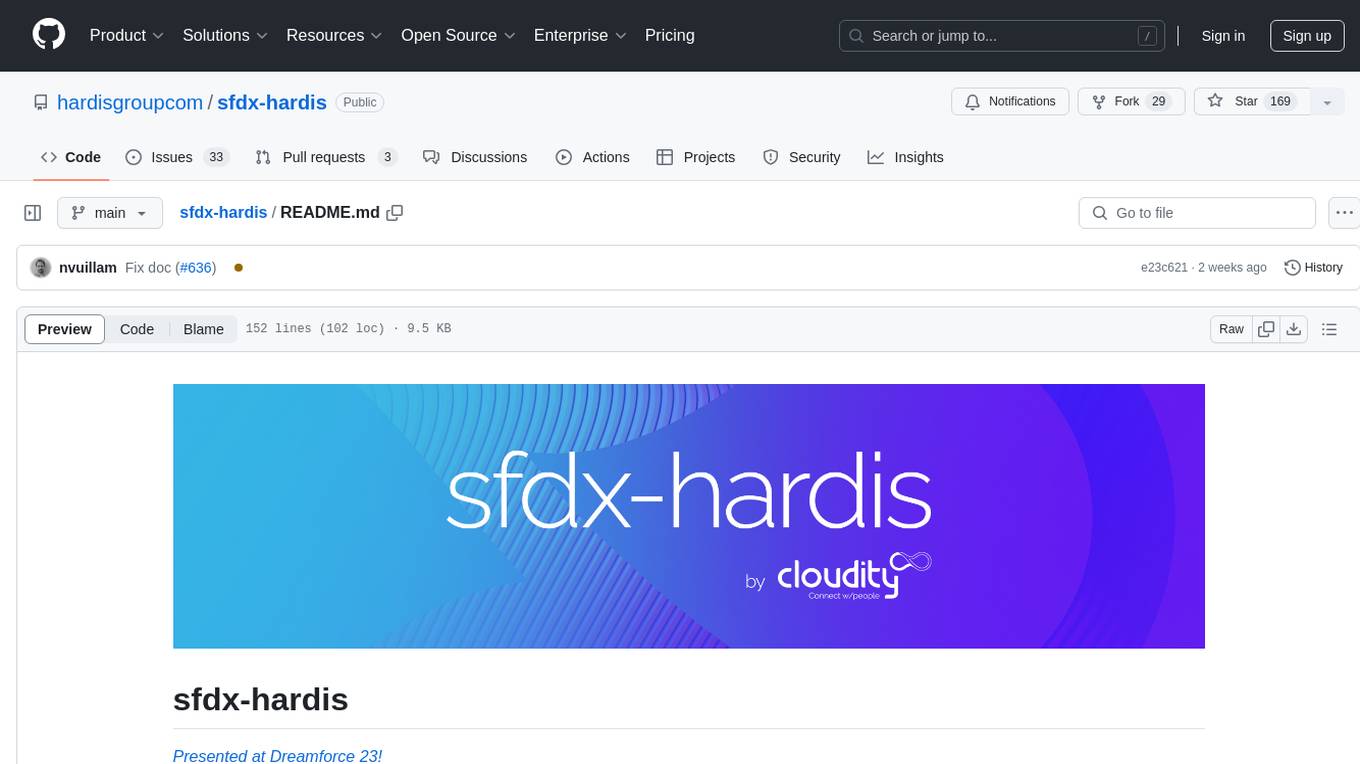
sfdx-hardis
sfdx-hardis is a toolbox for Salesforce DX, developed by Cloudity, that simplifies tasks which would otherwise take minutes or hours to complete manually. It enables users to define complete CI/CD pipelines for Salesforce projects, backup metadata, and monitor any Salesforce org. The tool offers a wide range of commands that can be accessed via the command line interface or through a Visual Studio Code extension. Additionally, sfdx-hardis provides Docker images for easy integration into CI workflows. The tool is designed to be natively compliant with various platforms and tools, making it a versatile solution for Salesforce developers.
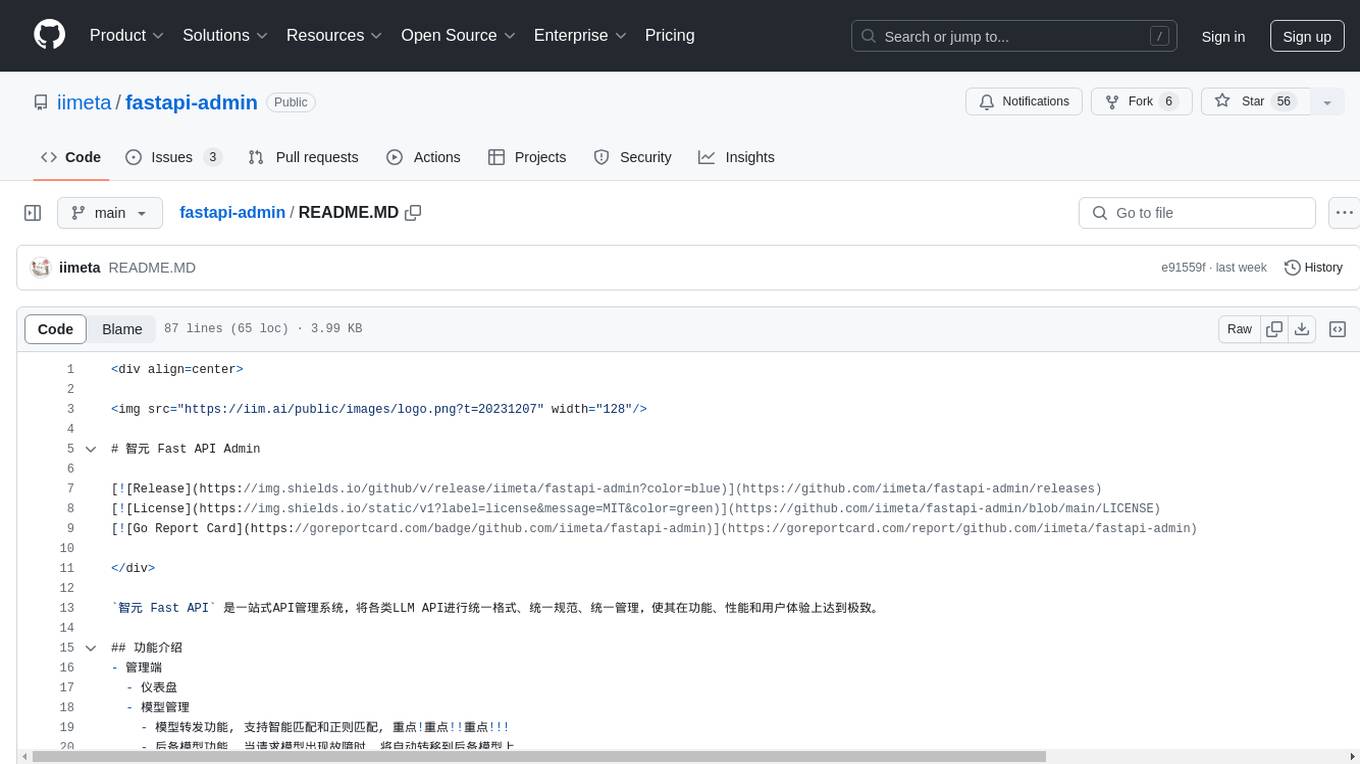
fastapi-admin
智元 Fast API is a one-stop API management system that unifies various LLM APIs in terms of format, standards, and management to achieve the ultimate in functionality, performance, and user experience. It includes features such as model management with intelligent and regex matching, backup model functionality, key management, proxy management, company management, user management, and chat management for both admin and user ends. The project supports cluster deployment, multi-site deployment, and cross-region deployment. It also provides a public API site for registration with a contact to the author for a 10 million quota. The tool offers a comprehensive dashboard, model management, application management, key management, and chat management functionalities for users.
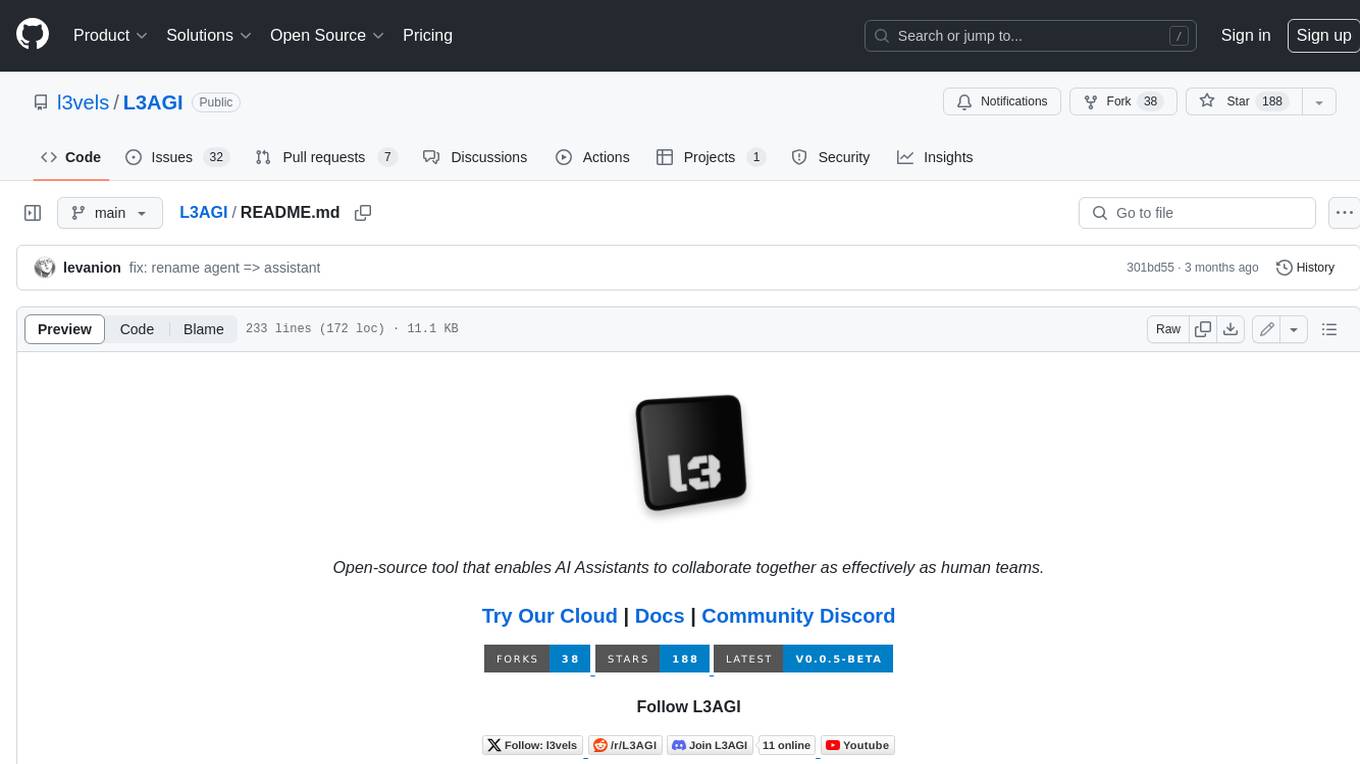
L3AGI
L3AGI is an open-source tool that enables AI Assistants to collaborate together as effectively as human teams. It provides a robust set of functionalities that empower users to design, supervise, and execute both autonomous AI Assistants and Teams of Assistants. Key features include the ability to create and manage Teams of AI Assistants, design and oversee standalone AI Assistants, equip AI Assistants with the ability to retain and recall information, connect AI Assistants to an array of data sources for efficient information retrieval and processing, and employ curated sets of tools for specific tasks. L3AGI also offers a user-friendly interface, APIs for integration with other systems, and a vibrant community for support and collaboration.
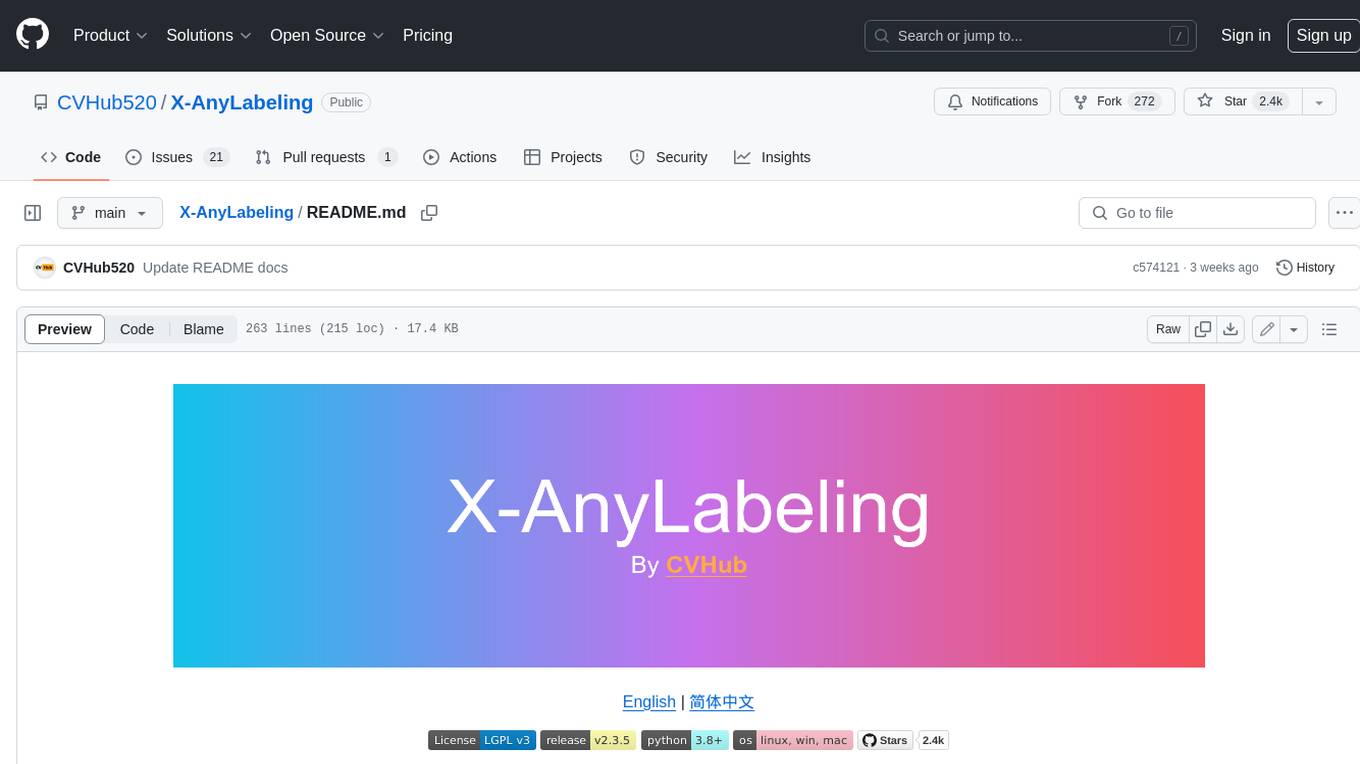
X-AnyLabeling
X-AnyLabeling is a robust annotation tool that seamlessly incorporates an AI inference engine alongside an array of sophisticated features. Tailored for practical applications, it is committed to delivering comprehensive, industrial-grade solutions for image data engineers. This tool excels in swiftly and automatically executing annotations across diverse and intricate tasks.
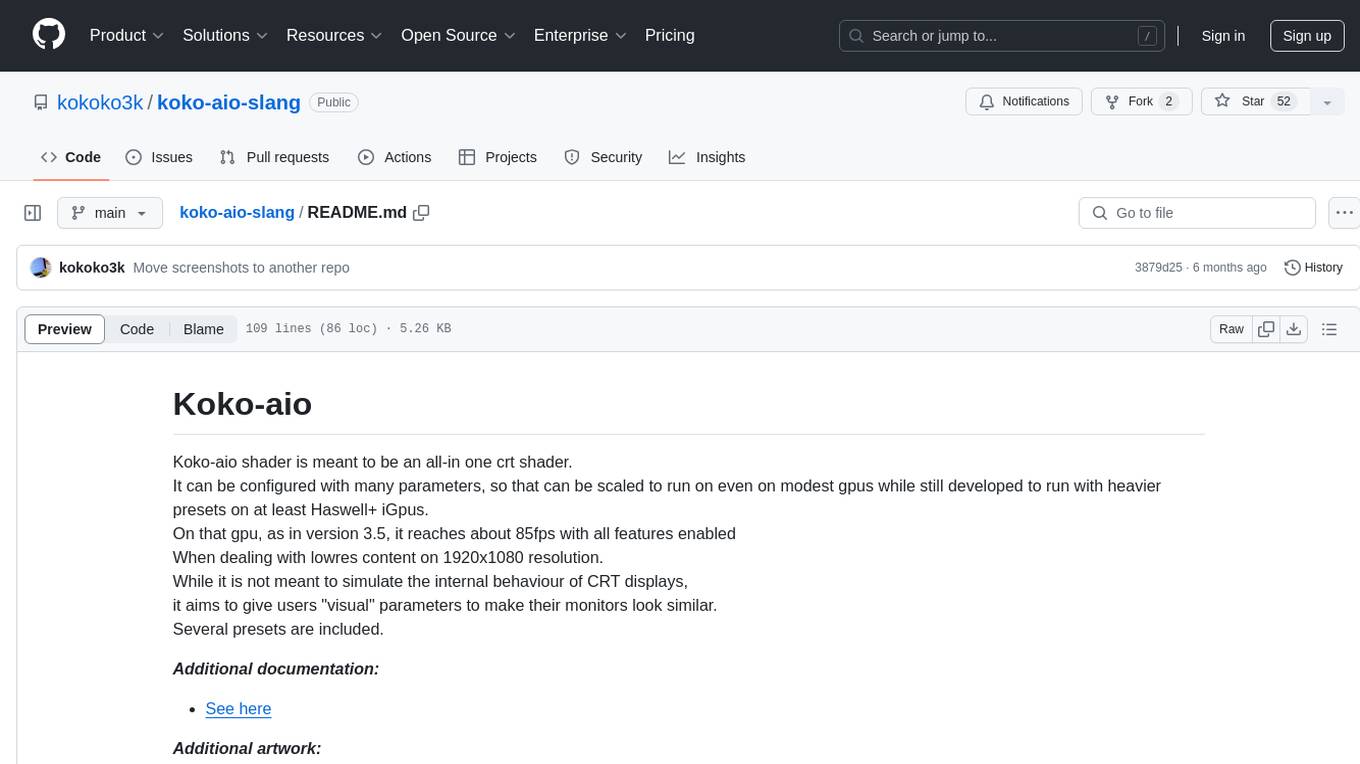
koko-aio-slang
Koko-aio shader is an all-in-one CRT shader tool that can be configured with various parameters to run on different GPUs. It aims to provide visual parameters to make monitors look similar to CRT displays without simulating their internal behavior. The tool includes features such as color corrections, B/W display colorization, antialiasing, noise effects, deconvergence, blurring/sharpening, interlacing, phosphor glow, and more. It also supports ambient lighting, vignette, integer scaling, and various image effects. Koko-aio is designed to enhance the visual experience of low-res content on high-resolution displays.
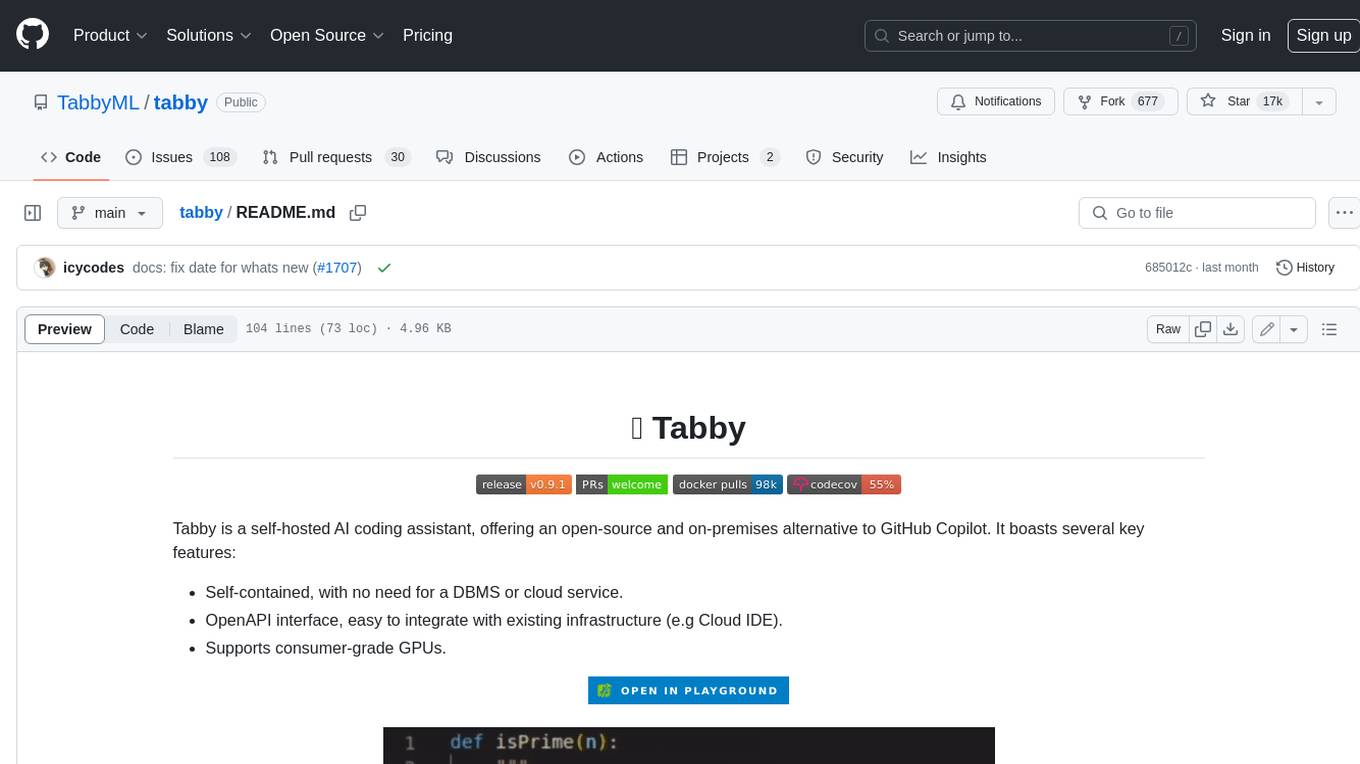
tabby
Tabby is a self-hosted AI coding assistant, offering an open-source and on-premises alternative to GitHub Copilot. It boasts several key features: * Self-contained, with no need for a DBMS or cloud service. * OpenAPI interface, easy to integrate with existing infrastructure (e.g Cloud IDE). * Supports consumer-grade GPUs.
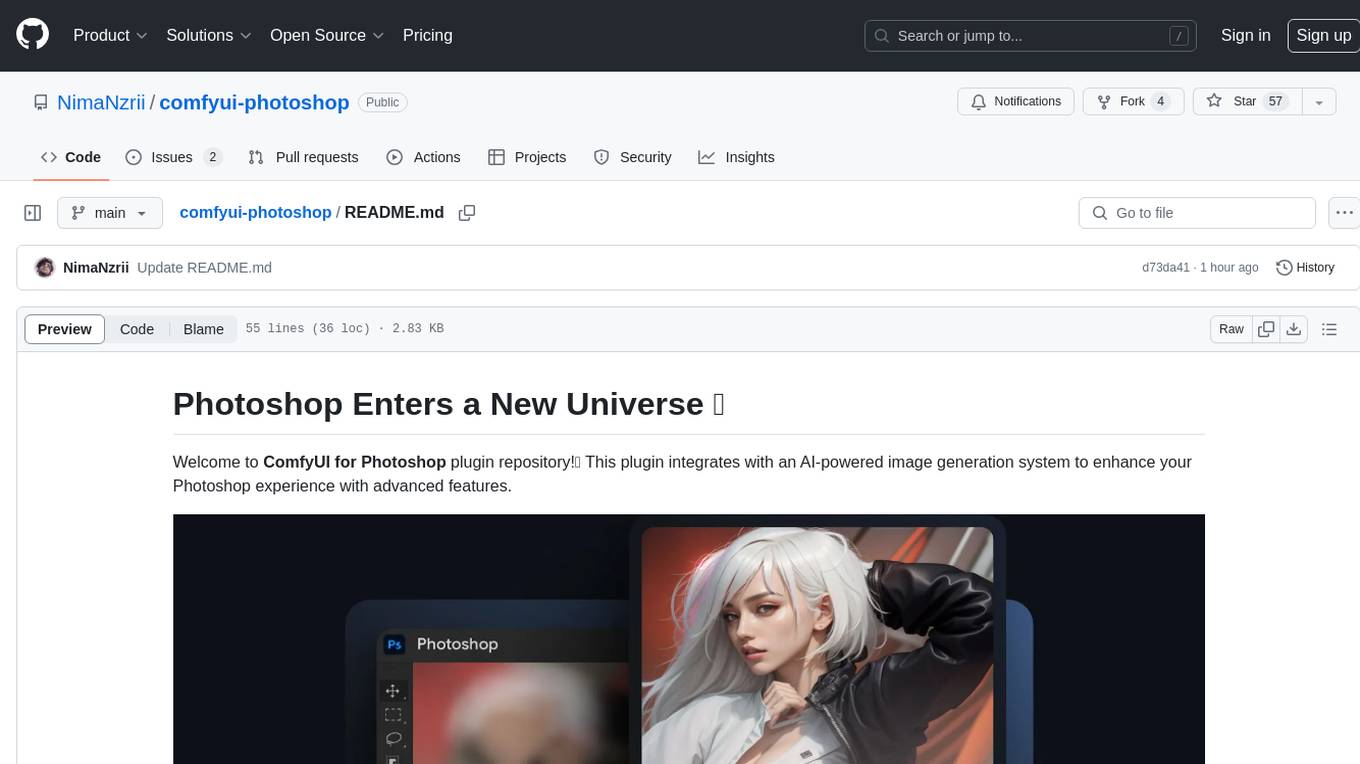
comfyui-photoshop
ComfyUI for Photoshop is a plugin that integrates with an AI-powered image generation system to enhance the Photoshop experience with features like unlimited generative fill, customizable back-end, AI-powered artistry, and one-click transformation. The plugin requires a minimum of 6GB graphics memory and 12GB RAM. Users can install the plugin and set up the ComfyUI workflow using provided links and files. Additionally, specific files like Check points, Loras, and Detailer Lora are required for different functionalities. Support and contributions are encouraged through GitHub.
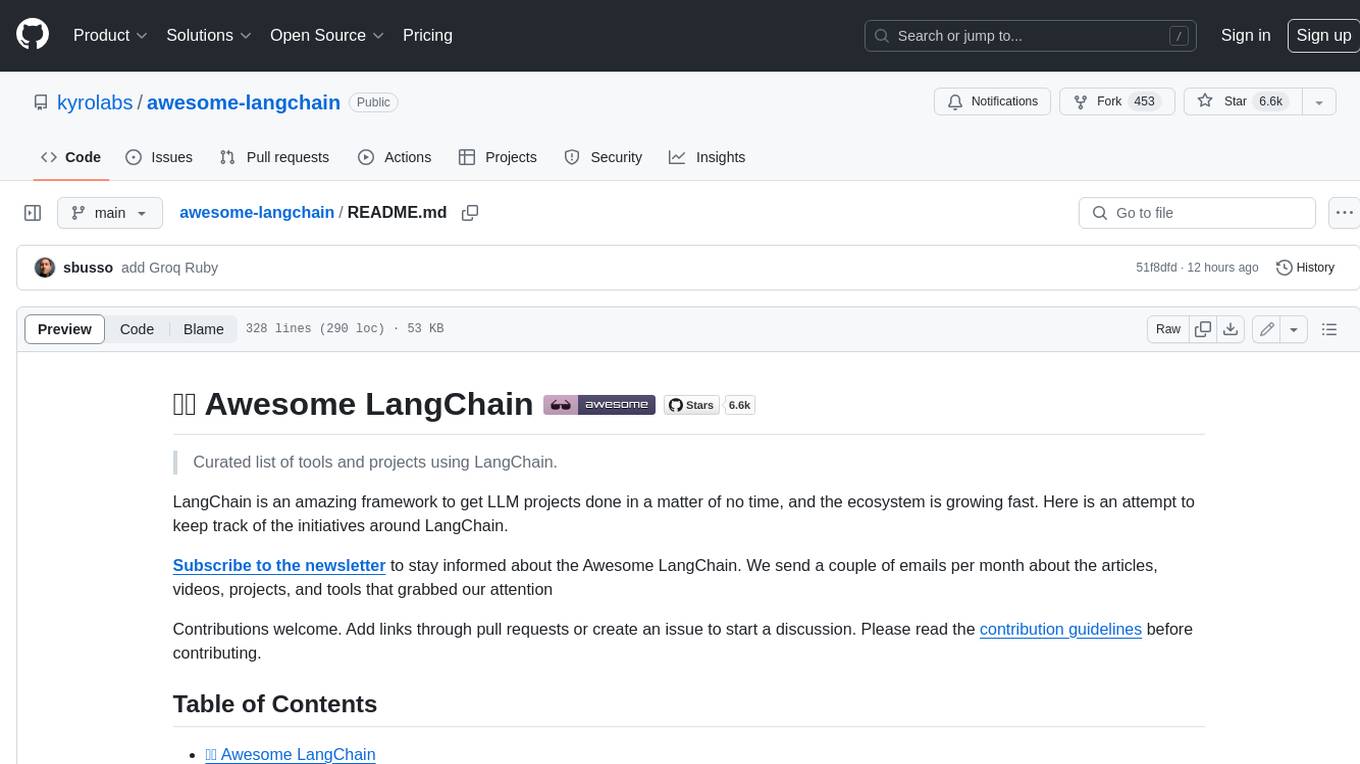
awesome-langchain
LangChain is an amazing framework to get LLM projects done in a matter of no time, and the ecosystem is growing fast. Here is an attempt to keep track of the initiatives around LangChain. Subscribe to the newsletter to stay informed about the Awesome LangChain. We send a couple of emails per month about the articles, videos, projects, and tools that grabbed our attention Contributions welcome. Add links through pull requests or create an issue to start a discussion. Please read the contribution guidelines before contributing.
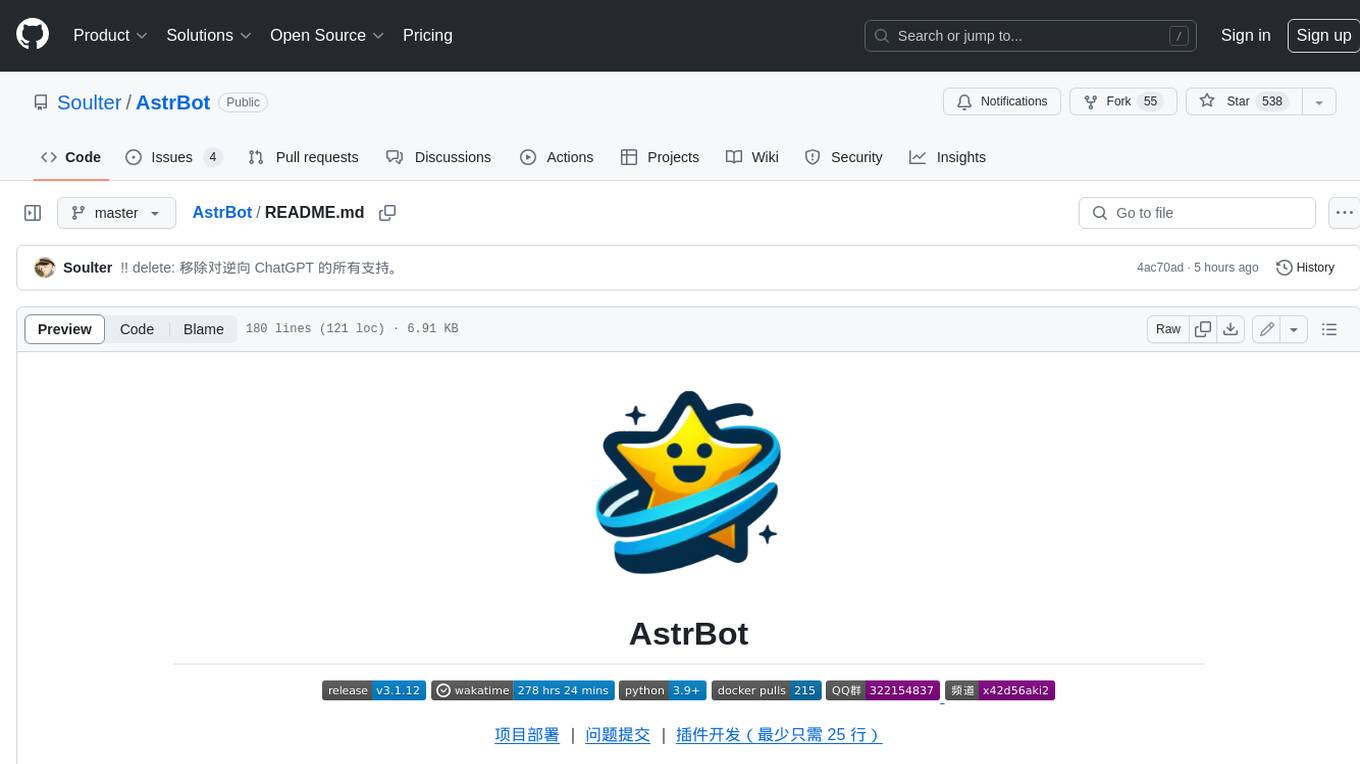
AstrBot
AstrBot is a powerful and versatile tool that leverages the capabilities of large language models (LLMs) like GPT-3, GPT-3.5, and GPT-4 to enhance communication and automate tasks. It seamlessly integrates with popular messaging platforms such as QQ, QQ Channel, and Telegram, enabling users to harness the power of AI within their daily conversations and workflows.
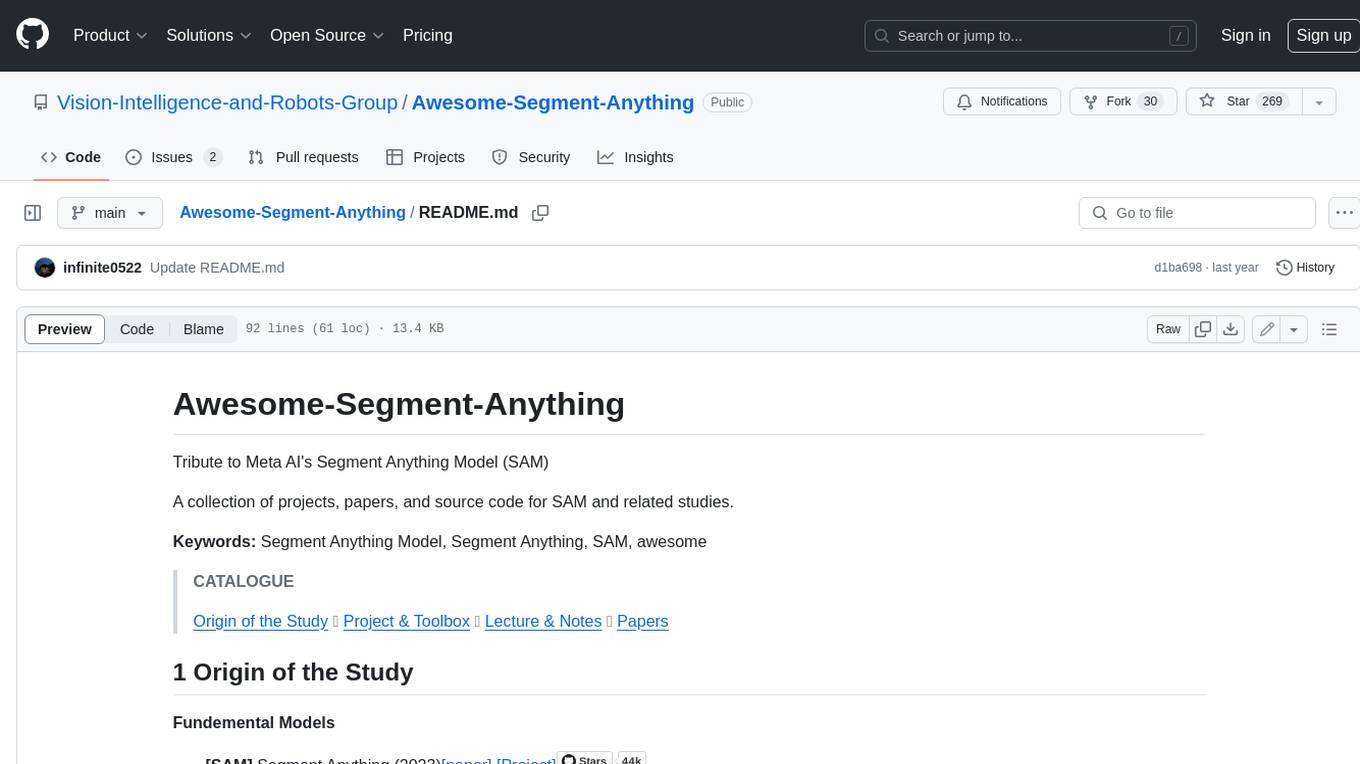
Awesome-Segment-Anything
The Segment Anything Model (SAM) is a powerful tool that allows users to segment any object in an image with just a few clicks. This makes it a great tool for a variety of tasks, such as object detection, tracking, and editing. SAM is also very easy to use, making it a great option for both beginners and experienced users.
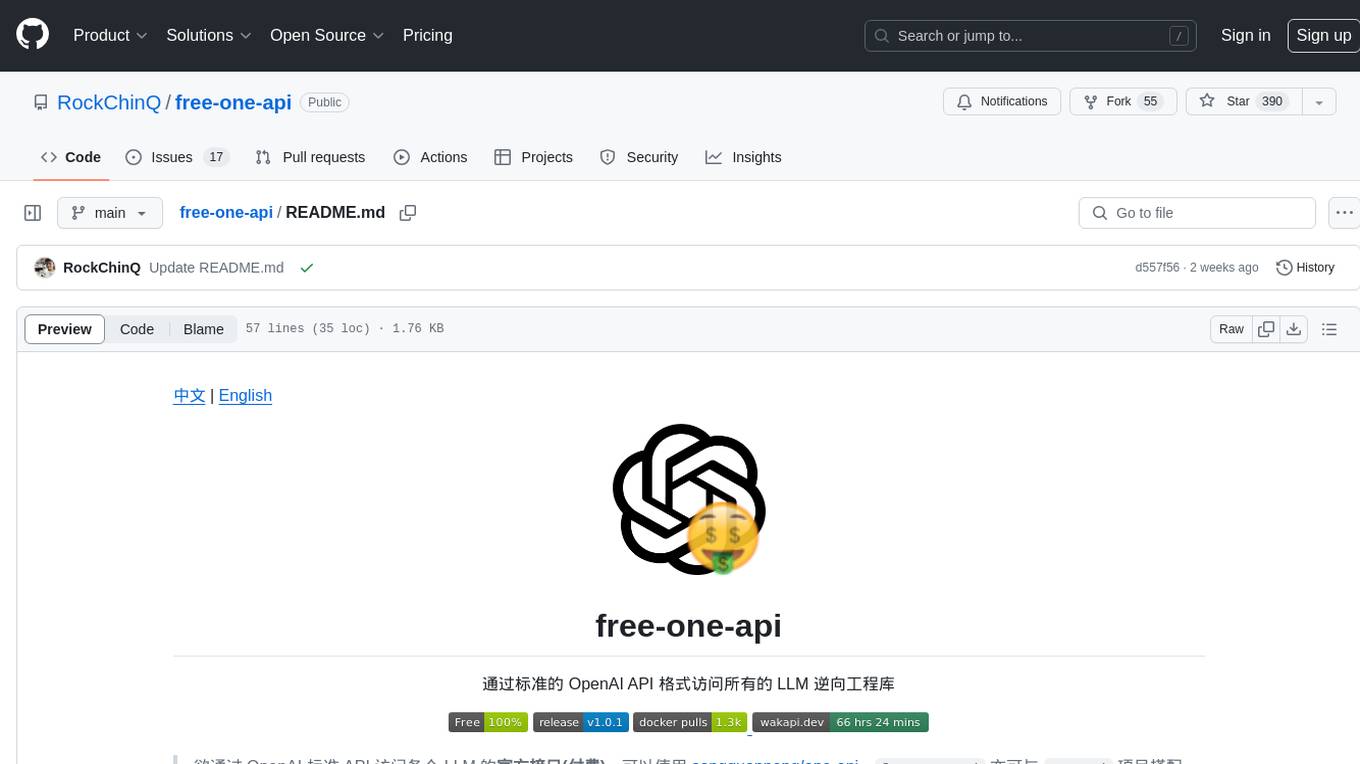
free-one-api
Free-one-api is a tool that allows access to all LLM reverse engineering libraries in a standard OpenAI API format. It supports automatic load balancing, Web UI, stream mode, multiple LLM reverse libraries, heartbeat detection mechanism, automatic disabling of unavailable channels, and runtime log recording. The tool is designed to work with the 'one-api' project and 'songquanpeng/one-api' for accessing official interfaces of various LLMs (paid). Contributors are needed to test adapters, find new reverse engineering libraries, and submit PRs.
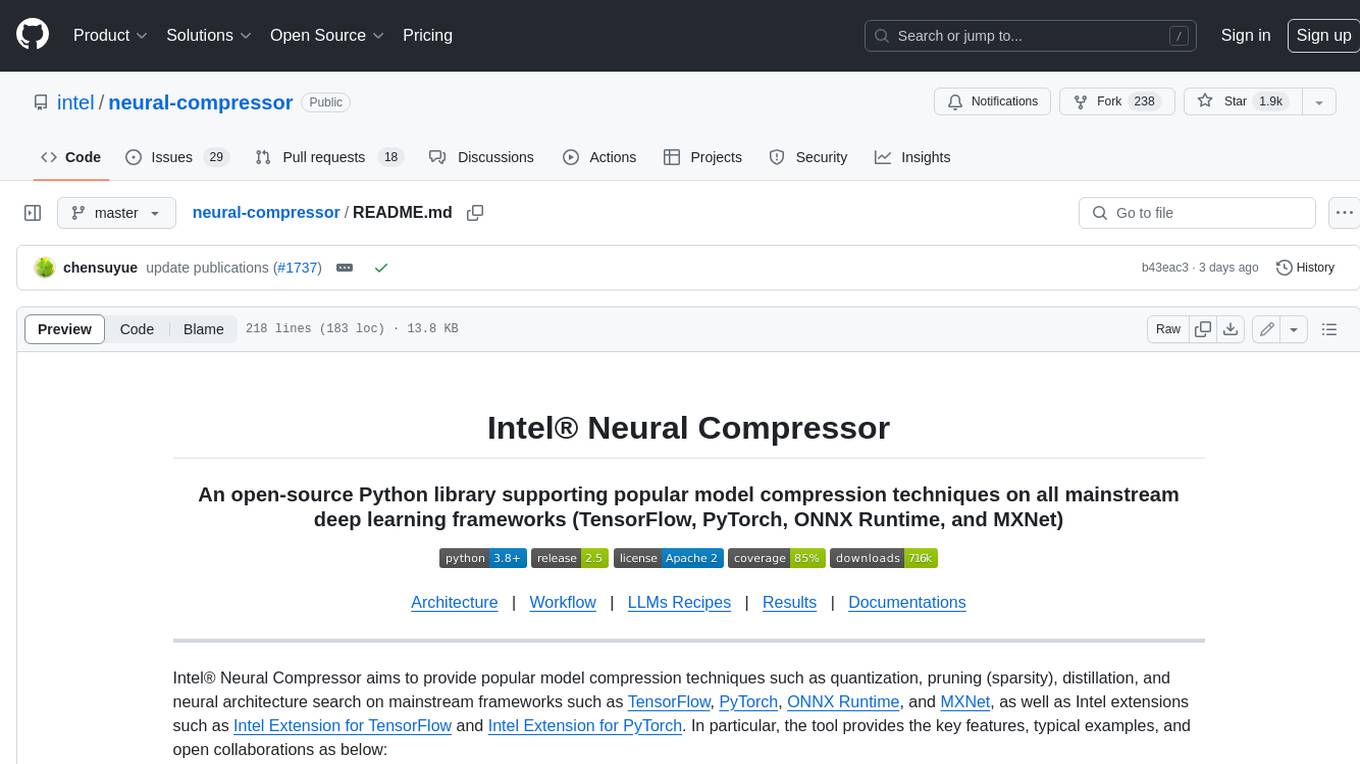
neural-compressor
Intel® Neural Compressor is an open-source Python library that supports popular model compression techniques such as quantization, pruning (sparsity), distillation, and neural architecture search on mainstream frameworks such as TensorFlow, PyTorch, ONNX Runtime, and MXNet. It provides key features, typical examples, and open collaborations, including support for a wide range of Intel hardware, validation of popular LLMs, and collaboration with cloud marketplaces, software platforms, and open AI ecosystems.
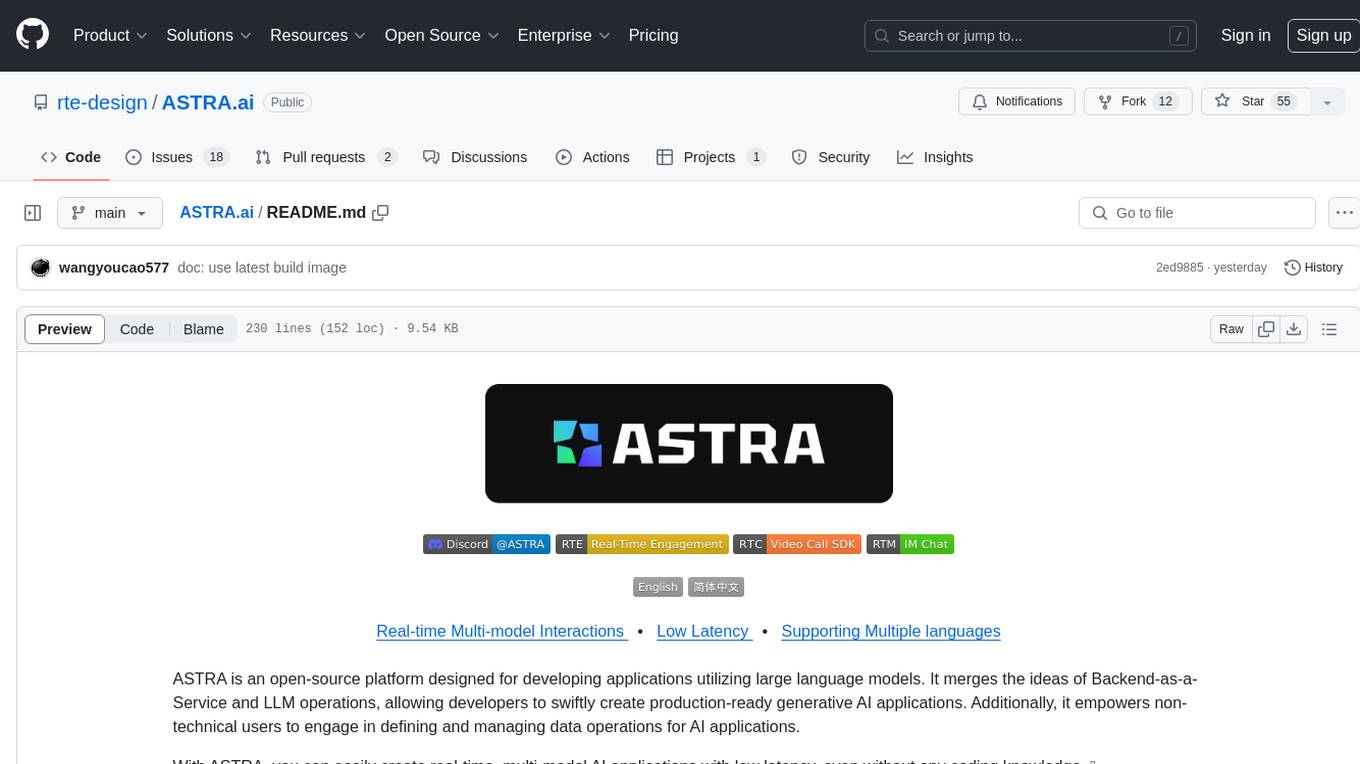
ASTRA.ai
ASTRA is an open-source platform designed for developing applications utilizing large language models. It merges the ideas of Backend-as-a-Service and LLM operations, allowing developers to swiftly create production-ready generative AI applications. Additionally, it empowers non-technical users to engage in defining and managing data operations for AI applications. With ASTRA, you can easily create real-time, multi-modal AI applications with low latency, even without any coding knowledge.
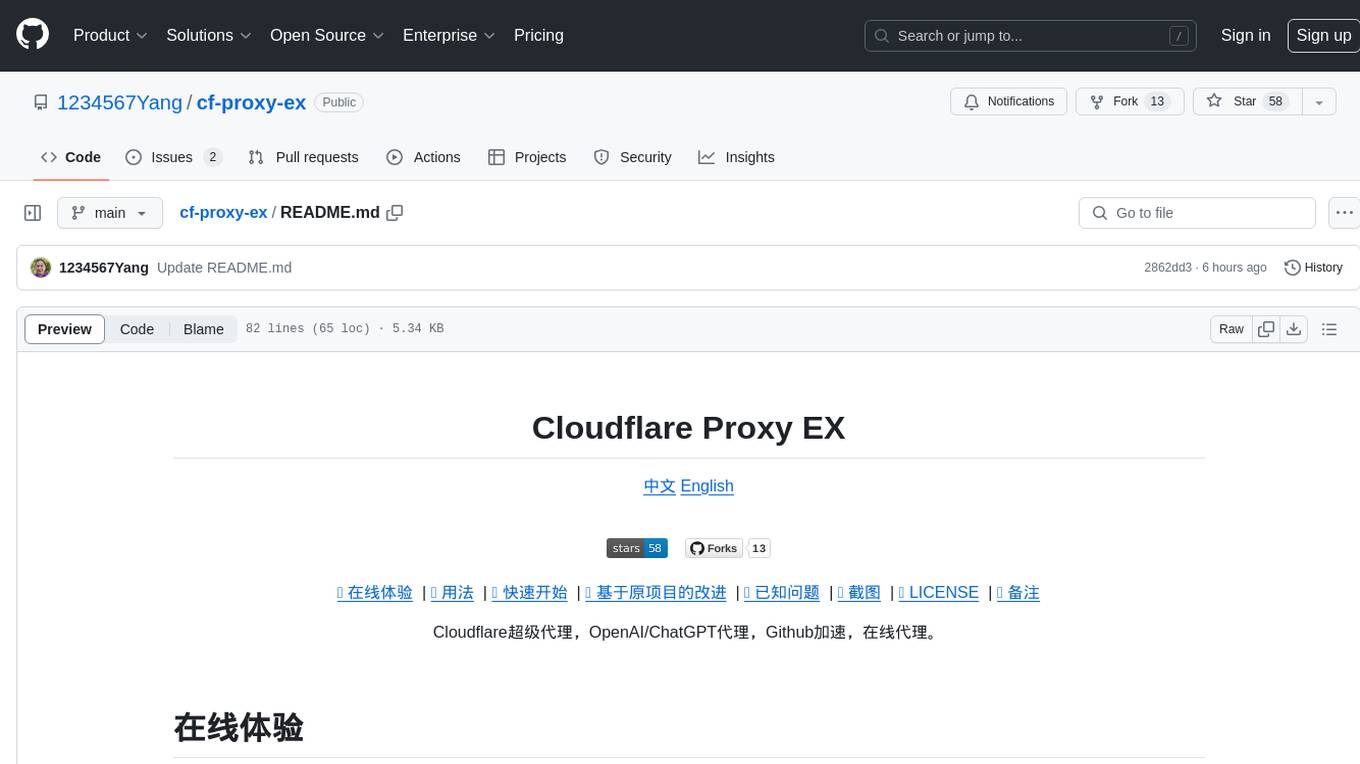
cf-proxy-ex
Cloudflare Proxy EX is a tool that provides Cloudflare super proxy, OpenAI/ChatGPT proxy, Github acceleration, and online proxy services. It allows users to create a worker in Cloudflare website by copying the content from worker.js file, and add their domain name before any URL to use the tool. The tool is an improvement based on gaboolic's cloudflare-reverse-proxy, offering features like removing '/proxy/', handling redirection events, modifying headers, converting relative paths to absolute paths, and more. It aims to enhance proxy functionality and address issues faced by some websites. However, users are advised not to log in to any website through the online proxy due to potential security risks.
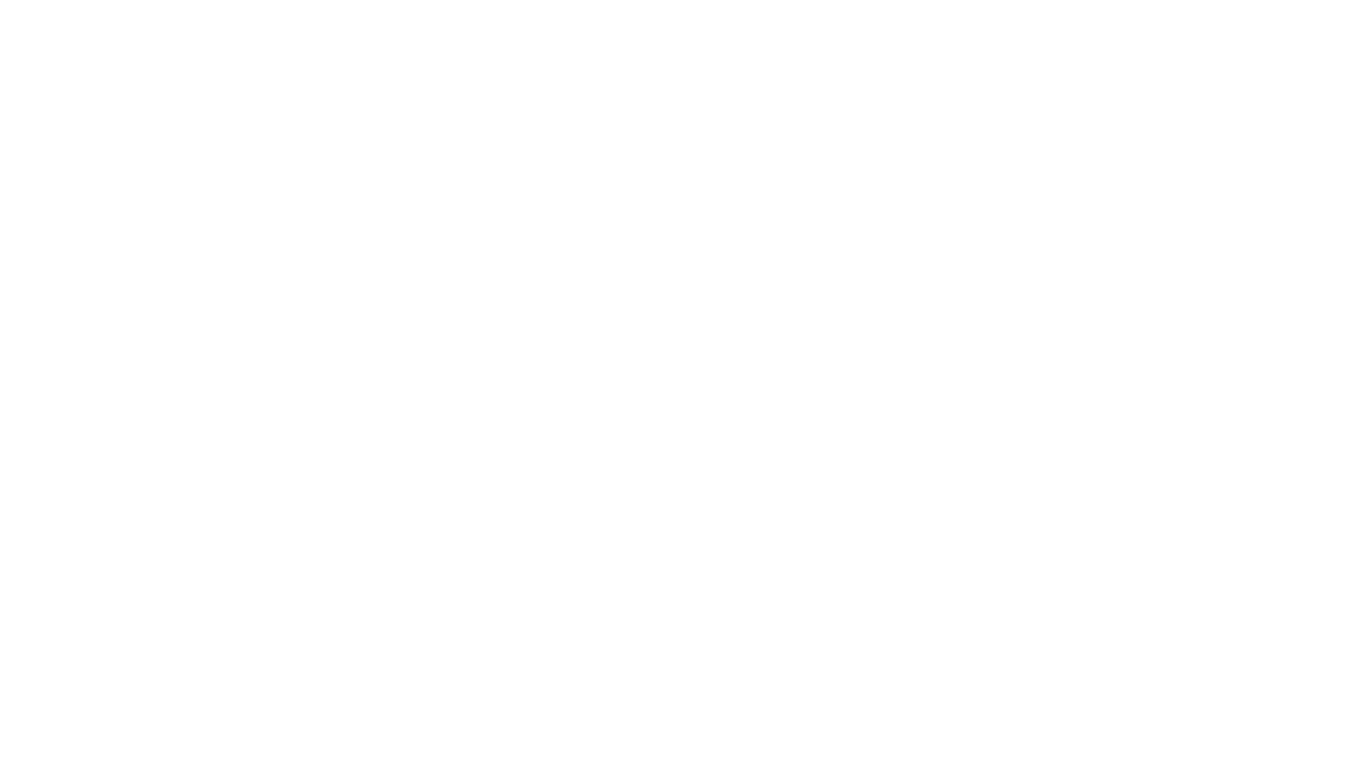
intel-extension-for-transformers
Intel® Extension for Transformers is an innovative toolkit designed to accelerate GenAI/LLM everywhere with the optimal performance of Transformer-based models on various Intel platforms, including Intel Gaudi2, Intel CPU, and Intel GPU. The toolkit provides the below key features and examples: * Seamless user experience of model compressions on Transformer-based models by extending [Hugging Face transformers](https://github.com/huggingface/transformers) APIs and leveraging [Intel® Neural Compressor](https://github.com/intel/neural-compressor) * Advanced software optimizations and unique compression-aware runtime (released with NeurIPS 2022's paper [Fast Distilbert on CPUs](https://arxiv.org/abs/2211.07715) and [QuaLA-MiniLM: a Quantized Length Adaptive MiniLM](https://arxiv.org/abs/2210.17114), and NeurIPS 2021's paper [Prune Once for All: Sparse Pre-Trained Language Models](https://arxiv.org/abs/2111.05754)) * Optimized Transformer-based model packages such as [Stable Diffusion](examples/huggingface/pytorch/text-to-image/deployment/stable_diffusion), [GPT-J-6B](examples/huggingface/pytorch/text-generation/deployment), [GPT-NEOX](examples/huggingface/pytorch/language-modeling/quantization#2-validated-model-list), [BLOOM-176B](examples/huggingface/pytorch/language-modeling/inference#BLOOM-176B), [T5](examples/huggingface/pytorch/summarization/quantization#2-validated-model-list), [Flan-T5](examples/huggingface/pytorch/summarization/quantization#2-validated-model-list), and end-to-end workflows such as [SetFit-based text classification](docs/tutorials/pytorch/text-classification/SetFit_model_compression_AGNews.ipynb) and [document level sentiment analysis (DLSA)](workflows/dlsa) * [NeuralChat](intel_extension_for_transformers/neural_chat), a customizable chatbot framework to create your own chatbot within minutes by leveraging a rich set of [plugins](https://github.com/intel/intel-extension-for-transformers/blob/main/intel_extension_for_transformers/neural_chat/docs/advanced_features.md) such as [Knowledge Retrieval](./intel_extension_for_transformers/neural_chat/pipeline/plugins/retrieval/README.md), [Speech Interaction](./intel_extension_for_transformers/neural_chat/pipeline/plugins/audio/README.md), [Query Caching](./intel_extension_for_transformers/neural_chat/pipeline/plugins/caching/README.md), and [Security Guardrail](./intel_extension_for_transformers/neural_chat/pipeline/plugins/security/README.md). This framework supports Intel Gaudi2/CPU/GPU. * [Inference](https://github.com/intel/neural-speed/tree/main) of Large Language Model (LLM) in pure C/C++ with weight-only quantization kernels for Intel CPU and Intel GPU (TBD), supporting [GPT-NEOX](https://github.com/intel/neural-speed/tree/main/neural_speed/models/gptneox), [LLAMA](https://github.com/intel/neural-speed/tree/main/neural_speed/models/llama), [MPT](https://github.com/intel/neural-speed/tree/main/neural_speed/models/mpt), [FALCON](https://github.com/intel/neural-speed/tree/main/neural_speed/models/falcon), [BLOOM-7B](https://github.com/intel/neural-speed/tree/main/neural_speed/models/bloom), [OPT](https://github.com/intel/neural-speed/tree/main/neural_speed/models/opt), [ChatGLM2-6B](https://github.com/intel/neural-speed/tree/main/neural_speed/models/chatglm), [GPT-J-6B](https://github.com/intel/neural-speed/tree/main/neural_speed/models/gptj), and [Dolly-v2-3B](https://github.com/intel/neural-speed/tree/main/neural_speed/models/gptneox). Support AMX, VNNI, AVX512F and AVX2 instruction set. We've boosted the performance of Intel CPUs, with a particular focus on the 4th generation Intel Xeon Scalable processor, codenamed [Sapphire Rapids](https://www.intel.com/content/www/us/en/products/docs/processors/xeon-accelerated/4th-gen-xeon-scalable-processors.html).
For similar tasks
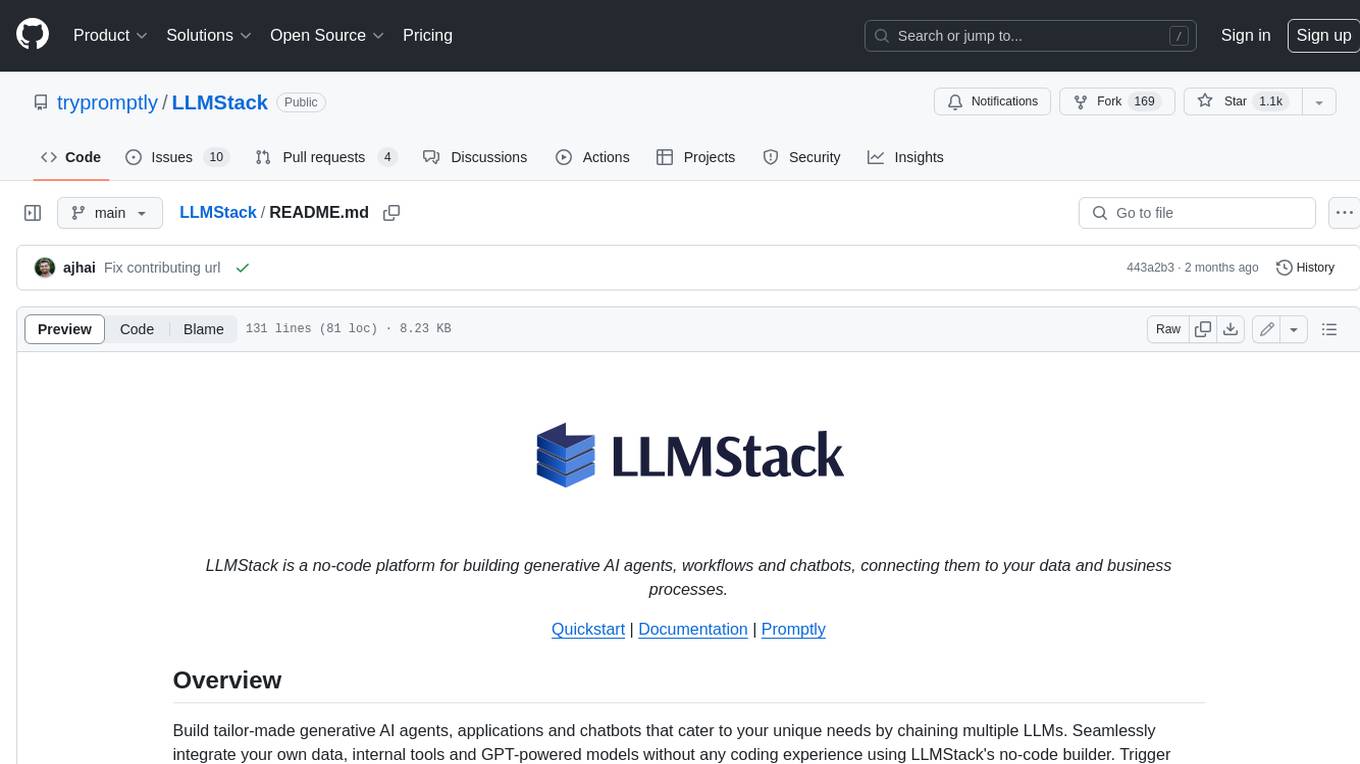
LLMStack
LLMStack is a no-code platform for building generative AI agents, workflows, and chatbots. It allows users to connect their own data, internal tools, and GPT-powered models without any coding experience. LLMStack can be deployed to the cloud or on-premise and can be accessed via HTTP API or triggered from Slack or Discord.
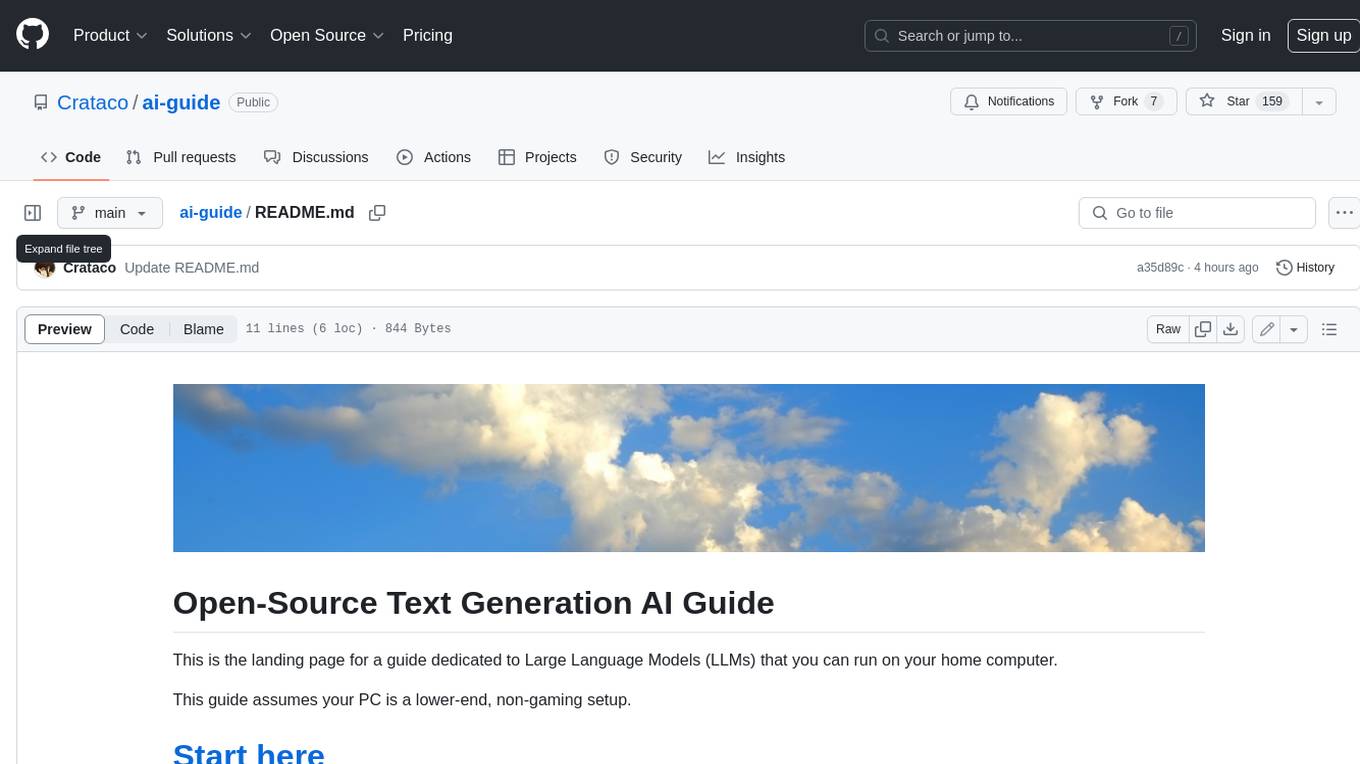
ai-guide
This guide is dedicated to Large Language Models (LLMs) that you can run on your home computer. It assumes your PC is a lower-end, non-gaming setup.
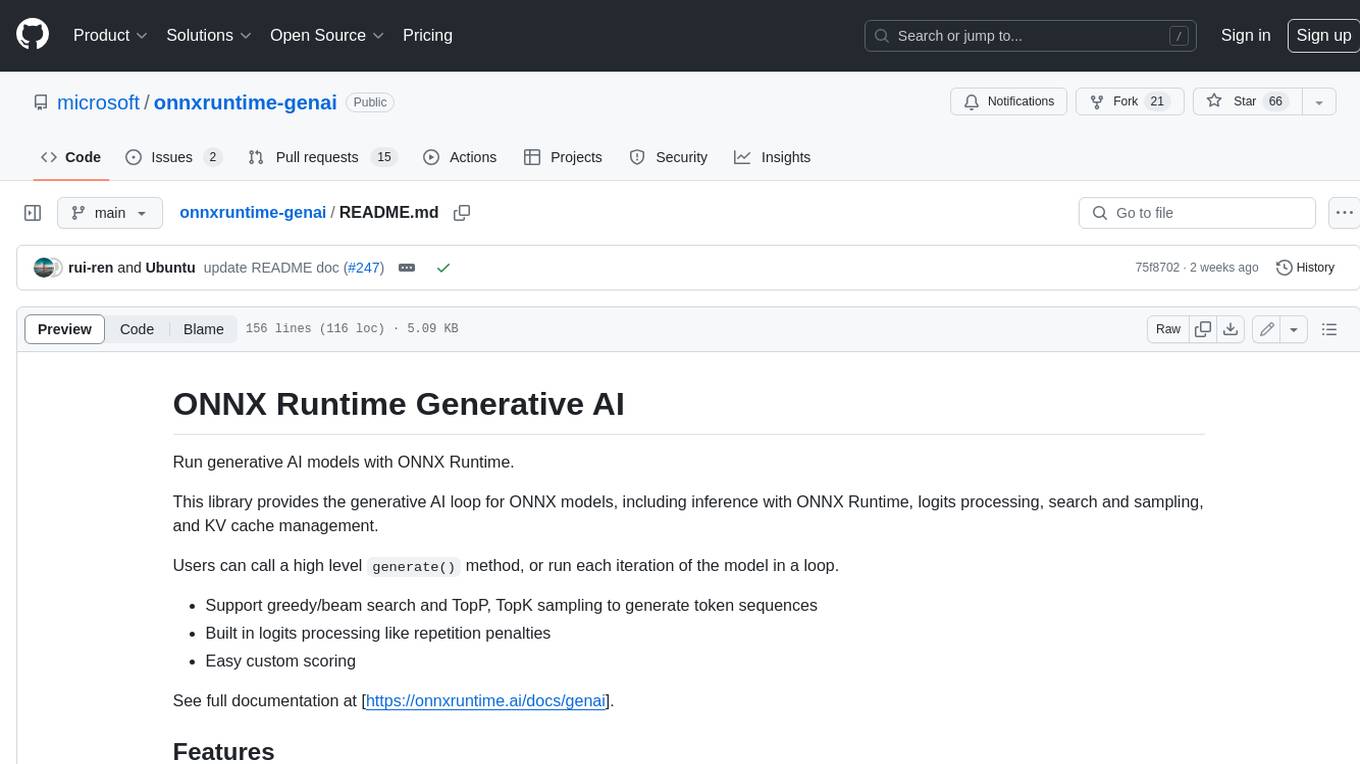
onnxruntime-genai
ONNX Runtime Generative AI is a library that provides the generative AI loop for ONNX models, including inference with ONNX Runtime, logits processing, search and sampling, and KV cache management. Users can call a high level `generate()` method, or run each iteration of the model in a loop. It supports greedy/beam search and TopP, TopK sampling to generate token sequences, has built in logits processing like repetition penalties, and allows for easy custom scoring.
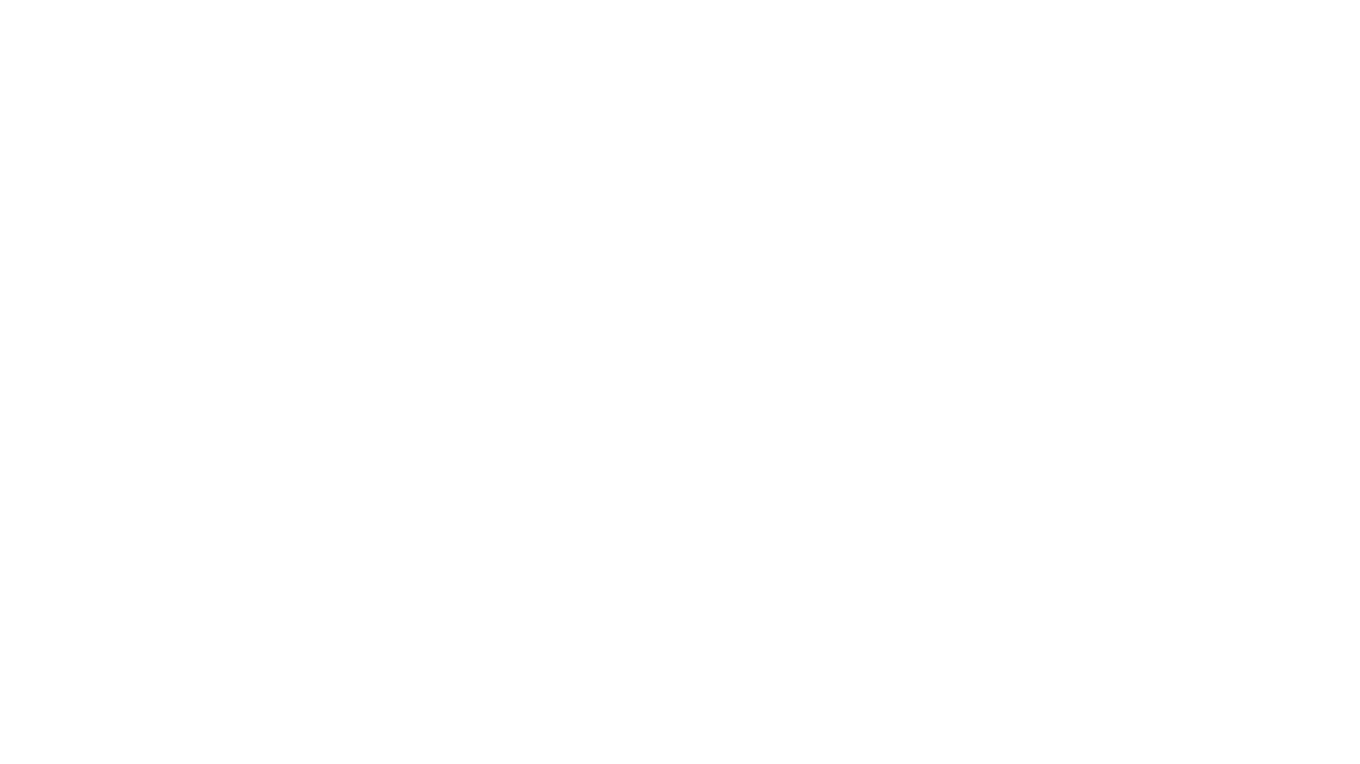
jupyter-ai
Jupyter AI connects generative AI with Jupyter notebooks. It provides a user-friendly and powerful way to explore generative AI models in notebooks and improve your productivity in JupyterLab and the Jupyter Notebook. Specifically, Jupyter AI offers: * An `%%ai` magic that turns the Jupyter notebook into a reproducible generative AI playground. This works anywhere the IPython kernel runs (JupyterLab, Jupyter Notebook, Google Colab, Kaggle, VSCode, etc.). * A native chat UI in JupyterLab that enables you to work with generative AI as a conversational assistant. * Support for a wide range of generative model providers, including AI21, Anthropic, AWS, Cohere, Gemini, Hugging Face, NVIDIA, and OpenAI. * Local model support through GPT4All, enabling use of generative AI models on consumer grade machines with ease and privacy.
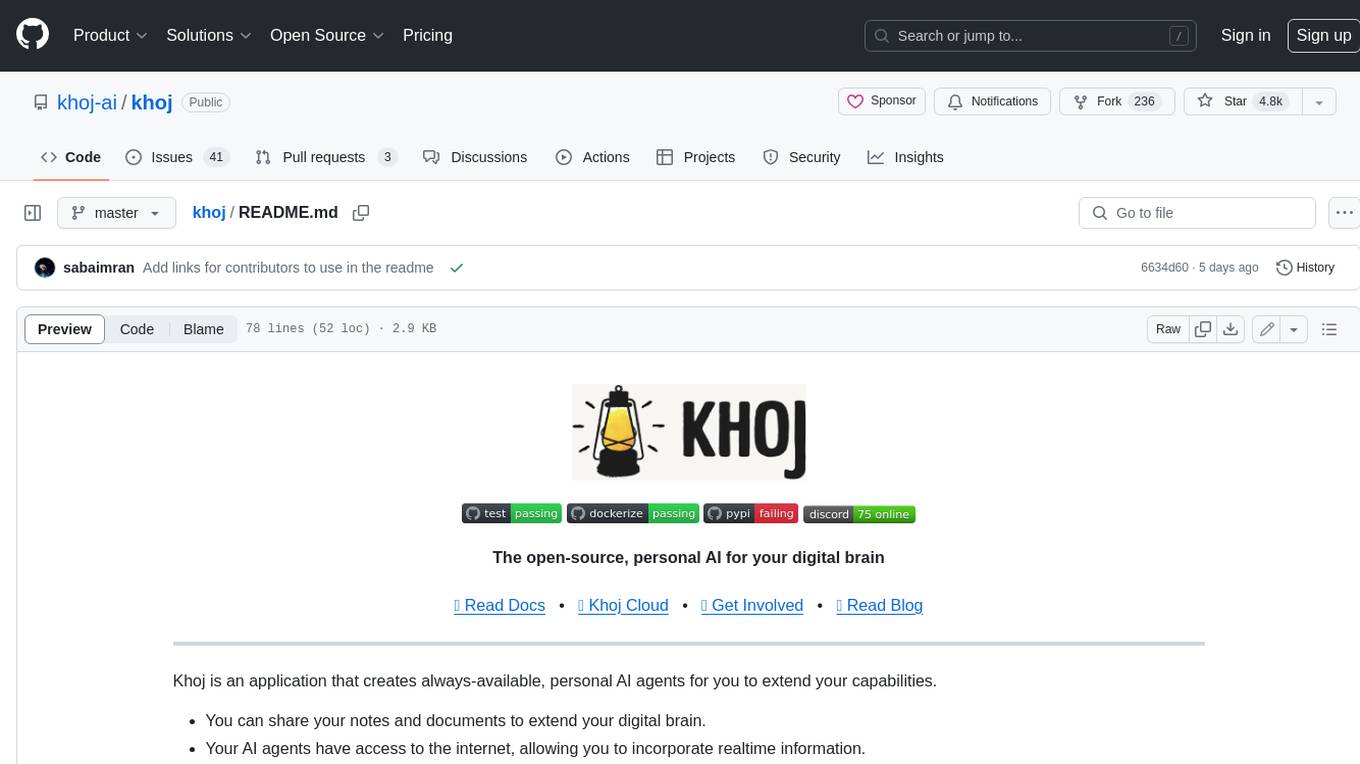
khoj
Khoj is an open-source, personal AI assistant that extends your capabilities by creating always-available AI agents. You can share your notes and documents to extend your digital brain, and your AI agents have access to the internet, allowing you to incorporate real-time information. Khoj is accessible on Desktop, Emacs, Obsidian, Web, and Whatsapp, and you can share PDF, markdown, org-mode, notion files, and GitHub repositories. You'll get fast, accurate semantic search on top of your docs, and your agents can create deeply personal images and understand your speech. Khoj is self-hostable and always will be.
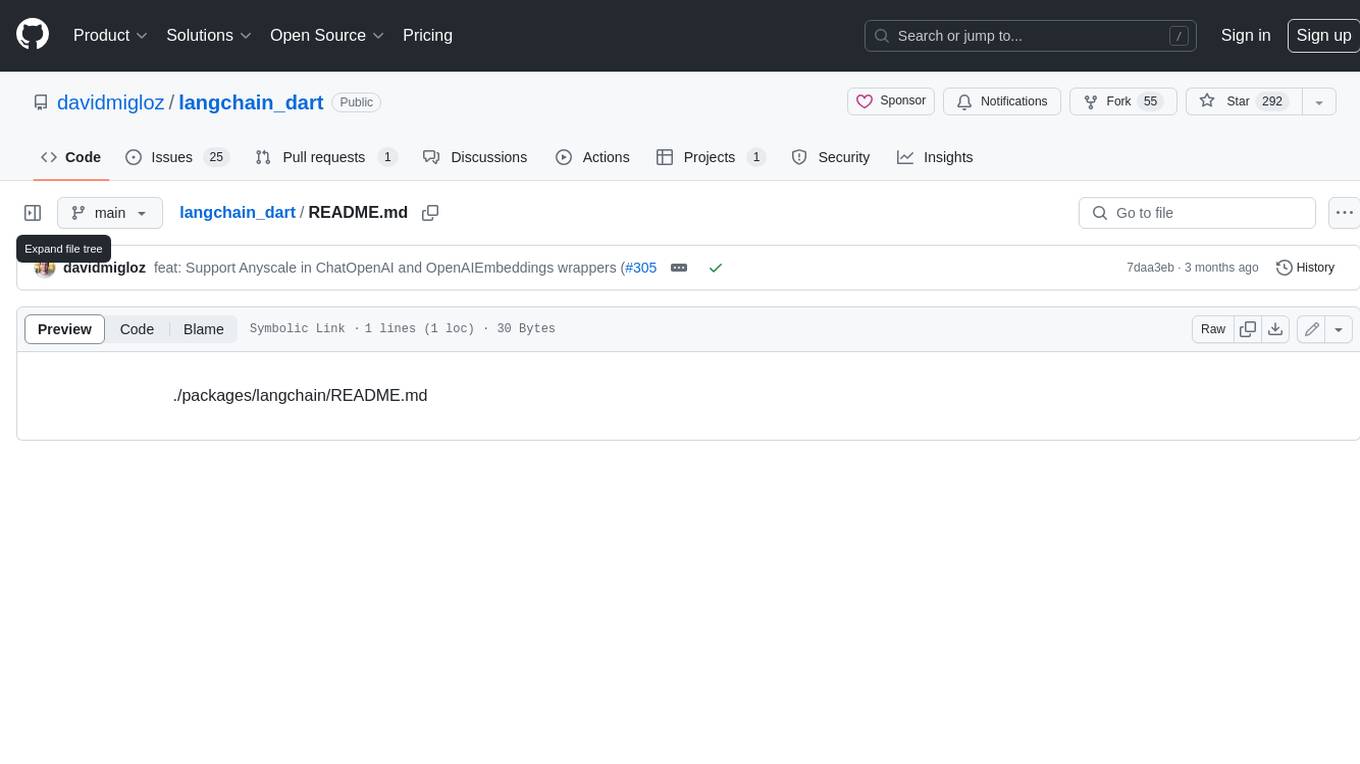
langchain_dart
LangChain.dart is a Dart port of the popular LangChain Python framework created by Harrison Chase. LangChain provides a set of ready-to-use components for working with language models and a standard interface for chaining them together to formulate more advanced use cases (e.g. chatbots, Q&A with RAG, agents, summarization, extraction, etc.). The components can be grouped into a few core modules: * **Model I/O:** LangChain offers a unified API for interacting with various LLM providers (e.g. OpenAI, Google, Mistral, Ollama, etc.), allowing developers to switch between them with ease. Additionally, it provides tools for managing model inputs (prompt templates and example selectors) and parsing the resulting model outputs (output parsers). * **Retrieval:** assists in loading user data (via document loaders), transforming it (with text splitters), extracting its meaning (using embedding models), storing (in vector stores) and retrieving it (through retrievers) so that it can be used to ground the model's responses (i.e. Retrieval-Augmented Generation or RAG). * **Agents:** "bots" that leverage LLMs to make informed decisions about which available tools (such as web search, calculators, database lookup, etc.) to use to accomplish the designated task. The different components can be composed together using the LangChain Expression Language (LCEL).
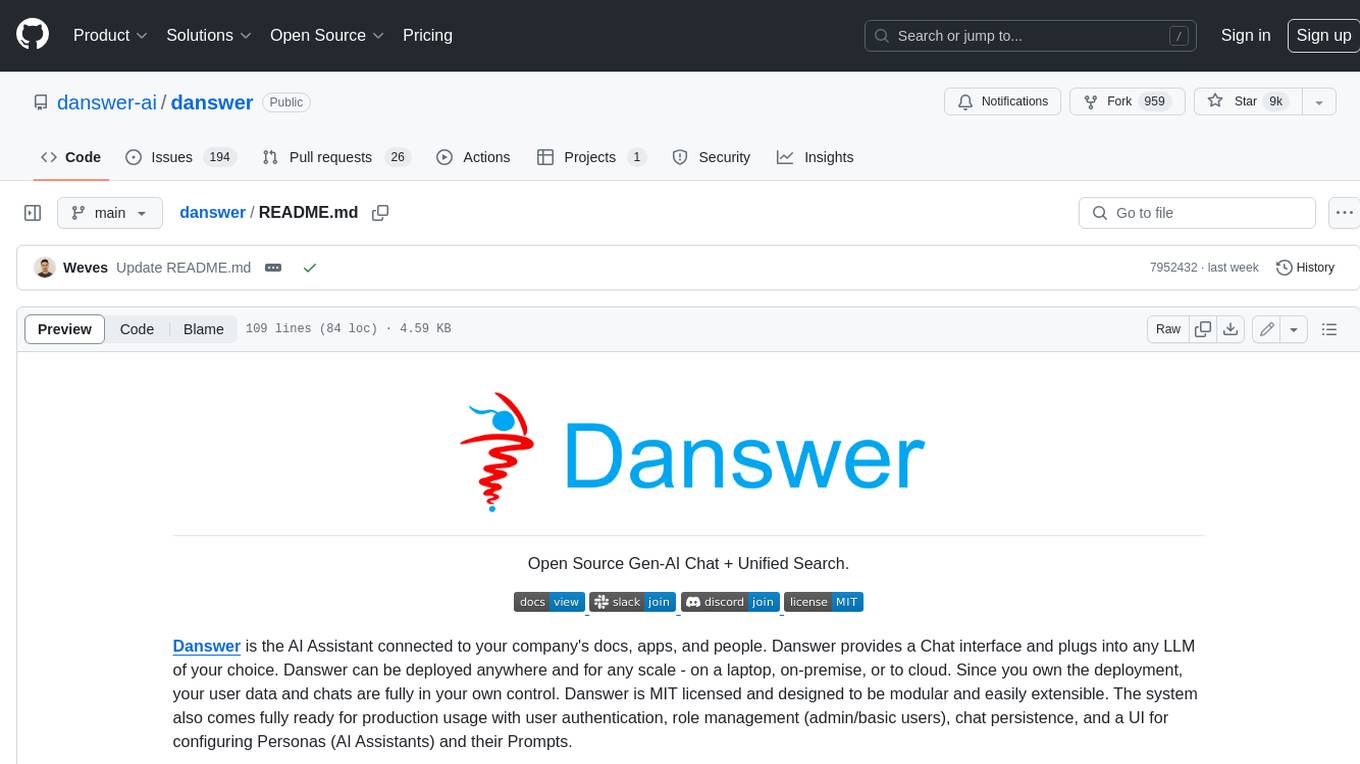
danswer
Danswer is an open-source Gen-AI Chat and Unified Search tool that connects to your company's docs, apps, and people. It provides a Chat interface and plugs into any LLM of your choice. Danswer can be deployed anywhere and for any scale - on a laptop, on-premise, or to cloud. Since you own the deployment, your user data and chats are fully in your own control. Danswer is MIT licensed and designed to be modular and easily extensible. The system also comes fully ready for production usage with user authentication, role management (admin/basic users), chat persistence, and a UI for configuring Personas (AI Assistants) and their Prompts. Danswer also serves as a Unified Search across all common workplace tools such as Slack, Google Drive, Confluence, etc. By combining LLMs and team specific knowledge, Danswer becomes a subject matter expert for the team. Imagine ChatGPT if it had access to your team's unique knowledge! It enables questions such as "A customer wants feature X, is this already supported?" or "Where's the pull request for feature Y?"
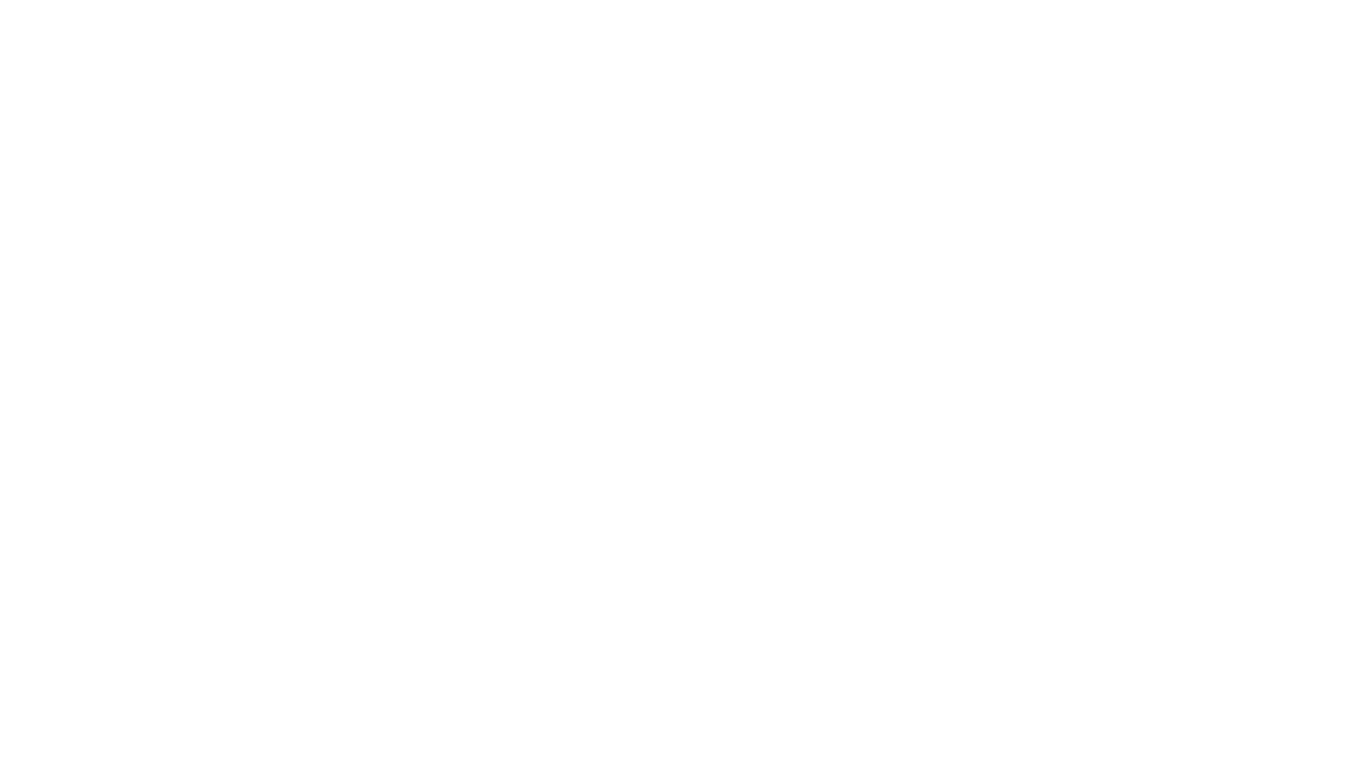
infinity
Infinity is an AI-native database designed for LLM applications, providing incredibly fast full-text and vector search capabilities. It supports a wide range of data types, including vectors, full-text, and structured data, and offers a fused search feature that combines multiple embeddings and full text. Infinity is easy to use, with an intuitive Python API and a single-binary architecture that simplifies deployment. It achieves high performance, with 0.1 milliseconds query latency on million-scale vector datasets and up to 15K QPS.
For similar jobs
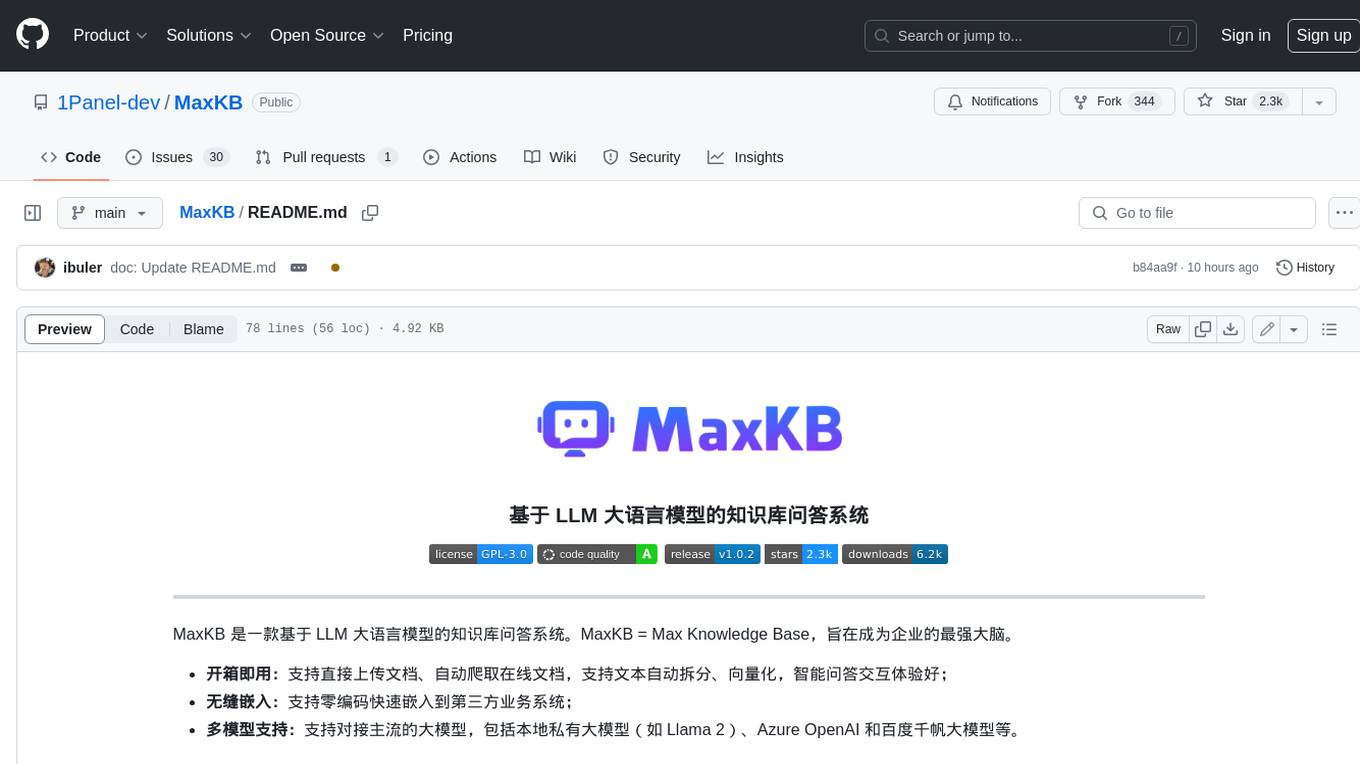
MaxKB
MaxKB is a knowledge base Q&A system based on the LLM large language model. MaxKB = Max Knowledge Base, which aims to become the most powerful brain of the enterprise.
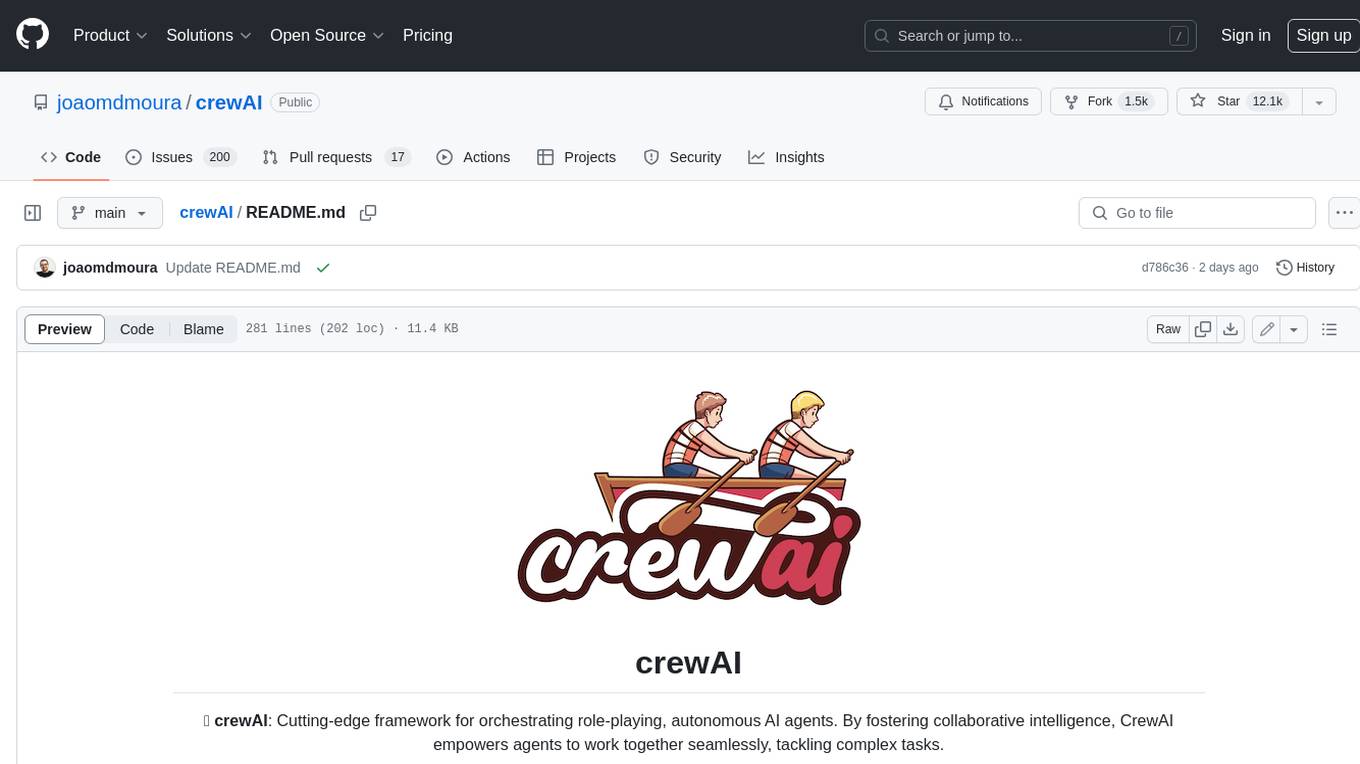
crewAI
crewAI is a cutting-edge framework for orchestrating role-playing, autonomous AI agents. By fostering collaborative intelligence, CrewAI empowers agents to work together seamlessly, tackling complex tasks. It provides a flexible and structured approach to AI collaboration, enabling users to define agents with specific roles, goals, and tools, and assign them tasks within a customizable process. crewAI supports integration with various LLMs, including OpenAI, and offers features such as autonomous task delegation, flexible task management, and output parsing. It is open-source and welcomes contributions, with a focus on improving the library based on usage data collected through anonymous telemetry.
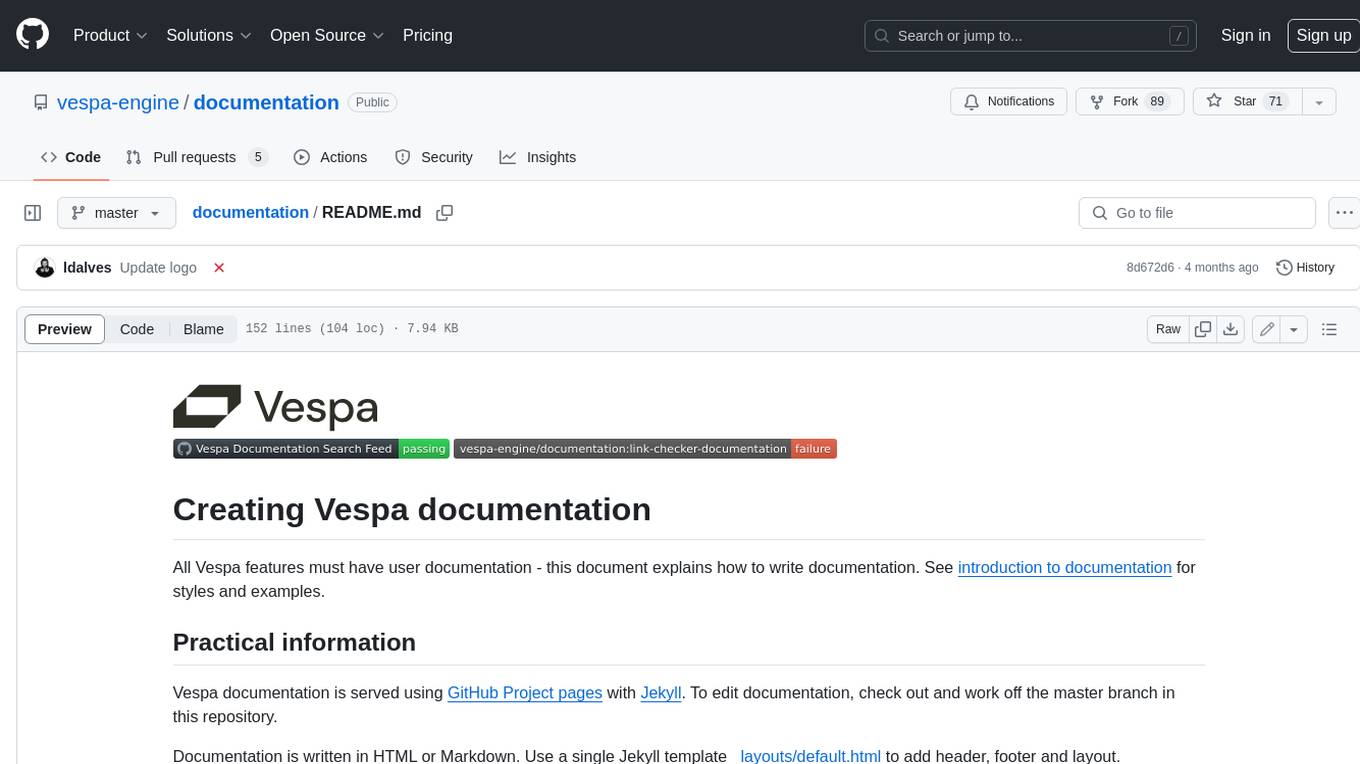
documentation
Vespa documentation is served using GitHub Project pages with Jekyll. To edit documentation, check out and work off the master branch in this repository. Documentation is written in HTML or Markdown. Use a single Jekyll template _layouts/default.html to add header, footer and layout. Install bundler, then $ bundle install $ bundle exec jekyll serve --incremental --drafts --trace to set up a local server at localhost:4000 to see the pages as they will look when served. If you get strange errors on bundle install try $ export PATH=“/usr/local/opt/[email protected]/bin:$PATH” $ export LDFLAGS=“-L/usr/local/opt/[email protected]/lib” $ export CPPFLAGS=“-I/usr/local/opt/[email protected]/include” $ export PKG_CONFIG_PATH=“/usr/local/opt/[email protected]/lib/pkgconfig” The output will highlight rendering/other problems when starting serving. Alternatively, use the docker image `jekyll/jekyll` to run the local server on Mac $ docker run -ti --rm --name doc \ --publish 4000:4000 -e JEKYLL_UID=$UID -v $(pwd):/srv/jekyll \ jekyll/jekyll jekyll serve or RHEL 8 $ podman run -it --rm --name doc -p 4000:4000 -e JEKYLL_ROOTLESS=true \ -v "$PWD":/srv/jekyll:Z docker.io/jekyll/jekyll jekyll serve The layout is written in denali.design, see _layouts/default.html for usage. Please do not add custom style sheets, as it is harder to maintain.
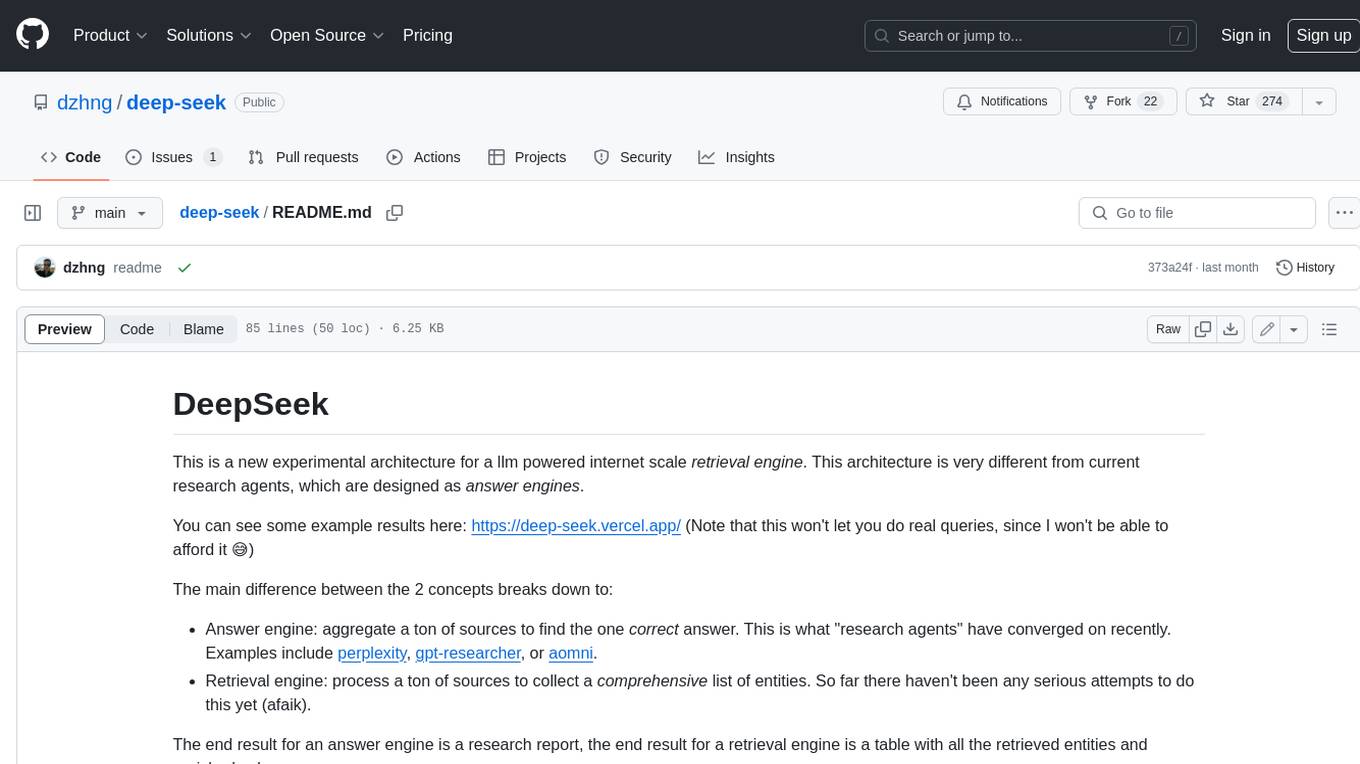
deep-seek
DeepSeek is a new experimental architecture for a large language model (LLM) powered internet-scale retrieval engine. Unlike current research agents designed as answer engines, DeepSeek aims to process a vast amount of sources to collect a comprehensive list of entities and enrich them with additional relevant data. The end result is a table with retrieved entities and enriched columns, providing a comprehensive overview of the topic. DeepSeek utilizes both standard keyword search and neural search to find relevant content, and employs an LLM to extract specific entities and their associated contents. It also includes a smaller answer agent to enrich the retrieved data, ensuring thoroughness. DeepSeek has the potential to revolutionize research and information gathering by providing a comprehensive and structured way to access information from the vastness of the internet.
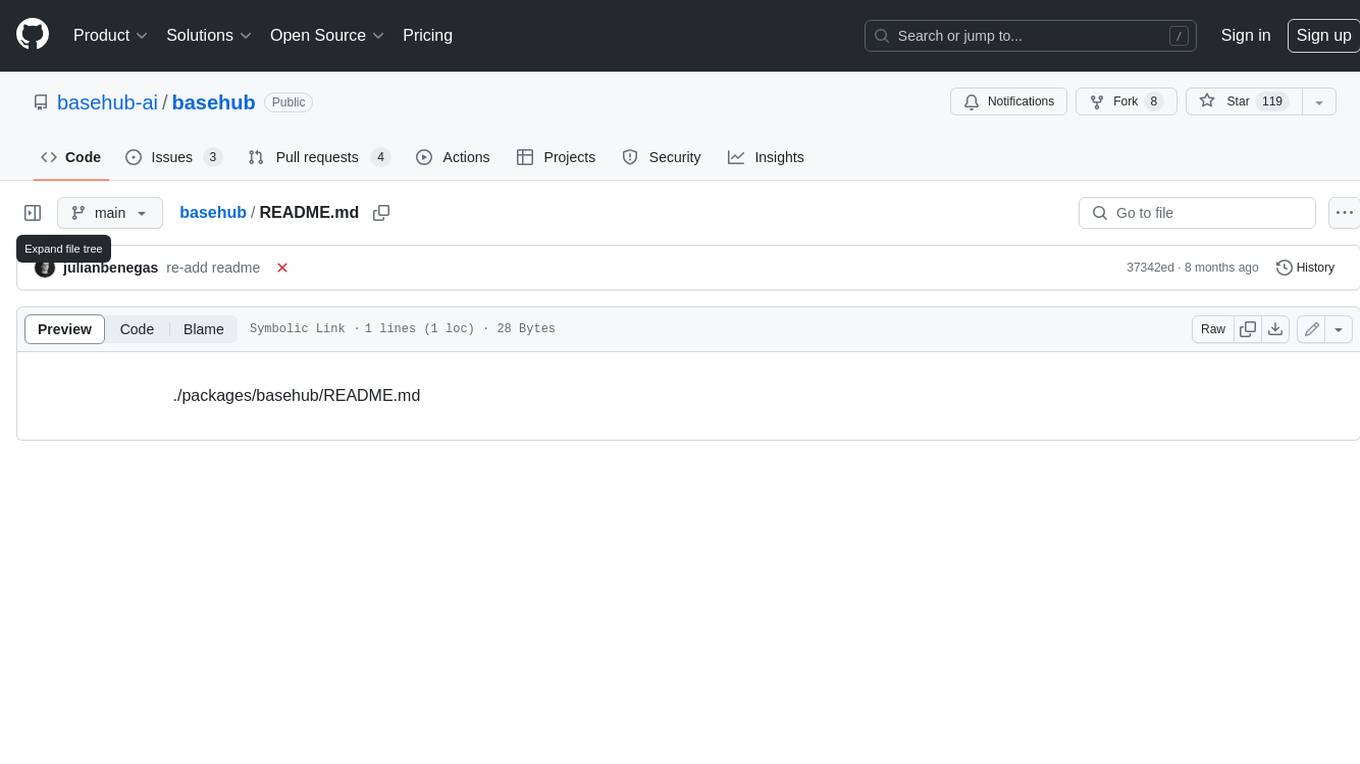
basehub
JavaScript / TypeScript SDK for BaseHub, the first AI-native content hub. **Features:** * ✨ Infers types from your BaseHub repository... _meaning IDE autocompletion works great._ * 🏎️ No dependency on graphql... _meaning your bundle is more lightweight._ * 🌐 Works everywhere `fetch` is supported... _meaning you can use it anywhere._
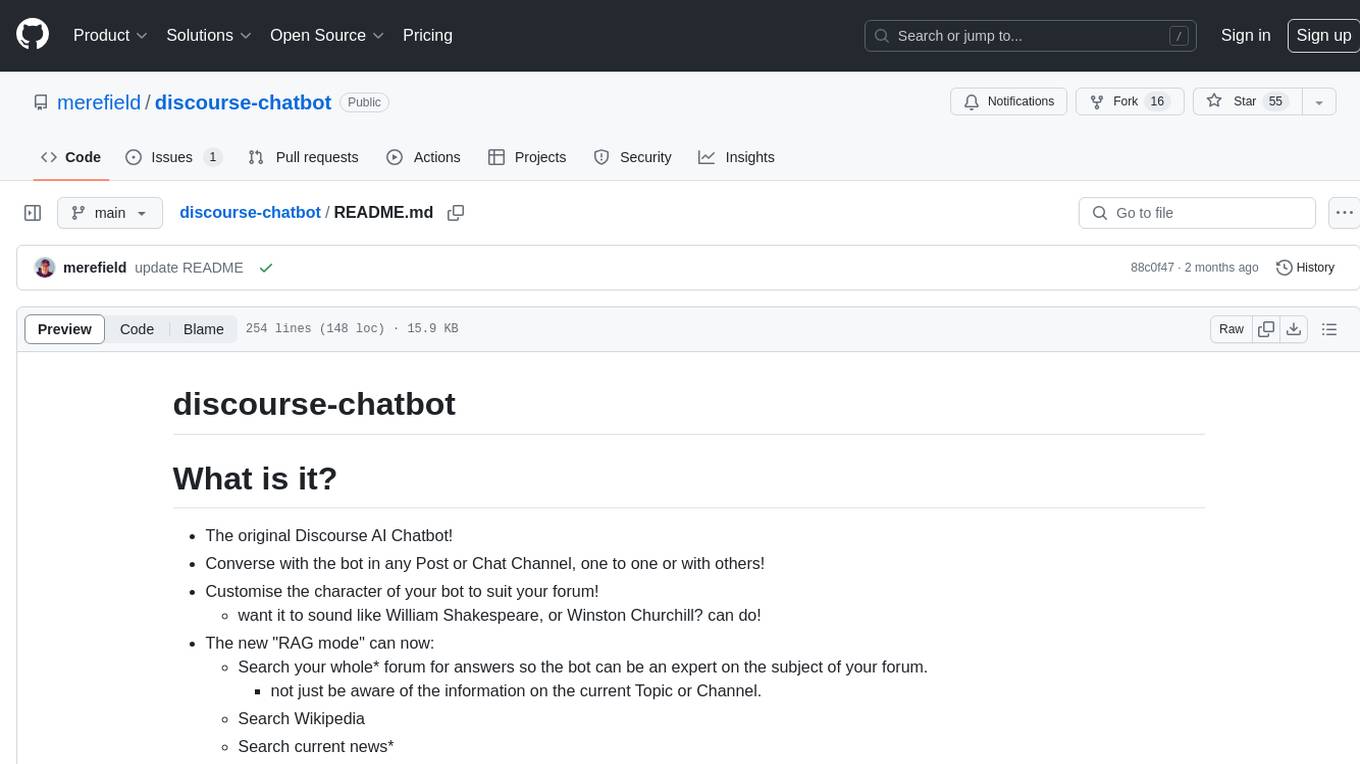
discourse-chatbot
The discourse-chatbot is an original AI chatbot for Discourse forums that allows users to converse with the bot in posts or chat channels. Users can customize the character of the bot, enable RAG mode for expert answers, search Wikipedia, news, and Google, provide market data, perform accurate math calculations, and experiment with vision support. The bot uses cutting-edge Open AI API and supports Azure and proxy server connections. It includes a quota system for access management and can be used in RAG mode or basic bot mode. The setup involves creating embeddings to make the bot aware of forum content and setting up bot access permissions based on trust levels. Users must obtain an API token from Open AI and configure group quotas to interact with the bot. The plugin is extensible to support other cloud bots and content search beyond the provided set.
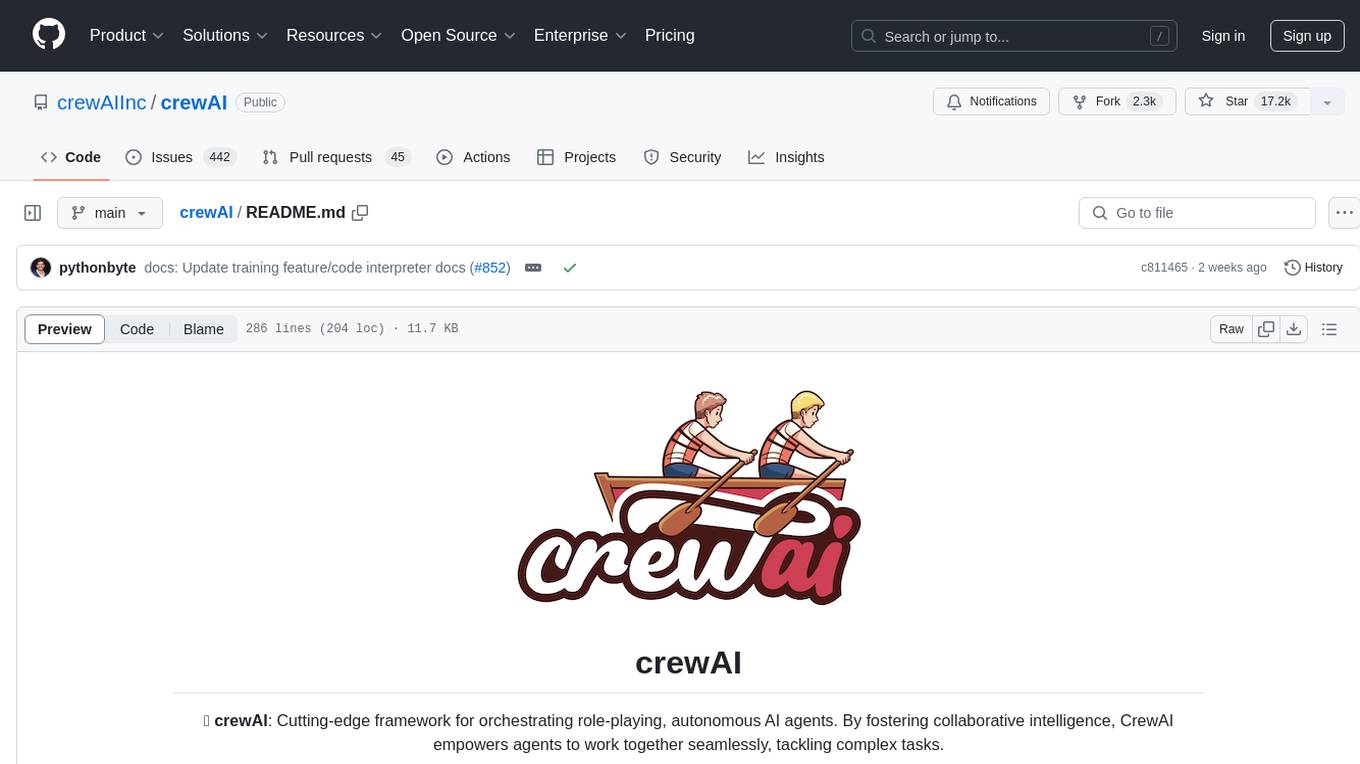
crewAI
CrewAI is a cutting-edge framework designed to orchestrate role-playing autonomous AI agents. By fostering collaborative intelligence, CrewAI empowers agents to work together seamlessly, tackling complex tasks. It enables AI agents to assume roles, share goals, and operate in a cohesive unit, much like a well-oiled crew. Whether you're building a smart assistant platform, an automated customer service ensemble, or a multi-agent research team, CrewAI provides the backbone for sophisticated multi-agent interactions. With features like role-based agent design, autonomous inter-agent delegation, flexible task management, and support for various LLMs, CrewAI offers a dynamic and adaptable solution for both development and production workflows.
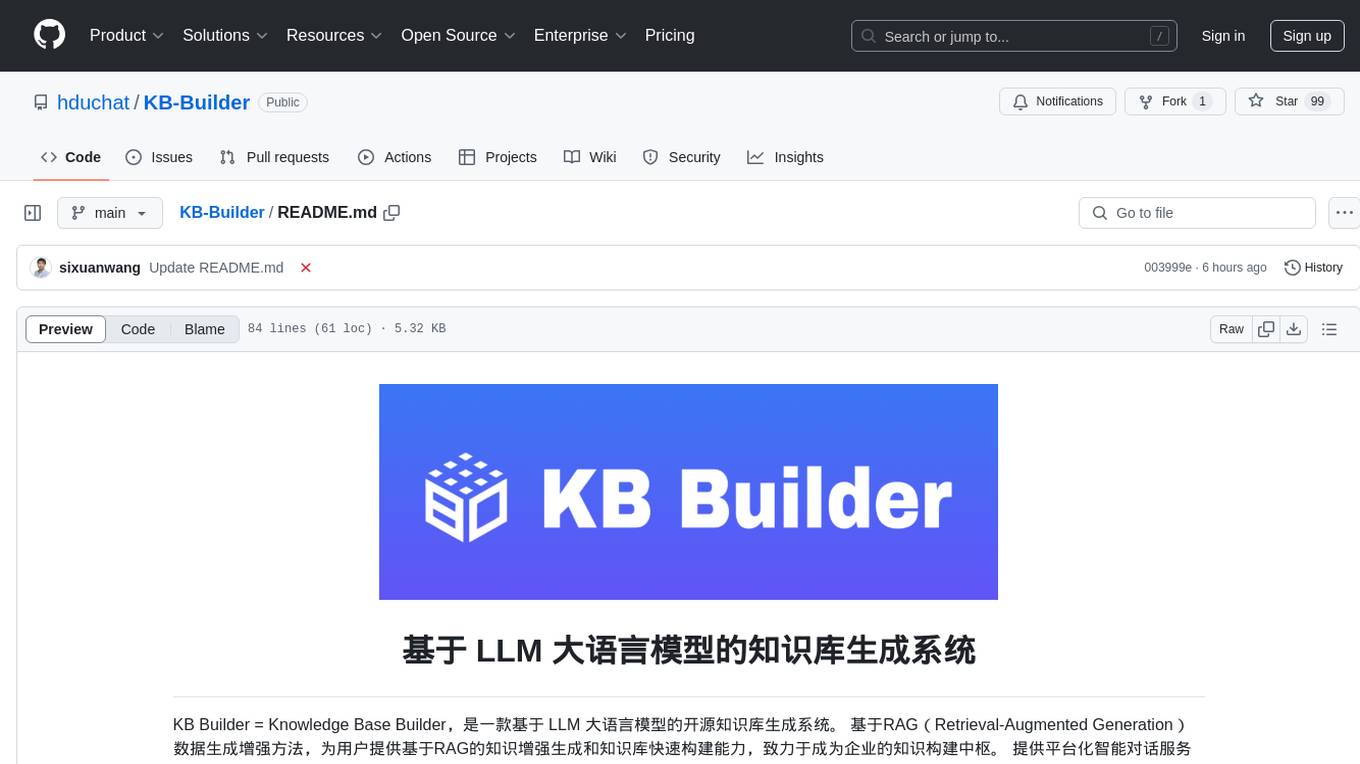
KB-Builder
KB Builder is an open-source knowledge base generation system based on the LLM large language model. It utilizes the RAG (Retrieval-Augmented Generation) data generation enhancement method to provide users with the ability to enhance knowledge generation and quickly build knowledge bases based on RAG. It aims to be the central hub for knowledge construction in enterprises, offering platform-based intelligent dialogue services and document knowledge base management functionality. Users can upload docx, pdf, txt, and md format documents and generate high-quality knowledge base question-answer pairs by invoking large models through the 'Parse Document' feature.