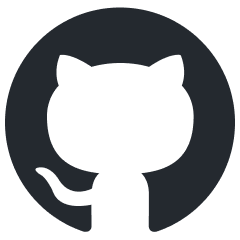
llama.rn
React Native binding of llama.cpp
Stars: 381
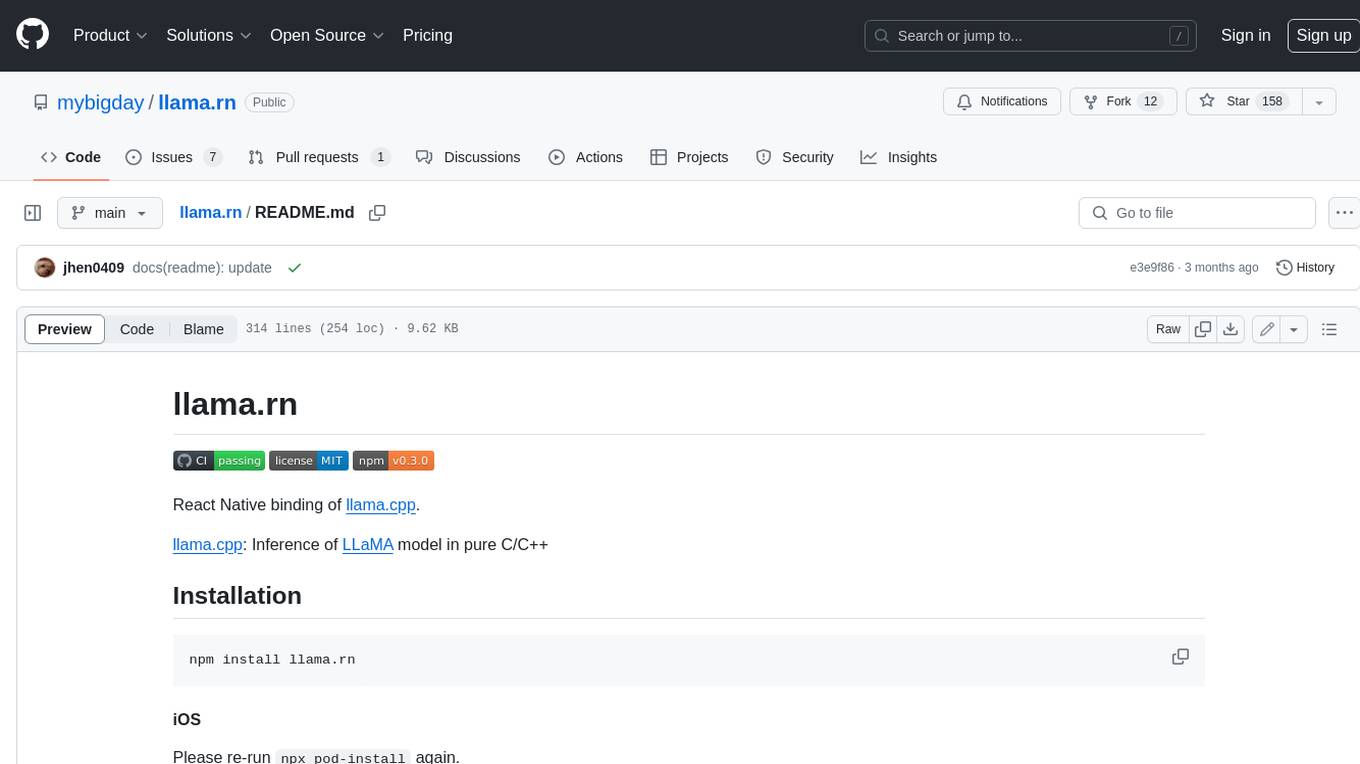
React Native binding of llama.cpp, which is an inference of LLaMA model in pure C/C++. This tool allows you to use the LLaMA model in your React Native applications for various tasks such as text completion, tokenization, detokenization, and embedding. It provides a convenient interface to interact with the LLaMA model and supports features like grammar sampling and mocking for testing purposes.
README:
React Native binding of llama.cpp.
llama.cpp: Inference of LLaMA model in pure C/C++
npm install llama.rn
Please re-run npx pod-install
again.
By default, llama.rn
will use pre-built rnllama.xcframework
for iOS. If you want to build from source, please set RNLLAMA_BUILD_FROM_SOURCE
to 1
in your Podfile.
Add proguard rule if it's enabled in project (android/app/proguard-rules.pro):
# llama.rn
-keep class com.rnllama.** { *; }
By default, llama.rn
will use pre-built libraries for Android. If you want to build from source, please set rnllamaBuildFromSource
to true
in android/gradle.properties
.
You can search HuggingFace for available models (Keyword: GGUF
).
For get a GGUF model or quantize manually, see Prepare and Quantize
section in llama.cpp.
Load model info only:
import { loadLlamaModelInfo } from 'llama.rn'
const modelPath = 'file://<path to gguf model>'
console.log('Model Info:', await loadLlamaModelInfo(modelPath))
Initialize a Llama context & do completion:
import { initLlama } from 'llama.rn'
// Initial a Llama context with the model (may take a while)
const context = await initLlama({
model: modelPath,
use_mlock: true,
n_ctx: 2048,
n_gpu_layers: 1, // > 0: enable Metal on iOS
// embedding: true, // use embedding
})
const stopWords = ['</s>', '<|end|>', '<|eot_id|>', '<|end_of_text|>', '<|im_end|>', '<|EOT|>', '<|END_OF_TURN_TOKEN|>', '<|end_of_turn|>', '<|endoftext|>']
// Do chat completion
const msgResult = await context.completion(
{
messages: [
{
role: 'system',
content: 'This is a conversation between user and assistant, a friendly chatbot.',
},
{
role: 'user',
content: 'Hello!',
},
],
n_predict: 100,
stop: stopWords,
// ...other params
},
(data) => {
// This is a partial completion callback
const { token } = data
},
)
console.log('Result:', msgResult.text)
console.log('Timings:', msgResult.timings)
// Or do text completion
const textResult = await context.completion(
{
prompt: 'This is a conversation between user and llama, a friendly chatbot. respond in simple markdown.\n\nUser: Hello!\nLlama:',
n_predict: 100,
stop: [...stopWords, 'Llama:', 'User:'],
// ...other params
},
(data) => {
// This is a partial completion callback
const { token } = data
},
)
console.log('Result:', textResult.text)
console.log('Timings:', textResult.timings)
The binding’s deisgn inspired by server.cpp example in llama.cpp, so you can map its API to LlamaContext:
-
/completion
and/chat/completions
:context.completion(params, partialCompletionCallback)
-
/tokenize
:context.tokenize(content)
-
/detokenize
:context.detokenize(tokens)
-
/embedding
:context.embedding(content)
- Other methods
context.loadSession(path)
context.saveSession(path)
context.stopCompletion()
context.release()
Please visit the Documentation for more details.
You can also visit the example to see how to use it.
GBNF (GGML BNF) is a format for defining formal grammars to constrain model outputs in llama.cpp
. For example, you can use it to force the model to generate valid JSON, or speak only in emojis.
You can see GBNF Guide for more details.
llama.rn
provided a built-in function to convert JSON Schema to GBNF:
import { initLlama, convertJsonSchemaToGrammar } from 'llama.rn'
const schema = {
/* JSON Schema, see below */
}
const context = await initLlama({
model: 'file://<path to gguf model>',
use_mlock: true,
n_ctx: 2048,
n_gpu_layers: 1, // > 0: enable Metal on iOS
// embedding: true, // use embedding
grammar: convertJsonSchemaToGrammar({
schema,
propOrder: { function: 0, arguments: 1 },
}),
})
const { text } = await context.completion({
prompt: 'Schedule a birthday party on Aug 14th 2023 at 8pm.',
})
console.log('Result:', text)
// Example output:
// {"function": "create_event","arguments":{"date": "Aug 14th 2023", "time": "8pm", "title": "Birthday Party"}}
JSON Schema example (Define function get_current_weather / create_event / image_search)
{
oneOf: [
{
type: 'object',
name: 'get_current_weather',
description: 'Get the current weather in a given location',
properties: {
function: {
const: 'get_current_weather',
},
arguments: {
type: 'object',
properties: {
location: {
type: 'string',
description: 'The city and state, e.g. San Francisco, CA',
},
unit: {
type: 'string',
enum: ['celsius', 'fahrenheit'],
},
},
required: ['location'],
},
},
},
{
type: 'object',
name: 'create_event',
description: 'Create a calendar event',
properties: {
function: {
const: 'create_event',
},
arguments: {
type: 'object',
properties: {
title: {
type: 'string',
description: 'The title of the event',
},
date: {
type: 'string',
description: 'The date of the event',
},
time: {
type: 'string',
description: 'The time of the event',
},
},
required: ['title', 'date', 'time'],
},
},
},
{
type: 'object',
name: 'image_search',
description: 'Search for an image',
properties: {
function: {
const: 'image_search',
},
arguments: {
type: 'object',
properties: {
query: {
type: 'string',
description: 'The search query',
},
},
required: ['query'],
},
},
},
],
}
Converted GBNF looks like
space ::= " "?
0-function ::= "\"get_current_weather\""
string ::= "\"" (
[^"\\] |
"\\" (["\\/bfnrt] | "u" [0-9a-fA-F] [0-9a-fA-F] [0-9a-fA-F] [0-9a-fA-F])
)* "\"" space
0-arguments-unit ::= "\"celsius\"" | "\"fahrenheit\""
0-arguments ::= "{" space "\"location\"" space ":" space string "," space "\"unit\"" space ":" space 0-arguments-unit "}" space
0 ::= "{" space "\"function\"" space ":" space 0-function "," space "\"arguments\"" space ":" space 0-arguments "}" space
1-function ::= "\"create_event\""
1-arguments ::= "{" space "\"date\"" space ":" space string "," space "\"time\"" space ":" space string "," space "\"title\"" space ":" space string "}" space
1 ::= "{" space "\"function\"" space ":" space 1-function "," space "\"arguments\"" space ":" space 1-arguments "}" space
2-function ::= "\"image_search\""
2-arguments ::= "{" space "\"query\"" space ":" space string "}" space
2 ::= "{" space "\"function\"" space ":" space 2-function "," space "\"arguments\"" space ":" space 2-arguments "}" space
root ::= 0 | 1 | 2
We have provided a mock version of llama.rn
for testing purpose you can use on Jest:
jest.mock('llama.rn', () => require('llama.rn/jest/mock'))
iOS:
- The Extended Virtual Addressing capability is recommended to enable on iOS project.
- Metal:
- We have tested to know some devices is not able to use Metal (GPU) due to llama.cpp used SIMD-scoped operation, you can check if your device is supported in Metal feature set tables, Apple7 GPU will be the minimum requirement.
- It's also not supported in iOS simulator due to this limitation, we used constant buffers more than 14.
Android:
- Currently only supported arm64-v8a / x86_64 platform, this means you can't initialize a context on another platforms. The 64-bit platform are recommended because it can allocate more memory for the model.
- No integrated any GPU backend yet.
See the contributing guide to learn how to contribute to the repository and the development workflow.
- BRICKS: Our product for building interactive signage in simple way. We provide LLM functions as Generator LLM/Assistant.
- ChatterUI: Simple frontend for LLMs built in react-native.
- PocketPal AI: An app that brings language models directly to your phone.
-
llama.node: An another Node.js binding of
llama.cpp
but made API same asllama.rn
.
MIT
Made with create-react-native-library
Built and maintained by BRICKS.
For Tasks:
Click tags to check more tools for each tasksFor Jobs:
Alternative AI tools for llama.rn
Similar Open Source Tools
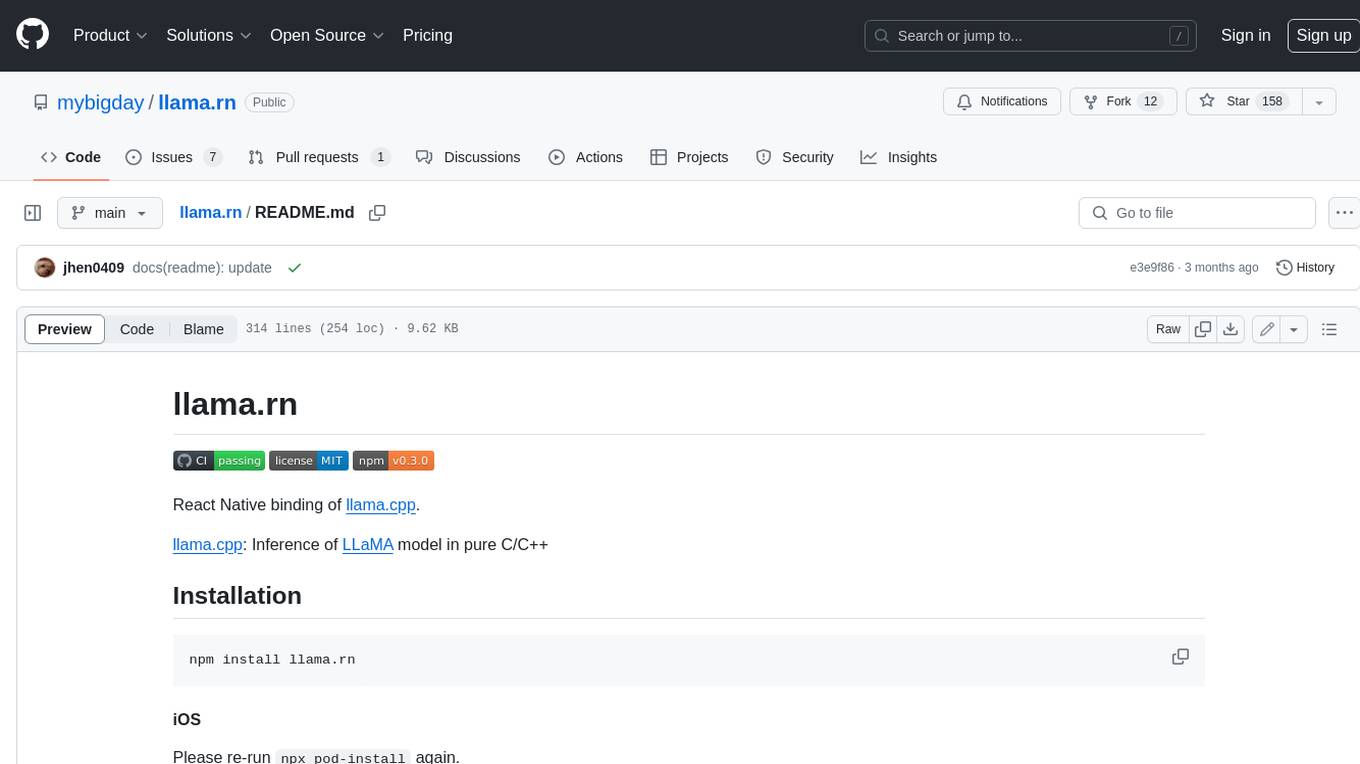
llama.rn
React Native binding of llama.cpp, which is an inference of LLaMA model in pure C/C++. This tool allows you to use the LLaMA model in your React Native applications for various tasks such as text completion, tokenization, detokenization, and embedding. It provides a convenient interface to interact with the LLaMA model and supports features like grammar sampling and mocking for testing purposes.
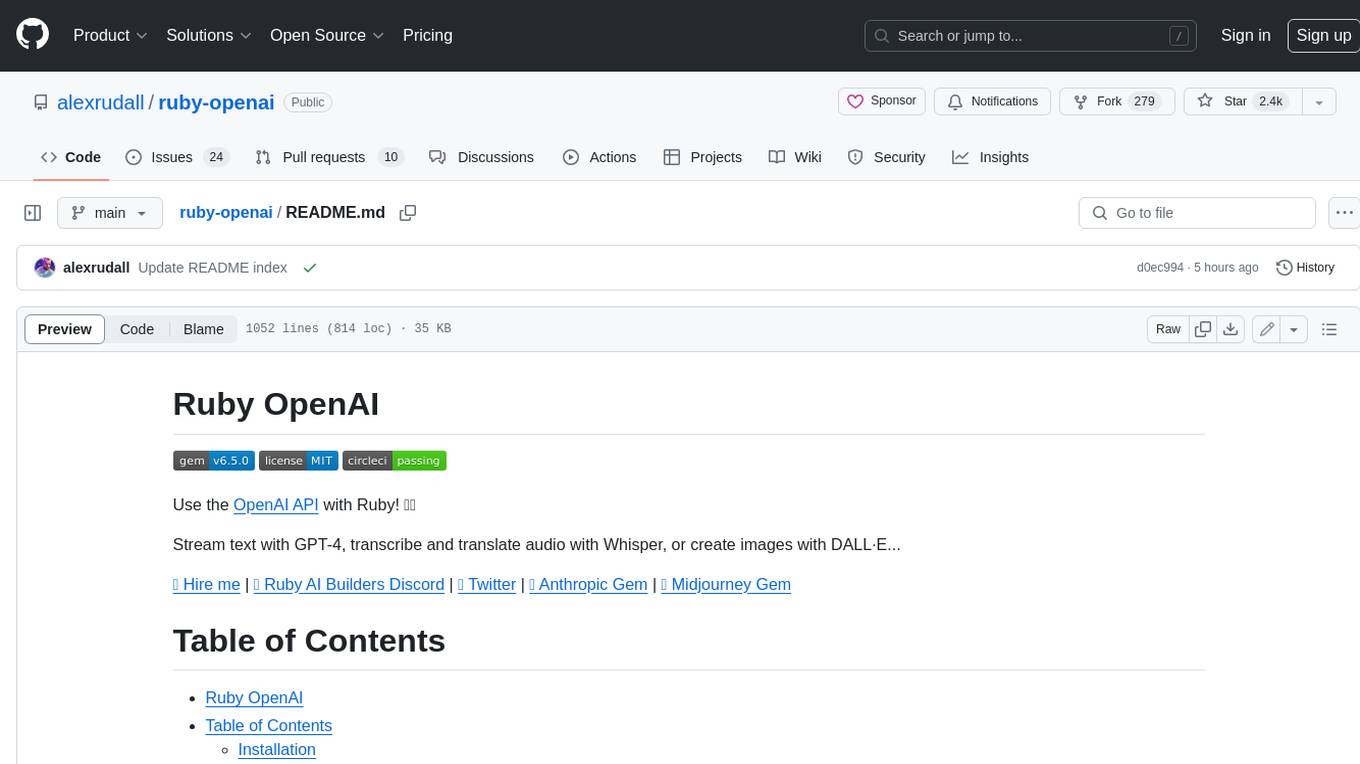
ruby-openai
Use the OpenAI API with Ruby! 🤖🩵 Stream text with GPT-4, transcribe and translate audio with Whisper, or create images with DALL·E... Hire me | 🎮 Ruby AI Builders Discord | 🐦 Twitter | 🧠 Anthropic Gem | 🚂 Midjourney Gem ## Table of Contents * Ruby OpenAI * Table of Contents * Installation * Bundler * Gem install * Usage * Quickstart * With Config * Custom timeout or base URI * Extra Headers per Client * Logging * Errors * Faraday middleware * Azure * Ollama * Counting Tokens * Models * Examples * Chat * Streaming Chat * Vision * JSON Mode * Functions * Edits * Embeddings * Batches * Files * Finetunes * Assistants * Threads and Messages * Runs * Runs involving function tools * Image Generation * DALL·E 2 * DALL·E 3 * Image Edit * Image Variations * Moderations * Whisper * Translate * Transcribe * Speech * Errors * Development * Release * Contributing * License * Code of Conduct
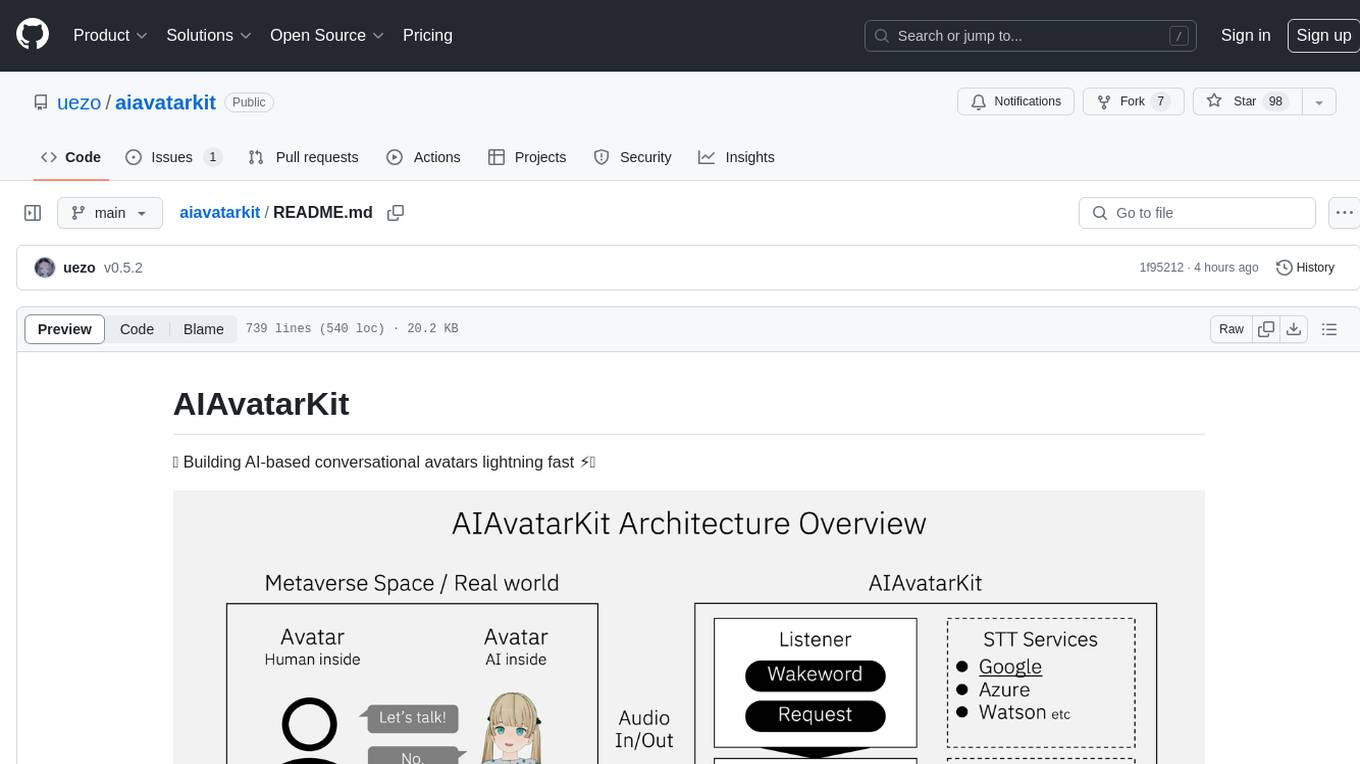
aiavatarkit
AIAvatarKit is a tool for building AI-based conversational avatars quickly. It supports various platforms like VRChat and cluster, along with real-world devices. The tool is extensible, allowing unlimited capabilities based on user needs. It requires VOICEVOX API, Google or Azure Speech Services API keys, and Python 3.10. Users can start conversations out of the box and enjoy seamless interactions with the avatars.
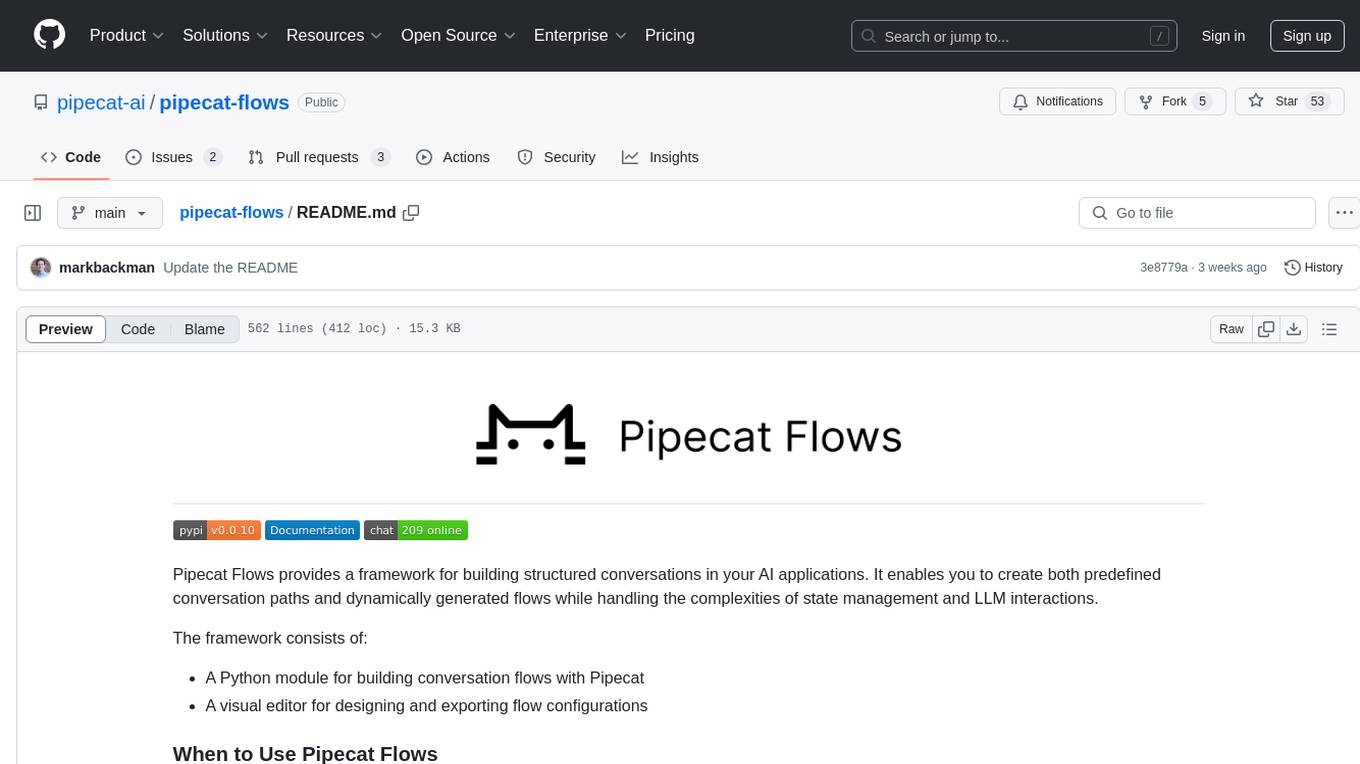
pipecat-flows
Pipecat Flows is a framework designed for building structured conversations in AI applications. It allows users to create both predefined conversation paths and dynamically generated flows, handling state management and LLM interactions. The framework includes a Python module for building conversation flows and a visual editor for designing and exporting flow configurations. Pipecat Flows is suitable for scenarios such as customer service scripts, intake forms, personalized experiences, and complex decision trees.
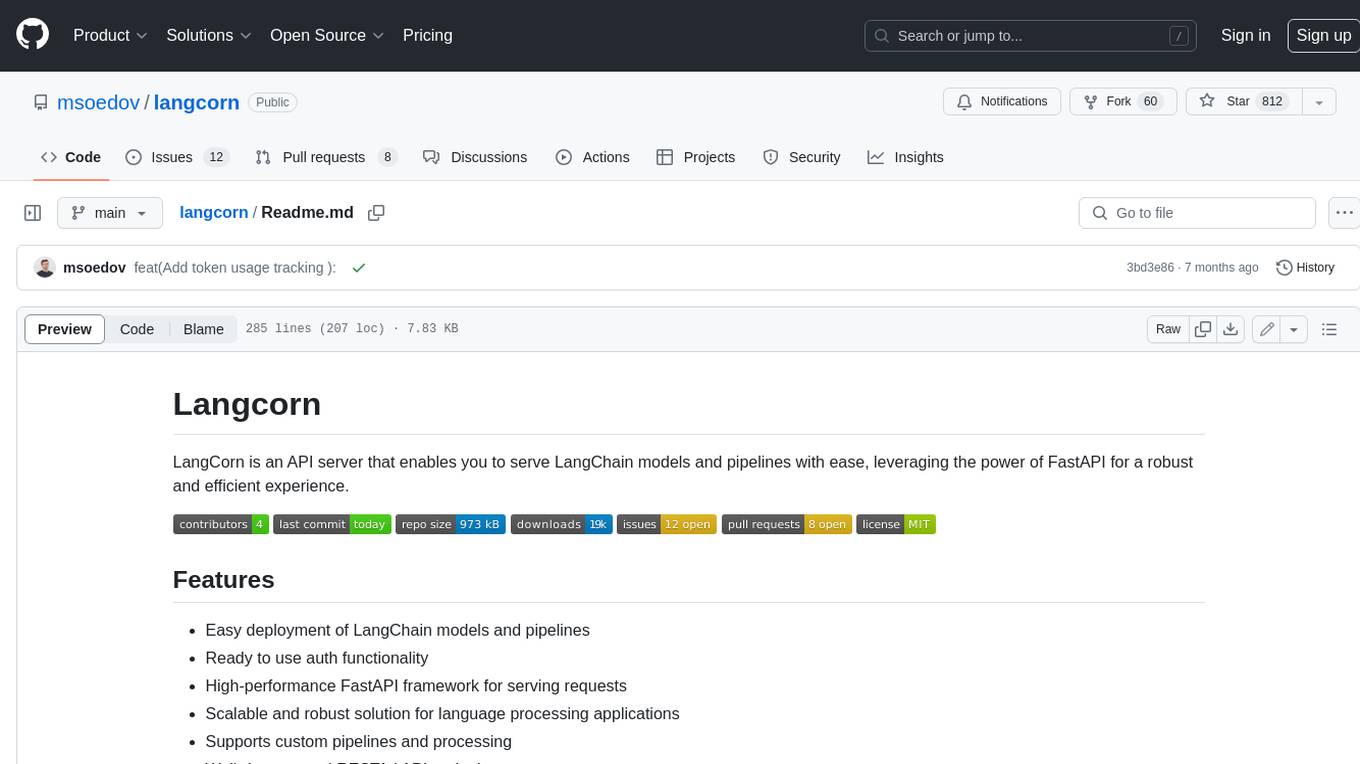
langcorn
LangCorn is an API server that enables you to serve LangChain models and pipelines with ease, leveraging the power of FastAPI for a robust and efficient experience. It offers features such as easy deployment of LangChain models and pipelines, ready-to-use authentication functionality, high-performance FastAPI framework for serving requests, scalability and robustness for language processing applications, support for custom pipelines and processing, well-documented RESTful API endpoints, and asynchronous processing for faster response times.
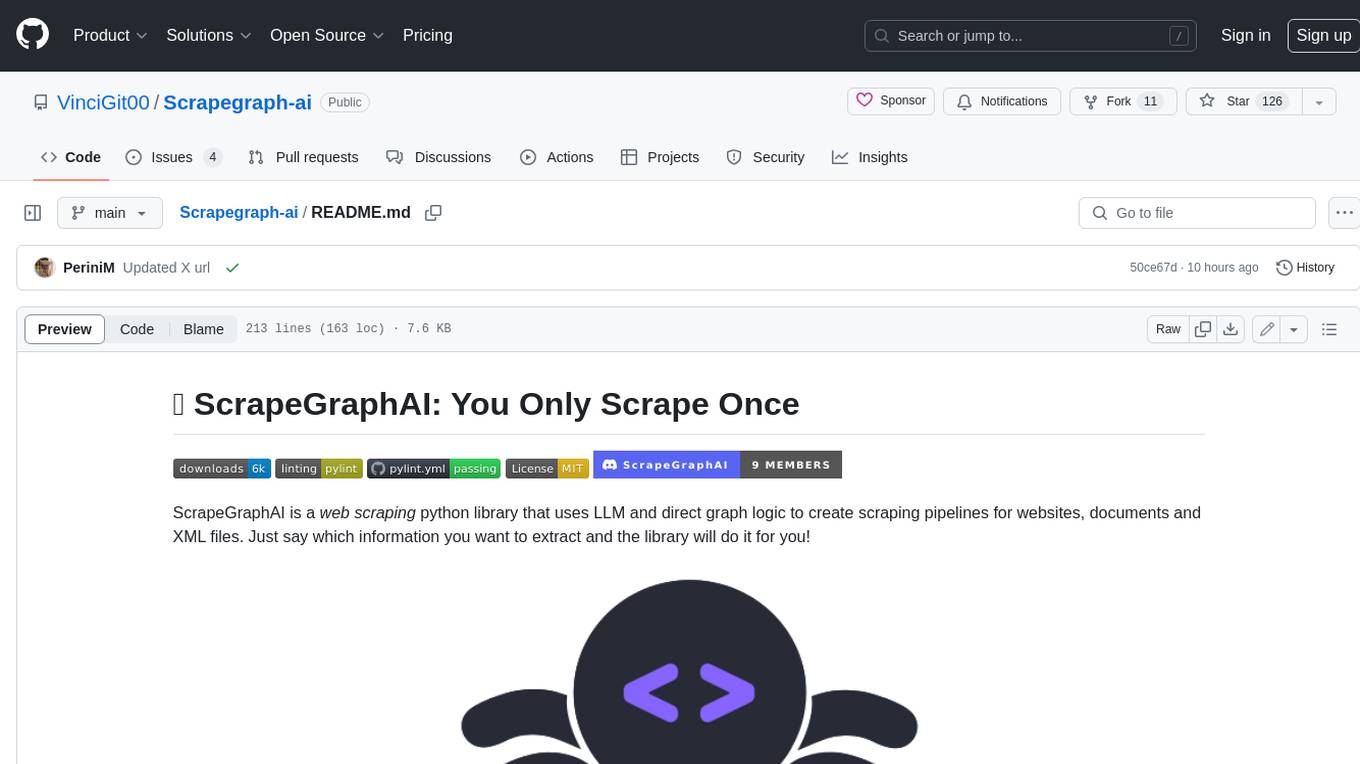
Scrapegraph-ai
ScrapeGraphAI is a Python library that uses Large Language Models (LLMs) and direct graph logic to create web scraping pipelines for websites, documents, and XML files. It allows users to extract specific information from web pages by providing a prompt describing the desired data. ScrapeGraphAI supports various LLMs, including Ollama, OpenAI, Gemini, and Docker, enabling users to choose the most suitable model for their needs. The library provides a user-friendly interface through its `SmartScraper` class, which simplifies the process of building and executing scraping pipelines. ScrapeGraphAI is open-source and available on GitHub, with extensive documentation and examples to guide users. It is particularly useful for researchers and data scientists who need to extract structured data from web pages for analysis and exploration.
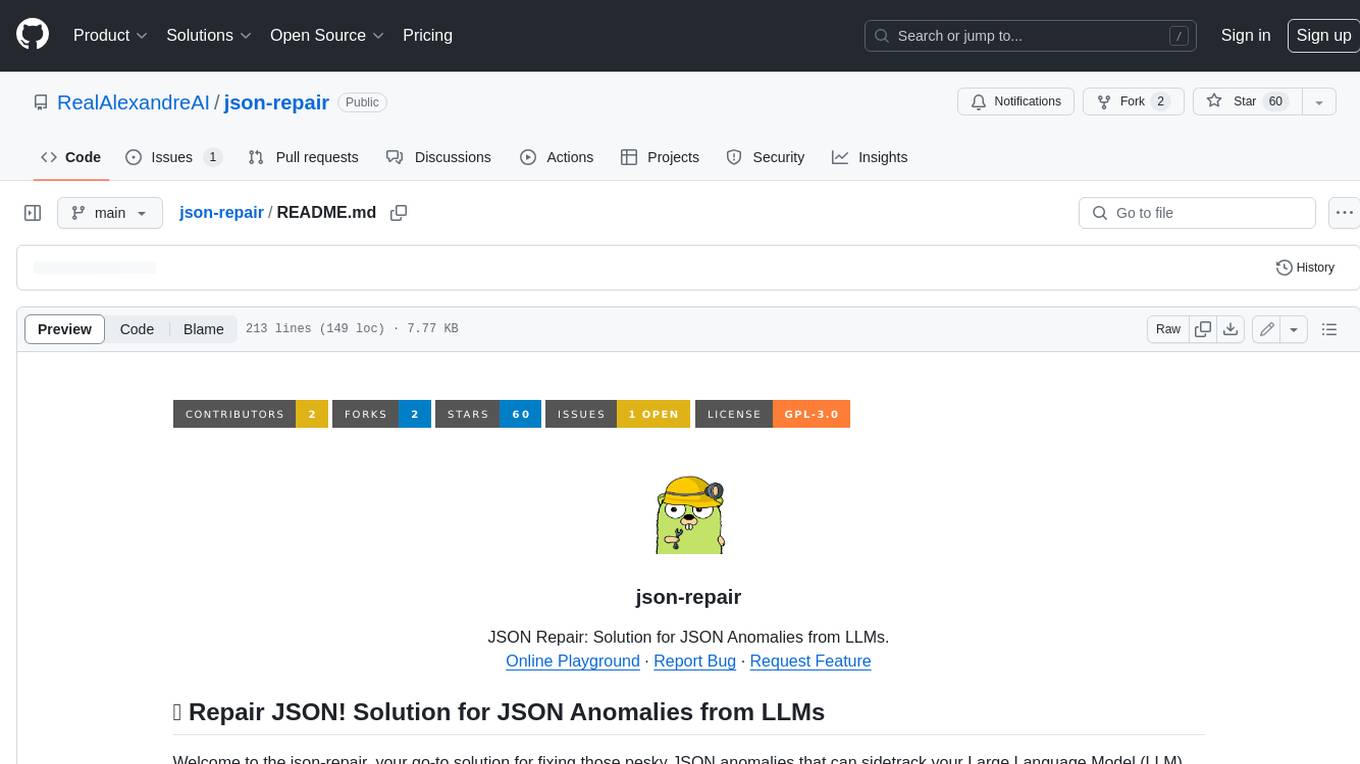
json-repair
JSON Repair is a toolkit designed to address JSON anomalies that can arise from Large Language Models (LLMs). It offers a comprehensive solution for repairing JSON strings, ensuring accuracy and reliability in your data processing. With its user-friendly interface and extensive capabilities, JSON Repair empowers developers to seamlessly integrate JSON repair into their workflows.
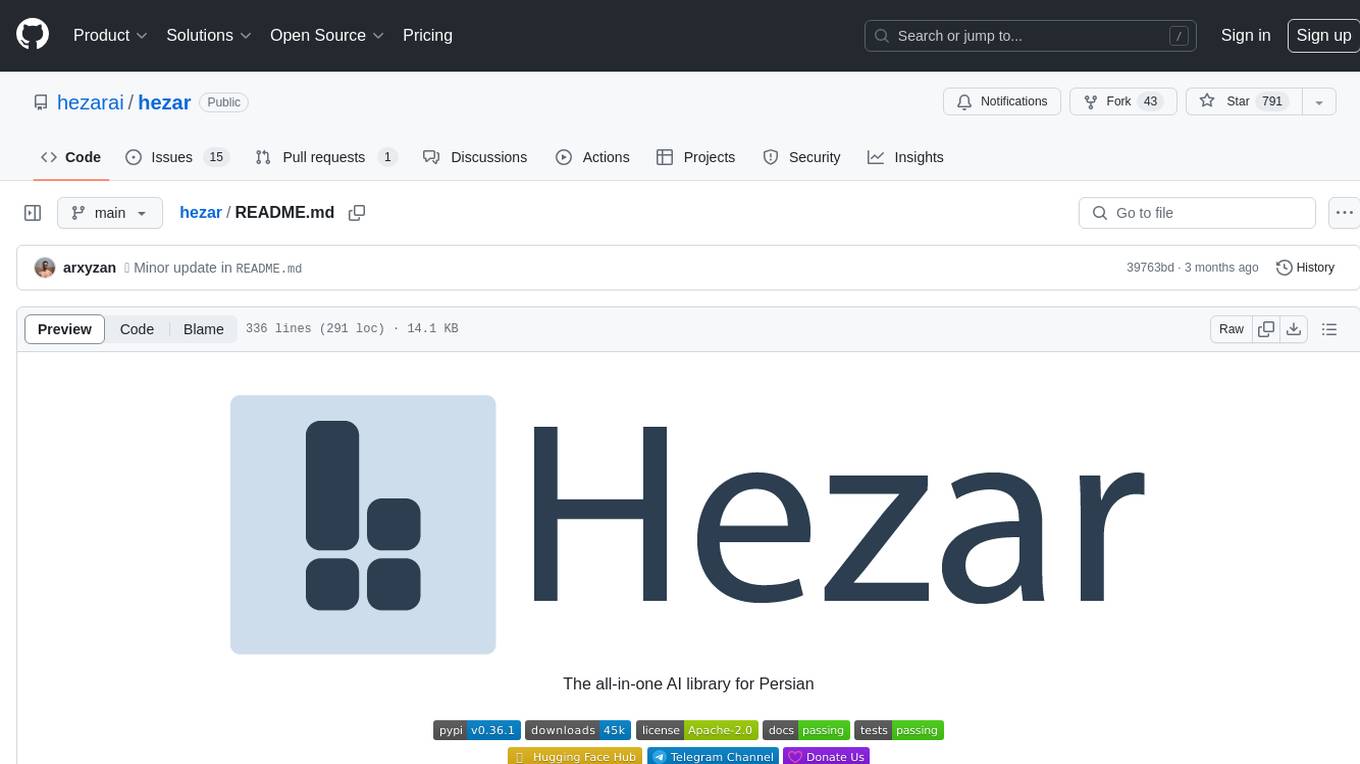
hezar
Hezar is an all-in-one AI library designed specifically for the Persian community. It brings together various AI models and tools, making it easy to use AI with just a few lines of code. The library seamlessly integrates with Hugging Face Hub, offering a developer-friendly interface and task-based model interface. In addition to models, Hezar provides tools like word embeddings, tokenizers, feature extractors, and more. It also includes supplementary ML tools for deployment, benchmarking, and optimization.
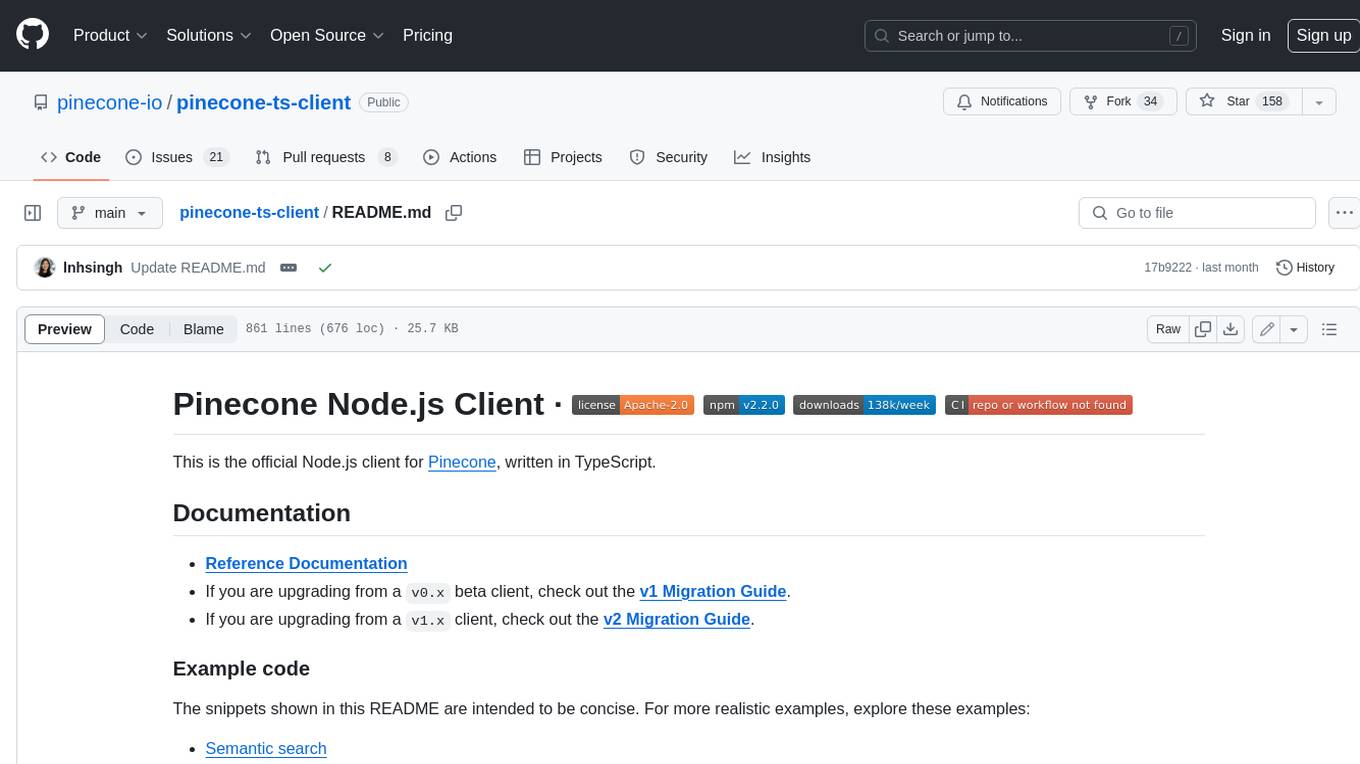
pinecone-ts-client
The official Node.js client for Pinecone, written in TypeScript. This client library provides a high-level interface for interacting with the Pinecone vector database service. With this client, you can create and manage indexes, upsert and query vector data, and perform other operations related to vector search and retrieval. The client is designed to be easy to use and provides a consistent and idiomatic experience for Node.js developers. It supports all the features and functionality of the Pinecone API, making it a comprehensive solution for building vector-powered applications in Node.js.
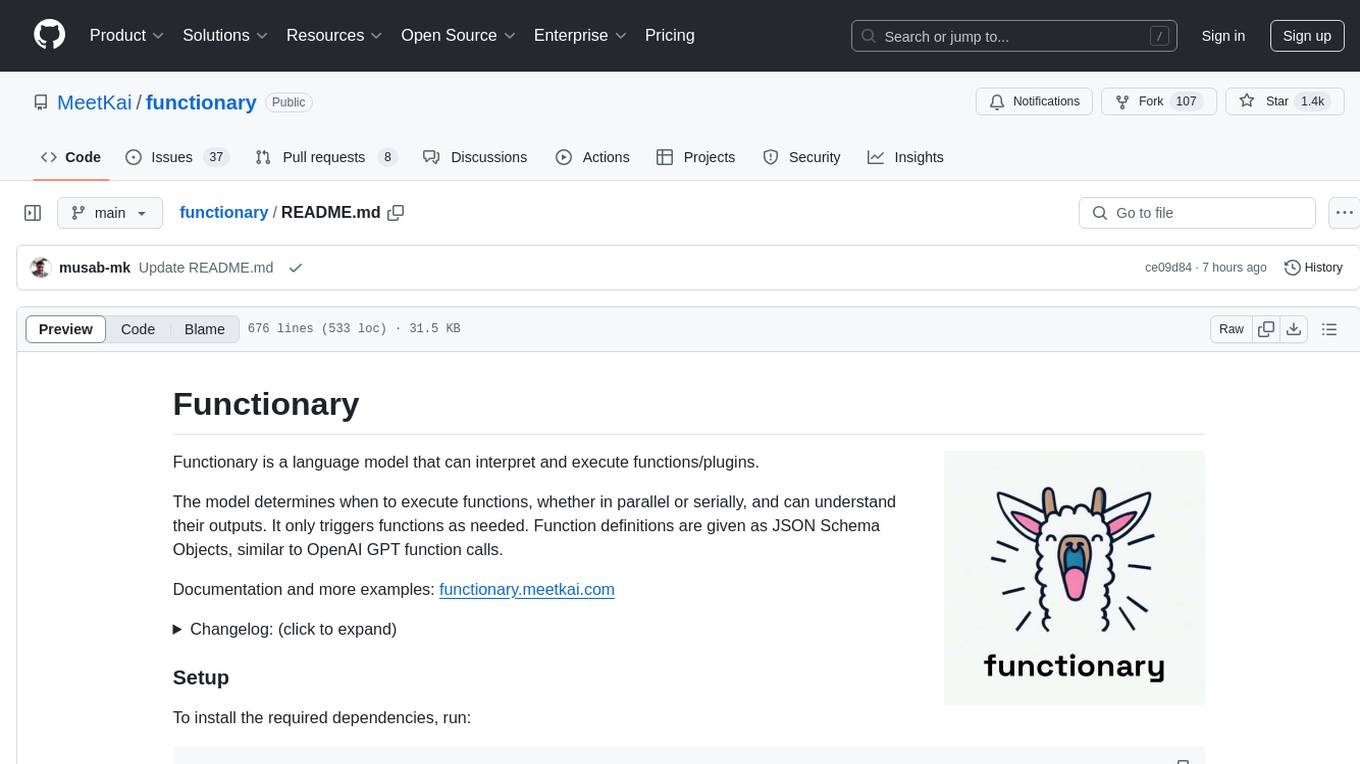
functionary
Functionary is a language model that interprets and executes functions/plugins. It determines when to execute functions, whether in parallel or serially, and understands their outputs. Function definitions are given as JSON Schema Objects, similar to OpenAI GPT function calls. It offers documentation and examples on functionary.meetkai.com. The newest model, meetkai/functionary-medium-v3.1, is ranked 2nd in the Berkeley Function-Calling Leaderboard. Functionary supports models with different context lengths and capabilities for function calling and code interpretation. It also provides grammar sampling for accurate function and parameter names. Users can deploy Functionary models serverlessly using Modal.com.
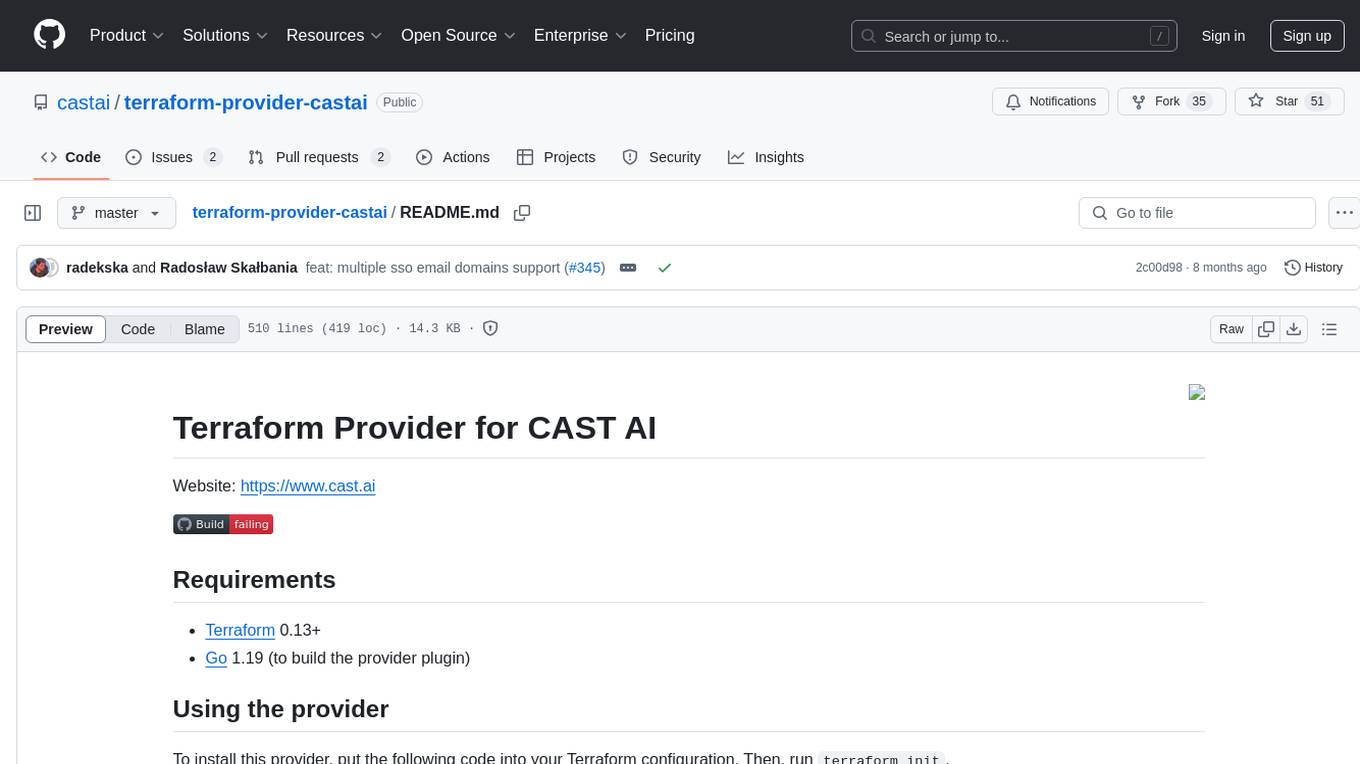
terraform-provider-castai
Terraform Provider for CAST AI is a tool that allows users to manage their CAST AI resources using Terraform. It provides a seamless integration between Terraform and CAST AI platform, enabling users to define and manage their infrastructure as code. The provider supports various features such as setting up cluster configurations, managing node templates, and configuring autoscaler policies. Users can easily install the provider, pass API keys, and leverage the provider's functionalities to automate the deployment and management of their CAST AI resources.
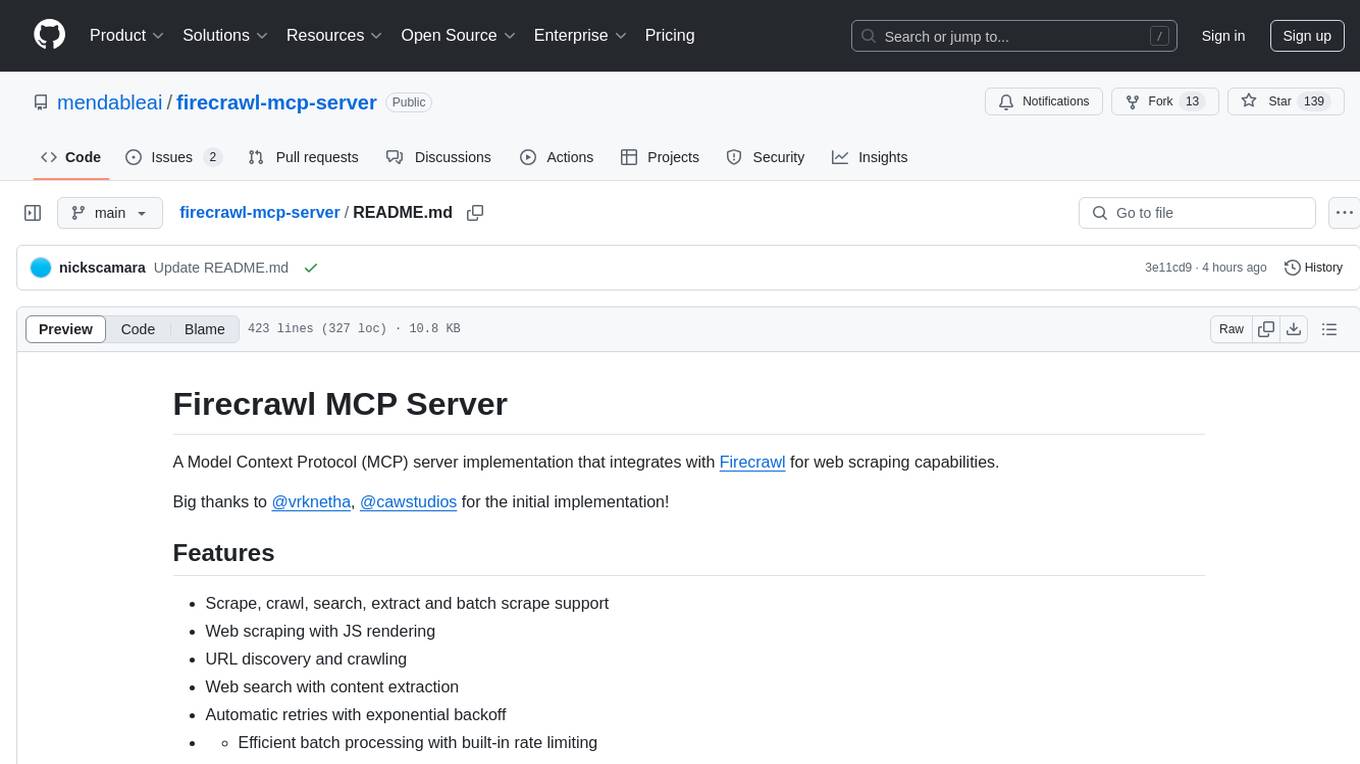
firecrawl-mcp-server
Firecrawl MCP Server is a Model Context Protocol (MCP) server implementation that integrates with Firecrawl for web scraping capabilities. It supports features like scrape, crawl, search, extract, and batch scrape. It provides web scraping with JS rendering, URL discovery, web search with content extraction, automatic retries with exponential backoff, credit usage monitoring, comprehensive logging system, support for cloud and self-hosted FireCrawl instances, mobile/desktop viewport support, and smart content filtering with tag inclusion/exclusion. The server includes configurable parameters for retry behavior and credit usage monitoring, rate limiting and batch processing capabilities, and tools for scraping, batch scraping, checking batch status, searching, crawling, and extracting structured information from web pages.
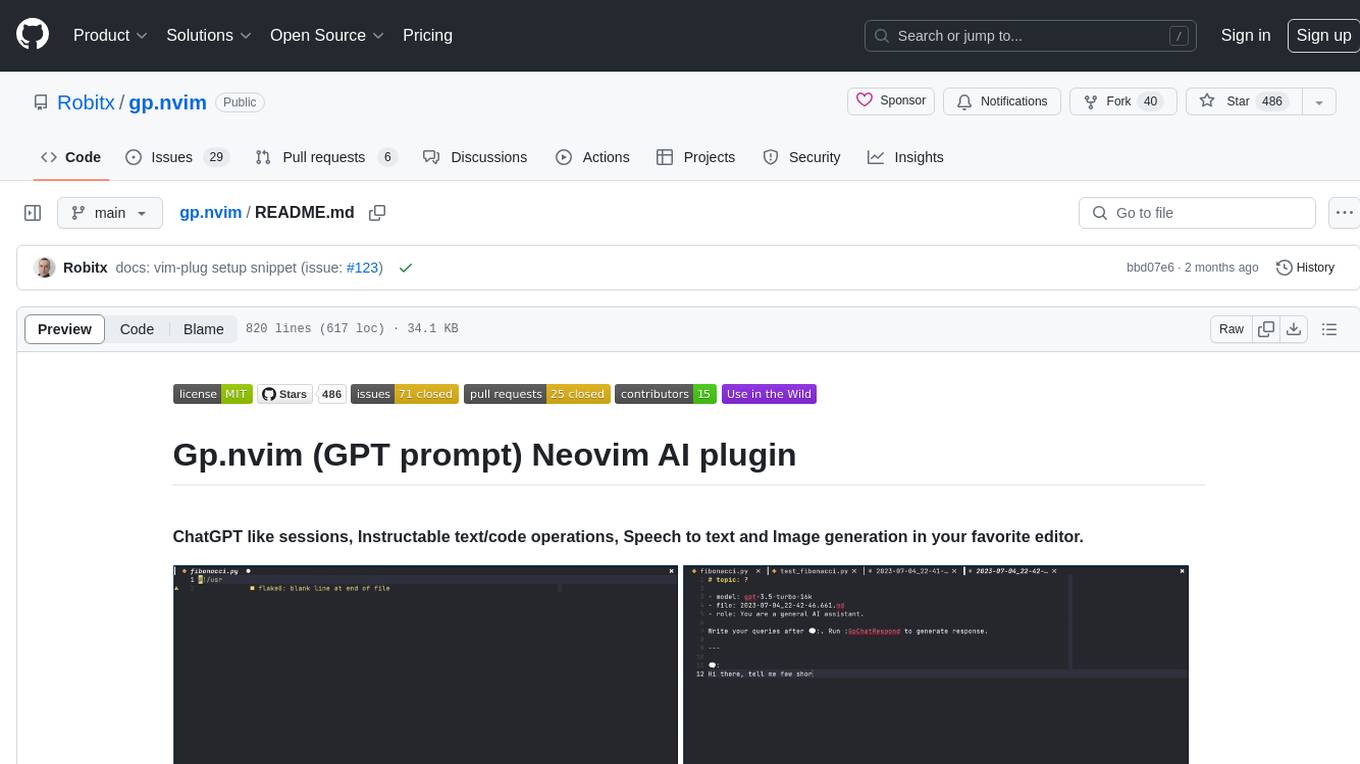
gp.nvim
Gp.nvim (GPT prompt) Neovim AI plugin provides a seamless integration of GPT models into Neovim, offering features like streaming responses, extensibility via hook functions, minimal dependencies, ChatGPT-like sessions, instructable text/code operations, speech-to-text support, and image generation directly within Neovim. The plugin aims to enhance the Neovim experience by leveraging the power of AI models in a user-friendly and native way.
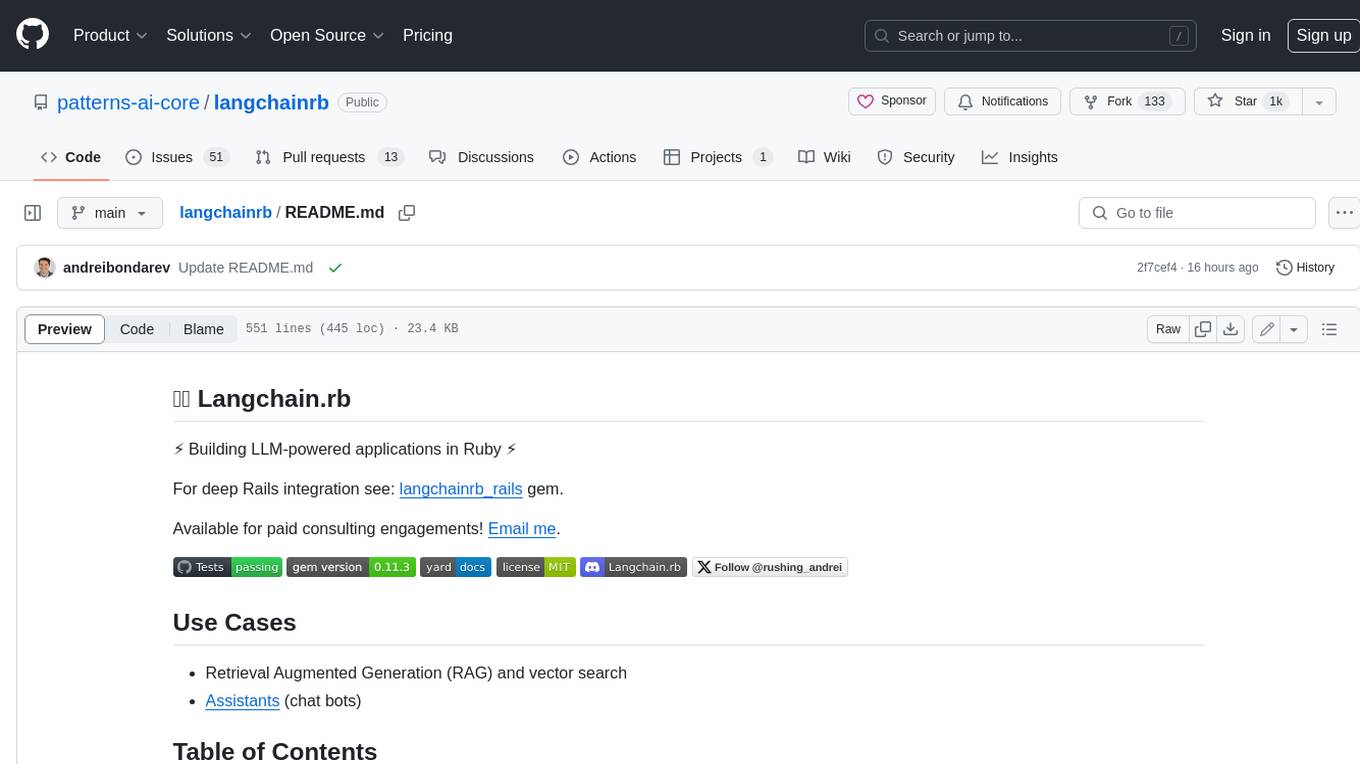
langchainrb
Langchain.rb is a Ruby library that makes it easy to build LLM-powered applications. It provides a unified interface to a variety of LLMs, vector search databases, and other tools, making it easy to build and deploy RAG (Retrieval Augmented Generation) systems and assistants. Langchain.rb is open source and available under the MIT License.
For similar tasks
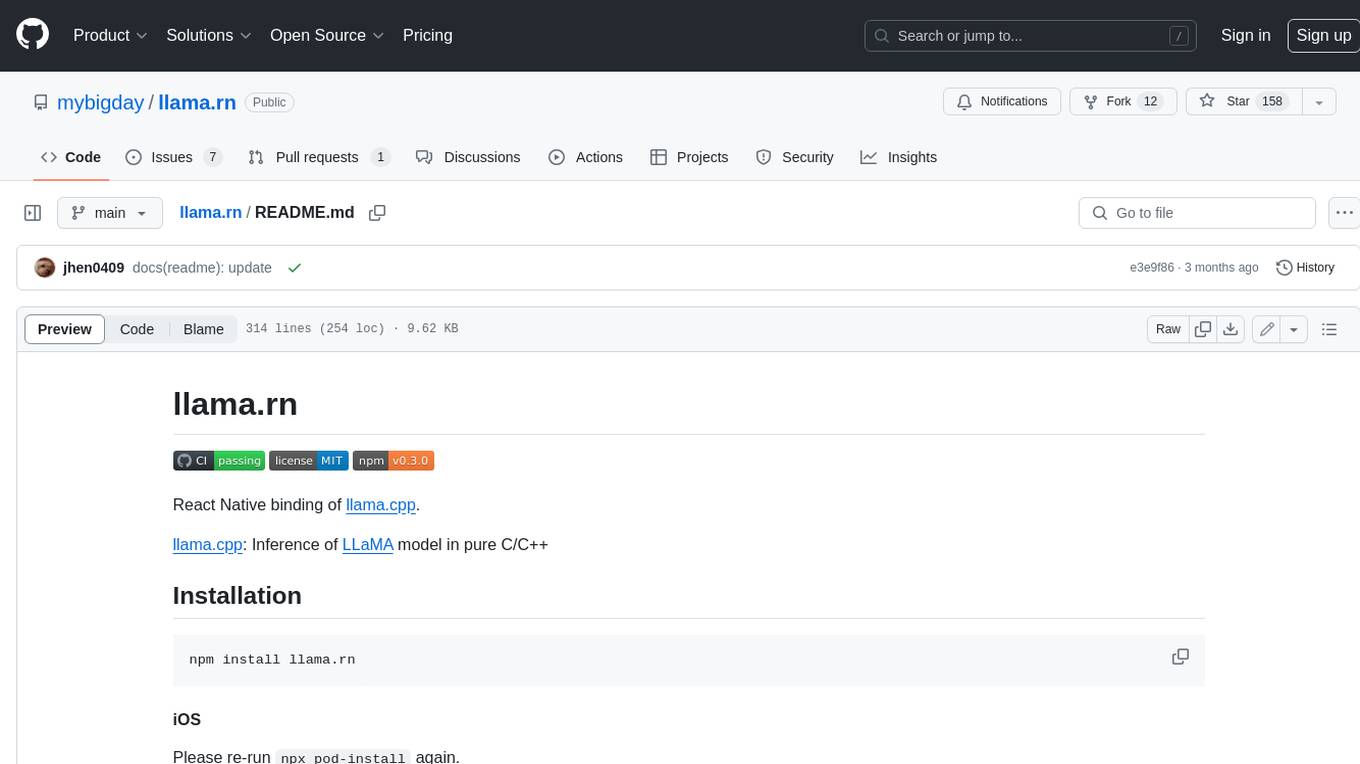
llama.rn
React Native binding of llama.cpp, which is an inference of LLaMA model in pure C/C++. This tool allows you to use the LLaMA model in your React Native applications for various tasks such as text completion, tokenization, detokenization, and embedding. It provides a convenient interface to interact with the LLaMA model and supports features like grammar sampling and mocking for testing purposes.
For similar jobs
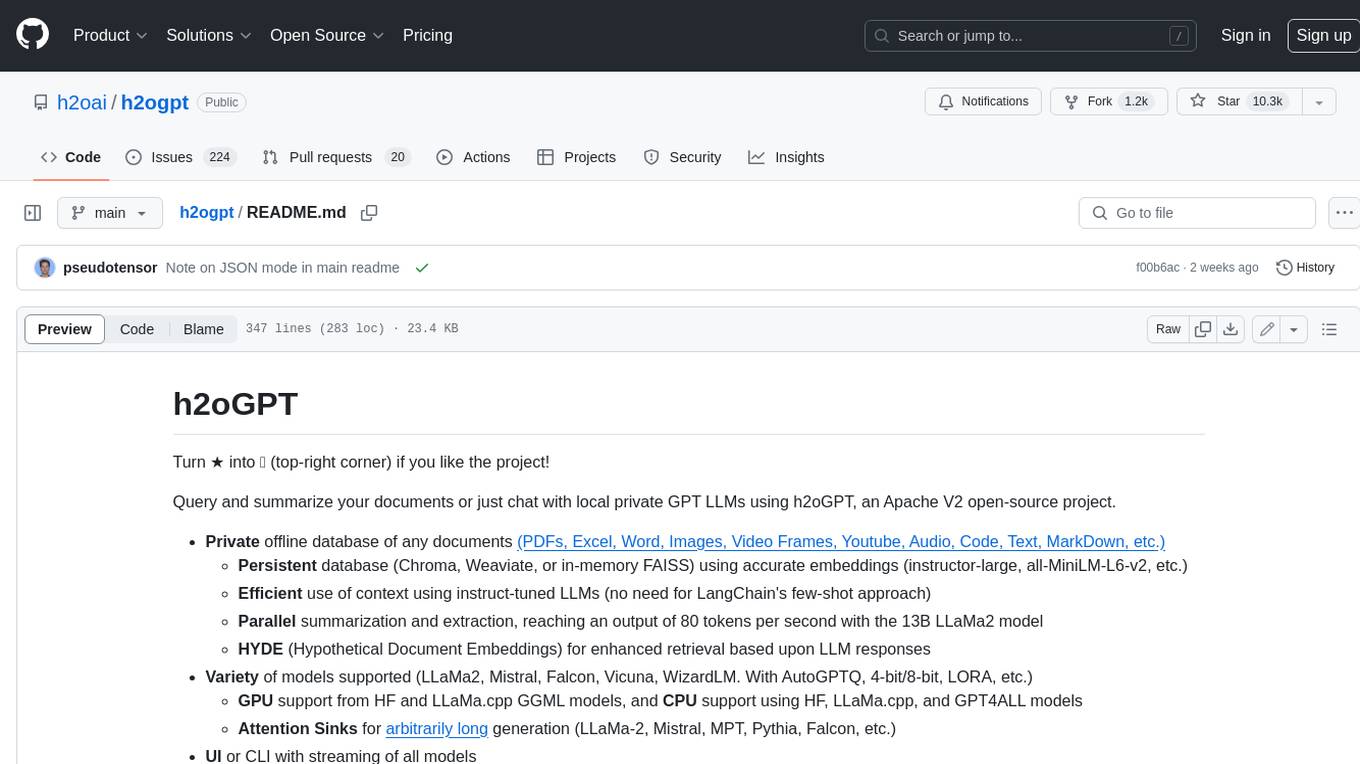
h2ogpt
h2oGPT is an Apache V2 open-source project that allows users to query and summarize documents or chat with local private GPT LLMs. It features a private offline database of any documents (PDFs, Excel, Word, Images, Video Frames, Youtube, Audio, Code, Text, MarkDown, etc.), a persistent database (Chroma, Weaviate, or in-memory FAISS) using accurate embeddings (instructor-large, all-MiniLM-L6-v2, etc.), and efficient use of context using instruct-tuned LLMs (no need for LangChain's few-shot approach). h2oGPT also offers parallel summarization and extraction, reaching an output of 80 tokens per second with the 13B LLaMa2 model, HYDE (Hypothetical Document Embeddings) for enhanced retrieval based upon LLM responses, a variety of models supported (LLaMa2, Mistral, Falcon, Vicuna, WizardLM. With AutoGPTQ, 4-bit/8-bit, LORA, etc.), GPU support from HF and LLaMa.cpp GGML models, and CPU support using HF, LLaMa.cpp, and GPT4ALL models. Additionally, h2oGPT provides Attention Sinks for arbitrarily long generation (LLaMa-2, Mistral, MPT, Pythia, Falcon, etc.), a UI or CLI with streaming of all models, the ability to upload and view documents through the UI (control multiple collaborative or personal collections), Vision Models LLaVa, Claude-3, Gemini-Pro-Vision, GPT-4-Vision, Image Generation Stable Diffusion (sdxl-turbo, sdxl) and PlaygroundAI (playv2), Voice STT using Whisper with streaming audio conversion, Voice TTS using MIT-Licensed Microsoft Speech T5 with multiple voices and Streaming audio conversion, Voice TTS using MPL2-Licensed TTS including Voice Cloning and Streaming audio conversion, AI Assistant Voice Control Mode for hands-free control of h2oGPT chat, Bake-off UI mode against many models at the same time, Easy Download of model artifacts and control over models like LLaMa.cpp through the UI, Authentication in the UI by user/password via Native or Google OAuth, State Preservation in the UI by user/password, Linux, Docker, macOS, and Windows support, Easy Windows Installer for Windows 10 64-bit (CPU/CUDA), Easy macOS Installer for macOS (CPU/M1/M2), Inference Servers support (oLLaMa, HF TGI server, vLLM, Gradio, ExLLaMa, Replicate, OpenAI, Azure OpenAI, Anthropic), OpenAI-compliant, Server Proxy API (h2oGPT acts as drop-in-replacement to OpenAI server), Python client API (to talk to Gradio server), JSON Mode with any model via code block extraction. Also supports MistralAI JSON mode, Claude-3 via function calling with strict Schema, OpenAI via JSON mode, and vLLM via guided_json with strict Schema, Web-Search integration with Chat and Document Q/A, Agents for Search, Document Q/A, Python Code, CSV frames (Experimental, best with OpenAI currently), Evaluate performance using reward models, and Quality maintained with over 1000 unit and integration tests taking over 4 GPU-hours.
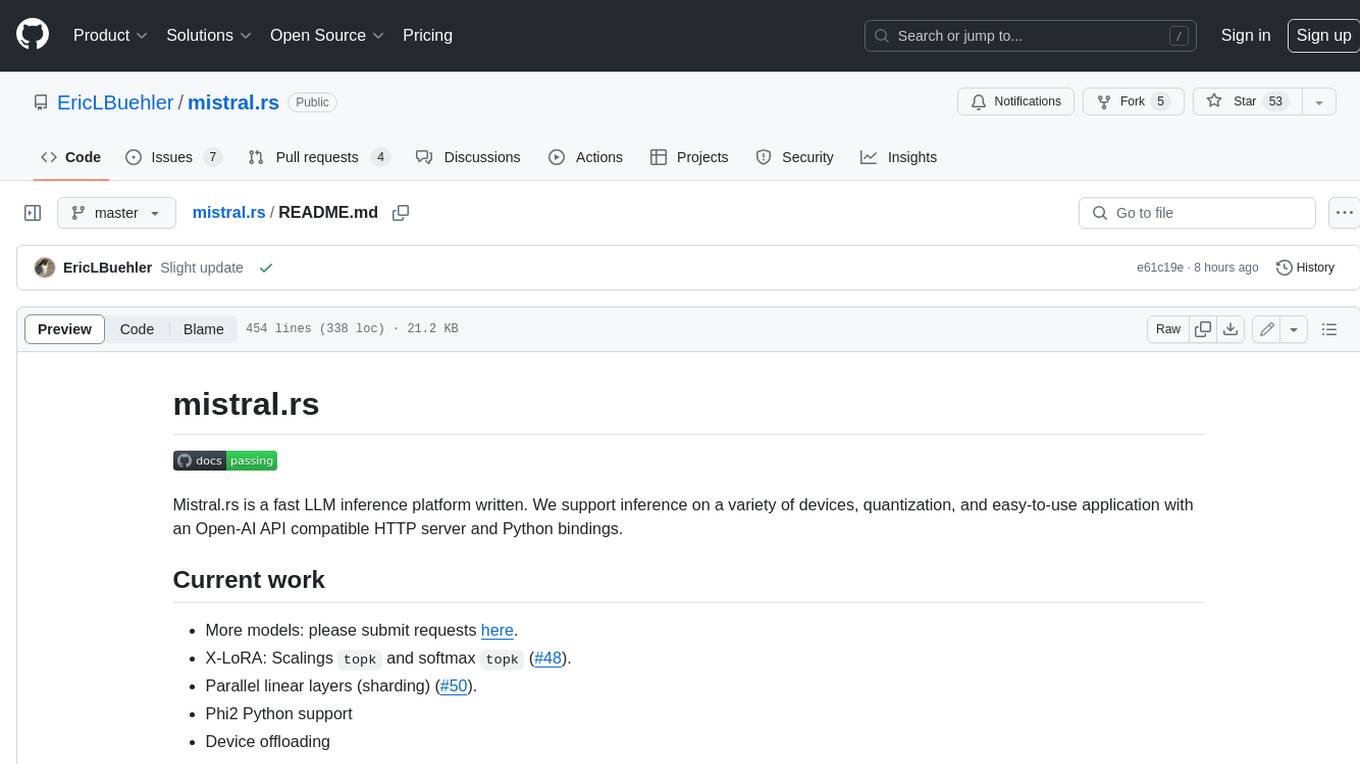
mistral.rs
Mistral.rs is a fast LLM inference platform written in Rust. We support inference on a variety of devices, quantization, and easy-to-use application with an Open-AI API compatible HTTP server and Python bindings.
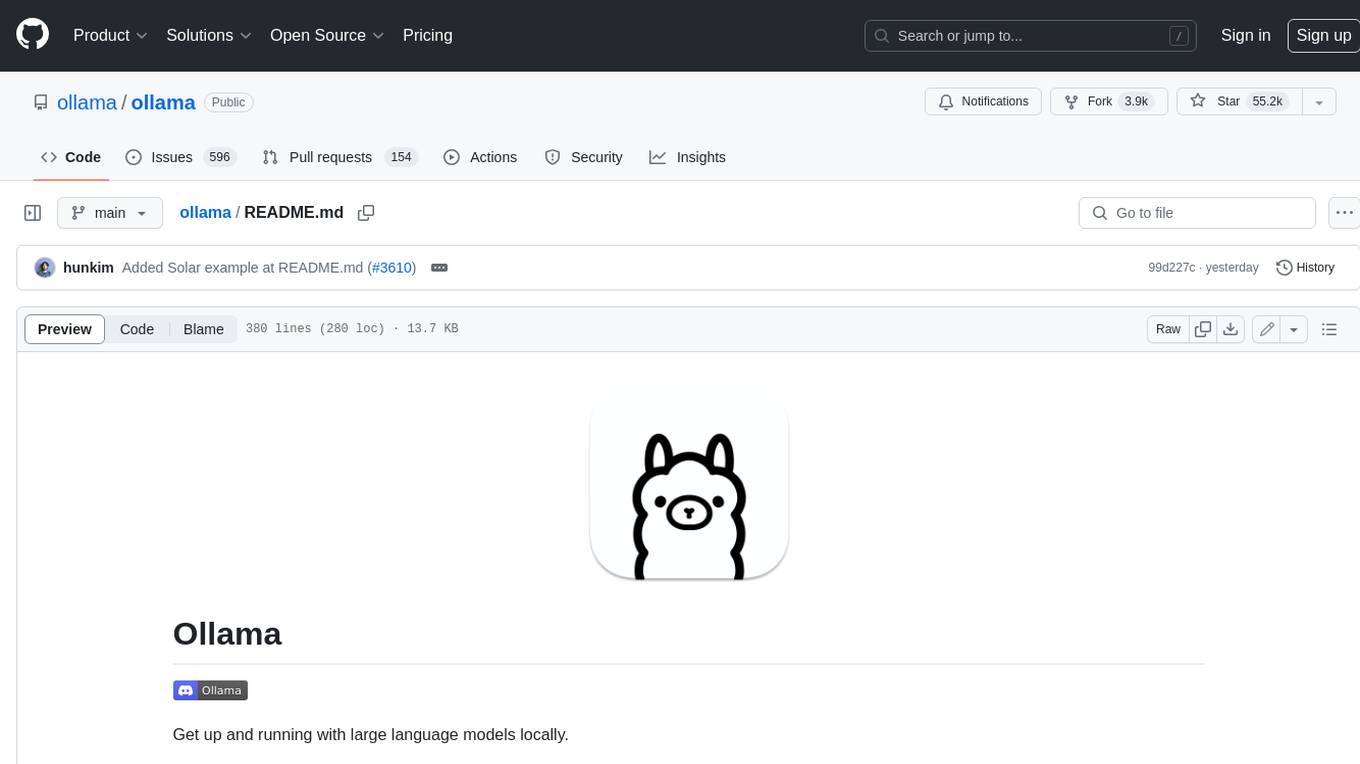
ollama
Ollama is a lightweight, extensible framework for building and running language models on the local machine. It provides a simple API for creating, running, and managing models, as well as a library of pre-built models that can be easily used in a variety of applications. Ollama is designed to be easy to use and accessible to developers of all levels. It is open source and available for free on GitHub.
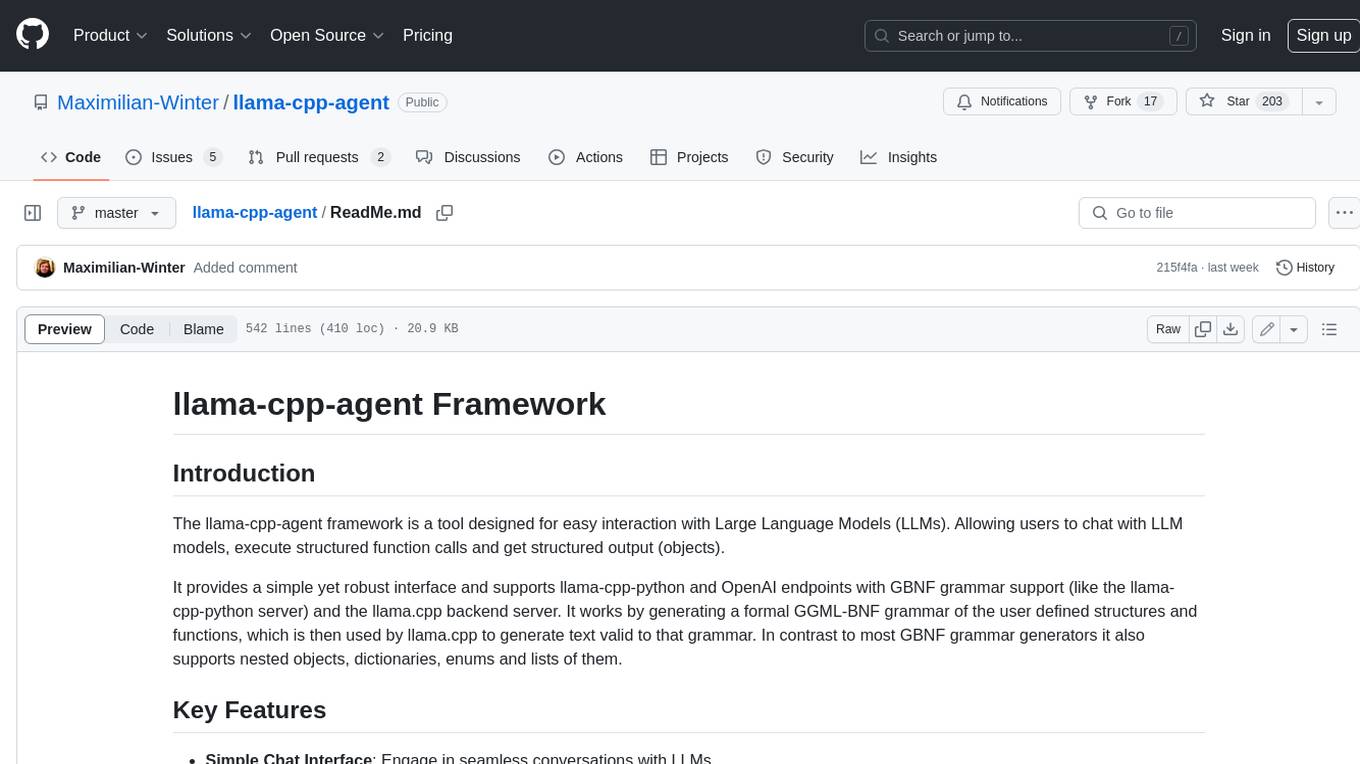
llama-cpp-agent
The llama-cpp-agent framework is a tool designed for easy interaction with Large Language Models (LLMs). Allowing users to chat with LLM models, execute structured function calls and get structured output (objects). It provides a simple yet robust interface and supports llama-cpp-python and OpenAI endpoints with GBNF grammar support (like the llama-cpp-python server) and the llama.cpp backend server. It works by generating a formal GGML-BNF grammar of the user defined structures and functions, which is then used by llama.cpp to generate text valid to that grammar. In contrast to most GBNF grammar generators it also supports nested objects, dictionaries, enums and lists of them.
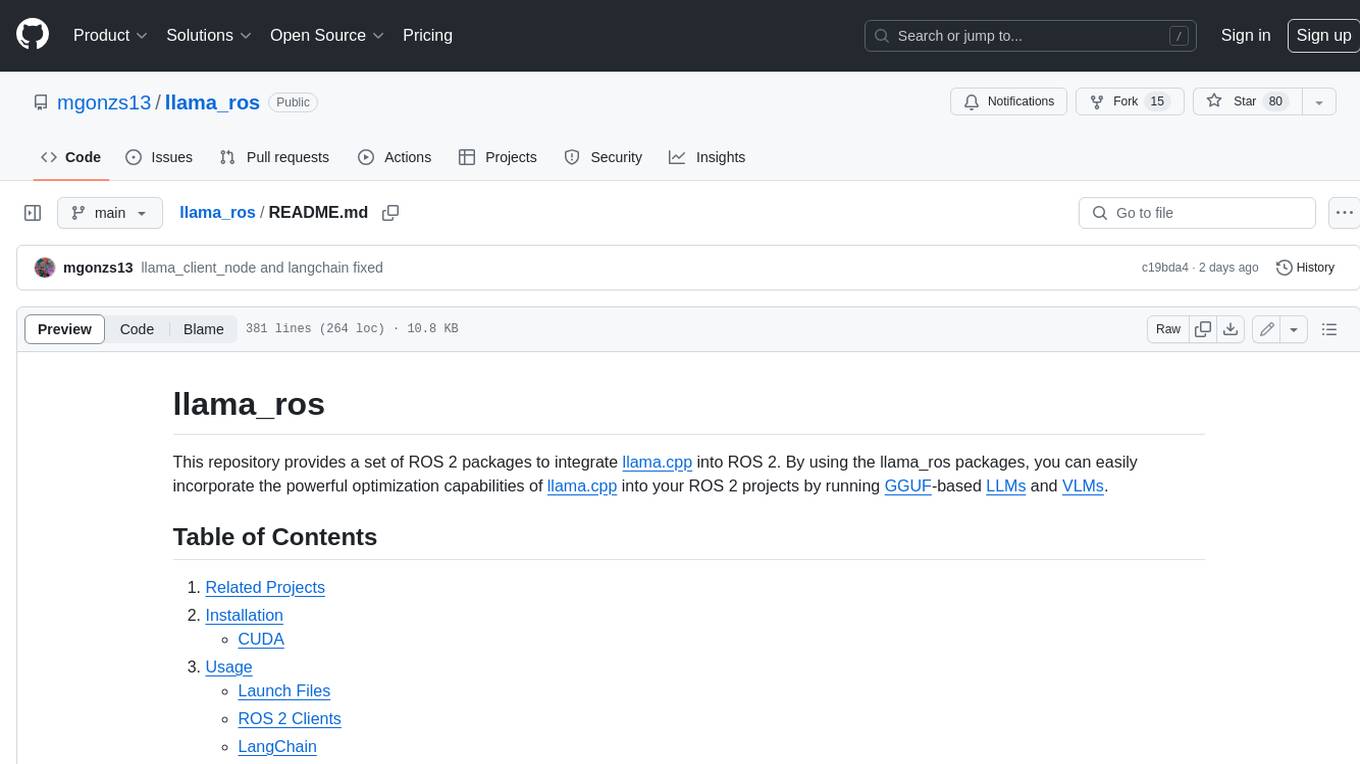
llama_ros
This repository provides a set of ROS 2 packages to integrate llama.cpp into ROS 2. By using the llama_ros packages, you can easily incorporate the powerful optimization capabilities of llama.cpp into your ROS 2 projects by running GGUF-based LLMs and VLMs.
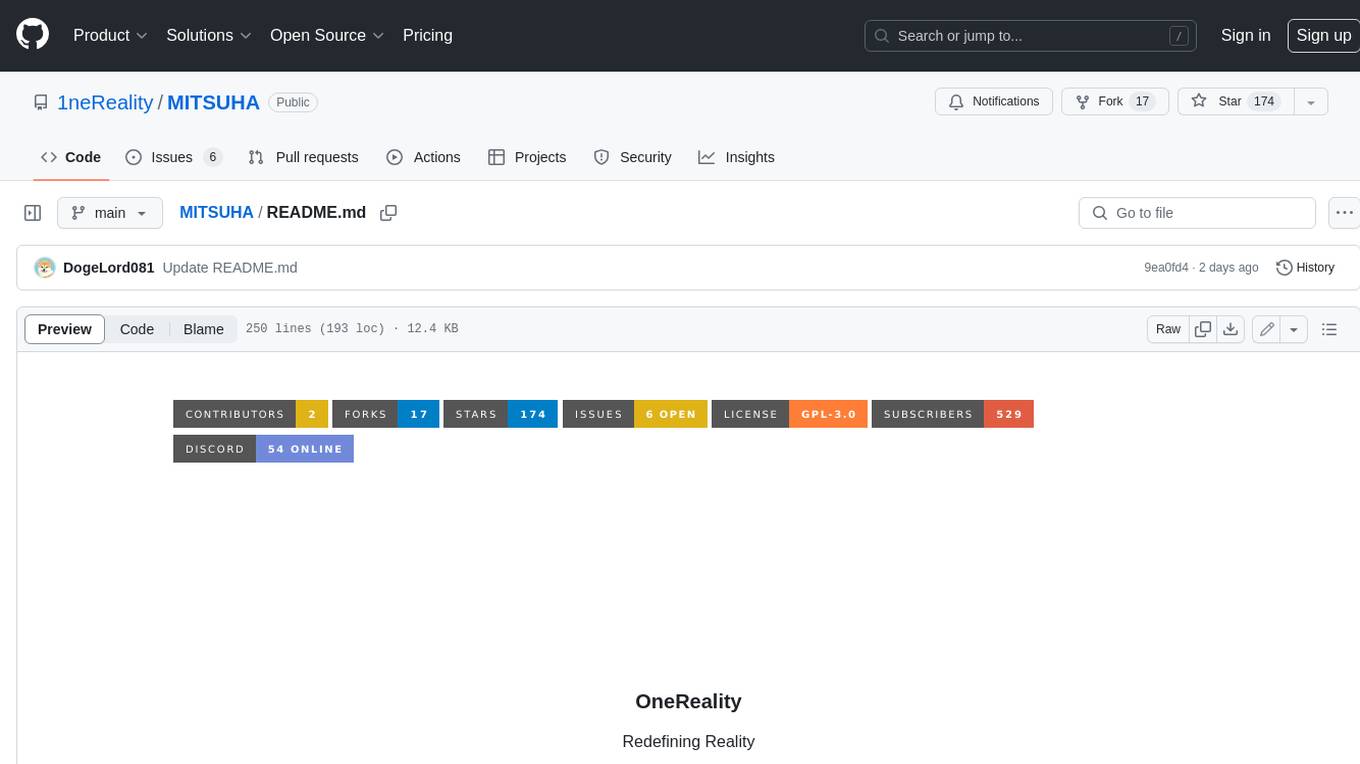
MITSUHA
OneReality is a virtual waifu/assistant that you can speak to through your mic and it'll speak back to you! It has many features such as: * You can speak to her with a mic * It can speak back to you * Has short-term memory and long-term memory * Can open apps * Smarter than you * Fluent in English, Japanese, Korean, and Chinese * Can control your smart home like Alexa if you set up Tuya (more info in Prerequisites) It is built with Python, Llama-cpp-python, Whisper, SpeechRecognition, PocketSphinx, VITS-fast-fine-tuning, VITS-simple-api, HyperDB, Sentence Transformers, and Tuya Cloud IoT.
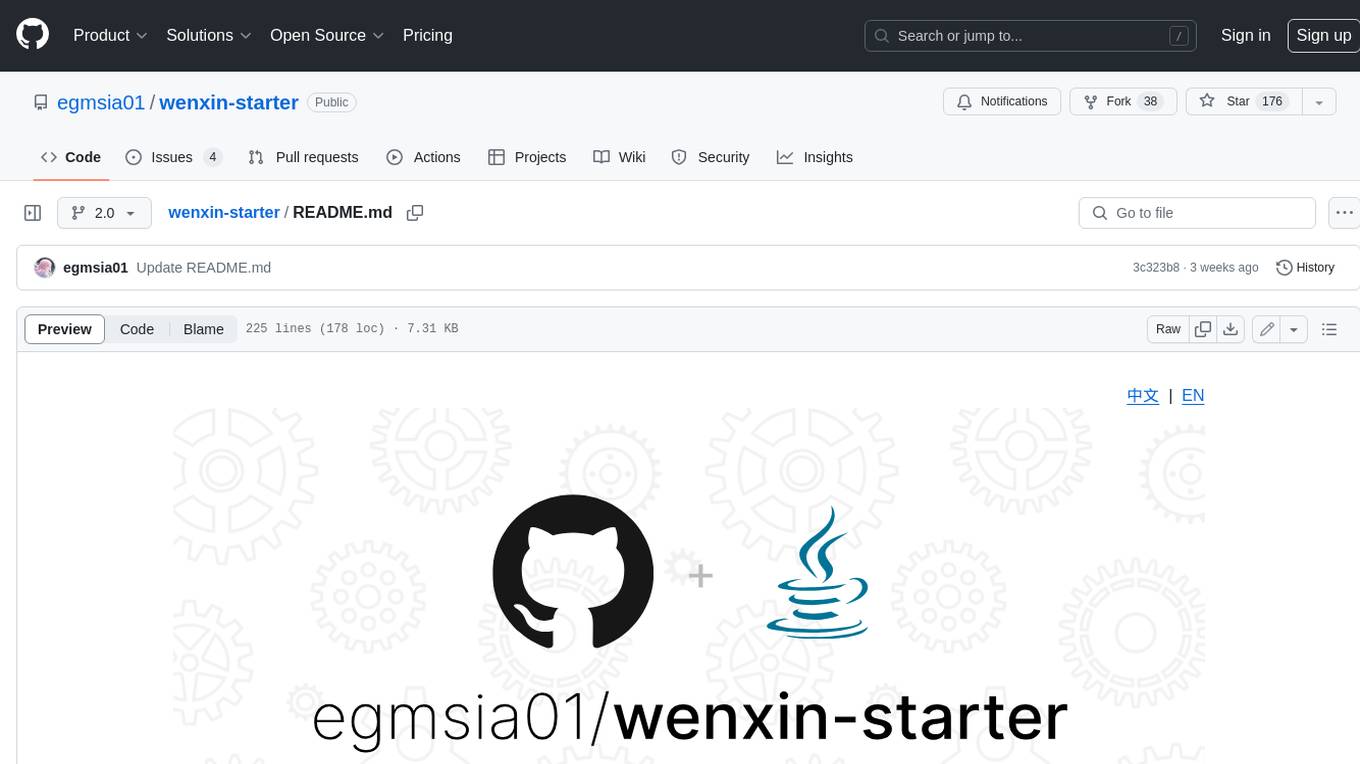
wenxin-starter
WenXin-Starter is a spring-boot-starter for Baidu's "Wenxin Qianfan WENXINWORKSHOP" large model, which can help you quickly access Baidu's AI capabilities. It fully integrates the official API documentation of Wenxin Qianfan. Supports text-to-image generation, built-in dialogue memory, and supports streaming return of dialogue. Supports QPS control of a single model and supports queuing mechanism. Plugins will be added soon.
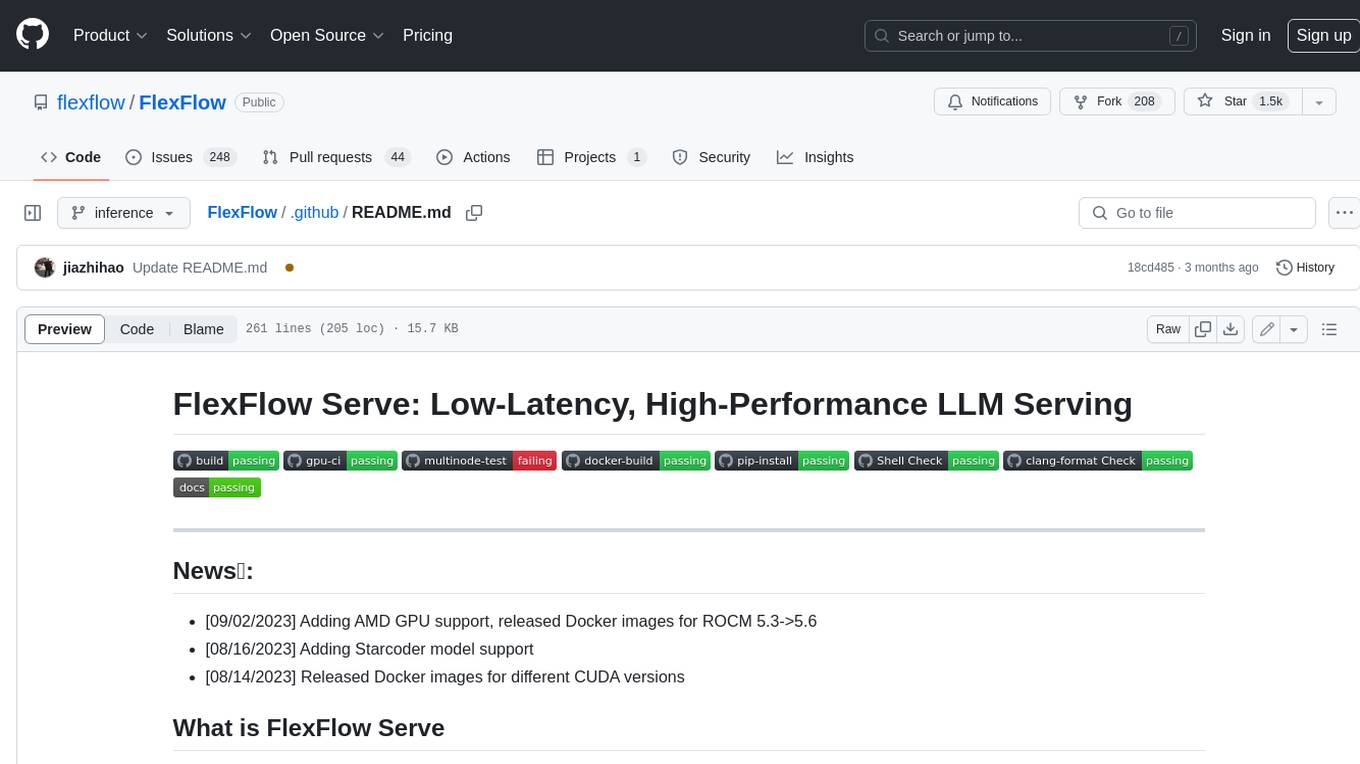
FlexFlow
FlexFlow Serve is an open-source compiler and distributed system for **low latency**, **high performance** LLM serving. FlexFlow Serve outperforms existing systems by 1.3-2.0x for single-node, multi-GPU inference and by 1.4-2.4x for multi-node, multi-GPU inference.