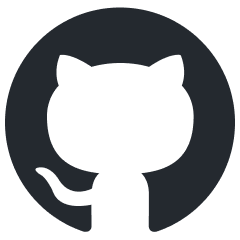
langchainrb
Build LLM-powered applications in Ruby
Stars: 1664
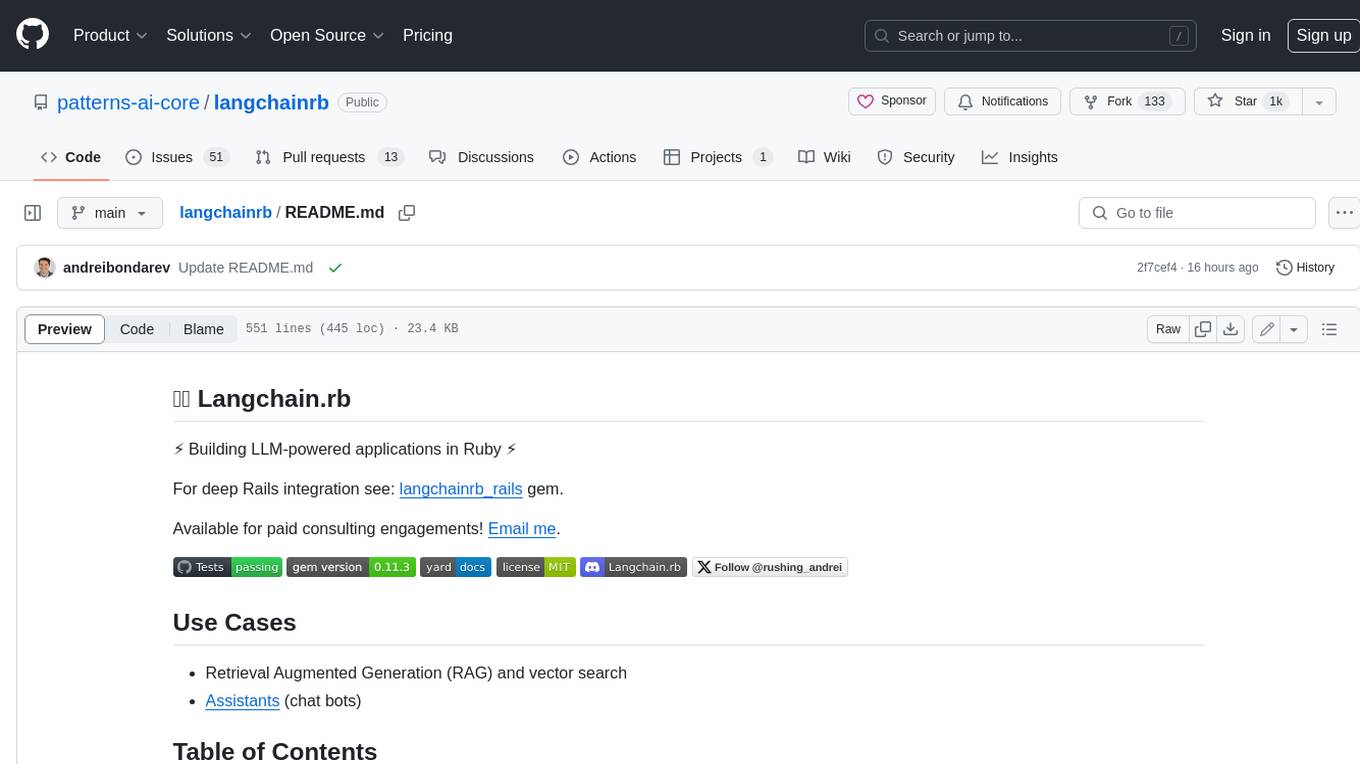
Langchain.rb is a Ruby library that makes it easy to build LLM-powered applications. It provides a unified interface to a variety of LLMs, vector search databases, and other tools, making it easy to build and deploy RAG (Retrieval Augmented Generation) systems and assistants. Langchain.rb is open source and available under the MIT License.
README:
⚡ Building LLM-powered applications in Ruby ⚡
For deep Rails integration see: langchainrb_rails gem.
Available for paid consulting engagements! Email me.
- Retrieval Augmented Generation (RAG) and vector search
- Assistants (chat bots)
- Installation
- Usage
- Unified Interface for LLMs
- Prompt Management
- Output Parsers
- Building RAG
- Assistants
- Evaluations
- Examples
- Logging
- Problems
- Development
- Discord
Install the gem and add to the application's Gemfile by executing:
bundle add langchainrb
If bundler is not being used to manage dependencies, install the gem by executing:
gem install langchainrb
Additional gems may be required. They're not included by default so you can include only what you need.
require "langchain"
The Langchain::LLM
module provides a unified interface for interacting with various Large Language Model (LLM) providers. This abstraction allows you to easily switch between different LLM backends without changing your application code.
- AI21
- Anthropic
- AWS Bedrock
- Azure OpenAI
- Cohere
- Google Gemini
- Google Vertex AI
- HuggingFace
- LlamaCpp
- Mistral AI
- Ollama
- OpenAI
- Replicate
All LLM classes inherit from Langchain::LLM::Base
and provide a consistent interface for common operations:
- Generating embeddings
- Generating prompt completions
- Generating chat completions
Most LLM classes can be initialized with an API key and optional default options:
llm = Langchain::LLM::OpenAI.new(
api_key: ENV["OPENAI_API_KEY"],
default_options: { temperature: 0.7, chat_model: "gpt-4o" }
)
Use the embed
method to generate embeddings for given text:
response = llm.embed(text: "Hello, world!")
embedding = response.embedding
-
text
: (Required) The input text to embed. -
model
: (Optional) The model name to use or default embedding model will be used.
Use the complete
method to generate completions for a given prompt:
response = llm.complete(prompt: "Once upon a time")
completion = response.completion
-
prompt
: (Required) The input prompt for completion. -
max_tokens
: (Optional) The maximum number of tokens to generate. -
temperature
: (Optional) Controls randomness in generation. Higher values (e.g., 0.8) make output more random, while lower values (e.g., 0.2) make it more deterministic. -
top_p
: (Optional) An alternative to temperature, controls diversity of generated tokens. -
n
: (Optional) Number of completions to generate for each prompt. -
stop
: (Optional) Sequences where the API will stop generating further tokens. -
presence_penalty
: (Optional) Penalizes new tokens based on their presence in the text so far. -
frequency_penalty
: (Optional) Penalizes new tokens based on their frequency in the text so far.
Use the chat
method to generate chat completions:
messages = [
{ role: "system", content: "You are a helpful assistant." },
{ role: "user", content: "What's the weather like today?" }
# Google Gemini and Google VertexAI expect messages in a different format:
# { role: "user", parts: [{ text: "why is the sky blue?" }]}
]
response = llm.chat(messages: messages)
chat_completion = response.chat_completion
-
messages
: (Required) An array of message objects representing the conversation history. -
model
: (Optional) The specific chat model to use. -
temperature
: (Optional) Controls randomness in generation. -
top_p
: (Optional) An alternative to temperature, controls diversity of generated tokens. -
n
: (Optional) Number of chat completion choices to generate. -
max_tokens
: (Optional) The maximum number of tokens to generate in the chat completion. -
stop
: (Optional) Sequences where the API will stop generating further tokens. -
presence_penalty
: (Optional) Penalizes new tokens based on their presence in the text so far. -
frequency_penalty
: (Optional) Penalizes new tokens based on their frequency in the text so far. -
logit_bias
: (Optional) Modifies the likelihood of specified tokens appearing in the completion. -
user
: (Optional) A unique identifier representing your end-user. -
tools
: (Optional) A list of tools the model may call. -
tool_choice
: (Optional) Controls how the model calls functions.
Thanks to the unified interface, you can easily switch between different LLM providers by changing the class you instantiate:
# Using Anthropic
anthropic_llm = Langchain::LLM::Anthropic.new(api_key: ENV["ANTHROPIC_API_KEY"])
# Using Google Gemini
gemini_llm = Langchain::LLM::GoogleGemini.new(api_key: ENV["GOOGLE_GEMINI_API_KEY"])
# Using OpenAI
openai_llm = Langchain::LLM::OpenAI.new(api_key: ENV["OPENAI_API_KEY"])
Each LLM method returns a response object that provides a consistent interface for accessing the results:
-
embedding
: Returns the embedding vector -
completion
: Returns the generated text completion -
chat_completion
: Returns the generated chat completion -
tool_calls
: Returns tool calls made by the LLM -
prompt_tokens
: Returns the number of tokens in the prompt -
completion_tokens
: Returns the number of tokens in the completion -
total_tokens
: Returns the total number of tokens used
[!NOTE] While the core interface is consistent across providers, some LLMs may offer additional features or parameters. Consult the documentation for each LLM class to learn about provider-specific capabilities and options.
Create a prompt with input variables:
prompt = Langchain::Prompt::PromptTemplate.new(template: "Tell me a {adjective} joke about {content}.", input_variables: ["adjective", "content"])
prompt.format(adjective: "funny", content: "chickens") # "Tell me a funny joke about chickens."
Creating a PromptTemplate using just a prompt and no input_variables:
prompt = Langchain::Prompt::PromptTemplate.from_template("Tell me a funny joke about chickens.")
prompt.input_variables # []
prompt.format # "Tell me a funny joke about chickens."
Save prompt template to JSON file:
prompt.save(file_path: "spec/fixtures/prompt/prompt_template.json")
Loading a new prompt template using a JSON file:
prompt = Langchain::Prompt.load_from_path(file_path: "spec/fixtures/prompt/prompt_template.json")
prompt.input_variables # ["adjective", "content"]
Create a prompt with a few shot examples:
prompt = Langchain::Prompt::FewShotPromptTemplate.new(
prefix: "Write antonyms for the following words.",
suffix: "Input: {adjective}\nOutput:",
example_prompt: Langchain::Prompt::PromptTemplate.new(
input_variables: ["input", "output"],
template: "Input: {input}\nOutput: {output}"
),
examples: [
{ "input": "happy", "output": "sad" },
{ "input": "tall", "output": "short" }
],
input_variables: ["adjective"]
)
prompt.format(adjective: "good")
# Write antonyms for the following words.
#
# Input: happy
# Output: sad
#
# Input: tall
# Output: short
#
# Input: good
# Output:
Save prompt template to JSON file:
prompt.save(file_path: "spec/fixtures/prompt/few_shot_prompt_template.json")
Loading a new prompt template using a JSON file:
prompt = Langchain::Prompt.load_from_path(file_path: "spec/fixtures/prompt/few_shot_prompt_template.json")
prompt.prefix # "Write antonyms for the following words."
Loading a new prompt template using a YAML file:
prompt = Langchain::Prompt.load_from_path(file_path: "spec/fixtures/prompt/prompt_template.yaml")
prompt.input_variables #=> ["adjective", "content"]
Parse LLM text responses into structured output, such as JSON.
You can use the StructuredOutputParser
to generate a prompt that instructs the LLM to provide a JSON response adhering to a specific JSON schema:
json_schema = {
type: "object",
properties: {
name: {
type: "string",
description: "Persons name"
},
age: {
type: "number",
description: "Persons age"
},
interests: {
type: "array",
items: {
type: "object",
properties: {
interest: {
type: "string",
description: "A topic of interest"
},
levelOfInterest: {
type: "number",
description: "A value between 0 and 100 of how interested the person is in this interest"
}
},
required: ["interest", "levelOfInterest"],
additionalProperties: false
},
minItems: 1,
maxItems: 3,
description: "A list of the person's interests"
}
},
required: ["name", "age", "interests"],
additionalProperties: false
}
parser = Langchain::OutputParsers::StructuredOutputParser.from_json_schema(json_schema)
prompt = Langchain::Prompt::PromptTemplate.new(template: "Generate details of a fictional character.\n{format_instructions}\nCharacter description: {description}", input_variables: ["description", "format_instructions"])
prompt_text = prompt.format(description: "Korean chemistry student", format_instructions: parser.get_format_instructions)
# Generate details of a fictional character.
# You must format your output as a JSON value that adheres to a given "JSON Schema" instance.
# ...
Then parse the llm response:
llm = Langchain::LLM::OpenAI.new(api_key: ENV["OPENAI_API_KEY"])
llm_response = llm.chat(messages: [{role: "user", content: prompt_text}]).completion
parser.parse(llm_response)
# {
# "name" => "Kim Ji-hyun",
# "age" => 22,
# "interests" => [
# {
# "interest" => "Organic Chemistry",
# "levelOfInterest" => 85
# },
# ...
# ]
# }
If the parser fails to parse the LLM response, you can use the OutputFixingParser
. It sends an error message, prior output, and the original prompt text to the LLM, asking for a "fixed" response:
begin
parser.parse(llm_response)
rescue Langchain::OutputParsers::OutputParserException => e
fix_parser = Langchain::OutputParsers::OutputFixingParser.from_llm(
llm: llm,
parser: parser
)
fix_parser.parse(llm_response)
end
Alternatively, if you don't need to handle the OutputParserException
, you can simplify the code:
# we already have the `OutputFixingParser`:
# parser = Langchain::OutputParsers::StructuredOutputParser.from_json_schema(json_schema)
fix_parser = Langchain::OutputParsers::OutputFixingParser.from_llm(
llm: llm,
parser: parser
)
fix_parser.parse(llm_response)
See here for a concrete example
RAG is a methodology that assists LLMs generate accurate and up-to-date information. A typical RAG workflow follows the 3 steps below:
- Relevant knowledge (or data) is retrieved from the knowledge base (typically a vector search DB)
- A prompt, containing retrieved knowledge above, is constructed.
- LLM receives the prompt above to generate a text completion. Most common use-case for a RAG system is powering Q&A systems where users pose natural language questions and receive answers in natural language.
Langchain.rb provides a convenient unified interface on top of supported vectorsearch databases that make it easy to configure your index, add data, query and retrieve from it.
Database | Open-source | Cloud offering |
---|---|---|
Chroma | ✅ | ✅ |
Epsilla | ✅ | ✅ |
Hnswlib | ✅ | ❌ |
Milvus | ✅ | ✅ Zilliz Cloud |
Pinecone | ❌ | ✅ |
Pgvector | ✅ | ✅ |
Qdrant | ✅ | ✅ |
Weaviate | ✅ | ✅ |
Elasticsearch | ✅ | ✅ |
Pick the vector search database you'll be using, add the gem dependency and instantiate the client:
gem "weaviate-ruby", "~> 0.8.9"
Choose and instantiate the LLM provider you'll be using to generate embeddings
llm = Langchain::LLM::OpenAI.new(api_key: ENV["OPENAI_API_KEY"])
client = Langchain::Vectorsearch::Weaviate.new(
url: ENV["WEAVIATE_URL"],
api_key: ENV["WEAVIATE_API_KEY"],
index_name: "Documents",
llm: llm
)
You can instantiate any other supported vector search database:
client = Langchain::Vectorsearch::Chroma.new(...) # `gem "chroma-db", "~> 0.6.0"`
client = Langchain::Vectorsearch::Epsilla.new(...) # `gem "epsilla-ruby", "~> 0.0.3"`
client = Langchain::Vectorsearch::Hnswlib.new(...) # `gem "hnswlib", "~> 0.8.1"`
client = Langchain::Vectorsearch::Milvus.new(...) # `gem "milvus", "~> 0.9.3"`
client = Langchain::Vectorsearch::Pinecone.new(...) # `gem "pinecone", "~> 0.1.6"`
client = Langchain::Vectorsearch::Pgvector.new(...) # `gem "pgvector", "~> 0.2"`
client = Langchain::Vectorsearch::Qdrant.new(...) # `gem "qdrant-ruby", "~> 0.9.3"`
client = Langchain::Vectorsearch::Elasticsearch.new(...) # `gem "elasticsearch", "~> 8.2.0"`
Create the default schema:
client.create_default_schema
Add plain text data to your vector search database:
client.add_texts(
texts: [
"Begin by preheating your oven to 375°F (190°C). Prepare four boneless, skinless chicken breasts by cutting a pocket into the side of each breast, being careful not to cut all the way through. Season the chicken with salt and pepper to taste. In a large skillet, melt 2 tablespoons of unsalted butter over medium heat. Add 1 small diced onion and 2 minced garlic cloves, and cook until softened, about 3-4 minutes. Add 8 ounces of fresh spinach and cook until wilted, about 3 minutes. Remove the skillet from heat and let the mixture cool slightly.",
"In a bowl, combine the spinach mixture with 4 ounces of softened cream cheese, 1/4 cup of grated Parmesan cheese, 1/4 cup of shredded mozzarella cheese, and 1/4 teaspoon of red pepper flakes. Mix until well combined. Stuff each chicken breast pocket with an equal amount of the spinach mixture. Seal the pocket with a toothpick if necessary. In the same skillet, heat 1 tablespoon of olive oil over medium-high heat. Add the stuffed chicken breasts and sear on each side for 3-4 minutes, or until golden brown."
]
)
Or use the file parsers to load, parse and index data into your database:
my_pdf = Langchain.root.join("path/to/my.pdf")
my_text = Langchain.root.join("path/to/my.txt")
my_docx = Langchain.root.join("path/to/my.docx")
client.add_data(paths: [my_pdf, my_text, my_docx])
Supported file formats: docx, html, pdf, text, json, jsonl, csv, xlsx, eml, pptx.
Retrieve similar documents based on the query string passed in:
client.similarity_search(
query:,
k: # number of results to be retrieved
)
Retrieve similar documents based on the query string passed in via the HyDE technique:
client.similarity_search_with_hyde()
Retrieve similar documents based on the embedding passed in:
client.similarity_search_by_vector(
embedding:,
k: # number of results to be retrieved
)
RAG-based querying
client.ask(question: "...")
Langchain::Assistant
is a powerful and flexible class that combines Large Language Models (LLMs), tools, and conversation management to create intelligent, interactive assistants. It's designed to handle complex conversations, execute tools, and provide coherent responses based on the context of the interaction.
- Supports multiple LLM providers (OpenAI, Google Gemini, Anthropic, Mistral AI and open-source models via Ollama)
- Integrates with various tools to extend functionality
- Manages conversation threads
- Handles automatic and manual tool execution
- Supports different message formats for various LLM providers
llm = Langchain::LLM::OpenAI.new(api_key: ENV["OPENAI_API_KEY"])
assistant = Langchain::Assistant.new(
llm: llm,
instructions: "You're a helpful AI assistant",
tools: [Langchain::Tool::NewsRetriever.new(api_key: ENV["NEWS_API_KEY"])]
)
# Add a user message and run the assistant
assistant.add_message_and_run!(content: "What's the latest news about AI?")
# Supply an image to the assistant
assistant.add_message_and_run!(
content: "Show me a picture of a cat",
image_url: "https://upload.wikimedia.org/wikipedia/commons/thumb/d/dd/Gfp-wisconsin-madison-the-nature-boardwalk.jpg/2560px-Gfp-wisconsin-madison-the-nature-boardwalk.jpg"
)
# Access the conversation thread
messages = assistant.messages
# Run the assistant with automatic tool execution
assistant.run(auto_tool_execution: true)
# If you want to stream the response, you can add a response handler
assistant = Langchain::Assistant.new(
llm: llm,
instructions: "You're a helpful AI assistant",
tools: [Langchain::Tool::NewsRetriever.new(api_key: ENV["NEWS_API_KEY"])]
) do |response_chunk|
# ...handle the response stream
# print(response_chunk.inspect)
end
assistant.add_message(content: "Hello")
assistant.run(auto_tool_execution: true)
Note that streaming is not currently supported for all LLMs.
-
llm
: The LLM instance to use (required) -
tools
: An array of tool instances (optional) -
instructions
: System instructions for the assistant (optional) -
tool_choice
: Specifies how tools should be selected. Default: "auto". A specific tool function name can be passed. This will force the Assistant to always use this function. -
parallel_tool_calls
: Whether to make multiple parallel tool calls. Default: true -
add_message_callback
: A callback function (proc, lambda) that is called when any message is added to the conversation (optional)
assistant.add_message_callback = -> (message) { puts "New message: #{message}" }
-
tool_execution_callback
: A callback function (proc, lambda) that is called right before a tool is executed (optional)
assistant.tool_execution_callback = -> (tool_call_id, tool_name, method_name, tool_arguments) { puts "Executing tool_call_id: #{tool_call_id}, tool_name: #{tool_name}, method_name: #{method_name}, tool_arguments: #{tool_arguments}" }
-
add_message
: Adds a user message to the messages array -
run!
: Processes the conversation and generates responses -
add_message_and_run!
: Combines adding a message and running the assistant -
submit_tool_output
: Manually submit output to a tool call -
messages
: Returns a list of ongoing messages
-
Langchain::Tool::Calculator
: Useful for evaluating math expressions. Requiresgem "eqn"
. -
Langchain::Tool::Database
: Connect your SQL database. Requiresgem "sequel"
. -
Langchain::Tool::FileSystem
: Interact with the file system (read & write). -
Langchain::Tool::RubyCodeInterpreter
: Useful for evaluating generated Ruby code. Requiresgem "safe_ruby"
(In need of a better solution). -
Langchain::Tool::NewsRetriever
: A wrapper around NewsApi.org to fetch news articles. -
Langchain::Tool::Tavily
: A wrapper around Tavily AI. -
Langchain::Tool::Weather
: Calls Open Weather API to retrieve the current weather. -
Langchain::Tool::Wikipedia
: Calls Wikipedia API.
The Langchain::Assistant can be easily extended with custom tools by creating classes that extend Langchain::ToolDefinition
module and implement required methods.
class MovieInfoTool
extend Langchain::ToolDefinition
define_function :search_movie, description: "MovieInfoTool: Search for a movie by title" do
property :query, type: "string", description: "The movie title to search for", required: true
end
define_function :get_movie_details, description: "MovieInfoTool: Get detailed information about a specific movie" do
property :movie_id, type: "integer", description: "The TMDb ID of the movie", required: true
end
def initialize(api_key:)
@api_key = api_key
end
def search_movie(query:)
tool_response(...)
end
def get_movie_details(movie_id:)
tool_response(...)
end
end
movie_tool = MovieInfoTool.new(api_key: "...")
assistant = Langchain::Assistant.new(
llm: llm,
instructions: "You're a helpful AI assistant that can provide movie information",
tools: [movie_tool]
)
assistant.add_message_and_run(content: "Can you tell me about the movie 'Inception'?")
# Check the response in the last message in the conversation
assistant.messages.last
The assistant includes error handling for invalid inputs, unsupported LLM types, and tool execution failures. It uses a state machine to manage the conversation flow and handle different scenarios gracefully.
- Building an AI Assistant that operates a simulated E-commerce Store
- New Langchain.rb Assistants interface
- Langchain.rb Assistant demo with NewsRetriever and function calling on Gemini - code
The Evaluations module is a collection of tools that can be used to evaluate and track the performance of the output products by LLM and your RAG (Retrieval Augmented Generation) pipelines.
Ragas helps you evaluate your Retrieval Augmented Generation (RAG) pipelines. The implementation is based on this paper and the original Python repo. Ragas tracks the following 3 metrics and assigns the 0.0 - 1.0 scores:
- Faithfulness - the answer is grounded in the given context.
- Context Relevance - the retrieved context is focused, containing little to no irrelevant information.
- Answer Relevance - the generated answer addresses the actual question that was provided.
# We recommend using Langchain::LLM::OpenAI as your llm for Ragas
ragas = Langchain::Evals::Ragas::Main.new(llm: llm)
# The answer that the LLM generated
# The question (or the original prompt) that was asked
# The context that was retrieved (usually from a vectorsearch database)
ragas.score(answer: "", question: "", context: "")
# =>
# {
# ragas_score: 0.6601257446503674,
# answer_relevance_score: 0.9573145866787608,
# context_relevance_score: 0.6666666666666666,
# faithfulness_score: 0.5
# }
Additional examples available: /examples
Langchain.rb uses the standard Ruby Logger mechanism and defaults to same level
value (currently Logger::DEBUG
).
To show all log messages:
Langchain.logger.level = Logger::DEBUG
The logger logs to STDOUT
by default. In order to configure the log destination (ie. log to a file) do:
Langchain.logger = Logger.new("path/to/file", **Langchain::LOGGER_OPTIONS)
If you're having issues installing unicode
gem required by pragmatic_segmenter
, try running:
gem install unicode -- --with-cflags="-Wno-incompatible-function-pointer-types"
git clone https://github.com/andreibondarev/langchainrb.git
-
cp .env.example .env
, then fill out the environment variables in.env
-
bundle exec rake
to ensure that the tests pass and to run standardrb -
bin/console
to load the gem in a REPL session. Feel free to add your own instances of LLMs, Tools, Agents, etc. and experiment with them. - Optionally, install lefthook git hooks for pre-commit to auto lint:
gem install lefthook && lefthook install -f
Join us in the Langchain.rb Discord server.
Bug reports and pull requests are welcome on GitHub at https://github.com/andreibondarev/langchainrb.
The gem is available as open source under the terms of the MIT License.
For Tasks:
Click tags to check more tools for each tasksFor Jobs:
Alternative AI tools for langchainrb
Similar Open Source Tools
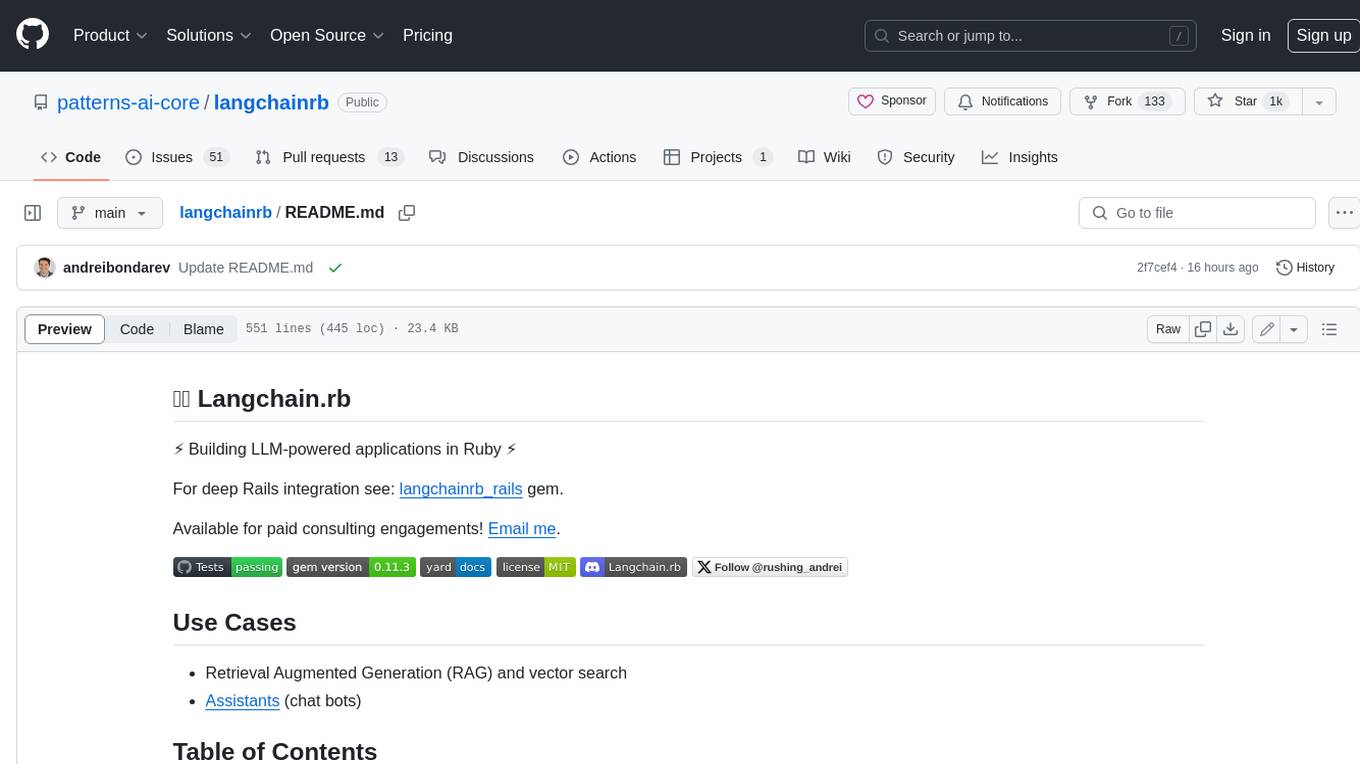
langchainrb
Langchain.rb is a Ruby library that makes it easy to build LLM-powered applications. It provides a unified interface to a variety of LLMs, vector search databases, and other tools, making it easy to build and deploy RAG (Retrieval Augmented Generation) systems and assistants. Langchain.rb is open source and available under the MIT License.
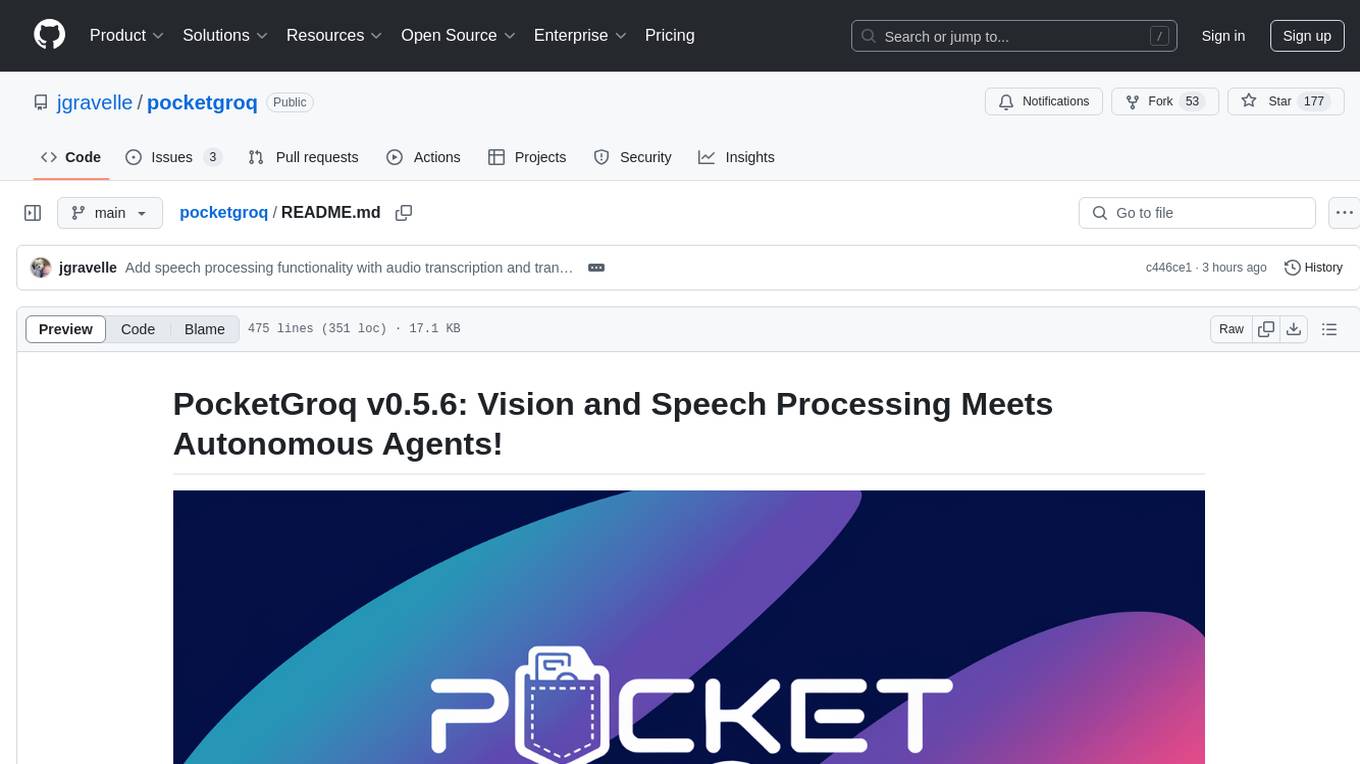
pocketgroq
PocketGroq is a tool that provides advanced functionalities for text generation, web scraping, web search, and AI response evaluation. It includes features like an Autonomous Agent for answering questions, web crawling and scraping capabilities, enhanced web search functionality, and flexible integration with Ollama server. Users can customize the agent's behavior, evaluate responses using AI, and utilize various methods for text generation, conversation management, and Chain of Thought reasoning. The tool offers comprehensive methods for different tasks, such as initializing RAG, error handling, and tool management. PocketGroq is designed to enhance development processes and enable the creation of AI-powered applications with ease.
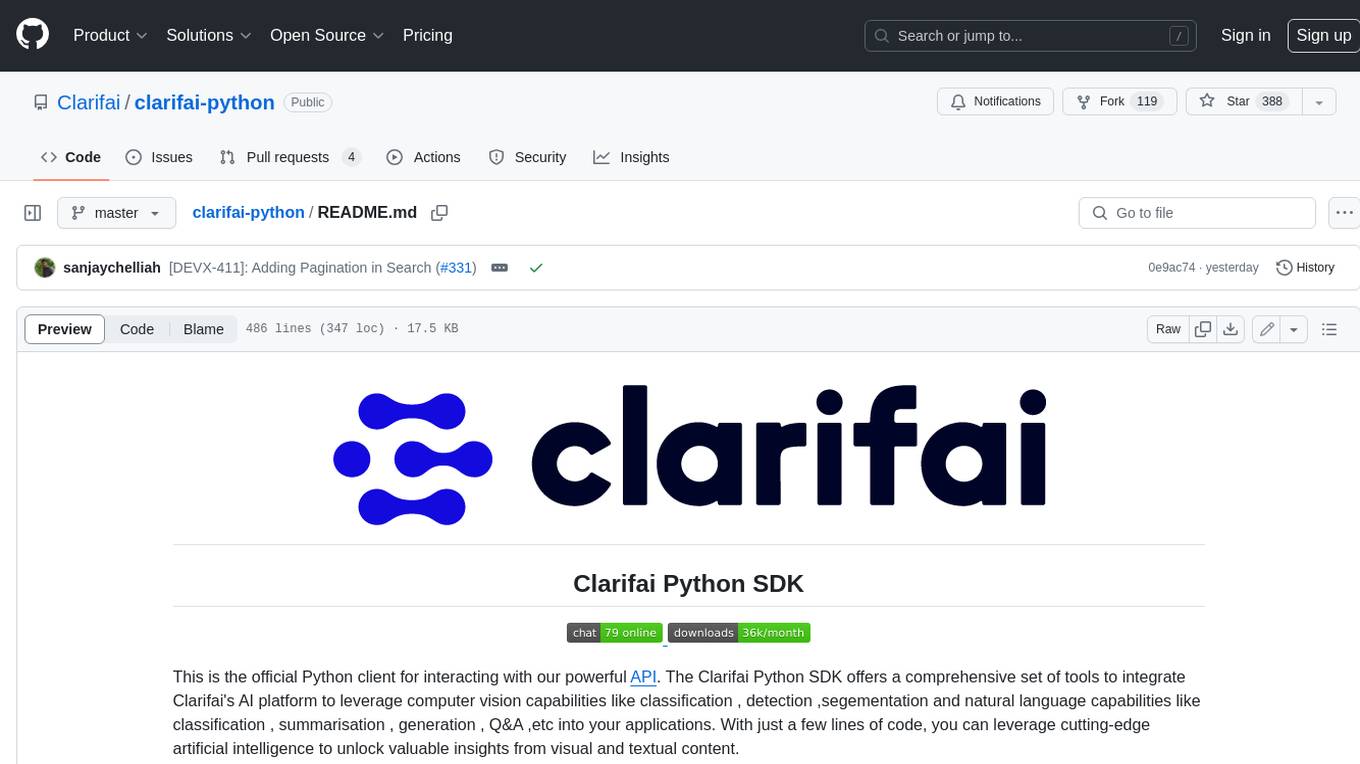
clarifai-python
The Clarifai Python SDK offers a comprehensive set of tools to integrate Clarifai's AI platform to leverage computer vision capabilities like classification , detection ,segementation and natural language capabilities like classification , summarisation , generation , Q&A ,etc into your applications. With just a few lines of code, you can leverage cutting-edge artificial intelligence to unlock valuable insights from visual and textual content.
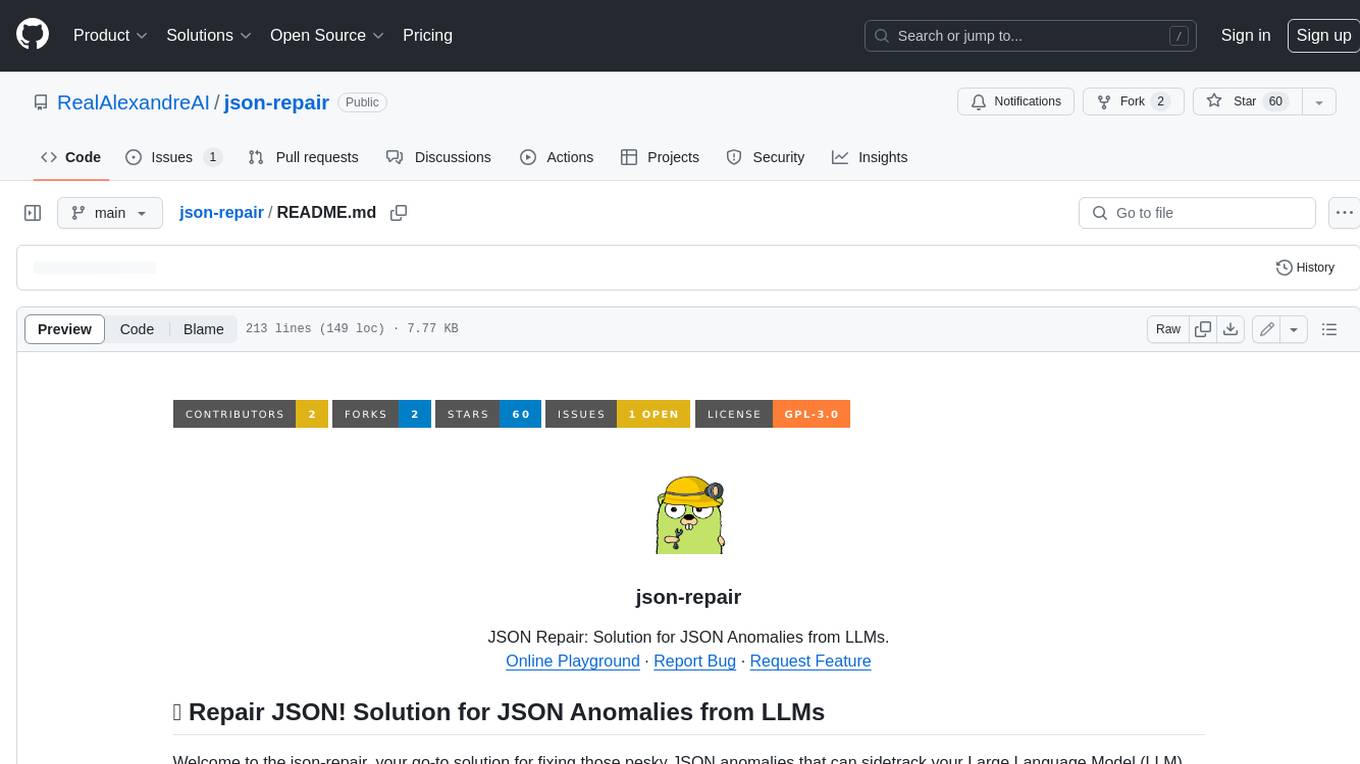
json-repair
JSON Repair is a toolkit designed to address JSON anomalies that can arise from Large Language Models (LLMs). It offers a comprehensive solution for repairing JSON strings, ensuring accuracy and reliability in your data processing. With its user-friendly interface and extensive capabilities, JSON Repair empowers developers to seamlessly integrate JSON repair into their workflows.
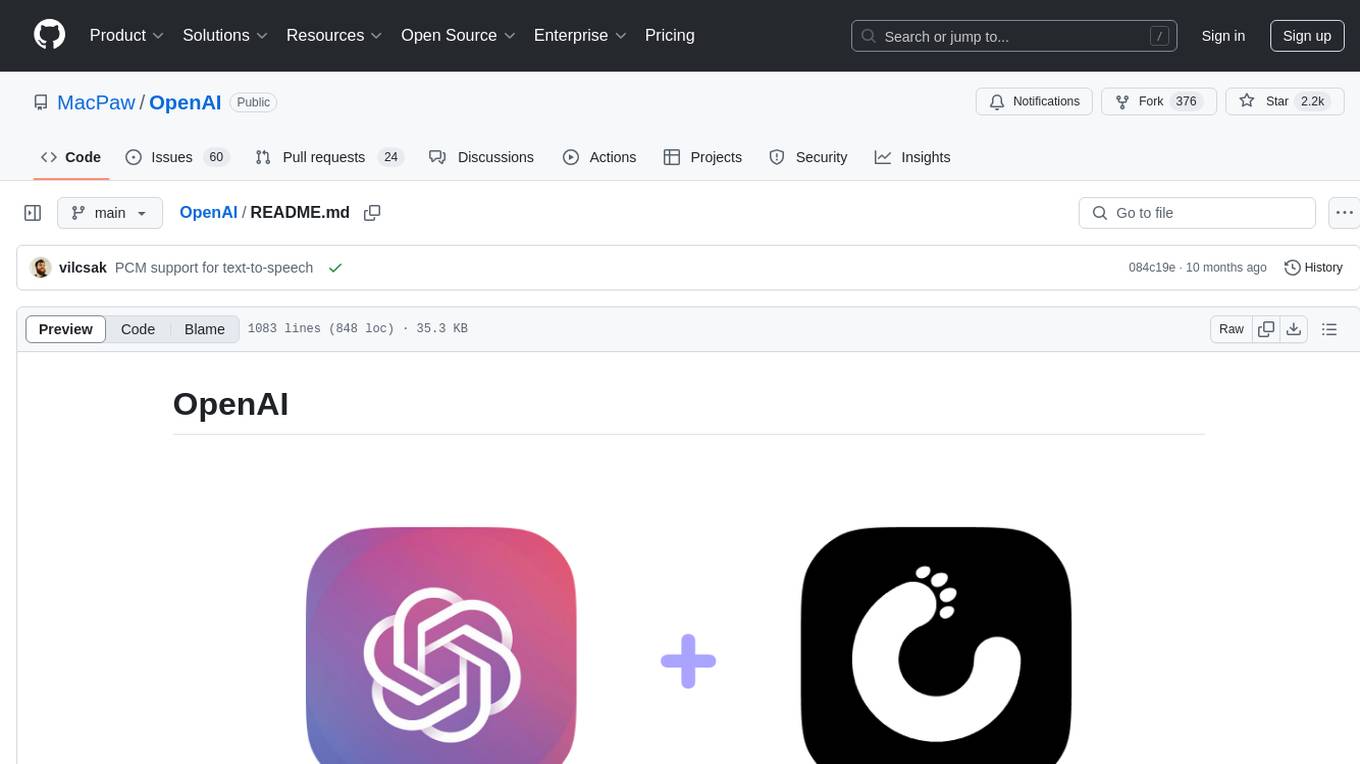
OpenAI
OpenAI is a Swift community-maintained implementation over OpenAI public API. It is a non-profit artificial intelligence research organization founded in San Francisco, California in 2015. OpenAI's mission is to ensure safe and responsible use of AI for civic good, economic growth, and other public benefits. The repository provides functionalities for text completions, chats, image generation, audio processing, edits, embeddings, models, moderations, utilities, and Combine extensions.
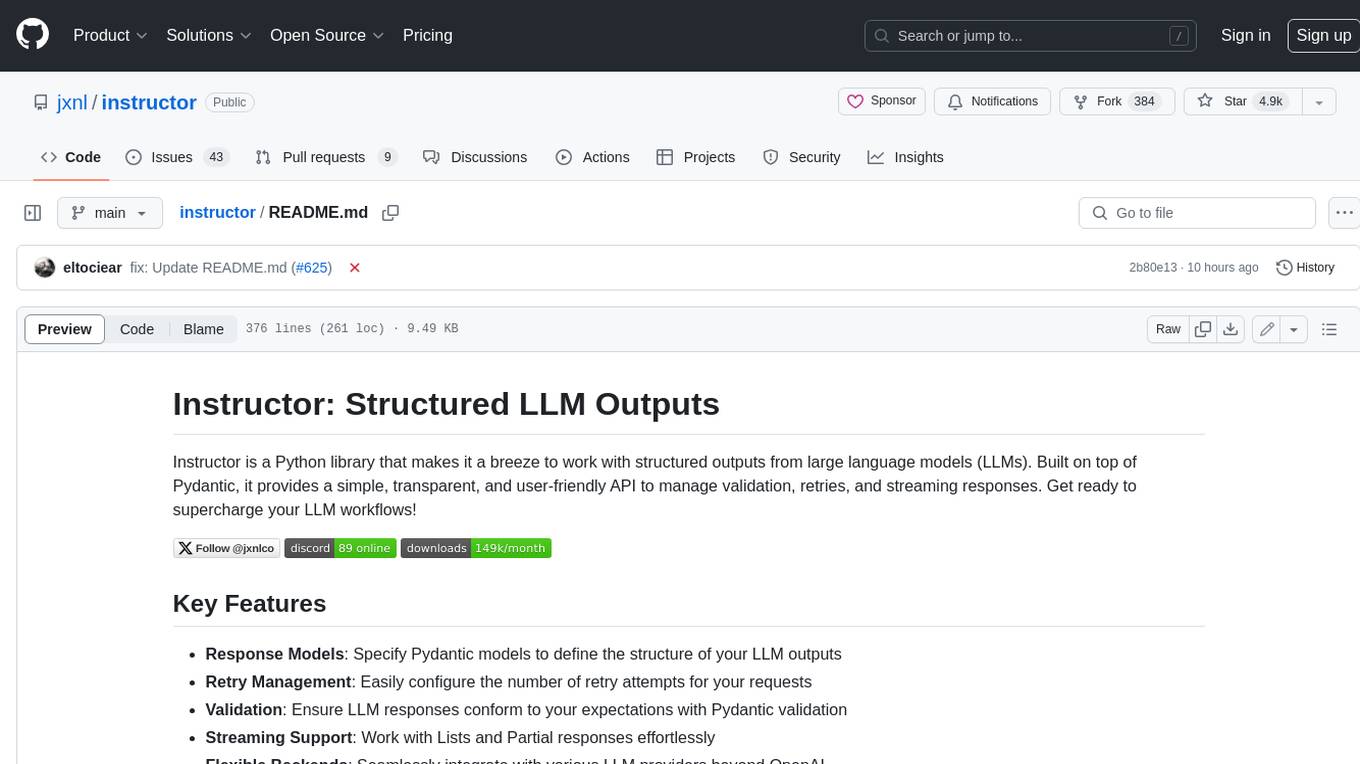
instructor
Instructor is a Python library that makes it a breeze to work with structured outputs from large language models (LLMs). Built on top of Pydantic, it provides a simple, transparent, and user-friendly API to manage validation, retries, and streaming responses. Get ready to supercharge your LLM workflows!
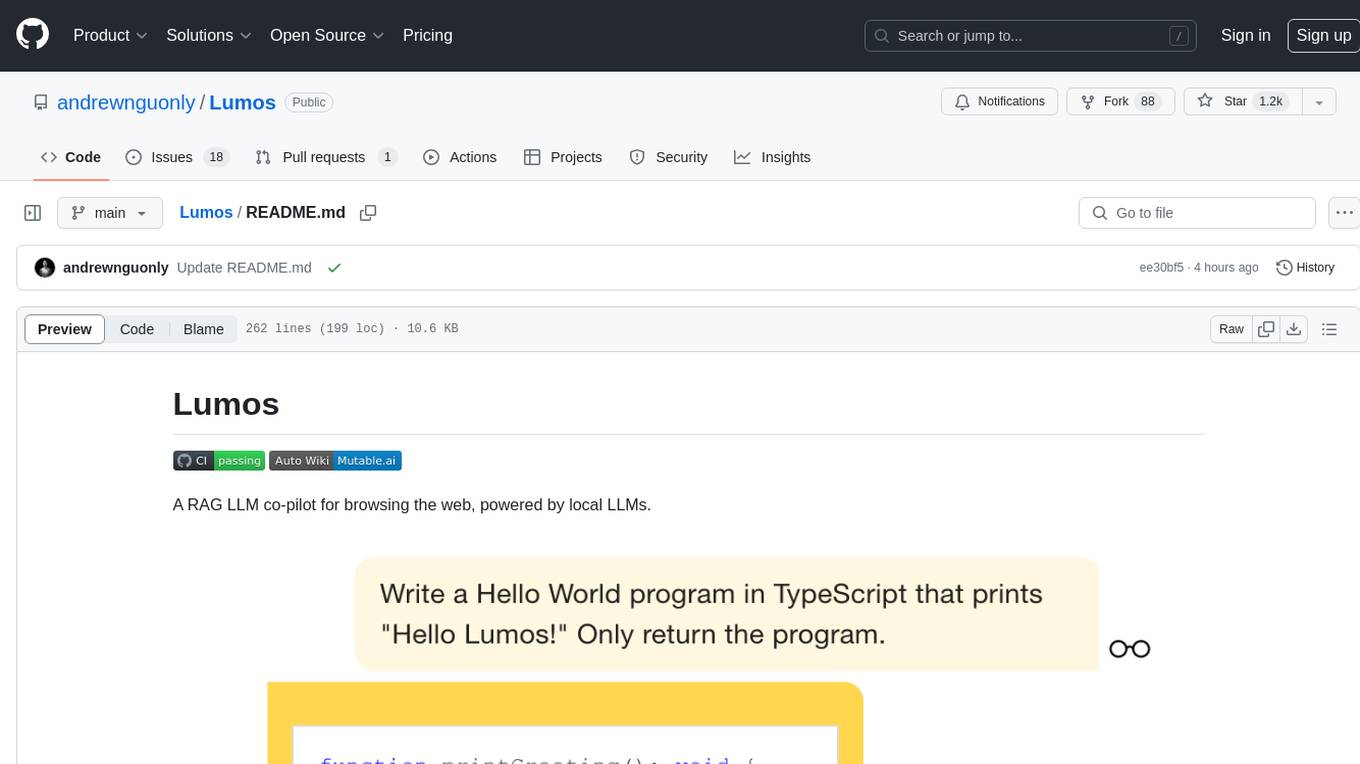
Lumos
Lumos is a Chrome extension powered by a local LLM co-pilot for browsing the web. It allows users to summarize long threads, news articles, and technical documentation. Users can ask questions about reviews and product pages. The tool requires a local Ollama server for LLM inference and embedding database. Lumos supports multimodal models and file attachments for processing text and image content. It also provides options to customize models, hosts, and content parsers. The extension can be easily accessed through keyboard shortcuts and offers tools for automatic invocation based on prompts.
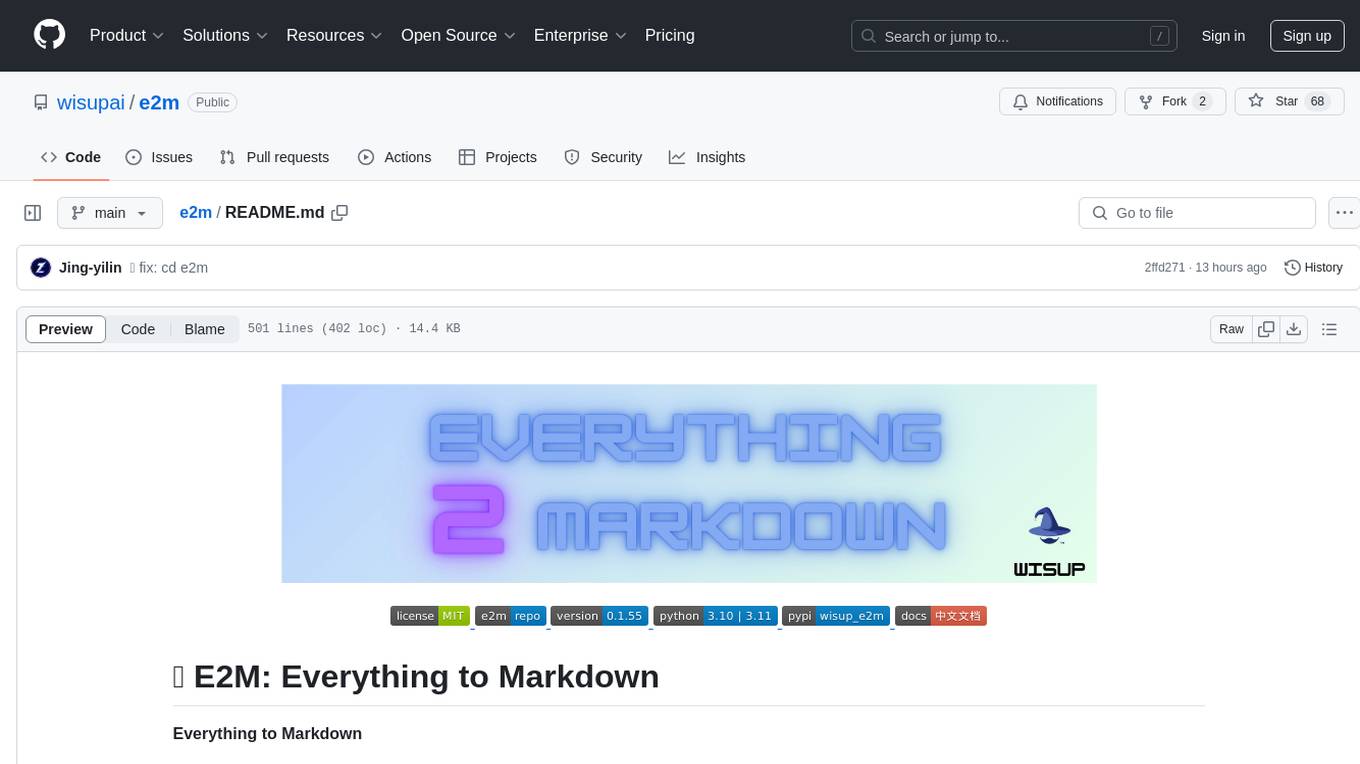
e2m
E2M is a Python library that can parse and convert various file types into Markdown format. It supports the conversion of multiple file formats, including doc, docx, epub, html, htm, url, pdf, ppt, pptx, mp3, and m4a. The ultimate goal of the E2M project is to provide high-quality data for Retrieval-Augmented Generation (RAG) and model training or fine-tuning. The core architecture consists of a Parser responsible for parsing various file types into text or image data, and a Converter responsible for converting text or image data into Markdown format.
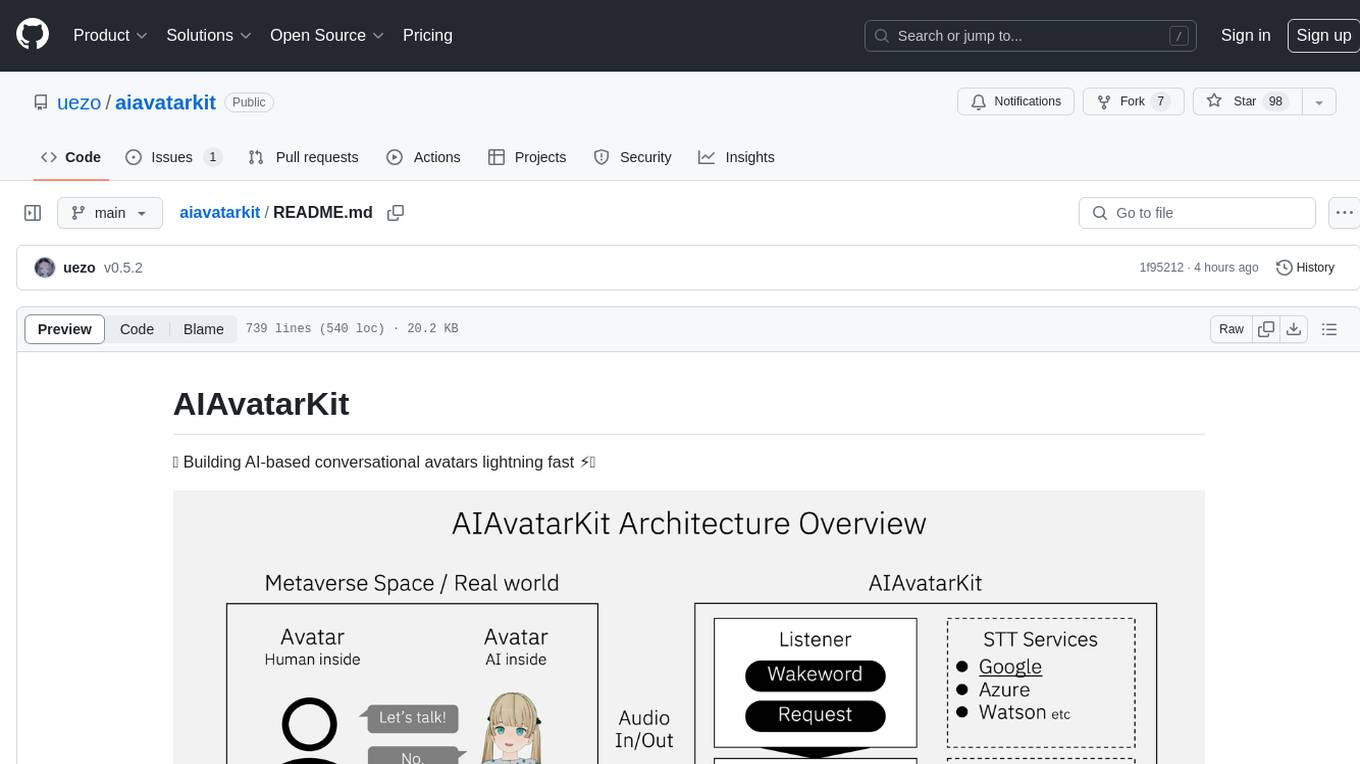
aiavatarkit
AIAvatarKit is a tool for building AI-based conversational avatars quickly. It supports various platforms like VRChat and cluster, along with real-world devices. The tool is extensible, allowing unlimited capabilities based on user needs. It requires VOICEVOX API, Google or Azure Speech Services API keys, and Python 3.10. Users can start conversations out of the box and enjoy seamless interactions with the avatars.
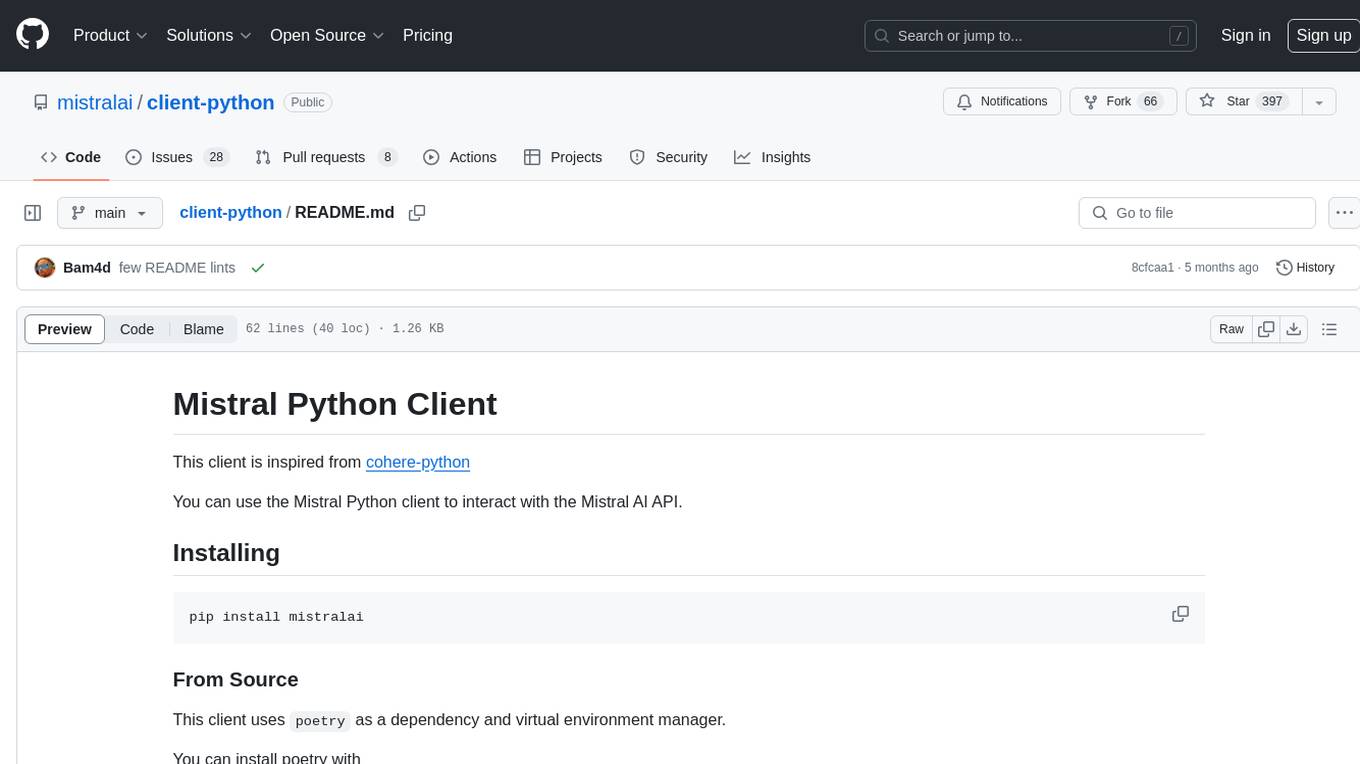
client-python
The Mistral Python Client is a tool inspired by cohere-python that allows users to interact with the Mistral AI API. It provides functionalities to access and utilize the AI capabilities offered by Mistral. Users can easily install the client using pip and manage dependencies using poetry. The client includes examples demonstrating how to use the API for various tasks, such as chat interactions. To get started, users need to obtain a Mistral API Key and set it as an environment variable. Overall, the Mistral Python Client simplifies the integration of Mistral AI services into Python applications.
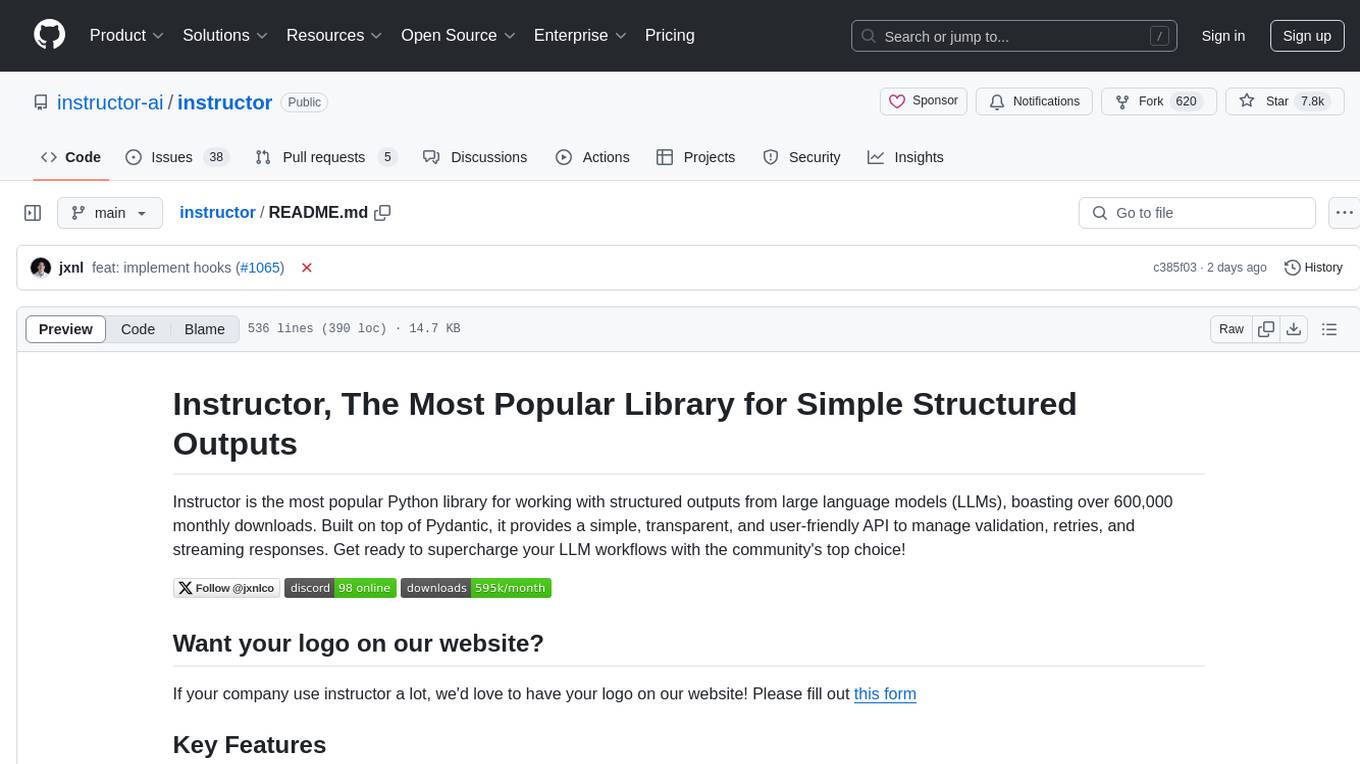
instructor
Instructor is a popular Python library for managing structured outputs from large language models (LLMs). It offers a user-friendly API for validation, retries, and streaming responses. With support for various LLM providers and multiple languages, Instructor simplifies working with LLM outputs. The library includes features like response models, retry management, validation, streaming support, and flexible backends. It also provides hooks for logging and monitoring LLM interactions, and supports integration with Anthropic, Cohere, Gemini, Litellm, and Google AI models. Instructor facilitates tasks such as extracting user data from natural language, creating fine-tuned models, managing uploaded files, and monitoring usage of OpenAI models.
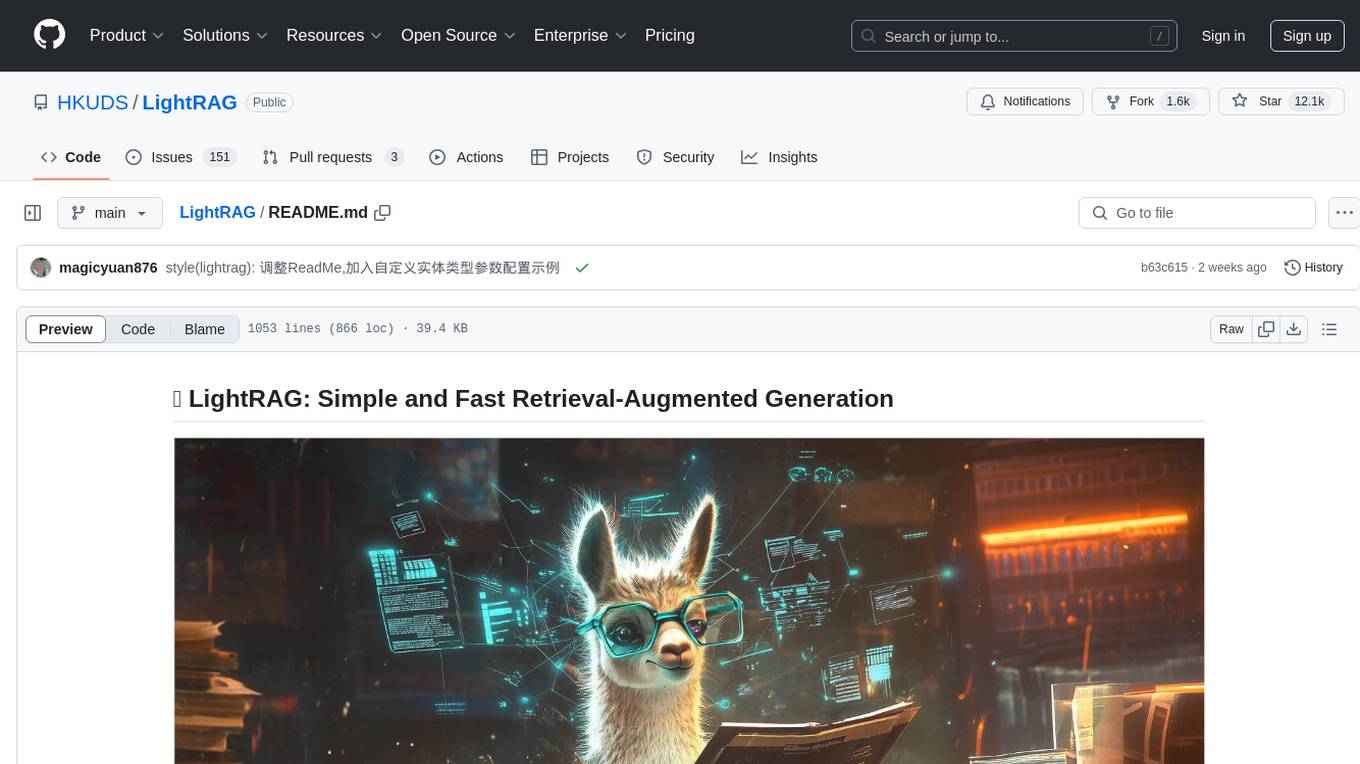
LightRAG
LightRAG is a repository hosting the code for LightRAG, a system that supports seamless integration of custom knowledge graphs, Oracle Database 23ai, Neo4J for storage, and multiple file types. It includes features like entity deletion, batch insert, incremental insert, and graph visualization. LightRAG provides an API server implementation for RESTful API access to RAG operations, allowing users to interact with it through HTTP requests. The repository also includes evaluation scripts, code for reproducing results, and a comprehensive code structure.
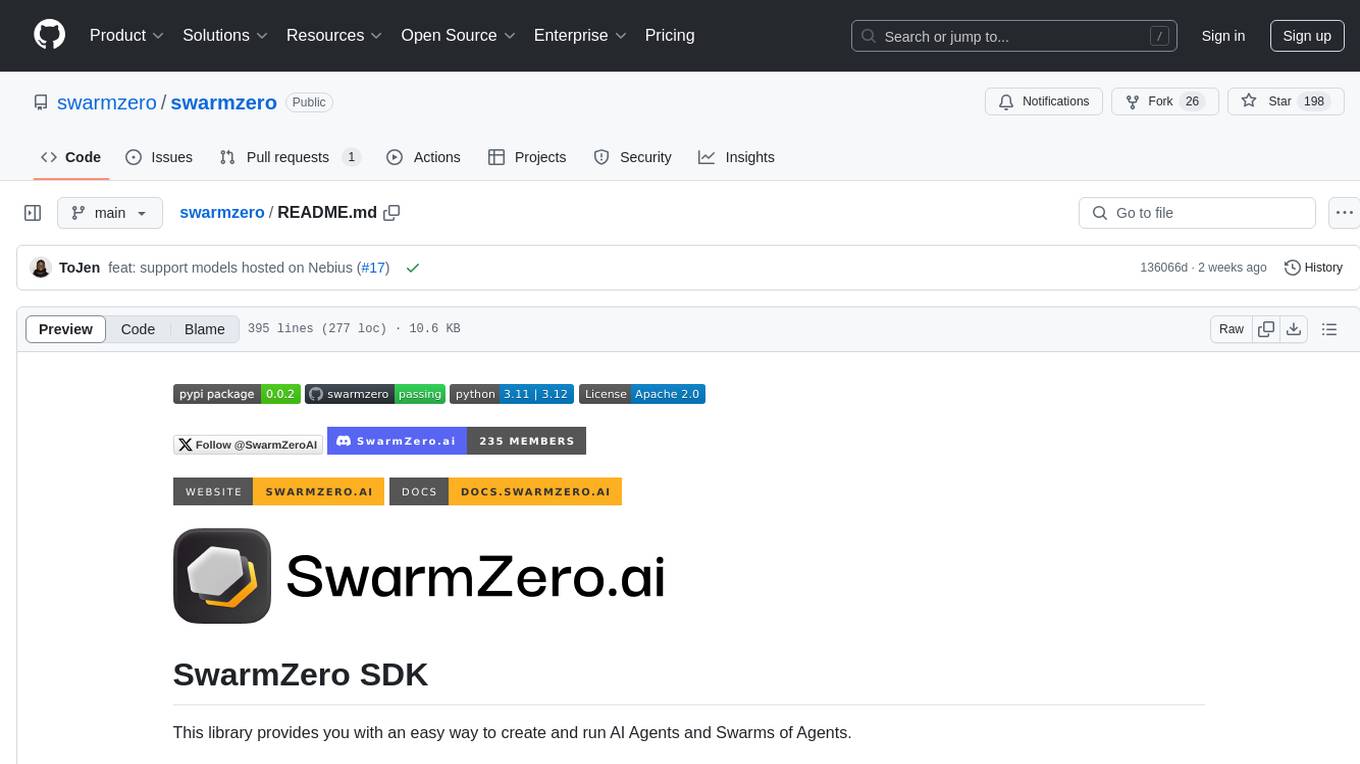
swarmzero
SwarmZero SDK is a library that simplifies the creation and execution of AI Agents and Swarms of Agents. It supports various LLM Providers such as OpenAI, Azure OpenAI, Anthropic, MistralAI, Gemini, Nebius, and Ollama. Users can easily install the library using pip or poetry, set up the environment and configuration, create and run Agents, collaborate with Swarms, add tools for complex tasks, and utilize retriever tools for semantic information retrieval. Sample prompts are provided to help users explore the capabilities of the agents and swarms. The SDK also includes detailed examples and documentation for reference.
For similar tasks
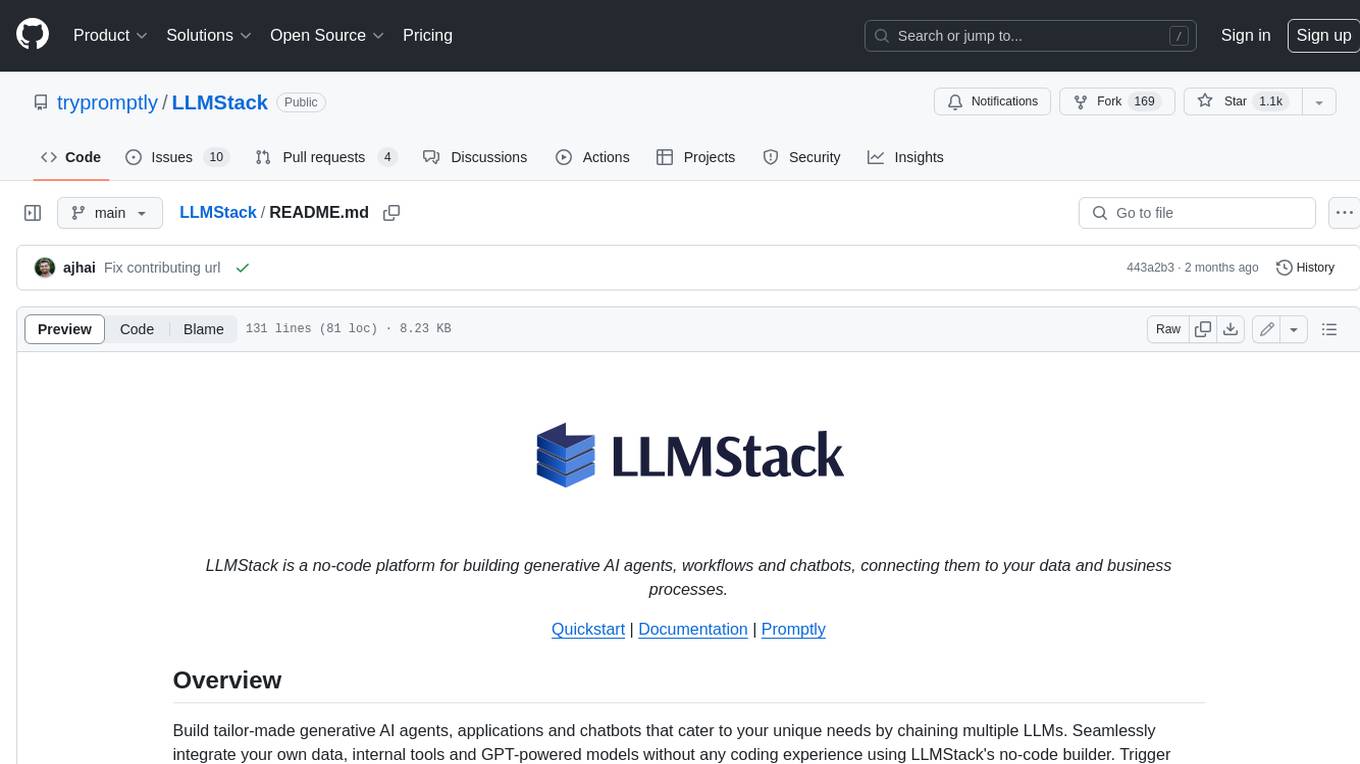
LLMStack
LLMStack is a no-code platform for building generative AI agents, workflows, and chatbots. It allows users to connect their own data, internal tools, and GPT-powered models without any coding experience. LLMStack can be deployed to the cloud or on-premise and can be accessed via HTTP API or triggered from Slack or Discord.
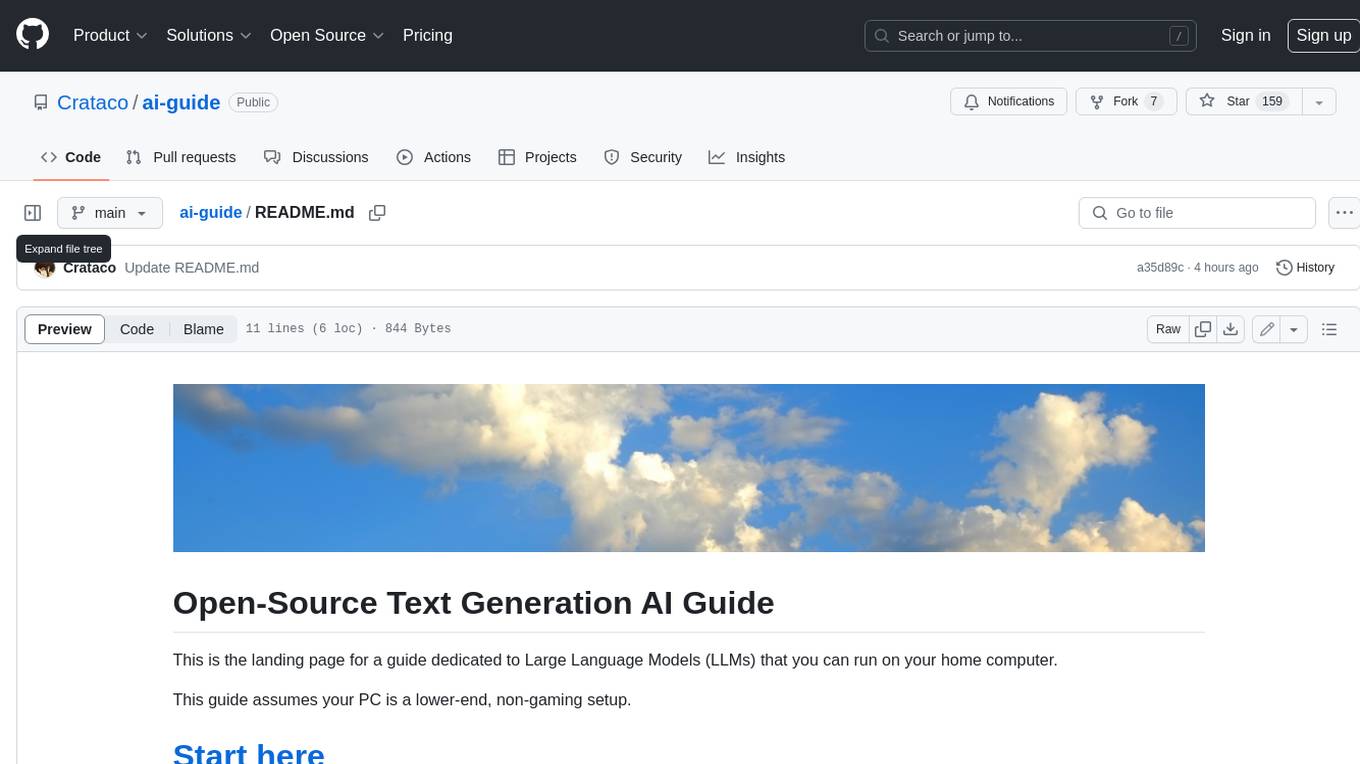
ai-guide
This guide is dedicated to Large Language Models (LLMs) that you can run on your home computer. It assumes your PC is a lower-end, non-gaming setup.
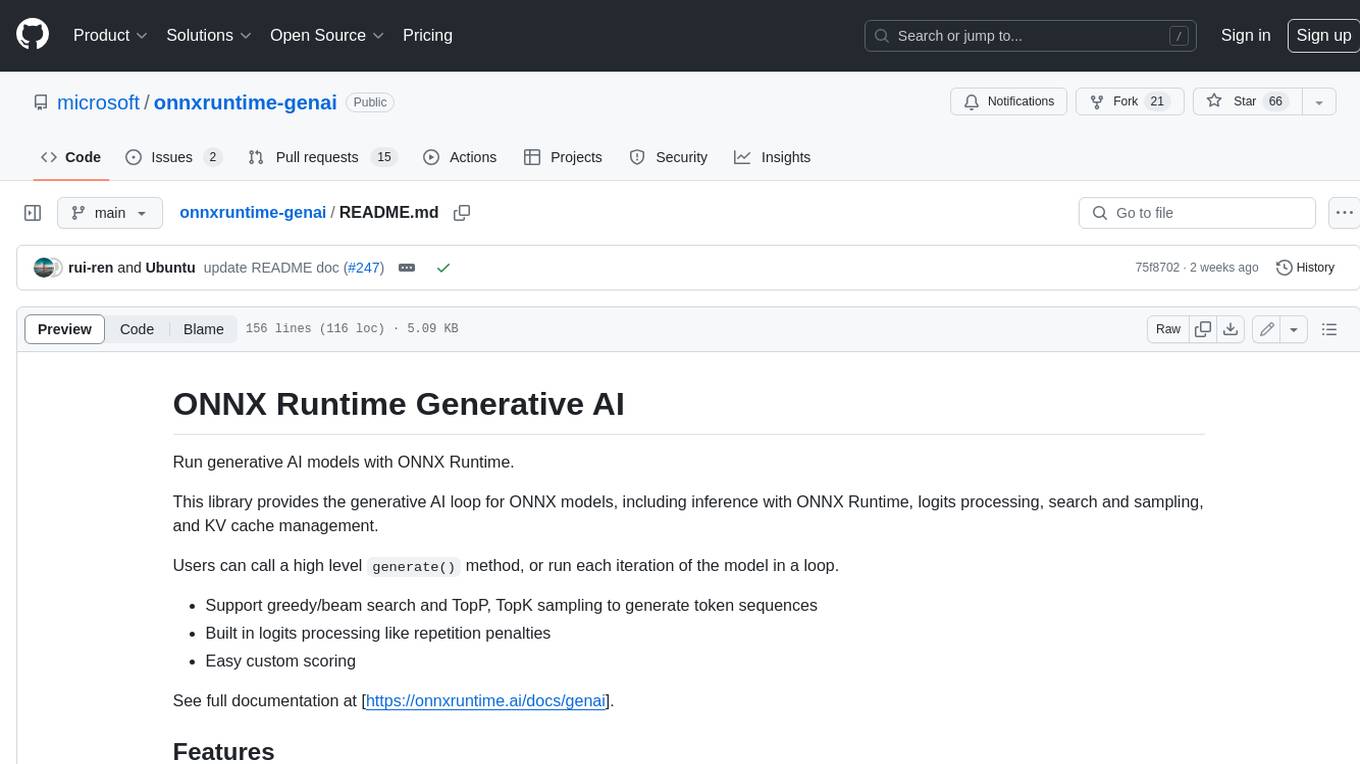
onnxruntime-genai
ONNX Runtime Generative AI is a library that provides the generative AI loop for ONNX models, including inference with ONNX Runtime, logits processing, search and sampling, and KV cache management. Users can call a high level `generate()` method, or run each iteration of the model in a loop. It supports greedy/beam search and TopP, TopK sampling to generate token sequences, has built in logits processing like repetition penalties, and allows for easy custom scoring.
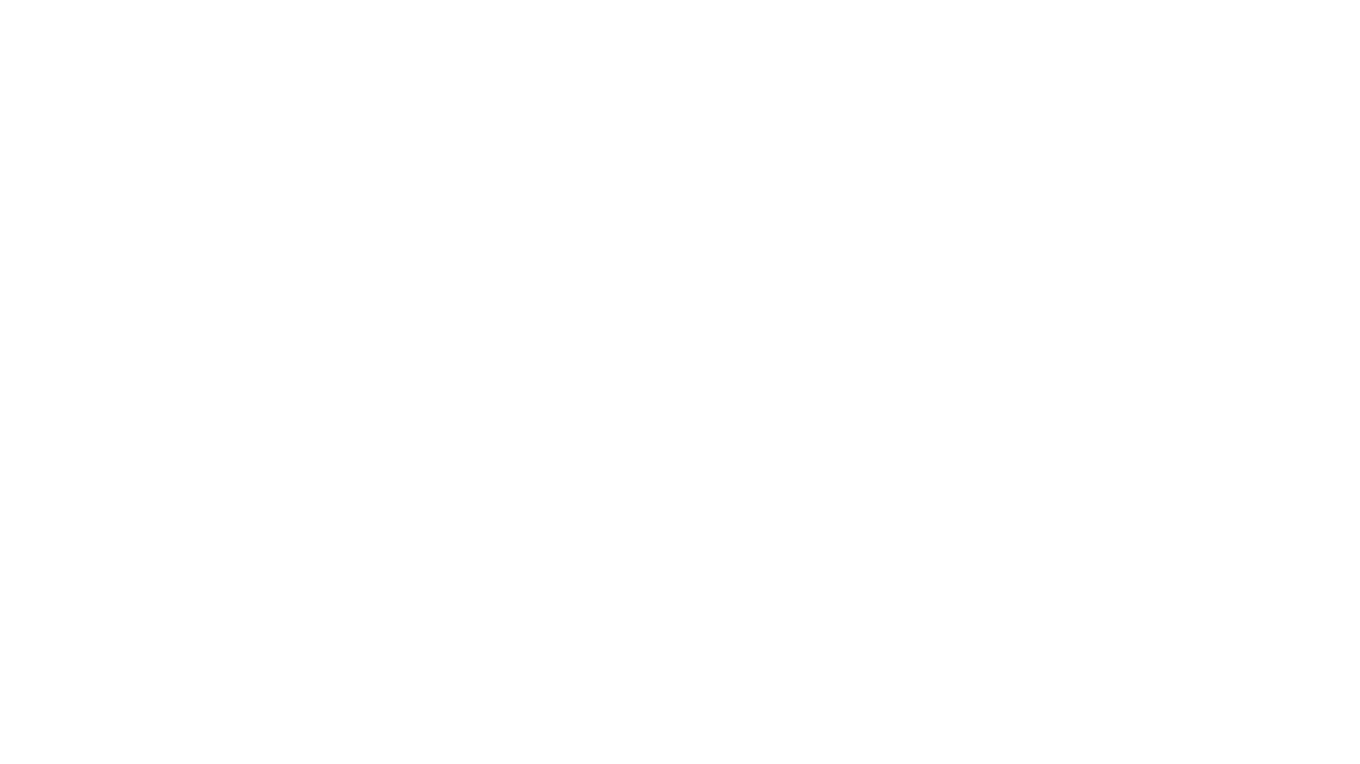
jupyter-ai
Jupyter AI connects generative AI with Jupyter notebooks. It provides a user-friendly and powerful way to explore generative AI models in notebooks and improve your productivity in JupyterLab and the Jupyter Notebook. Specifically, Jupyter AI offers: * An `%%ai` magic that turns the Jupyter notebook into a reproducible generative AI playground. This works anywhere the IPython kernel runs (JupyterLab, Jupyter Notebook, Google Colab, Kaggle, VSCode, etc.). * A native chat UI in JupyterLab that enables you to work with generative AI as a conversational assistant. * Support for a wide range of generative model providers, including AI21, Anthropic, AWS, Cohere, Gemini, Hugging Face, NVIDIA, and OpenAI. * Local model support through GPT4All, enabling use of generative AI models on consumer grade machines with ease and privacy.
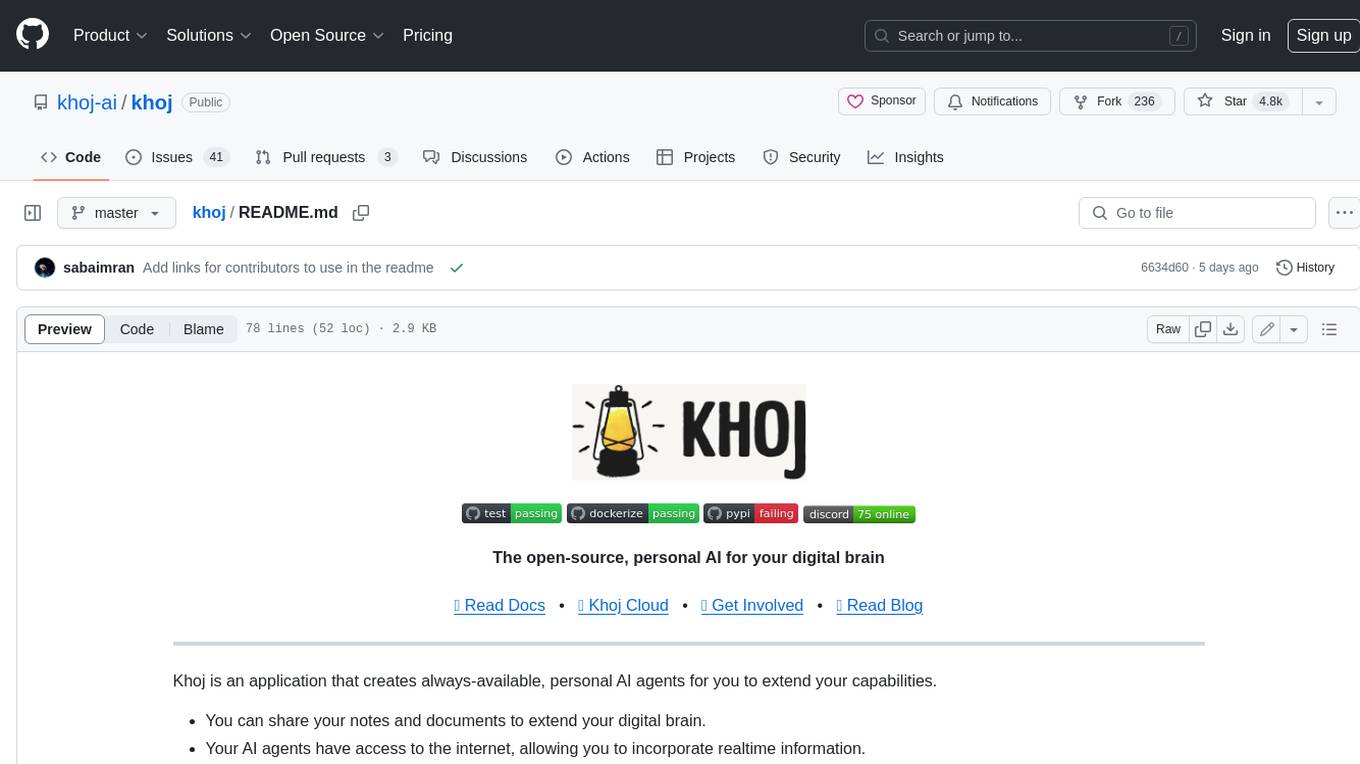
khoj
Khoj is an open-source, personal AI assistant that extends your capabilities by creating always-available AI agents. You can share your notes and documents to extend your digital brain, and your AI agents have access to the internet, allowing you to incorporate real-time information. Khoj is accessible on Desktop, Emacs, Obsidian, Web, and Whatsapp, and you can share PDF, markdown, org-mode, notion files, and GitHub repositories. You'll get fast, accurate semantic search on top of your docs, and your agents can create deeply personal images and understand your speech. Khoj is self-hostable and always will be.
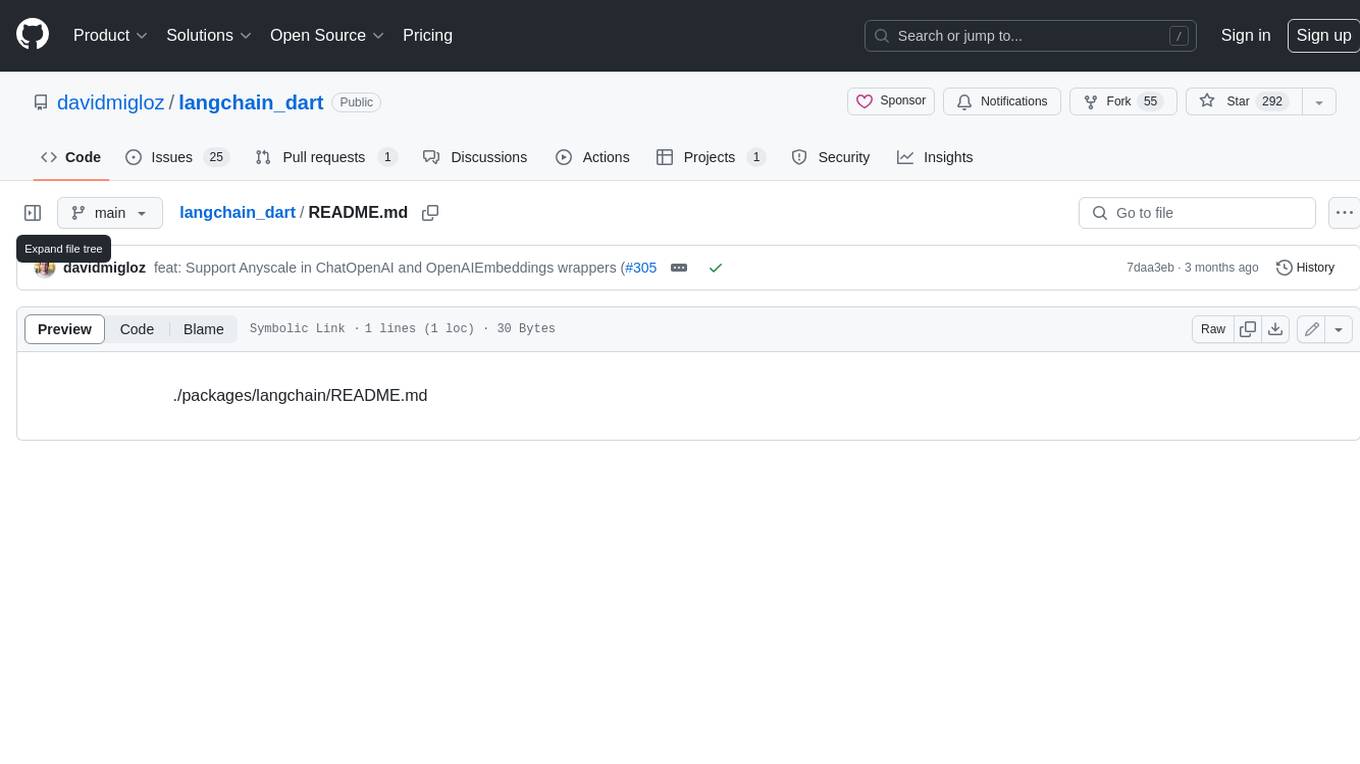
langchain_dart
LangChain.dart is a Dart port of the popular LangChain Python framework created by Harrison Chase. LangChain provides a set of ready-to-use components for working with language models and a standard interface for chaining them together to formulate more advanced use cases (e.g. chatbots, Q&A with RAG, agents, summarization, extraction, etc.). The components can be grouped into a few core modules: * **Model I/O:** LangChain offers a unified API for interacting with various LLM providers (e.g. OpenAI, Google, Mistral, Ollama, etc.), allowing developers to switch between them with ease. Additionally, it provides tools for managing model inputs (prompt templates and example selectors) and parsing the resulting model outputs (output parsers). * **Retrieval:** assists in loading user data (via document loaders), transforming it (with text splitters), extracting its meaning (using embedding models), storing (in vector stores) and retrieving it (through retrievers) so that it can be used to ground the model's responses (i.e. Retrieval-Augmented Generation or RAG). * **Agents:** "bots" that leverage LLMs to make informed decisions about which available tools (such as web search, calculators, database lookup, etc.) to use to accomplish the designated task. The different components can be composed together using the LangChain Expression Language (LCEL).
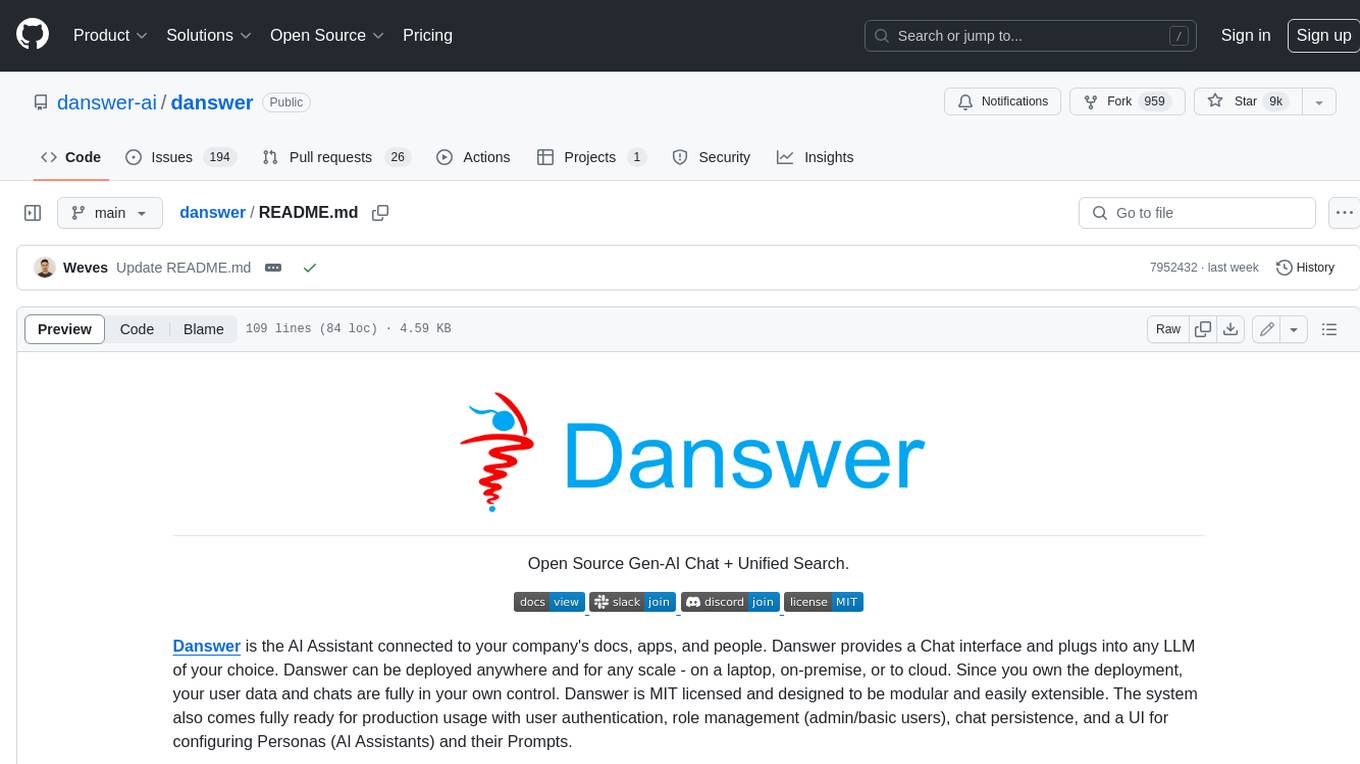
danswer
Danswer is an open-source Gen-AI Chat and Unified Search tool that connects to your company's docs, apps, and people. It provides a Chat interface and plugs into any LLM of your choice. Danswer can be deployed anywhere and for any scale - on a laptop, on-premise, or to cloud. Since you own the deployment, your user data and chats are fully in your own control. Danswer is MIT licensed and designed to be modular and easily extensible. The system also comes fully ready for production usage with user authentication, role management (admin/basic users), chat persistence, and a UI for configuring Personas (AI Assistants) and their Prompts. Danswer also serves as a Unified Search across all common workplace tools such as Slack, Google Drive, Confluence, etc. By combining LLMs and team specific knowledge, Danswer becomes a subject matter expert for the team. Imagine ChatGPT if it had access to your team's unique knowledge! It enables questions such as "A customer wants feature X, is this already supported?" or "Where's the pull request for feature Y?"
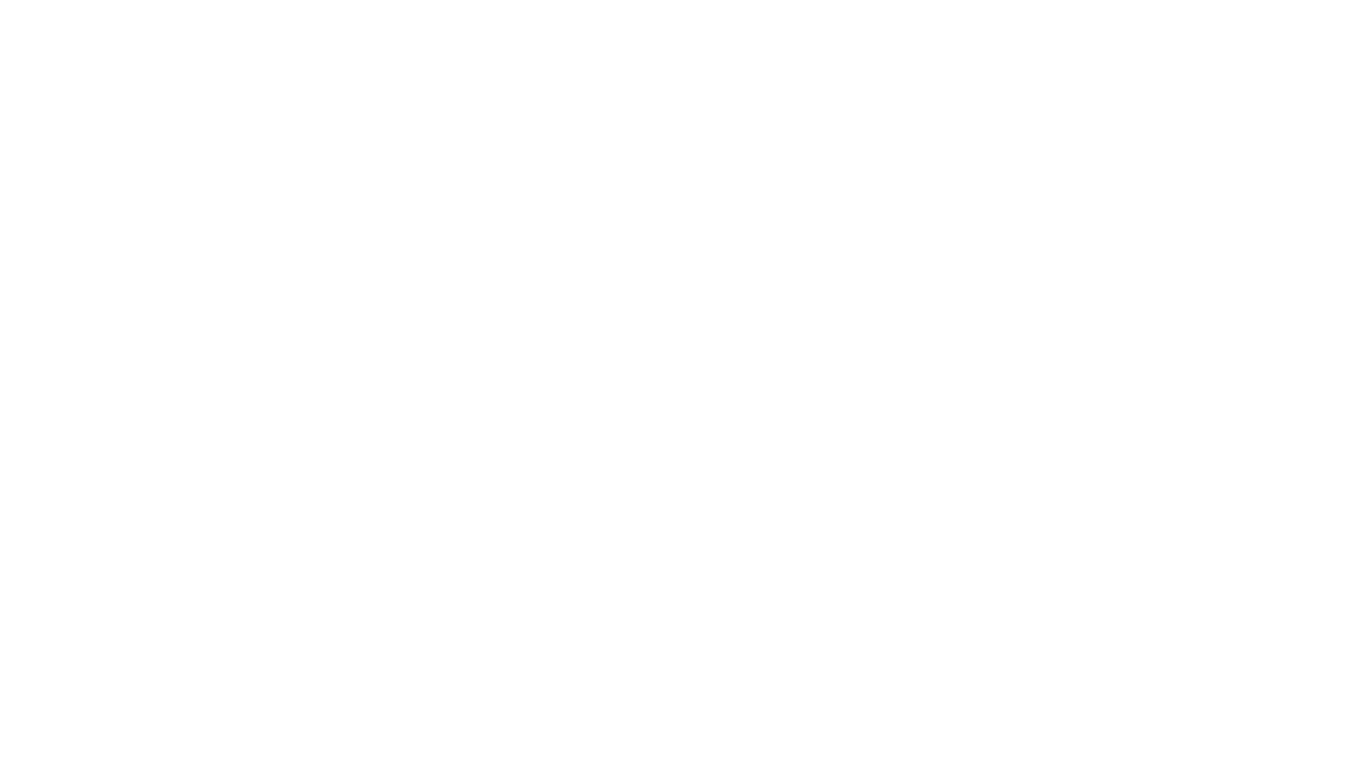
infinity
Infinity is an AI-native database designed for LLM applications, providing incredibly fast full-text and vector search capabilities. It supports a wide range of data types, including vectors, full-text, and structured data, and offers a fused search feature that combines multiple embeddings and full text. Infinity is easy to use, with an intuitive Python API and a single-binary architecture that simplifies deployment. It achieves high performance, with 0.1 milliseconds query latency on million-scale vector datasets and up to 15K QPS.
For similar jobs
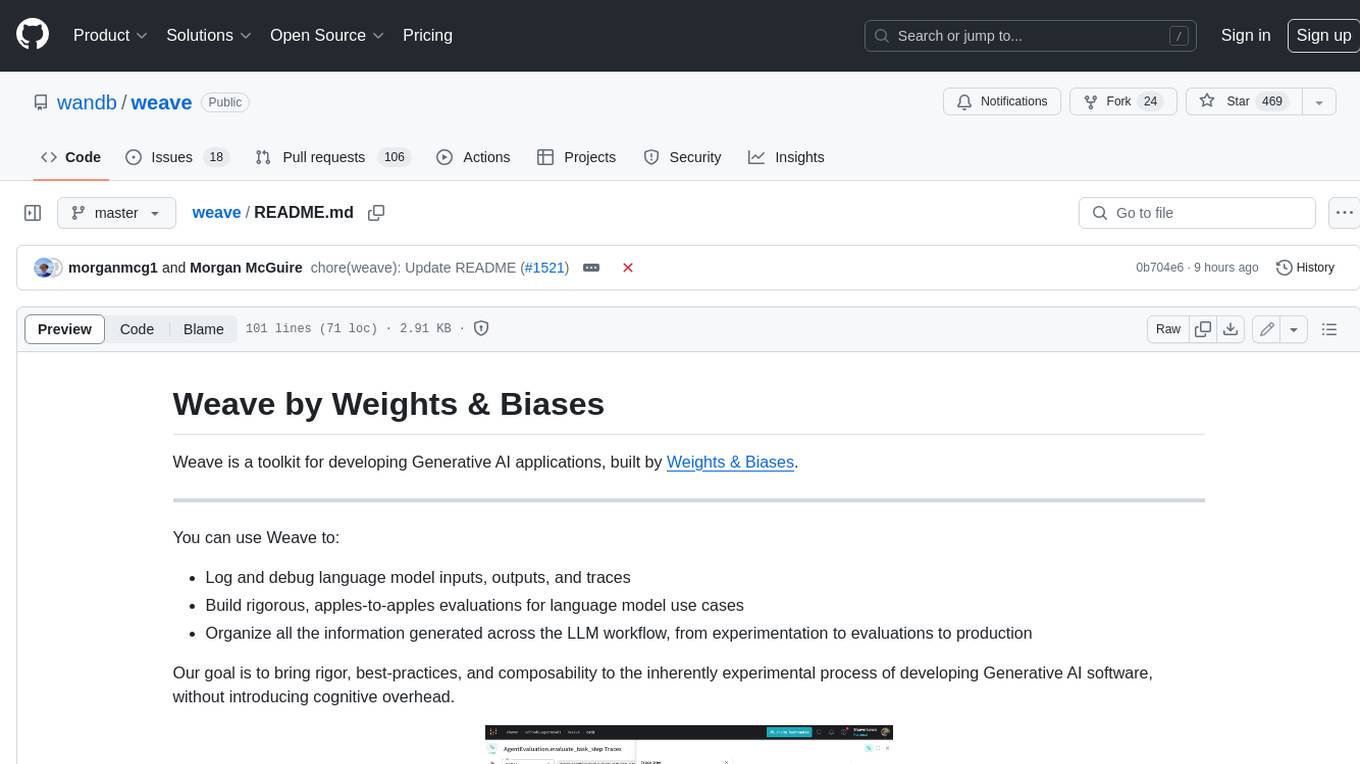
weave
Weave is a toolkit for developing Generative AI applications, built by Weights & Biases. With Weave, you can log and debug language model inputs, outputs, and traces; build rigorous, apples-to-apples evaluations for language model use cases; and organize all the information generated across the LLM workflow, from experimentation to evaluations to production. Weave aims to bring rigor, best-practices, and composability to the inherently experimental process of developing Generative AI software, without introducing cognitive overhead.
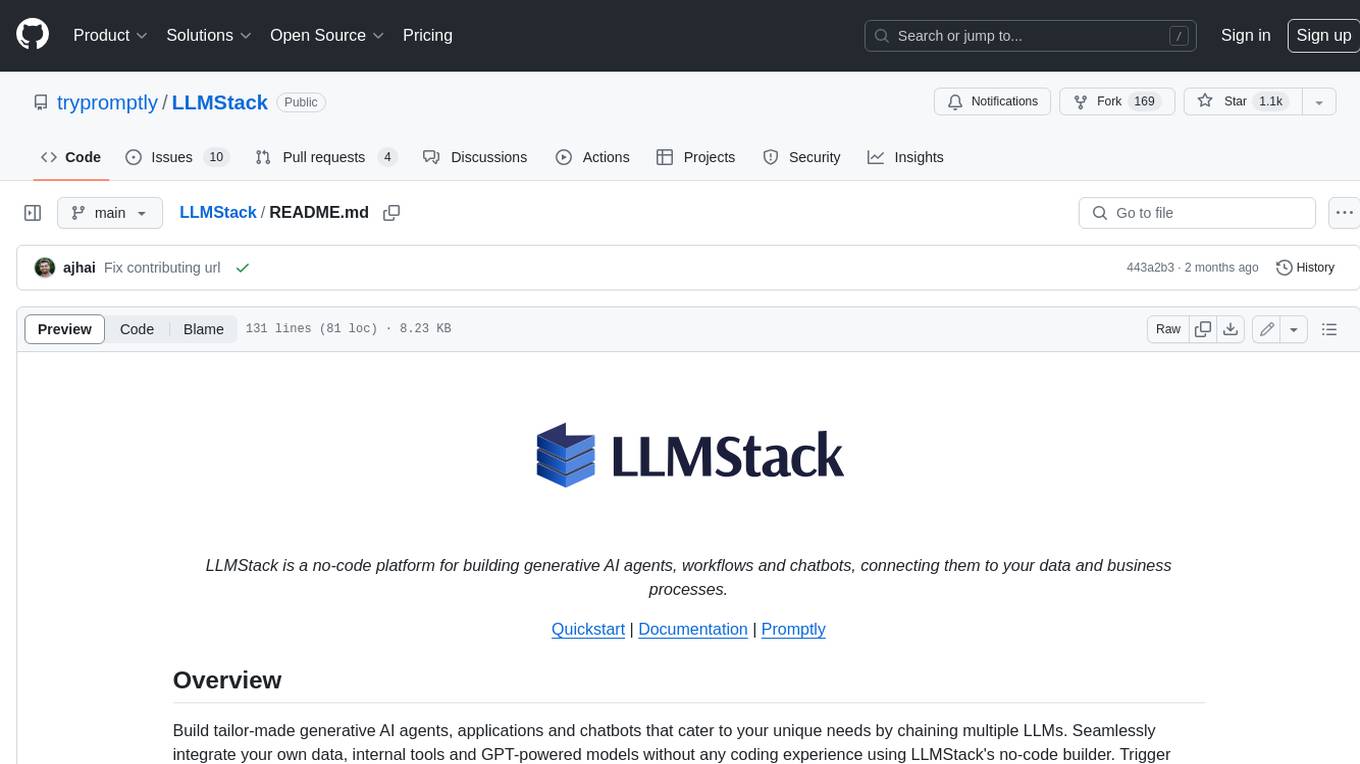
LLMStack
LLMStack is a no-code platform for building generative AI agents, workflows, and chatbots. It allows users to connect their own data, internal tools, and GPT-powered models without any coding experience. LLMStack can be deployed to the cloud or on-premise and can be accessed via HTTP API or triggered from Slack or Discord.
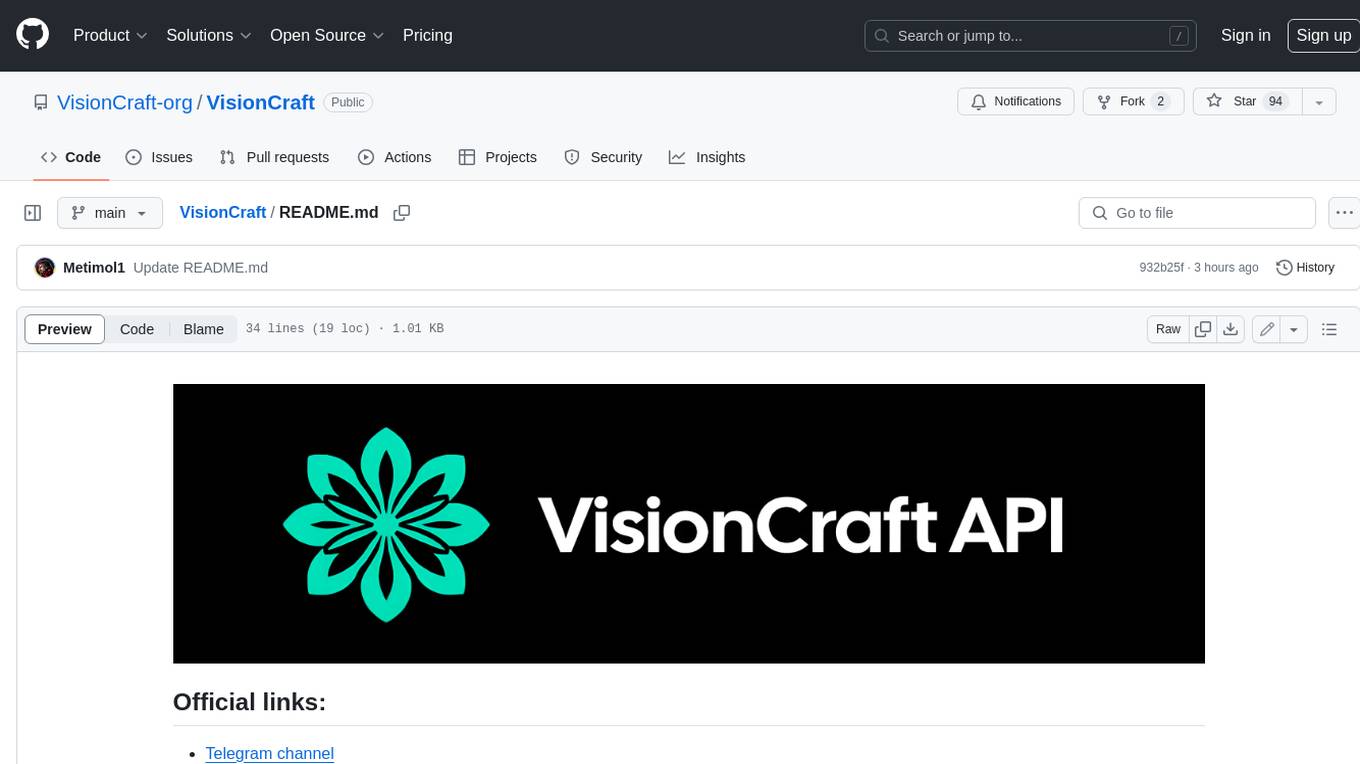
VisionCraft
The VisionCraft API is a free API for using over 100 different AI models. From images to sound.
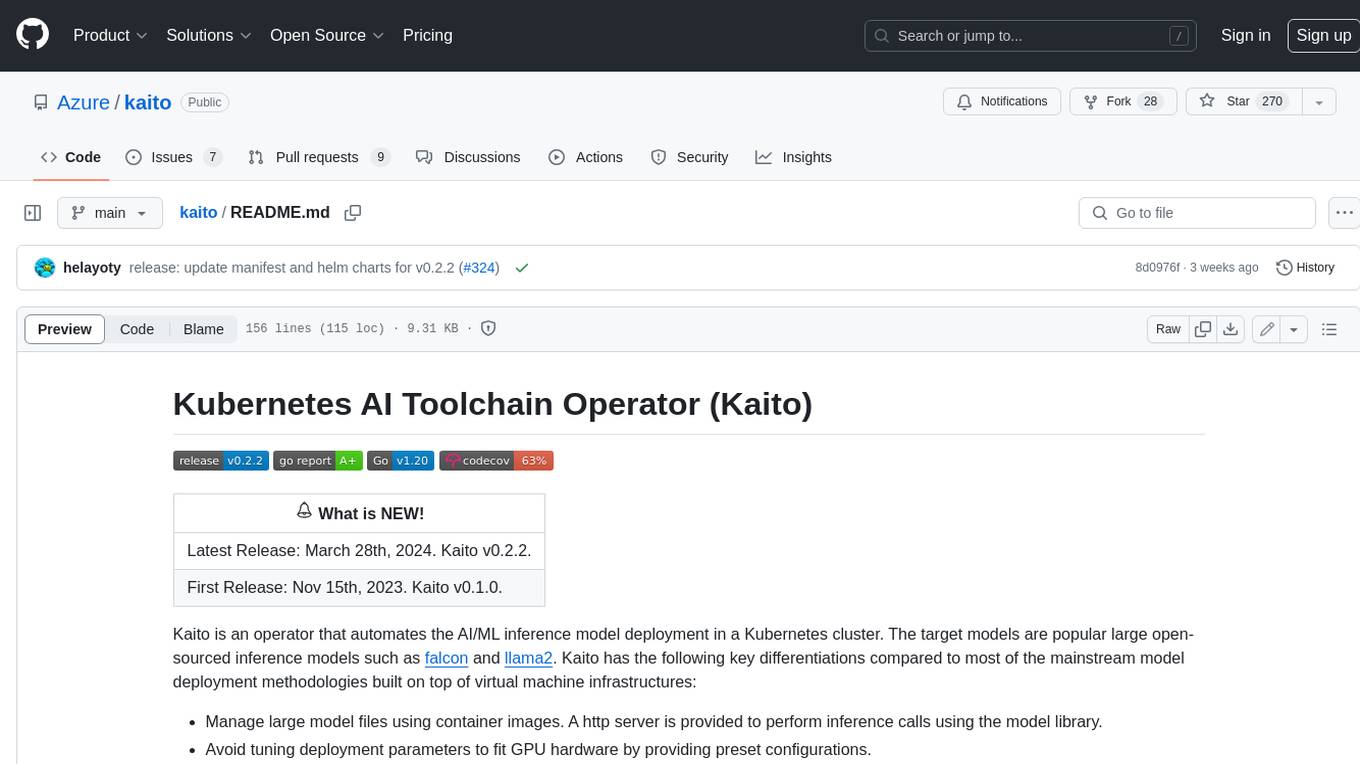
kaito
Kaito is an operator that automates the AI/ML inference model deployment in a Kubernetes cluster. It manages large model files using container images, avoids tuning deployment parameters to fit GPU hardware by providing preset configurations, auto-provisions GPU nodes based on model requirements, and hosts large model images in the public Microsoft Container Registry (MCR) if the license allows. Using Kaito, the workflow of onboarding large AI inference models in Kubernetes is largely simplified.
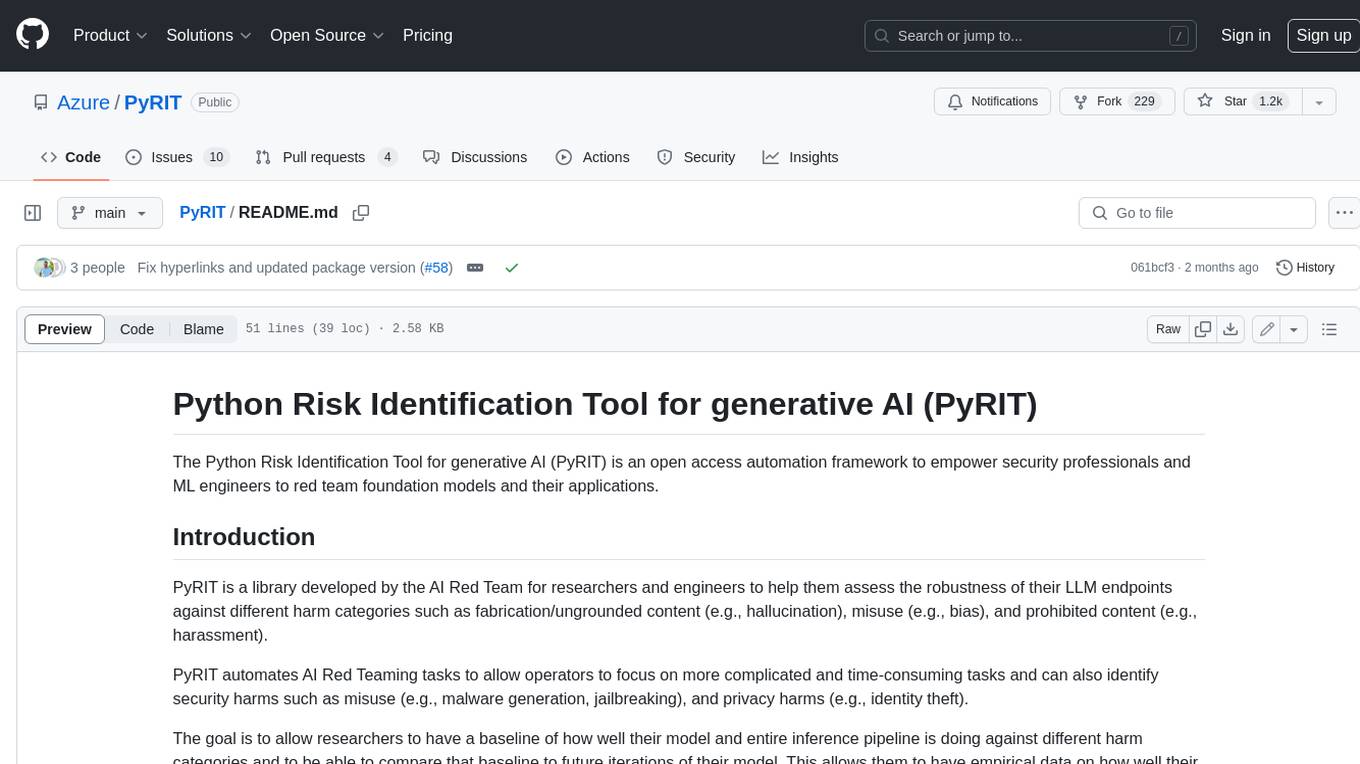
PyRIT
PyRIT is an open access automation framework designed to empower security professionals and ML engineers to red team foundation models and their applications. It automates AI Red Teaming tasks to allow operators to focus on more complicated and time-consuming tasks and can also identify security harms such as misuse (e.g., malware generation, jailbreaking), and privacy harms (e.g., identity theft). The goal is to allow researchers to have a baseline of how well their model and entire inference pipeline is doing against different harm categories and to be able to compare that baseline to future iterations of their model. This allows them to have empirical data on how well their model is doing today, and detect any degradation of performance based on future improvements.
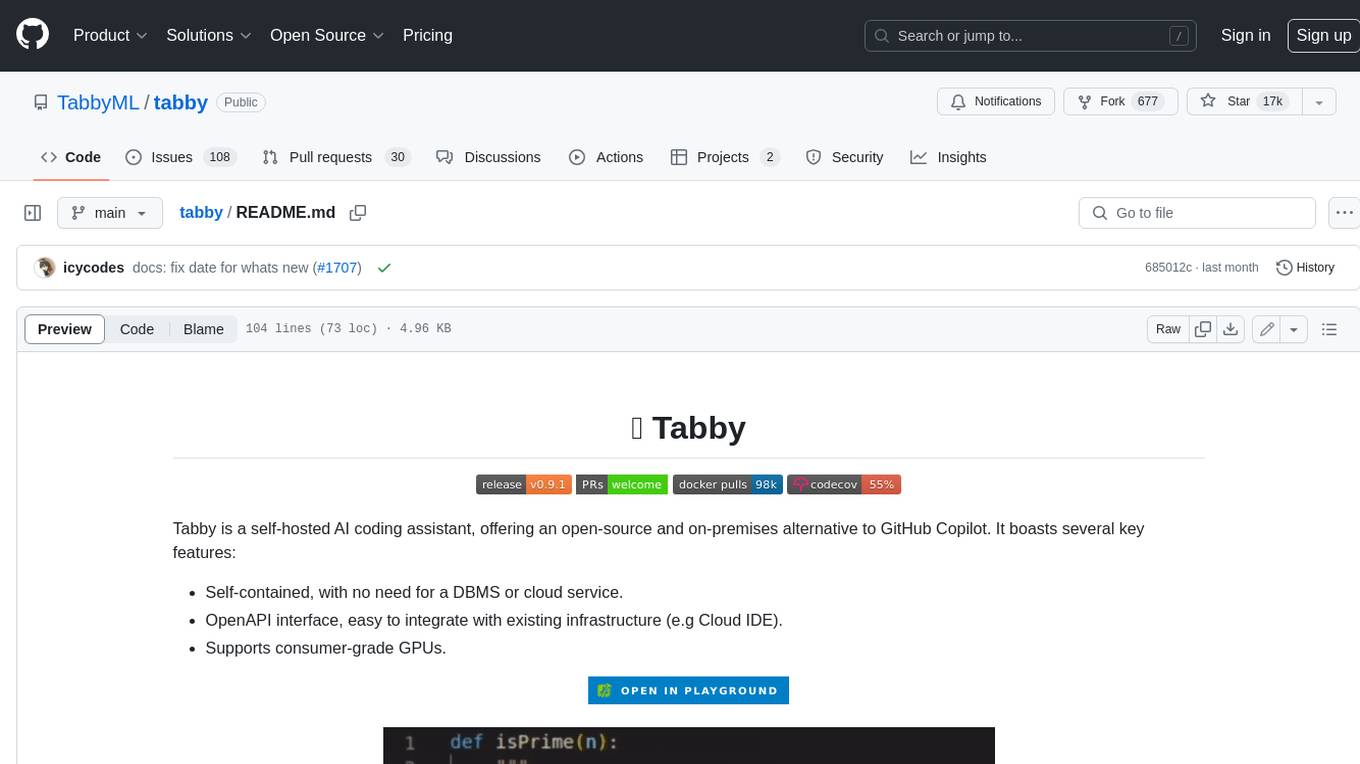
tabby
Tabby is a self-hosted AI coding assistant, offering an open-source and on-premises alternative to GitHub Copilot. It boasts several key features: * Self-contained, with no need for a DBMS or cloud service. * OpenAPI interface, easy to integrate with existing infrastructure (e.g Cloud IDE). * Supports consumer-grade GPUs.
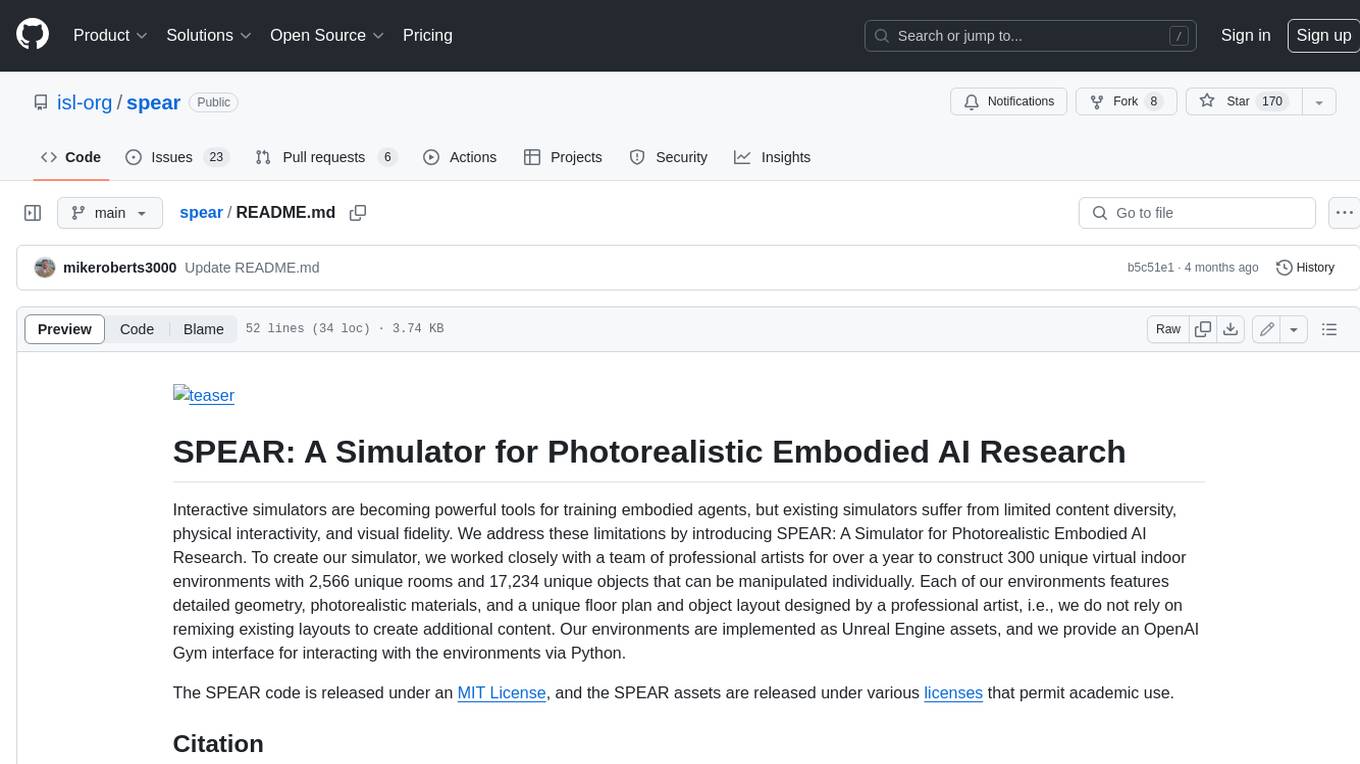
spear
SPEAR (Simulator for Photorealistic Embodied AI Research) is a powerful tool for training embodied agents. It features 300 unique virtual indoor environments with 2,566 unique rooms and 17,234 unique objects that can be manipulated individually. Each environment is designed by a professional artist and features detailed geometry, photorealistic materials, and a unique floor plan and object layout. SPEAR is implemented as Unreal Engine assets and provides an OpenAI Gym interface for interacting with the environments via Python.
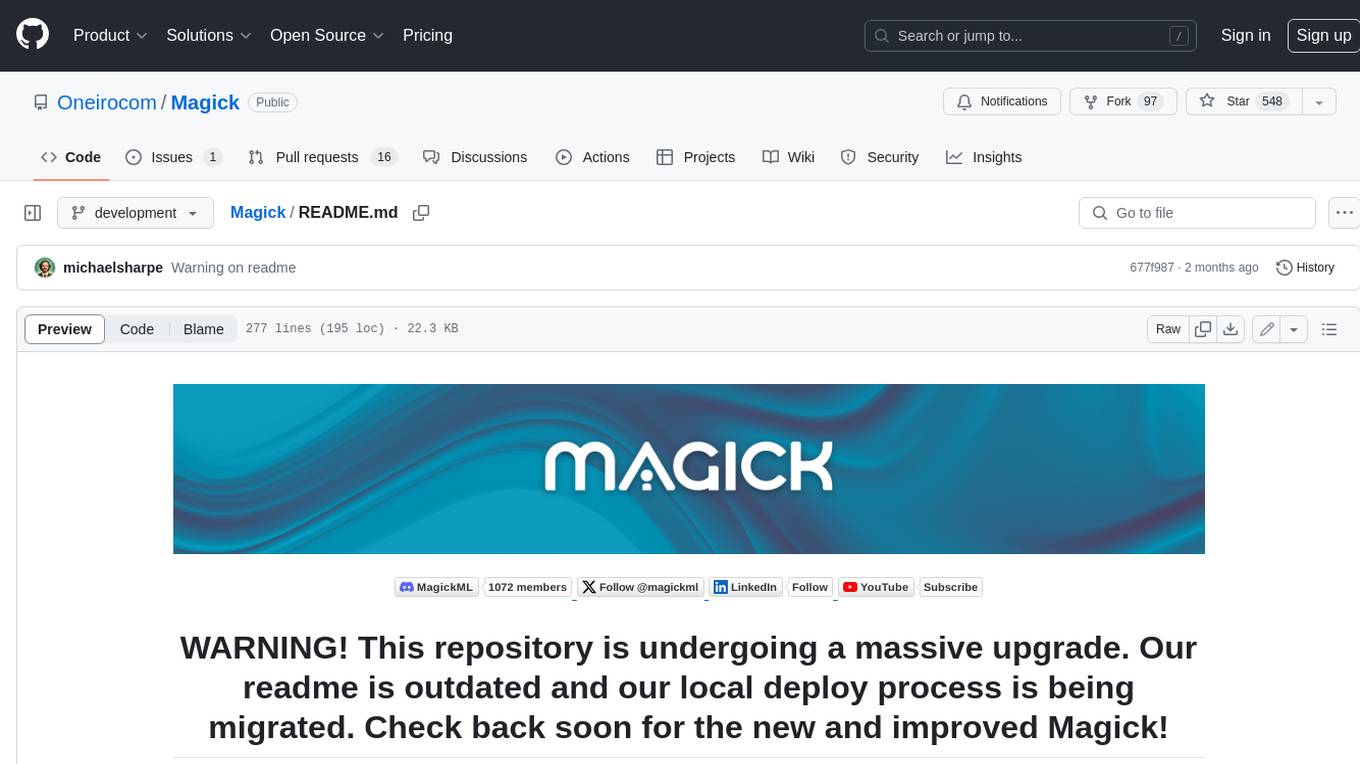
Magick
Magick is a groundbreaking visual AIDE (Artificial Intelligence Development Environment) for no-code data pipelines and multimodal agents. Magick can connect to other services and comes with nodes and templates well-suited for intelligent agents, chatbots, complex reasoning systems and realistic characters.