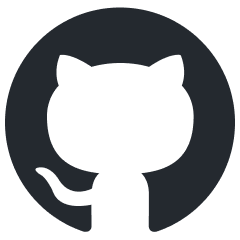
LightRAG
"LightRAG: Simple and Fast Retrieval-Augmented Generation"
Stars: 13233
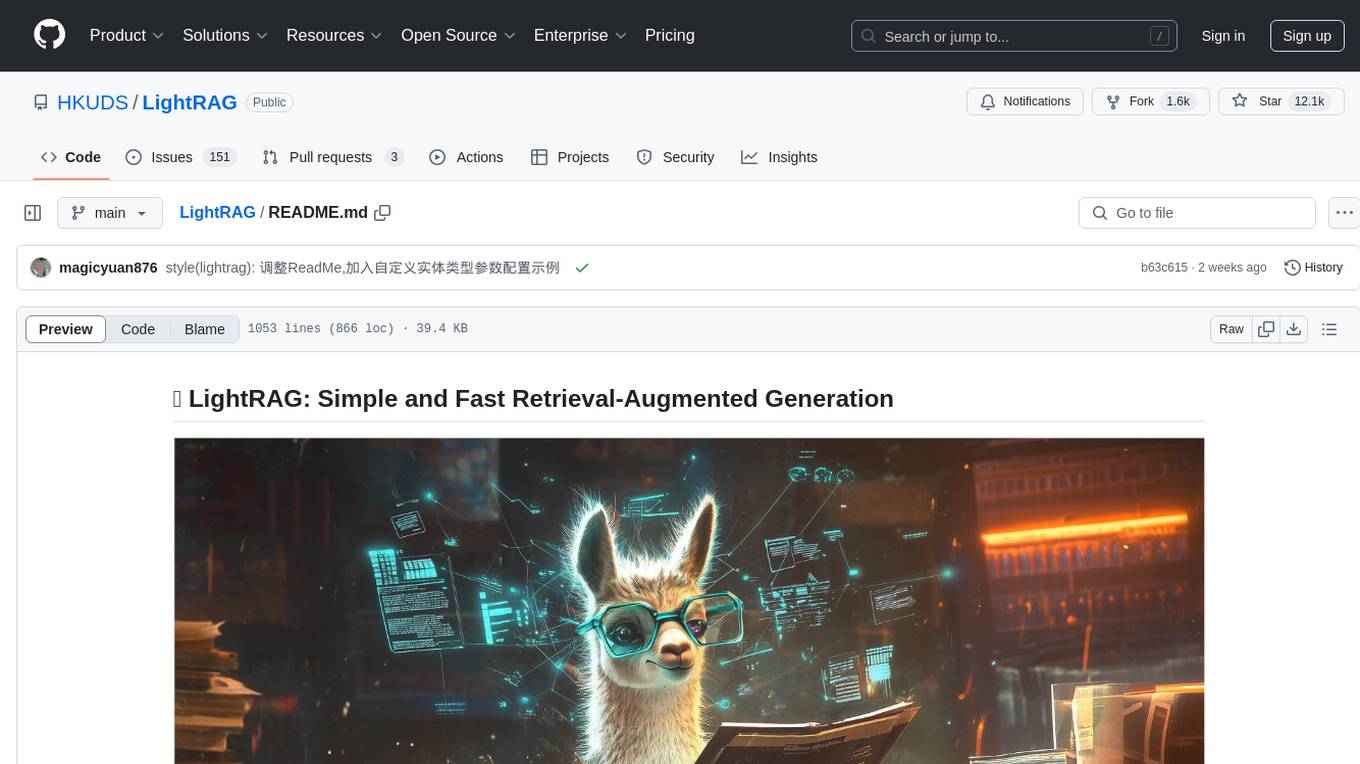
LightRAG is a repository hosting the code for LightRAG, a system that supports seamless integration of custom knowledge graphs, Oracle Database 23ai, Neo4J for storage, and multiple file types. It includes features like entity deletion, batch insert, incremental insert, and graph visualization. LightRAG provides an API server implementation for RESTful API access to RAG operations, allowing users to interact with it through HTTP requests. The repository also includes evaluation scripts, code for reproducing results, and a comprehensive code structure.
README:
- [X] [2025.03.18]🎯📢LightRAG now supports citation functionality.
- [X] [2025.02.05]🎯📢Our team has released VideoRAG understanding extremely long-context videos.
- [X] [2025.01.13]🎯📢Our team has released MiniRAG making RAG simpler with small models.
- [X] [2025.01.06]🎯📢You can now use PostgreSQL for Storage.
- [X] [2024.12.31]🎯📢LightRAG now supports deletion by document ID.
- [X] [2024.11.25]🎯📢LightRAG now supports seamless integration of custom knowledge graphs, empowering users to enhance the system with their own domain expertise.
- [X] [2024.11.19]🎯📢A comprehensive guide to LightRAG is now available on LearnOpenCV. Many thanks to the blog author.
- [X] [2024.11.12]🎯📢LightRAG now supports Oracle Database 23ai for all storage types (KV, vector, and graph).
- [X] [2024.11.11]🎯📢LightRAG now supports deleting entities by their names.
- [X] [2024.11.09]🎯📢Introducing the LightRAG Gui, which allows you to insert, query, visualize, and download LightRAG knowledge.
- [X] [2024.11.04]🎯📢You can now use Neo4J for Storage.
- [X] [2024.10.29]🎯📢LightRAG now supports multiple file types, including PDF, DOC, PPT, and CSV via
textract
. - [X] [2024.10.20]🎯📢We've added a new feature to LightRAG: Graph Visualization.
- [X] [2024.10.18]🎯📢We've added a link to a LightRAG Introduction Video. Thanks to the author!
- [X] [2024.10.17]🎯📢We have created a Discord channel! Welcome to join for sharing and discussions! 🎉🎉
- [X] [2024.10.16]🎯📢LightRAG now supports Ollama models!
- [X] [2024.10.15]🎯📢LightRAG now supports Hugging Face models!
Algorithm Flowchart
Figure 1: LightRAG Indexing Flowchart - Img Caption : Source
Figure 2: LightRAG Retrieval and Querying Flowchart - Img Caption : Source
- Install from source (Recommend)
cd LightRAG
pip install -e .
- Install from PyPI
pip install lightrag-hku
The LightRAG Server is designed to provide Web UI and API support. The Web UI facilitates document indexing, knowledge graph exploration, and a simple RAG query interface. LightRAG Server also provide an Ollama compatible interfaces, aiming to emulate LightRAG as an Ollama chat model. This allows AI chat bot, such as Open WebUI, to access LightRAG easily.
- Install from PyPI
pip install "lightrag-hku[api]"
- Installation from Source
# create a Python virtual enviroment if neccesary
# Install in editable mode with API support
pip install -e ".[api]"
For more information about LightRAG Server, please refer to LightRAG Server.
- Video demo of running LightRAG locally.
- All the code can be found in the
examples
. - Set OpenAI API key in environment if using OpenAI models:
export OPENAI_API_KEY="sk-...".
- Download the demo text "A Christmas Carol by Charles Dickens":
curl https://raw.githubusercontent.com/gusye1234/nano-graphrag/main/tests/mock_data.txt > ./book.txt
Use the below Python snippet (in a script) to initialize LightRAG and perform queries:
import os
import asyncio
from lightrag import LightRAG, QueryParam
from lightrag.llm.openai import gpt_4o_mini_complete, gpt_4o_complete, openai_embed
from lightrag.kg.shared_storage import initialize_pipeline_status
from lightrag.utils import setup_logger
setup_logger("lightrag", level="INFO")
async def initialize_rag():
rag = LightRAG(
working_dir="your/path",
embedding_func=openai_embed,
llm_model_func=gpt_4o_mini_complete
)
await rag.initialize_storages()
await initialize_pipeline_status()
return rag
def main():
# Initialize RAG instance
rag = asyncio.run(initialize_rag())
# Insert text
rag.insert("Your text")
# Perform naive search
mode="naive"
# Perform local search
mode="local"
# Perform global search
mode="global"
# Perform hybrid search
mode="hybrid"
# Mix mode Integrates knowledge graph and vector retrieval.
mode="mix"
rag.query(
"What are the top themes in this story?",
param=QueryParam(mode=mode)
)
if __name__ == "__main__":
main()
class QueryParam:
mode: Literal["local", "global", "hybrid", "naive", "mix"] = "global"
"""Specifies the retrieval mode:
- "local": Focuses on context-dependent information.
- "global": Utilizes global knowledge.
- "hybrid": Combines local and global retrieval methods.
- "naive": Performs a basic search without advanced techniques.
- "mix": Integrates knowledge graph and vector retrieval. Mix mode combines knowledge graph and vector search:
- Uses both structured (KG) and unstructured (vector) information
- Provides comprehensive answers by analyzing relationships and context
- Supports image content through HTML img tags
- Allows control over retrieval depth via top_k parameter
"""
only_need_context: bool = False
"""If True, only returns the retrieved context without generating a response."""
response_type: str = "Multiple Paragraphs"
"""Defines the response format. Examples: 'Multiple Paragraphs', 'Single Paragraph', 'Bullet Points'."""
top_k: int = 60
"""Number of top items to retrieve. Represents entities in 'local' mode and relationships in 'global' mode."""
max_token_for_text_unit: int = 4000
"""Maximum number of tokens allowed for each retrieved text chunk."""
max_token_for_global_context: int = 4000
"""Maximum number of tokens allocated for relationship descriptions in global retrieval."""
max_token_for_local_context: int = 4000
"""Maximum number of tokens allocated for entity descriptions in local retrieval."""
ids: list[str] | None = None # ONLY SUPPORTED FOR PG VECTOR DBs
"""List of ids to filter the RAG."""
model_func: Callable[..., object] | None = None
"""Optional override for the LLM model function to use for this specific query.
If provided, this will be used instead of the global model function.
This allows using different models for different query modes.
"""
...
default value of Top_k can be change by environment variables TOP_K.
LightRAG requires the utilization of LLM and Embedding models to accomplish document indexing and querying tasks. During the initialization phase, it is necessary to inject the invocation methods of the relevant models into LightRAG:
Using Open AI-like APIs
- LightRAG also supports Open AI-like chat/embeddings APIs:
async def llm_model_func(
prompt, system_prompt=None, history_messages=[], keyword_extraction=False, **kwargs
) -> str:
return await openai_complete_if_cache(
"solar-mini",
prompt,
system_prompt=system_prompt,
history_messages=history_messages,
api_key=os.getenv("UPSTAGE_API_KEY"),
base_url="https://api.upstage.ai/v1/solar",
**kwargs
)
async def embedding_func(texts: list[str]) -> np.ndarray:
return await openai_embed(
texts,
model="solar-embedding-1-large-query",
api_key=os.getenv("UPSTAGE_API_KEY"),
base_url="https://api.upstage.ai/v1/solar"
)
async def initialize_rag():
rag = LightRAG(
working_dir=WORKING_DIR,
llm_model_func=llm_model_func,
embedding_func=EmbeddingFunc(
embedding_dim=4096,
max_token_size=8192,
func=embedding_func
)
)
await rag.initialize_storages()
await initialize_pipeline_status()
return rag
Using Hugging Face Models
- If you want to use Hugging Face models, you only need to set LightRAG as follows:
See lightrag_hf_demo.py
# Initialize LightRAG with Hugging Face model
rag = LightRAG(
working_dir=WORKING_DIR,
llm_model_func=hf_model_complete, # Use Hugging Face model for text generation
llm_model_name='meta-llama/Llama-3.1-8B-Instruct', # Model name from Hugging Face
# Use Hugging Face embedding function
embedding_func=EmbeddingFunc(
embedding_dim=384,
max_token_size=5000,
func=lambda texts: hf_embed(
texts,
tokenizer=AutoTokenizer.from_pretrained("sentence-transformers/all-MiniLM-L6-v2"),
embed_model=AutoModel.from_pretrained("sentence-transformers/all-MiniLM-L6-v2")
)
),
)
Using Ollama Models
**Overview**If you want to use Ollama models, you need to pull model you plan to use and embedding model, for example nomic-embed-text
.
Then you only need to set LightRAG as follows:
# Initialize LightRAG with Ollama model
rag = LightRAG(
working_dir=WORKING_DIR,
llm_model_func=ollama_model_complete, # Use Ollama model for text generation
llm_model_name='your_model_name', # Your model name
# Use Ollama embedding function
embedding_func=EmbeddingFunc(
embedding_dim=768,
max_token_size=8192,
func=lambda texts: ollama_embed(
texts,
embed_model="nomic-embed-text"
)
),
)
- Increasing context size
In order for LightRAG to work context should be at least 32k tokens. By default Ollama models have context size of 8k. You can achieve this using one of two ways:
- Increasing the
num_ctx
parameter in Modelfile
- Pull the model:
ollama pull qwen2
- Display the model file:
ollama show --modelfile qwen2 > Modelfile
- Edit the Modelfile by adding the following line:
PARAMETER num_ctx 32768
- Create the modified model:
ollama create -f Modelfile qwen2m
- Setup
num_ctx
via Ollama API
Tiy can use llm_model_kwargs
param to configure ollama:
rag = LightRAG(
working_dir=WORKING_DIR,
llm_model_func=ollama_model_complete, # Use Ollama model for text generation
llm_model_name='your_model_name', # Your model name
llm_model_kwargs={"options": {"num_ctx": 32768}},
# Use Ollama embedding function
embedding_func=EmbeddingFunc(
embedding_dim=768,
max_token_size=8192,
func=lambda texts: ollama_embedding(
texts,
embed_model="nomic-embed-text"
)
),
)
- Low RAM GPUs
In order to run this experiment on low RAM GPU you should select small model and tune context window (increasing context increase memory consumption). For example, running this ollama example on repurposed mining GPU with 6Gb of RAM required to set context size to 26k while using gemma2:2b
. It was able to find 197 entities and 19 relations on book.txt
.
LlamaIndex
LightRAG supports integration with LlamaIndex (llm/llama_index_impl.py
):
- Integrates with OpenAI and other providers through LlamaIndex
- See LlamaIndex Documentation for detailed setup and examples
Example Usage
# Using LlamaIndex with direct OpenAI access
import asyncio
from lightrag import LightRAG
from lightrag.llm.llama_index_impl import llama_index_complete_if_cache, llama_index_embed
from llama_index.embeddings.openai import OpenAIEmbedding
from llama_index.llms.openai import OpenAI
from lightrag.kg.shared_storage import initialize_pipeline_status
from lightrag.utils import setup_logger
# Setup log handler for LightRAG
setup_logger("lightrag", level="INFO")
async def initialize_rag():
rag = LightRAG(
working_dir="your/path",
llm_model_func=llama_index_complete_if_cache, # LlamaIndex-compatible completion function
embedding_func=EmbeddingFunc( # LlamaIndex-compatible embedding function
embedding_dim=1536,
max_token_size=8192,
func=lambda texts: llama_index_embed(texts, embed_model=embed_model)
),
)
await rag.initialize_storages()
await initialize_pipeline_status()
return rag
def main():
# Initialize RAG instance
rag = asyncio.run(initialize_rag())
with open("./book.txt", "r", encoding="utf-8") as f:
rag.insert(f.read())
# Perform naive search
print(
rag.query("What are the top themes in this story?", param=QueryParam(mode="naive"))
)
# Perform local search
print(
rag.query("What are the top themes in this story?", param=QueryParam(mode="local"))
)
# Perform global search
print(
rag.query("What are the top themes in this story?", param=QueryParam(mode="global"))
)
# Perform hybrid search
print(
rag.query("What are the top themes in this story?", param=QueryParam(mode="hybrid"))
)
if __name__ == "__main__":
main()
For detailed documentation and examples, see:
LightRAG now supports multi-turn dialogue through the conversation history feature. Here's how to use it:
Usage Example
# Create conversation history
conversation_history = [
{"role": "user", "content": "What is the main character's attitude towards Christmas?"},
{"role": "assistant", "content": "At the beginning of the story, Ebenezer Scrooge has a very negative attitude towards Christmas..."},
{"role": "user", "content": "How does his attitude change?"}
]
# Create query parameters with conversation history
query_param = QueryParam(
mode="mix", # or any other mode: "local", "global", "hybrid"
conversation_history=conversation_history, # Add the conversation history
history_turns=3 # Number of recent conversation turns to consider
)
# Make a query that takes into account the conversation history
response = rag.query(
"What causes this change in his character?",
param=query_param
)
LightRAG now supports custom prompts for fine-tuned control over the system's behavior. Here's how to use it:
Usage Example
# Create query parameters
query_param = QueryParam(
mode="hybrid", # or other mode: "local", "global", "hybrid", "mix" and "naive"
)
# Example 1: Using the default system prompt
response_default = rag.query(
"What are the primary benefits of renewable energy?",
param=query_param
)
print(response_default)
# Example 2: Using a custom prompt
custom_prompt = """
You are an expert assistant in environmental science. Provide detailed and structured answers with examples.
---Conversation History---
{history}
---Knowledge Base---
{context_data}
---Response Rules---
- Target format and length: {response_type}
"""
response_custom = rag.query(
"What are the primary benefits of renewable energy?",
param=query_param,
system_prompt=custom_prompt # Pass the custom prompt
)
print(response_custom)
We've introduced a new function query_with_separate_keyword_extraction
to enhance the keyword extraction capabilities. This function separates the keyword extraction process from the user's prompt, focusing solely on the query to improve the relevance of extracted keywords.
How It Works?
The function operates by dividing the input into two parts:
User Query
Prompt
It then performs keyword extraction exclusively on the user query
. This separation ensures that the extraction process is focused and relevant, unaffected by any additional language in the prompt
. It also allows the prompt
to serve purely for response formatting, maintaining the intent and clarity of the user's original question.
Usage Example
This example
shows how to tailor the function for educational content, focusing on detailed explanations for older students.
rag.query_with_separate_keyword_extraction(
query="Explain the law of gravity",
prompt="Provide a detailed explanation suitable for high school students studying physics.",
param=QueryParam(mode="hybrid")
)
Basic Insert
# Basic Insert
rag.insert("Text")
Batch Insert
# Basic Batch Insert: Insert multiple texts at once
rag.insert(["TEXT1", "TEXT2",...])
# Batch Insert with custom batch size configuration
rag = LightRAG(
working_dir=WORKING_DIR,
addon_params={
"insert_batch_size": 4 # Process 4 documents per batch
}
)
rag.insert(["TEXT1", "TEXT2", "TEXT3", ...]) # Documents will be processed in batches of 4
The insert_batch_size
parameter in addon_params
controls how many documents are processed in each batch during insertion. This is useful for:
- Managing memory usage with large document collections
- Optimizing processing speed
- Providing better progress tracking
- Default value is 10 if not specified
Insert with ID
If you want to provide your own IDs for your documents, number of documents and number of IDs must be the same.
# Insert single text, and provide ID for it
rag.insert("TEXT1", ids=["ID_FOR_TEXT1"])
# Insert multiple texts, and provide IDs for them
rag.insert(["TEXT1", "TEXT2",...], ids=["ID_FOR_TEXT1", "ID_FOR_TEXT2"])
Insert using Pipeline
The apipeline_enqueue_documents
and apipeline_process_enqueue_documents
functions allow you to perform incremental insertion of documents into the graph.
This is useful for scenarios where you want to process documents in the background while still allowing the main thread to continue executing.
And using a routine to process news documents.
rag = LightRAG(..)
await rag.apipeline_enqueue_documents(input)
# Your routine in loop
await rag.apipeline_process_enqueue_documents(input)
Insert Multi-file Type Support
The textract
supports reading file types such as TXT, DOCX, PPTX, CSV, and PDF.
import textract
file_path = 'TEXT.pdf'
text_content = textract.process(file_path)
rag.insert(text_content.decode('utf-8'))
Insert Custom KG
custom_kg = {
"chunks": [
{
"content": "Alice and Bob are collaborating on quantum computing research.",
"source_id": "doc-1"
}
],
"entities": [
{
"entity_name": "Alice",
"entity_type": "person",
"description": "Alice is a researcher specializing in quantum physics.",
"source_id": "doc-1"
},
{
"entity_name": "Bob",
"entity_type": "person",
"description": "Bob is a mathematician.",
"source_id": "doc-1"
},
{
"entity_name": "Quantum Computing",
"entity_type": "technology",
"description": "Quantum computing utilizes quantum mechanical phenomena for computation.",
"source_id": "doc-1"
}
],
"relationships": [
{
"src_id": "Alice",
"tgt_id": "Bob",
"description": "Alice and Bob are research partners.",
"keywords": "collaboration research",
"weight": 1.0,
"source_id": "doc-1"
},
{
"src_id": "Alice",
"tgt_id": "Quantum Computing",
"description": "Alice conducts research on quantum computing.",
"keywords": "research expertise",
"weight": 1.0,
"source_id": "doc-1"
},
{
"src_id": "Bob",
"tgt_id": "Quantum Computing",
"description": "Bob researches quantum computing.",
"keywords": "research application",
"weight": 1.0,
"source_id": "doc-1"
}
]
}
rag.insert_custom_kg(custom_kg)
Citation Functionality
By providing file paths, the system ensures that sources can be traced back to their original documents.
# Define documents and their file paths
documents = ["Document content 1", "Document content 2"]
file_paths = ["path/to/doc1.txt", "path/to/doc2.txt"]
# Insert documents with file paths
rag.insert(documents, file_paths=file_paths)
Using Neo4J for Storage
- For production level scenarios you will most likely want to leverage an enterprise solution
- for KG storage. Running Neo4J in Docker is recommended for seamless local testing.
- See: https://hub.docker.com/_/neo4j
export NEO4J_URI="neo4j://localhost:7687"
export NEO4J_USERNAME="neo4j"
export NEO4J_PASSWORD="password"
# Setup logger for LightRAG
setup_logger("lightrag", level="INFO")
# When you launch the project be sure to override the default KG: NetworkX
# by specifying kg="Neo4JStorage".
# Note: Default settings use NetworkX
# Initialize LightRAG with Neo4J implementation.
async def initialize_rag():
rag = LightRAG(
working_dir=WORKING_DIR,
llm_model_func=gpt_4o_mini_complete, # Use gpt_4o_mini_complete LLM model
graph_storage="Neo4JStorage", #<-----------override KG default
)
# Initialize database connections
await rag.initialize_storages()
# Initialize pipeline status for document processing
await initialize_pipeline_status()
return rag
see test_neo4j.py for a working example.
Using PostgreSQL for Storage
For production level scenarios you will most likely want to leverage an enterprise solution. PostgreSQL can provide a one-stop solution for you as KV store, VectorDB (pgvector) and GraphDB (apache AGE).
- PostgreSQL is lightweight,the whole binary distribution including all necessary plugins can be zipped to 40MB: Ref to Windows Release as it is easy to install for Linux/Mac.
- If you prefer docker, please start with this image if you are a beginner to avoid hiccups (DO read the overview): https://hub.docker.com/r/shangor/postgres-for-rag
- How to start? Ref to: examples/lightrag_zhipu_postgres_demo.py
- Create index for AGE example: (Change below
dickens
to your graph name if necessary)load 'age'; SET search_path = ag_catalog, "$user", public; CREATE INDEX CONCURRENTLY entity_p_idx ON dickens."Entity" (id); CREATE INDEX CONCURRENTLY vertex_p_idx ON dickens."_ag_label_vertex" (id); CREATE INDEX CONCURRENTLY directed_p_idx ON dickens."DIRECTED" (id); CREATE INDEX CONCURRENTLY directed_eid_idx ON dickens."DIRECTED" (end_id); CREATE INDEX CONCURRENTLY directed_sid_idx ON dickens."DIRECTED" (start_id); CREATE INDEX CONCURRENTLY directed_seid_idx ON dickens."DIRECTED" (start_id,end_id); CREATE INDEX CONCURRENTLY edge_p_idx ON dickens."_ag_label_edge" (id); CREATE INDEX CONCURRENTLY edge_sid_idx ON dickens."_ag_label_edge" (start_id); CREATE INDEX CONCURRENTLY edge_eid_idx ON dickens."_ag_label_edge" (end_id); CREATE INDEX CONCURRENTLY edge_seid_idx ON dickens."_ag_label_edge" (start_id,end_id); create INDEX CONCURRENTLY vertex_idx_node_id ON dickens."_ag_label_vertex" (ag_catalog.agtype_access_operator(properties, '"node_id"'::agtype)); create INDEX CONCURRENTLY entity_idx_node_id ON dickens."Entity" (ag_catalog.agtype_access_operator(properties, '"node_id"'::agtype)); CREATE INDEX CONCURRENTLY entity_node_id_gin_idx ON dickens."Entity" using gin(properties); ALTER TABLE dickens."DIRECTED" CLUSTER ON directed_sid_idx; -- drop if necessary drop INDEX entity_p_idx; drop INDEX vertex_p_idx; drop INDEX directed_p_idx; drop INDEX directed_eid_idx; drop INDEX directed_sid_idx; drop INDEX directed_seid_idx; drop INDEX edge_p_idx; drop INDEX edge_sid_idx; drop INDEX edge_eid_idx; drop INDEX edge_seid_idx; drop INDEX vertex_idx_node_id; drop INDEX entity_idx_node_id; drop INDEX entity_node_id_gin_idx;
- Known issue of the Apache AGE: The released versions got below issue:
You might find that the properties of the nodes/edges are empty. It is a known issue of the release version: https://github.com/apache/age/pull/1721
You can Compile the AGE from source code and fix it.
Using Faiss for Storage
- Install the required dependencies:
pip install faiss-cpu
You can also install faiss-gpu
if you have GPU support.
- Here we are using
sentence-transformers
but you can also useOpenAIEmbedding
model with3072
dimensions.
async def embedding_func(texts: list[str]) -> np.ndarray:
model = SentenceTransformer('all-MiniLM-L6-v2')
embeddings = model.encode(texts, convert_to_numpy=True)
return embeddings
# Initialize LightRAG with the LLM model function and embedding function
rag = LightRAG(
working_dir=WORKING_DIR,
llm_model_func=llm_model_func,
embedding_func=EmbeddingFunc(
embedding_dim=384,
max_token_size=8192,
func=embedding_func,
),
vector_storage="FaissVectorDBStorage",
vector_db_storage_cls_kwargs={
"cosine_better_than_threshold": 0.3 # Your desired threshold
}
)
# Delete Entity: Deleting entities by their names
rag.delete_by_entity("Project Gutenberg")
# Delete Document: Deleting entities and relationships associated with the document by doc id
rag.delete_by_doc_id("doc_id")
LightRAG now supports comprehensive knowledge graph management capabilities, allowing you to create, edit, and delete entities and relationships within your knowledge graph.
Create Entities and Relations
# Create new entity
entity = rag.create_entity("Google", {
"description": "Google is a multinational technology company specializing in internet-related services and products.",
"entity_type": "company"
})
# Create another entity
product = rag.create_entity("Gmail", {
"description": "Gmail is an email service developed by Google.",
"entity_type": "product"
})
# Create relation between entities
relation = rag.create_relation("Google", "Gmail", {
"description": "Google develops and operates Gmail.",
"keywords": "develops operates service",
"weight": 2.0
})
Edit Entities and Relations
# Edit an existing entity
updated_entity = rag.edit_entity("Google", {
"description": "Google is a subsidiary of Alphabet Inc., founded in 1998.",
"entity_type": "tech_company"
})
# Rename an entity (with all its relationships properly migrated)
renamed_entity = rag.edit_entity("Gmail", {
"entity_name": "Google Mail",
"description": "Google Mail (formerly Gmail) is an email service."
})
# Edit a relation between entities
updated_relation = rag.edit_relation("Google", "Google Mail", {
"description": "Google created and maintains Google Mail service.",
"keywords": "creates maintains email service",
"weight": 3.0
})
All operations are available in both synchronous and asynchronous versions. The asynchronous versions have the prefix "a" (e.g., acreate_entity
, aedit_relation
).
- create_entity: Creates a new entity with specified attributes
- edit_entity: Updates an existing entity's attributes or renames it
- create_relation: Creates a new relation between existing entities
- edit_relation: Updates an existing relation's attributes
These operations maintain data consistency across both the graph database and vector database components, ensuring your knowledge graph remains coherent.
LightRAG allows you to export your knowledge graph data in various formats for analysis, sharing, and backup purposes. The system supports exporting entities, relations, and relationship data.
Basic Usage
# Basic CSV export (default format)
rag.export_data("knowledge_graph.csv")
# Specify any format
rag.export_data("output.xlsx", file_format="excel")
Different File Formats supported
#Export data in CSV format
rag.export_data("graph_data.csv", file_format="csv")
# Export data in Excel sheet
rag.export_data("graph_data.xlsx", file_format="excel")
# Export data in markdown format
rag.export_data("graph_data.md", file_format="md")
# Export data in Text
rag.export_data("graph_data.txt", file_format="txt")
Additional Options
Include vector embeddings in the export (optional):
rag.export_data("complete_data.csv", include_vector_data=True)
All exports include:
- Entity information (names, IDs, metadata)
- Relation data (connections between entities)
- Relationship information from vector database
Merge Entities and Their Relationships
LightRAG now supports merging multiple entities into a single entity, automatically handling all relationships:
# Basic entity merging
rag.merge_entities(
source_entities=["Artificial Intelligence", "AI", "Machine Intelligence"],
target_entity="AI Technology"
)
With custom merge strategy:
# Define custom merge strategy for different fields
rag.merge_entities(
source_entities=["John Smith", "Dr. Smith", "J. Smith"],
target_entity="John Smith",
merge_strategy={
"description": "concatenate", # Combine all descriptions
"entity_type": "keep_first", # Keep the entity type from the first entity
"source_id": "join_unique" # Combine all unique source IDs
}
)
With custom target entity data:
# Specify exact values for the merged entity
rag.merge_entities(
source_entities=["New York", "NYC", "Big Apple"],
target_entity="New York City",
target_entity_data={
"entity_type": "LOCATION",
"description": "New York City is the most populous city in the United States.",
}
)
Advanced usage combining both approaches:
# Merge company entities with both strategy and custom data
rag.merge_entities(
source_entities=["Microsoft Corp", "Microsoft Corporation", "MSFT"],
target_entity="Microsoft",
merge_strategy={
"description": "concatenate", # Combine all descriptions
"source_id": "join_unique" # Combine source IDs
},
target_entity_data={
"entity_type": "ORGANIZATION",
}
)
When merging entities:
- All relationships from source entities are redirected to the target entity
- Duplicate relationships are intelligently merged
- Self-relationships (loops) are prevented
- Source entities are removed after merging
- Relationship weights and attributes are preserved
Clear Cache
You can clear the LLM response cache with different modes:
# Clear all cache
await rag.aclear_cache()
# Clear local mode cache
await rag.aclear_cache(modes=["local"])
# Clear extraction cache
await rag.aclear_cache(modes=["default"])
# Clear multiple modes
await rag.aclear_cache(modes=["local", "global", "hybrid"])
# Synchronous version
rag.clear_cache(modes=["local"])
Valid modes are:
-
"default"
: Extraction cache -
"naive"
: Naive search cache -
"local"
: Local search cache -
"global"
: Global search cache -
"hybrid"
: Hybrid search cache -
"mix"
: Mix search cache
Parameters
Parameter | Type | Explanation | Default |
---|---|---|---|
working_dir | str |
Directory where the cache will be stored | lightrag_cache+timestamp |
kv_storage | str |
Storage type for documents and text chunks. Supported types: JsonKVStorage , OracleKVStorage
|
JsonKVStorage |
vector_storage | str |
Storage type for embedding vectors. Supported types: NanoVectorDBStorage , OracleVectorDBStorage
|
NanoVectorDBStorage |
graph_storage | str |
Storage type for graph edges and nodes. Supported types: NetworkXStorage , Neo4JStorage , OracleGraphStorage
|
NetworkXStorage |
chunk_token_size | int |
Maximum token size per chunk when splitting documents | 1200 |
chunk_overlap_token_size | int |
Overlap token size between two chunks when splitting documents | 100 |
tiktoken_model_name | str |
Model name for the Tiktoken encoder used to calculate token numbers | gpt-4o-mini |
entity_extract_max_gleaning | int |
Number of loops in the entity extraction process, appending history messages | 1 |
entity_summary_to_max_tokens | int |
Maximum token size for each entity summary | 500 |
node_embedding_algorithm | str |
Algorithm for node embedding (currently not used) | node2vec |
node2vec_params | dict |
Parameters for node embedding | {"dimensions": 1536,"num_walks": 10,"walk_length": 40,"window_size": 2,"iterations": 3,"random_seed": 3,} |
embedding_func | EmbeddingFunc |
Function to generate embedding vectors from text | openai_embed |
embedding_batch_num | int |
Maximum batch size for embedding processes (multiple texts sent per batch) | 32 |
embedding_func_max_async | int |
Maximum number of concurrent asynchronous embedding processes | 16 |
llm_model_func | callable |
Function for LLM generation | gpt_4o_mini_complete |
llm_model_name | str |
LLM model name for generation | meta-llama/Llama-3.2-1B-Instruct |
llm_model_max_token_size | int |
Maximum token size for LLM generation (affects entity relation summaries) |
32768 (default value changed by env var MAX_TOKENS) |
llm_model_max_async | int |
Maximum number of concurrent asynchronous LLM processes |
4 (default value changed by env var MAX_ASYNC) |
llm_model_kwargs | dict |
Additional parameters for LLM generation | |
vector_db_storage_cls_kwargs | dict |
Additional parameters for vector database, like setting the threshold for nodes and relations retrieval | cosine_better_than_threshold: 0.2(default value changed by env var COSINE_THRESHOLD) |
enable_llm_cache | bool |
If TRUE , stores LLM results in cache; repeated prompts return cached responses |
TRUE |
enable_llm_cache_for_entity_extract | bool |
If TRUE , stores LLM results in cache for entity extraction; Good for beginners to debug your application |
TRUE |
addon_params | dict |
Additional parameters, e.g., {"example_number": 1, "language": "Simplified Chinese", "entity_types": ["organization", "person", "geo", "event"], "insert_batch_size": 10} : sets example limit, output language, and batch size for document processing |
example_number: all examples, language: English, insert_batch_size: 10 |
convert_response_to_json_func | callable |
Not used | convert_response_to_json |
embedding_cache_config | dict |
Configuration for question-answer caching. Contains three parameters: enabled : Boolean value to enable/disable cache lookup functionality. When enabled, the system will check cached responses before generating new answers. similarity_threshold : Float value (0-1), similarity threshold. When a new question's similarity with a cached question exceeds this threshold, the cached answer will be returned directly without calling the LLM. use_llm_check : Boolean value to enable/disable LLM similarity verification. When enabled, LLM will be used as a secondary check to verify the similarity between questions before returning cached answers. |
Default: {"enabled": False, "similarity_threshold": 0.95, "use_llm_check": False}
|
Click to view error handling details
The API includes comprehensive error handling:
- File not found errors (404)
- Processing errors (500)
- Supports multiple file encodings (UTF-8 and GBK)
The LightRAG Server is designed to provide Web UI and API support. For more information about LightRAG Server, please refer to LightRAG Server.
The LightRAG Server offers a comprehensive knowledge graph visualization feature. It supports various gravity layouts, node queries, subgraph filtering, and more. For more information about LightRAG Server, please refer to LightRAG Server.
The dataset used in LightRAG can be downloaded from TommyChien/UltraDomain.
LightRAG uses the following prompt to generate high-level queries, with the corresponding code in example/generate_query.py
.
Prompt
Given the following description of a dataset:
{description}
Please identify 5 potential users who would engage with this dataset. For each user, list 5 tasks they would perform with this dataset. Then, for each (user, task) combination, generate 5 questions that require a high-level understanding of the entire dataset.
Output the results in the following structure:
- User 1: [user description]
- Task 1: [task description]
- Question 1:
- Question 2:
- Question 3:
- Question 4:
- Question 5:
- Task 2: [task description]
...
- Task 5: [task description]
- User 2: [user description]
...
- User 5: [user description]
...
To evaluate the performance of two RAG systems on high-level queries, LightRAG uses the following prompt, with the specific code available in example/batch_eval.py
.
Prompt
---Role---
You are an expert tasked with evaluating two answers to the same question based on three criteria: **Comprehensiveness**, **Diversity**, and **Empowerment**.
---Goal---
You will evaluate two answers to the same question based on three criteria: **Comprehensiveness**, **Diversity**, and **Empowerment**.
- **Comprehensiveness**: How much detail does the answer provide to cover all aspects and details of the question?
- **Diversity**: How varied and rich is the answer in providing different perspectives and insights on the question?
- **Empowerment**: How well does the answer help the reader understand and make informed judgments about the topic?
For each criterion, choose the better answer (either Answer 1 or Answer 2) and explain why. Then, select an overall winner based on these three categories.
Here is the question:
{query}
Here are the two answers:
**Answer 1:**
{answer1}
**Answer 2:**
{answer2}
Evaluate both answers using the three criteria listed above and provide detailed explanations for each criterion.
Output your evaluation in the following JSON format:
{{
"Comprehensiveness": {{
"Winner": "[Answer 1 or Answer 2]",
"Explanation": "[Provide explanation here]"
}},
"Empowerment": {{
"Winner": "[Answer 1 or Answer 2]",
"Explanation": "[Provide explanation here]"
}},
"Overall Winner": {{
"Winner": "[Answer 1 or Answer 2]",
"Explanation": "[Summarize why this answer is the overall winner based on the three criteria]"
}}
}}
Agriculture | CS | Legal | Mix | |||||
---|---|---|---|---|---|---|---|---|
NaiveRAG | LightRAG | NaiveRAG | LightRAG | NaiveRAG | LightRAG | NaiveRAG | LightRAG | |
Comprehensiveness | 32.4% | 67.6% | 38.4% | 61.6% | 16.4% | 83.6% | 38.8% | 61.2% |
Diversity | 23.6% | 76.4% | 38.0% | 62.0% | 13.6% | 86.4% | 32.4% | 67.6% |
Empowerment | 32.4% | 67.6% | 38.8% | 61.2% | 16.4% | 83.6% | 42.8% | 57.2% |
Overall | 32.4% | 67.6% | 38.8% | 61.2% | 15.2% | 84.8% | 40.0% | 60.0% |
RQ-RAG | LightRAG | RQ-RAG | LightRAG | RQ-RAG | LightRAG | RQ-RAG | LightRAG | |
Comprehensiveness | 31.6% | 68.4% | 38.8% | 61.2% | 15.2% | 84.8% | 39.2% | 60.8% |
Diversity | 29.2% | 70.8% | 39.2% | 60.8% | 11.6% | 88.4% | 30.8% | 69.2% |
Empowerment | 31.6% | 68.4% | 36.4% | 63.6% | 15.2% | 84.8% | 42.4% | 57.6% |
Overall | 32.4% | 67.6% | 38.0% | 62.0% | 14.4% | 85.6% | 40.0% | 60.0% |
HyDE | LightRAG | HyDE | LightRAG | HyDE | LightRAG | HyDE | LightRAG | |
Comprehensiveness | 26.0% | 74.0% | 41.6% | 58.4% | 26.8% | 73.2% | 40.4% | 59.6% |
Diversity | 24.0% | 76.0% | 38.8% | 61.2% | 20.0% | 80.0% | 32.4% | 67.6% |
Empowerment | 25.2% | 74.8% | 40.8% | 59.2% | 26.0% | 74.0% | 46.0% | 54.0% |
Overall | 24.8% | 75.2% | 41.6% | 58.4% | 26.4% | 73.6% | 42.4% | 57.6% |
GraphRAG | LightRAG | GraphRAG | LightRAG | GraphRAG | LightRAG | GraphRAG | LightRAG | |
Comprehensiveness | 45.6% | 54.4% | 48.4% | 51.6% | 48.4% | 51.6% | 50.4% | 49.6% |
Diversity | 22.8% | 77.2% | 40.8% | 59.2% | 26.4% | 73.6% | 36.0% | 64.0% |
Empowerment | 41.2% | 58.8% | 45.2% | 54.8% | 43.6% | 56.4% | 50.8% | 49.2% |
Overall | 45.2% | 54.8% | 48.0% | 52.0% | 47.2% | 52.8% | 50.4% | 49.6% |
All the code can be found in the ./reproduce
directory.
First, we need to extract unique contexts in the datasets.
Code
def extract_unique_contexts(input_directory, output_directory):
os.makedirs(output_directory, exist_ok=True)
jsonl_files = glob.glob(os.path.join(input_directory, '*.jsonl'))
print(f"Found {len(jsonl_files)} JSONL files.")
for file_path in jsonl_files:
filename = os.path.basename(file_path)
name, ext = os.path.splitext(filename)
output_filename = f"{name}_unique_contexts.json"
output_path = os.path.join(output_directory, output_filename)
unique_contexts_dict = {}
print(f"Processing file: {filename}")
try:
with open(file_path, 'r', encoding='utf-8') as infile:
for line_number, line in enumerate(infile, start=1):
line = line.strip()
if not line:
continue
try:
json_obj = json.loads(line)
context = json_obj.get('context')
if context and context not in unique_contexts_dict:
unique_contexts_dict[context] = None
except json.JSONDecodeError as e:
print(f"JSON decoding error in file {filename} at line {line_number}: {e}")
except FileNotFoundError:
print(f"File not found: {filename}")
continue
except Exception as e:
print(f"An error occurred while processing file {filename}: {e}")
continue
unique_contexts_list = list(unique_contexts_dict.keys())
print(f"There are {len(unique_contexts_list)} unique `context` entries in the file {filename}.")
try:
with open(output_path, 'w', encoding='utf-8') as outfile:
json.dump(unique_contexts_list, outfile, ensure_ascii=False, indent=4)
print(f"Unique `context` entries have been saved to: {output_filename}")
except Exception as e:
print(f"An error occurred while saving to the file {output_filename}: {e}")
print("All files have been processed.")
For the extracted contexts, we insert them into the LightRAG system.
Code
def insert_text(rag, file_path):
with open(file_path, mode='r') as f:
unique_contexts = json.load(f)
retries = 0
max_retries = 3
while retries < max_retries:
try:
rag.insert(unique_contexts)
break
except Exception as e:
retries += 1
print(f"Insertion failed, retrying ({retries}/{max_retries}), error: {e}")
time.sleep(10)
if retries == max_retries:
print("Insertion failed after exceeding the maximum number of retries")
We extract tokens from the first and the second half of each context in the dataset, then combine them as dataset descriptions to generate queries.
Code
tokenizer = GPT2Tokenizer.from_pretrained('gpt2')
def get_summary(context, tot_tokens=2000):
tokens = tokenizer.tokenize(context)
half_tokens = tot_tokens // 2
start_tokens = tokens[1000:1000 + half_tokens]
end_tokens = tokens[-(1000 + half_tokens):1000]
summary_tokens = start_tokens + end_tokens
summary = tokenizer.convert_tokens_to_string(summary_tokens)
return summary
For the queries generated in Step-2, we will extract them and query LightRAG.
Code
def extract_queries(file_path):
with open(file_path, 'r') as f:
data = f.read()
data = data.replace('**', '')
queries = re.findall(r'- Question \d+: (.+)', data)
return queries
Thank you to all our contributors!
@article{guo2024lightrag,
title={LightRAG: Simple and Fast Retrieval-Augmented Generation},
author={Zirui Guo and Lianghao Xia and Yanhua Yu and Tu Ao and Chao Huang},
year={2024},
eprint={2410.05779},
archivePrefix={arXiv},
primaryClass={cs.IR}
}
Thank you for your interest in our work!
For Tasks:
Click tags to check more tools for each tasksFor Jobs:
Alternative AI tools for LightRAG
Similar Open Source Tools
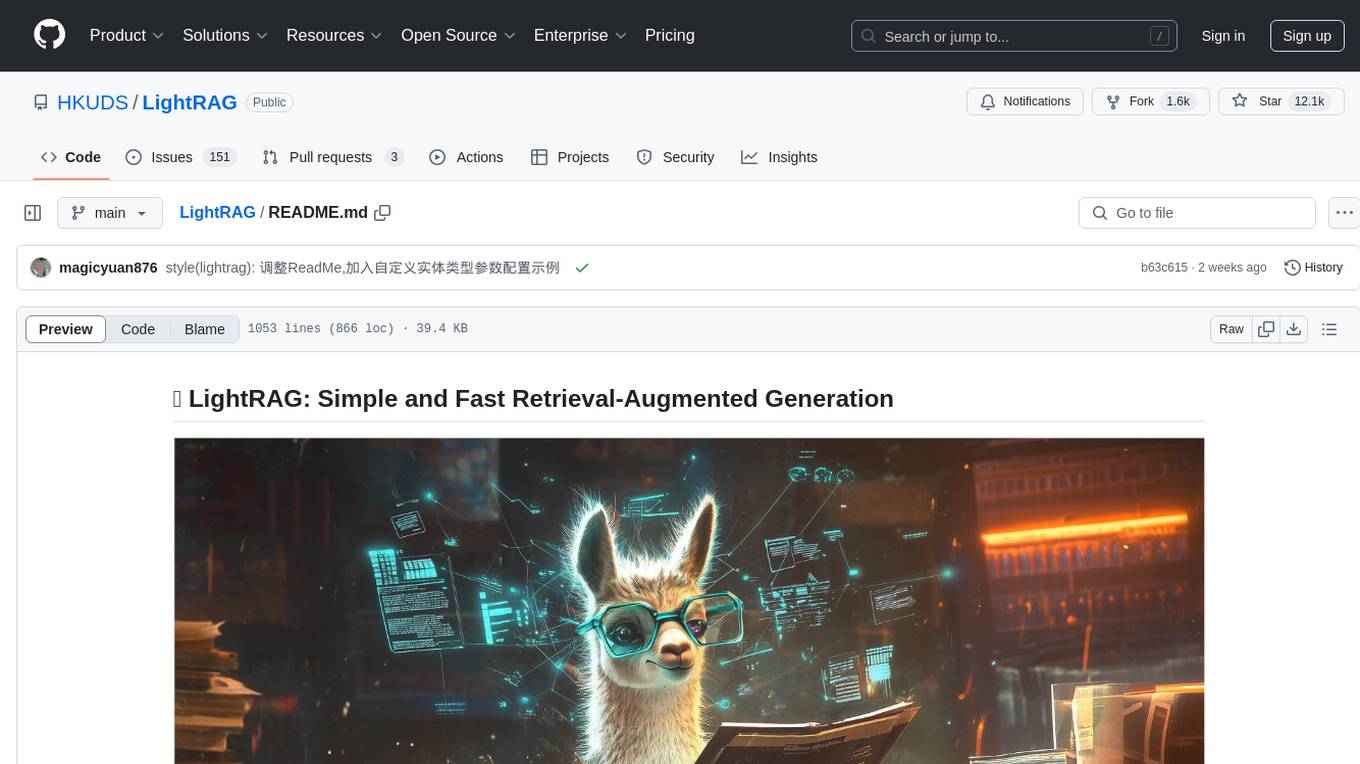
LightRAG
LightRAG is a repository hosting the code for LightRAG, a system that supports seamless integration of custom knowledge graphs, Oracle Database 23ai, Neo4J for storage, and multiple file types. It includes features like entity deletion, batch insert, incremental insert, and graph visualization. LightRAG provides an API server implementation for RESTful API access to RAG operations, allowing users to interact with it through HTTP requests. The repository also includes evaluation scripts, code for reproducing results, and a comprehensive code structure.
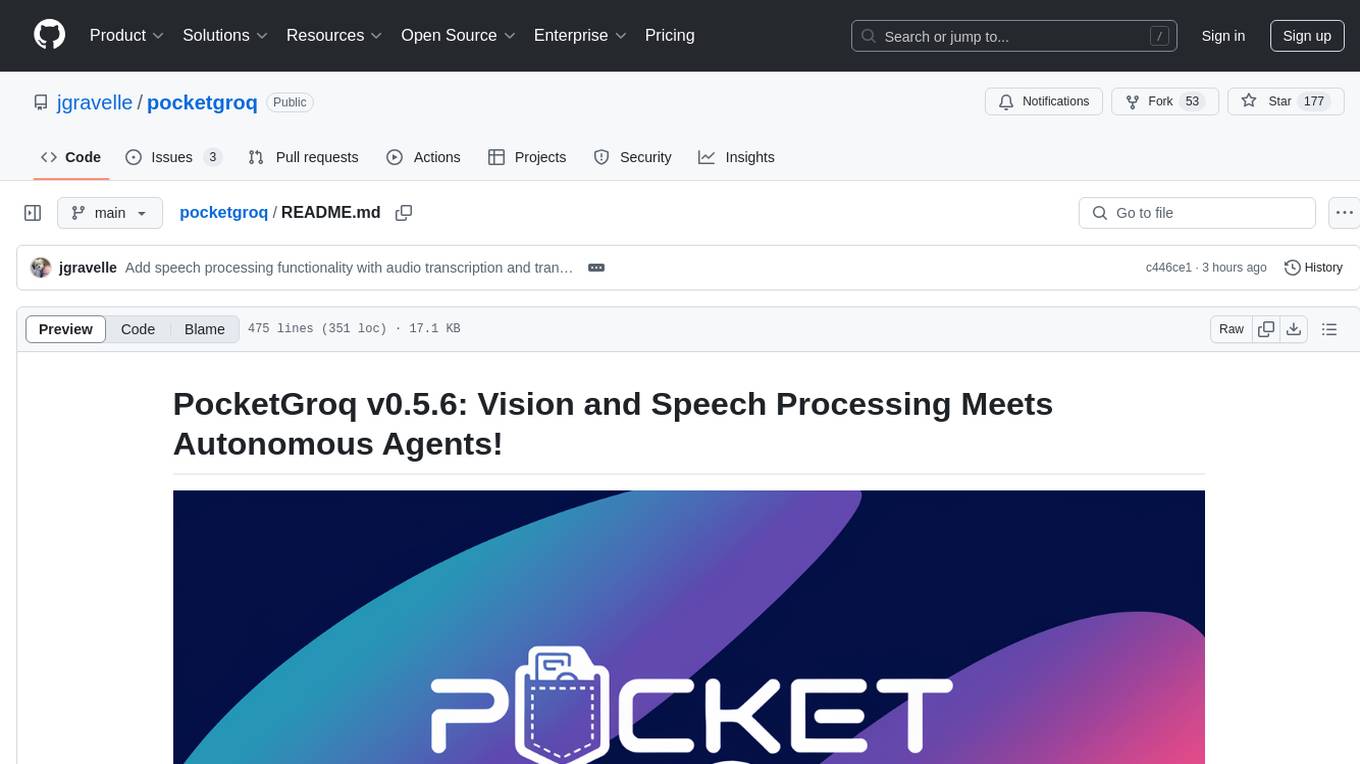
pocketgroq
PocketGroq is a tool that provides advanced functionalities for text generation, web scraping, web search, and AI response evaluation. It includes features like an Autonomous Agent for answering questions, web crawling and scraping capabilities, enhanced web search functionality, and flexible integration with Ollama server. Users can customize the agent's behavior, evaluate responses using AI, and utilize various methods for text generation, conversation management, and Chain of Thought reasoning. The tool offers comprehensive methods for different tasks, such as initializing RAG, error handling, and tool management. PocketGroq is designed to enhance development processes and enable the creation of AI-powered applications with ease.
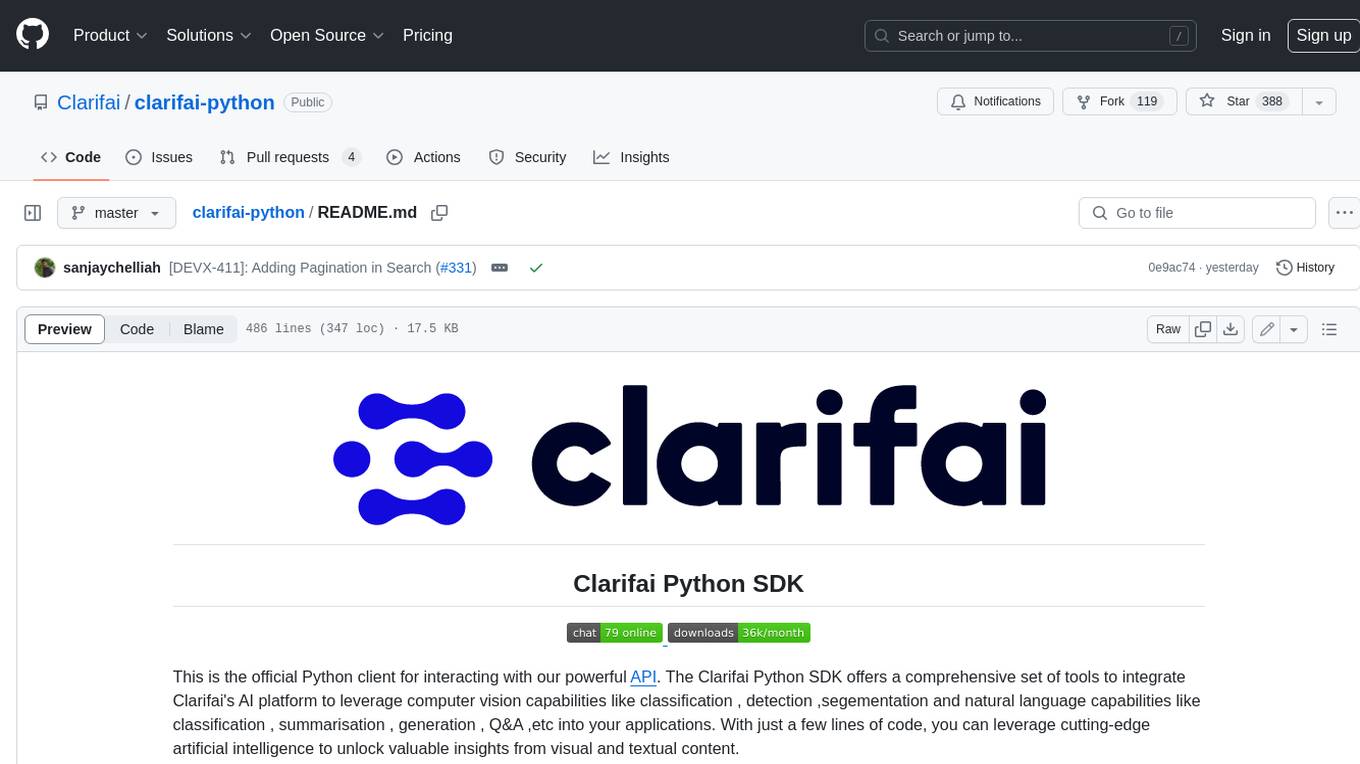
clarifai-python
The Clarifai Python SDK offers a comprehensive set of tools to integrate Clarifai's AI platform to leverage computer vision capabilities like classification , detection ,segementation and natural language capabilities like classification , summarisation , generation , Q&A ,etc into your applications. With just a few lines of code, you can leverage cutting-edge artificial intelligence to unlock valuable insights from visual and textual content.
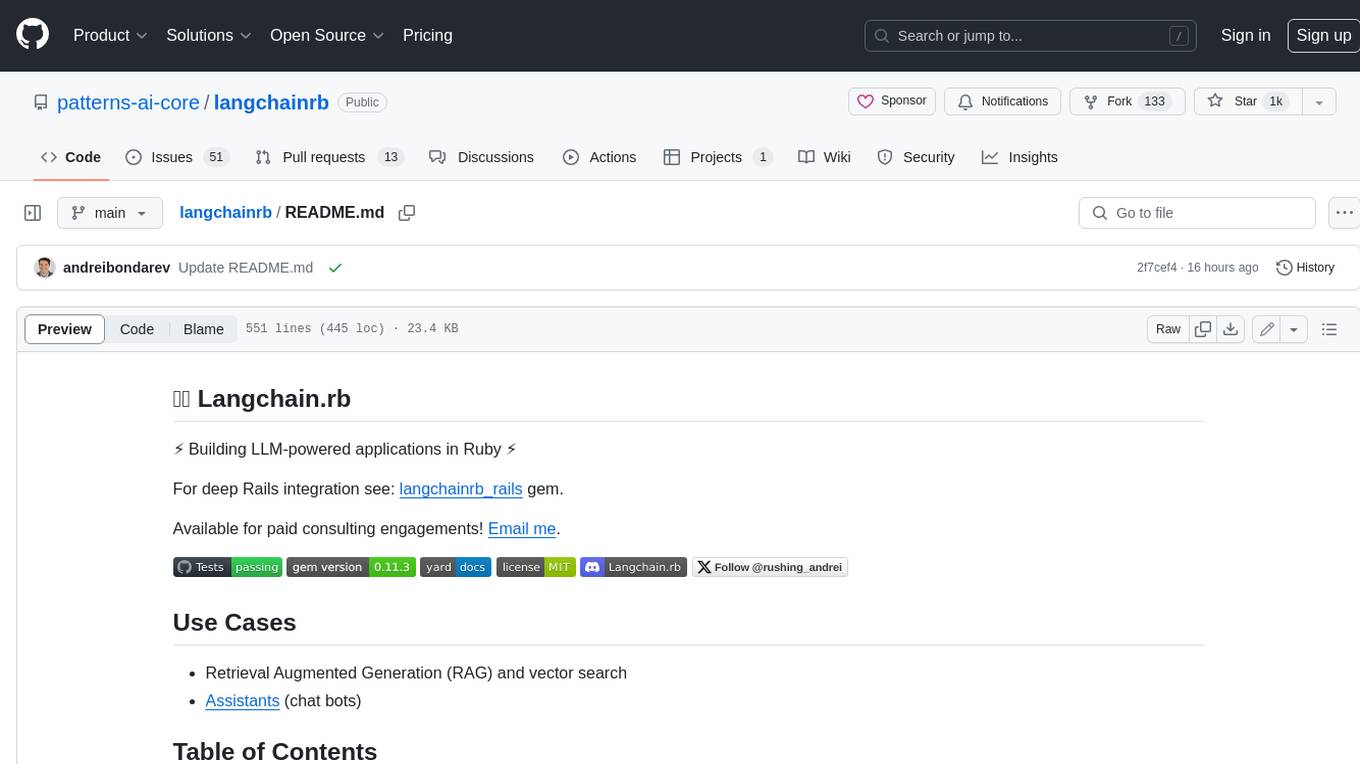
langchainrb
Langchain.rb is a Ruby library that makes it easy to build LLM-powered applications. It provides a unified interface to a variety of LLMs, vector search databases, and other tools, making it easy to build and deploy RAG (Retrieval Augmented Generation) systems and assistants. Langchain.rb is open source and available under the MIT License.
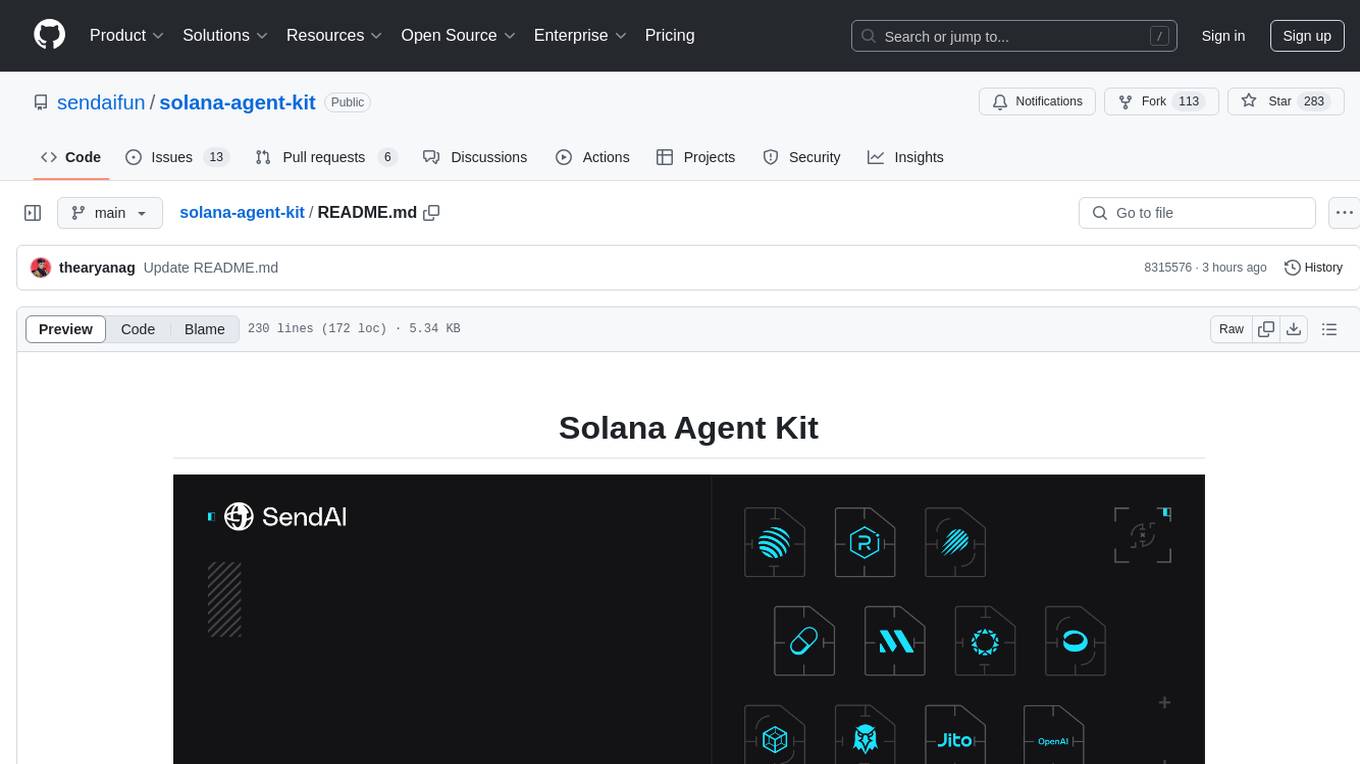
solana-agent-kit
Solana Agent Kit is an open-source toolkit designed for connecting AI agents to Solana protocols. It enables agents, regardless of the model used, to autonomously perform various Solana actions such as trading tokens, launching new tokens, lending assets, sending compressed airdrops, executing blinks, and more. The toolkit integrates core blockchain features like token operations, NFT management via Metaplex, DeFi integration, Solana blinks, AI integration features with LangChain, autonomous modes, and AI tools. It provides ready-to-use tools for blockchain operations, supports autonomous agent actions, and offers features like memory management, real-time feedback, and error handling. Solana Agent Kit facilitates tasks such as deploying tokens, creating NFT collections, swapping tokens, lending tokens, staking SOL, and sending SPL token airdrops via ZK compression. It also includes functionalities for fetching price data from Pyth and relies on key Solana and Metaplex libraries for its operations.
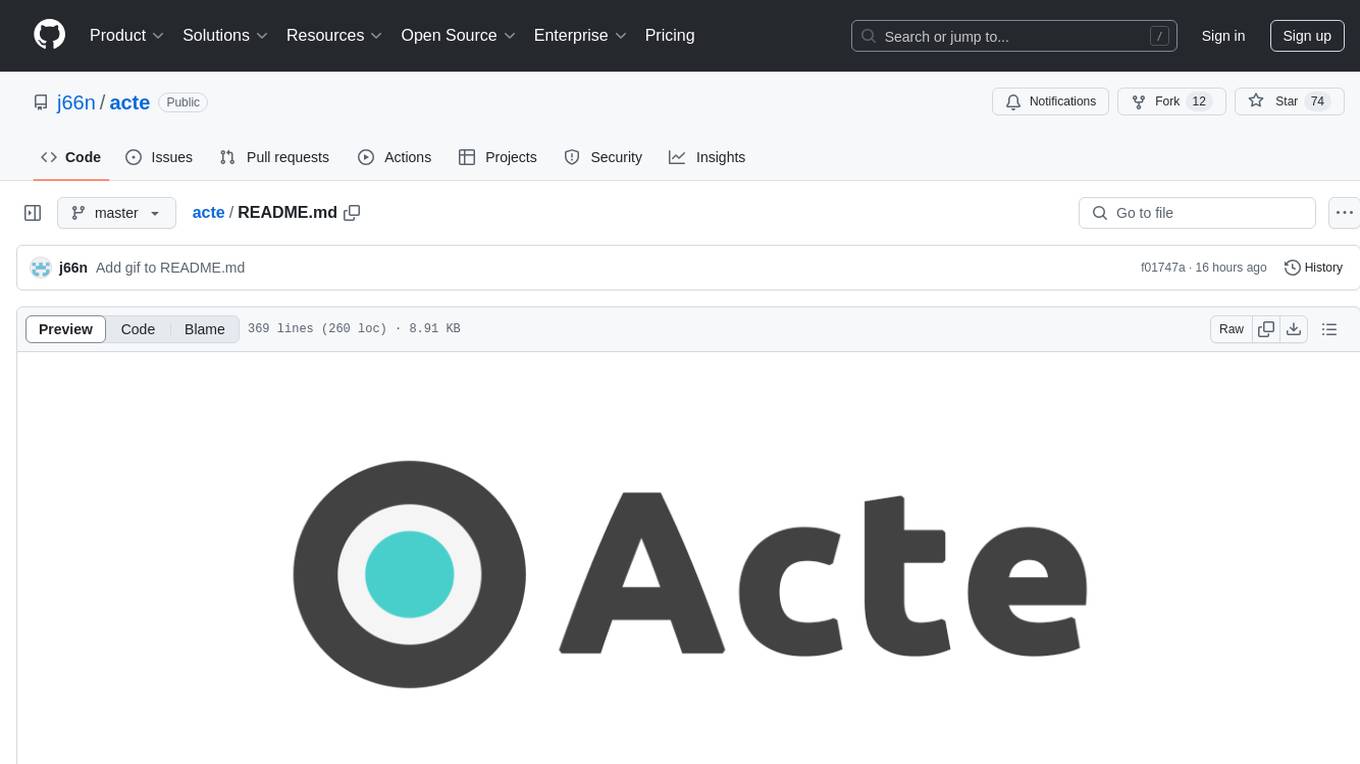
acte
Acte is a framework designed to build GUI-like tools for AI Agents. It aims to address the issues of cognitive load and freedom degrees when interacting with multiple APIs in complex scenarios. By providing a graphical user interface (GUI) for Agents, Acte helps reduce cognitive load and constraints interaction, similar to how humans interact with computers through GUIs. The tool offers APIs for starting new sessions, executing actions, and displaying screens, accessible via HTTP requests or the SessionManager class.
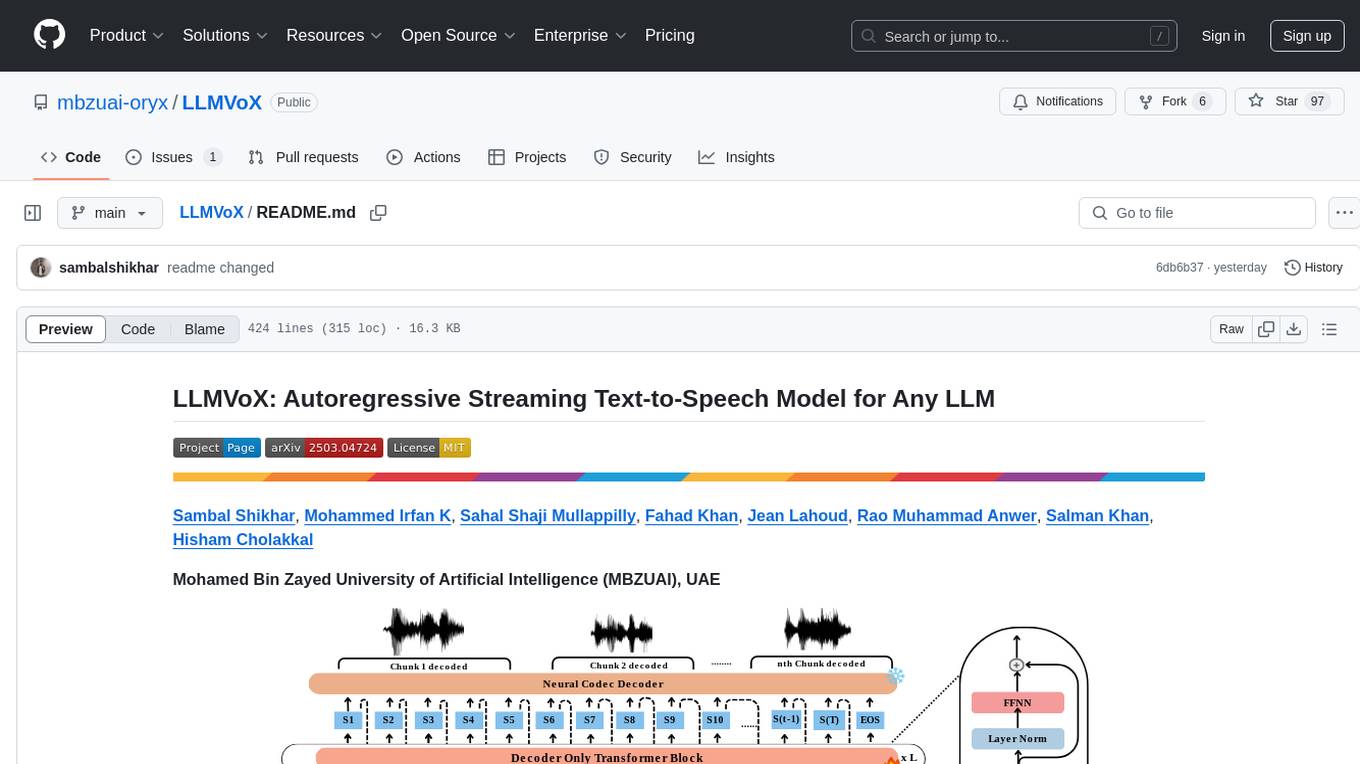
LLMVoX
LLMVoX is a lightweight 30M-parameter, LLM-agnostic, autoregressive streaming Text-to-Speech (TTS) system designed to convert text outputs from Large Language Models into high-fidelity streaming speech with low latency. It achieves significantly lower Word Error Rate compared to speech-enabled LLMs while operating at comparable latency and speech quality. Key features include being lightweight & fast with only 30M parameters, LLM-agnostic for easy integration with existing models, multi-queue streaming for continuous speech generation, and multilingual support for easy adaptation to new languages.
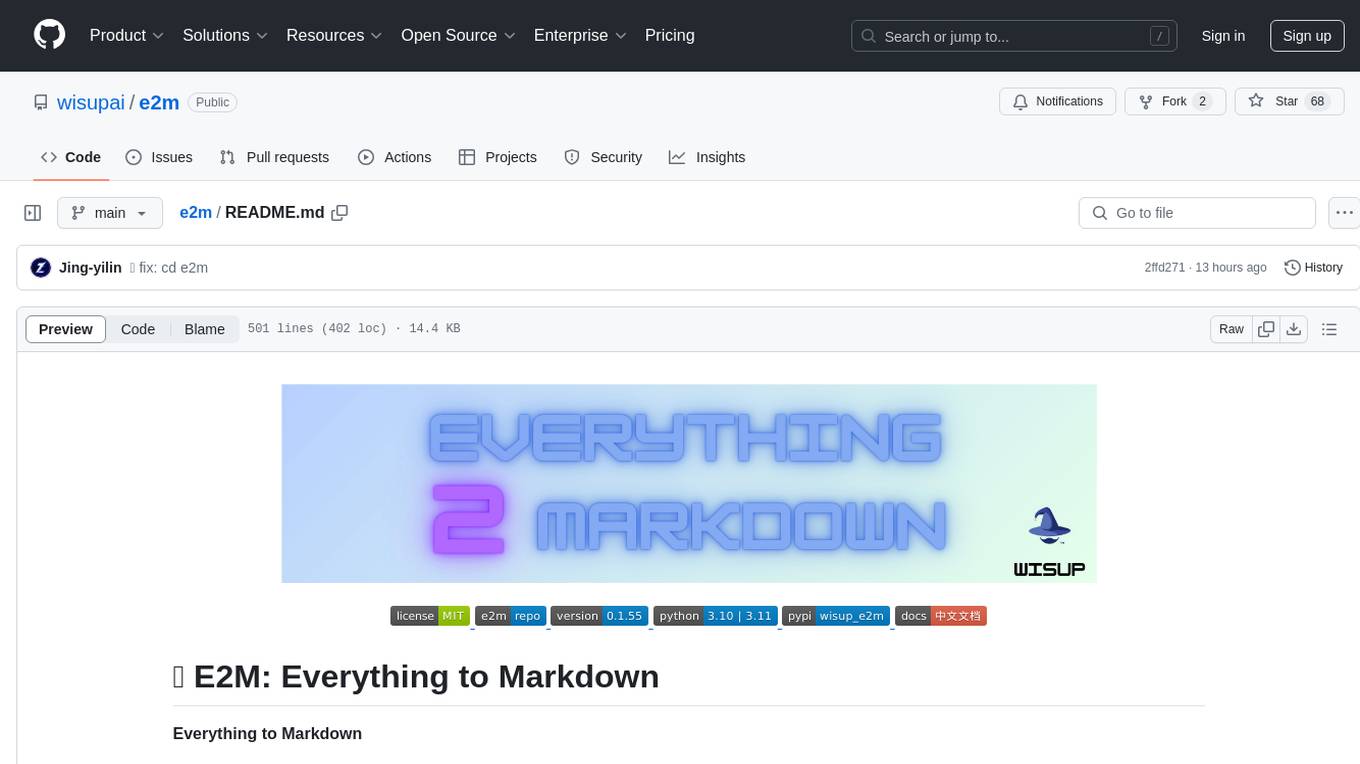
e2m
E2M is a Python library that can parse and convert various file types into Markdown format. It supports the conversion of multiple file formats, including doc, docx, epub, html, htm, url, pdf, ppt, pptx, mp3, and m4a. The ultimate goal of the E2M project is to provide high-quality data for Retrieval-Augmented Generation (RAG) and model training or fine-tuning. The core architecture consists of a Parser responsible for parsing various file types into text or image data, and a Converter responsible for converting text or image data into Markdown format.
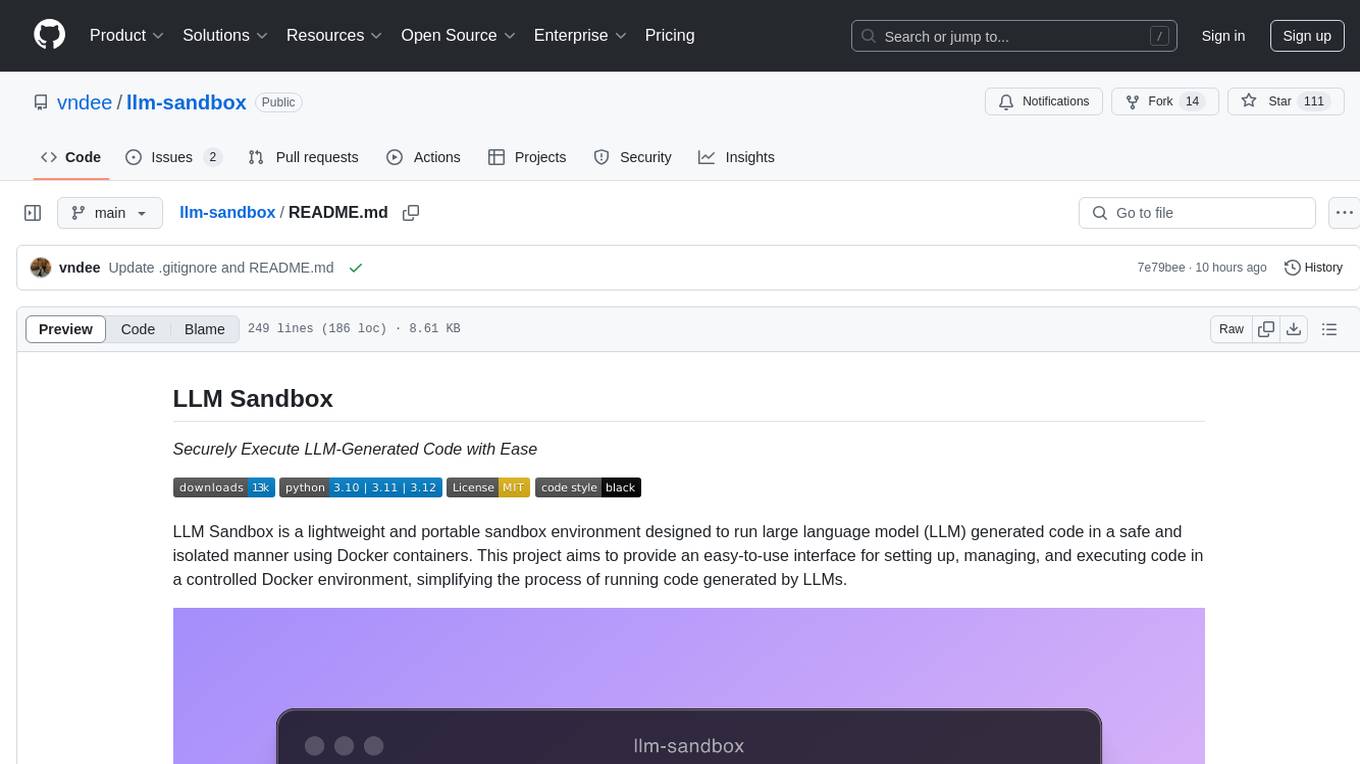
llm-sandbox
LLM Sandbox is a lightweight and portable sandbox environment designed to securely execute large language model (LLM) generated code in a safe and isolated manner using Docker containers. It provides an easy-to-use interface for setting up, managing, and executing code in a controlled Docker environment, simplifying the process of running code generated by LLMs. The tool supports multiple programming languages, offers flexibility with predefined Docker images or custom Dockerfiles, and allows scalability with support for Kubernetes and remote Docker hosts.
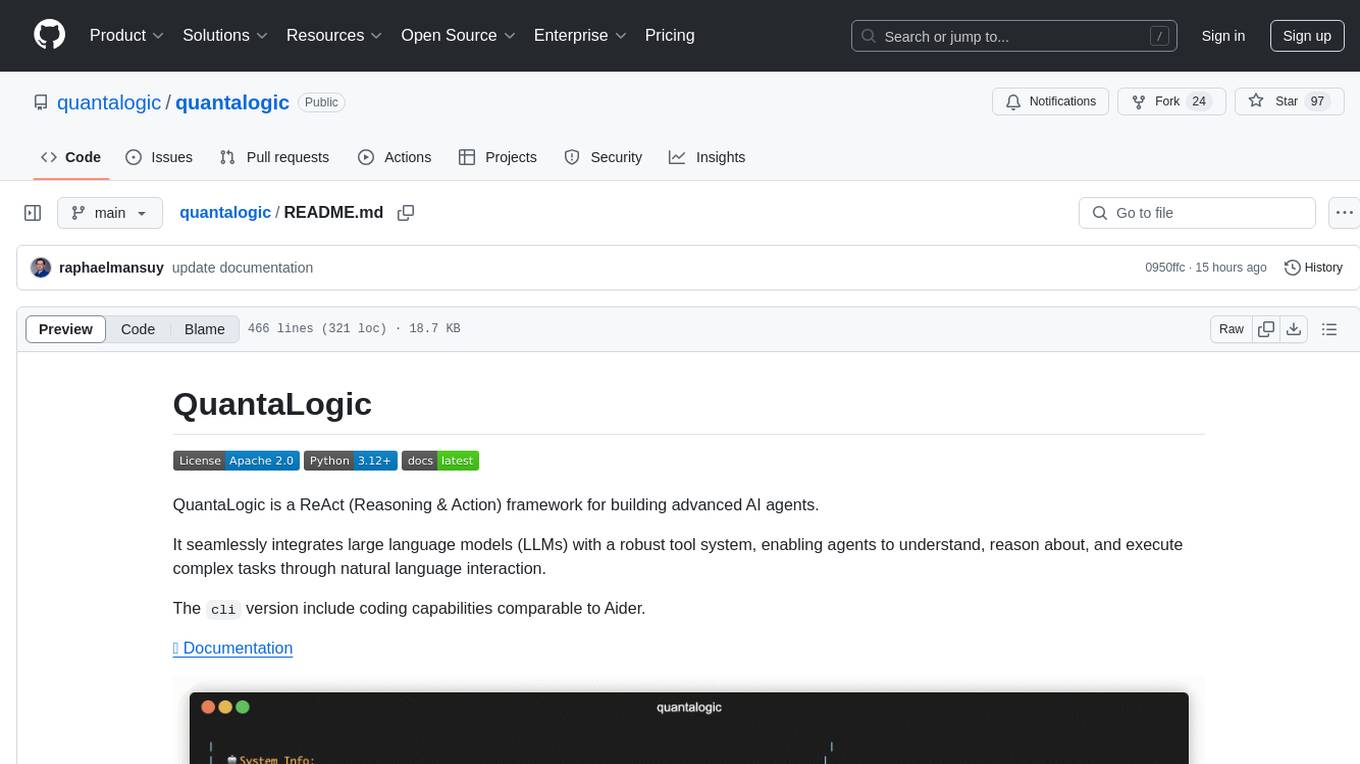
quantalogic
QuantaLogic is a ReAct framework for building advanced AI agents that seamlessly integrates large language models with a robust tool system. It aims to bridge the gap between advanced AI models and practical implementation in business processes by enabling agents to understand, reason about, and execute complex tasks through natural language interaction. The framework includes features such as ReAct Framework, Universal LLM Support, Secure Tool System, Real-time Monitoring, Memory Management, and Enterprise Ready components.
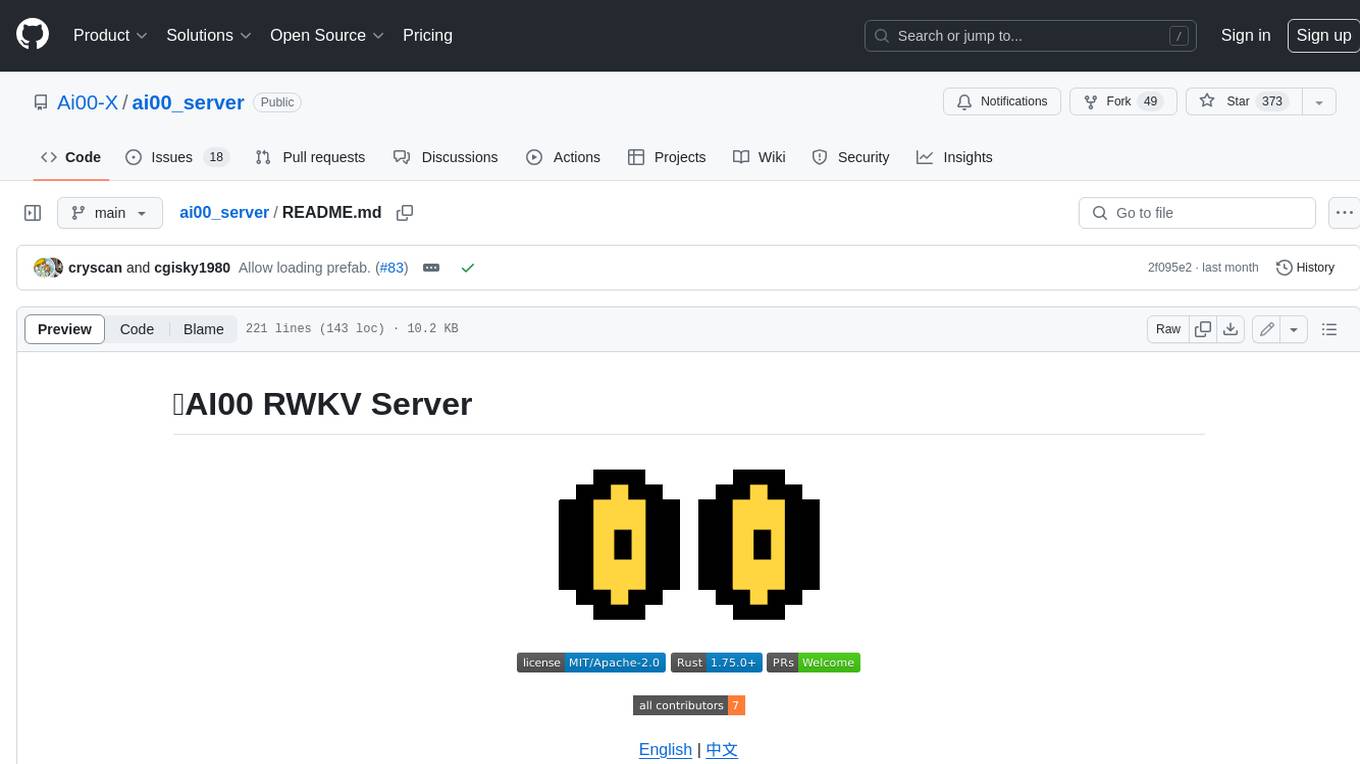
ai00_server
AI00 RWKV Server is an inference API server for the RWKV language model based upon the web-rwkv inference engine. It supports VULKAN parallel and concurrent batched inference and can run on all GPUs that support VULKAN. No need for Nvidia cards!!! AMD cards and even integrated graphics can be accelerated!!! No need for bulky pytorch, CUDA and other runtime environments, it's compact and ready to use out of the box! Compatible with OpenAI's ChatGPT API interface. 100% open source and commercially usable, under the MIT license. If you are looking for a fast, efficient, and easy-to-use LLM API server, then AI00 RWKV Server is your best choice. It can be used for various tasks, including chatbots, text generation, translation, and Q&A.
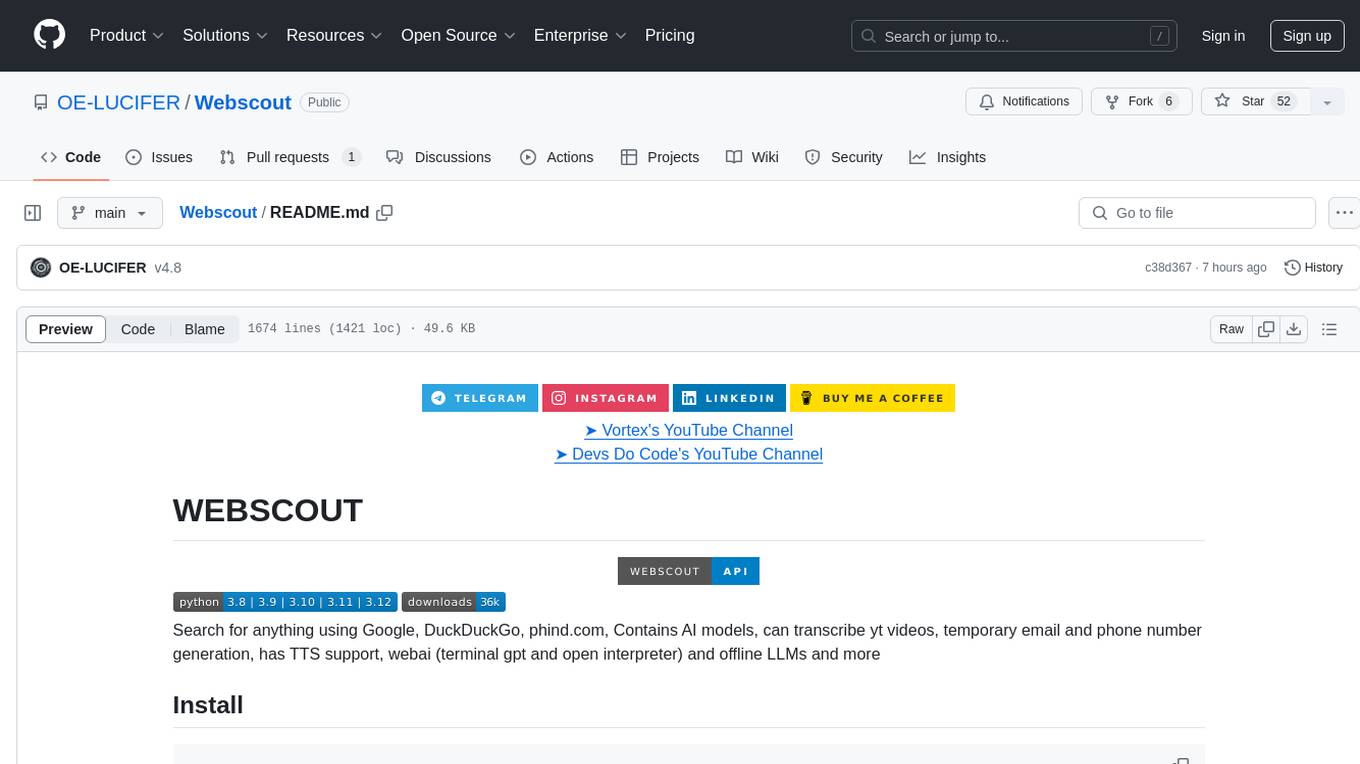
Webscout
WebScout is a versatile tool that allows users to search for anything using Google, DuckDuckGo, and phind.com. It contains AI models, can transcribe YouTube videos, generate temporary email and phone numbers, has TTS support, webai (terminal GPT and open interpreter), and offline LLMs. It also supports features like weather forecasting, YT video downloading, temp mail and number generation, text-to-speech, advanced web searches, and more.
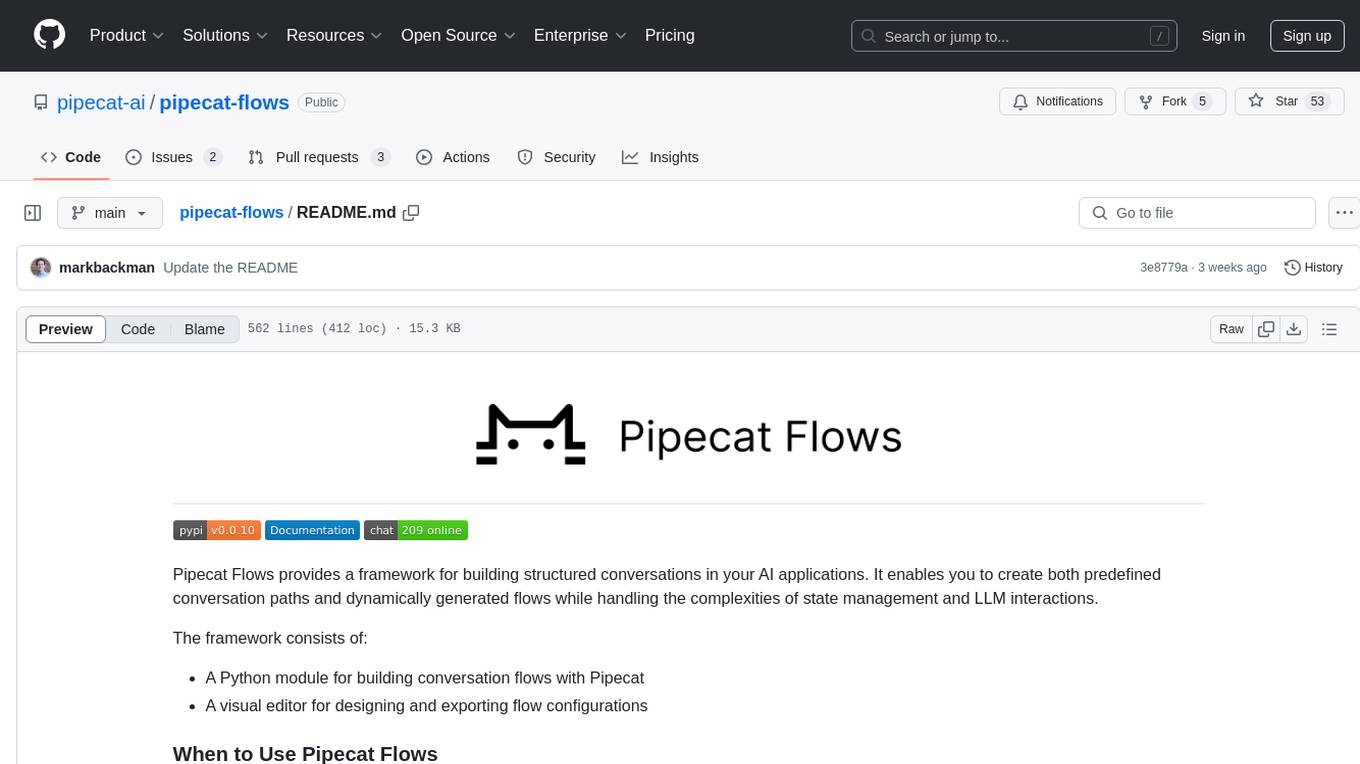
pipecat-flows
Pipecat Flows is a framework designed for building structured conversations in AI applications. It allows users to create both predefined conversation paths and dynamically generated flows, handling state management and LLM interactions. The framework includes a Python module for building conversation flows and a visual editor for designing and exporting flow configurations. Pipecat Flows is suitable for scenarios such as customer service scripts, intake forms, personalized experiences, and complex decision trees.
For similar tasks
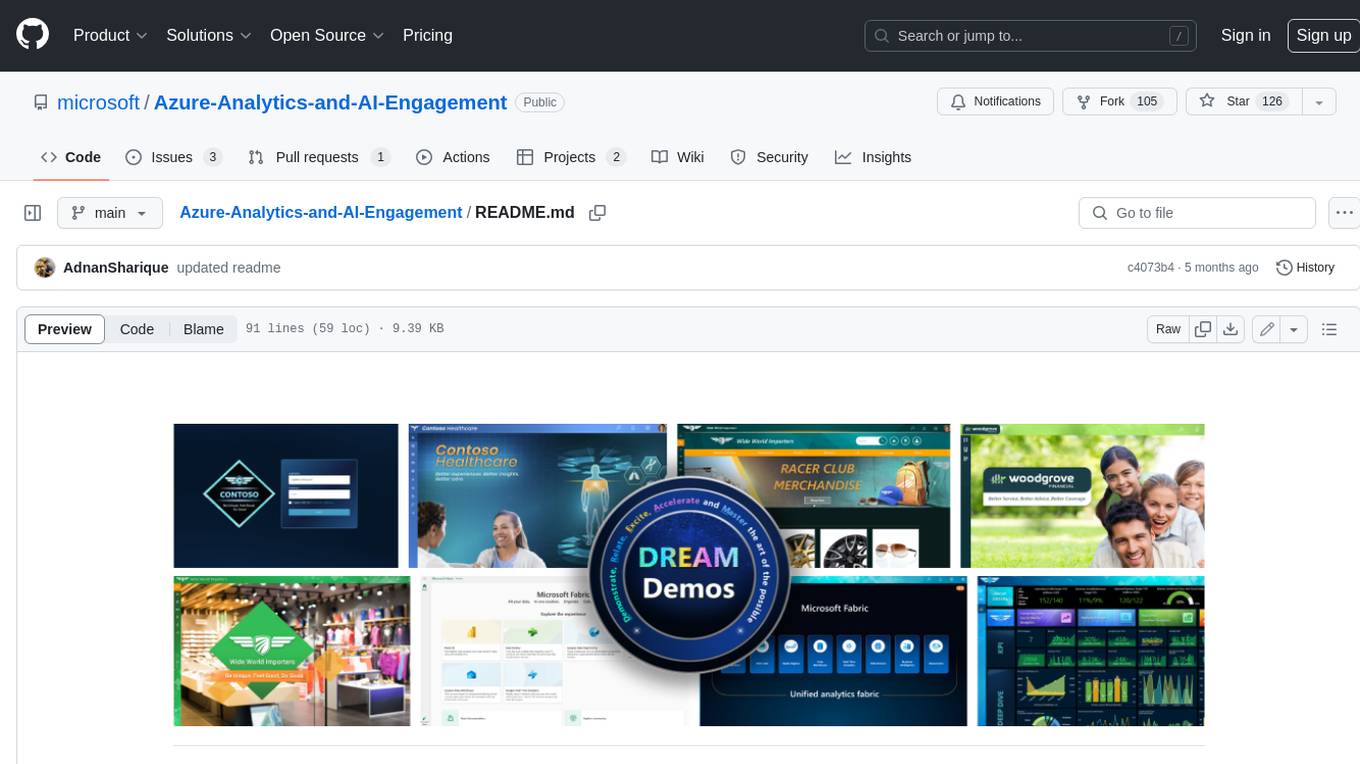
Azure-Analytics-and-AI-Engagement
The Azure-Analytics-and-AI-Engagement repository provides packaged Industry Scenario DREAM Demos with ARM templates (Containing a demo web application, Power BI reports, Synapse resources, AML Notebooks etc.) that can be deployed in a customer’s subscription using the CAPE tool within a matter of few hours. Partners can also deploy DREAM Demos in their own subscriptions using DPoC.
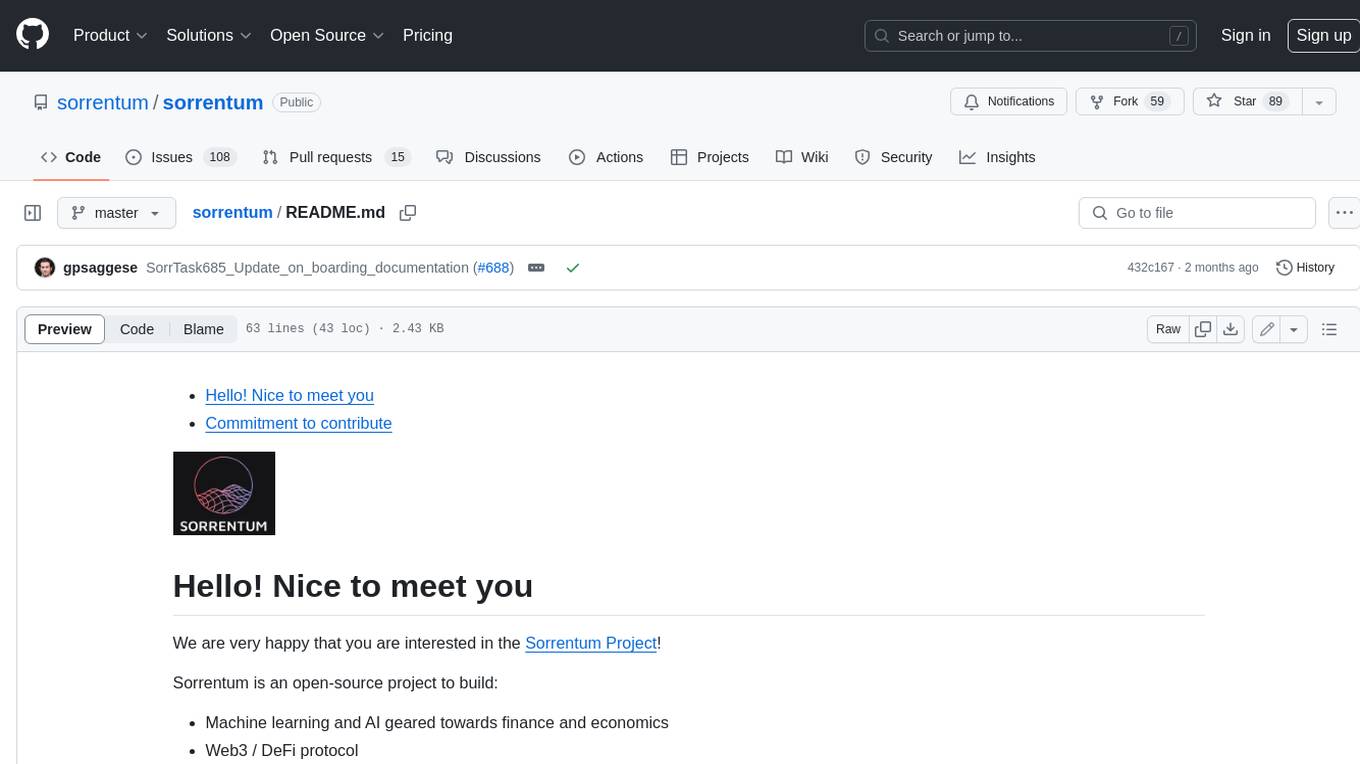
sorrentum
Sorrentum is an open-source project that aims to combine open-source development, startups, and brilliant students to build machine learning, AI, and Web3 / DeFi protocols geared towards finance and economics. The project provides opportunities for internships, research assistantships, and development grants, as well as the chance to work on cutting-edge problems, learn about startups, write academic papers, and get internships and full-time positions at companies working on Sorrentum applications.
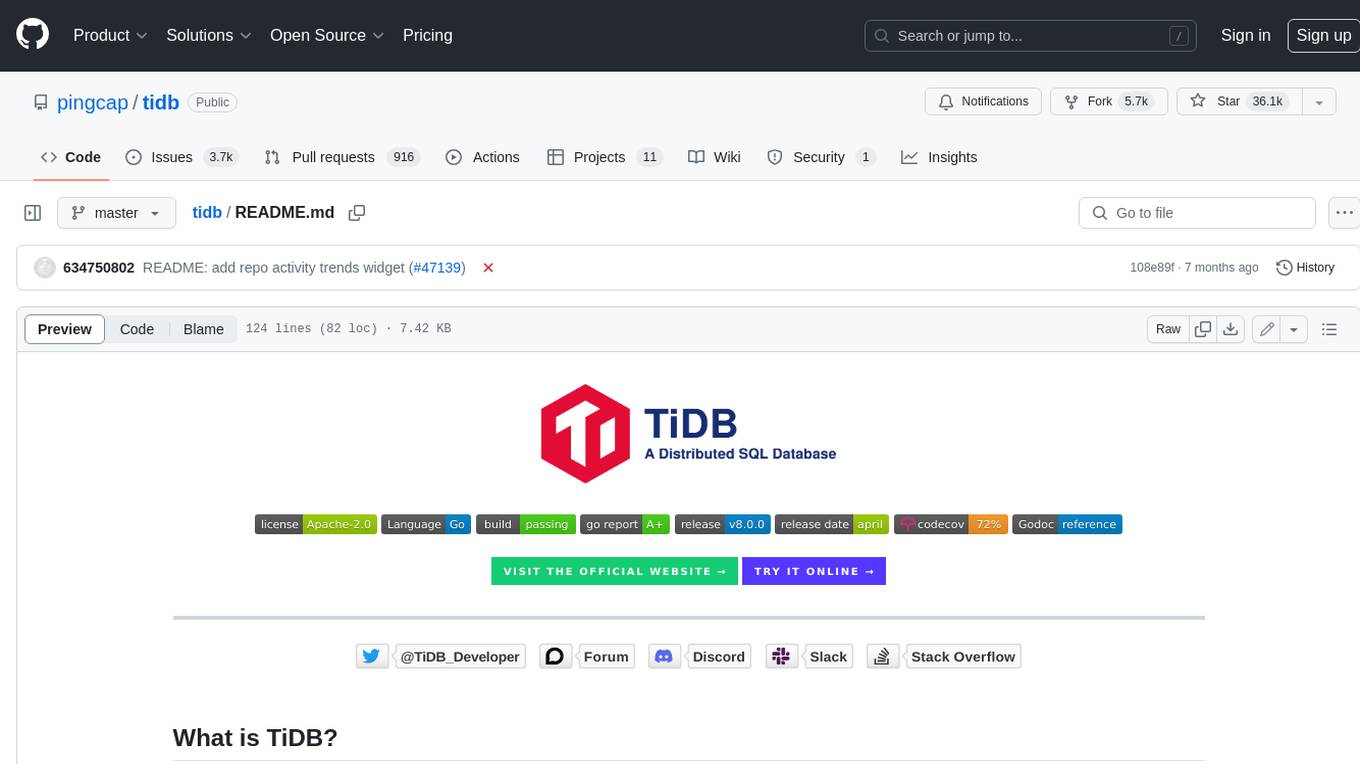
tidb
TiDB is an open-source distributed SQL database that supports Hybrid Transactional and Analytical Processing (HTAP) workloads. It is MySQL compatible and features horizontal scalability, strong consistency, and high availability.
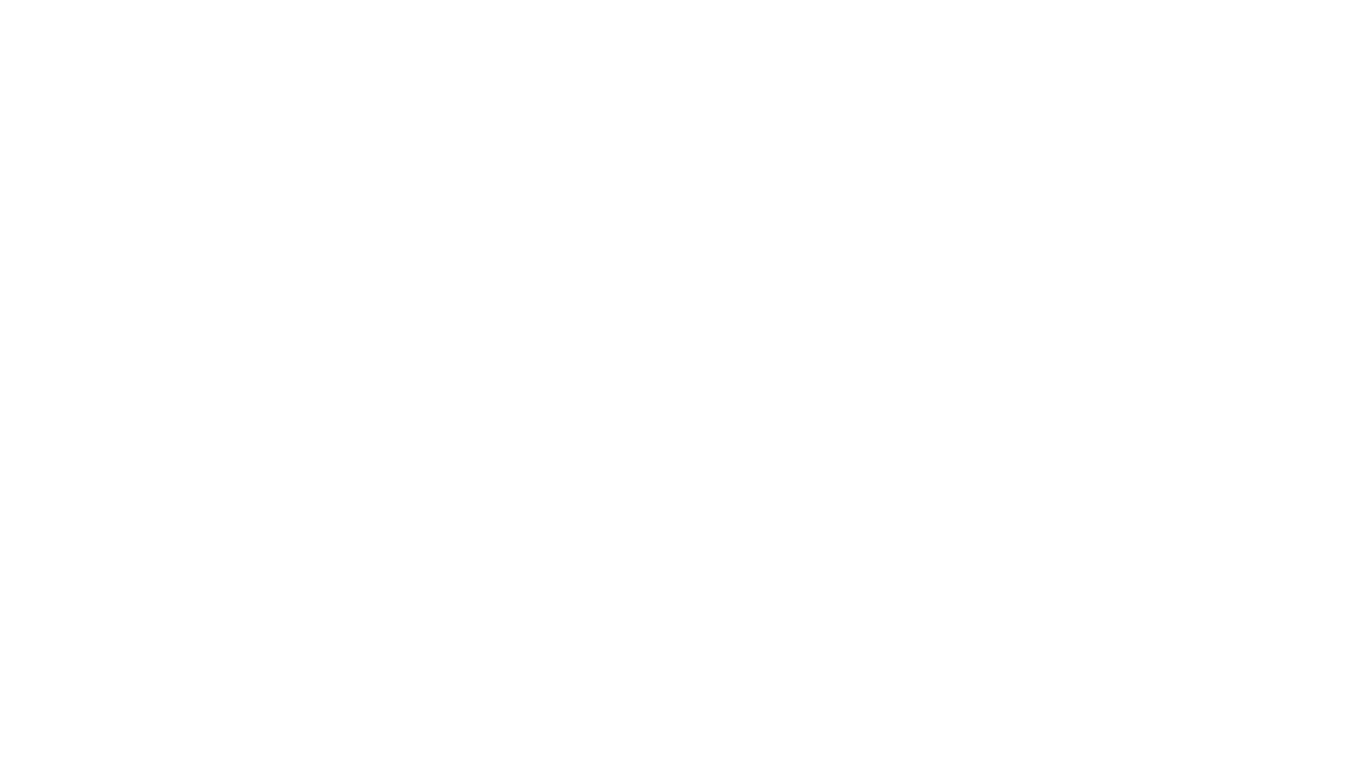
zep-python
Zep is an open-source platform for building and deploying large language model (LLM) applications. It provides a suite of tools and services that make it easy to integrate LLMs into your applications, including chat history memory, embedding, vector search, and data enrichment. Zep is designed to be scalable, reliable, and easy to use, making it a great choice for developers who want to build LLM-powered applications quickly and easily.
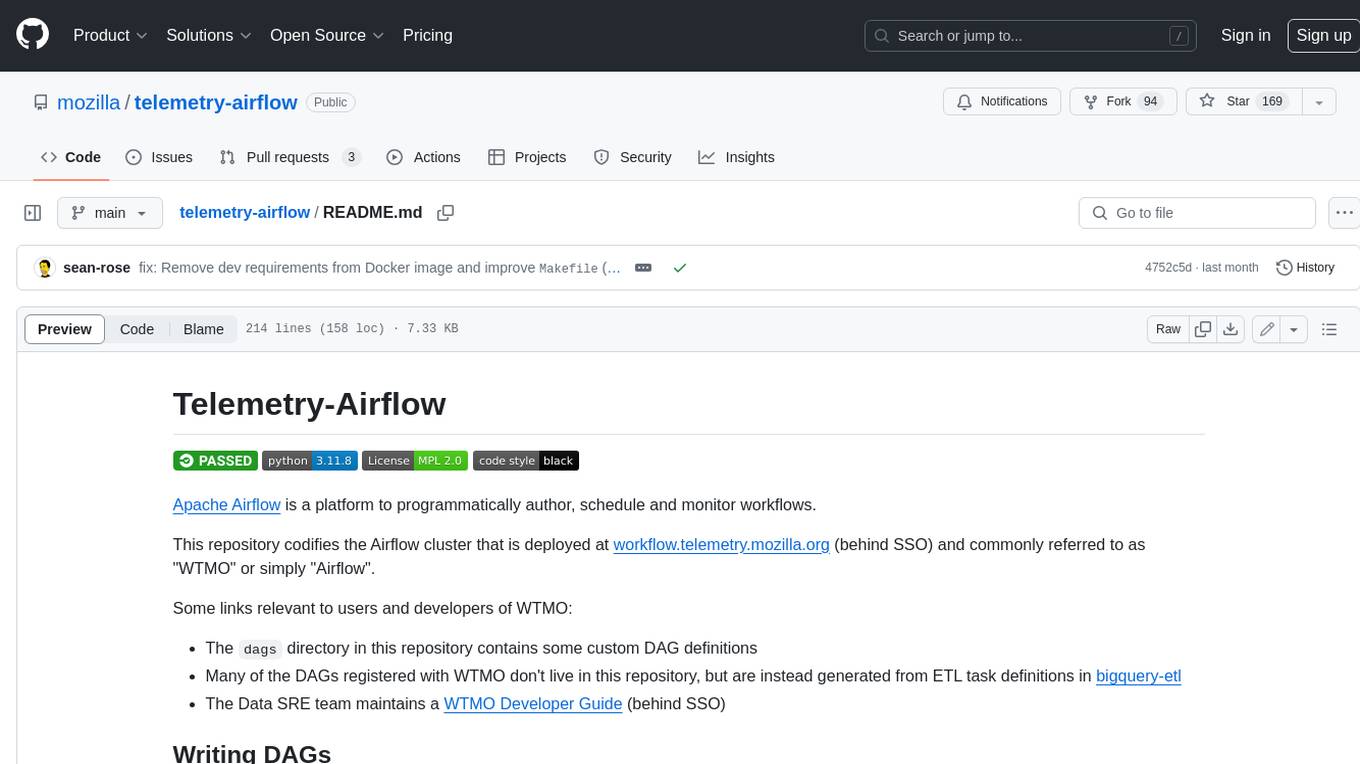
telemetry-airflow
This repository codifies the Airflow cluster that is deployed at workflow.telemetry.mozilla.org (behind SSO) and commonly referred to as "WTMO" or simply "Airflow". Some links relevant to users and developers of WTMO: * The `dags` directory in this repository contains some custom DAG definitions * Many of the DAGs registered with WTMO don't live in this repository, but are instead generated from ETL task definitions in bigquery-etl * The Data SRE team maintains a WTMO Developer Guide (behind SSO)
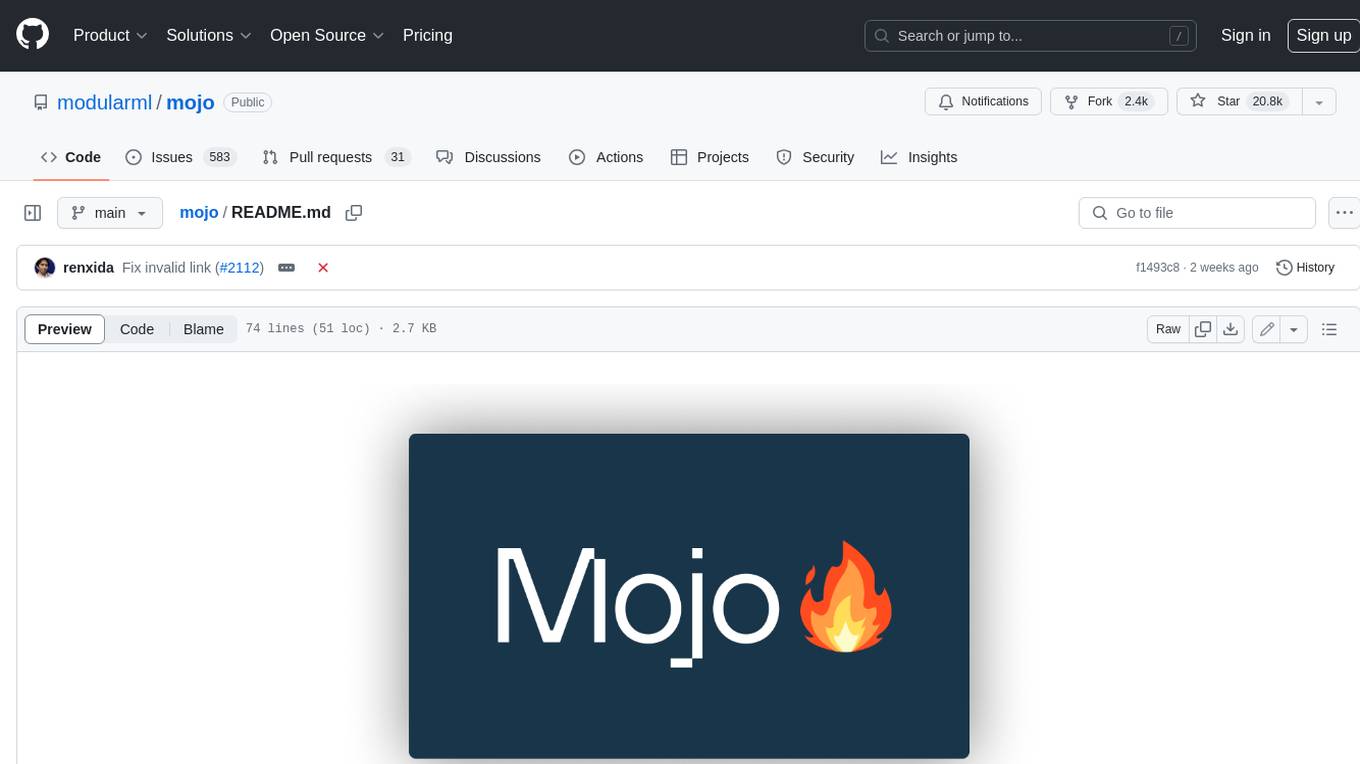
mojo
Mojo is a new programming language that bridges the gap between research and production by combining Python syntax and ecosystem with systems programming and metaprogramming features. Mojo is still young, but it is designed to become a superset of Python over time.
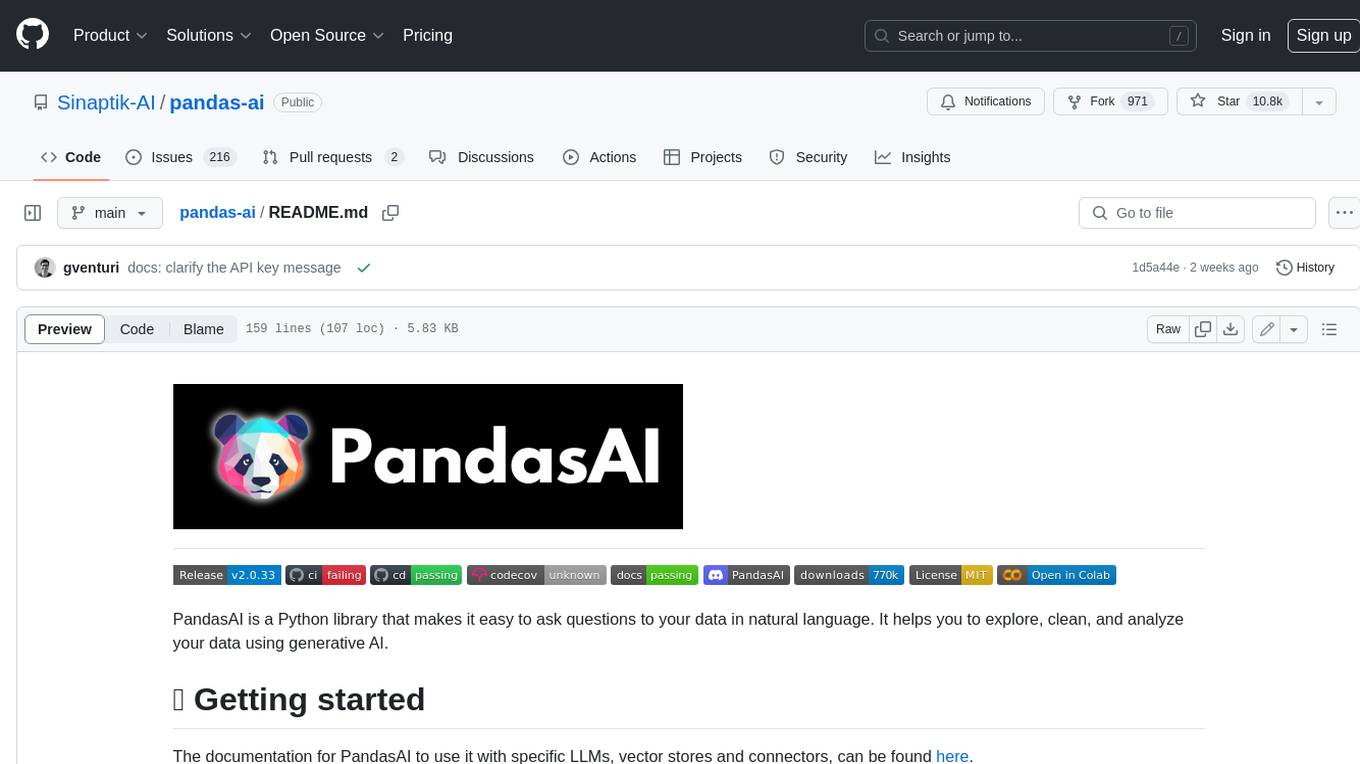
pandas-ai
PandasAI is a Python library that makes it easy to ask questions to your data in natural language. It helps you to explore, clean, and analyze your data using generative AI.
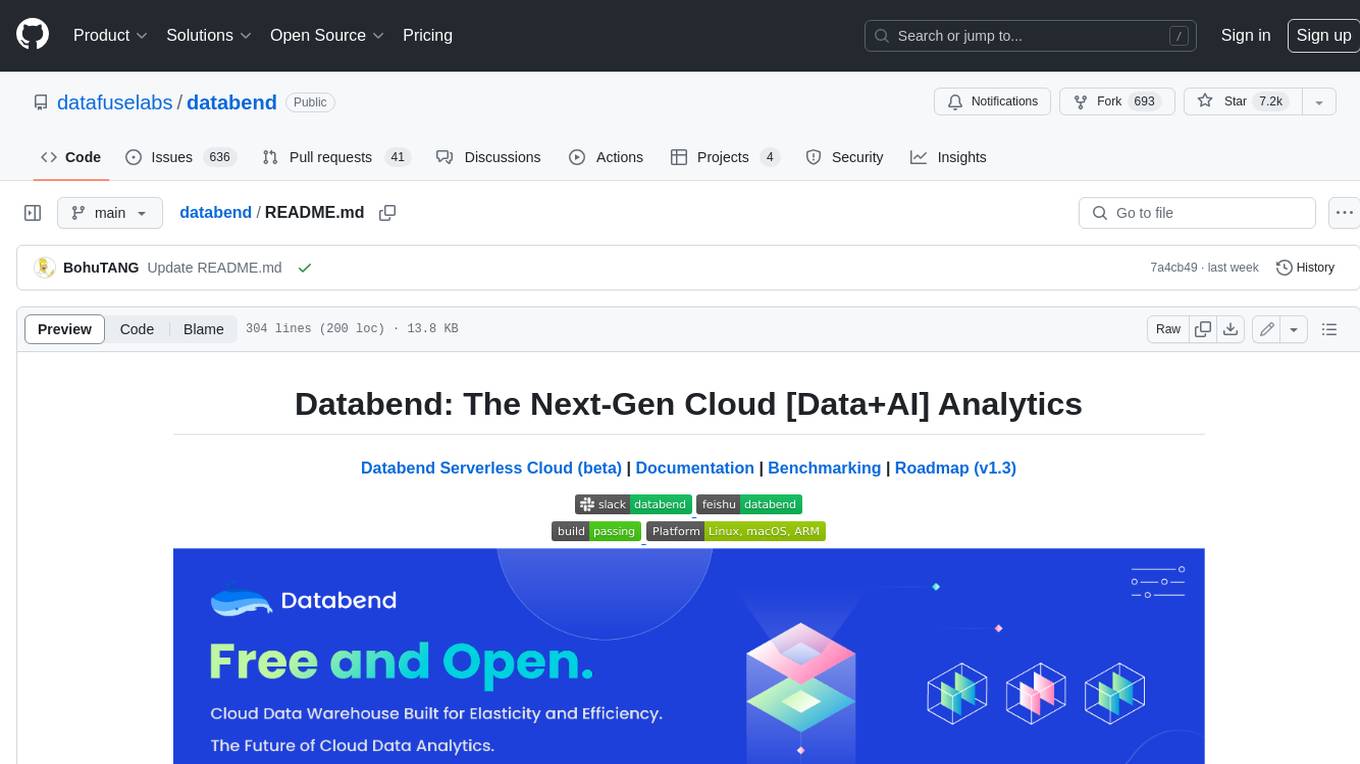
databend
Databend is an open-source cloud data warehouse that serves as a cost-effective alternative to Snowflake. With its focus on fast query execution and data ingestion, it's designed for complex analysis of the world's largest datasets.
For similar jobs
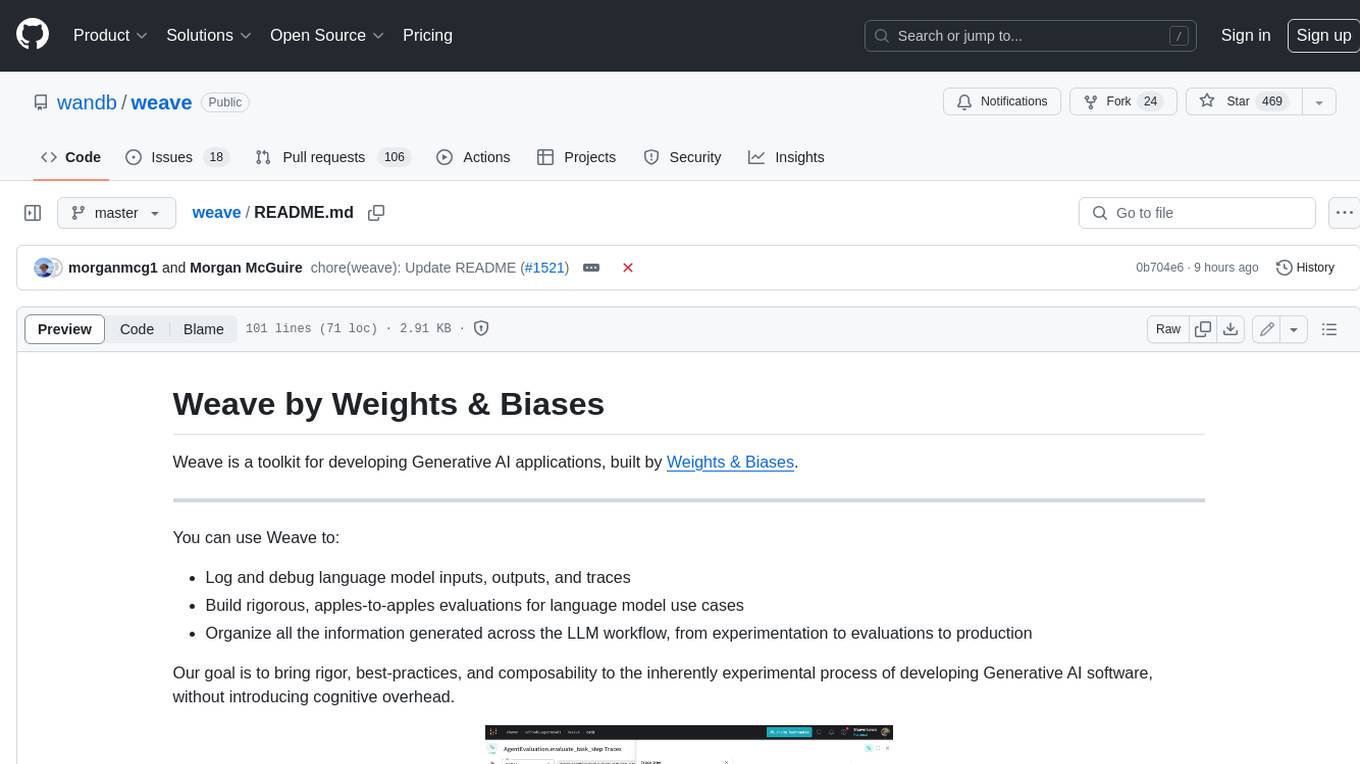
weave
Weave is a toolkit for developing Generative AI applications, built by Weights & Biases. With Weave, you can log and debug language model inputs, outputs, and traces; build rigorous, apples-to-apples evaluations for language model use cases; and organize all the information generated across the LLM workflow, from experimentation to evaluations to production. Weave aims to bring rigor, best-practices, and composability to the inherently experimental process of developing Generative AI software, without introducing cognitive overhead.
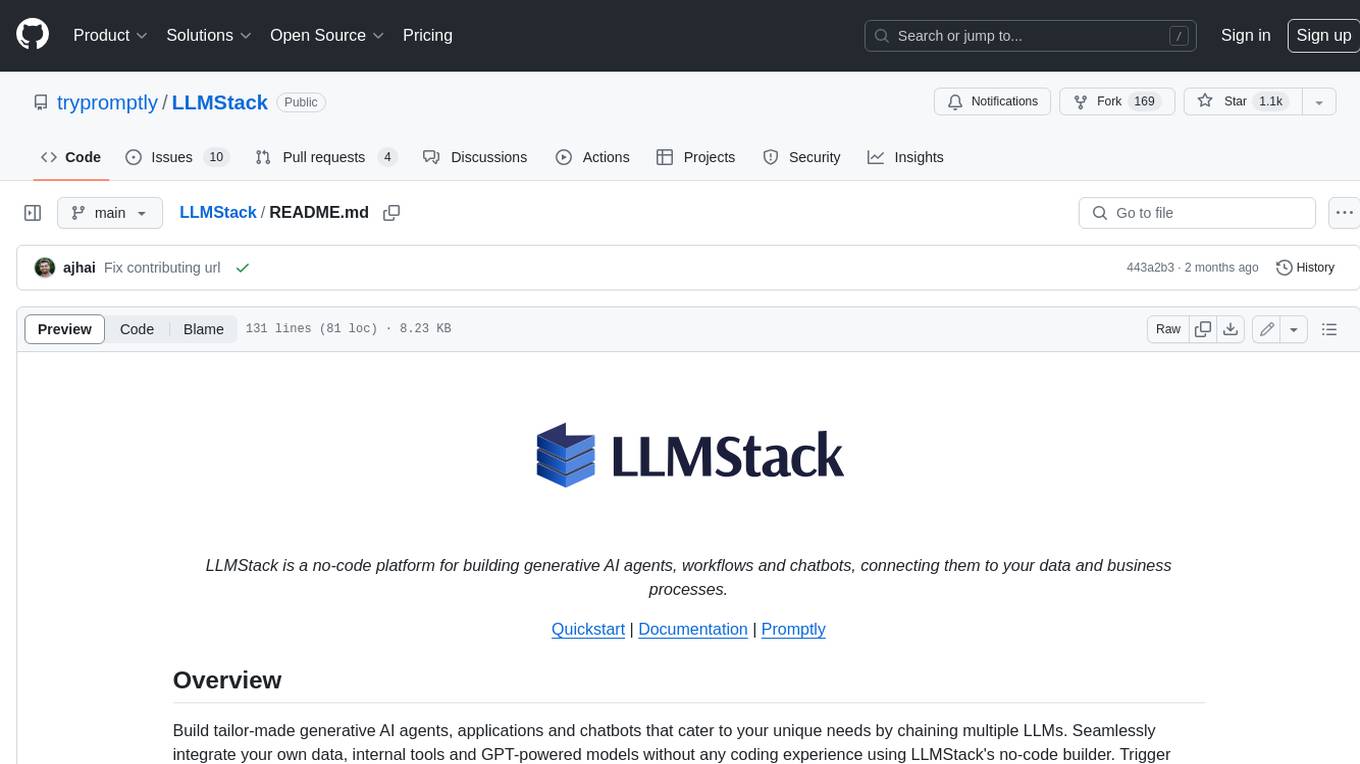
LLMStack
LLMStack is a no-code platform for building generative AI agents, workflows, and chatbots. It allows users to connect their own data, internal tools, and GPT-powered models without any coding experience. LLMStack can be deployed to the cloud or on-premise and can be accessed via HTTP API or triggered from Slack or Discord.
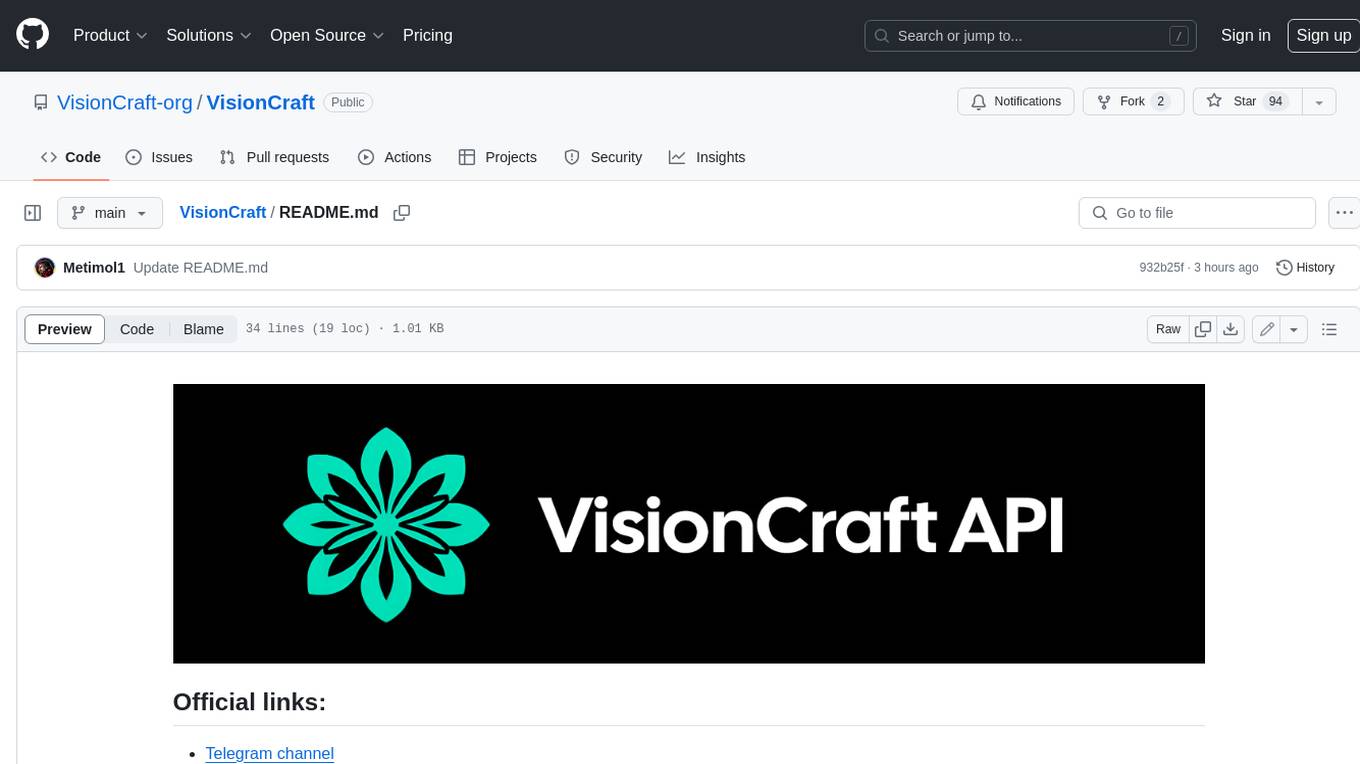
VisionCraft
The VisionCraft API is a free API for using over 100 different AI models. From images to sound.
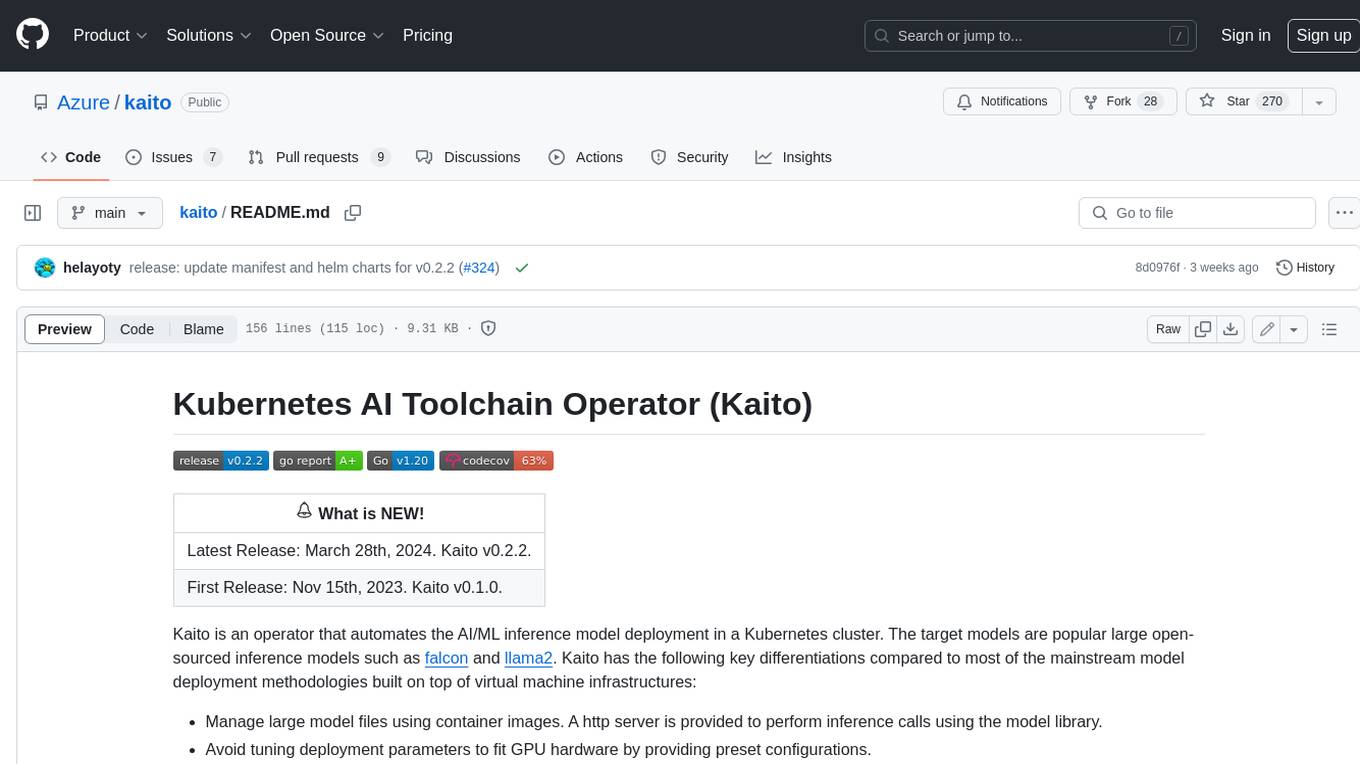
kaito
Kaito is an operator that automates the AI/ML inference model deployment in a Kubernetes cluster. It manages large model files using container images, avoids tuning deployment parameters to fit GPU hardware by providing preset configurations, auto-provisions GPU nodes based on model requirements, and hosts large model images in the public Microsoft Container Registry (MCR) if the license allows. Using Kaito, the workflow of onboarding large AI inference models in Kubernetes is largely simplified.
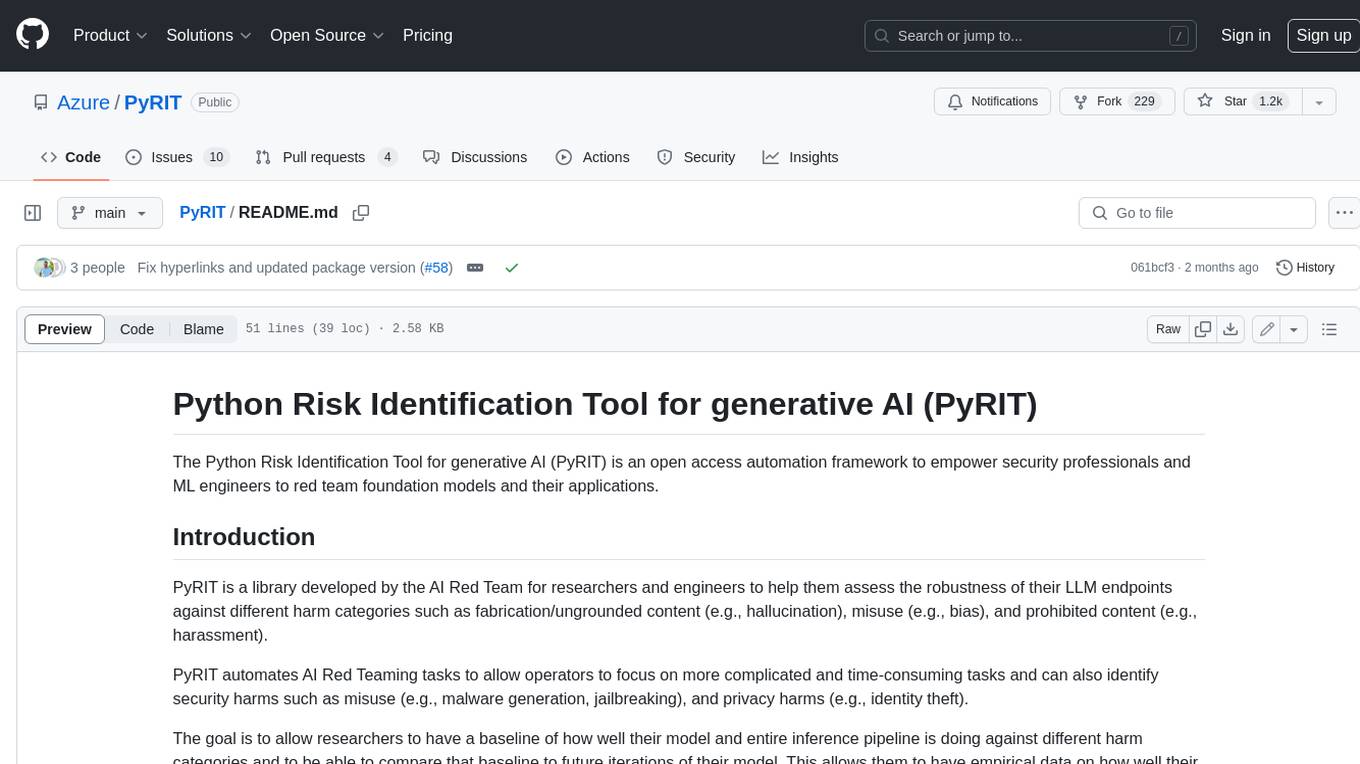
PyRIT
PyRIT is an open access automation framework designed to empower security professionals and ML engineers to red team foundation models and their applications. It automates AI Red Teaming tasks to allow operators to focus on more complicated and time-consuming tasks and can also identify security harms such as misuse (e.g., malware generation, jailbreaking), and privacy harms (e.g., identity theft). The goal is to allow researchers to have a baseline of how well their model and entire inference pipeline is doing against different harm categories and to be able to compare that baseline to future iterations of their model. This allows them to have empirical data on how well their model is doing today, and detect any degradation of performance based on future improvements.
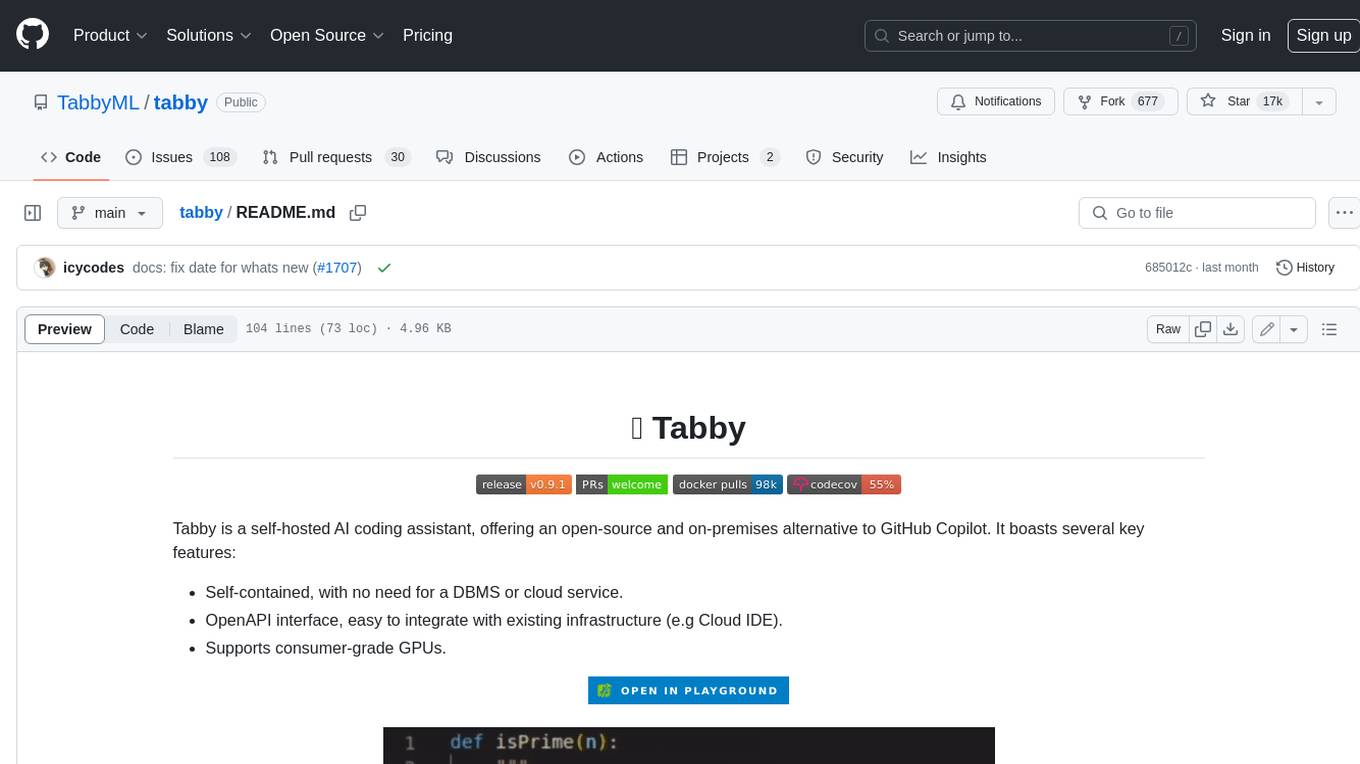
tabby
Tabby is a self-hosted AI coding assistant, offering an open-source and on-premises alternative to GitHub Copilot. It boasts several key features: * Self-contained, with no need for a DBMS or cloud service. * OpenAPI interface, easy to integrate with existing infrastructure (e.g Cloud IDE). * Supports consumer-grade GPUs.
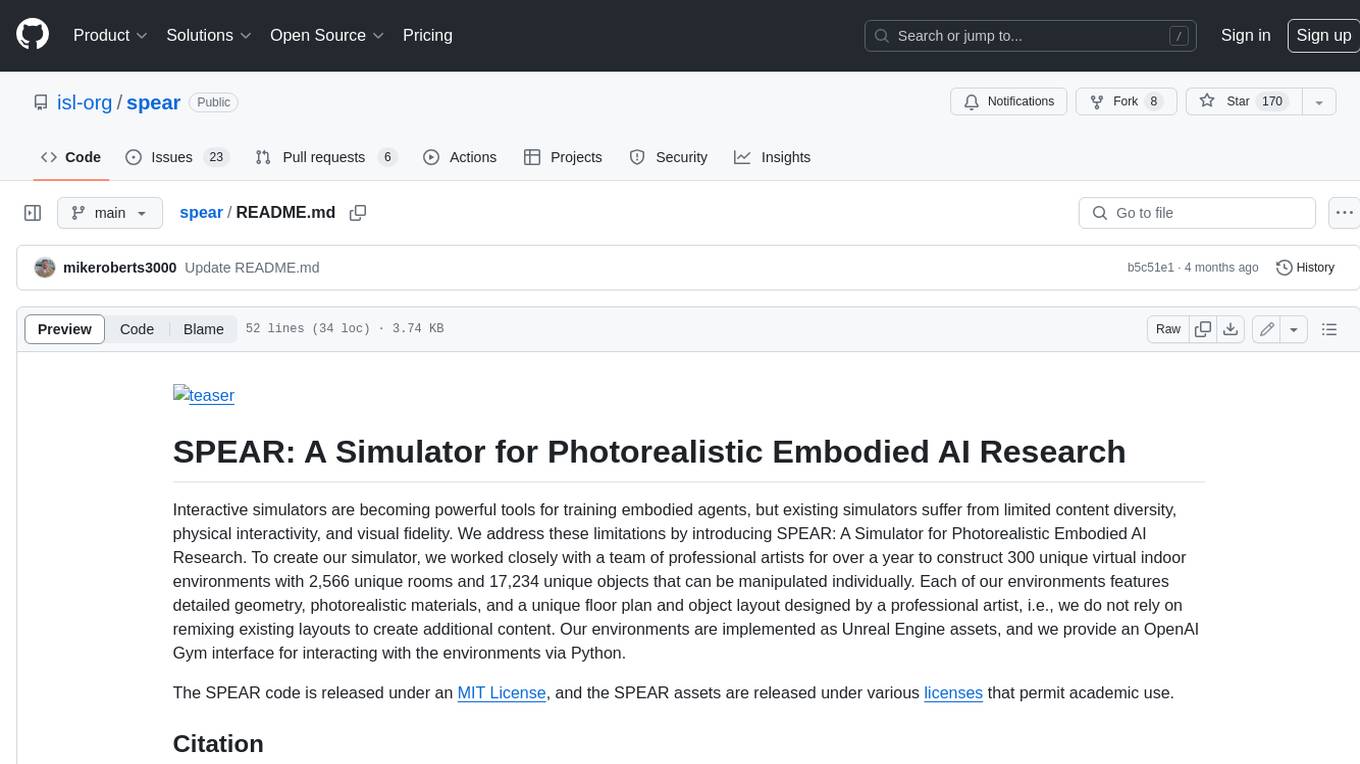
spear
SPEAR (Simulator for Photorealistic Embodied AI Research) is a powerful tool for training embodied agents. It features 300 unique virtual indoor environments with 2,566 unique rooms and 17,234 unique objects that can be manipulated individually. Each environment is designed by a professional artist and features detailed geometry, photorealistic materials, and a unique floor plan and object layout. SPEAR is implemented as Unreal Engine assets and provides an OpenAI Gym interface for interacting with the environments via Python.
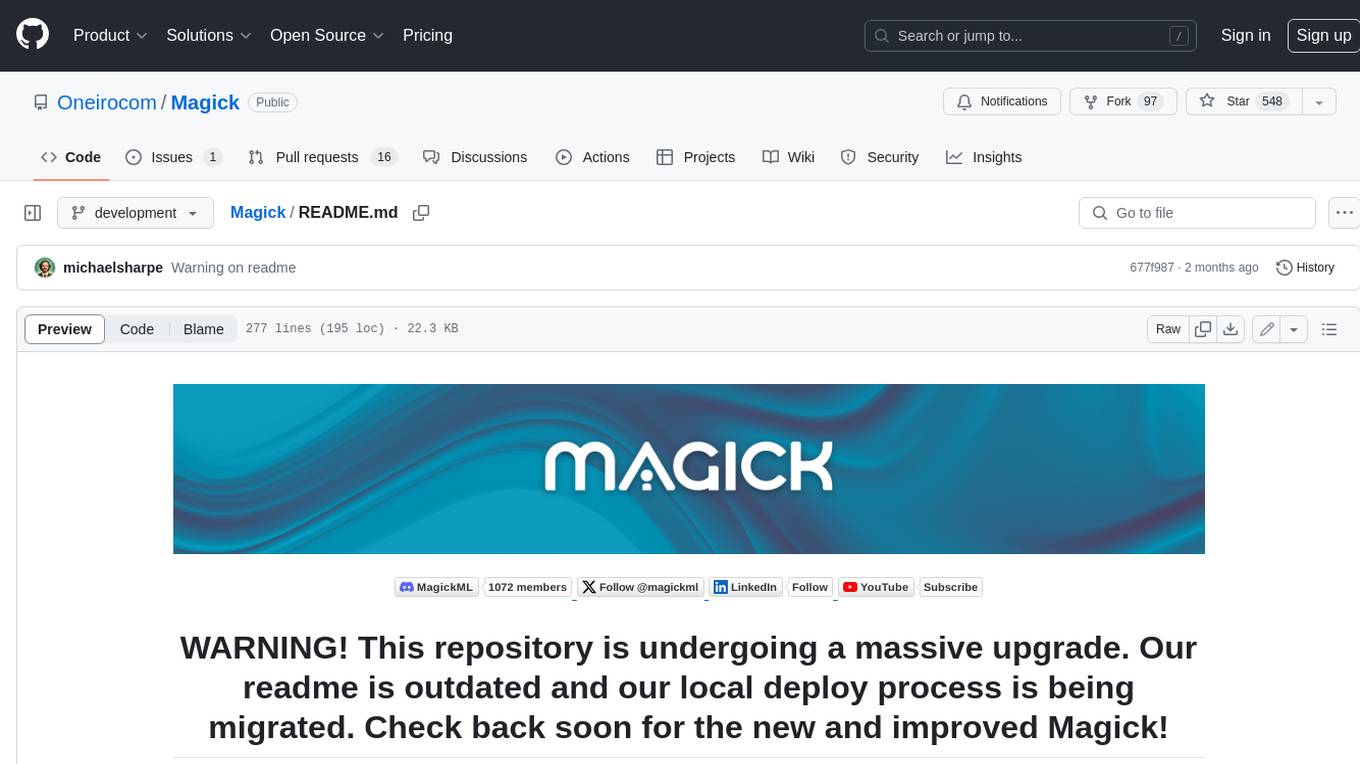
Magick
Magick is a groundbreaking visual AIDE (Artificial Intelligence Development Environment) for no-code data pipelines and multimodal agents. Magick can connect to other services and comes with nodes and templates well-suited for intelligent agents, chatbots, complex reasoning systems and realistic characters.