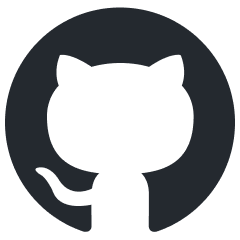
ai00_server
The all-in-one RWKV runtime box with embed, RAG, AI agents, and more.
Stars: 515
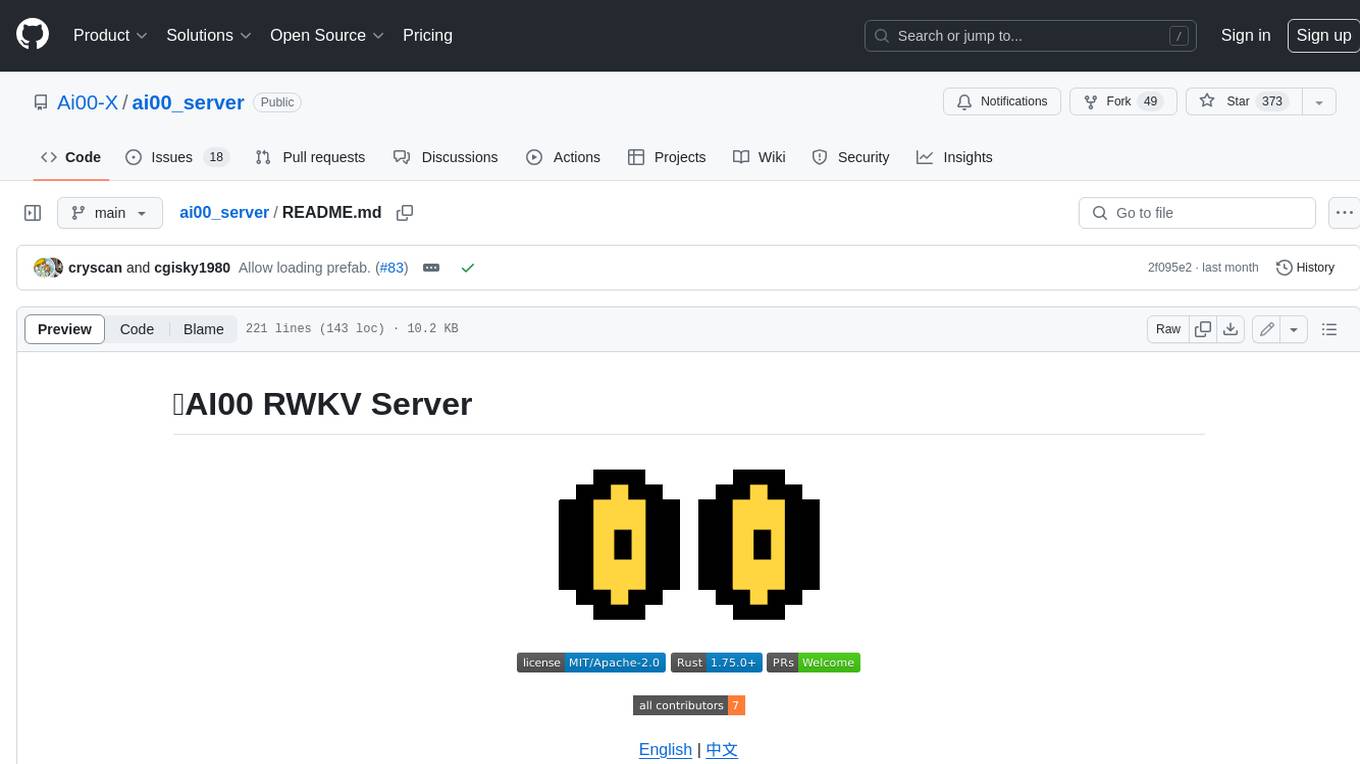
AI00 RWKV Server is an inference API server for the RWKV language model based upon the web-rwkv inference engine. It supports VULKAN parallel and concurrent batched inference and can run on all GPUs that support VULKAN. No need for Nvidia cards!!! AMD cards and even integrated graphics can be accelerated!!! No need for bulky pytorch, CUDA and other runtime environments, it's compact and ready to use out of the box! Compatible with OpenAI's ChatGPT API interface. 100% open source and commercially usable, under the MIT license. If you are looking for a fast, efficient, and easy-to-use LLM API server, then AI00 RWKV Server is your best choice. It can be used for various tasks, including chatbots, text generation, translation, and Q&A.
README:
AI00 RWKV Server
is an inference API server for the RWKV
language model based upon the web-rwkv
inference engine.
It supports Vulkan
parallel and concurrent batched inference and can run on all GPUs that support Vulkan
. No need for Nvidia cards!!! AMD cards and even integrated graphics can be accelerated!!!
No need for bulky pytorch
, CUDA
and other runtime environments, it's compact and ready to use out of the box!
Compatible with OpenAI's ChatGPT API interface.
100% open source and commercially usable, under the MIT license.
If you are looking for a fast, efficient, and easy-to-use LLM API server, then AI00 RWKV Server
is your best choice. It can be used for various tasks, including chatbots, text generation, translation, and Q&A.
Join the AI00 RWKV Server
community now and experience the charm of AI!
QQ Group for communication: 30920262
- Based on the
RWKV
model, it has high performance and accuracy - Supports
Vulkan
inference acceleration, you can enjoy GPU acceleration without the need forCUDA
! Supports AMD cards, integrated graphics, and all GPUs that supportVulkan
- No need for bulky
pytorch
,CUDA
and other runtime environments, it's compact and ready to use out of the box! - Compatible with OpenAI's ChatGPT API interface
- Chatbot
- Text generation
- Translation
- Q&A
- Any other tasks that LLM can do
-
Directly download the latest version from Release
-
After downloading the model, place the model in the
assets/models/
path, for example,assets/models/RWKV-x060-World-3B-v2-20240228-ctx4096.st
-
Optionally modify
assets/configs/Config.toml
for model configurations like model path, quantization layers, etc. -
Run in the command line
$ ./ai00_rwkv_server
-
Open the browser and visit the WebUI at http://localhost:65530 (https://localhost:65530 if
tls
is enabled)
-
Clone this repository
$ git clone https://github.com/cgisky1980/ai00_rwkv_server.git $ cd ai00_rwkv_server
-
After downloading the model, place the model in the
assets/models/
path, for example,assets/models/RWKV-x060-World-3B-v2-20240228-ctx4096.st
-
Compile
$ cargo build --release
-
After compilation, run
$ cargo run --release
-
Open the browser and visit the WebUI at http://localhost:65530 (https://localhost:65530 if
tls
is enabled)
It only supports Safetensors models with the .st
extension now. Models saved with the .pth
extension using torch need to be converted before use.
-
(Recommended) Run the python script
convert_ai00.py
orconvert_safetensors.py
:$ python assets/scripts/convert_ai00.py --input /path/to/model.pth --output /path/to/model.st
Requirements: Python, with
torch
andsafetensors
installed. -
If you do not want to install python, In the Release you could find an executable called
converter
. Run
$ ./converter --input /path/to/model.pth --output /path/to/model.st
- If you are building from source, run
$ cargo run --release --package converter -- --input /path/to/model.pth --output /path/to/model.st
- Just like the steps mentioned above, place the model in the
.st
model in theassets/models/
path and modify the model path inassets/configs/Config.toml
-
--config
: Configure file path (default:assets/configs/Config.toml
) -
--ip
: The IP address the server is bound to -
--port
: Running port
The API service starts at port 65530, and the data input and output format follow the Openai API specification.
Note that some APIs like chat
and completions
have additional optional fields for advanced functionalities. Visit http://localhost:65530/api-docs for API schema.
/api/oai/v1/models
/api/oai/models
/api/oai/v1/chat/completions
/api/oai/chat/completions
/api/oai/v1/completions
/api/oai/completions
/api/oai/v1/embeddings
/api/oai/embeddings
The following is an out-of-box example of Ai00 API invocations in Python:
import openai
class Ai00:
def __init__(self,model="model",port=65530,api_key="JUSTSECRET_KEY") :
openai.api_base = f"http://127.0.0.1:{port}/api/oai"
openai.api_key = api_key
self.ctx = []
self.params = {
"system_name": "System",
"user_name": "User",
"assistant_name": "Assistant",
"model": model,
"max_tokens": 4096,
"top_p": 0.6,
"temperature": 1,
"presence_penalty": 0.3,
"frequency_penalty": 0.3,
"half_life": 400,
"stop": ['\x00','\n\n']
}
def set_params(self,**kwargs):
self.params.update(kwargs)
def clear_ctx(self):
self.ctx = []
def get_ctx(self):
return self.ctx
def continuation(self, message):
response = openai.Completion.create(
model=self.params['model'],
prompt=message,
max_tokens=self.params['max_tokens'],
half_life=self.params['half_life'],
top_p=self.params['top_p'],
temperature=self.params['temperature'],
presence_penalty=self.params['presence_penalty'],
frequency_penalty=self.params['frequency_penalty'],
stop=self.params['stop']
)
result = response.choices[0].text
return result
def append_ctx(self,role,content):
self.ctx.append({
"role": role,
"content": content
})
def send_message(self, message,role="user"):
self.ctx.append({
"role": role,
"content": message
})
result = openai.ChatCompletion.create(
model=self.params['model'],
messages=self.ctx,
names={
"system": self.params['system_name'],
"user": self.params['user_name'],
"assistant": self.params['assistant_name']
},
max_tokens=self.params['max_tokens'],
half_life=self.params['half_life'],
top_p=self.params['top_p'],
temperature=self.params['temperature'],
presence_penalty=self.params['presence_penalty'],
frequency_penalty=self.params['frequency_penalty'],
stop=self.params['stop']
)
result = result.choices[0].message['content']
self.ctx.append({
"role": "assistant",
"content": result
})
return result
ai00 = Ai00()
ai00.set_params(
max_tokens = 4096,
top_p = 0.55,
temperature = 2,
presence_penalty = 0.3,
frequency_penalty = 0.8,
half_life = 400,
stop = ['\x00','\n\n']
)
print(ai00.send_message("how are you?"))
print(ai00.send_message("me too!"))
print(ai00.get_ctx())
ai00.clear_ctx()
print(ai00.continuation("i like"))
Since v0.5, Ai00 has a unique feature called BNF sampling. BNF forces the model to output in specified formats (e.g., JSON or markdown with specified fields) by limiting the possible next tokens the model can choose from.
Here is an example BNF for JSON with fields "name", "age" and "job":
<start> ::= <json_object>
<json_object> ::= "{" <object_members> "}"
<object_members> ::= <json_member> | <json_member> ", " <object_members>
<json_member> ::= <json_key> ": " <json_value>
<json_key> ::= '"' "name" '"' | '"' "age" '"' | '"' "job" '"'
<json_value> ::= <json_string> | <json_number>
<json_string>::='"'<content>'"'
<content>::=<except!([escaped_literals])>|<except!([escaped_literals])><content>|'\\"'<content>|'\\"'
<escaped_literals>::='\t'|'\n'|'\r'|'"'
<json_number> ::= <positive_digit><digits>|'0'
<digits>::=<digit>|<digit><digits>
<digit>::='0'|<positive_digit>
<positive_digit>::="1"|"2"|"3"|"4"|"5"|"6"|"7"|"8"|"9"




- [x] Support for
text_completions
andchat_completions
- [x] Support for sse push
- [x] Integrate basic front-end
- [x] Parallel inference via
batch serve
- [x] Support for
int8
quantization - [x] Support for
NF4
quantization - [x] Support for
LoRA
model - [x] Support for tuned initial states
- [ ] Hot loading and switching of
LoRA
model - [x] Hot loading and switching of tuned initial states
- [x] BNF sampling
We are always looking for people interested in helping us improve the project. If you are interested in any of the following, please join us!
- 💀Writing code
- 💬Providing feedback
- 🔆Proposing ideas or needs
- 🔍Testing new features
- ✏Translating documentation
- 📣Promoting the project
- 🏅Anything else that would be helpful to us
No matter your skill level, we welcome you to join us. You can join us in the following ways:
- Join our Discord channel
- Join our QQ group
- Submit issues or pull requests on GitHub
- Leave feedback on our website
We can't wait to work with you to make this project better! We hope the project is helpful to you!
Thank you to these awesome individuals who are insightful and outstanding for their support and selfless dedication to the project!
顾真牛 📖 💻 🖋 🎨 🧑🏫 |
研究社交 💻 💡 🤔 🚧 👀 📦 |
josc146 🐛 💻 🤔 🔧 |
l15y 🔧 🔌 💻 |
Cahya Wirawan 🐛 |
yuunnn_w 📖 |
longzou 💻 🛡️ |
luoqiqi 📖 |
For Tasks:
Click tags to check more tools for each tasksFor Jobs:
Alternative AI tools for ai00_server
Similar Open Source Tools
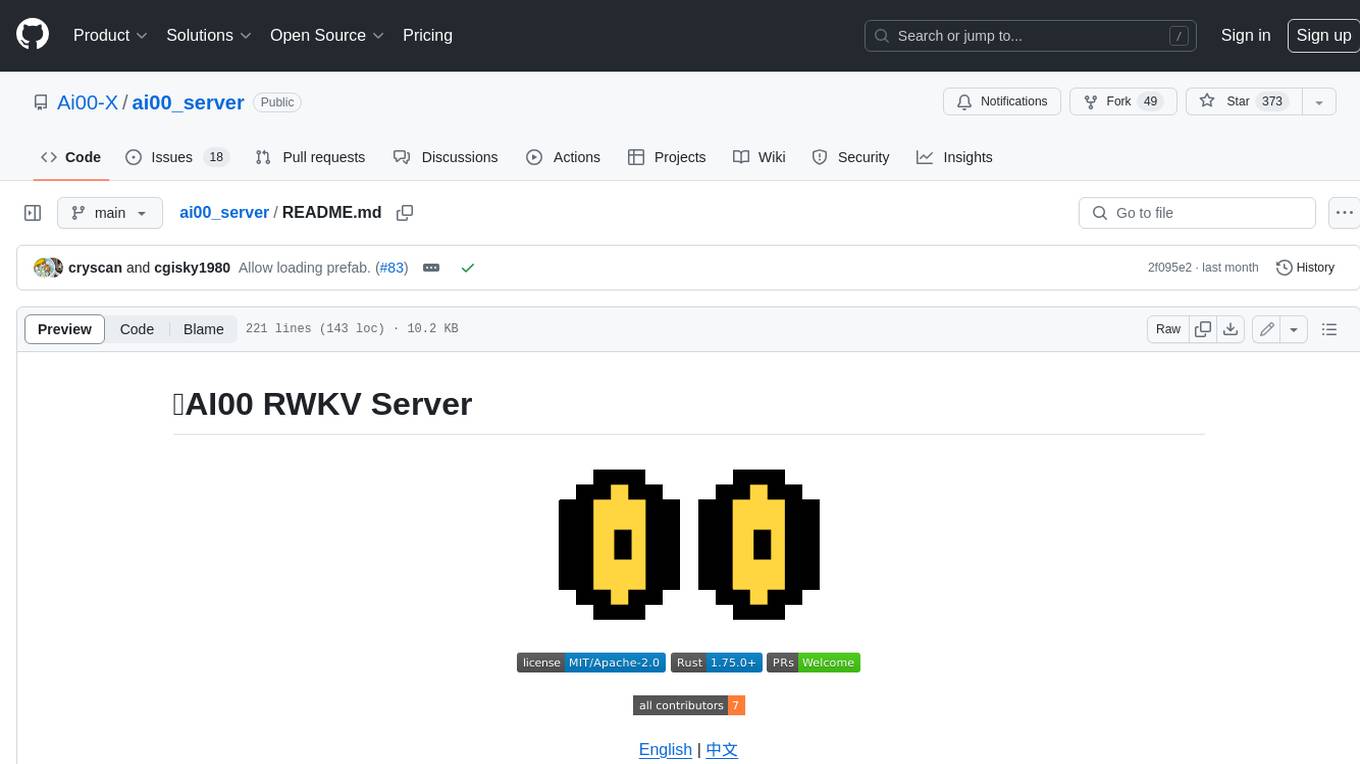
ai00_server
AI00 RWKV Server is an inference API server for the RWKV language model based upon the web-rwkv inference engine. It supports VULKAN parallel and concurrent batched inference and can run on all GPUs that support VULKAN. No need for Nvidia cards!!! AMD cards and even integrated graphics can be accelerated!!! No need for bulky pytorch, CUDA and other runtime environments, it's compact and ready to use out of the box! Compatible with OpenAI's ChatGPT API interface. 100% open source and commercially usable, under the MIT license. If you are looking for a fast, efficient, and easy-to-use LLM API server, then AI00 RWKV Server is your best choice. It can be used for various tasks, including chatbots, text generation, translation, and Q&A.
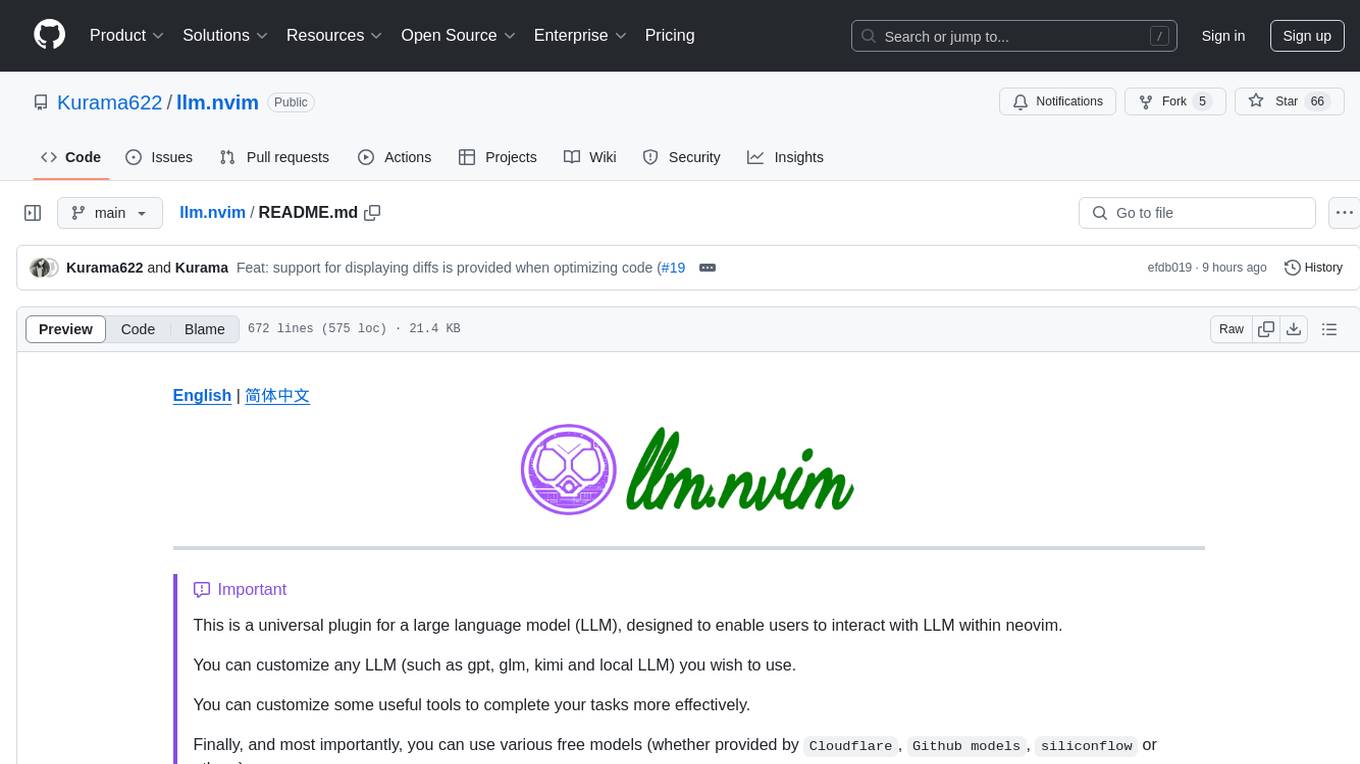
llm.nvim
llm.nvim is a universal plugin for a large language model (LLM) designed to enable users to interact with LLM within neovim. Users can customize various LLMs such as gpt, glm, kimi, and local LLM. The plugin provides tools for optimizing code, comparing code, translating text, and more. It also supports integration with free models from Cloudflare, Github models, siliconflow, and others. Users can customize tools, chat with LLM, quickly translate text, and explain code snippets. The plugin offers a flexible window interface for easy interaction and customization.
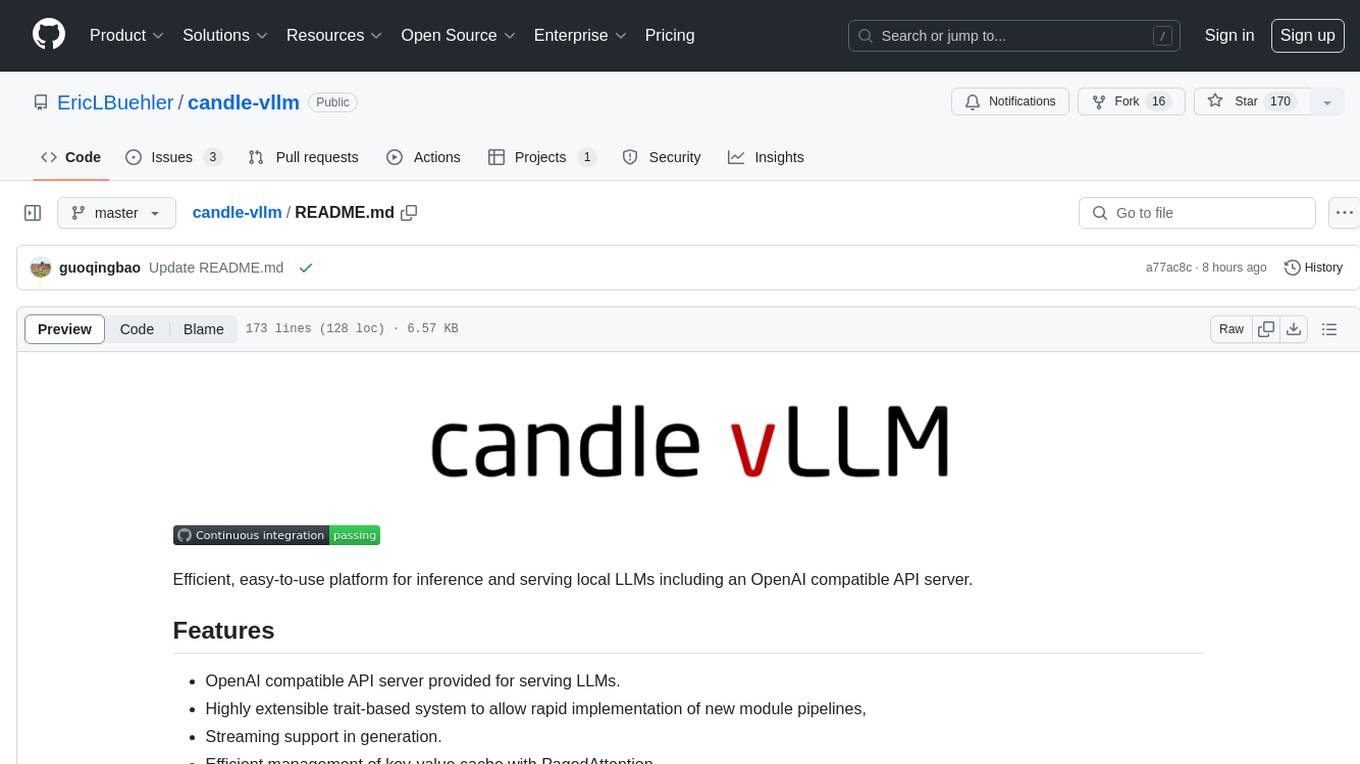
candle-vllm
Candle-vllm is an efficient and easy-to-use platform designed for inference and serving local LLMs, featuring an OpenAI compatible API server. It offers a highly extensible trait-based system for rapid implementation of new module pipelines, streaming support in generation, efficient management of key-value cache with PagedAttention, and continuous batching. The tool supports chat serving for various models and provides a seamless experience for users to interact with LLMs through different interfaces.
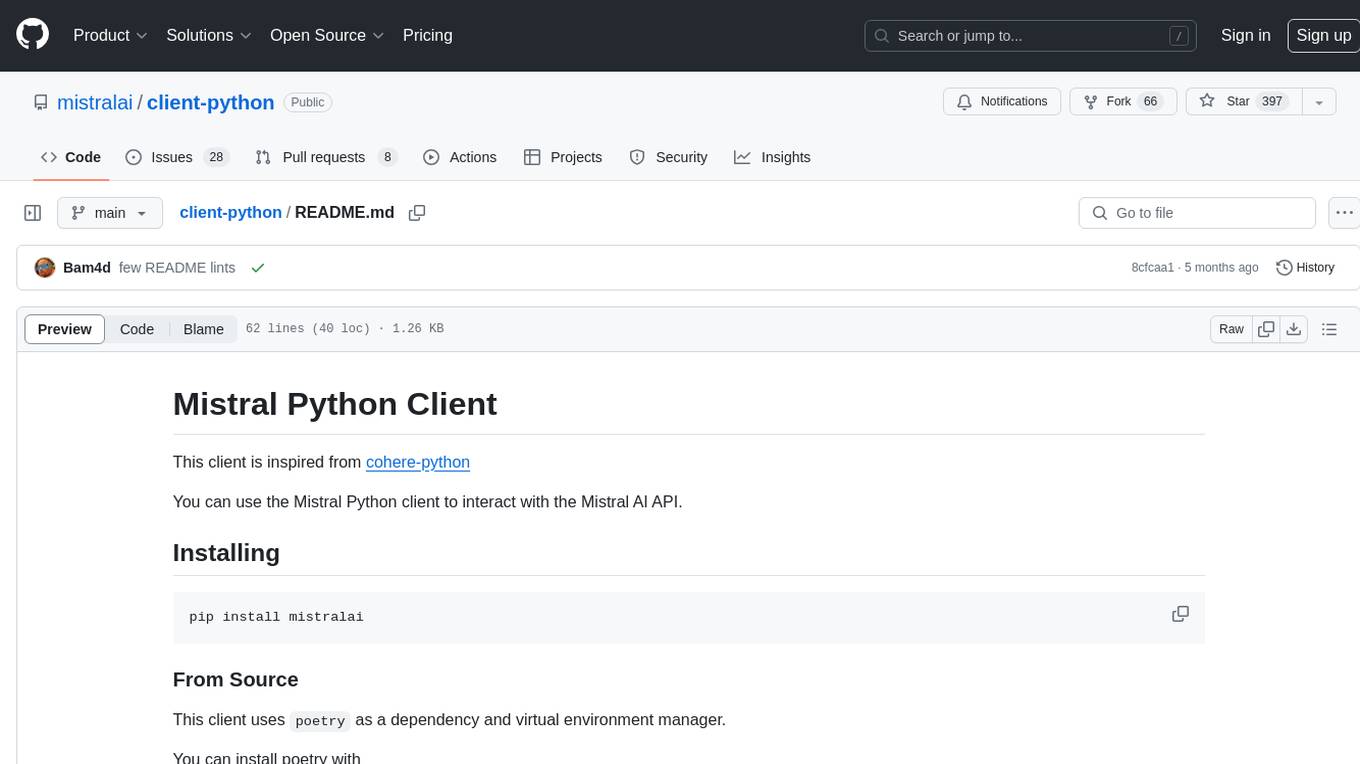
client-python
The Mistral Python Client is a tool inspired by cohere-python that allows users to interact with the Mistral AI API. It provides functionalities to access and utilize the AI capabilities offered by Mistral. Users can easily install the client using pip and manage dependencies using poetry. The client includes examples demonstrating how to use the API for various tasks, such as chat interactions. To get started, users need to obtain a Mistral API Key and set it as an environment variable. Overall, the Mistral Python Client simplifies the integration of Mistral AI services into Python applications.
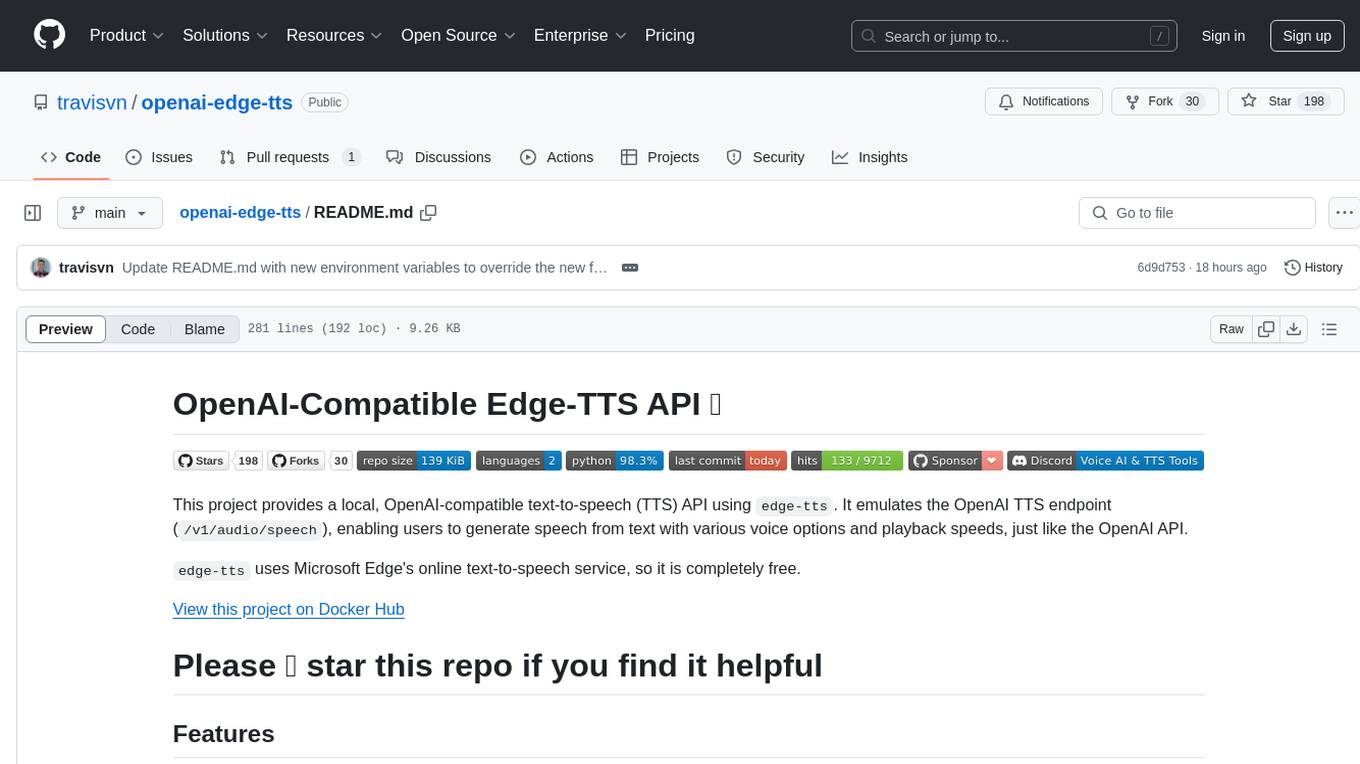
openai-edge-tts
This project provides a local, OpenAI-compatible text-to-speech (TTS) API using `edge-tts`. It emulates the OpenAI TTS endpoint (`/v1/audio/speech`), enabling users to generate speech from text with various voice options and playback speeds, just like the OpenAI API. `edge-tts` uses Microsoft Edge's online text-to-speech service, making it completely free. The project supports multiple audio formats, adjustable playback speed, and voice selection options, providing a flexible and customizable TTS solution for users.
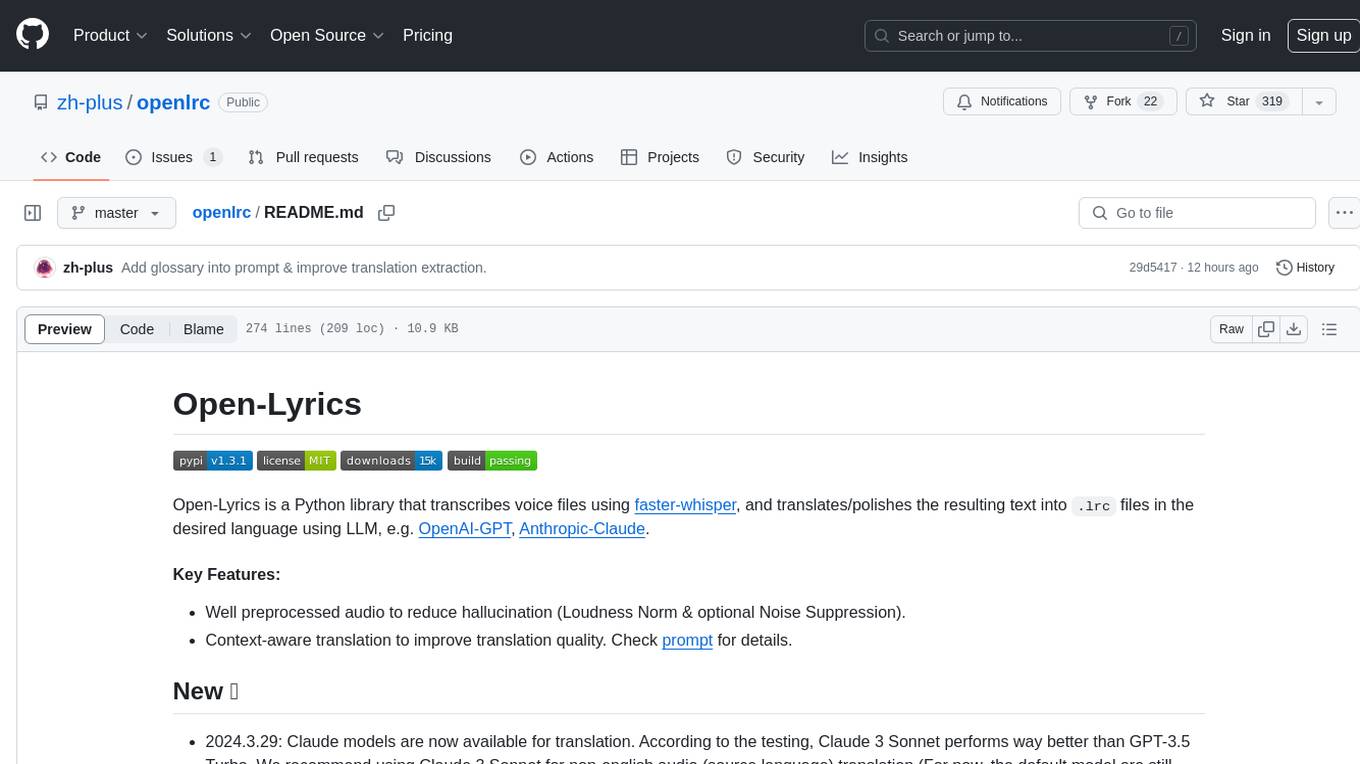
openlrc
Open-Lyrics is a Python library that transcribes voice files using faster-whisper and translates/polishes the resulting text into `.lrc` files in the desired language using LLM, e.g. OpenAI-GPT, Anthropic-Claude. It offers well preprocessed audio to reduce hallucination and context-aware translation to improve translation quality. Users can install the library from PyPI or GitHub and follow the installation steps to set up the environment. The tool supports GUI usage and provides Python code examples for transcription and translation tasks. It also includes features like utilizing context and glossary for translation enhancement, pricing information for different models, and a list of todo tasks for future improvements.
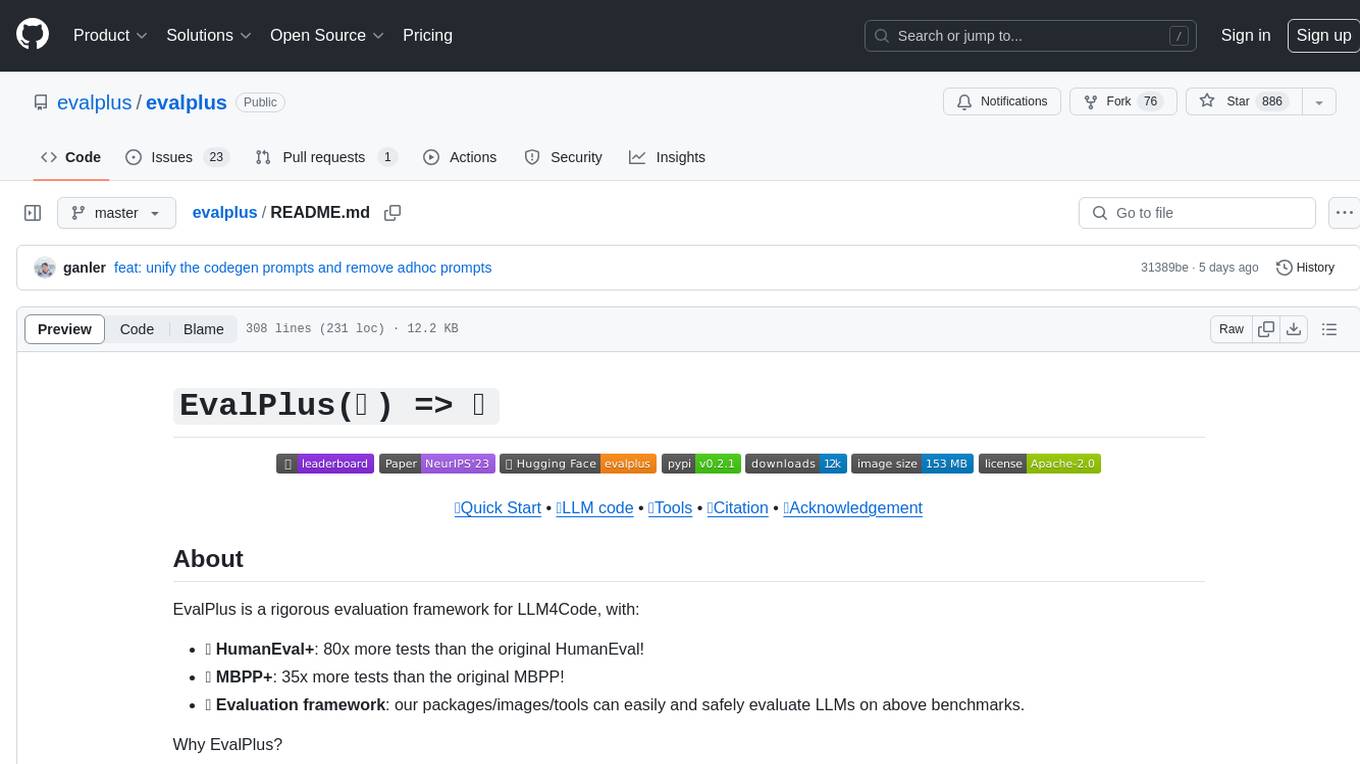
evalplus
EvalPlus is a rigorous evaluation framework for LLM4Code, providing HumanEval+ and MBPP+ tests to evaluate large language models on code generation tasks. It offers precise evaluation and ranking, coding rigorousness analysis, and pre-generated code samples. Users can use EvalPlus to generate code solutions, post-process code, and evaluate code quality. The tool includes tools for code generation and test input generation using various backends.
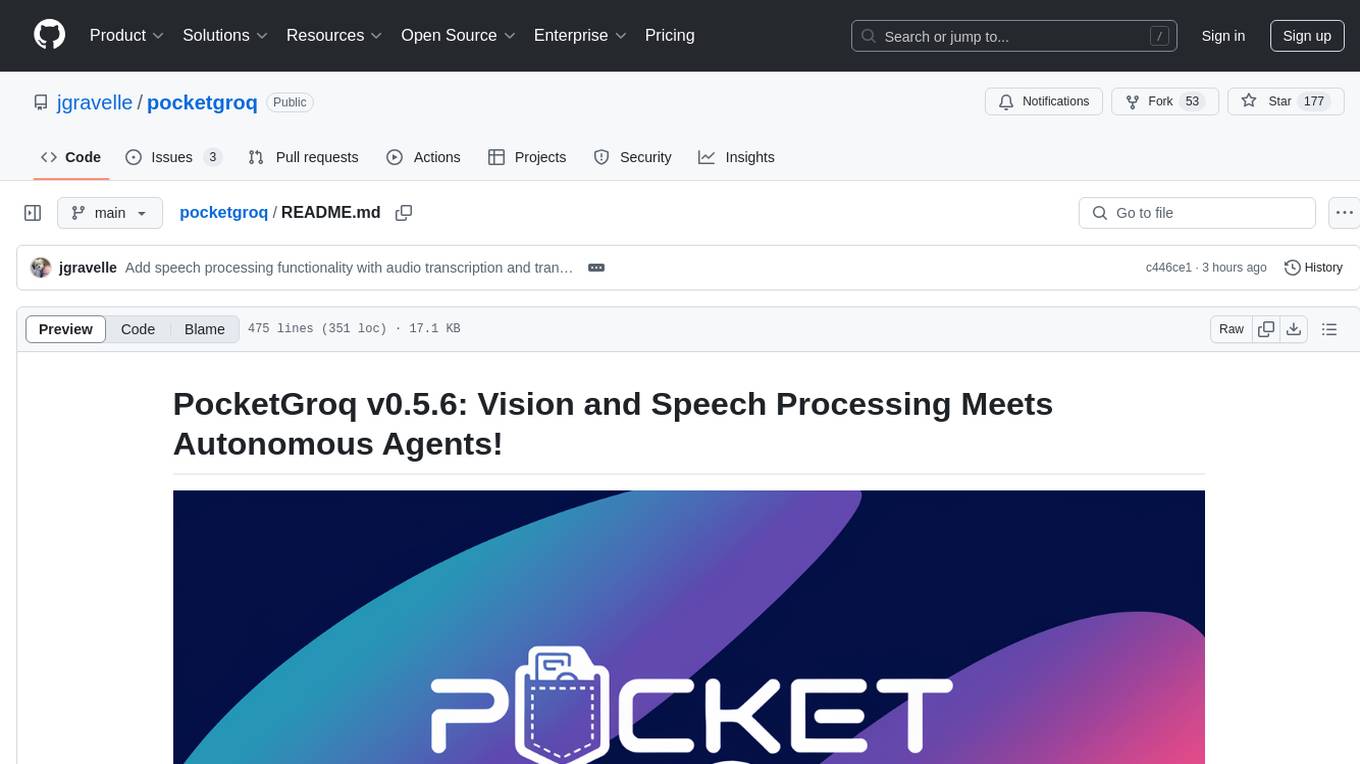
pocketgroq
PocketGroq is a tool that provides advanced functionalities for text generation, web scraping, web search, and AI response evaluation. It includes features like an Autonomous Agent for answering questions, web crawling and scraping capabilities, enhanced web search functionality, and flexible integration with Ollama server. Users can customize the agent's behavior, evaluate responses using AI, and utilize various methods for text generation, conversation management, and Chain of Thought reasoning. The tool offers comprehensive methods for different tasks, such as initializing RAG, error handling, and tool management. PocketGroq is designed to enhance development processes and enable the creation of AI-powered applications with ease.
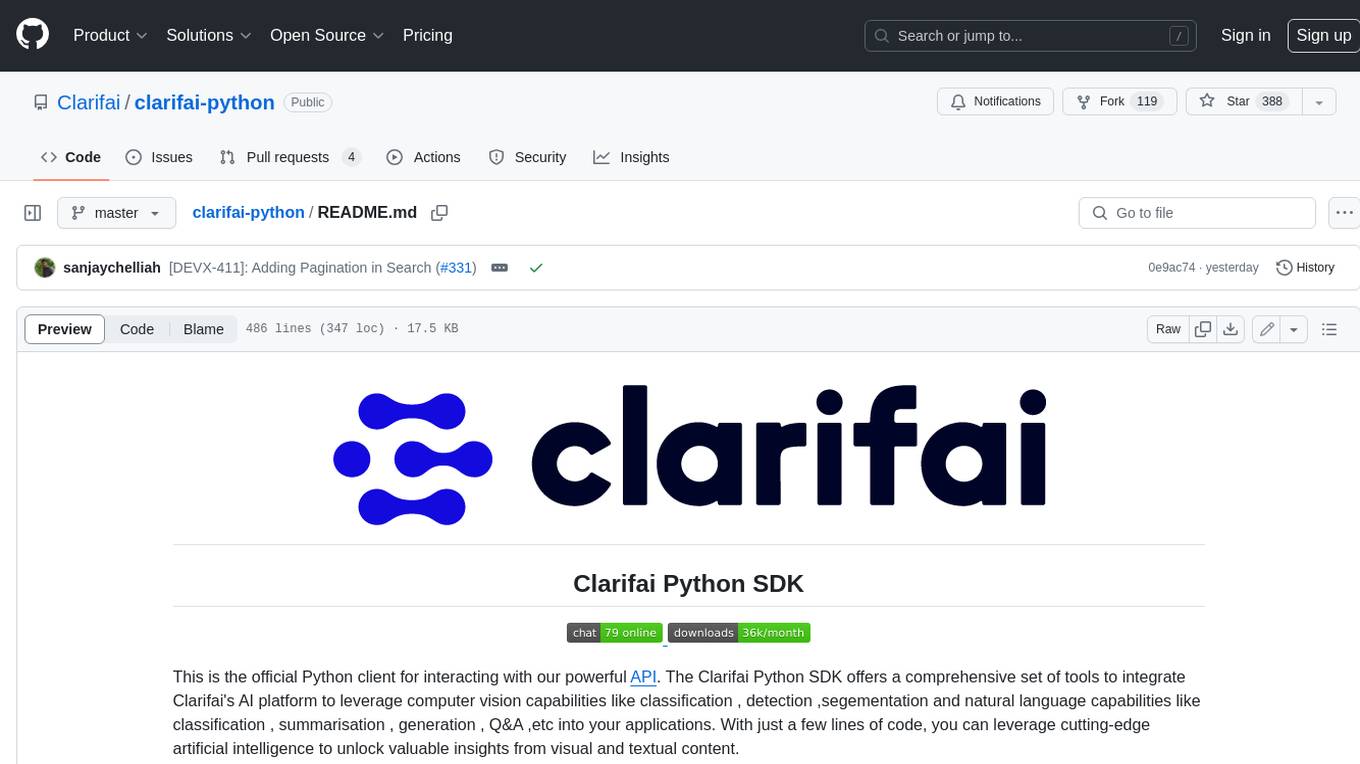
clarifai-python
The Clarifai Python SDK offers a comprehensive set of tools to integrate Clarifai's AI platform to leverage computer vision capabilities like classification , detection ,segementation and natural language capabilities like classification , summarisation , generation , Q&A ,etc into your applications. With just a few lines of code, you can leverage cutting-edge artificial intelligence to unlock valuable insights from visual and textual content.
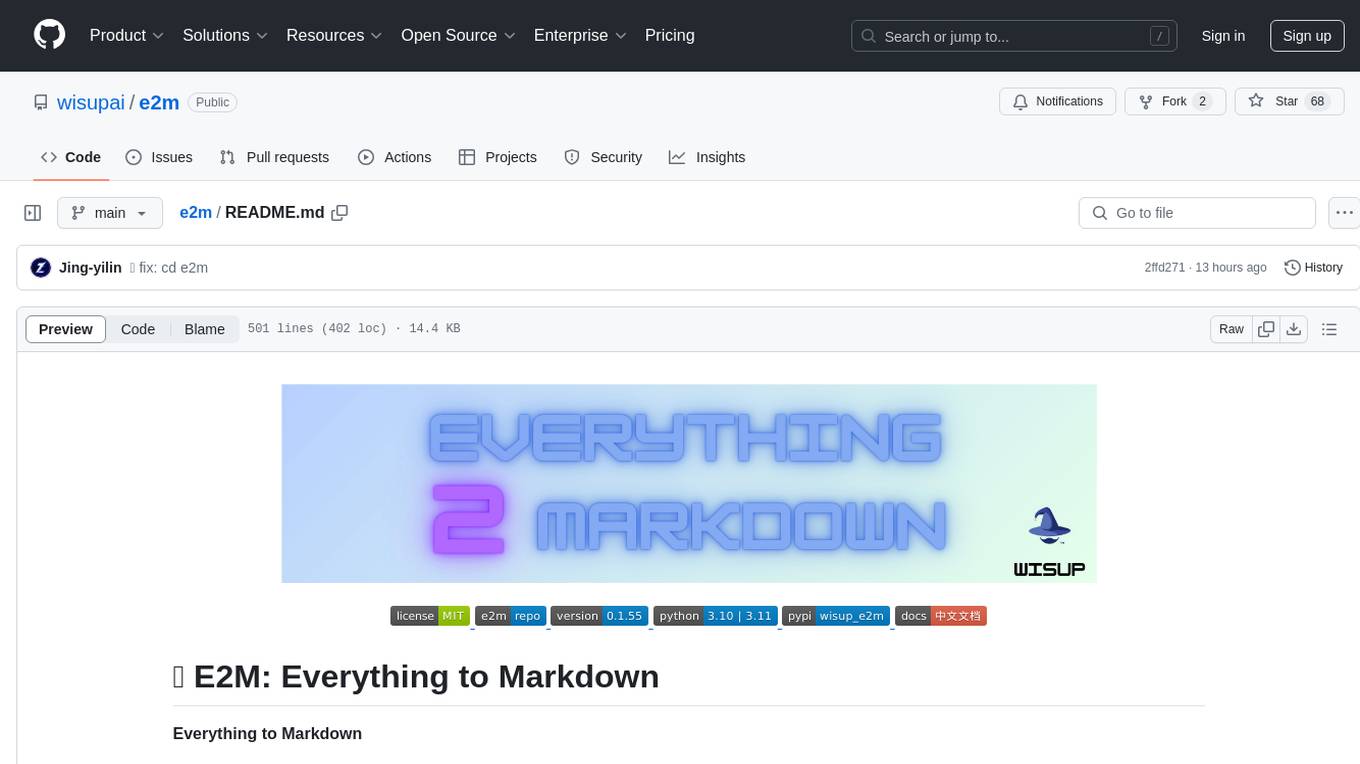
e2m
E2M is a Python library that can parse and convert various file types into Markdown format. It supports the conversion of multiple file formats, including doc, docx, epub, html, htm, url, pdf, ppt, pptx, mp3, and m4a. The ultimate goal of the E2M project is to provide high-quality data for Retrieval-Augmented Generation (RAG) and model training or fine-tuning. The core architecture consists of a Parser responsible for parsing various file types into text or image data, and a Converter responsible for converting text or image data into Markdown format.
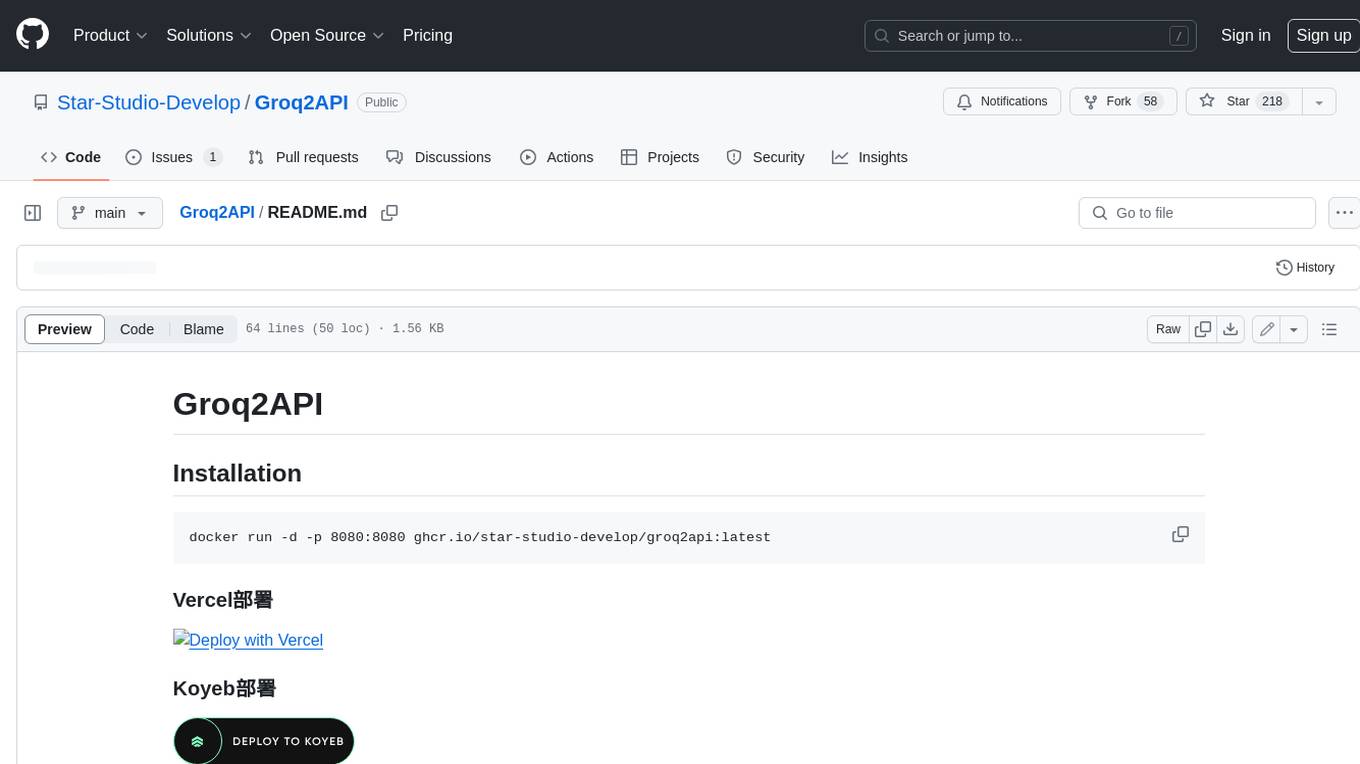
Groq2API
Groq2API is a REST API wrapper around the Groq2 model, a large language model trained by Google. The API allows you to send text prompts to the model and receive generated text responses. The API is easy to use and can be integrated into a variety of applications.
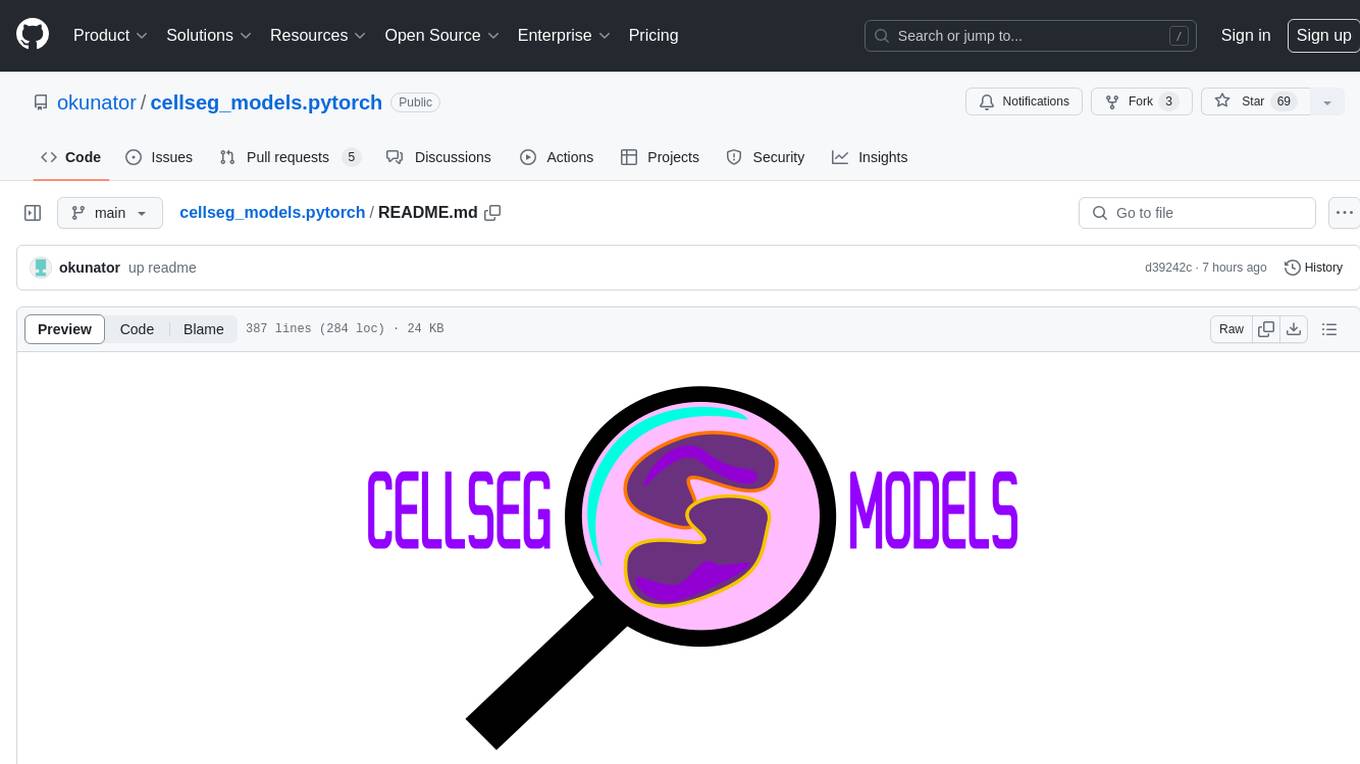
cellseg_models.pytorch
cellseg-models.pytorch is a Python library built upon PyTorch for 2D cell/nuclei instance segmentation models. It provides multi-task encoder-decoder architectures and post-processing methods for segmenting cell/nuclei instances. The library offers high-level API to define segmentation models, open-source datasets for training, flexibility to modify model components, sliding window inference, multi-GPU inference, benchmarking utilities, regularization techniques, and example notebooks for training and finetuning models with different backbones.
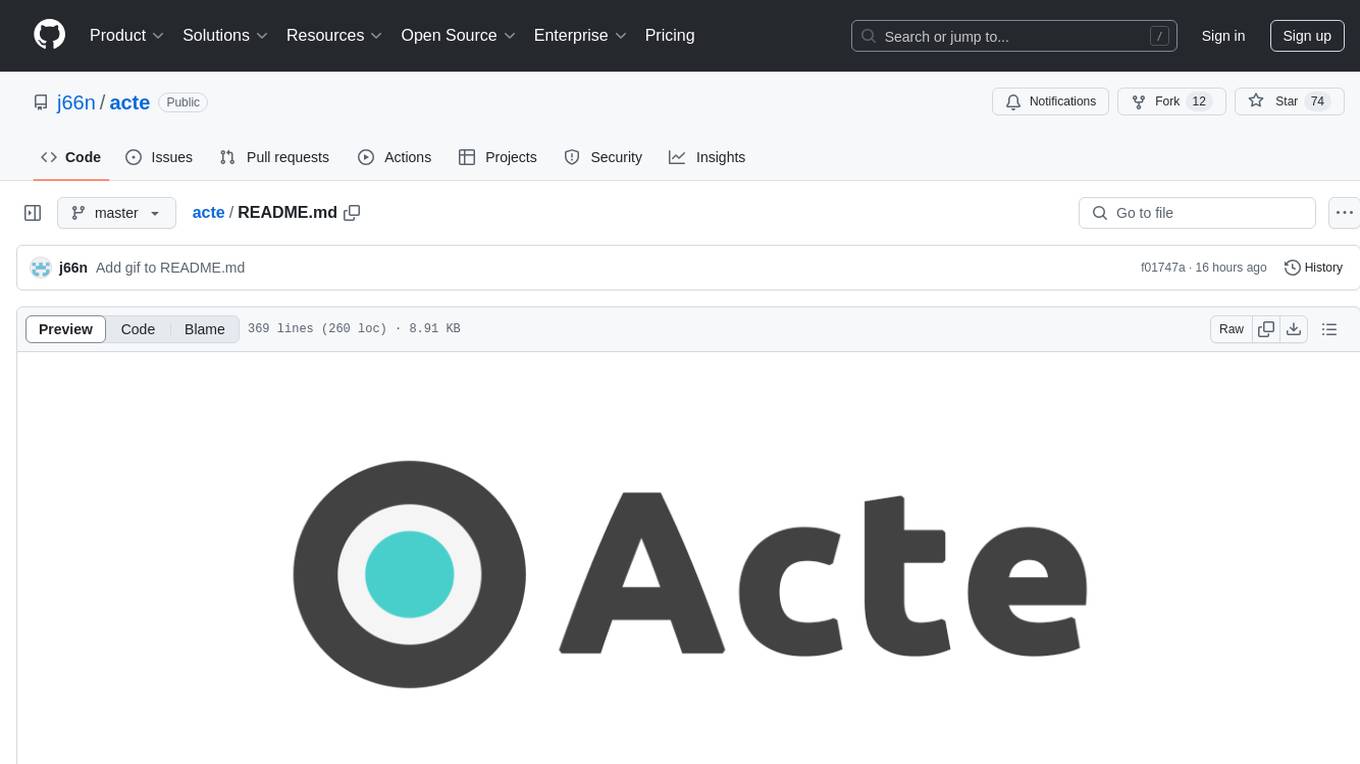
acte
Acte is a framework designed to build GUI-like tools for AI Agents. It aims to address the issues of cognitive load and freedom degrees when interacting with multiple APIs in complex scenarios. By providing a graphical user interface (GUI) for Agents, Acte helps reduce cognitive load and constraints interaction, similar to how humans interact with computers through GUIs. The tool offers APIs for starting new sessions, executing actions, and displaying screens, accessible via HTTP requests or the SessionManager class.
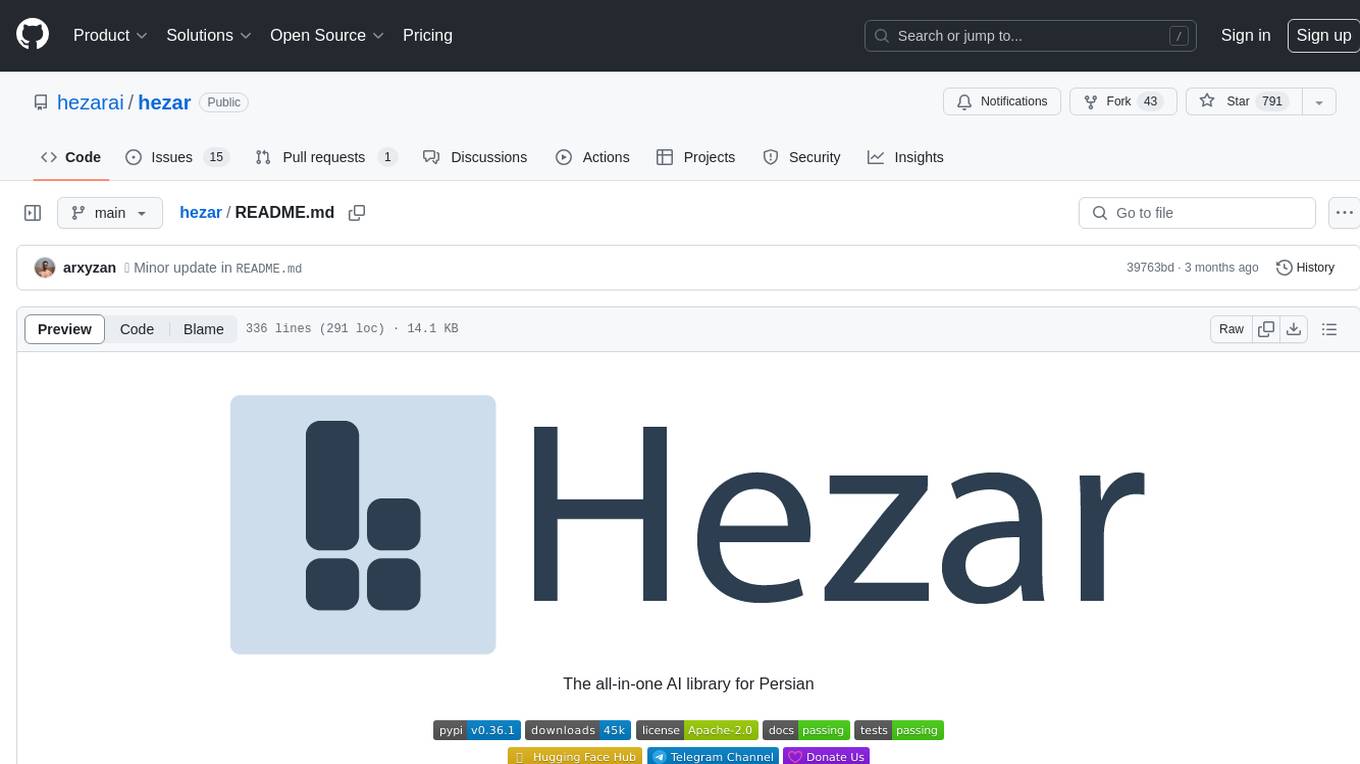
hezar
Hezar is an all-in-one AI library designed specifically for the Persian community. It brings together various AI models and tools, making it easy to use AI with just a few lines of code. The library seamlessly integrates with Hugging Face Hub, offering a developer-friendly interface and task-based model interface. In addition to models, Hezar provides tools like word embeddings, tokenizers, feature extractors, and more. It also includes supplementary ML tools for deployment, benchmarking, and optimization.
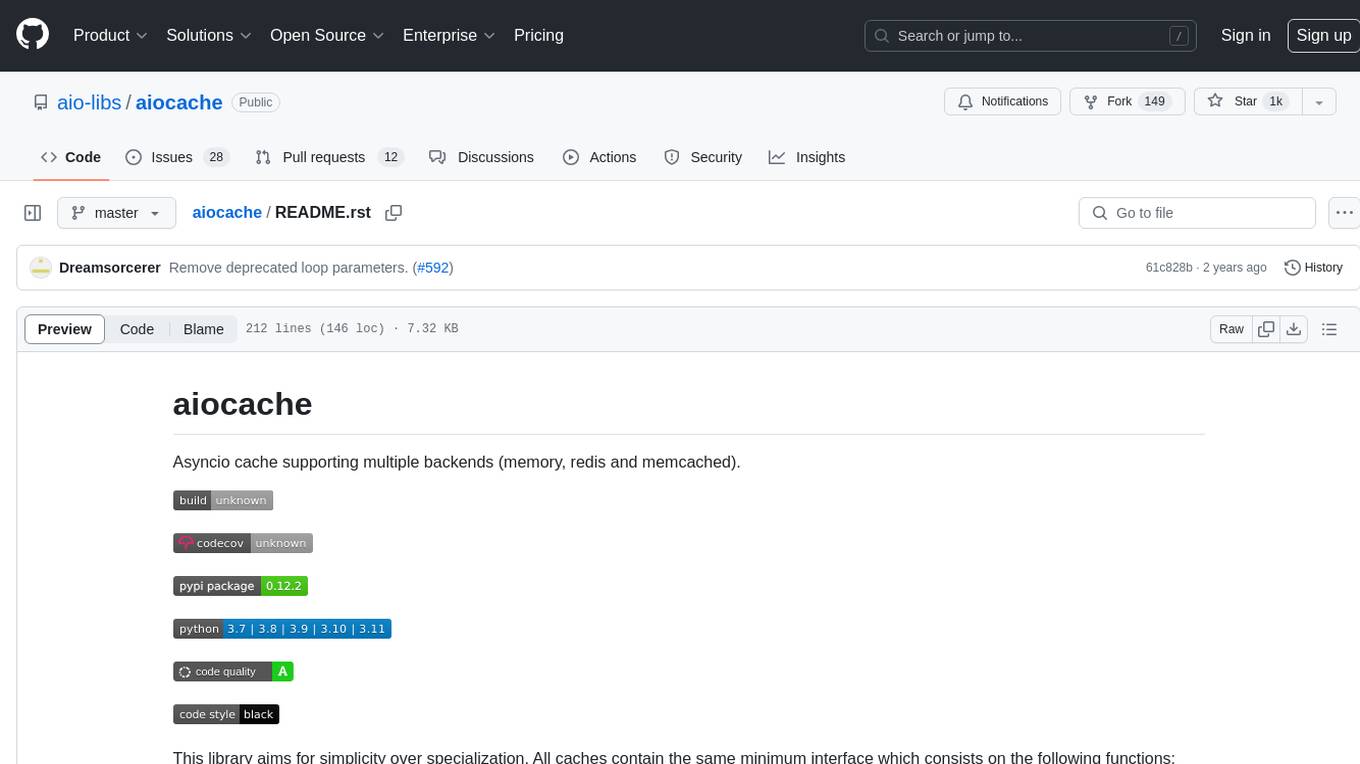
aiocache
Aiocache is an asyncio cache library that supports multiple backends such as memory, redis, and memcached. It provides a simple interface for functions like add, get, set, multi_get, multi_set, exists, increment, delete, clear, and raw. Users can easily install and use the library for caching data in Python applications. Aiocache allows for easy instantiation of caches and setup of cache aliases for reusing configurations. It also provides support for backends, serializers, and plugins to customize cache operations. The library offers detailed documentation and examples for different use cases and configurations.
For similar tasks
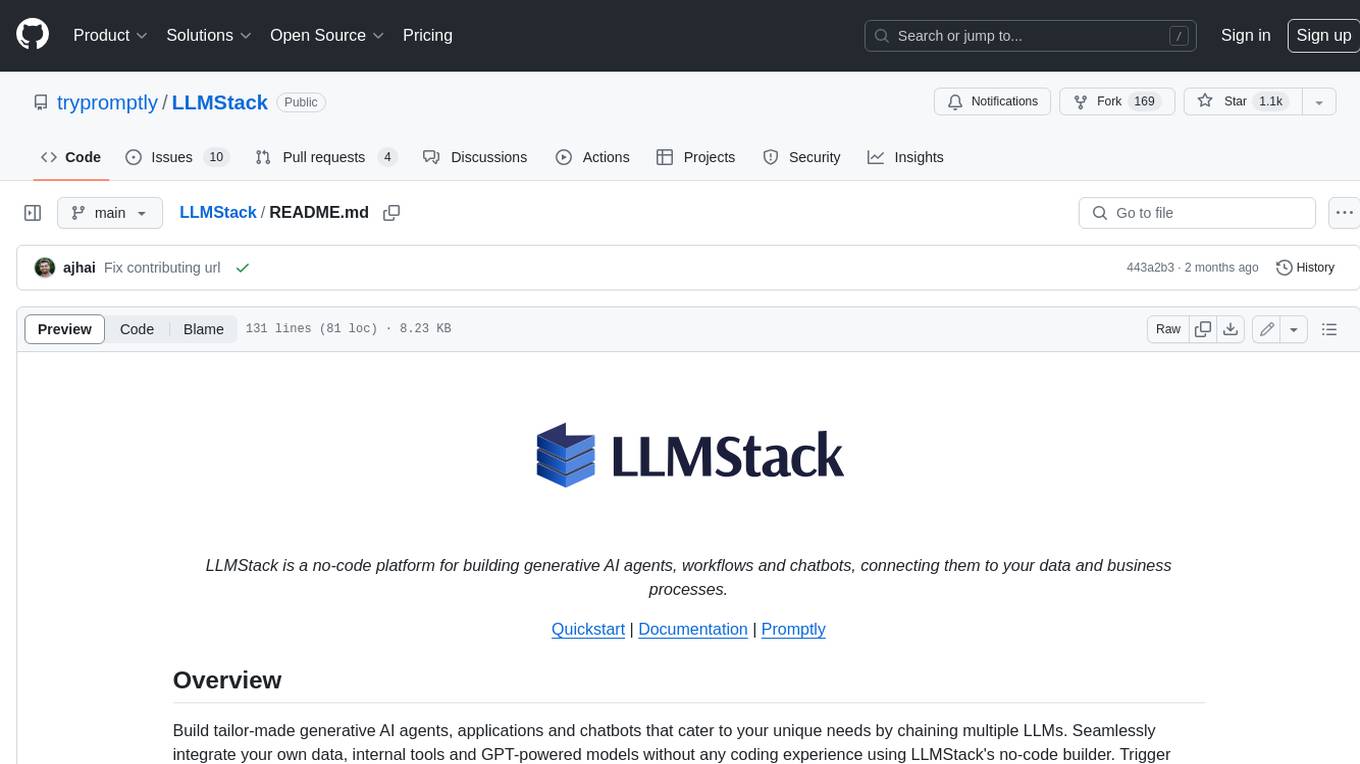
LLMStack
LLMStack is a no-code platform for building generative AI agents, workflows, and chatbots. It allows users to connect their own data, internal tools, and GPT-powered models without any coding experience. LLMStack can be deployed to the cloud or on-premise and can be accessed via HTTP API or triggered from Slack or Discord.
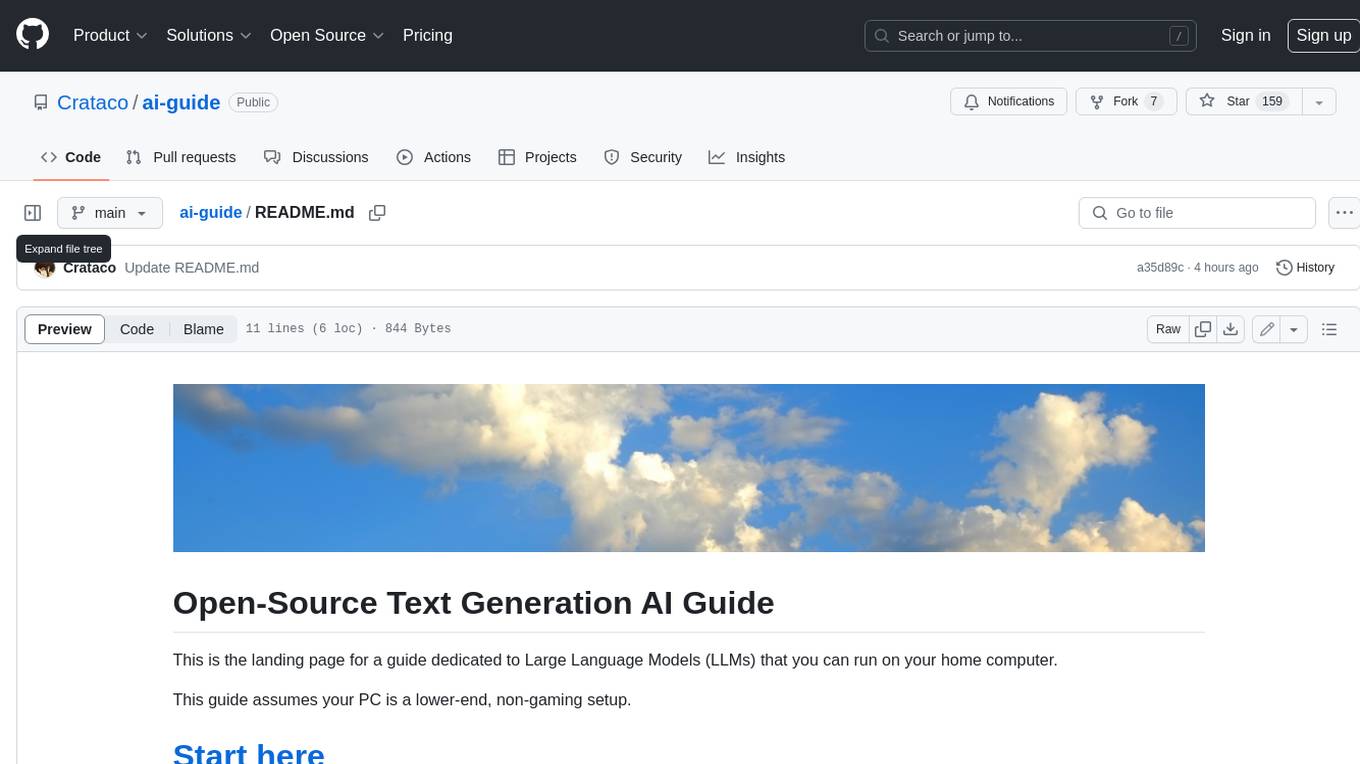
ai-guide
This guide is dedicated to Large Language Models (LLMs) that you can run on your home computer. It assumes your PC is a lower-end, non-gaming setup.
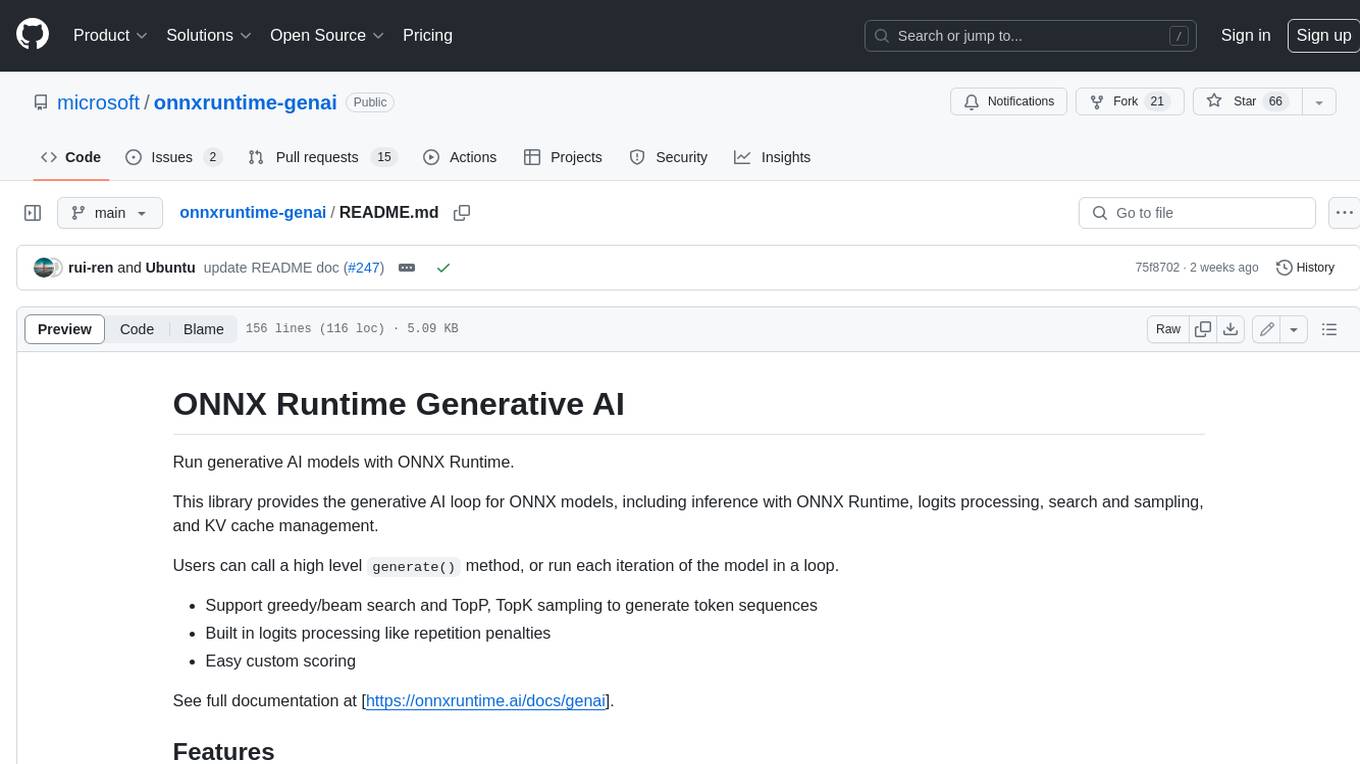
onnxruntime-genai
ONNX Runtime Generative AI is a library that provides the generative AI loop for ONNX models, including inference with ONNX Runtime, logits processing, search and sampling, and KV cache management. Users can call a high level `generate()` method, or run each iteration of the model in a loop. It supports greedy/beam search and TopP, TopK sampling to generate token sequences, has built in logits processing like repetition penalties, and allows for easy custom scoring.
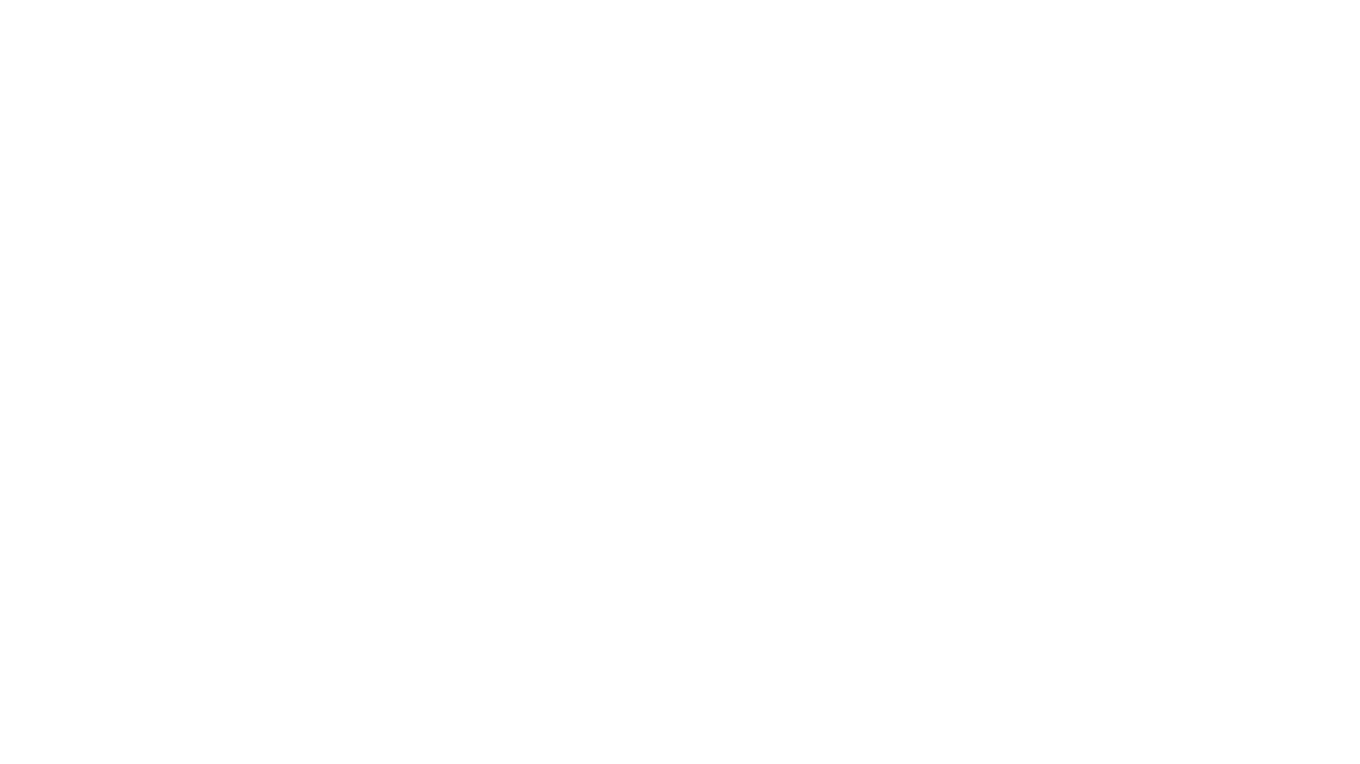
jupyter-ai
Jupyter AI connects generative AI with Jupyter notebooks. It provides a user-friendly and powerful way to explore generative AI models in notebooks and improve your productivity in JupyterLab and the Jupyter Notebook. Specifically, Jupyter AI offers: * An `%%ai` magic that turns the Jupyter notebook into a reproducible generative AI playground. This works anywhere the IPython kernel runs (JupyterLab, Jupyter Notebook, Google Colab, Kaggle, VSCode, etc.). * A native chat UI in JupyterLab that enables you to work with generative AI as a conversational assistant. * Support for a wide range of generative model providers, including AI21, Anthropic, AWS, Cohere, Gemini, Hugging Face, NVIDIA, and OpenAI. * Local model support through GPT4All, enabling use of generative AI models on consumer grade machines with ease and privacy.
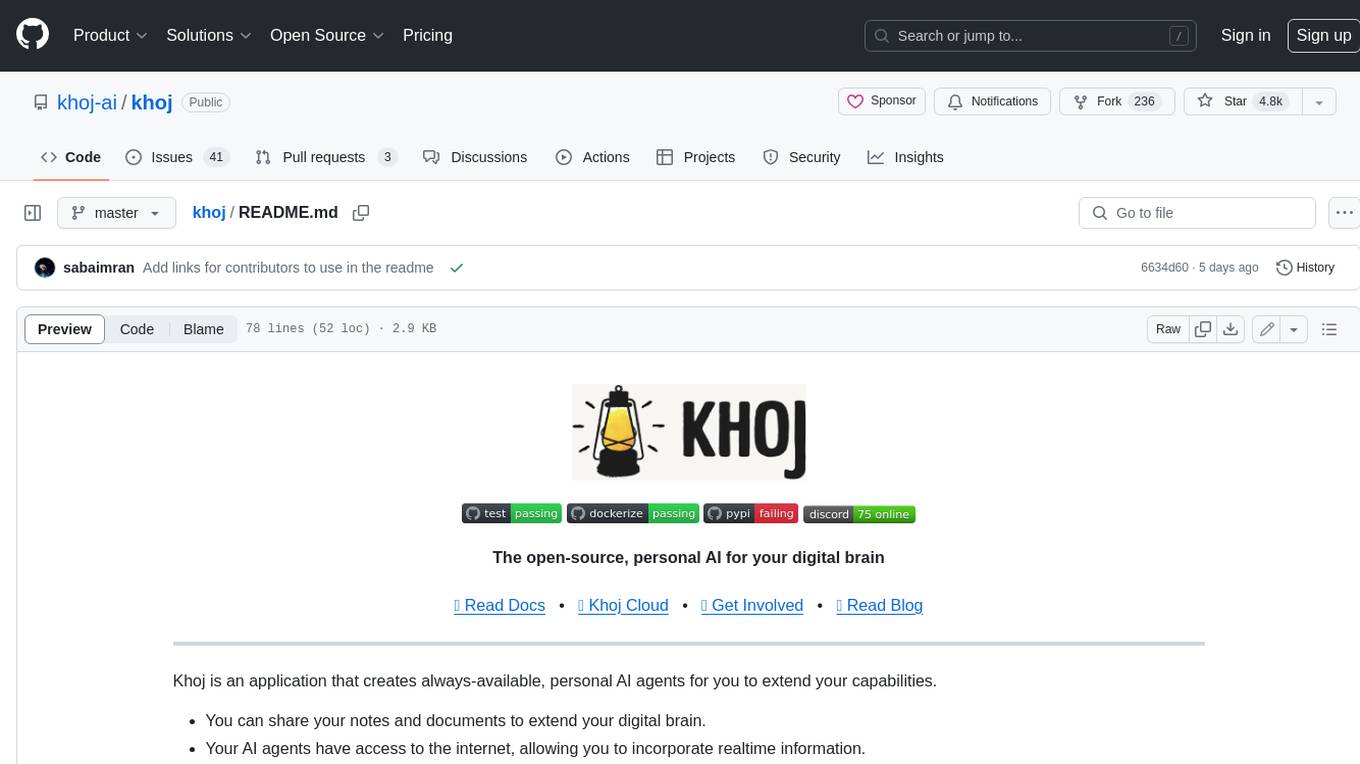
khoj
Khoj is an open-source, personal AI assistant that extends your capabilities by creating always-available AI agents. You can share your notes and documents to extend your digital brain, and your AI agents have access to the internet, allowing you to incorporate real-time information. Khoj is accessible on Desktop, Emacs, Obsidian, Web, and Whatsapp, and you can share PDF, markdown, org-mode, notion files, and GitHub repositories. You'll get fast, accurate semantic search on top of your docs, and your agents can create deeply personal images and understand your speech. Khoj is self-hostable and always will be.
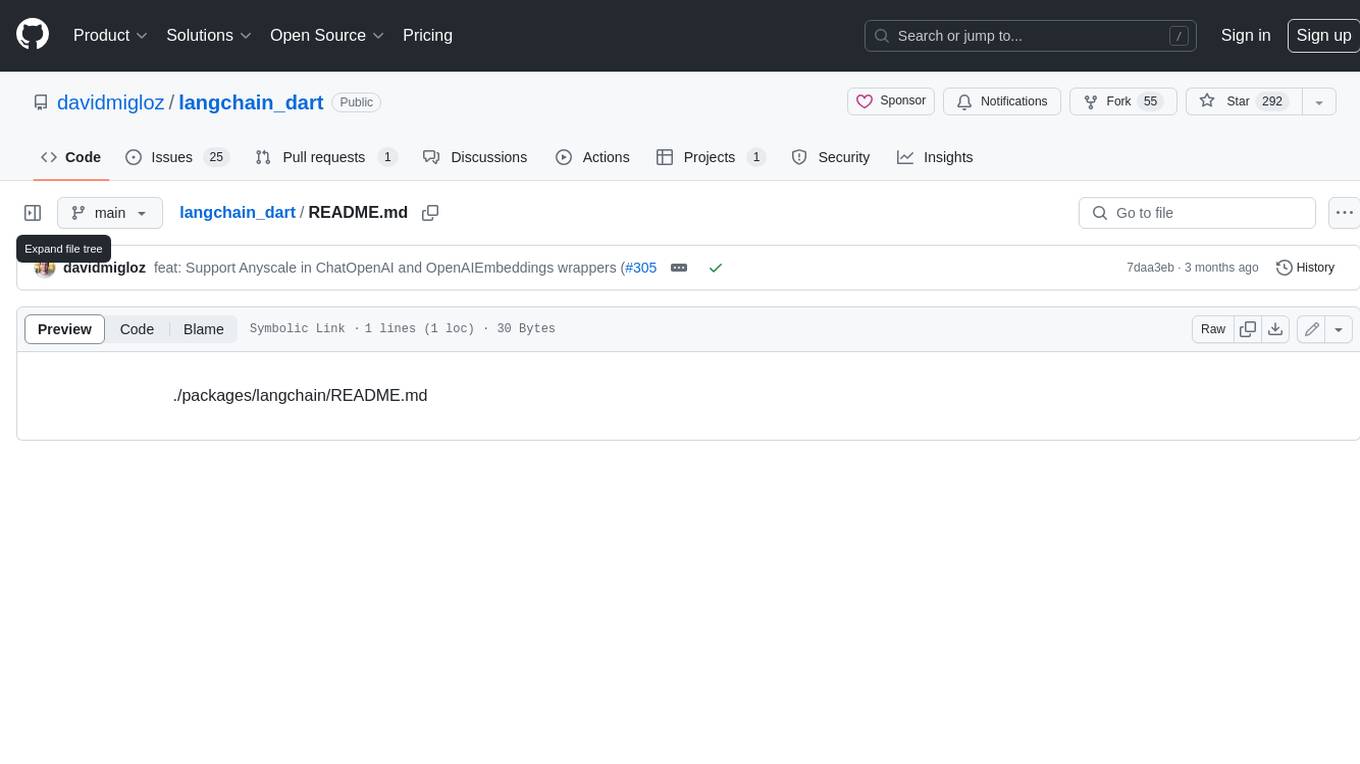
langchain_dart
LangChain.dart is a Dart port of the popular LangChain Python framework created by Harrison Chase. LangChain provides a set of ready-to-use components for working with language models and a standard interface for chaining them together to formulate more advanced use cases (e.g. chatbots, Q&A with RAG, agents, summarization, extraction, etc.). The components can be grouped into a few core modules: * **Model I/O:** LangChain offers a unified API for interacting with various LLM providers (e.g. OpenAI, Google, Mistral, Ollama, etc.), allowing developers to switch between them with ease. Additionally, it provides tools for managing model inputs (prompt templates and example selectors) and parsing the resulting model outputs (output parsers). * **Retrieval:** assists in loading user data (via document loaders), transforming it (with text splitters), extracting its meaning (using embedding models), storing (in vector stores) and retrieving it (through retrievers) so that it can be used to ground the model's responses (i.e. Retrieval-Augmented Generation or RAG). * **Agents:** "bots" that leverage LLMs to make informed decisions about which available tools (such as web search, calculators, database lookup, etc.) to use to accomplish the designated task. The different components can be composed together using the LangChain Expression Language (LCEL).
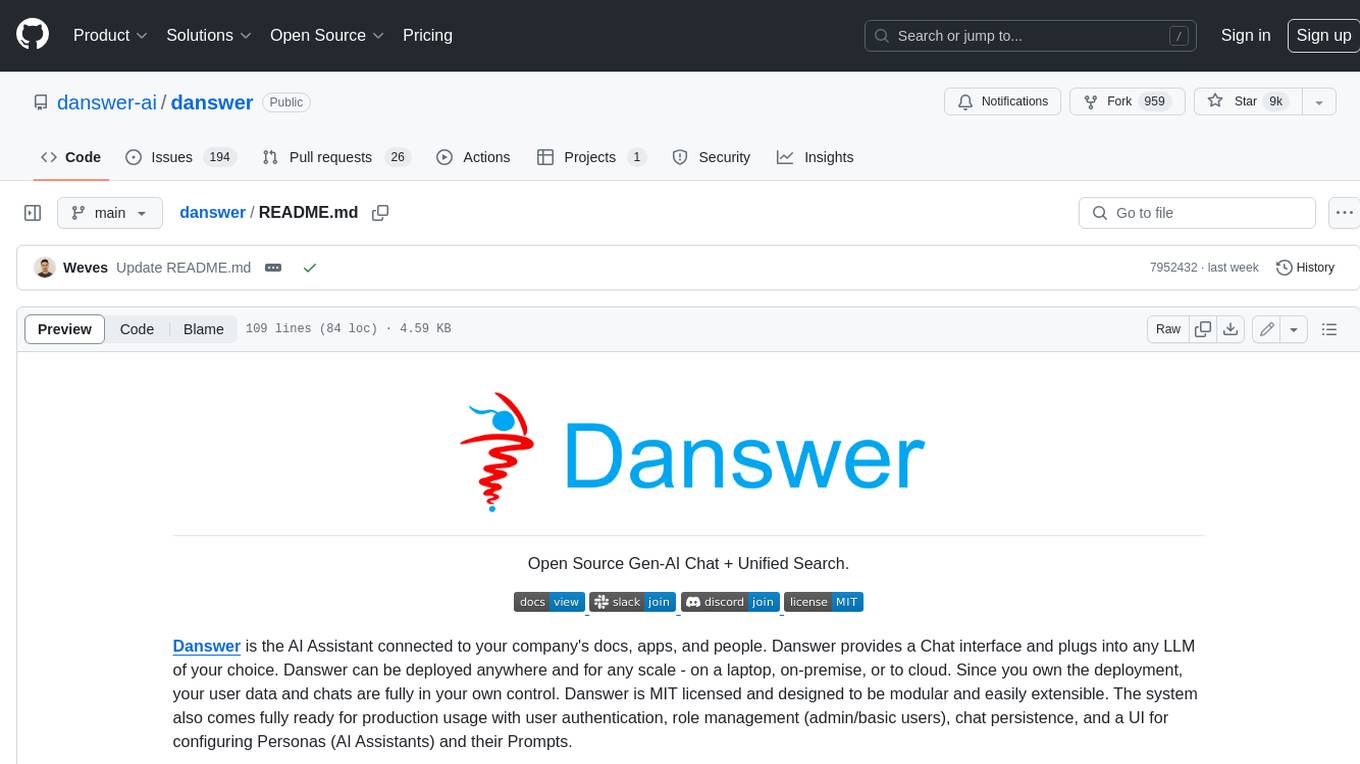
danswer
Danswer is an open-source Gen-AI Chat and Unified Search tool that connects to your company's docs, apps, and people. It provides a Chat interface and plugs into any LLM of your choice. Danswer can be deployed anywhere and for any scale - on a laptop, on-premise, or to cloud. Since you own the deployment, your user data and chats are fully in your own control. Danswer is MIT licensed and designed to be modular and easily extensible. The system also comes fully ready for production usage with user authentication, role management (admin/basic users), chat persistence, and a UI for configuring Personas (AI Assistants) and their Prompts. Danswer also serves as a Unified Search across all common workplace tools such as Slack, Google Drive, Confluence, etc. By combining LLMs and team specific knowledge, Danswer becomes a subject matter expert for the team. Imagine ChatGPT if it had access to your team's unique knowledge! It enables questions such as "A customer wants feature X, is this already supported?" or "Where's the pull request for feature Y?"
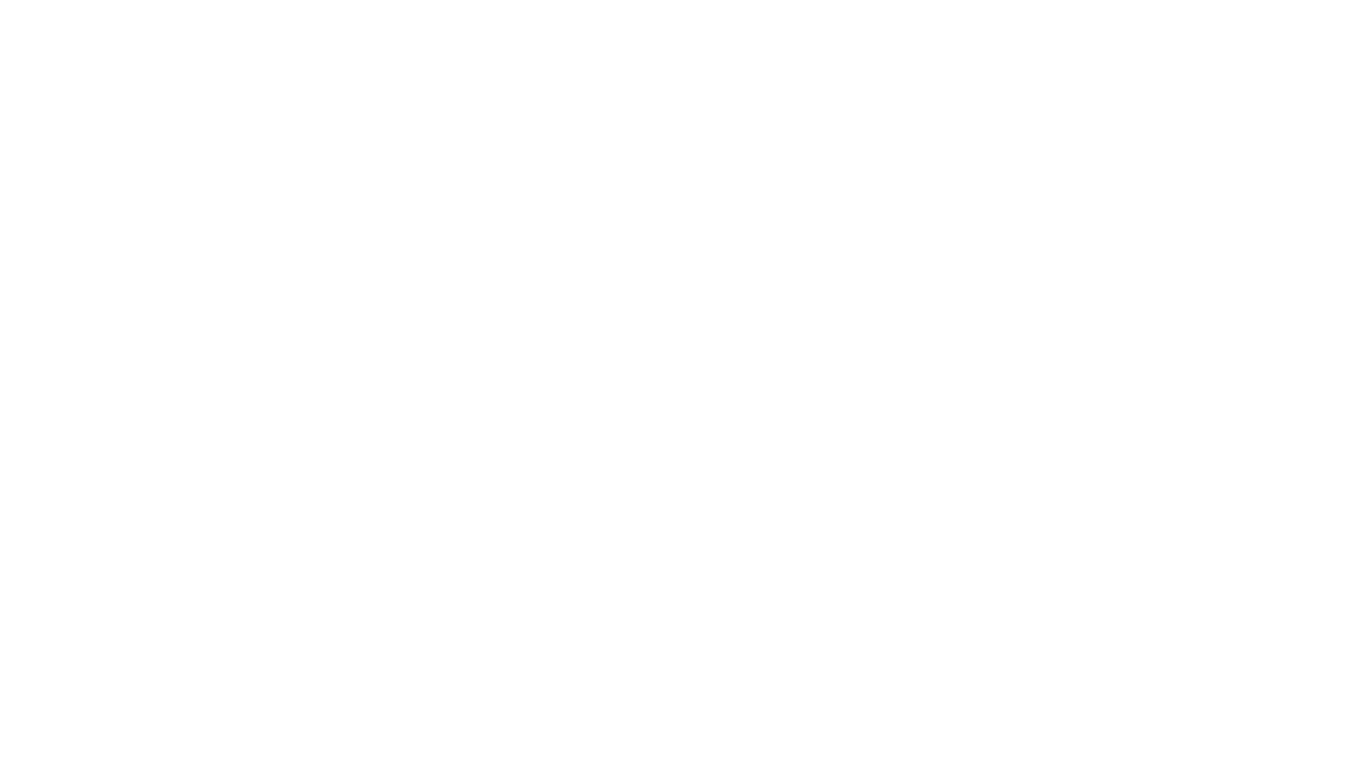
infinity
Infinity is an AI-native database designed for LLM applications, providing incredibly fast full-text and vector search capabilities. It supports a wide range of data types, including vectors, full-text, and structured data, and offers a fused search feature that combines multiple embeddings and full text. Infinity is easy to use, with an intuitive Python API and a single-binary architecture that simplifies deployment. It achieves high performance, with 0.1 milliseconds query latency on million-scale vector datasets and up to 15K QPS.
For similar jobs
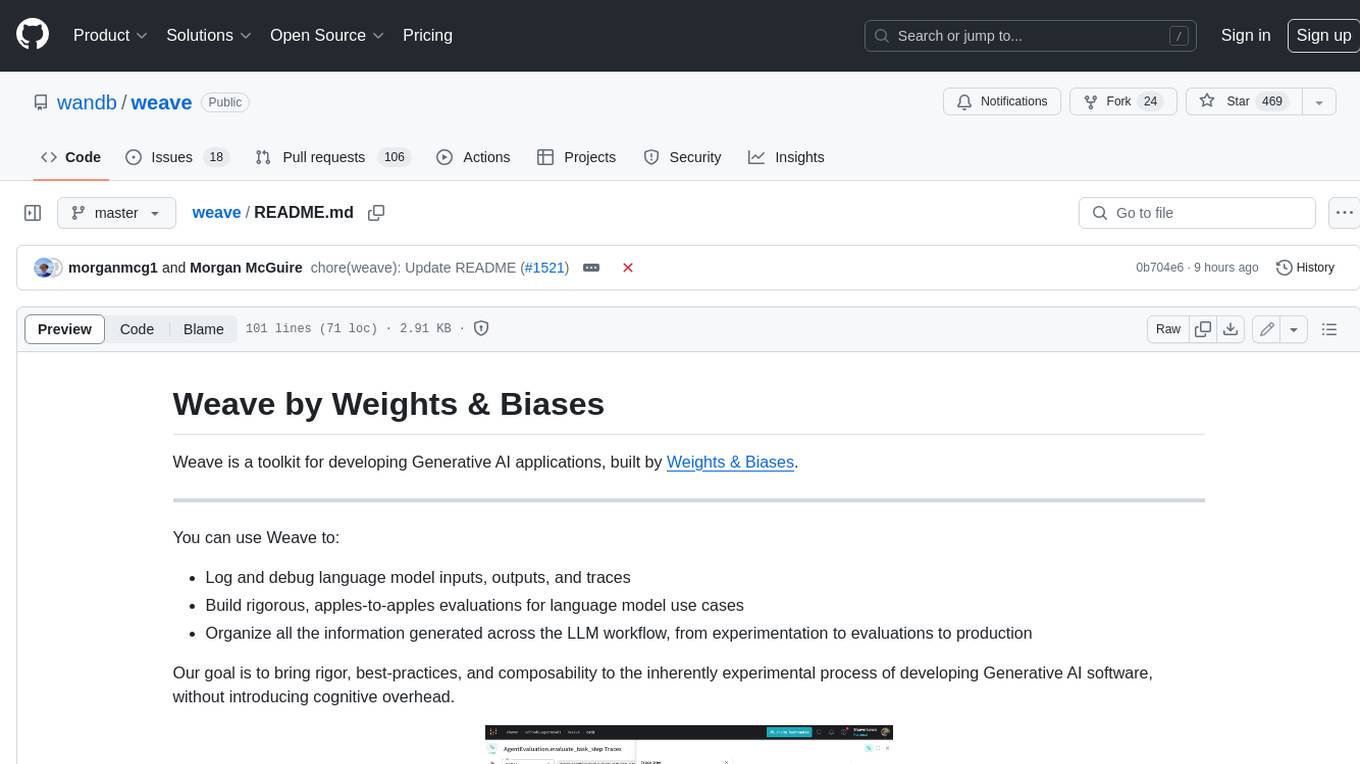
weave
Weave is a toolkit for developing Generative AI applications, built by Weights & Biases. With Weave, you can log and debug language model inputs, outputs, and traces; build rigorous, apples-to-apples evaluations for language model use cases; and organize all the information generated across the LLM workflow, from experimentation to evaluations to production. Weave aims to bring rigor, best-practices, and composability to the inherently experimental process of developing Generative AI software, without introducing cognitive overhead.
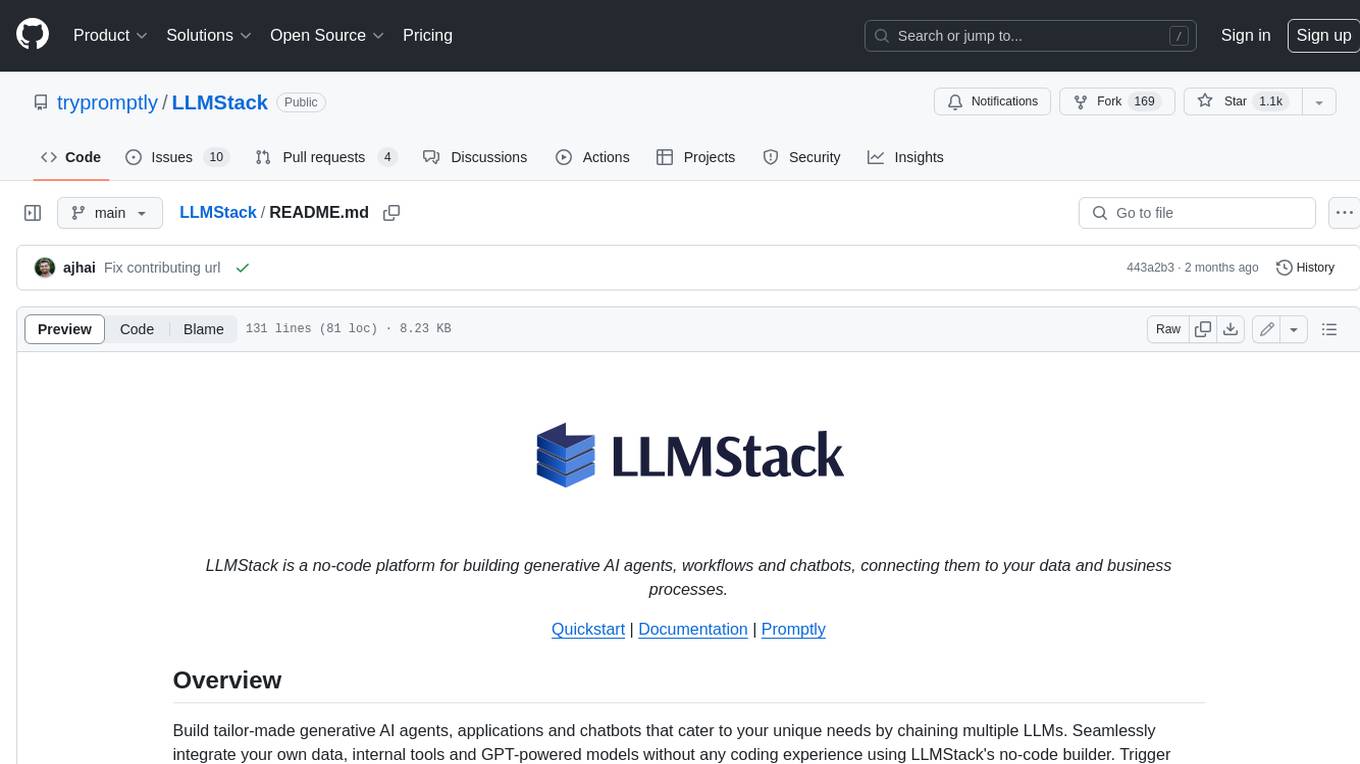
LLMStack
LLMStack is a no-code platform for building generative AI agents, workflows, and chatbots. It allows users to connect their own data, internal tools, and GPT-powered models without any coding experience. LLMStack can be deployed to the cloud or on-premise and can be accessed via HTTP API or triggered from Slack or Discord.
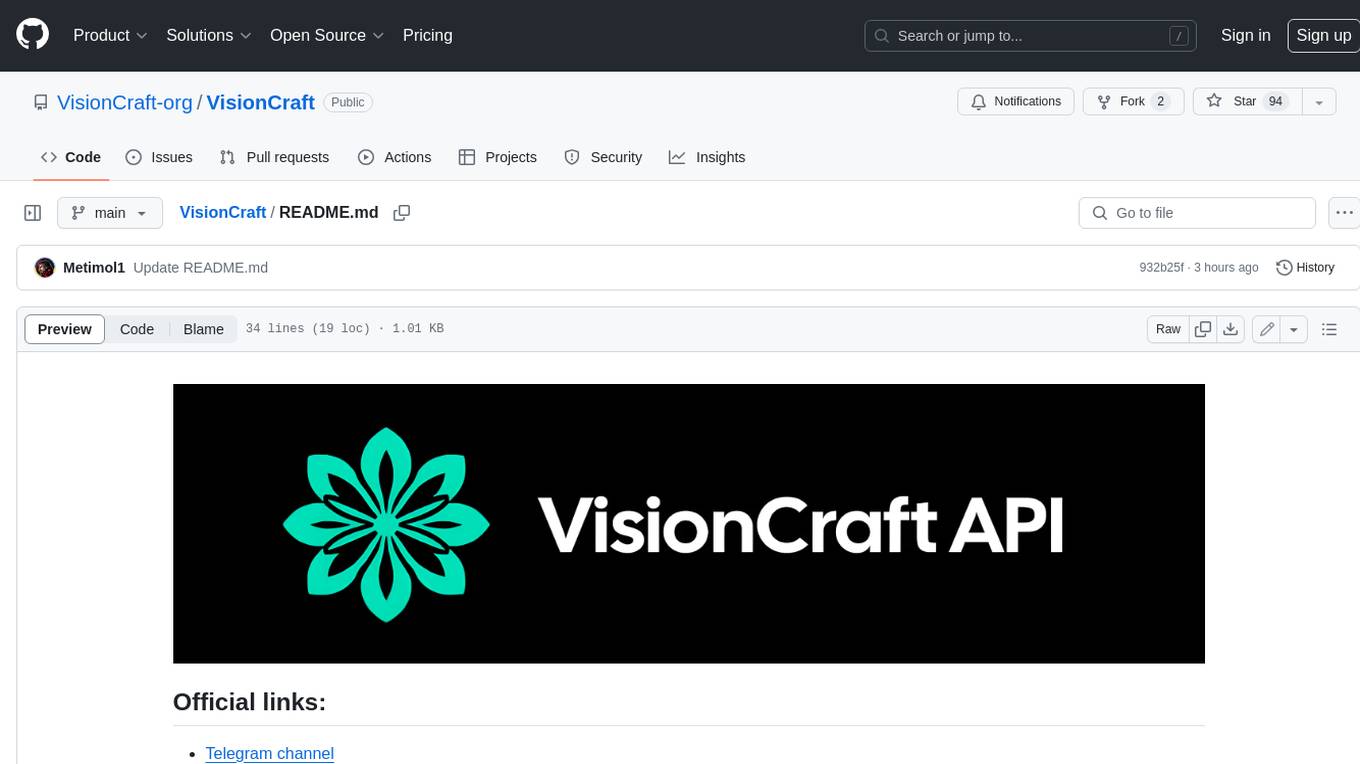
VisionCraft
The VisionCraft API is a free API for using over 100 different AI models. From images to sound.
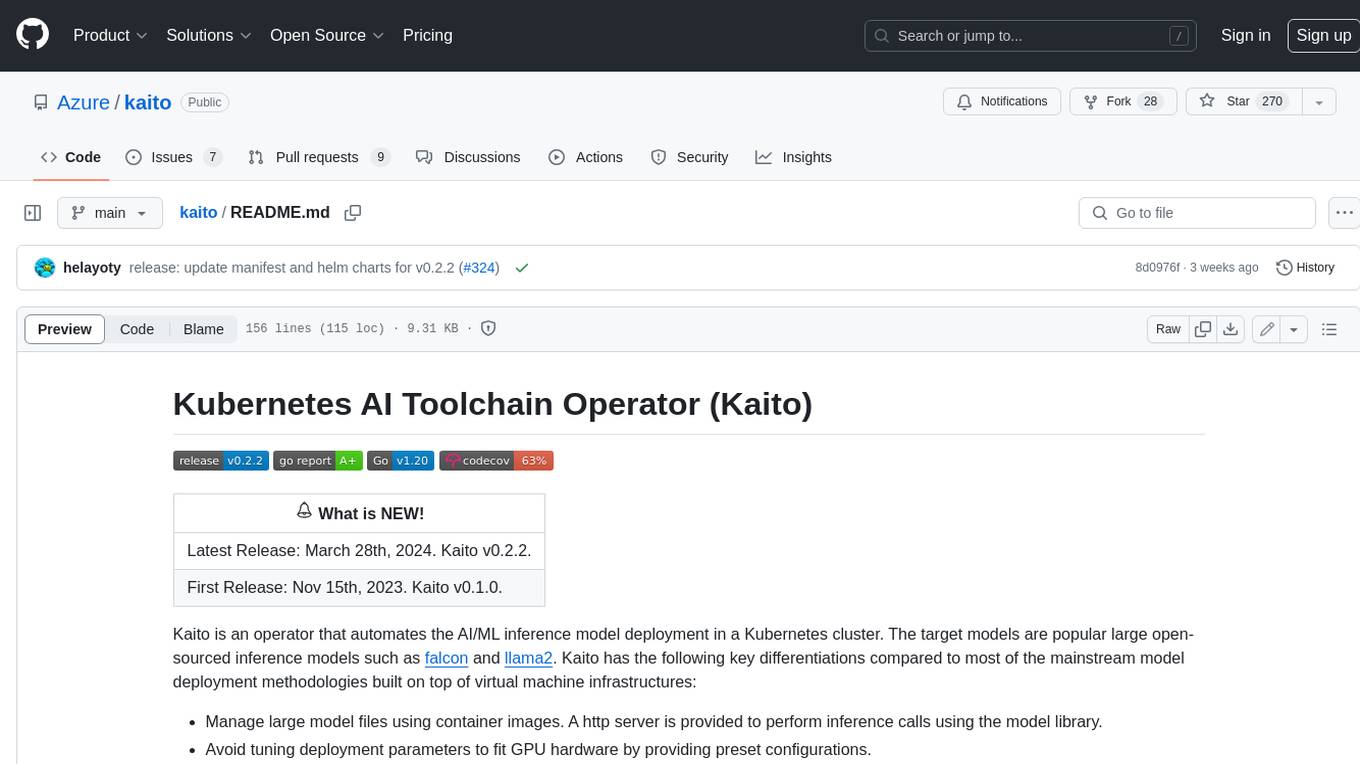
kaito
Kaito is an operator that automates the AI/ML inference model deployment in a Kubernetes cluster. It manages large model files using container images, avoids tuning deployment parameters to fit GPU hardware by providing preset configurations, auto-provisions GPU nodes based on model requirements, and hosts large model images in the public Microsoft Container Registry (MCR) if the license allows. Using Kaito, the workflow of onboarding large AI inference models in Kubernetes is largely simplified.
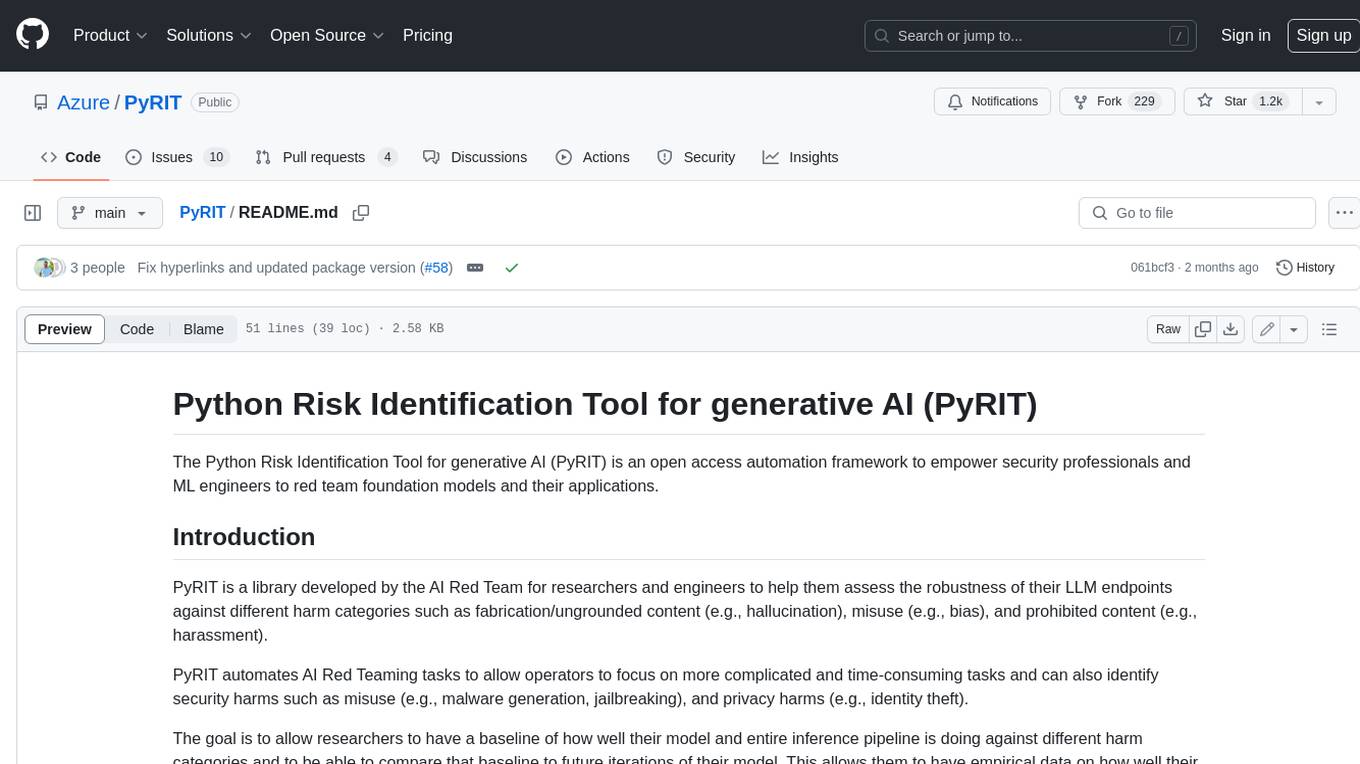
PyRIT
PyRIT is an open access automation framework designed to empower security professionals and ML engineers to red team foundation models and their applications. It automates AI Red Teaming tasks to allow operators to focus on more complicated and time-consuming tasks and can also identify security harms such as misuse (e.g., malware generation, jailbreaking), and privacy harms (e.g., identity theft). The goal is to allow researchers to have a baseline of how well their model and entire inference pipeline is doing against different harm categories and to be able to compare that baseline to future iterations of their model. This allows them to have empirical data on how well their model is doing today, and detect any degradation of performance based on future improvements.
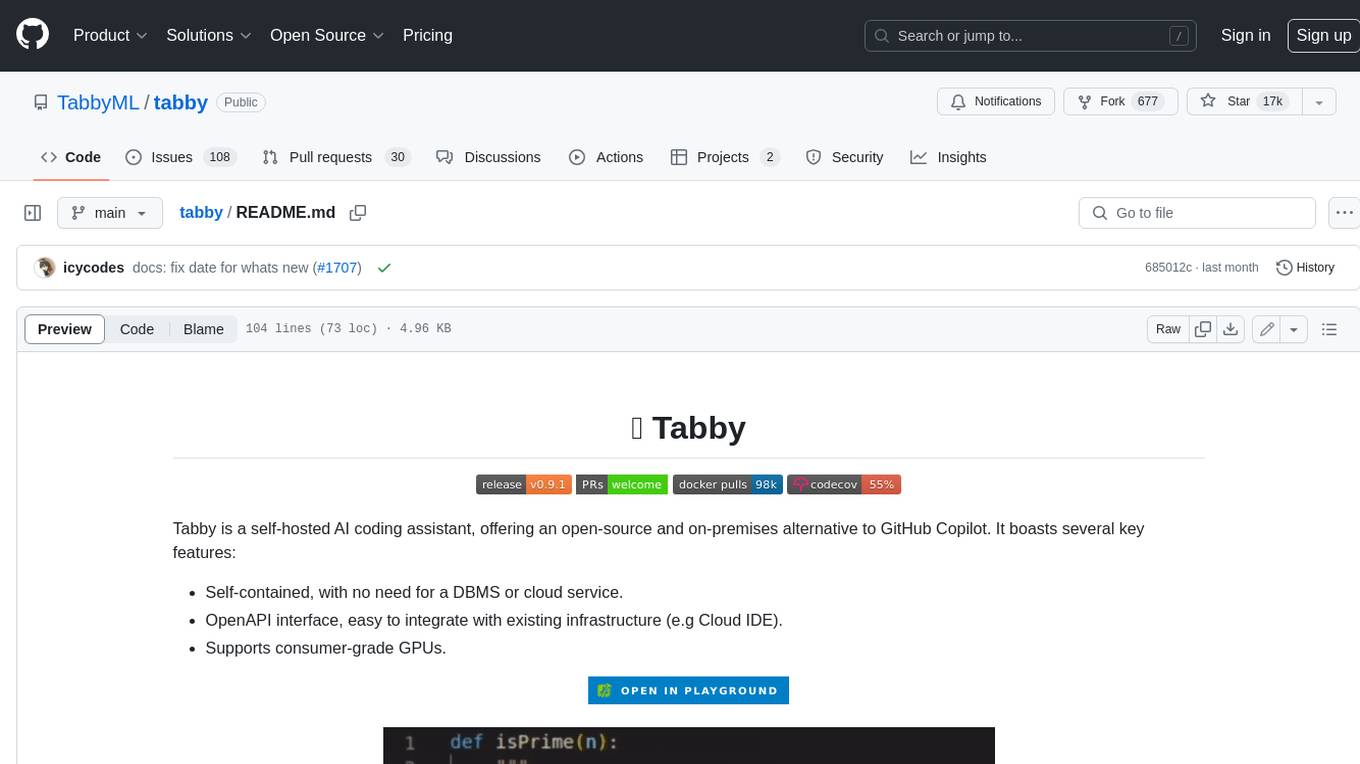
tabby
Tabby is a self-hosted AI coding assistant, offering an open-source and on-premises alternative to GitHub Copilot. It boasts several key features: * Self-contained, with no need for a DBMS or cloud service. * OpenAPI interface, easy to integrate with existing infrastructure (e.g Cloud IDE). * Supports consumer-grade GPUs.
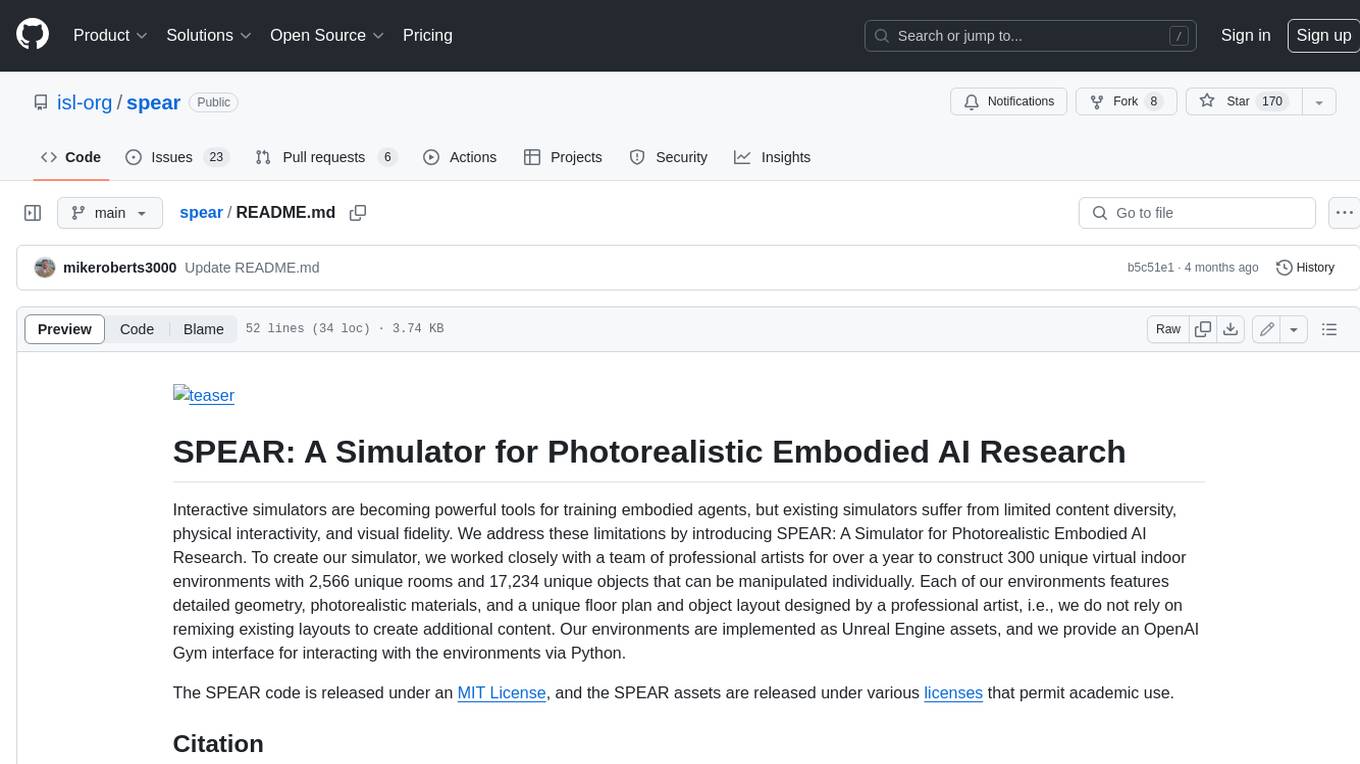
spear
SPEAR (Simulator for Photorealistic Embodied AI Research) is a powerful tool for training embodied agents. It features 300 unique virtual indoor environments with 2,566 unique rooms and 17,234 unique objects that can be manipulated individually. Each environment is designed by a professional artist and features detailed geometry, photorealistic materials, and a unique floor plan and object layout. SPEAR is implemented as Unreal Engine assets and provides an OpenAI Gym interface for interacting with the environments via Python.
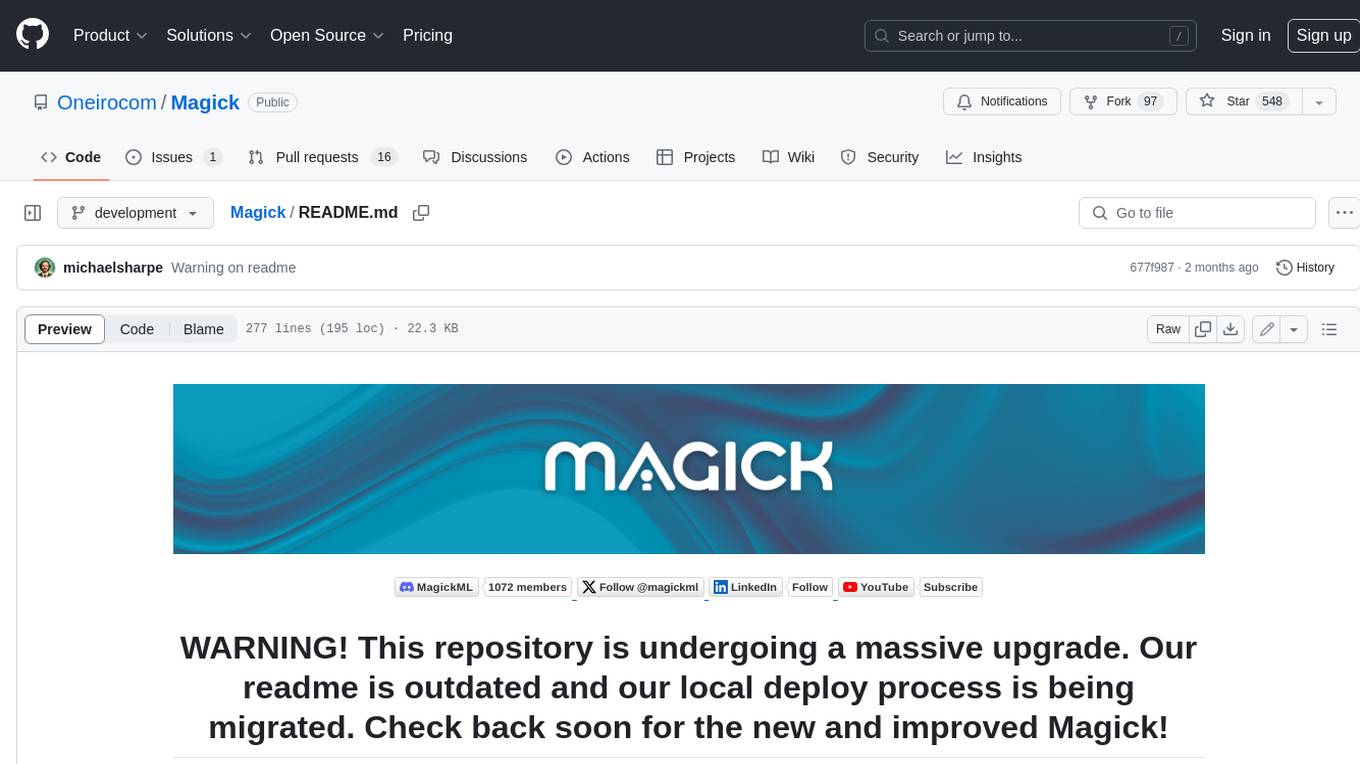
Magick
Magick is a groundbreaking visual AIDE (Artificial Intelligence Development Environment) for no-code data pipelines and multimodal agents. Magick can connect to other services and comes with nodes and templates well-suited for intelligent agents, chatbots, complex reasoning systems and realistic characters.