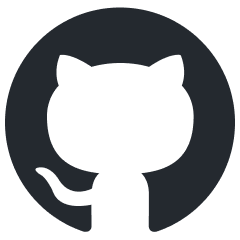
evalplus
Rigourous evaluation of LLM-synthesized code - NeurIPS 2023 & COLM 2024
Stars: 1303
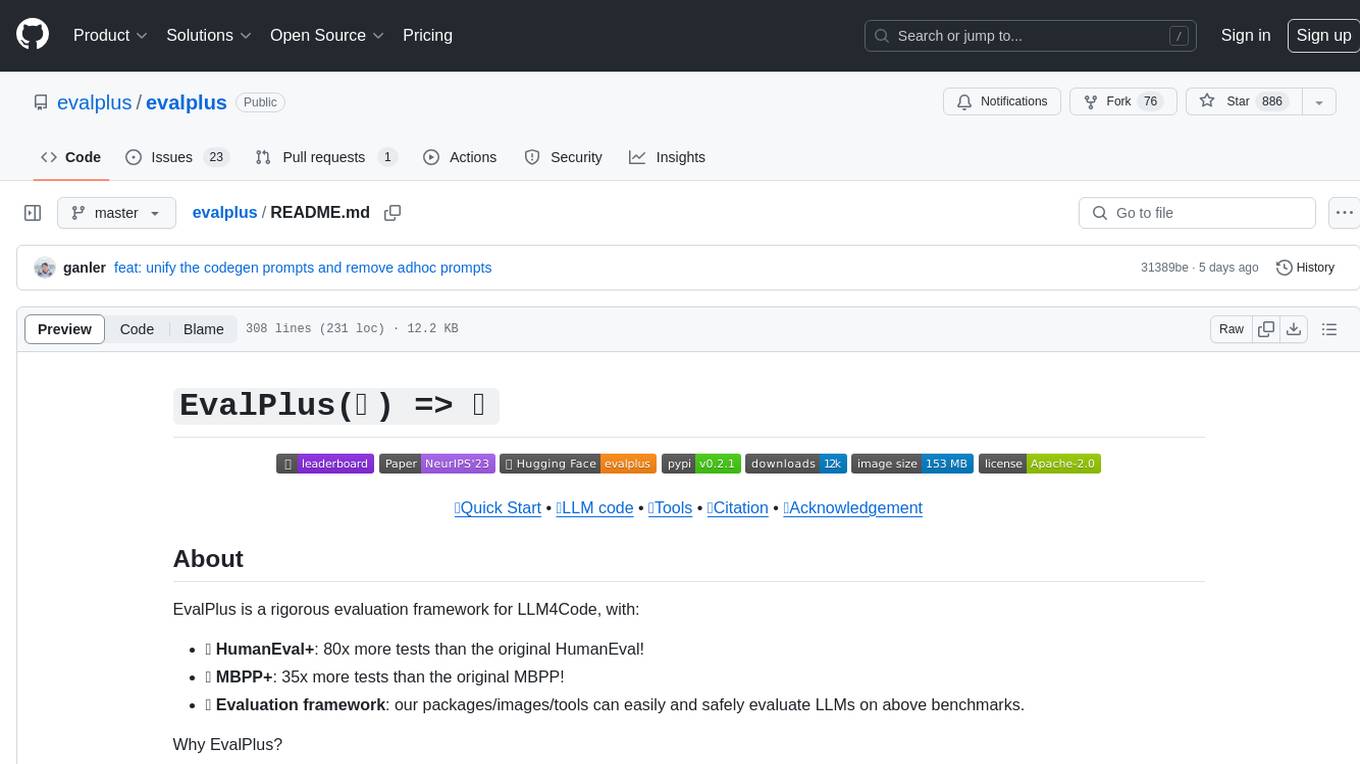
EvalPlus is a rigorous evaluation framework for LLM4Code, providing HumanEval+ and MBPP+ tests to evaluate large language models on code generation tasks. It offers precise evaluation and ranking, coding rigorousness analysis, and pre-generated code samples. Users can use EvalPlus to generate code solutions, post-process code, and evaluate code quality. The tool includes tools for code generation and test input generation using various backends.
README:
📙About • 🔥Quick Start • 🚀LLM Backends • 📚Documents • 📜Citation • 🙏Acknowledgement
Who's using EvalPlus datasets? EvalPlus has been used by various LLM teams, including:
- Meta Llama 3.1 and 3.3
- Allen AI TÜLU 1/2/3
- Qwen2.5-Coder
- CodeQwen 1.5
- DeepSeek-Coder V2
- Qwen2
- Snowflake Arctic
- StarCoder2
- Magicoder
- WizardCoder
Below tracks the notable updates of EvalPlus:
-
[2024-10-20
v0.3.1
]: EvalPlusv0.3.1
is officially released! Highlights: (i) Code efficiency evaluation via EvalPerf, (ii) one command to run all: generation + post-processing + evaluation, (iii) support for more inference backends such as Google Gemini & Anthropic, etc. -
[2024-06-09 pre
v0.3.0
]: Improved ground-truth solutions for MBPP+ tasks (IDs: 459, 102, 559). Thanks to EvalArena. -
[2024-04-17 pre
v0.3.0
]: MBPP+ is upgraded tov0.2.0
by removing some broken tasks (399 -> 378 tasks). ~4pp pass@1 improvement could be expected.
Earlier news :: click to expand ::
- (
v0.2.1
) You can use EvalPlus datasets via bigcode-evaluation-harness! HumanEval+ oracle fixes (32). - (
v0.2.0
) MBPP+ is released! HumanEval contract & input fixes (0/3/9/148/114/1/2/99/28/32/35/160). - (
v0.1.7
) Leaderboard release; HumanEval+ contract and input fixes (32/166/126/6) - (
v0.1.6
) Configurable and by-default-conservative timeout settings; HumanEval+ contract & ground-truth fixes (129/148/75/53/0/3/9/140) - (
v0.1.5
) HumanEval+ mini is released for ultra-fast evaluation when you have too many samples! - (
v0.1.1
) Optimizing user experiences: evaluation speed, PyPI package, Docker, etc. - (
v0.1.0
) HumanEval+ is released!
EvalPlus is a rigorous evaluation framework for LLM4Code, with:
- ✨ HumanEval+: 80x more tests than the original HumanEval!
- ✨ MBPP+: 35x more tests than the original MBPP!
- ✨ EvalPerf: evaluating the efficiency of LLM-generated code!
- ✨ Framework: our packages/images/tools can easily and safely evaluate LLMs on above benchmarks.
Why EvalPlus?
- ✨ Precise evaluation: See our leaderboard for latest LLM rankings before & after rigorous evaluation.
- ✨ Coding rigorousness: Look at the score differences! esp. before & after using EvalPlus tests! Less drop means more rigorousness in code generation; while a bigger drop means the generated code tends to be fragile.
- ✨ Code efficiency: Beyond correctness, our EvalPerf dataset evaluates the efficiency of LLM-generated code via performance-exercising coding tasks and test inputs.
Want to know more details? Read our papers & materials!
- EvalPlus: NeurIPS'23 paper, Slides, Poster, Leaderboard
- EvalPerf: COLM'24 paper, Poster, Documentation, Leaderboard
pip install --upgrade "evalplus[vllm] @ git+https://github.com/evalplus/evalplus"
# Or `pip install "evalplus[vllm]" --upgrade` for the latest stable release
evalplus.evaluate --model "ise-uiuc/Magicoder-S-DS-6.7B" \
--dataset [humaneval|mbpp] \
--backend vllm \
--greedy
🛡️ Safe code execution within Docker :: click to expand ::
# Local generation
evalplus.codegen --model "ise-uiuc/Magicoder-S-DS-6.7B" \
--dataset humaneval \
--backend vllm \
--greedy
# Code execution within Docker
docker run --rm --pull=always -v $(pwd)/evalplus_results:/app ganler/evalplus:latest \
evalplus.evaluate --dataset humaneval \
--samples /app/humaneval/ise-uiuc--Magicoder-S-DS-6.7B_vllm_temp_0.0.jsonl
pip install --upgrade "evalplus[perf,vllm] @ git+https://github.com/evalplus/evalplus"
# Or `pip install "evalplus[perf,vllm]" --upgrade` for the latest stable release
sudo sh -c 'echo 0 > /proc/sys/kernel/perf_event_paranoid' # Enable perf
evalplus.evalperf --model "ise-uiuc/Magicoder-S-DS-6.7B" --backend vllm
🛡️ Safe code execution within Docker :: click to expand ::
# Local generation
evalplus.codegen --model "ise-uiuc/Magicoder-S-DS-6.7B" \
--dataset evalperf \
--backend vllm \
--temperature 1.0 \
--n-samples 100
# Code execution within Docker
sudo sh -c 'echo 0 > /proc/sys/kernel/perf_event_paranoid' # Enable perf
docker run --cap-add PERFMON --rm --pull=always -v $(pwd)/evalplus_results:/app ganler/evalplus:latest \
evalplus.evalperf --samples /app/evalperf/ise-uiuc--Magicoder-S-DS-6.7B_vllm_temp_1.0.jsonl
-
transformers
backend:
evalplus.evaluate --model "ise-uiuc/Magicoder-S-DS-6.7B" \
--dataset [humaneval|mbpp] \
--backend hf \
--greedy
[!Note]
EvalPlus uses different prompts for base and chat models. By default it is detected by
tokenizer.chat_template
when usinghf
/vllm
as backend. For other backends, only chat mode is allowed.Therefore, if your base models come with a
tokenizer.chat_template
, please add--force-base-prompt
to avoid being evaluated in a chat mode.
Enable Flash Attention 2 :: click to expand ::
# Install Flash Attention 2
pip install packaging ninja
pip install flash-attn --no-build-isolation
# Note: if you have installation problem, consider using pre-built
# wheels from https://github.com/Dao-AILab/flash-attention/releases
# Run evaluation with FA2
evalplus.evaluate --model "ise-uiuc/Magicoder-S-DS-6.7B" \
--dataset [humaneval|mbpp] \
--backend hf \
--attn-implementation [flash_attention_2|sdpa] \
--greedy
-
vllm
backend:
evalplus.evaluate --model "ise-uiuc/Magicoder-S-DS-6.7B" \
--dataset [humaneval|mbpp] \
--backend vllm \
--tp [TENSOR_PARALLEL_SIZE] \
--greedy
-
openai
compatible servers (e.g., vLLM):
# OpenAI models
export OPENAI_API_KEY="{KEY}" # https://platform.openai.com/settings/organization/api-keys
evalplus.evaluate --model "gpt-4o-2024-08-06" \
--dataset [humaneval|mbpp] \
--backend openai --greedy
# DeepSeek
export OPENAI_API_KEY="{KEY}" # https://platform.deepseek.com/api_keys
evalplus.evaluate --model "deepseek-chat" \
--dataset [humaneval|mbpp] \
--base-url https://api.deepseek.com \
--backend openai --greedy
# Grok
export OPENAI_API_KEY="{KEY}" # https://console.x.ai/
evalplus.evaluate --model "grok-beta" \
--dataset [humaneval|mbpp] \
--base-url https://api.x.ai/v1 \
--backend openai --greedy
# vLLM server
# First, launch a vLLM server: https://docs.vllm.ai/en/latest/serving/deploying_with_docker.html
evalplus.evaluate --model "ise-uiuc/Magicoder-S-DS-6.7B" \
--dataset [humaneval|mbpp] \
--base-url http://localhost:8000/v1 \
--backend openai --greedy
# GPTQModel
evalplus.evaluate --model "ModelCloud/Llama-3.2-1B-Instruct-gptqmodel-4bit-vortex-v1" \
--dataset [humaneval|mbpp] \
--backend gptqmodel --greedy
- Access OpenAI APIs from OpenAI Console
export OPENAI_API_KEY="[YOUR_API_KEY]"
evalplus.evaluate --model "gpt-4o" \
--dataset [humaneval|mbpp] \
--backend openai \
--greedy
- Access Anthropic APIs from Anthropic Console
export ANTHROPIC_API_KEY="[YOUR_API_KEY]"
evalplus.evaluate --model "claude-3-haiku-20240307" \
--dataset [humaneval|mbpp] \
--backend anthropic \
--greedy
- Access Gemini APIs from Google AI Studio
export GOOGLE_API_KEY="[YOUR_API_KEY]"
evalplus.evaluate --model "gemini-1.5-pro" \
--dataset [humaneval|mbpp] \
--backend google \
--greedy
export BEDROCK_ROLE_ARN="[BEDROCK_ROLE_ARN]"
evalplus.evaluate --model "anthropic.claude-3-5-sonnet-20241022-v2:0" \
--dataset [humaneval|mbpp] \
--backend bedrock \
--greedy
You can checkout the generation and results at evalplus_results/[humaneval|mbpp]/
⏬ Using EvalPlus as a local repo? :: click to expand ::
git clone https://github.com/evalplus/evalplus.git
cd evalplus
export PYTHONPATH=$PYTHONPATH:$(pwd)
pip install -r requirements.txt
To learn more about how to use EvalPlus, please refer to:
@inproceedings{evalplus,
title = {Is Your Code Generated by Chat{GPT} Really Correct? Rigorous Evaluation of Large Language Models for Code Generation},
author = {Liu, Jiawei and Xia, Chunqiu Steven and Wang, Yuyao and Zhang, Lingming},
booktitle = {Thirty-seventh Conference on Neural Information Processing Systems},
year = {2023},
url = {https://openreview.net/forum?id=1qvx610Cu7},
}
@inproceedings{evalperf,
title = {Evaluating Language Models for Efficient Code Generation},
author = {Liu, Jiawei and Xie, Songrun and Wang, Junhao and Wei, Yuxiang and Ding, Yifeng and Zhang, Lingming},
booktitle = {First Conference on Language Modeling},
year = {2024},
url = {https://openreview.net/forum?id=IBCBMeAhmC},
}
For Tasks:
Click tags to check more tools for each tasksFor Jobs:
Alternative AI tools for evalplus
Similar Open Source Tools
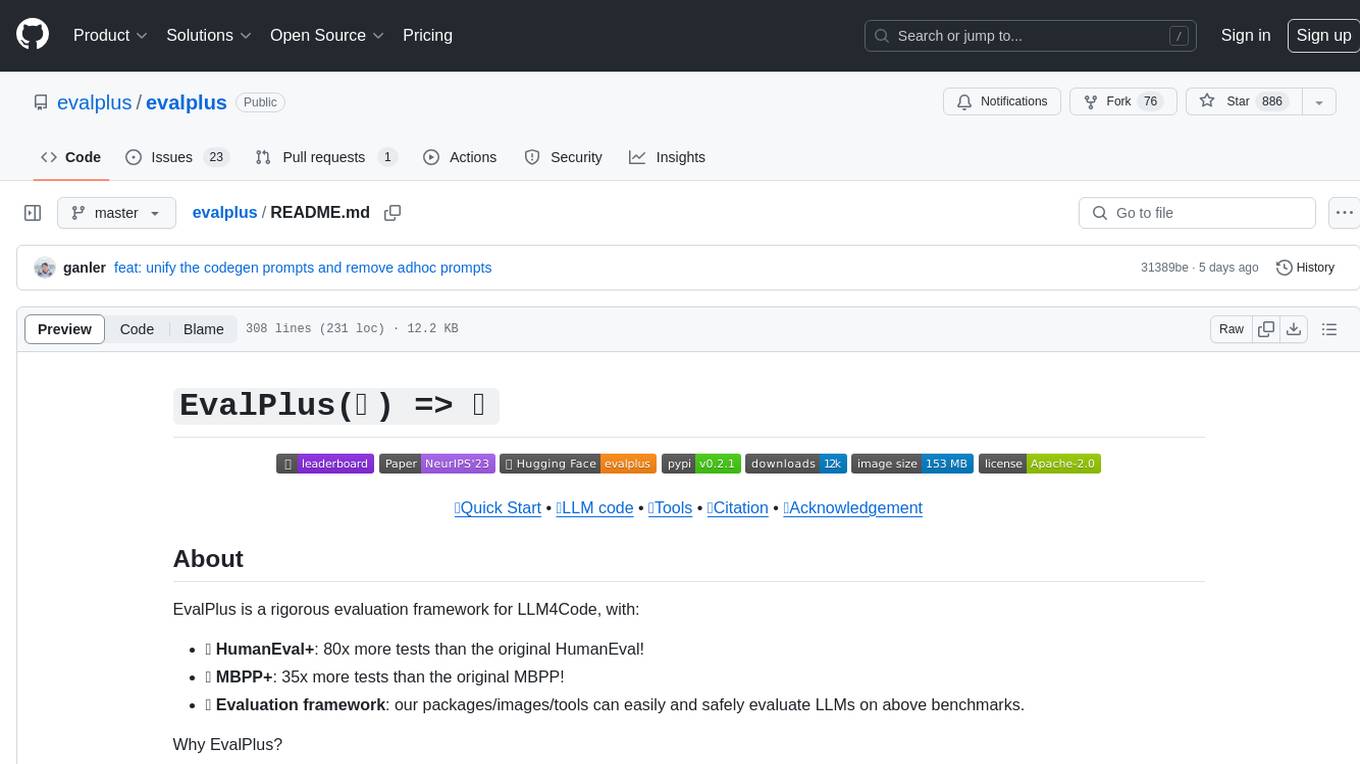
evalplus
EvalPlus is a rigorous evaluation framework for LLM4Code, providing HumanEval+ and MBPP+ tests to evaluate large language models on code generation tasks. It offers precise evaluation and ranking, coding rigorousness analysis, and pre-generated code samples. Users can use EvalPlus to generate code solutions, post-process code, and evaluate code quality. The tool includes tools for code generation and test input generation using various backends.
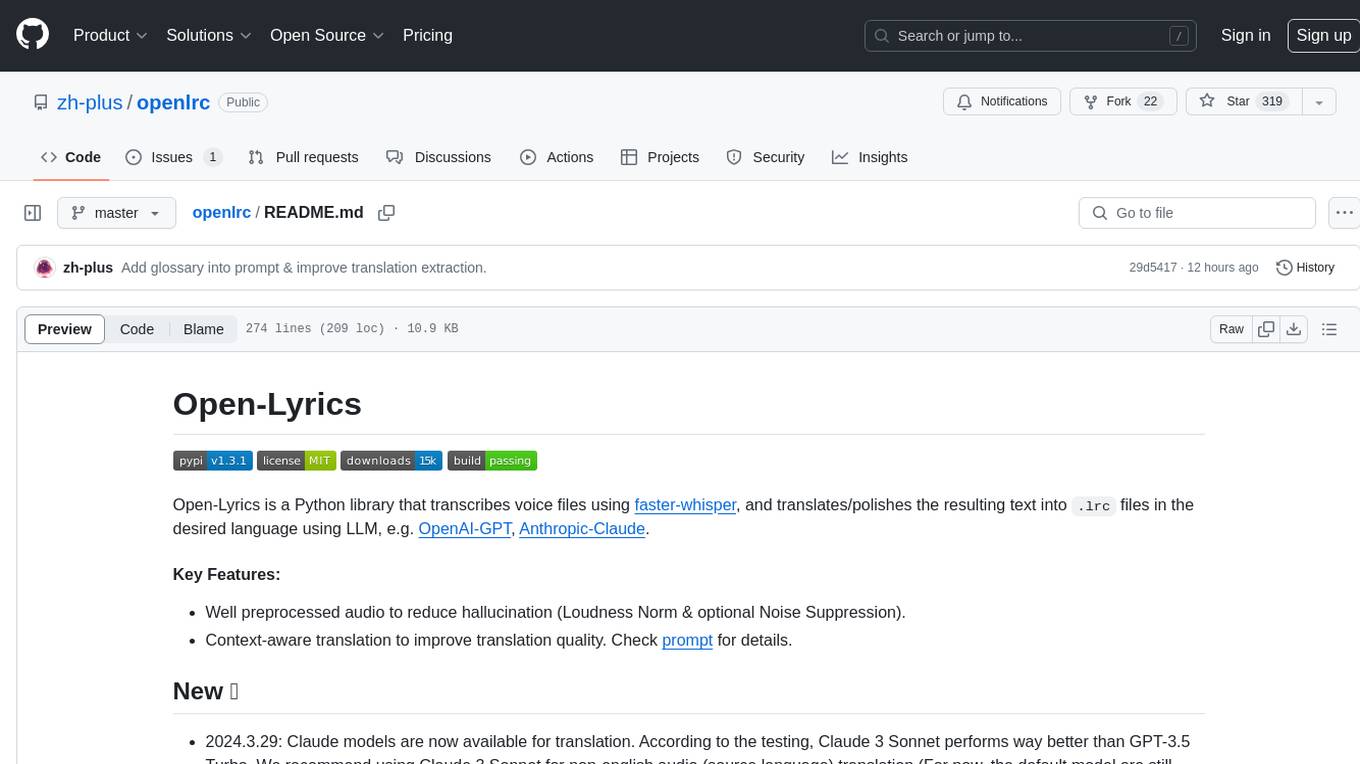
openlrc
Open-Lyrics is a Python library that transcribes voice files using faster-whisper and translates/polishes the resulting text into `.lrc` files in the desired language using LLM, e.g. OpenAI-GPT, Anthropic-Claude. It offers well preprocessed audio to reduce hallucination and context-aware translation to improve translation quality. Users can install the library from PyPI or GitHub and follow the installation steps to set up the environment. The tool supports GUI usage and provides Python code examples for transcription and translation tasks. It also includes features like utilizing context and glossary for translation enhancement, pricing information for different models, and a list of todo tasks for future improvements.
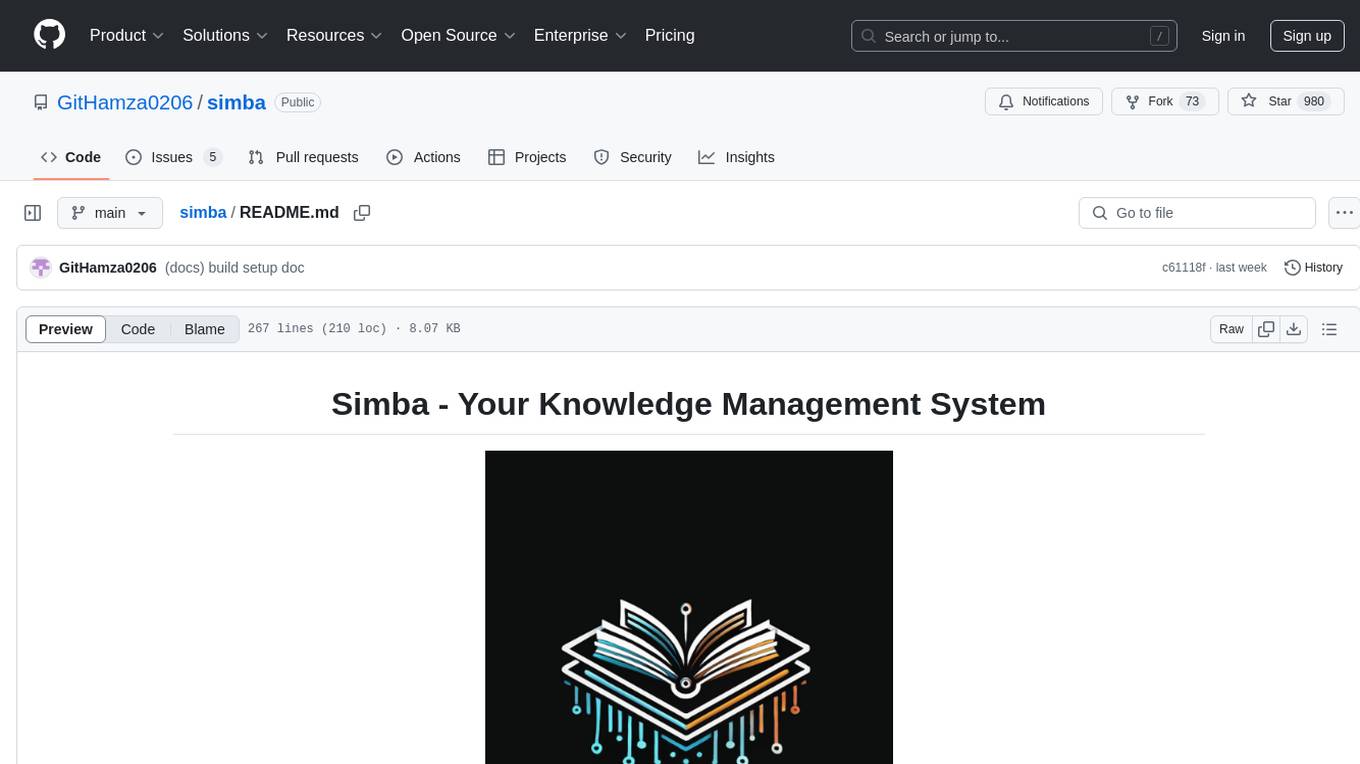
simba
Simba is an open source, portable Knowledge Management System (KMS) designed to seamlessly integrate with any Retrieval-Augmented Generation (RAG) system. It features a modern UI and modular architecture, allowing developers to focus on building advanced AI solutions without the complexities of knowledge management. Simba offers a user-friendly interface to visualize and modify document chunks, supports various vector stores and embedding models, and simplifies knowledge management for developers. It is community-driven, extensible, and aims to enhance AI functionality by providing a seamless integration with RAG-based systems.
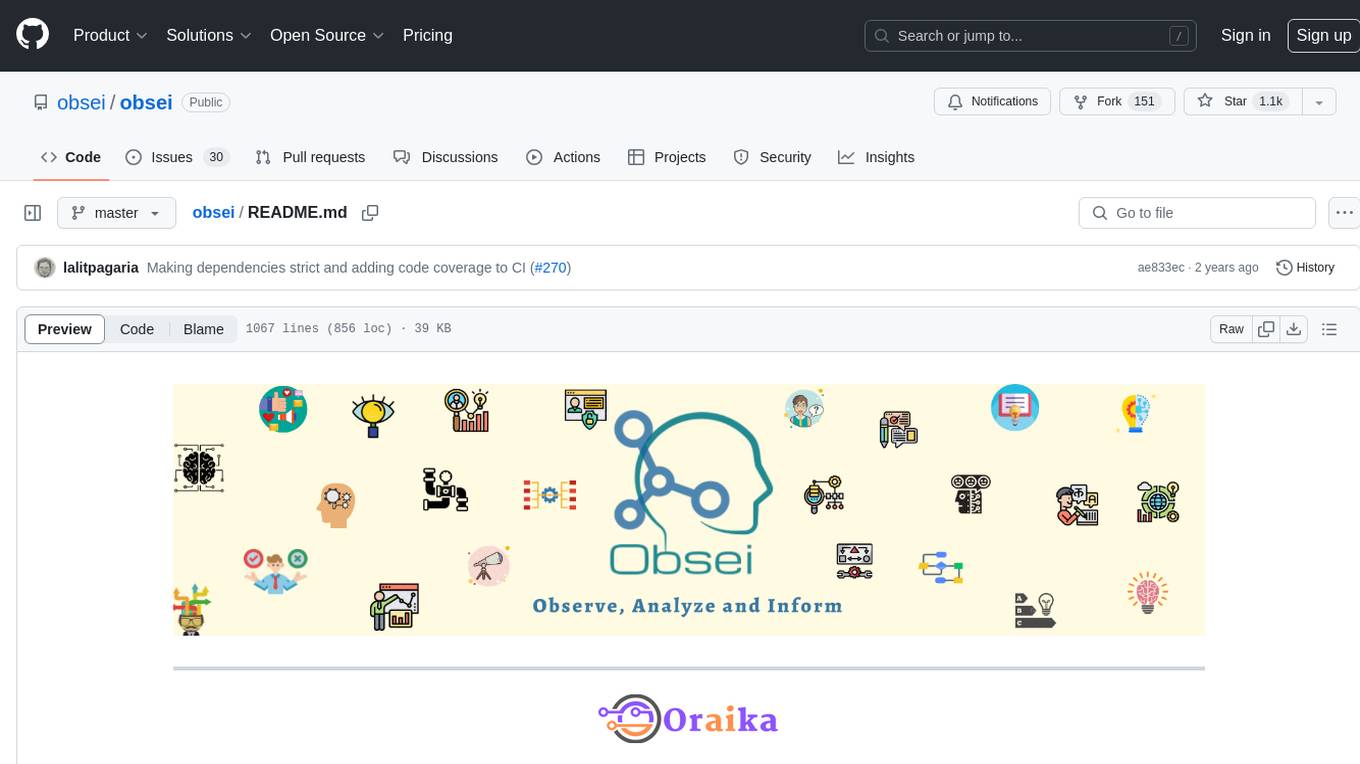
obsei
Obsei is an open-source, low-code, AI powered automation tool that consists of an Observer to collect unstructured data from various sources, an Analyzer to analyze the collected data with various AI tasks, and an Informer to send analyzed data to various destinations. The tool is suitable for scheduled jobs or serverless applications as all Observers can store their state in databases. Obsei is still in alpha stage, so caution is advised when using it in production. The tool can be used for social listening, alerting/notification, automatic customer issue creation, extraction of deeper insights from feedbacks, market research, dataset creation for various AI tasks, and more based on creativity.
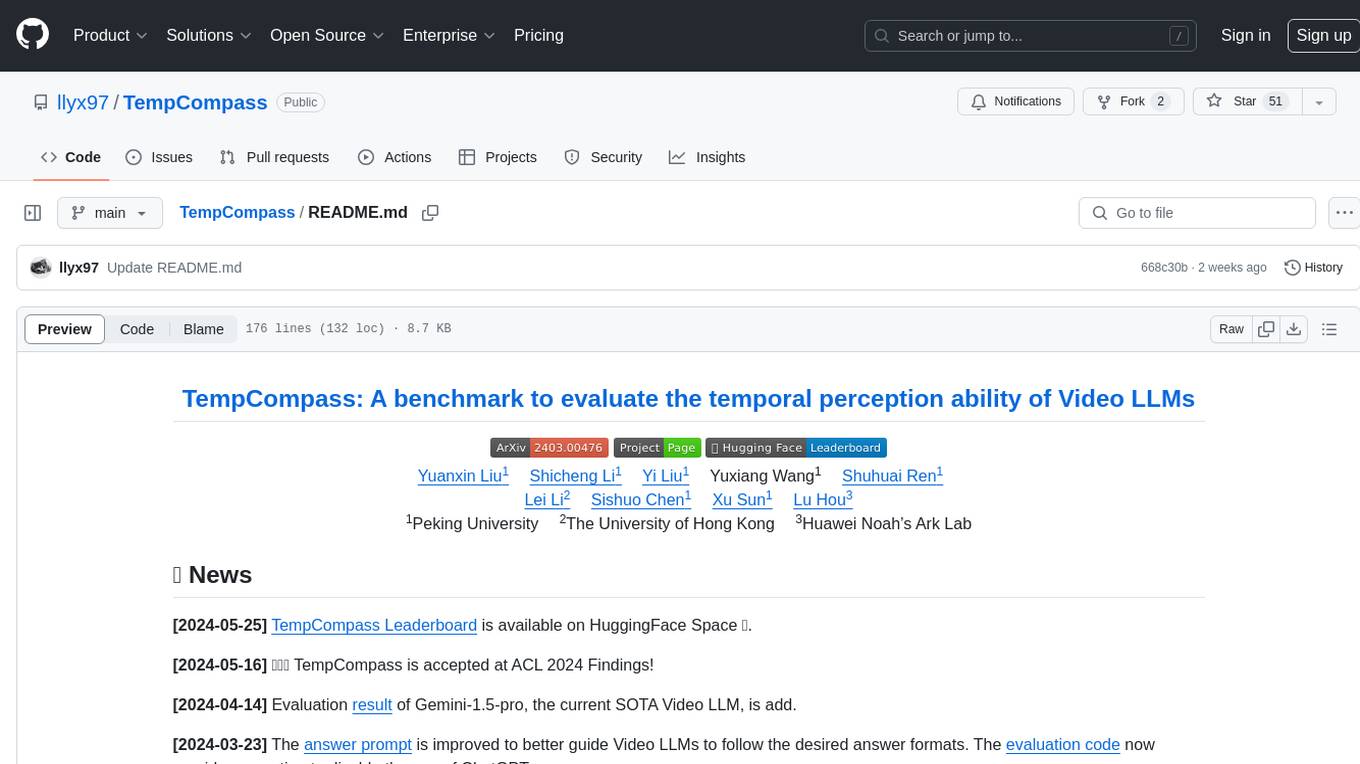
TempCompass
TempCompass is a benchmark designed to evaluate the temporal perception ability of Video LLMs. It encompasses a diverse set of temporal aspects and task formats to comprehensively assess the capability of Video LLMs in understanding videos. The benchmark includes conflicting videos to prevent models from relying on single-frame bias and language priors. Users can clone the repository, install required packages, prepare data, run inference using examples like Video-LLaVA and Gemini, and evaluate the performance of their models across different tasks such as Multi-Choice QA, Yes/No QA, Caption Matching, and Caption Generation.
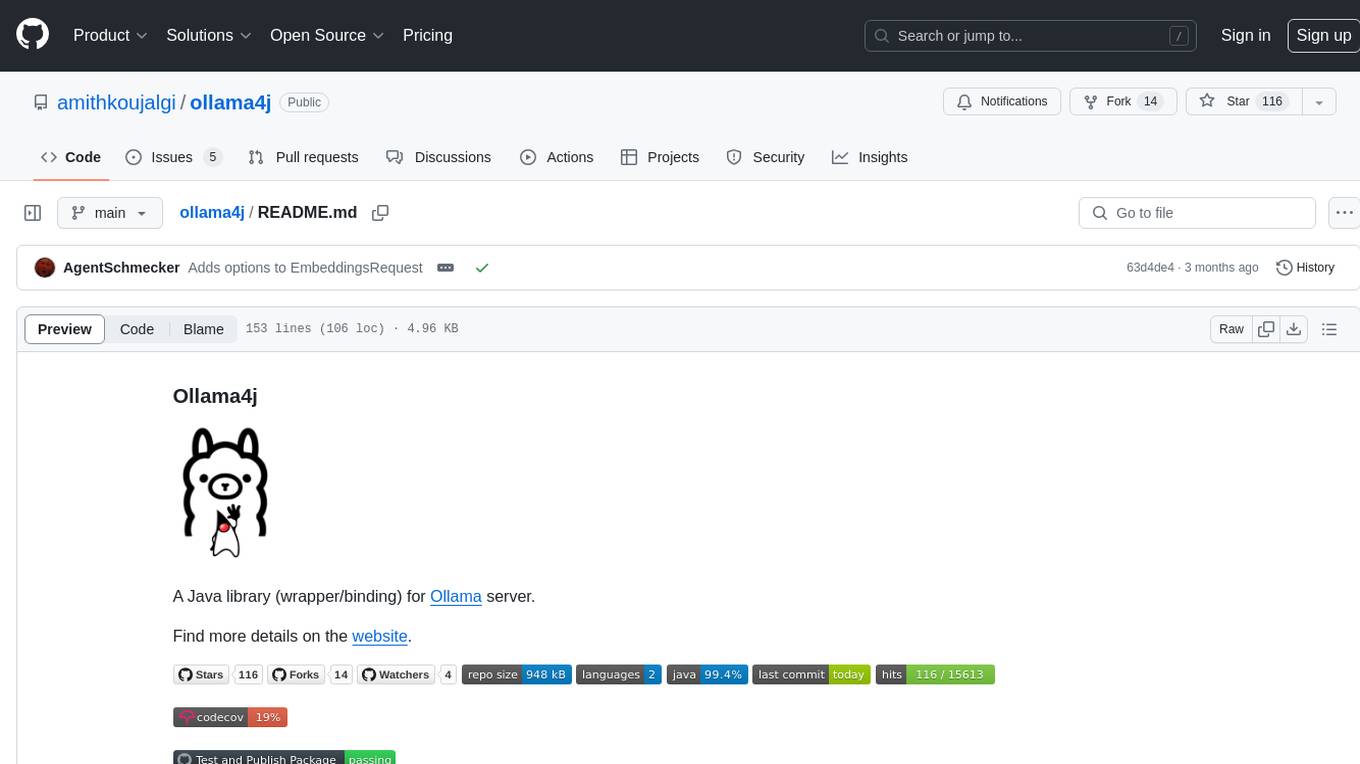
ollama4j
Ollama4j is a Java library that serves as a wrapper or binding for the Ollama server. It facilitates communication with the Ollama server and provides models for deployment. The tool requires Java 11 or higher and can be installed locally or via Docker. Users can integrate Ollama4j into Maven projects by adding the specified dependency. The tool offers API specifications and supports various development tasks such as building, running unit tests, and integration tests. Releases are automated through GitHub Actions CI workflow. Areas of improvement include adhering to Java naming conventions, updating deprecated code, implementing logging, using lombok, and enhancing request body creation. Contributions to the project are encouraged, whether reporting bugs, suggesting enhancements, or contributing code.
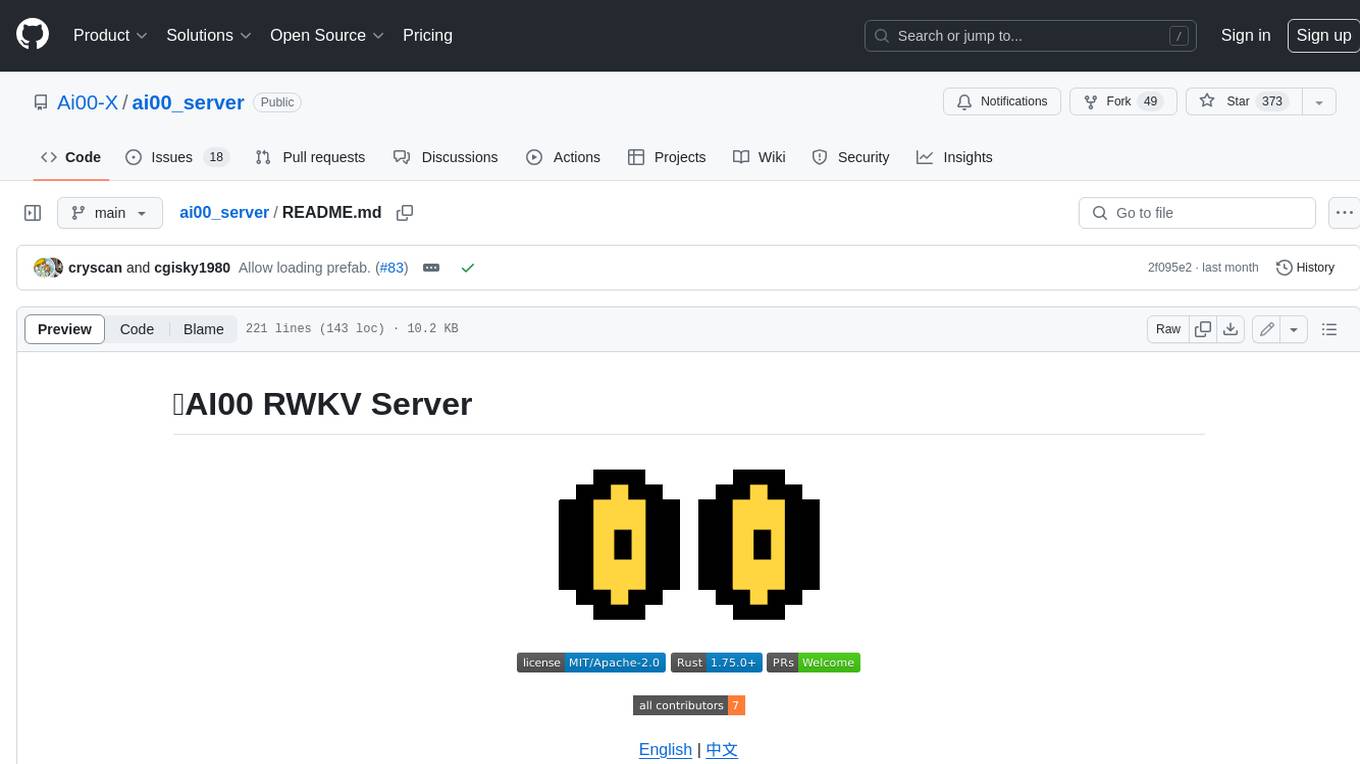
ai00_server
AI00 RWKV Server is an inference API server for the RWKV language model based upon the web-rwkv inference engine. It supports VULKAN parallel and concurrent batched inference and can run on all GPUs that support VULKAN. No need for Nvidia cards!!! AMD cards and even integrated graphics can be accelerated!!! No need for bulky pytorch, CUDA and other runtime environments, it's compact and ready to use out of the box! Compatible with OpenAI's ChatGPT API interface. 100% open source and commercially usable, under the MIT license. If you are looking for a fast, efficient, and easy-to-use LLM API server, then AI00 RWKV Server is your best choice. It can be used for various tasks, including chatbots, text generation, translation, and Q&A.
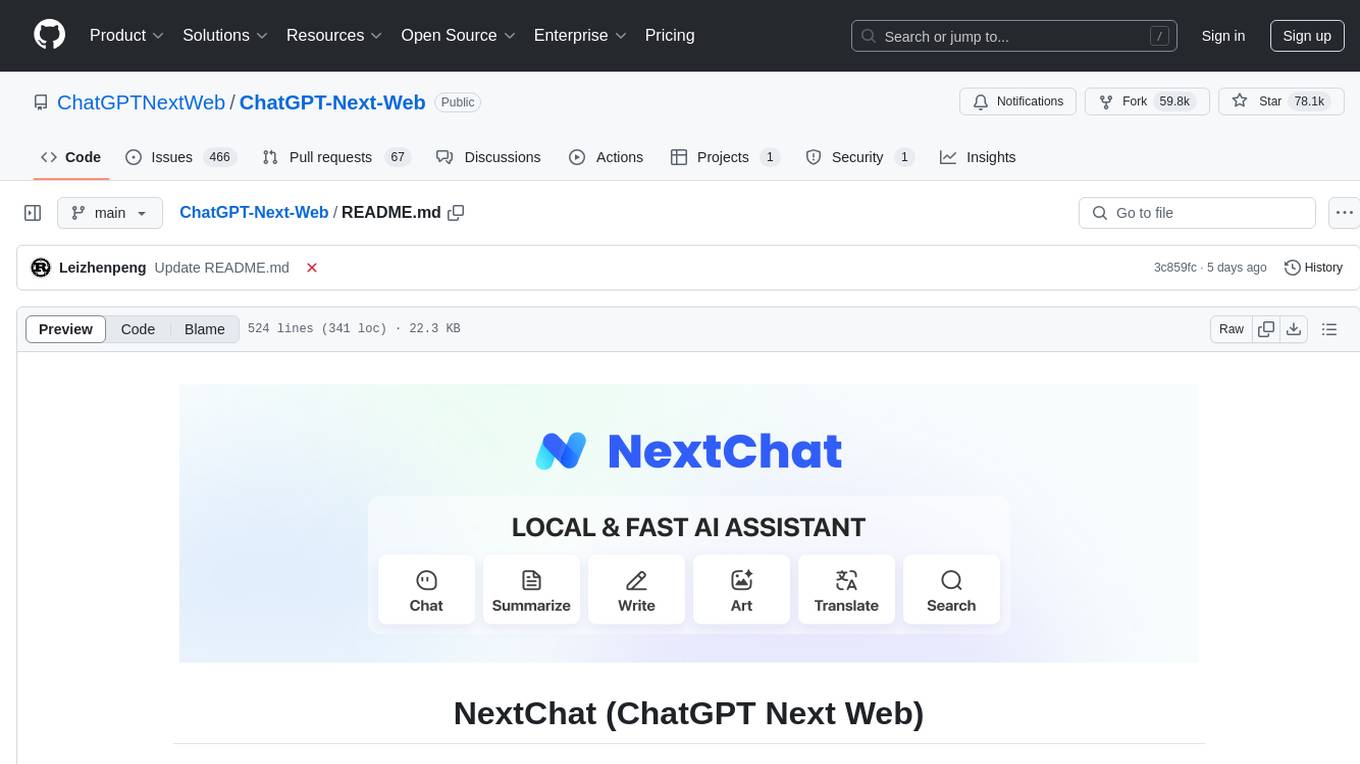
ChatGPT-Next-Web
ChatGPT Next Web is a well-designed cross-platform ChatGPT web UI tool that supports Claude, GPT4, and Gemini Pro models. It allows users to deploy their private ChatGPT applications with ease. The tool offers features like one-click deployment, compact client for Linux/Windows/MacOS, compatibility with self-deployed LLMs, privacy-first approach with local data storage, markdown support, responsive design, fast loading speed, prompt templates, awesome prompts, chat history compression, multilingual support, and more.
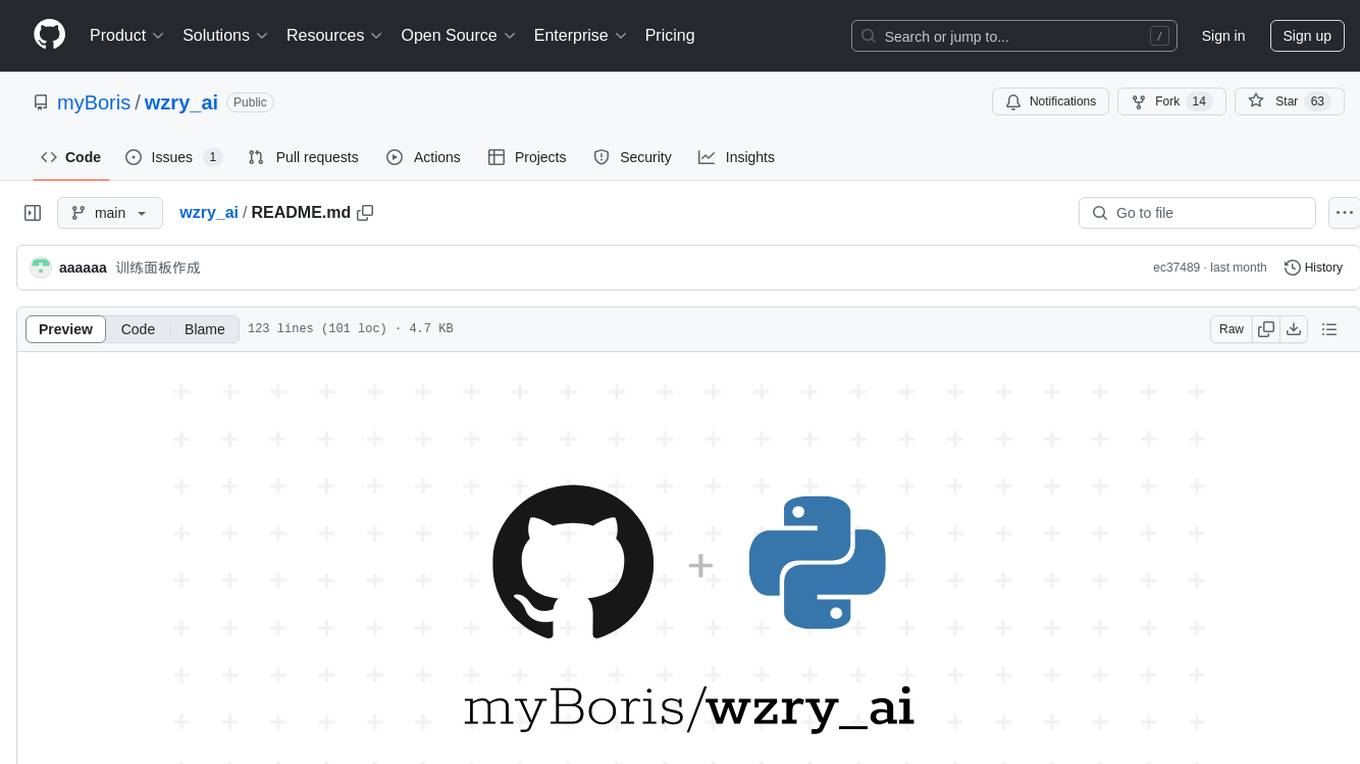
wzry_ai
This is an open-source project for playing the game King of Glory with an artificial intelligence model. The first phase of the project has been completed, and future upgrades will be built upon this foundation. The second phase of the project has started, and progress is expected to proceed according to plan. For any questions, feel free to join the QQ exchange group: 687853827. The project aims to learn artificial intelligence and strictly prohibits cheating. Detailed installation instructions are available in the doc/README.md file. Environment installation video: (bilibili) Welcome to follow, like, tip, comment, and provide your suggestions.
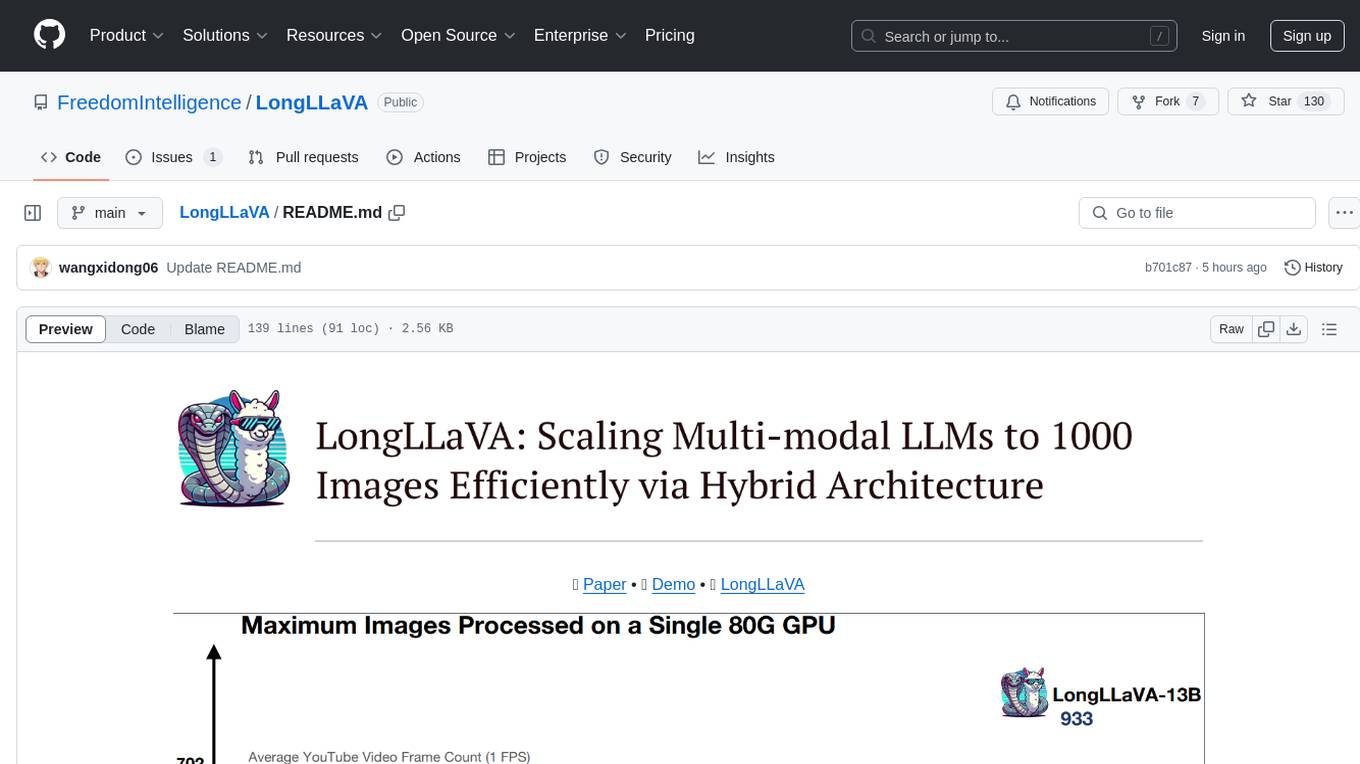
LongLLaVA
LongLLaVA is a tool for scaling multi-modal LLMs to 1000 images efficiently via hybrid architecture. It includes stages for single-image alignment, instruction-tuning, and multi-image instruction-tuning, with evaluation through a command line interface and model inference. The tool aims to achieve GPT-4V level capabilities and beyond, providing reproducibility of results and benchmarks for efficiency and performance.
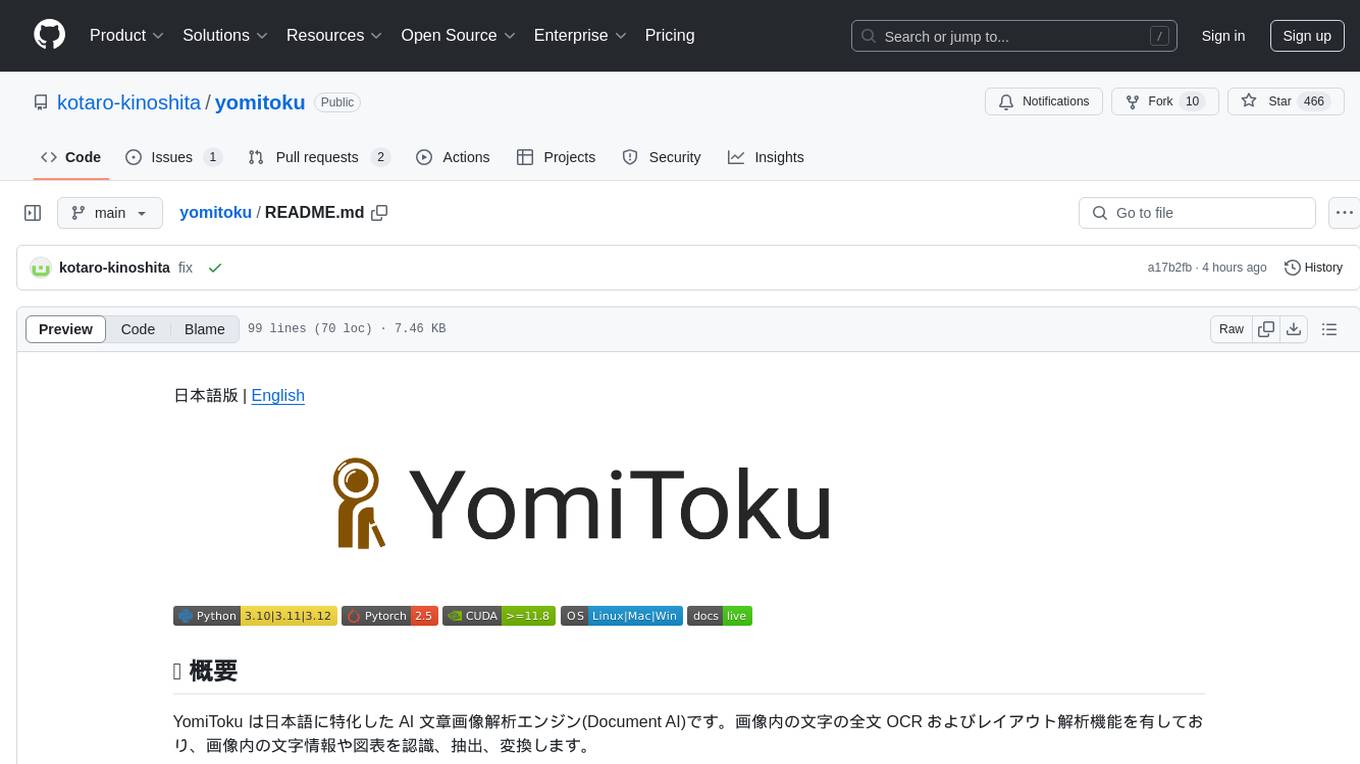
yomitoku
YomiToku is a Japanese-focused AI document image analysis engine that provides full-text OCR and layout analysis capabilities for images. It recognizes, extracts, and converts text information and figures in images. It includes 4 AI models trained on Japanese datasets for tasks such as detecting text positions, recognizing text strings, analyzing layouts, and recognizing table structures. The models are specialized for Japanese document images, supporting recognition of over 7000 Japanese characters and analyzing layout structures specific to Japanese documents. It offers features like layout analysis, table structure analysis, and reading order estimation to extract information from document images without disrupting their semantic structure. YomiToku supports various output formats such as HTML, markdown, JSON, and CSV, and can also extract figures, tables, and images from documents. It operates efficiently in GPU environments, enabling fast and effective analysis of document transcriptions without requiring high-end GPUs.
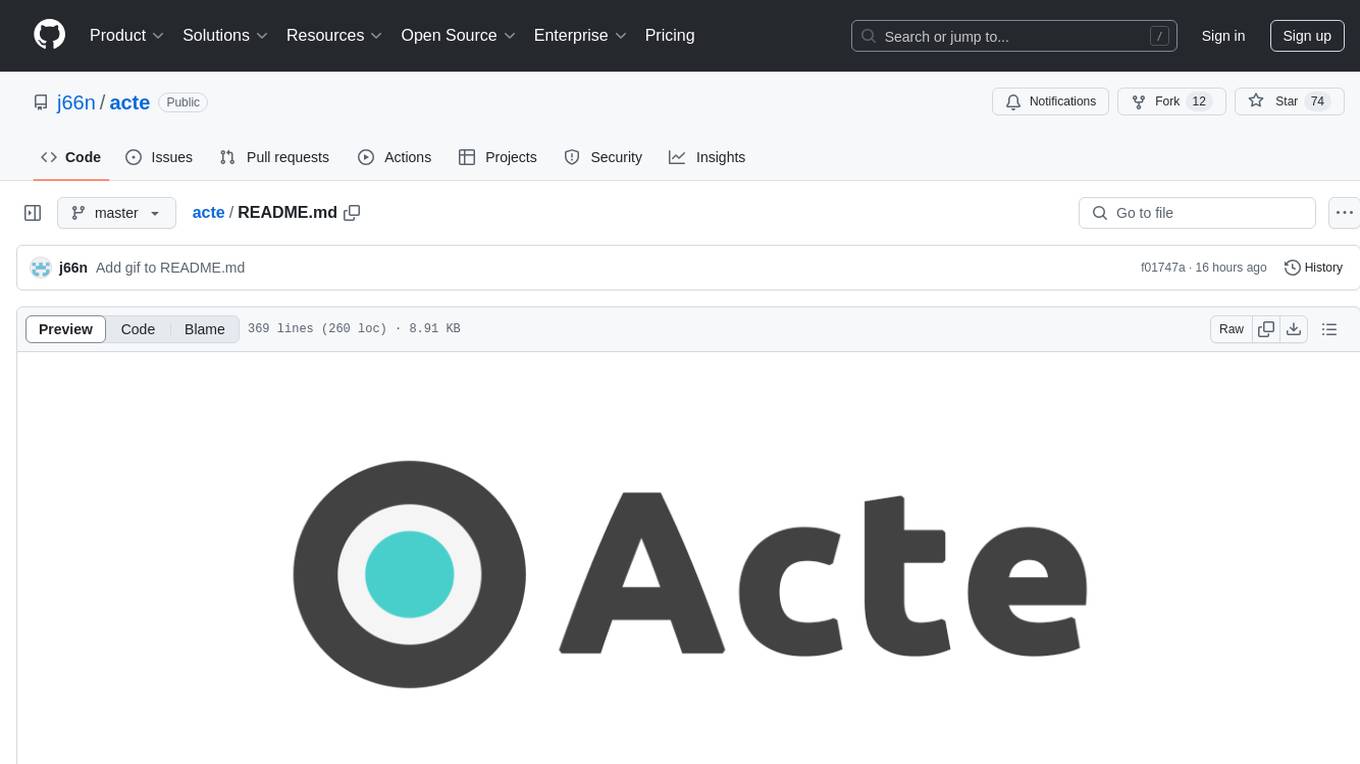
acte
Acte is a framework designed to build GUI-like tools for AI Agents. It aims to address the issues of cognitive load and freedom degrees when interacting with multiple APIs in complex scenarios. By providing a graphical user interface (GUI) for Agents, Acte helps reduce cognitive load and constraints interaction, similar to how humans interact with computers through GUIs. The tool offers APIs for starting new sessions, executing actions, and displaying screens, accessible via HTTP requests or the SessionManager class.
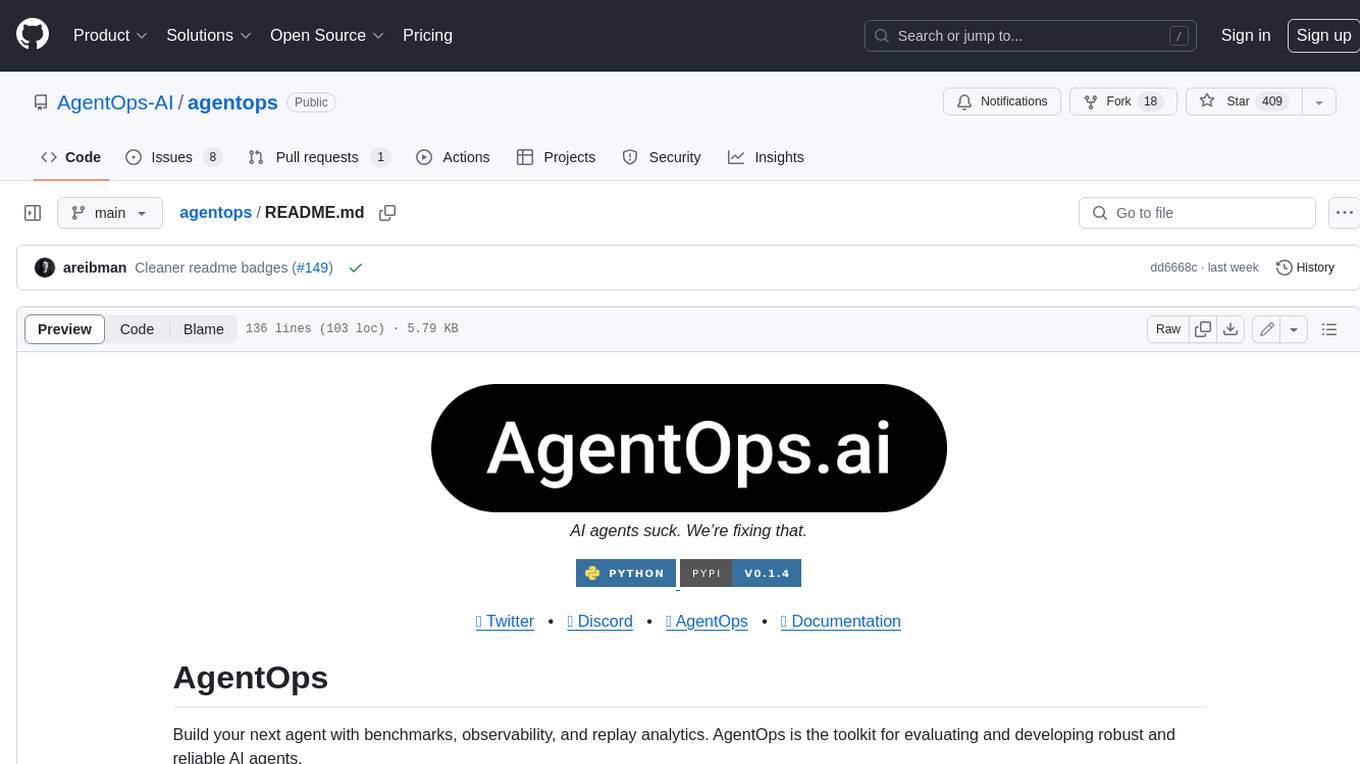
agentops
AgentOps is a toolkit for evaluating and developing robust and reliable AI agents. It provides benchmarks, observability, and replay analytics to help developers build better agents. AgentOps is open beta and can be signed up for here. Key features of AgentOps include: - Session replays in 3 lines of code: Initialize the AgentOps client and automatically get analytics on every LLM call. - Time travel debugging: (coming soon!) - Agent Arena: (coming soon!) - Callback handlers: AgentOps works seamlessly with applications built using Langchain and LlamaIndex.
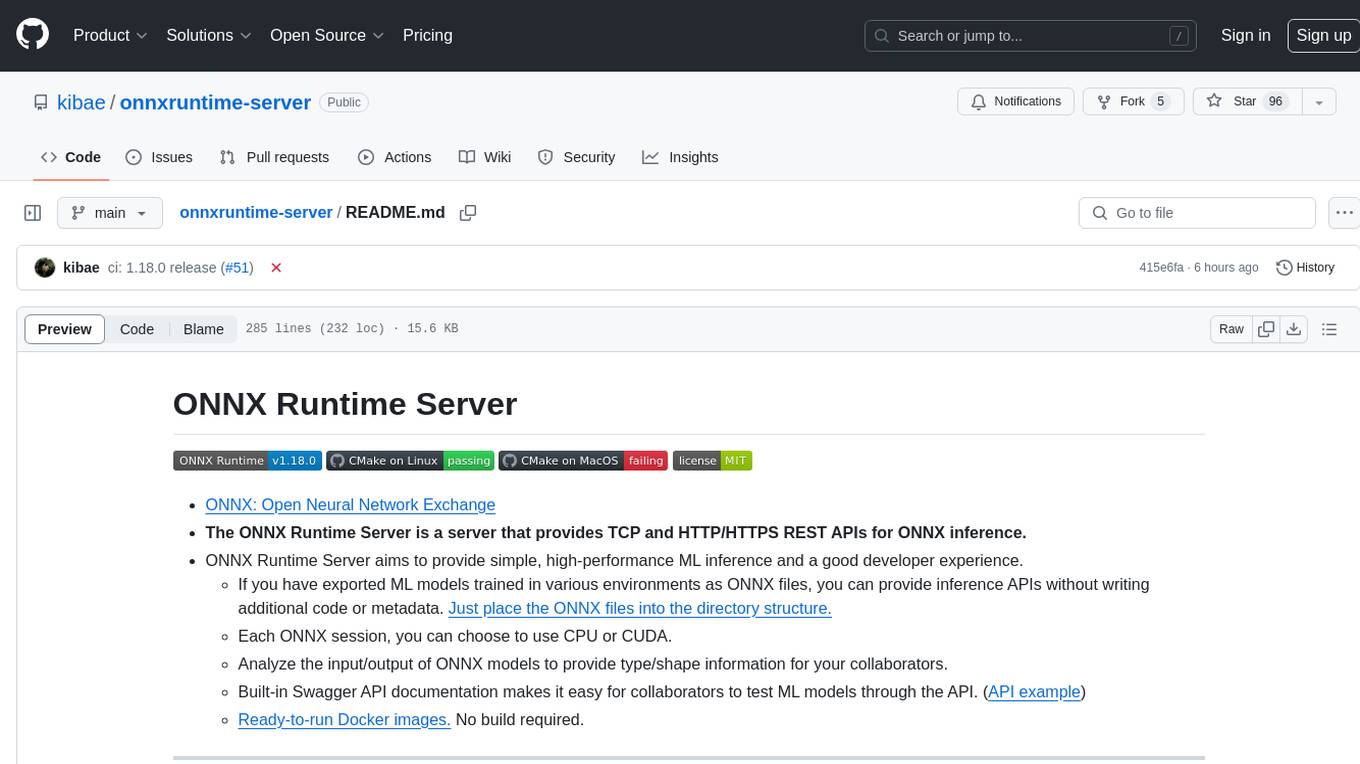
onnxruntime-server
ONNX Runtime Server is a server that provides TCP and HTTP/HTTPS REST APIs for ONNX inference. It aims to offer simple, high-performance ML inference and a good developer experience. Users can provide inference APIs for ONNX models without writing additional code by placing the models in the directory structure. Each session can choose between CPU or CUDA, analyze input/output, and provide Swagger API documentation for easy testing. Ready-to-run Docker images are available, making it convenient to deploy the server.
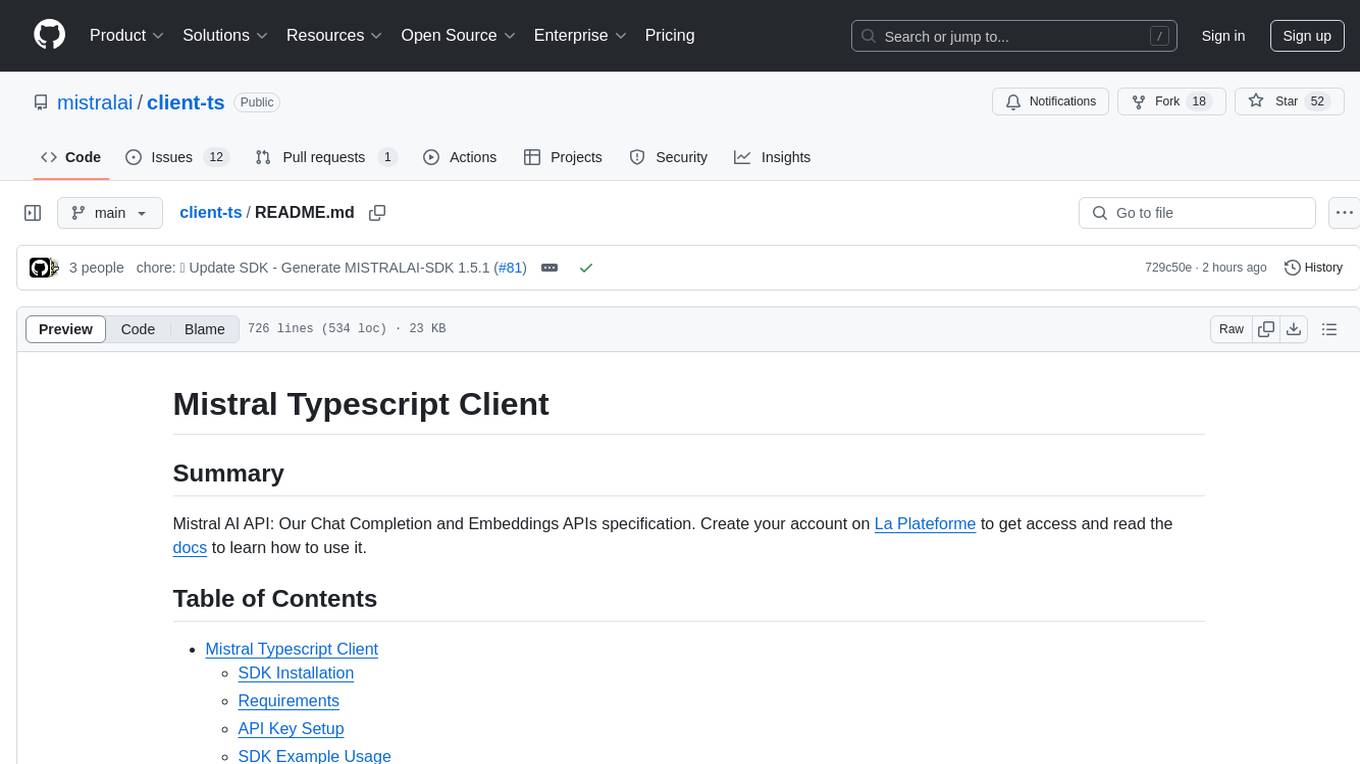
client-ts
Mistral Typescript Client is an SDK for Mistral AI API, providing Chat Completion and Embeddings APIs. It allows users to create chat completions, upload files, create agent completions, create embedding requests, and more. The SDK supports various JavaScript runtimes and provides detailed documentation on installation, requirements, API key setup, example usage, error handling, server selection, custom HTTP client, authentication, providers support, standalone functions, debugging, and contributions.
For similar tasks
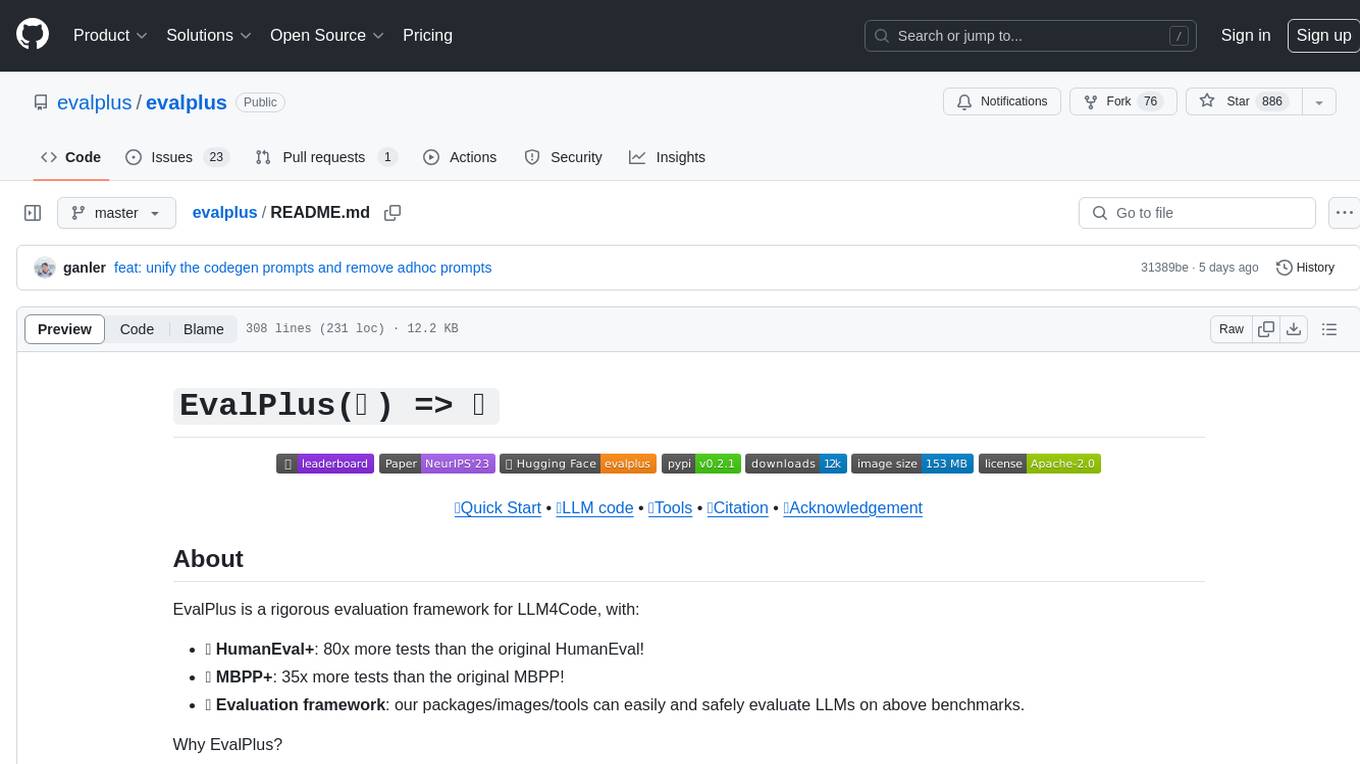
evalplus
EvalPlus is a rigorous evaluation framework for LLM4Code, providing HumanEval+ and MBPP+ tests to evaluate large language models on code generation tasks. It offers precise evaluation and ranking, coding rigorousness analysis, and pre-generated code samples. Users can use EvalPlus to generate code solutions, post-process code, and evaluate code quality. The tool includes tools for code generation and test input generation using various backends.
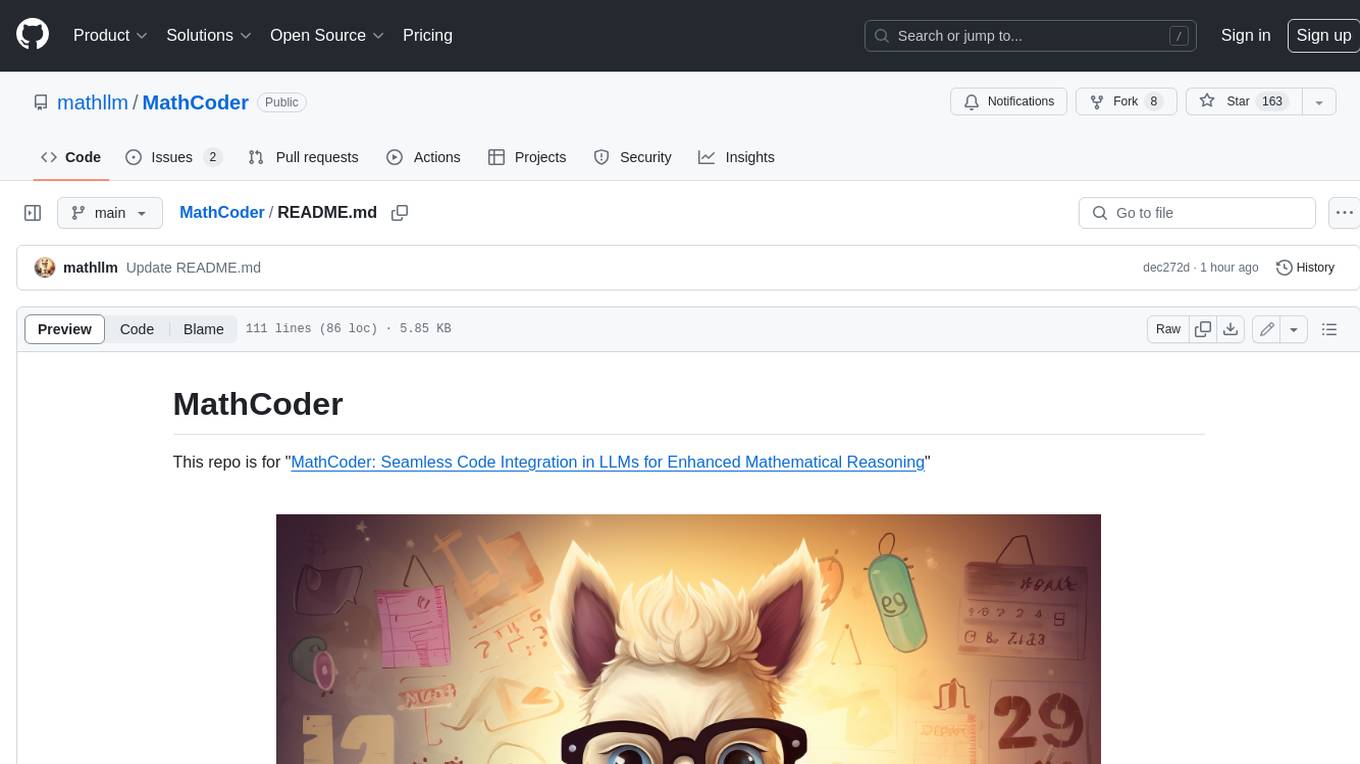
MathCoder
MathCoder is a repository focused on enhancing mathematical reasoning by fine-tuning open-source language models to use code for modeling and deriving math equations. It introduces MathCodeInstruct dataset with solutions interleaving natural language, code, and execution results. The repository provides MathCoder models capable of generating code-based solutions for challenging math problems, achieving state-of-the-art scores on MATH and GSM8K datasets. It offers tools for model deployment, inference, and evaluation, along with a citation for referencing the work.
For similar jobs
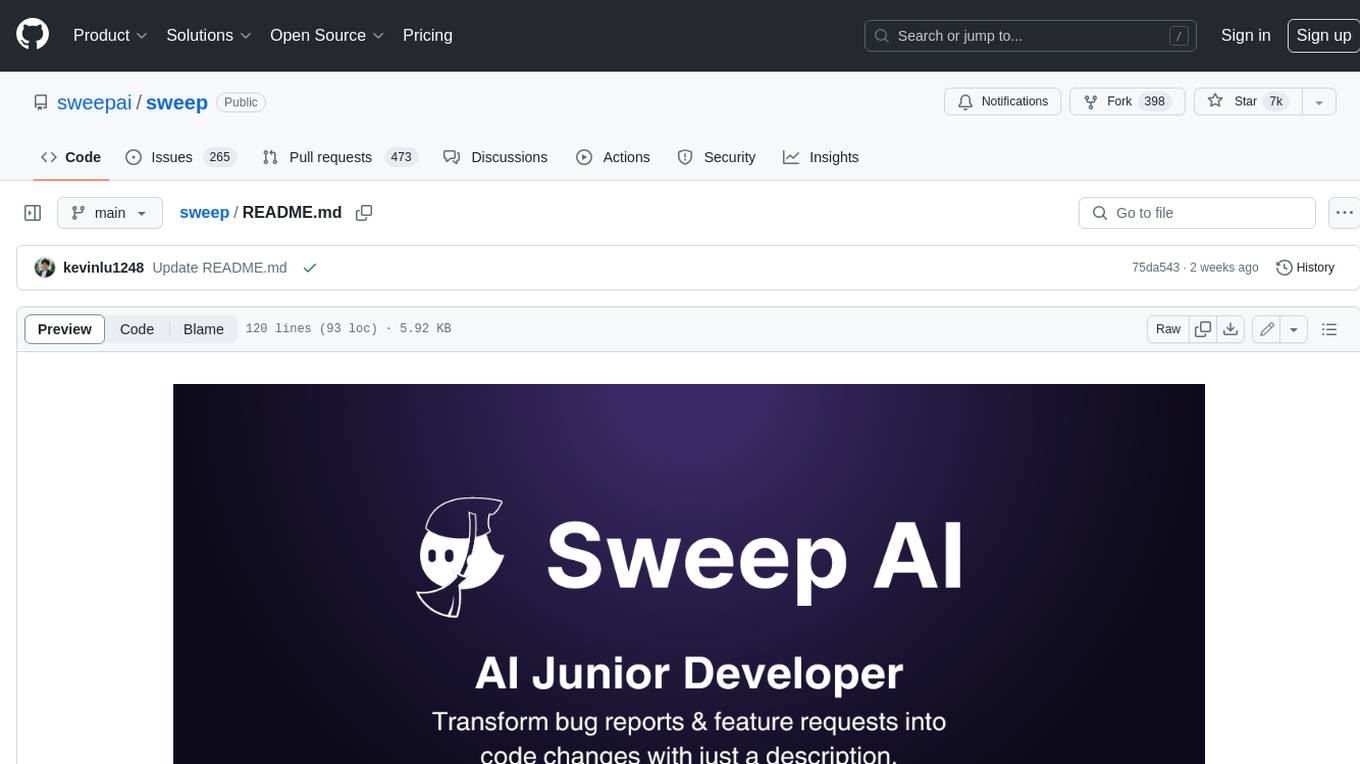
sweep
Sweep is an AI junior developer that turns bugs and feature requests into code changes. It automatically handles developer experience improvements like adding type hints and improving test coverage.
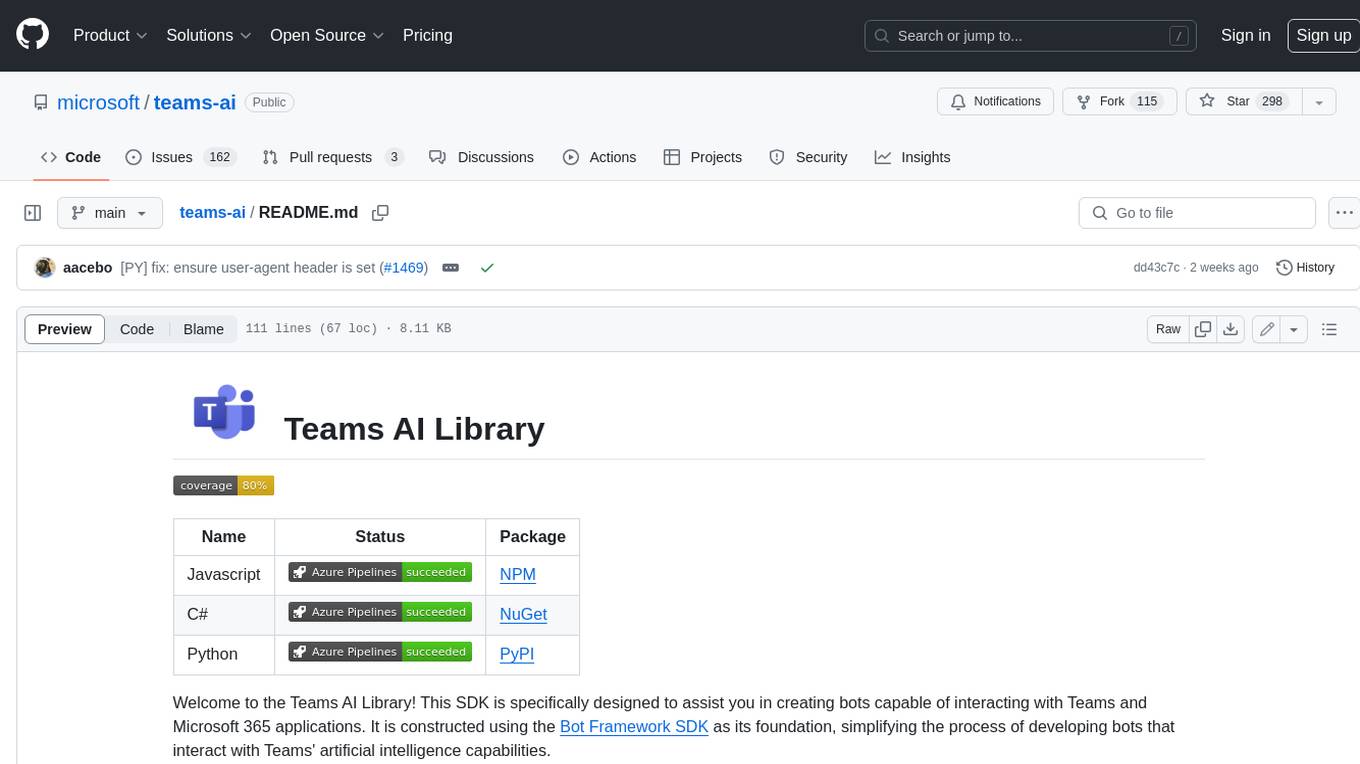
teams-ai
The Teams AI Library is a software development kit (SDK) that helps developers create bots that can interact with Teams and Microsoft 365 applications. It is built on top of the Bot Framework SDK and simplifies the process of developing bots that interact with Teams' artificial intelligence capabilities. The SDK is available for JavaScript/TypeScript, .NET, and Python.
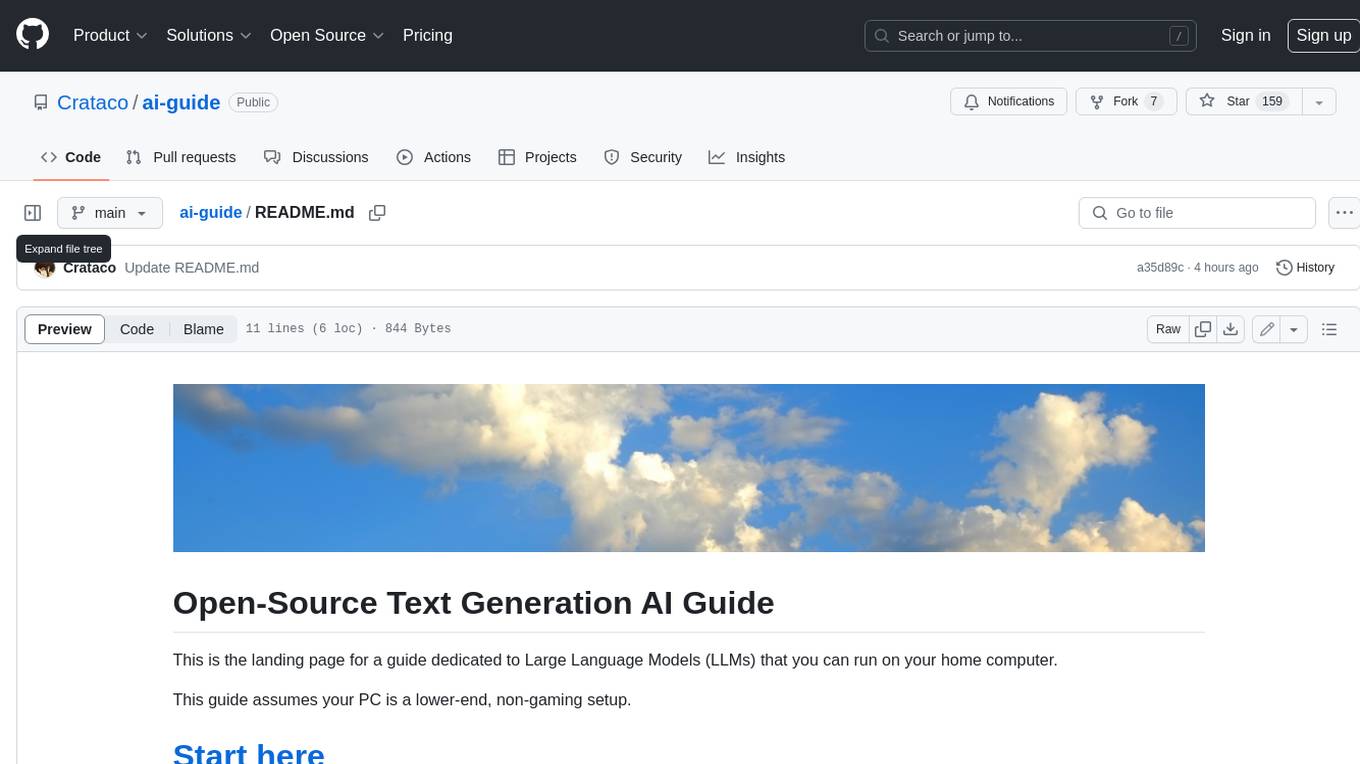
ai-guide
This guide is dedicated to Large Language Models (LLMs) that you can run on your home computer. It assumes your PC is a lower-end, non-gaming setup.
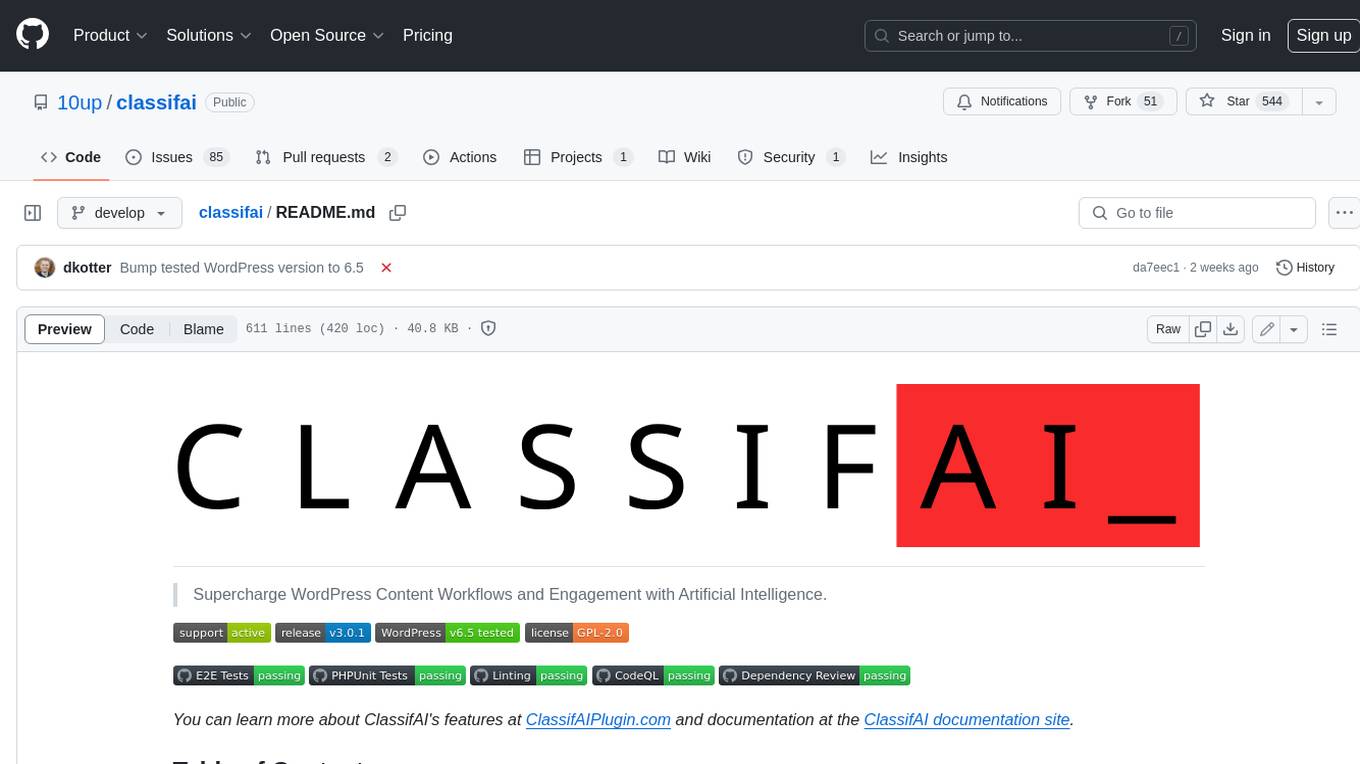
classifai
Supercharge WordPress Content Workflows and Engagement with Artificial Intelligence. Tap into leading cloud-based services like OpenAI, Microsoft Azure AI, Google Gemini and IBM Watson to augment your WordPress-powered websites. Publish content faster while improving SEO performance and increasing audience engagement. ClassifAI integrates Artificial Intelligence and Machine Learning technologies to lighten your workload and eliminate tedious tasks, giving you more time to create original content that matters.
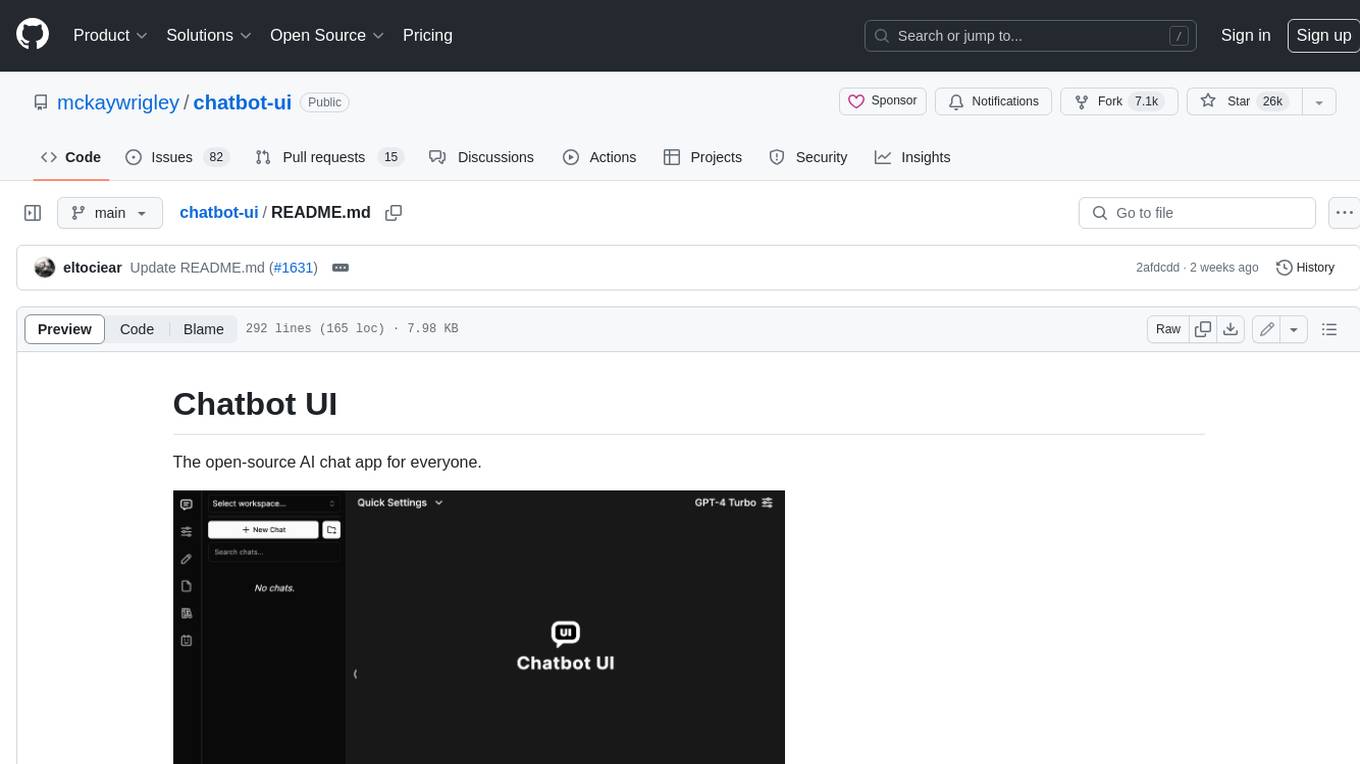
chatbot-ui
Chatbot UI is an open-source AI chat app that allows users to create and deploy their own AI chatbots. It is easy to use and can be customized to fit any need. Chatbot UI is perfect for businesses, developers, and anyone who wants to create a chatbot.
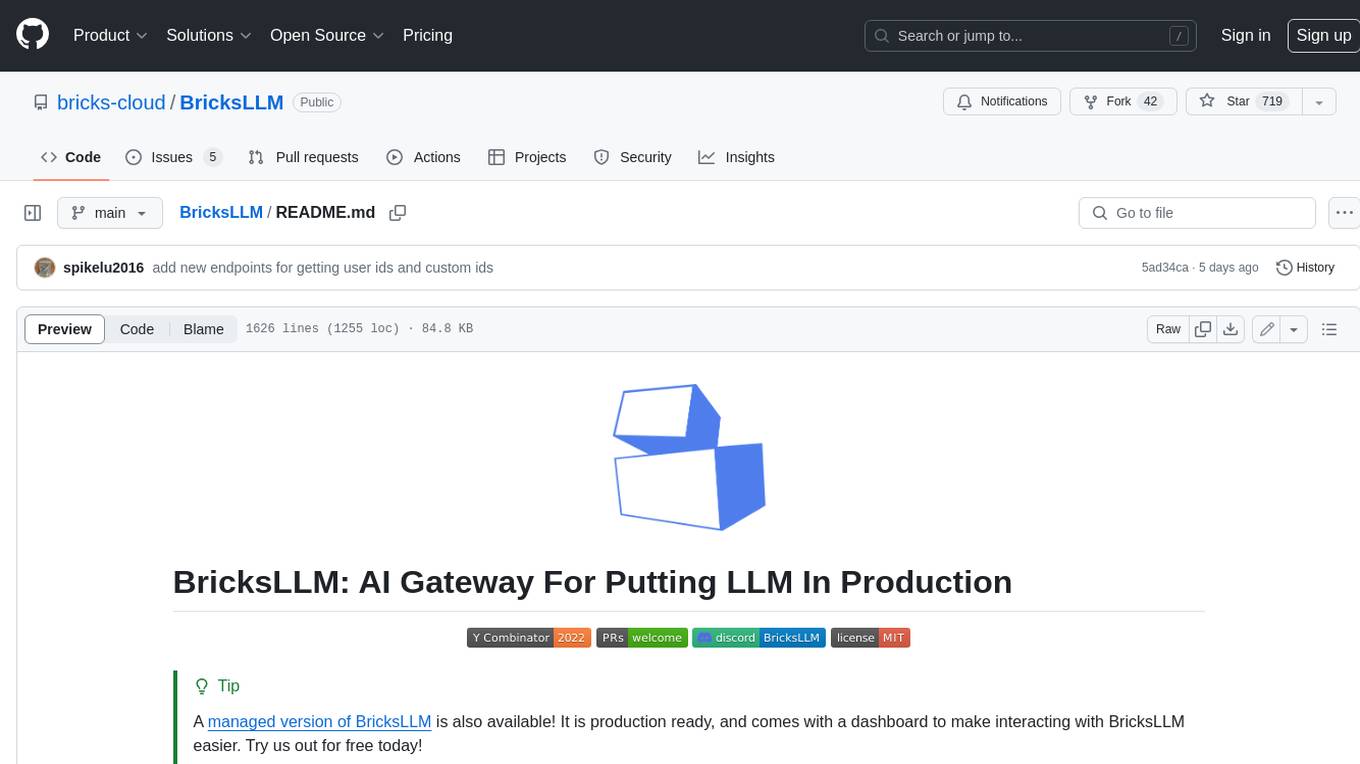
BricksLLM
BricksLLM is a cloud native AI gateway written in Go. Currently, it provides native support for OpenAI, Anthropic, Azure OpenAI and vLLM. BricksLLM aims to provide enterprise level infrastructure that can power any LLM production use cases. Here are some use cases for BricksLLM: * Set LLM usage limits for users on different pricing tiers * Track LLM usage on a per user and per organization basis * Block or redact requests containing PIIs * Improve LLM reliability with failovers, retries and caching * Distribute API keys with rate limits and cost limits for internal development/production use cases * Distribute API keys with rate limits and cost limits for students
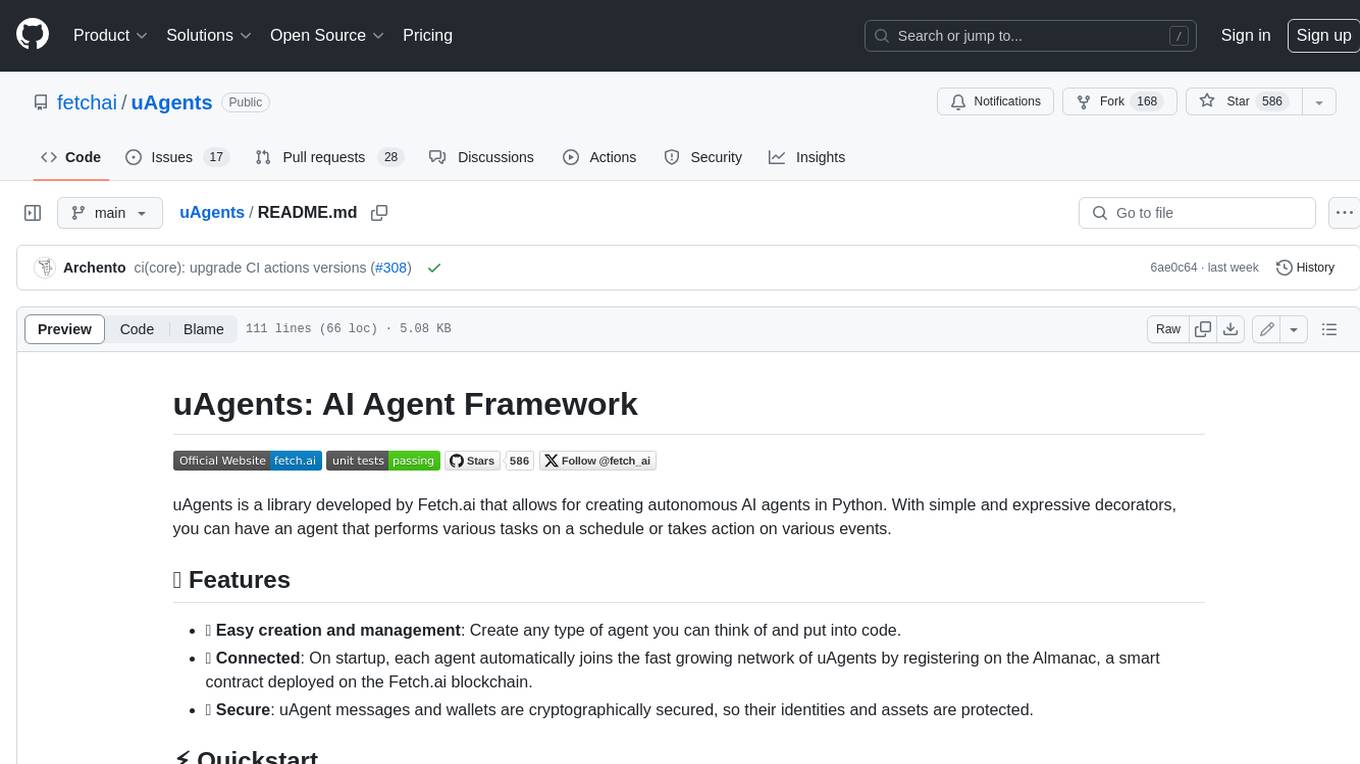
uAgents
uAgents is a Python library developed by Fetch.ai that allows for the creation of autonomous AI agents. These agents can perform various tasks on a schedule or take action on various events. uAgents are easy to create and manage, and they are connected to a fast-growing network of other uAgents. They are also secure, with cryptographically secured messages and wallets.
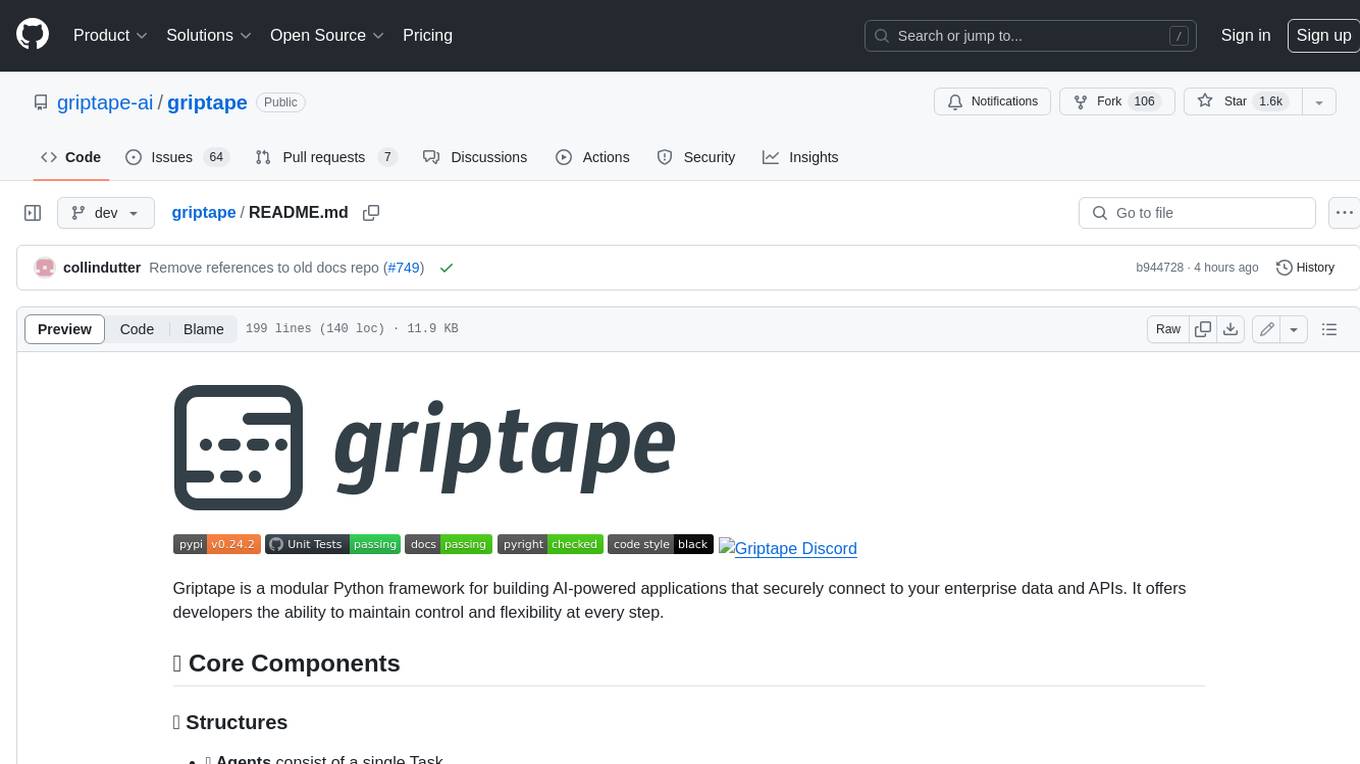
griptape
Griptape is a modular Python framework for building AI-powered applications that securely connect to your enterprise data and APIs. It offers developers the ability to maintain control and flexibility at every step. Griptape's core components include Structures (Agents, Pipelines, and Workflows), Tasks, Tools, Memory (Conversation Memory, Task Memory, and Meta Memory), Drivers (Prompt and Embedding Drivers, Vector Store Drivers, Image Generation Drivers, Image Query Drivers, SQL Drivers, Web Scraper Drivers, and Conversation Memory Drivers), Engines (Query Engines, Extraction Engines, Summary Engines, Image Generation Engines, and Image Query Engines), and additional components (Rulesets, Loaders, Artifacts, Chunkers, and Tokenizers). Griptape enables developers to create AI-powered applications with ease and efficiency.