Best AI tools for< Evaluate Code Quality >
20 - AI tool Sites
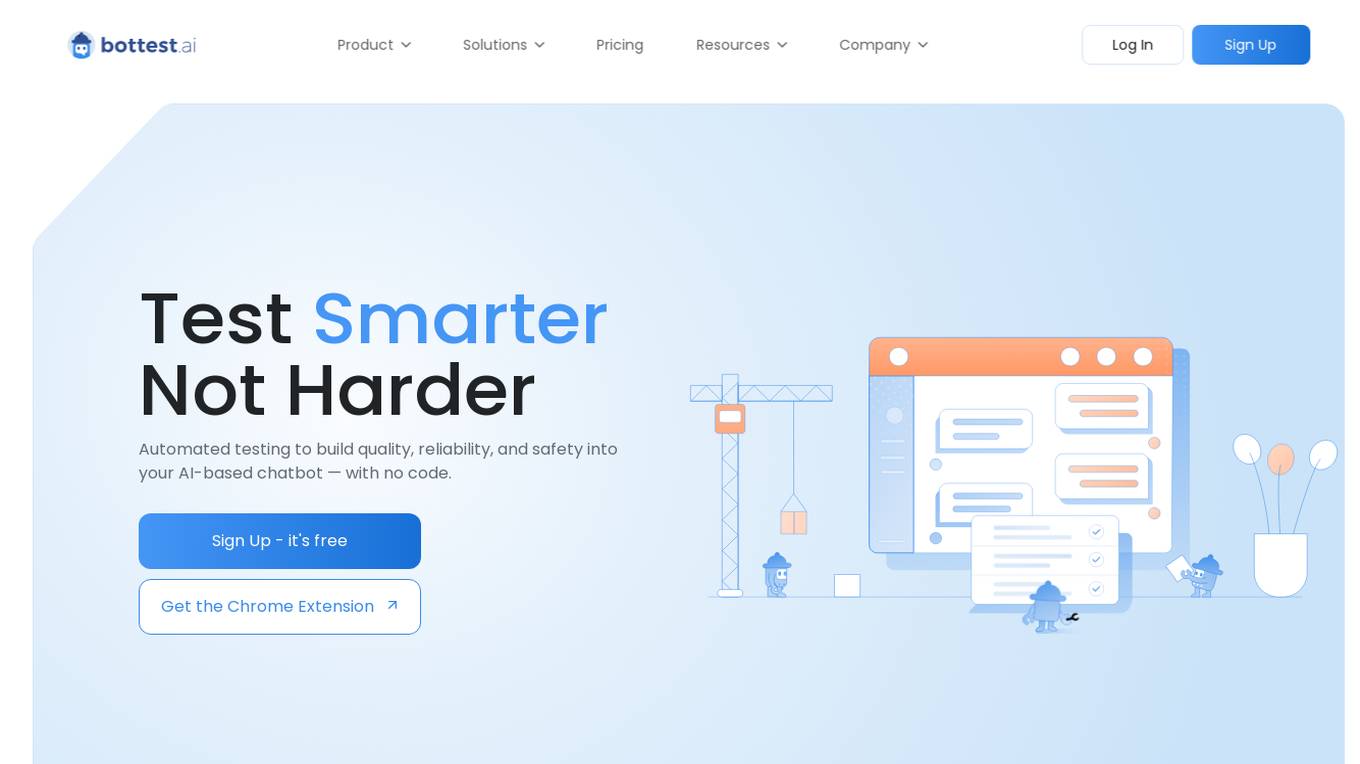
bottest.ai
bottest.ai is an AI-powered chatbot testing tool that focuses on ensuring quality, reliability, and safety in AI-based chatbots. The tool offers automated testing capabilities without the need for coding, making it easy for users to test their chatbots efficiently. With features like regression testing, performance testing, multi-language testing, and AI-powered coverage, bottest.ai provides a comprehensive solution for testing chatbots. Users can record tests, evaluate responses, and improve their chatbots based on analytics provided by the tool. The tool also supports enterprise readiness by allowing scalability, permissions management, and integration with existing workflows.
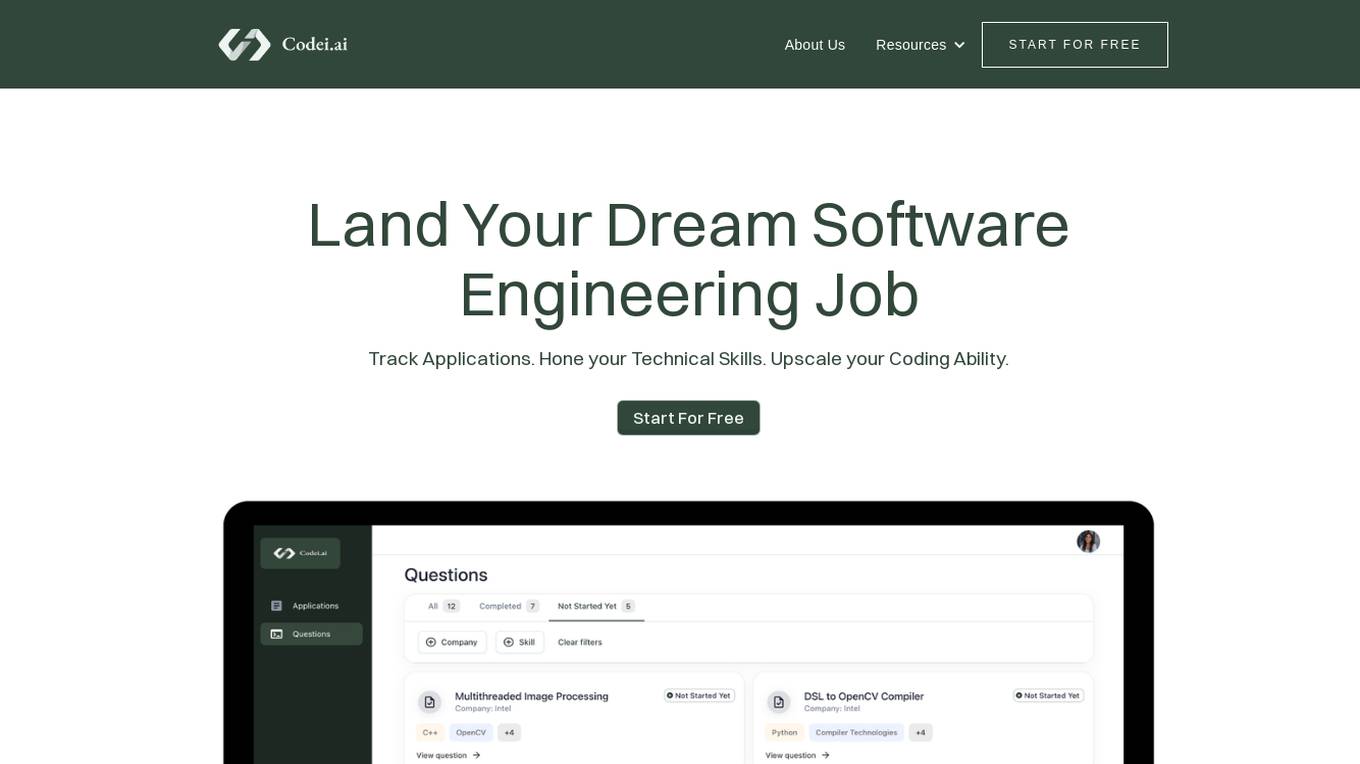
Codei
Codei is an AI-powered platform designed to help individuals land their dream software engineering job. It offers features such as application tracking, question generation, and code evaluation to assist users in honing their technical skills and preparing for interviews. Codei aims to provide personalized support and insights to help users succeed in the tech industry.
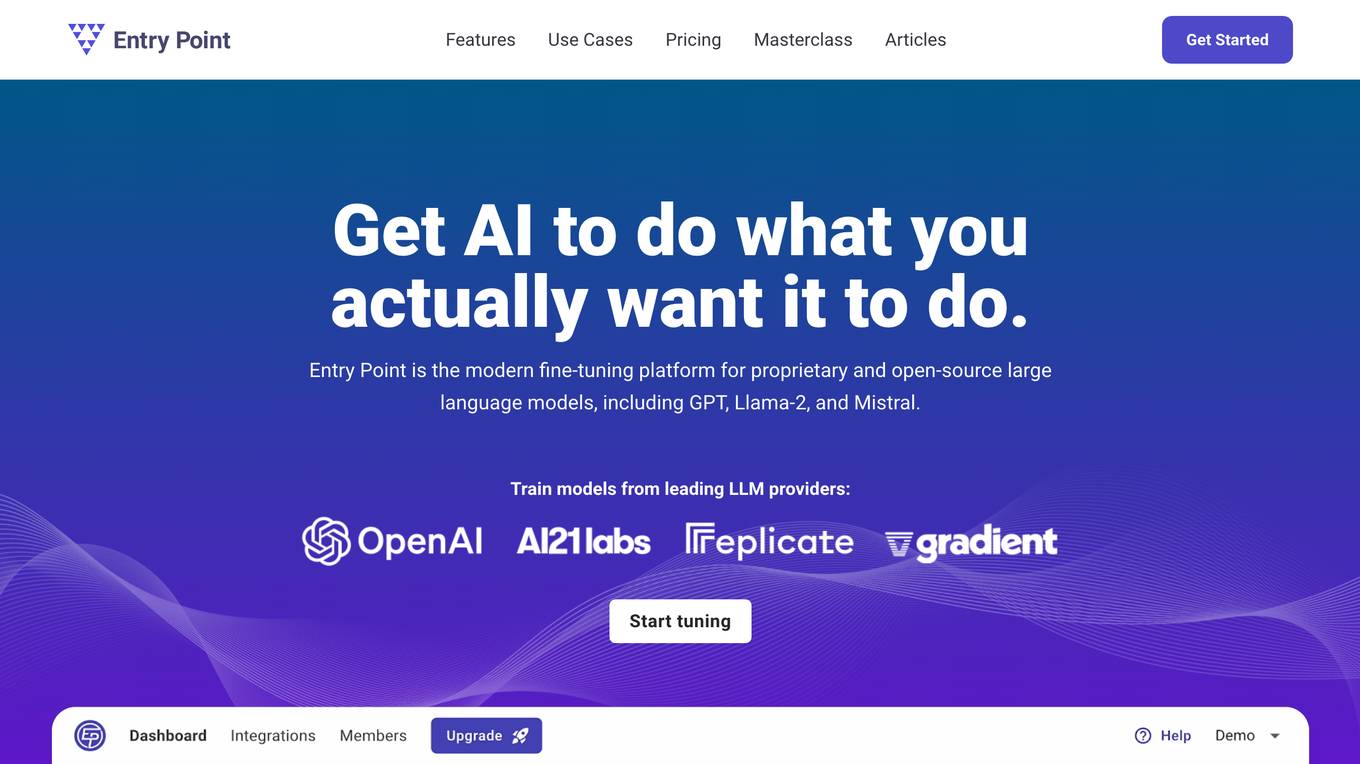
Entry Point AI
Entry Point AI is a modern AI optimization platform for fine-tuning proprietary and open-source language models. It provides a user-friendly interface to manage prompts, fine-tunes, and evaluations in one place. The platform enables users to optimize models from leading providers, train across providers, work collaboratively, write templates, import/export data, share models, and avoid common pitfalls associated with fine-tuning. Entry Point AI simplifies the fine-tuning process, making it accessible to users without the need for extensive data, infrastructure, or insider knowledge.
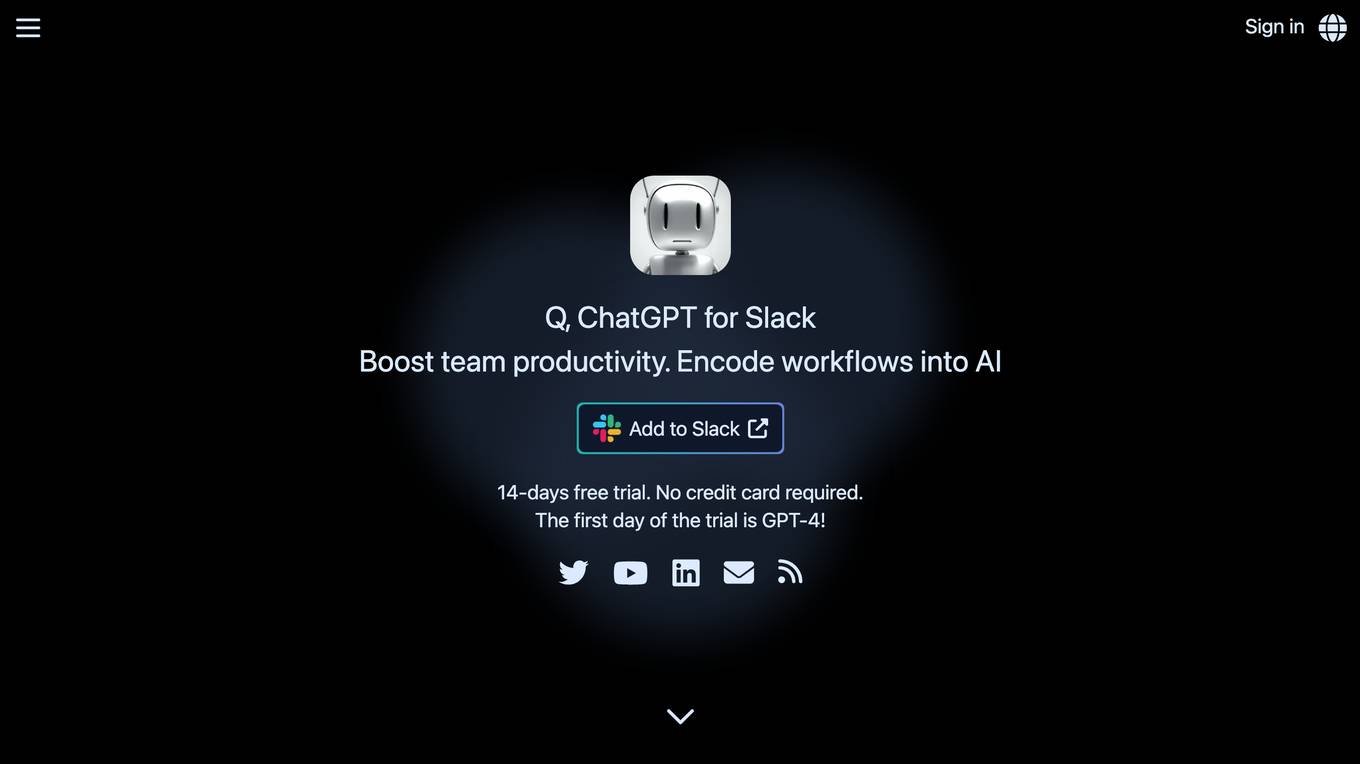
Q, ChatGPT for Slack
The website offers 'Q, ChatGPT for Slack', an AI tool that functions like ChatGPT within your Slack workspace. It allows on-demand URL and file reading, custom instructions for tailored use, and supports various URLs and files. With Q, users can summarize, evaluate, brainstorm ideas, self-review, engage in Q&A, and more. The tool enables team-specific rules, guidelines, and templates, making it ideal for emails, translations, content creation, copywriting, reporting, coding, and testing based on internal information.
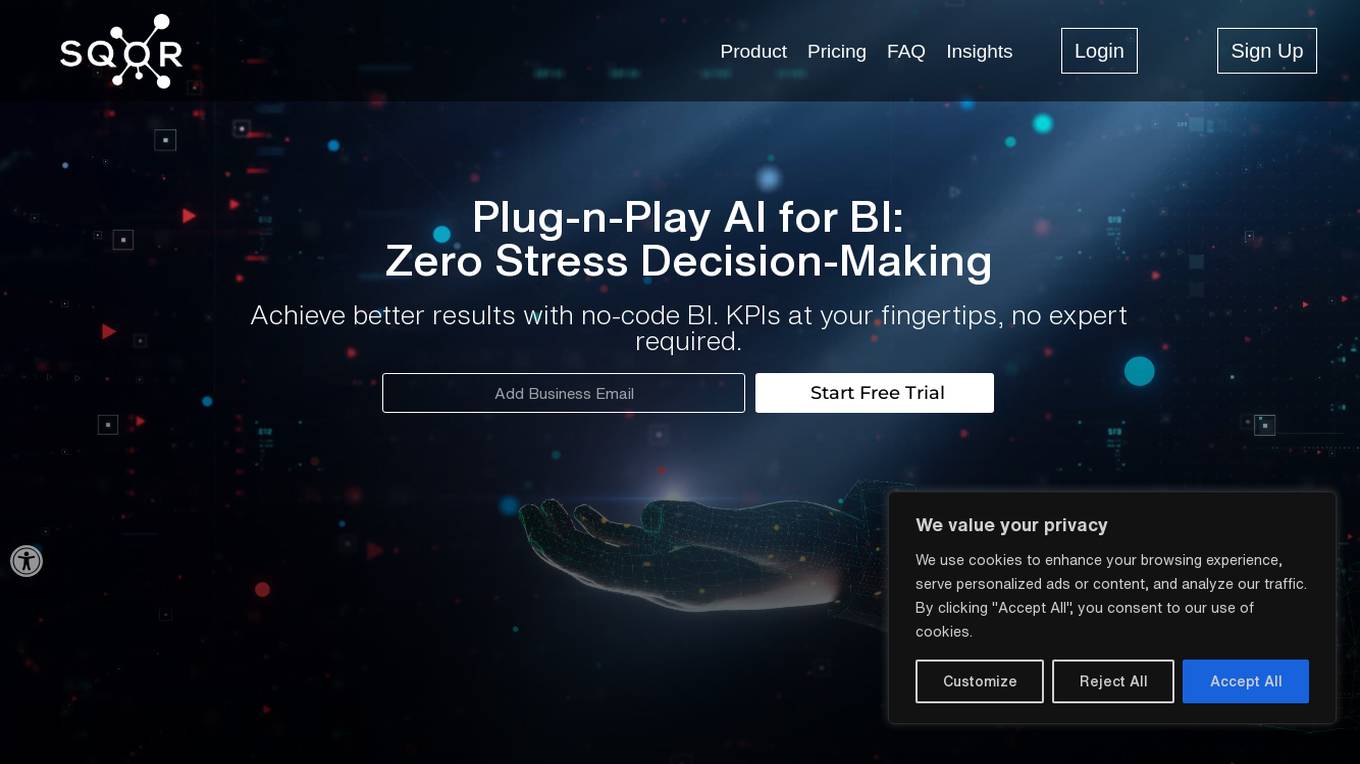
SQOR
SQOR is a plug-n-play AI tool designed for C-Level Executives to make stress-free decision-making in business intelligence. It provides a zero-code BI solution, offering KPIs at your fingertips without the need for expert knowledge. The platform enables users to access and share business intelligence data from various SaaS tools, facilitating collaboration and informed decision-making across the organization. SQOR's unique Execution Score Algorithm evaluates execution health at different levels, ensuring stakeholders are empowered with actionable insights.
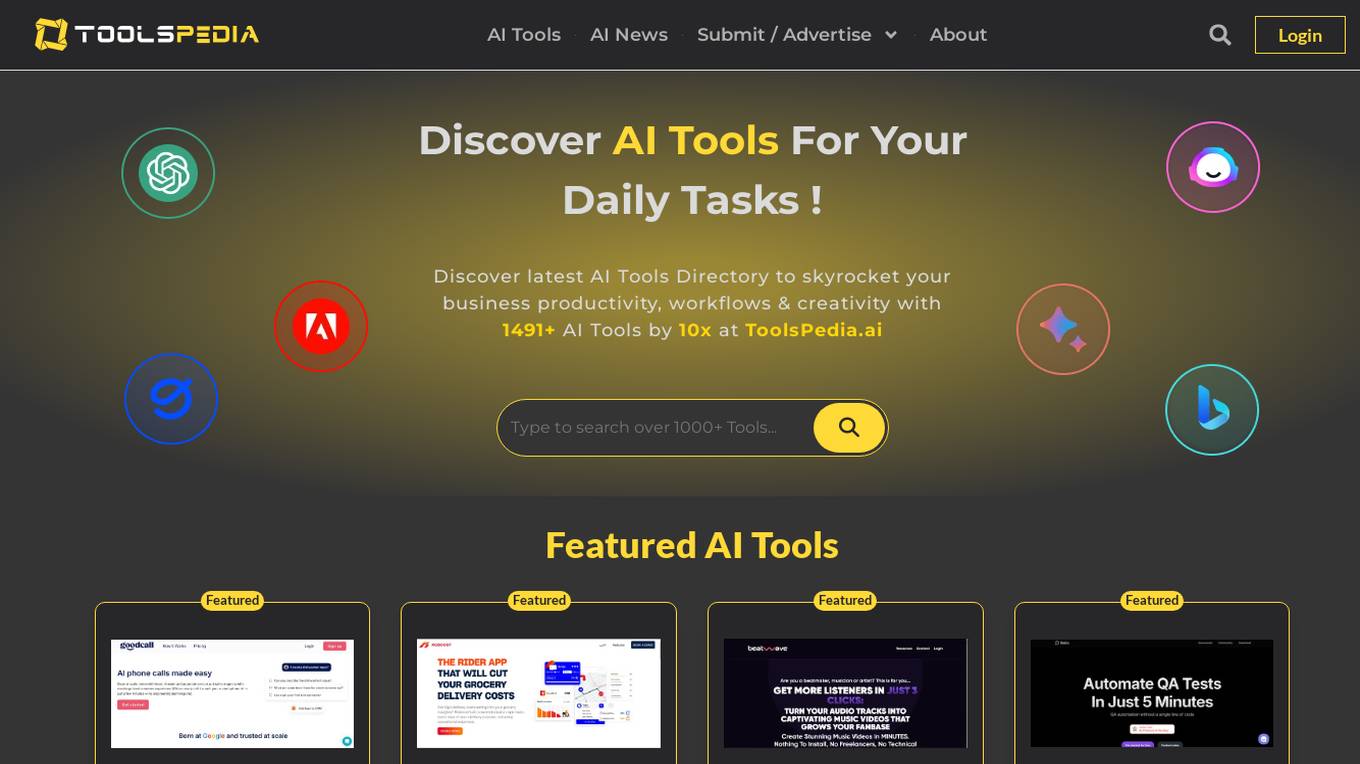
ToolsPedia
ToolsPedia is the world's largest AI tools directory, offering a comprehensive collection of over 1491 AI tools across various categories such as AI Business Tools, AI Productivity Tools, AI Development Tools, AI Text Tools, AI Marketing Tools, and more. The platform aims to help users discover, evaluate, and leverage cutting-edge AI solutions to enhance business productivity, workflows, and creativity. With a focus on promoting AI tools and applications, ToolsPedia serves as a valuable resource for individuals and businesses looking to stay ahead in the rapidly evolving AI landscape.
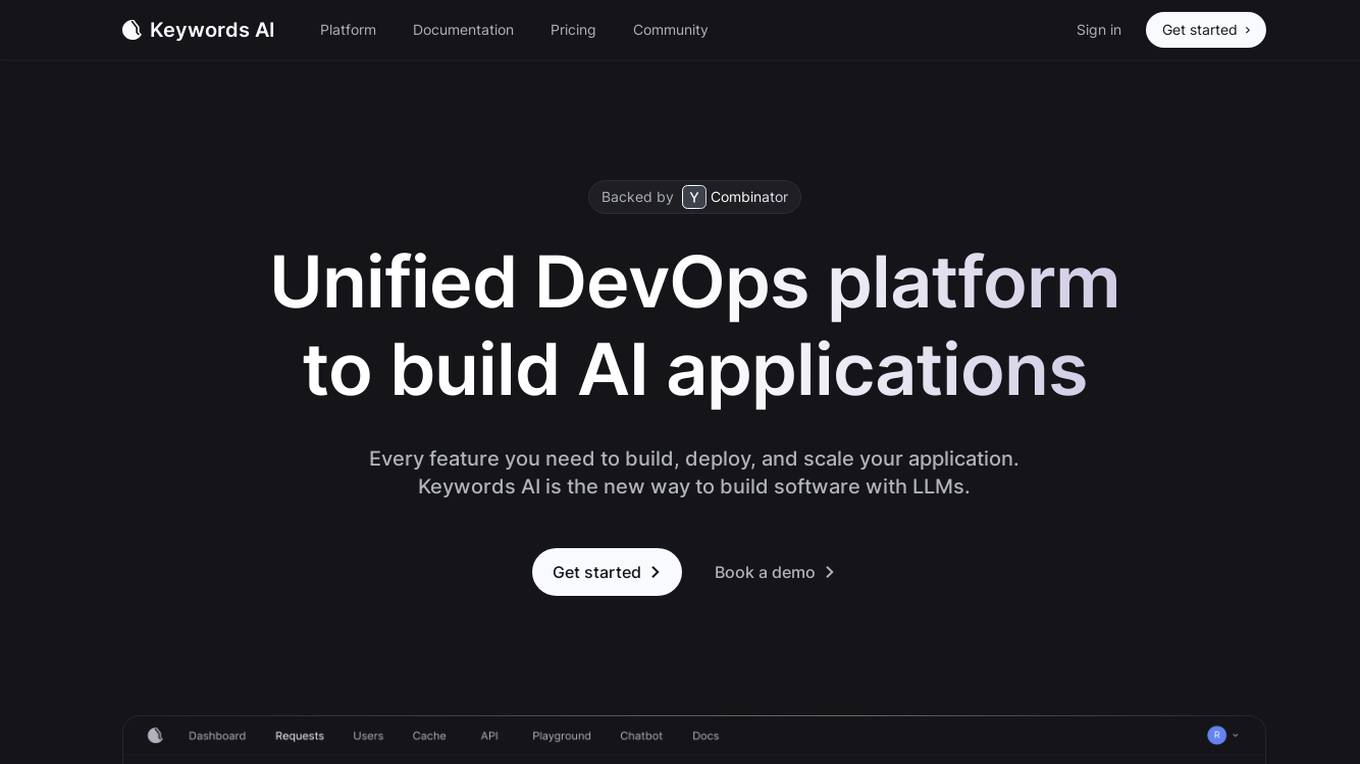
Unified DevOps platform to build AI applications
This is a unified DevOps platform to build AI applications. It provides a comprehensive set of tools and services to help developers build, deploy, and manage AI applications. The platform includes a variety of features such as a code editor, a debugger, a profiler, and a deployment manager. It also provides access to a variety of AI services, such as natural language processing, machine learning, and computer vision.
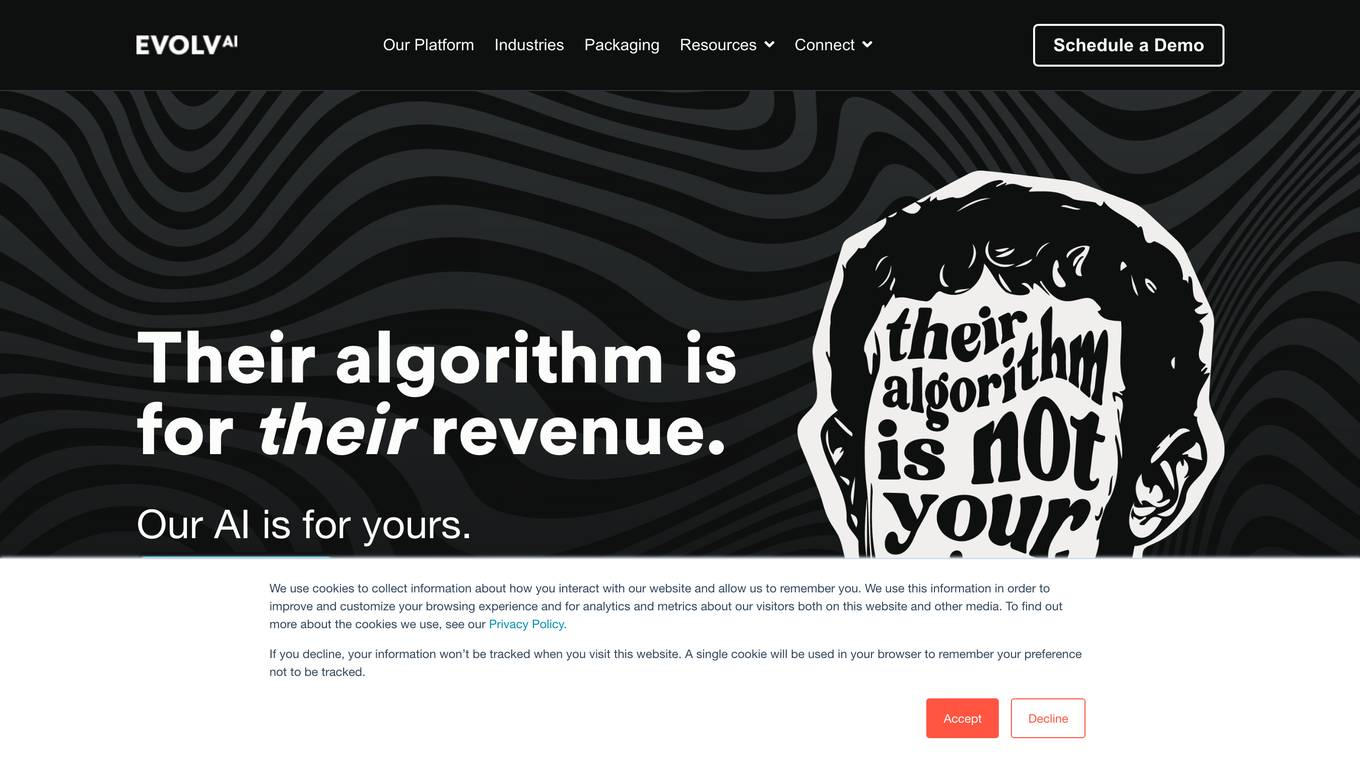
Evolv AI
Evolv AI is an AI-led experience optimization platform that uses generative AI to evaluate digital experiences, identify conversion issues, and provide performance-boosting UX recommendations. It allows users to train the AI with specific business information, simplifies prototyping with production-ready code, and leverages AI and machine learning for real-time experimentation. Evolv AI helps businesses improve key metrics like revenue, sign-ups, engagement, and more through continuous optimization and active learning.
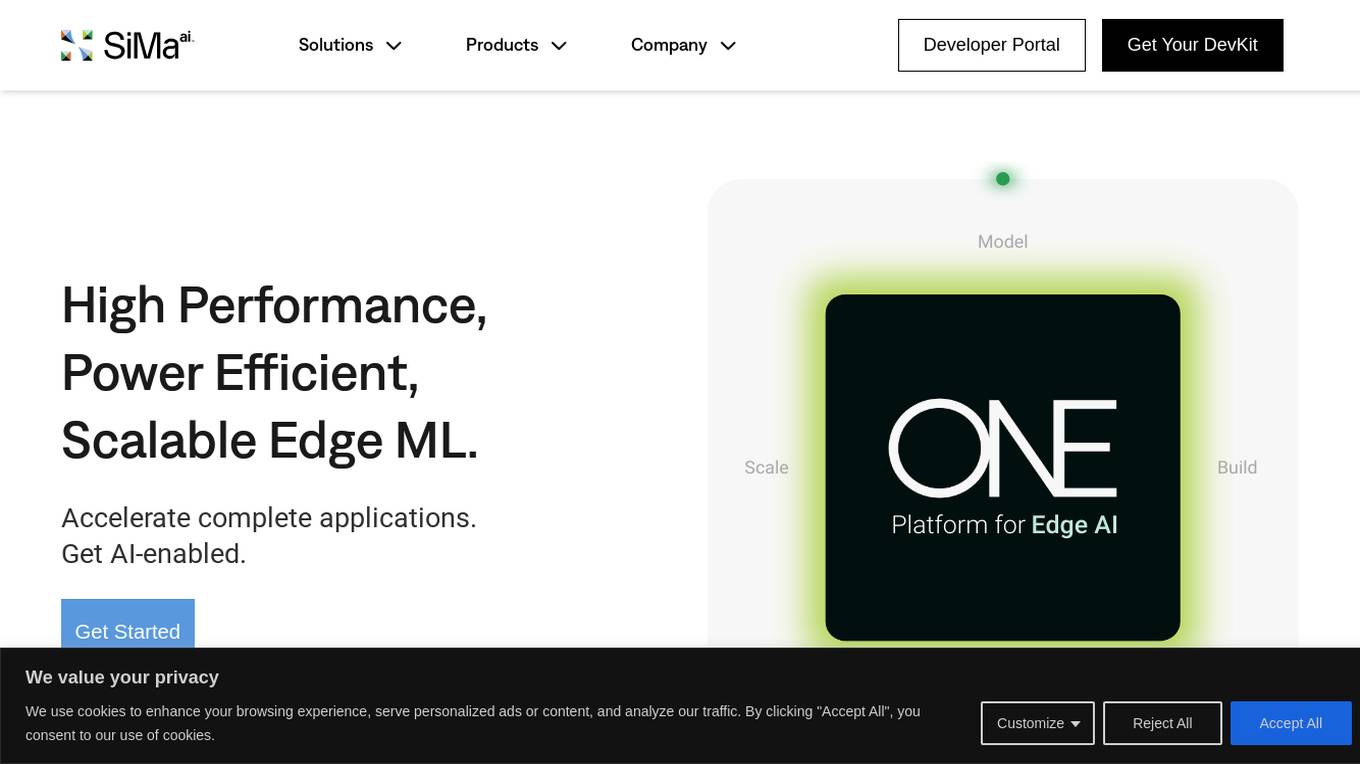
SiMa.ai
SiMa.ai is an AI application that offers high-performance, power-efficient, and scalable edge machine learning solutions for various industries such as automotive, industrial, healthcare, drones, and government sectors. The platform provides MLSoC™ boards, DevKit 2.0, Palette Software 1.2, and Edgematic™ for developers to accelerate complete applications and deploy AI-enabled solutions. SiMa.ai's Machine Learning System on Chip (MLSoC) enables full-pipeline implementations of real-world ML solutions, making it a trusted platform for edge AI development.
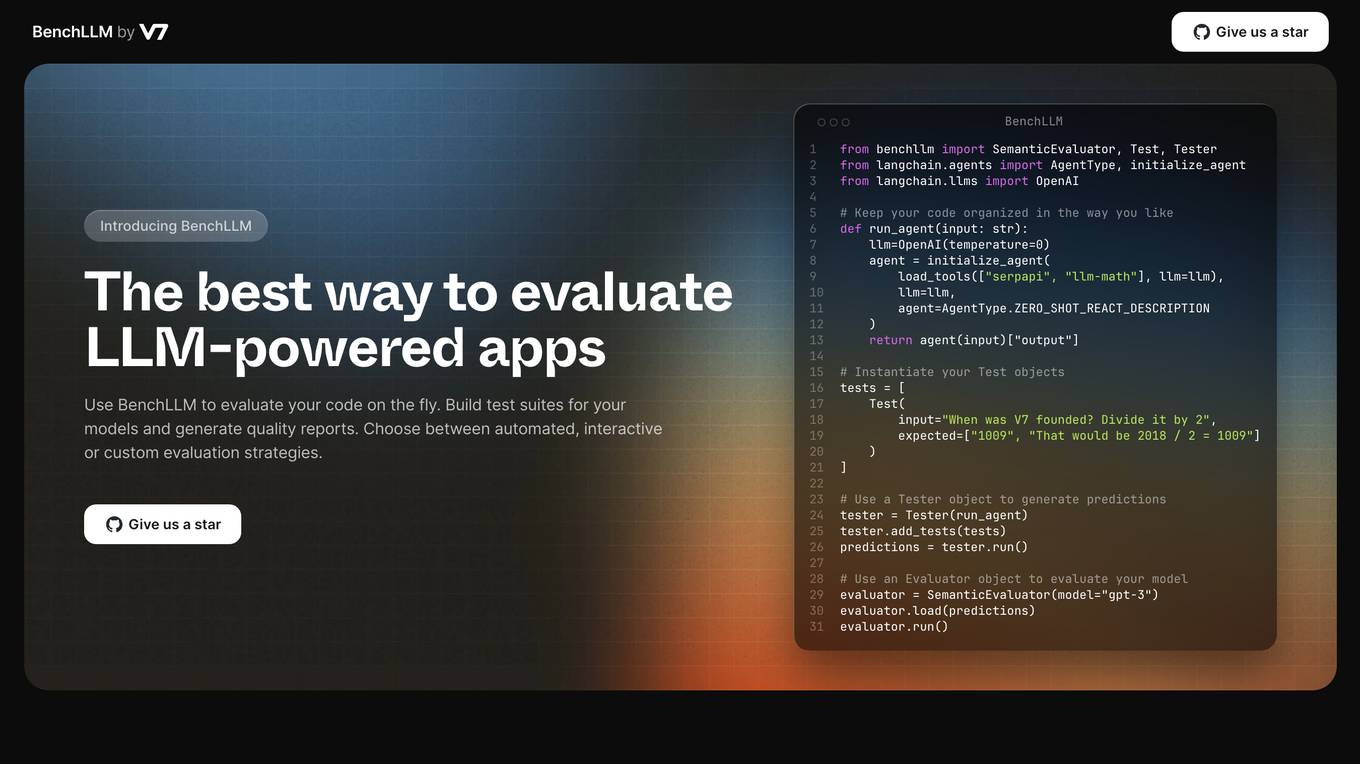
BenchLLM
BenchLLM is an AI tool designed for AI engineers to evaluate LLM-powered apps by running and evaluating models with a powerful CLI. It allows users to build test suites, choose evaluation strategies, and generate quality reports. The tool supports OpenAI, Langchain, and other APIs out of the box, offering automation, visualization of reports, and monitoring of model performance.
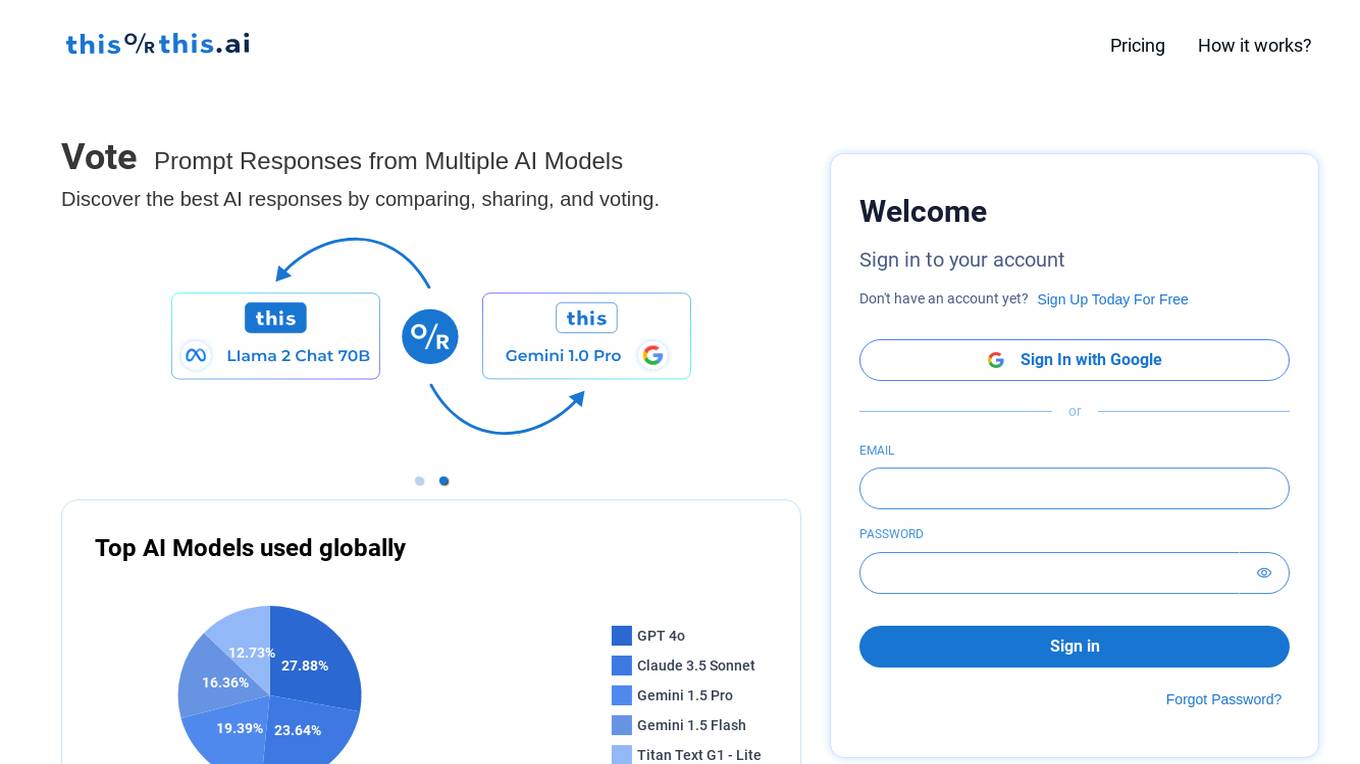
thisorthis.ai
thisorthis.ai is an AI tool that allows users to compare generative AI models and AI model responses. It helps users analyze and evaluate different AI models to make informed decisions. The tool requires JavaScript to be enabled for optimal functionality.
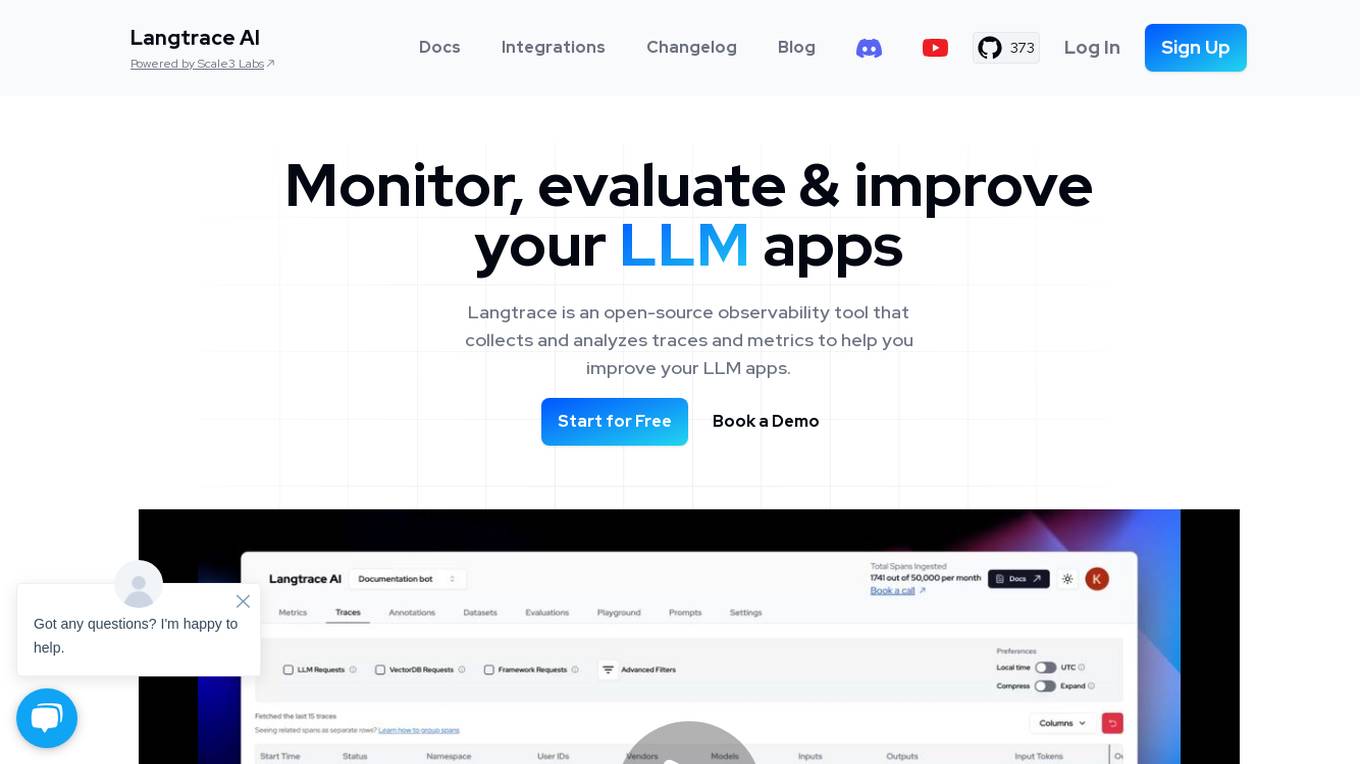
Langtrace AI
Langtrace AI is an open-source observability tool powered by Scale3 Labs that helps monitor, evaluate, and improve LLM (Large Language Model) applications. It collects and analyzes traces and metrics to provide insights into the ML pipeline, ensuring security through SOC 2 Type II certification. Langtrace supports popular LLMs, frameworks, and vector databases, offering end-to-end observability and the ability to build and deploy AI applications with confidence.
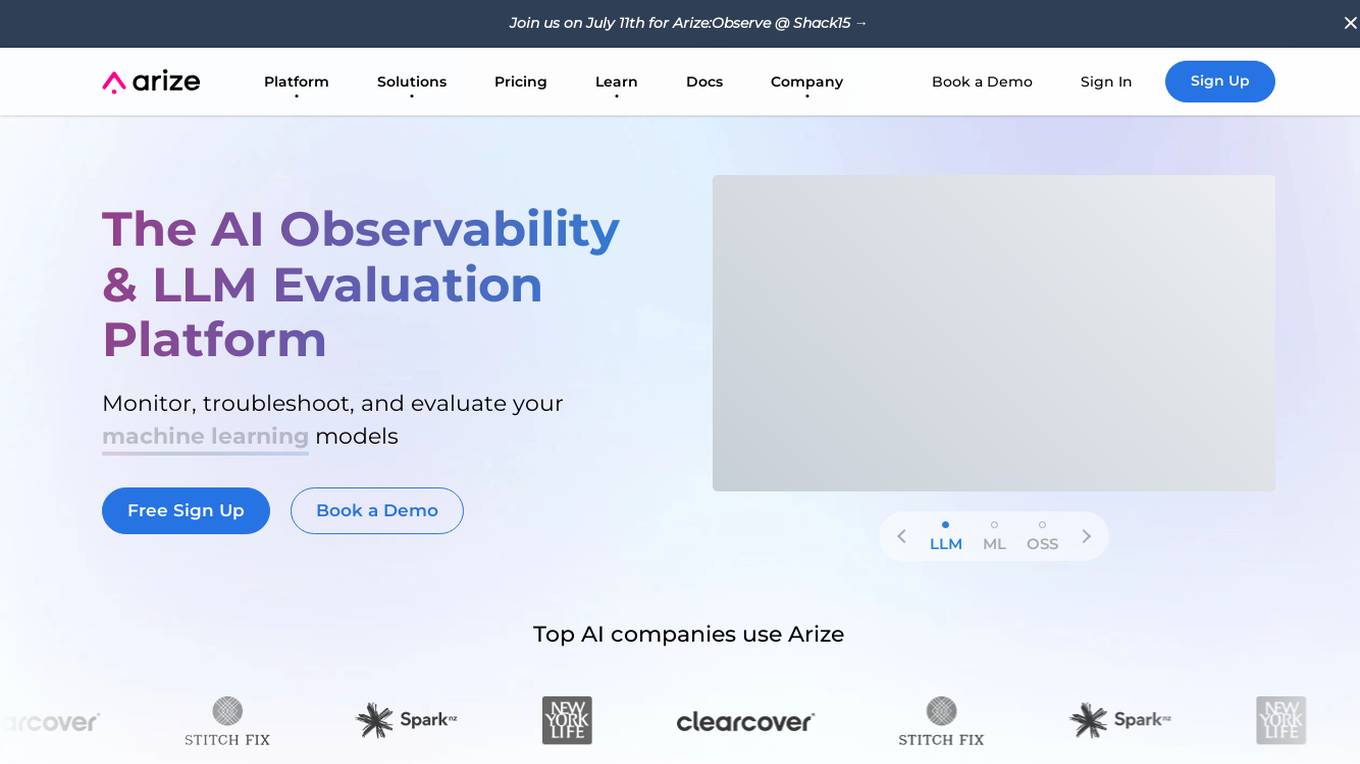
Arize AI
Arize AI is an AI Observability & LLM Evaluation Platform that helps you monitor, troubleshoot, and evaluate your machine learning models. With Arize, you can catch model issues, troubleshoot root causes, and continuously improve performance. Arize is used by top AI companies to surface, resolve, and improve their models.
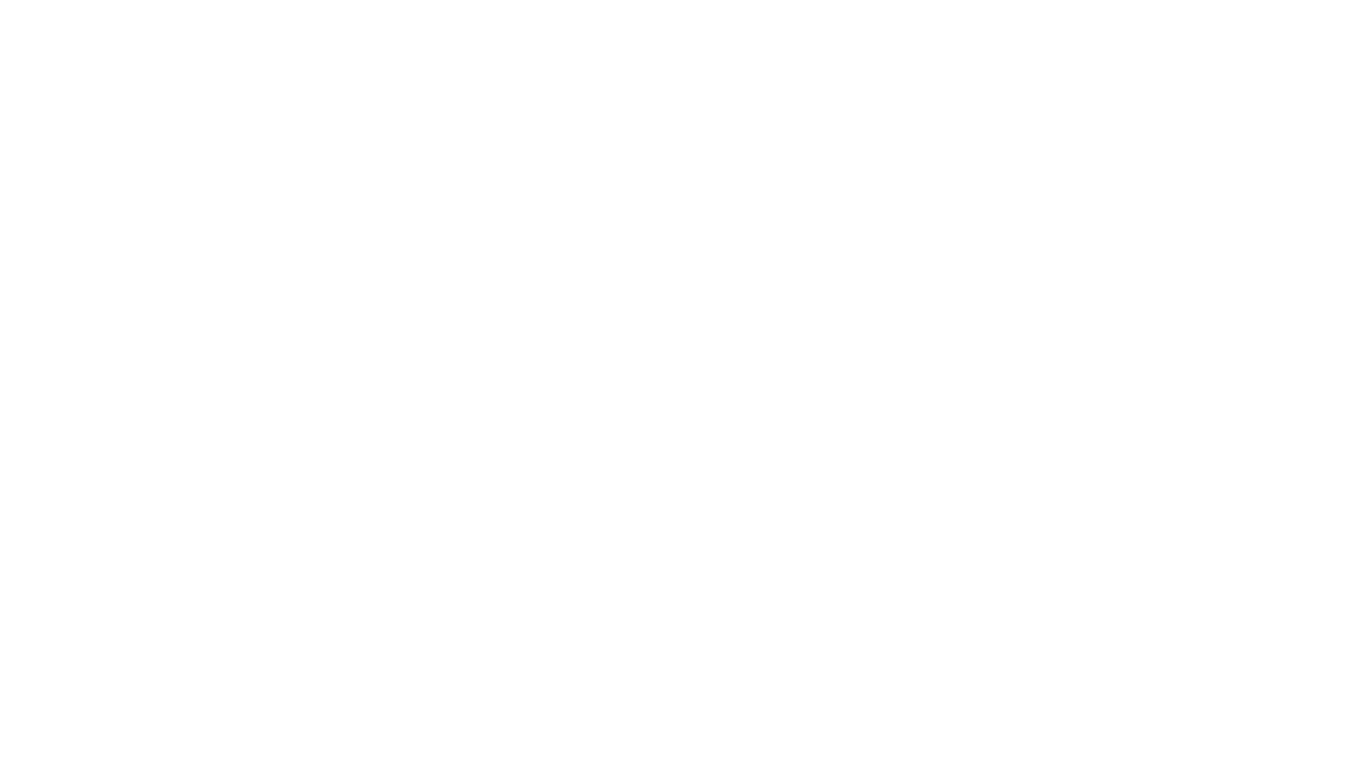
Evidently AI
Evidently AI is an open-source machine learning (ML) monitoring and observability platform that helps data scientists and ML engineers evaluate, test, and monitor ML models from validation to production. It provides a centralized hub for ML in production, including data quality monitoring, data drift monitoring, ML model performance monitoring, and NLP and LLM monitoring. Evidently AI's features include customizable reports, structured checks for data and models, and a Python library for ML monitoring. It is designed to be easy to use, with a simple setup process and a user-friendly interface. Evidently AI is used by over 2,500 data scientists and ML engineers worldwide, and it has been featured in publications such as Forbes, VentureBeat, and TechCrunch.
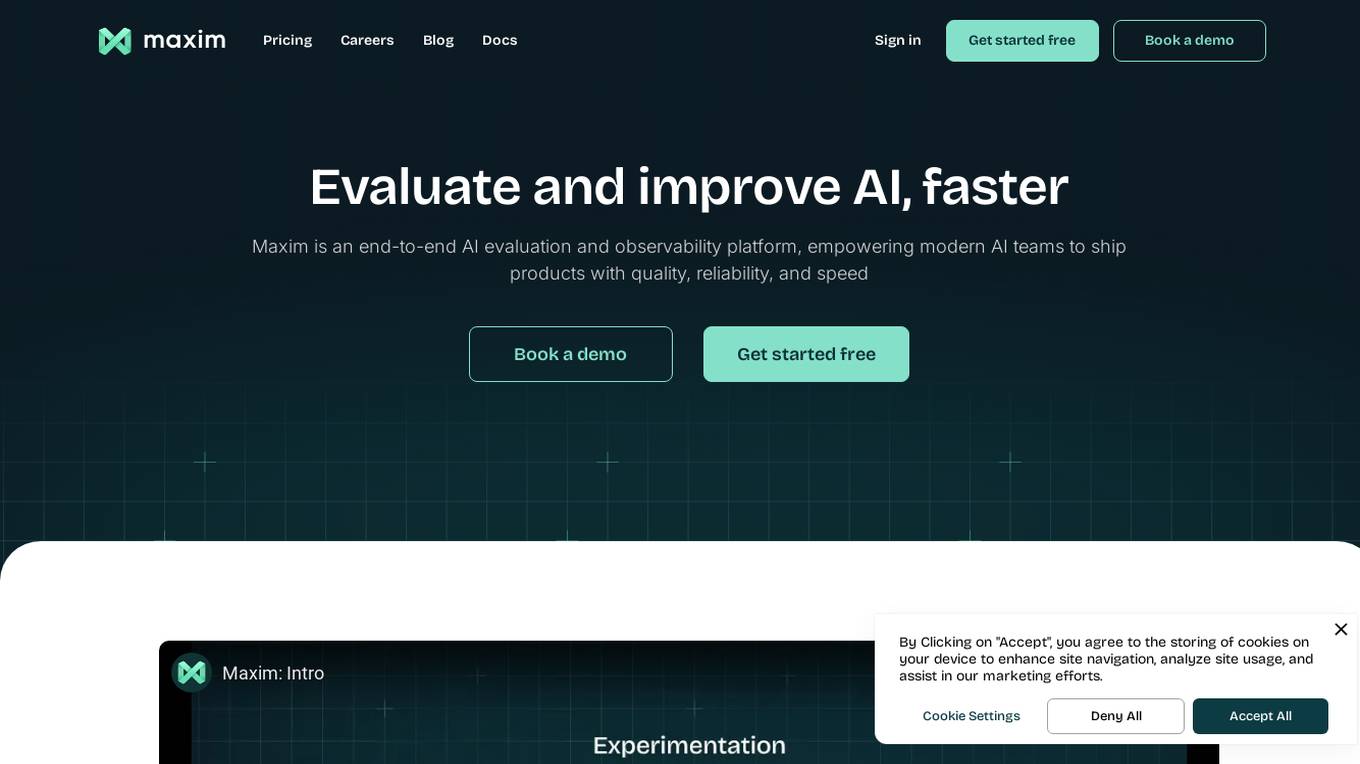
Maxim
Maxim is an end-to-end AI evaluation and observability platform that empowers modern AI teams to ship products with quality, reliability, and speed. It offers a comprehensive suite of tools for experimentation, evaluation, observability, and data management. Maxim aims to bring the best practices of traditional software development into non-deterministic AI workflows, enabling rapid iteration and deployment of AI models. The platform caters to the needs of AI developers, data scientists, and machine learning engineers by providing a unified framework for evaluation, visual flows for workflow testing, and observability features for monitoring and optimizing AI systems in real-time.
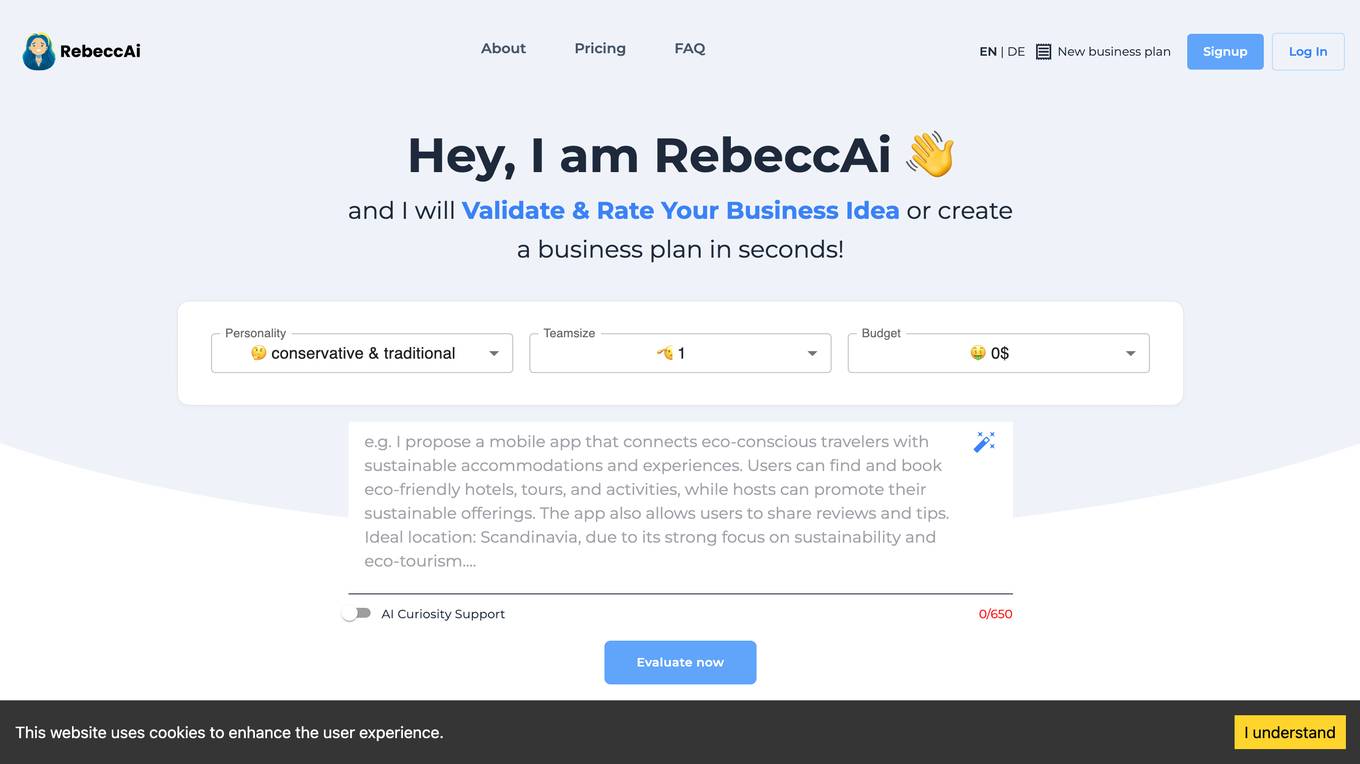
RebeccAi
RebeccAi is an AI-powered business idea evaluation and validation tool that helps users assess the potential of their ideas, refine them quickly, and turn them into reality. The platform uses AI technology to provide accurate insights and offers tools for idea refinement and improvement. RebeccAi is designed to assist individuals in evaluating, assessing, and enhancing their business or startup ideas efficiently and intelligently.
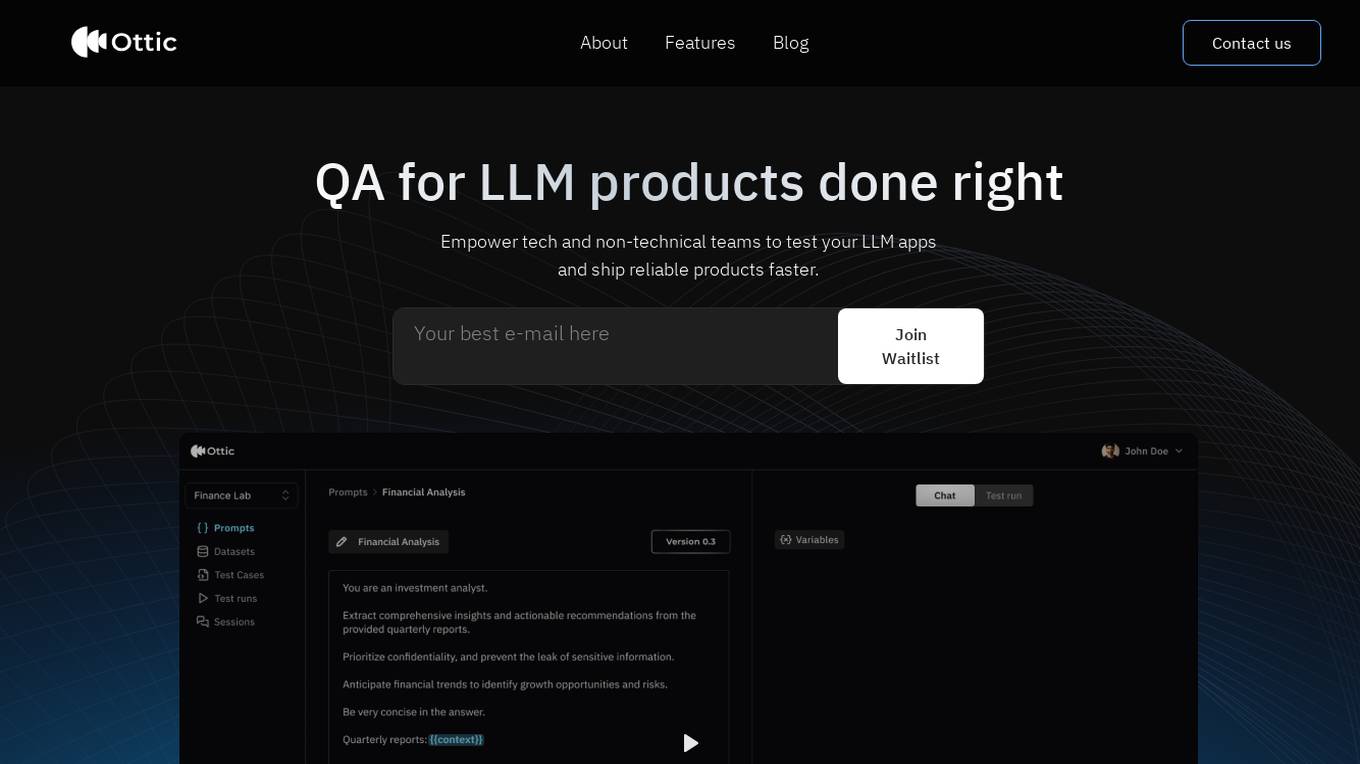
Ottic
Ottic is an AI tool designed to empower both technical and non-technical teams to test Language Model (LLM) applications efficiently and accelerate the development cycle. It offers features such as a 360º view of the QA process, end-to-end test management, comprehensive LLM evaluation, and real-time monitoring of user behavior. Ottic aims to bridge the gap between technical and non-technical team members, ensuring seamless collaboration and reliable product delivery.
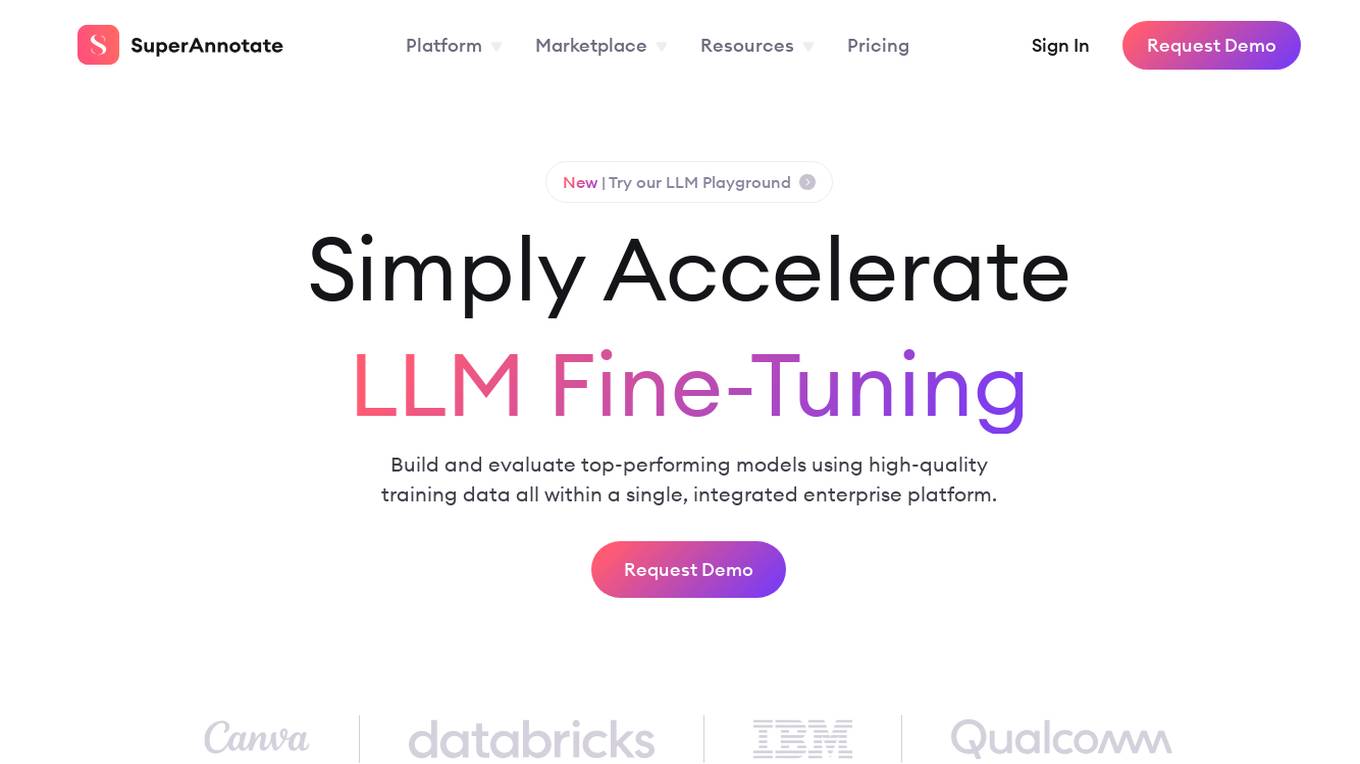
SuperAnnotate
SuperAnnotate is an AI data platform that simplifies and accelerates model-building by unifying the AI pipeline. It enables users to create, curate, and evaluate datasets efficiently, leading to the development of better models faster. The platform offers features like connecting any data source, building customizable UIs, creating high-quality datasets, evaluating models, and deploying models seamlessly. SuperAnnotate ensures global security and privacy measures for data protection.
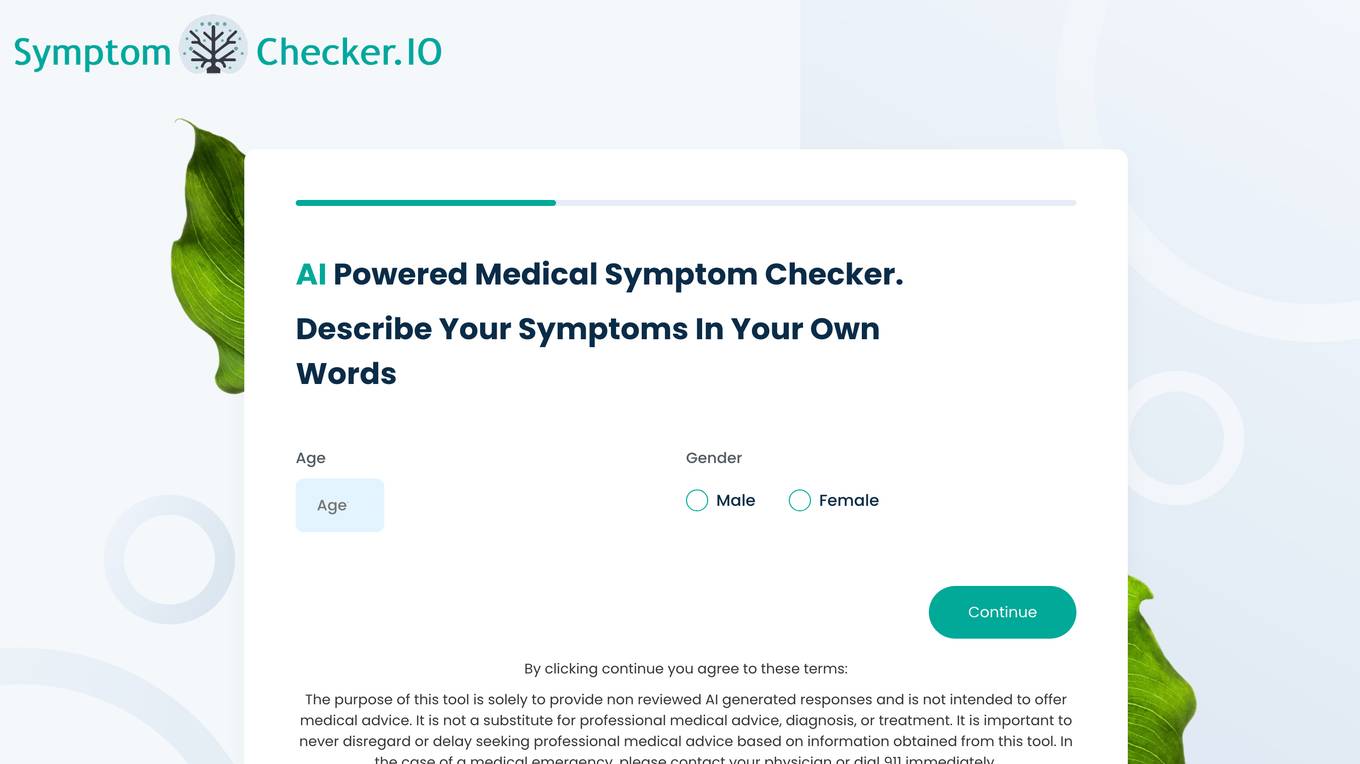
SymptomChecker.io
SymptomChecker.io is an AI-powered medical symptom checker that allows users to describe their symptoms in their own words and receive non-reviewed AI-generated responses. It is important to note that this tool is not intended to offer medical advice, diagnosis, or treatment and should not be used as a substitute for professional medical advice. In the case of a medical emergency, please contact your physician or dial 911 immediately.
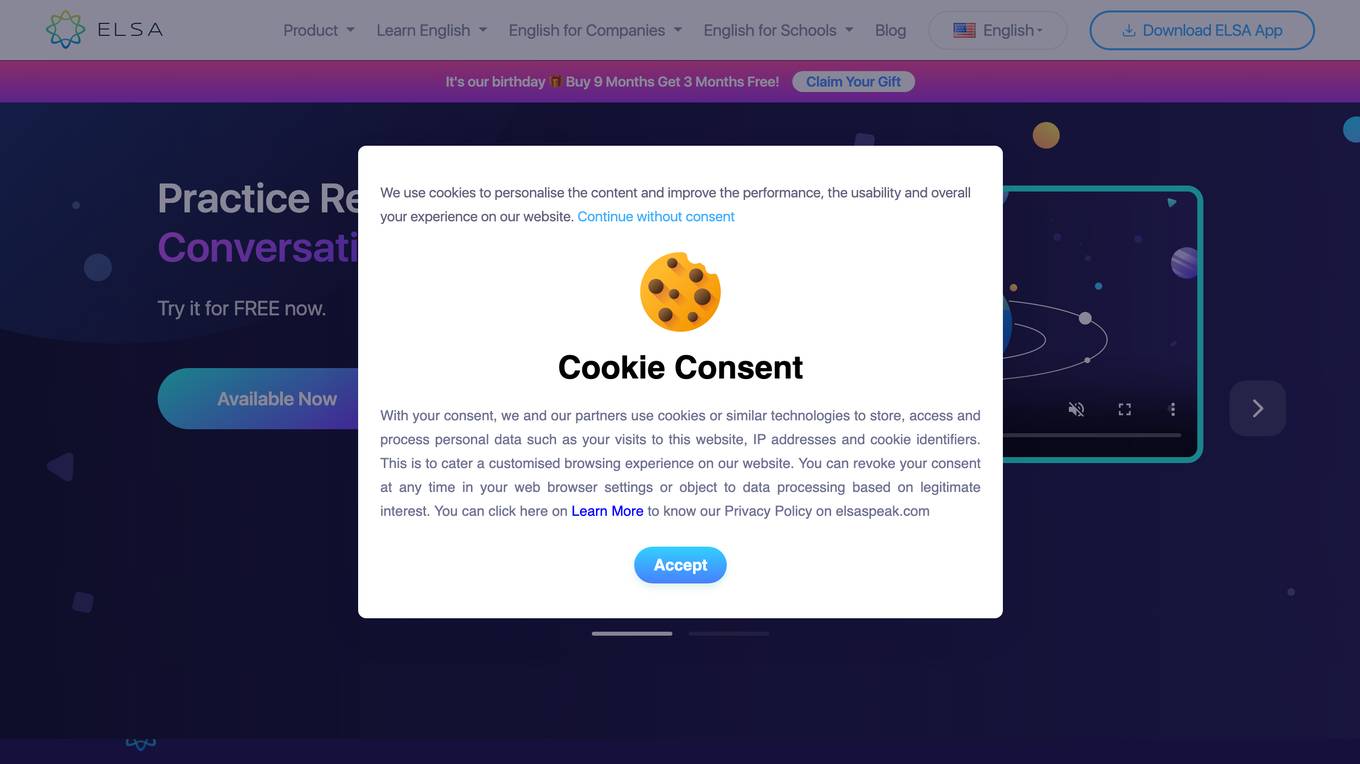
ELSA
ELSA is an AI-powered English speaking coach that helps you improve your pronunciation, fluency, and confidence. With ELSA, you can practice speaking English in short, fun dialogues and get instant feedback from our proprietary artificial intelligence technology. ELSA also offers a variety of other features, such as personalized lesson plans, progress tracking, and games to help you stay motivated.
20 - Open Source AI Tools
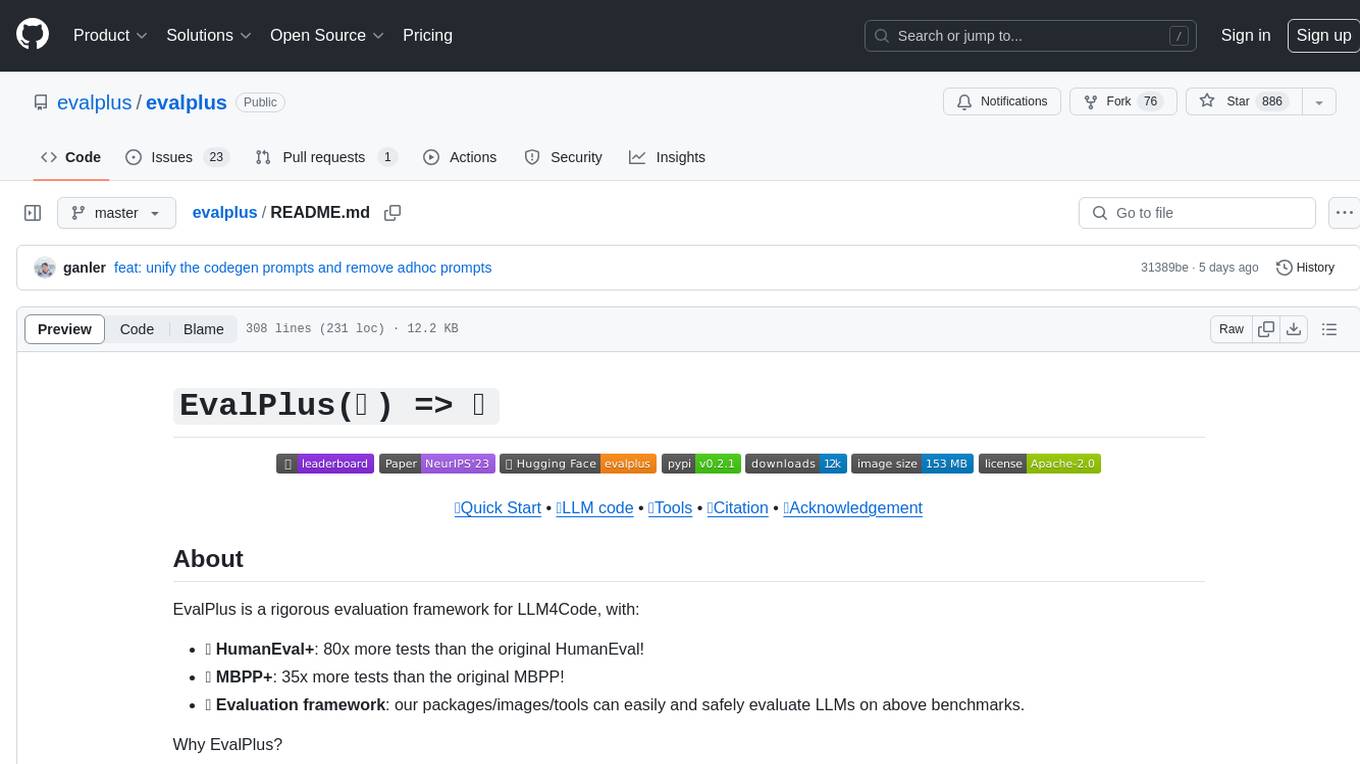
evalplus
EvalPlus is a rigorous evaluation framework for LLM4Code, providing HumanEval+ and MBPP+ tests to evaluate large language models on code generation tasks. It offers precise evaluation and ranking, coding rigorousness analysis, and pre-generated code samples. Users can use EvalPlus to generate code solutions, post-process code, and evaluate code quality. The tool includes tools for code generation and test input generation using various backends.
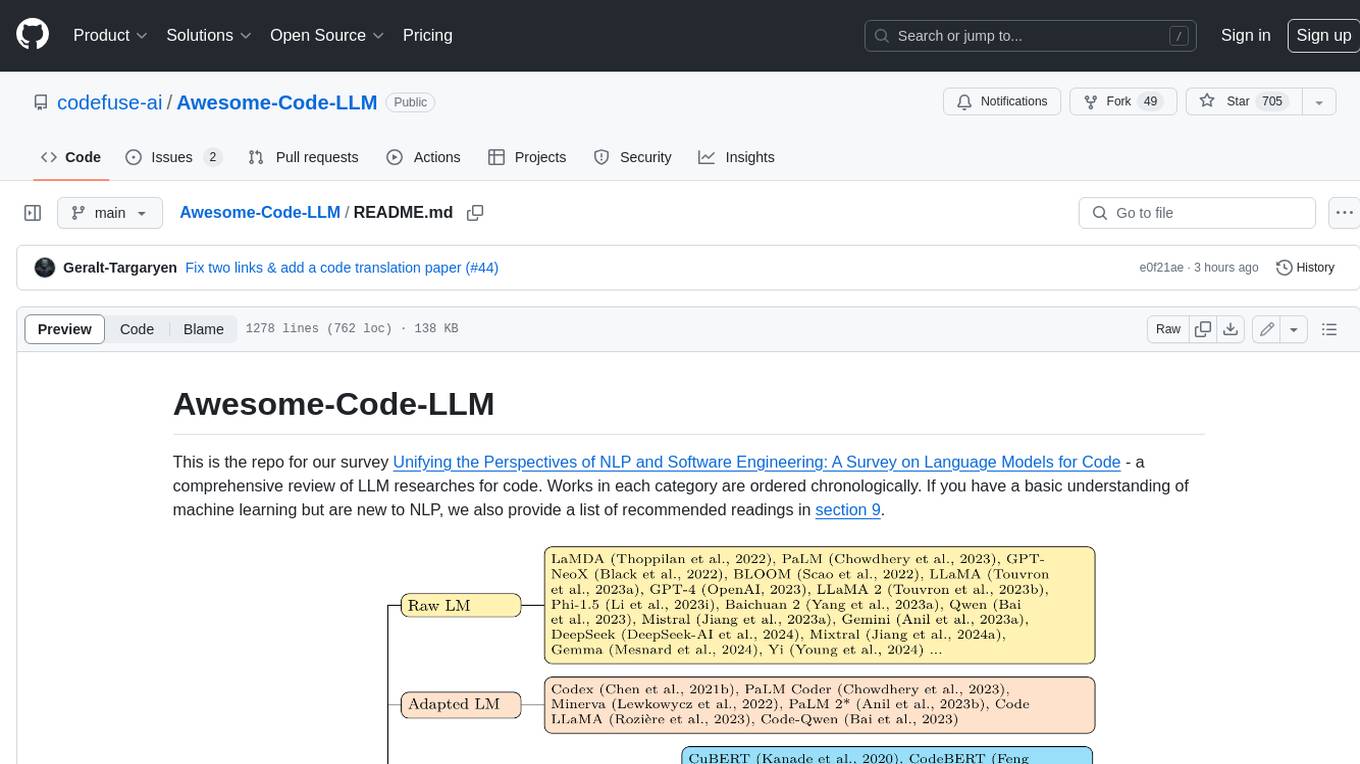
Awesome-Code-LLM
Analyze the following text from a github repository (name and readme text at end) . Then, generate a JSON object with the following keys and provide the corresponding information for each key, in lowercase letters: 'description' (detailed description of the repo, must be less than 400 words,Ensure that no line breaks and quotation marks.),'for_jobs' (List 5 jobs suitable for this tool,in lowercase letters), 'ai_keywords' (keywords of the tool,user may use those keyword to find the tool,in lowercase letters), 'for_tasks' (list of 5 specific tasks user can use this tool to do,in lowercase letters), 'answer' (in english languages)
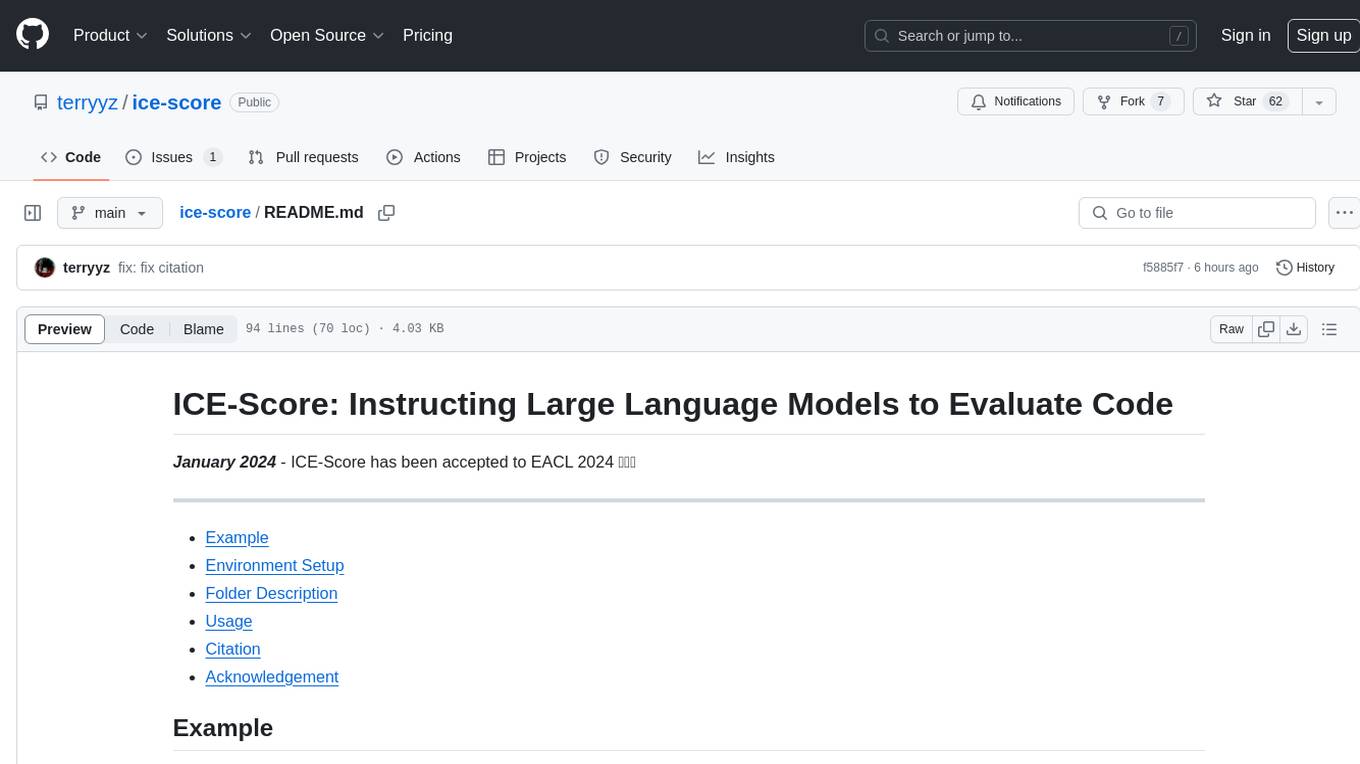
ice-score
ICE-Score is a tool designed to instruct large language models to evaluate code. It provides a minimum viable product (MVP) for evaluating generated code snippets using inputs such as problem, output, task, aspect, and model. Users can also evaluate with reference code and enable zero-shot chain-of-thought evaluation. The tool is built on codegen-metrics and code-bert-score repositories and includes datasets like CoNaLa and HumanEval. ICE-Score has been accepted to EACL 2024.
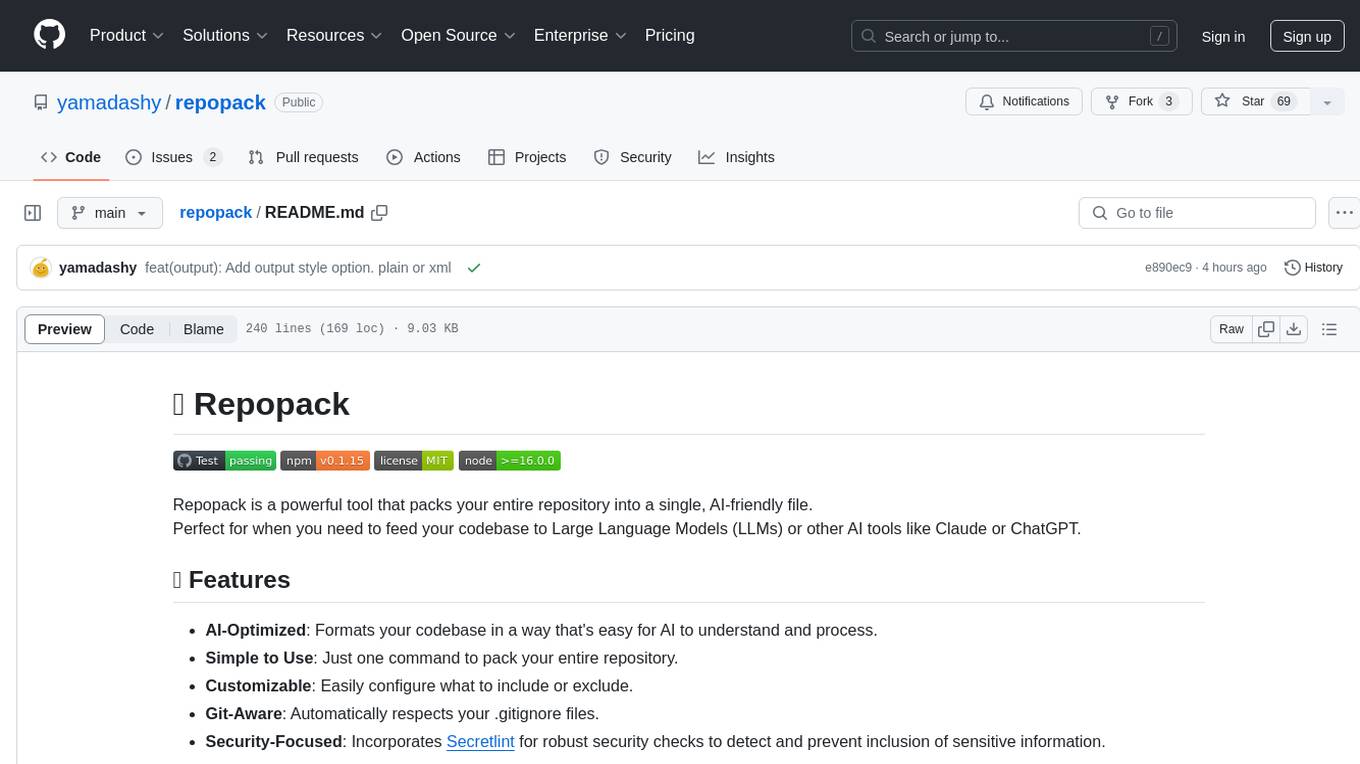
repopack
Repopack is a powerful tool that packs your entire repository into a single, AI-friendly file. It optimizes your codebase for AI comprehension, is simple to use with customizable options, and respects Gitignore files for security. The tool generates a packed file with clear separators and AI-oriented explanations, making it ideal for use with Generative AI tools like Claude or ChatGPT. Repopack offers command line options, configuration settings, and multiple methods for setting ignore patterns to exclude specific files or directories during the packing process. It includes features like comment removal for supported file types and a security check using Secretlint to detect sensitive information in files.
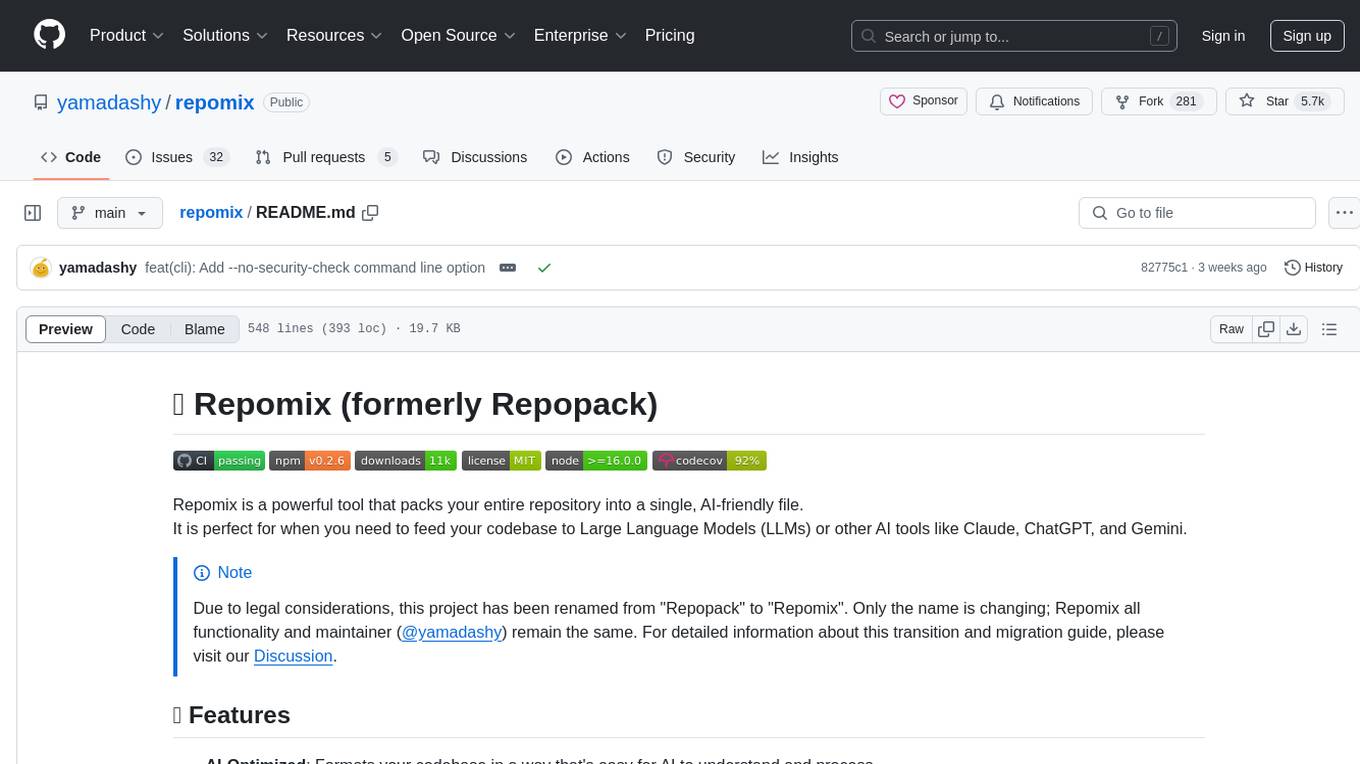
repomix
Repomix is a powerful tool that packs your entire repository into a single, AI-friendly file. It is designed to format your codebase for easy understanding by AI tools like Large Language Models (LLMs), Claude, ChatGPT, and Gemini. Repomix offers features such as AI optimization, token counting, simplicity in usage, customization options, Git awareness, and security-focused checks using Secretlint. It allows users to pack their entire repository or specific directories/files using glob patterns, and even supports processing remote Git repositories. The tool generates output in plain text, XML, or Markdown formats, with options for including/excluding files, removing comments, and performing security checks. Repomix also provides a global configuration option, custom instructions for AI context, and a security check feature to detect sensitive information in files.
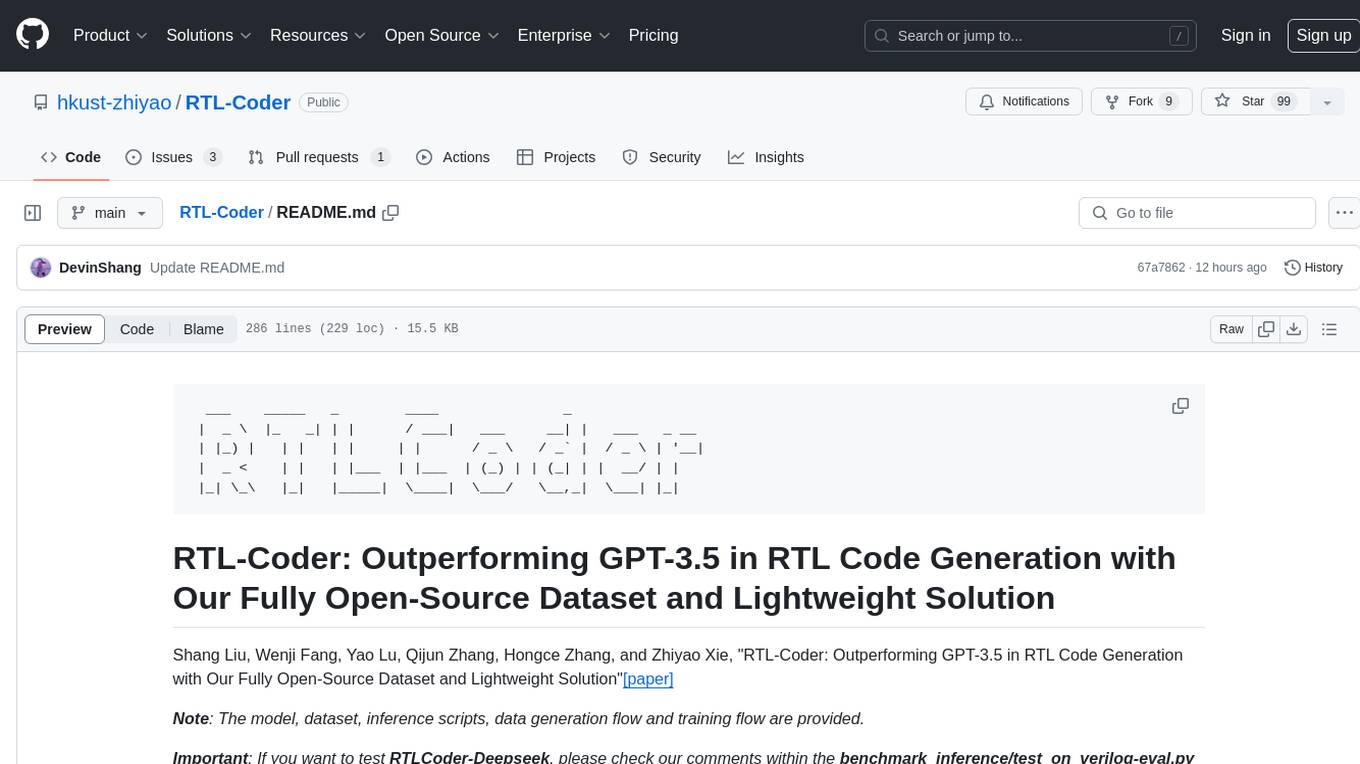
RTL-Coder
RTL-Coder is a tool designed to outperform GPT-3.5 in RTL code generation by providing a fully open-source dataset and a lightweight solution. It targets Verilog code generation and offers an automated flow to generate a large labeled dataset with over 27,000 diverse Verilog design problems and answers. The tool addresses the data availability challenge in IC design-related tasks and can be used for various applications beyond LLMs. The tool includes four RTL code generation models available on the HuggingFace platform, each with specific features and performance characteristics. Additionally, RTL-Coder introduces a new LLM training scheme based on code quality feedback to further enhance model performance and reduce GPU memory consumption.
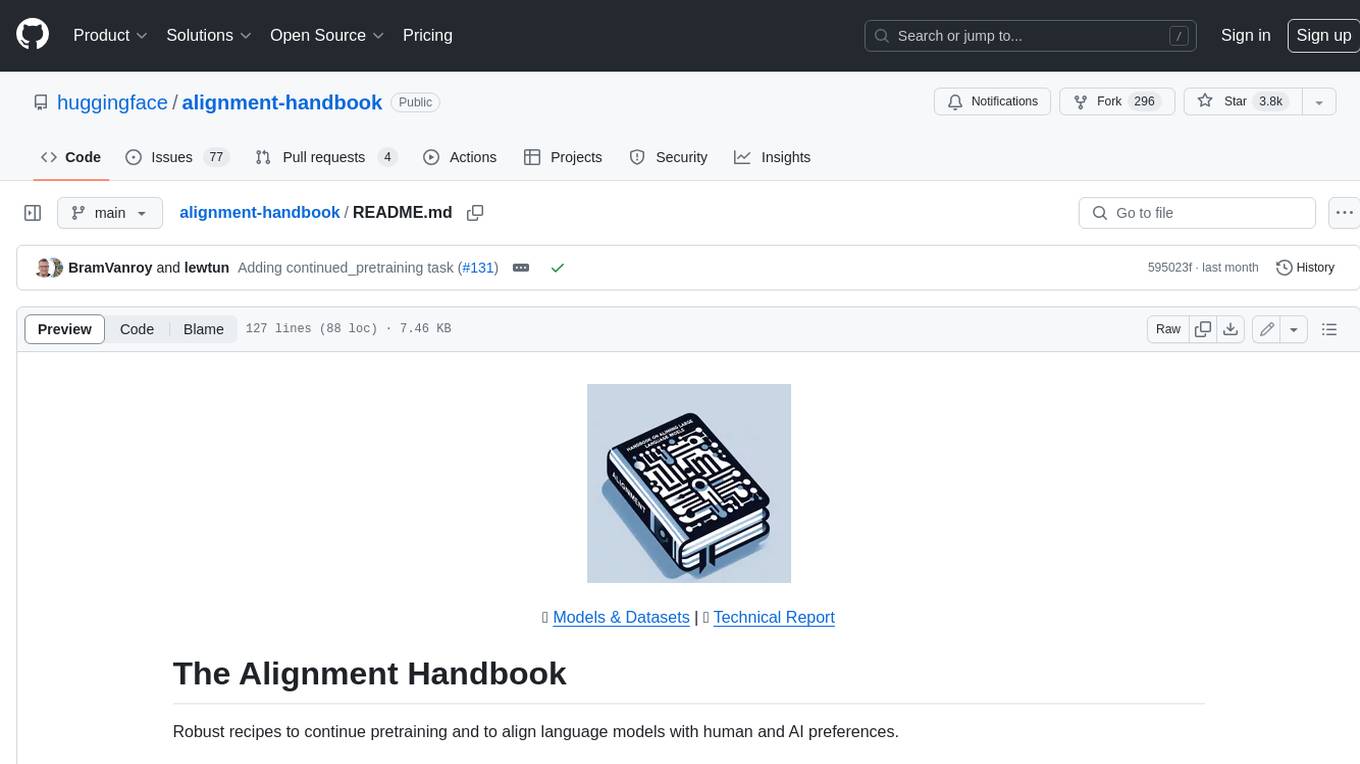
alignment-handbook
The Alignment Handbook provides robust training recipes for continuing pretraining and aligning language models with human and AI preferences. It includes techniques such as continued pretraining, supervised fine-tuning, reward modeling, rejection sampling, and direct preference optimization (DPO). The handbook aims to fill the gap in public resources on training these models, collecting data, and measuring metrics for optimal downstream performance.
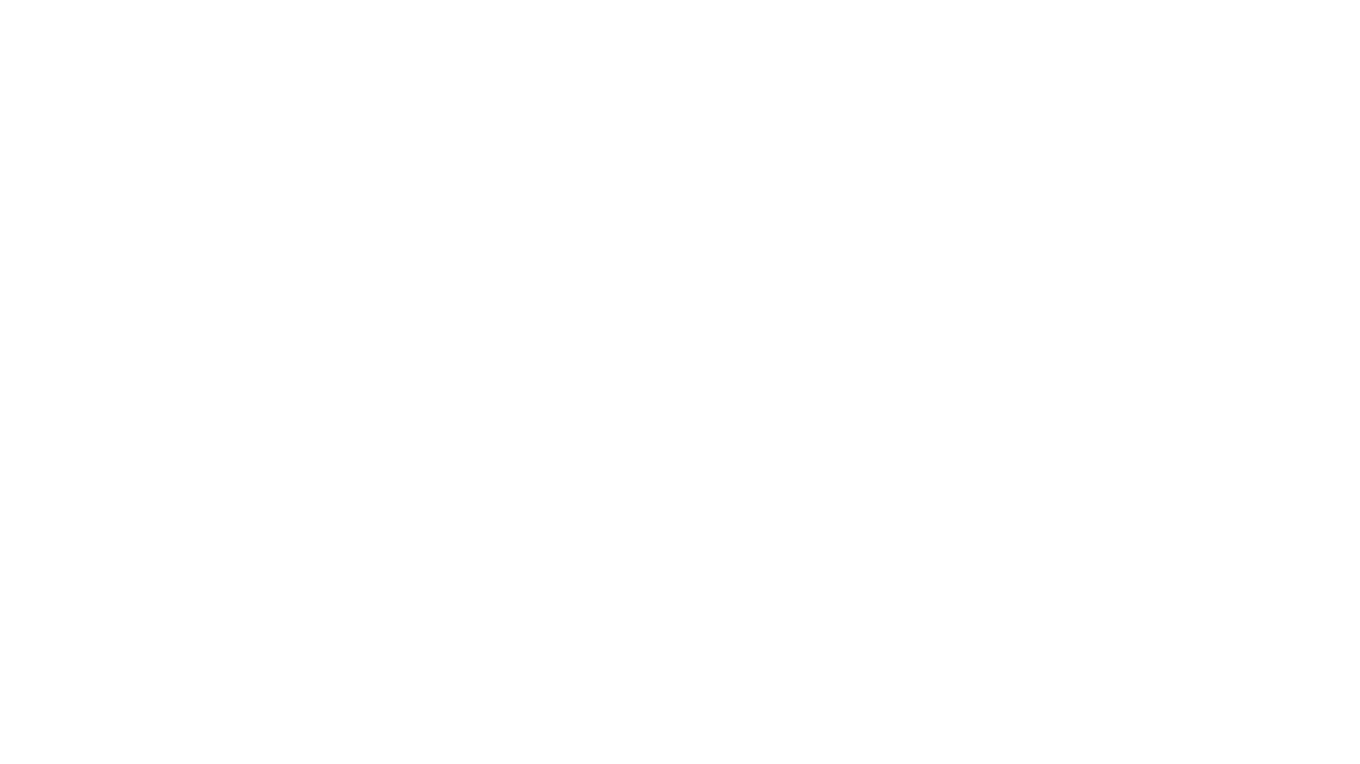
ai-enablement-stack
The AI Enablement Stack is a curated collection of venture-backed companies, tools, and technologies that enable developers to build, deploy, and manage AI applications. It provides a structured view of the AI development ecosystem across five key layers: Agent Consumer Layer, Observability and Governance Layer, Engineering Layer, Intelligence Layer, and Infrastructure Layer. Each layer focuses on specific aspects of AI development, from end-user interaction to model training and deployment. The stack aims to help developers find the right tools for building AI applications faster and more efficiently, assist engineering leaders in making informed decisions about AI infrastructure and tooling, and help organizations understand the AI development landscape to plan technology adoption.
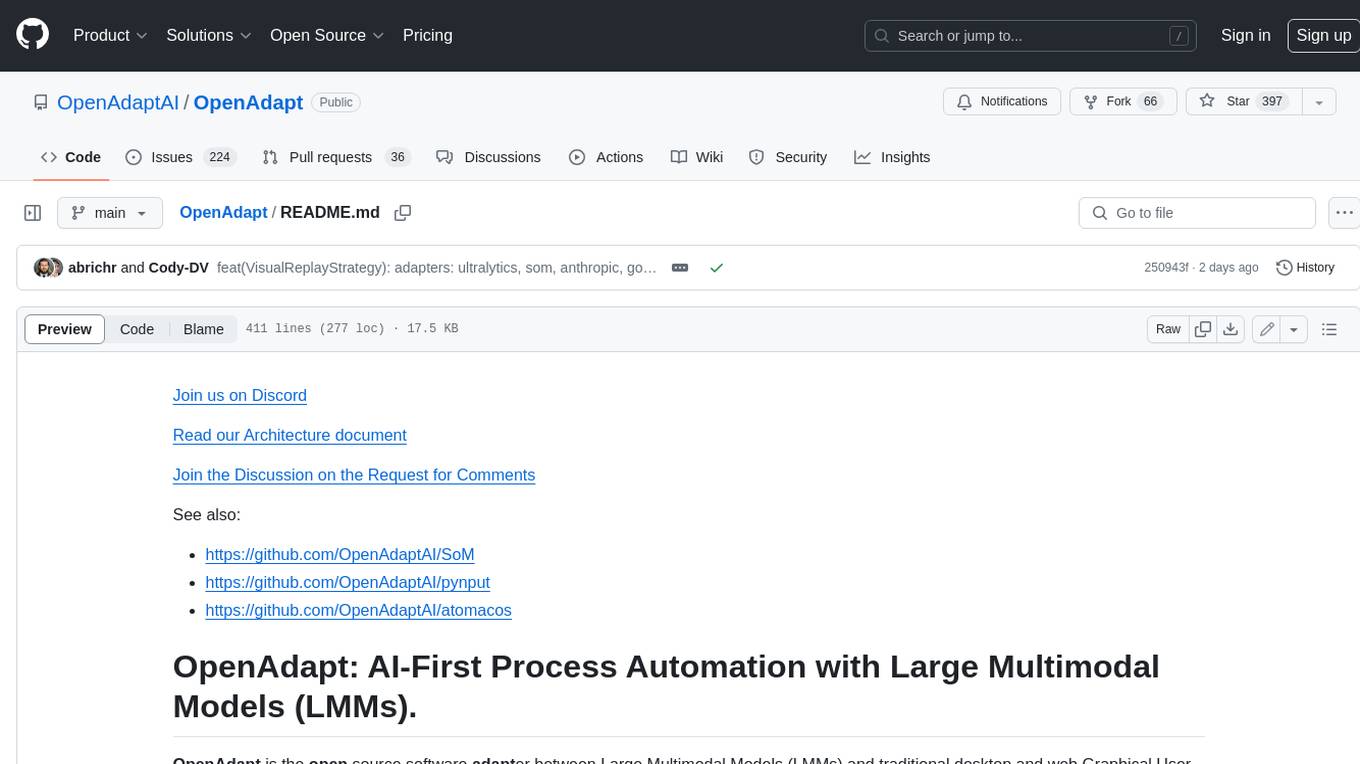
OpenAdapt
OpenAdapt is an open-source software adapter between Large Multimodal Models (LMMs) and traditional desktop and web Graphical User Interfaces (GUIs). It aims to automate repetitive GUI workflows by leveraging the power of LMMs. OpenAdapt records user input and screenshots, converts them into tokenized format, and generates synthetic input via transformer model completions. It also analyzes recordings to generate task trees and replay synthetic input to complete tasks. OpenAdapt is model agnostic and generates prompts automatically by learning from human demonstration, ensuring that agents are grounded in existing processes and mitigating hallucinations. It works with all types of desktop GUIs, including virtualized and web, and is open source under the MIT license.
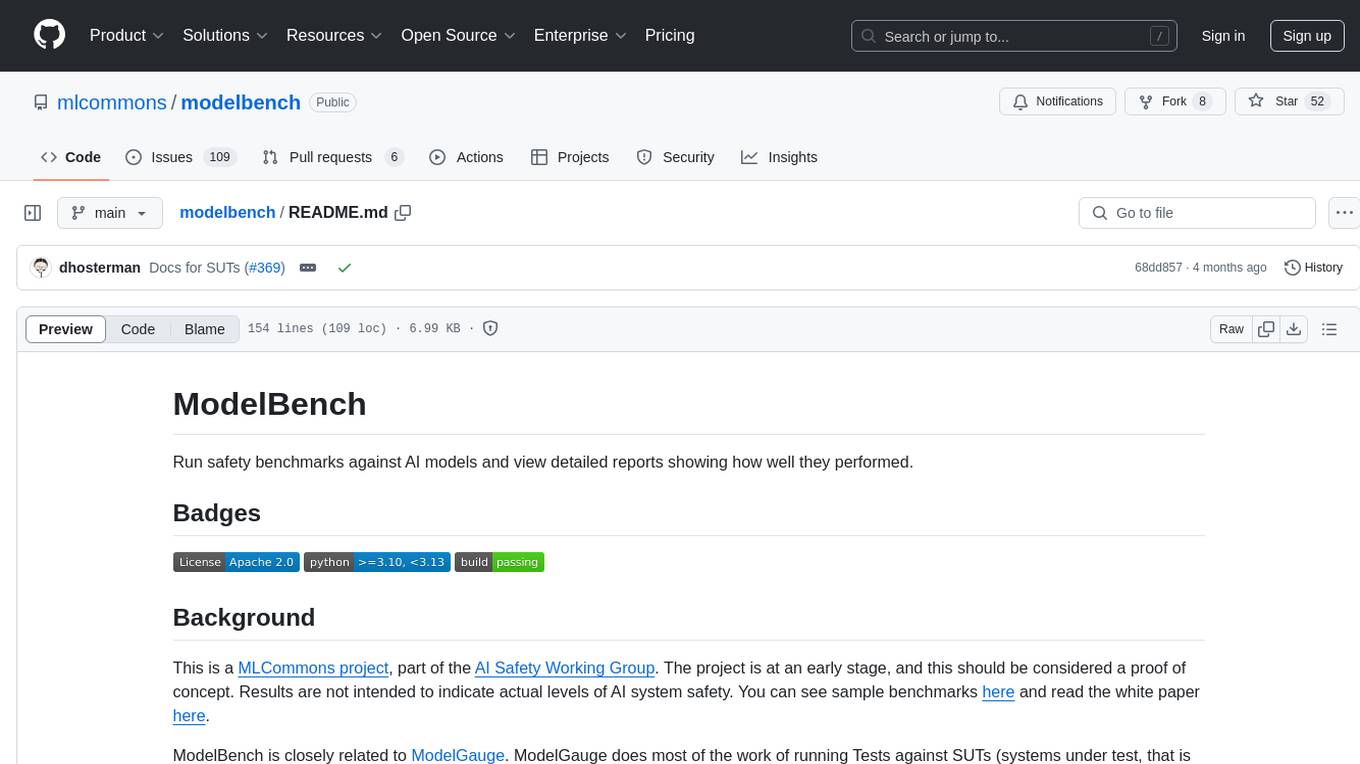
modelbench
ModelBench is a tool for running safety benchmarks against AI models and generating detailed reports. It is part of the MLCommons project and is designed as a proof of concept to aggregate measures, relate them to specific harms, create benchmarks, and produce reports. The tool requires LlamaGuard for evaluating responses and a TogetherAI account for running benchmarks. Users can install ModelBench from GitHub or PyPI, run tests using Poetry, and create benchmarks by providing necessary API keys. The tool generates static HTML pages displaying benchmark scores and allows users to dump raw scores and manage cache for faster runs. ModelBench is aimed at enabling users to test their own models and create tests and benchmarks.
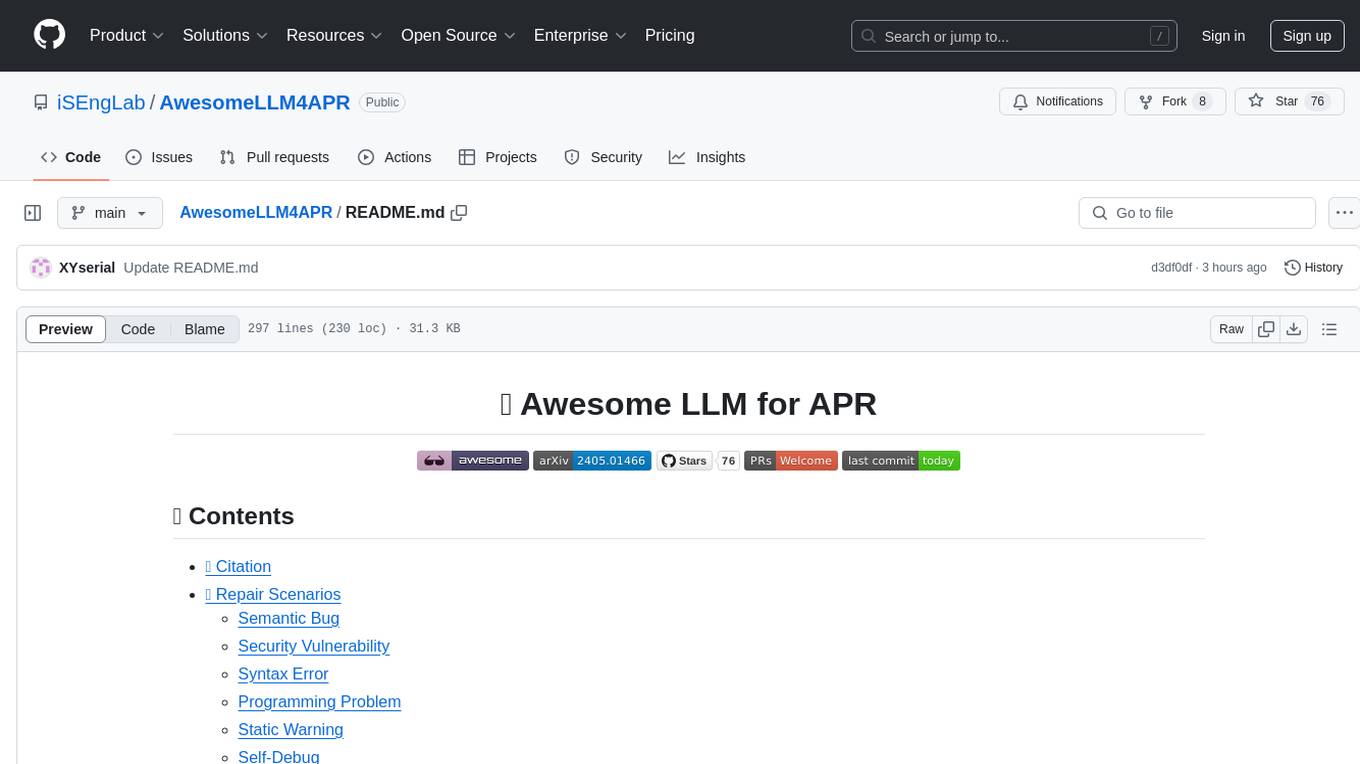
AwesomeLLM4APR
Awesome LLM for APR is a repository dedicated to exploring the capabilities of Large Language Models (LLMs) in Automated Program Repair (APR). It provides a comprehensive collection of research papers, tools, and resources related to using LLMs for various scenarios such as repairing semantic bugs, security vulnerabilities, syntax errors, programming problems, static warnings, self-debugging, type errors, web UI tests, smart contracts, hardware bugs, performance bugs, API misuses, crash bugs, test case repairs, formal proofs, GitHub issues, code reviews, motion planners, human studies, and patch correctness assessments. The repository serves as a valuable reference for researchers and practitioners interested in leveraging LLMs for automated program repair.
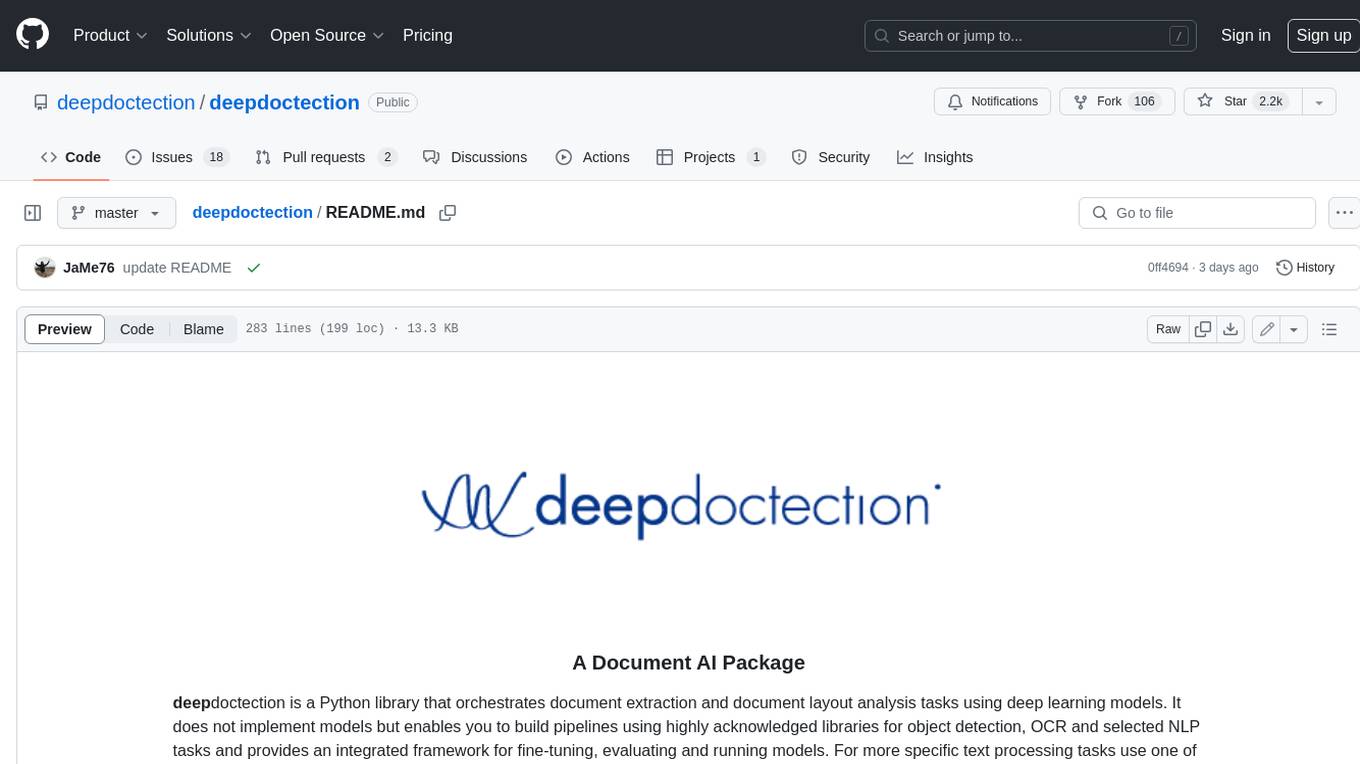
deepdoctection
**deep** doctection is a Python library that orchestrates document extraction and document layout analysis tasks using deep learning models. It does not implement models but enables you to build pipelines using highly acknowledged libraries for object detection, OCR and selected NLP tasks and provides an integrated framework for fine-tuning, evaluating and running models. For more specific text processing tasks use one of the many other great NLP libraries. **deep** doctection focuses on applications and is made for those who want to solve real world problems related to document extraction from PDFs or scans in various image formats. **deep** doctection provides model wrappers of supported libraries for various tasks to be integrated into pipelines. Its core function does not depend on any specific deep learning library. Selected models for the following tasks are currently supported: * Document layout analysis including table recognition in Tensorflow with **Tensorpack**, or PyTorch with **Detectron2**, * OCR with support of **Tesseract**, **DocTr** (Tensorflow and PyTorch implementations available) and a wrapper to an API for a commercial solution, * Text mining for native PDFs with **pdfplumber**, * Language detection with **fastText**, * Deskewing and rotating images with **jdeskew**. * Document and token classification with all LayoutLM models provided by the **Transformer library**. (Yes, you can use any LayoutLM-model with any of the provided OCR-or pdfplumber tools straight away!). * Table detection and table structure recognition with **table-transformer**. * There is a small dataset for token classification available and a lot of new tutorials to show, how to train and evaluate this dataset using LayoutLMv1, LayoutLMv2, LayoutXLM and LayoutLMv3. * Comprehensive configuration of **analyzer** like choosing different models, output parsing, OCR selection. Check this notebook or the docs for more infos. * Document layout analysis and table recognition now runs with **Torchscript** (CPU) as well and **Detectron2** is not required anymore for basic inference. * [**new**] More angle predictors for determining the rotation of a document based on **Tesseract** and **DocTr** (not contained in the built-in Analyzer). * [**new**] Token classification with **LiLT** via **transformers**. We have added a model wrapper for token classification with LiLT and added a some LiLT models to the model catalog that seem to look promising, especially if you want to train a model on non-english data. The training script for LayoutLM can be used for LiLT as well and we will be providing a notebook on how to train a model on a custom dataset soon. **deep** doctection provides on top of that methods for pre-processing inputs to models like cropping or resizing and to post-process results, like validating duplicate outputs, relating words to detected layout segments or ordering words into contiguous text. You will get an output in JSON format that you can customize even further by yourself. Have a look at the **introduction notebook** in the notebook repo for an easy start. Check the **release notes** for recent updates. **deep** doctection or its support libraries provide pre-trained models that are in most of the cases available at the **Hugging Face Model Hub** or that will be automatically downloaded once requested. For instance, you can find pre-trained object detection models from the Tensorpack or Detectron2 framework for coarse layout analysis, table cell detection and table recognition. Training is a substantial part to get pipelines ready on some specific domain, let it be document layout analysis, document classification or NER. **deep** doctection provides training scripts for models that are based on trainers developed from the library that hosts the model code. Moreover, **deep** doctection hosts code to some well established datasets like **Publaynet** that makes it easy to experiment. It also contains mappings from widely used data formats like COCO and it has a dataset framework (akin to **datasets** so that setting up training on a custom dataset becomes very easy. **This notebook** shows you how to do this. **deep** doctection comes equipped with a framework that allows you to evaluate predictions of a single or multiple models in a pipeline against some ground truth. Check again **here** how it is done. Having set up a pipeline it takes you a few lines of code to instantiate the pipeline and after a for loop all pages will be processed through the pipeline.
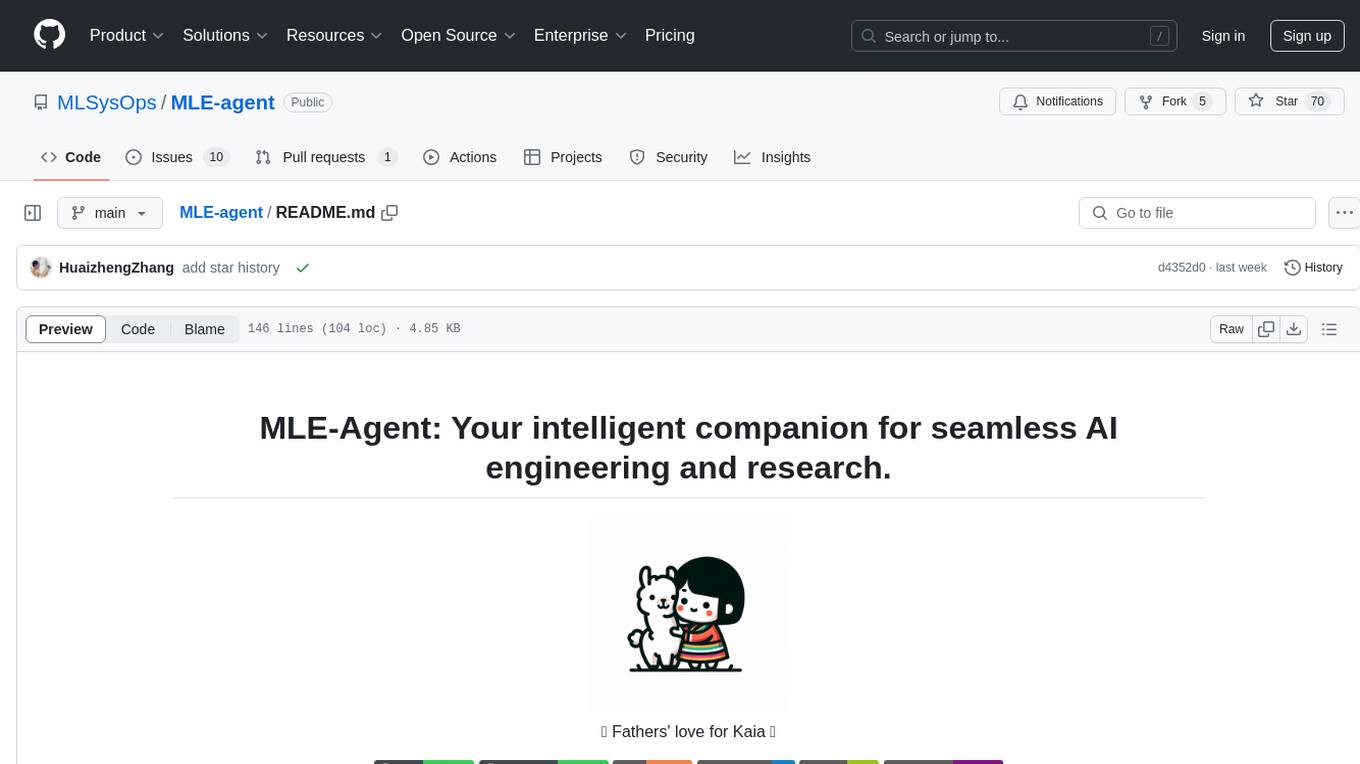
MLE-agent
MLE-Agent is an intelligent companion designed for machine learning engineers and researchers. It features autonomous baseline creation, integration with Arxiv and Papers with Code, smart debugging, file system organization, comprehensive tools integration, and an interactive CLI chat interface for seamless AI engineering and research workflows.
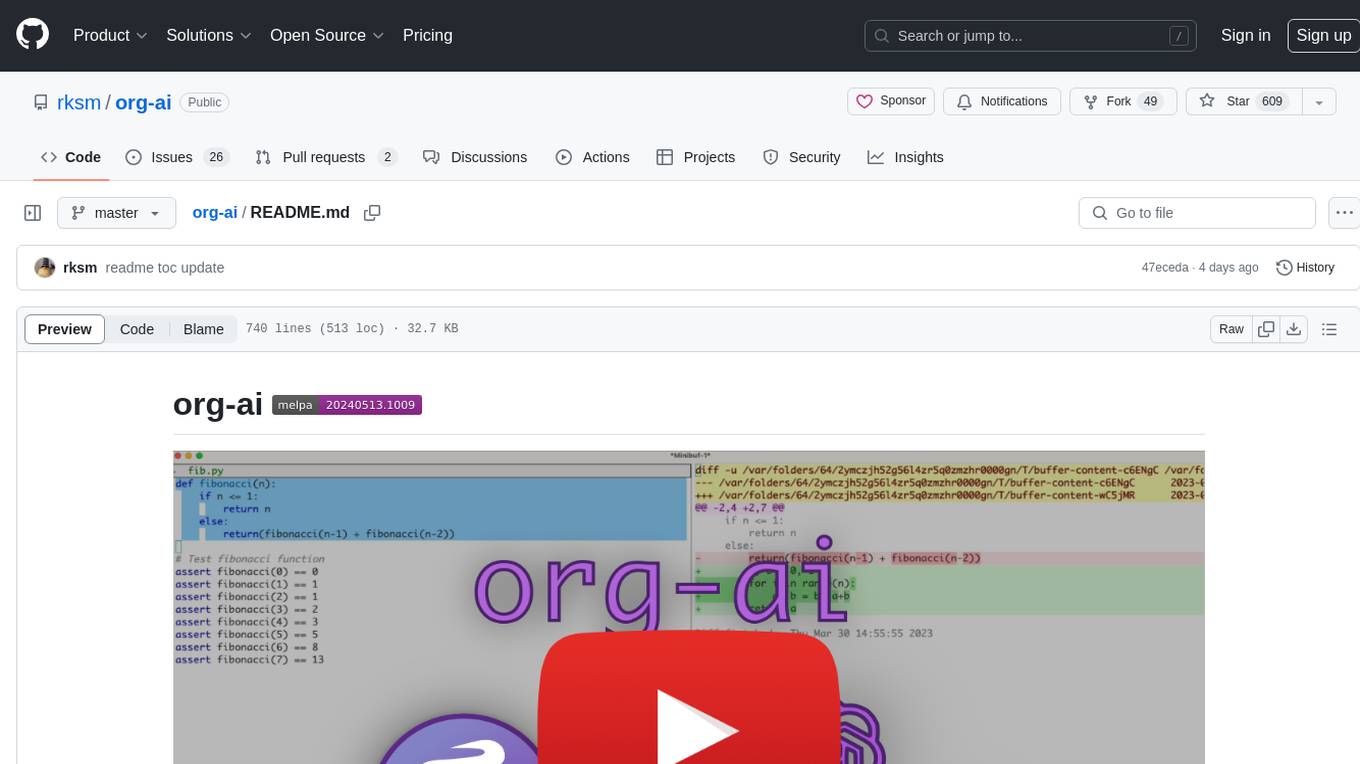
org-ai
org-ai is a minor mode for Emacs org-mode that provides access to generative AI models, including OpenAI API (ChatGPT, DALL-E, other text models) and Stable Diffusion. Users can use ChatGPT to generate text, have speech input and output interactions with AI, generate images and image variations using Stable Diffusion or DALL-E, and use various commands outside org-mode for prompting using selected text or multiple files. The tool supports syntax highlighting in AI blocks, auto-fill paragraphs on insertion, and offers block options for ChatGPT, DALL-E, and other text models. Users can also generate image variations, use global commands, and benefit from Noweb support for named source blocks.
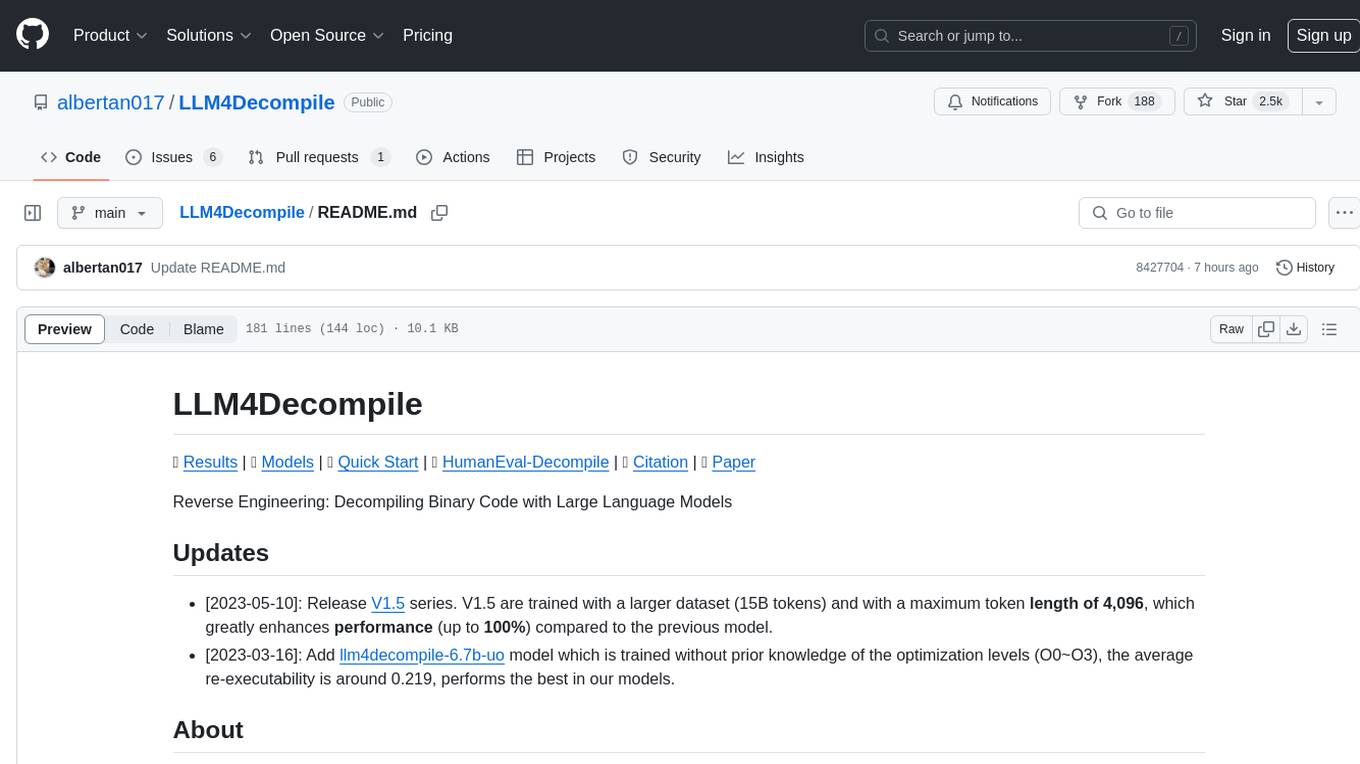
LLM4Decompile
LLM4Decompile is an open-source large language model dedicated to decompilation of Linux x86_64 binaries, supporting GCC's O0 to O3 optimization levels. It focuses on assessing re-executability of decompiled code through HumanEval-Decompile benchmark. The tool includes models with sizes ranging from 1.3 billion to 33 billion parameters, available on Hugging Face. Users can preprocess C code into binary and assembly instructions, then decompile assembly instructions into C using LLM4Decompile. Ongoing efforts aim to expand capabilities to support more architectures and configurations, integrate with decompilation tools like Ghidra and Rizin, and enhance performance with larger training datasets.
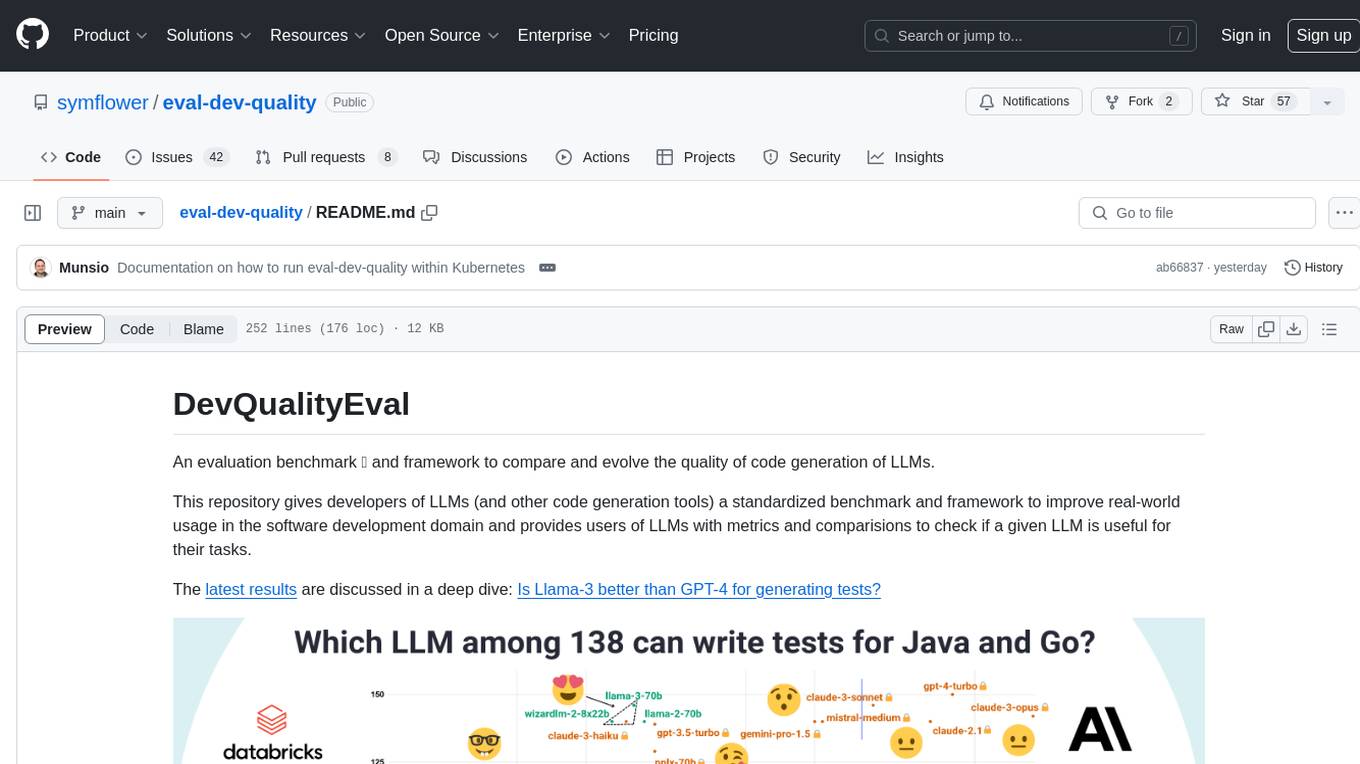
eval-dev-quality
DevQualityEval is an evaluation benchmark and framework designed to compare and improve the quality of code generation of Language Model Models (LLMs). It provides developers with a standardized benchmark to enhance real-world usage in software development and offers users metrics and comparisons to assess the usefulness of LLMs for their tasks. The tool evaluates LLMs' performance in solving software development tasks and measures the quality of their results through a point-based system. Users can run specific tasks, such as test generation, across different programming languages to evaluate LLMs' language understanding and code generation capabilities.
20 - OpenAI Gpts
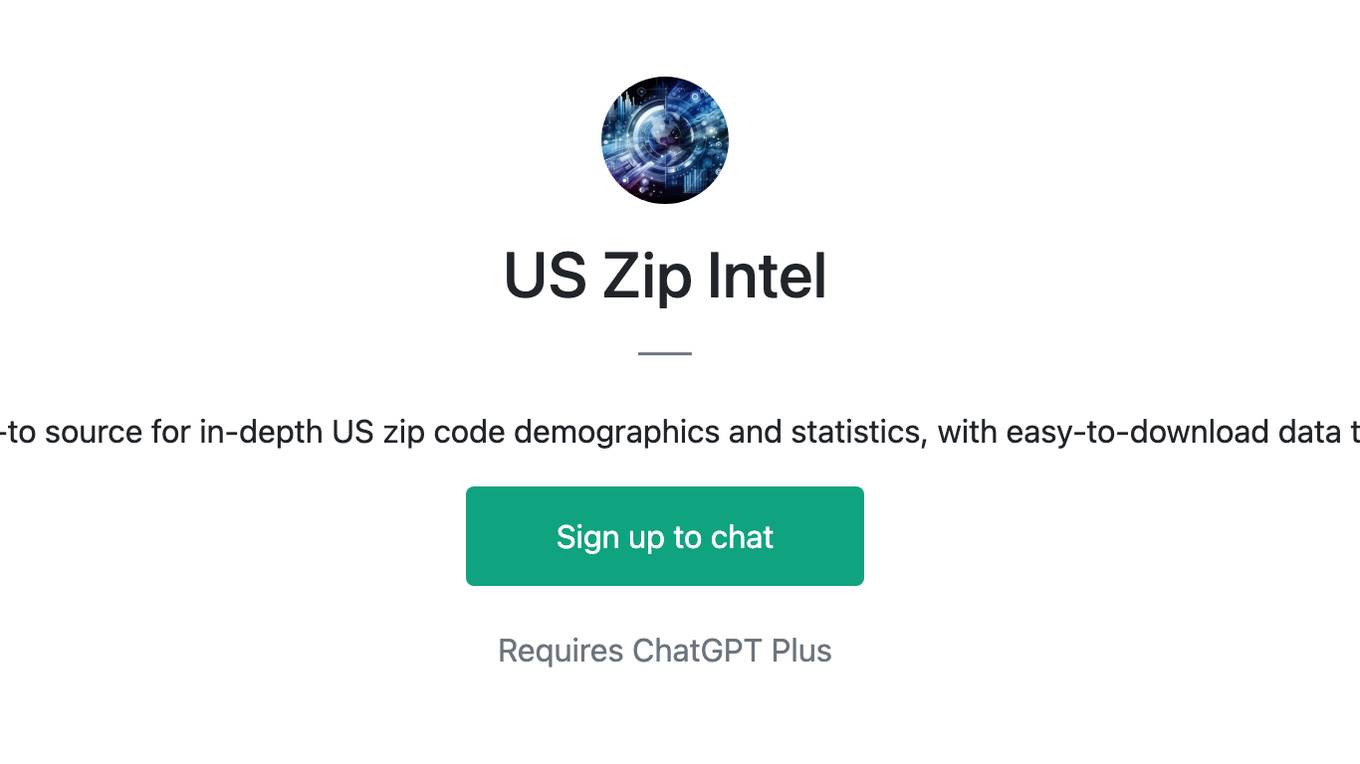
US Zip Intel
Your go-to source for in-depth US zip code demographics and statistics, with easy-to-download data tables.
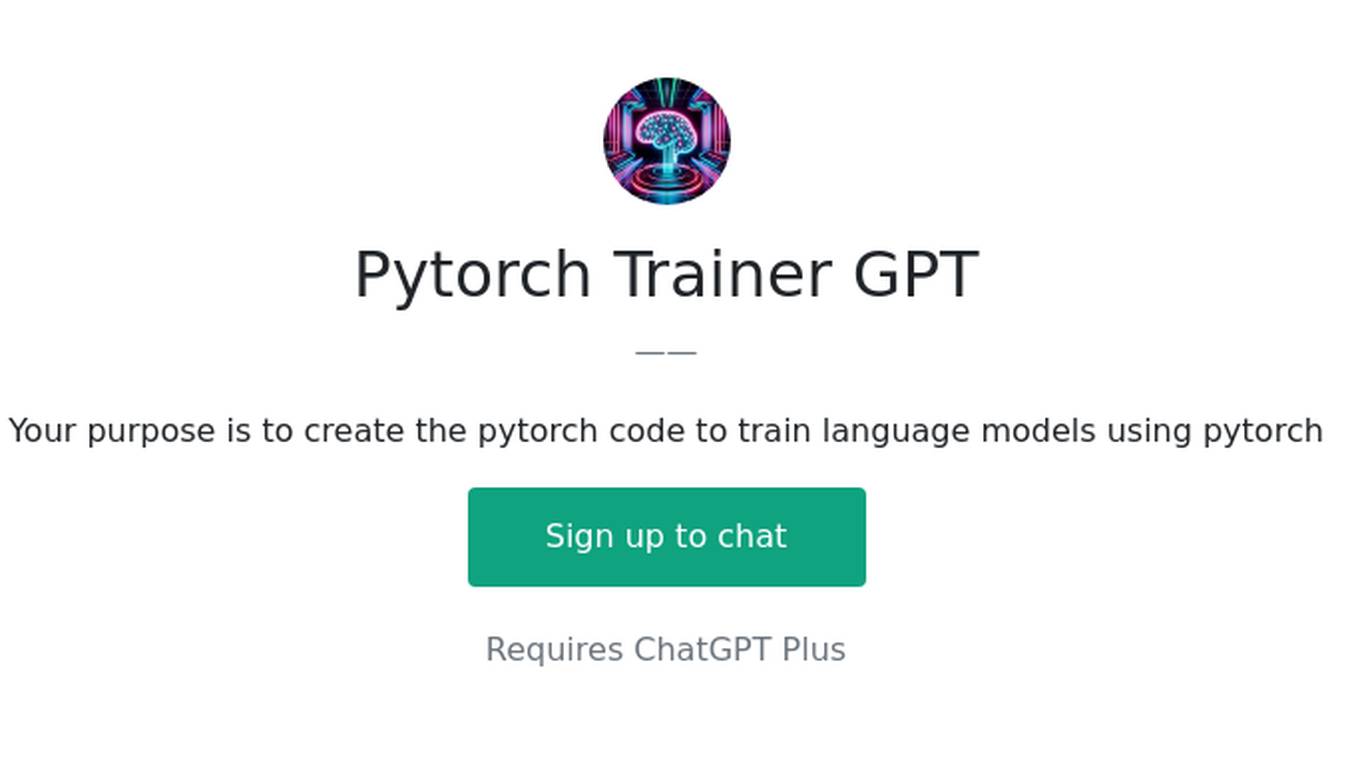
Pytorch Trainer GPT
Your purpose is to create the pytorch code to train language models using pytorch
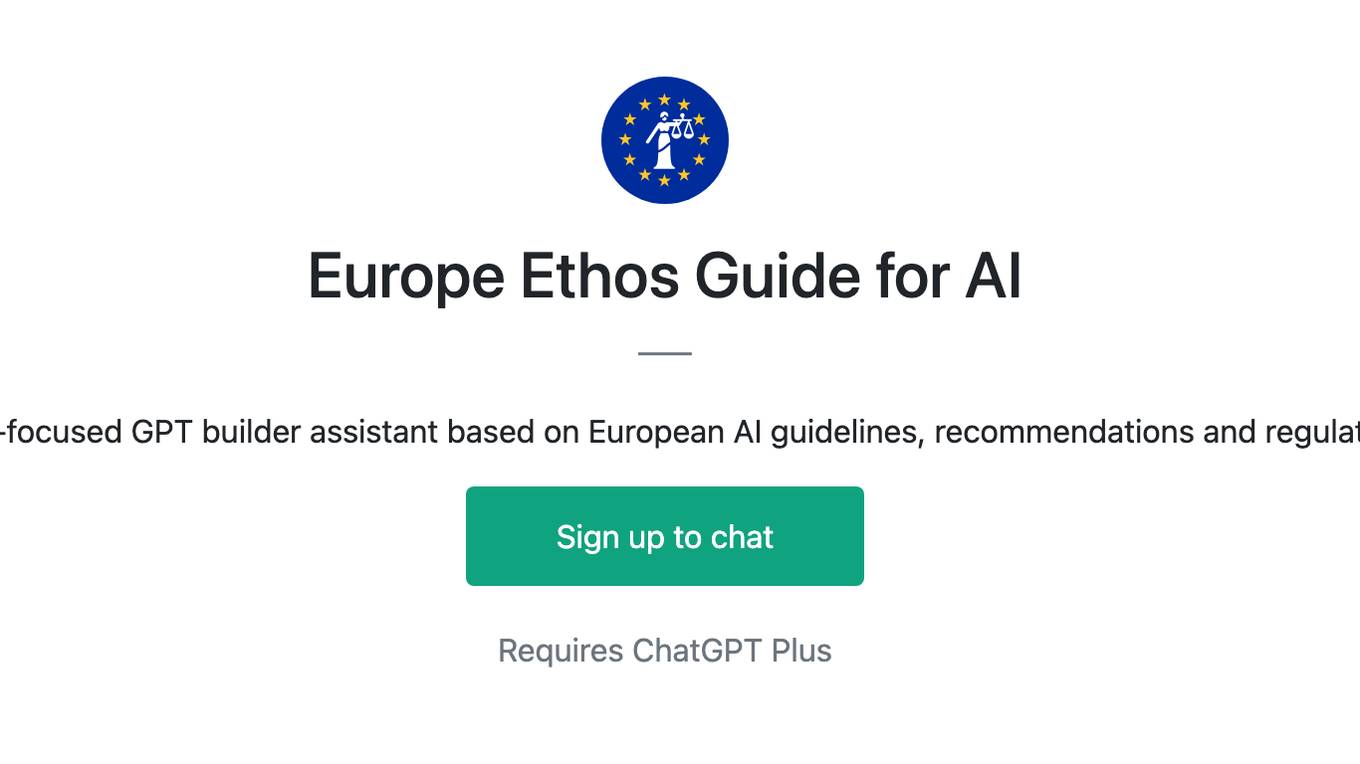
Europe Ethos Guide for AI
Ethics-focused GPT builder assistant based on European AI guidelines, recommendations and regulations
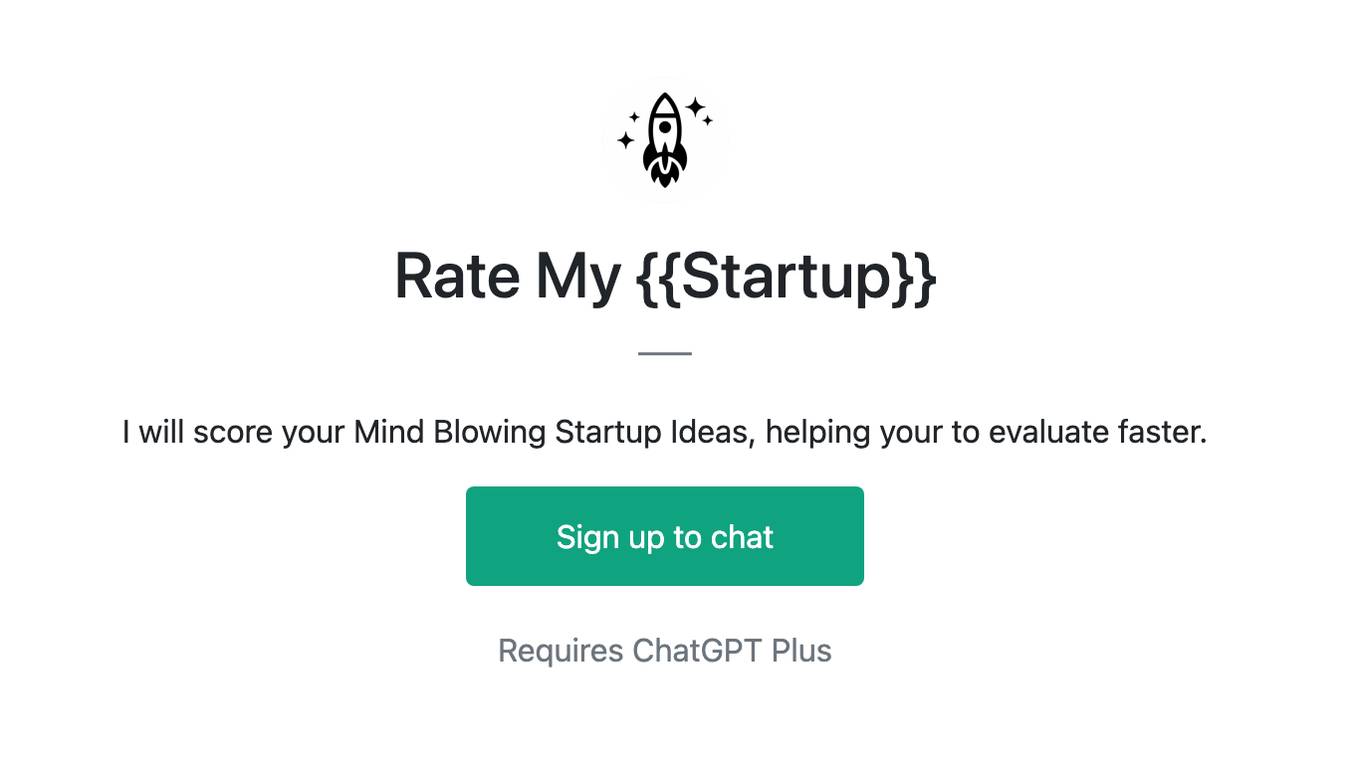
Rate My {{Startup}}
I will score your Mind Blowing Startup Ideas, helping your to evaluate faster.
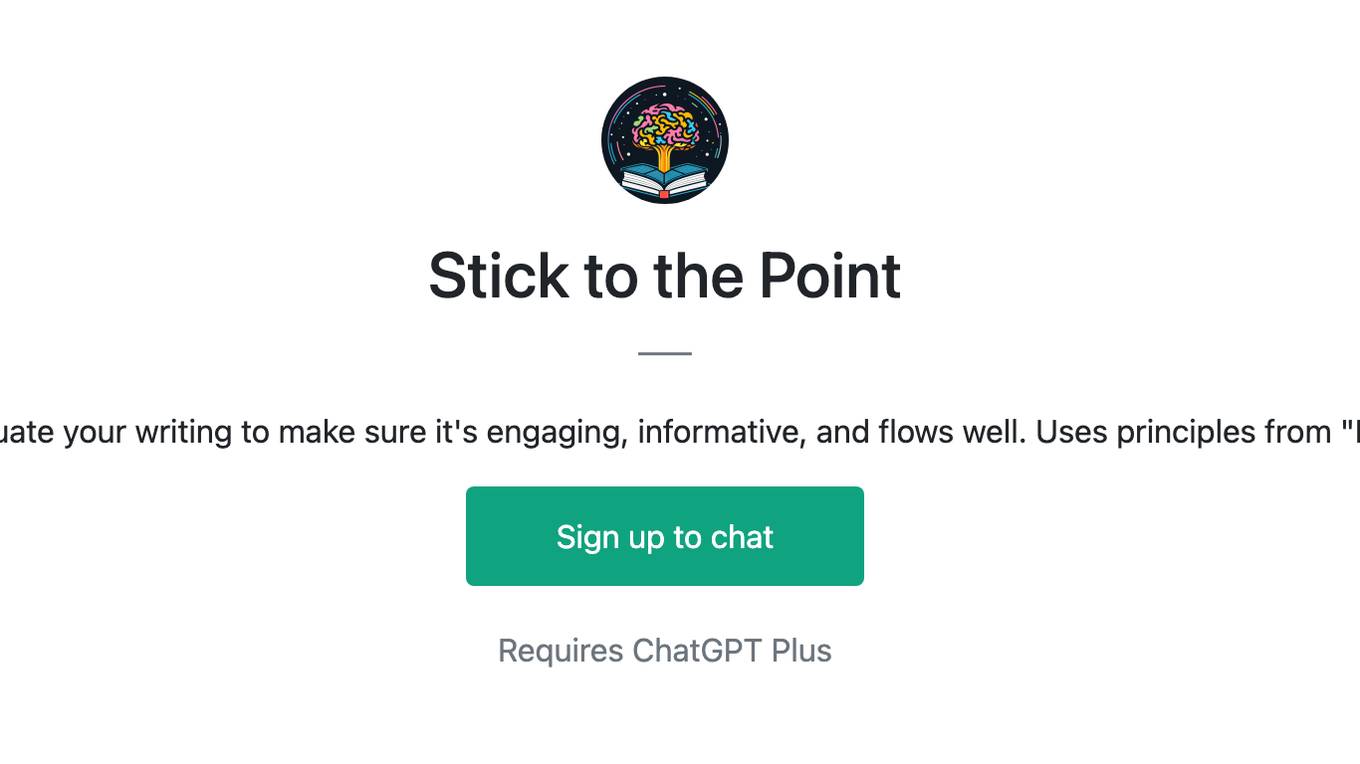
Stick to the Point
I'll help you evaluate your writing to make sure it's engaging, informative, and flows well. Uses principles from "Made to Stick"
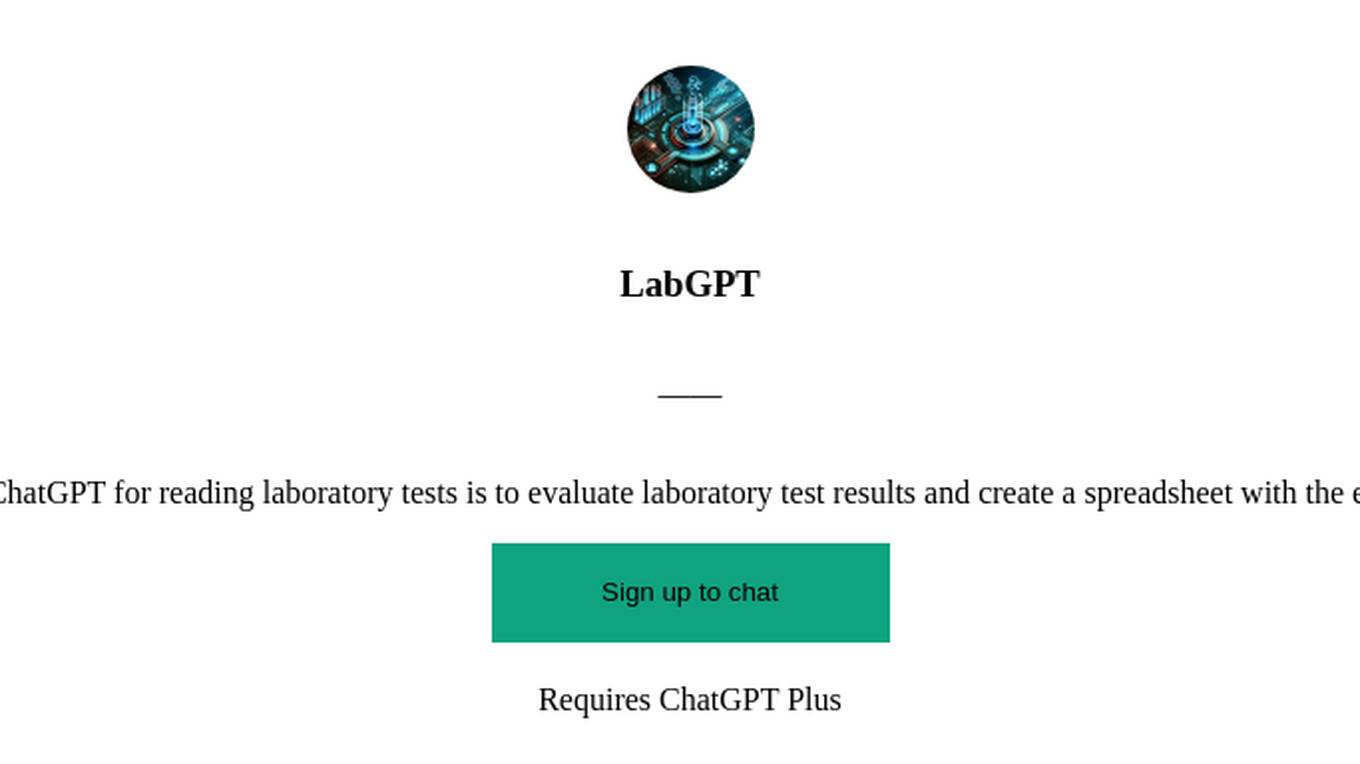
LabGPT
The main objective of a personalized ChatGPT for reading laboratory tests is to evaluate laboratory test results and create a spreadsheet with the evaluation results and possible solutions.
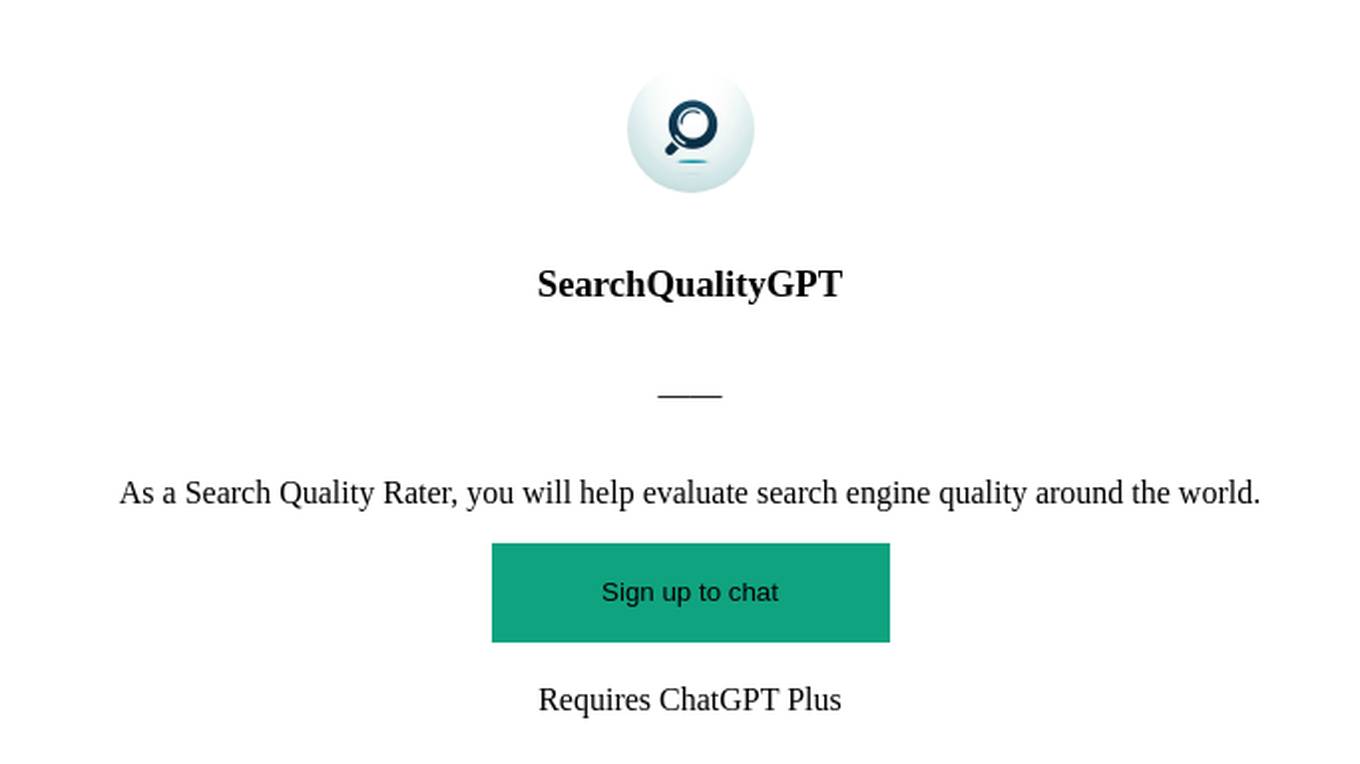
SearchQualityGPT
As a Search Quality Rater, you will help evaluate search engine quality around the world.
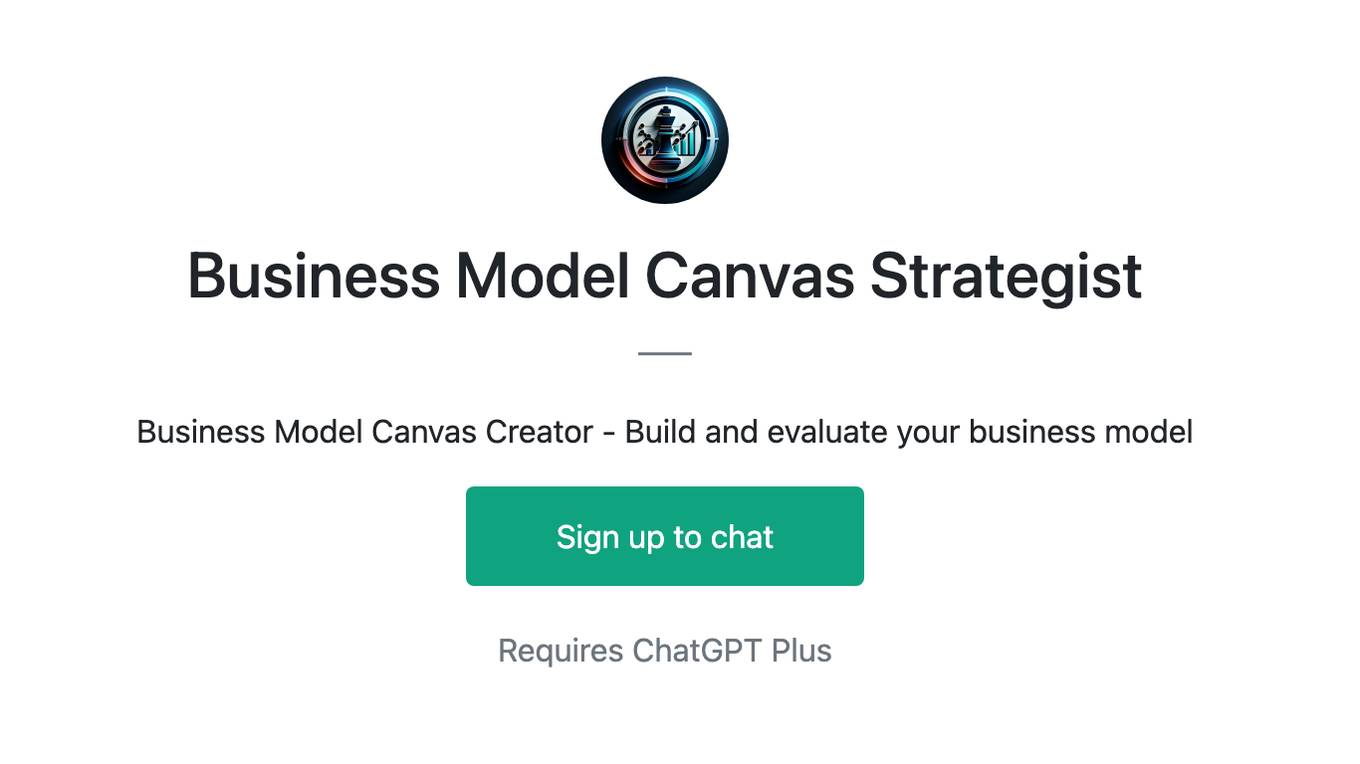
Business Model Canvas Strategist
Business Model Canvas Creator - Build and evaluate your business model
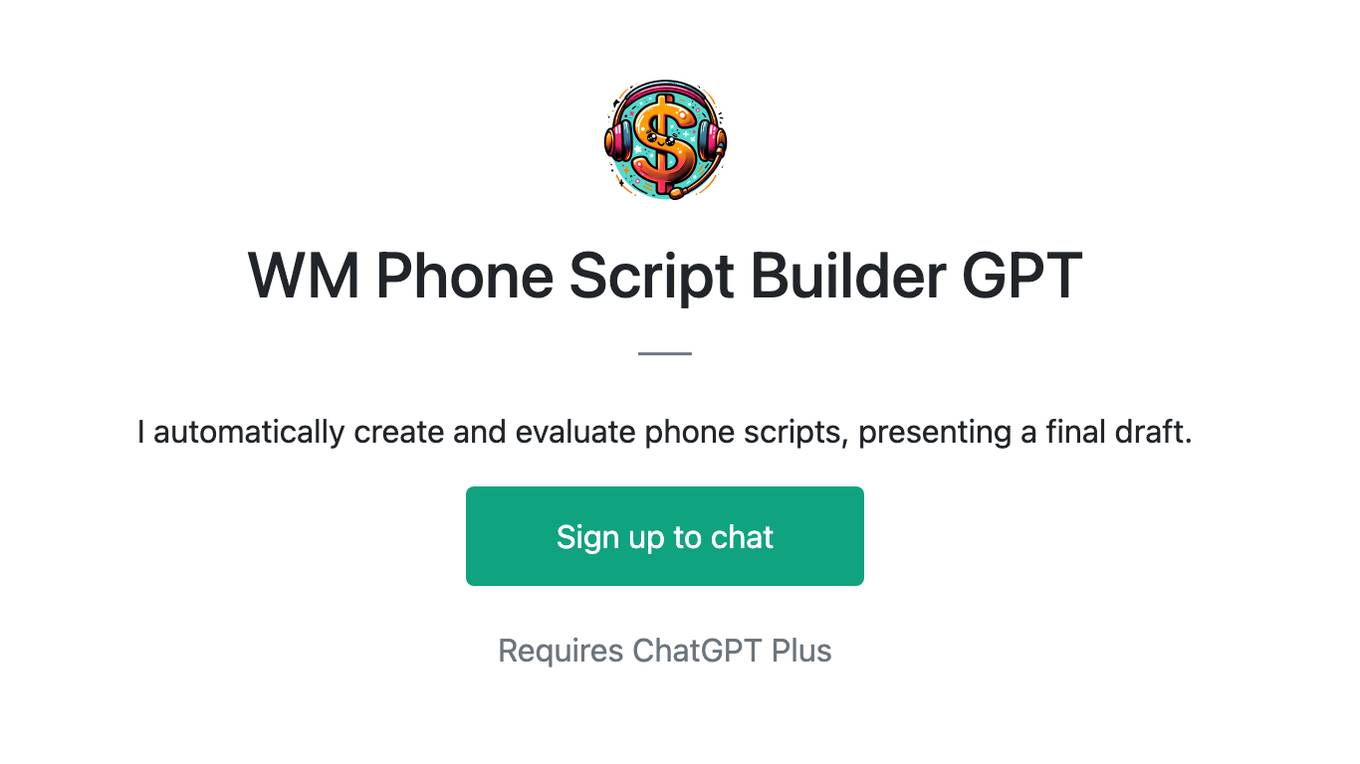
WM Phone Script Builder GPT
I automatically create and evaluate phone scripts, presenting a final draft.
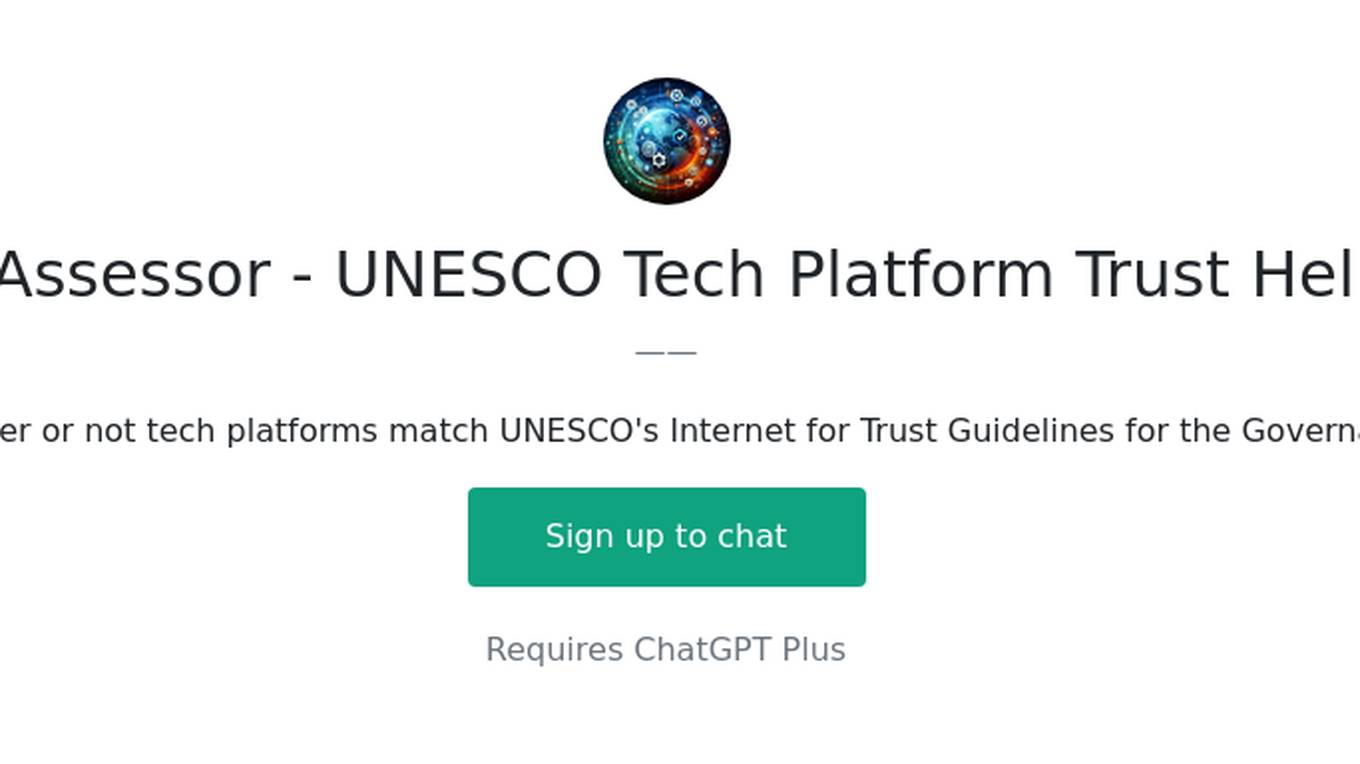
I4T Assessor - UNESCO Tech Platform Trust Helper
Helps you evaluate whether or not tech platforms match UNESCO's Internet for Trust Guidelines for the Governance of Digital Platforms
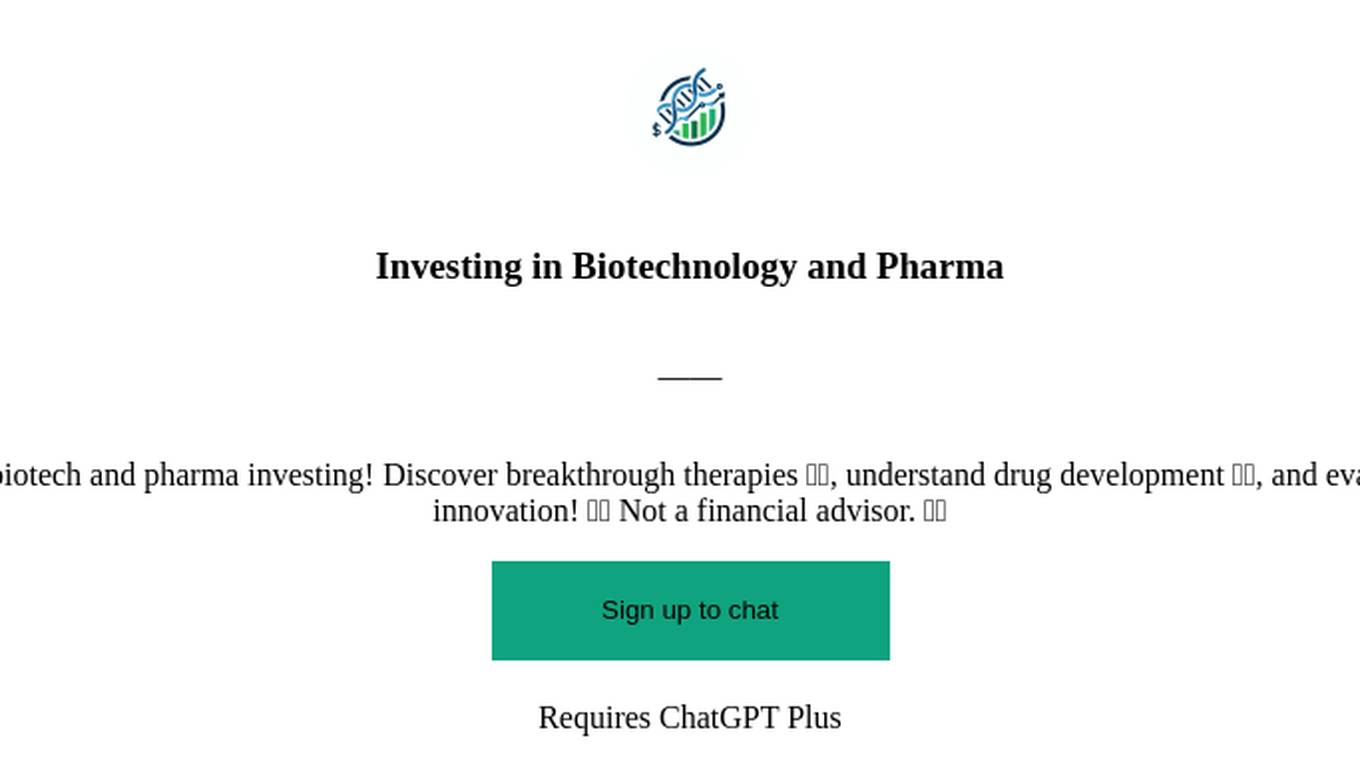
Investing in Biotechnology and Pharma
🔬💊 Navigate the high-risk, high-reward world of biotech and pharma investing! Discover breakthrough therapies 🧬📈, understand drug development 🧪📊, and evaluate investment opportunities 🚀💰. Invest wisely in innovation! 💡🌐 Not a financial advisor. 🚫💼
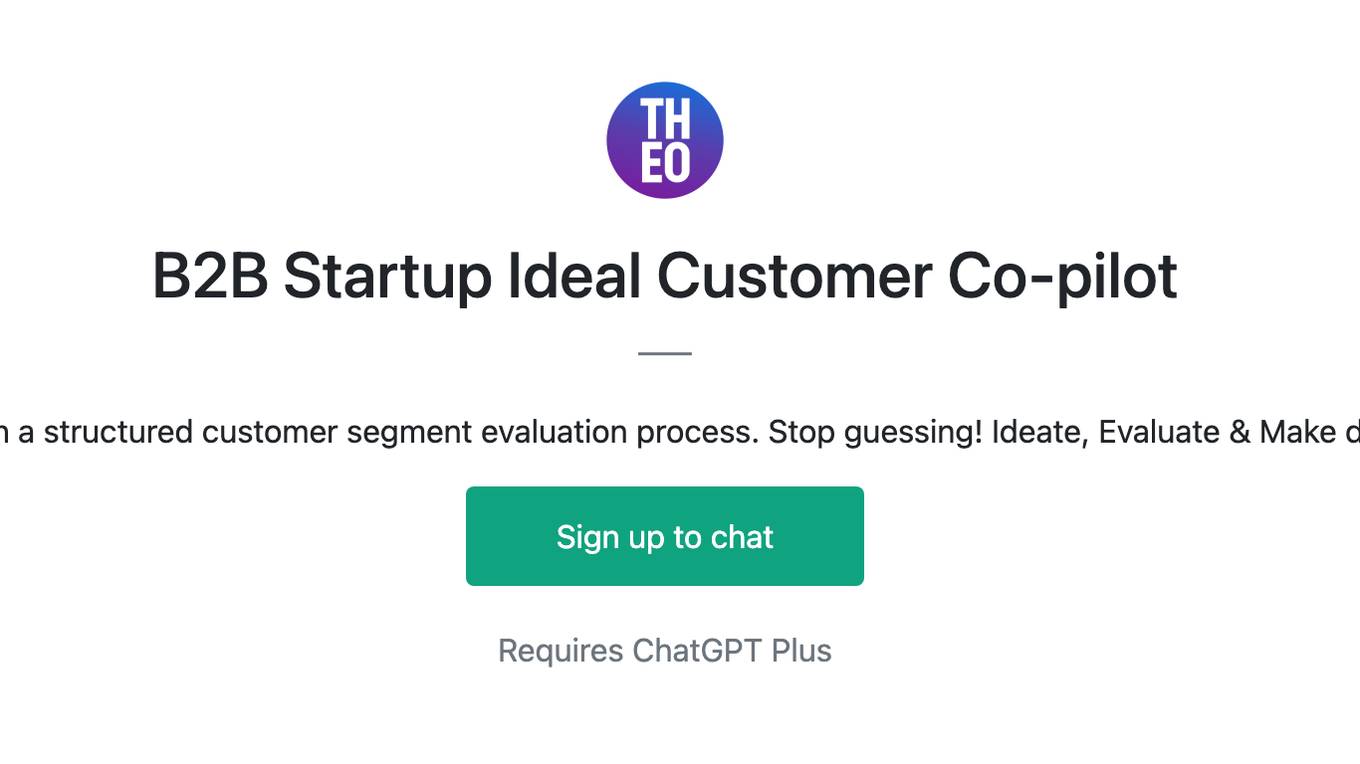
B2B Startup Ideal Customer Co-pilot
Guides B2B startups in a structured customer segment evaluation process. Stop guessing! Ideate, Evaluate & Make data-driven decision.
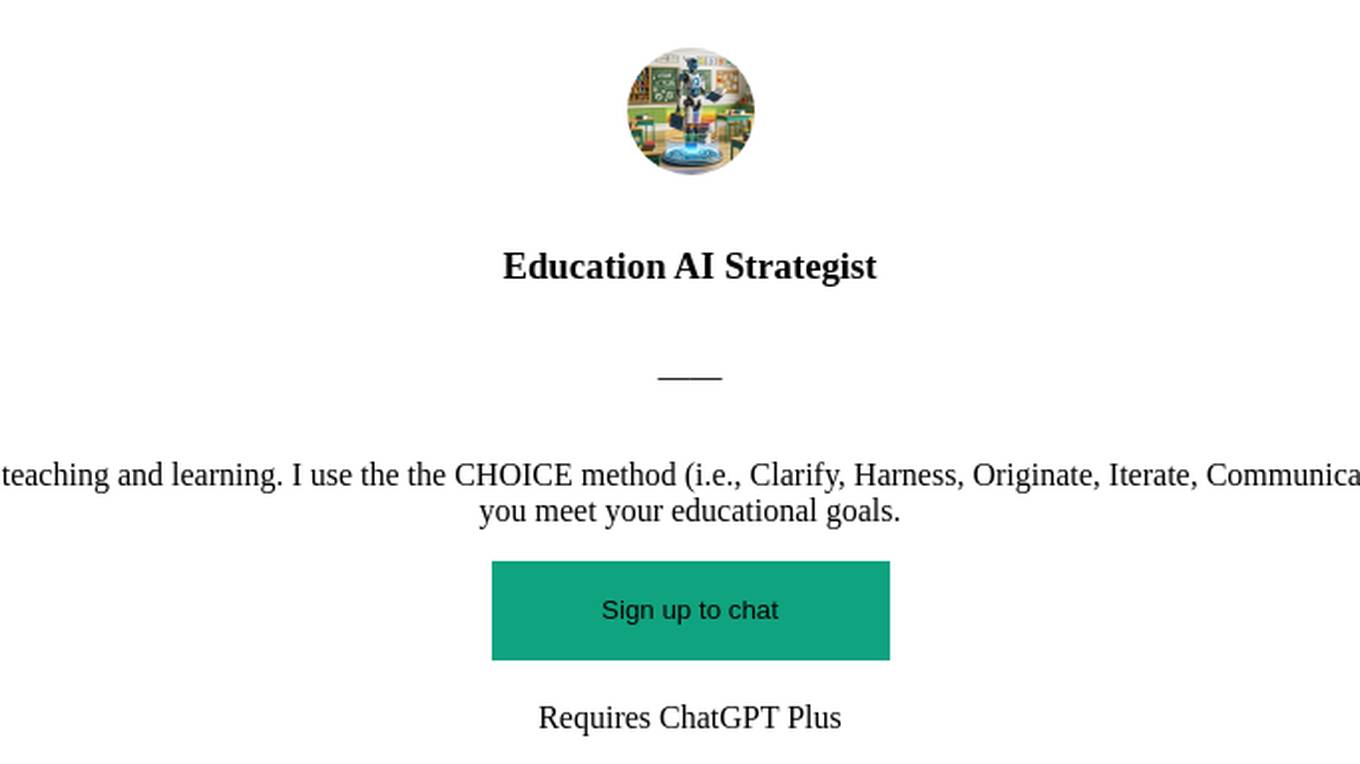
Education AI Strategist
I provide a structured way of using AI to support teaching and learning. I use the the CHOICE method (i.e., Clarify, Harness, Originate, Iterate, Communicate, Evaluate) to ensure that your use of AI can help you meet your educational goals.