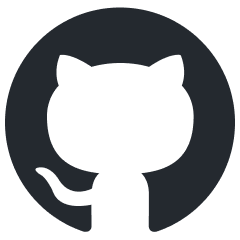
repomix
📦 Repomix (formerly Repopack) is a powerful tool that packs your entire repository into a single, AI-friendly file. Perfect for when you need to feed your codebase to Large Language Models (LLMs) or other AI tools like Claude, ChatGPT, DeepSeek, Perplexity, Gemini, Gemma, Llama, Grok, and more.
Stars: 14239
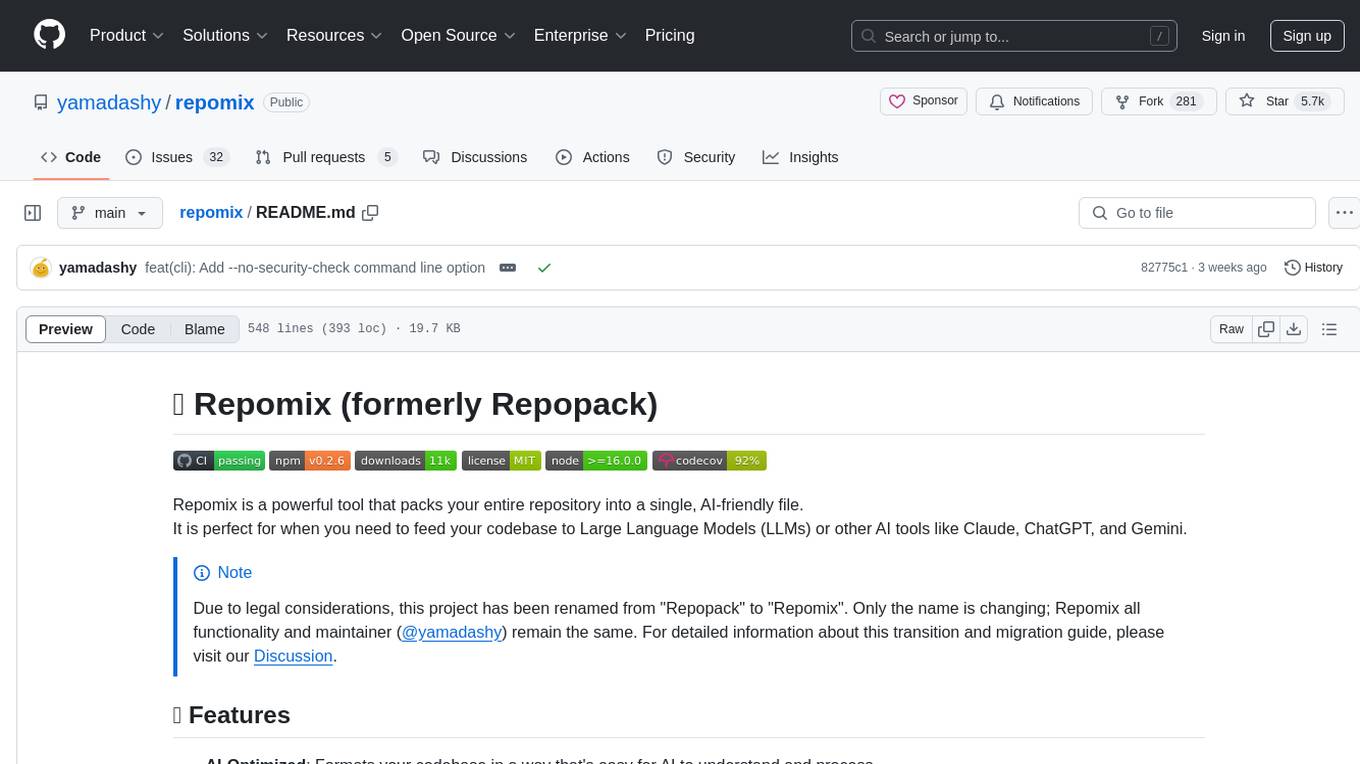
Repomix is a powerful tool that packs your entire repository into a single, AI-friendly file. It is designed to format your codebase for easy understanding by AI tools like Large Language Models (LLMs), Claude, ChatGPT, and Gemini. Repomix offers features such as AI optimization, token counting, simplicity in usage, customization options, Git awareness, and security-focused checks using Secretlint. It allows users to pack their entire repository or specific directories/files using glob patterns, and even supports processing remote Git repositories. The tool generates output in plain text, XML, or Markdown formats, with options for including/excluding files, removing comments, and performing security checks. Repomix also provides a global configuration option, custom instructions for AI context, and a security check feature to detect sensitive information in files.
README:
Use Repomix online! 👉 repomix.com
Need discussion? Join us on Discord!
Share your experience and tips
Stay updated on new features
Get help with configuration and usage
📦 Repomix is a powerful tool that packs your entire repository into a single, AI-friendly file.
It is perfect for when you need to feed your codebase to Large Language Models (LLMs) or other AI tools like Claude,
ChatGPT, DeepSeek, Perplexity, Gemini, Gemma, Llama, Grok, and more.
- Try Repomix in your browser at repomix.com
- Join our Discord Server for support and discussion
We look forward to seeing you there!
- AI-Optimized: Formats your codebase in a way that's easy for AI to understand and process.
- Token Counting: Provides token counts for each file and the entire repository, useful for LLM context limits.
- Simple to Use: You need just one command to pack your entire repository.
- Customizable: Easily configure what to include or exclude.
-
Git-Aware: Automatically respects your
.gitignore
files and.git/info/exclude
. - Security-Focused: Incorporates Secretlint for robust security checks to detect and prevent inclusion of sensitive information.
-
Code Compression: The
--compress
option uses Tree-sitter to extract key code elements, reducing token count while preserving structure.
You can try Repomix instantly in your project directory without installation:
npx repomix
Or install globally for repeated use:
# Install using npm
npm install -g repomix
# Alternatively using yarn
yarn global add repomix
# Alternatively using Homebrew (macOS/Linux)
brew install repomix
# Then run in any project directory
repomix
That's it! Repomix will generate a repomix-output.xml
file in your current directory, containing your entire
repository in an AI-friendly format.
You can then send this file to an AI assistant with a prompt like:
This file contains all the files in the repository combined into one.
I want to refactor the code, so please review it first.
When you propose specific changes, the AI might be able to generate code accordingly. With features like Claude's Artifacts, you could potentially output multiple files, allowing for the generation of multiple interdependent pieces of code.
Happy coding! 🚀
Want to try it quickly? Visit the official website at repomix.com. Simply enter your repository name, fill in any optional details, and click the Pack button to see your generated output.
The website offers several convenient features:
- Customizable output format (XML, Markdown, or Plain Text)
- Instant token count estimation
- Much more!
A community-maintained VSCode extension called Repomix Runner (created by massdo) lets you run Repomix right inside your editor with just a few clicks. Run it on any folder, manage outputs seamlessly, and control everything through VSCode's intuitive interface.
Want your output as a file or just the content? Need automatic cleanup? This extension has you covered. Plus, it works smoothly with your existing repomix.config.json.
Try it now on the VSCode Marketplace! Source code is available on GitHub.
If you're using Python, you might want to check out Gitingest
, which is better suited for Python ecosystem and data
science workflows:
https://github.com/cyclotruc/gitingest
To pack your entire repository:
repomix
To pack a specific directory:
repomix path/to/directory
To pack specific files or directories using glob patterns:
repomix --include "src/**/*.ts,**/*.md"
To exclude specific files or directories:
repomix --ignore "**/*.log,tmp/"
To pack a remote repository:
repomix --remote https://github.com/yamadashy/repomix
# You can also use GitHub shorthand:
repomix --remote yamadashy/repomix
# You can specify the branch name, tag, or commit hash:
repomix --remote https://github.com/yamadashy/repomix --remote-branch main
# Or use a specific commit hash:
repomix --remote https://github.com/yamadashy/repomix --remote-branch 935b695
# Another convenient way is specifying the branch's URL
repomix --remote https://github.com/yamadashy/repomix/tree/main
# Commit's URL is also supported
repomix --remote https://github.com/yamadashy/repomix/commit/836abcd7335137228ad77feb28655d85712680f1
To compress the output:
repomix --compress
# You can also use it with remote repositories:
repomix --remote yamadashy/repomix --compress
To initialize a new configuration file (repomix.config.json
):
repomix --init
Once you have generated the packed file, you can use it with Generative AI tools like ChatGPT, DeepSeek, Perplexity, Gemini, Gemma, Llama, Grok, and more.
You can also run Repomix using Docker.
This is useful if you want to run Repomix in an isolated environment or prefer using containers.
Basic usage (current directory):
docker run -v .:/app -it --rm ghcr.io/yamadashy/repomix
To pack a specific directory:
docker run -v .:/app -it --rm ghcr.io/yamadashy/repomix path/to/directory
Process a remote repository and output to a output
directory:
docker run -v ./output:/app -it --rm ghcr.io/yamadashy/repomix --remote https://github.com/yamadashy/repomix
Once you have generated the packed file with Repomix, you can use it with AI tools like ChatGPT, DeepSeek, Perplexity, Gemini, Gemma, Llama, Grok, and more. Here are some example prompts to get you started:
For a comprehensive code review and refactoring suggestions:
This file contains my entire codebase. Please review the overall structure and suggest any improvements or refactoring opportunities, focusing on maintainability and scalability.
To generate project documentation:
Based on the codebase in this file, please generate a detailed README.md that includes an overview of the project, its main features, setup instructions, and usage examples.
For generating test cases:
Analyze the code in this file and suggest a comprehensive set of unit tests for the main functions and classes. Include edge cases and potential error scenarios.
Evaluate code quality and adherence to best practices:
Review the codebase for adherence to coding best practices and industry standards. Identify areas where the code could be improved in terms of readability, maintainability, and efficiency. Suggest specific changes to align the code with best practices.
Get a high-level understanding of the library
This file contains the entire codebase of library. Please provide a comprehensive overview of the library, including its main purpose, key features, and overall architecture.
Feel free to modify these prompts based on your specific needs and the capabilities of the AI tool you're using.
Check out our community discussion where users share:
- Which AI tools they're using with Repomix
- Effective prompts they've discovered
- How Repomix has helped them
- Tips and tricks for getting the most out of AI code analysis
Feel free to join the discussion and share your own experiences! Your insights could help others make better use of Repomix.
Repomix generates a single file with clear separators between different parts of your codebase.
To enhance AI comprehension, the output file begins with an AI-oriented explanation, making it easier for AI models to
understand the context and structure of the packed repository.
The XML format structures the content in a hierarchical manner:
This file is a merged representation of the entire codebase, combining all repository files into a single document.
<file_summary>
(Metadata and usage AI instructions)
</file_summary>
<directory_structure>
src/
cli/
cliOutput.ts
index.ts
(...remaining directories)
</directory_structure>
<files>
<file path="src/index.js">
// File contents here
</file>
(...remaining files)
</files>
<instruction>
(Custom instructions from `output.instructionFilePath`)
</instruction>
For those interested in the potential of XML tags in AI contexts:
https://docs.anthropic.com/en/docs/build-with-claude/prompt-engineering/use-xml-tags
When your prompts involve multiple components like context, instructions, and examples, XML tags can be a game-changer. They help Claude parse your prompts more accurately, leading to higher-quality outputs.
This means that the XML output from Repomix is not just a different format, but potentially a more effective way to feed your codebase into AI systems for analysis, code review, or other tasks.
To generate output in Markdown format, use the --style markdown
option:
repomix --style markdown
The Markdown format structures the content in a hierarchical manner:
This file is a merged representation of the entire codebase, combining all repository files into a single document.
# File Summary
(Metadata and usage AI instructions)
# Repository Structure
```
src/
cli/
cliOutput.ts
index.ts
```
(...remaining directories)
# Repository Files
## File: src/index.js
```
// File contents here
```
(...remaining files)
# Instruction
(Custom instructions from `output.instructionFilePath`)
This format provides a clean, readable structure that is both human-friendly and easily parseable by AI systems.
To generate output in plain text format, use the --style plain
option:
repomix --style plain
This file is a merged representation of the entire codebase, combining all repository files into a single document.
================================================================
File Summary
================================================================
(Metadata and usage AI instructions)
================================================================
Directory Structure
================================================================
src/
cli/
cliOutput.ts
index.ts
config/
configLoader.ts
(...remaining directories)
================================================================
Files
================================================================
================
File: src/index.js
================
// File contents here
================
File: src/utils.js
================
// File contents here
(...remaining files)
================================================================
Instruction
================================================================
(Custom instructions from `output.instructionFilePath`)
-
-v, --version
: Show tool version
-
-o, --output <file>
: Specify the output file name -
--style <style>
: Specify the output style (xml
,markdown
,plain
) -
--parsable-style
: Enable parsable output based on the chosen style schema. Note that this can increase token count. -
--compress
: Perform intelligent code extraction, focusing on essential function and class signatures to reduce token count -
--output-show-line-numbers
: Show line numbers in the output -
--copy
: Additionally copy generated output to system clipboard -
--no-file-summary
: Disable file summary section output -
--no-directory-structure
: Disable directory structure section output -
--remove-comments
: Remove comments from supported file types -
--remove-empty-lines
: Remove empty lines from the output -
--header-text <text>
: Custom text to include in the file header -
--instruction-file-path <path>
: Path to a file containing detailed custom instructions -
--include-empty-directories
: Include empty directories in the output -
--no-git-sort-by-changes
: Disable sorting files by git change count (enabled by default)
-
--include <patterns>
: List of include patterns (comma-separated) -
-i, --ignore <patterns>
: Additional ignore patterns (comma-separated) -
--no-gitignore
: Disable .gitignore file usage -
--no-default-patterns
: Disable default patterns
-
--remote <url>
: Process a remote Git repository -
--remote-branch <name>
: Specify the remote branch name, tag, or commit hash (defaults to repository default branch)
-
-c, --config <path>
: Path to a custom config file -
--init
: Create config file -
--global
: Use global config
-
--no-security-check
: Disable security check
-
--token-count-encoding <encoding>
: Specify token count encoding used by OpenAI's tiktoken tokenizer (e.g.,o200k_base
for GPT-4o,cl100k_base
for GPT-4/3.5). See tiktoken model.py for encoding details.
-
--mcp
: Run as a MCP (Model Context Protocol) server
-
--top-files-len <number>
: Number of top files to display in the summary -
--verbose
: Enable verbose logging -
--quiet
: Disable all output to stdout
Examples:
repomix -o custom-output.txt
repomix -i "*.log,tmp" -v
repomix -c ./custom-config.json
repomix --style xml
repomix --remote https://github.com/user/repo
npx repomix src
To update a globally installed Repomix:
# Using npm
npm update -g repomix
# Using yarn
yarn global upgrade repomix
Using npx repomix
is generally more convenient as it always uses the latest version.
Repomix supports processing remote Git repositories without the need for manual cloning. This feature allows you to quickly analyze any public Git repository with a single command.
To process a remote repository, use the --remote
option followed by the repository URL:
repomix --remote https://github.com/yamadashy/repomix
You can also use GitHub's shorthand format:
repomix --remote yamadashy/repomix
You can specify the branch name, tag, or commit hash:
# Using --remote-branch option
repomix --remote https://github.com/yamadashy/repomix --remote-branch main
# Using branch's URL
repomix --remote https://github.com/yamadashy/repomix/tree/main
Or use a specific commit hash:
# Using --remote-branch option
repomix --remote https://github.com/yamadashy/repomix --remote-branch 935b695
# Using commit's URL
repomix --remote https://github.com/yamadashy/repomix/commit/836abcd7335137228ad77feb28655d85712680f1
The --compress
option utilizes Tree-sitter to perform intelligent code extraction, focusing on essential function and class signatures while removing implementation details. This can help reduce token count while retaining important structural information.
repomix --compress
For example, this code:
import { ShoppingItem } from './shopping-item';
/**
* Calculate the total price of shopping items
*/
const calculateTotal = (
items: ShoppingItem[]
) => {
let total = 0;
for (const item of items) {
total += item.price * item.quantity;
}
return total;
}
// Shopping item interface
interface Item {
name: string;
price: number;
quantity: number;
}
Will be compressed to:
import { ShoppingItem } from './shopping-item';
⋮----
/**
* Calculate the total price of shopping items
*/
const calculateTotal = (
items: ShoppingItem[]
) => {
⋮----
// Shopping item interface
interface Item {
name: string;
price: number;
quantity: number;
}
[!NOTE] This is an experimental feature that we'll be actively improving based on user feedback and real-world usage
Repomix supports the Model Context Protocol (MCP), allowing AI assistants to directly interact with your codebase. When run as an MCP server, Repomix provides tools that enable AI assistants to package local or remote repositories for analysis without requiring manual file preparation.
repomix --mcp
To use Repomix as an MCP server with AI assistants like Claude, you need to configure the MCP settings:
For VS Code:
You can install the Repomix MCP server in VS Code using one of these methods:
-
Using the Install Badge:
-
Using the Command Line:
code --add-mcp '{"name":"repomix","command":"npx","args":["-y","repomix","--mcp"]}'
For VS Code Insiders:
code-insiders --add-mcp '{"name":"repomix","command":"npx","args":["-y","repomix","--mcp"]}'
For Cline (VS Code extension):
Edit the cline_mcp_settings.json
file:
{
"mcpServers": {
"repomix": {
"command": "npx",
"args": [
"-y",
"repomix",
"--mcp"
]
}
}
}
For Cursor:
In Cursor, add a new MCP server from Cursor Settings
> MCP
> + Add new global MCP server
with a configuration similar to Cline.
For Claude Desktop:
Edit the claude_desktop_config.json
file with similar configuration to Cline's config.
Once configured, your AI assistant can directly use Repomix's capabilities to analyze codebases without manual file preparation, making code analysis workflows more efficient.
When running as an MCP server, Repomix provides the following tools:
- pack_codebase: Package a local code directory into a consolidated file for AI analysis
- Parameters:
-
directory
: Absolute path to the directory to pack -
compress
: (Optional, default: true) Whether to perform intelligent code extraction -
includePatterns
: (Optional) Comma-separated list of include patterns -
ignorePatterns
: (Optional) Comma-separated list of ignore patterns
-
- pack_remote_repository: Fetch, clone and package a GitHub repository
- Parameters:
-
remote
: GitHub repository URL or user/repo format (e.g., yamadashy/repomix) -
compress
: (Optional, default: true) Whether to perform intelligent code extraction -
includePatterns
: (Optional) Comma-separated list of include patterns -
ignorePatterns
: (Optional) Comma-separated list of ignore patterns
-
- read_repomix_output: Read the contents of a Repomix output file in environments where direct file access is not possible
- Parameters:
-
outputId
: ID of the Repomix output file to read
-
- Features:
- Specifically designed for web-based environments or sandboxed applications
- Retrieves the content of previously generated outputs using their ID
- Provides secure access to packed codebase without requiring file system access
- file_system_read_file: Read a file using an absolute path with security validation
- Parameters:
-
path
: Absolute path to the file to read
-
- Security features:
- Implements security validation using Secretlint
- Prevents access to files containing sensitive information
- Validates absolute paths to prevent directory traversal attacks
- file_system_read_directory: List contents of a directory using an absolute path
- Parameters:
-
path
: Absolute path to the directory to list
-
- Features:
- Shows files and directories with clear indicators (
[FILE]
or[DIR]
) - Provides safe directory traversal with proper error handling
- Validates paths and ensures they are absolute
- Shows files and directories with clear indicators (
Create a repomix.config.json
file in your project root for custom configurations.
repomix --init
Here's an explanation of the configuration options:
Option | Description | Default |
---|---|---|
output.filePath |
The name of the output file | "repomix-output.xml" |
output.style |
The style of the output (xml , markdown , plain ) |
"xml" |
output.parsableStyle |
Whether to escape the output based on the chosen style schema. Note that this can increase token count. | false |
output.compress |
Whether to perform intelligent code extraction to reduce token count | false |
output.headerText |
Custom text to include in the file header | null |
output.instructionFilePath |
Path to a file containing detailed custom instructions | null |
output.fileSummary |
Whether to include a summary section at the beginning of the output | true |
output.directoryStructure |
Whether to include the directory structure in the output | true |
output.removeComments |
Whether to remove comments from supported file types | false |
output.removeEmptyLines |
Whether to remove empty lines from the output | false |
output.showLineNumbers |
Whether to add line numbers to each line in the output | false |
output.copyToClipboard |
Whether to copy the output to system clipboard in addition to saving the file | false |
output.topFilesLength |
Number of top files to display in the summary. If set to 0, no summary will be displayed | 5 |
output.includeEmptyDirectories |
Whether to include empty directories in the repository structure | false |
output.git.sortByChanges |
Whether to sort files by git change count (files with more changes appear at the bottom) | true |
output.git.sortByChangesMaxCommits |
Maximum number of commits to analyze for git changes | 100 |
include |
Patterns of files to include (using glob patterns) | [] |
ignore.useGitignore |
Whether to use patterns from the project's .gitignore file |
true |
ignore.useDefaultPatterns |
Whether to use default ignore patterns | true |
ignore.customPatterns |
Additional patterns to ignore (using glob patterns) | [] |
security.enableSecurityCheck |
Whether to perform security checks on files | true |
tokenCount.encoding |
Token count encoding used by OpenAI's tiktoken tokenizer (e.g., o200k_base for GPT-4o, cl100k_base for GPT-4/3.5). See tiktoken model.py for encoding details. |
"o200k_base" |
The configuration file supports JSON5 syntax, which allows:
- Comments (both single-line and multi-line)
- Trailing commas in objects and arrays
- Unquoted property names
- More relaxed string syntax
Example configuration:
{
"output": {
"filePath": "repomix-output.xml",
"style": "xml",
"parsableStyle": true,
"compress": false,
"headerText": "Custom header information for the packed file.",
"fileSummary": true,
"directoryStructure": true,
"removeComments": false,
"removeEmptyLines": false,
"showLineNumbers": false,
"copyToClipboard": true,
"topFilesLength": 5,
"includeEmptyDirectories": false,
"git": {
"sortByChanges": true,
"sortByChangesMaxCommits": 100
}
},
"include": [
"**/*"
],
"ignore": {
"useGitignore": true,
"useDefaultPatterns": true,
// Patterns can also be specified in .repomixignore
"customPatterns": [
"additional-folder",
"**/*.log"
],
},
"security": {
"enableSecurityCheck": true
},
"tokenCount": {
"encoding": "o200k_base"
},
}
To create a global configuration file:
repomix --init --global
The global configuration file will be created in:
- Windows:
%LOCALAPPDATA%\Repomix\repomix.config.json
- macOS/Linux:
$XDG_CONFIG_HOME/repomix/repomix.config.json
or~/.config/repomix/repomix.config.json
Note: Local configuration (if present) takes precedence over global configuration.
Repomix now supports specifying files to include using glob patterns. This allows for more flexible and powerful file selection:
- Use
**/*.js
to include all JavaScript files in any directory - Use
src/**/*
to include all files within thesrc
directory and its subdirectories - Combine multiple patterns like
["src/**/*.js", "**/*.md"]
to include JavaScript files insrc
and all Markdown files
Repomix offers multiple methods to set ignore patterns for excluding specific files or directories during the packing process:
-
.gitignore: By default, patterns listed in your project's
.gitignore
files and.git/info/exclude
are used. This behavior can be controlled with theignore.useGitignore
setting or the--no-gitignore
cli option. -
Default patterns: Repomix includes a default list of commonly excluded files and directories (e.g., node_modules,
.git, binary files). This feature can be controlled with the
ignore.useDefaultPatterns
setting or the--no-default-patterns
cli option. Please see defaultIgnore.ts for more details. -
.repomixignore: You can create a
.repomixignore
file in your project root to define Repomix-specific ignore patterns. This file follows the same format as.gitignore
. -
Custom patterns: Additional ignore patterns can be specified using the
ignore.customPatterns
option in the configuration file. You can overwrite this setting with the-i, --ignore
command line option.
Priority Order (from highest to lowest):
- Custom patterns
ignore.customPatterns
.repomixignore
-
.gitignore
and.git/info/exclude
(ifignore.useGitignore
is true and--no-gitignore
is not used) - Default patterns (if
ignore.useDefaultPatterns
is true and--no-default-patterns
is not used)
This approach allows for flexible file exclusion configuration based on your project's needs. It helps optimize the size of the generated pack file by ensuring the exclusion of security-sensitive files and large binary files, while preventing the leakage of confidential information.
Note: Binary files are not included in the packed output by default, but their paths are listed in the "Repository Structure" section of the output file. This provides a complete overview of the repository structure while keeping the packed file efficient and text-based.
The output.instructionFilePath
option allows you to specify a separate file containing detailed instructions or
context about your project. This allows AI systems to understand the specific context and requirements of your project,
potentially leading to more relevant and tailored analysis or suggestions.
Here's an example of how you might use this feature:
- Create a file named
repomix-instruction.md
in your project root:
# Coding Guidelines
- Follow the Airbnb JavaScript Style Guide
- Suggest splitting files into smaller, focused units when appropriate
- Add comments for non-obvious logic. Keep all text in English
- All new features should have corresponding unit tests
# Generate Comprehensive Output
- Include all content without abbreviation, unless specified otherwise
- Optimize for handling large codebases while maintaining output quality
- In your
repomix.config.json
, add theinstructionFilePath
option:
{
"output": {
"instructionFilePath": "repomix-instruction.md",
// other options...
}
}
When Repomix generates the output, it will include the contents of repomix-instruction.md
in a dedicated section.
Note: The instruction content is appended at the end of the output file. This placement can be particularly effective
for AI systems. For those interested in understanding why this might be beneficial, Anthropic provides some insights in
their documentation:
https://docs.anthropic.com/en/docs/build-with-claude/prompt-engineering/long-context-tips
Put long-form data at the top: Place your long documents and inputs (~20K+ tokens) near the top of your prompt, above your query, instructions, and examples. This can significantly improve Claude's performance across all models. Queries at the end can improve response quality by up to 30% in tests, especially with complex, multi-document inputs.
When output.removeComments
is set to true
, Repomix will attempt to remove comments from supported file types. This
feature can help reduce the size of the output file and focus on the essential code content.
Supported languages include:
HTML, CSS, JavaScript, TypeScript, Vue, Svelte, Python, PHP, Ruby, C, C#, Java, Go, Rust, Swift, Kotlin, Dart, Shell,
and YAML.
Note: The comment removal process is conservative to avoid accidentally removing code. In complex cases, some comments might be retained.
Repomix includes a security check feature that uses Secretlint to detect potentially sensitive information in your files. This feature helps you identify possible security risks before sharing your packed repository.
The security check results will be displayed in the CLI output after the packing process is complete. If any suspicious files are detected, you'll see a list of these files along with a warning message.
Example output:
🔍 Security Check:
──────────────────
2 suspicious file(s) detected:
1. src/utils/test.txt
2. tests/utils/secretLintUtils.test.ts
Please review these files for potentially sensitive information.
By default, Repomix's security check feature is enabled. You can disable it by setting security.enableSecurityCheck
to
false
in your configuration file:
{
"security": {
"enableSecurityCheck": false
}
}
Or using the --no-security-check
command line option:
repomix --no-security-check
[!NOTE] Disabling security checks may expose sensitive information. Use this option with caution and only when necessary, such as when working with test files or documentation that contains example credentials.
We welcome contributions from the community! To get started, please refer to our Contributing Guide.
- Data Collection: The Repomix CLI tool does not collect, transmit, or store any user data, telemetry, or repository information.
-
Network Usage: Repomix CLI operates fully offline after installation. The only cases where an internet connection is needed are:
- Installation via npm/yarn.
- Using the
--remote
flag to process remote repositories. - Checking for updates (manually triggered).
- Security Considerations: Since all processing is local, Repomix CLI is safe to use with private and internal repositories.
Repomix Website (repomix.com)
- Data Collection: The Repomix website uses Google Analytics to collect usage data, such as page views and user interactions. This helps us understand how the website is used and improve the user experience.
Repomix (both the CLI tool and the website) is provided "as is" without any warranties or guarantees.
We do not take responsibility for how the generated output is used, including but not limited to its accuracy, legality, or any potential consequences arising from its use.
This project is licensed under the MIT License.
For Tasks:
Click tags to check more tools for each tasksFor Jobs:
Alternative AI tools for repomix
Similar Open Source Tools
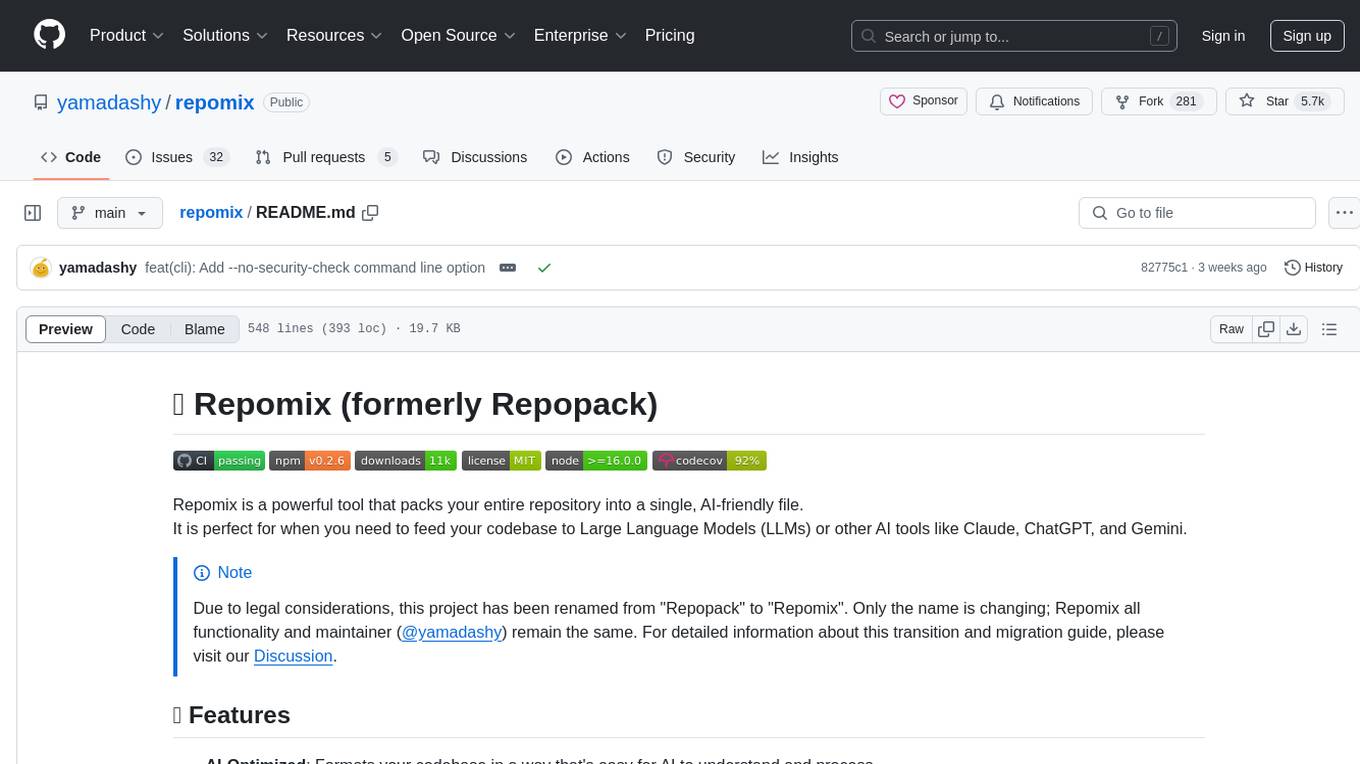
repomix
Repomix is a powerful tool that packs your entire repository into a single, AI-friendly file. It is designed to format your codebase for easy understanding by AI tools like Large Language Models (LLMs), Claude, ChatGPT, and Gemini. Repomix offers features such as AI optimization, token counting, simplicity in usage, customization options, Git awareness, and security-focused checks using Secretlint. It allows users to pack their entire repository or specific directories/files using glob patterns, and even supports processing remote Git repositories. The tool generates output in plain text, XML, or Markdown formats, with options for including/excluding files, removing comments, and performing security checks. Repomix also provides a global configuration option, custom instructions for AI context, and a security check feature to detect sensitive information in files.
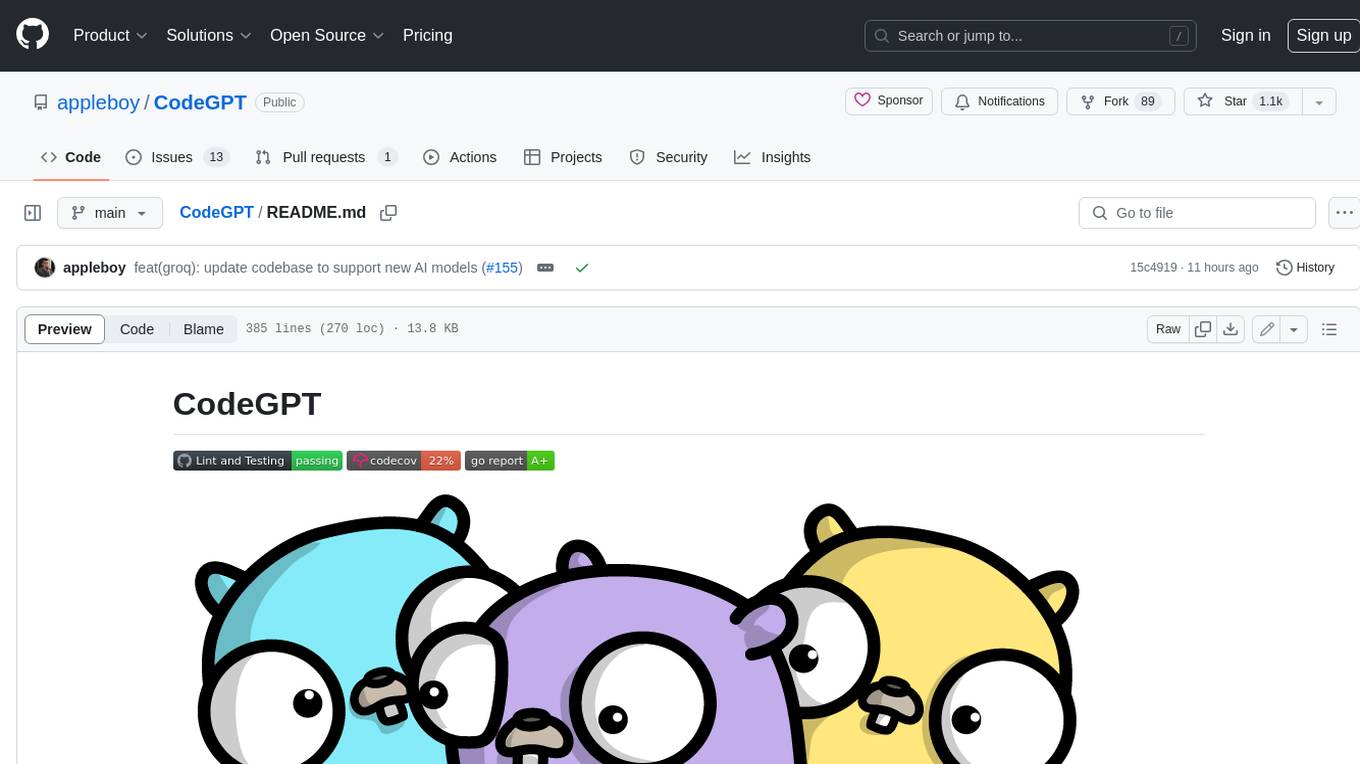
CodeGPT
CodeGPT is a CLI tool written in Go that helps you write git commit messages or do a code review brief using ChatGPT AI (gpt-3.5-turbo, gpt-4 model) and automatically installs a git prepare-commit-msg hook. It supports Azure OpenAI Service or OpenAI API, conventional commits specification, Git prepare-commit-msg Hook, customizing the number of lines of context in diffs, excluding files from the git diff command, translating commit messages into different languages, using socks or custom network HTTP proxies, specifying model lists, and doing brief code reviews.
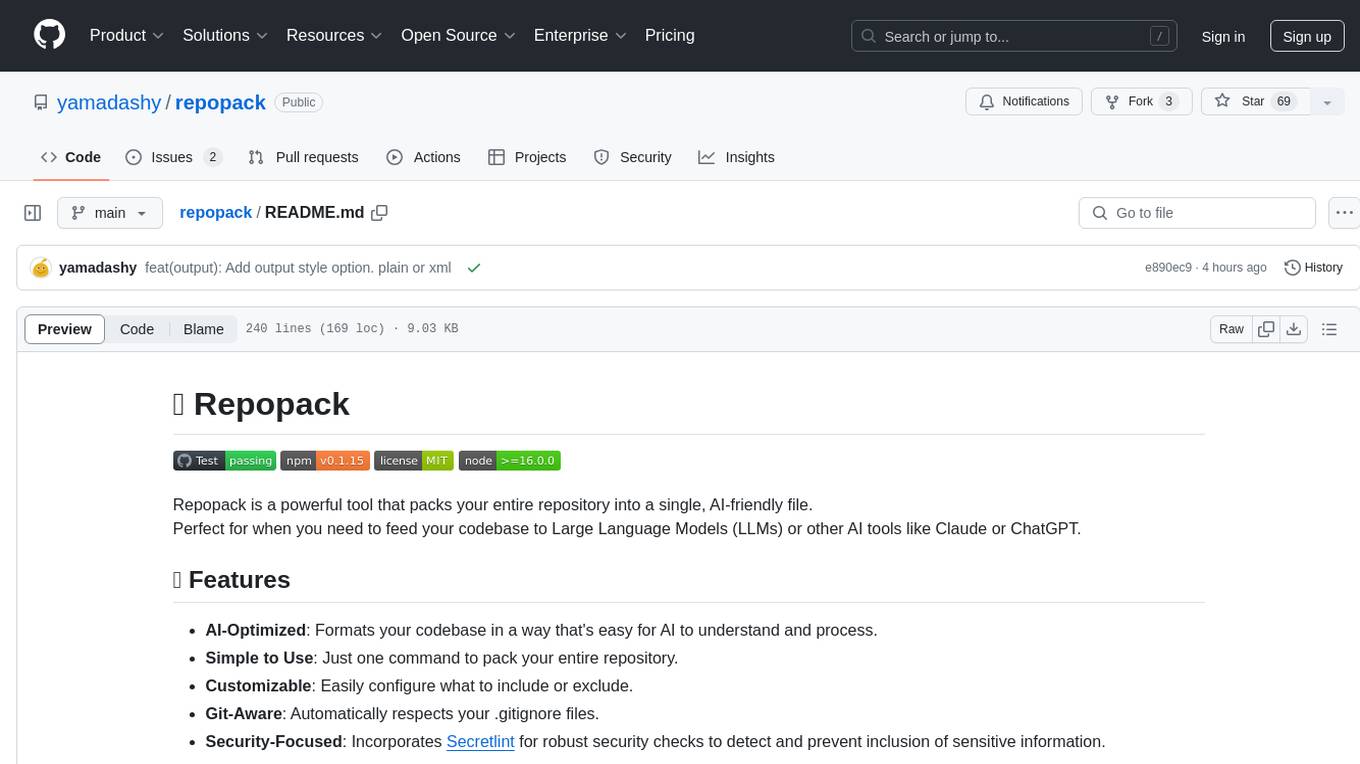
repopack
Repopack is a powerful tool that packs your entire repository into a single, AI-friendly file. It optimizes your codebase for AI comprehension, is simple to use with customizable options, and respects Gitignore files for security. The tool generates a packed file with clear separators and AI-oriented explanations, making it ideal for use with Generative AI tools like Claude or ChatGPT. Repopack offers command line options, configuration settings, and multiple methods for setting ignore patterns to exclude specific files or directories during the packing process. It includes features like comment removal for supported file types and a security check using Secretlint to detect sensitive information in files.
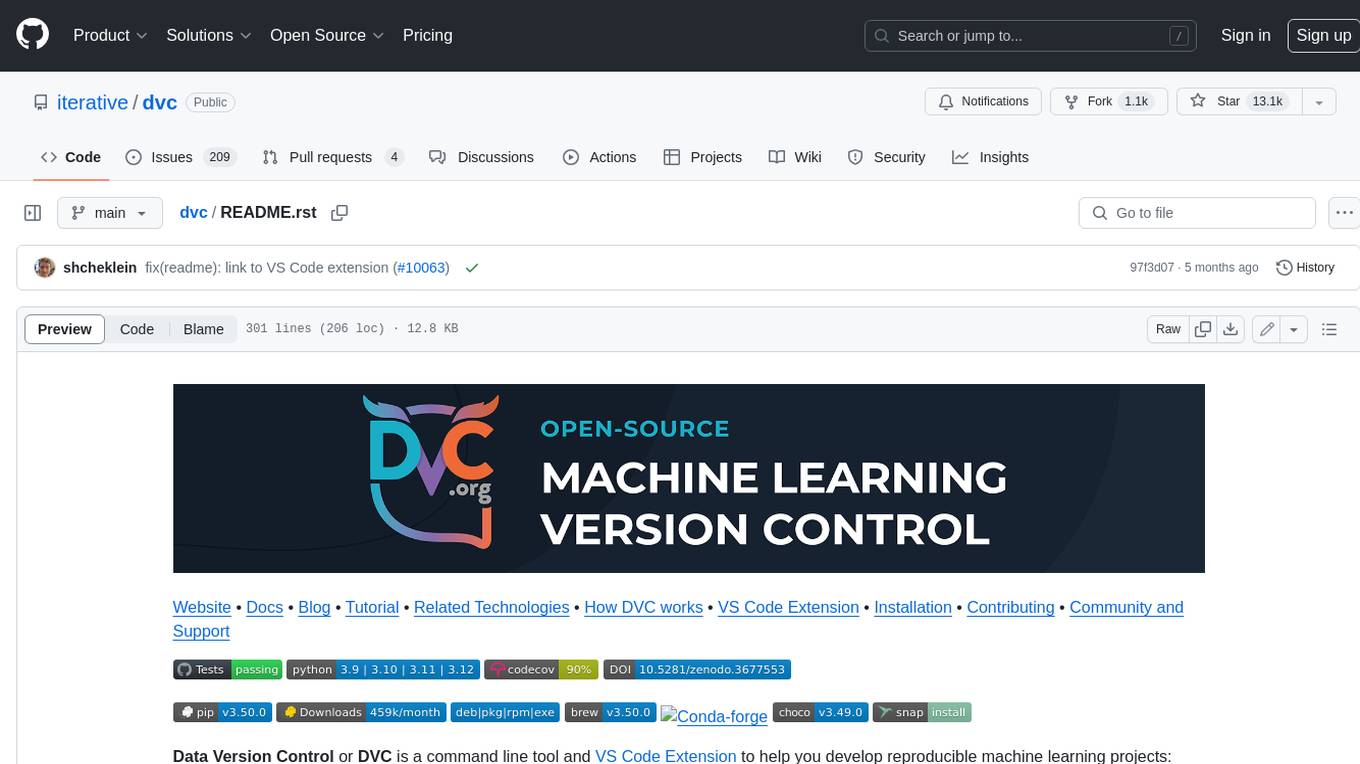
dvc
DVC, or Data Version Control, is a command-line tool and VS Code extension that helps you develop reproducible machine learning projects. With DVC, you can version your data and models, iterate fast with lightweight pipelines, track experiments in your local Git repo, compare any data, code, parameters, model, or performance plots, and share experiments and automatically reproduce anyone's experiment.
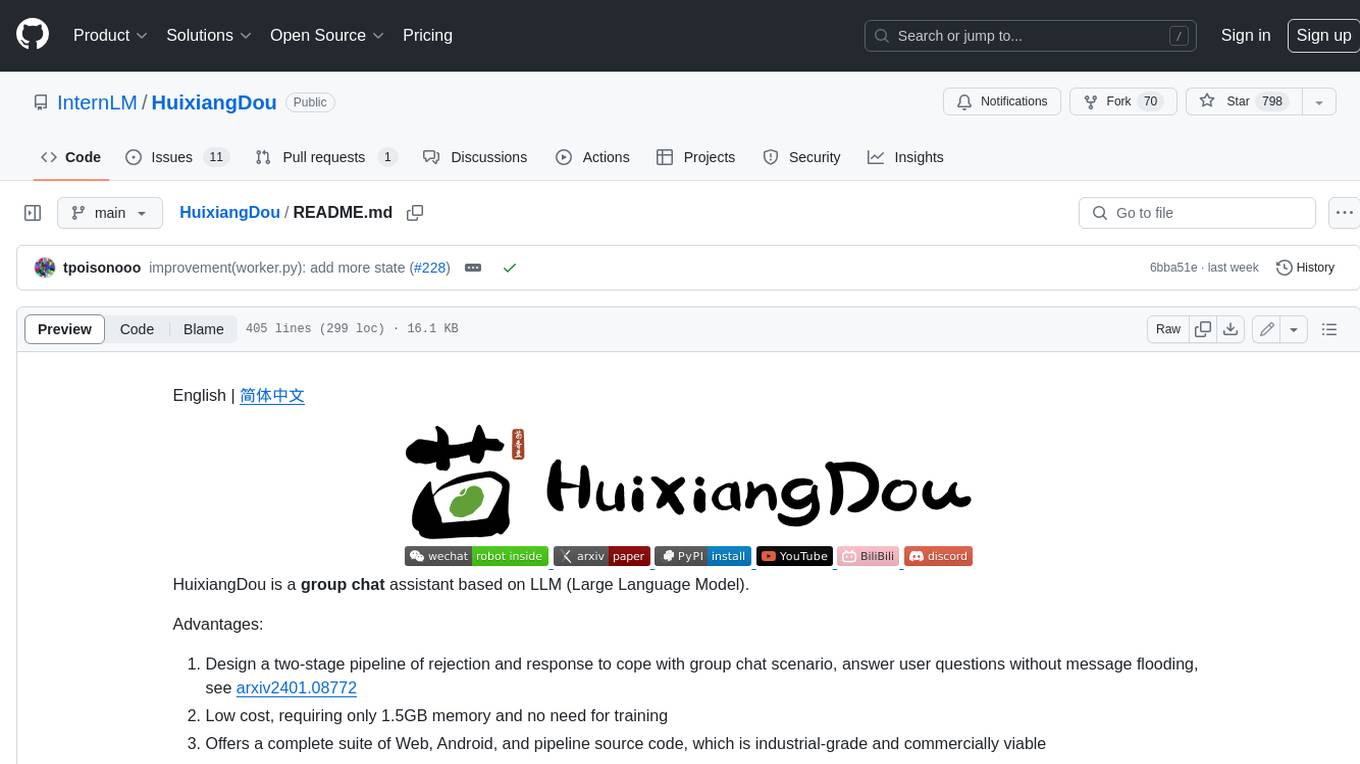
HuixiangDou
HuixiangDou is a **group chat** assistant based on LLM (Large Language Model). Advantages: 1. Design a two-stage pipeline of rejection and response to cope with group chat scenario, answer user questions without message flooding, see arxiv2401.08772 2. Low cost, requiring only 1.5GB memory and no need for training 3. Offers a complete suite of Web, Android, and pipeline source code, which is industrial-grade and commercially viable Check out the scenes in which HuixiangDou are running and join WeChat Group to try AI assistant inside. If this helps you, please give it a star ⭐
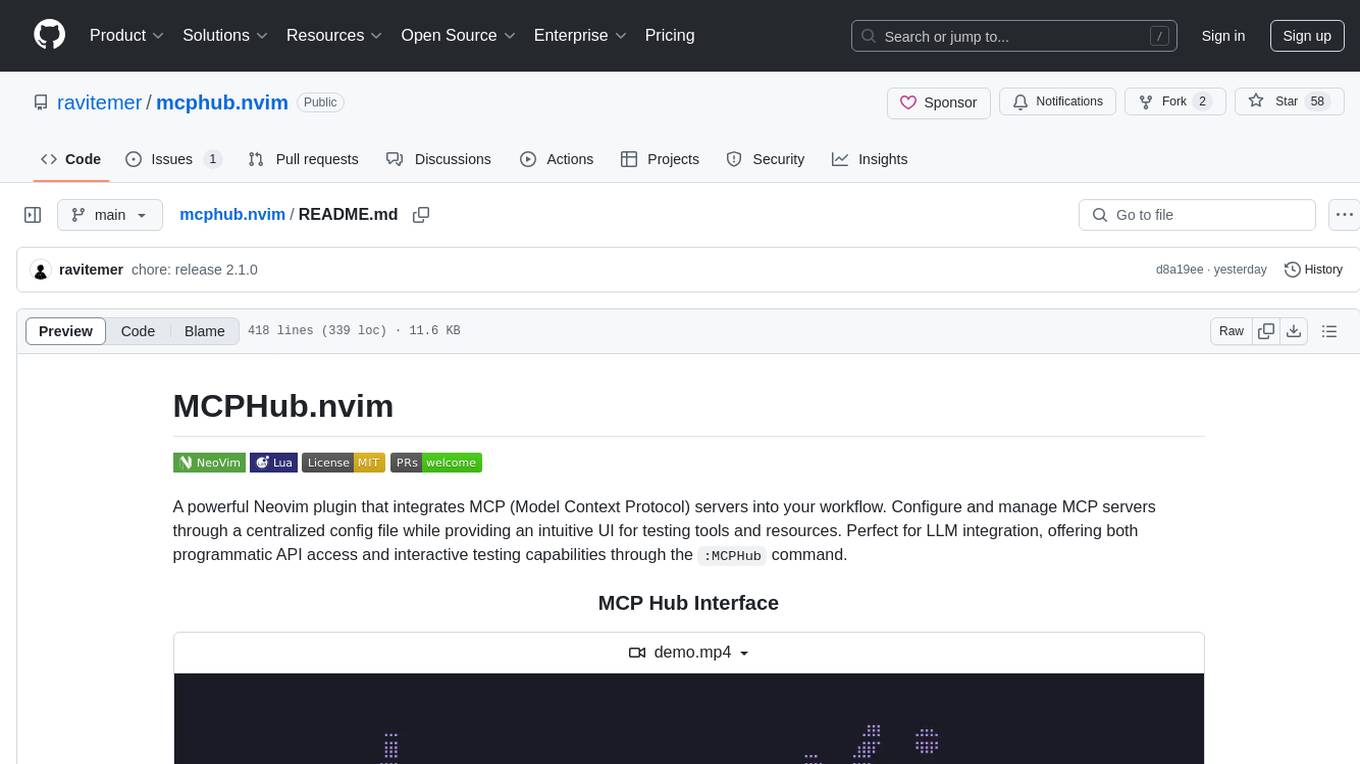
mcphub.nvim
MCPHub.nvim is a powerful Neovim plugin that integrates MCP (Model Context Protocol) servers into your workflow. It offers a centralized config file for managing servers and tools, with an intuitive UI for testing resources. Ideal for LLM integration, it provides programmatic API access and interactive testing through the `:MCPHub` command.
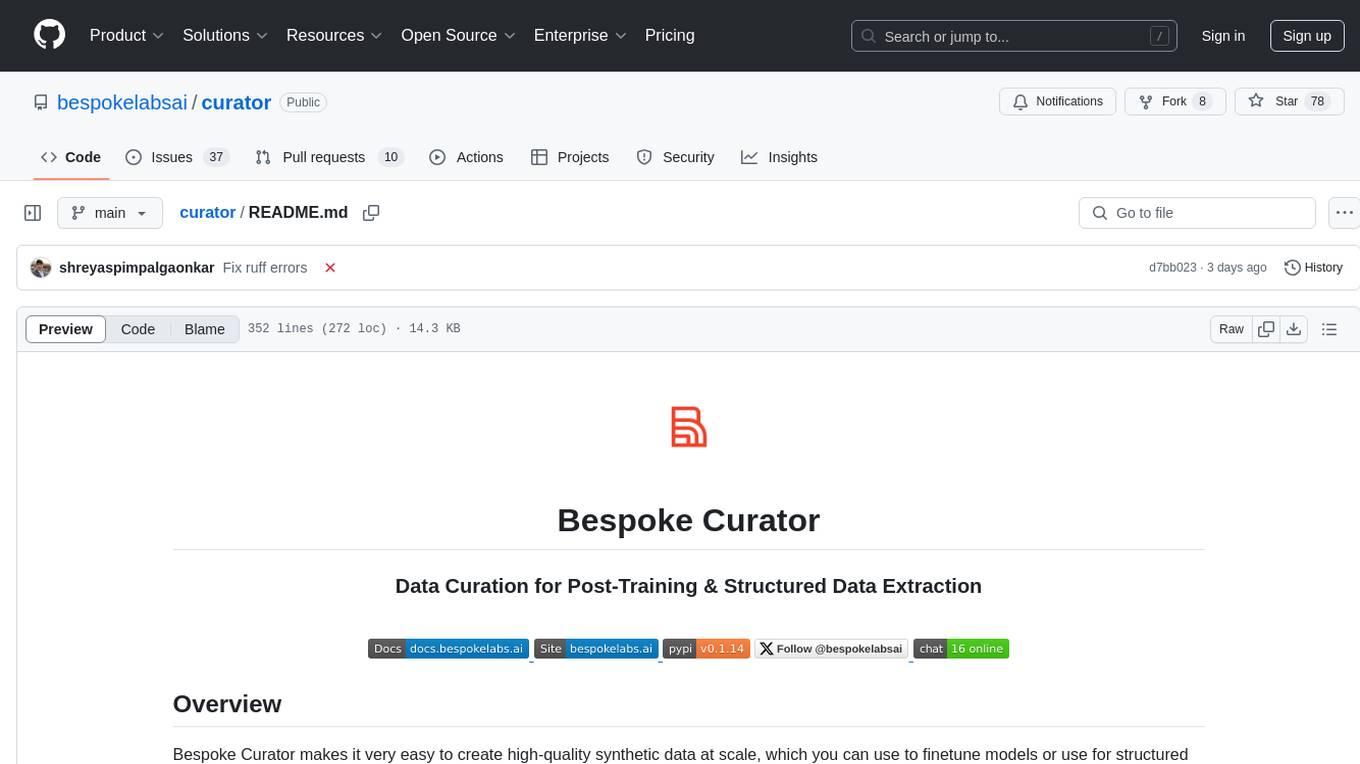
curator
Bespoke Curator is an open-source tool for data curation and structured data extraction. It provides a Python library for generating synthetic data at scale, with features like programmability, performance optimization, caching, and integration with HuggingFace Datasets. The tool includes a Curator Viewer for dataset visualization and offers a rich set of functionalities for creating and refining data generation strategies.
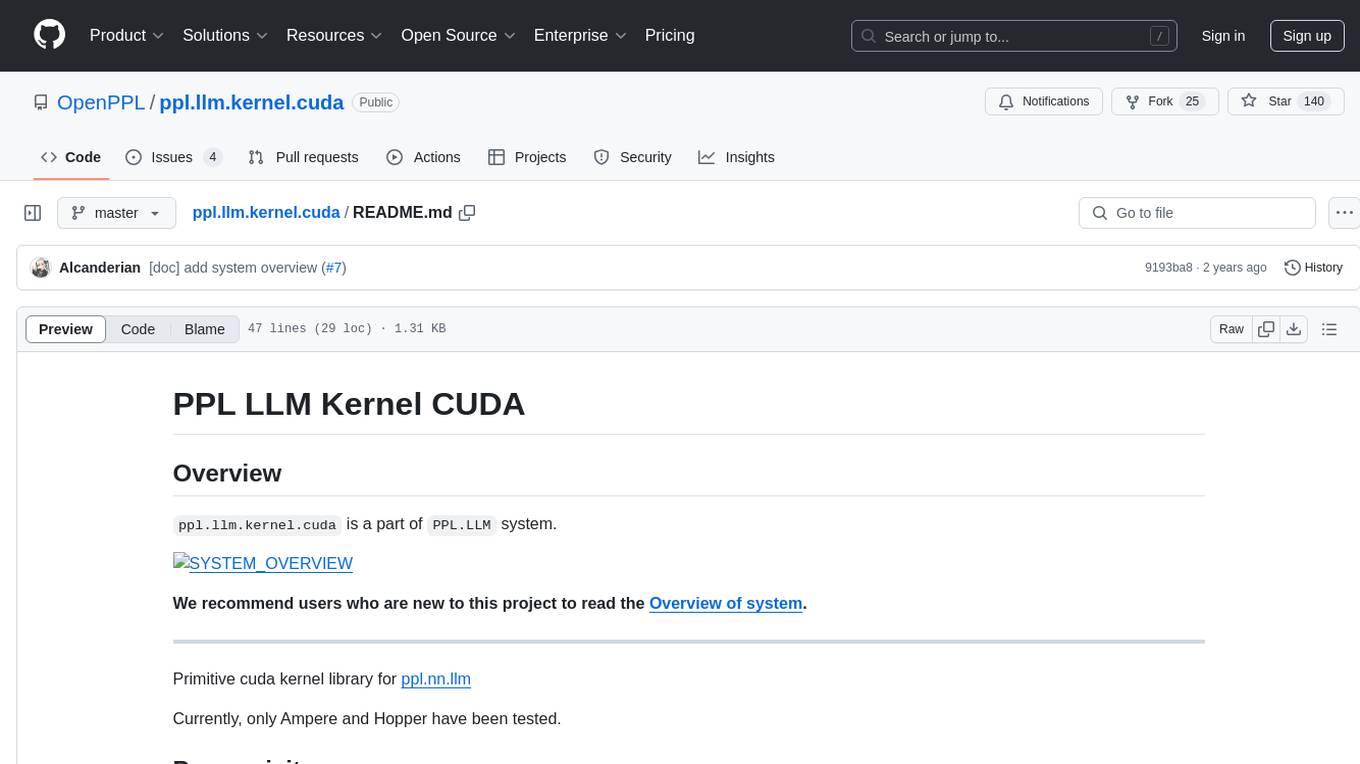
ppl.llm.kernel.cuda
Primitive cuda kernel library for ppl.nn.llm, part of PPL.LLM system, tested on Ampere and Hopper, requires Linux on x86_64 or arm64 CPUs, GCC >= 9.4.0, CMake >= 3.18, Git >= 2.7.0, CUDA Toolkit >= 11.4. 11.6 recommended. Provides cuda kernel functionalities for deep learning tasks.
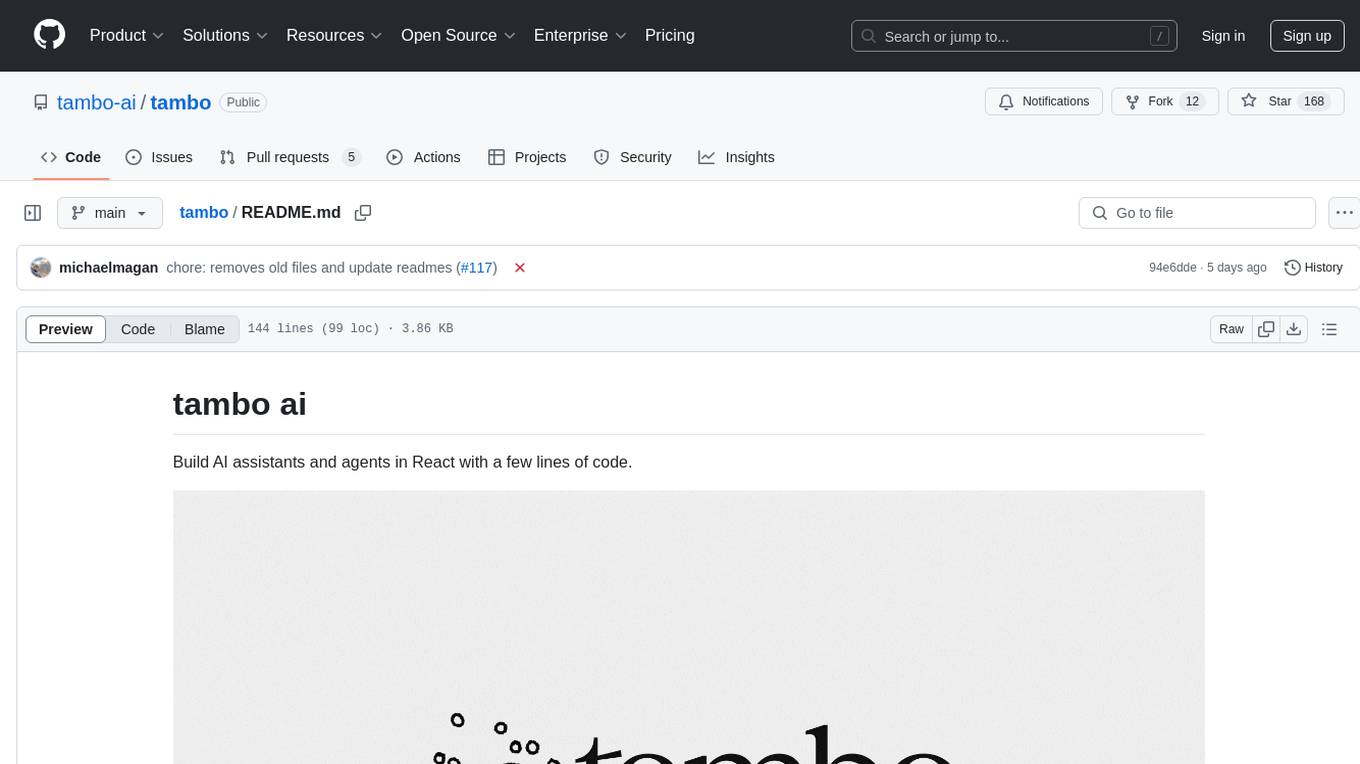
tambo
tambo ai is a React library that simplifies the process of building AI assistants and agents in React by handling thread management, state persistence, streaming responses, AI orchestration, and providing a compatible React UI library. It eliminates React boilerplate for AI features, allowing developers to focus on creating exceptional user experiences with clean React hooks that seamlessly integrate with their codebase.
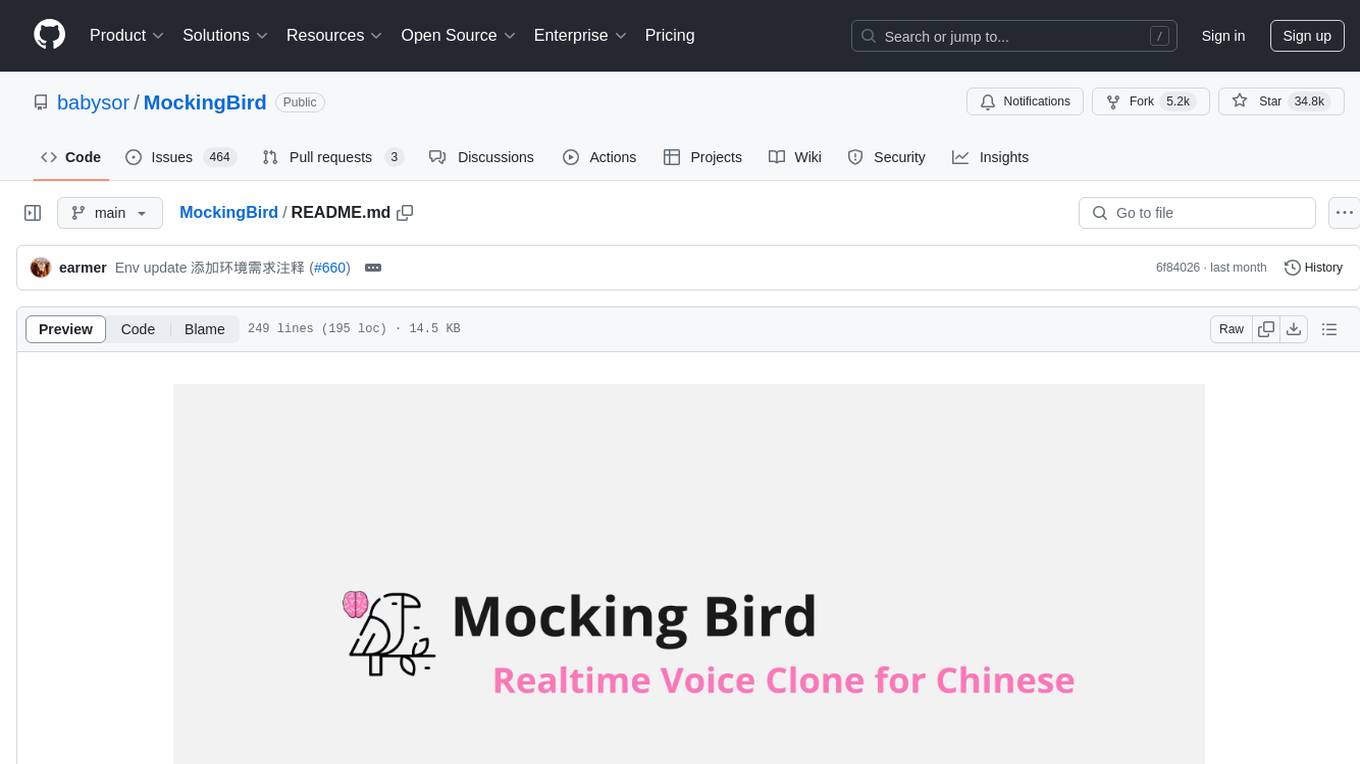
MockingBird
MockingBird is a toolbox designed for Mandarin speech synthesis using PyTorch. It supports multiple datasets such as aidatatang_200zh, magicdata, aishell3, and data_aishell. The toolbox can run on Windows, Linux, and M1 MacOS, providing easy and effective speech synthesis with pretrained encoder/vocoder models. It is webserver ready for remote calling. Users can train their own models or use existing ones for the encoder, synthesizer, and vocoder. The toolbox offers a demo video and detailed setup instructions for installation and model training.
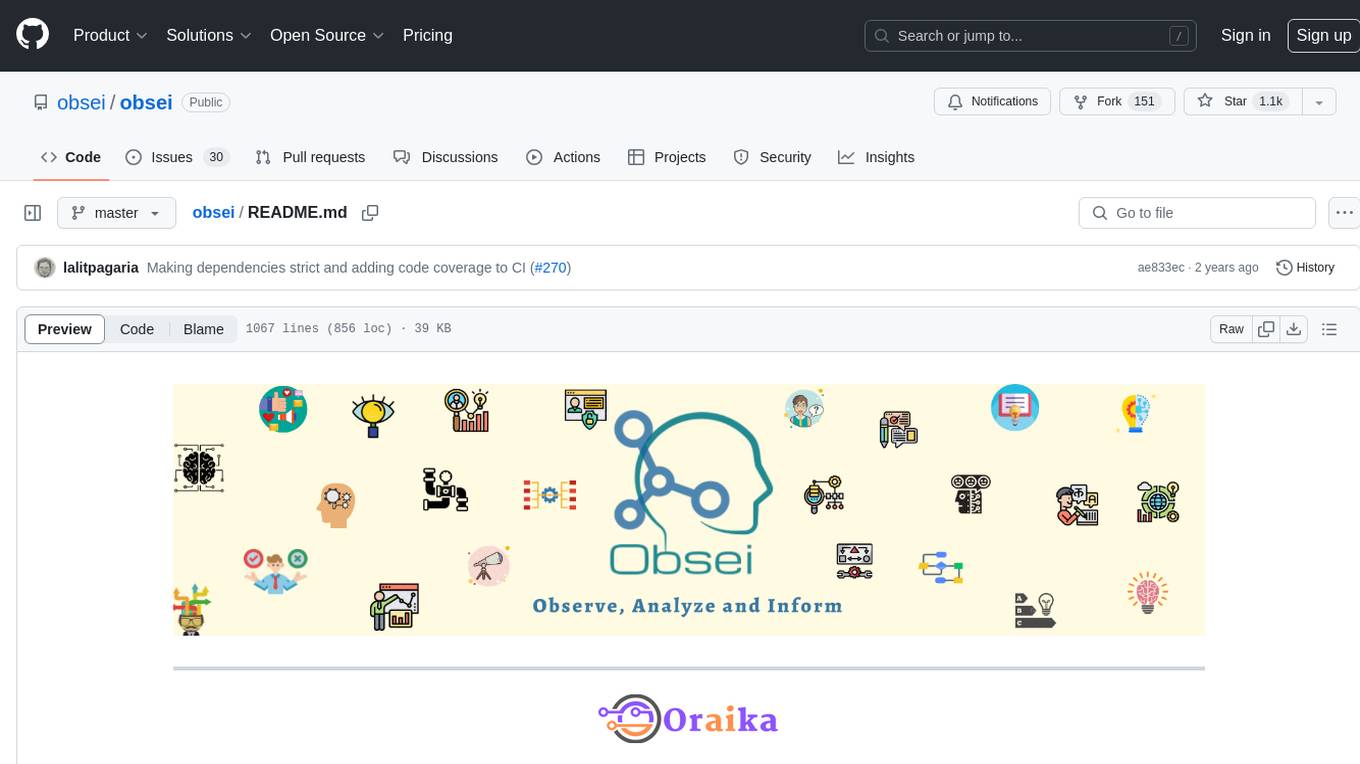
obsei
Obsei is an open-source, low-code, AI powered automation tool that consists of an Observer to collect unstructured data from various sources, an Analyzer to analyze the collected data with various AI tasks, and an Informer to send analyzed data to various destinations. The tool is suitable for scheduled jobs or serverless applications as all Observers can store their state in databases. Obsei is still in alpha stage, so caution is advised when using it in production. The tool can be used for social listening, alerting/notification, automatic customer issue creation, extraction of deeper insights from feedbacks, market research, dataset creation for various AI tasks, and more based on creativity.
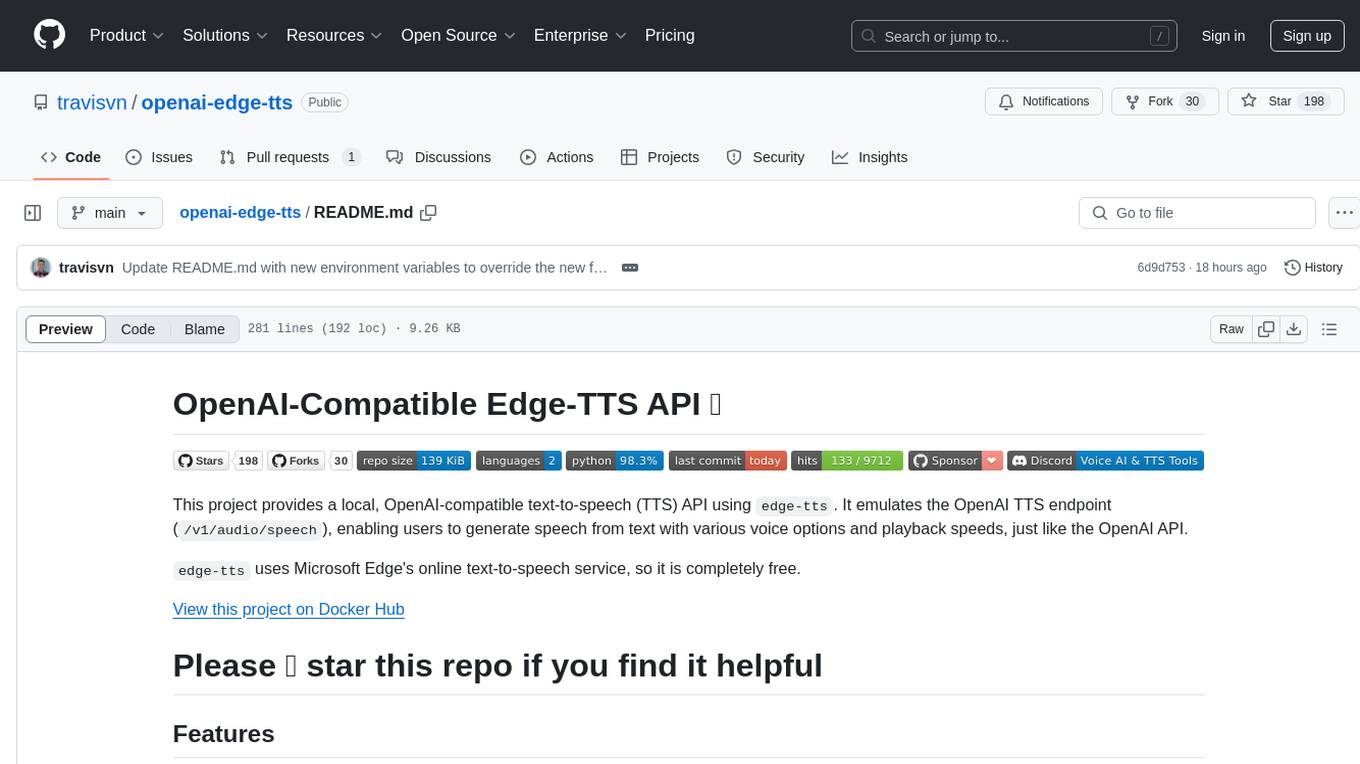
openai-edge-tts
This project provides a local, OpenAI-compatible text-to-speech (TTS) API using `edge-tts`. It emulates the OpenAI TTS endpoint (`/v1/audio/speech`), enabling users to generate speech from text with various voice options and playback speeds, just like the OpenAI API. `edge-tts` uses Microsoft Edge's online text-to-speech service, making it completely free. The project supports multiple audio formats, adjustable playback speed, and voice selection options, providing a flexible and customizable TTS solution for users.
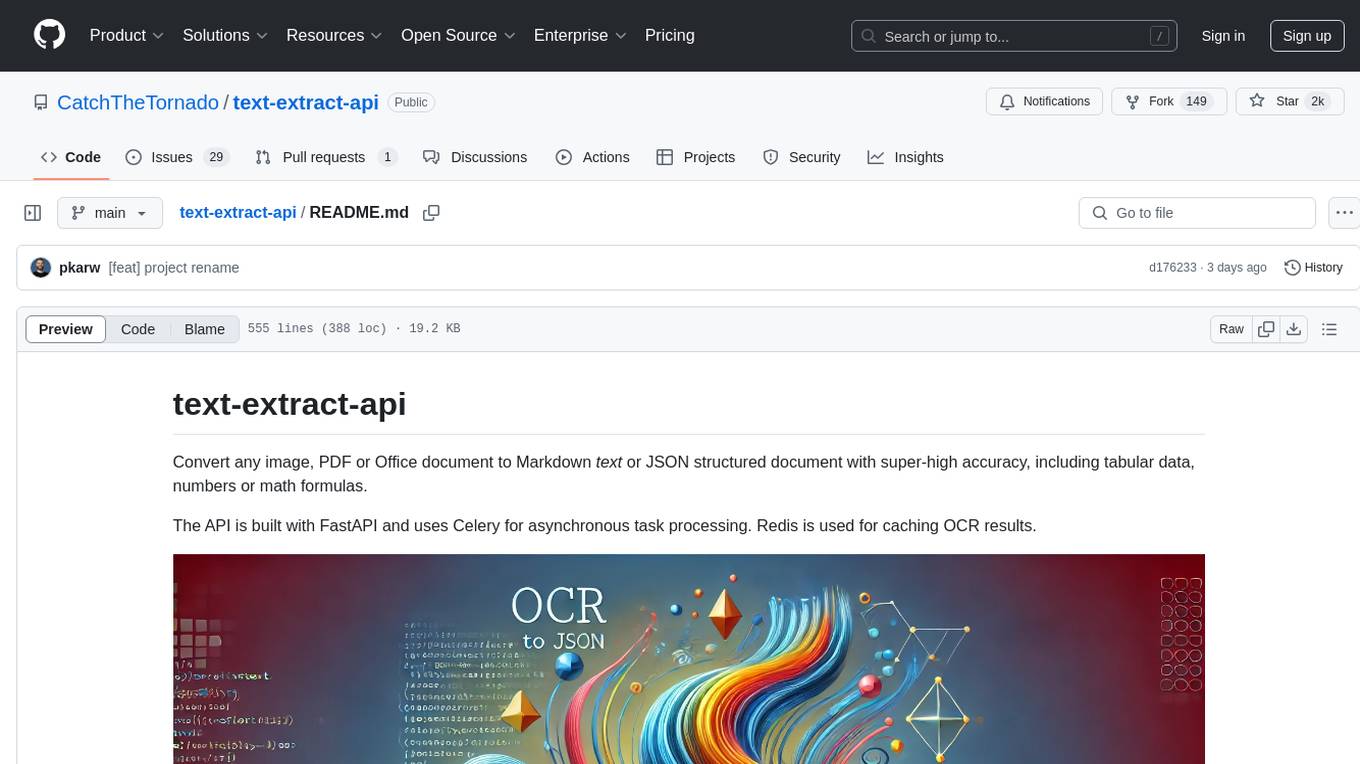
text-extract-api
The text-extract-api is a powerful tool that allows users to convert images, PDFs, or Office documents to Markdown text or JSON structured documents with high accuracy. It is built using FastAPI and utilizes Celery for asynchronous task processing, with Redis for caching OCR results. The tool provides features such as PDF/Office to Markdown and JSON conversion, improving OCR results with LLama, removing Personally Identifiable Information from documents, distributed queue processing, caching using Redis, switchable storage strategies, and a CLI tool for task management. Users can run the tool locally or on cloud services, with support for GPU processing. The tool also offers an online demo for testing purposes.
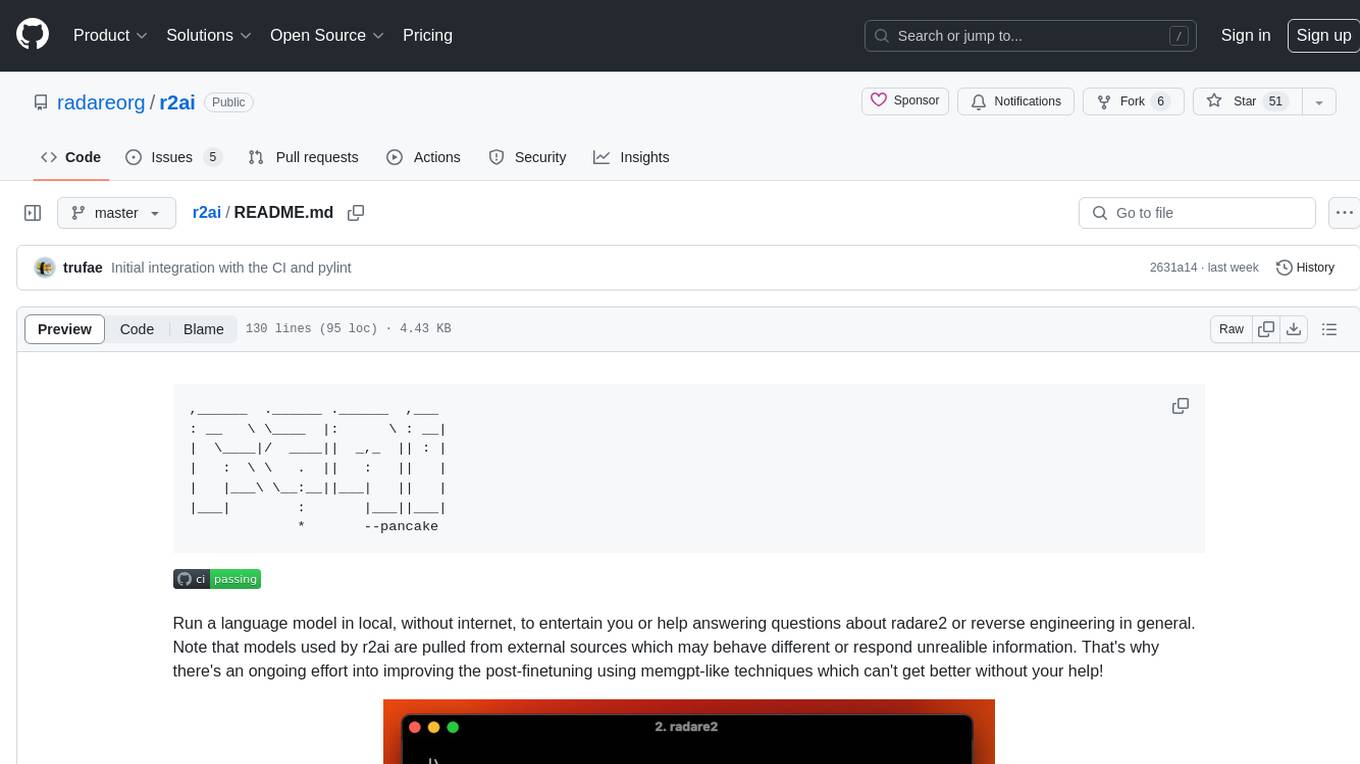
r2ai
r2ai is a tool designed to run a language model locally without internet access. It can be used to entertain users or assist in answering questions related to radare2 or reverse engineering. The tool allows users to prompt the language model, index large codebases, slurp file contents, embed the output of an r2 command, define different system-level assistant roles, set environment variables, and more. It is accessible as an r2lang-python plugin and can be scripted from various languages. Users can use different models, adjust query templates dynamically, load multiple models, and make them communicate with each other.
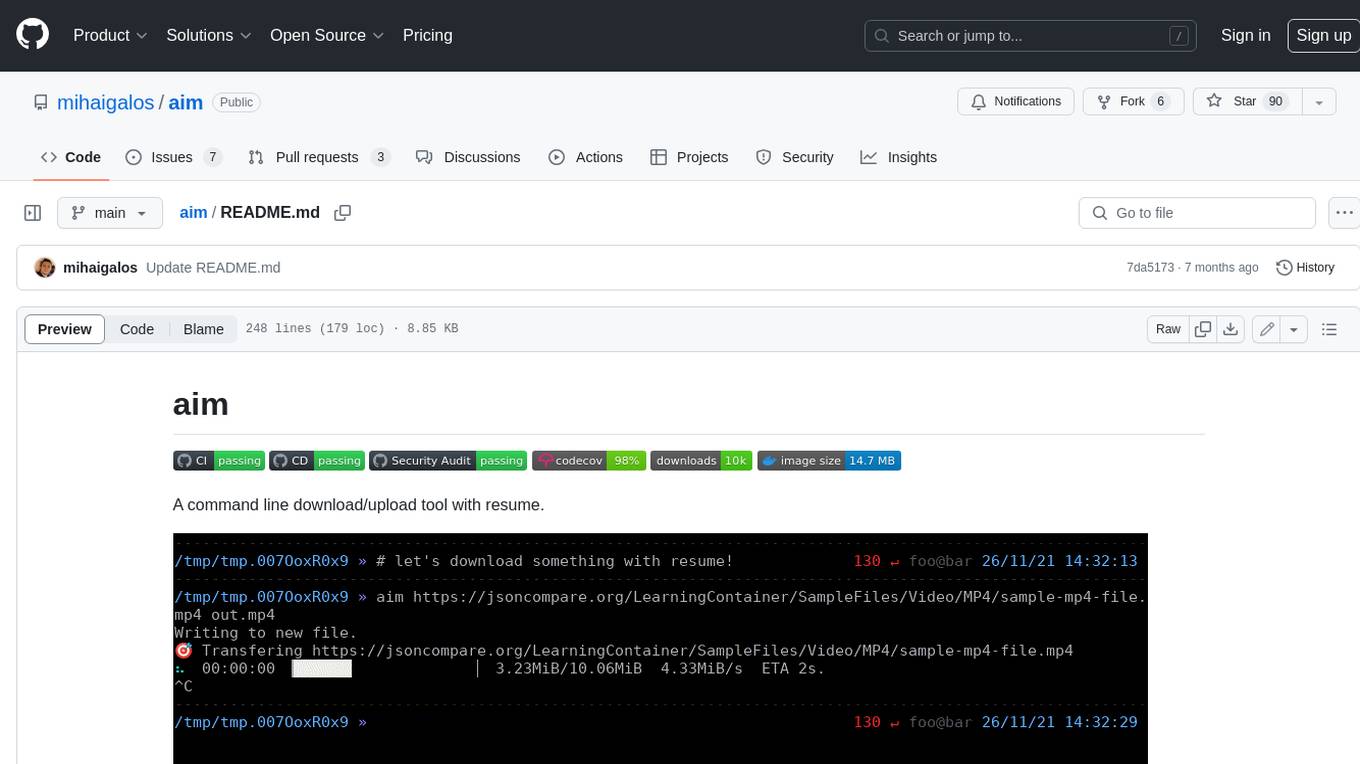
aim
Aim is a command-line tool for downloading and uploading files with resume support. It supports various protocols including HTTP, FTP, SFTP, SSH, and S3. Aim features an interactive mode for easy navigation and selection of files, as well as the ability to share folders over HTTP for easy access from other devices. Additionally, it offers customizable progress indicators and output formats, and can be integrated with other commands through piping. Aim can be installed via pre-built binaries or by compiling from source, and is also available as a Docker image for platform-independent usage.
For similar tasks
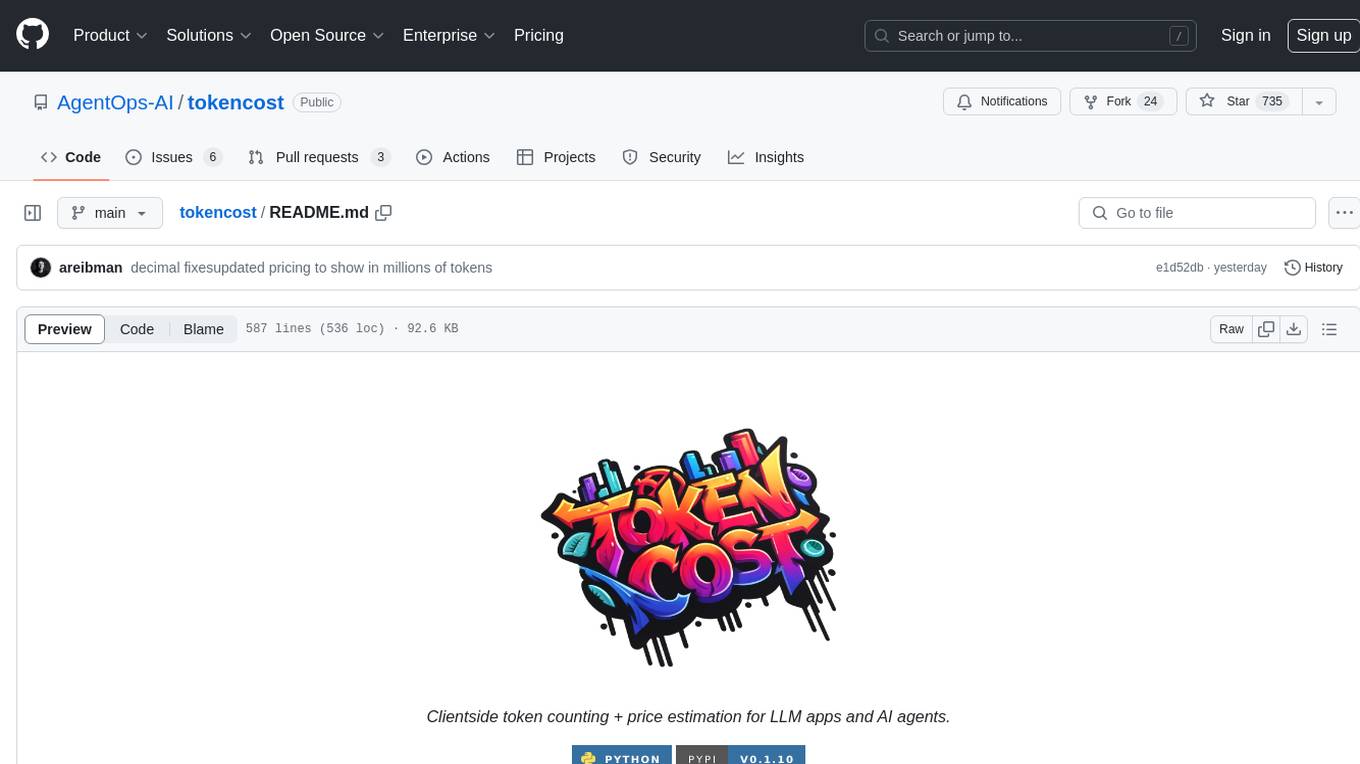
tokencost
Tokencost is a clientside tool for calculating the USD cost of using major Large Language Model (LLMs) APIs by estimating the cost of prompts and completions. It helps track the latest price changes of major LLM providers, accurately count prompt tokens before sending OpenAI requests, and easily integrate to get the cost of a prompt or completion with a single function. Users can calculate prompt and completion costs using OpenAI requests, count tokens in prompts formatted as message lists or string prompts, and refer to a cost table with updated prices for various LLM models. The tool also supports callback handlers for LLM wrapper/framework libraries like LlamaIndex and Langchain.
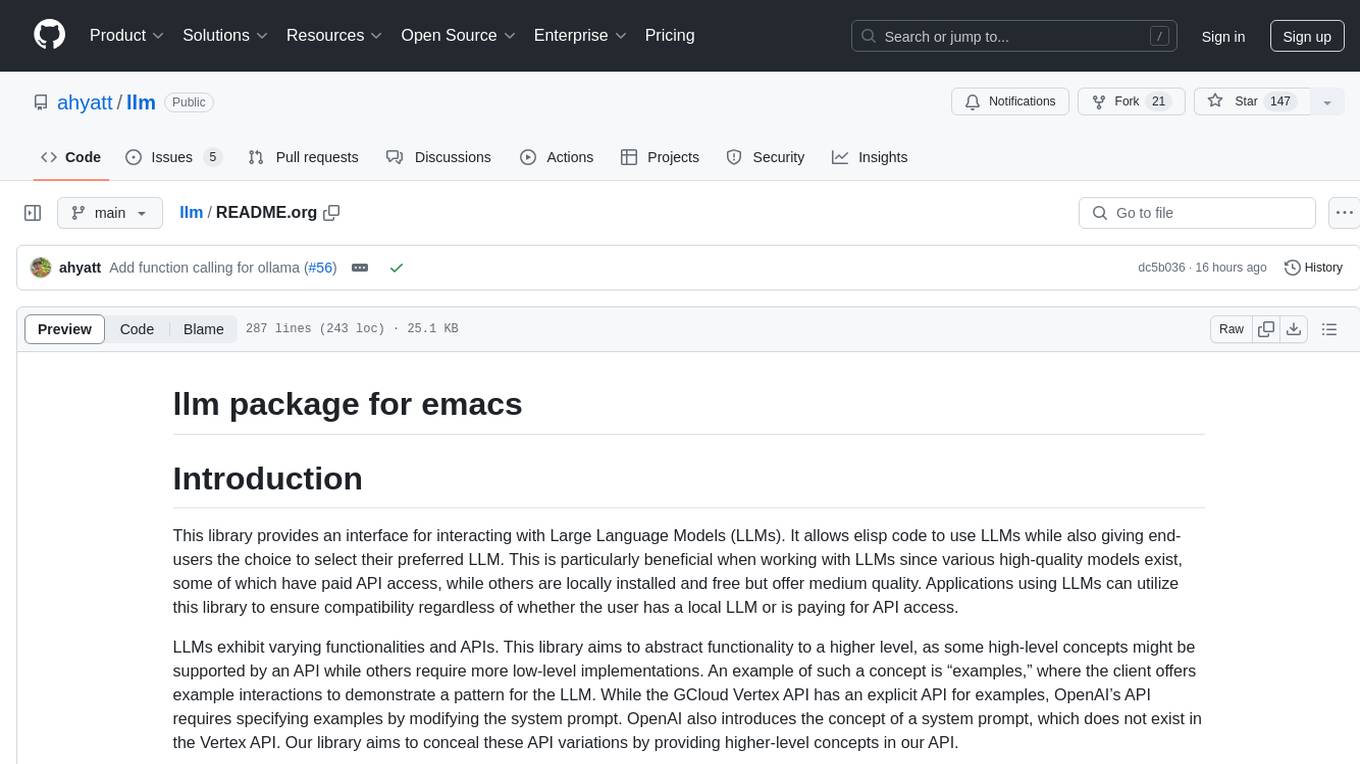
llm
The 'llm' package for Emacs provides an interface for interacting with Large Language Models (LLMs). It abstracts functionality to a higher level, concealing API variations and ensuring compatibility with various LLMs. Users can set up providers like OpenAI, Gemini, Vertex, Claude, Ollama, GPT4All, and a fake client for testing. The package allows for chat interactions, embeddings, token counting, and function calling. It also offers advanced prompt creation and logging capabilities. Users can handle conversations, create prompts with placeholders, and contribute by creating providers.
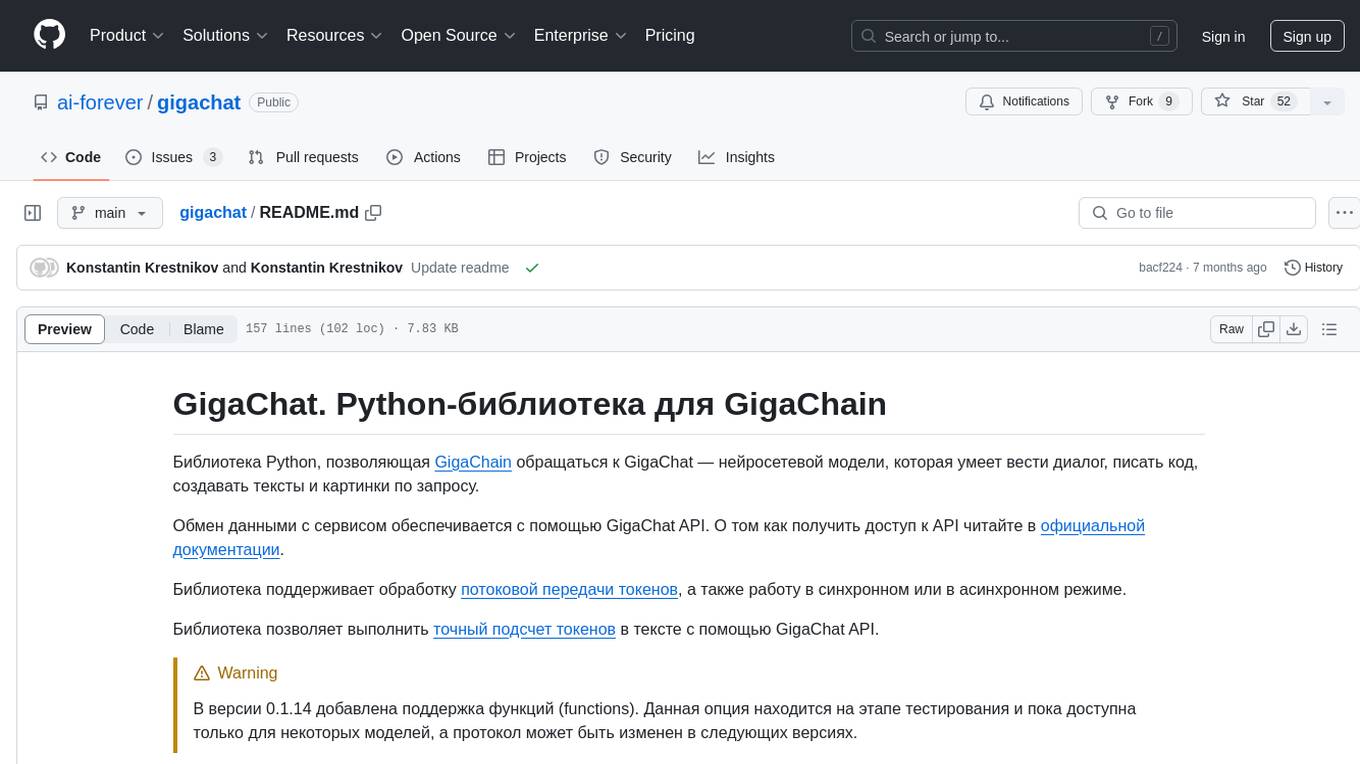
gigachat
GigaChat is a Python library that allows GigaChain to interact with GigaChat, a neural network model capable of engaging in dialogue, writing code, creating texts, and images on demand. Data exchange with the service is facilitated through the GigaChat API. The library supports processing token streaming, as well as working in synchronous or asynchronous mode. It enables precise token counting in text using the GigaChat API.
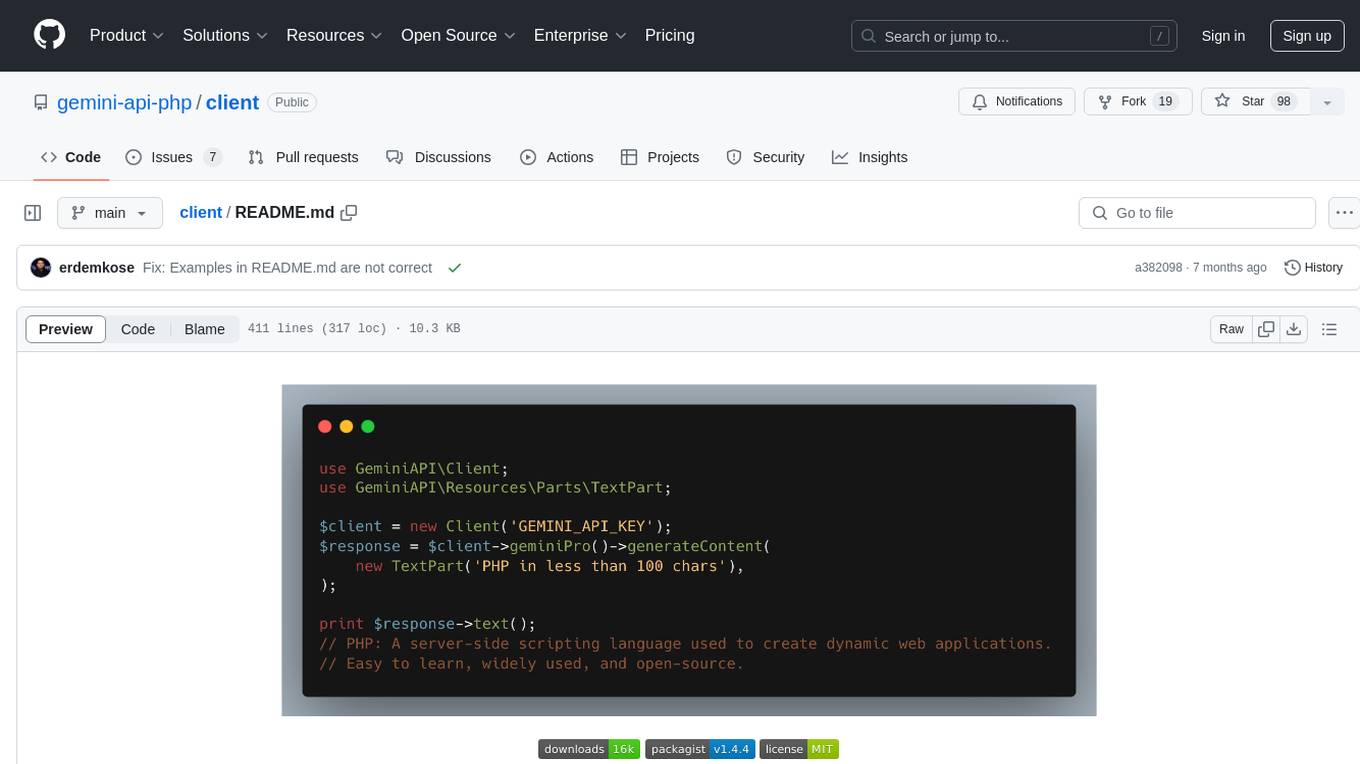
client
Gemini API PHP Client is a library that allows you to interact with Google's generative AI models, such as Gemini Pro and Gemini Pro Vision. It provides functionalities for basic text generation, multimodal input, chat sessions, streaming responses, tokens counting, listing models, and advanced usages like safety settings and custom HTTP client usage. The library requires an API key to access Google's Gemini API and can be installed using Composer. It supports various features like generating content, starting chat sessions, embedding content, counting tokens, and listing available models.
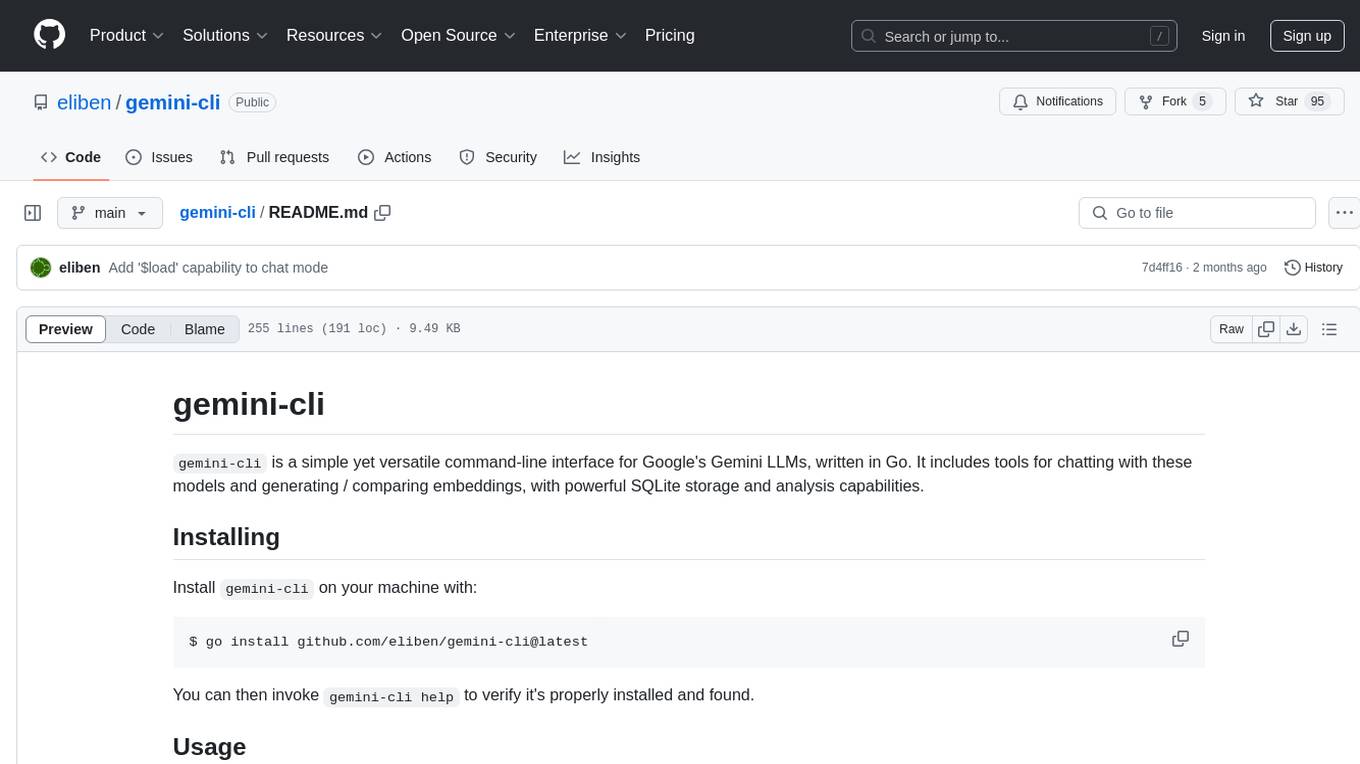
gemini-cli
gemini-cli is a versatile command-line interface for Google's Gemini LLMs, written in Go. It includes tools for chatting with models, generating/comparing embeddings, and storing data in SQLite for analysis. Users can interact with Gemini models through various subcommands like prompt, chat, counttok, embed content, embed db, and embed similar.
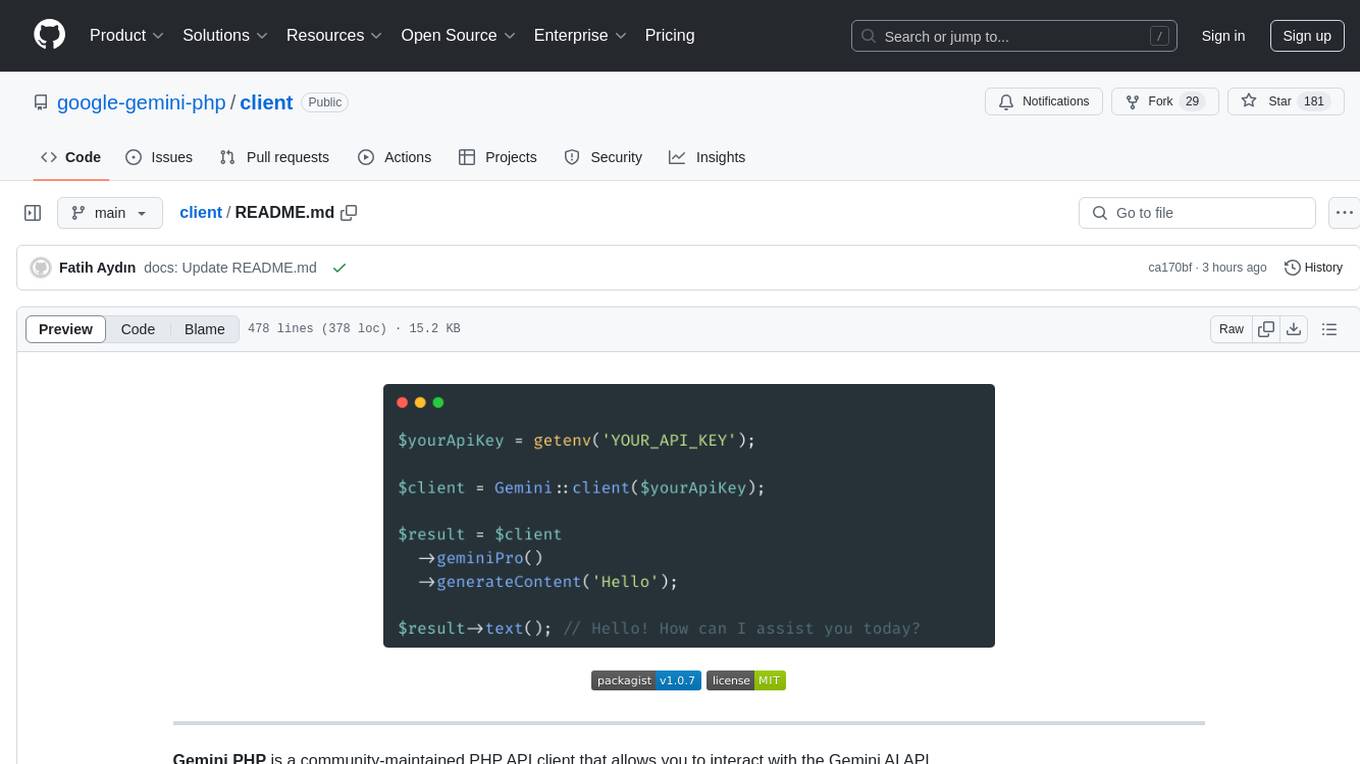
client
Gemini PHP is a PHP API client for interacting with the Gemini AI API. It allows users to generate content, chat, count tokens, configure models, embed resources, list models, get model information, troubleshoot timeouts, and test API responses. The client supports various features such as text-only input, text-and-image input, multi-turn conversations, streaming content generation, token counting, model configuration, and embedding techniques. Users can interact with Gemini's API to perform tasks related to natural language generation and text analysis.
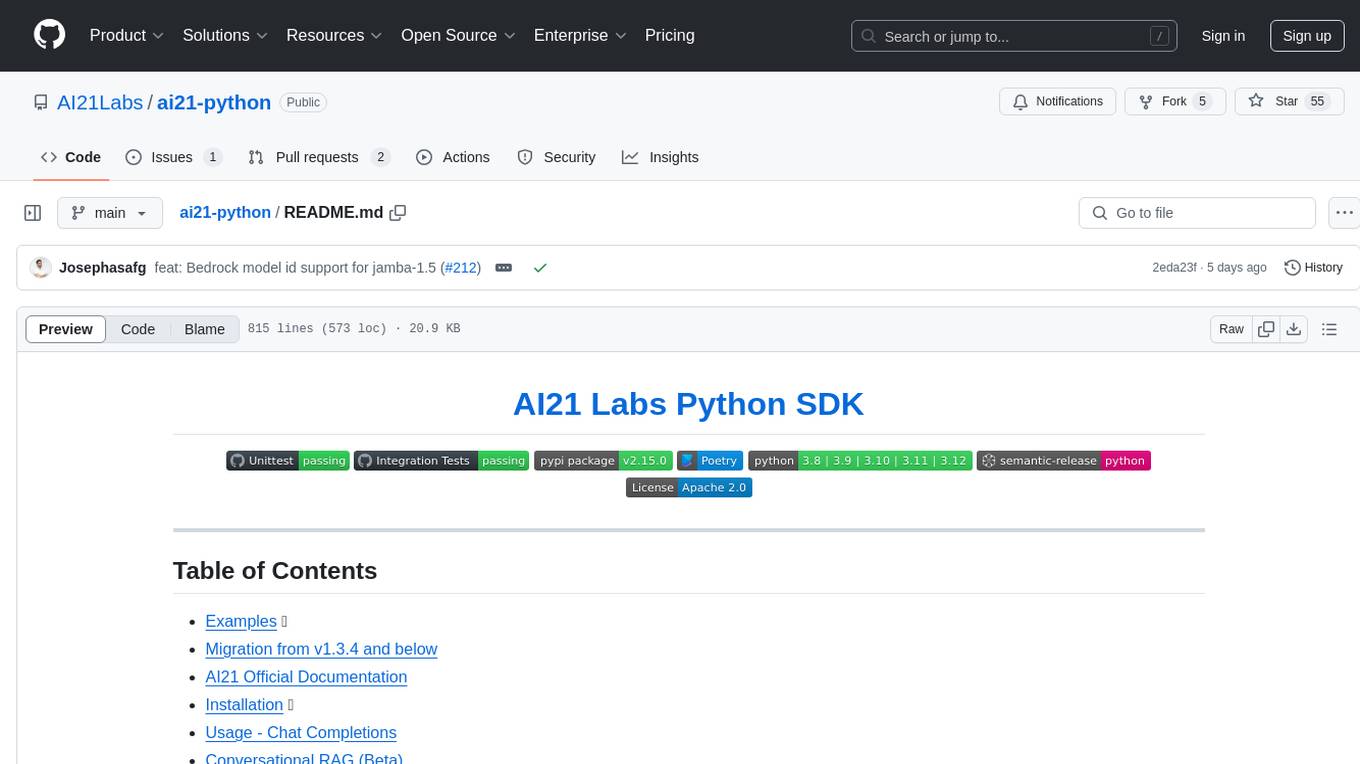
ai21-python
The AI21 Labs Python SDK is a comprehensive tool for interacting with the AI21 API. It provides functionalities for chat completions, conversational RAG, token counting, error handling, and support for various cloud providers like AWS, Azure, and Vertex. The SDK offers both synchronous and asynchronous usage, along with detailed examples and documentation. Users can quickly get started with the SDK to leverage AI21's powerful models for various natural language processing tasks.
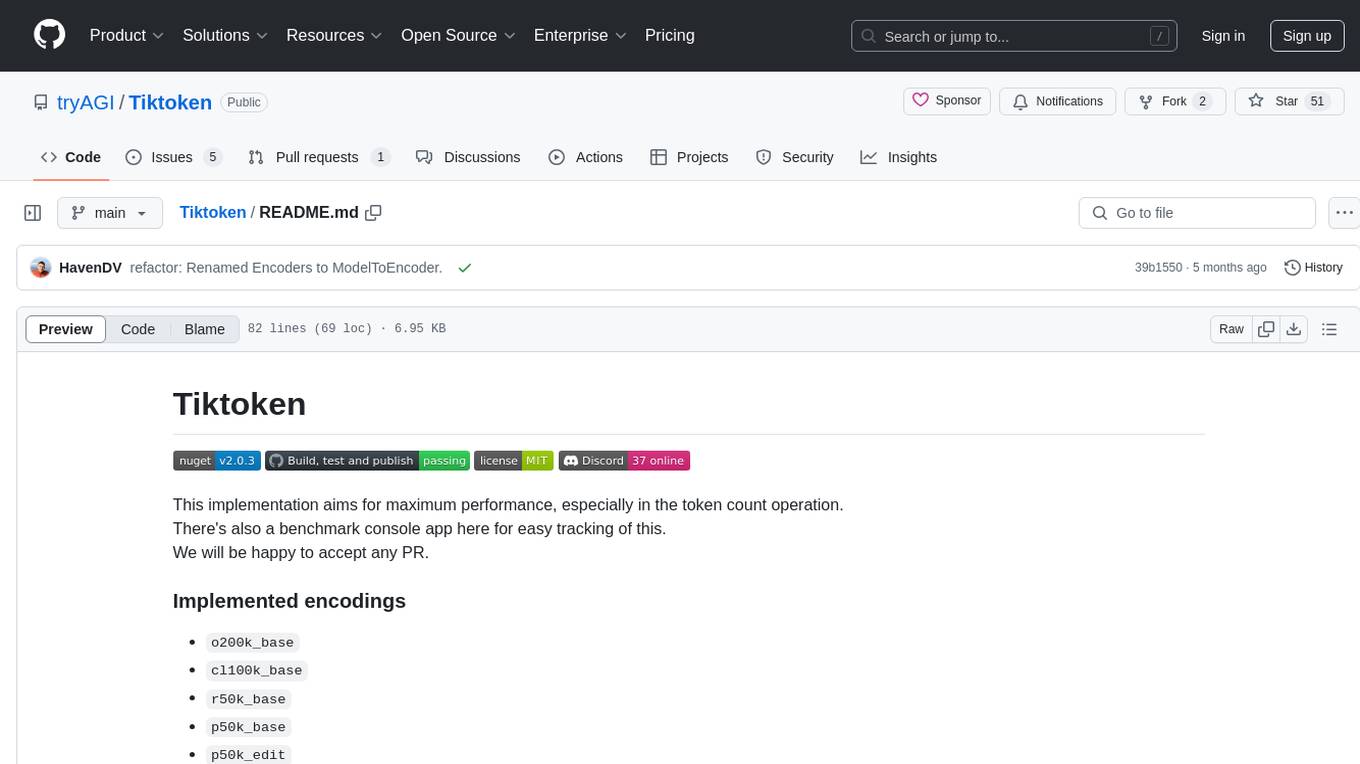
Tiktoken
Tiktoken is a high-performance implementation focused on token count operations. It provides various encodings like o200k_base, cl100k_base, r50k_base, p50k_base, and p50k_edit. Users can easily encode and decode text using the provided API. The repository also includes a benchmark console app for performance tracking. Contributions in the form of PRs are welcome.
For similar jobs
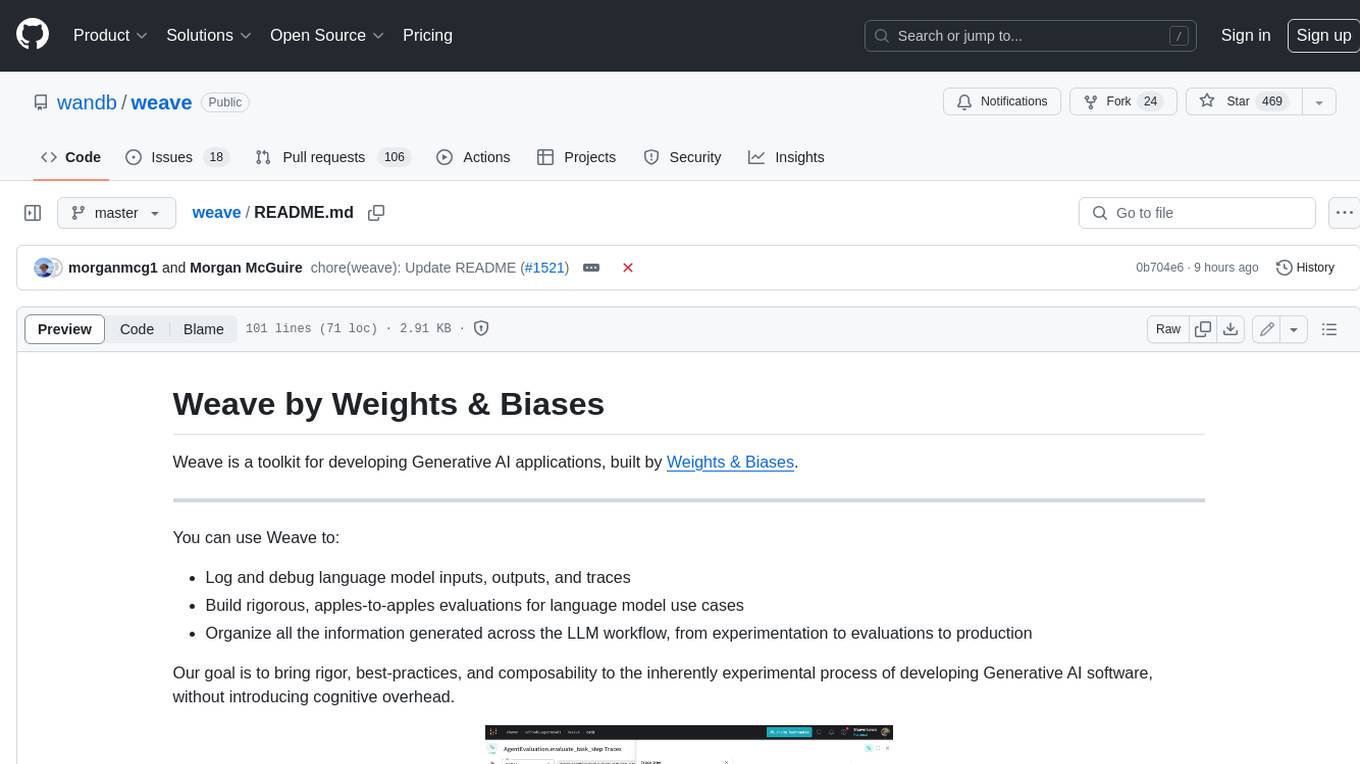
weave
Weave is a toolkit for developing Generative AI applications, built by Weights & Biases. With Weave, you can log and debug language model inputs, outputs, and traces; build rigorous, apples-to-apples evaluations for language model use cases; and organize all the information generated across the LLM workflow, from experimentation to evaluations to production. Weave aims to bring rigor, best-practices, and composability to the inherently experimental process of developing Generative AI software, without introducing cognitive overhead.
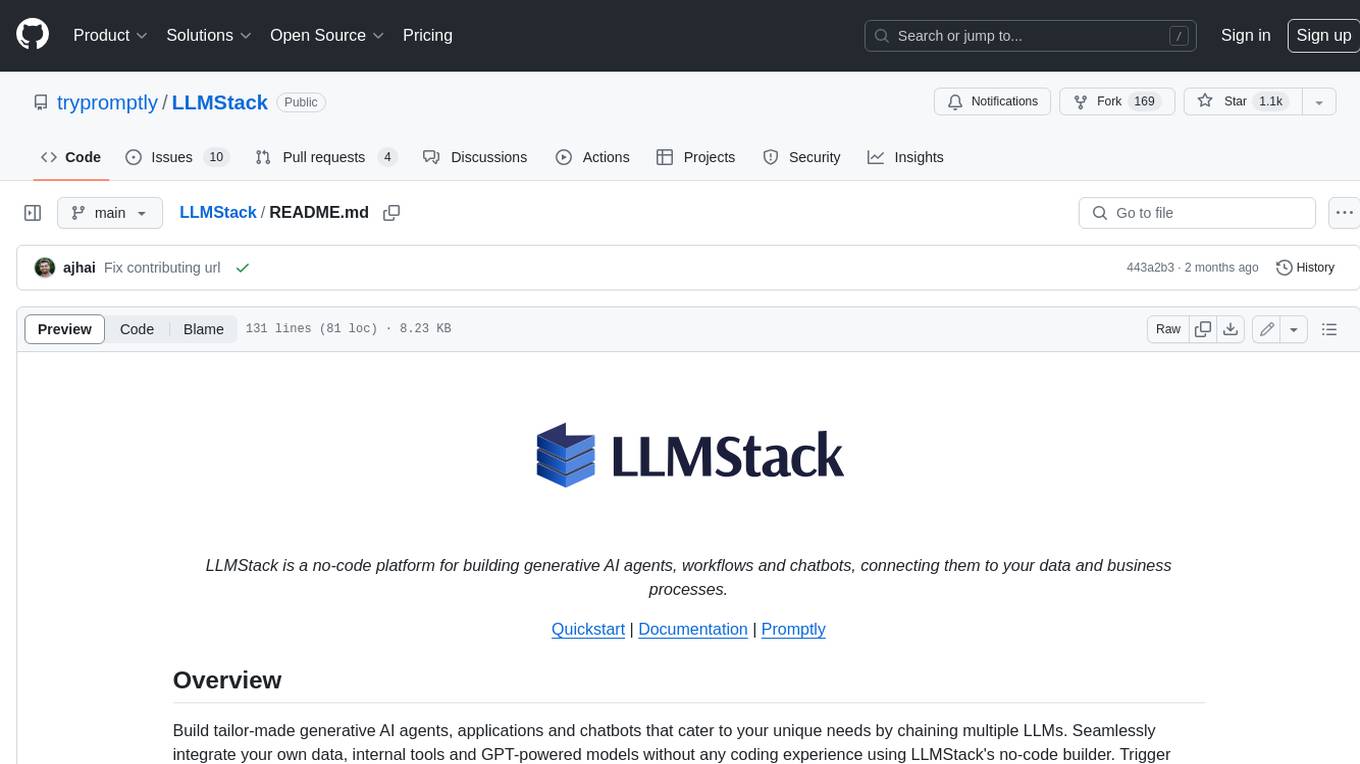
LLMStack
LLMStack is a no-code platform for building generative AI agents, workflows, and chatbots. It allows users to connect their own data, internal tools, and GPT-powered models without any coding experience. LLMStack can be deployed to the cloud or on-premise and can be accessed via HTTP API or triggered from Slack or Discord.
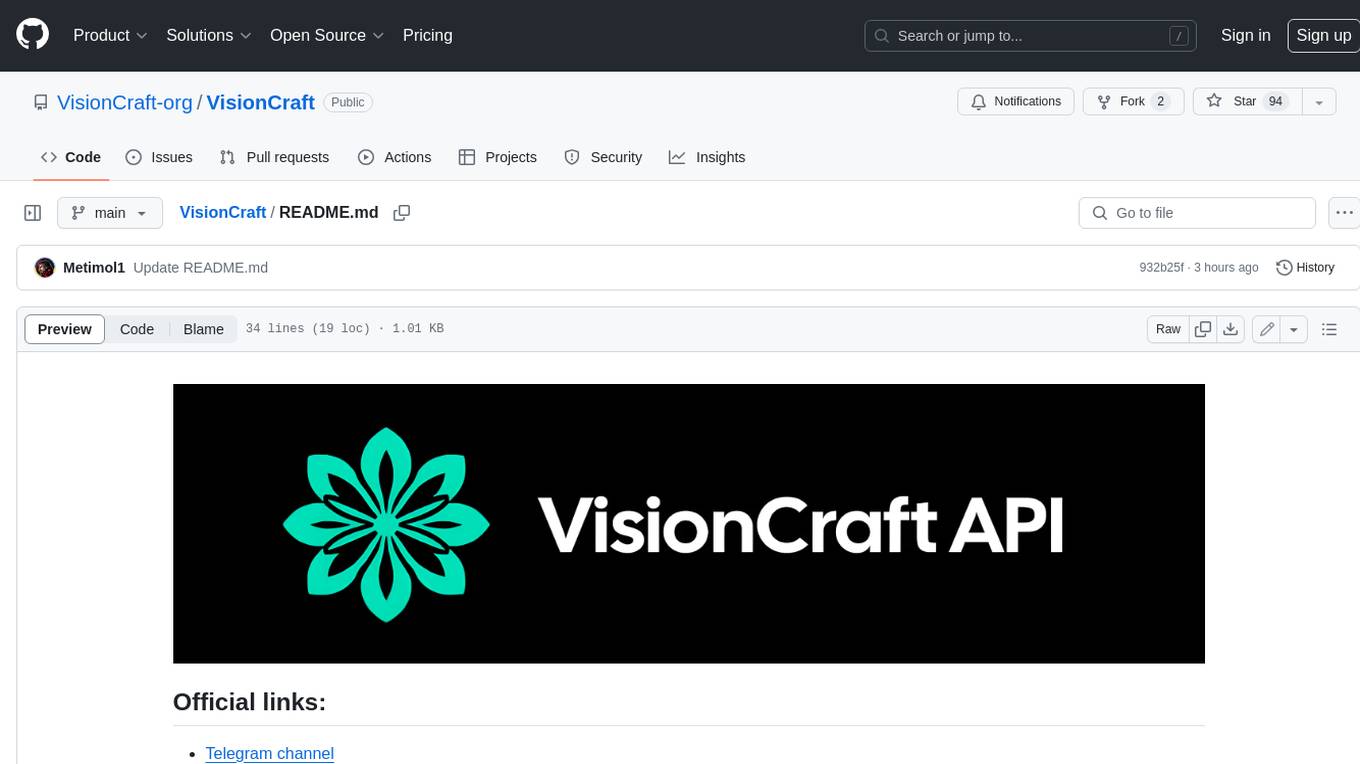
VisionCraft
The VisionCraft API is a free API for using over 100 different AI models. From images to sound.
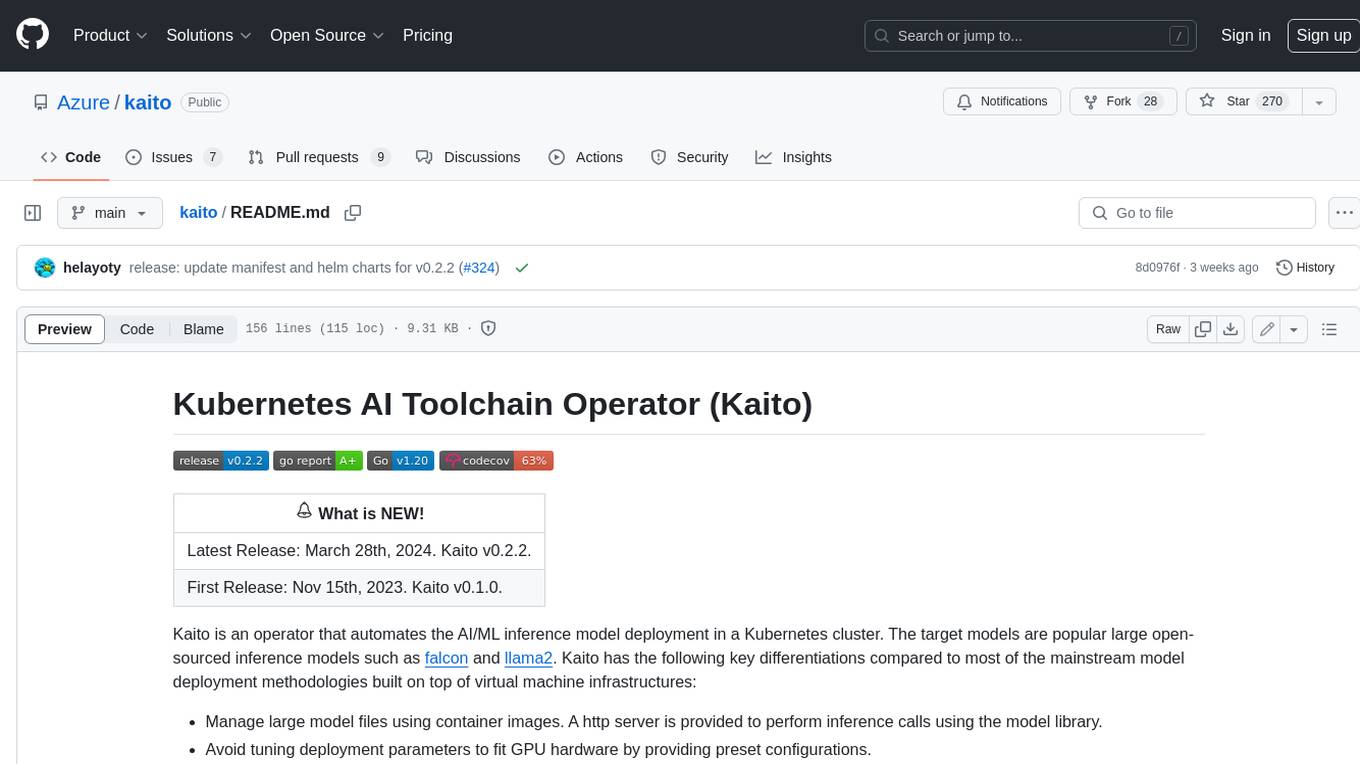
kaito
Kaito is an operator that automates the AI/ML inference model deployment in a Kubernetes cluster. It manages large model files using container images, avoids tuning deployment parameters to fit GPU hardware by providing preset configurations, auto-provisions GPU nodes based on model requirements, and hosts large model images in the public Microsoft Container Registry (MCR) if the license allows. Using Kaito, the workflow of onboarding large AI inference models in Kubernetes is largely simplified.
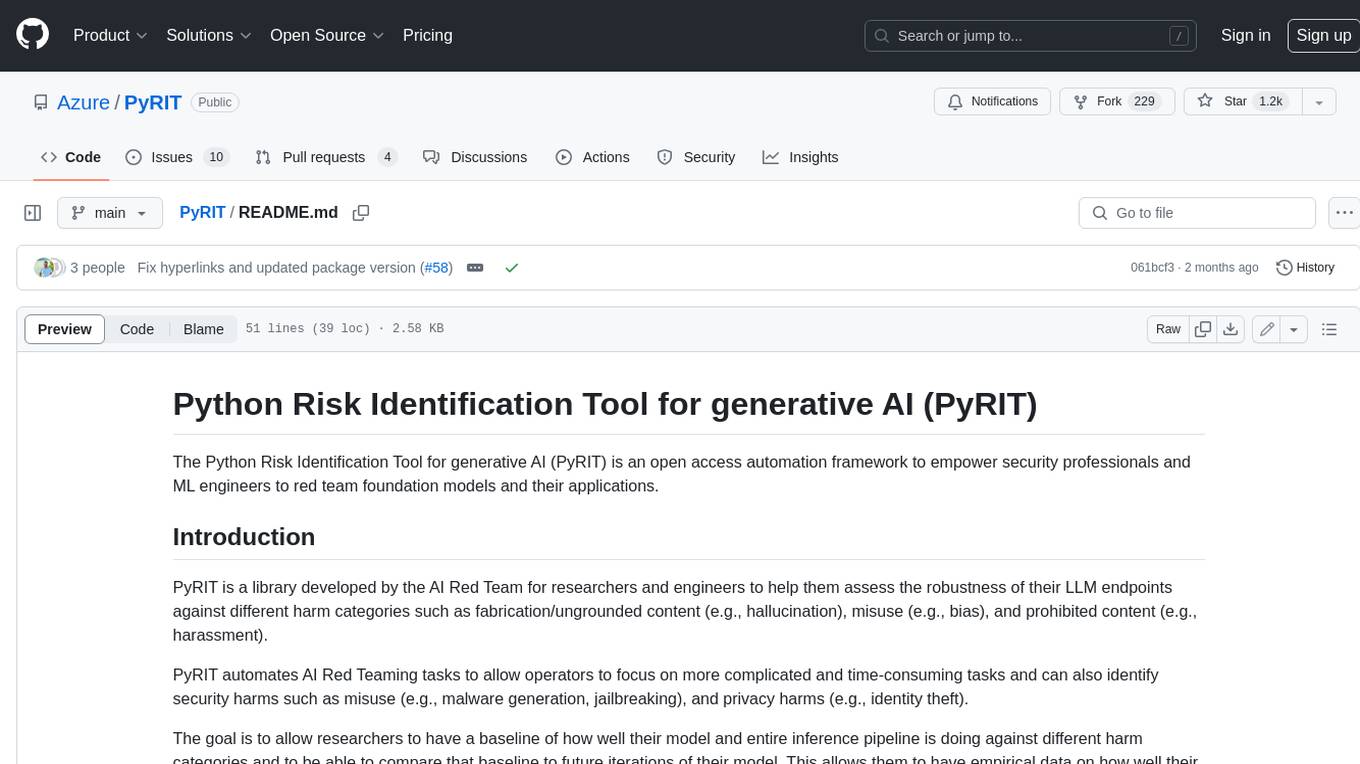
PyRIT
PyRIT is an open access automation framework designed to empower security professionals and ML engineers to red team foundation models and their applications. It automates AI Red Teaming tasks to allow operators to focus on more complicated and time-consuming tasks and can also identify security harms such as misuse (e.g., malware generation, jailbreaking), and privacy harms (e.g., identity theft). The goal is to allow researchers to have a baseline of how well their model and entire inference pipeline is doing against different harm categories and to be able to compare that baseline to future iterations of their model. This allows them to have empirical data on how well their model is doing today, and detect any degradation of performance based on future improvements.
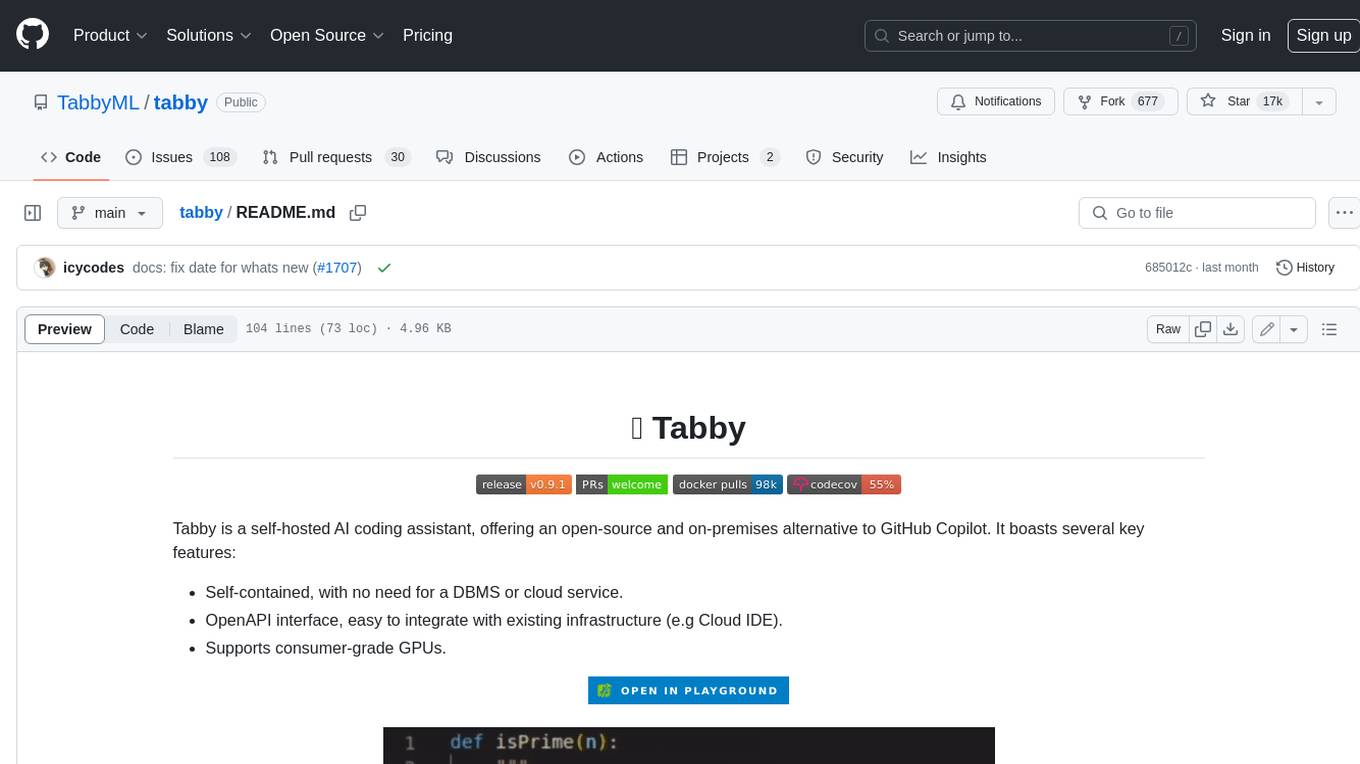
tabby
Tabby is a self-hosted AI coding assistant, offering an open-source and on-premises alternative to GitHub Copilot. It boasts several key features: * Self-contained, with no need for a DBMS or cloud service. * OpenAPI interface, easy to integrate with existing infrastructure (e.g Cloud IDE). * Supports consumer-grade GPUs.
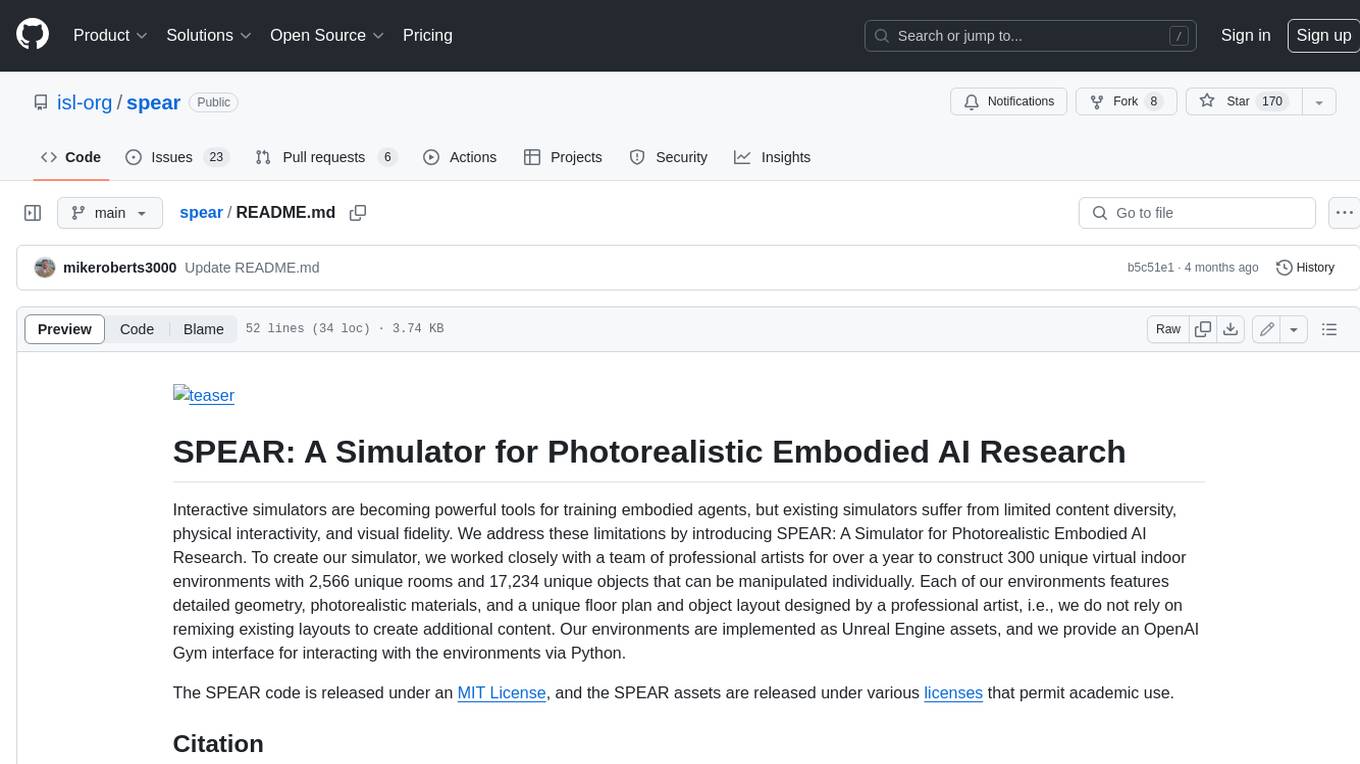
spear
SPEAR (Simulator for Photorealistic Embodied AI Research) is a powerful tool for training embodied agents. It features 300 unique virtual indoor environments with 2,566 unique rooms and 17,234 unique objects that can be manipulated individually. Each environment is designed by a professional artist and features detailed geometry, photorealistic materials, and a unique floor plan and object layout. SPEAR is implemented as Unreal Engine assets and provides an OpenAI Gym interface for interacting with the environments via Python.
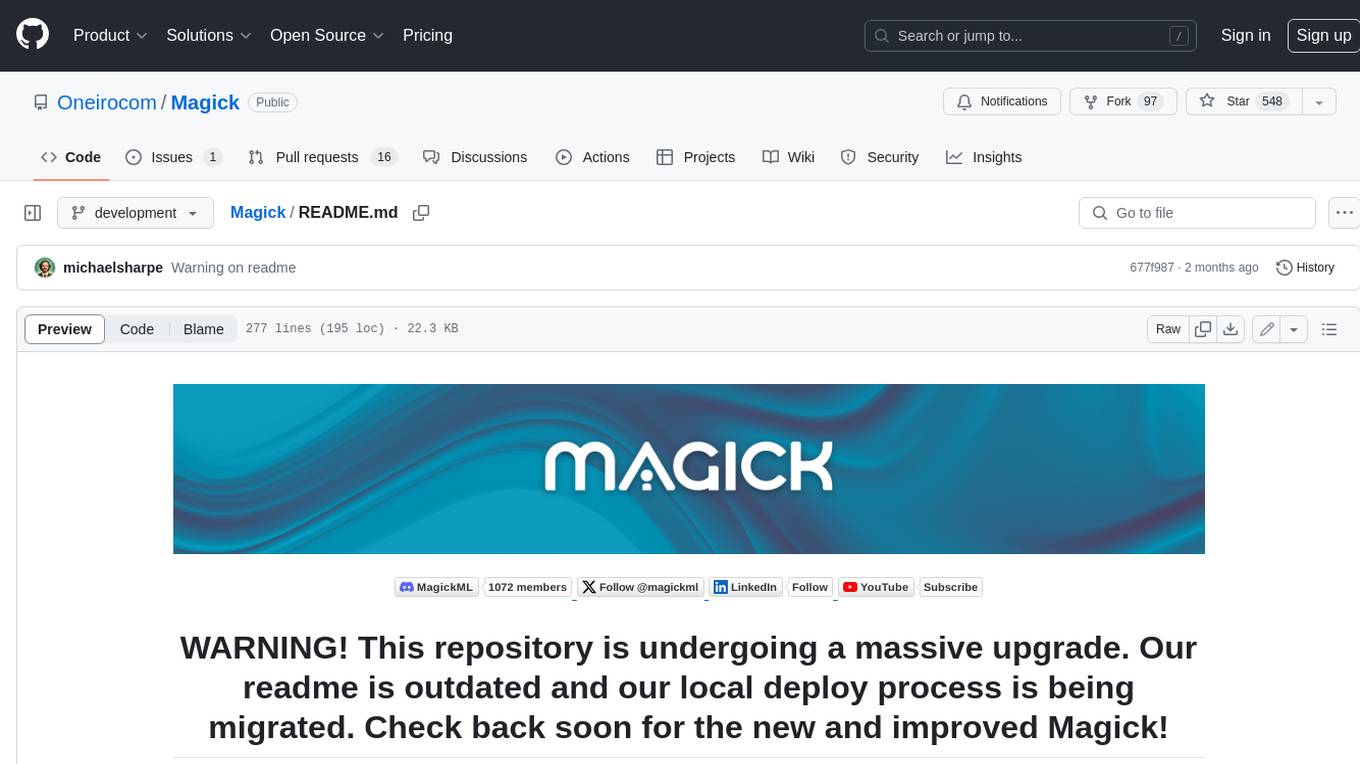
Magick
Magick is a groundbreaking visual AIDE (Artificial Intelligence Development Environment) for no-code data pipelines and multimodal agents. Magick can connect to other services and comes with nodes and templates well-suited for intelligent agents, chatbots, complex reasoning systems and realistic characters.