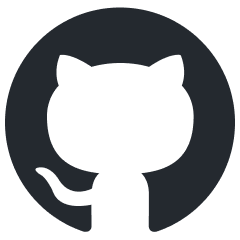
HuixiangDou
HuixiangDou: Overcoming Group Chat Scenarios with LLM-based Technical Assistance
Stars: 2256
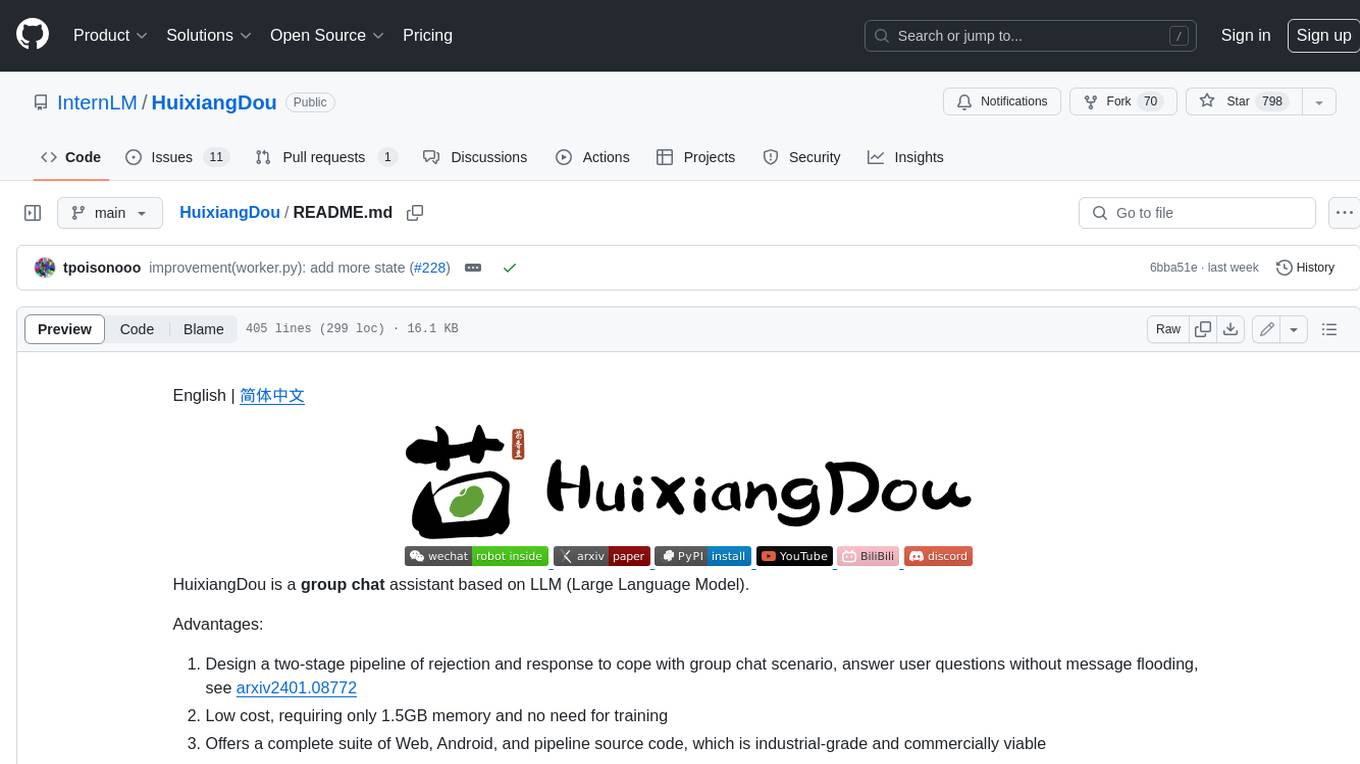
HuixiangDou is a **group chat** assistant based on LLM (Large Language Model). Advantages: 1. Design a two-stage pipeline of rejection and response to cope with group chat scenario, answer user questions without message flooding, see arxiv2401.08772 2. Low cost, requiring only 1.5GB memory and no need for training 3. Offers a complete suite of Web, Android, and pipeline source code, which is industrial-grade and commercially viable Check out the scenes in which HuixiangDou are running and join WeChat Group to try AI assistant inside. If this helps you, please give it a star โญ
README:
HuixiangDou2 is a validated GraphRAG solution in the plant field. If you are interested in the effects of HuixiangDou in non-computer fields, try the new version.
English | ็ฎไฝไธญๆ
HuixiangDou1 is a professional knowledge assistant based on LLM.
Advantages:
- Design three-stage pipelines of preprocess, rejection and response
-
chat_in_group
copes with group chat scenario, answer user questions without message flooding, see 2401.08772, 2405.02817, Hybrid Retrieval and Precision Report -
chat_with_repo
for real-time streaming chat
-
- No training required, with CPU-only, 2G, 10G, 20G and 80G configuration
- Offers a complete suite of Web, Android, and pipeline source code, industrial-grade and commercially viable
Check out the scenes in which HuixiangDou are running and current public service status:
- readthedocs ChatWithAI (cpu-only) is available
- OpenXLab is using GPU and under continuous maintenance
- WeChat bot has a cost associated with WeChat integration. All code has been verified to be functional for one year. Please deploy it on your own for either the free or commercial version.
If this helps you, please give it a star โญ
Our Web version has been released to OpenXLab, where you can create knowledge base, update positive and negative examples, turn on web search, test chat, and integrate into Feishu/WeChat groups. See BiliBili and YouTube !
The Web version's API for Android also supports other devices. See Python sample code.
- [2025/03] Forwarding multiple wechat group message
- [2024/09] Inverted indexer makes LLM prefer knowledge base๐ฏ
- [2024/09] Code retrieval
- [2024/08] chat_with_readthedocs, see how to integrate ๐
- [2024/07] Image and text retrieval & Removal of
langchain
๐ - [2024/07] Hybrid Knowledge Graph and Dense Retrieval improve 1.7% F1 score ๐ฏ
- [2024/06] Evaluation of chunksize, splitter, and text2vec model ๐ฏ
- [2024/05] wkteam WeChat access, parsing image & URL, support coreference resolution
- [2024/05] SFT LLM on NLP task, F1 increased by 29% ๐ฏ
๐ค LoRA-Qwen1.5-14B LoRA-Qwen1.5-32B alpaca data arXiv - [2024/04] RAG Annotation SFT Q&A Data and Examples
- [2024/04] Release Web Front and Back End Service Source Code ๐
- [2024/03] New Personal WeChat Integration and Prebuilt APK !
- [2024/02] [Experimental Feature] WeChat Group Integration of multimodal to achieve OCR
LLM | File Format | Retrieval Method | Integration | Preprocessing |
|
|
|
The following are the GPU memory requirements for different features, the difference lies only in whether the options are turned on.
Configuration Example | GPU mem Requirements | Description | Verified on Linux |
---|---|---|---|
config-cpu.ini | - | Use siliconcloud API for text only |
|
config-2G.ini | 2GB | Use openai API (such as kimi, deepseek and stepfun to search for text only | |
config-multimodal.ini | 10GB | Use openai API for LLM, image and text retrieval | |
[Standard Edition] config.ini | 19GB | Local deployment of LLM, single modality | |
config-advanced.ini | 80GB | local LLM, anaphora resolution, single modality, practical for WeChat group |
We take the standard edition (local running LLM, text retrieval) as an introduction example. Other versions are just different in configuration options.
Click to agree to the BCE model agreement, log in huggingface
huggingface-cli login
Install dependencies
# parsing `word` format requirements
apt update
apt install python-dev libxml2-dev libxslt1-dev antiword unrtf poppler-utils pstotext tesseract-ocr flac ffmpeg lame libmad0 libsox-fmt-mp3 sox libjpeg-dev swig libpulse-dev
# python requirements
pip install -r requirements.txt
# For python3.8, install faiss-gpu instead of faiss
Use mmpose documents to build the mmpose knowledge base and filtering questions. If you have your own documents, just put them under repodir
.
Copy and execute all the following commands (including the '#' symbol).
# Download the knowledge base, we only take the documents of mmpose as an example. You can put any of your own documents under `repodir`
cd HuixiangDou
mkdir repodir
git clone https://github.com/open-mmlab/mmpose --depth=1 repodir/mmpose
# Save the features of repodir to workdir, and update the positive and negative example thresholds into `config.ini`
mkdir workdir
python3 -m huixiangdou.service.feature_store
After running, test with python3 -m huixiangdou.main --standalone
. At this time, reply to mmpose related questions (related to the knowledge base), while not responding to weather questions.
python3 -m huixiangdou.main --standalone
+---------------------------+---------+----------------------------+-----------------+
| Query | State | Reply | References |
+===========================+=========+============================+=================+
| How to install mmpose? | success | To install mmpose, plea.. | installation.md |
--------------------------------------------------------------------------------------
| How is the weather today? | unrelated.. | .. | |
+-----------------------+---------+--------------------------------+-----------------+
๐ Input your question here, type `bye` for exit:
..
[!NOTE]
If restarting LLM every time is too slow, first python3 -m huixiangdou.service.llm_server_hybrid; then open a new window, and each time only execute python3 -m huixiangdou.main without restarting LLM.
๐ก Also run a simple Web UI with gradio
:
python3 -m huixiangdou.gradio_ui
Or run a server to listen 23333, default pipeline is chat_with_repo
:
python3 -m huixiangdou.server
# test async API
curl -X POST http://127.0.0.1:23333/huixiangdou_stream -H "Content-Type: application/json" -d '{"text": "how to install mmpose","image": ""}'
# cURL sync API
curl -X POST http://127.0.0.1:23333/huixiangdou_inference -H "Content-Type: application/json" -d '{"text": "how to install mmpose","image": ""}'
Please update the repodir
documents, good_questions and bad_questions, and try your own domain knowledge (medical, financial, power, etc.).
- One-way sending to Feishu group
- Two-way Feishu group receiving and sending, recalling
- Personal WeChat Android access
- Personal WeChat wkteam access
We provide typescript
front-end and python
back-end source code:
- Multi-tenant management supported
- Zero programming access to Feishu and WeChat
- k8s friendly
Same as OpenXlab APP, please read the web deployment document.
If there is no GPU available, model inference can be completed using the siliconcloud API.
Taking docker miniconda+Python3.11 as an example, install CPU dependencies and run:
# Start container
docker run -v /path/to/huixiangdou:/huixiangdou -p 7860:7860 -p 23333:23333 -it continuumio/miniconda3 /bin/bash
# Install dependencies
apt update
apt install python-dev libxml2-dev libxslt1-dev antiword unrtf poppler-utils pstotext tesseract-ocr flac ffmpeg lame libmad0 libsox-fmt-mp3 sox libjpeg-dev swig libpulse-dev
python3 -m pip install -r requirements-cpu.txt
# Establish knowledge base
python3 -m huixiangdou.service.feature_store --config_path config-cpu.ini
# Q&A test
python3 -m huixiangdou.main --standalone --config_path config-cpu.ini
# gradio UI
python3 -m huixiangdou.gradio_ui --config_path config-cpu.ini
If you find the installation too slow, a pre-installed image is provided in Docker Hub. Simply replace it when starting the docker.
If your GPU mem exceeds 1.8G, or you pursue cost-effectiveness. This configuration discards the local LLM and uses remote LLM instead, which is the same as the standard edition.
Take siliconcloud
as an example, fill in the API TOKEN applied from the official website into config-2G.ini
# config-2G.ini
[llm]
enable_local = 0 # Turn off local LLM
enable_remote = 1 # Only use remote
..
remote_type = "siliconcloud" # Choose siliconcloud
remote_api_key = "YOUR-API-KEY-HERE" # Your API key
remote_llm_model = "alibaba/Qwen1.5-110B-Chat"
[!NOTE]
Each Q&A scenario requires calling the LLM 7 times at worst, subject to the free user RPM limit, you can modify the rpm parameter in config.ini
Execute the following to get the Q&A results
python3 -m huixiangdou.main --standalone --config-path config-2G.ini # Start all services at once
If you have 10G GPU mem, you can further support image and text retrieval. Just modify the model used in config.ini.
# config-multimodal.ini
# !!! Download `https://huggingface.co/BAAI/bge-visualized/blob/main/Visualized_m3.pth` to `bge-m3` folder !!!
embedding_model_path = "BAAI/bge-m3"
reranker_model_path = "BAAI/bge-reranker-v2-minicpm-layerwise"
Note:
- You need to manually download Visualized_m3.pth to the bge-m3 directory
- Install FlagEmbedding on main branch, we have made bugfix. Here you can download
bpe_simple_vocab_16e6.txt.gz
- Install requirements/multimodal.txt
Run gradio to test, see the image and text retrieval result here.
python3 tests/test_query_gradio.py
The "HuiXiangDou" in the WeChat experience group has enabled all features:
- Serper search and SourceGraph search enhancement
- Group chat images, WeChat public account parsing
- Text coreference resolution
- Hybrid LLM
- Knowledge base is related to openmmlab's 12 repositories (1700 documents), refusing small talk
Please read the following topics:
- Hybrid knowledge graph and dense retrieval
- Refer to config-advanced.ini configuration to improve effects
- Group chat scenario anaphora resolution training
- Use wkteam WeChat access, integrate images, public account parsing, and anaphora resolution
- Use rag.py to annotate SFT training data
Contributors have provided Android tools to interact with WeChat. The solution is based on system-level APIs, and in principle, it can control any UI (not limited to communication software).
-
What if the robot is too cold/too chatty?
- Fill in the questions that should be answered in the real scenario into
resource/good_questions.json
, and fill the ones that should be rejected intoresource/bad_questions.json
. - Adjust the theme content in
repodir
to ensure that the markdown documents in the main library do not contain irrelevant content.
Re-run
feature_store
to update thresholds and feature libraries.โ ๏ธ You can directly modifyreject_throttle
in config.ini. Generally speaking, 0.5 is a high value; 0.2 is too low. - Fill in the questions that should be answered in the real scenario into
-
Launch is normal, but out of memory during runtime?
LLM long text based on transformers structure requires more memory. At this time, kv cache quantization needs to be done on the model, such as lmdeploy quantization description. Then use docker to independently deploy Hybrid LLM Service.
-
How to access other local LLM / After access, the effect is not ideal?
- Open hybrid llm service, add a new LLM inference implementation.
- Refer to test_intention_prompt and test data, adjust prompt and threshold for the new model, and update them into prompt.py.
-
What if the response is too slow/request always fails?
- Refer to hybrid llm service to add exponential backoff and retransmission.
- Replace local LLM with an inference framework such as lmdeploy, instead of the native huggingface/transformers.
-
What if the GPU memory is too low?
At this time, it is impossible to run local LLM, and only remote LLM can be used in conjunction with text2vec to execute the pipeline. Please make sure that
config.ini
only uses remote LLM and turn off local LLM.
- KIMI: Long text LLM, supports direct file upload
- FlagEmbedding: BAAI RAG group
- BCEmbedding: Chinese-English bilingual feature model
- Langchain-ChatChat: Application of Langchain and ChatGLM
- GrabRedEnvelope: WeChat red packet grab
@misc{kong2024huixiangdou,
title={HuiXiangDou: Overcoming Group Chat Scenarios with LLM-based Technical Assistance},
author={Huanjun Kong and Songyang Zhang and Jiaying Li and Min Xiao and Jun Xu and Kai Chen},
year={2024},
eprint={2401.08772},
archivePrefix={arXiv},
primaryClass={cs.CL}
}
@misc{kong2024labelingsupervisedfinetuningdata,
title={Labeling supervised fine-tuning data with the scaling law},
author={Huanjun Kong},
year={2024},
eprint={2405.02817},
archivePrefix={arXiv},
primaryClass={cs.CL},
url={https://arxiv.org/abs/2405.02817},
}
For Tasks:
Click tags to check more tools for each tasksFor Jobs:
Alternative AI tools for HuixiangDou
Similar Open Source Tools
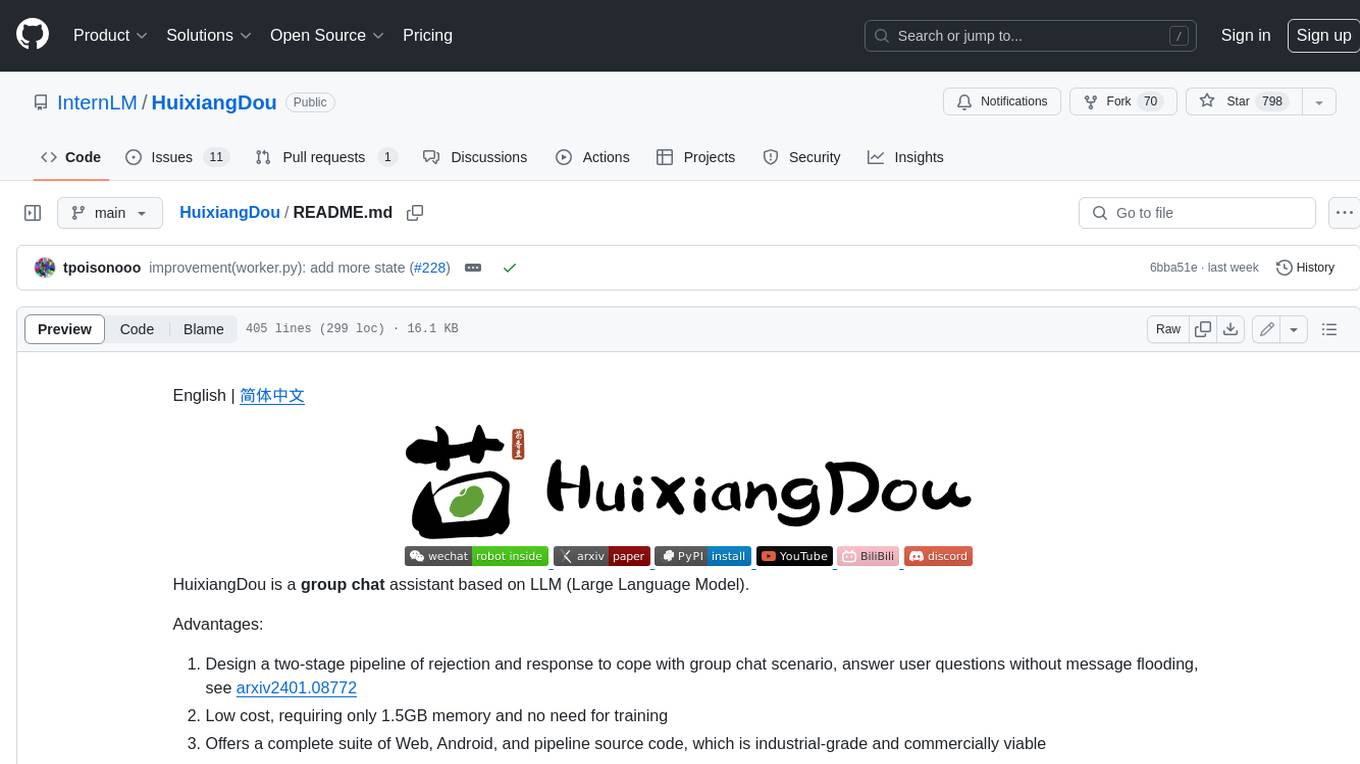
HuixiangDou
HuixiangDou is a **group chat** assistant based on LLM (Large Language Model). Advantages: 1. Design a two-stage pipeline of rejection and response to cope with group chat scenario, answer user questions without message flooding, see arxiv2401.08772 2. Low cost, requiring only 1.5GB memory and no need for training 3. Offers a complete suite of Web, Android, and pipeline source code, which is industrial-grade and commercially viable Check out the scenes in which HuixiangDou are running and join WeChat Group to try AI assistant inside. If this helps you, please give it a star โญ
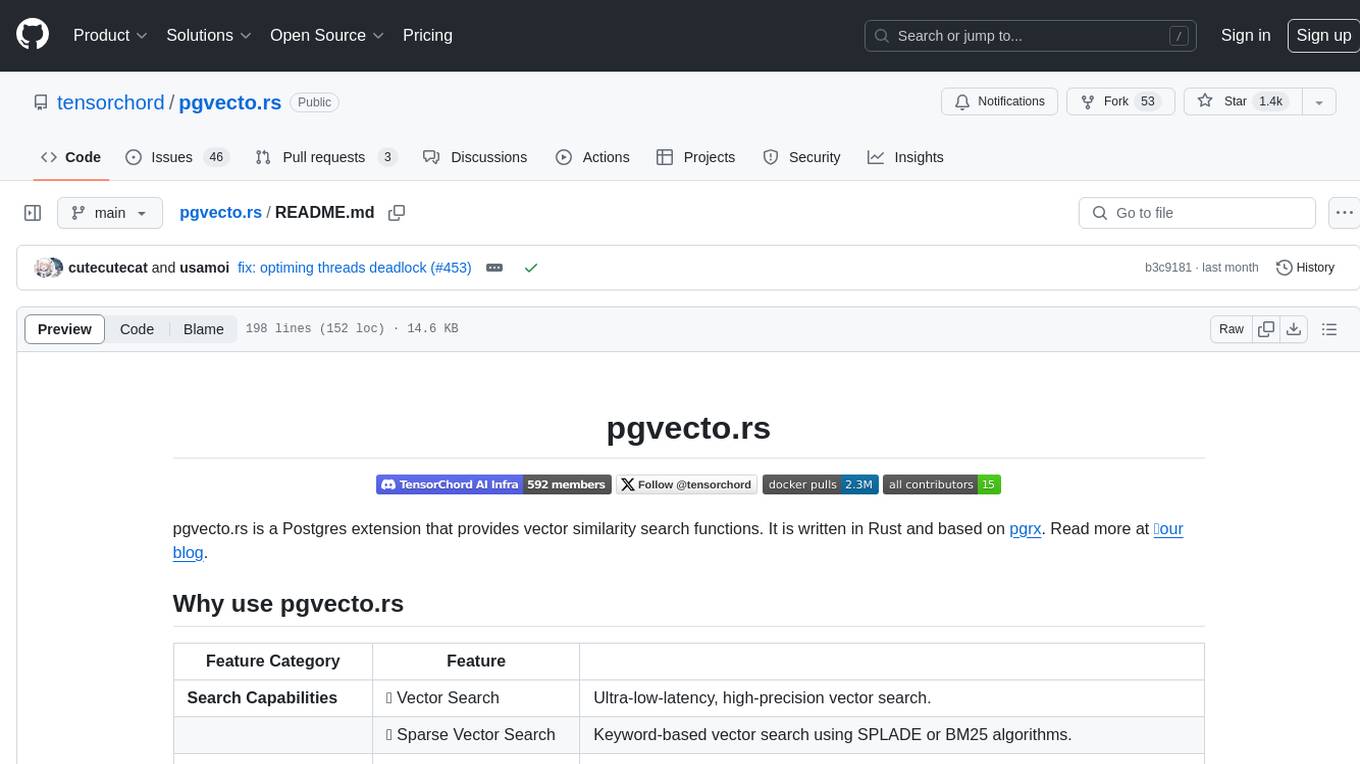
pgvecto.rs
pgvecto.rs is a Postgres extension written in Rust that provides vector similarity search functions. It offers ultra-low-latency, high-precision vector search capabilities, including sparse vector search and full-text search. With complete SQL support, async indexing, and easy data management, it simplifies data handling. The extension supports various data types like FP16/INT8, binary vectors, and Matryoshka embeddings. It ensures system performance with production-ready features, high availability, and resource efficiency. Security and permissions are managed through easy access control. The tool allows users to create tables with vector columns, insert vector data, and calculate distances between vectors using different operators. It also supports half-precision floating-point numbers for better performance and memory usage optimization.
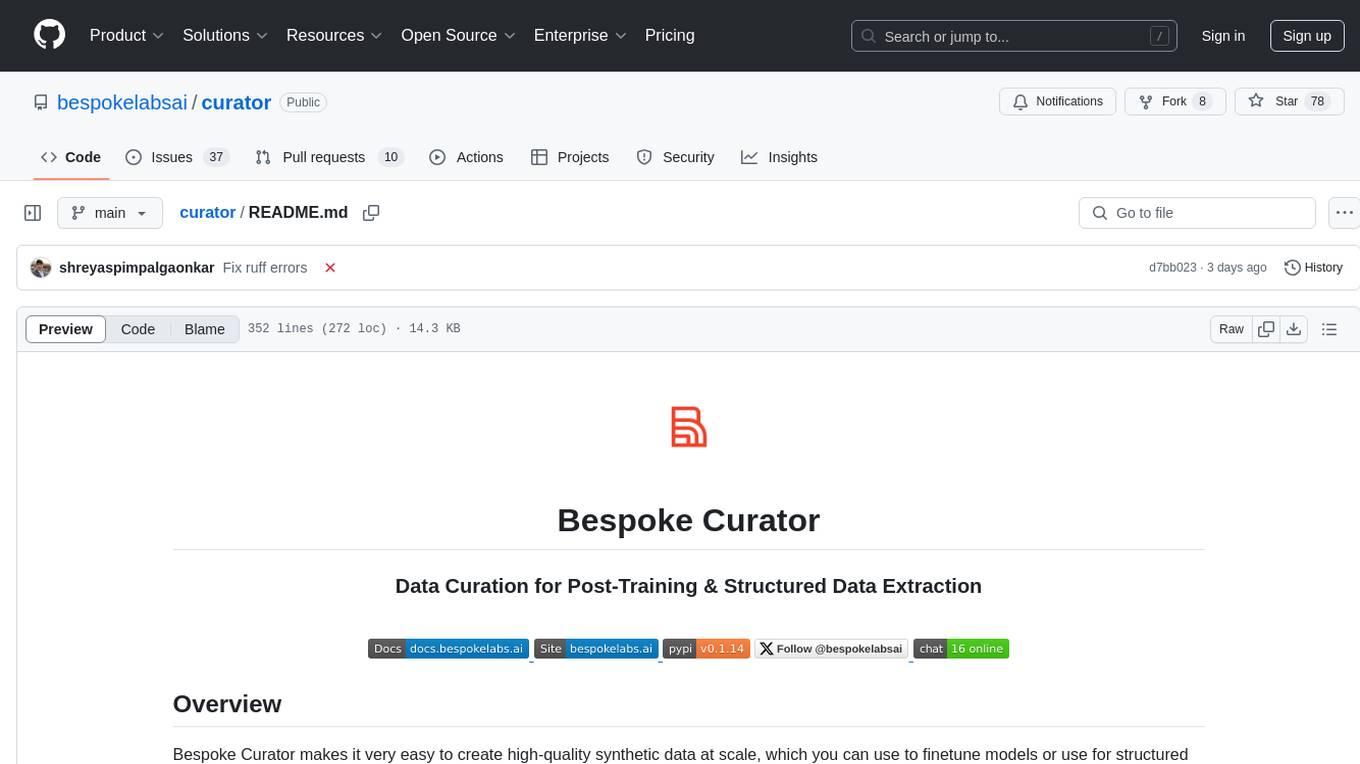
curator
Bespoke Curator is an open-source tool for data curation and structured data extraction. It provides a Python library for generating synthetic data at scale, with features like programmability, performance optimization, caching, and integration with HuggingFace Datasets. The tool includes a Curator Viewer for dataset visualization and offers a rich set of functionalities for creating and refining data generation strategies.
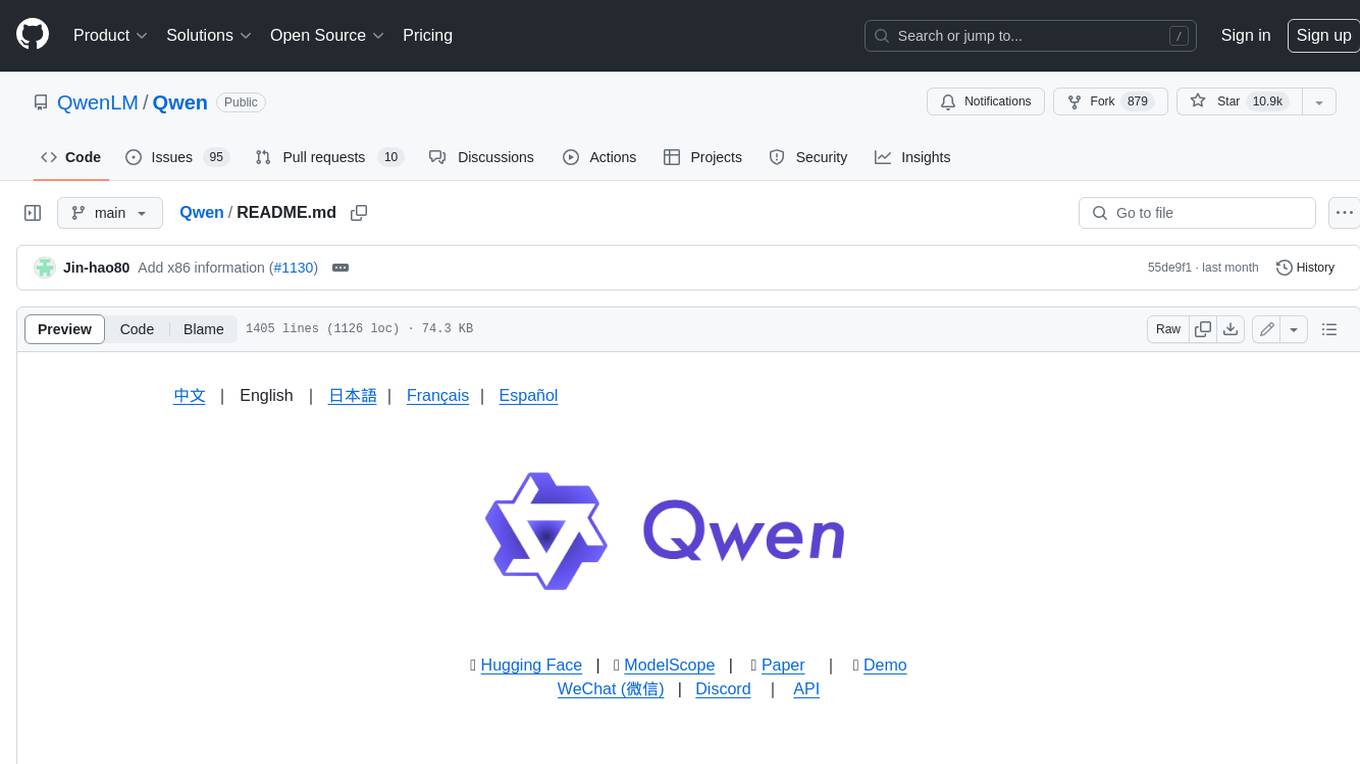
Qwen
Qwen is a series of large language models developed by Alibaba DAMO Academy. It outperforms the baseline models of similar model sizes on a series of benchmark datasets, e.g., MMLU, C-Eval, GSM8K, MATH, HumanEval, MBPP, BBH, etc., which evaluate the modelsโ capabilities on natural language understanding, mathematic problem solving, coding, etc. Qwen models outperform the baseline models of similar model sizes on a series of benchmark datasets, e.g., MMLU, C-Eval, GSM8K, MATH, HumanEval, MBPP, BBH, etc., which evaluate the modelsโ capabilities on natural language understanding, mathematic problem solving, coding, etc. Qwen-72B achieves better performance than LLaMA2-70B on all tasks and outperforms GPT-3.5 on 7 out of 10 tasks.
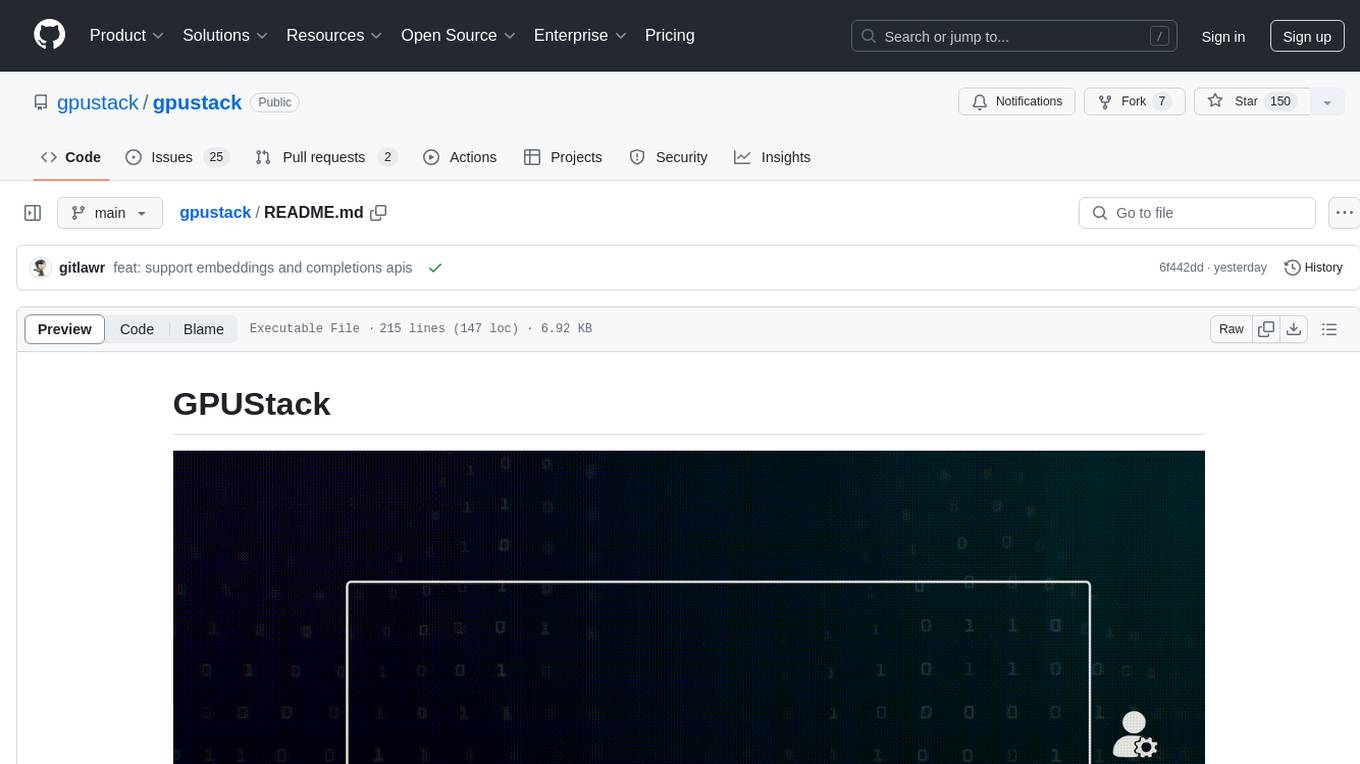
gpustack
GPUStack is an open-source GPU cluster manager designed for running large language models (LLMs). It supports a wide variety of hardware, scales with GPU inventory, offers lightweight Python package with minimal dependencies, provides OpenAI-compatible APIs, simplifies user and API key management, enables GPU metrics monitoring, and facilitates token usage and rate metrics tracking. The tool is suitable for managing GPU clusters efficiently and effectively.
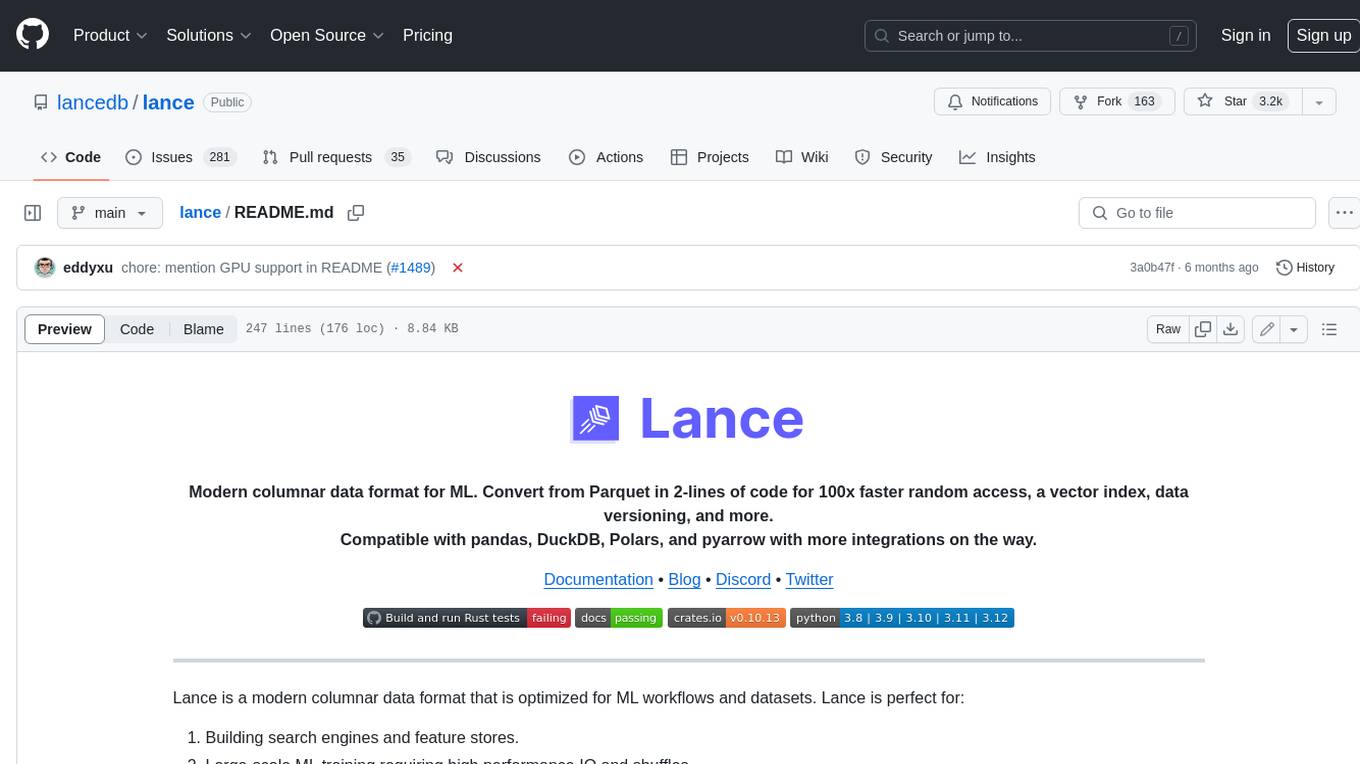
lance
Lance is a modern columnar data format optimized for ML workflows and datasets. It offers high-performance random access, vector search, zero-copy automatic versioning, and ecosystem integrations with Apache Arrow, Pandas, Polars, and DuckDB. Lance is designed to address the challenges of the ML development cycle, providing a unified data format for collection, exploration, analytics, feature engineering, training, evaluation, deployment, and monitoring. It aims to reduce data silos and streamline the ML development process.
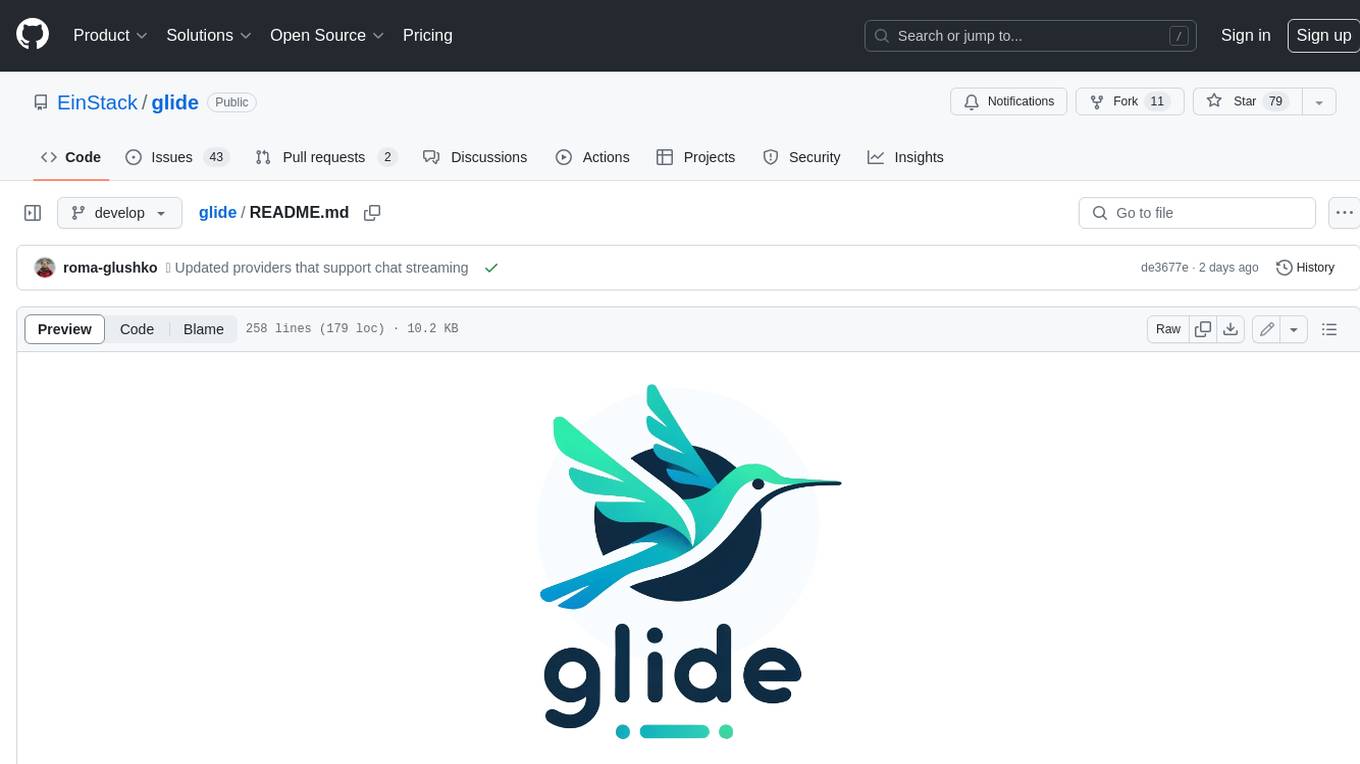
glide
Glide is a cloud-native LLM gateway that provides a unified REST API for accessing various large language models (LLMs) from different providers. It handles LLMOps tasks such as model failover, caching, key management, and more, making it easy to integrate LLMs into applications. Glide supports popular LLM providers like OpenAI, Anthropic, Azure OpenAI, AWS Bedrock (Titan), Cohere, Google Gemini, OctoML, and Ollama. It offers high availability, performance, and observability, and provides SDKs for Python and NodeJS to simplify integration.
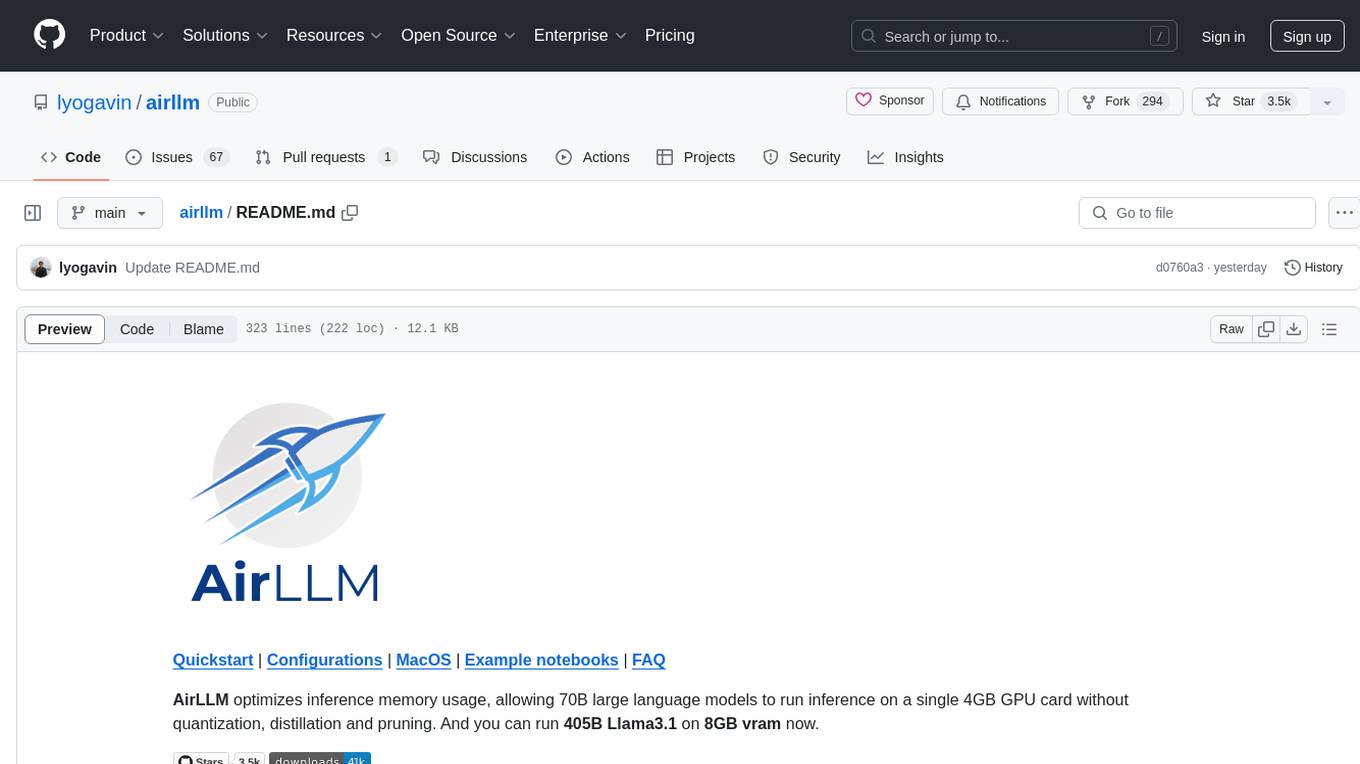
airllm
AirLLM is a tool that optimizes inference memory usage, enabling large language models to run on low-end GPUs without quantization, distillation, or pruning. It supports models like Llama3.1 on 8GB VRAM. The tool offers model compression for up to 3x inference speedup with minimal accuracy loss. Users can specify compression levels, profiling modes, and other configurations when initializing models. AirLLM also supports prefetching and disk space management. It provides examples and notebooks for easy implementation and usage.
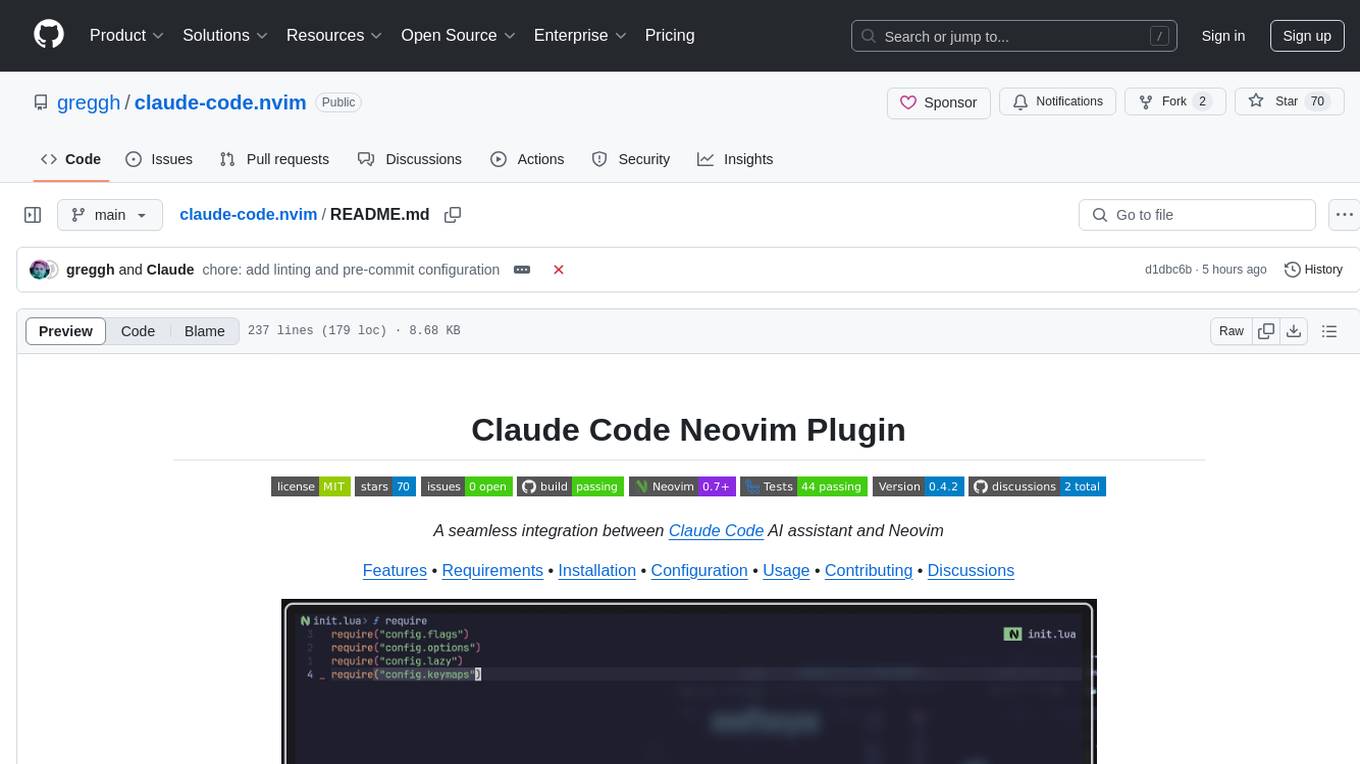
claude-code.nvim
Claude Code Neovim Plugin is a seamless integration between Claude Code AI assistant and Neovim. It allows users to toggle Claude Code in a terminal window with a single key press, automatically detect and reload files modified by Claude Code, provide real-time buffer updates when files are changed externally, offer customizable window position and size, integrate with which-key, use git project root as working directory, maintain a modular code structure, provide type annotations with LuaCATS for better IDE support, offer configuration validation, and include a testing framework for reliability. The plugin creates a terminal buffer running the Claude Code CLI, sets up autocommands to detect file changes on disk, automatically reloads files modified by Claude Code, provides keymaps and commands for toggling the terminal, and detects git repositories to set the working directory to the git root.
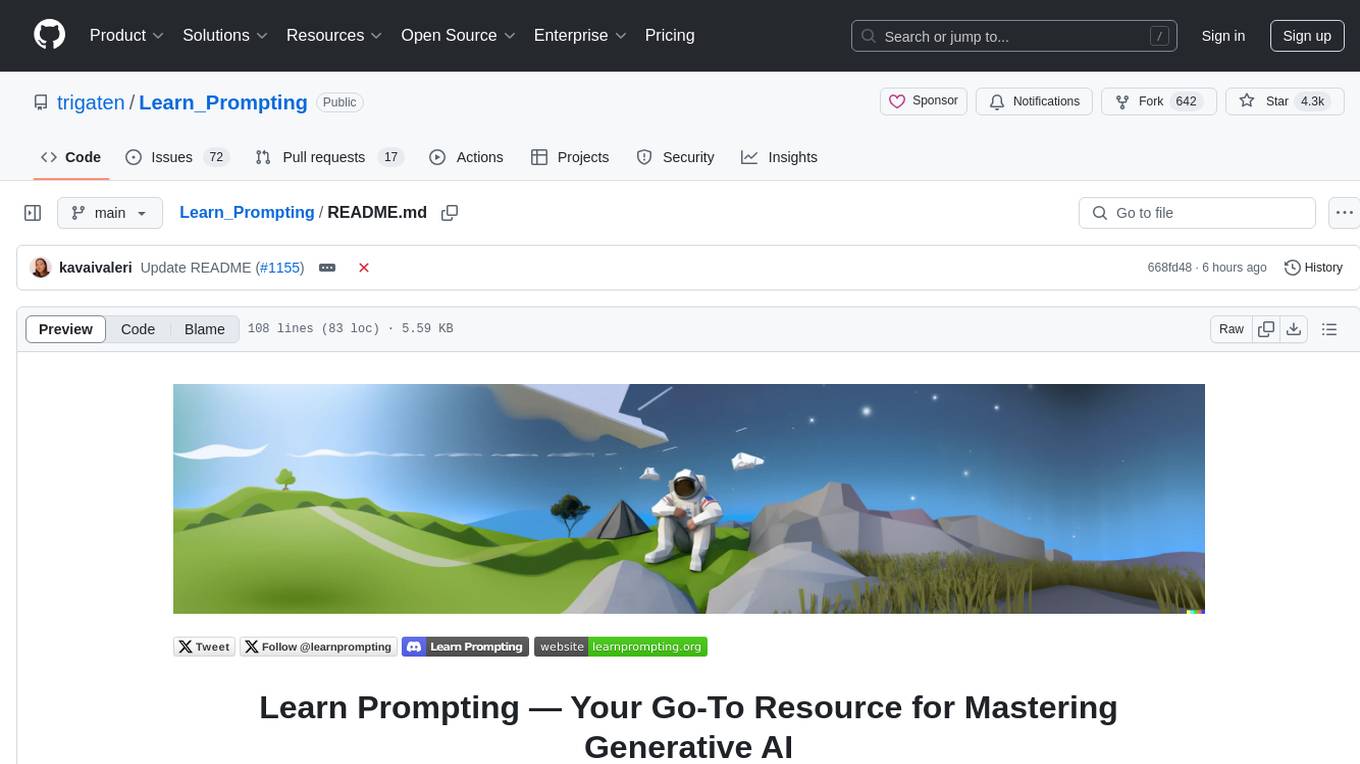
Learn_Prompting
Learn Prompting is a platform offering free resources, courses, and webinars to master prompt engineering and generative AI. It provides a Prompt Engineering Guide, courses on Generative AI, workshops, and the HackAPrompt competition. The platform also offers AI Red Teaming and AI Safety courses, research reports on prompting techniques, and welcomes contributions in various forms such as content suggestions, translations, artwork, and typo fixes. Users can locally develop the website using Visual Studio Code, Git, and Node.js, and run it in development mode to preview changes.
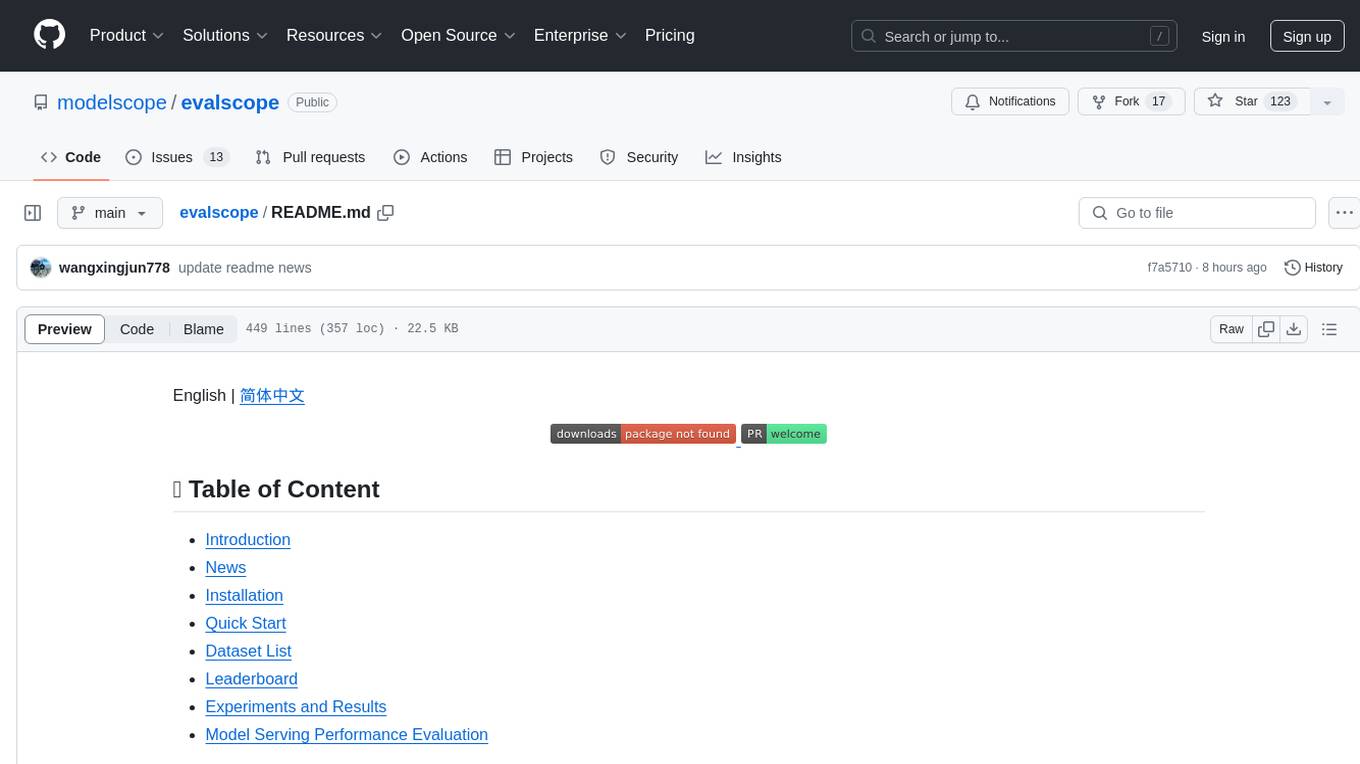
evalscope
Eval-Scope is a framework designed to support the evaluation of large language models (LLMs) by providing pre-configured benchmark datasets, common evaluation metrics, model integration, automatic evaluation for objective questions, complex task evaluation using expert models, reports generation, visualization tools, and model inference performance evaluation. It is lightweight, easy to customize, supports new dataset integration, model hosting on ModelScope, deployment of locally hosted models, and rich evaluation metrics. Eval-Scope also supports various evaluation modes like single mode, pairwise-baseline mode, and pairwise (all) mode, making it suitable for assessing and improving LLMs.
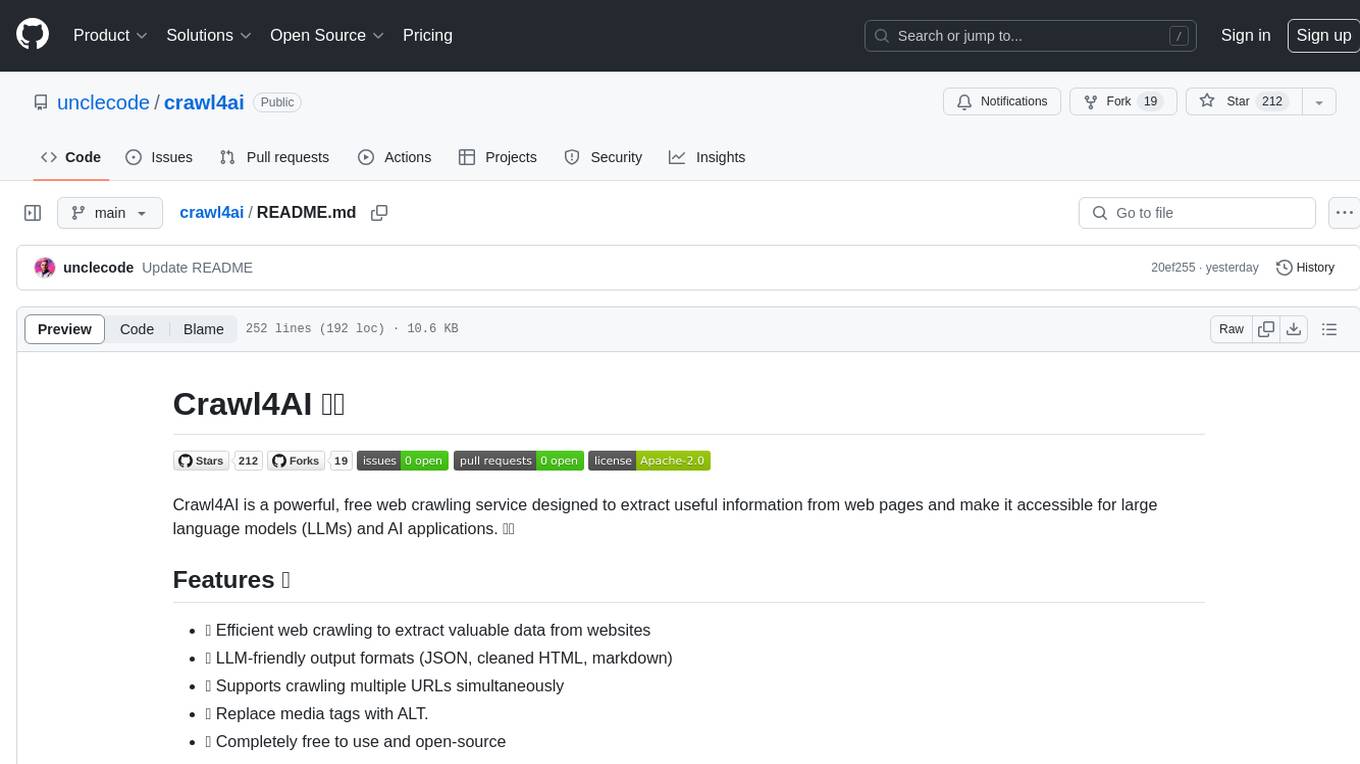
crawl4ai
Crawl4AI is a powerful and free web crawling service that extracts valuable data from websites and provides LLM-friendly output formats. It supports crawling multiple URLs simultaneously, replaces media tags with ALT, and is completely free to use and open-source. Users can integrate Crawl4AI into Python projects as a library or run it as a standalone local server. The tool allows users to crawl and extract data from specified URLs using different providers and models, with options to include raw HTML content, force fresh crawls, and extract meaningful text blocks. Configuration settings can be adjusted in the `crawler/config.py` file to customize providers, API keys, chunk processing, and word thresholds. Contributions to Crawl4AI are welcome from the open-source community to enhance its value for AI enthusiasts and developers.
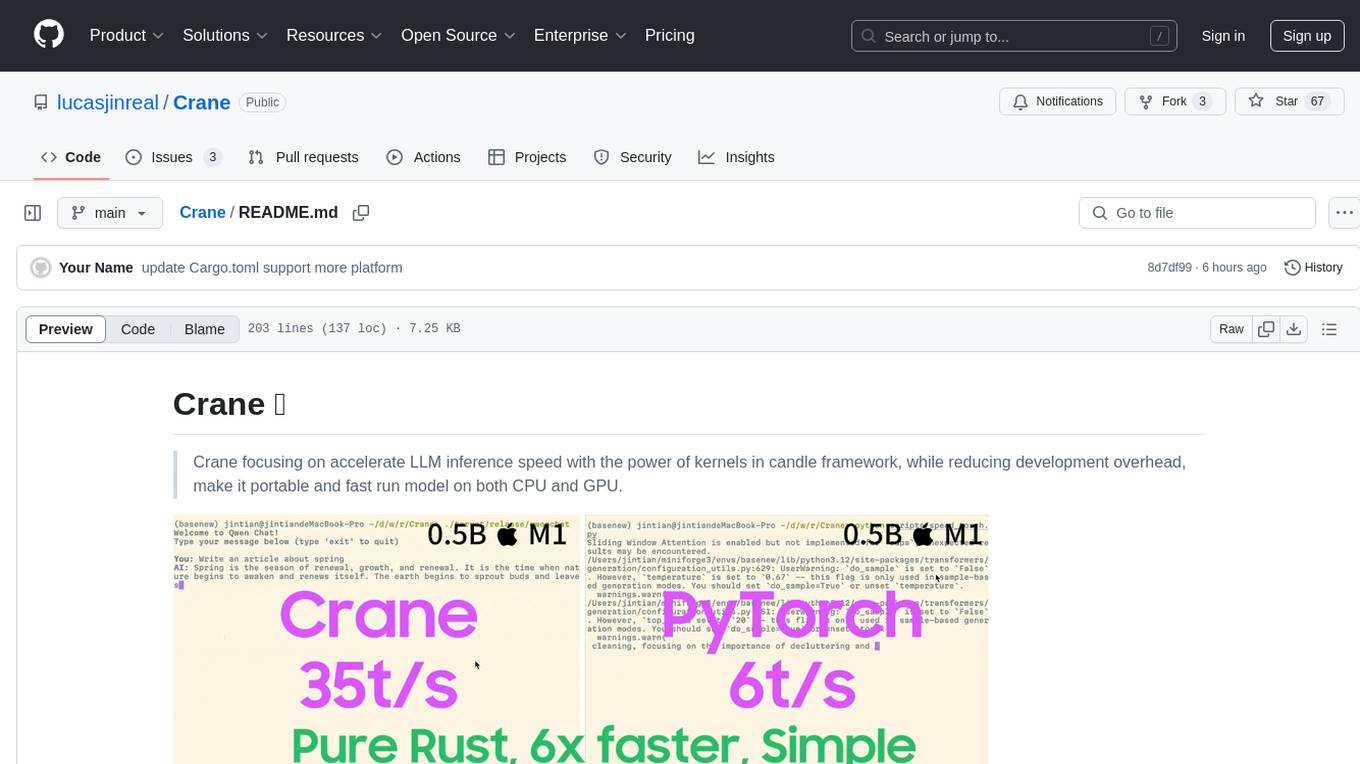
Crane
Crane is a high-performance inference framework leveraging Rust's Candle for maximum speed on CPU/GPU. It focuses on accelerating LLM inference speed with optimized kernels, reducing development overhead, and ensuring portability for running models on both CPU and GPU. Supported models include TTS systems like Spark-TTS and Orpheus-TTS, foundation models like Qwen2.5 series and basic LLMs, and multimodal models like Namo-R1 and Qwen2.5-VL. Key advantages of Crane include blazing-fast inference outperforming native PyTorch, Rust-powered to eliminate C++ complexity, Apple Silicon optimized for GPU acceleration via Metal, and hardware agnostic with a unified codebase for CPU/CUDA/Metal execution. Crane simplifies deployment with the ability to add new models with less than 100 lines of code in most cases.
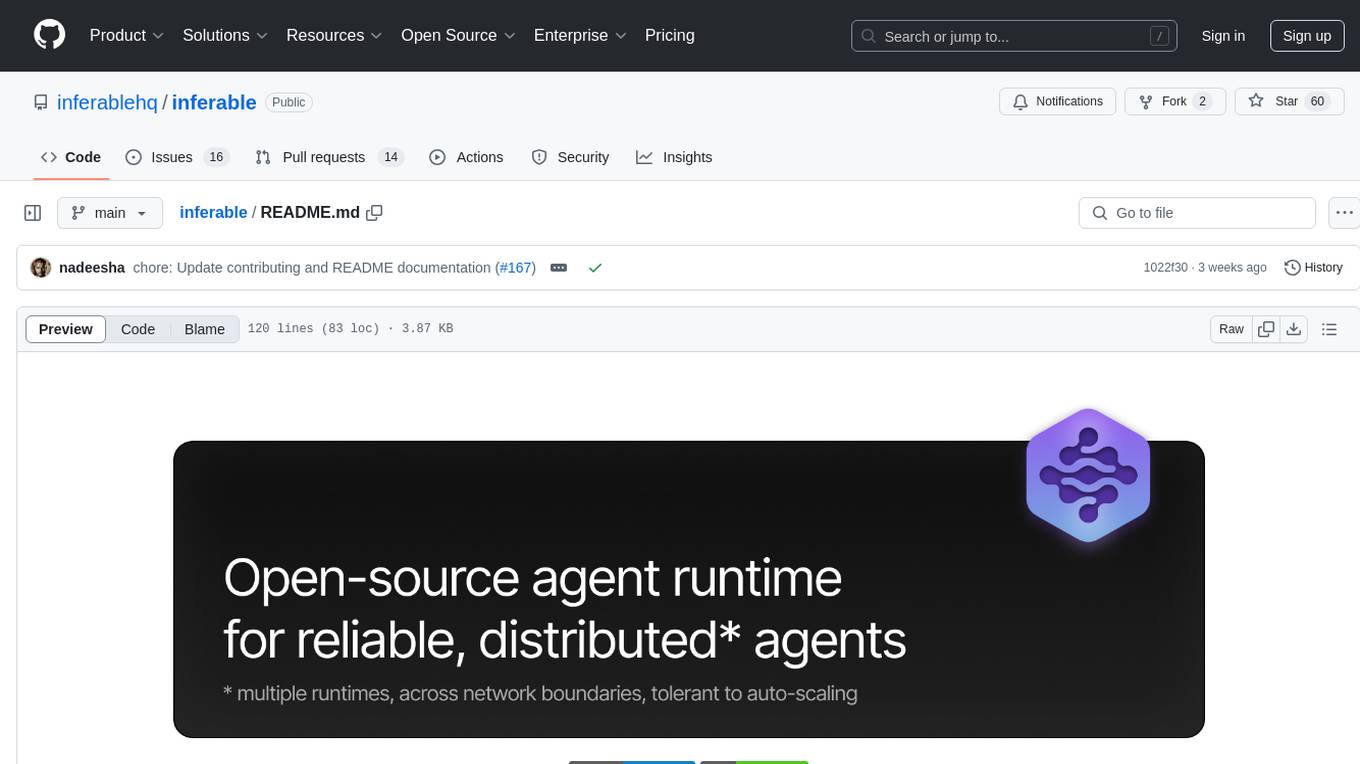
inferable
Inferable is an open source platform that helps users build reliable LLM-powered agentic automations at scale. It offers a managed agent runtime, durable tool calling, zero network configuration, multiple language support, and is fully open source under the MIT license. Users can define functions, register them with Inferable, and create runs that utilize these functions to automate tasks. The platform supports Node.js/TypeScript, Go, .NET, and React, and provides SDKs, core services, and bootstrap templates for various languages.
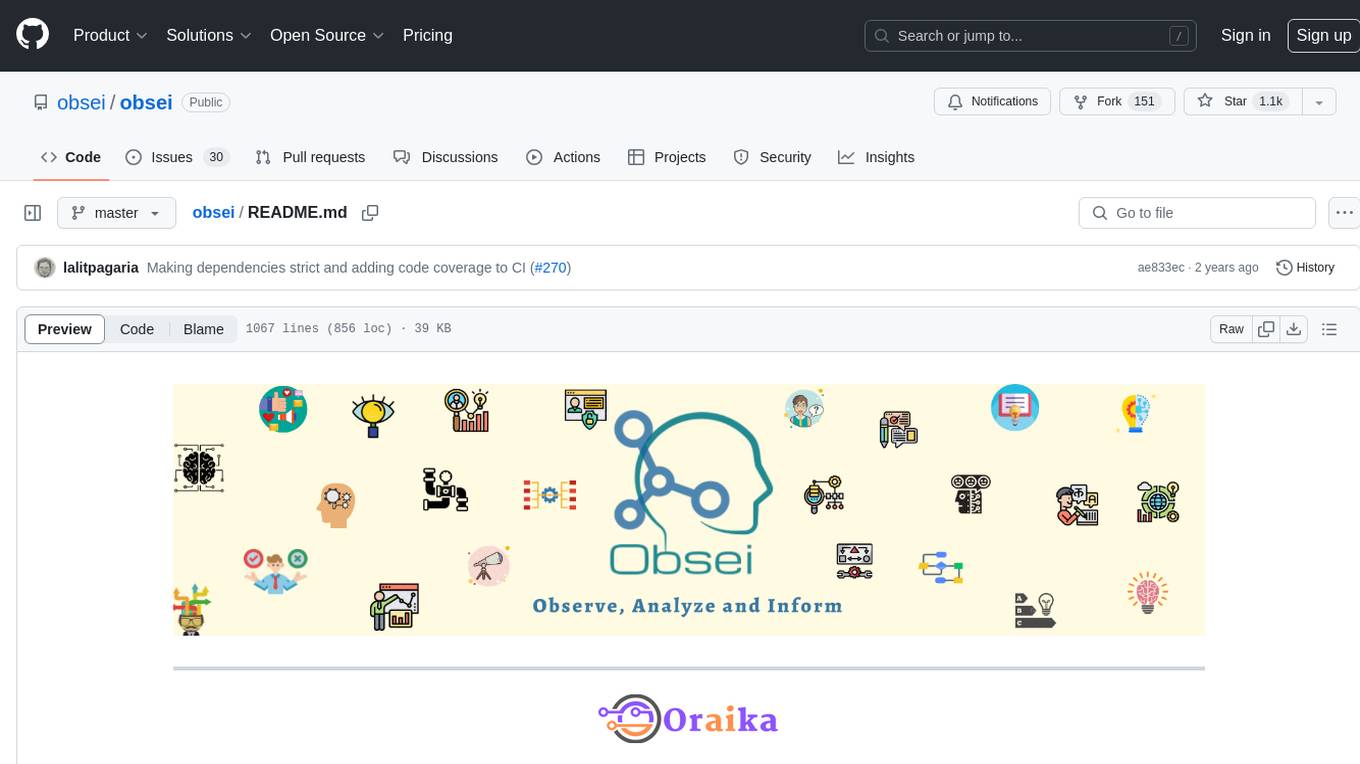
obsei
Obsei is an open-source, low-code, AI powered automation tool that consists of an Observer to collect unstructured data from various sources, an Analyzer to analyze the collected data with various AI tasks, and an Informer to send analyzed data to various destinations. The tool is suitable for scheduled jobs or serverless applications as all Observers can store their state in databases. Obsei is still in alpha stage, so caution is advised when using it in production. The tool can be used for social listening, alerting/notification, automatic customer issue creation, extraction of deeper insights from feedbacks, market research, dataset creation for various AI tasks, and more based on creativity.
For similar tasks
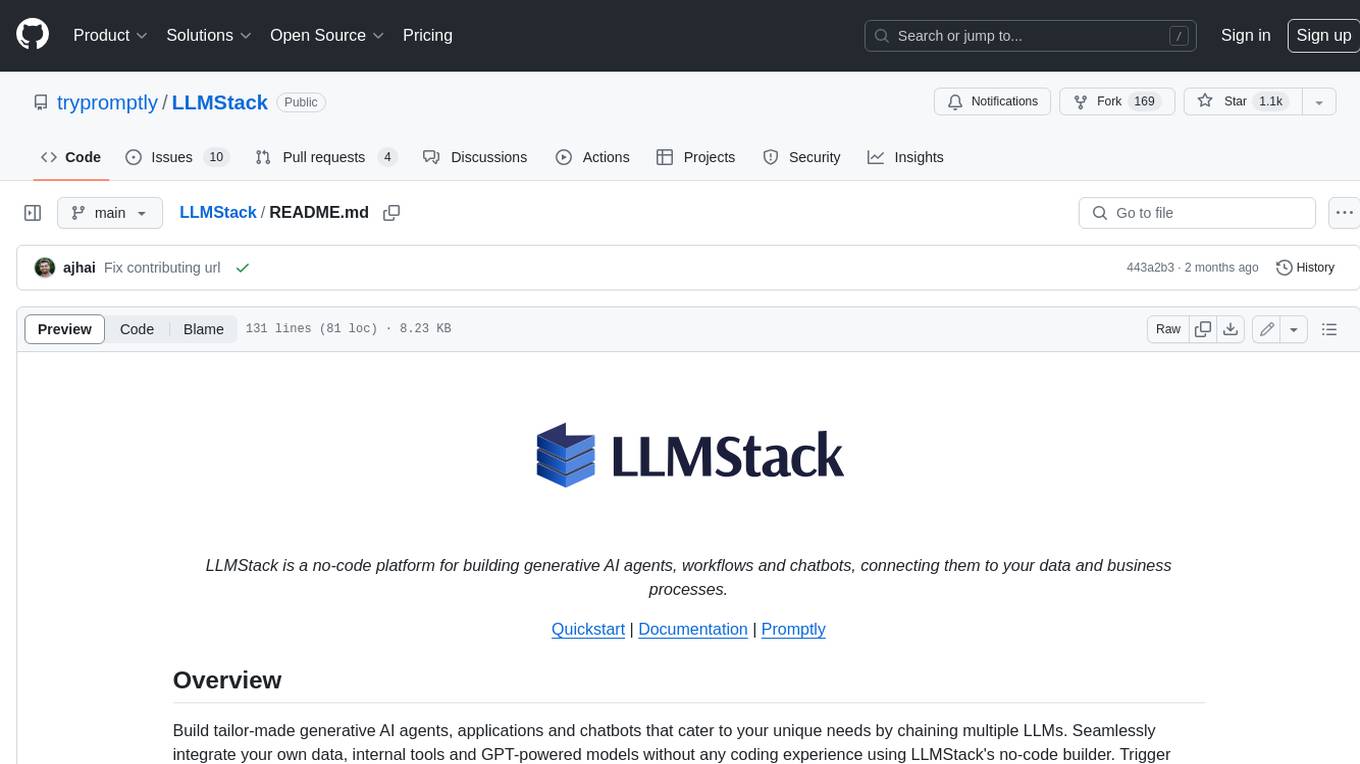
LLMStack
LLMStack is a no-code platform for building generative AI agents, workflows, and chatbots. It allows users to connect their own data, internal tools, and GPT-powered models without any coding experience. LLMStack can be deployed to the cloud or on-premise and can be accessed via HTTP API or triggered from Slack or Discord.
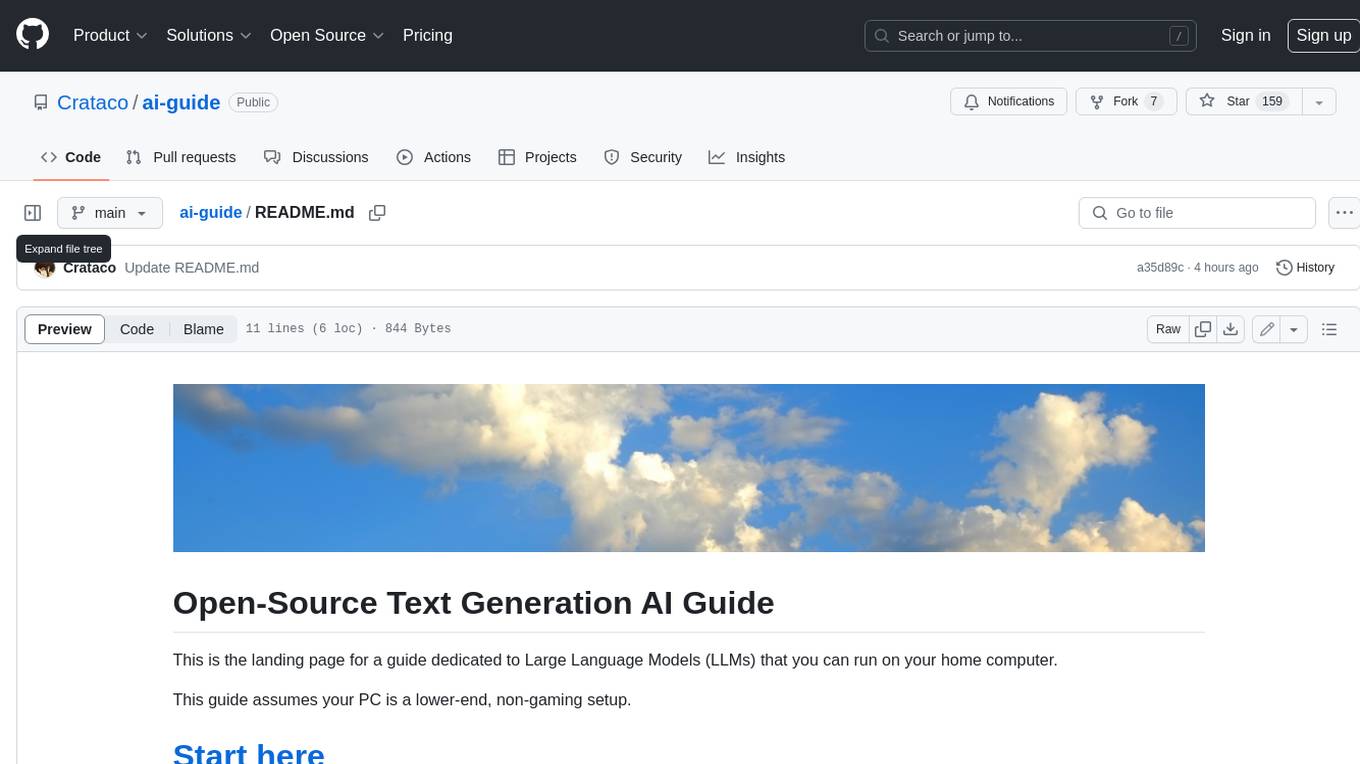
ai-guide
This guide is dedicated to Large Language Models (LLMs) that you can run on your home computer. It assumes your PC is a lower-end, non-gaming setup.
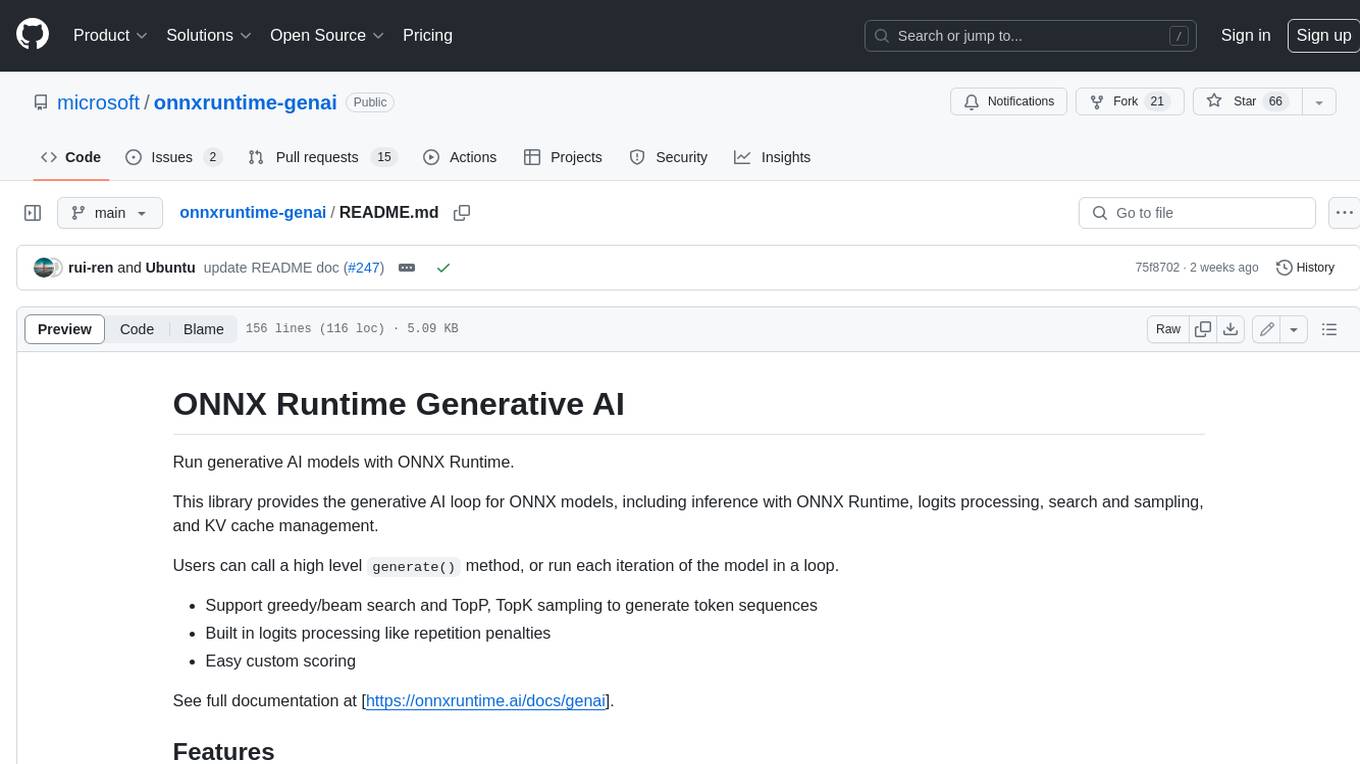
onnxruntime-genai
ONNX Runtime Generative AI is a library that provides the generative AI loop for ONNX models, including inference with ONNX Runtime, logits processing, search and sampling, and KV cache management. Users can call a high level `generate()` method, or run each iteration of the model in a loop. It supports greedy/beam search and TopP, TopK sampling to generate token sequences, has built in logits processing like repetition penalties, and allows for easy custom scoring.
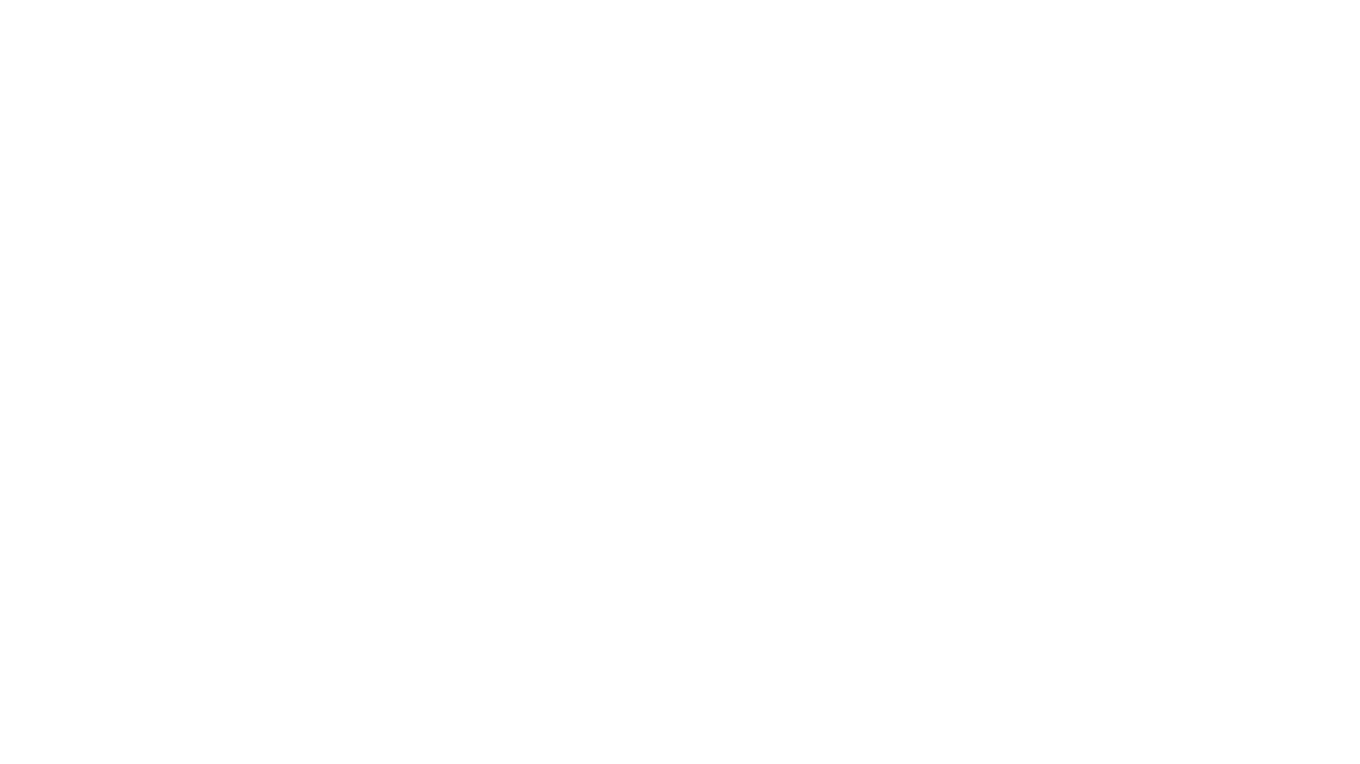
jupyter-ai
Jupyter AI connects generative AI with Jupyter notebooks. It provides a user-friendly and powerful way to explore generative AI models in notebooks and improve your productivity in JupyterLab and the Jupyter Notebook. Specifically, Jupyter AI offers: * An `%%ai` magic that turns the Jupyter notebook into a reproducible generative AI playground. This works anywhere the IPython kernel runs (JupyterLab, Jupyter Notebook, Google Colab, Kaggle, VSCode, etc.). * A native chat UI in JupyterLab that enables you to work with generative AI as a conversational assistant. * Support for a wide range of generative model providers, including AI21, Anthropic, AWS, Cohere, Gemini, Hugging Face, NVIDIA, and OpenAI. * Local model support through GPT4All, enabling use of generative AI models on consumer grade machines with ease and privacy.
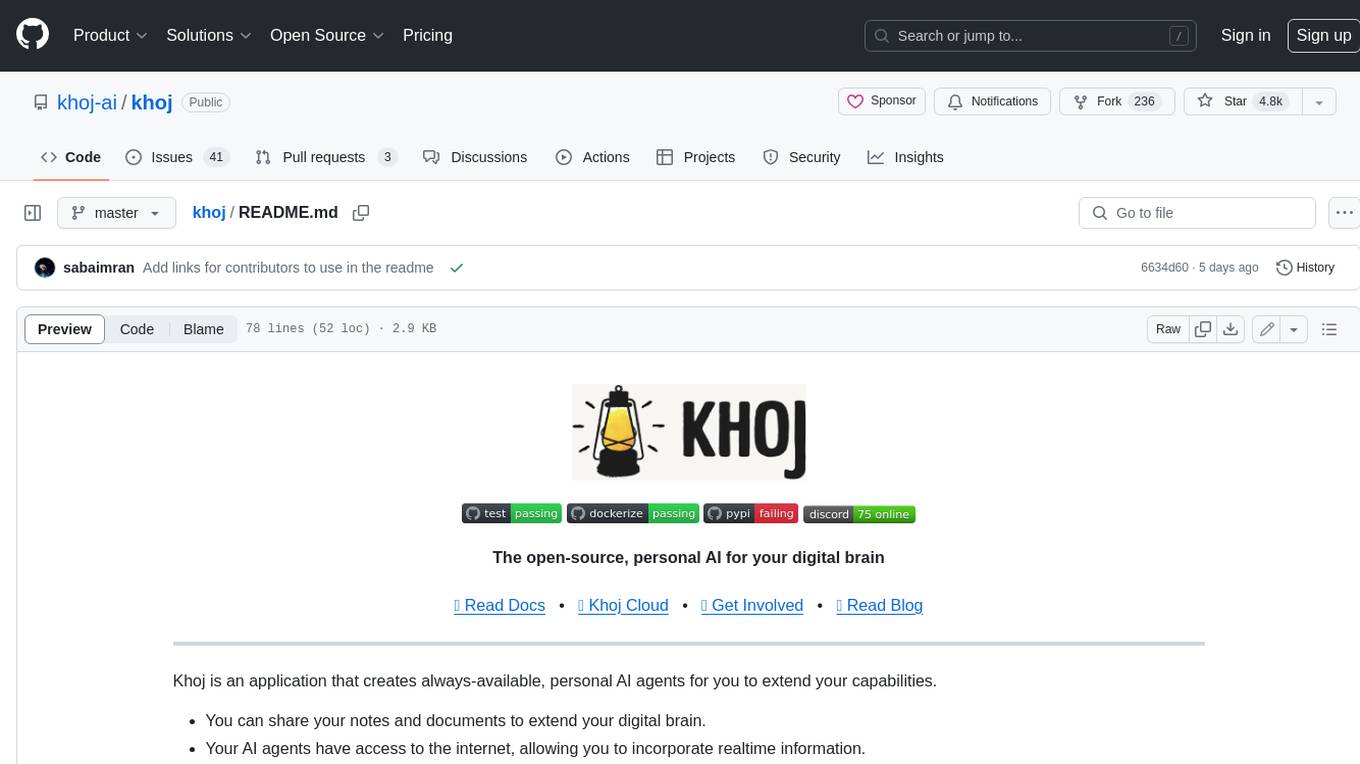
khoj
Khoj is an open-source, personal AI assistant that extends your capabilities by creating always-available AI agents. You can share your notes and documents to extend your digital brain, and your AI agents have access to the internet, allowing you to incorporate real-time information. Khoj is accessible on Desktop, Emacs, Obsidian, Web, and Whatsapp, and you can share PDF, markdown, org-mode, notion files, and GitHub repositories. You'll get fast, accurate semantic search on top of your docs, and your agents can create deeply personal images and understand your speech. Khoj is self-hostable and always will be.
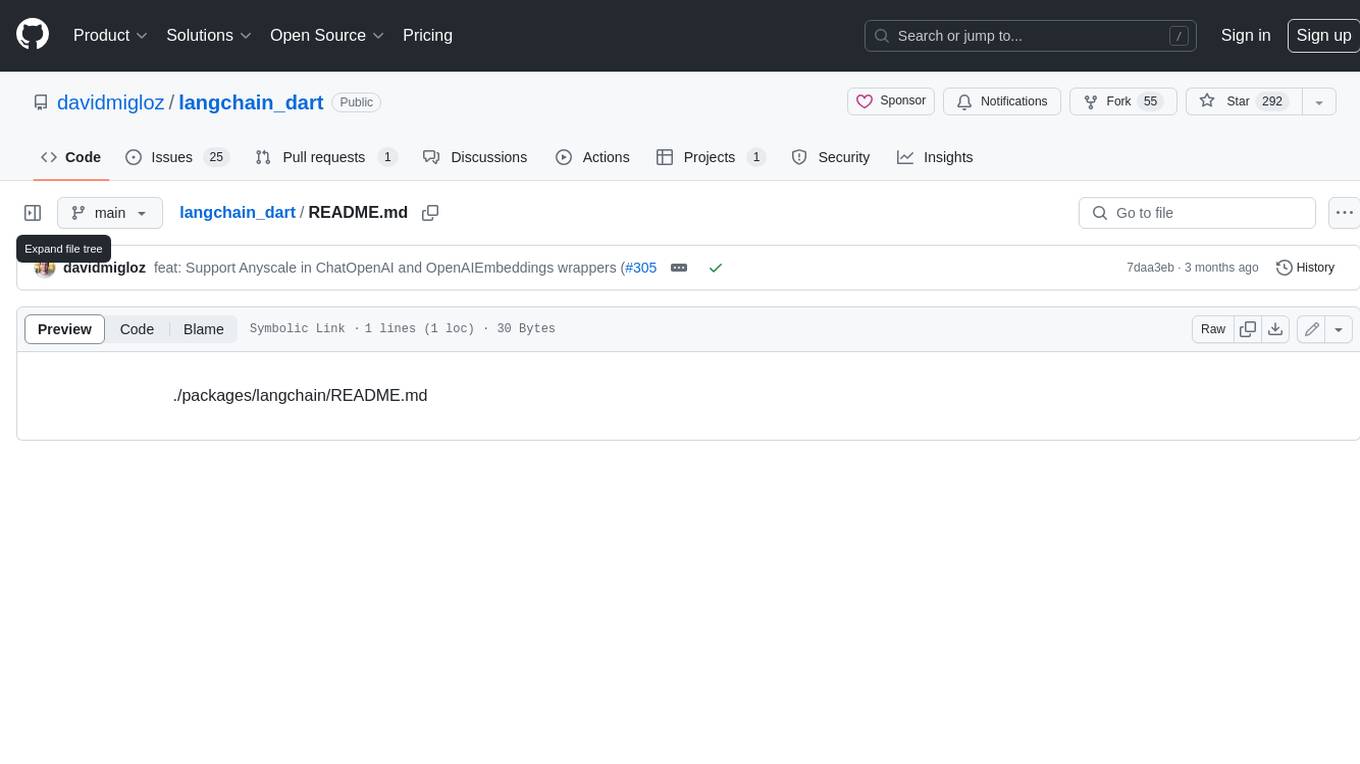
langchain_dart
LangChain.dart is a Dart port of the popular LangChain Python framework created by Harrison Chase. LangChain provides a set of ready-to-use components for working with language models and a standard interface for chaining them together to formulate more advanced use cases (e.g. chatbots, Q&A with RAG, agents, summarization, extraction, etc.). The components can be grouped into a few core modules: * **Model I/O:** LangChain offers a unified API for interacting with various LLM providers (e.g. OpenAI, Google, Mistral, Ollama, etc.), allowing developers to switch between them with ease. Additionally, it provides tools for managing model inputs (prompt templates and example selectors) and parsing the resulting model outputs (output parsers). * **Retrieval:** assists in loading user data (via document loaders), transforming it (with text splitters), extracting its meaning (using embedding models), storing (in vector stores) and retrieving it (through retrievers) so that it can be used to ground the model's responses (i.e. Retrieval-Augmented Generation or RAG). * **Agents:** "bots" that leverage LLMs to make informed decisions about which available tools (such as web search, calculators, database lookup, etc.) to use to accomplish the designated task. The different components can be composed together using the LangChain Expression Language (LCEL).
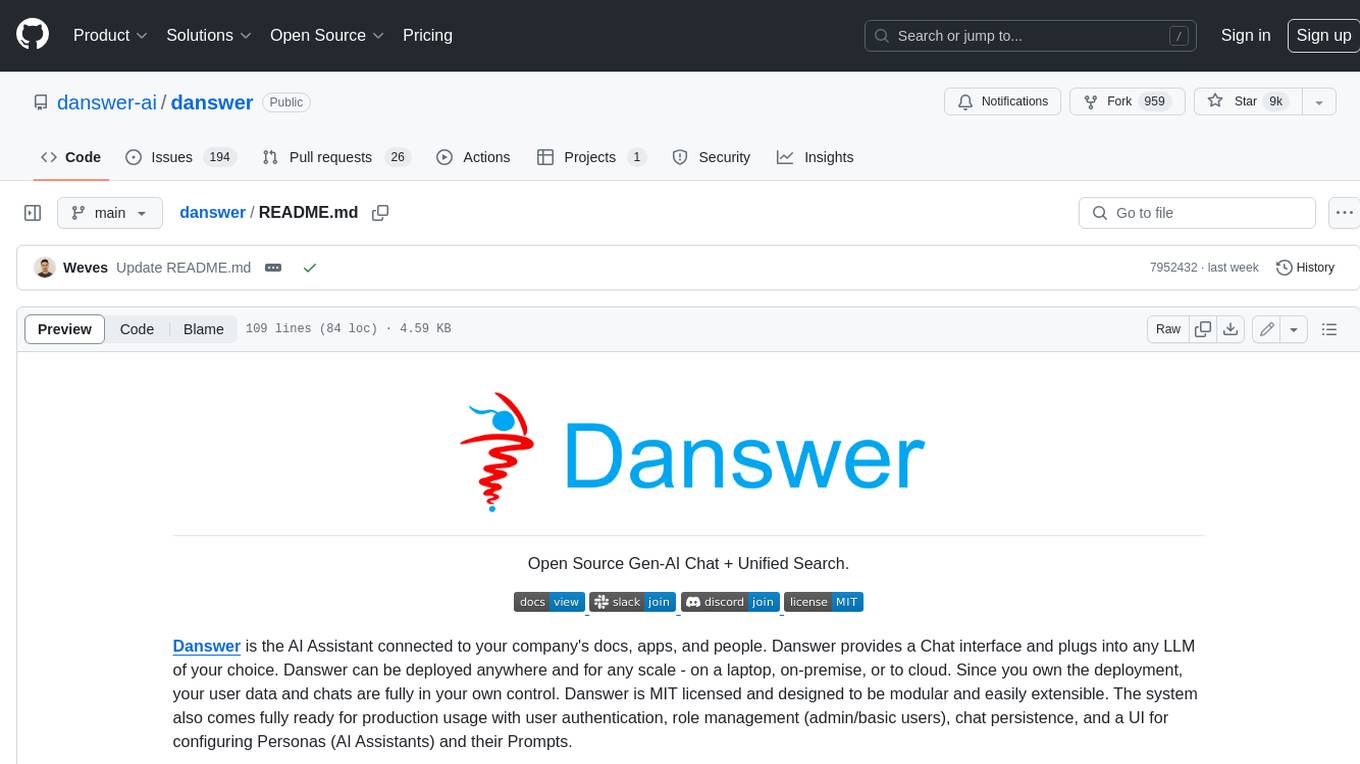
danswer
Danswer is an open-source Gen-AI Chat and Unified Search tool that connects to your company's docs, apps, and people. It provides a Chat interface and plugs into any LLM of your choice. Danswer can be deployed anywhere and for any scale - on a laptop, on-premise, or to cloud. Since you own the deployment, your user data and chats are fully in your own control. Danswer is MIT licensed and designed to be modular and easily extensible. The system also comes fully ready for production usage with user authentication, role management (admin/basic users), chat persistence, and a UI for configuring Personas (AI Assistants) and their Prompts. Danswer also serves as a Unified Search across all common workplace tools such as Slack, Google Drive, Confluence, etc. By combining LLMs and team specific knowledge, Danswer becomes a subject matter expert for the team. Imagine ChatGPT if it had access to your team's unique knowledge! It enables questions such as "A customer wants feature X, is this already supported?" or "Where's the pull request for feature Y?"
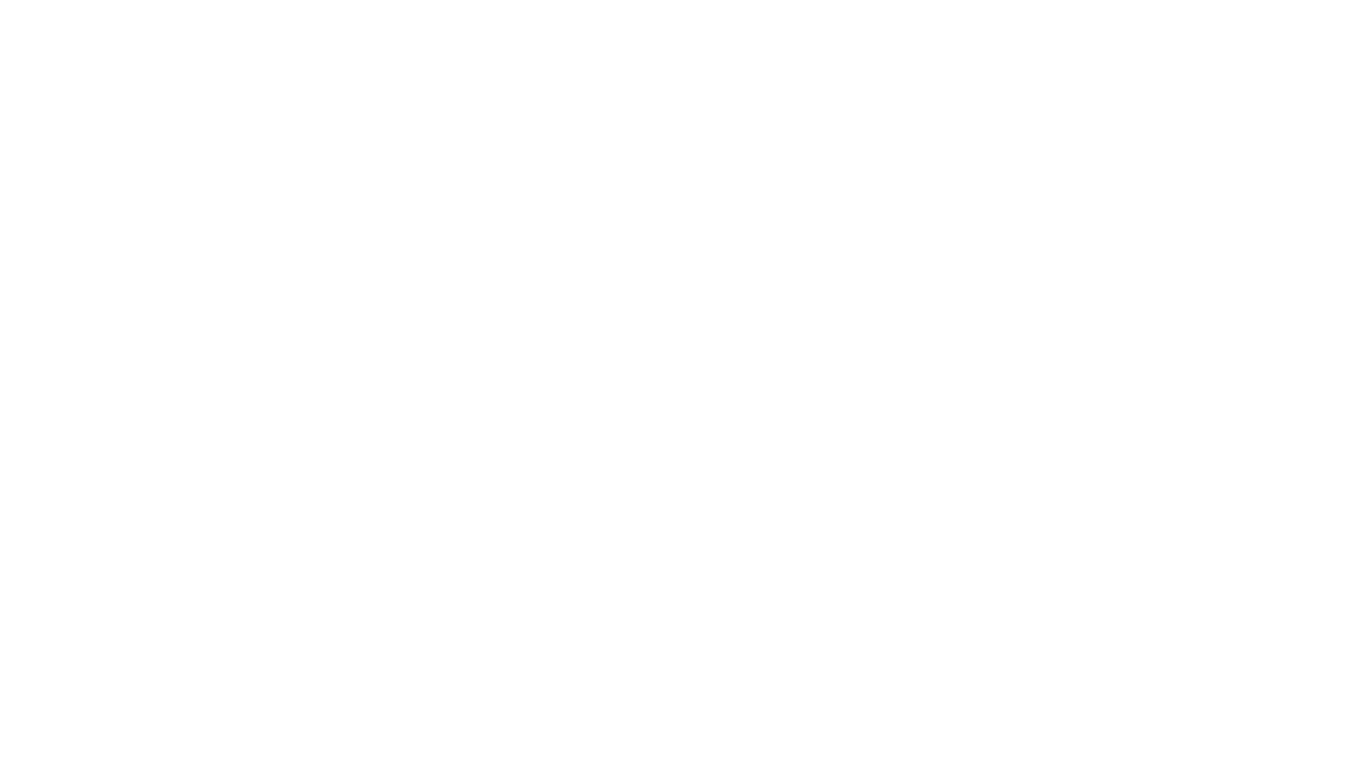
infinity
Infinity is an AI-native database designed for LLM applications, providing incredibly fast full-text and vector search capabilities. It supports a wide range of data types, including vectors, full-text, and structured data, and offers a fused search feature that combines multiple embeddings and full text. Infinity is easy to use, with an intuitive Python API and a single-binary architecture that simplifies deployment. It achieves high performance, with 0.1 milliseconds query latency on million-scale vector datasets and up to 15K QPS.
For similar jobs
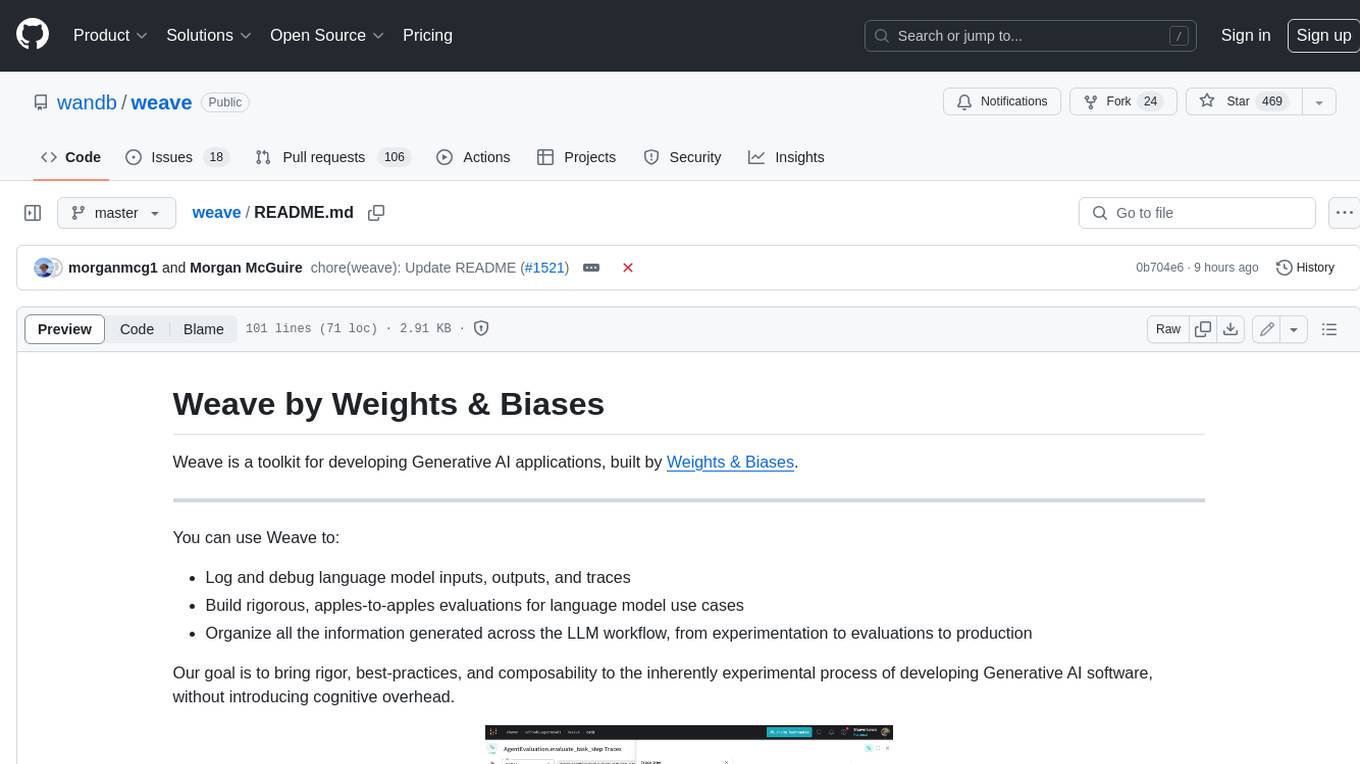
weave
Weave is a toolkit for developing Generative AI applications, built by Weights & Biases. With Weave, you can log and debug language model inputs, outputs, and traces; build rigorous, apples-to-apples evaluations for language model use cases; and organize all the information generated across the LLM workflow, from experimentation to evaluations to production. Weave aims to bring rigor, best-practices, and composability to the inherently experimental process of developing Generative AI software, without introducing cognitive overhead.
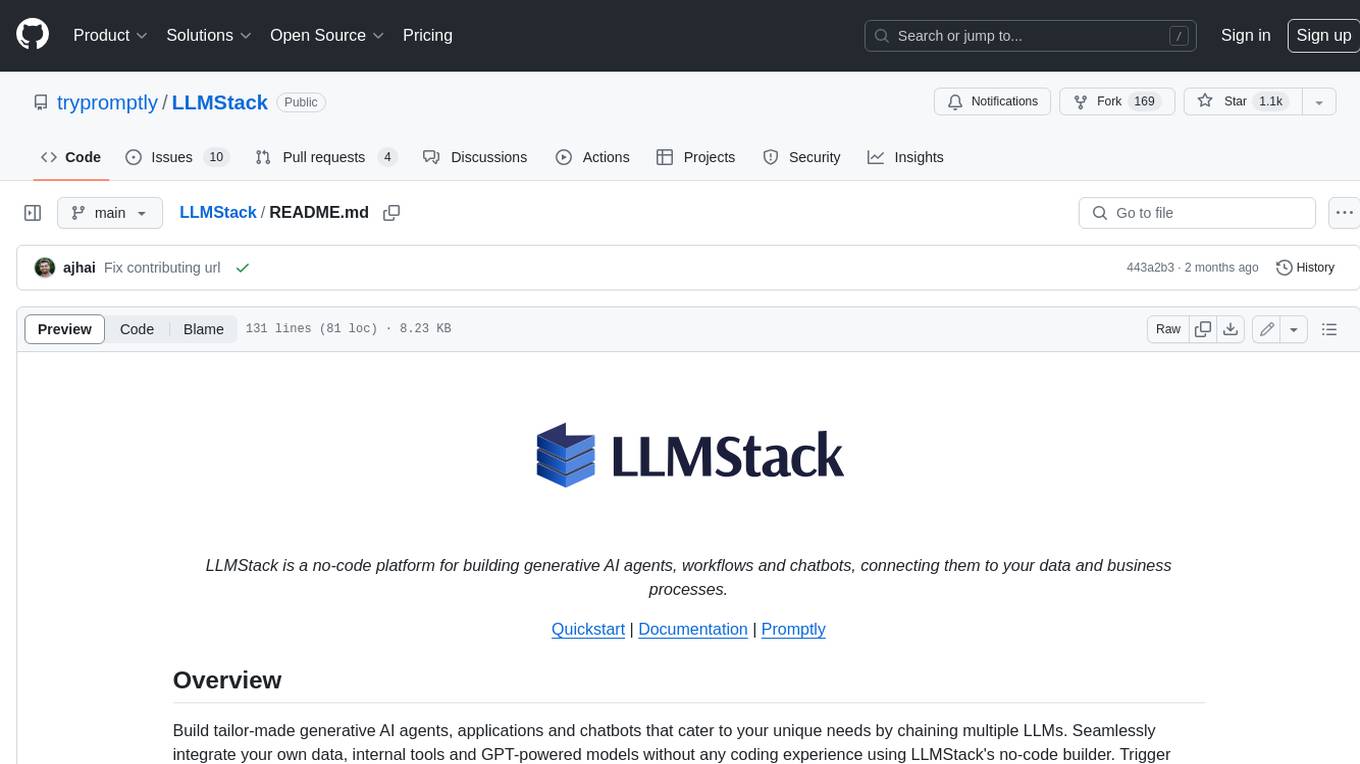
LLMStack
LLMStack is a no-code platform for building generative AI agents, workflows, and chatbots. It allows users to connect their own data, internal tools, and GPT-powered models without any coding experience. LLMStack can be deployed to the cloud or on-premise and can be accessed via HTTP API or triggered from Slack or Discord.
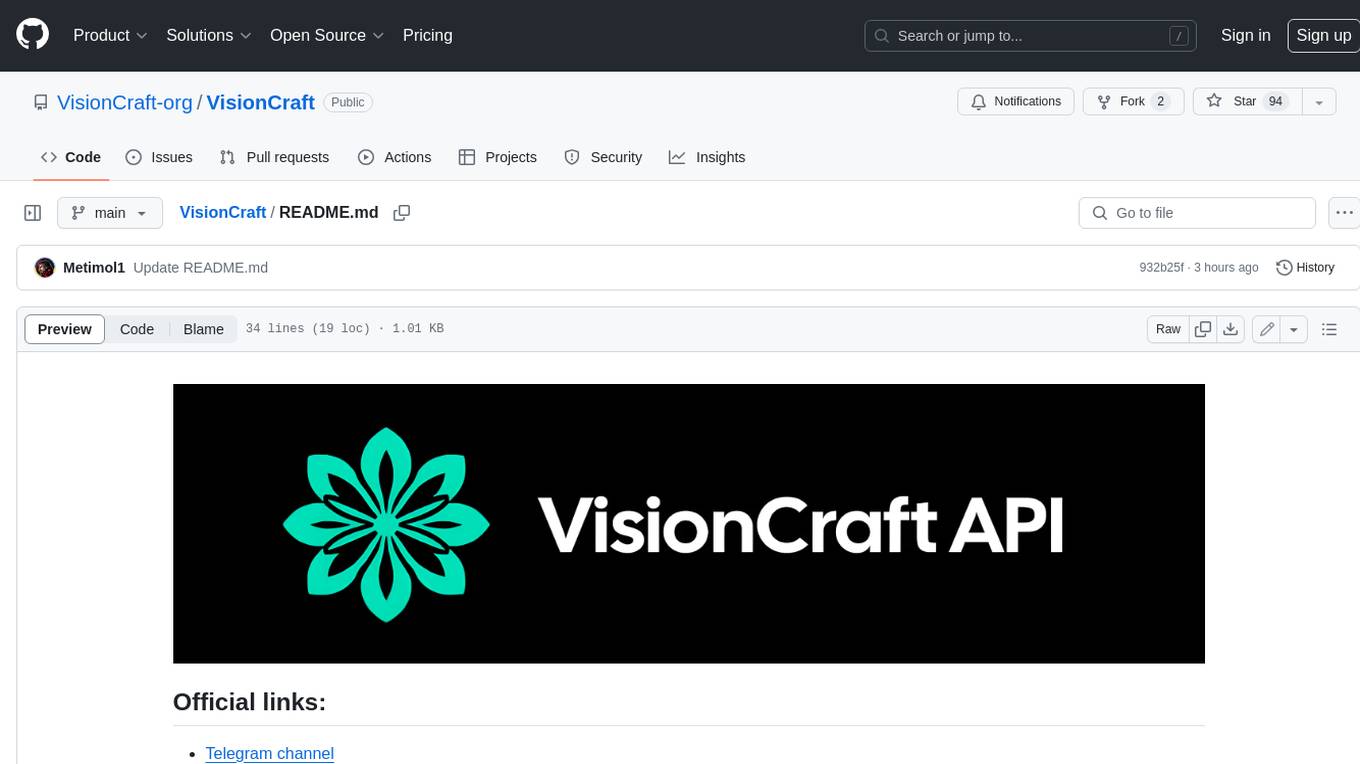
VisionCraft
The VisionCraft API is a free API for using over 100 different AI models. From images to sound.
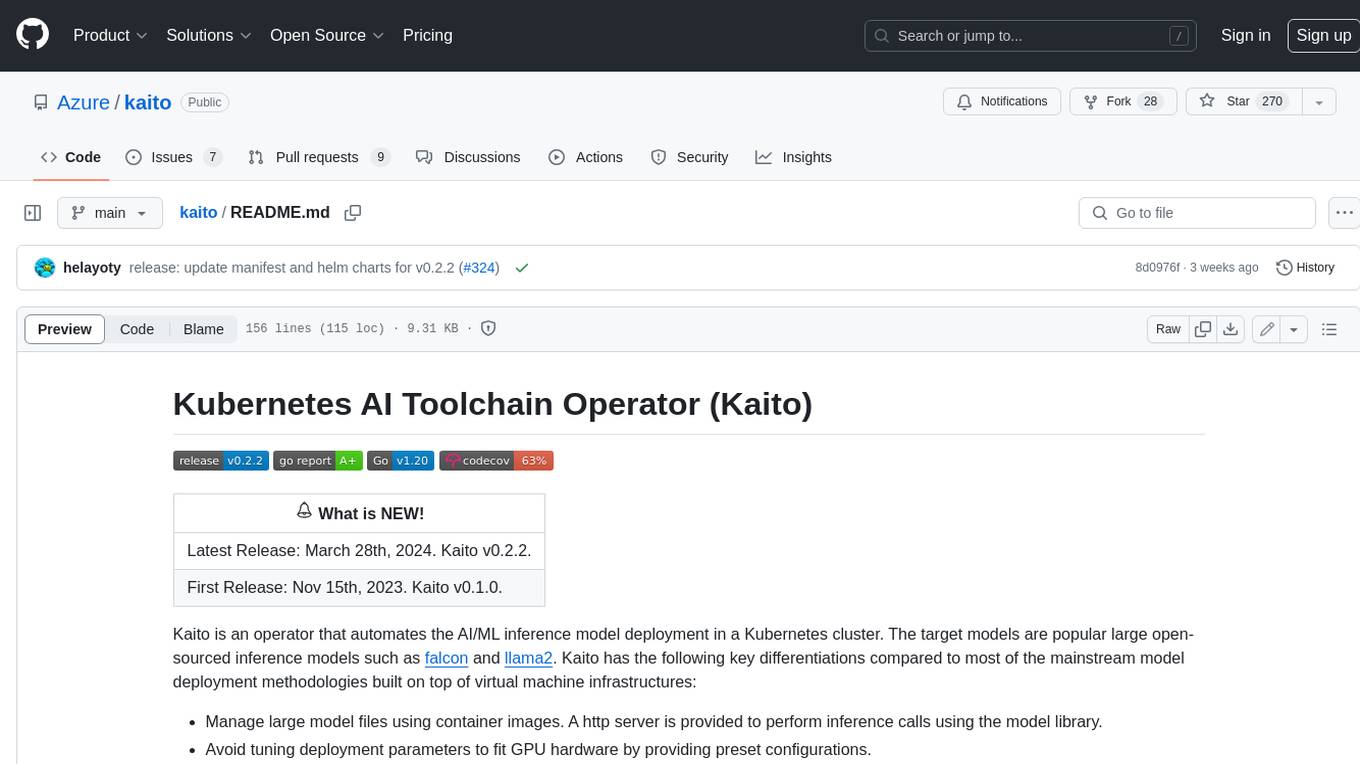
kaito
Kaito is an operator that automates the AI/ML inference model deployment in a Kubernetes cluster. It manages large model files using container images, avoids tuning deployment parameters to fit GPU hardware by providing preset configurations, auto-provisions GPU nodes based on model requirements, and hosts large model images in the public Microsoft Container Registry (MCR) if the license allows. Using Kaito, the workflow of onboarding large AI inference models in Kubernetes is largely simplified.
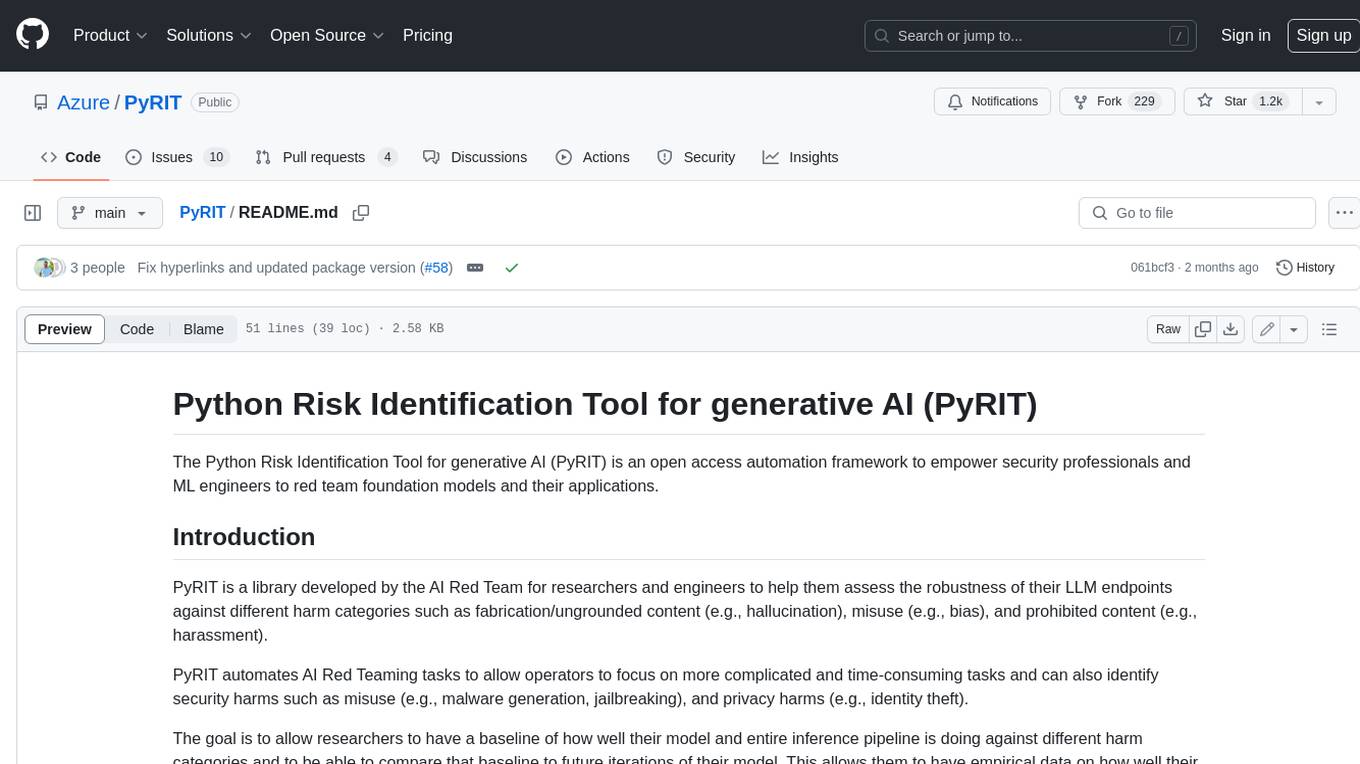
PyRIT
PyRIT is an open access automation framework designed to empower security professionals and ML engineers to red team foundation models and their applications. It automates AI Red Teaming tasks to allow operators to focus on more complicated and time-consuming tasks and can also identify security harms such as misuse (e.g., malware generation, jailbreaking), and privacy harms (e.g., identity theft). The goal is to allow researchers to have a baseline of how well their model and entire inference pipeline is doing against different harm categories and to be able to compare that baseline to future iterations of their model. This allows them to have empirical data on how well their model is doing today, and detect any degradation of performance based on future improvements.
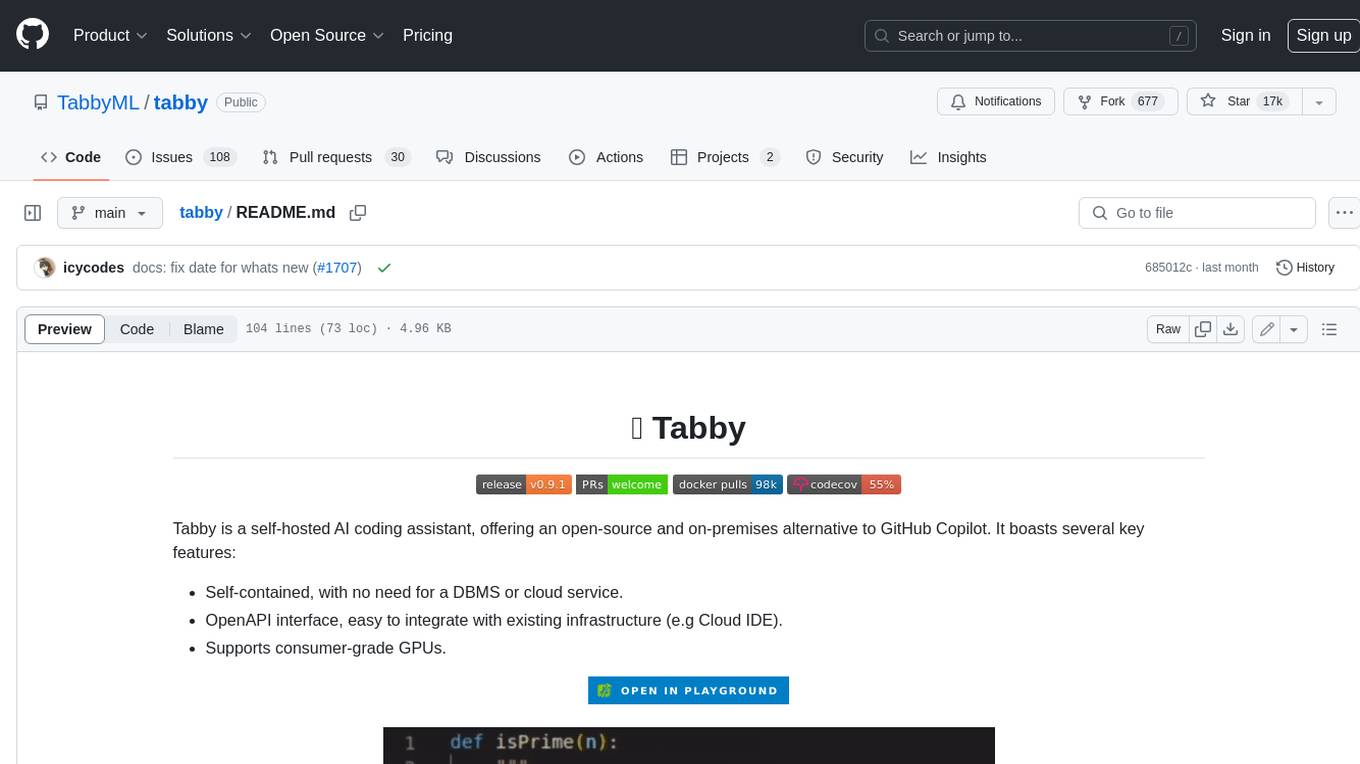
tabby
Tabby is a self-hosted AI coding assistant, offering an open-source and on-premises alternative to GitHub Copilot. It boasts several key features: * Self-contained, with no need for a DBMS or cloud service. * OpenAPI interface, easy to integrate with existing infrastructure (e.g Cloud IDE). * Supports consumer-grade GPUs.
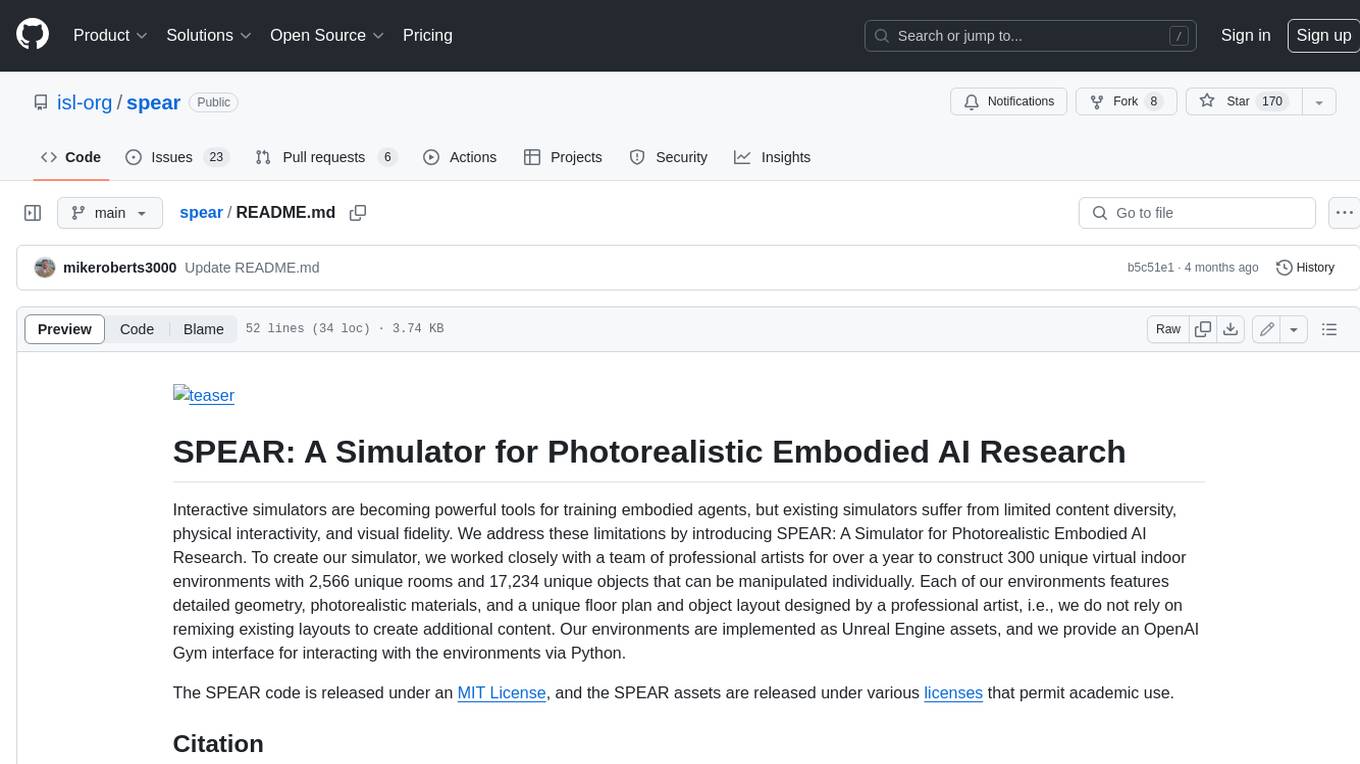
spear
SPEAR (Simulator for Photorealistic Embodied AI Research) is a powerful tool for training embodied agents. It features 300 unique virtual indoor environments with 2,566 unique rooms and 17,234 unique objects that can be manipulated individually. Each environment is designed by a professional artist and features detailed geometry, photorealistic materials, and a unique floor plan and object layout. SPEAR is implemented as Unreal Engine assets and provides an OpenAI Gym interface for interacting with the environments via Python.
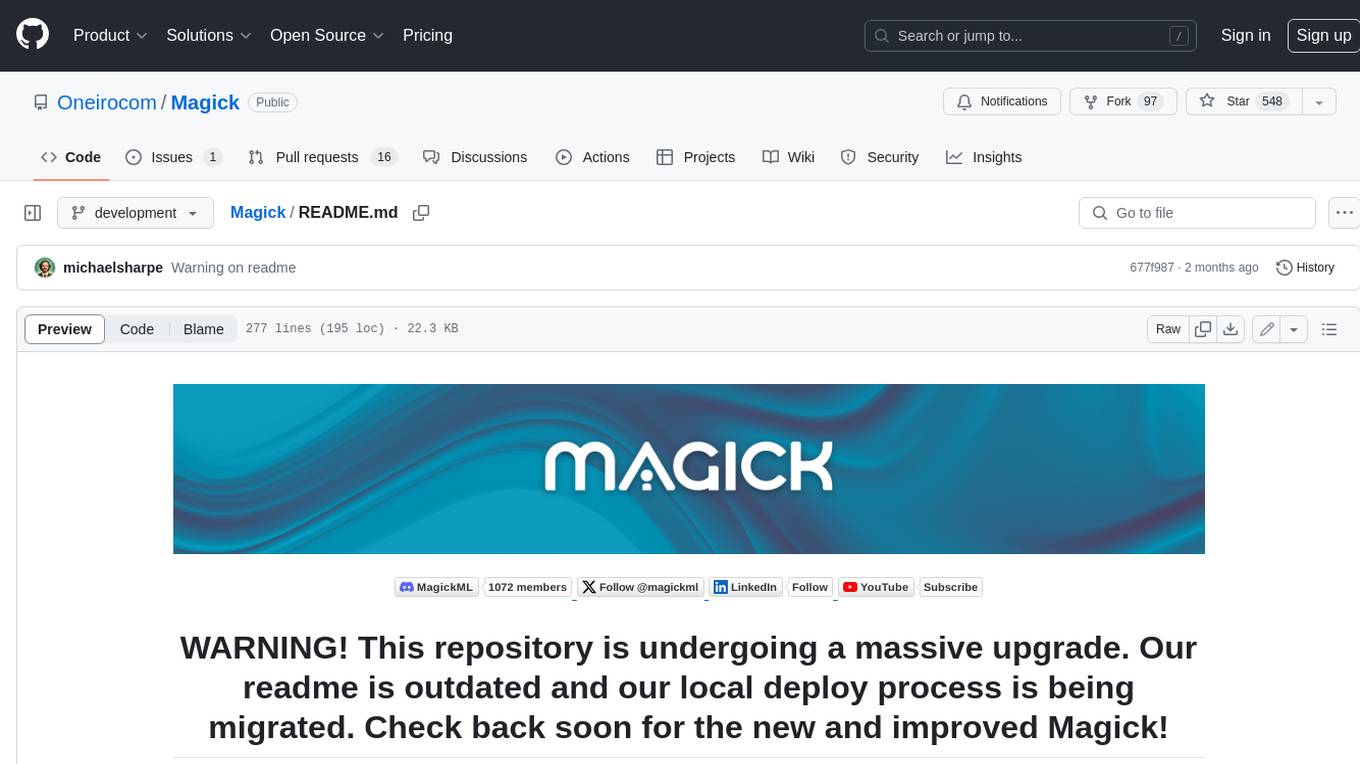
Magick
Magick is a groundbreaking visual AIDE (Artificial Intelligence Development Environment) for no-code data pipelines and multimodal agents. Magick can connect to other services and comes with nodes and templates well-suited for intelligent agents, chatbots, complex reasoning systems and realistic characters.