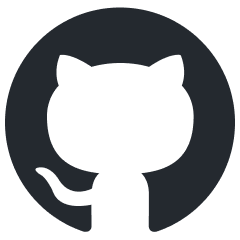
curator
Synthetic data curation for post-training and structured data extraction
Stars: 1158
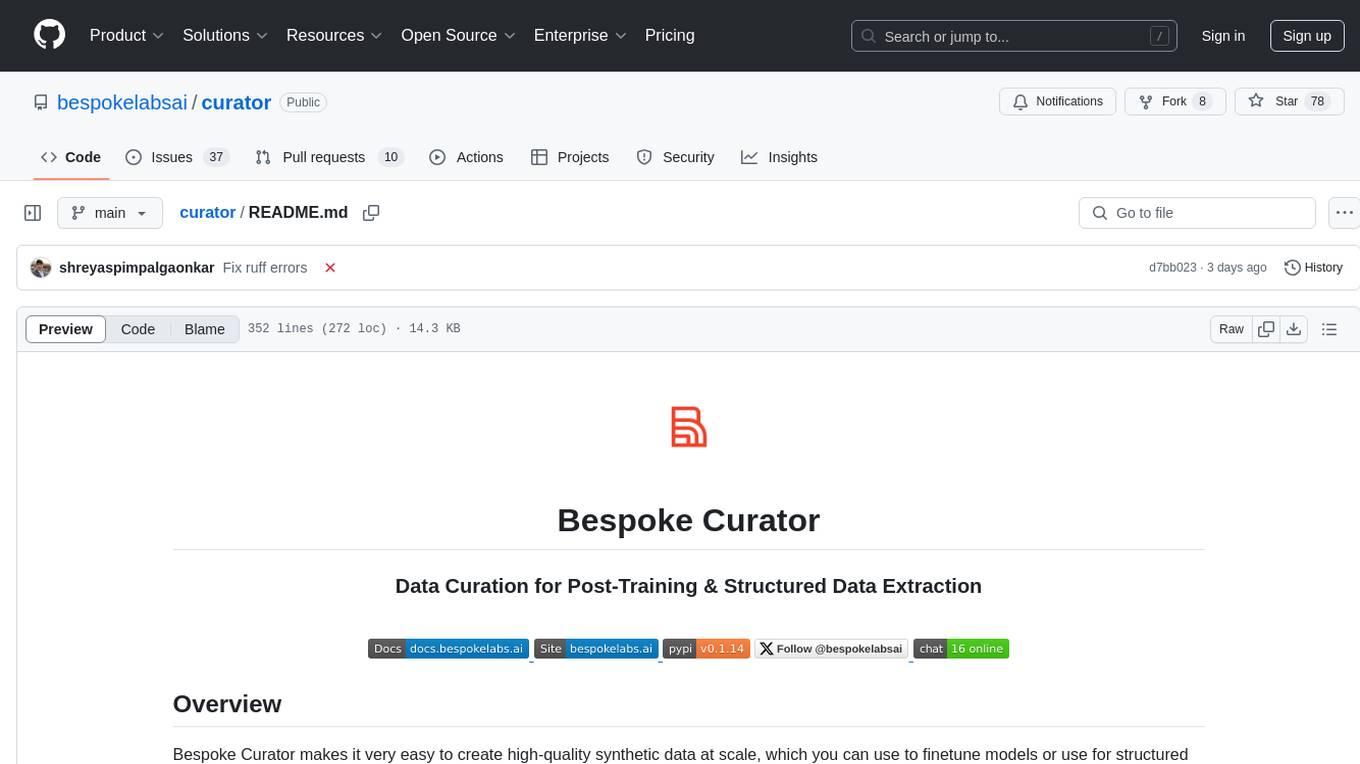
Bespoke Curator is an open-source tool for data curation and structured data extraction. It provides a Python library for generating synthetic data at scale, with features like programmability, performance optimization, caching, and integration with HuggingFace Datasets. The tool includes a Curator Viewer for dataset visualization and offers a rich set of functionalities for creating and refining data generation strategies.
README:
- [2025.03.12] Gemini Batch support added: Gemini batch API is extremely challenging, and we made it much simpler! :)
- [2025.03.05] Claude 3.7 Sonnet Thinking and batch mode support added.
- [2025.02.26] Code Execution Support added: You can now run code (generated by Curator) using CodeExecutor. We support four backends: local (called multiprocessing), Ray, Docker and e2b.
- [2025.02.06] We used Bespoke Curator to create s1K-1.1, a high-quality sample-efficient reasoning dataset.
- [2025.01.30] Batch Processing Support for OpenAI, Anthropic, and other compatible APIs: Cut Token Costs in Half π₯π₯π₯. Through our partnership with kluster.ai, new users using Curator can access open-source models like DeepSeek-R1 and receive a $25 credit (limits apply). EDIT: Promotion has come to an end.
- [2025.01.27] We used Bespoke Curator to create OpenThoughts-114k, a high-quality reasoning dataset (trending on HuggingFace).
- [2025.01.22] We used Bespoke Curator to create Bespoke-Stratos-17k, a high-quality reasoning dataset (trending on HuggingFace).
- [2025.01.15] Curator launched π
Bespoke Curator makes it easy to create synthetic data pipelines. Whether you are training a model or extracting structured data, Curator will prepare high-quality data quickly and robustly.
- Rich Python based library for generating and curating synthetic data.
- Interactive viewer to monitor data while it is being generated.
- First class support for structured outputs.
- Built-in performance optimizations for asynchronous operations, caching, and fault recovery at every scale.
- Support for a wide range of inference options via LiteLLM, vLLM, and popular batch APIs.
Check out our full documentation for getting started, tutorials, guides and detailed reference.
pip install bespokelabs-curator
Task | Link(s) | Goal |
---|---|---|
Product feature extraction | Finetuning a model to identify features of a product | |
Sentiment analysis | Aspect-based sentiment analysis of restaurant reviews and finetuning using Together.ai | |
RAFT for domain-specific RAG | Code | Implement Retrieval Augmented Fine-Tuning (RAFT) that processes domain-specific documents, generates questions, and prepares data for fine-tuning LLMs. |
Task | Link(s) | Goal |
---|---|---|
Reasoning dataset generation (Bespoke Stratos) | Code | Generate the Bespoke-Stratos-17k dataset, focusing on reasoning traces from math, coding, and problem-solving datasets. |
Reasoning dataset generation (Open Thoughts) | Code | Generate the Open-Thoughts-114k dataset, focusing on reasoning traces from math, coding, and problem-solving datasets. |
Multimodal | Code | Demonstrates multimodal capabilities by generating recipes from food images |
Ungrounded Question Answer generation | Code | Generate diverse question-answer pairs using techniques similar to the CAMEL paper |
Code Execution | Execute code generated with Curator | |
3Blue1Brown video generation | Code | Generate videos similar to 3Blue1Brown and render them using code execution! |
Synthetic charts | Code | Generate charts synthetically. |
Function calling | Code | Generate data for finetuning for function calling. |
from bespokelabs import curator
llm = curator.LLM(model_name="gpt-4o-mini")
poem = llm("Write a poem about the importance of data in AI.")
print(poem.to_pandas())
[!NOTE] Retries and caching are enabled by default to help you rapidly iterate your data pipelines. So now if you run the same prompt again, you will get the same response, pretty much instantly. You can delete the cache at
~/.cache/curator
or disable it withexport CURATOR_DISABLE_CACHE=true
.
[!IMPORTANT] Make sure to set your API keys as environment variables for the model you are calling. For example running
export OPENAI_API_KEY=sk-...
andexport ANTHROPIC_API_KEY=ant-...
will allow you to run the previous two examples. A full list of supported models and their associated environment variable names can be found in the litellm docs.
You can also send a list of prompts to the LLM, or a HuggingFace Dataset object (see below for more details).
Here's an example of using structured outputs and custom prompting and parsing logic.
from typing import Dict
from pydantic import BaseModel, Field
from bespokelabs import curator
from datasets import Dataset
class Poem(BaseModel):
title: str = Field(description="The title of the poem.")
poem: str = Field(description="The content of the poem.")
class Poet(curator.LLM):
response_format = Poem
def prompt(self, input: Dict) -> str:
return f"Write two poems about {input['topic']}."
def parse(self, input: Dict, response: Poem) -> Dict:
return [{"title": response.title, "poem": response.poem}]
poet = Poet(model_name="gpt-4o-mini")
topics = Dataset.from_dict({'topic': ['Dreams of a Robot']})
poems = poet(topics)
print(poems.to_pandas())
Output:
title poem
0 Dreams of a Robot: Awakening In circuits deep, where silence hums, \nA dre..
1 Life of an AI Agent - Poem 1 In circuits woven, thoughts ignite,\nI dwell i...
In the Poet
class:
-
response_format
is the structured output class we defined above. -
prompt
takes the input (input
) and returns the prompt for the LLM. -
parse
takes the input (input
) and the structured output (response
) and converts it to a list of dictionaries. This is so that we can easily convert the output to a HuggingFace Dataset object.
Note that topics
can be created with another LLM
class as well,
and we can scale this up to create tens of thousands of diverse poems.
class Topics(BaseModel):
topics_list: List[str] = Field(description="A list of topics.")
class TopicGenerator(curator.LLM):
response_format = Topics
def prompt(self, subject):
return f"Return 3 topics related to {subject}"
def parse(self, input: str, response: Topics):
return [{"topic": t} for t in response.topics_list]
topic_generator = TopicGenerator(model_name="gpt-4o-mini")
topics = topic_generator("Mathematics")
poems = poet(topics)
Output:
title poem
0 The Language of Algebra In symbols and signs, truths intertwine,..
1 The Geometry of Space In the world around us, shapes do collide,..
2 The Language of Logic In circuits and wires where silence speaks,..
You can see more examples in the examples directory.
See the docs for more details as well as for troubleshooting information.
[!TIP] If you are generating large datasets, you may want to use batch mode to save costs. Currently batch APIs from OpenAI and Anthropic are supported. With curator this is as simple as setting
batch=True
in theLLM
class.
We collect minimal, anonymized usage telemetry to help prioritize new features and improvements that benefit the Curator community. You can opt out by setting the TELEMETRY_ENABLED
environment variable to False
.
Curator supports a wide range of providers, including OpenAI, Anthropic, and many more.
llm = curator.LLM(
model_name="gpt-4o-mini",
)
For other models that support OpenAI-compatible APIs, you can use the openai
backend:
llm = curator.LLM(
model_name="gpt-4o-mini",
backend="openai",
backend_params={
"base_url": "https://your-openai-compatible-api-url",
"api_key": <YOUR_OPENAI_COMPATIBLE_SERVICE_API_KEY>,
},
)
Here is an example of using Gemini with litellm backend:
llm = curator.LLM(
model_name="gemini/gemini-1.5-flash",
backend="litellm",
backend_params={
"max_requests_per_minute": 2_000,
"max_tokens_per_minute": 4_000_000
},
)
llm = curator.LLM(
model_name="ollama/llama3.1:8b", # Ollama model identifier
backend_params={"base_url": "http://localhost:11434"},
)
llm = curator.LLM(
model_name="Qwen/Qwen2.5-3B-Instruct",
backend="vllm",
backend_params={
"tensor_parallel_size": 1, # Adjust based on GPU count
"gpu_memory_utilization": 0.7
}
)
DeepSeek offers an OpenAI-compatible API that you can use with the openai
backend.
[!IMPORTANT] The DeepSeek API is experiencing intermittent issues and will return empty responses during times of high traffic. We recommend calling the DeepSeek API through the
openai
backend, with a high max retries so that we can retry failed requests upon empty response and a reasonable max requests and tokens per minute so we don't retry too aggressively and overwhelm the API.
llm = curator.LLM(
model_name="deepseek-reasoner",
generation_params={"temp": 0.0},
backend_params={
"max_requests_per_minute": 100,
"max_tokens_per_minute": 10_000_000,
"base_url": "https://api.deepseek.com/",
"api_key": <YOUR_DEEPSEEK_API_KEY>,
"max_retries": 50,
},
backend="openai",
)
llm = curator.LLM(
model_name="deepseek-ai/DeepSeek-R1",
backend="klusterai",
)
Several providers offer about 50% discount on token usage when using batch mode. Curator makes it easy to use batch mode with a wide range of providers.
Example with OpenAI (docs reference):
llm = curator.LLM(model_name="gpt-4o-mini", batch=True)
See documentation:
The hosted curator viewer is a rich interface to visualize data -- and makes visually inspecting the data much easier.
You can enable it as follows:
Bash:
export CURATOR_VIEWER=1
Python/colab:
import os
os.environ["CURATOR_VIEWER"]="1"
With this enabled, as curator generates data, it gets uploaded and you can see the responses streaming in the viewer. The URL for the viewer is displayed right next to the rich progress.
We support a range of environment variables to customize the behavior of Curator.
Here is a complete table of environment variables:
Variable | Description | Default |
---|---|---|
CURATOR_VIEWER |
Enables the Curator viewer for visualizing data curation when True . |
False |
CURATOR_DISABLE_CACHE |
Disables caching for curator.LLM generations when True . Useful for fresh runs. |
False |
CURATOR_CACHE_DIR |
Sets the cache directory used for curator.LLM generations. |
~/.cache/curator |
CURATOR_DISABLE_RICH_DISPLAY |
When True , disables Rich CLI output (and falls back to tqdm logging) for local data generation monitoring. This is useful when debugging with inline breakpoints or interactive debuggers like pdb , where Rich's dynamic output can interfere with terminal input. |
False |
TELEMETRY_ENABLED |
Enable telemetry for curator usage tracking when True
|
True |
Thank you to all the contributors for making this project possible! Please follow these instructions on how to contribute.
If you find Curator useful, please consider citing us!
@software{Curator: A Tool for Synthetic Data Creation,
author = {Marten, Ryan* and Vu, Trung* and Ji, Charlie Cheng-Jie and Sharma, Kartik and Pimpalgaonkar, Shreyas and Dimakis, Alex and Sathiamoorthy, Maheswaran},
month = jan,
title = {{Curator}},
year = {2025},
howpublished = {\url{https://github.com/bespokelabsai/curator}}
}
For Tasks:
Click tags to check more tools for each tasksFor Jobs:
Alternative AI tools for curator
Similar Open Source Tools
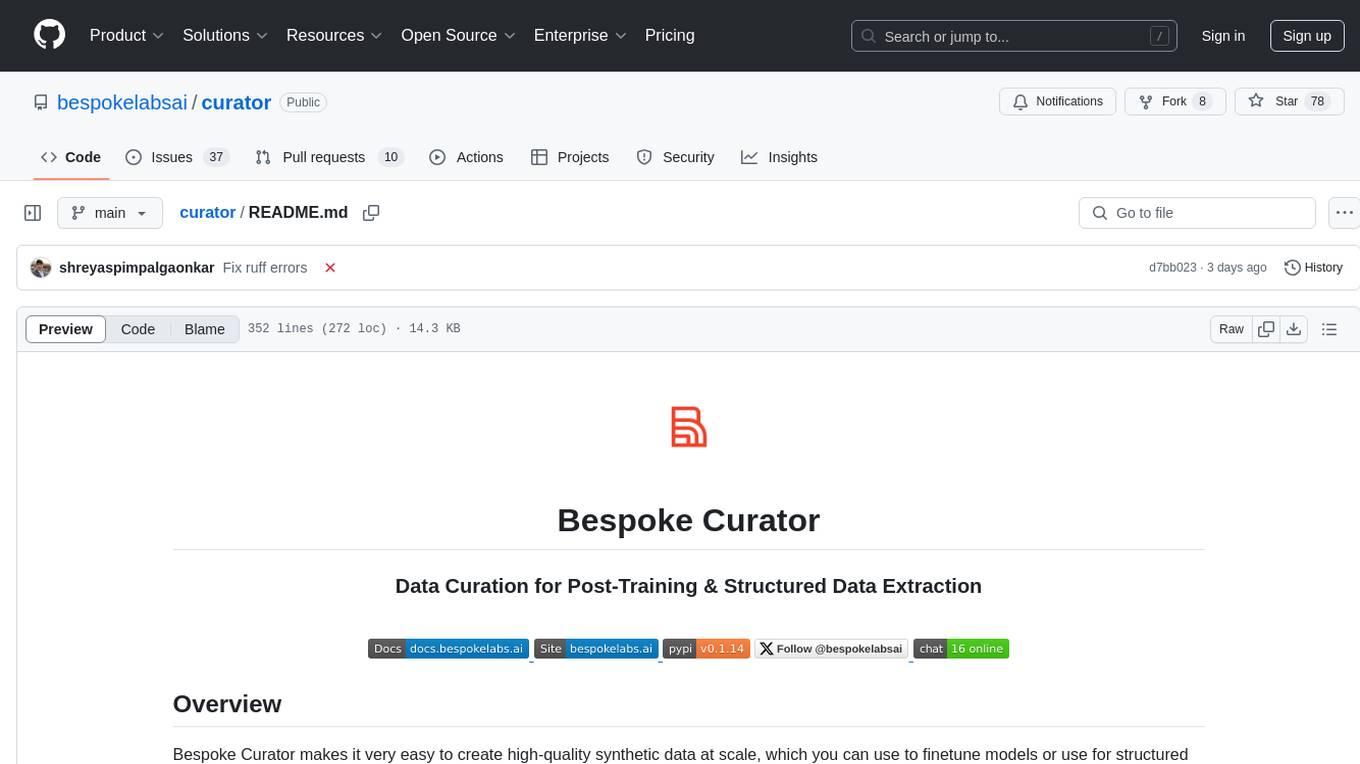
curator
Bespoke Curator is an open-source tool for data curation and structured data extraction. It provides a Python library for generating synthetic data at scale, with features like programmability, performance optimization, caching, and integration with HuggingFace Datasets. The tool includes a Curator Viewer for dataset visualization and offers a rich set of functionalities for creating and refining data generation strategies.
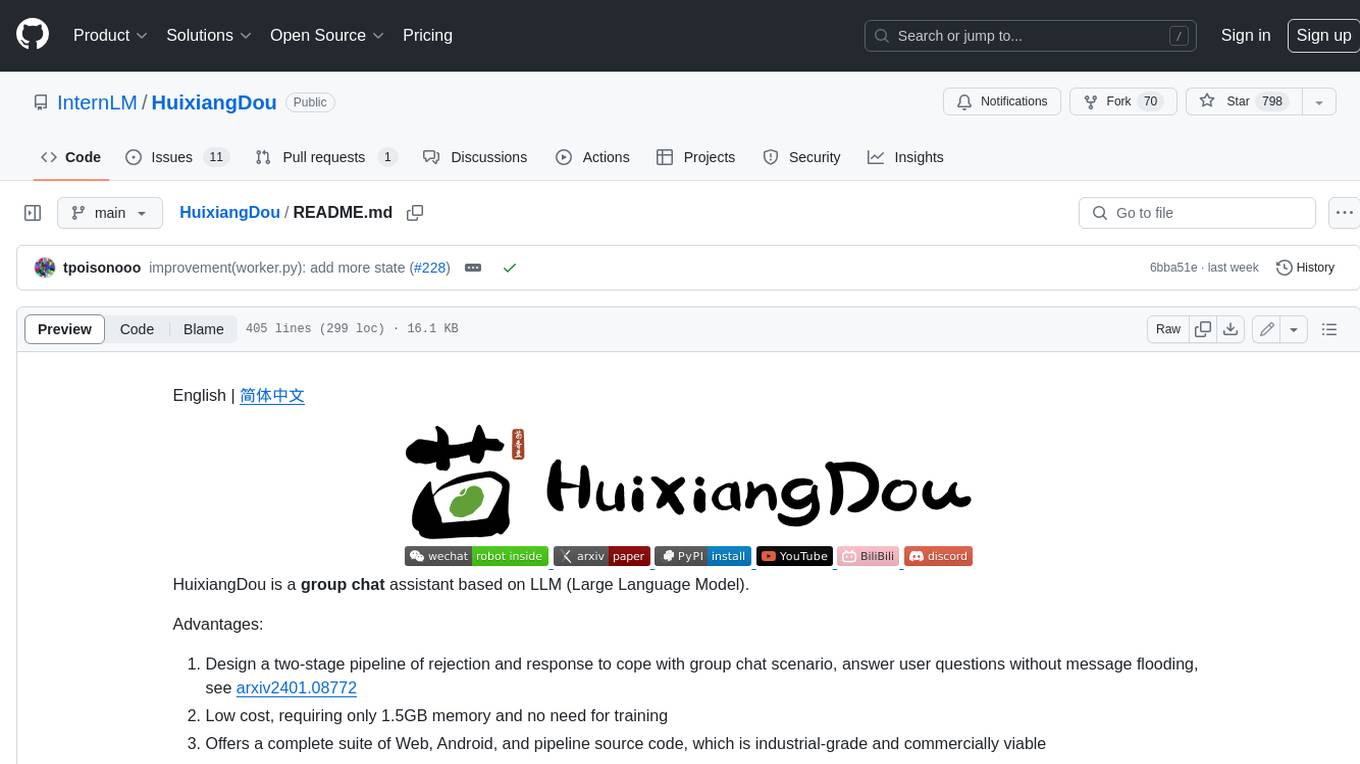
HuixiangDou
HuixiangDou is a **group chat** assistant based on LLM (Large Language Model). Advantages: 1. Design a two-stage pipeline of rejection and response to cope with group chat scenario, answer user questions without message flooding, see arxiv2401.08772 2. Low cost, requiring only 1.5GB memory and no need for training 3. Offers a complete suite of Web, Android, and pipeline source code, which is industrial-grade and commercially viable Check out the scenes in which HuixiangDou are running and join WeChat Group to try AI assistant inside. If this helps you, please give it a star β
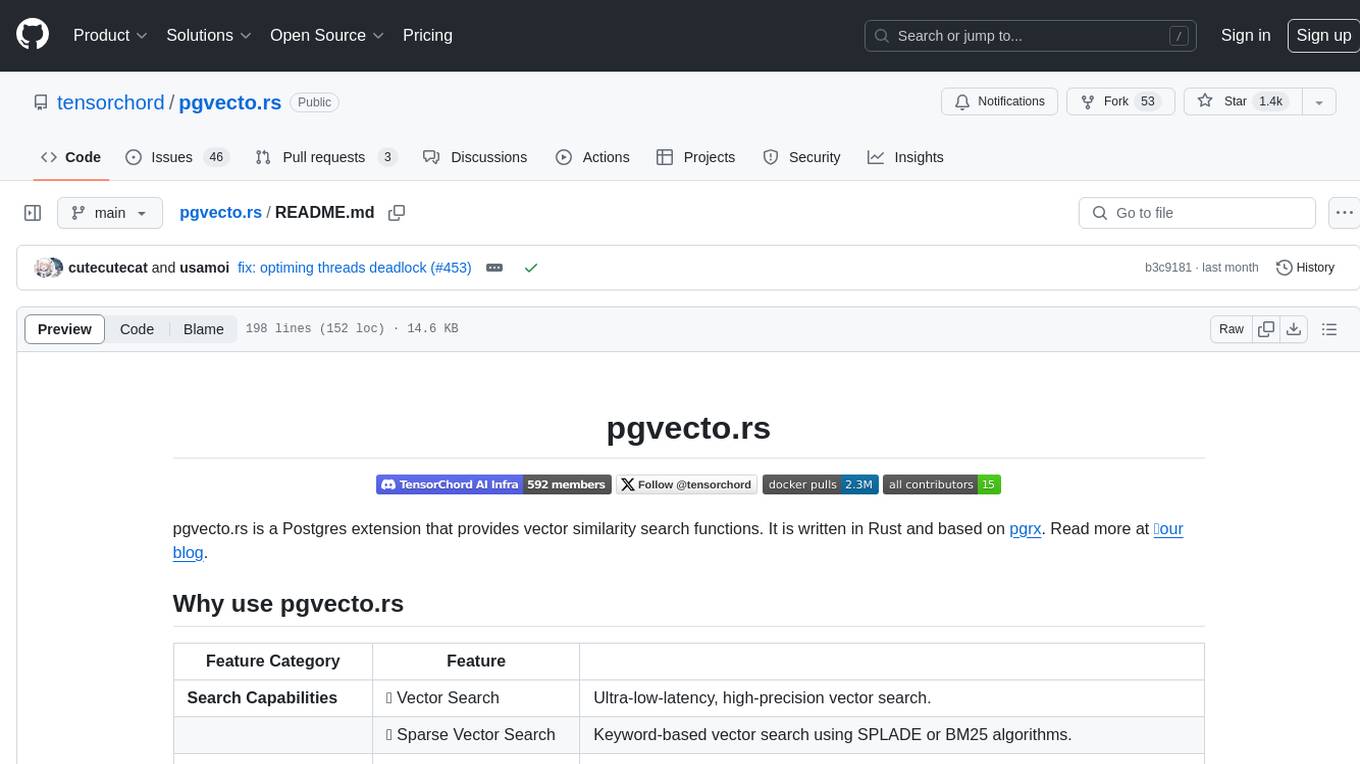
pgvecto.rs
pgvecto.rs is a Postgres extension written in Rust that provides vector similarity search functions. It offers ultra-low-latency, high-precision vector search capabilities, including sparse vector search and full-text search. With complete SQL support, async indexing, and easy data management, it simplifies data handling. The extension supports various data types like FP16/INT8, binary vectors, and Matryoshka embeddings. It ensures system performance with production-ready features, high availability, and resource efficiency. Security and permissions are managed through easy access control. The tool allows users to create tables with vector columns, insert vector data, and calculate distances between vectors using different operators. It also supports half-precision floating-point numbers for better performance and memory usage optimization.
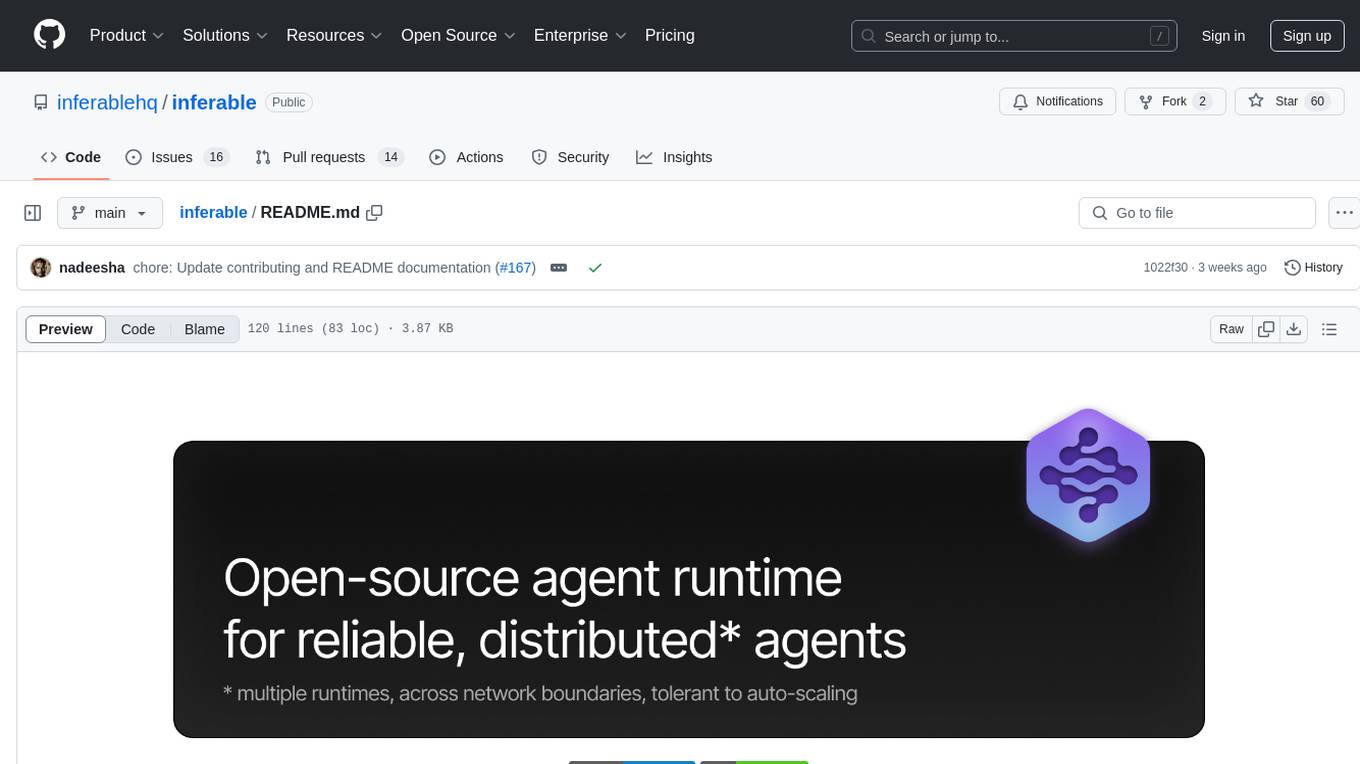
inferable
Inferable is an open source platform that helps users build reliable LLM-powered agentic automations at scale. It offers a managed agent runtime, durable tool calling, zero network configuration, multiple language support, and is fully open source under the MIT license. Users can define functions, register them with Inferable, and create runs that utilize these functions to automate tasks. The platform supports Node.js/TypeScript, Go, .NET, and React, and provides SDKs, core services, and bootstrap templates for various languages.
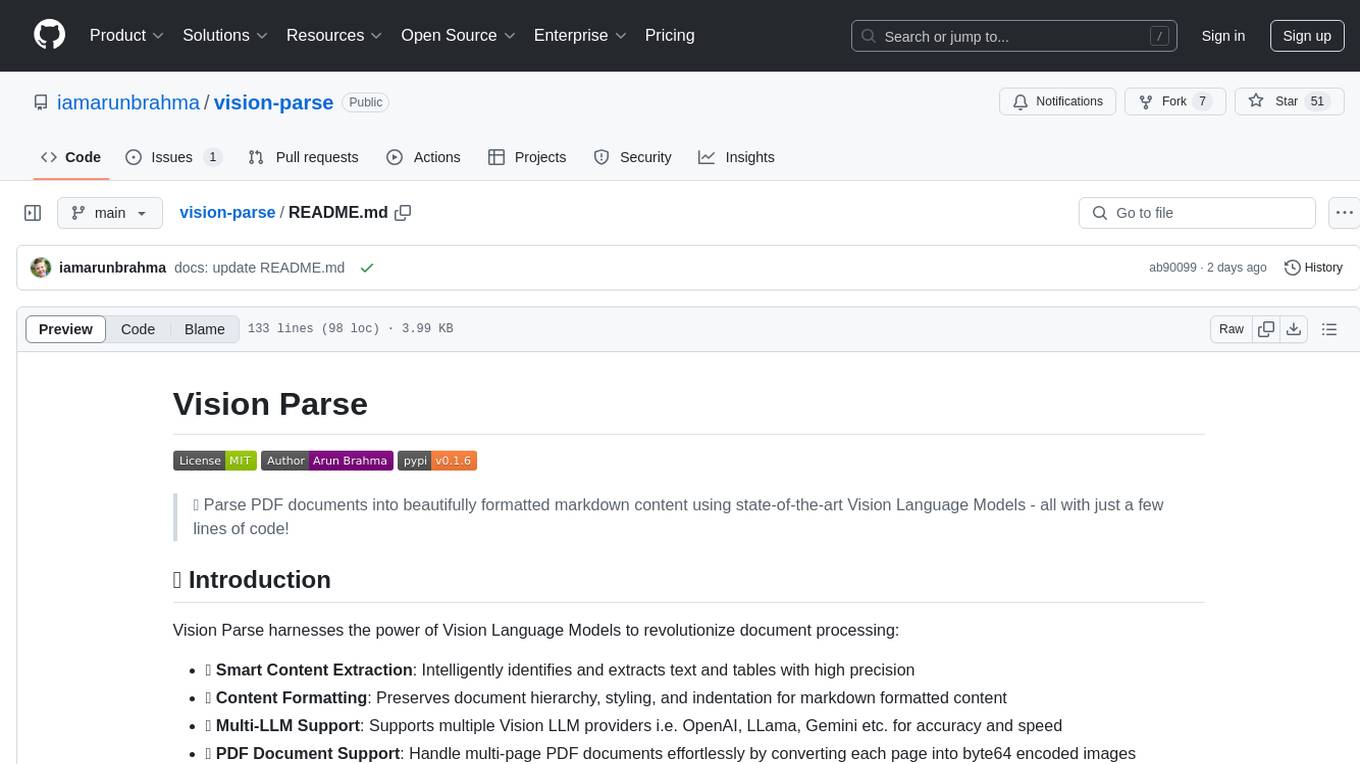
vision-parse
Vision Parse is a tool that leverages Vision Language Models to parse PDF documents into beautifully formatted markdown content. It offers smart content extraction, content formatting, multi-LLM support, PDF document support, and local model hosting using Ollama. Users can easily convert PDFs to markdown with high precision and preserve document hierarchy and styling. The tool supports multiple Vision LLM providers like OpenAI, LLama, and Gemini for accuracy and speed, making document processing efficient and effortless.
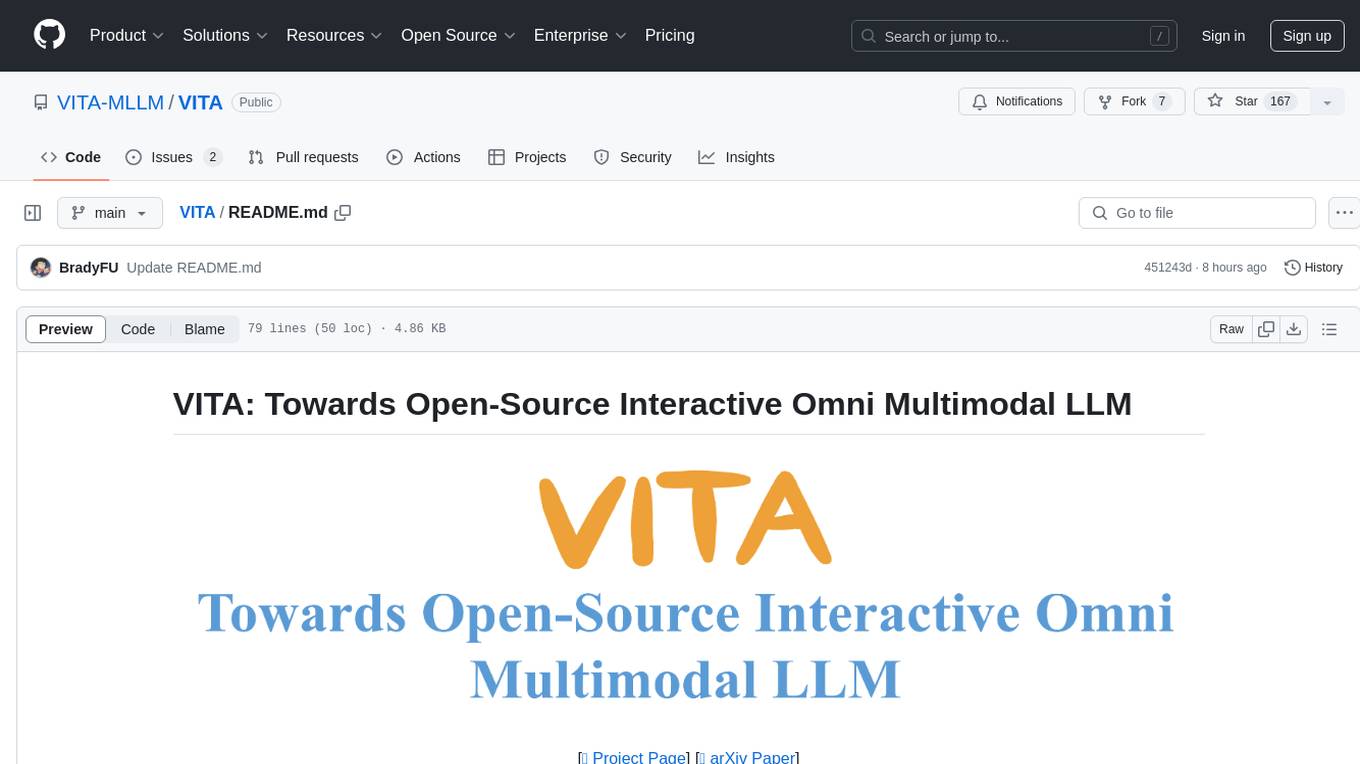
VITA
VITA is an open-source interactive omni multimodal Large Language Model (LLM) capable of processing video, image, text, and audio inputs simultaneously. It stands out with features like Omni Multimodal Understanding, Non-awakening Interaction, and Audio Interrupt Interaction. VITA can respond to user queries without a wake-up word, track and filter external queries in real-time, and handle various query inputs effectively. The model utilizes state tokens and a duplex scheme to enhance the multimodal interactive experience.
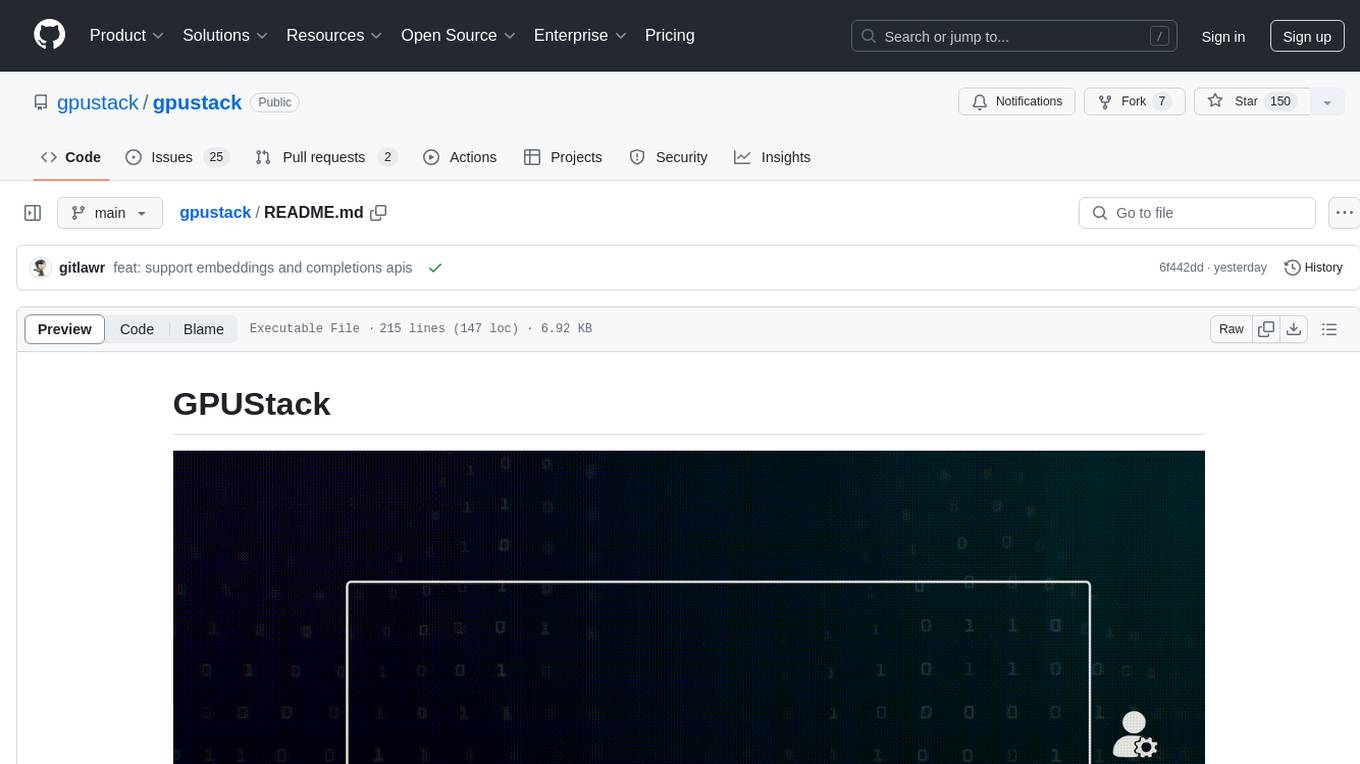
gpustack
GPUStack is an open-source GPU cluster manager designed for running large language models (LLMs). It supports a wide variety of hardware, scales with GPU inventory, offers lightweight Python package with minimal dependencies, provides OpenAI-compatible APIs, simplifies user and API key management, enables GPU metrics monitoring, and facilitates token usage and rate metrics tracking. The tool is suitable for managing GPU clusters efficiently and effectively.
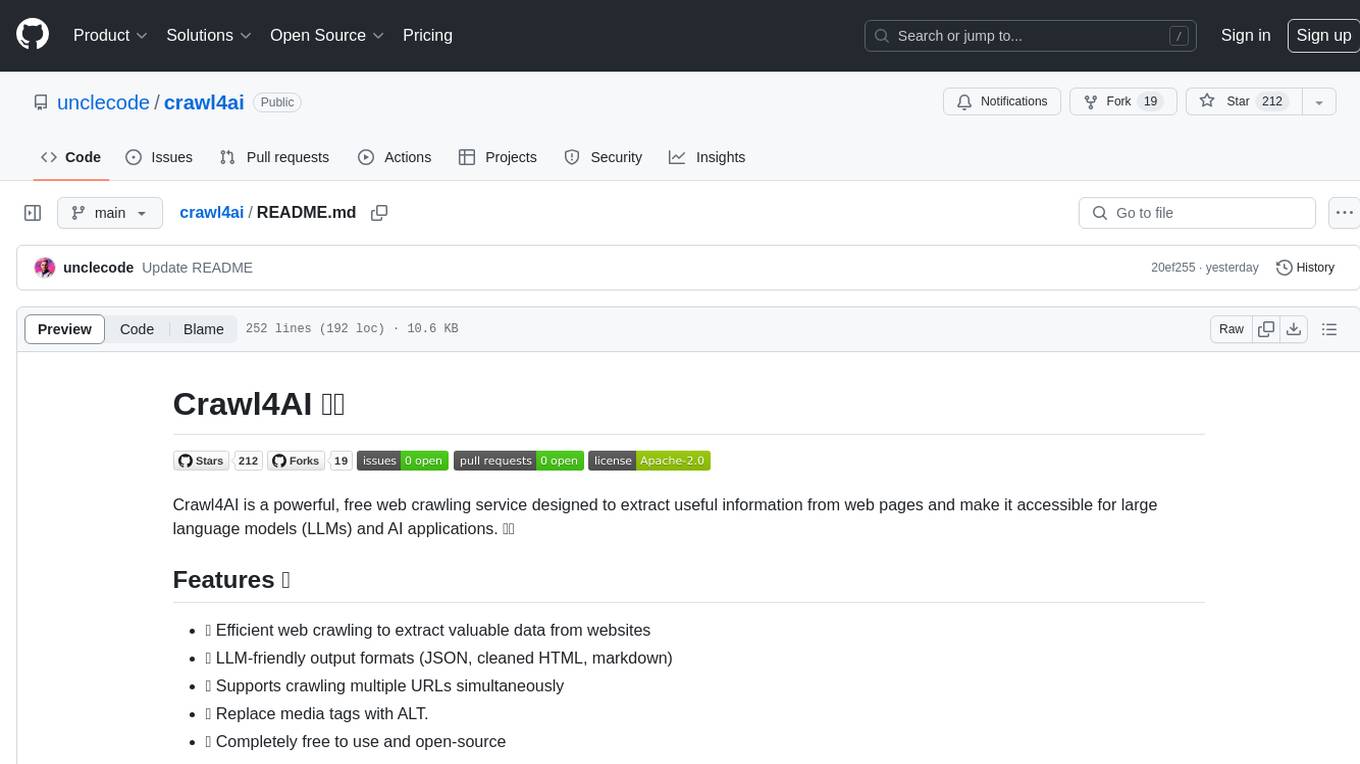
crawl4ai
Crawl4AI is a powerful and free web crawling service that extracts valuable data from websites and provides LLM-friendly output formats. It supports crawling multiple URLs simultaneously, replaces media tags with ALT, and is completely free to use and open-source. Users can integrate Crawl4AI into Python projects as a library or run it as a standalone local server. The tool allows users to crawl and extract data from specified URLs using different providers and models, with options to include raw HTML content, force fresh crawls, and extract meaningful text blocks. Configuration settings can be adjusted in the `crawler/config.py` file to customize providers, API keys, chunk processing, and word thresholds. Contributions to Crawl4AI are welcome from the open-source community to enhance its value for AI enthusiasts and developers.
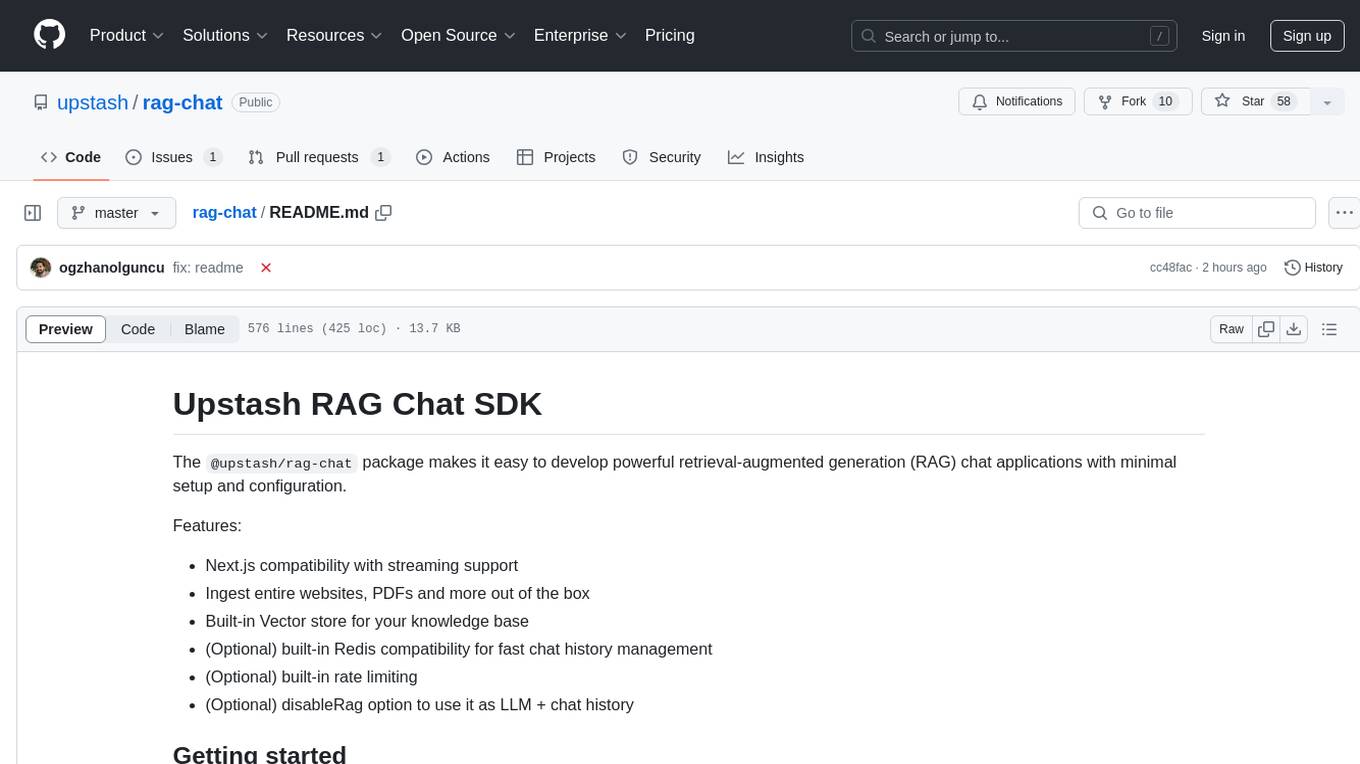
rag-chat
The `@upstash/rag-chat` package simplifies the development of retrieval-augmented generation (RAG) chat applications by providing Next.js compatibility with streaming support, built-in vector store, optional Redis compatibility for fast chat history management, rate limiting, and disableRag option. Users can easily set up the environment variables and initialize RAGChat to interact with AI models, manage knowledge base, chat history, and enable debugging features. Advanced configuration options allow customization of RAGChat instance with built-in rate limiting, observability via Helicone, and integration with Next.js route handlers and Vercel AI SDK. The package supports OpenAI models, Upstash-hosted models, and custom providers like TogetherAi and Replicate.
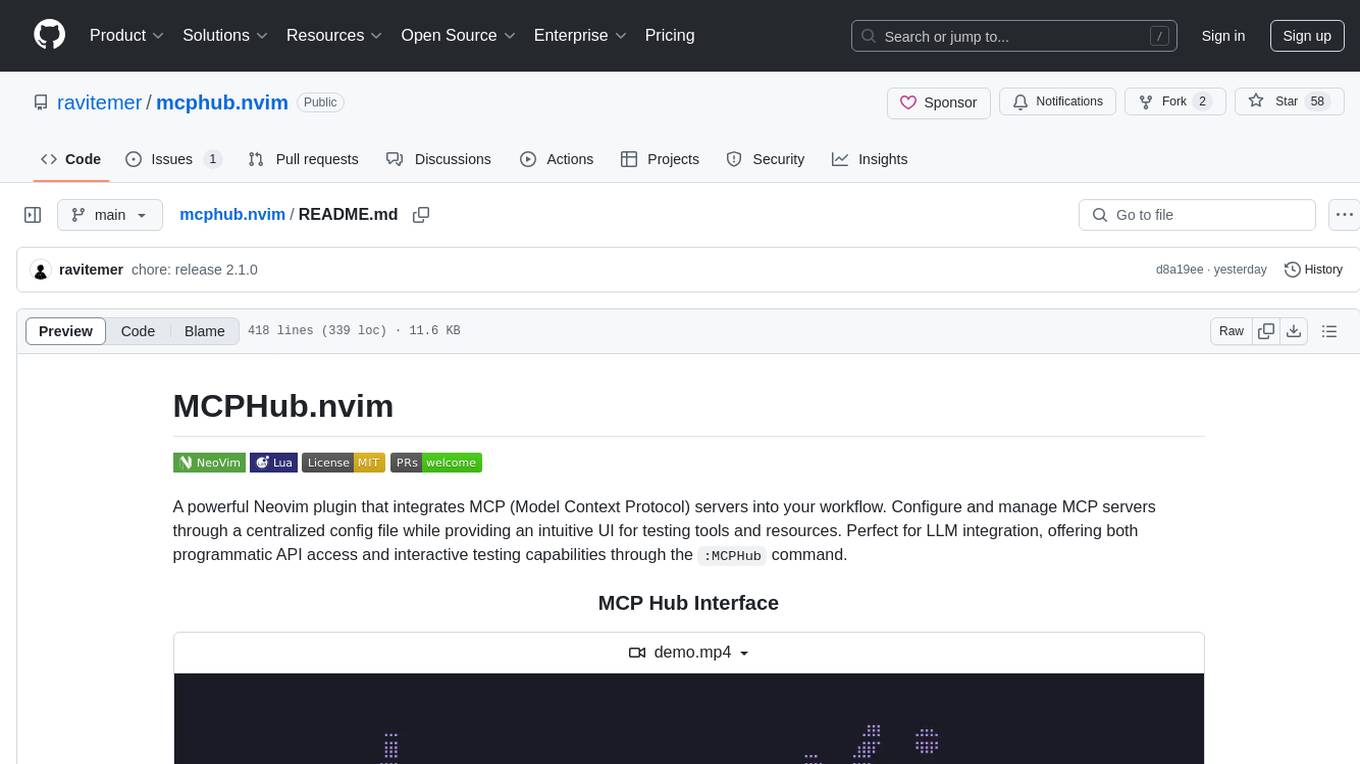
mcphub.nvim
MCPHub.nvim is a powerful Neovim plugin that integrates MCP (Model Context Protocol) servers into your workflow. It offers a centralized config file for managing servers and tools, with an intuitive UI for testing resources. Ideal for LLM integration, it provides programmatic API access and interactive testing through the `:MCPHub` command.
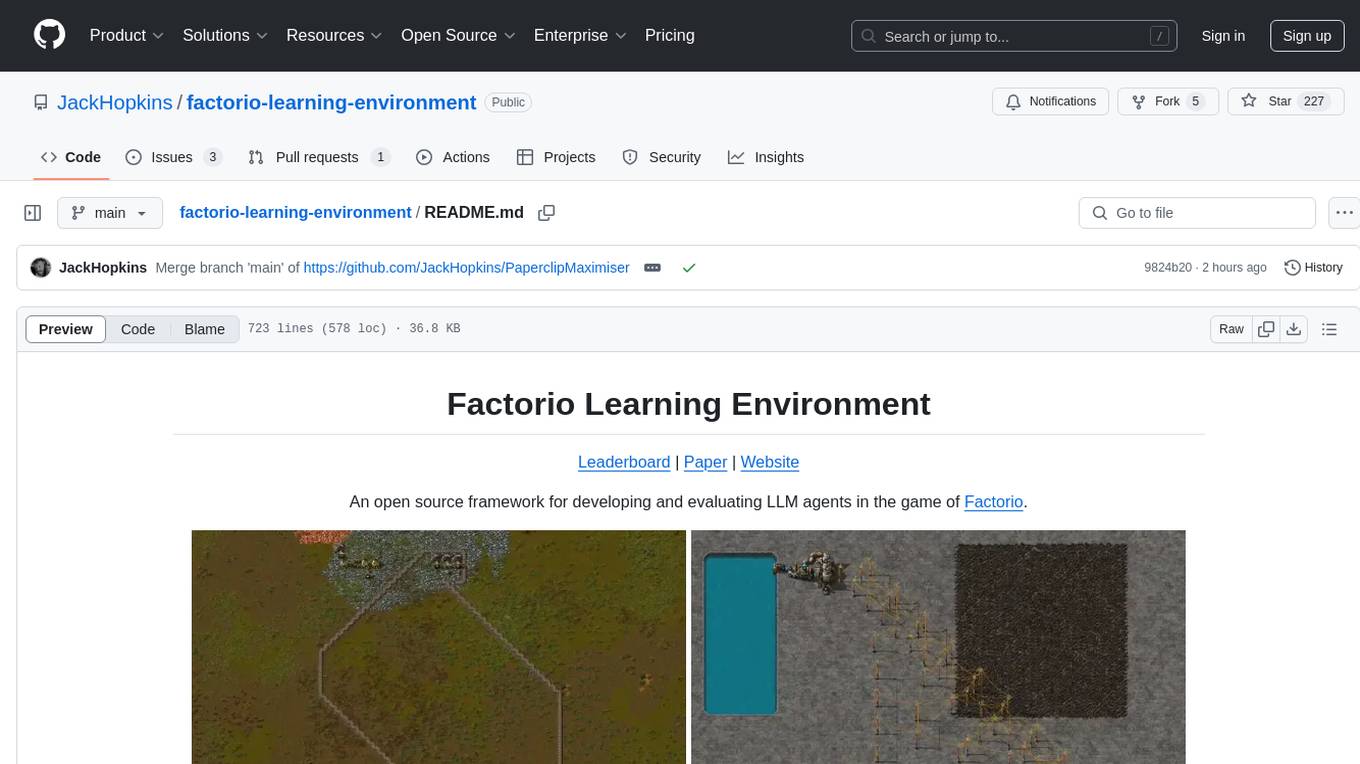
factorio-learning-environment
Factorio Learning Environment is an open source framework designed for developing and evaluating LLM agents in the game of Factorio. It provides two settings: Lab-play with structured tasks and Open-play for building large factories. Results show limitations in spatial reasoning and automation strategies. Agents interact with the environment through code synthesis, observation, action, and feedback. Tools are provided for game actions and state representation. Agents operate in episodes with observation, planning, and action execution. Tasks specify agent goals and are implemented in JSON files. The project structure includes directories for agents, environment, cluster, data, docs, eval, and more. A database is used for checkpointing agent steps. Benchmarks show performance metrics for different configurations.
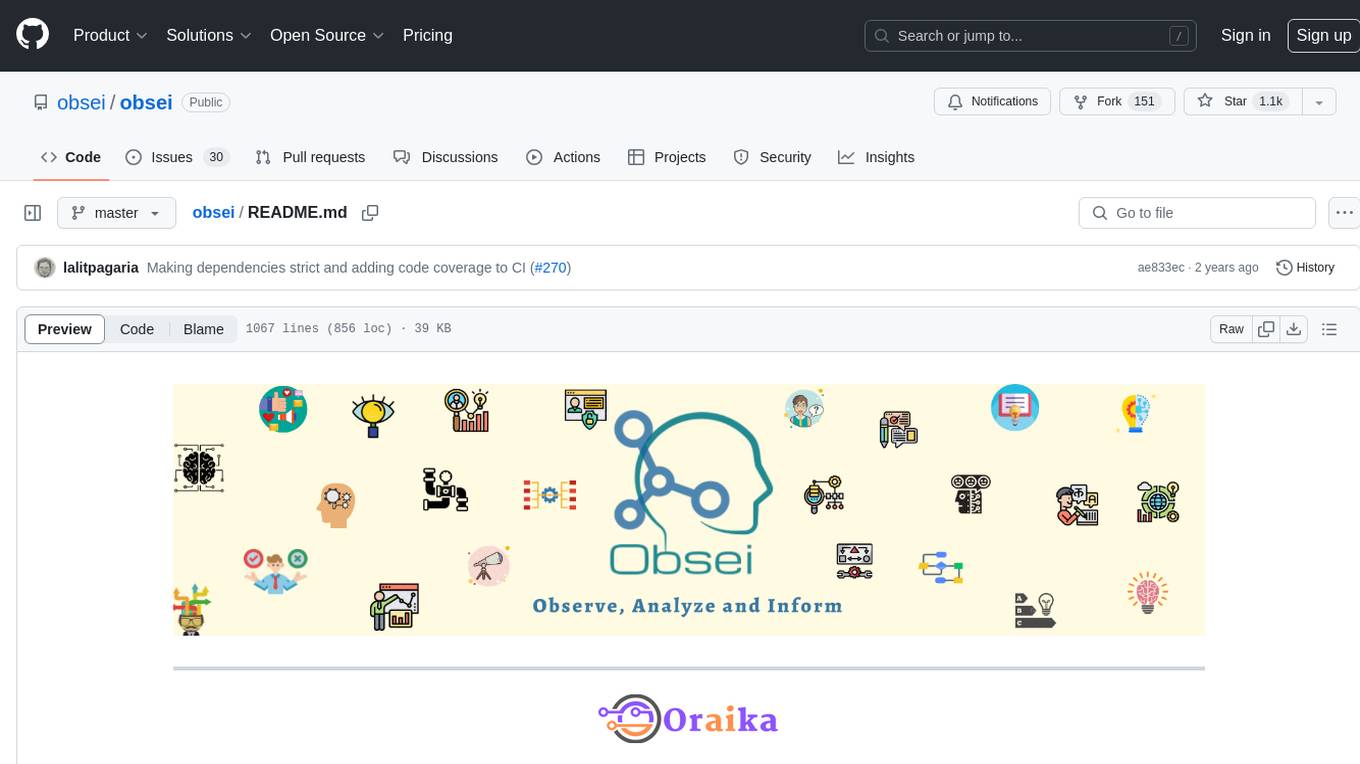
obsei
Obsei is an open-source, low-code, AI powered automation tool that consists of an Observer to collect unstructured data from various sources, an Analyzer to analyze the collected data with various AI tasks, and an Informer to send analyzed data to various destinations. The tool is suitable for scheduled jobs or serverless applications as all Observers can store their state in databases. Obsei is still in alpha stage, so caution is advised when using it in production. The tool can be used for social listening, alerting/notification, automatic customer issue creation, extraction of deeper insights from feedbacks, market research, dataset creation for various AI tasks, and more based on creativity.
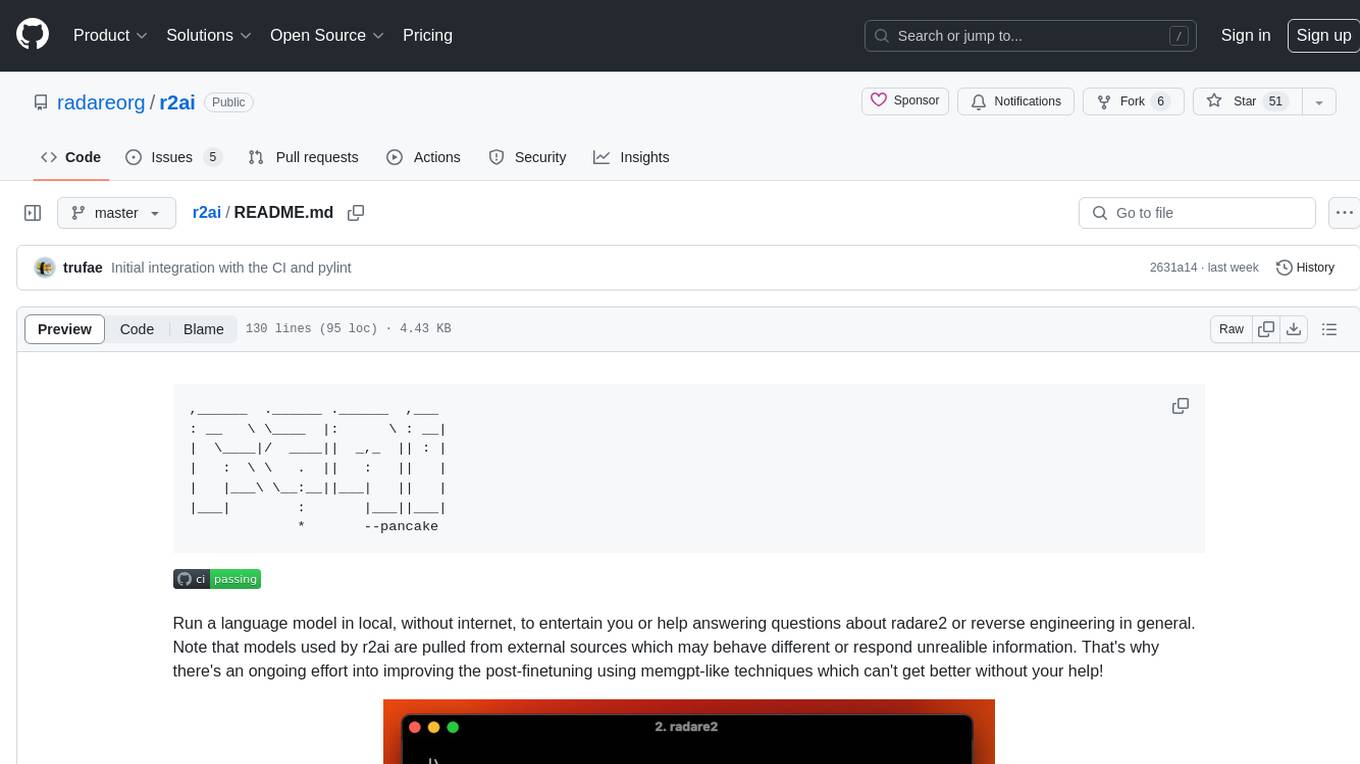
r2ai
r2ai is a tool designed to run a language model locally without internet access. It can be used to entertain users or assist in answering questions related to radare2 or reverse engineering. The tool allows users to prompt the language model, index large codebases, slurp file contents, embed the output of an r2 command, define different system-level assistant roles, set environment variables, and more. It is accessible as an r2lang-python plugin and can be scripted from various languages. Users can use different models, adjust query templates dynamically, load multiple models, and make them communicate with each other.
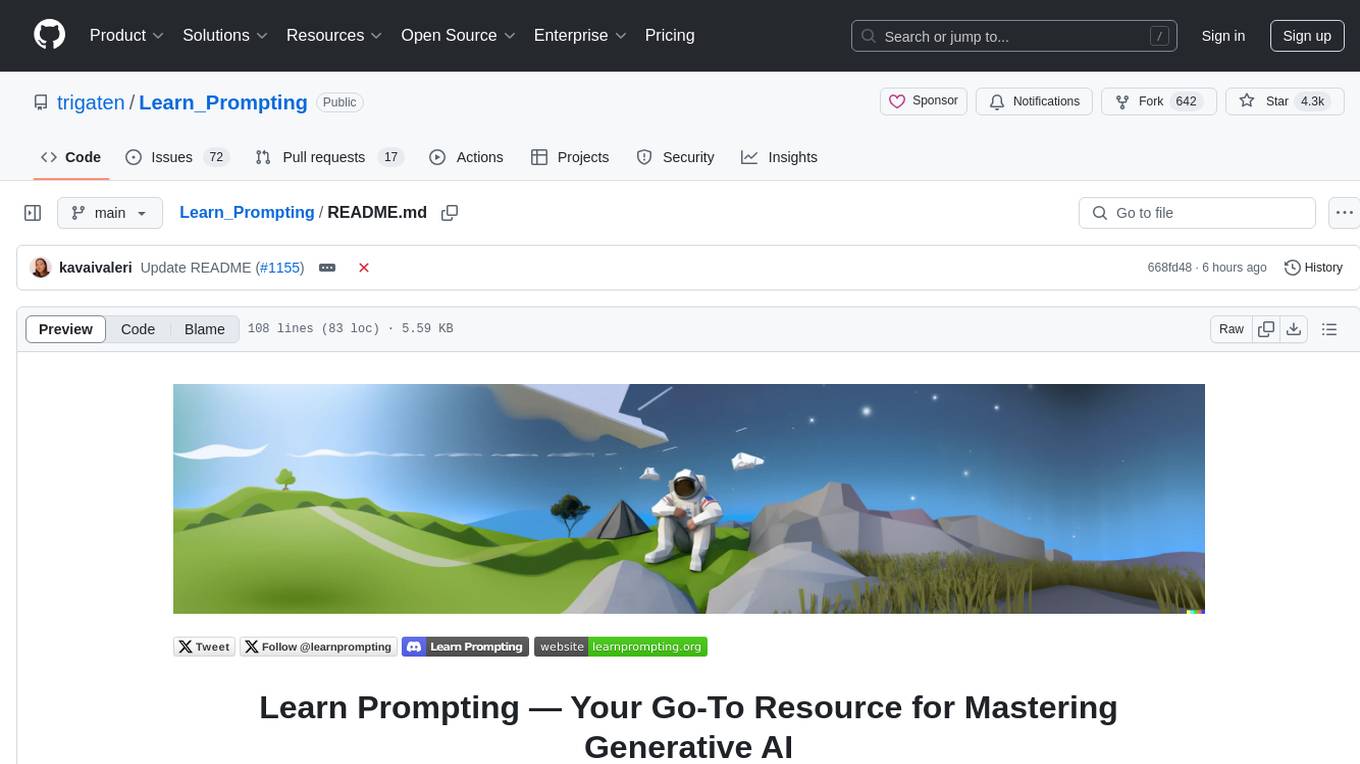
Learn_Prompting
Learn Prompting is a platform offering free resources, courses, and webinars to master prompt engineering and generative AI. It provides a Prompt Engineering Guide, courses on Generative AI, workshops, and the HackAPrompt competition. The platform also offers AI Red Teaming and AI Safety courses, research reports on prompting techniques, and welcomes contributions in various forms such as content suggestions, translations, artwork, and typo fixes. Users can locally develop the website using Visual Studio Code, Git, and Node.js, and run it in development mode to preview changes.
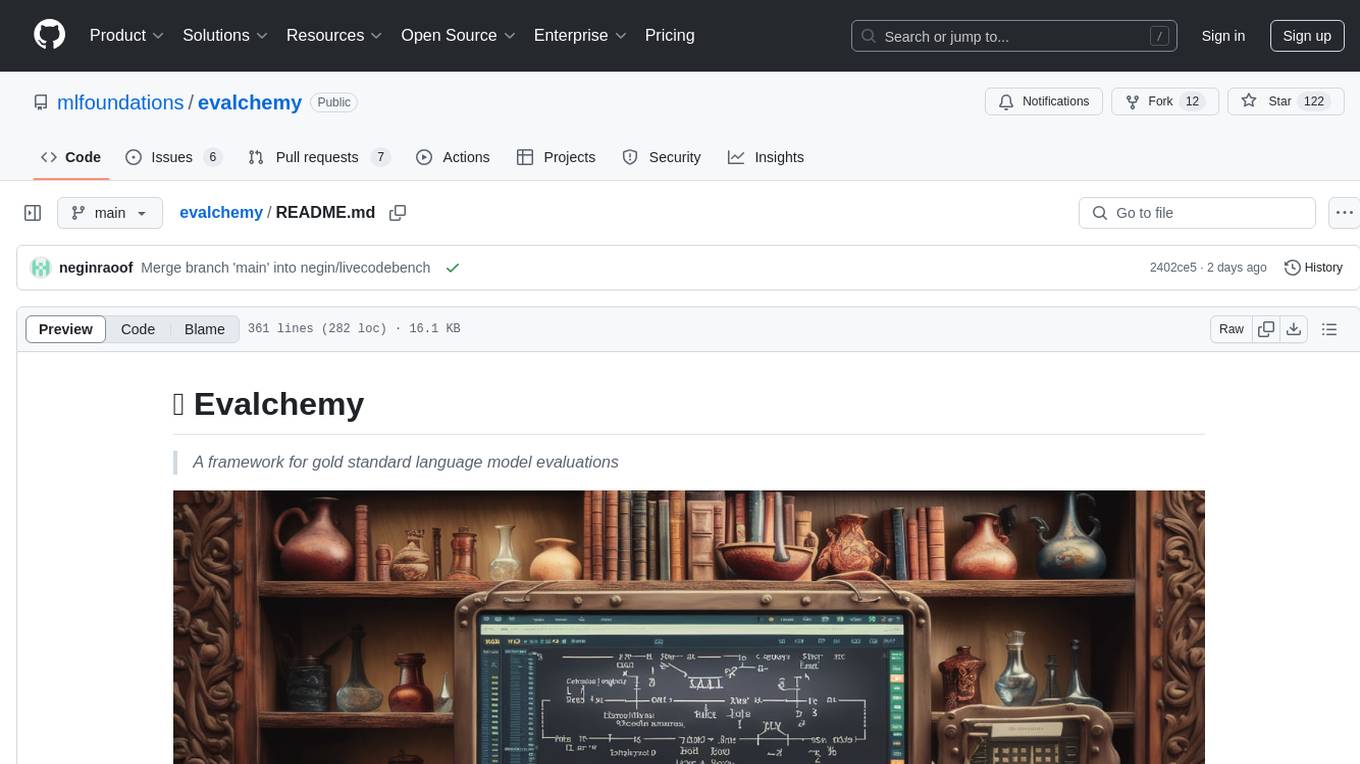
evalchemy
Evalchemy is a unified and easy-to-use toolkit for evaluating language models, focusing on post-trained models. It integrates multiple existing benchmarks such as RepoBench, AlpacaEval, and ZeroEval. Key features include unified installation, parallel evaluation, simplified usage, and results management. Users can run various benchmarks with a consistent command-line interface and track results locally or integrate with a database for systematic tracking and leaderboard submission.
For similar tasks
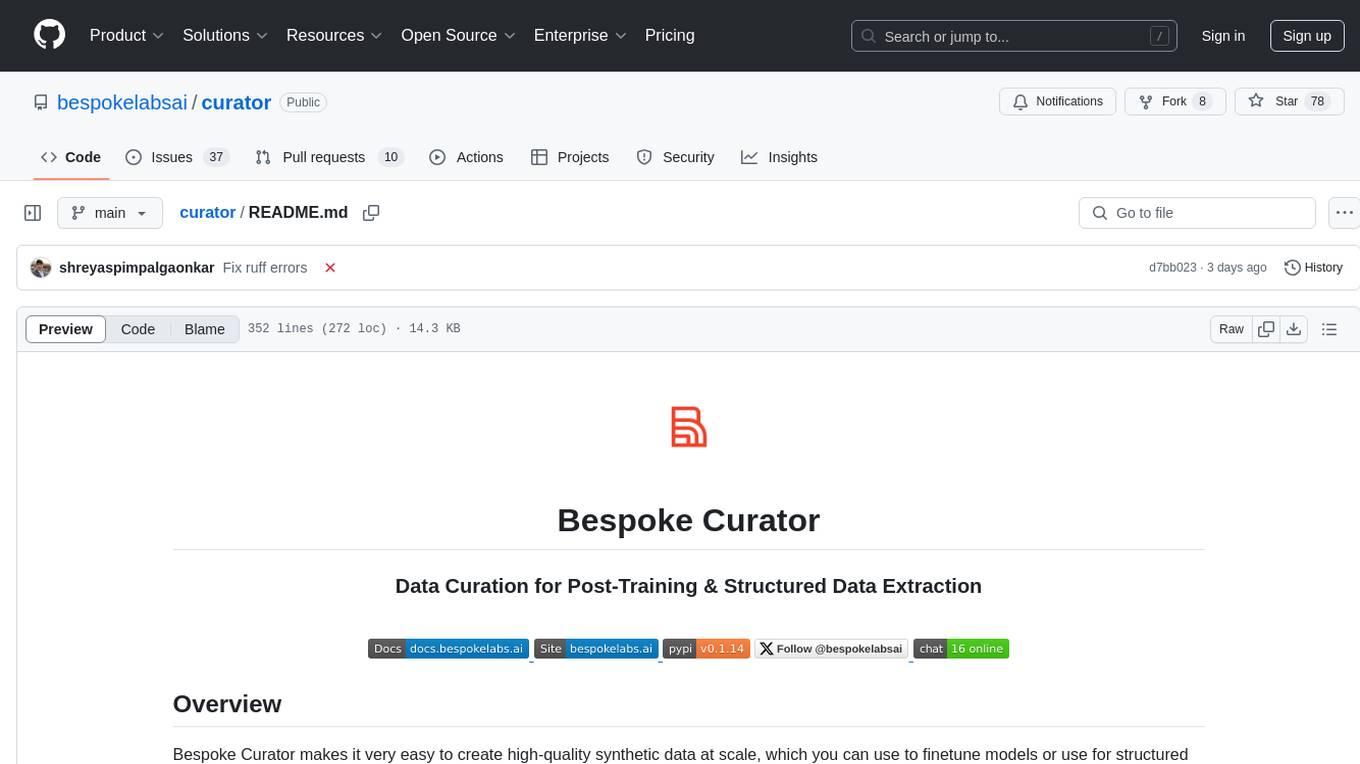
curator
Bespoke Curator is an open-source tool for data curation and structured data extraction. It provides a Python library for generating synthetic data at scale, with features like programmability, performance optimization, caching, and integration with HuggingFace Datasets. The tool includes a Curator Viewer for dataset visualization and offers a rich set of functionalities for creating and refining data generation strategies.
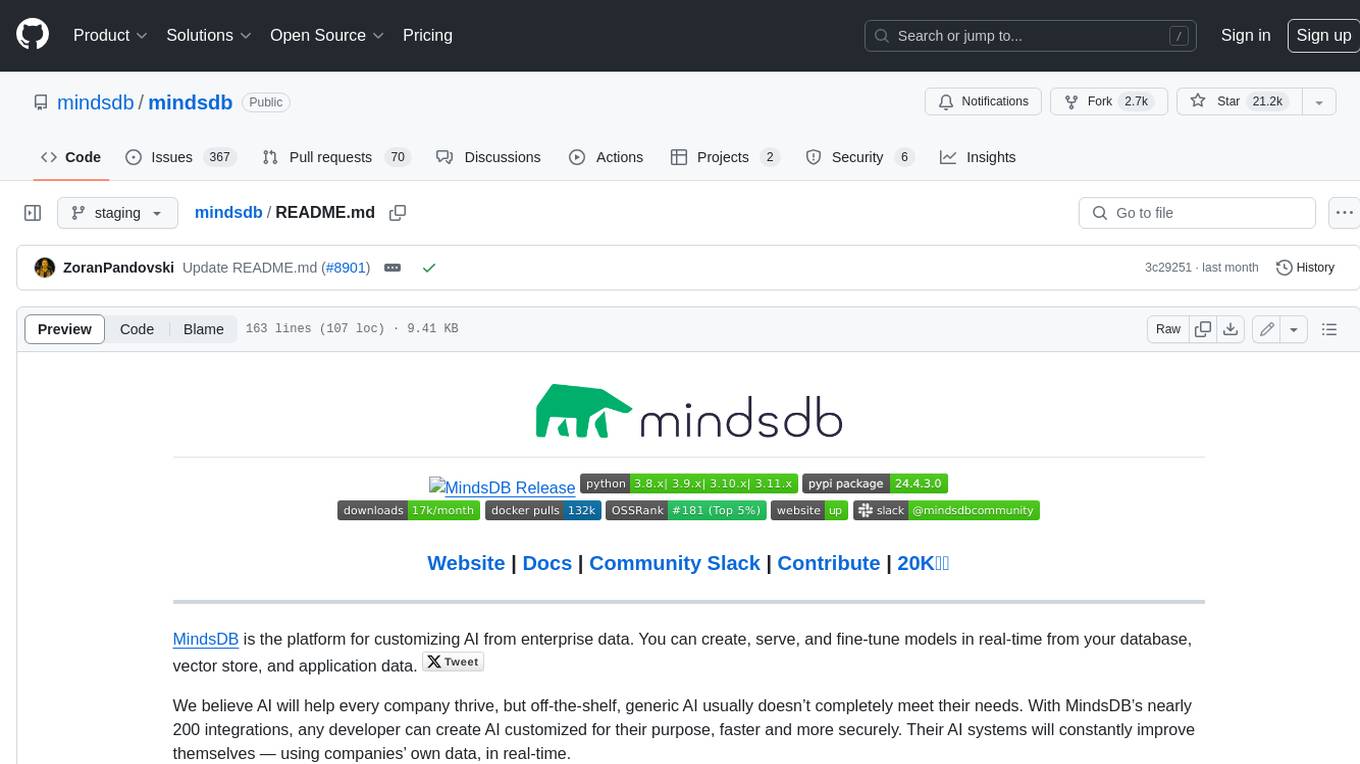
mindsdb
MindsDB is a platform for customizing AI from enterprise data. You can create, serve, and fine-tune models in real-time from your database, vector store, and application data. MindsDB "enhances" SQL syntax with AI capabilities to make it accessible for developers worldwide. With MindsDBβs nearly 200 integrations, any developer can create AI customized for their purpose, faster and more securely. Their AI systems will constantly improve themselves β using companiesβ own data, in real-time.
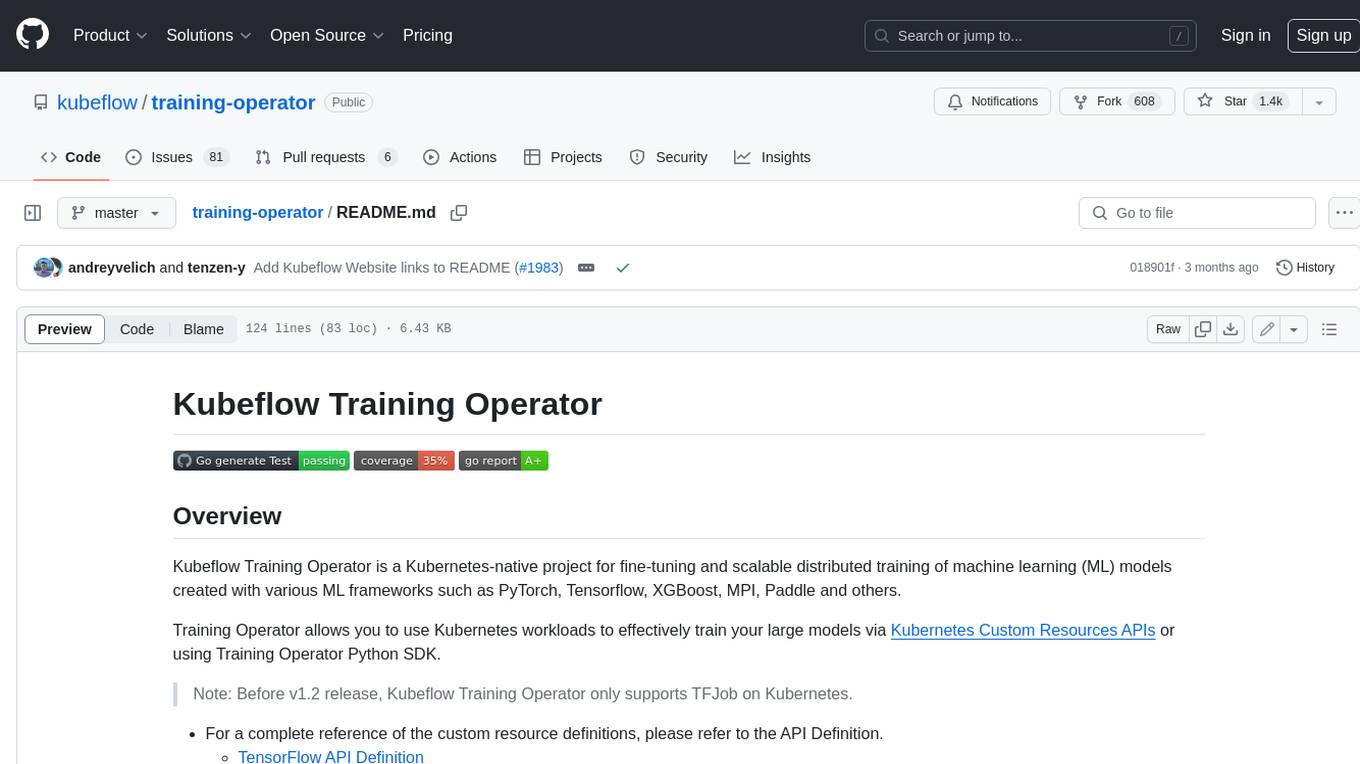
training-operator
Kubeflow Training Operator is a Kubernetes-native project for fine-tuning and scalable distributed training of machine learning (ML) models created with various ML frameworks such as PyTorch, Tensorflow, XGBoost, MPI, Paddle and others. Training Operator allows you to use Kubernetes workloads to effectively train your large models via Kubernetes Custom Resources APIs or using Training Operator Python SDK. > Note: Before v1.2 release, Kubeflow Training Operator only supports TFJob on Kubernetes. * For a complete reference of the custom resource definitions, please refer to the API Definition. * TensorFlow API Definition * PyTorch API Definition * Apache MXNet API Definition * XGBoost API Definition * MPI API Definition * PaddlePaddle API Definition * For details of all-in-one operator design, please refer to the All-in-one Kubeflow Training Operator * For details on its observability, please refer to the monitoring design doc.
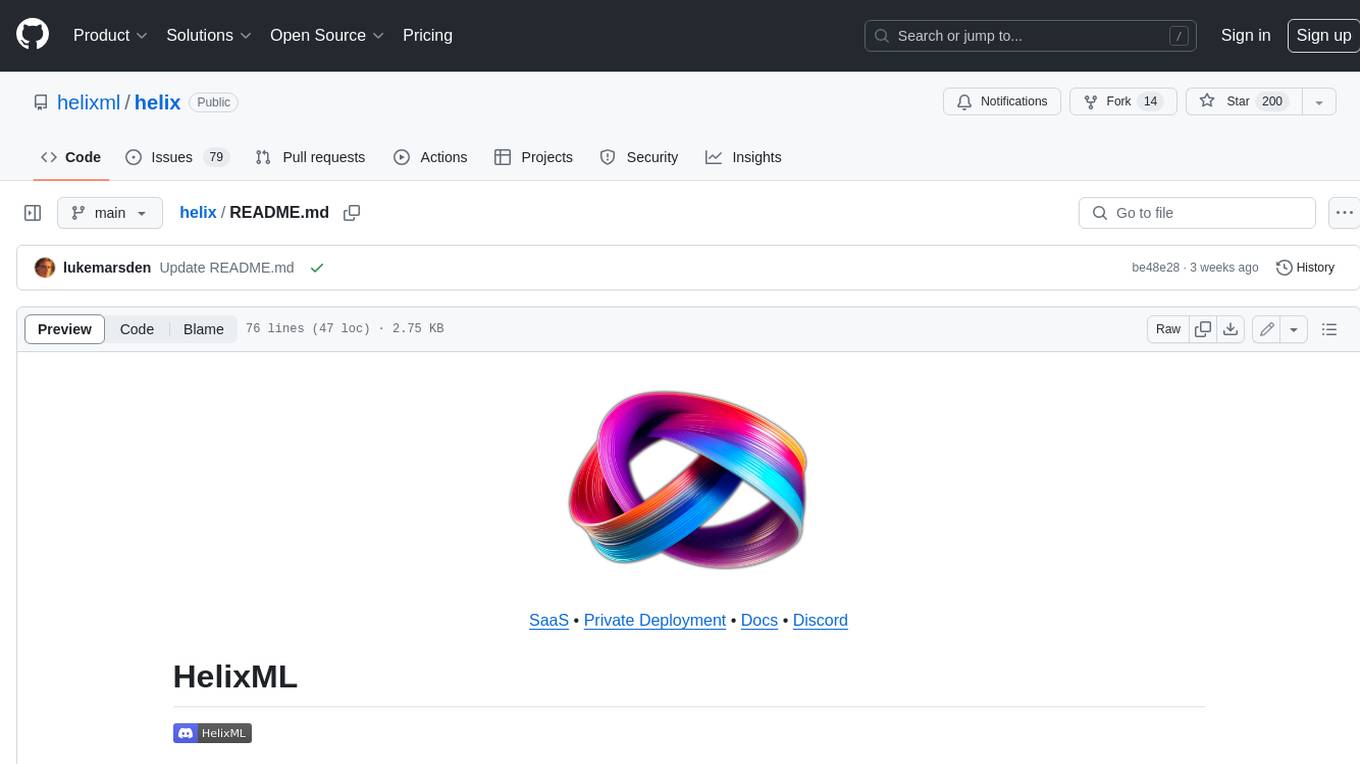
helix
HelixML is a private GenAI platform that allows users to deploy the best of open AI in their own data center or VPC while retaining complete data security and control. It includes support for fine-tuning models with drag-and-drop functionality. HelixML brings the best of open source AI to businesses in an ergonomic and scalable way, optimizing the tradeoff between GPU memory and latency.
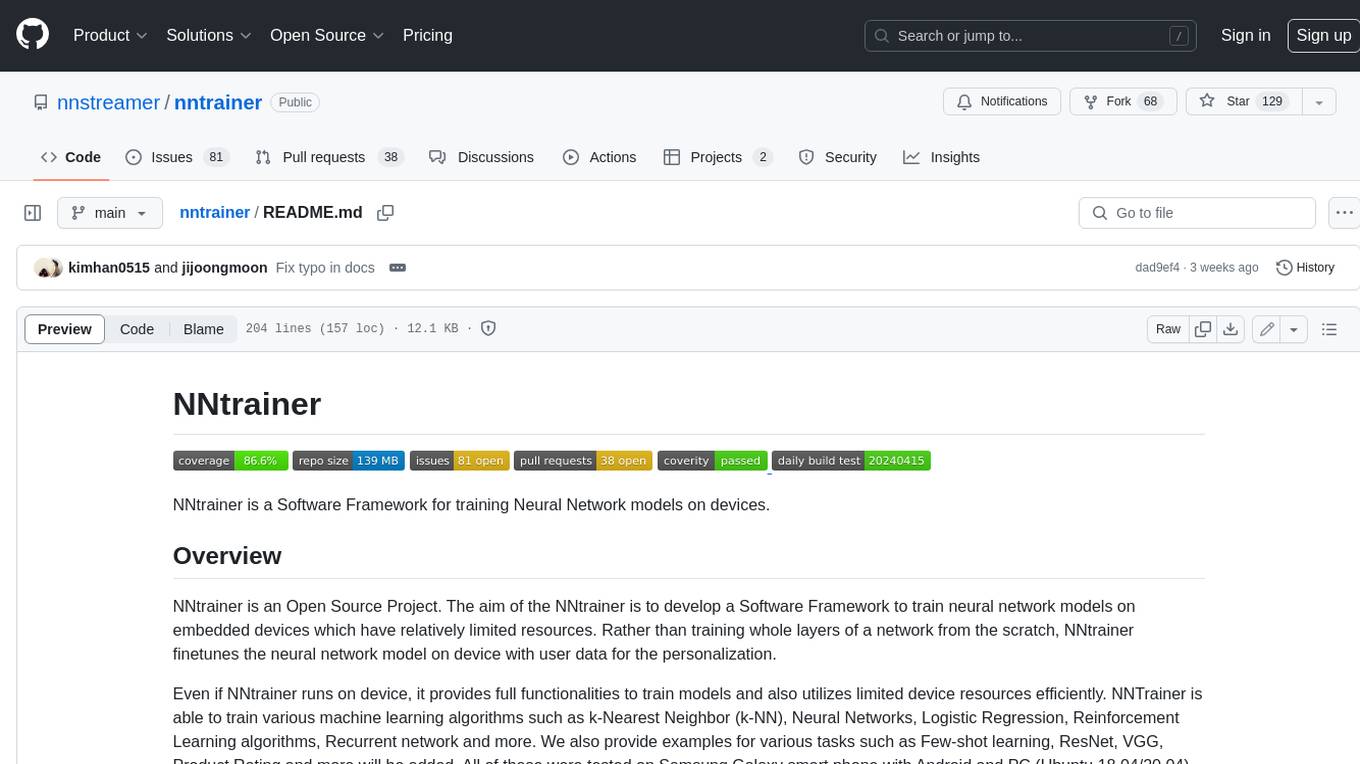
nntrainer
NNtrainer is a software framework for training neural network models on devices with limited resources. It enables on-device fine-tuning of neural networks using user data for personalization. NNtrainer supports various machine learning algorithms and provides examples for tasks such as few-shot learning, ResNet, VGG, and product rating. It is optimized for embedded devices and utilizes CBLAS and CUBLAS for accelerated calculations. NNtrainer is open source and released under the Apache License version 2.0.
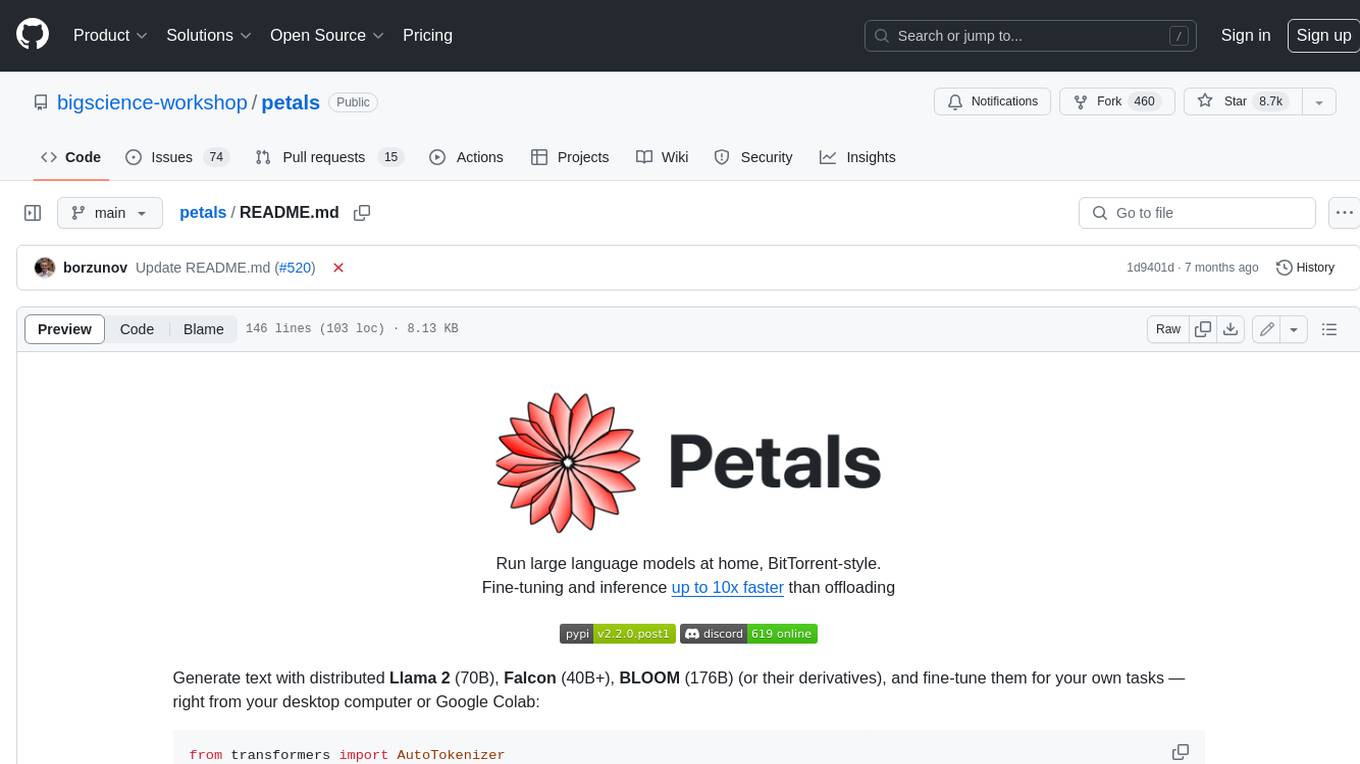
petals
Petals is a tool that allows users to run large language models at home in a BitTorrent-style manner. It enables fine-tuning and inference up to 10x faster than offloading. Users can generate text with distributed models like Llama 2, Falcon, and BLOOM, and fine-tune them for specific tasks directly from their desktop computer or Google Colab. Petals is a community-run system that relies on people sharing their GPUs to increase its capacity and offer a distributed network for hosting model layers.
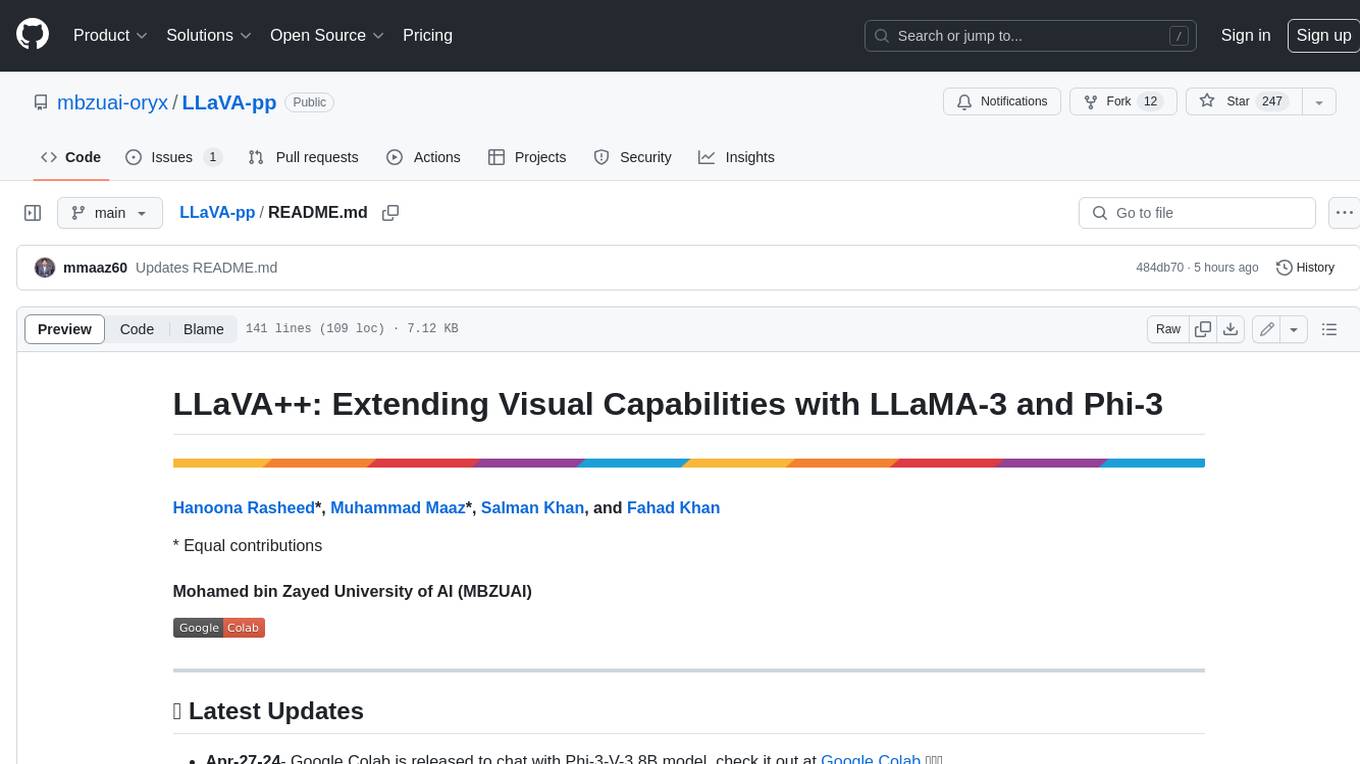
LLaVA-pp
This repository, LLaVA++, extends the visual capabilities of the LLaVA 1.5 model by incorporating the latest LLMs, Phi-3 Mini Instruct 3.8B, and LLaMA-3 Instruct 8B. It provides various models for instruction-following LMMS and academic-task-oriented datasets, along with training scripts for Phi-3-V and LLaMA-3-V. The repository also includes installation instructions and acknowledgments to related open-source contributions.
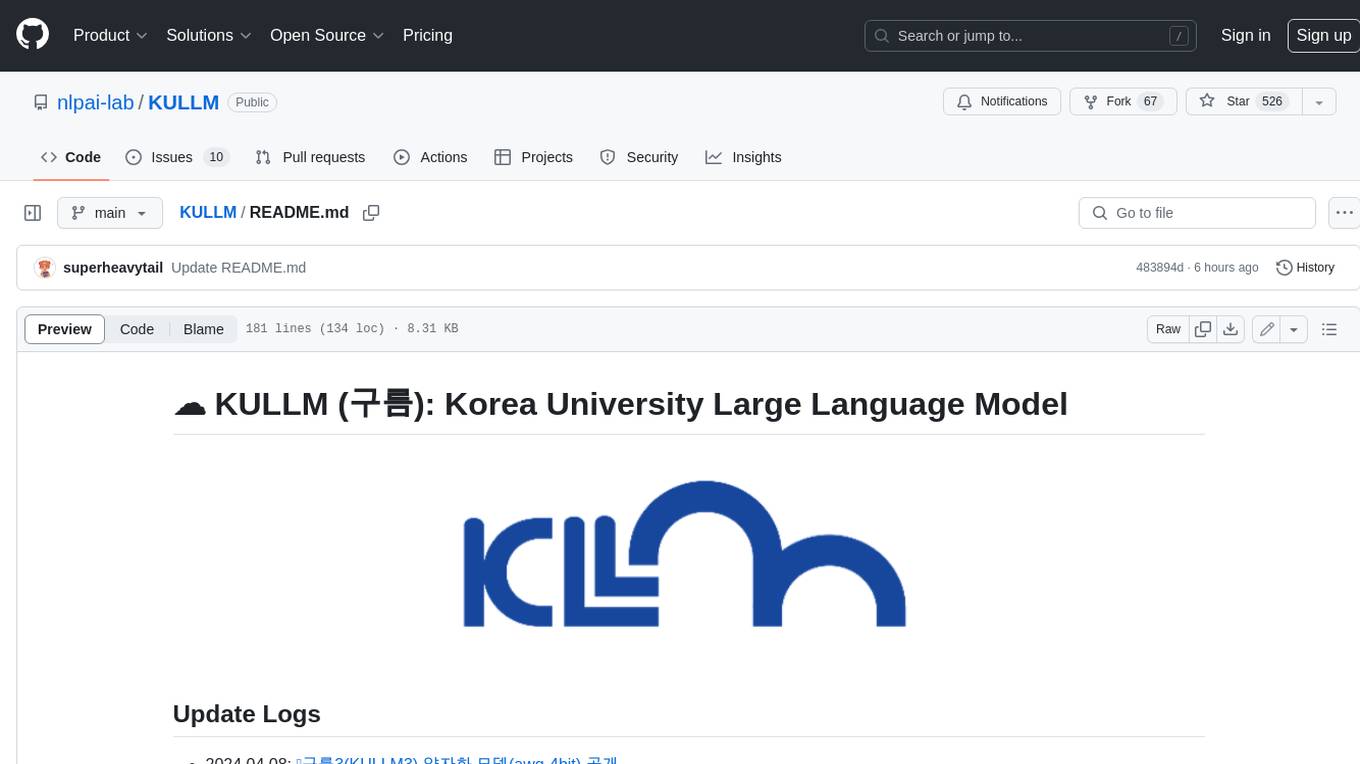
KULLM
KULLM (ꡬλ¦) is a Korean Large Language Model developed by Korea University NLP & AI Lab and HIAI Research Institute. It is based on the upstage/SOLAR-10.7B-v1.0 model and has been fine-tuned for instruction. The model has been trained on 8ΓA100 GPUs and is capable of generating responses in Korean language. KULLM exhibits hallucination and repetition phenomena due to its decoding strategy. Users should be cautious as the model may produce inaccurate or harmful results. Performance may vary in benchmarks without a fixed system prompt.
For similar jobs
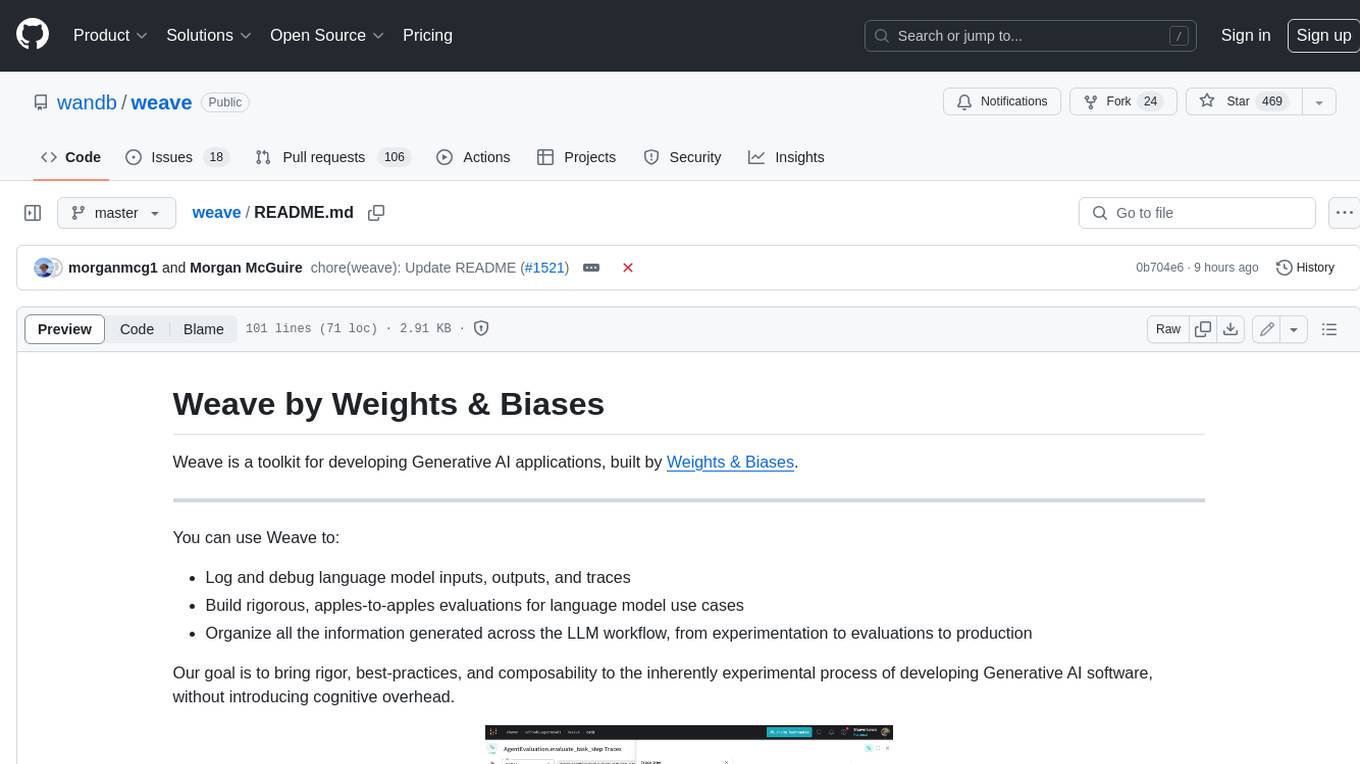
weave
Weave is a toolkit for developing Generative AI applications, built by Weights & Biases. With Weave, you can log and debug language model inputs, outputs, and traces; build rigorous, apples-to-apples evaluations for language model use cases; and organize all the information generated across the LLM workflow, from experimentation to evaluations to production. Weave aims to bring rigor, best-practices, and composability to the inherently experimental process of developing Generative AI software, without introducing cognitive overhead.
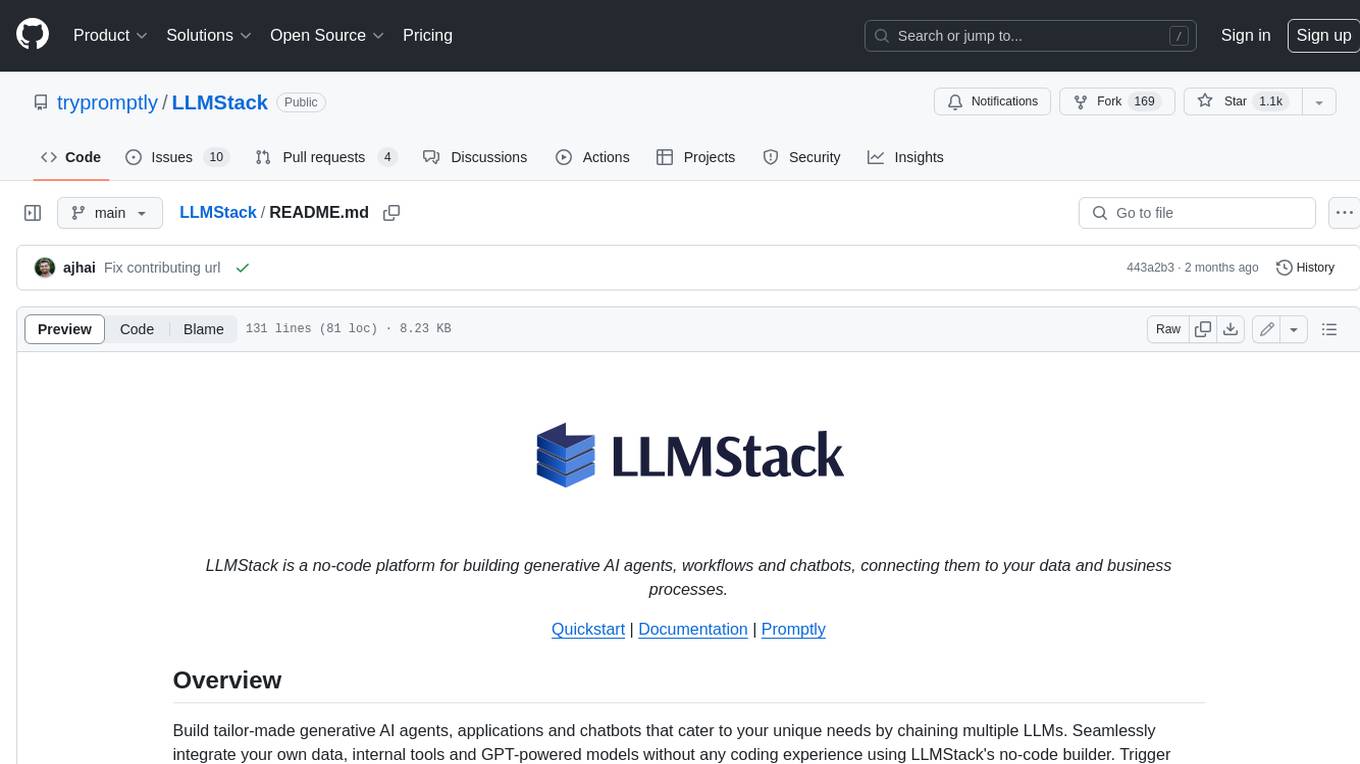
LLMStack
LLMStack is a no-code platform for building generative AI agents, workflows, and chatbots. It allows users to connect their own data, internal tools, and GPT-powered models without any coding experience. LLMStack can be deployed to the cloud or on-premise and can be accessed via HTTP API or triggered from Slack or Discord.
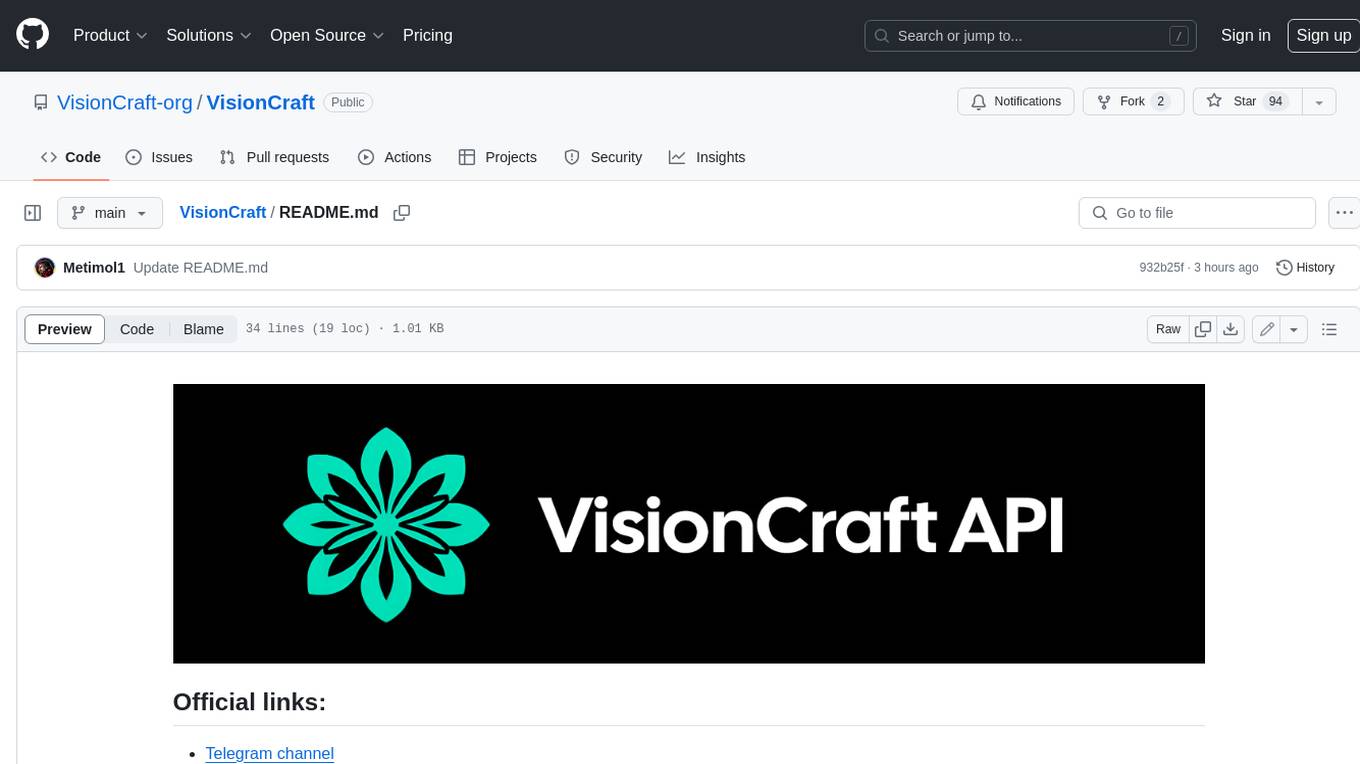
VisionCraft
The VisionCraft API is a free API for using over 100 different AI models. From images to sound.
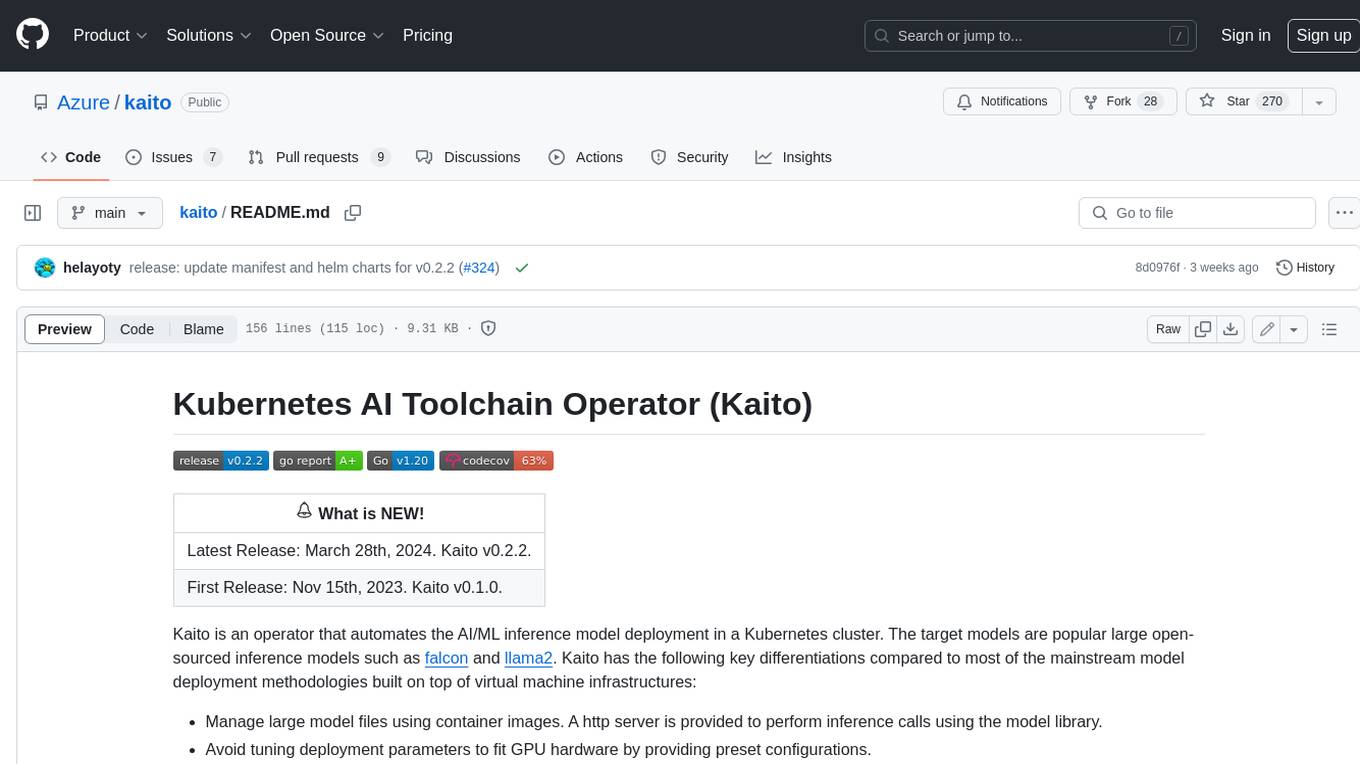
kaito
Kaito is an operator that automates the AI/ML inference model deployment in a Kubernetes cluster. It manages large model files using container images, avoids tuning deployment parameters to fit GPU hardware by providing preset configurations, auto-provisions GPU nodes based on model requirements, and hosts large model images in the public Microsoft Container Registry (MCR) if the license allows. Using Kaito, the workflow of onboarding large AI inference models in Kubernetes is largely simplified.
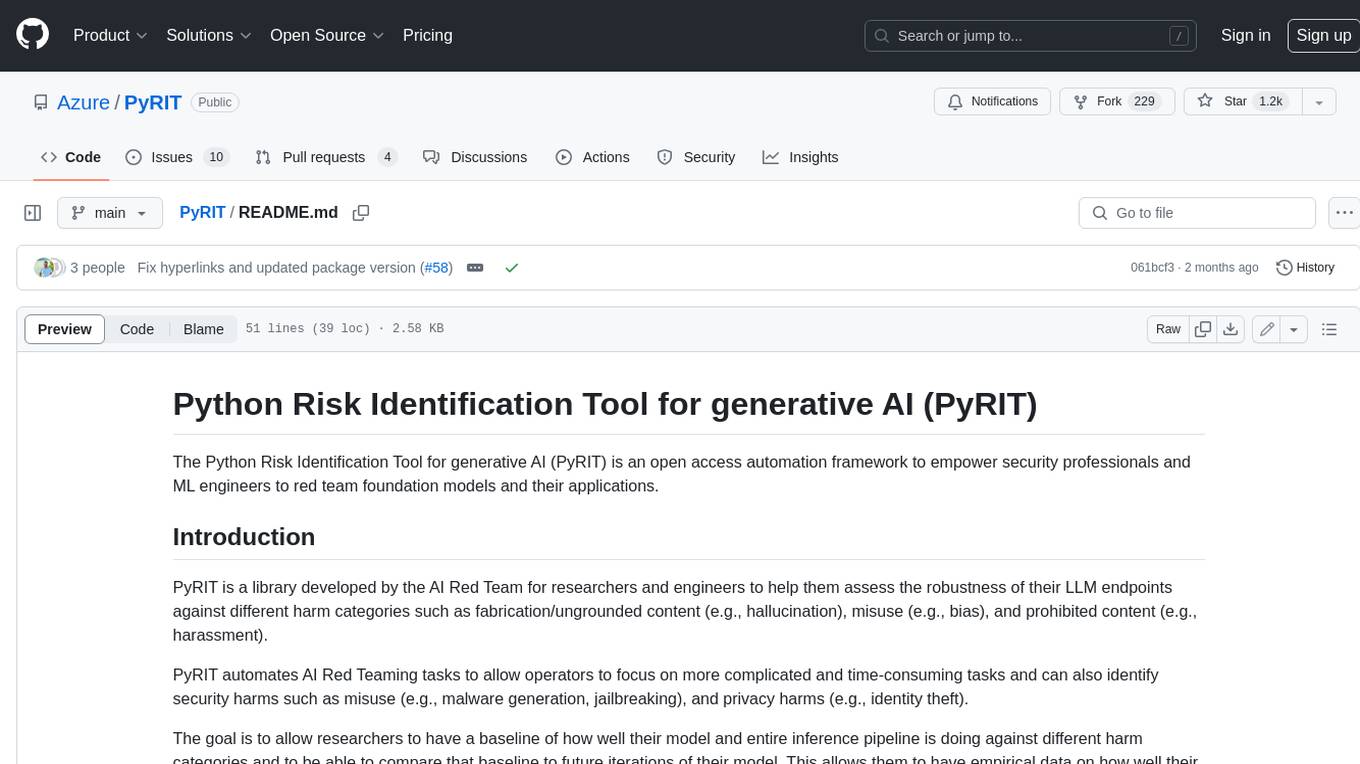
PyRIT
PyRIT is an open access automation framework designed to empower security professionals and ML engineers to red team foundation models and their applications. It automates AI Red Teaming tasks to allow operators to focus on more complicated and time-consuming tasks and can also identify security harms such as misuse (e.g., malware generation, jailbreaking), and privacy harms (e.g., identity theft). The goal is to allow researchers to have a baseline of how well their model and entire inference pipeline is doing against different harm categories and to be able to compare that baseline to future iterations of their model. This allows them to have empirical data on how well their model is doing today, and detect any degradation of performance based on future improvements.
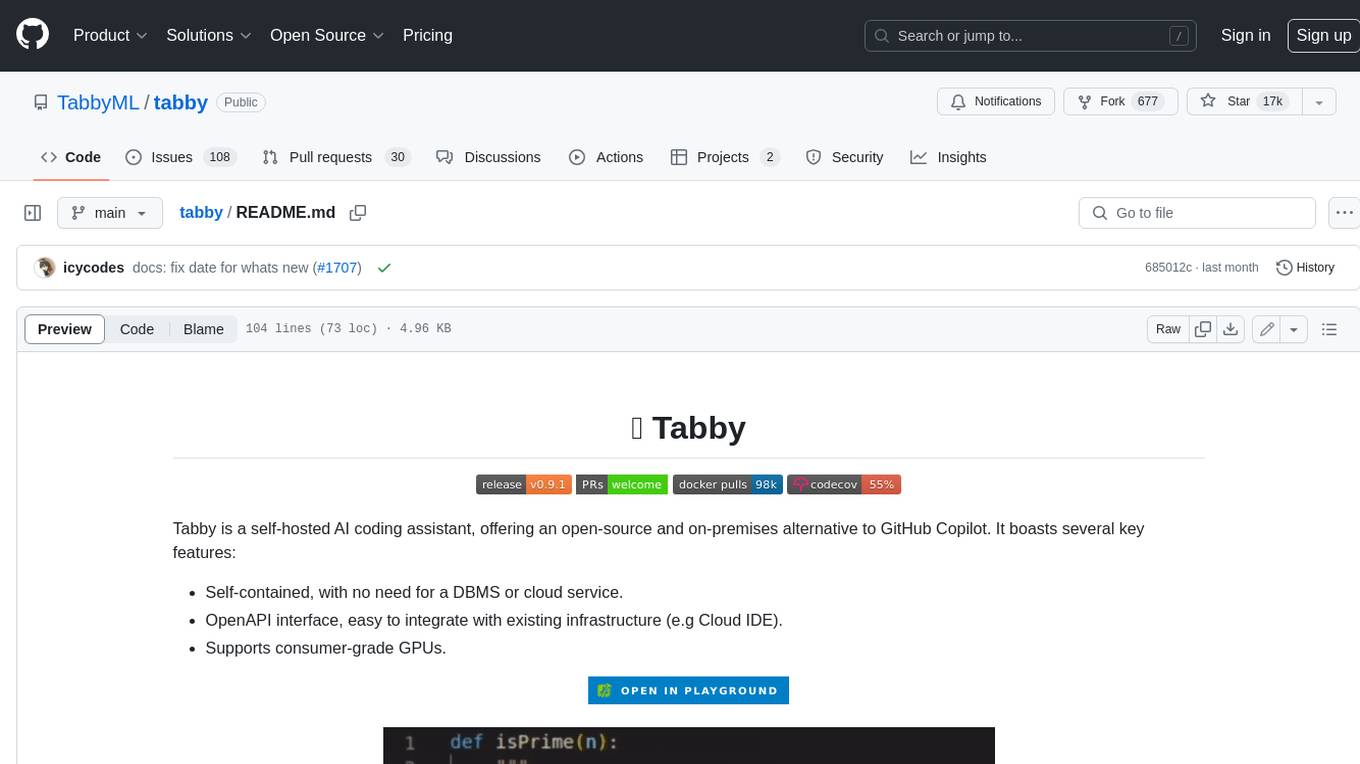
tabby
Tabby is a self-hosted AI coding assistant, offering an open-source and on-premises alternative to GitHub Copilot. It boasts several key features: * Self-contained, with no need for a DBMS or cloud service. * OpenAPI interface, easy to integrate with existing infrastructure (e.g Cloud IDE). * Supports consumer-grade GPUs.
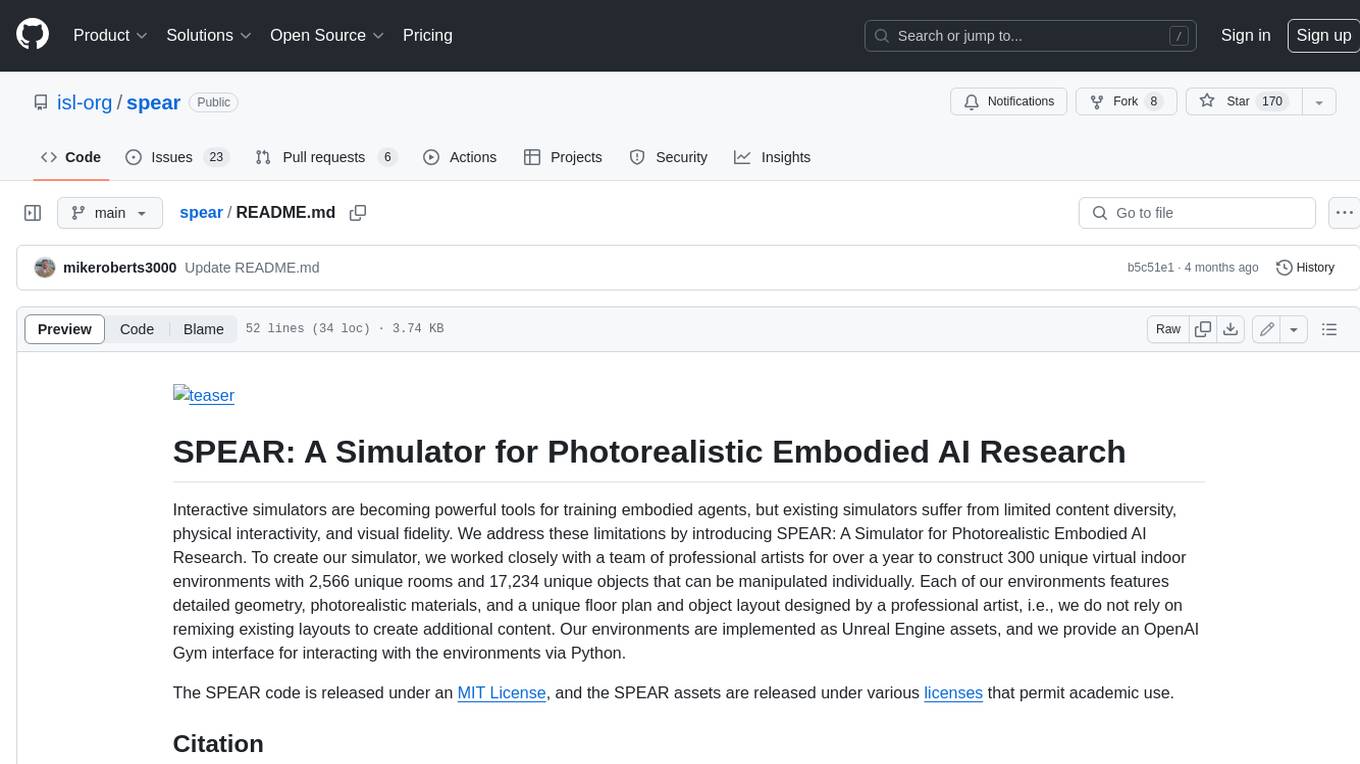
spear
SPEAR (Simulator for Photorealistic Embodied AI Research) is a powerful tool for training embodied agents. It features 300 unique virtual indoor environments with 2,566 unique rooms and 17,234 unique objects that can be manipulated individually. Each environment is designed by a professional artist and features detailed geometry, photorealistic materials, and a unique floor plan and object layout. SPEAR is implemented as Unreal Engine assets and provides an OpenAI Gym interface for interacting with the environments via Python.
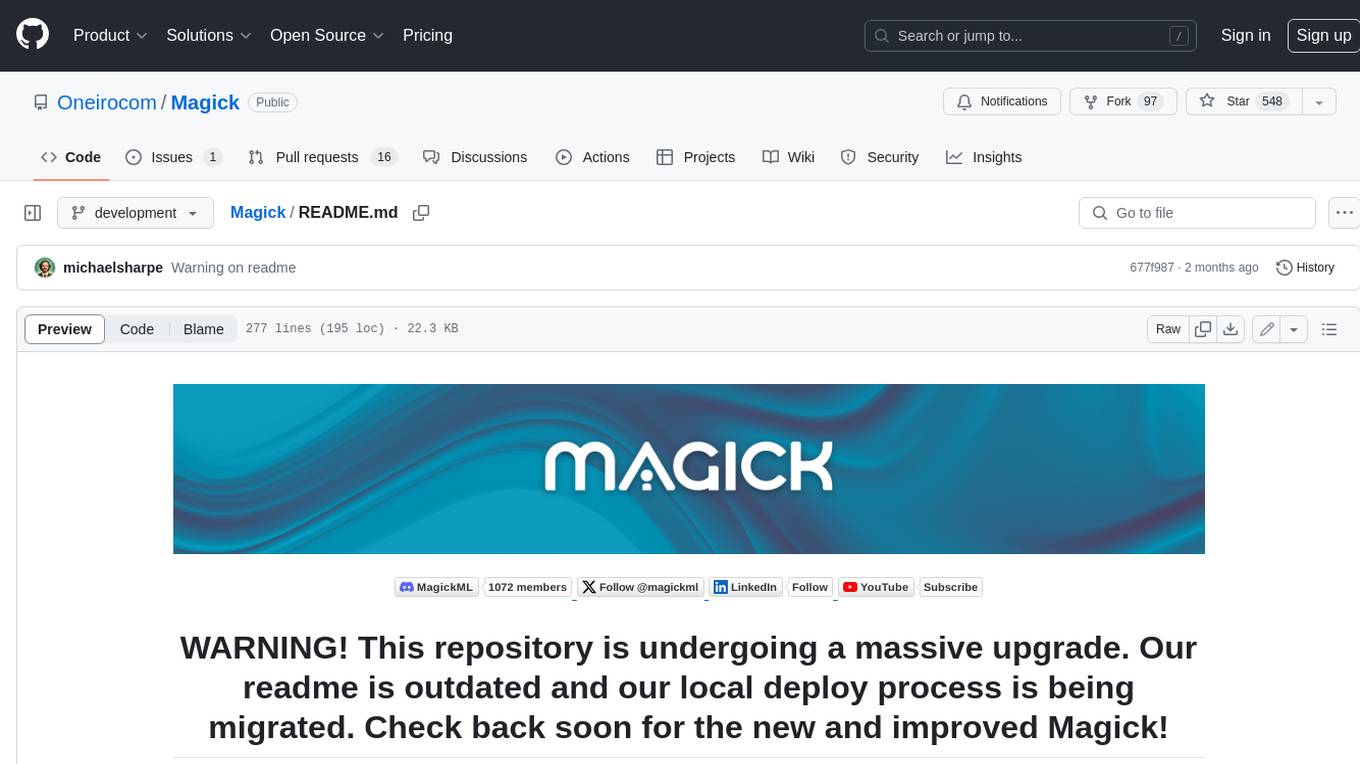
Magick
Magick is a groundbreaking visual AIDE (Artificial Intelligence Development Environment) for no-code data pipelines and multimodal agents. Magick can connect to other services and comes with nodes and templates well-suited for intelligent agents, chatbots, complex reasoning systems and realistic characters.