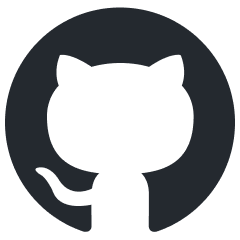
zo2
ZO2 (Zeroth-Order Offloading): Full Parameter Fine-Tuning 175B LLMs with 18GB GPU Memory
Stars: 72
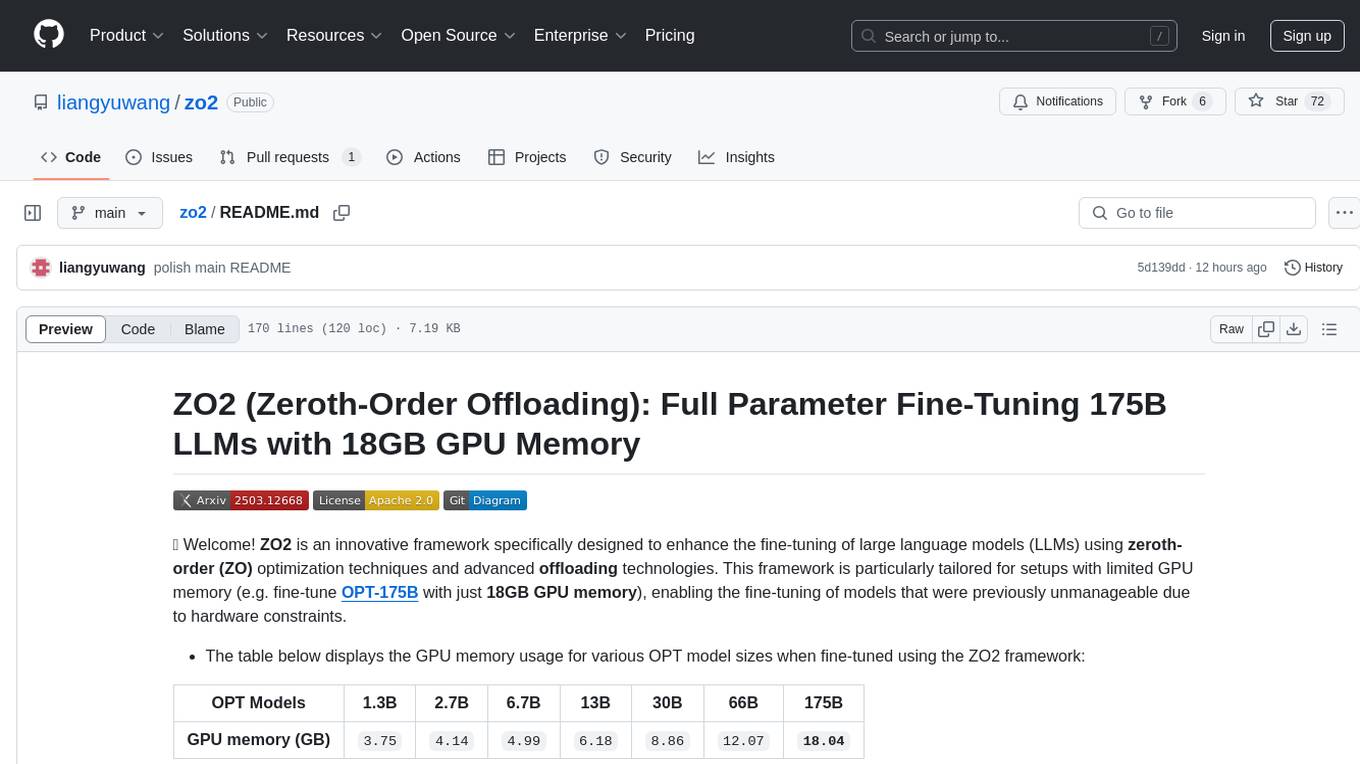
README:
👋 Welcome! ZO2 is an innovative framework specifically designed to enhance the fine-tuning of large language models (LLMs) using zeroth-order (ZO) optimization techniques and advanced offloading technologies. This framework is particularly tailored for setups with limited GPU memory (e.g. fine-tune OPT-175B with just 18GB GPU memory), enabling the fine-tuning of models that were previously unmanageable due to hardware constraints.
- The table below displays the GPU memory usage for various OPT model sizes when fine-tuned using the ZO2 framework:
OPT Models | 1.3B | 2.7B | 6.7B | 13B | 30B | 66B | 175B |
---|---|---|---|---|---|---|---|
GPU memory (GB) | 3.75 |
4.14 |
4.99 |
6.18 |
8.86 |
12.07 |
18.04 |
- Install the package and execute the following test to see the memory usage:
bash test/mezo_sgd/hf_opt/record_zo2_memory.sh
- 06/03/2025: We have open-sourced ZO2!
-
Optimized ZO CPU Offloading: ZO2 leverages
zeroth-order (ZO)
methods to efficiently useCPU offloading
, avoiding redundant data transfers and significantly reducing GPU memory demands. This allows for handling large-scale models on hardware with limited GPU resources. -
Dynamic Scheduling: Incorporates a high-performance scheduler to optimize the
computation-communication overlap
, enhancing GPU utilization and preventing training delays. -
Capability for Very Large Models: Enables the fine-tuning of extraordinarily large models, such as those with over
175 billion parameters
, on single GPUs with as little as18GB
of memory, previously impossible with traditional methods. -
Empirical Validation: ZO2 has demonstrated through rigorous testing that it can efficiently fine-tune massive models
without extra time costs or accuracy losses
, confirming its effectiveness for large-scale model training.
We offer two installation options, and you only need to use one of them to install ZO2:
- To experiment with our examples, tutorials, or tests, follow these steps to set up the ZO2 environment:
git clone https://github.com/liangyuwang/zo2.git
cd zo2/
conda env create -f env.yml
conda activate zo2
-
If you want to use ZO2 as a package in your own code, you can install it directly in your Python environment.
Before installing the ZO2 package, ensure you have the required dependencies:
- PyTorch >= 2.4.0, CUDA >= 12.1
Once the dependencies are installed, you can install the ZO2 package using pip:
pip install git+https://github.com/liangyuwang/zo2.git
We utilize the OPT models and MeZO-SGD as examples. For additional information, please refer to the section on Supported Models and ZO methods.
1. Using MeZO-Runner to Evaluate Fine-tuning Tasks
Before running the following commands, please ensure that you have cloned the entire project. If you installed ZO2 using option 2, you will need to run "git clone https://github.com/liangyuwang/zo2.git" to obtain the complete project, then navigate to the zo2 folder by "cd zo2".
cd example/mezo_runner/
export CUDA_VISIBLE_DEVICES=0
MODEL=facebook/opt-2.7b TASK=SST2 MODE=ft LR=1e-7 EPS=1e-3 STEPS=20000 EVAL_STEPS=4000 bash mezo.sh
2. Fine-Tuning HF Models with ZOTrainer / ZOSFTTrainer [Trainer]
from zo2 import ZOConfig, zo_hf_init
from zo2.trainer.hf_transformers import ZOTrainer
from zo2.trainer.hf_trl import ZOSFTTrainer
from transformers import TrainingArguments
# Model and optimizer init
zo_config = ZOConfig(method="mezo-sgd", zo2=True, offloading_device='cpu', working_device='cuda', lr=1e-5)
with zo_hf_init(zo_config):
from transformers import OPTForCausalLM
model = OPTForCausalLM.from_pretrained("facebook/opt-125m")
model.zo_init(zo_config)
training_args = TrainingArguments("test-trainer")
trainer = ZOTrainer( # or ZOSFTTrainer
model,
args = training_args,
train_dataset=..., # get training dataset
eval_dataset=..., # get eval dataset
data_collator=..., # get data_collator
tokenizer=..., # use suitable tokenizer
...
)
trainer.train()
3. Train HF Models with Custom Training Loop [demo]
from zo2 import ZOConfig, zo_hf_init
# Model and optimizer init
zo_config = ZOConfig(method="mezo-sgd", zo2=True, offloading_device='cpu', working_device='cuda', lr=1e-5)
with zo_hf_init(zo_config):
from transformers import OPTForCausalLM
model = OPTForCausalLM.from_pretrained("facebook/opt-125m")
model.zo_init(zo_config)
# Training loop
for i in range(max_training_step):
# Train
training_input_ids, training_labels = ... # get training data batch
model.zo_train()
loss = model(input_ids=training_input_ids, labels=training_labels)
# Evaluate
eval_input_ids, eval_labels = ... # get eval data batch
model.zo_eval()
output = model(input_ids=eval_input_ids, labels=eval_labels)
Please refer to tutorial.
-
Models:
- NanoGPT (mainly for idea evaluation)
- Transformers: OPT
-
ZO methods:
-
Tasks: Please refer to MeZO-Runner
Please refer to test.
- [ ] Support more models like LLaMA, DeepSeek, and Qwen
- [ ] Support more ZO methods
- [ ] Support more offloading strategies (Disk offloading)
Feel free to submit issues and pull requests to improve the project!
- Liangyu Wang: [email protected]
@article{wang2025zo2,
title={ZO2: Scalable Zeroth-Order Fine-Tuning for Extremely Large Language Models with Limited GPU Memory},
author={Wang, Liangyu and Ren, Jie and Xu, Hang and Wang, Junxiao and Xie, Huanyi and Keyes, David E and Wang, Di},
journal={arXiv preprint arXiv:2503.12668},
year={2025}
}
For Tasks:
Click tags to check more tools for each tasksFor Jobs:
Alternative AI tools for zo2
Similar Open Source Tools
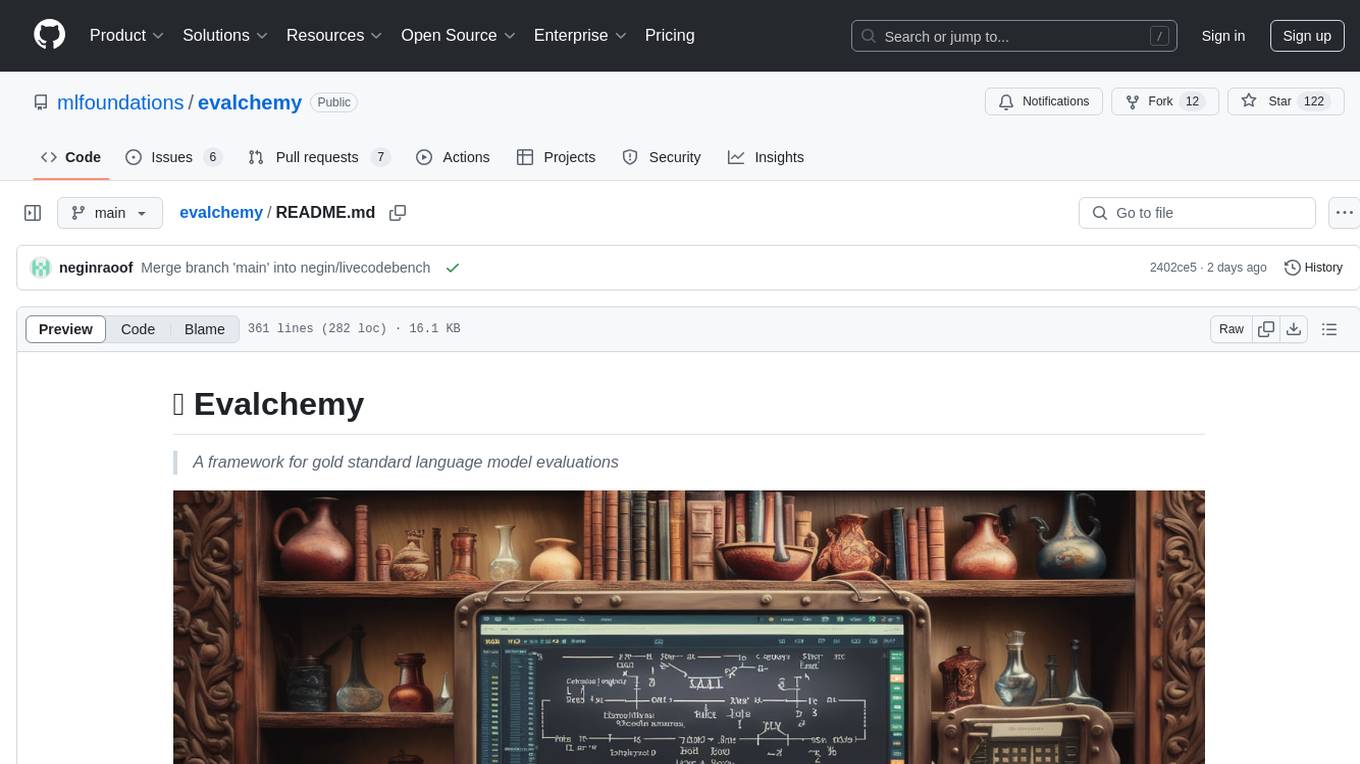
evalchemy
Evalchemy is a unified and easy-to-use toolkit for evaluating language models, focusing on post-trained models. It integrates multiple existing benchmarks such as RepoBench, AlpacaEval, and ZeroEval. Key features include unified installation, parallel evaluation, simplified usage, and results management. Users can run various benchmarks with a consistent command-line interface and track results locally or integrate with a database for systematic tracking and leaderboard submission.
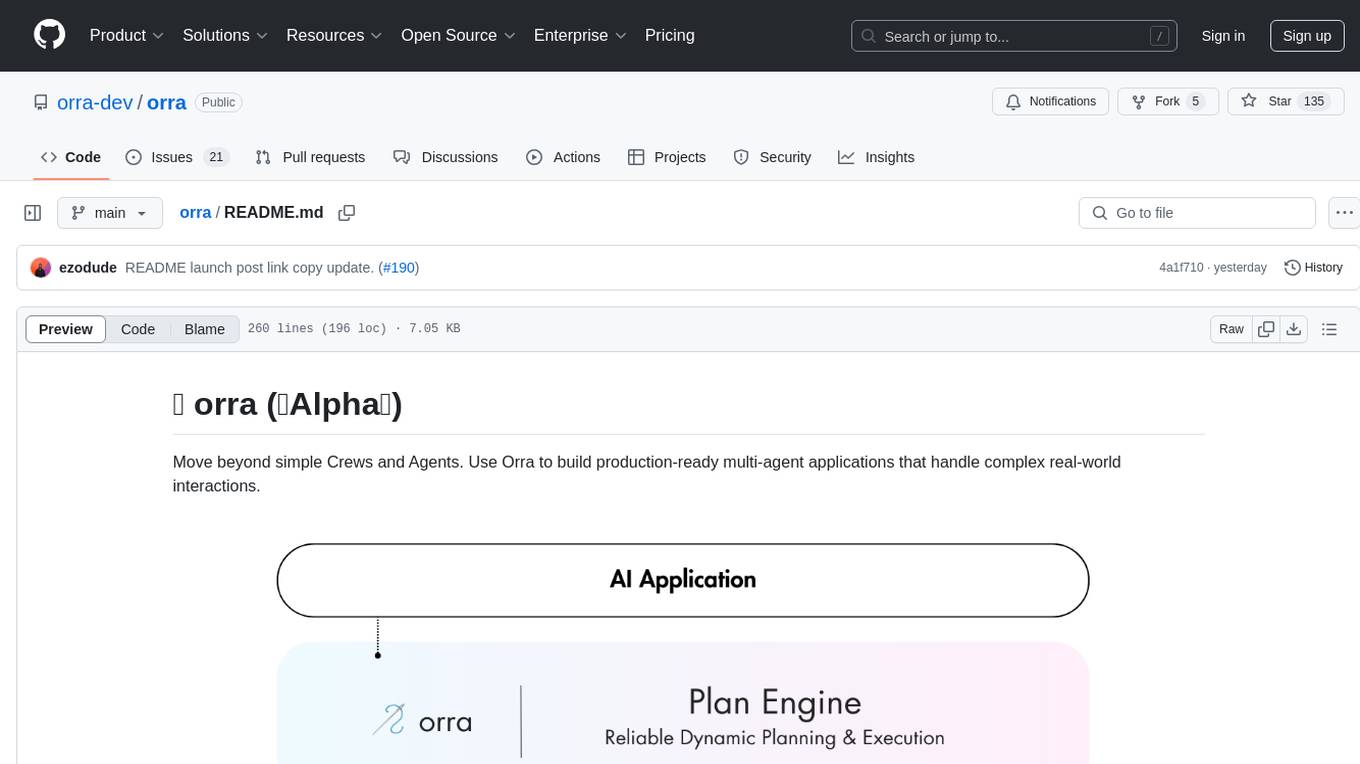
orra
Orra is a tool for building production-ready multi-agent applications that handle complex real-world interactions. It coordinates tasks across existing stack, agents, and tools run as services using intelligent reasoning. With features like smart pre-evaluated execution plans, domain grounding, durable execution, and automatic service health monitoring, Orra enables users to go fast with tools as services and revert state to handle failures. It provides real-time status tracking and webhook result delivery, making it ideal for developers looking to move beyond simple crews and agents.
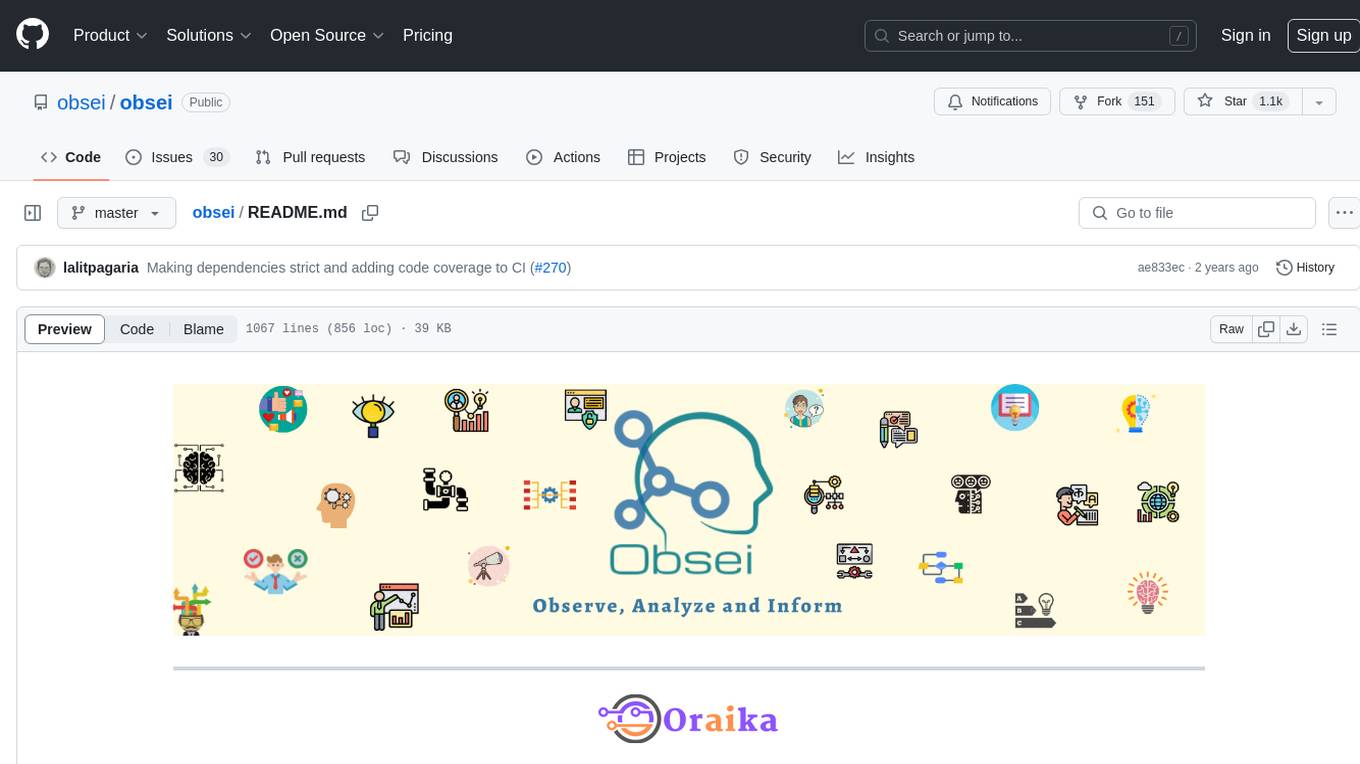
obsei
Obsei is an open-source, low-code, AI powered automation tool that consists of an Observer to collect unstructured data from various sources, an Analyzer to analyze the collected data with various AI tasks, and an Informer to send analyzed data to various destinations. The tool is suitable for scheduled jobs or serverless applications as all Observers can store their state in databases. Obsei is still in alpha stage, so caution is advised when using it in production. The tool can be used for social listening, alerting/notification, automatic customer issue creation, extraction of deeper insights from feedbacks, market research, dataset creation for various AI tasks, and more based on creativity.
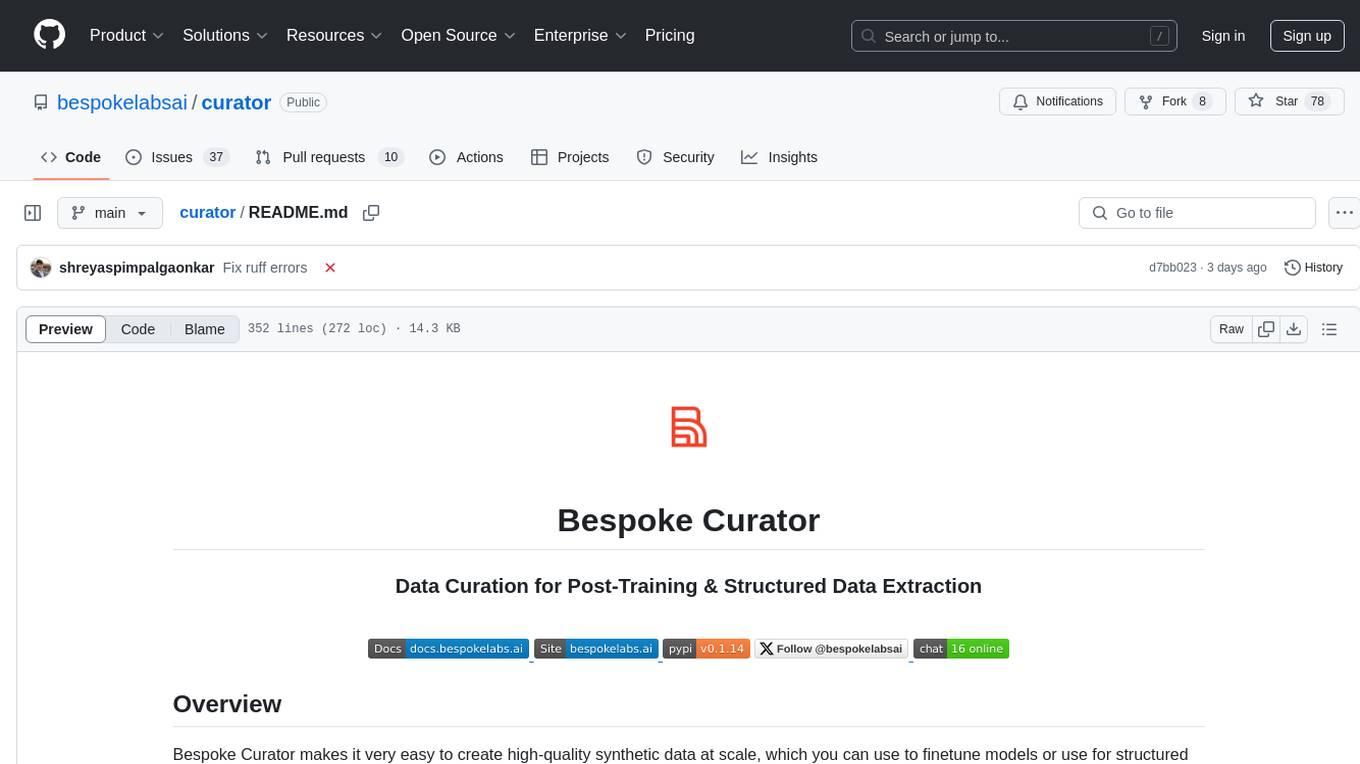
curator
Bespoke Curator is an open-source tool for data curation and structured data extraction. It provides a Python library for generating synthetic data at scale, with features like programmability, performance optimization, caching, and integration with HuggingFace Datasets. The tool includes a Curator Viewer for dataset visualization and offers a rich set of functionalities for creating and refining data generation strategies.
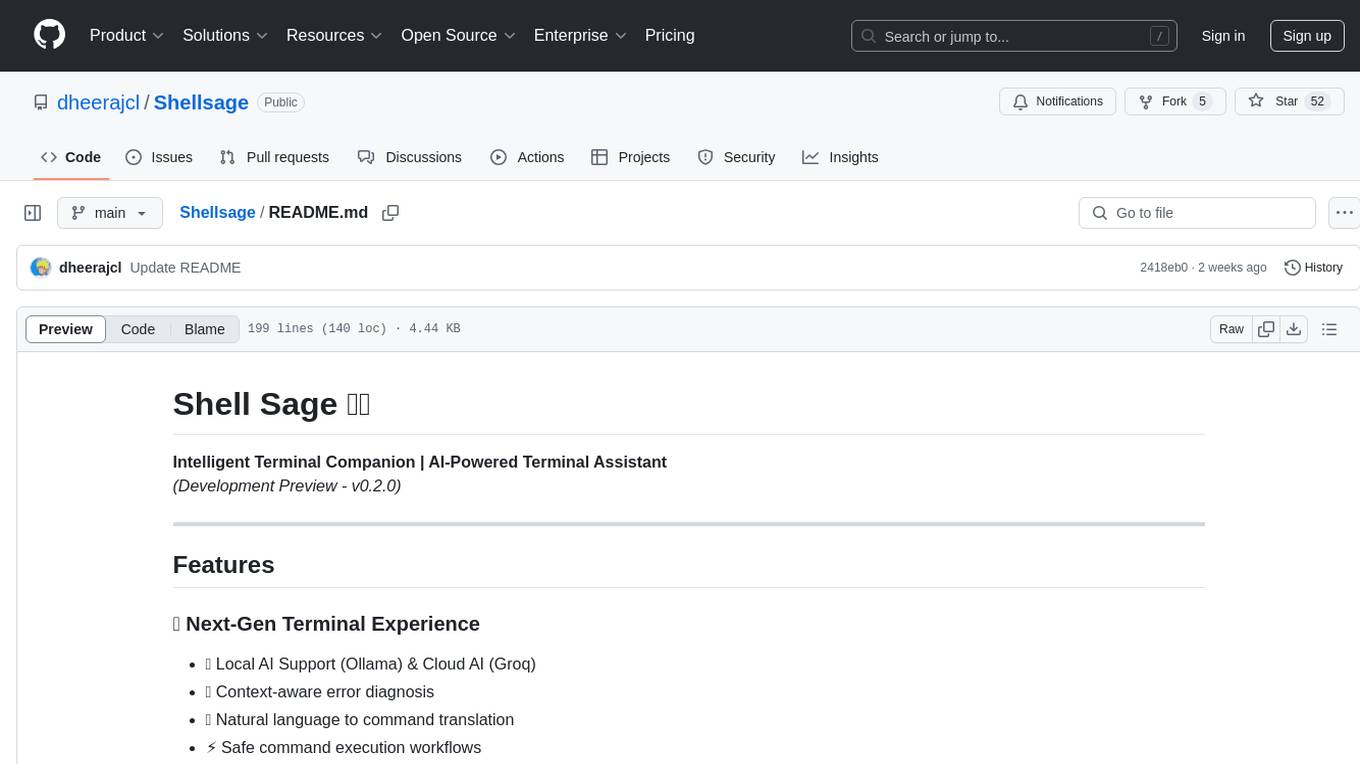
Shellsage
Shell Sage is an intelligent terminal companion and AI-powered terminal assistant that enhances the terminal experience with features like local and cloud AI support, context-aware error diagnosis, natural language to command translation, and safe command execution workflows. It offers interactive workflows, supports various API providers, and allows for custom model selection. Users can configure the tool for local or API mode, select specific models, and switch between modes easily. Currently in alpha development, Shell Sage has known limitations like limited Windows support and occasional false positives in error detection. The roadmap includes improvements like better context awareness, Windows PowerShell integration, Tmux integration, and CI/CD error pattern database.
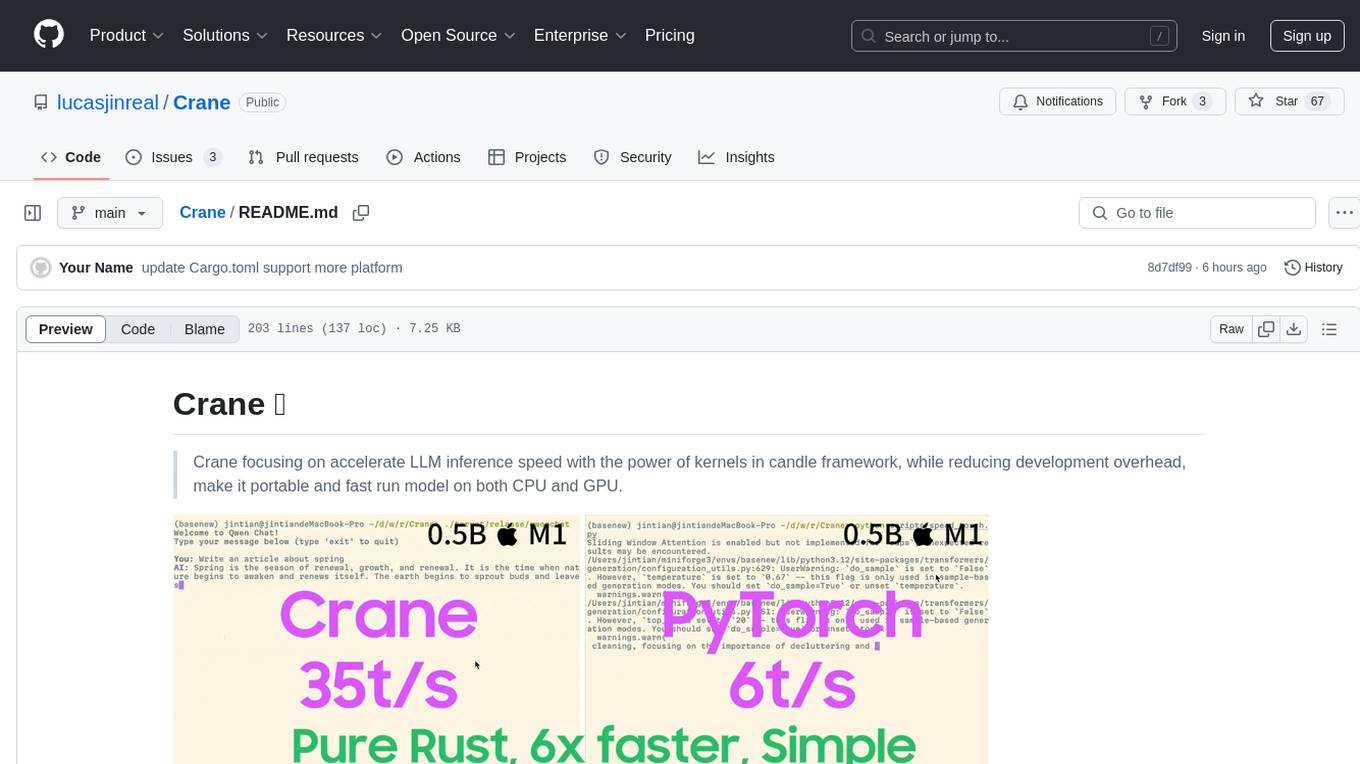
Crane
Crane is a high-performance inference framework leveraging Rust's Candle for maximum speed on CPU/GPU. It focuses on accelerating LLM inference speed with optimized kernels, reducing development overhead, and ensuring portability for running models on both CPU and GPU. Supported models include TTS systems like Spark-TTS and Orpheus-TTS, foundation models like Qwen2.5 series and basic LLMs, and multimodal models like Namo-R1 and Qwen2.5-VL. Key advantages of Crane include blazing-fast inference outperforming native PyTorch, Rust-powered to eliminate C++ complexity, Apple Silicon optimized for GPU acceleration via Metal, and hardware agnostic with a unified codebase for CPU/CUDA/Metal execution. Crane simplifies deployment with the ability to add new models with less than 100 lines of code in most cases.
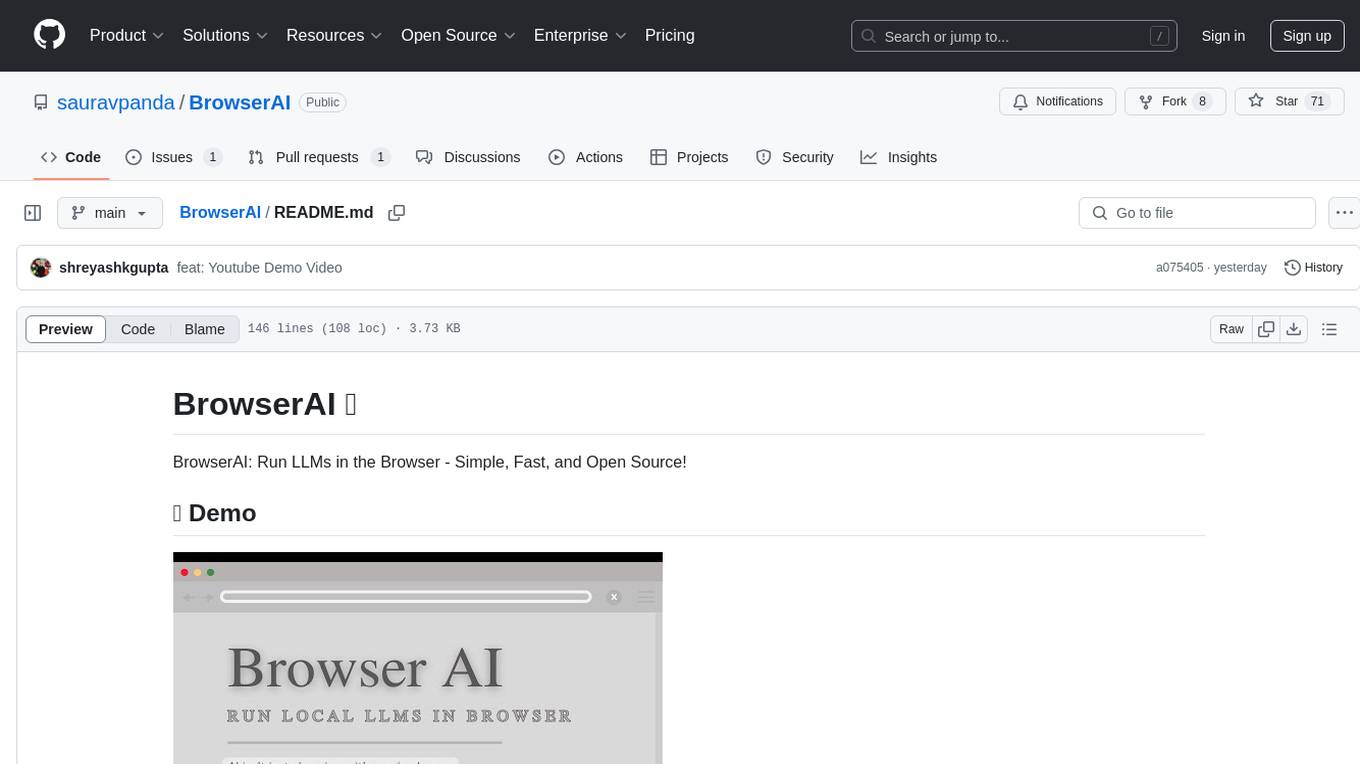
BrowserAI
BrowserAI is a tool that allows users to run large language models (LLMs) directly in the browser, providing a simple, fast, and open-source solution. It prioritizes privacy by processing data locally, is cost-effective with no server costs, works offline after initial download, and offers WebGPU acceleration for high performance. It is developer-friendly with a simple API, supports multiple engines, and comes with pre-configured models for easy use. Ideal for web developers, companies needing privacy-conscious AI solutions, researchers experimenting with browser-based AI, and hobbyists exploring AI without infrastructure overhead.
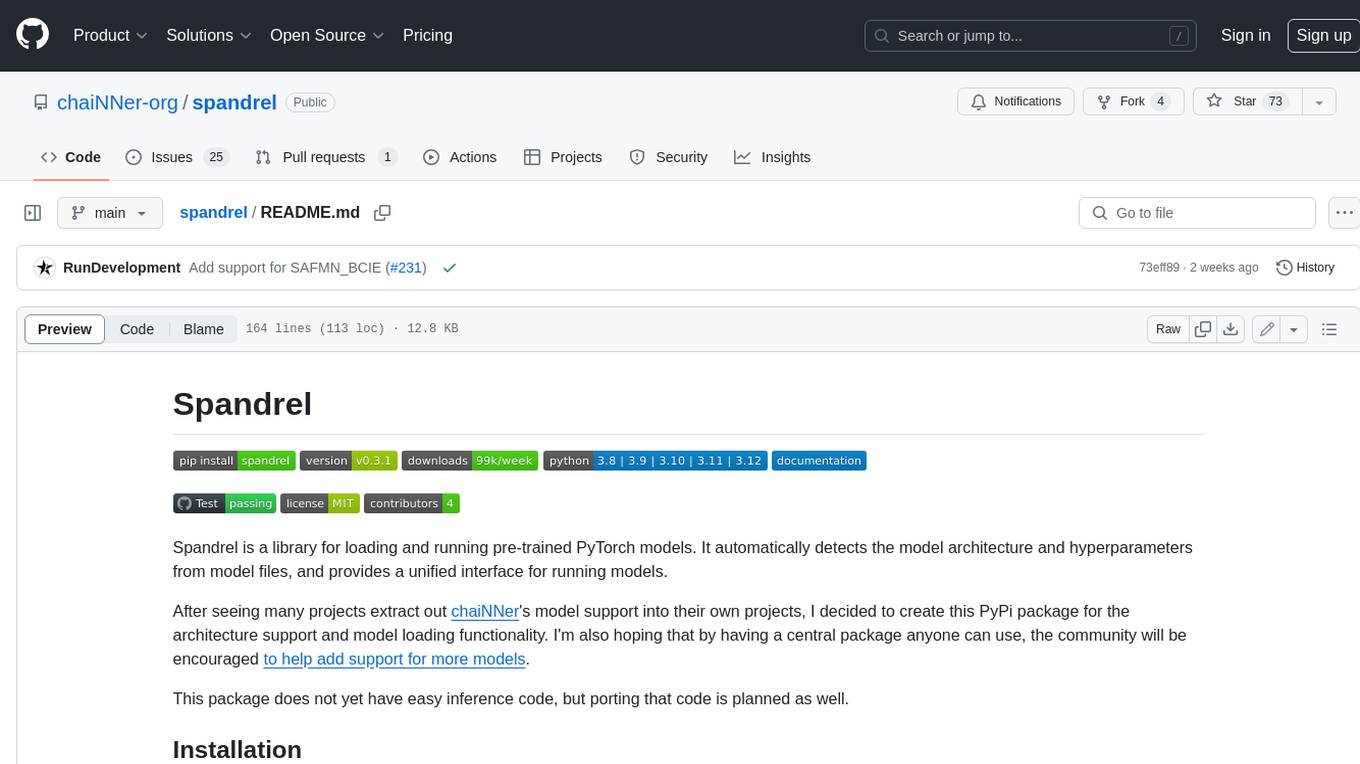
spandrel
Spandrel is a library for loading and running pre-trained PyTorch models. It automatically detects the model architecture and hyperparameters from model files, and provides a unified interface for running models.
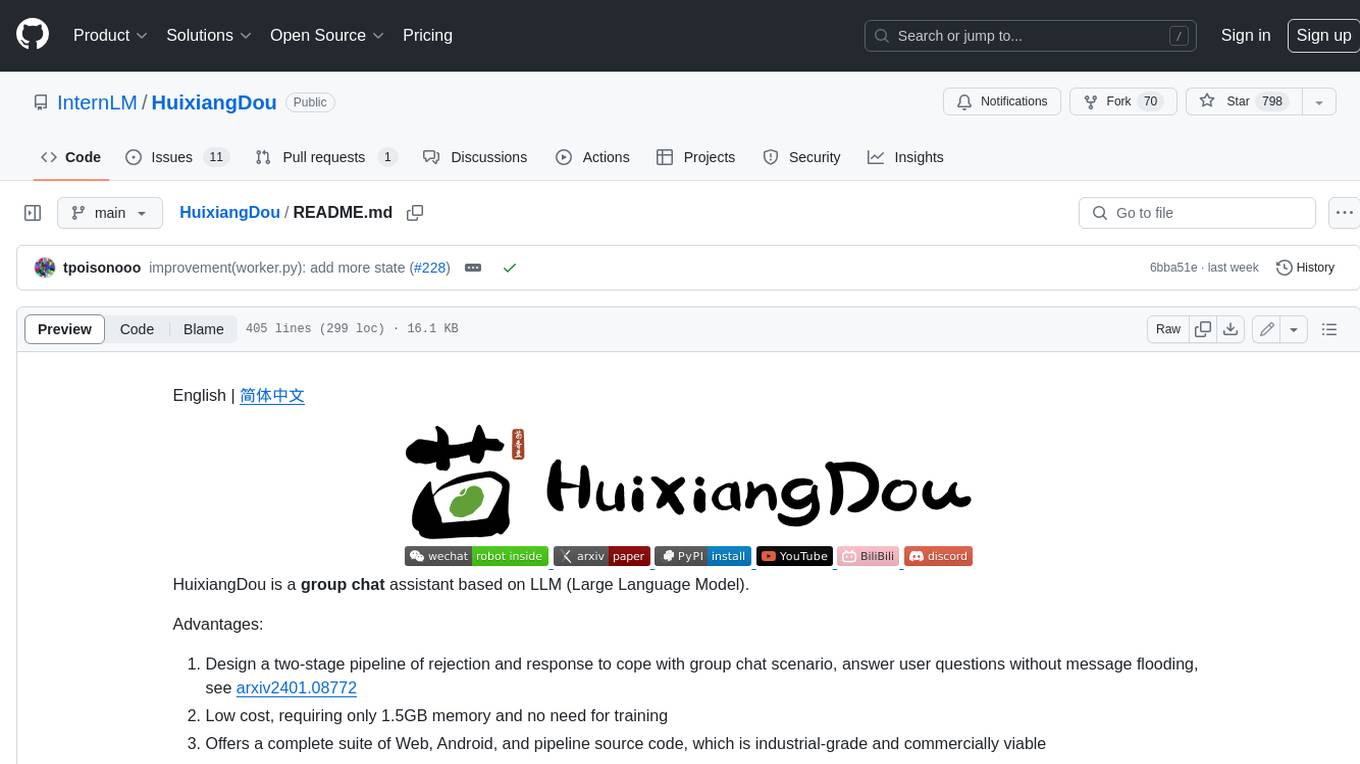
HuixiangDou
HuixiangDou is a **group chat** assistant based on LLM (Large Language Model). Advantages: 1. Design a two-stage pipeline of rejection and response to cope with group chat scenario, answer user questions without message flooding, see arxiv2401.08772 2. Low cost, requiring only 1.5GB memory and no need for training 3. Offers a complete suite of Web, Android, and pipeline source code, which is industrial-grade and commercially viable Check out the scenes in which HuixiangDou are running and join WeChat Group to try AI assistant inside. If this helps you, please give it a star ⭐
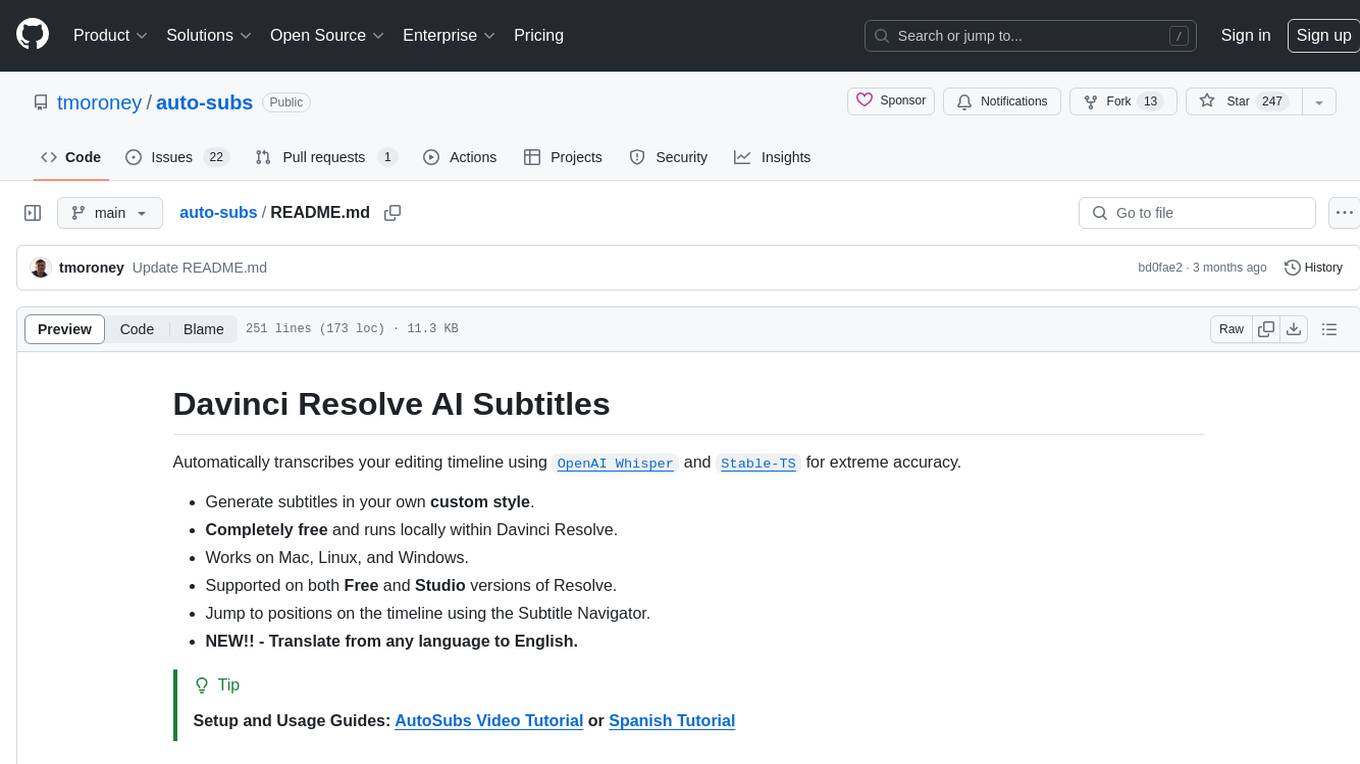
auto-subs
Auto-subs is a tool designed to automatically transcribe editing timelines using OpenAI Whisper and Stable-TS for extreme accuracy. It generates subtitles in a custom style, is completely free, and runs locally within Davinci Resolve. It works on Mac, Linux, and Windows, supporting both Free and Studio versions of Resolve. Users can jump to positions on the timeline using the Subtitle Navigator and translate from any language to English. The tool provides a user-friendly interface for creating and customizing subtitles for video content.
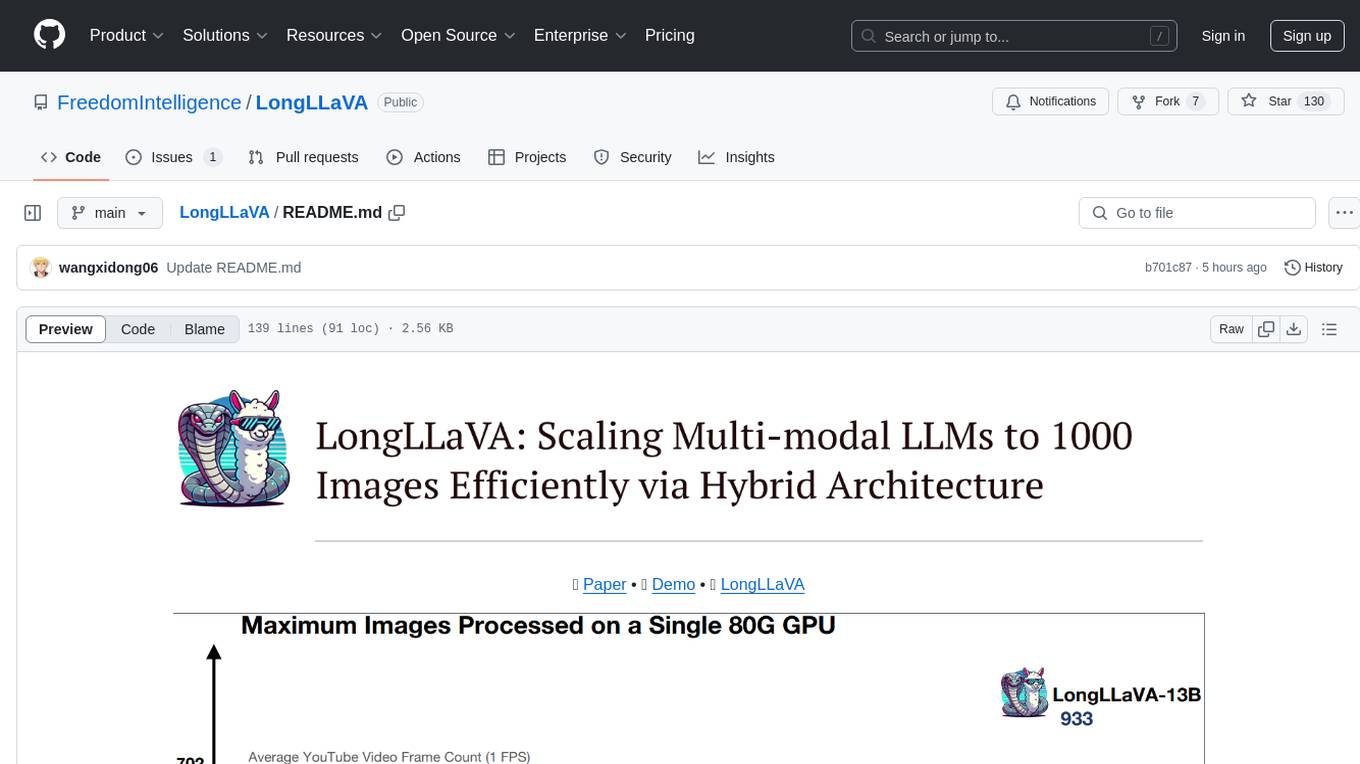
LongLLaVA
LongLLaVA is a tool for scaling multi-modal LLMs to 1000 images efficiently via hybrid architecture. It includes stages for single-image alignment, instruction-tuning, and multi-image instruction-tuning, with evaluation through a command line interface and model inference. The tool aims to achieve GPT-4V level capabilities and beyond, providing reproducibility of results and benchmarks for efficiency and performance.
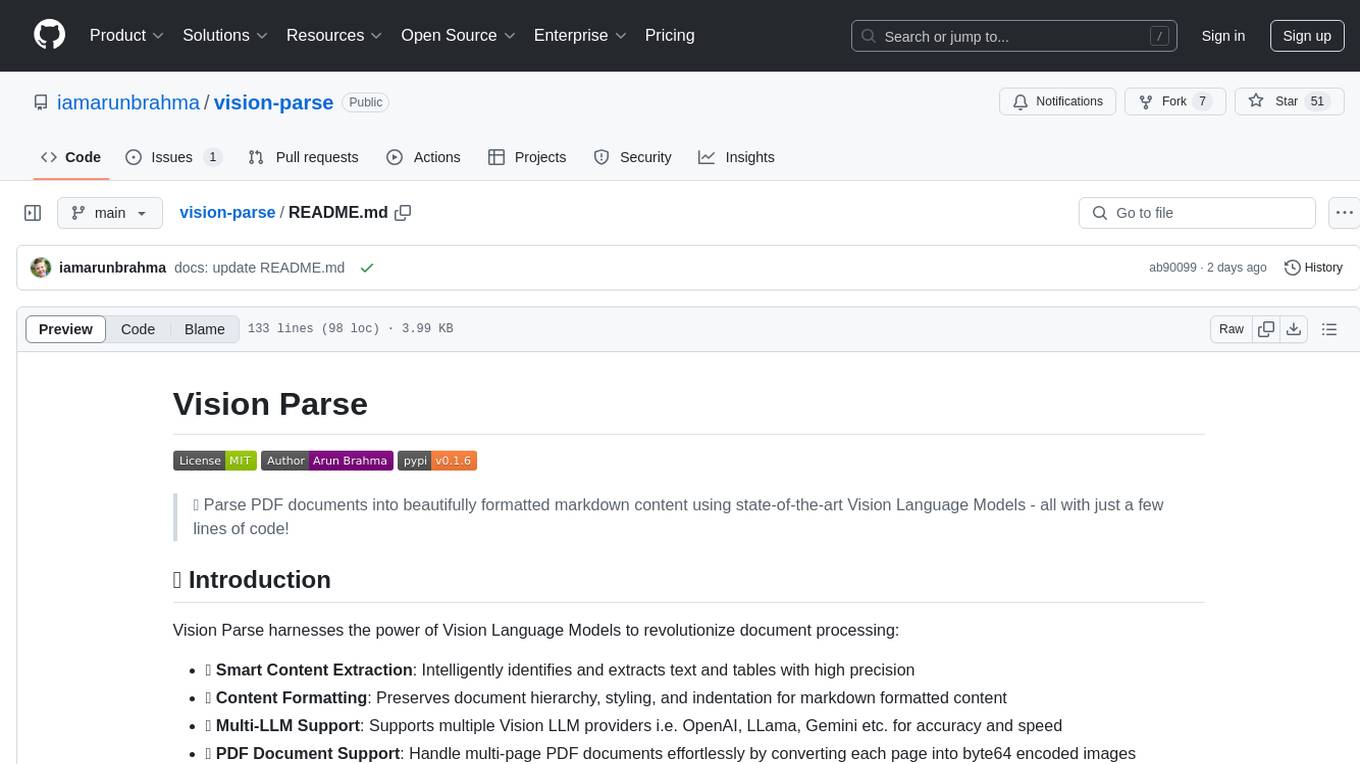
vision-parse
Vision Parse is a tool that leverages Vision Language Models to parse PDF documents into beautifully formatted markdown content. It offers smart content extraction, content formatting, multi-LLM support, PDF document support, and local model hosting using Ollama. Users can easily convert PDFs to markdown with high precision and preserve document hierarchy and styling. The tool supports multiple Vision LLM providers like OpenAI, LLama, and Gemini for accuracy and speed, making document processing efficient and effortless.
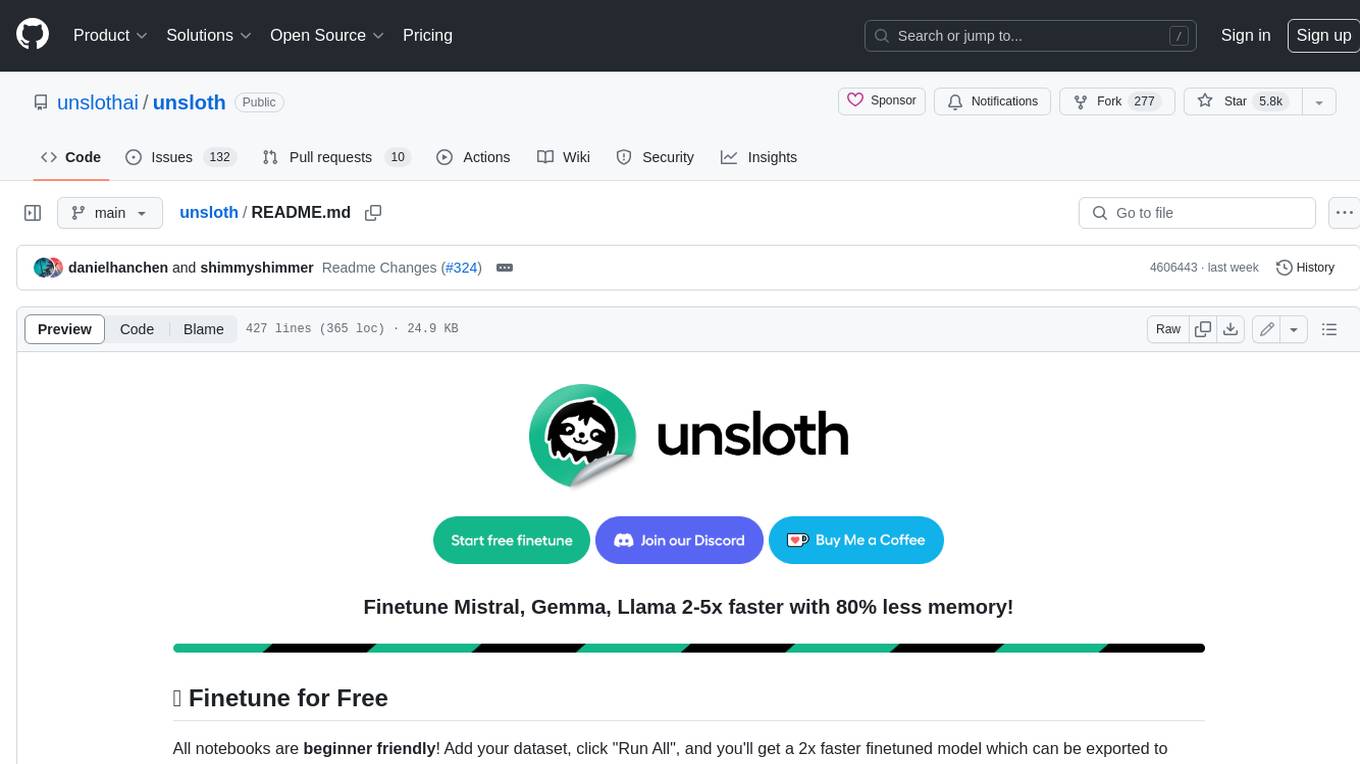
unsloth
Unsloth is a tool that allows users to fine-tune large language models (LLMs) 2-5x faster with 80% less memory. It is a free and open-source tool that can be used to fine-tune LLMs such as Gemma, Mistral, Llama 2-5, TinyLlama, and CodeLlama 34b. Unsloth supports 4-bit and 16-bit QLoRA / LoRA fine-tuning via bitsandbytes. It also supports DPO (Direct Preference Optimization), PPO, and Reward Modelling. Unsloth is compatible with Hugging Face's TRL, Trainer, Seq2SeqTrainer, and Pytorch code. It is also compatible with NVIDIA GPUs since 2018+ (minimum CUDA Capability 7.0).
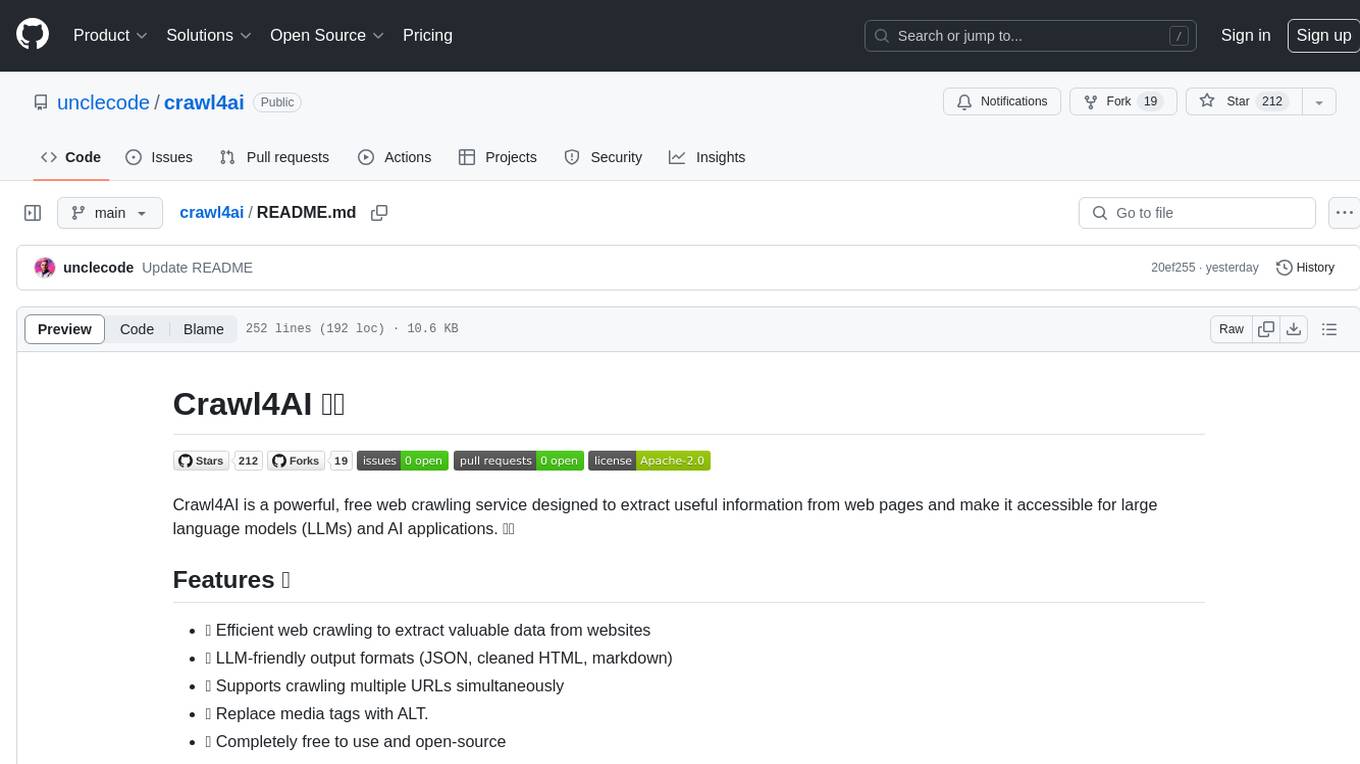
crawl4ai
Crawl4AI is a powerful and free web crawling service that extracts valuable data from websites and provides LLM-friendly output formats. It supports crawling multiple URLs simultaneously, replaces media tags with ALT, and is completely free to use and open-source. Users can integrate Crawl4AI into Python projects as a library or run it as a standalone local server. The tool allows users to crawl and extract data from specified URLs using different providers and models, with options to include raw HTML content, force fresh crawls, and extract meaningful text blocks. Configuration settings can be adjusted in the `crawler/config.py` file to customize providers, API keys, chunk processing, and word thresholds. Contributions to Crawl4AI are welcome from the open-source community to enhance its value for AI enthusiasts and developers.
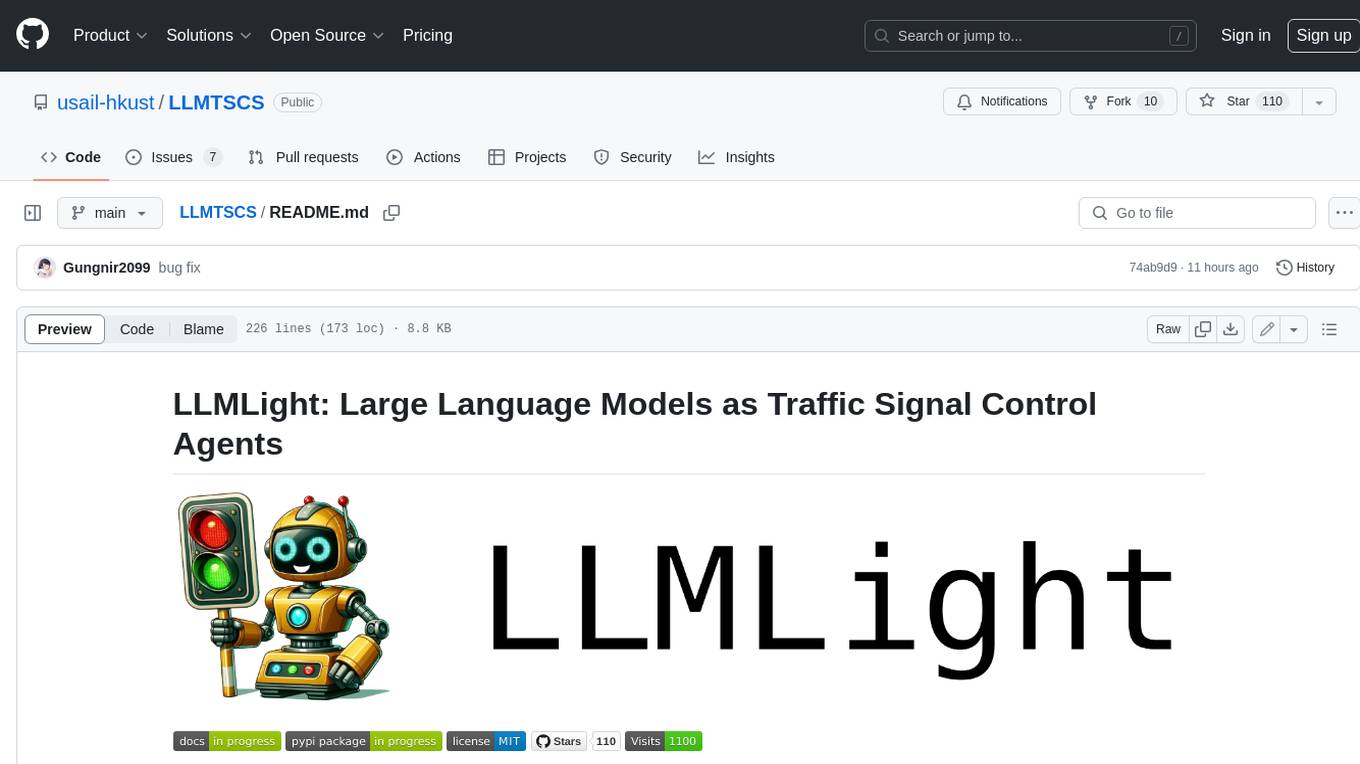
LLMTSCS
LLMLight is a novel framework that employs Large Language Models (LLMs) as decision-making agents for Traffic Signal Control (TSC). The framework leverages the advanced generalization capabilities of LLMs to engage in a reasoning and decision-making process akin to human intuition for effective traffic control. LLMLight has been demonstrated to be remarkably effective, generalizable, and interpretable against various transportation-based and RL-based baselines on nine real-world and synthetic datasets.