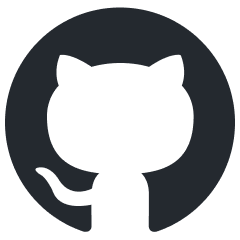
obsei
Obsei is a low code AI powered automation tool. It can be used in various business flows like social listening, AI based alerting, brand image analysis, comparative study and more .
Stars: 1204
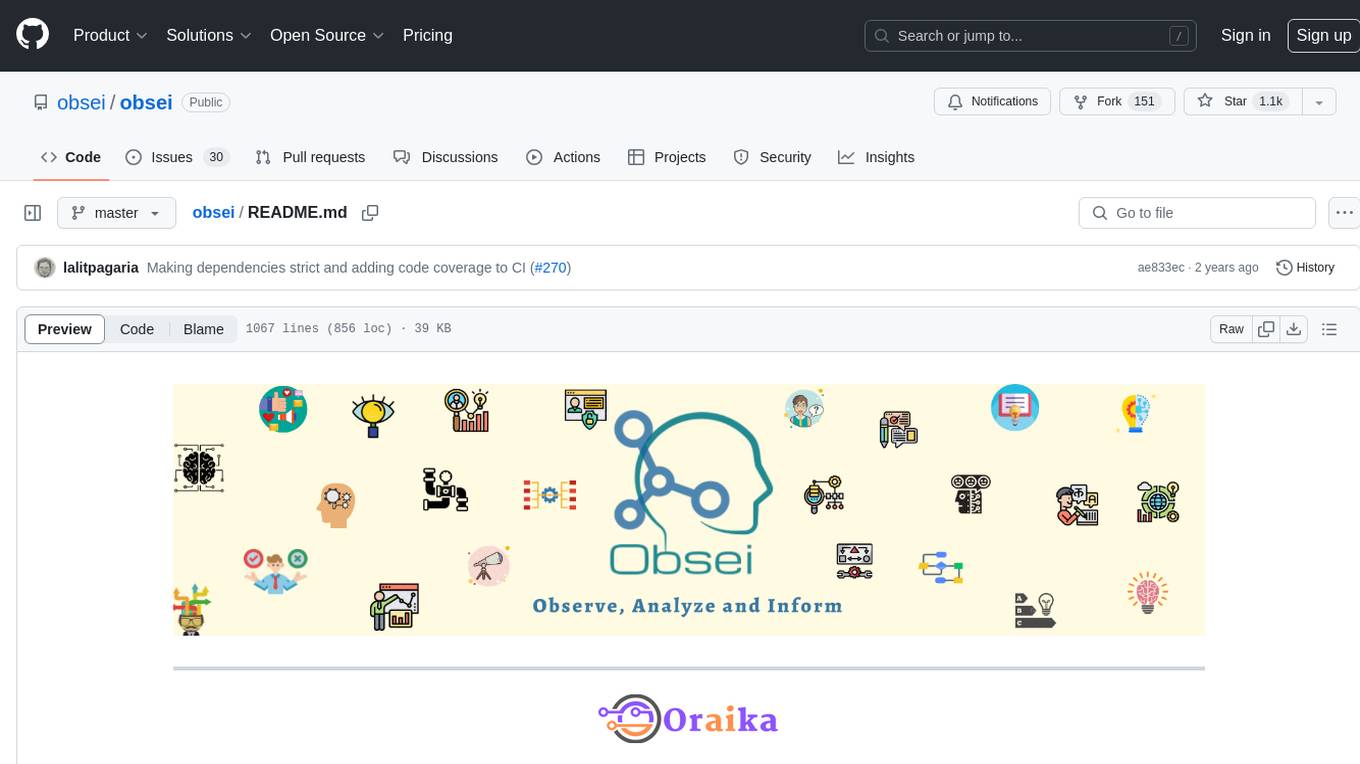
Obsei is an open-source, low-code, AI powered automation tool that consists of an Observer to collect unstructured data from various sources, an Analyzer to analyze the collected data with various AI tasks, and an Informer to send analyzed data to various destinations. The tool is suitable for scheduled jobs or serverless applications as all Observers can store their state in databases. Obsei is still in alpha stage, so caution is advised when using it in production. The tool can be used for social listening, alerting/notification, automatic customer issue creation, extraction of deeper insights from feedbacks, market research, dataset creation for various AI tasks, and more based on creativity.
README:
Note: Obsei is still in alpha stage hence carefully use it in Production. Also, as it is constantly undergoing development hence master branch may contain many breaking changes. Please use released version.
Obsei (pronounced "Ob see" | /əb-'sē/) is an open-source, low-code, AI powered automation tool. Obsei consists of -
- Observer: Collect unstructured data from various sources like tweets from Twitter, Subreddit comments on Reddit, page post's comments from Facebook, App Stores reviews, Google reviews, Amazon reviews, News, Website, etc.
- Analyzer: Analyze unstructured data collected with various AI tasks like classification, sentiment analysis, translation, PII, etc.
- Informer: Send analyzed data to various destinations like ticketing platforms, data storage, dataframe, etc so that the user can take further actions and perform analysis on the data.
All the Observers can store their state in databases (Sqlite, Postgres, MySQL, etc.), making Obsei suitable for scheduled jobs or serverless applications.
- Text, Image, Audio, Documents and Video oriented workflows
- Collect data from every possible private and public channels
- Add every possible workflow to an AI downstream application to automate manual cognitive workflows
Obsei use cases are following, but not limited to -
- Social listening: Listening about social media posts, comments, customer feedback, etc.
- Alerting/Notification: To get auto-alerts for events such as customer complaints, qualified sales leads, etc.
- Automatic customer issue creation based on customer complaints on Social Media, Email, etc.
- Automatic assignment of proper tags to tickets based content of customer complaint for example login issue, sign up issue, delivery issue, etc.
- Extraction of deeper insight from feedbacks on various platforms
- Market research
- Creation of dataset for various AI tasks
- Many more based on creativity 💡
Install the following (if not present already) -
- Install Python 3.7+
- Install PIP
You can install Obsei either via PIP or Conda based on your preference. To install latest released version -
pip install obsei[all]
Install from master branch (if you want to try the latest features) -
git clone https://github.com/obsei/obsei.git
cd obsei
pip install --editable .[all]
Note: all
option will install all the dependencies which might not be needed for your workflow, alternatively
following options are available to install minimal dependencies as per need -
-
pip install obsei[source]
: To install dependencies related to all observers -
pip install obsei[sink]
: To install dependencies related to all informers -
pip install obsei[analyzer]
: To install dependencies related to all analyzers, it will install pytorch as well -
pip install obsei[twitter-api]
: To install dependencies related to Twitter observer -
pip install obsei[google-play-scraper]
: To install dependencies related to Play Store review scrapper observer -
pip install obsei[google-play-api]
: To install dependencies related to Google official play store review API based observer -
pip install obsei[app-store-scraper]
: To install dependencies related to Apple App Store review scrapper observer -
pip install obsei[reddit-scraper]
: To install dependencies related to Reddit post and comment scrapper observer -
pip install obsei[reddit-api]
: To install dependencies related to Reddit official api based observer -
pip install obsei[pandas]
: To install dependencies related to TSV/CSV/Pandas based observer and informer -
pip install obsei[google-news-scraper]
: To install dependencies related to Google news scrapper observer -
pip install obsei[facebook-api]
: To install dependencies related to Facebook official page post and comments api based observer -
pip install obsei[atlassian-api]
: To install dependencies related to Jira official api based informer -
pip install obsei[elasticsearch]
: To install dependencies related to elasticsearch informer -
pip install obsei[slack-api]
:To install dependencies related to Slack official api based informer
You can also mix multiple dependencies together in single installation command. For example to install dependencies Twitter observer, all analyzer, and Slack informer use following command -
pip install obsei[twitter-api, analyzer, slack-api]
Expand the following steps and create a workflow -
Step 1: Configure Source/Observer
Step 2: Configure Analyzer
Note: To run transformers in an offline mode, check transformers offline mode.
Some analyzer support GPU and to utilize pass device parameter. List of possible values of device parameter (default value auto):
- auto: GPU (cuda:0) will be used if available otherwise CPU will be used
- cpu: CPU will be used
- cuda:{id} - GPU will be used with provided CUDA device id
Step 3: Configure Sink/Informer
Step 4: Join and create workflow
source
will fetch data from the selected source, then feed it to the analyzer
for processing, whose output we feed into a sink
to get notified at that sink.
# Uncomment if you want logger
# import logging
# import sys
# logger = logging.getLogger(__name__)
# logging.basicConfig(stream=sys.stdout, level=logging.INFO)
# This will fetch information from configured source ie twitter, app store etc
source_response_list = source.lookup(source_config)
# Uncomment if you want to log source response
# for idx, source_response in enumerate(source_response_list):
# logger.info(f"source_response#'{idx}'='{source_response.__dict__}'")
# This will execute analyzer (Sentiment, classification etc) on source data with provided analyzer_config
analyzer_response_list = text_analyzer.analyze_input(
source_response_list=source_response_list,
analyzer_config=analyzer_config
)
# Uncomment if you want to log analyzer response
# for idx, an_response in enumerate(analyzer_response_list):
# logger.info(f"analyzer_response#'{idx}'='{an_response.__dict__}'")
# Analyzer output added to segmented_data
# Uncomment to log it
# for idx, an_response in enumerate(analyzer_response_list):
# logger.info(f"analyzed_data#'{idx}'='{an_response.segmented_data.__dict__}'")
# This will send analyzed output to configure sink ie Slack, Zendesk etc
sink_response_list = sink.send_data(analyzer_response_list, sink_config)
# Uncomment if you want to log sink response
# for sink_response in sink_response_list:
# if sink_response is not None:
# logger.info(f"sink_response='{sink_response}'")
Step 5: Execute workflow
Copy the code snippets from Steps 1 to 4 into a python file, for exampleexample.py
and execute the following command -
python example.py
We have a minimal streamlit based UI that you can use to test Obsei.
(Note: Sometimes the Streamlit demo might not work due to rate limiting, use the docker image (locally) in such cases.)
To test locally, just run
docker run -d --name obesi-ui -p 8501:8501 obsei/obsei-ui-demo
# You can find the UI at http://localhost:8501
To run Obsei workflow easily using GitHub Actions (no sign ups and cloud hosting required), refer to this repo.
Here are some companies/projects (alphabetical order) using Obsei. To add your company/project to the list, please raise a PR or contact us via email.
- Oraika: Contextually understand customer feedback
- 1Page: Giving a better context in meetings and calls
- Spacepulse: The operating system for spaces
- Superblog: A blazing fast alternative to WordPress and Medium
- Zolve: Creating a financial world beyond borders
- Utilize: No-code app builder for businesses with a deskless workforce
Sr. No. | Title | Author |
---|---|---|
1 | AI based Comparative Customer Feedback Analysis Using Obsei | Reena Bapna |
2 | LinkedIn App - User Feedback Analysis | Himanshu Sharma |
For detailed installation instructions, usages and examples, refer to our documentation.
Linux | Mac | Windows | Remark | |
---|---|---|---|---|
Tests | ✅ | ✅ | ✅ | Low Coverage as difficult to test 3rd party libs |
PIP | ✅ | ✅ | ✅ | Fully Supported |
Conda | ❌ | ❌ | ❌ | Not Supported |
Discussion about Obsei can be done at community forum
Refer releases for changelogs
For any security issue please contact us via email
This project is being maintained by Oraika Technologies. Lalit Pagaria and Girish Patel are maintainers of this project.
- Copyright holder: Oraika Technologies
- Overall Apache 2.0 and you can read License file.
- Multiple other secondary permissive or weak copyleft licenses (LGPL, MIT, BSD etc.) for third-party components refer Attribution.
- To make project more commercial friendly, we void third party components which have strong copyleft licenses (GPL, AGPL etc.) into the project.
This could not have been possible without these open source softwares.
First off, thank you for even considering contributing to this package, every contribution big or small is greatly appreciated. Please refer our Contribution Guideline and Code of Conduct.
Thanks so much to all our contributors
For Tasks:
Click tags to check more tools for each tasksFor Jobs:
Alternative AI tools for obsei
Similar Open Source Tools
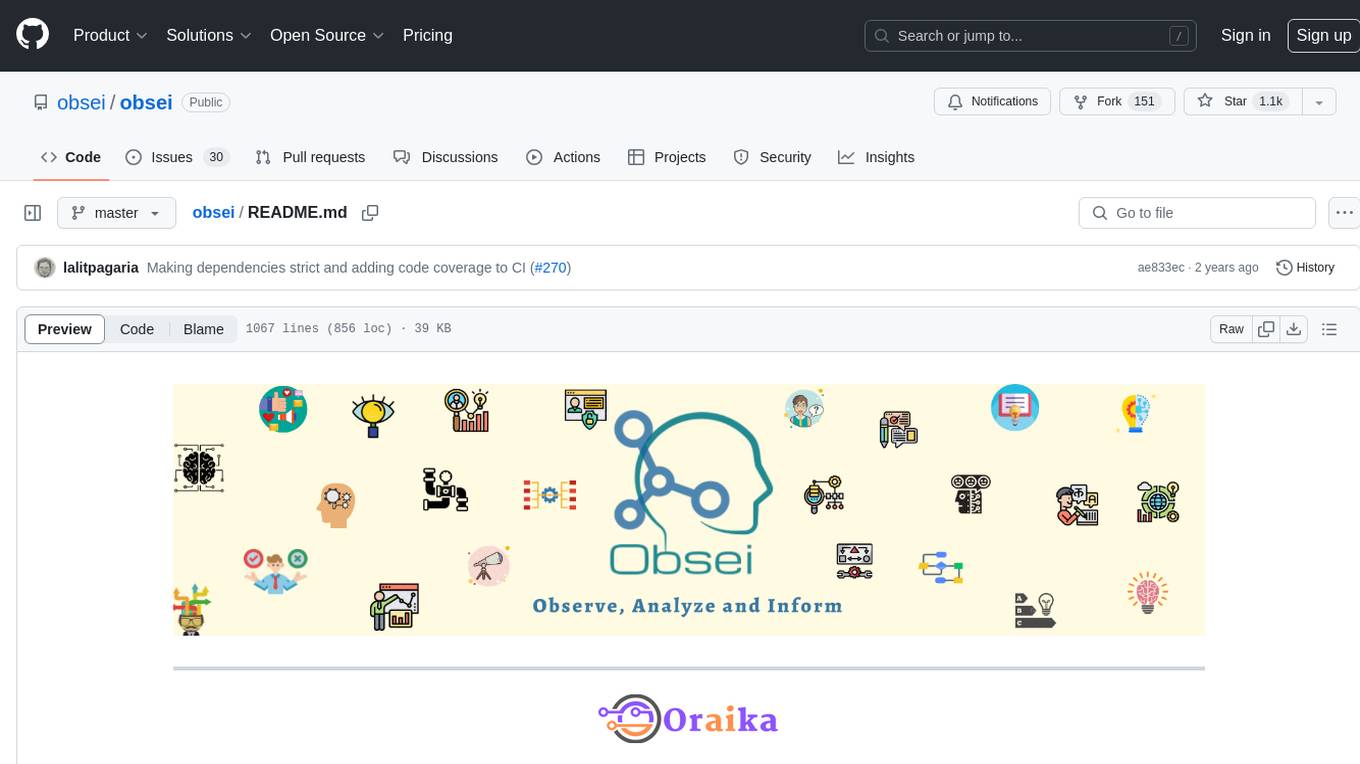
obsei
Obsei is an open-source, low-code, AI powered automation tool that consists of an Observer to collect unstructured data from various sources, an Analyzer to analyze the collected data with various AI tasks, and an Informer to send analyzed data to various destinations. The tool is suitable for scheduled jobs or serverless applications as all Observers can store their state in databases. Obsei is still in alpha stage, so caution is advised when using it in production. The tool can be used for social listening, alerting/notification, automatic customer issue creation, extraction of deeper insights from feedbacks, market research, dataset creation for various AI tasks, and more based on creativity.
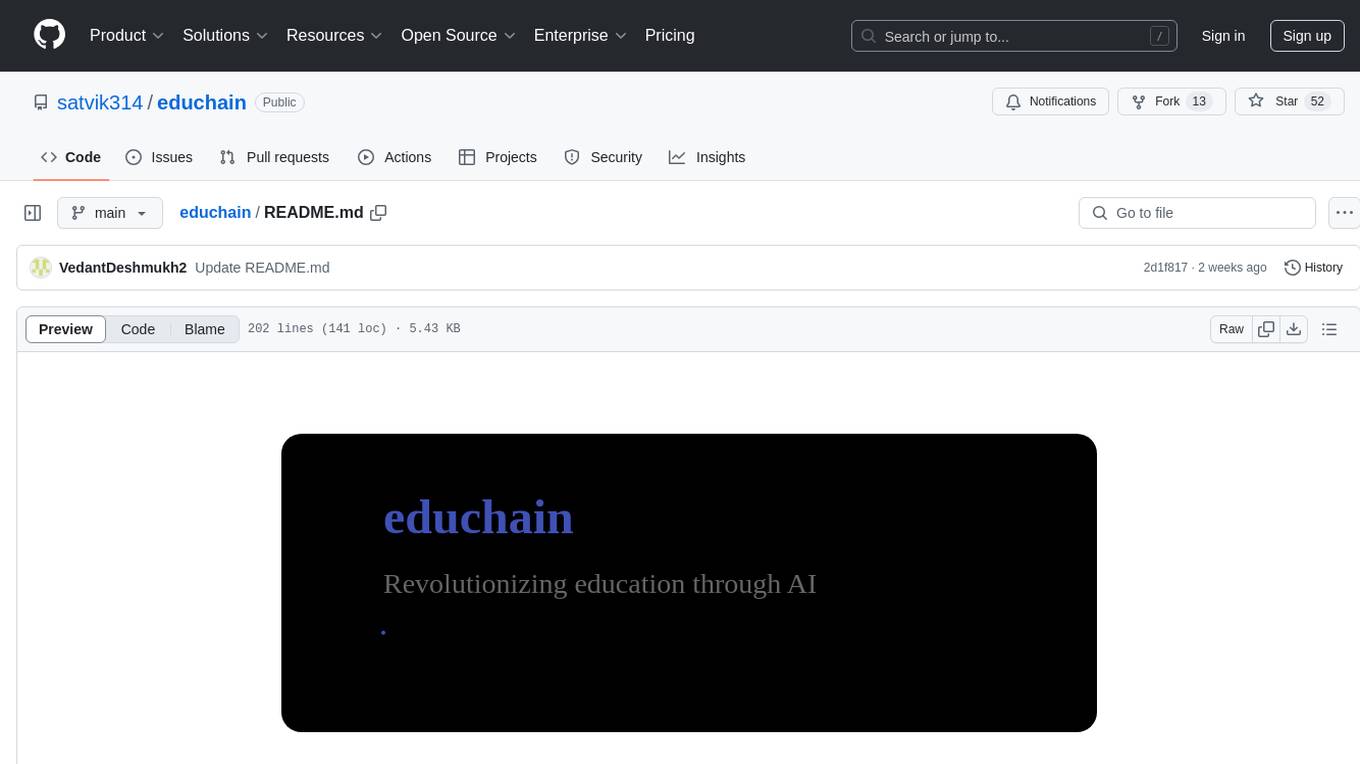
educhain
Educhain is a powerful Python package that leverages Generative AI to create engaging and personalized educational content. It enables users to generate multiple-choice questions, create lesson plans, and support various LLM models. Users can export questions to JSON, PDF, and CSV formats, customize prompt templates, and generate questions from text, PDF, URL files, youtube videos, and images. Educhain outperforms traditional methods in content generation speed and quality. It offers advanced configuration options and has a roadmap for future enhancements, including integration with popular Learning Management Systems and a mobile app for content generation on-the-go.
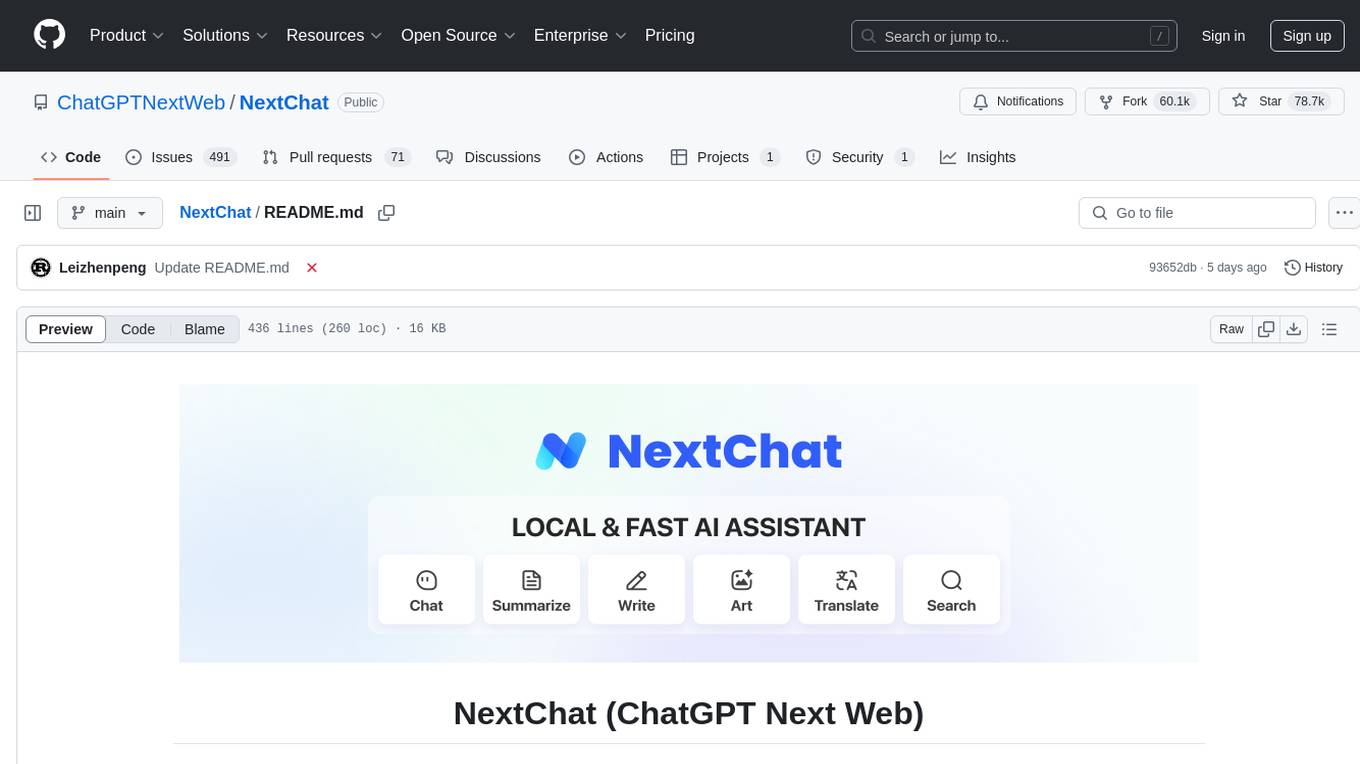
NextChat
NextChat is a well-designed cross-platform ChatGPT web UI tool that supports Claude, GPT4, and Gemini Pro. It offers a compact client for Linux, Windows, and MacOS, with features like self-deployed LLMs compatibility, privacy-first data storage, markdown support, responsive design, and fast loading speed. Users can create, share, and debug chat tools with prompt templates, access various prompts, compress chat history, and use multiple languages. The tool also supports enterprise-level privatization and customization deployment, with features like brand customization, resource integration, permission control, knowledge integration, security auditing, private deployment, and continuous updates.
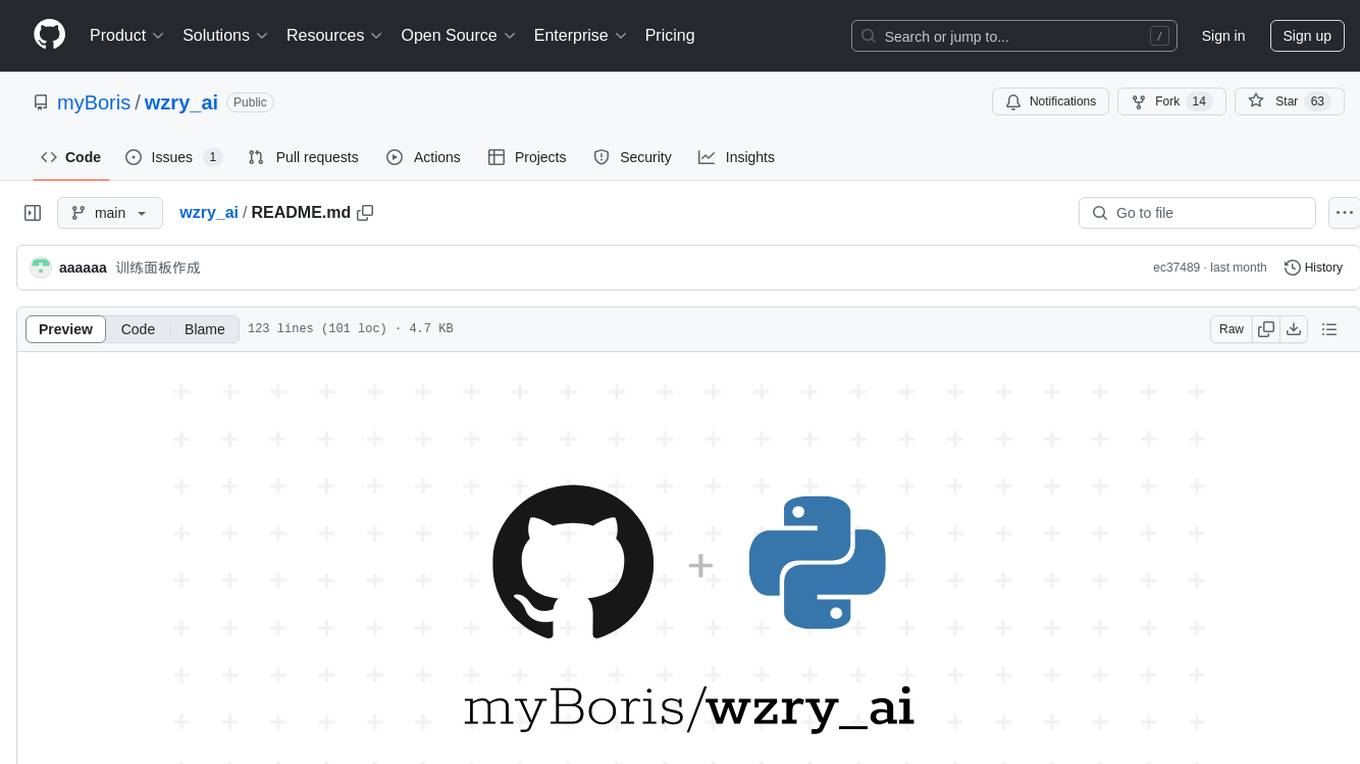
wzry_ai
This is an open-source project for playing the game King of Glory with an artificial intelligence model. The first phase of the project has been completed, and future upgrades will be built upon this foundation. The second phase of the project has started, and progress is expected to proceed according to plan. For any questions, feel free to join the QQ exchange group: 687853827. The project aims to learn artificial intelligence and strictly prohibits cheating. Detailed installation instructions are available in the doc/README.md file. Environment installation video: (bilibili) Welcome to follow, like, tip, comment, and provide your suggestions.
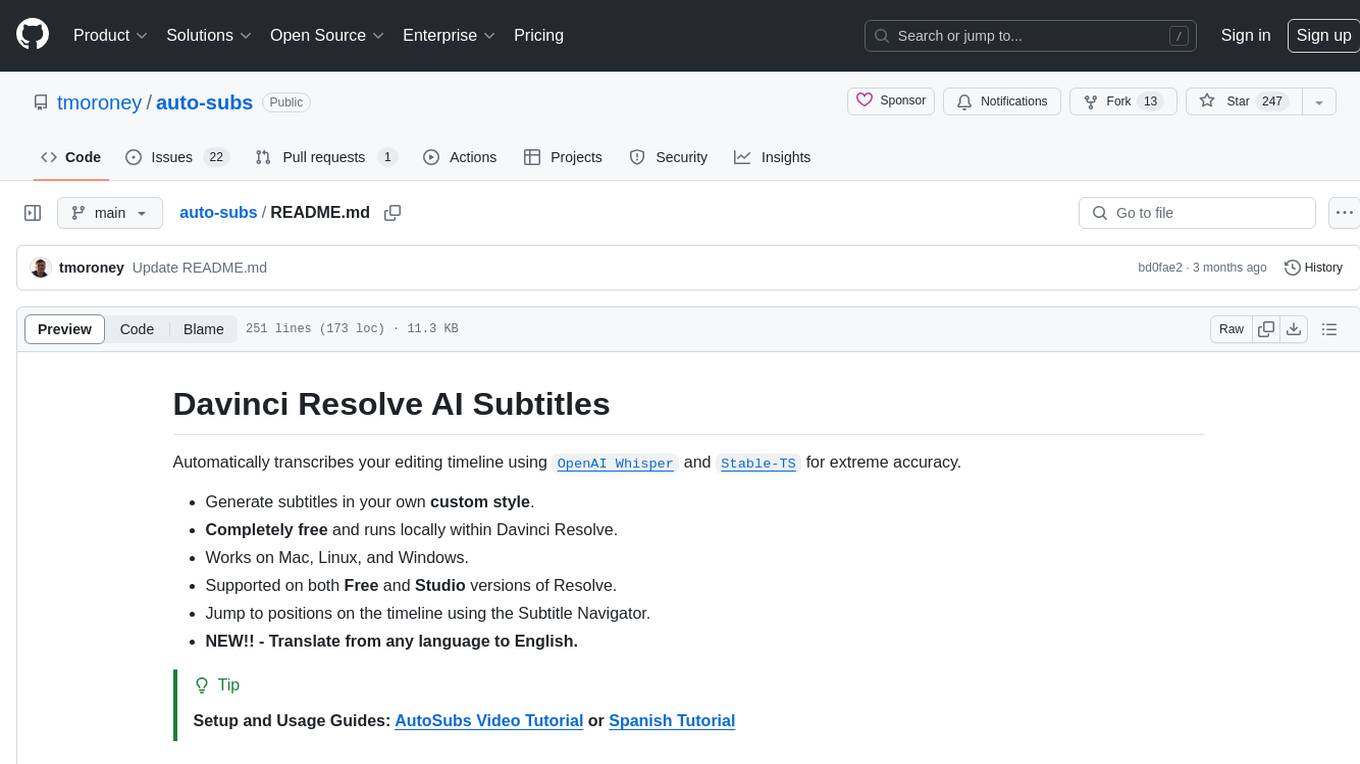
auto-subs
Auto-subs is a tool designed to automatically transcribe editing timelines using OpenAI Whisper and Stable-TS for extreme accuracy. It generates subtitles in a custom style, is completely free, and runs locally within Davinci Resolve. It works on Mac, Linux, and Windows, supporting both Free and Studio versions of Resolve. Users can jump to positions on the timeline using the Subtitle Navigator and translate from any language to English. The tool provides a user-friendly interface for creating and customizing subtitles for video content.
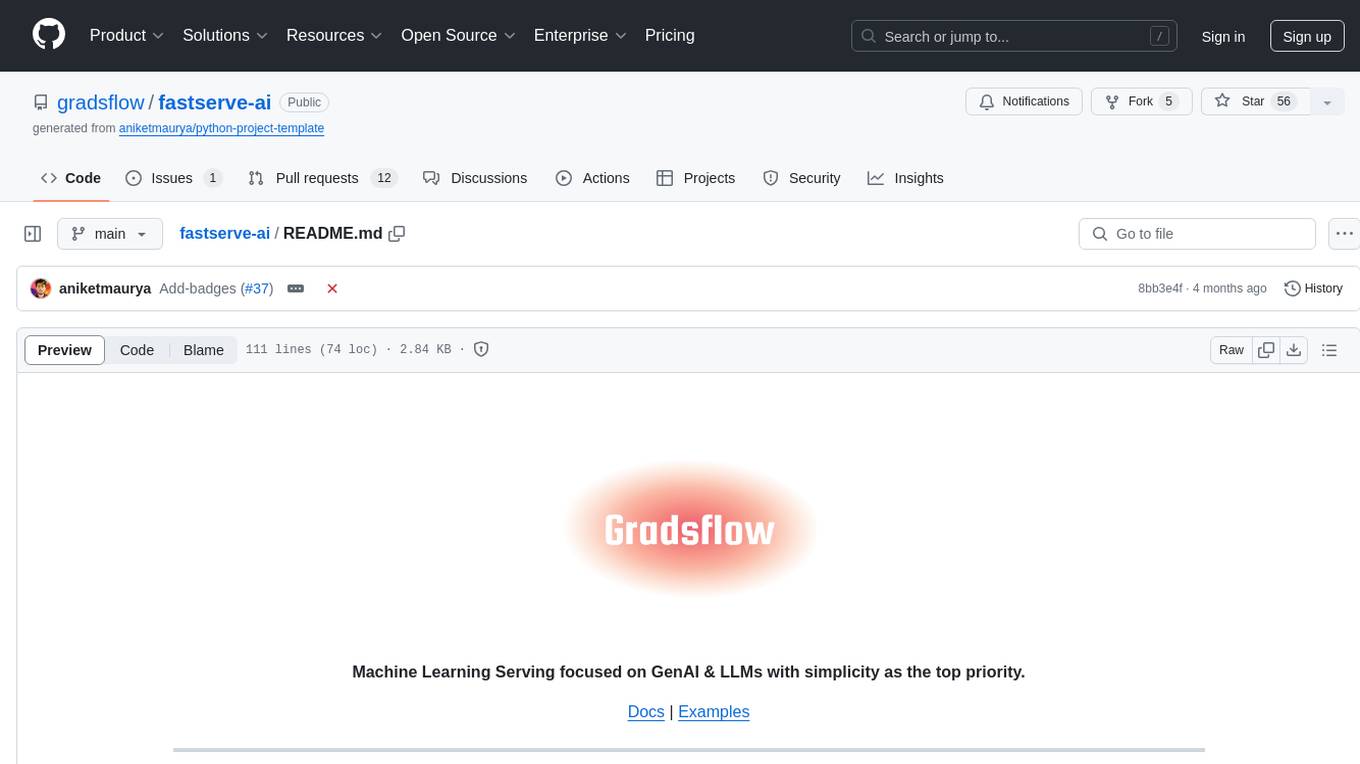
fastserve-ai
FastServe-AI is a machine learning serving tool focused on GenAI & LLMs with simplicity as the top priority. It allows users to easily serve custom models by implementing the 'handle' method for 'FastServe'. The tool provides a FastAPI server for custom models and can be deployed using Lightning AI Studio. Users can install FastServe-AI via pip and run it to serve their own GPT-like LLM models in minutes.
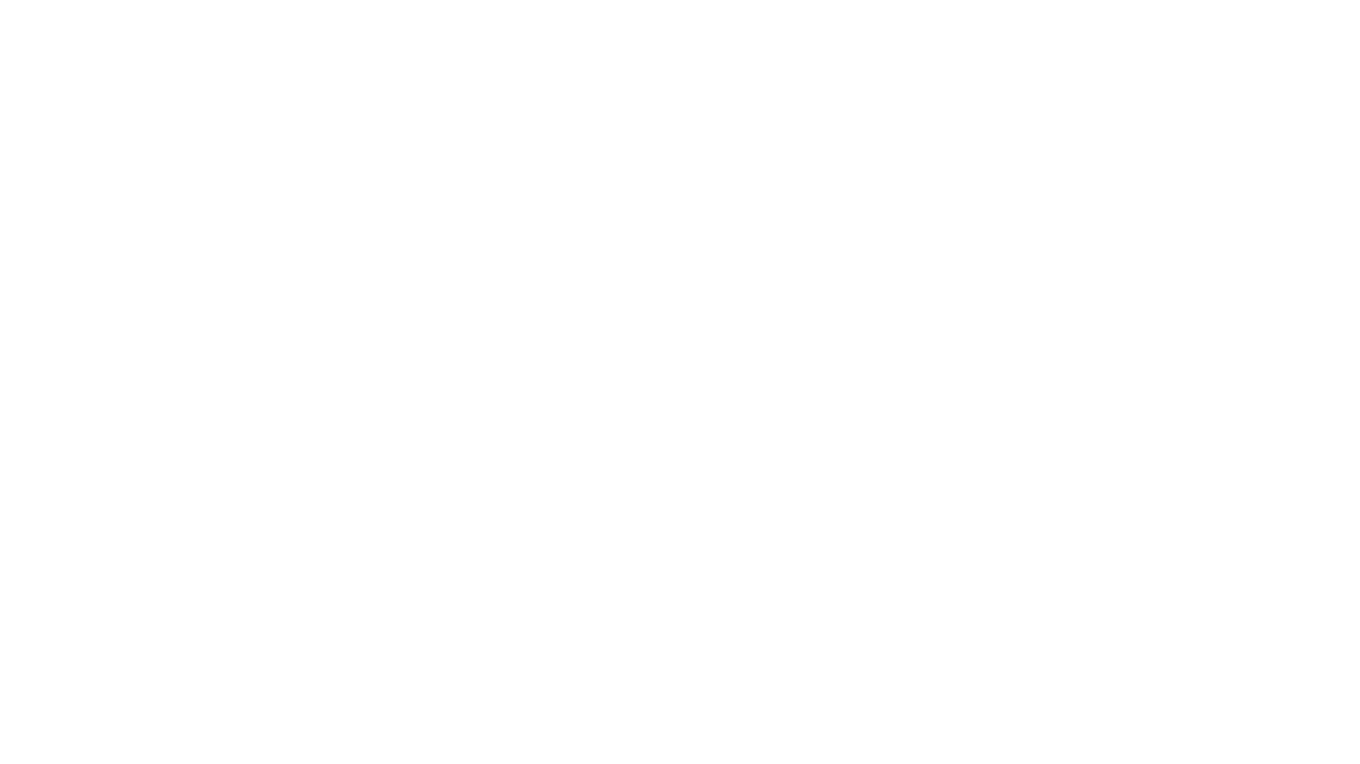
ebook2audiobook
ebook2audiobook is a CPU/GPU converter tool that converts eBooks to audiobooks with chapters and metadata using tools like Calibre, ffmpeg, XTTSv2, and Fairseq. It supports voice cloning and a wide range of languages. The tool is designed to run on 4GB RAM and provides a new v2.0 Web GUI interface for user-friendly interaction. Users can convert eBooks to text format, split eBooks into chapters, and utilize high-quality text-to-speech functionalities. Supported languages include Arabic, Chinese, English, French, German, Hindi, and many more. The tool can be used for legal, non-DRM eBooks only and should be used responsibly in compliance with applicable laws.
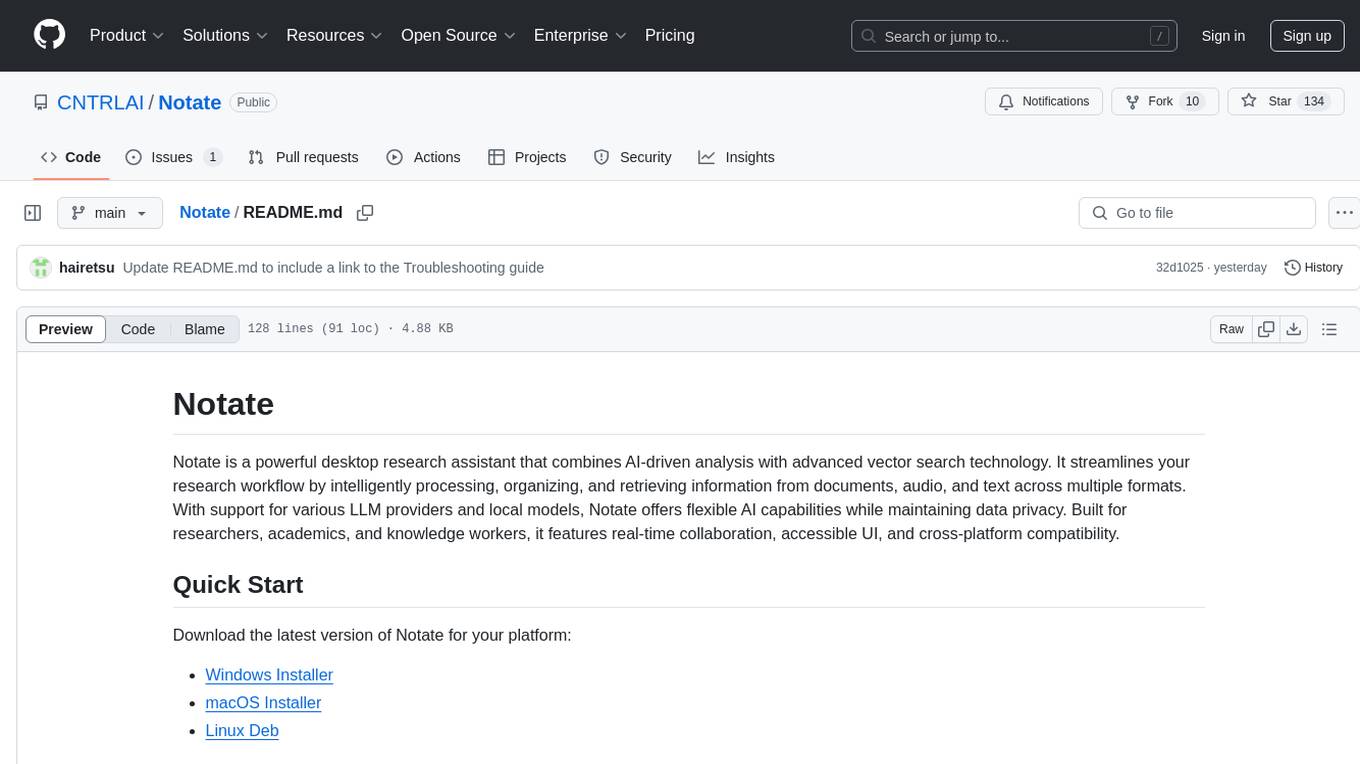
Notate
Notate is a powerful desktop research assistant that combines AI-driven analysis with advanced vector search technology. It streamlines research workflow by processing, organizing, and retrieving information from documents, audio, and text. Notate offers flexible AI capabilities with support for various LLM providers and local models, ensuring data privacy. Built for researchers, academics, and knowledge workers, it features real-time collaboration, accessible UI, and cross-platform compatibility.
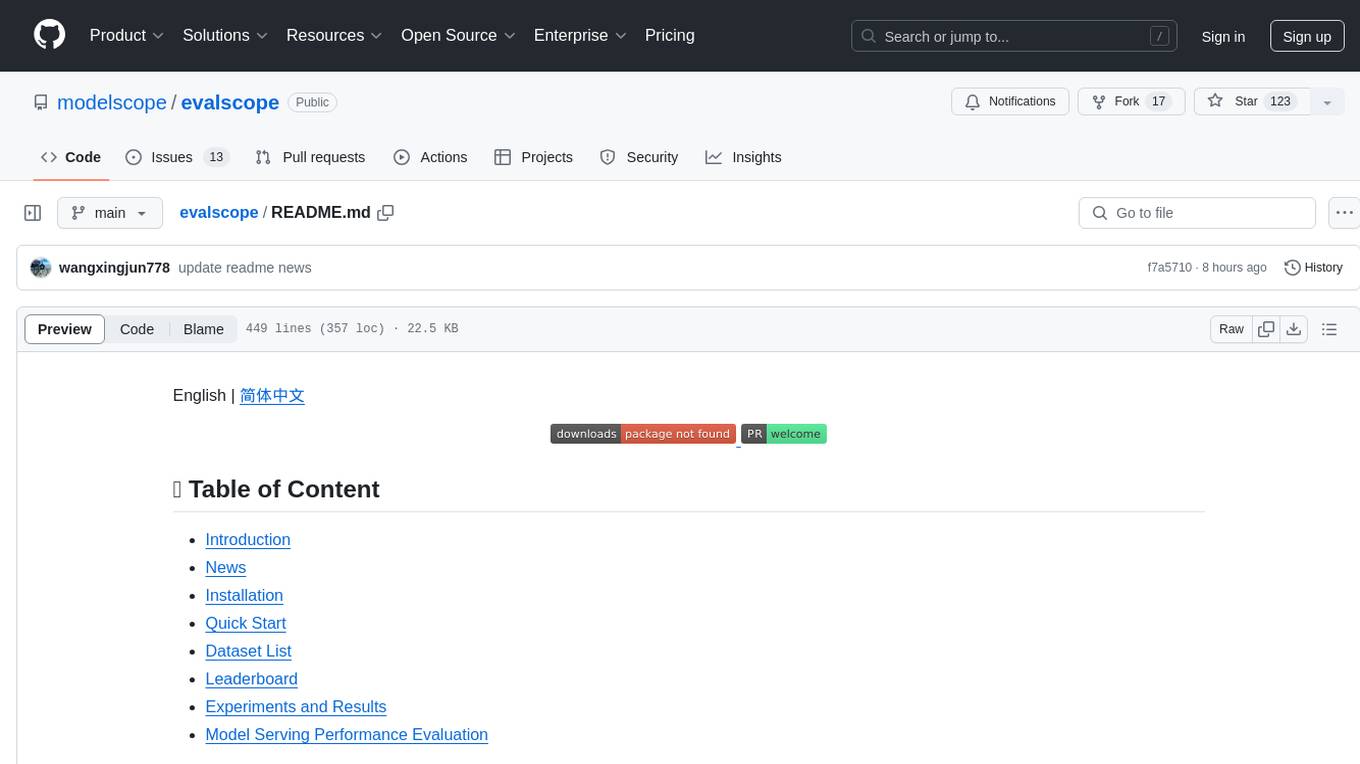
evalscope
Eval-Scope is a framework designed to support the evaluation of large language models (LLMs) by providing pre-configured benchmark datasets, common evaluation metrics, model integration, automatic evaluation for objective questions, complex task evaluation using expert models, reports generation, visualization tools, and model inference performance evaluation. It is lightweight, easy to customize, supports new dataset integration, model hosting on ModelScope, deployment of locally hosted models, and rich evaluation metrics. Eval-Scope also supports various evaluation modes like single mode, pairwise-baseline mode, and pairwise (all) mode, making it suitable for assessing and improving LLMs.
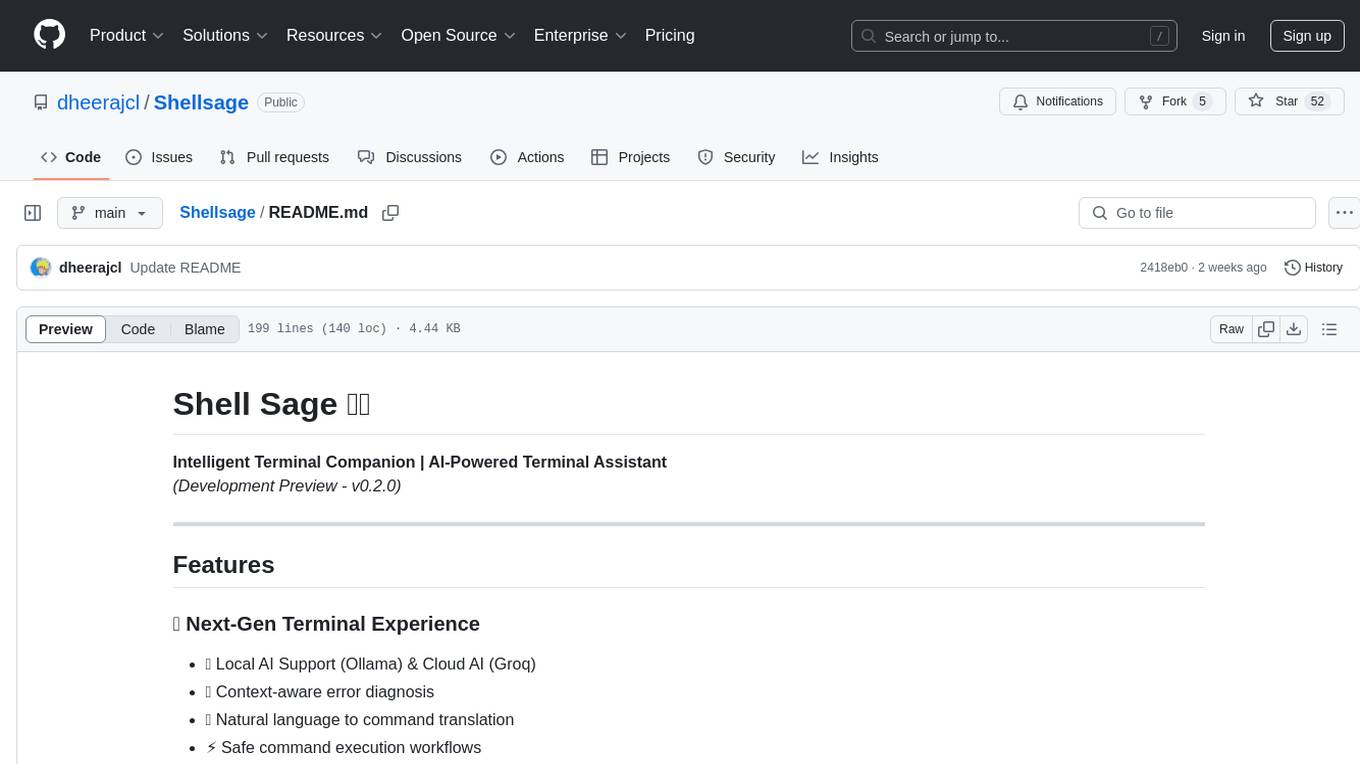
Shellsage
Shell Sage is an intelligent terminal companion and AI-powered terminal assistant that enhances the terminal experience with features like local and cloud AI support, context-aware error diagnosis, natural language to command translation, and safe command execution workflows. It offers interactive workflows, supports various API providers, and allows for custom model selection. Users can configure the tool for local or API mode, select specific models, and switch between modes easily. Currently in alpha development, Shell Sage has known limitations like limited Windows support and occasional false positives in error detection. The roadmap includes improvements like better context awareness, Windows PowerShell integration, Tmux integration, and CI/CD error pattern database.
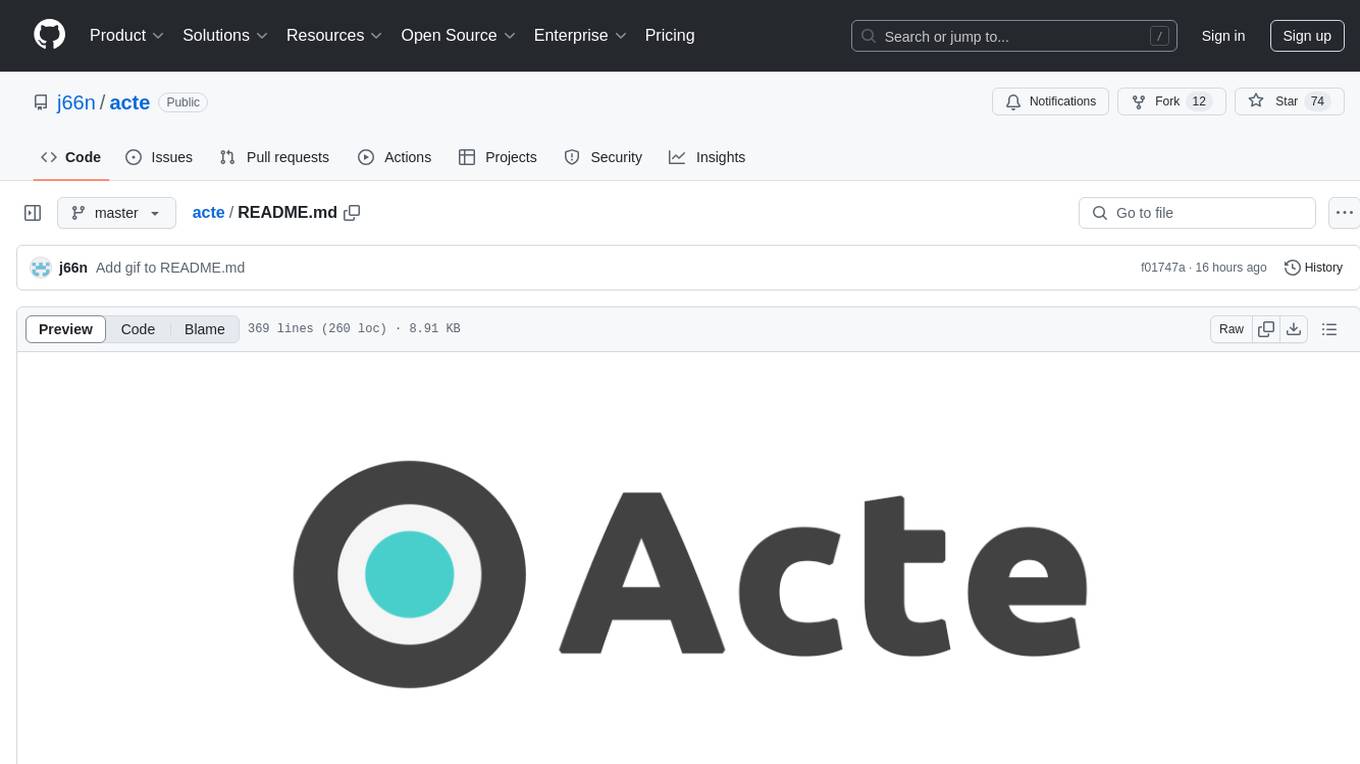
acte
Acte is a framework designed to build GUI-like tools for AI Agents. It aims to address the issues of cognitive load and freedom degrees when interacting with multiple APIs in complex scenarios. By providing a graphical user interface (GUI) for Agents, Acte helps reduce cognitive load and constraints interaction, similar to how humans interact with computers through GUIs. The tool offers APIs for starting new sessions, executing actions, and displaying screens, accessible via HTTP requests or the SessionManager class.
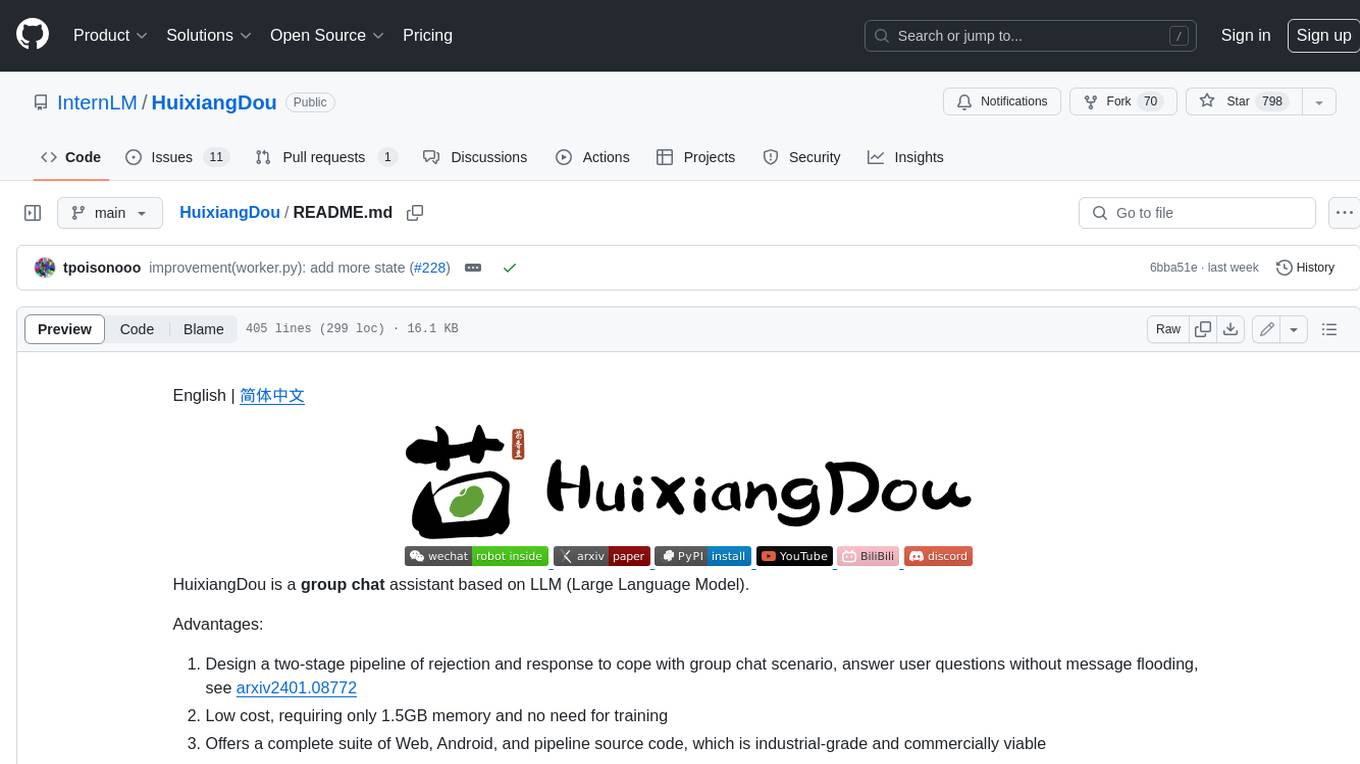
HuixiangDou
HuixiangDou is a **group chat** assistant based on LLM (Large Language Model). Advantages: 1. Design a two-stage pipeline of rejection and response to cope with group chat scenario, answer user questions without message flooding, see arxiv2401.08772 2. Low cost, requiring only 1.5GB memory and no need for training 3. Offers a complete suite of Web, Android, and pipeline source code, which is industrial-grade and commercially viable Check out the scenes in which HuixiangDou are running and join WeChat Group to try AI assistant inside. If this helps you, please give it a star ⭐
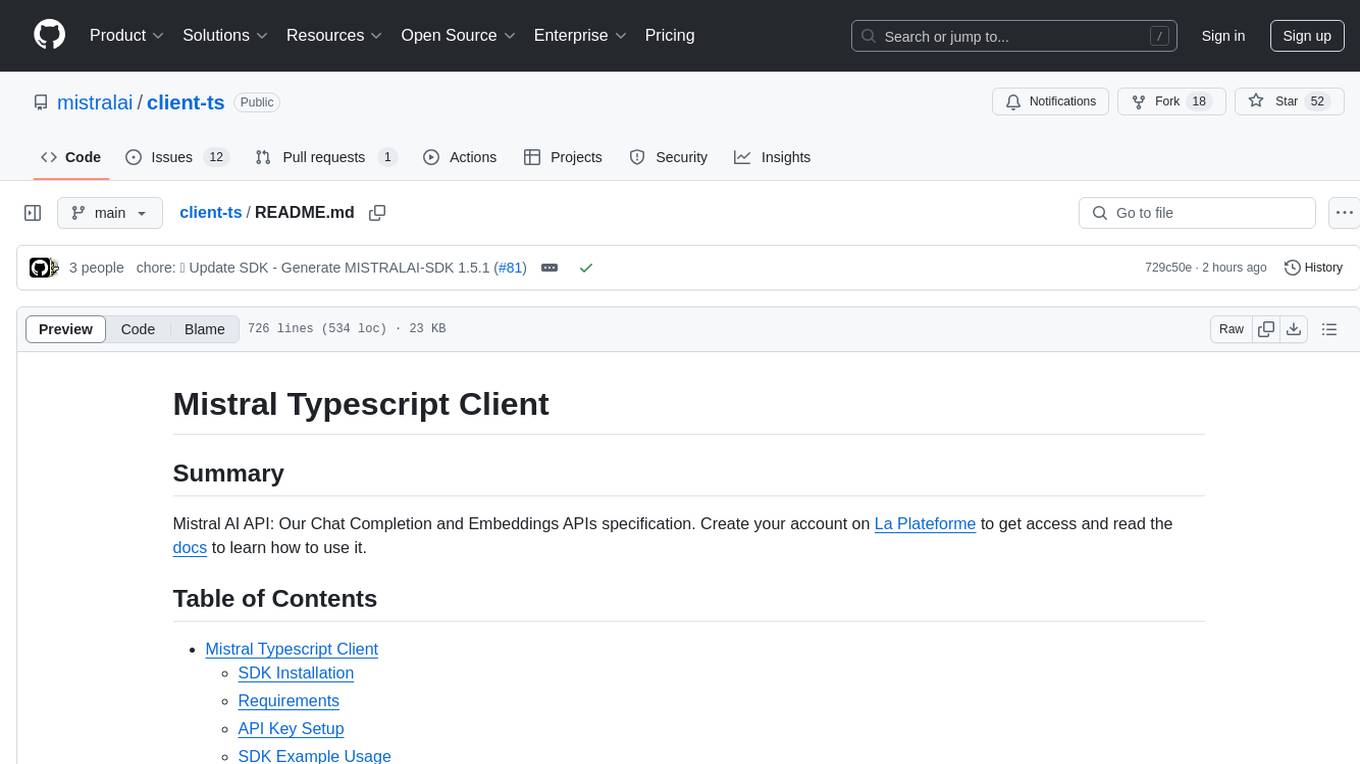
client-ts
Mistral Typescript Client is an SDK for Mistral AI API, providing Chat Completion and Embeddings APIs. It allows users to create chat completions, upload files, create agent completions, create embedding requests, and more. The SDK supports various JavaScript runtimes and provides detailed documentation on installation, requirements, API key setup, example usage, error handling, server selection, custom HTTP client, authentication, providers support, standalone functions, debugging, and contributions.
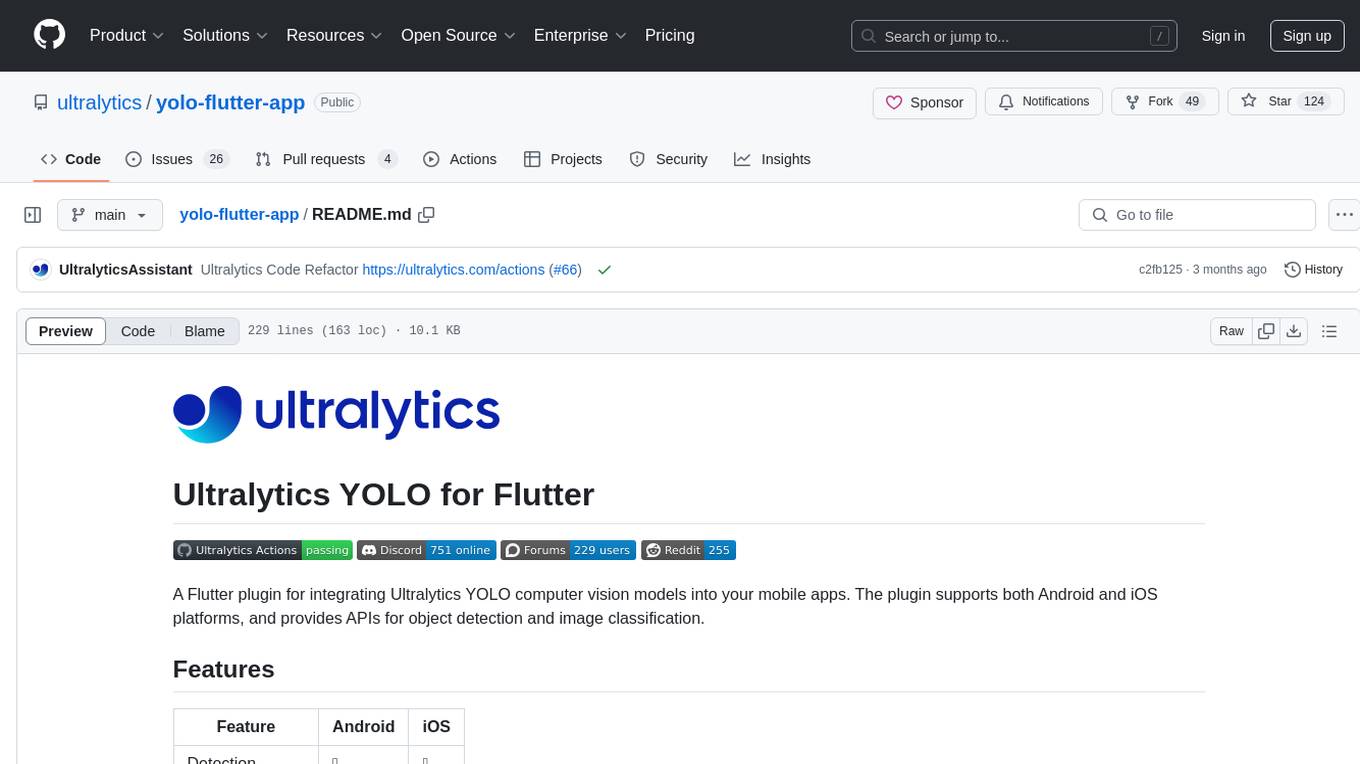
yolo-flutter-app
Ultralytics YOLO for Flutter is a Flutter plugin that allows you to integrate Ultralytics YOLO computer vision models into your mobile apps. It supports both Android and iOS platforms, providing APIs for object detection and image classification. The plugin leverages Flutter Platform Channels for seamless communication between the client and host, handling all processing natively. Before using the plugin, you need to export the required models in `.tflite` and `.mlmodel` formats. The plugin provides support for tasks like detection and classification, with specific instructions for Android and iOS platforms. It also includes features like camera preview and methods for object detection and image classification on images. Ultralytics YOLO thrives on community collaboration and offers different licensing paths for open-source and commercial use cases.
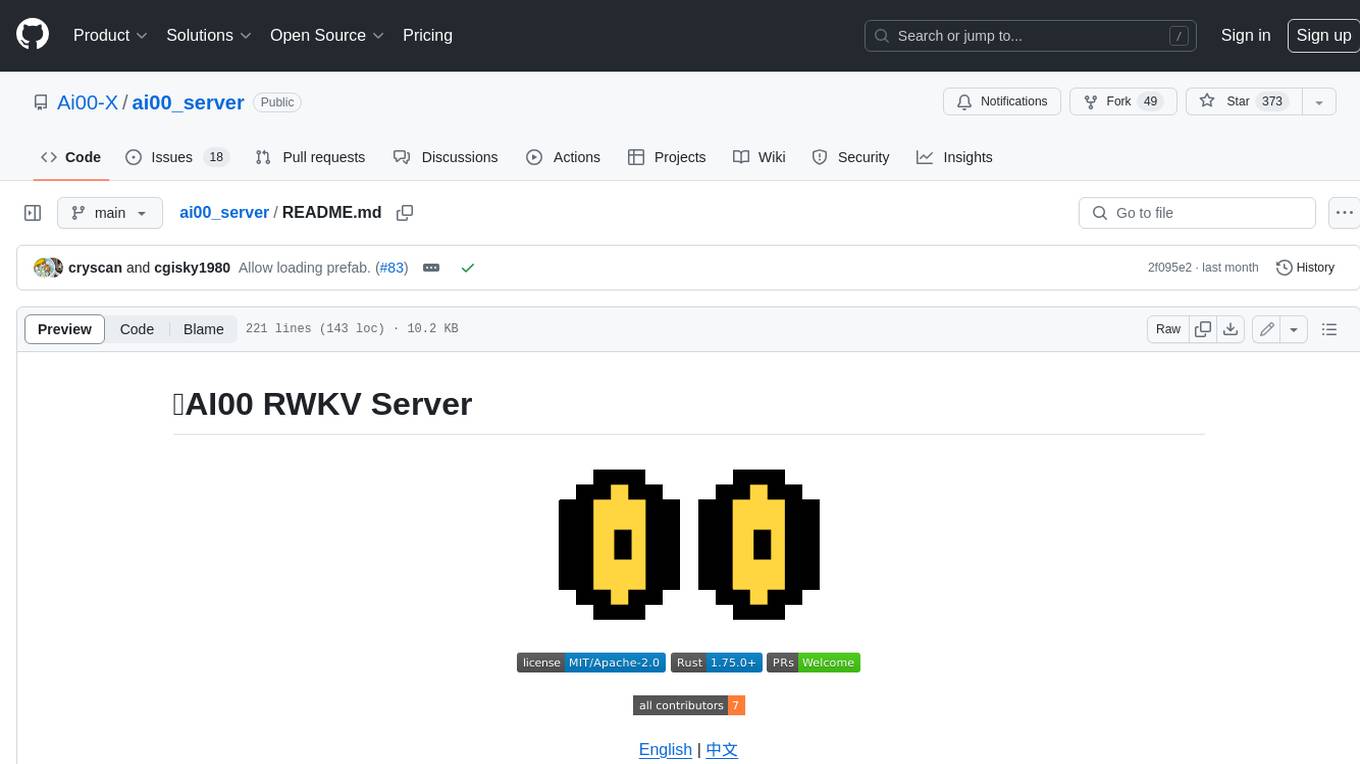
ai00_server
AI00 RWKV Server is an inference API server for the RWKV language model based upon the web-rwkv inference engine. It supports VULKAN parallel and concurrent batched inference and can run on all GPUs that support VULKAN. No need for Nvidia cards!!! AMD cards and even integrated graphics can be accelerated!!! No need for bulky pytorch, CUDA and other runtime environments, it's compact and ready to use out of the box! Compatible with OpenAI's ChatGPT API interface. 100% open source and commercially usable, under the MIT license. If you are looking for a fast, efficient, and easy-to-use LLM API server, then AI00 RWKV Server is your best choice. It can be used for various tasks, including chatbots, text generation, translation, and Q&A.
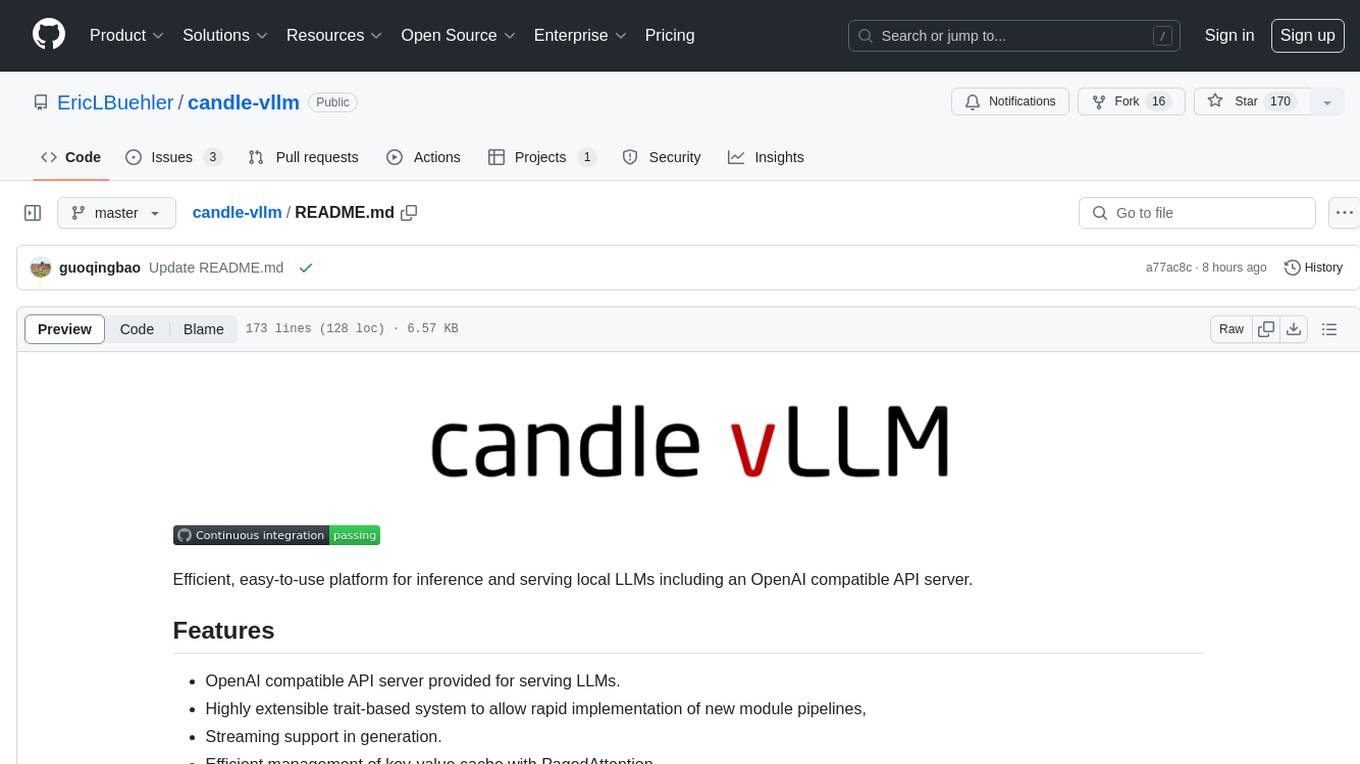
candle-vllm
Candle-vllm is an efficient and easy-to-use platform designed for inference and serving local LLMs, featuring an OpenAI compatible API server. It offers a highly extensible trait-based system for rapid implementation of new module pipelines, streaming support in generation, efficient management of key-value cache with PagedAttention, and continuous batching. The tool supports chat serving for various models and provides a seamless experience for users to interact with LLMs through different interfaces.
For similar tasks
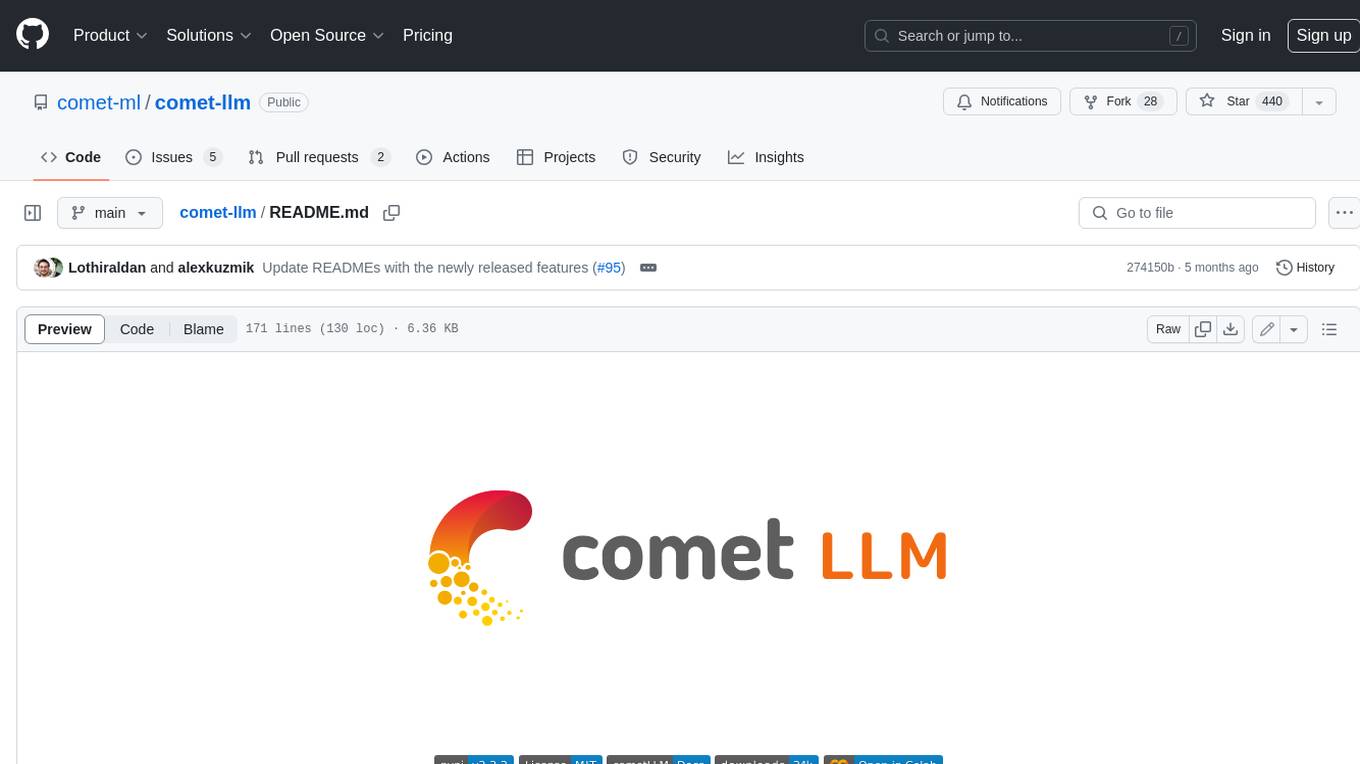
comet-llm
CometLLM is a tool to log and visualize your LLM prompts and chains. Use CometLLM to identify effective prompt strategies, streamline your troubleshooting, and ensure reproducible workflows!
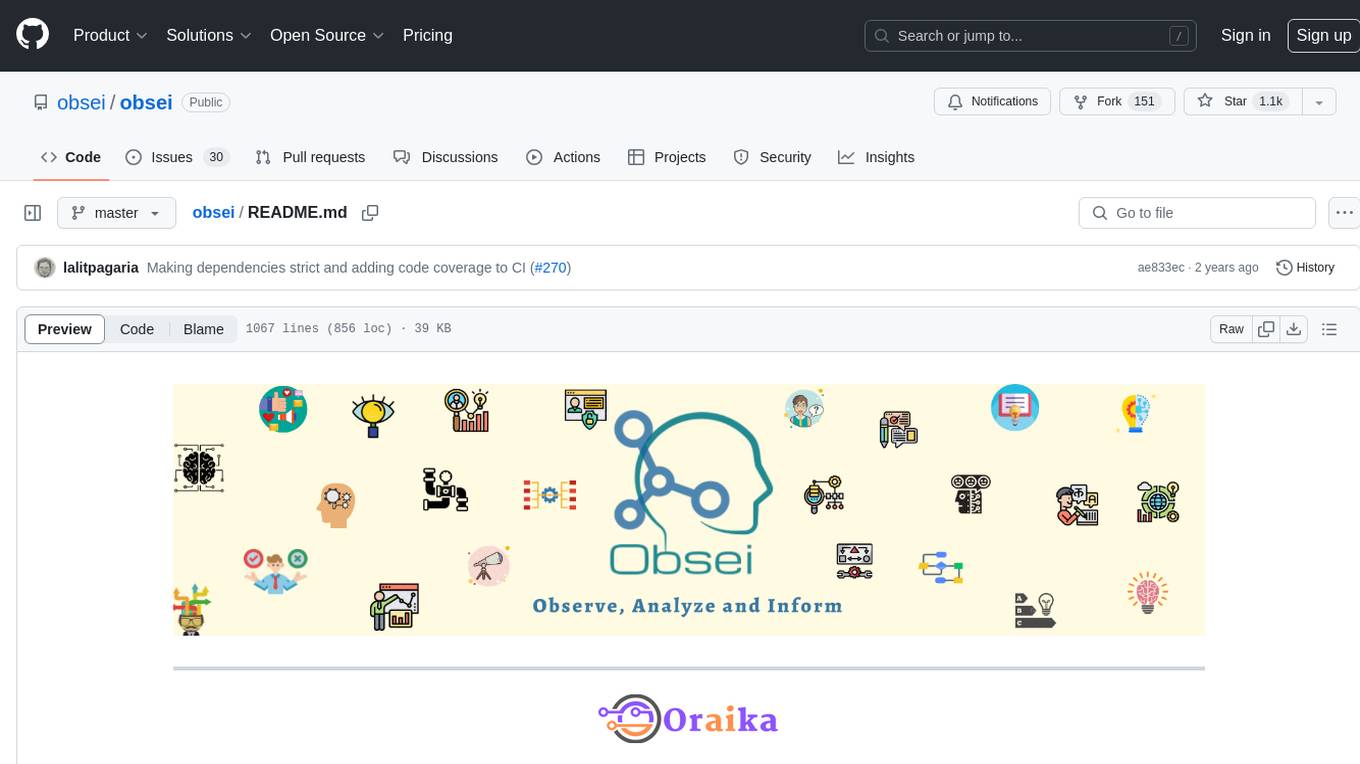
obsei
Obsei is an open-source, low-code, AI powered automation tool that consists of an Observer to collect unstructured data from various sources, an Analyzer to analyze the collected data with various AI tasks, and an Informer to send analyzed data to various destinations. The tool is suitable for scheduled jobs or serverless applications as all Observers can store their state in databases. Obsei is still in alpha stage, so caution is advised when using it in production. The tool can be used for social listening, alerting/notification, automatic customer issue creation, extraction of deeper insights from feedbacks, market research, dataset creation for various AI tasks, and more based on creativity.
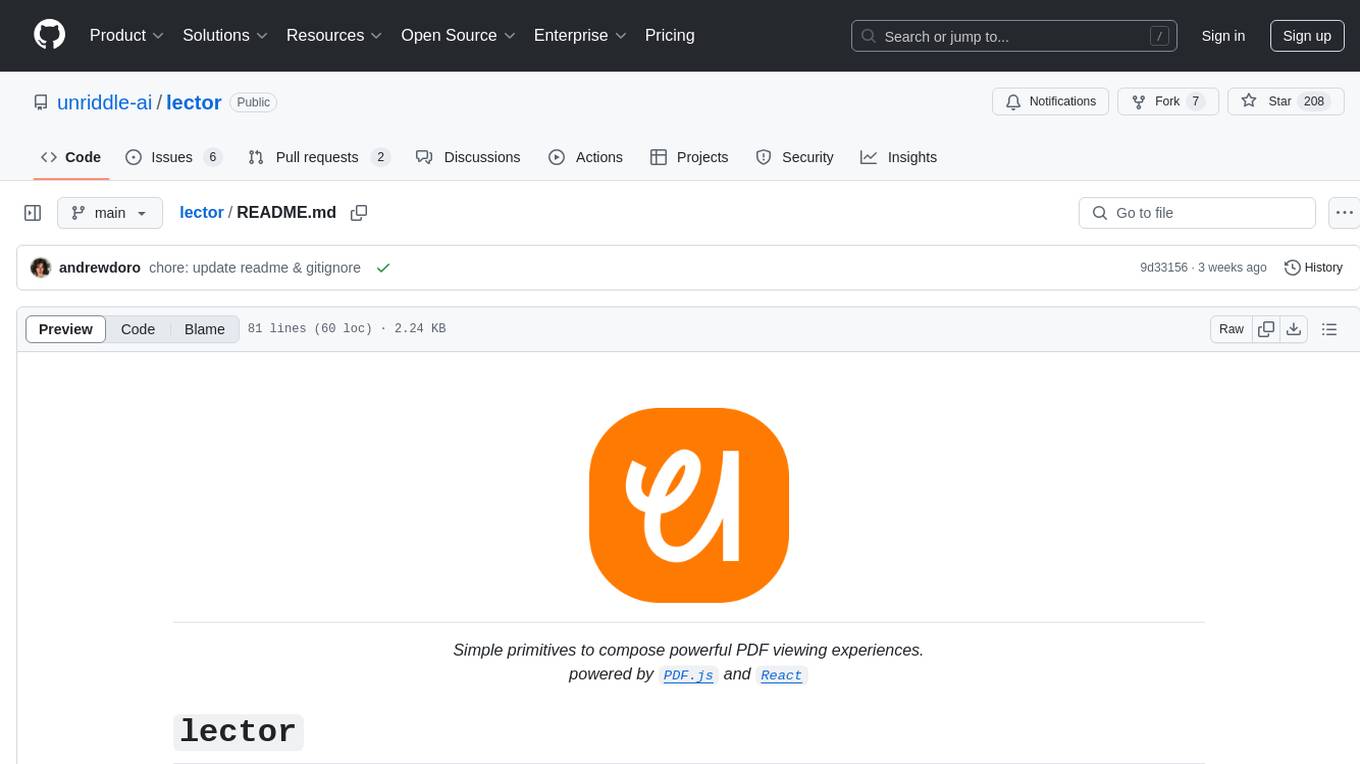
lector
Lector is a text analysis tool that helps users extract insights from unstructured text data. It provides functionalities such as sentiment analysis, keyword extraction, entity recognition, and text summarization. With Lector, users can easily analyze large volumes of text data to uncover patterns, trends, and valuable information. The tool is designed to be user-friendly and efficient, making it suitable for both beginners and experienced users in the field of natural language processing and text mining.
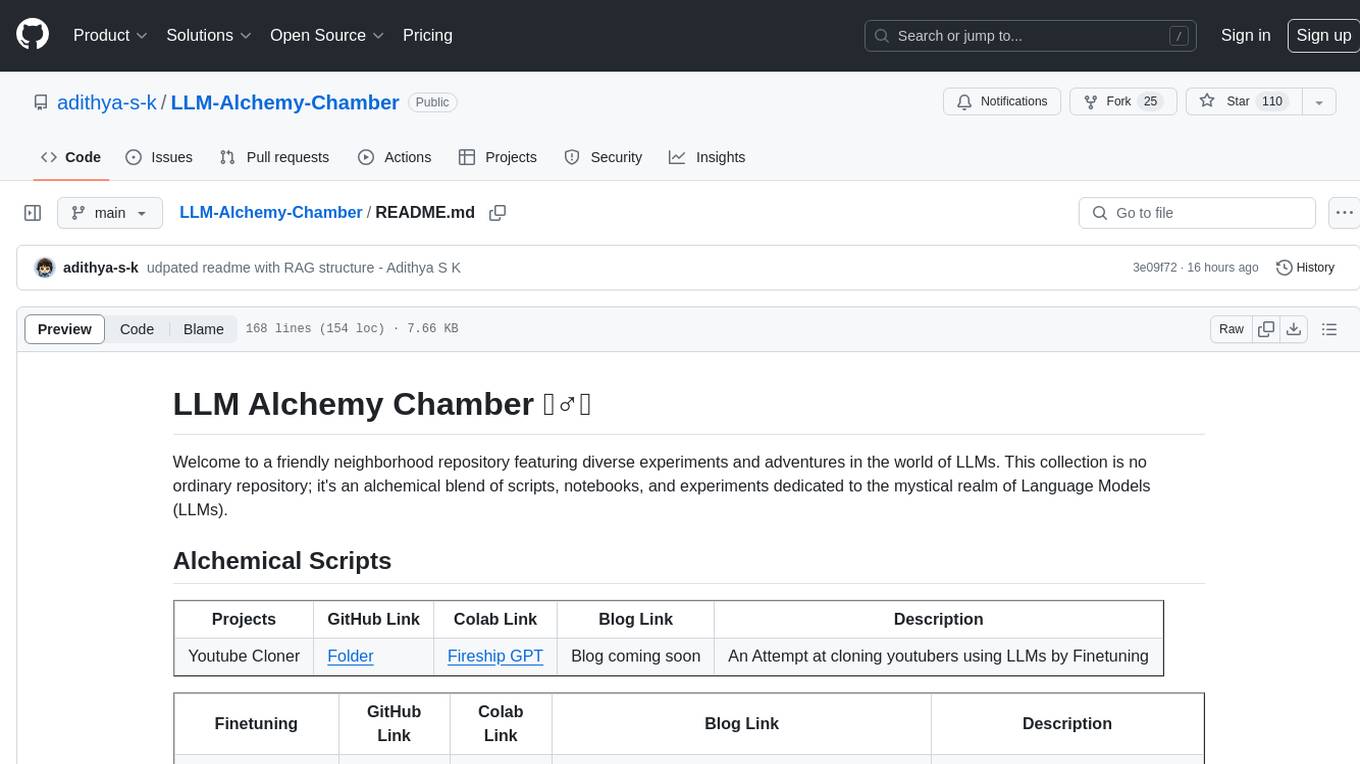
LLM-Alchemy-Chamber
LLM Alchemy Chamber is a repository dedicated to exploring the world of Language Models (LLMs) through various experiments and projects. It contains scripts, notebooks, and experiments focused on tasks such as fine-tuning different LLM models, quantization for performance optimization, dataset generation for instruction/QA tasks, and more. The repository offers a collection of resources for beginners and enthusiasts interested in delving into the mystical realm of LLMs.
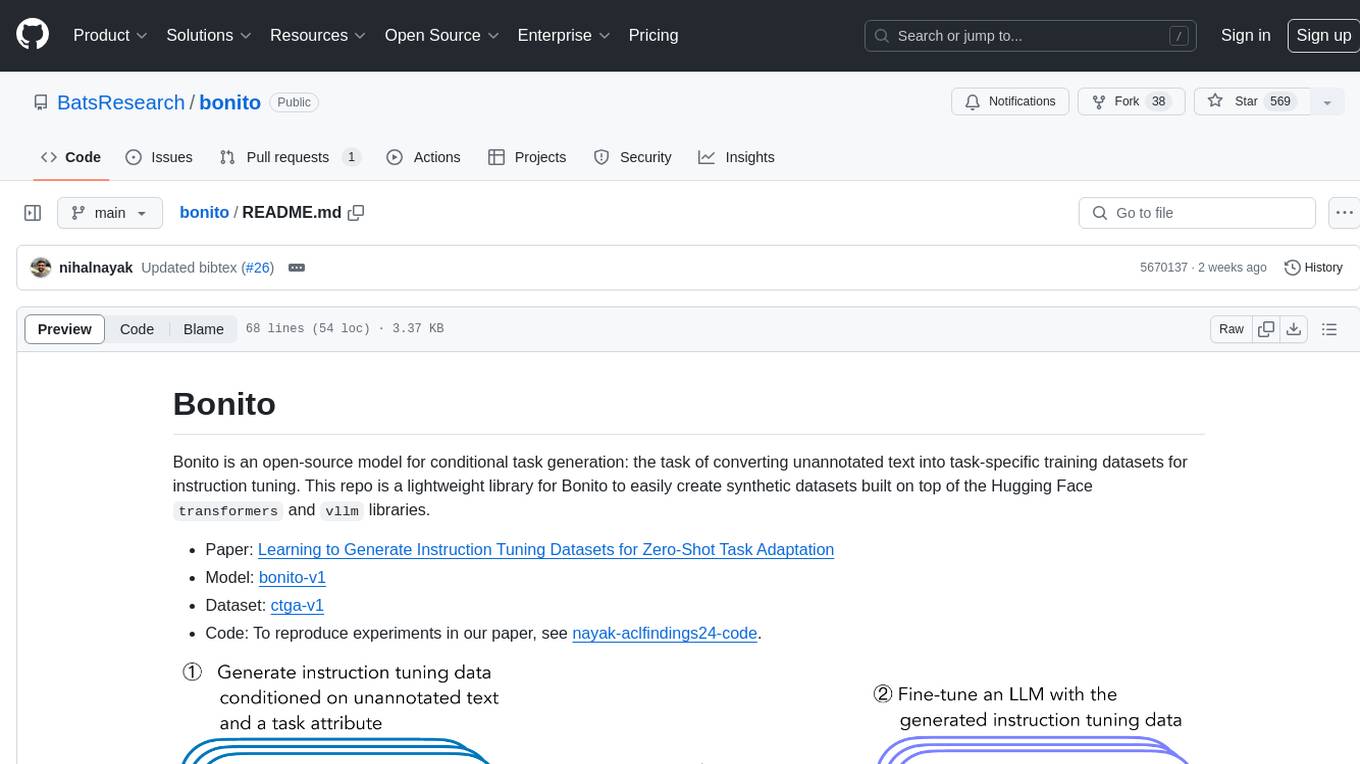
bonito
Bonito is an open-source model for conditional task generation, converting unannotated text into task-specific training datasets for instruction tuning. It is a lightweight library built on top of Hugging Face `transformers` and `vllm` libraries. The tool supports various task types such as question answering, paraphrase generation, sentiment analysis, summarization, and more. Users can easily generate synthetic instruction tuning datasets using Bonito for zero-shot task adaptation.
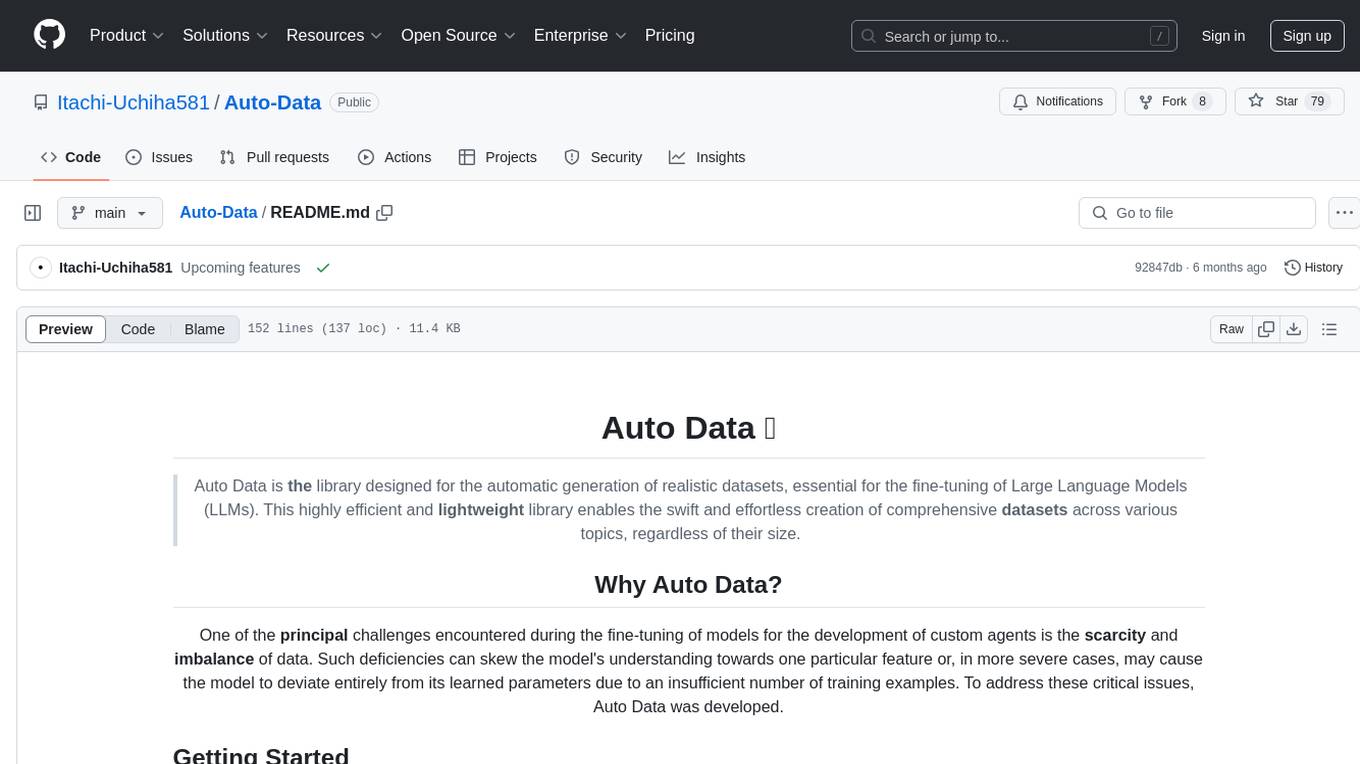
Auto-Data
Auto Data is a library designed for the automatic generation of realistic datasets, essential for the fine-tuning of Large Language Models (LLMs). This highly efficient and lightweight library enables the swift and effortless creation of comprehensive datasets across various topics, regardless of their size. It addresses challenges encountered during model fine-tuning due to data scarcity and imbalance, ensuring models are trained with sufficient examples.
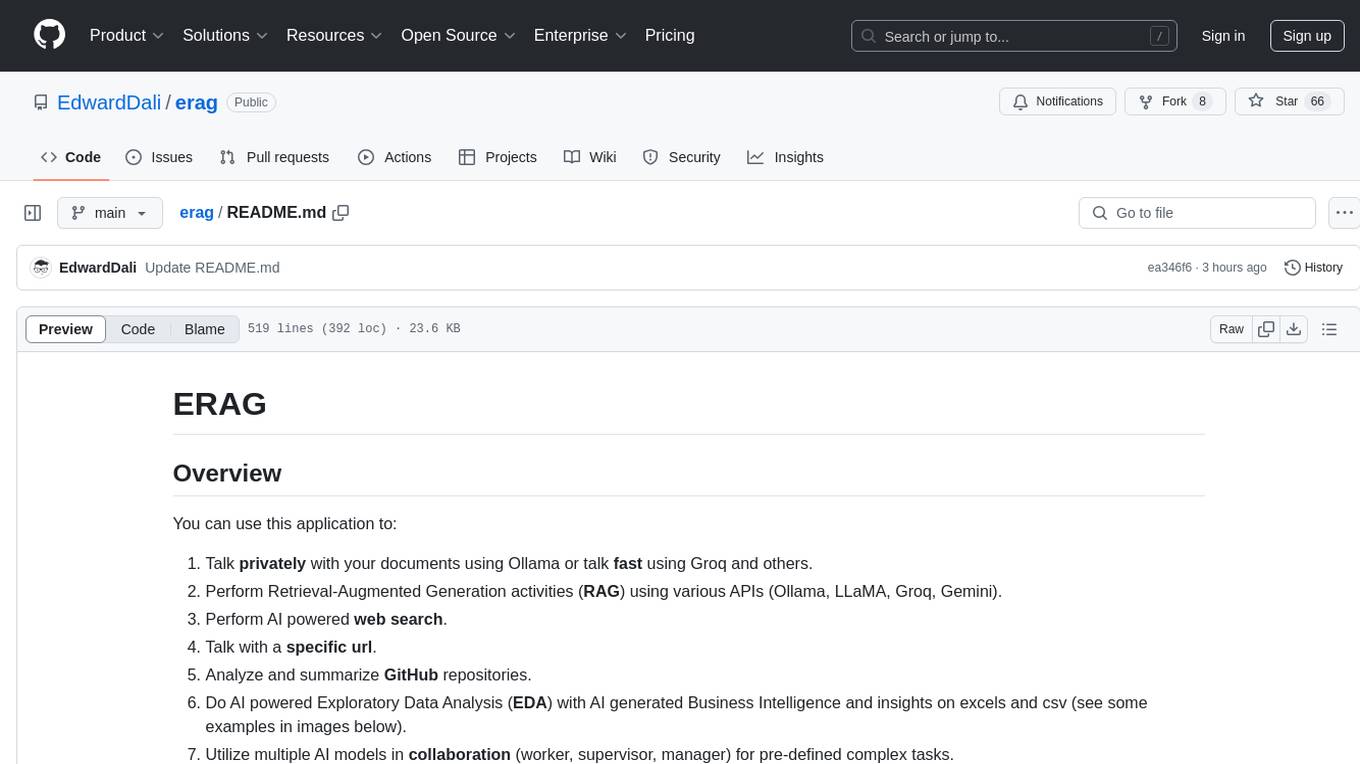
erag
ERAG is an advanced system that combines lexical, semantic, text, and knowledge graph searches with conversation context to provide accurate and contextually relevant responses. This tool processes various document types, creates embeddings, builds knowledge graphs, and uses this information to answer user queries intelligently. It includes modules for interacting with web content, GitHub repositories, and performing exploratory data analysis using various language models.
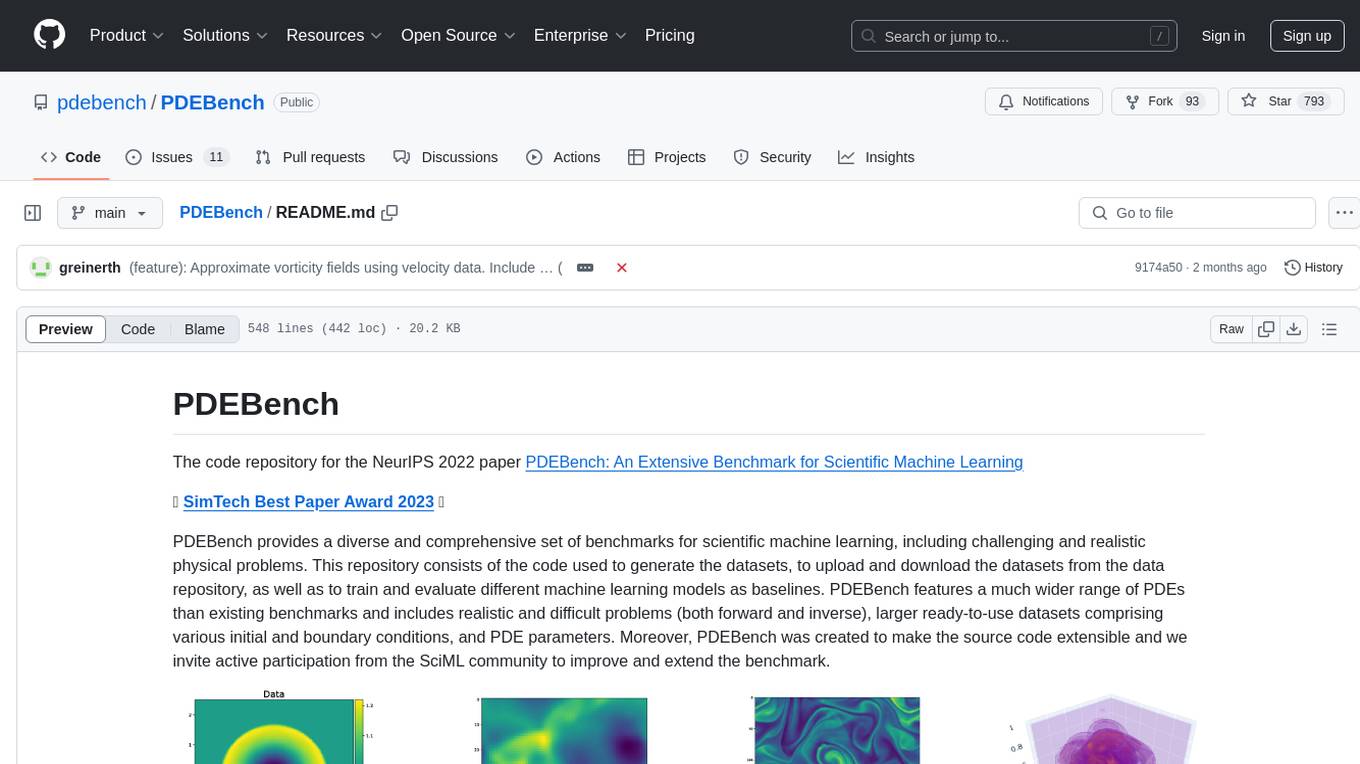
PDEBench
PDEBench provides a diverse and comprehensive set of benchmarks for scientific machine learning, including challenging and realistic physical problems. The repository consists of code for generating datasets, uploading and downloading datasets, training and evaluating machine learning models as baselines. It features a wide range of PDEs, realistic and difficult problems, ready-to-use datasets with various conditions and parameters. PDEBench aims for extensibility and invites participation from the SciML community to improve and extend the benchmark.
For similar jobs
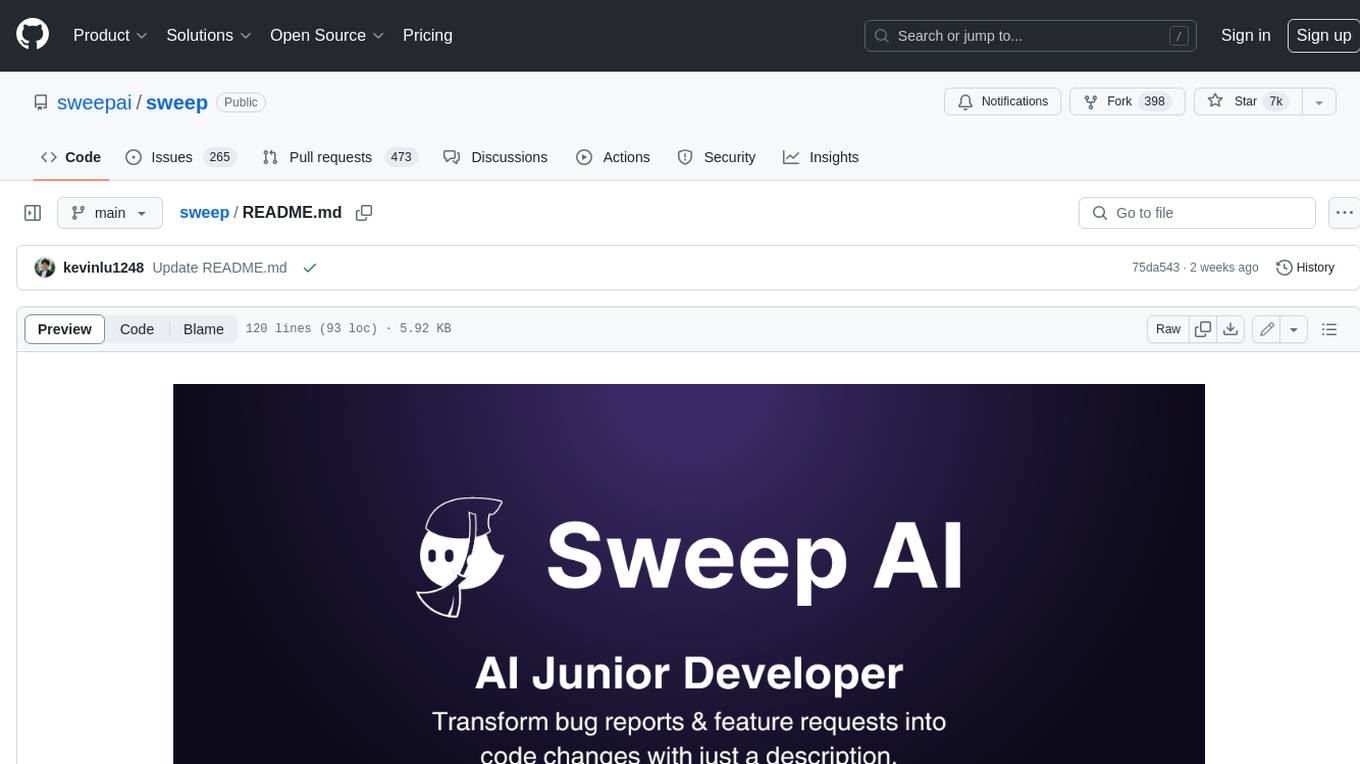
sweep
Sweep is an AI junior developer that turns bugs and feature requests into code changes. It automatically handles developer experience improvements like adding type hints and improving test coverage.
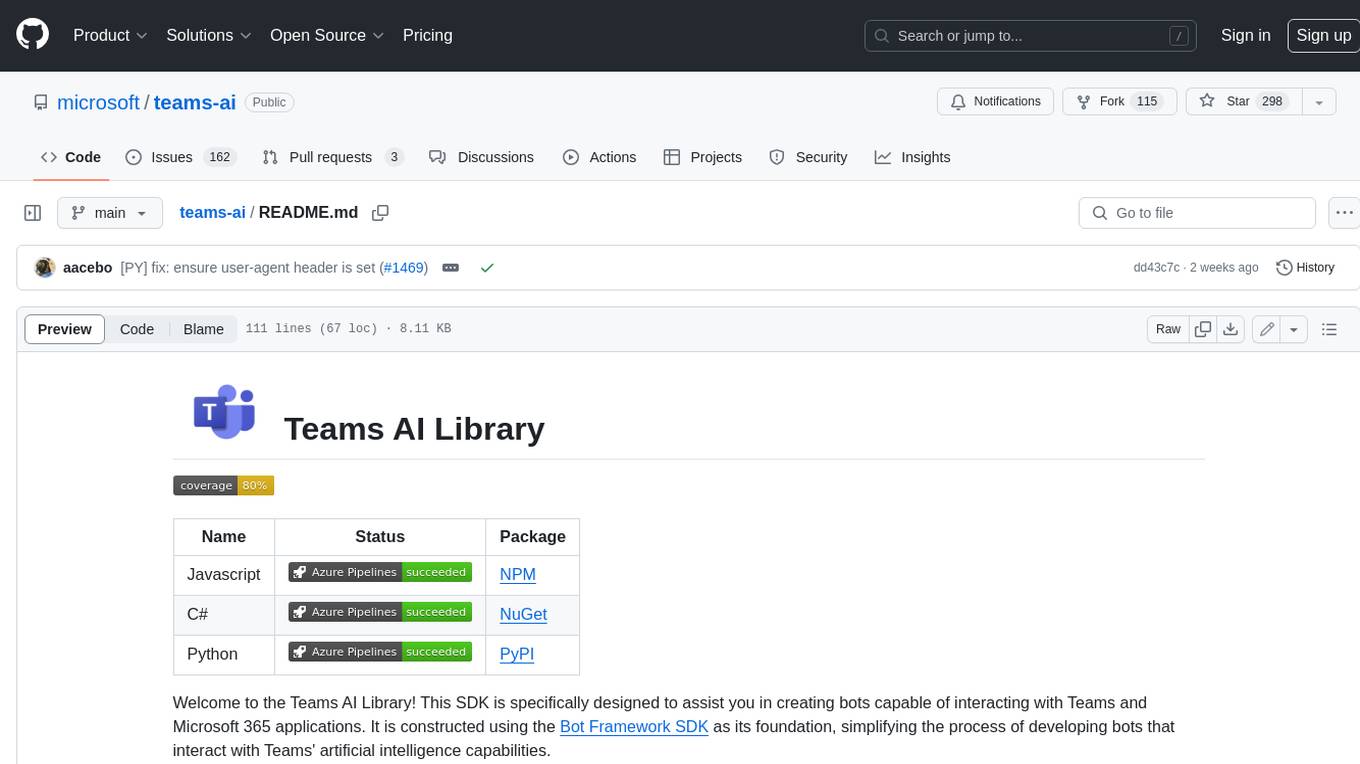
teams-ai
The Teams AI Library is a software development kit (SDK) that helps developers create bots that can interact with Teams and Microsoft 365 applications. It is built on top of the Bot Framework SDK and simplifies the process of developing bots that interact with Teams' artificial intelligence capabilities. The SDK is available for JavaScript/TypeScript, .NET, and Python.
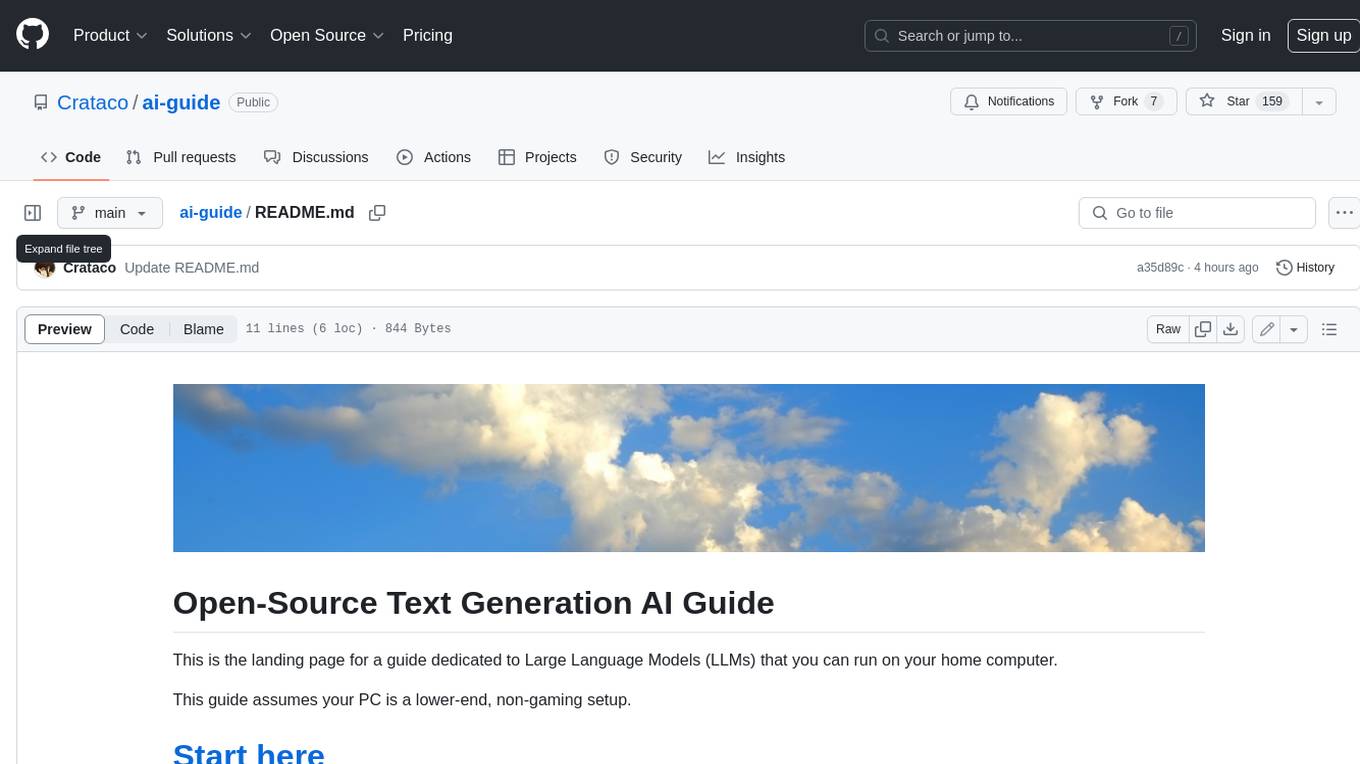
ai-guide
This guide is dedicated to Large Language Models (LLMs) that you can run on your home computer. It assumes your PC is a lower-end, non-gaming setup.
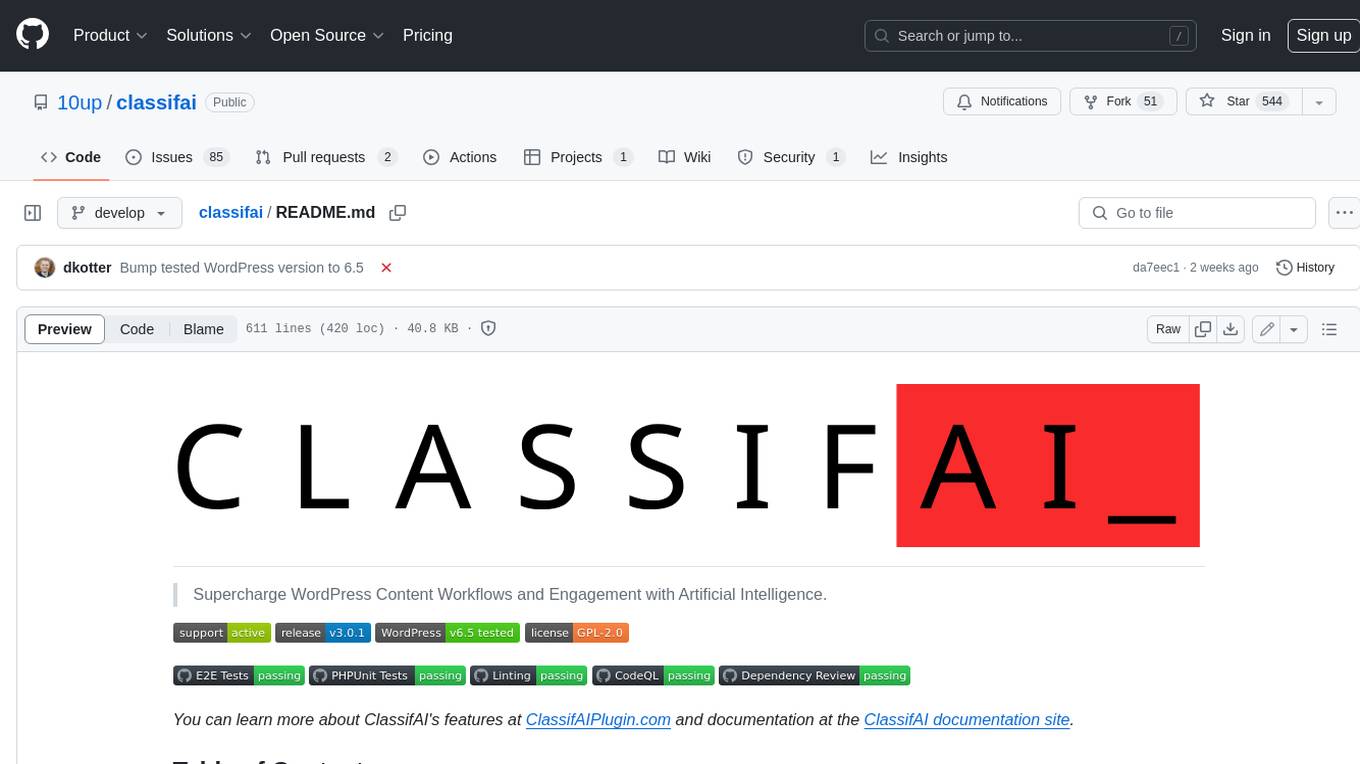
classifai
Supercharge WordPress Content Workflows and Engagement with Artificial Intelligence. Tap into leading cloud-based services like OpenAI, Microsoft Azure AI, Google Gemini and IBM Watson to augment your WordPress-powered websites. Publish content faster while improving SEO performance and increasing audience engagement. ClassifAI integrates Artificial Intelligence and Machine Learning technologies to lighten your workload and eliminate tedious tasks, giving you more time to create original content that matters.
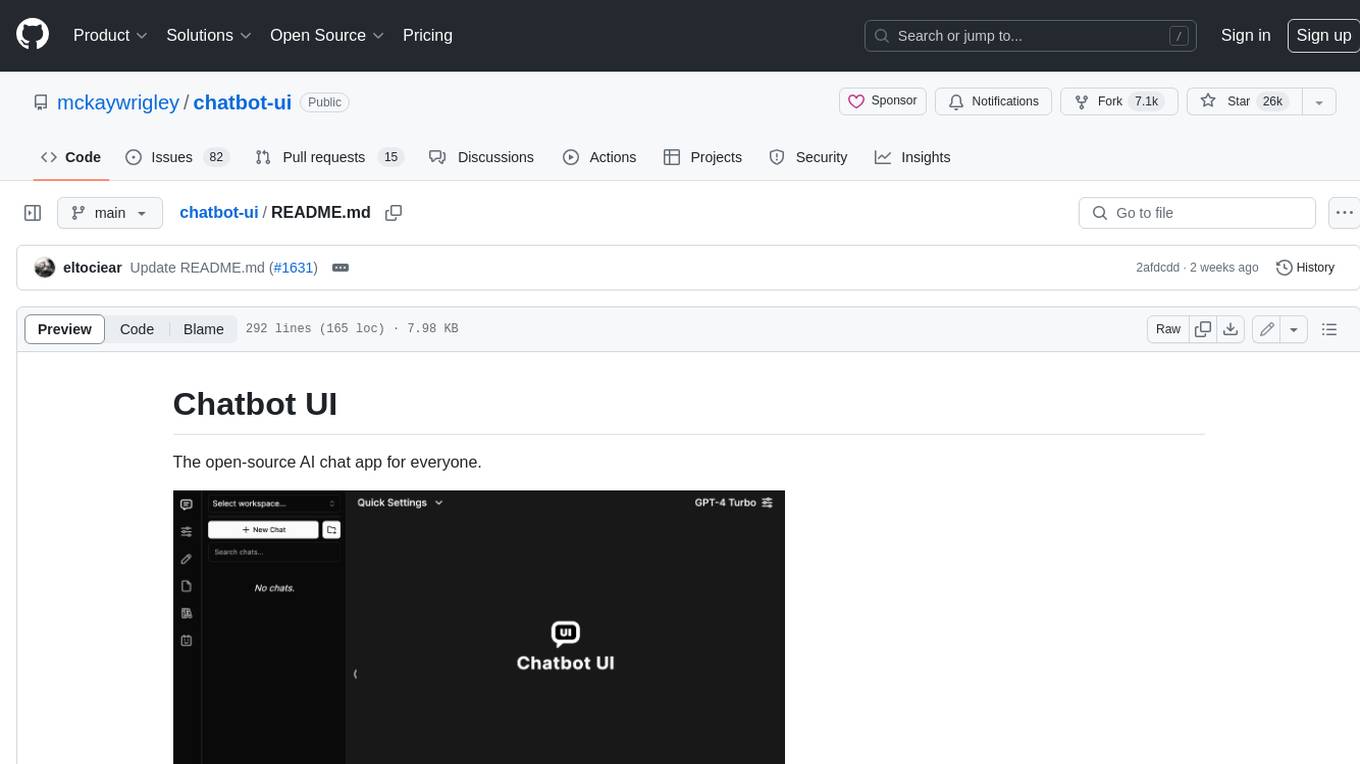
chatbot-ui
Chatbot UI is an open-source AI chat app that allows users to create and deploy their own AI chatbots. It is easy to use and can be customized to fit any need. Chatbot UI is perfect for businesses, developers, and anyone who wants to create a chatbot.
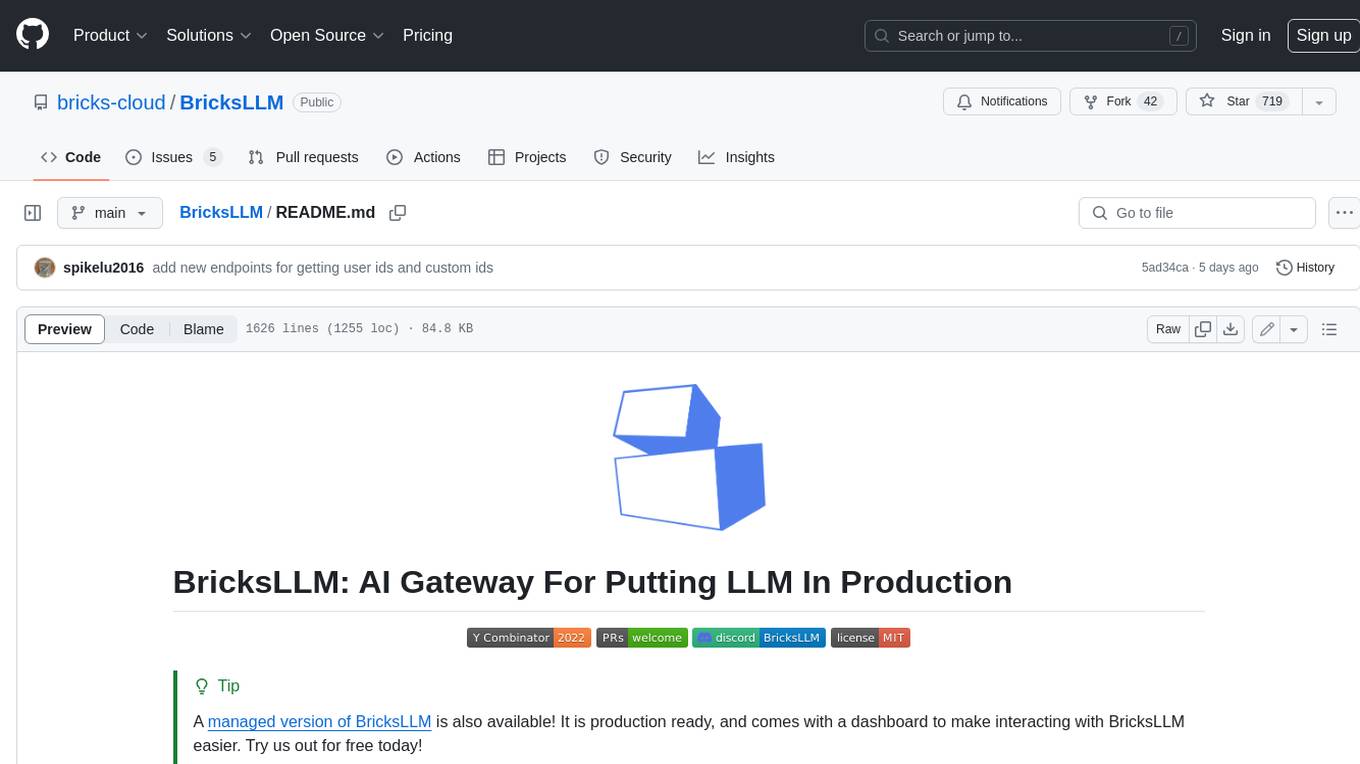
BricksLLM
BricksLLM is a cloud native AI gateway written in Go. Currently, it provides native support for OpenAI, Anthropic, Azure OpenAI and vLLM. BricksLLM aims to provide enterprise level infrastructure that can power any LLM production use cases. Here are some use cases for BricksLLM: * Set LLM usage limits for users on different pricing tiers * Track LLM usage on a per user and per organization basis * Block or redact requests containing PIIs * Improve LLM reliability with failovers, retries and caching * Distribute API keys with rate limits and cost limits for internal development/production use cases * Distribute API keys with rate limits and cost limits for students
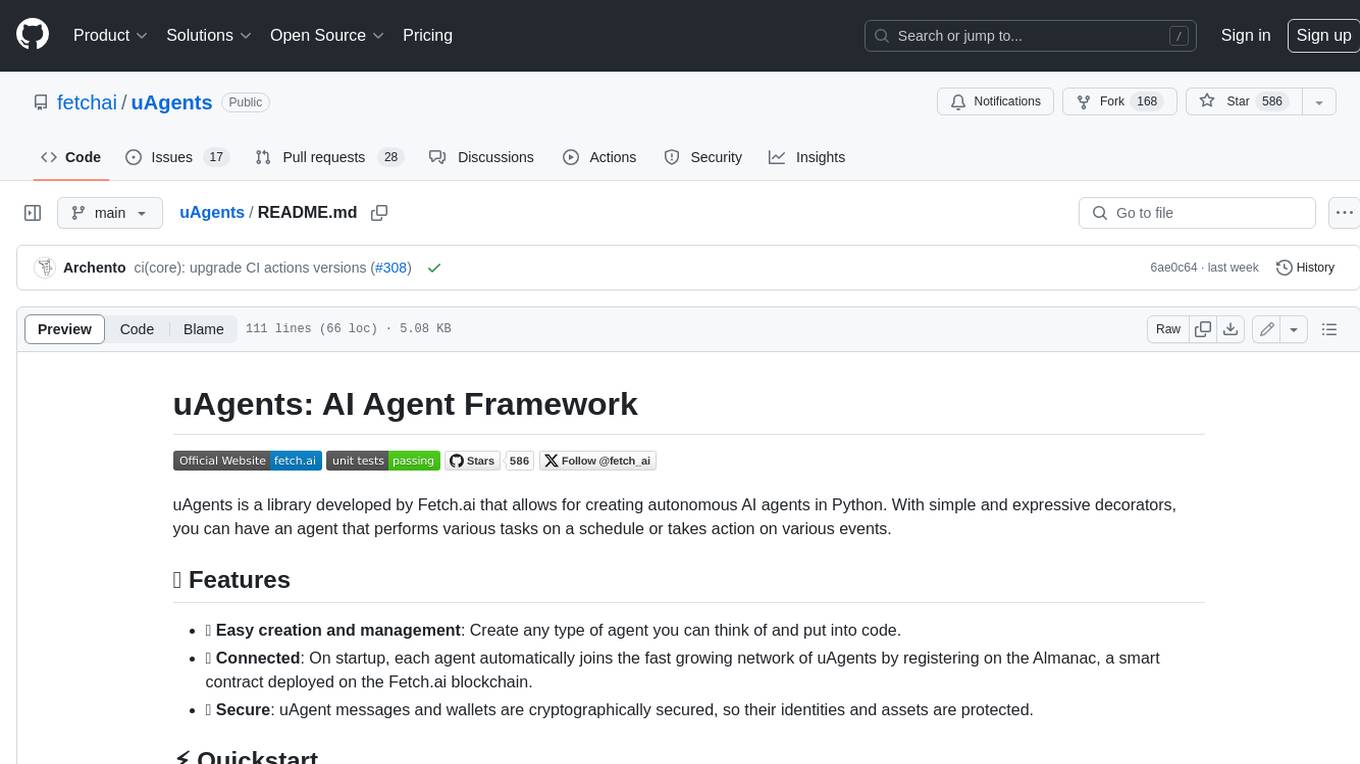
uAgents
uAgents is a Python library developed by Fetch.ai that allows for the creation of autonomous AI agents. These agents can perform various tasks on a schedule or take action on various events. uAgents are easy to create and manage, and they are connected to a fast-growing network of other uAgents. They are also secure, with cryptographically secured messages and wallets.
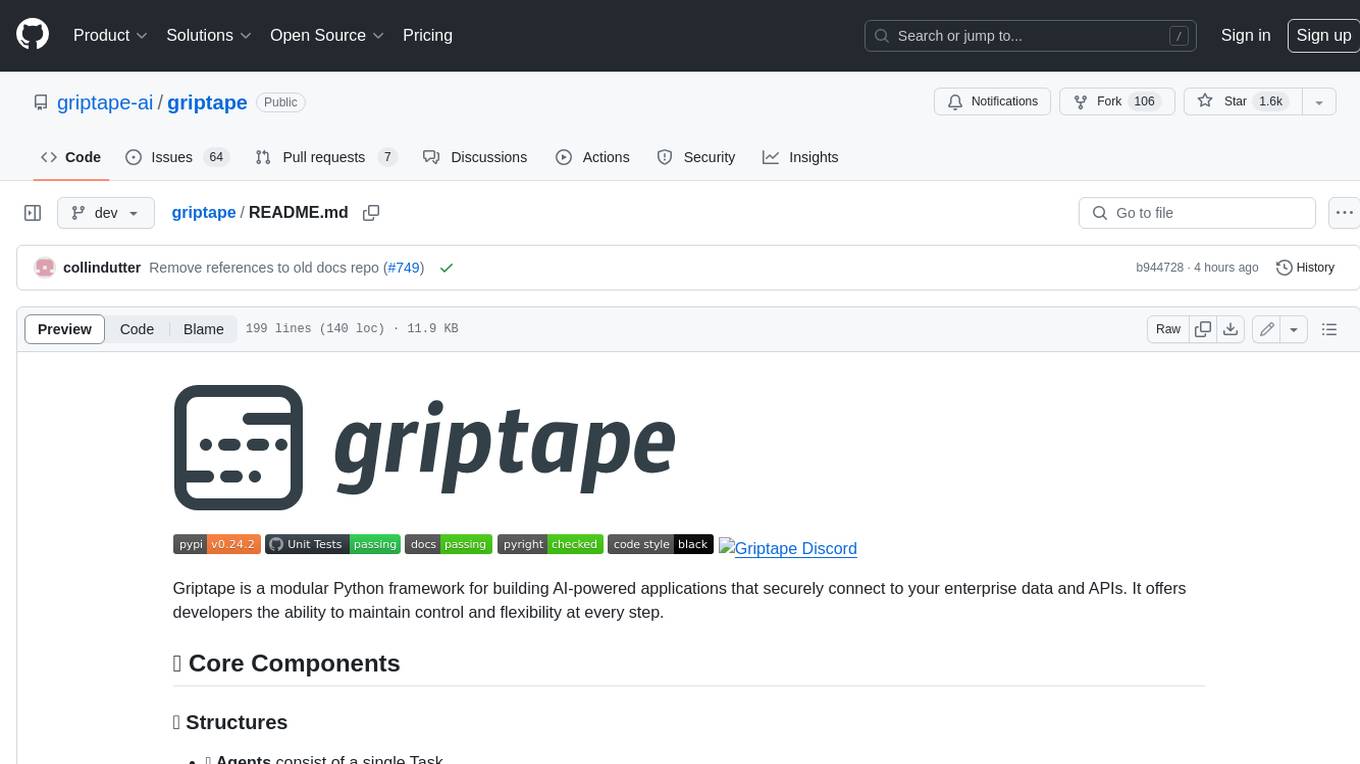
griptape
Griptape is a modular Python framework for building AI-powered applications that securely connect to your enterprise data and APIs. It offers developers the ability to maintain control and flexibility at every step. Griptape's core components include Structures (Agents, Pipelines, and Workflows), Tasks, Tools, Memory (Conversation Memory, Task Memory, and Meta Memory), Drivers (Prompt and Embedding Drivers, Vector Store Drivers, Image Generation Drivers, Image Query Drivers, SQL Drivers, Web Scraper Drivers, and Conversation Memory Drivers), Engines (Query Engines, Extraction Engines, Summary Engines, Image Generation Engines, and Image Query Engines), and additional components (Rulesets, Loaders, Artifacts, Chunkers, and Tokenizers). Griptape enables developers to create AI-powered applications with ease and efficiency.