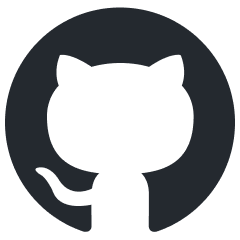
unsloth
Finetune Llama 3.3, DeepSeek-R1, Gemma 3 & Reasoning LLMs 2x faster with 70% less memory! 🦥
Stars: 35208
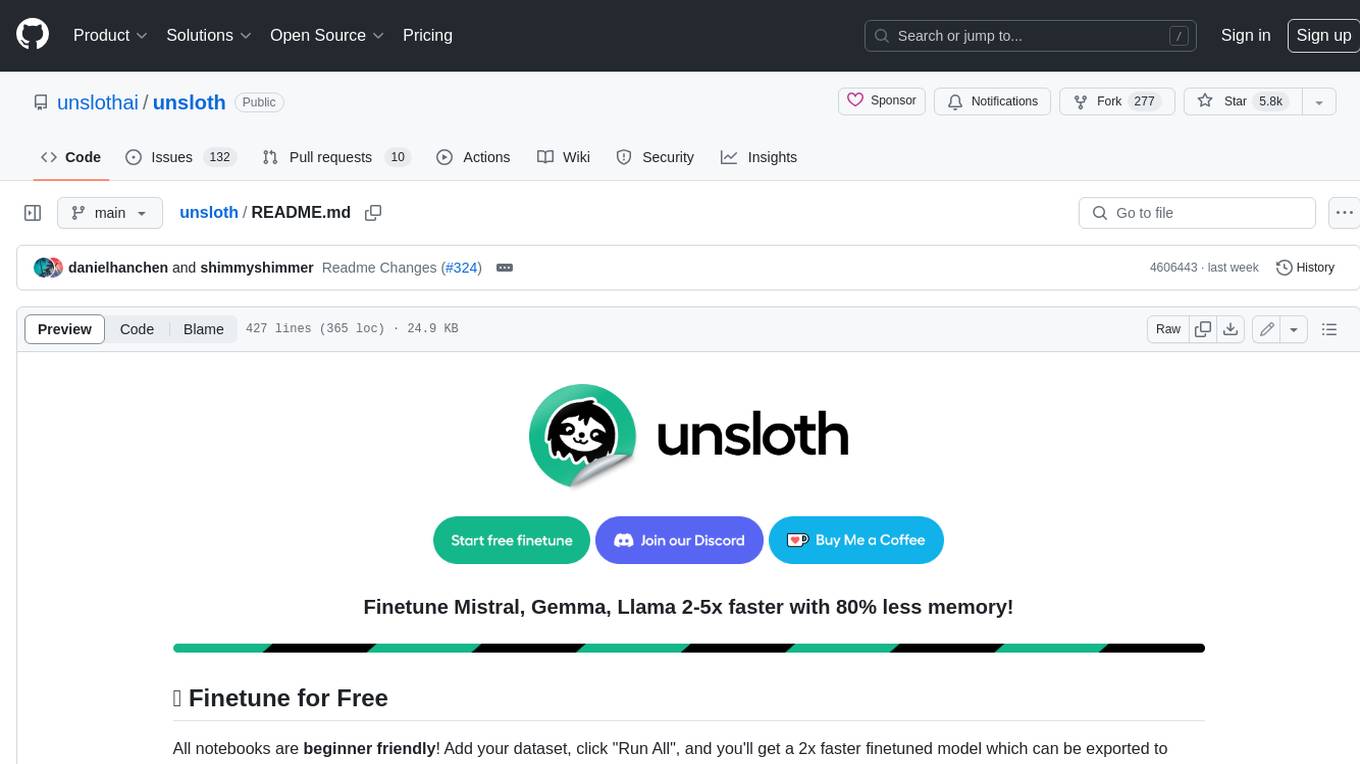
Unsloth is a tool that allows users to fine-tune large language models (LLMs) 2-5x faster with 80% less memory. It is a free and open-source tool that can be used to fine-tune LLMs such as Gemma, Mistral, Llama 2-5, TinyLlama, and CodeLlama 34b. Unsloth supports 4-bit and 16-bit QLoRA / LoRA fine-tuning via bitsandbytes. It also supports DPO (Direct Preference Optimization), PPO, and Reward Modelling. Unsloth is compatible with Hugging Face's TRL, Trainer, Seq2SeqTrainer, and Pytorch code. It is also compatible with NVIDIA GPUs since 2018+ (minimum CUDA Capability 7.0).
README:
Notebooks are beginner friendly. Read our guide. Add your dataset, click "Run All", and export your finetuned model to GGUF, Ollama, vLLM or Hugging Face.
Unsloth supports | Free Notebooks | Performance | Memory use |
---|---|---|---|
GRPO (R1 reasoning) | 2x faster | 80% less | |
Gemma 3 (4B) | 1.6x faster | 60% less | |
Llama 3.2 (3B) | 2x faster | 70% less | |
Phi-4 (14B) | 2x faster | 70% less | |
Llama 3.2 Vision (11B) | 2x faster | 50% less | |
Llama 3.1 (8B) | 2x faster | 70% less | |
Qwen 2.5 (7B) | 2x faster | 70% less | |
Mistral v0.3 (7B) | 2.2x faster | 75% less | |
Ollama | 1.9x faster | 60% less | |
DPO Zephyr | 1.9x faster | 50% less |
- See all our notebooks and all our models
- Kaggle Notebooks for Llama 3.2 Kaggle notebook, Llama 3.1 (8B), Phi-4 (14B), Mistral (7B)
- See detailed documentation for Unsloth here.
- Install with pip (recommended) for Linux devices:
pip install unsloth
For Windows install instructions, see here.
- 📣 NEW! EVERYTHING is now supported incuding: FFT, ALL models (Mixtral, MOE, Cohere, Mamba) and all training algorithms (KTO, DoRA) etc. MultiGPU support coming very soon.
To enable full-finetuning, set
full_finetuning = True
and for 8-bit finetuning, setload_in_8bit = True
- 📣 NEW! Gemma 3 by Google: Read Blog. We uploaded GGUFs, 4-bit models.
- 📣 NEW! Introducing Long-context Reasoning (GRPO) in Unsloth. Train your own reasoning model with just 5GB VRAM. Transform Llama, Phi, Mistral etc. into reasoning LLMs!
- 📣 NEW! DeepSeek-R1 - the most powerful open reasoning models with Llama & Qwen distillations. Run or fine-tune them now with our guide. All model uploads: here.
- 📣 NEW! Phi-4 by Microsoft: We also fixed bugs in Phi-4 and uploaded GGUFs, 4-bit.
- 📣 NEW! Llama 3.3 (70B), Meta's latest model is supported.
- 📣 Introducing Unsloth Dynamic 4-bit Quantization! We dynamically opt not to quantize certain parameters and this greatly increases accuracy while only using <10% more VRAM than BnB 4-bit. See our collection on Hugging Face here.
- 📣 Vision models now supported! Llama 3.2 Vision (11B), Qwen 2.5 VL (7B) and Pixtral (12B) 2409
Click for more news
- 📣 NEW! We worked with Apple to add Cut Cross Entropy. Unsloth now supports 89K context for Meta's Llama 3.3 (70B) on a 80GB GPU - 13x longer than HF+FA2. For Llama 3.1 (8B), Unsloth enables 342K context, surpassing its native 128K support.
- 📣 We found and helped fix a gradient accumulation bug! Please update Unsloth and transformers.
- 📣 Try out Chat interface!
- 📣 NEW! Qwen-2.5 including Coder models are now supported with bugfixes. 14b fits in a Colab GPU! Qwen 2.5 conversational notebook
- 📣 NEW! Mistral Small 22b notebook finetuning fits in under 16GB of VRAM!
- 📣 NEW!
pip install unsloth
now works! Head over to pypi to check it out! This allows non git pull installs. Usepip install unsloth[colab-new]
for non dependency installs. - 📣 NEW! Continued Pretraining notebook for other languages like Korean!
- 📣 2x faster inference added for all our models
- 📣 We cut memory usage by a further 30% and now support 4x longer context windows!
Type | Links |
---|---|
📚 Documentation & Wiki | Read Our Docs |
|
Follow us on X |
💾 Installation | Pip install |
🔮 Our Models | Unsloth Releases |
✍️ Blog | Read our Blogs |
|
Join our Reddit page |
- Supports full-finetuning, pretraining, 4b-bit, 16-bit and 8-bit training
- All kernels written in OpenAI's Triton language. Manual backprop engine.
- 0% loss in accuracy - no approximation methods - all exact.
- No change of hardware. Supports NVIDIA GPUs since 2018+. Minimum CUDA Capability 7.0 (V100, T4, Titan V, RTX 20, 30, 40x, A100, H100, L40 etc) Check your GPU! GTX 1070, 1080 works, but is slow.
- Works on Linux and Windows
- Supports 4bit and 16bit QLoRA / LoRA finetuning via bitsandbytes.
- If you trained a model with 🦥Unsloth, you can use this cool sticker!
You can also see our documentation for more detailed installation and updating instructions here.
Install with pip (recommended) for Linux devices:
pip install unsloth
See here for advanced pip install instructions.
[!warning] Python 3.13 does not support Unsloth. Use 3.12, 3.11 or 3.10
-
Install NVIDIA Video Driver: You should install the latest version of your GPUs driver. Download drivers here: NVIDIA GPU Drive.
-
Install Visual Studio C++: You will need Visual Studio, with C++ installed. By default, C++ is not installed with Visual Studio, so make sure you select all of the C++ options. Also select options for Windows 10/11 SDK. For detailed instructions with options, see here.
-
Install CUDA Toolkit: Follow the instructions to install CUDA Toolkit.
-
Install PyTorch: You will need the correct version of PyTorch that is compatibile with your CUDA drivers, so make sure to select them carefully. Install PyTorch.
-
Install Unsloth:
pip install unsloth
To run Unsloth directly on Windows:
- Install Triton from this Windows fork and follow the instructions here (be aware that the Windows fork requires PyTorch >= 2.4 and CUDA 12)
- In the SFTTrainer, set
dataset_num_proc=1
to avoid a crashing issue:
trainer = SFTTrainer(
dataset_num_proc=1,
...
)
For advanced installation instructions or if you see weird errors during installations:
- Install
torch
andtriton
. Go to https://pytorch.org to install it. For examplepip install torch torchvision torchaudio triton
- Confirm if CUDA is installated correctly. Try
nvcc
. If that fails, you need to installcudatoolkit
or CUDA drivers. - Install
xformers
manually. You can try installingvllm
and seeing ifvllm
succeeds. Check ifxformers
succeeded withpython -m xformers.info
Go to https://github.com/facebookresearch/xformers. Another option is to installflash-attn
for Ampere GPUs. - Double check that your versions of Python, CUDA, CUDNN,
torch
,triton
, andxformers
are compatible with one another. The PyTorch Compatibility Matrix may be useful. - Finally, install
bitsandbytes
and check it withpython -m bitsandbytes
⚠️Only use Conda if you have it. If not, use Pip
. Select either pytorch-cuda=11.8,12.1
for CUDA 11.8 or CUDA 12.1. We support python=3.10,3.11,3.12
.
conda create --name unsloth_env \
python=3.11 \
pytorch-cuda=12.1 \
pytorch cudatoolkit xformers -c pytorch -c nvidia -c xformers \
-y
conda activate unsloth_env
pip install unsloth
If you're looking to install Conda in a Linux environment, read here, or run the below 🔽
mkdir -p ~/miniconda3
wget https://repo.anaconda.com/miniconda/Miniconda3-latest-Linux-x86_64.sh -O ~/miniconda3/miniconda.sh
bash ~/miniconda3/miniconda.sh -b -u -p ~/miniconda3
rm -rf ~/miniconda3/miniconda.sh
~/miniconda3/bin/conda init bash
~/miniconda3/bin/conda init zsh
⚠️Do **NOT** use this if you have Conda.
Pip is a bit more complex since there are dependency issues. The pip command is different for torch 2.2,2.3,2.4,2.5
and CUDA versions.
For other torch versions, we support torch211
, torch212
, torch220
, torch230
, torch240
and for CUDA versions, we support cu118
and cu121
and cu124
. For Ampere devices (A100, H100, RTX3090) and above, use cu118-ampere
or cu121-ampere
or cu124-ampere
.
For example, if you have torch 2.4
and CUDA 12.1
, use:
pip install --upgrade pip
pip install "unsloth[cu121-torch240] @ git+https://github.com/unslothai/unsloth.git"
Another example, if you have torch 2.5
and CUDA 12.4
, use:
pip install --upgrade pip
pip install "unsloth[cu124-torch250] @ git+https://github.com/unslothai/unsloth.git"
And other examples:
pip install "unsloth[cu121-ampere-torch240] @ git+https://github.com/unslothai/unsloth.git"
pip install "unsloth[cu118-ampere-torch240] @ git+https://github.com/unslothai/unsloth.git"
pip install "unsloth[cu121-torch240] @ git+https://github.com/unslothai/unsloth.git"
pip install "unsloth[cu118-torch240] @ git+https://github.com/unslothai/unsloth.git"
pip install "unsloth[cu121-torch230] @ git+https://github.com/unslothai/unsloth.git"
pip install "unsloth[cu121-ampere-torch230] @ git+https://github.com/unslothai/unsloth.git"
pip install "unsloth[cu121-torch250] @ git+https://github.com/unslothai/unsloth.git"
pip install "unsloth[cu124-ampere-torch250] @ git+https://github.com/unslothai/unsloth.git"
Or, run the below in a terminal to get the optimal pip installation command:
wget -qO- https://raw.githubusercontent.com/unslothai/unsloth/main/unsloth/_auto_install.py | python -
Or, run the below manually in a Python REPL:
try: import torch
except: raise ImportError('Install torch via `pip install torch`')
from packaging.version import Version as V
v = V(torch.__version__)
cuda = str(torch.version.cuda)
is_ampere = torch.cuda.get_device_capability()[0] >= 8
if cuda != "12.1" and cuda != "11.8" and cuda != "12.4": raise RuntimeError(f"CUDA = {cuda} not supported!")
if v <= V('2.1.0'): raise RuntimeError(f"Torch = {v} too old!")
elif v <= V('2.1.1'): x = 'cu{}{}-torch211'
elif v <= V('2.1.2'): x = 'cu{}{}-torch212'
elif v < V('2.3.0'): x = 'cu{}{}-torch220'
elif v < V('2.4.0'): x = 'cu{}{}-torch230'
elif v < V('2.5.0'): x = 'cu{}{}-torch240'
elif v < V('2.6.0'): x = 'cu{}{}-torch250'
else: raise RuntimeError(f"Torch = {v} too new!")
x = x.format(cuda.replace(".", ""), "-ampere" if is_ampere else "")
print(f'pip install --upgrade pip && pip install "unsloth[{x}] @ git+https://github.com/unslothai/unsloth.git"')
- Go to our official Documentation for saving to GGUF, checkpointing, evaluation and more!
- We support Huggingface's TRL, Trainer, Seq2SeqTrainer or even Pytorch code!
- We're in 🤗Hugging Face's official docs! Check out the SFT docs and DPO docs!
- If you want to download models from the ModelScope community, please use an environment variable:
UNSLOTH_USE_MODELSCOPE=1
, and install the modelscope library by:pip install modelscope -U
.
unsloth_cli.py also supports
UNSLOTH_USE_MODELSCOPE=1
to download models and datasets. please remember to use the model and dataset id in the ModelScope community.
from unsloth import FastLanguageModel
import torch
from trl import SFTTrainer, SFTConfig
from datasets import load_dataset
max_seq_length = 2048 # Supports RoPE Scaling interally, so choose any!
# Get LAION dataset
url = "https://huggingface.co/datasets/laion/OIG/resolve/main/unified_chip2.jsonl"
dataset = load_dataset("json", data_files = {"train" : url}, split = "train")
# 4bit pre quantized models we support for 4x faster downloading + no OOMs.
fourbit_models = [
"unsloth/Meta-Llama-3.1-8B-bnb-4bit", # Llama-3.1 2x faster
"unsloth/Meta-Llama-3.1-8B-Instruct-bnb-4bit",
"unsloth/Meta-Llama-3.1-70B-bnb-4bit",
"unsloth/Meta-Llama-3.1-405B-bnb-4bit", # 4bit for 405b!
"unsloth/Mistral-Small-Instruct-2409", # Mistral 22b 2x faster!
"unsloth/mistral-7b-instruct-v0.3-bnb-4bit",
"unsloth/Phi-3.5-mini-instruct", # Phi-3.5 2x faster!
"unsloth/Phi-3-medium-4k-instruct",
"unsloth/gemma-2-9b-bnb-4bit",
"unsloth/gemma-2-27b-bnb-4bit", # Gemma 2x faster!
"unsloth/Llama-3.2-1B-bnb-4bit", # NEW! Llama 3.2 models
"unsloth/Llama-3.2-1B-Instruct-bnb-4bit",
"unsloth/Llama-3.2-3B-bnb-4bit",
"unsloth/Llama-3.2-3B-Instruct-bnb-4bit",
"unsloth/Llama-3.3-70B-Instruct-bnb-4bit" # NEW! Llama 3.3 70B!
] # More models at https://huggingface.co/unsloth
model, tokenizer = FastModel.from_pretrained(
model_name = "unsloth/gemma-3-4B-it",
max_seq_length = 2048, # Choose any for long context!
load_in_4bit = True, # 4 bit quantization to reduce memory
load_in_8bit = False, # [NEW!] A bit more accurate, uses 2x memory
full_finetuning = False, # [NEW!] We have full finetuning now!
# token = "hf_...", # use one if using gated models
)
# Do model patching and add fast LoRA weights
model = FastLanguageModel.get_peft_model(
model,
r = 16,
target_modules = ["q_proj", "k_proj", "v_proj", "o_proj",
"gate_proj", "up_proj", "down_proj",],
lora_alpha = 16,
lora_dropout = 0, # Supports any, but = 0 is optimized
bias = "none", # Supports any, but = "none" is optimized
# [NEW] "unsloth" uses 30% less VRAM, fits 2x larger batch sizes!
use_gradient_checkpointing = "unsloth", # True or "unsloth" for very long context
random_state = 3407,
max_seq_length = max_seq_length,
use_rslora = False, # We support rank stabilized LoRA
loftq_config = None, # And LoftQ
)
trainer = SFTTrainer(
model = model,
train_dataset = dataset,
tokenizer = tokenizer,
args = SFTConfig(
dataset_text_field = "text",
max_seq_length = max_seq_length,
per_device_train_batch_size = 2,
gradient_accumulation_steps = 4,
warmup_steps = 10,
max_steps = 60,
logging_steps = 1,
output_dir = "outputs",
optim = "adamw_8bit",
seed = 3407,
),
)
trainer.train()
# Go to https://github.com/unslothai/unsloth/wiki for advanced tips like
# (1) Saving to GGUF / merging to 16bit for vLLM
# (2) Continued training from a saved LoRA adapter
# (3) Adding an evaluation loop / OOMs
# (4) Customized chat templates
RL including DPO, GRPO, PPO, Reward Modelling, Online DPO all work with Unsloth. We're in 🤗Hugging Face's official docs! We're on the SFT docs and the DPO docs! List of RL notebooks:
Click for DPO code
import os
os.environ["CUDA_VISIBLE_DEVICES"] = "0" # Optional set GPU device ID
from unsloth import FastLanguageModel
import torch
from trl import DPOTrainer, DPOConfig
max_seq_length = 2048
model, tokenizer = FastLanguageModel.from_pretrained(
model_name = "unsloth/zephyr-sft-bnb-4bit",
max_seq_length = max_seq_length,
load_in_4bit = True,
)
# Do model patching and add fast LoRA weights
model = FastLanguageModel.get_peft_model(
model,
r = 64,
target_modules = ["q_proj", "k_proj", "v_proj", "o_proj",
"gate_proj", "up_proj", "down_proj",],
lora_alpha = 64,
lora_dropout = 0, # Supports any, but = 0 is optimized
bias = "none", # Supports any, but = "none" is optimized
# [NEW] "unsloth" uses 30% less VRAM, fits 2x larger batch sizes!
use_gradient_checkpointing = "unsloth", # True or "unsloth" for very long context
random_state = 3407,
max_seq_length = max_seq_length,
)
dpo_trainer = DPOTrainer(
model = model,
ref_model = None,
train_dataset = YOUR_DATASET_HERE,
# eval_dataset = YOUR_DATASET_HERE,
tokenizer = tokenizer,
args = DPOConfig(
per_device_train_batch_size = 4,
gradient_accumulation_steps = 8,
warmup_ratio = 0.1,
num_train_epochs = 3,
logging_steps = 1,
optim = "adamw_8bit",
seed = 42,
output_dir = "outputs",
max_length = 1024,
max_prompt_length = 512,
beta = 0.1,
),
)
dpo_trainer.train()
- For our most detailed benchmarks, read our Llama 3.3 Blog.
- Benchmarking of Unsloth was also conducted by 🤗Hugging Face.
We tested using the Alpaca Dataset, a batch size of 2, gradient accumulation steps of 4, rank = 32, and applied QLoRA on all linear layers (q, k, v, o, gate, up, down):
Model | VRAM | 🦥 Unsloth speed | 🦥 VRAM reduction | 🦥 Longer context | 😊 Hugging Face + FA2 |
---|---|---|---|---|---|
Llama 3.3 (70B) | 80GB | 2x | >75% | 13x longer | 1x |
Llama 3.1 (8B) | 80GB | 2x | >70% | 12x longer | 1x |
We tested Llama 3.1 (8B) Instruct and did 4bit QLoRA on all linear layers (Q, K, V, O, gate, up and down) with rank = 32 with a batch size of 1. We padded all sequences to a certain maximum sequence length to mimic long context finetuning workloads.
GPU VRAM | 🦥Unsloth context length | Hugging Face + FA2 |
---|---|---|
8 GB | 2,972 | OOM |
12 GB | 21,848 | 932 |
16 GB | 40,724 | 2,551 |
24 GB | 78,475 | 5,789 |
40 GB | 153,977 | 12,264 |
48 GB | 191,728 | 15,502 |
80 GB | 342,733 | 28,454 |
We tested Llama 3.3 (70B) Instruct on a 80GB A100 and did 4bit QLoRA on all linear layers (Q, K, V, O, gate, up and down) with rank = 32 with a batch size of 1. We padded all sequences to a certain maximum sequence length to mimic long context finetuning workloads.
GPU VRAM | 🦥Unsloth context length | Hugging Face + FA2 |
---|---|---|
48 GB | 12,106 | OOM |
80 GB | 89,389 | 6,916 |
You can cite the Unsloth repo as follows:
@software{unsloth,
author = {Daniel Han, Michael Han and Unsloth team},
title = {Unsloth},
url = {http://github.com/unslothai/unsloth},
year = {2023}
}
- Hugging Face's TRL library which serves as the basis foundation for Unsloth
- Erik for his help adding Apple's ML Cross Entropy in Unsloth
- HuyNguyen-hust for making RoPE Embeddings 28% faster
- RandomInternetPreson for confirming WSL support
- 152334H for experimental DPO support
For Tasks:
Click tags to check more tools for each tasksFor Jobs:
Alternative AI tools for unsloth
Similar Open Source Tools
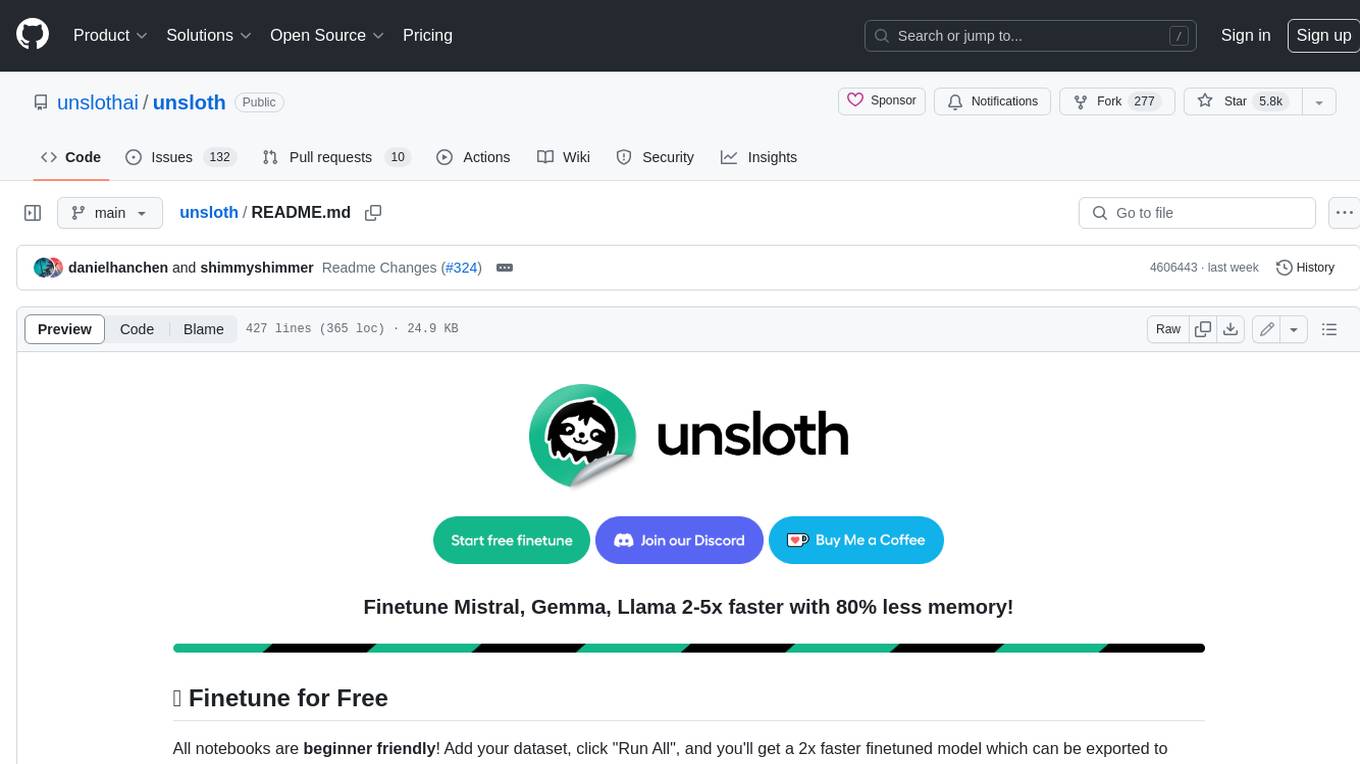
unsloth
Unsloth is a tool that allows users to fine-tune large language models (LLMs) 2-5x faster with 80% less memory. It is a free and open-source tool that can be used to fine-tune LLMs such as Gemma, Mistral, Llama 2-5, TinyLlama, and CodeLlama 34b. Unsloth supports 4-bit and 16-bit QLoRA / LoRA fine-tuning via bitsandbytes. It also supports DPO (Direct Preference Optimization), PPO, and Reward Modelling. Unsloth is compatible with Hugging Face's TRL, Trainer, Seq2SeqTrainer, and Pytorch code. It is also compatible with NVIDIA GPUs since 2018+ (minimum CUDA Capability 7.0).
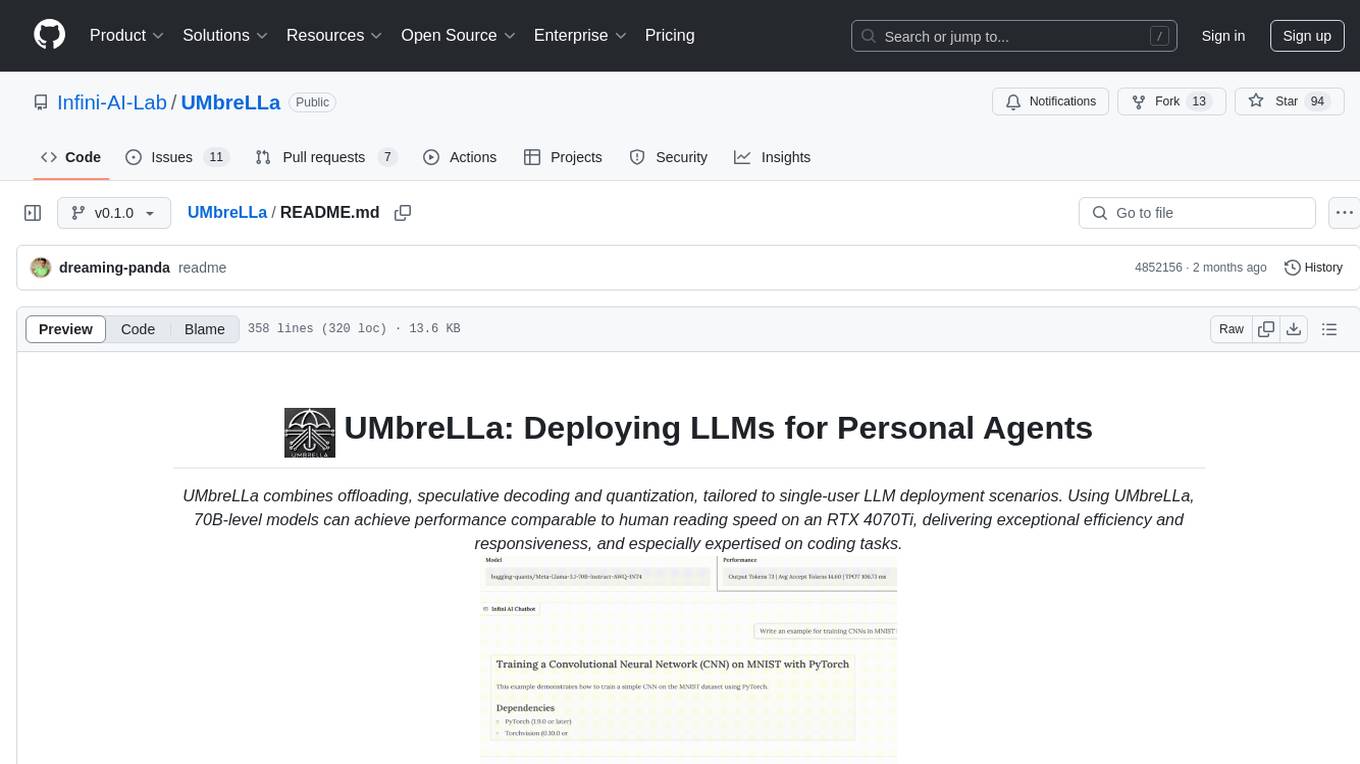
UMbreLLa
UMbreLLa is a tool designed for deploying Large Language Models (LLMs) for personal agents. It combines offloading, speculative decoding, and quantization to optimize single-user LLM deployment scenarios. With UMbreLLa, 70B-level models can achieve performance comparable to human reading speed on an RTX 4070Ti, delivering exceptional efficiency and responsiveness, especially for coding tasks. The tool supports deploying models on various GPUs and offers features like code completion and CLI/Gradio chatbots. Users can configure the LLM engine for optimal performance based on their hardware setup.
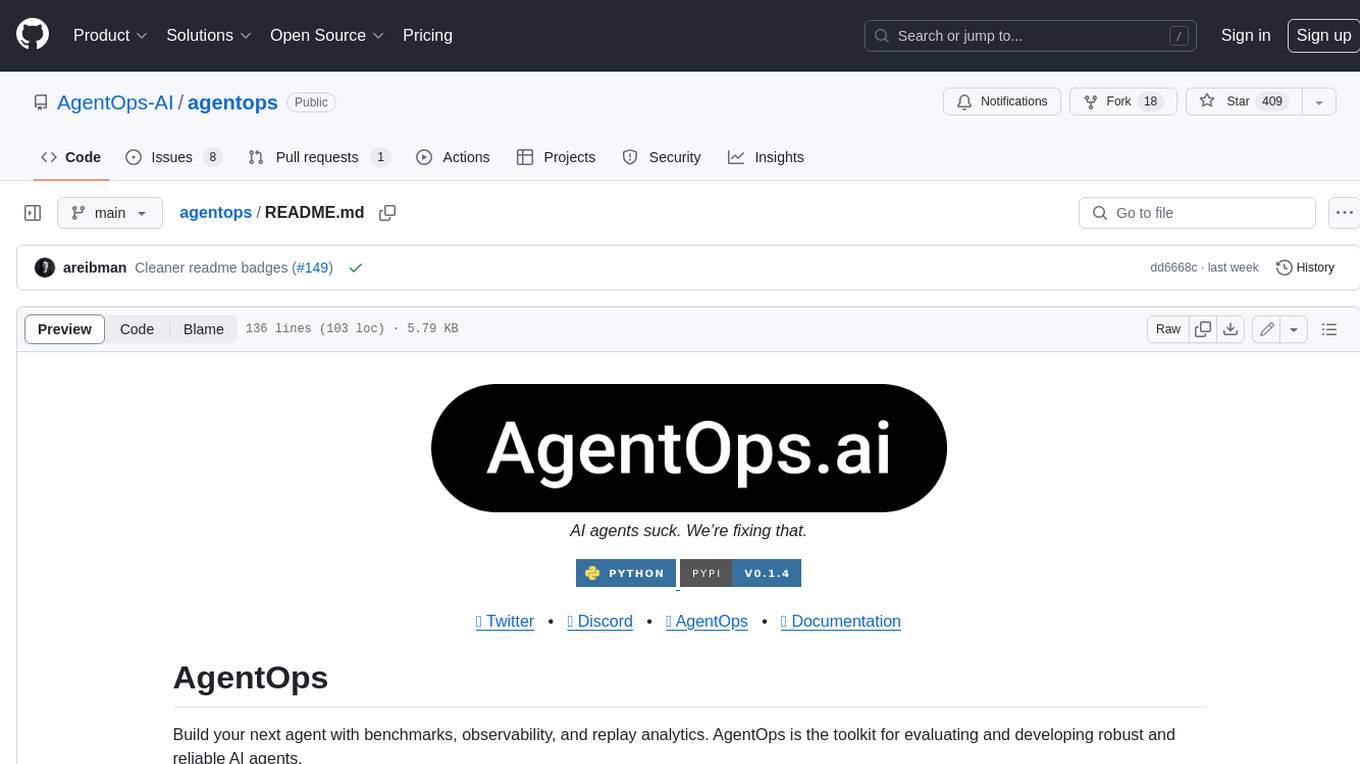
agentops
AgentOps is a toolkit for evaluating and developing robust and reliable AI agents. It provides benchmarks, observability, and replay analytics to help developers build better agents. AgentOps is open beta and can be signed up for here. Key features of AgentOps include: - Session replays in 3 lines of code: Initialize the AgentOps client and automatically get analytics on every LLM call. - Time travel debugging: (coming soon!) - Agent Arena: (coming soon!) - Callback handlers: AgentOps works seamlessly with applications built using Langchain and LlamaIndex.
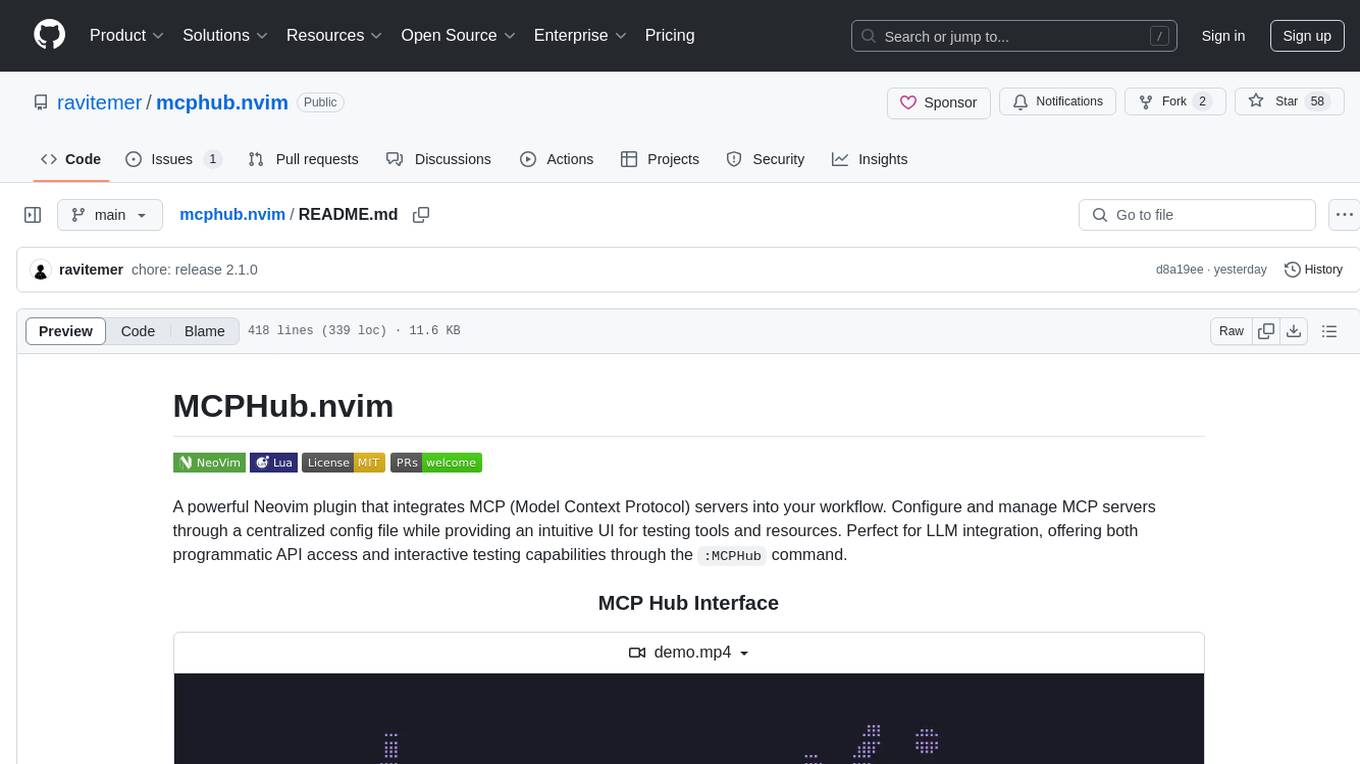
mcphub.nvim
MCPHub.nvim is a powerful Neovim plugin that integrates MCP (Model Context Protocol) servers into your workflow. It offers a centralized config file for managing servers and tools, with an intuitive UI for testing resources. Ideal for LLM integration, it provides programmatic API access and interactive testing through the `:MCPHub` command.
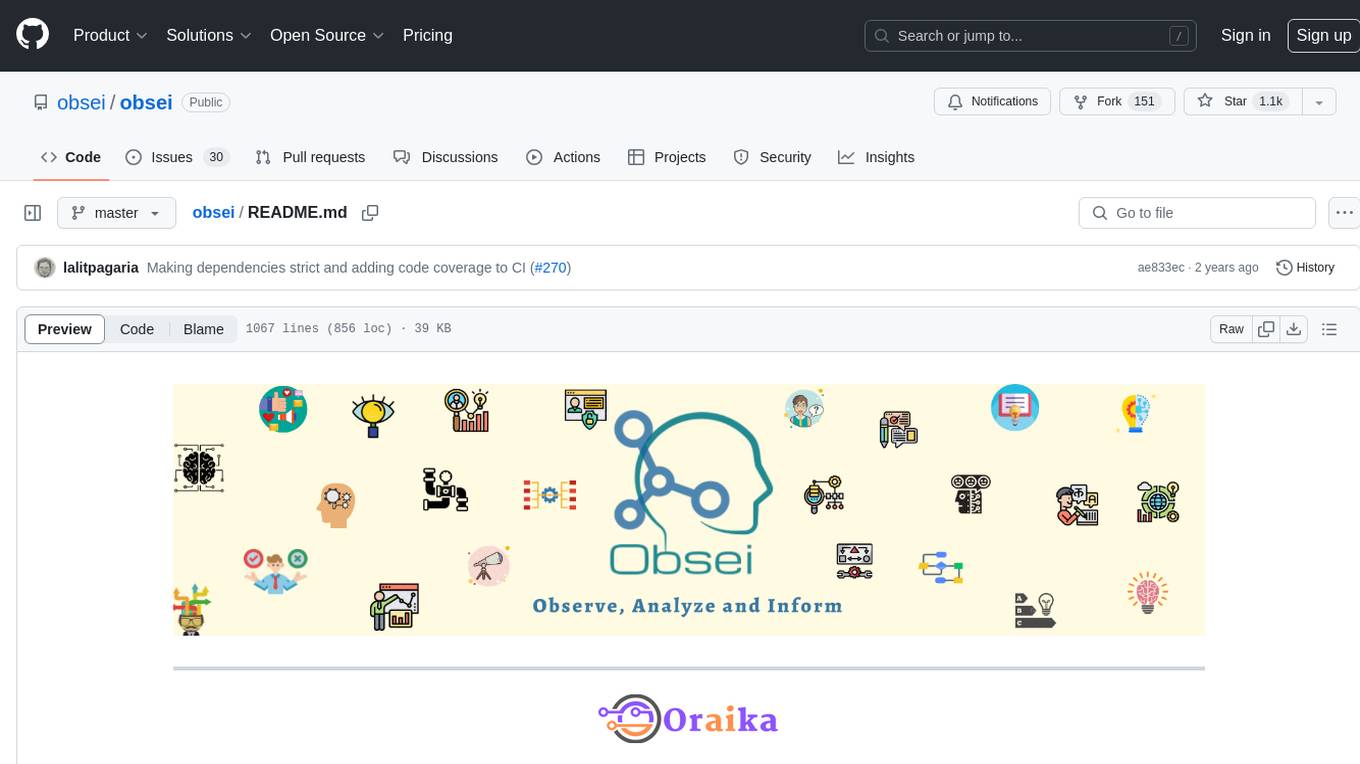
obsei
Obsei is an open-source, low-code, AI powered automation tool that consists of an Observer to collect unstructured data from various sources, an Analyzer to analyze the collected data with various AI tasks, and an Informer to send analyzed data to various destinations. The tool is suitable for scheduled jobs or serverless applications as all Observers can store their state in databases. Obsei is still in alpha stage, so caution is advised when using it in production. The tool can be used for social listening, alerting/notification, automatic customer issue creation, extraction of deeper insights from feedbacks, market research, dataset creation for various AI tasks, and more based on creativity.
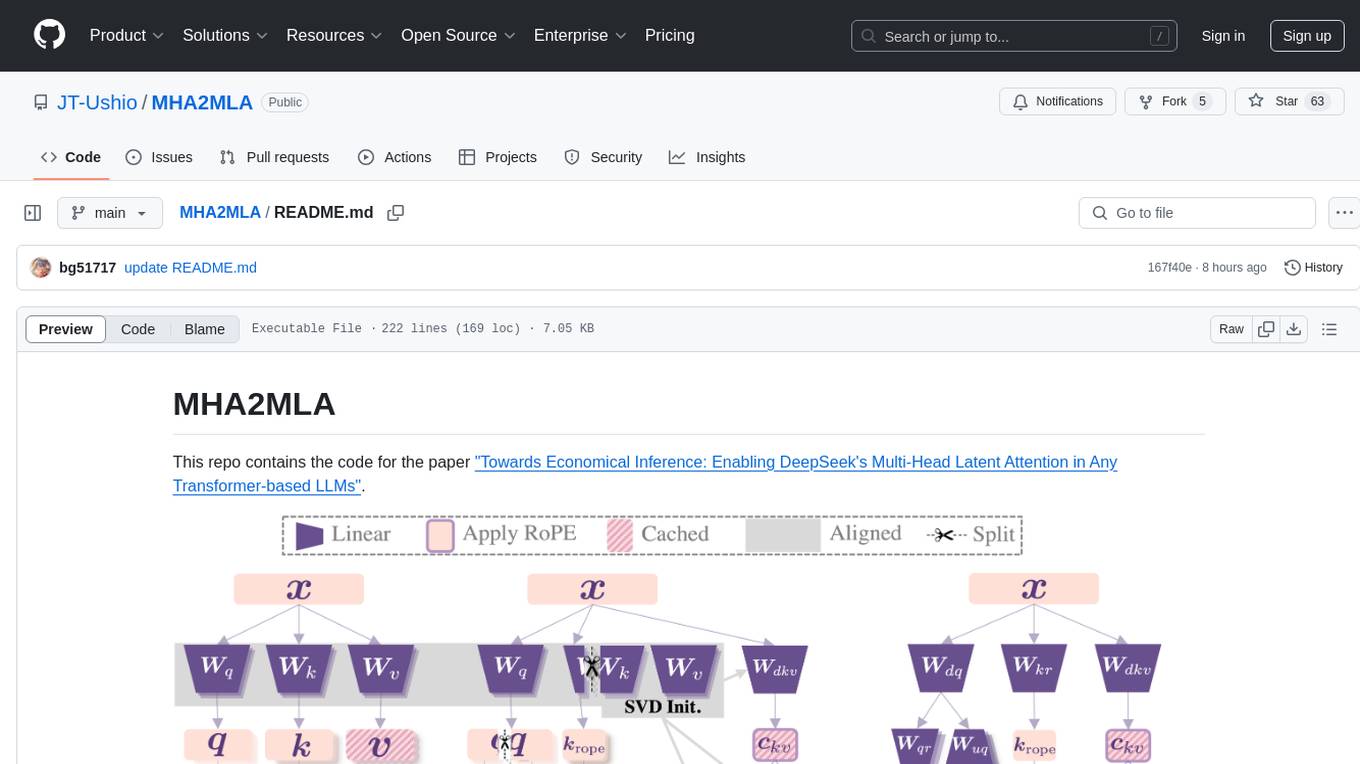
MHA2MLA
This repository contains the code for the paper 'Towards Economical Inference: Enabling DeepSeek's Multi-Head Latent Attention in Any Transformer-based LLMs'. It provides tools for fine-tuning and evaluating Llama models, converting models between different frameworks, processing datasets, and performing specific model training tasks like Partial-RoPE Fine-Tuning and Multiple-Head Latent Attention Fine-Tuning. The repository also includes commands for model evaluation using Lighteval and LongBench, along with necessary environment setup instructions.
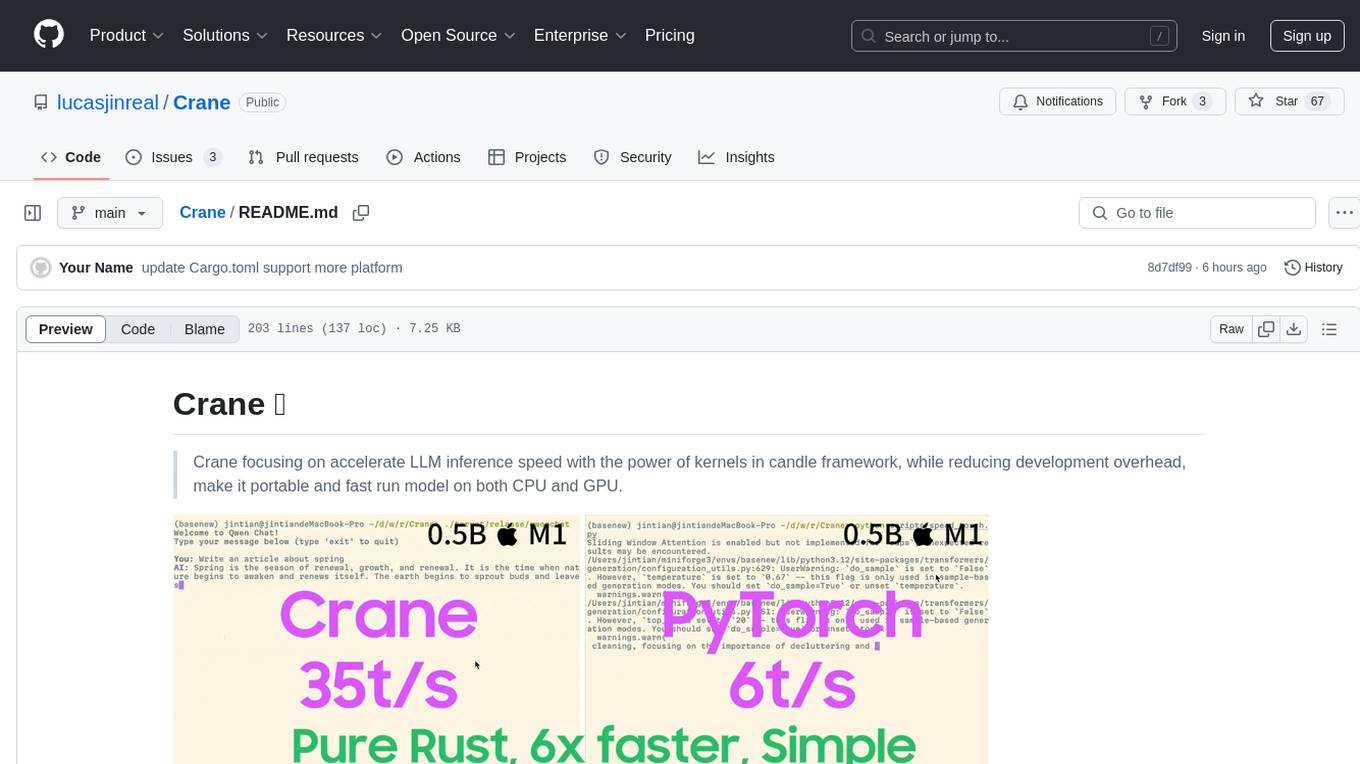
Crane
Crane is a high-performance inference framework leveraging Rust's Candle for maximum speed on CPU/GPU. It focuses on accelerating LLM inference speed with optimized kernels, reducing development overhead, and ensuring portability for running models on both CPU and GPU. Supported models include TTS systems like Spark-TTS and Orpheus-TTS, foundation models like Qwen2.5 series and basic LLMs, and multimodal models like Namo-R1 and Qwen2.5-VL. Key advantages of Crane include blazing-fast inference outperforming native PyTorch, Rust-powered to eliminate C++ complexity, Apple Silicon optimized for GPU acceleration via Metal, and hardware agnostic with a unified codebase for CPU/CUDA/Metal execution. Crane simplifies deployment with the ability to add new models with less than 100 lines of code in most cases.
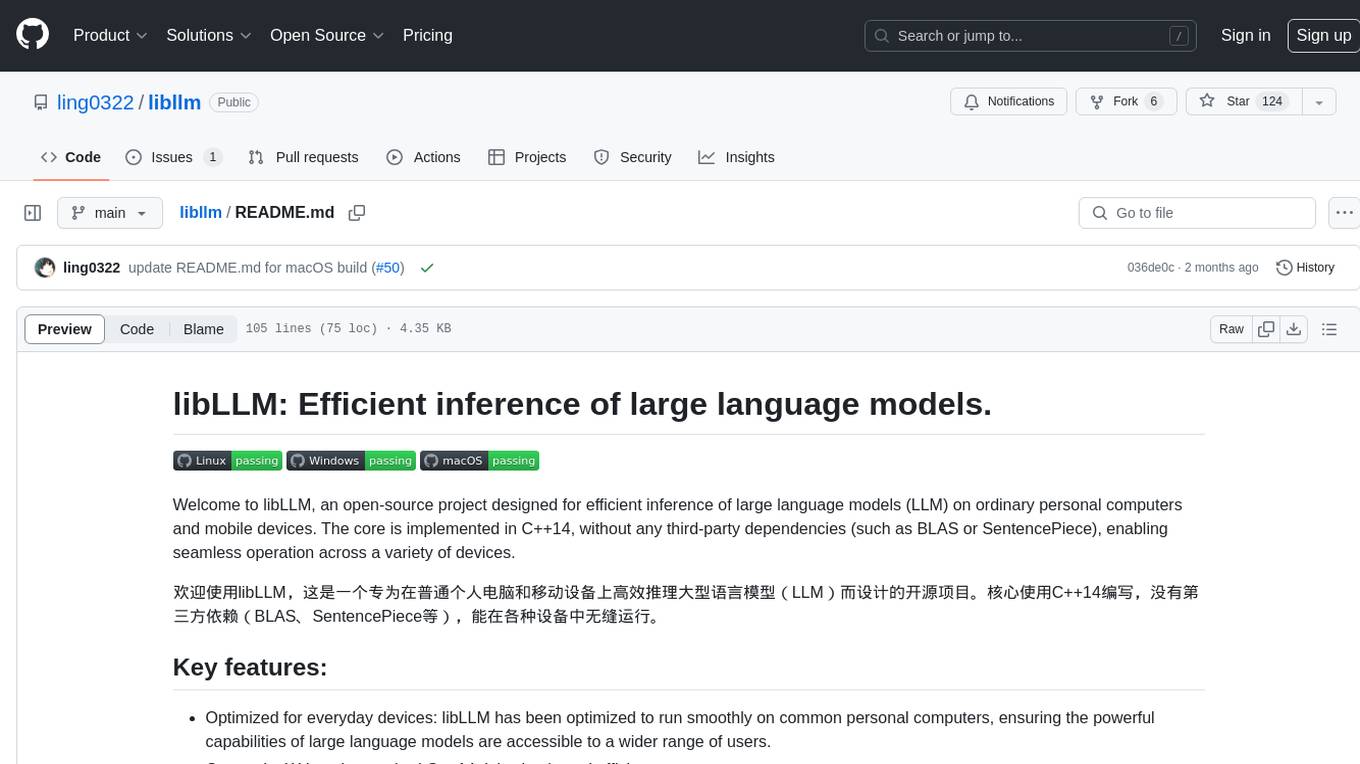
libllm
libLLM is an open-source project designed for efficient inference of large language models (LLM) on personal computers and mobile devices. It is optimized to run smoothly on common devices, written in C++14 without external dependencies, and supports CUDA for accelerated inference. Users can build the tool for CPU only or with CUDA support, and run libLLM from the command line. Additionally, there are API examples available for Python and the tool can export Huggingface models.
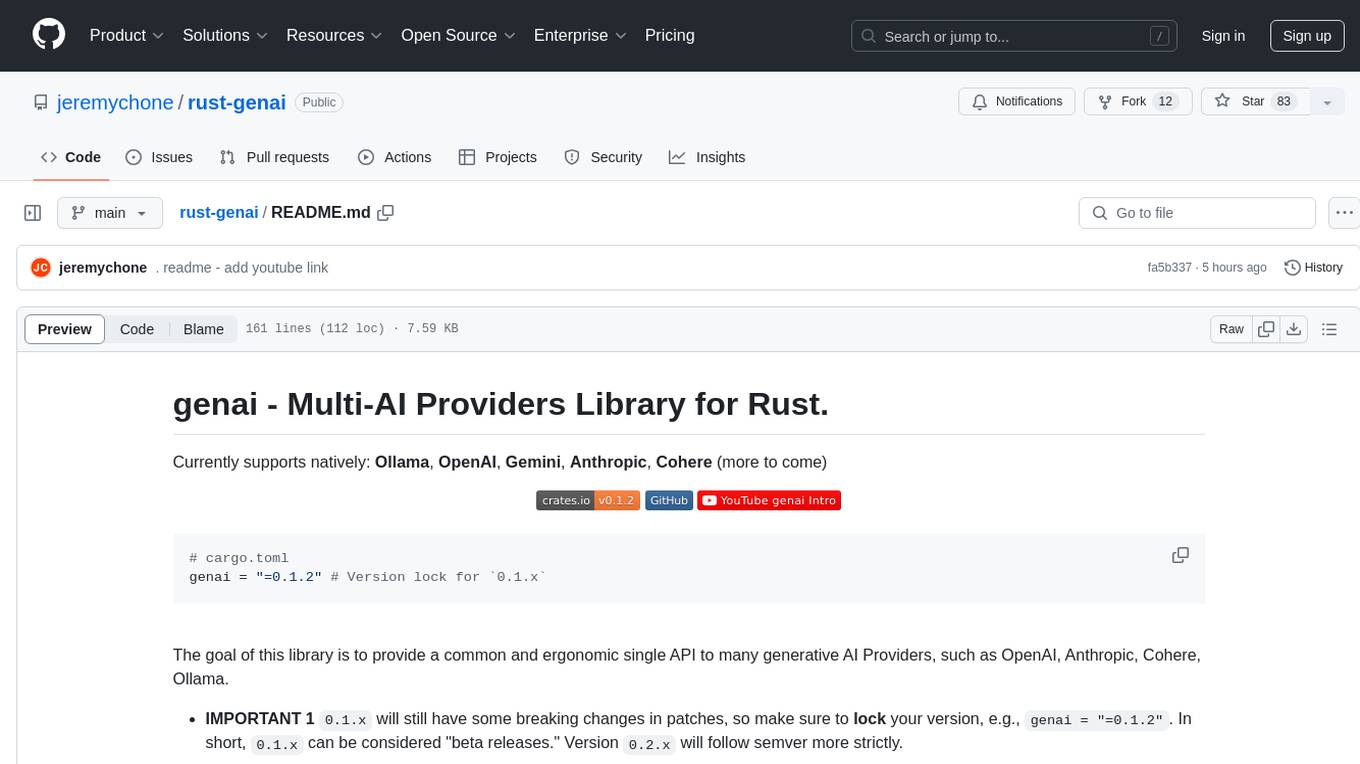
rust-genai
genai is a multi-AI providers library for Rust that aims to provide a common and ergonomic single API to various generative AI providers such as OpenAI, Anthropic, Cohere, Ollama, and Gemini. It focuses on standardizing chat completion APIs across major AI services, prioritizing ergonomics and commonality. The library initially focuses on text chat APIs and plans to expand to support images, function calling, and more in the future versions. Version 0.1.x will have breaking changes in patches, while version 0.2.x will follow semver more strictly. genai does not provide a full representation of a given AI provider but aims to simplify the differences at a lower layer for ease of use.
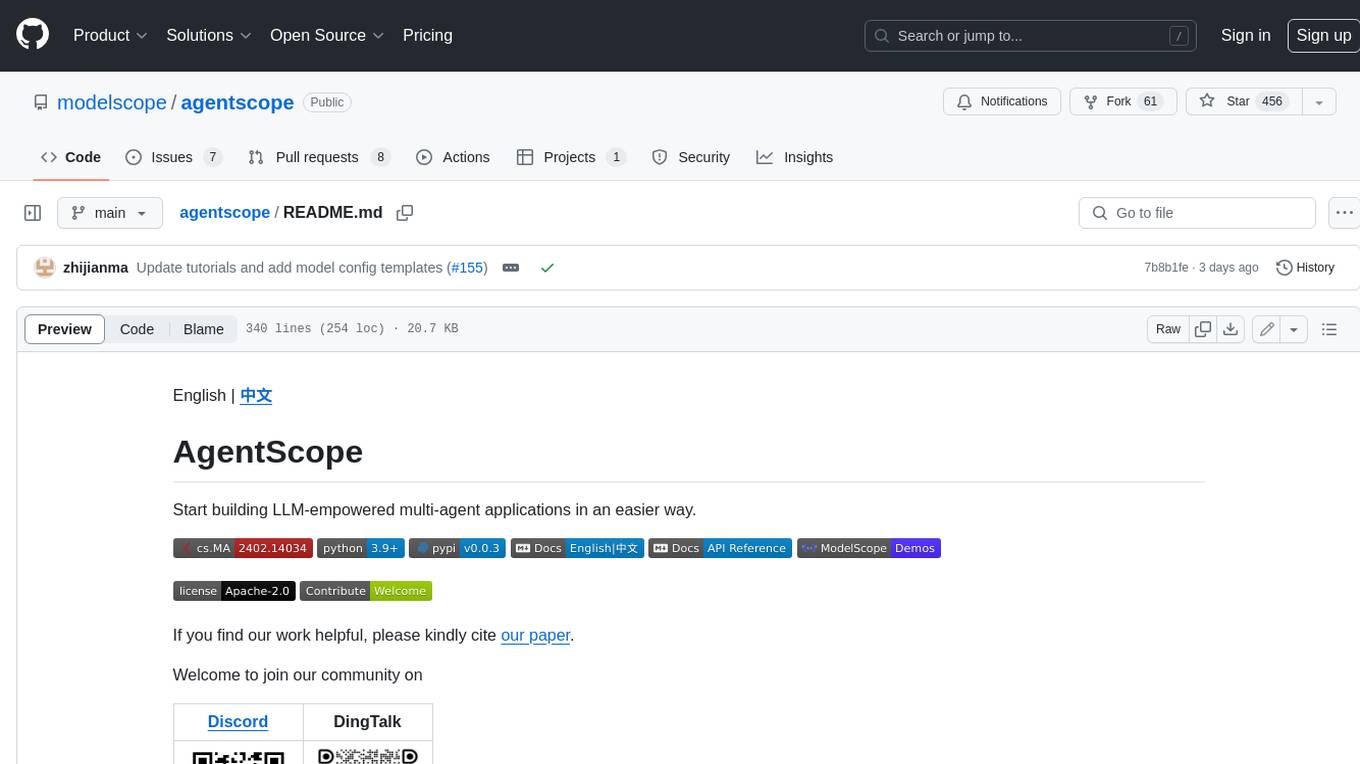
agentscope
AgentScope is a multi-agent platform designed to empower developers to build multi-agent applications with large-scale models. It features three high-level capabilities: Easy-to-Use, High Robustness, and Actor-Based Distribution. AgentScope provides a list of `ModelWrapper` to support both local model services and third-party model APIs, including OpenAI API, DashScope API, Gemini API, and ollama. It also enables developers to rapidly deploy local model services using libraries such as ollama (CPU inference), Flask + Transformers, Flask + ModelScope, FastChat, and vllm. AgentScope supports various services, including Web Search, Data Query, Retrieval, Code Execution, File Operation, and Text Processing. Example applications include Conversation, Game, and Distribution. AgentScope is released under Apache License 2.0 and welcomes contributions.
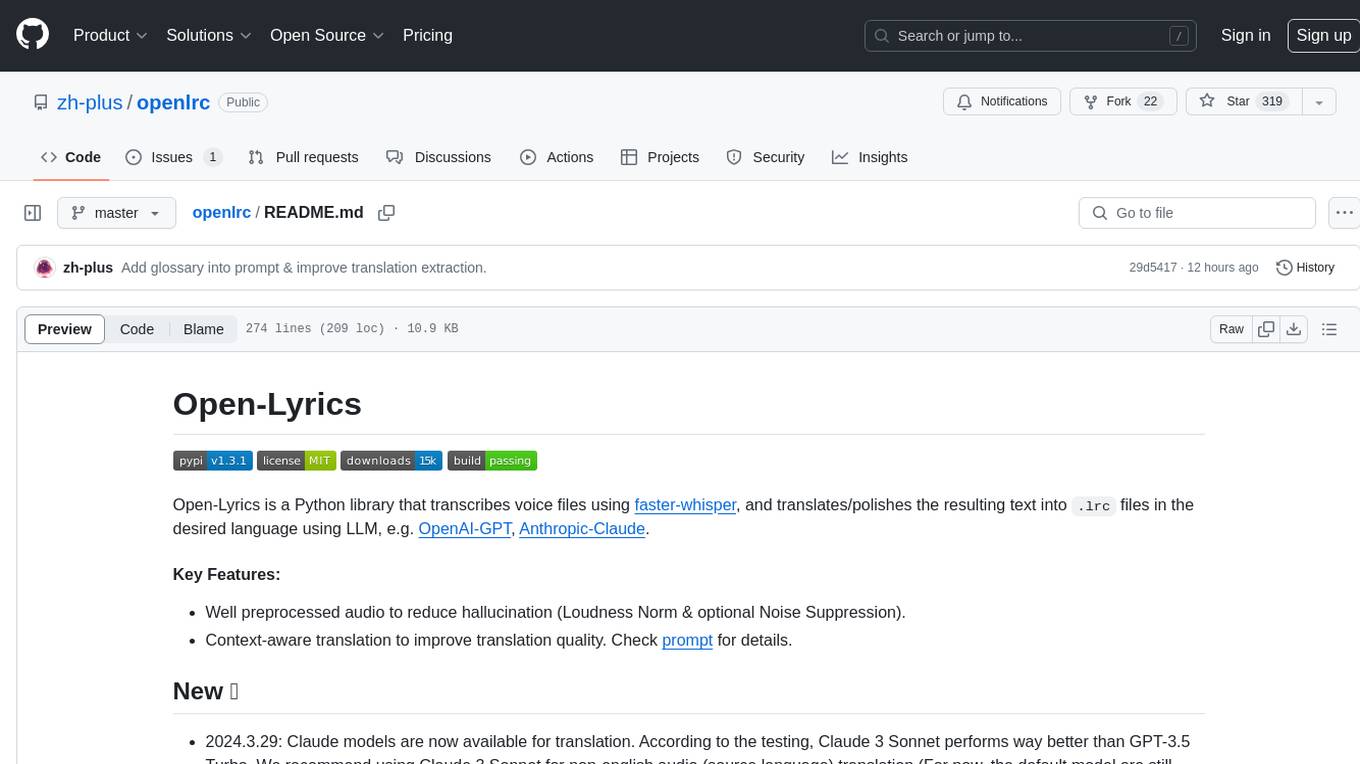
openlrc
Open-Lyrics is a Python library that transcribes voice files using faster-whisper and translates/polishes the resulting text into `.lrc` files in the desired language using LLM, e.g. OpenAI-GPT, Anthropic-Claude. It offers well preprocessed audio to reduce hallucination and context-aware translation to improve translation quality. Users can install the library from PyPI or GitHub and follow the installation steps to set up the environment. The tool supports GUI usage and provides Python code examples for transcription and translation tasks. It also includes features like utilizing context and glossary for translation enhancement, pricing information for different models, and a list of todo tasks for future improvements.
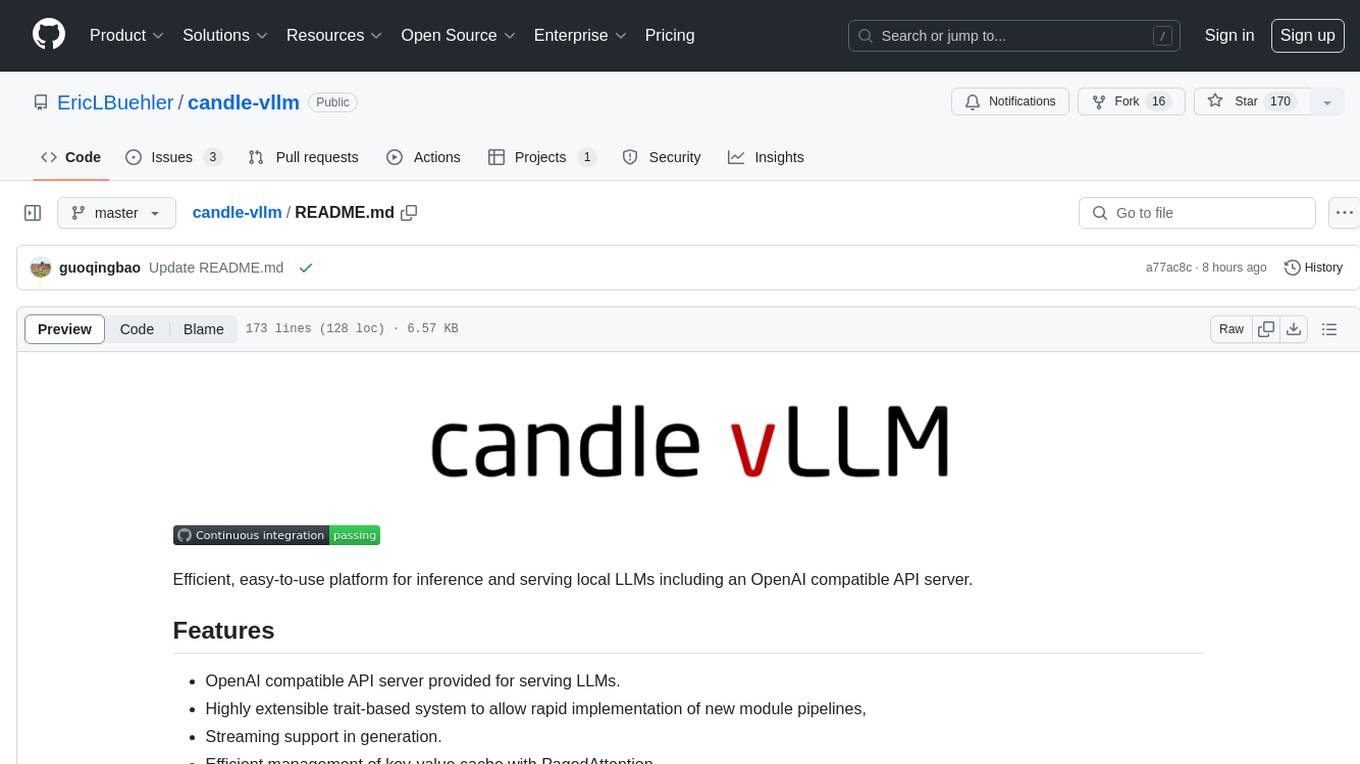
candle-vllm
Candle-vllm is an efficient and easy-to-use platform designed for inference and serving local LLMs, featuring an OpenAI compatible API server. It offers a highly extensible trait-based system for rapid implementation of new module pipelines, streaming support in generation, efficient management of key-value cache with PagedAttention, and continuous batching. The tool supports chat serving for various models and provides a seamless experience for users to interact with LLMs through different interfaces.
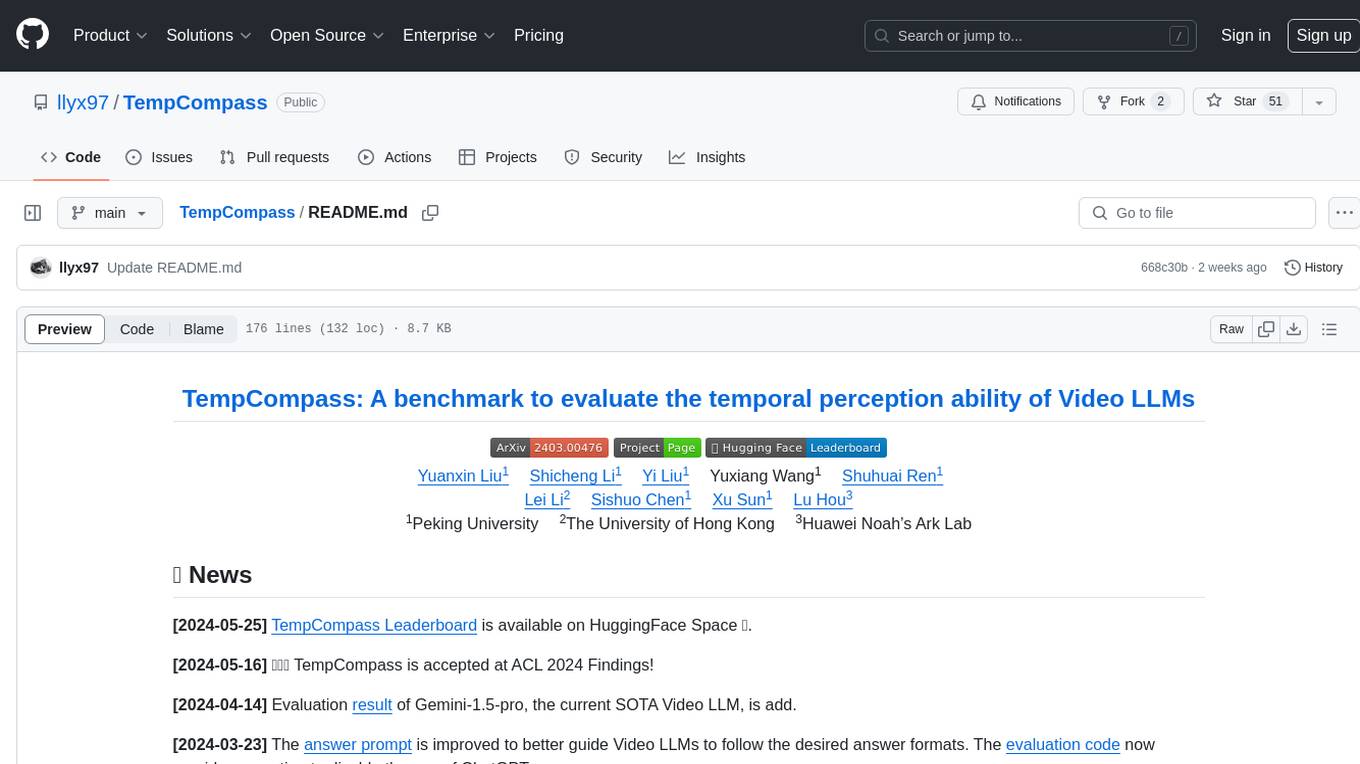
TempCompass
TempCompass is a benchmark designed to evaluate the temporal perception ability of Video LLMs. It encompasses a diverse set of temporal aspects and task formats to comprehensively assess the capability of Video LLMs in understanding videos. The benchmark includes conflicting videos to prevent models from relying on single-frame bias and language priors. Users can clone the repository, install required packages, prepare data, run inference using examples like Video-LLaVA and Gemini, and evaluate the performance of their models across different tasks such as Multi-Choice QA, Yes/No QA, Caption Matching, and Caption Generation.
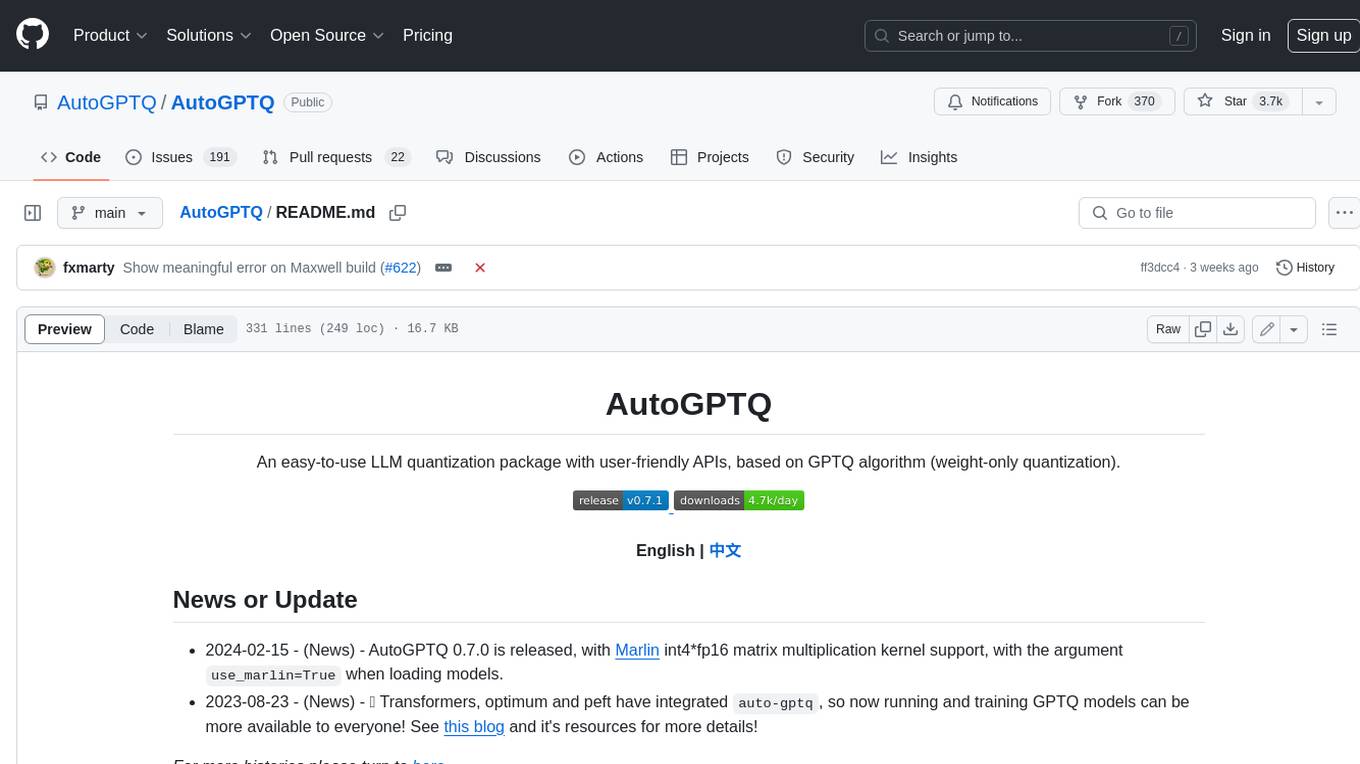
AutoGPTQ
AutoGPTQ is an easy-to-use LLM quantization package with user-friendly APIs, based on GPTQ algorithm (weight-only quantization). It provides a simple and efficient way to quantize large language models (LLMs) to reduce their size and computational cost while maintaining their performance. AutoGPTQ supports a wide range of LLM models, including GPT-2, GPT-J, OPT, and BLOOM. It also supports various evaluation tasks, such as language modeling, sequence classification, and text summarization. With AutoGPTQ, users can easily quantize their LLM models and deploy them on resource-constrained devices, such as mobile phones and embedded systems.
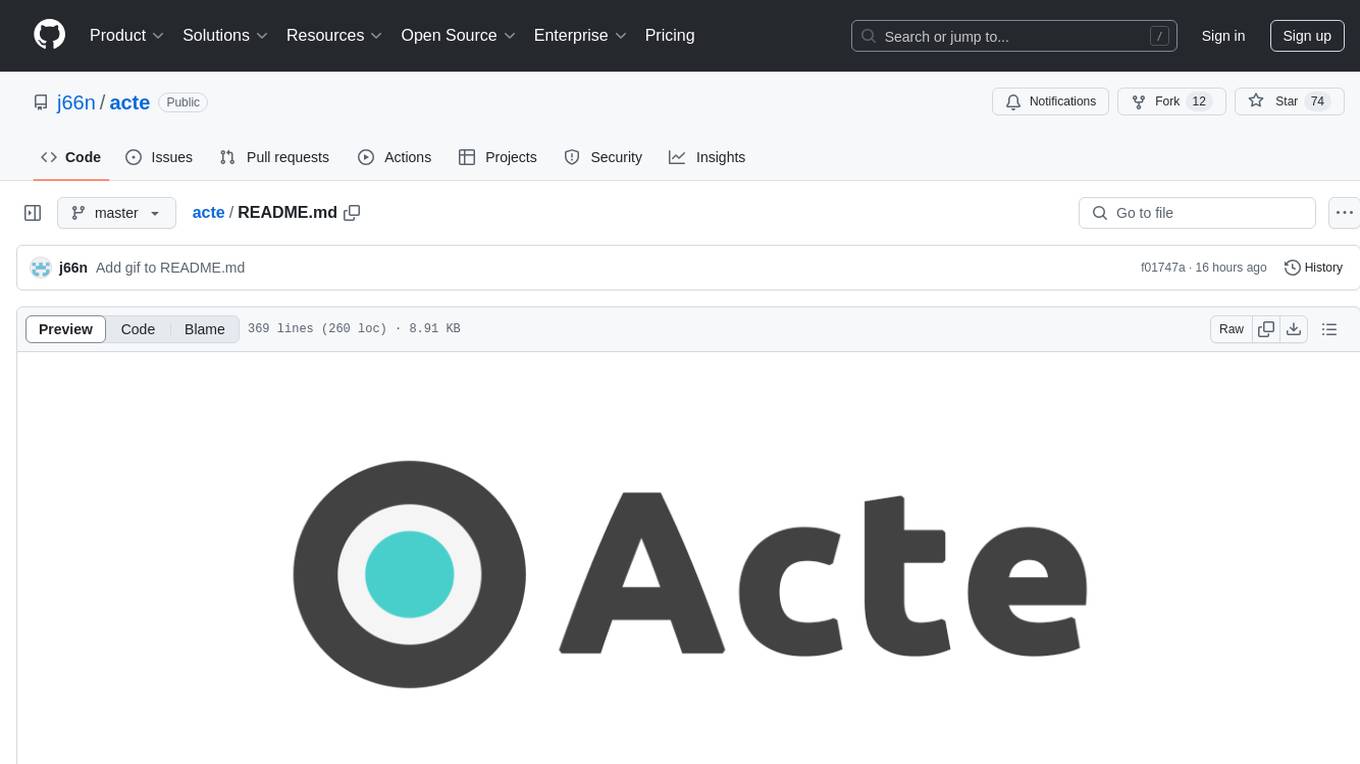
acte
Acte is a framework designed to build GUI-like tools for AI Agents. It aims to address the issues of cognitive load and freedom degrees when interacting with multiple APIs in complex scenarios. By providing a graphical user interface (GUI) for Agents, Acte helps reduce cognitive load and constraints interaction, similar to how humans interact with computers through GUIs. The tool offers APIs for starting new sessions, executing actions, and displaying screens, accessible via HTTP requests or the SessionManager class.
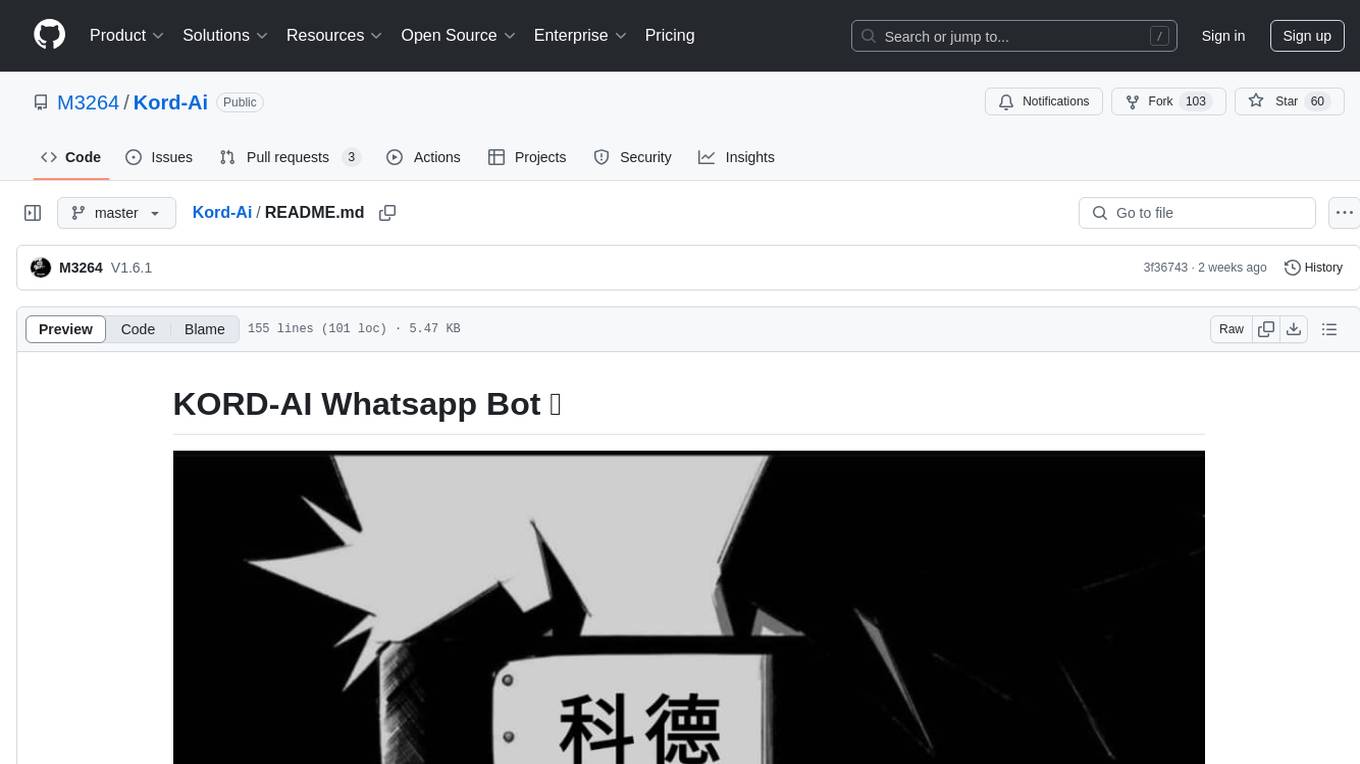
Kord-Ai
Kord-Ai is a WhatsApp bot designed to automate interactions on WhatsApp by executing predefined commands or responding to user inputs. It can handle tasks like sending messages, sharing media, and managing group activities, providing convenience and efficiency for users and businesses. The bot offers features for deployment on various platforms, including Heroku, Replit, Koyeb, Glitch, Codespace, Render, Railway, VPS, and PC. Users can deploy the bot by obtaining a session ID, forking the repository, setting configurations in the Config.js file, and starting/stopping the bot using npm commands. It is important to note that Kord-Ai is a bot created by M3264, not affiliated with WhatsApp, and users should be cautious in its usage.
For similar tasks
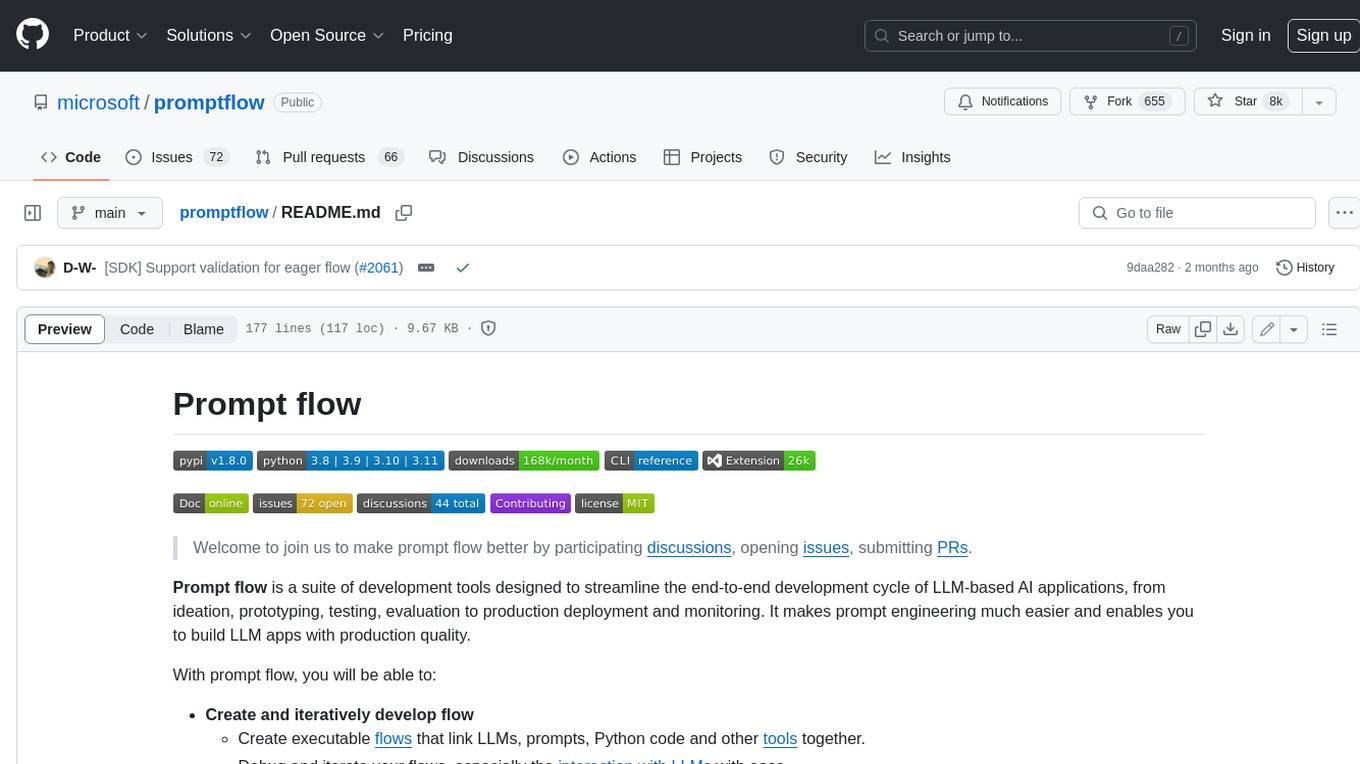
promptflow
**Prompt flow** is a suite of development tools designed to streamline the end-to-end development cycle of LLM-based AI applications, from ideation, prototyping, testing, evaluation to production deployment and monitoring. It makes prompt engineering much easier and enables you to build LLM apps with production quality.
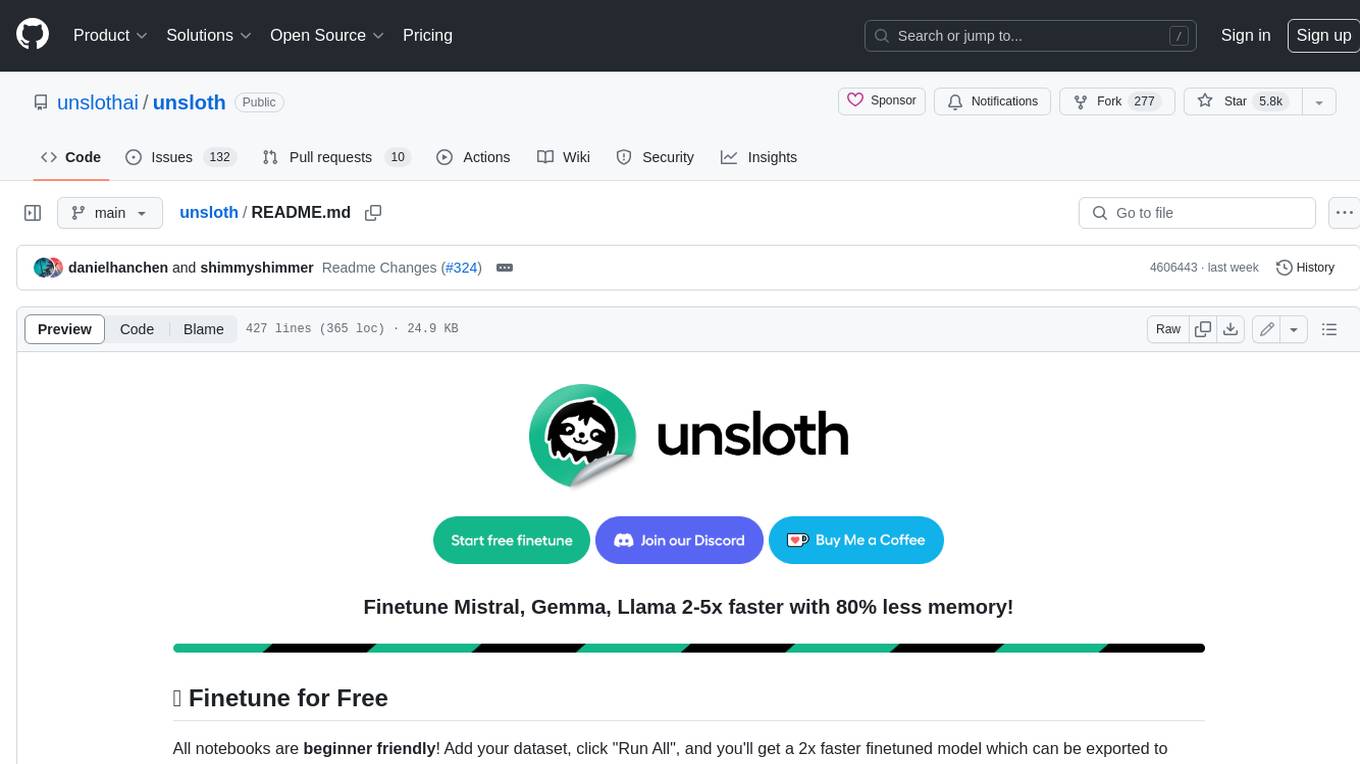
unsloth
Unsloth is a tool that allows users to fine-tune large language models (LLMs) 2-5x faster with 80% less memory. It is a free and open-source tool that can be used to fine-tune LLMs such as Gemma, Mistral, Llama 2-5, TinyLlama, and CodeLlama 34b. Unsloth supports 4-bit and 16-bit QLoRA / LoRA fine-tuning via bitsandbytes. It also supports DPO (Direct Preference Optimization), PPO, and Reward Modelling. Unsloth is compatible with Hugging Face's TRL, Trainer, Seq2SeqTrainer, and Pytorch code. It is also compatible with NVIDIA GPUs since 2018+ (minimum CUDA Capability 7.0).
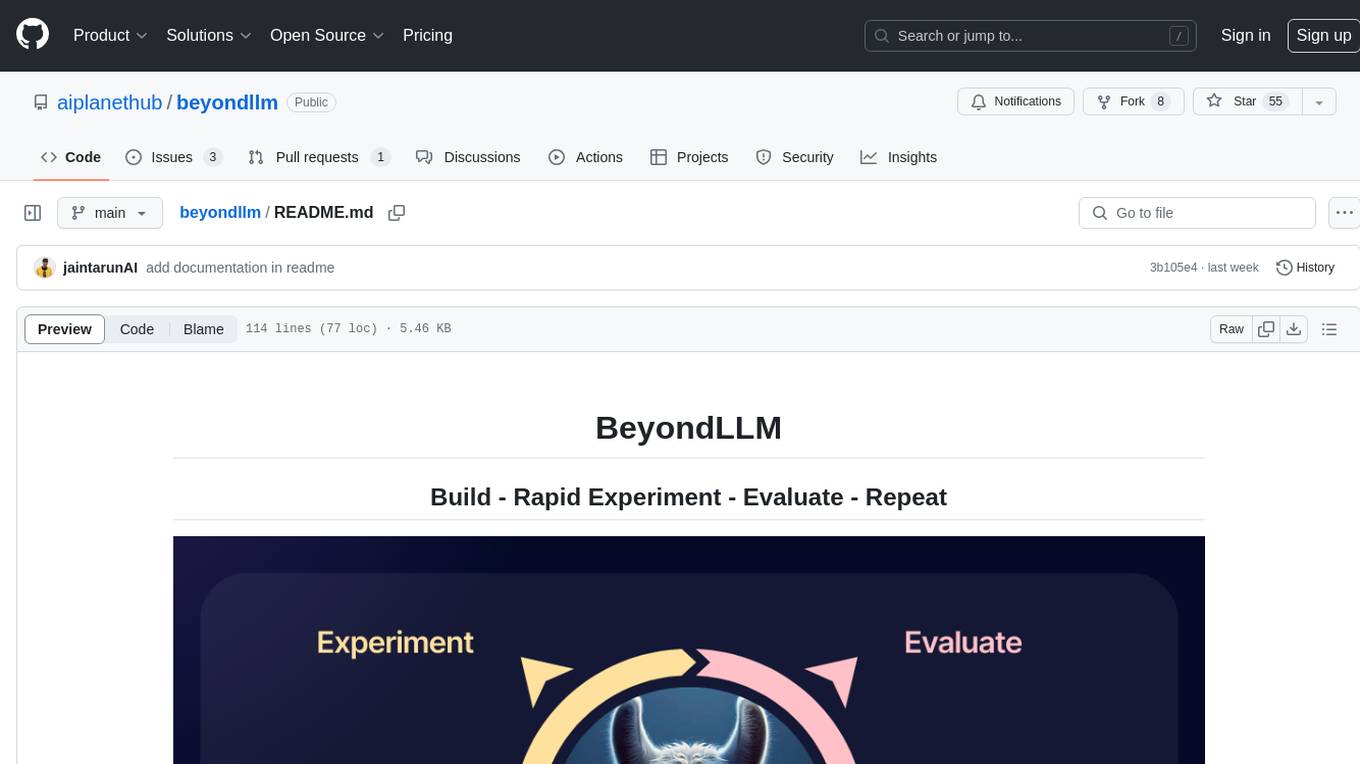
beyondllm
Beyond LLM offers an all-in-one toolkit for experimentation, evaluation, and deployment of Retrieval-Augmented Generation (RAG) systems. It simplifies the process with automated integration, customizable evaluation metrics, and support for various Large Language Models (LLMs) tailored to specific needs. The aim is to reduce LLM hallucination risks and enhance reliability.
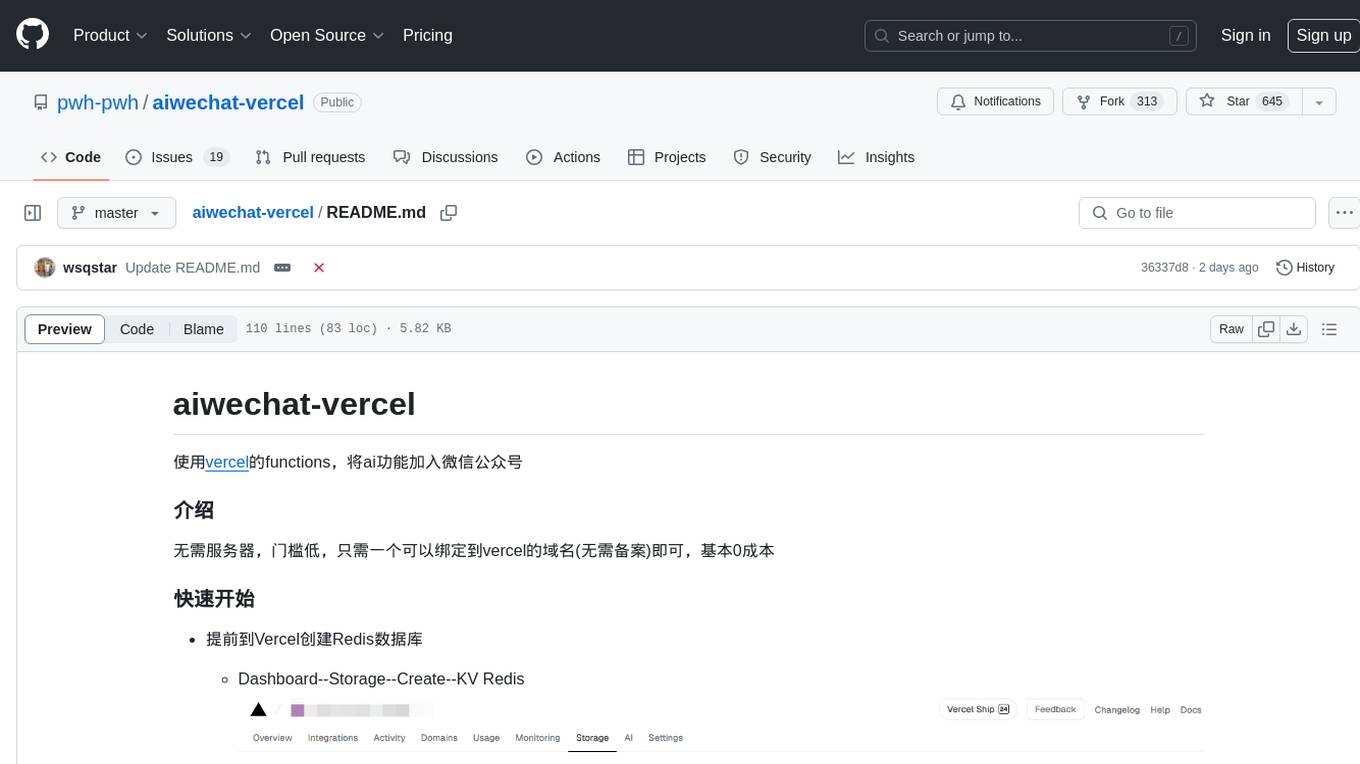
aiwechat-vercel
aiwechat-vercel is a tool that integrates AI capabilities into WeChat public accounts using Vercel functions. It requires minimal server setup, low entry barriers, and only needs a domain name that can be bound to Vercel, with almost zero cost. The tool supports various AI models, continuous Q&A sessions, chat functionality, system prompts, and custom commands. It aims to provide a platform for learning and experimentation with AI integration in WeChat public accounts.
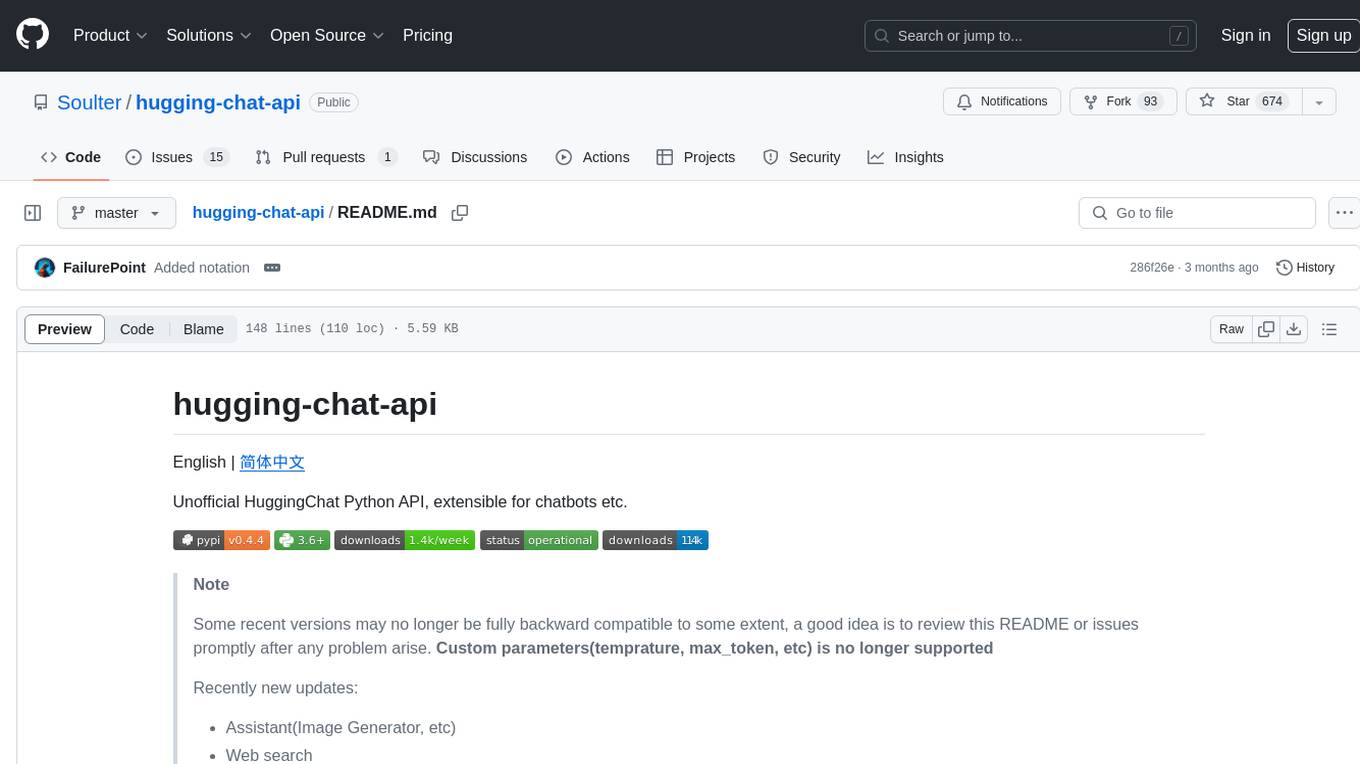
hugging-chat-api
Unofficial HuggingChat Python API for creating chatbots, supporting features like image generation, web search, memorizing context, and changing LLMs. Users can log in, chat with the ChatBot, perform web searches, create new conversations, manage conversations, switch models, get conversation info, use assistants, and delete conversations. The API also includes a CLI mode with various commands for interacting with the tool. Users are advised not to use the application for high-stakes decisions or advice and to avoid high-frequency requests to preserve server resources.
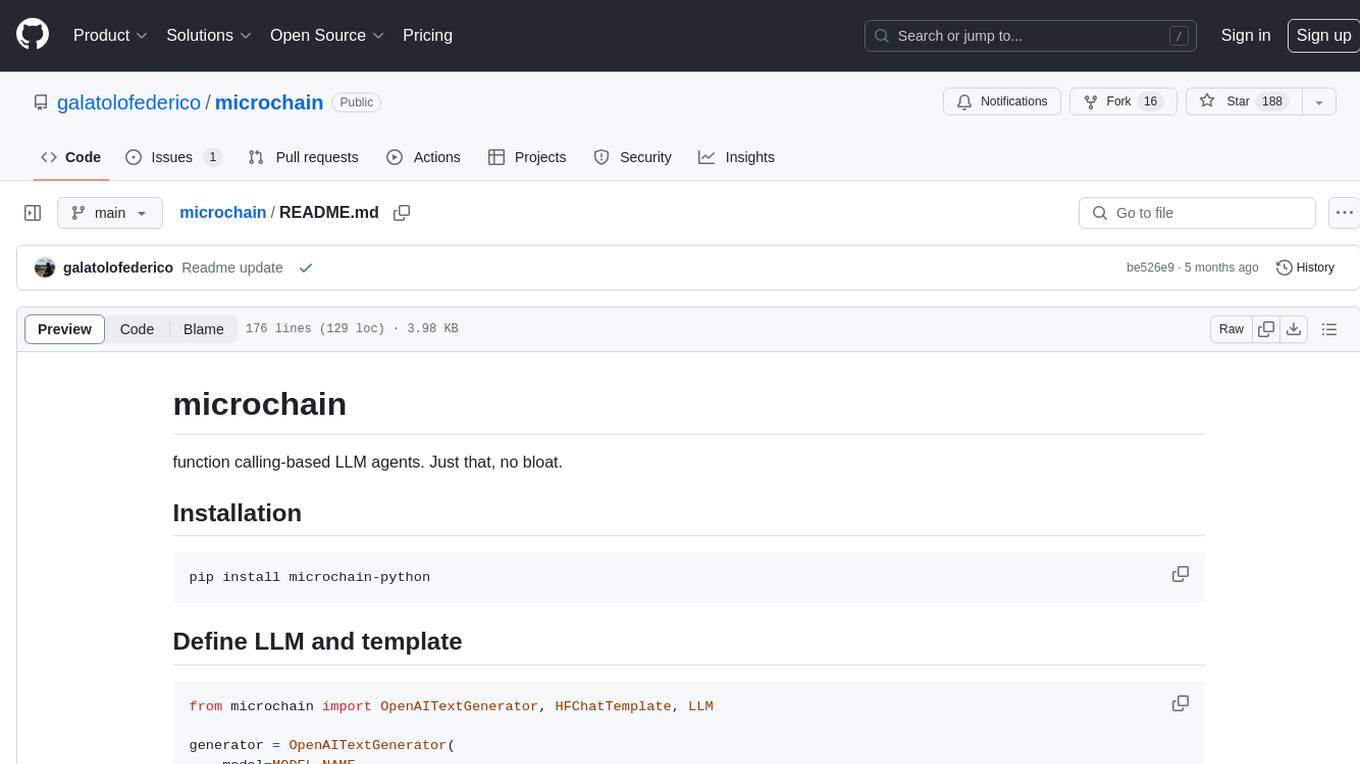
microchain
Microchain is a function calling-based LLM agents tool with no bloat. It allows users to define LLM and templates, use various functions like Sum and Product, and create LLM agents for specific tasks. The tool provides a simple and efficient way to interact with OpenAI models and create conversational agents for various applications.
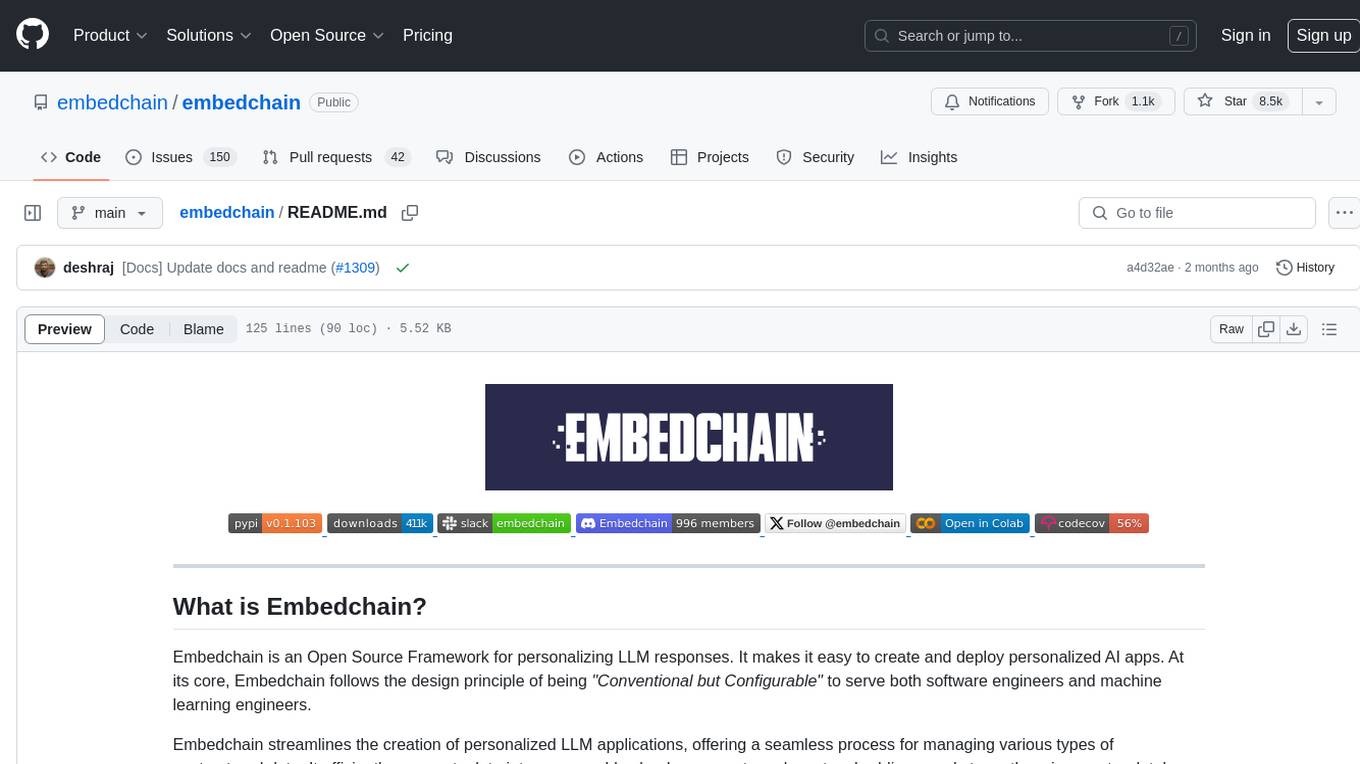
embedchain
Embedchain is an Open Source Framework for personalizing LLM responses. It simplifies the creation and deployment of personalized AI applications by efficiently managing unstructured data, generating relevant embeddings, and storing them in a vector database. With diverse APIs, users can extract contextual information, find precise answers, and engage in interactive chat conversations tailored to their data. The framework follows the design principle of being 'Conventional but Configurable' to cater to both software engineers and machine learning engineers.
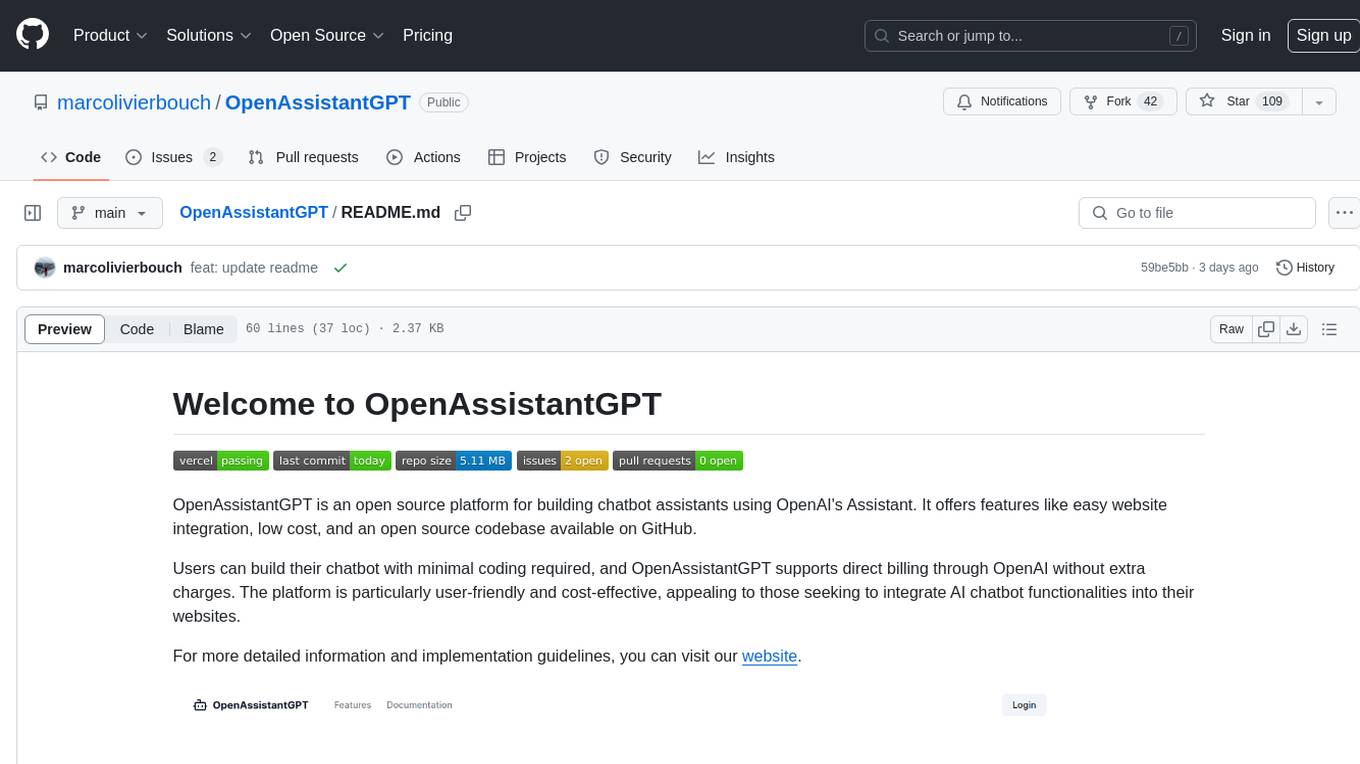
OpenAssistantGPT
OpenAssistantGPT is an open source platform for building chatbot assistants using OpenAI's Assistant. It offers features like easy website integration, low cost, and an open source codebase available on GitHub. Users can build their chatbot with minimal coding required, and OpenAssistantGPT supports direct billing through OpenAI without extra charges. The platform is user-friendly and cost-effective, appealing to those seeking to integrate AI chatbot functionalities into their websites.
For similar jobs
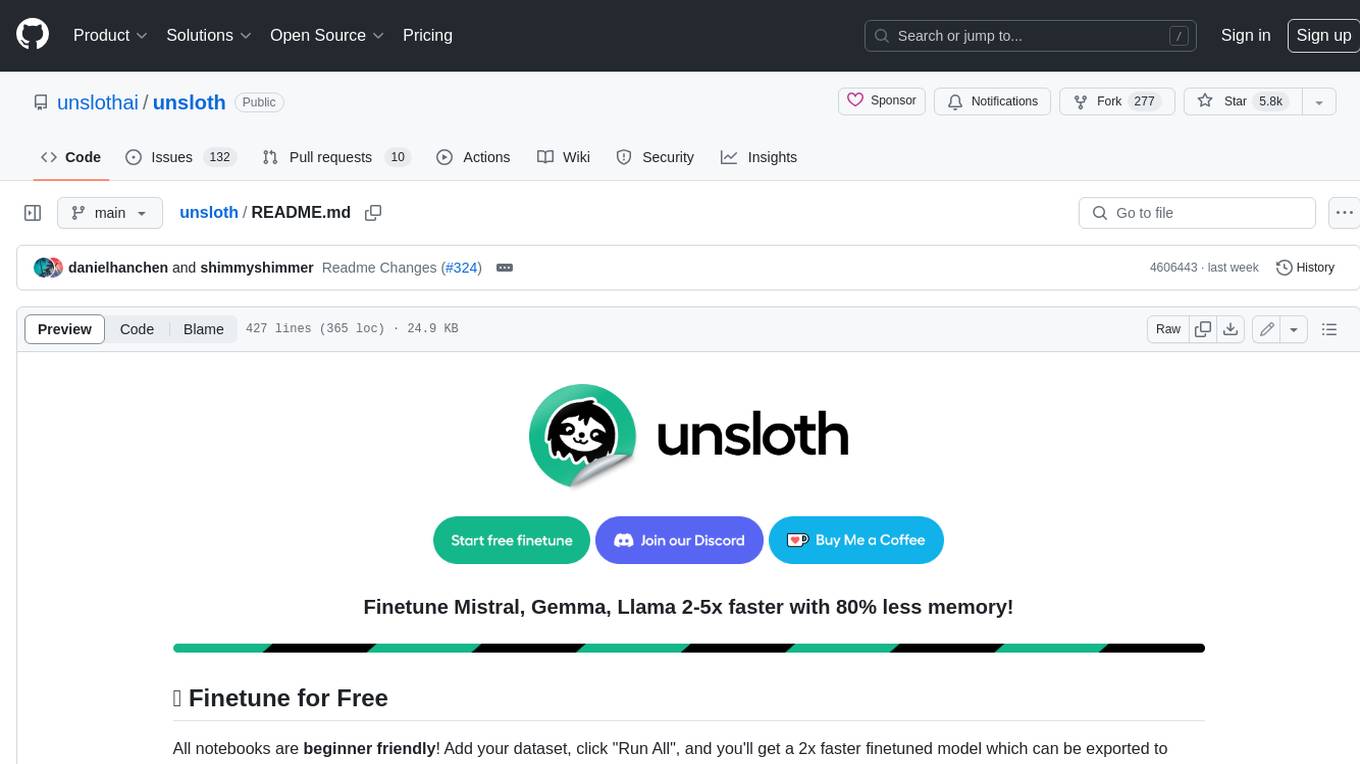
unsloth
Unsloth is a tool that allows users to fine-tune large language models (LLMs) 2-5x faster with 80% less memory. It is a free and open-source tool that can be used to fine-tune LLMs such as Gemma, Mistral, Llama 2-5, TinyLlama, and CodeLlama 34b. Unsloth supports 4-bit and 16-bit QLoRA / LoRA fine-tuning via bitsandbytes. It also supports DPO (Direct Preference Optimization), PPO, and Reward Modelling. Unsloth is compatible with Hugging Face's TRL, Trainer, Seq2SeqTrainer, and Pytorch code. It is also compatible with NVIDIA GPUs since 2018+ (minimum CUDA Capability 7.0).