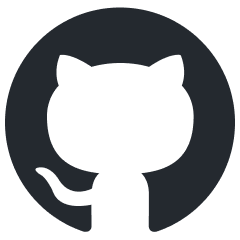
UMbreLLa
LLM Inference on consumer devices
Stars: 94
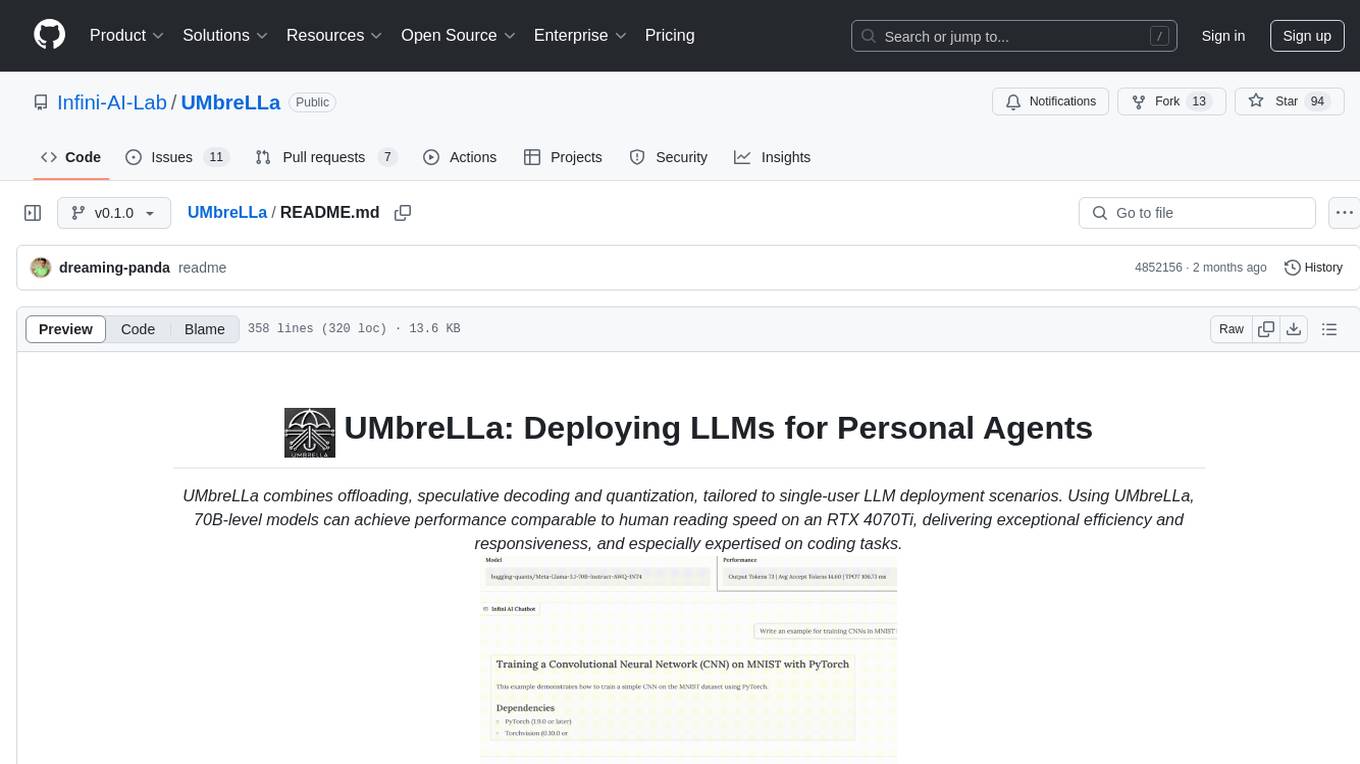
UMbreLLa is a tool designed for deploying Large Language Models (LLMs) for personal agents. It combines offloading, speculative decoding, and quantization to optimize single-user LLM deployment scenarios. With UMbreLLa, 70B-level models can achieve performance comparable to human reading speed on an RTX 4070Ti, delivering exceptional efficiency and responsiveness, especially for coding tasks. The tool supports deploying models on various GPUs and offers features like code completion and CLI/Gradio chatbots. Users can configure the LLM engine for optimal performance based on their hardware setup.
README:
The throughput is measured with a batch size of 1 to directly mirror the user experience.
GPU | Model | Draft | Throughput (tokens/sec) | |
---|---|---|---|---|
Stochastic | Greedy | |||
RTX 4090 | Llama3.1-70B-Instruct-AWQ | Llama3.1-8B-Instruct-AWQ | 7.2 | 8.6 |
Llama3.3-70B-Instruct-AWQ | Llama3.1-8B-Instruct-AWQ | 7.0 | 7.4 | |
Llama3.1-8B-Instruct | Llama3.2-1B-Instruct | 100.7 | 108.1 | |
RTX 4080 SUPER | Llama3.1-70B-Instruct-AWQ | Llama3.1-8B-Instruct-AWQ | 7.4 | 8.4 |
Llama3.3-70B-Instruct-AWQ | Llama3.1-8B-Instruct-AWQ | 6.7 | 7.2 | |
RTX 4070 Ti | Llama3.1-70B-Instruct-AWQ | Llama3.2-1B-Instruct | 5.5 | 6.1 |
Llama3.3-70B-Instruct-AWQ | Llama3.2-1B-Instruct | 5.2 | 5.5 | |
L40 | Llama3.1-70B-Instruct-AWQ | Llama3.2-1B-Instruct | 37.0 | 38.5 |
Llama3.3-70B-Instruct-AWQ | Llama3.2-1B-Instruct | 36.3 | 37.1 |
Evaluated on ananyarn/Algorithm_and_Python_Source_Code
.
GPU | Model | Draft | Throughput (tokens/sec) |
---|---|---|---|
RTX 4090 | Llama3.1-70B-Instruct-AWQ | Llama3.1-8B-Instruct-AWQ | 11.4 |
Llama3.3-70B-Instruct-AWQ | Llama3.1-8B-Instruct-AWQ | 11.2 | |
Llama3.1-8B-Instruct | CodeDrafter-500M | 174.8 | |
RTX 4080 SUPER | Llama3.1-70B-Instruct-AWQ | Llama3.1-8B-Instruct-AWQ | 12.2 |
Llama3.3-70B-Instruct-AWQ | Llama3.1-8B-Instruct-AWQ | 12.1 | |
Llama3.1-8B-Instruct-AWQ | CodeDrafter-500M | 195.3 | |
RTX 4070 Ti | Llama3.1-70B-Instruct-AWQ | Llama3.2-1B-Instruct | 9.7 |
Llama3.3-70B-Instruct-AWQ | Llama3.2-1B-Instruct | 9.6 | |
Llama3.1-8B-Instruct-AWQ | CodeDrafter-500M | 162.3 | |
L40 | Llama3.1-70B-Instruct-AWQ | CodeDrafter-500M | 45.6 |
Llama3.3-70B-Instruct-AWQ | CodeDrafter-500M | 45.0 |
Offloading experiments heavily rely on the status of PCIE, and may vary across instances.
❌ UMbreLLa is not designed for large-scale LLM serving.
conda create -n umbrella python=3.10
bash install.sh
cd app
python chatbot.py --configuration ../configs/chat_config_24gb.json
Then you can chat with the LLM specified in chat_config_24gb.json
.
cd app
python gradio_chat.py --configuration ../configs/chat_config_24gb.json
Then you can chat with the LLM specified in chat_config_24gb.json
in Gradio.
cd app
python api.py --configuration ../configs/chat_config_24gb.json --max_client 1 --port 65432
configuration
specifies the LLM and speculative decoding details.
max_client
is the maximum clients that can connect to the server.
port
is the port of the server.
After the server is started, Client can be started and connect to the server by
from umbrella.api.client import APIClient
client = APIClient(port=port) #port should be the same as the server
client.run()
To get the LLM output,
input1 = {"context": text1, "max_new_tokens": 512, "temperature": 0.0}
output1 = client.get_output(**input1)
{
"model": "hugging-quants/Meta-Llama-3.1-70B-Instruct-AWQ-INT4",
"draft_model": "meta-llama/Llama-3.2-1B-Instruct",
"offload": true,
"cuda_graph": false,
"max_length": 4096,
"num_cache_layers": 0,
"generation_length": 256,
"max_turns": 12,
"topk": 32,
"temperature": 0.6,
"topp": 0.9,
"repetition_penalty": 1.05,
"growmap_path": "../umbrella/trees/sequoia_tree-3x4.json",
"width": 16,
"num_beams": 24,
"depth": 16,
"engine": "dynamic",
"template": "meta-llama3"
}
-
model: Specifies the target LLM to serve, e.g.,
"hugging-quants/Meta-Llama-3.1-70B-Instruct-AWQ-INT4"
. -
draft_model: Lightweight draft model, e.g.,
"meta-llama/Llama-3.2-1B-Instruct"
. -
offload: Enables offloading of the target model to host memory or disk (
true
orfalse
). - cuda_graph: Toggles CUDA graph optimization for the draft model (currently unsupported for AWQ models).
- max_length: The maximum token length for input and output combined.
- num_cache_layers: Sets the number of layers cached during inference (e.g., for memory optimization).
- generation_length: Maximum length of generated responses in tokens.
- max_turns: Limits the number of conversational turns retained in memory.
-
topk: Limits token selection during generation to the top
k
most likely tokens. - temperature: Controls randomness in token selection (lower values = more deterministic outputs).
-
topp: Enables nucleus sampling by limiting token selection to those with cumulative probability ≤
p
. - repetition_penalty: Penalizes repetitive text generation (values > 1 discourage repetition).
-
growmap_path: Path to the speculative decoding tree used by the static engine (e.g.,
"../umbrella/trees/sequoia_tree-3x4.json"
).
-
engine: Defines the decoding strategy. Choose between:
-
"static"
: Optimized for on-device execution. -
"dynamic"
: Designed for offloading scenarios.
-
- width, num_beams, depth: Hyperparameters for speculative decoding in dynamic engines.
-
template: Defines the structure for input prompts. Supported values include:
-
"llama3-code"
: Optimized for code-related tasks. -
"meta-llama3"
: General-purpose instruction-following template.
-
./configs
and ./umbrella/trees
.
from umbrella.speculation.auto_engine import AutoEngine
DEVICE = "cuda:0"
engine = AutoEngine.from_config(device=DEVICE, **config)
engine.initialize()
GEN_LEN = 512
text1 = "Tell me what you know about Reinforcement Learning in 100 words."
text2 = "Tell me what you know about LSH in 100 words."
engine.prefill(text1) # The first operation must be prefilling
engine.speculative_decoding(max_new_tokens=GEN_LEN)
engine.append(text2)
engine.speculative_decoding(max_new_tokens=GEN_LEN)
output = engine.generate(
context=prompt,
max_new_tokens=max_new_tokens,
temperature=temperature,
top_p=top_p,
repetition_penalty=repetition_penalty,
)
# return a dict containing token ids and detokenized texts
# context=prompt (str) can be replaced by input_ids=tokens list[int]
stream = engine.generate_stream(
context=prompt,
max_new_tokens=max_new_tokens,
temperature=temperature,
top_p=top_p,
repetition_penalty=repetition_penalty,
)
# return a stream containing detokenized texts
# context=prompt (str) can be replaced by input_ids=tokens list[int]
@article{chen2024sequoia,
title={Sequoia: Scalable, Robust, and Hardware-aware Speculative Decoding},
author={Chen, Zhuoming and May, Avner and Svirschevski, Ruslan and Huang, Yuhsun and Ryabinin, Max and Jia, Zhihao and Chen, Beidi},
journal={arXiv preprint arXiv:2402.12374},
year={2024}
}
@article{svirschevski2024specexec,
title={SpecExec: Massively Parallel Speculative Decoding for Interactive LLM Inference on Consumer Devices},
author={Svirschevski, Ruslan and May, Avner and Chen, Zhuoming and Chen, Beidi and Jia, Zhihao and Ryabinin, Max},
journal={arXiv preprint arXiv:2406.02532},
year={2024}
}
For Tasks:
Click tags to check more tools for each tasksFor Jobs:
Alternative AI tools for UMbreLLa
Similar Open Source Tools
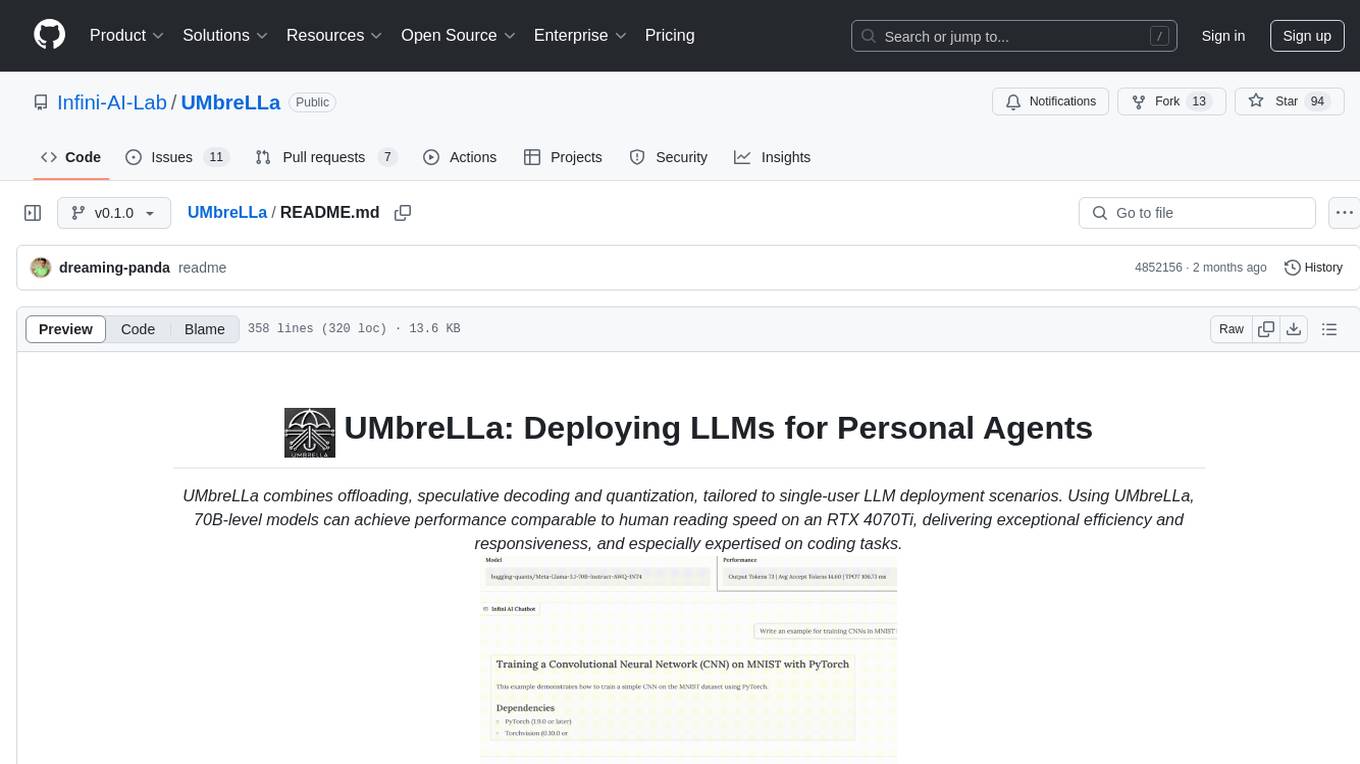
UMbreLLa
UMbreLLa is a tool designed for deploying Large Language Models (LLMs) for personal agents. It combines offloading, speculative decoding, and quantization to optimize single-user LLM deployment scenarios. With UMbreLLa, 70B-level models can achieve performance comparable to human reading speed on an RTX 4070Ti, delivering exceptional efficiency and responsiveness, especially for coding tasks. The tool supports deploying models on various GPUs and offers features like code completion and CLI/Gradio chatbots. Users can configure the LLM engine for optimal performance based on their hardware setup.
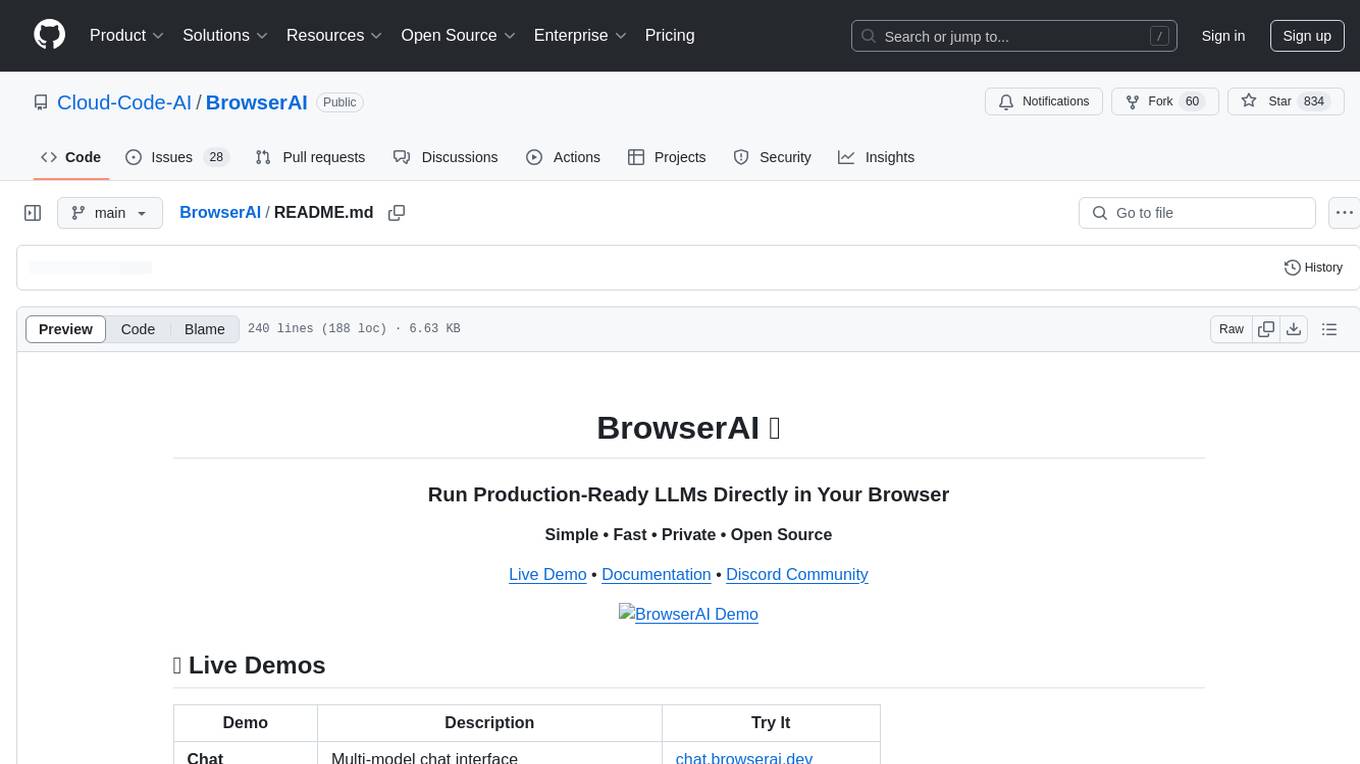
BrowserAI
BrowserAI is a production-ready tool that allows users to run AI models directly in the browser, offering simplicity, speed, privacy, and open-source capabilities. It provides WebGPU acceleration for fast inference, zero server costs, offline capability, and developer-friendly features. Perfect for web developers, companies seeking privacy-conscious AI solutions, researchers experimenting with browser-based AI, and hobbyists exploring AI without infrastructure overhead. The tool supports various AI tasks like text generation, speech recognition, and text-to-speech, with pre-configured popular models ready to use. It offers a simple SDK with multiple engine support and seamless switching between MLC and Transformers engines.
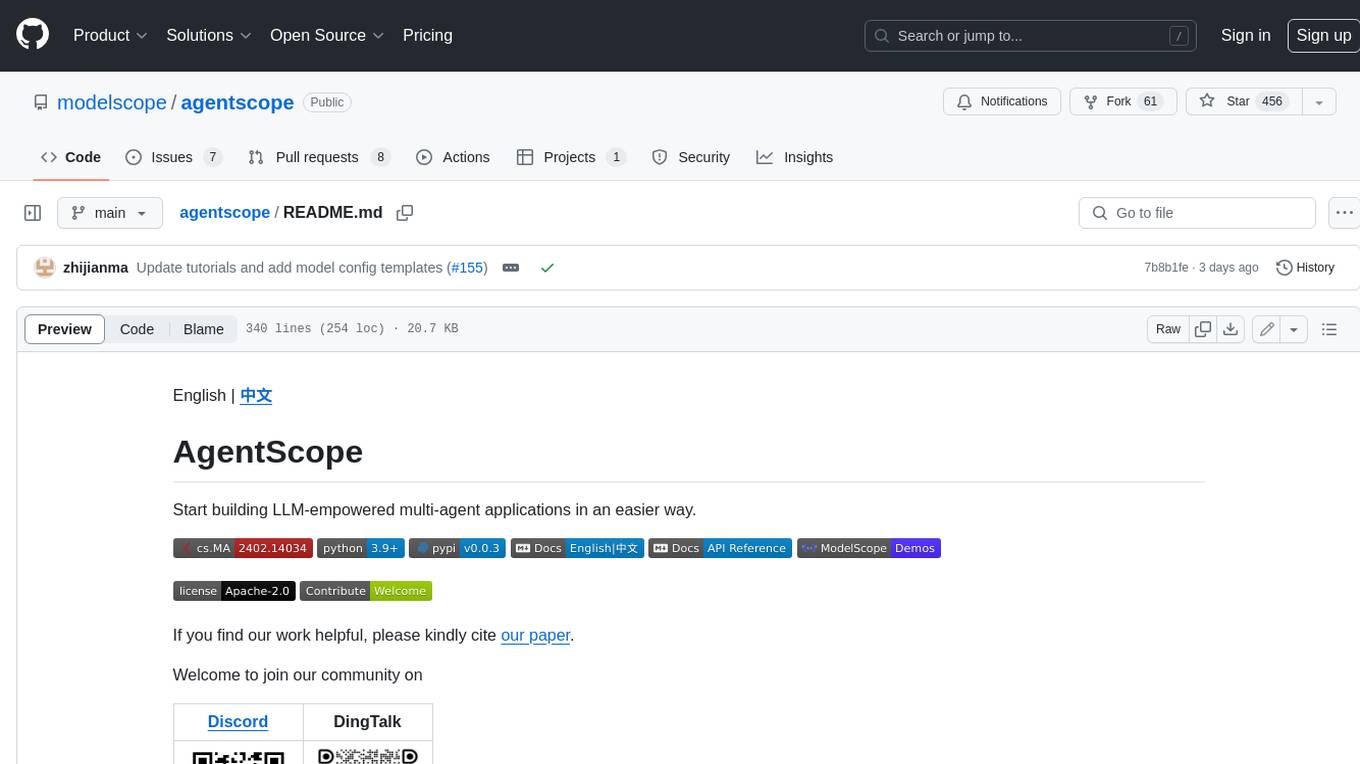
agentscope
AgentScope is a multi-agent platform designed to empower developers to build multi-agent applications with large-scale models. It features three high-level capabilities: Easy-to-Use, High Robustness, and Actor-Based Distribution. AgentScope provides a list of `ModelWrapper` to support both local model services and third-party model APIs, including OpenAI API, DashScope API, Gemini API, and ollama. It also enables developers to rapidly deploy local model services using libraries such as ollama (CPU inference), Flask + Transformers, Flask + ModelScope, FastChat, and vllm. AgentScope supports various services, including Web Search, Data Query, Retrieval, Code Execution, File Operation, and Text Processing. Example applications include Conversation, Game, and Distribution. AgentScope is released under Apache License 2.0 and welcomes contributions.
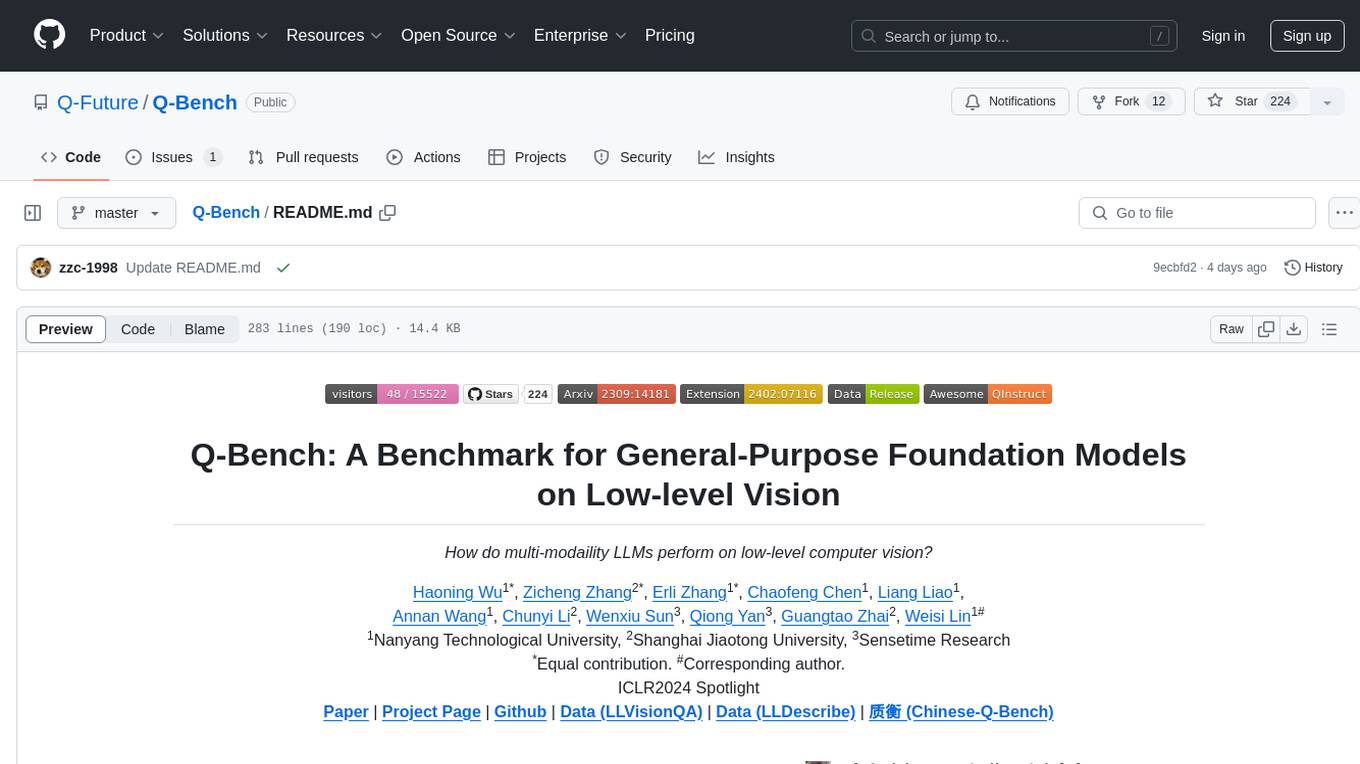
Q-Bench
Q-Bench is a benchmark for general-purpose foundation models on low-level vision, focusing on multi-modality LLMs performance. It includes three realms for low-level vision: perception, description, and assessment. The benchmark datasets LLVisionQA and LLDescribe are collected for perception and description tasks, with open submission-based evaluation. An abstract evaluation code is provided for assessment using public datasets. The tool can be used with the datasets API for single images and image pairs, allowing for automatic download and usage. Various tasks and evaluations are available for testing MLLMs on low-level vision tasks.
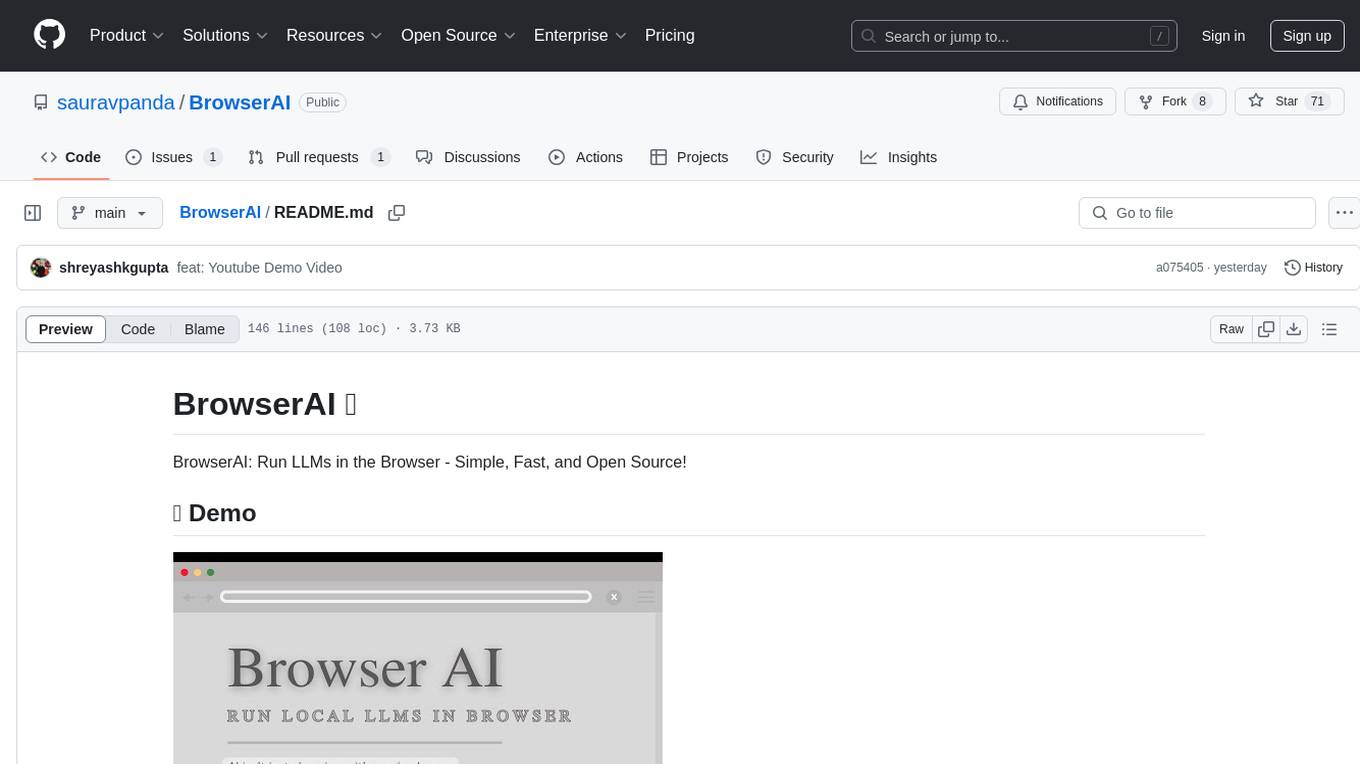
BrowserAI
BrowserAI is a tool that allows users to run large language models (LLMs) directly in the browser, providing a simple, fast, and open-source solution. It prioritizes privacy by processing data locally, is cost-effective with no server costs, works offline after initial download, and offers WebGPU acceleration for high performance. It is developer-friendly with a simple API, supports multiple engines, and comes with pre-configured models for easy use. Ideal for web developers, companies needing privacy-conscious AI solutions, researchers experimenting with browser-based AI, and hobbyists exploring AI without infrastructure overhead.
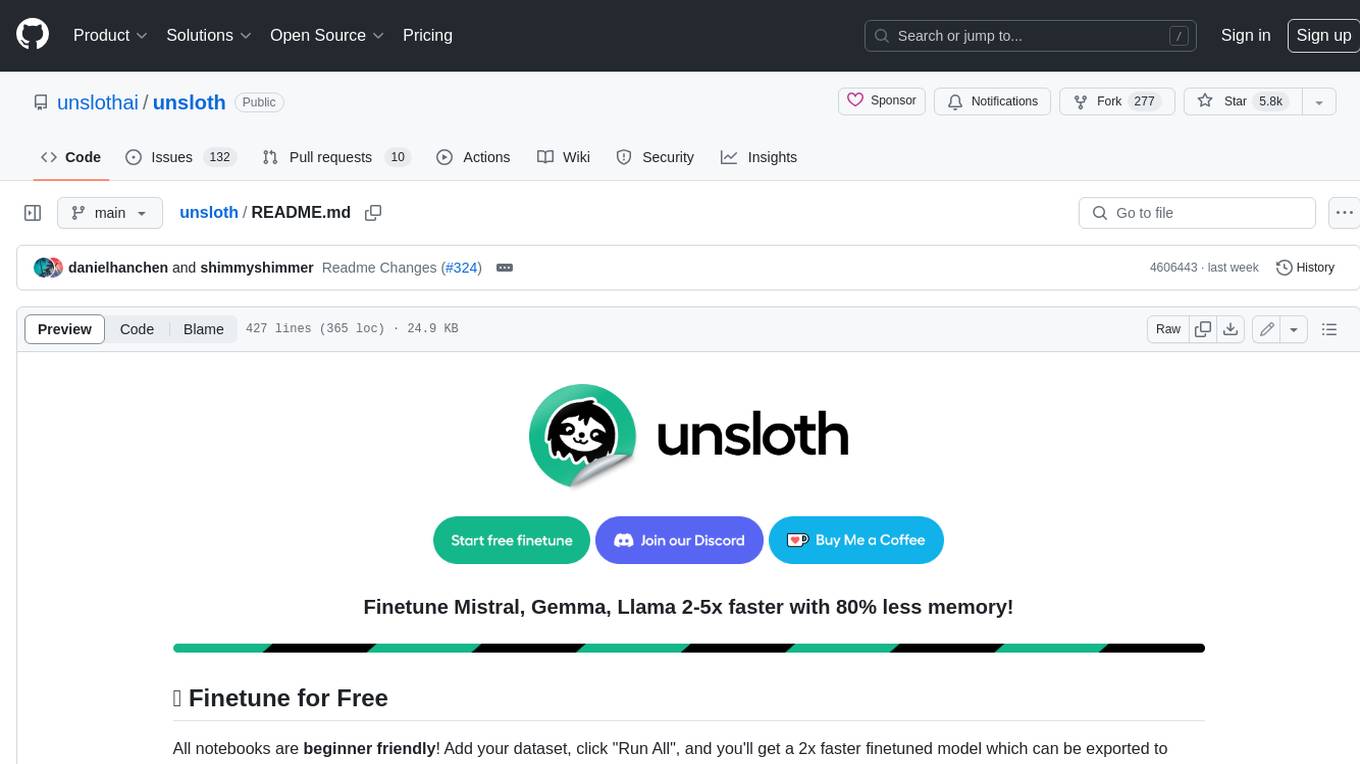
unsloth
Unsloth is a tool that allows users to fine-tune large language models (LLMs) 2-5x faster with 80% less memory. It is a free and open-source tool that can be used to fine-tune LLMs such as Gemma, Mistral, Llama 2-5, TinyLlama, and CodeLlama 34b. Unsloth supports 4-bit and 16-bit QLoRA / LoRA fine-tuning via bitsandbytes. It also supports DPO (Direct Preference Optimization), PPO, and Reward Modelling. Unsloth is compatible with Hugging Face's TRL, Trainer, Seq2SeqTrainer, and Pytorch code. It is also compatible with NVIDIA GPUs since 2018+ (minimum CUDA Capability 7.0).
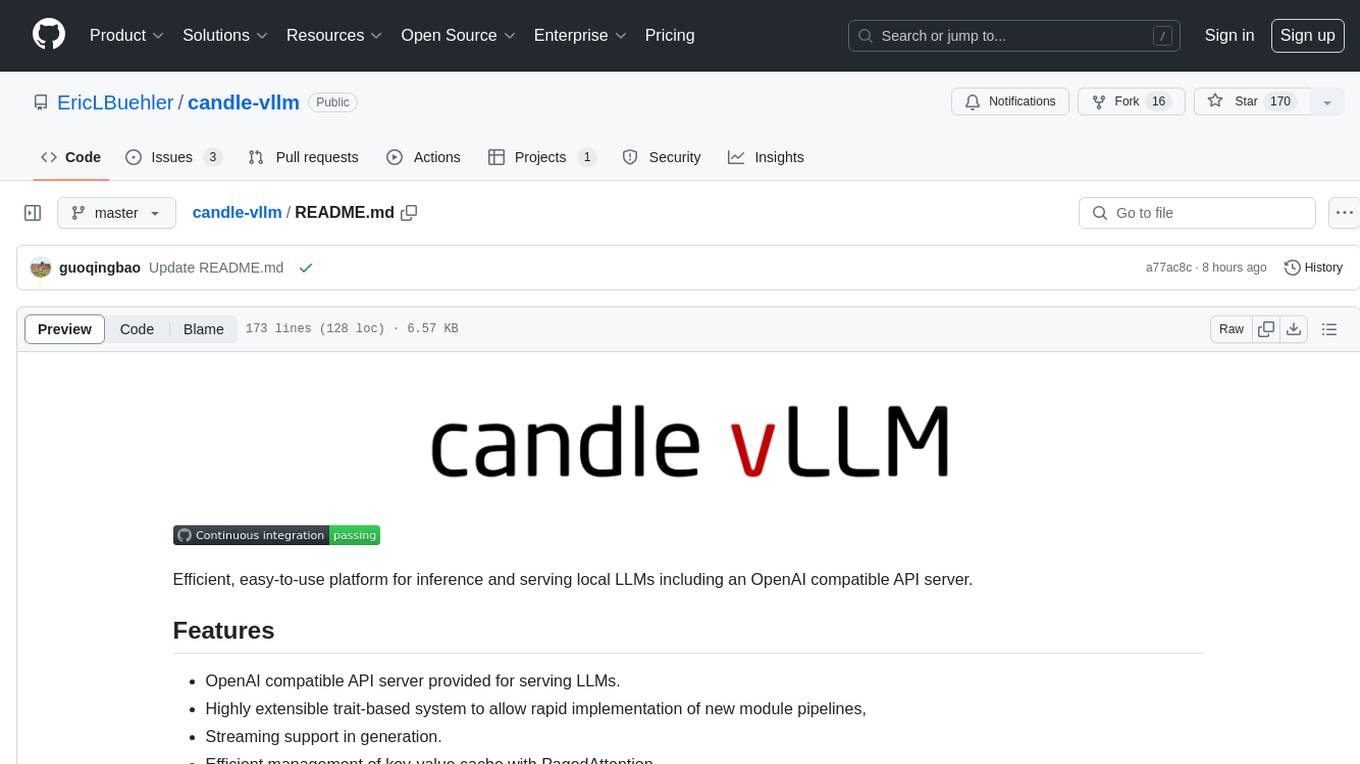
candle-vllm
Candle-vllm is an efficient and easy-to-use platform designed for inference and serving local LLMs, featuring an OpenAI compatible API server. It offers a highly extensible trait-based system for rapid implementation of new module pipelines, streaming support in generation, efficient management of key-value cache with PagedAttention, and continuous batching. The tool supports chat serving for various models and provides a seamless experience for users to interact with LLMs through different interfaces.
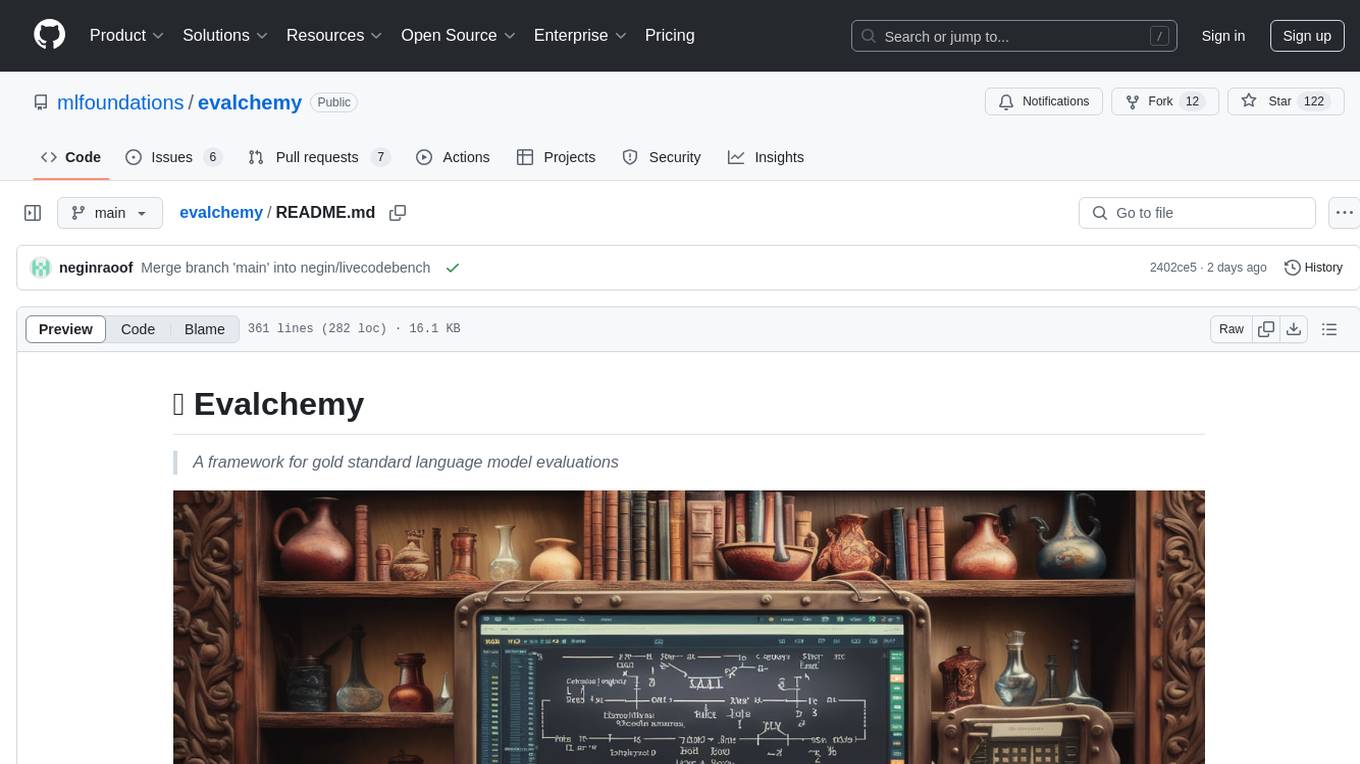
evalchemy
Evalchemy is a unified and easy-to-use toolkit for evaluating language models, focusing on post-trained models. It integrates multiple existing benchmarks such as RepoBench, AlpacaEval, and ZeroEval. Key features include unified installation, parallel evaluation, simplified usage, and results management. Users can run various benchmarks with a consistent command-line interface and track results locally or integrate with a database for systematic tracking and leaderboard submission.
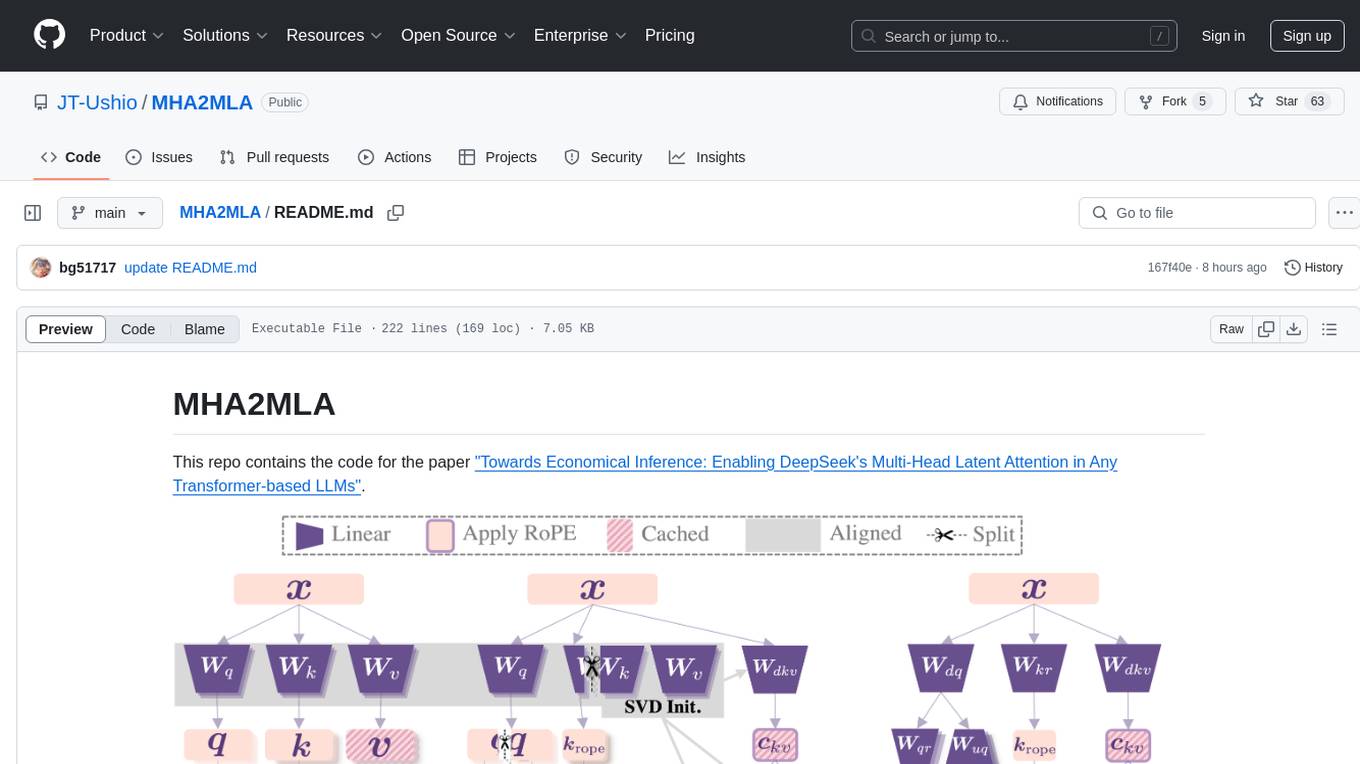
MHA2MLA
This repository contains the code for the paper 'Towards Economical Inference: Enabling DeepSeek's Multi-Head Latent Attention in Any Transformer-based LLMs'. It provides tools for fine-tuning and evaluating Llama models, converting models between different frameworks, processing datasets, and performing specific model training tasks like Partial-RoPE Fine-Tuning and Multiple-Head Latent Attention Fine-Tuning. The repository also includes commands for model evaluation using Lighteval and LongBench, along with necessary environment setup instructions.
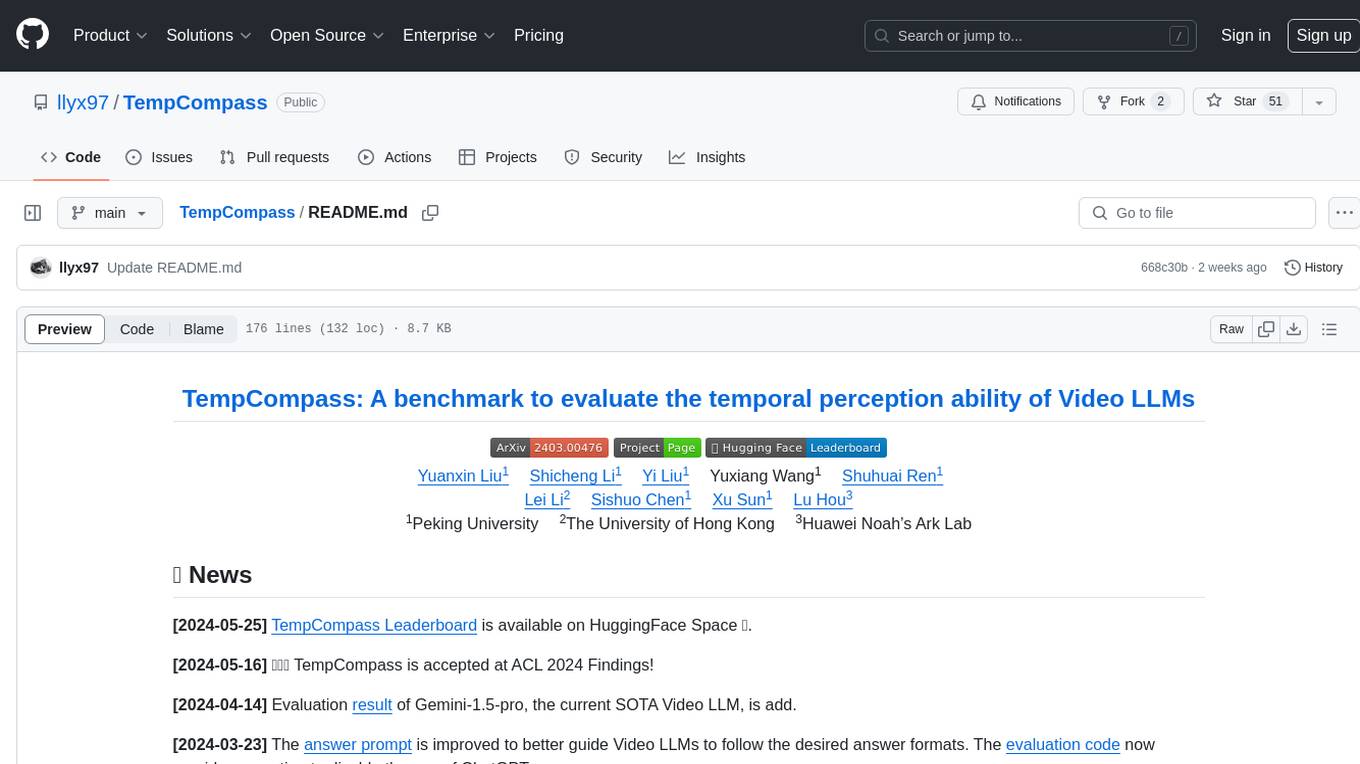
TempCompass
TempCompass is a benchmark designed to evaluate the temporal perception ability of Video LLMs. It encompasses a diverse set of temporal aspects and task formats to comprehensively assess the capability of Video LLMs in understanding videos. The benchmark includes conflicting videos to prevent models from relying on single-frame bias and language priors. Users can clone the repository, install required packages, prepare data, run inference using examples like Video-LLaVA and Gemini, and evaluate the performance of their models across different tasks such as Multi-Choice QA, Yes/No QA, Caption Matching, and Caption Generation.
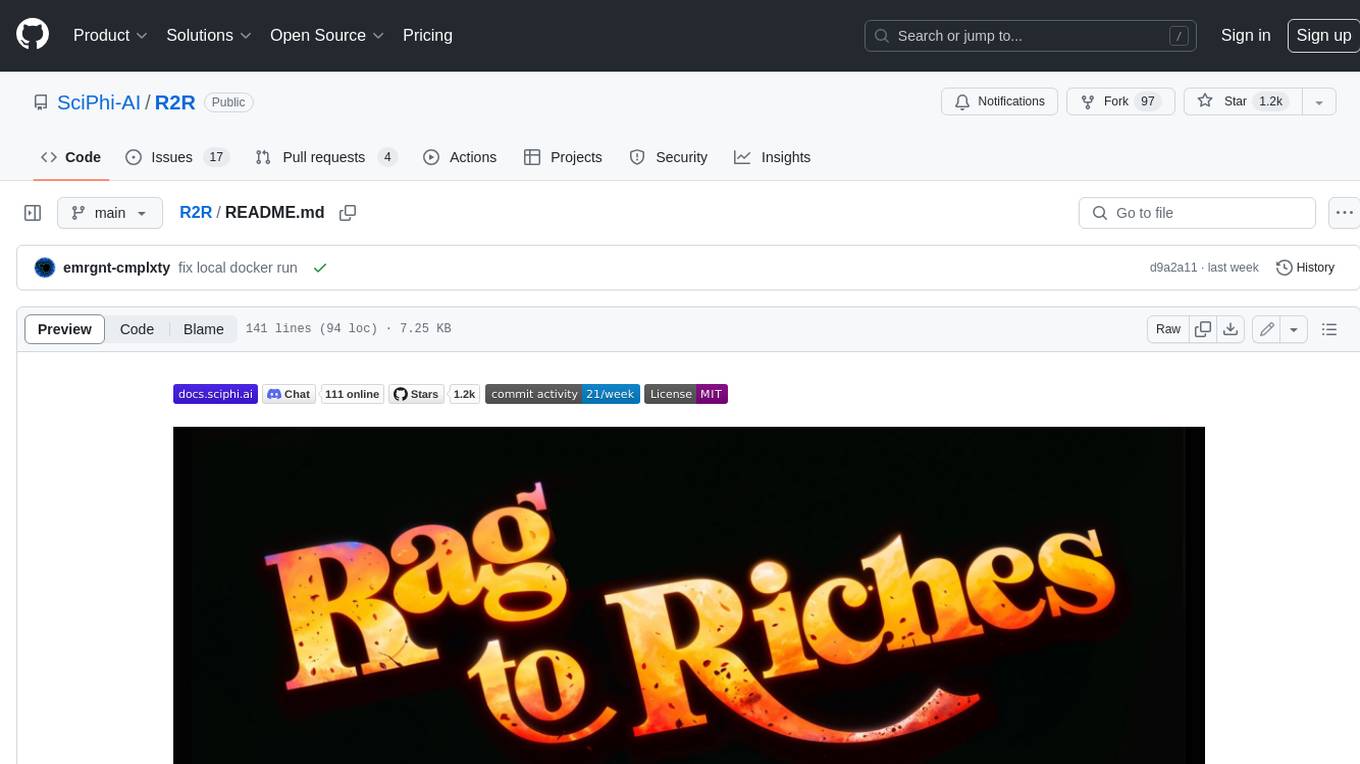
R2R
R2R (RAG to Riches) is a fast and efficient framework for serving high-quality Retrieval-Augmented Generation (RAG) to end users. The framework is designed with customizable pipelines and a feature-rich FastAPI implementation, enabling developers to quickly deploy and scale RAG-based applications. R2R was conceived to bridge the gap between local LLM experimentation and scalable production solutions. **R2R is to LangChain/LlamaIndex what NextJS is to React**. A JavaScript client for R2R deployments can be found here. ### Key Features * **🚀 Deploy** : Instantly launch production-ready RAG pipelines with streaming capabilities. * **🧩 Customize** : Tailor your pipeline with intuitive configuration files. * **🔌 Extend** : Enhance your pipeline with custom code integrations. * **⚖️ Autoscale** : Scale your pipeline effortlessly in the cloud using SciPhi. * **🤖 OSS** : Benefit from a framework developed by the open-source community, designed to simplify RAG deployment.
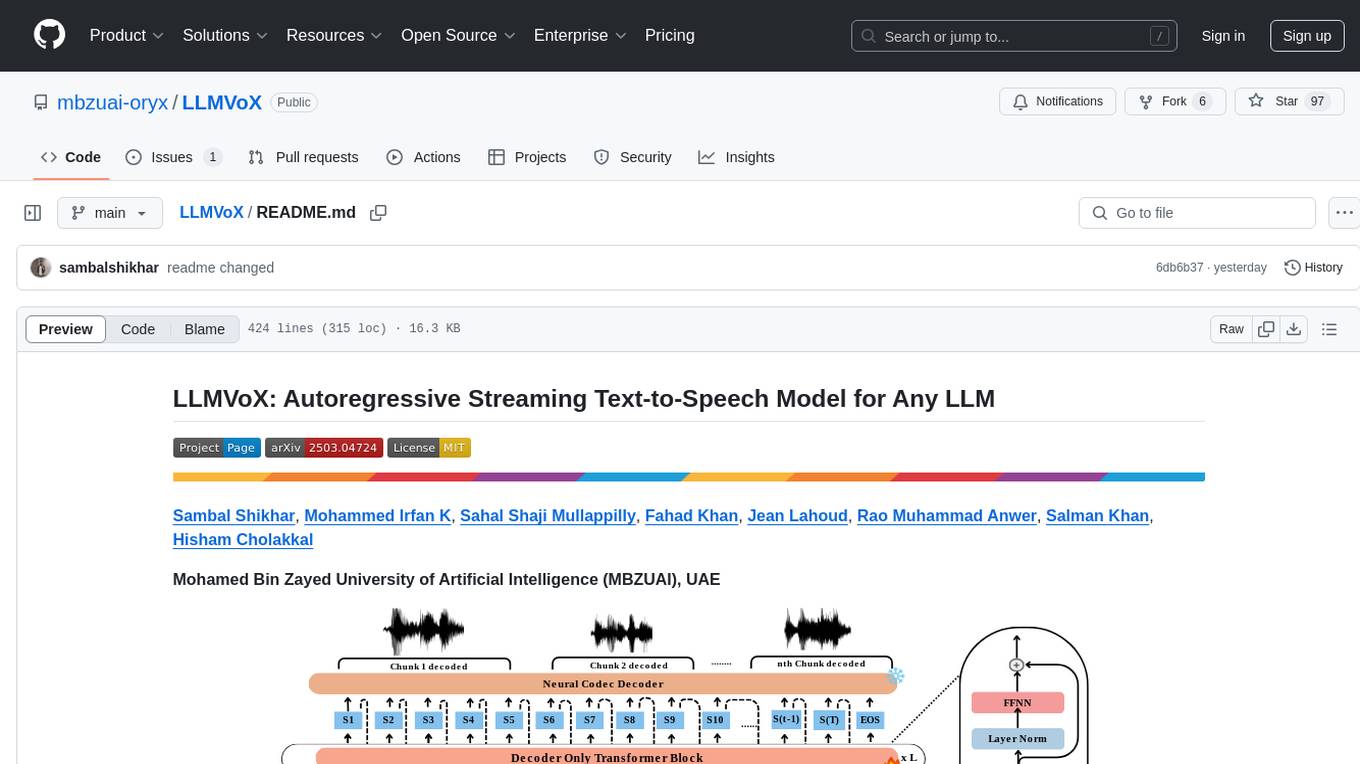
LLMVoX
LLMVoX is a lightweight 30M-parameter, LLM-agnostic, autoregressive streaming Text-to-Speech (TTS) system designed to convert text outputs from Large Language Models into high-fidelity streaming speech with low latency. It achieves significantly lower Word Error Rate compared to speech-enabled LLMs while operating at comparable latency and speech quality. Key features include being lightweight & fast with only 30M parameters, LLM-agnostic for easy integration with existing models, multi-queue streaming for continuous speech generation, and multilingual support for easy adaptation to new languages.
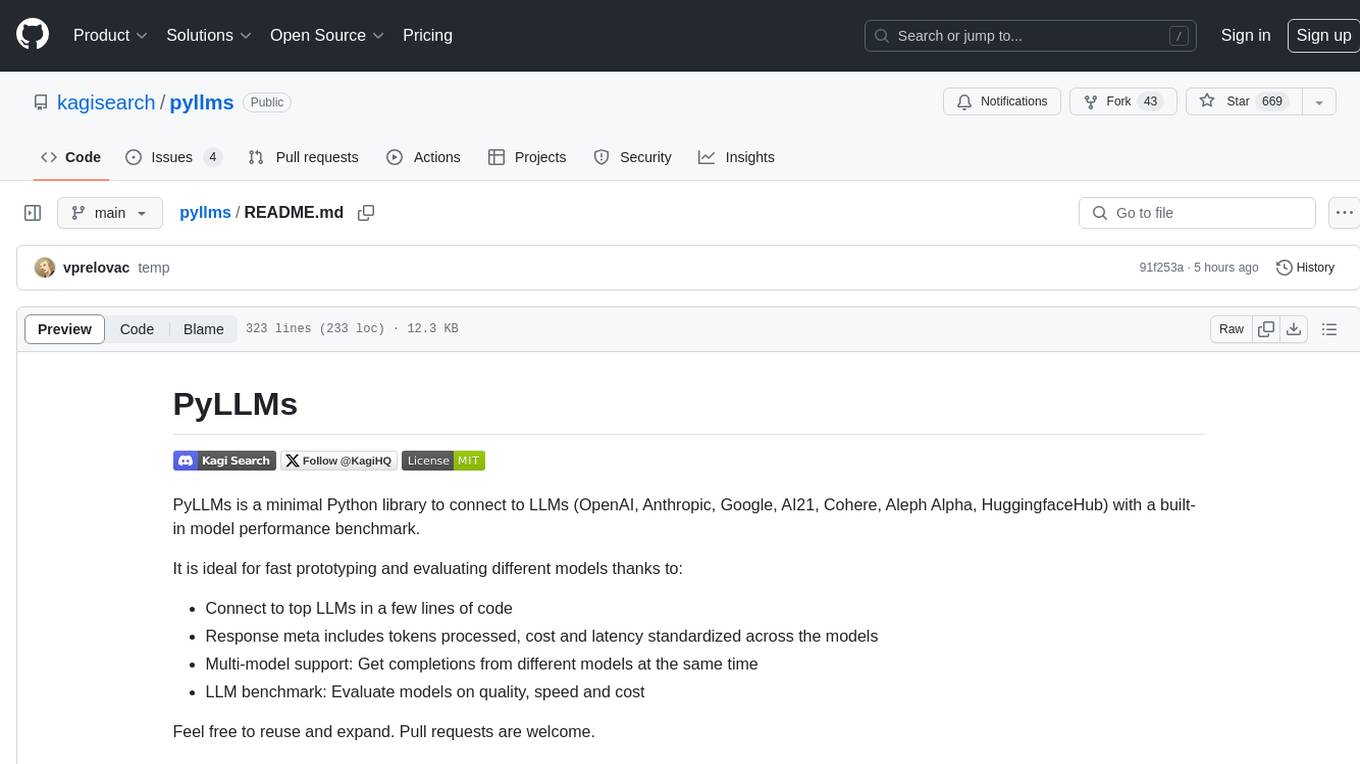
pyllms
PyLLMs is a minimal Python library designed to connect to various Language Model Models (LLMs) such as OpenAI, Anthropic, Google, AI21, Cohere, Aleph Alpha, and HuggingfaceHub. It provides a built-in model performance benchmark for fast prototyping and evaluating different models. Users can easily connect to top LLMs, get completions from multiple models simultaneously, and evaluate models on quality, speed, and cost. The library supports asynchronous completion, streaming from compatible models, and multi-model initialization for testing and comparison. Additionally, it offers features like passing chat history, system messages, counting tokens, and benchmarking models based on quality, speed, and cost.
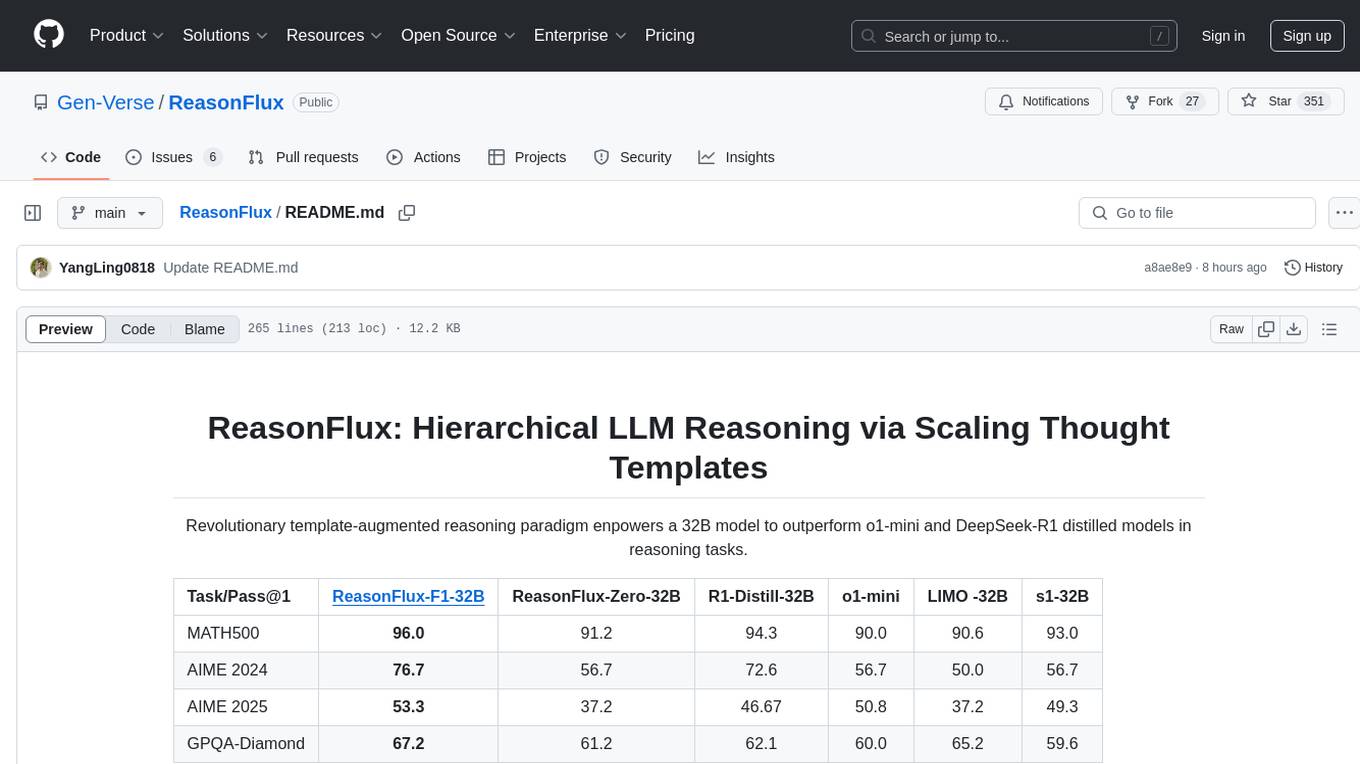
ReasonFlux
ReasonFlux is a revolutionary template-augmented reasoning paradigm that empowers a 32B model to outperform other models in reasoning tasks. The repository provides official resources for the paper 'ReasonFlux: Hierarchical LLM Reasoning via Scaling Thought Templates', including the latest released model ReasonFlux-F1-32B. It includes updates, dataset links, model zoo, getting started guide, training instructions, evaluation details, inference examples, performance comparisons, reasoning examples, preliminary work references, and citation information.
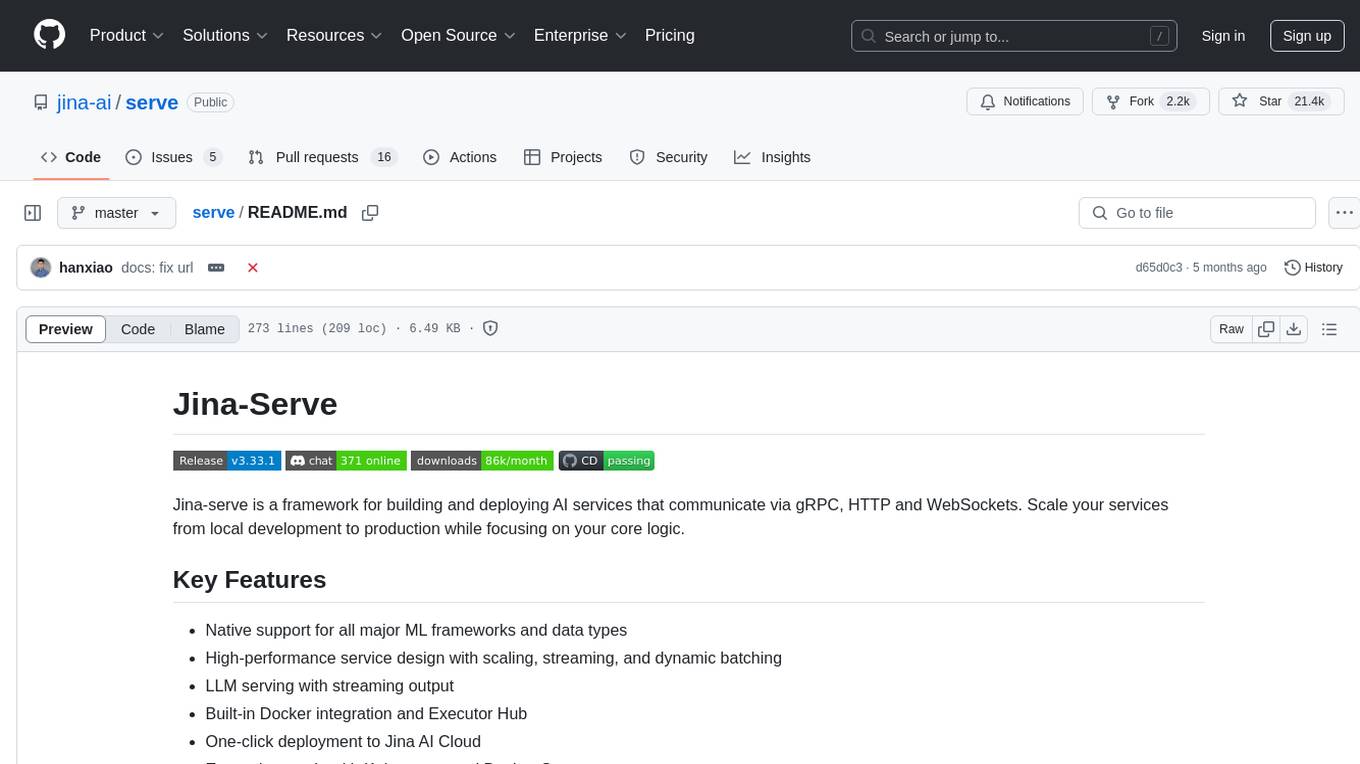
serve
Jina-Serve is a framework for building and deploying AI services that communicate via gRPC, HTTP and WebSockets. It provides native support for major ML frameworks and data types, high-performance service design with scaling and dynamic batching, LLM serving with streaming output, built-in Docker integration and Executor Hub, one-click deployment to Jina AI Cloud, and enterprise-ready features with Kubernetes and Docker Compose support. Users can create gRPC-based AI services, build pipelines, scale services locally with replicas, shards, and dynamic batching, deploy to the cloud using Kubernetes, Docker Compose, or JCloud, and enable token-by-token streaming for responsive LLM applications.
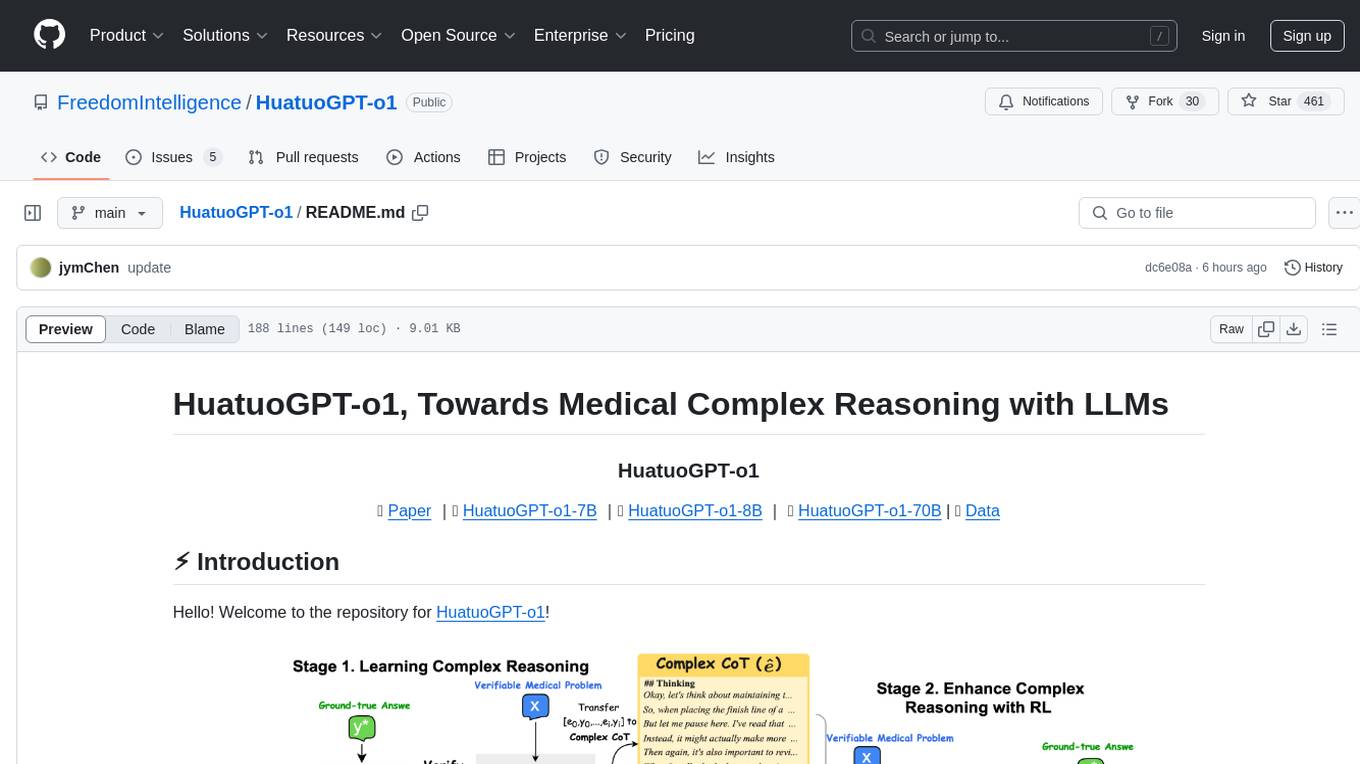
HuatuoGPT-o1
HuatuoGPT-o1 is a medical language model designed for advanced medical reasoning. It can identify mistakes, explore alternative strategies, and refine answers. The model leverages verifiable medical problems and a specialized medical verifier to guide complex reasoning trajectories and enhance reasoning through reinforcement learning. The repository provides access to models, data, and code for HuatuoGPT-o1, allowing users to deploy the model for medical reasoning tasks.
For similar tasks
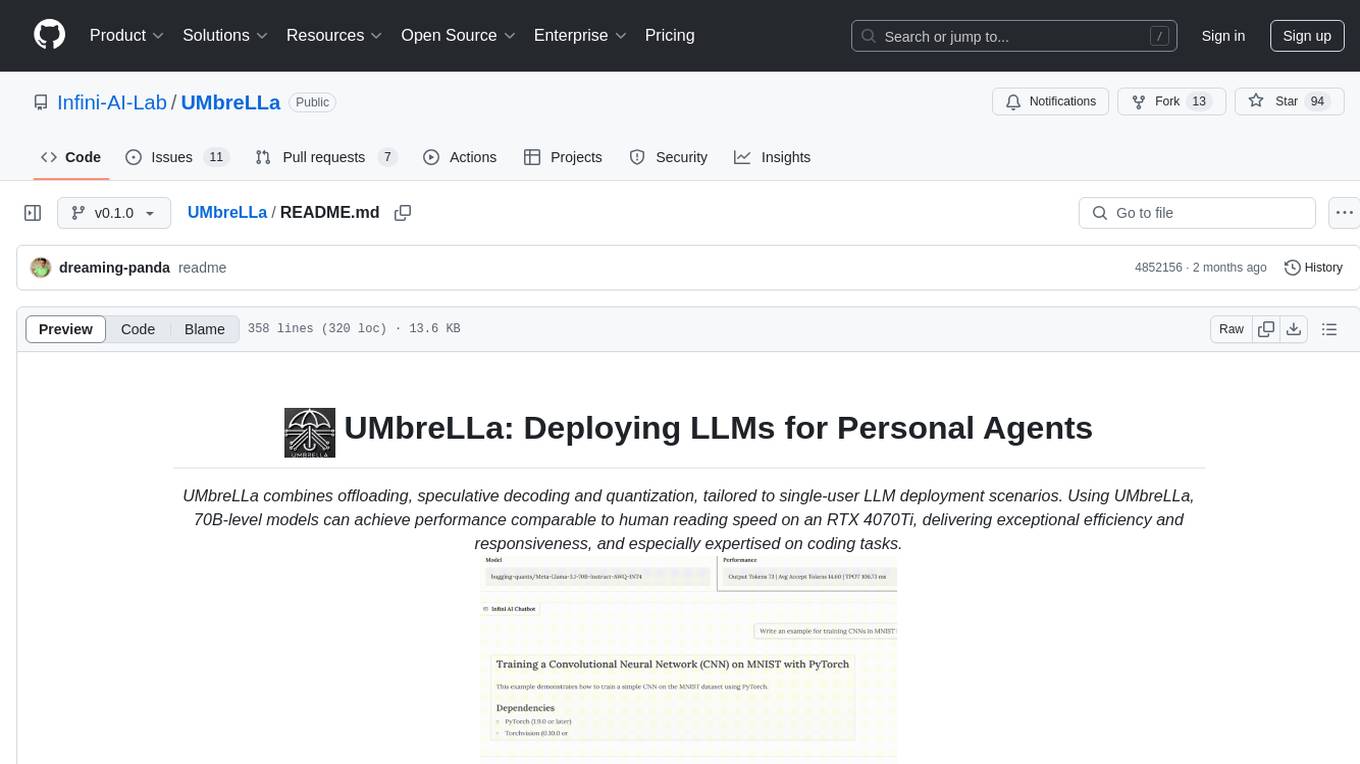
UMbreLLa
UMbreLLa is a tool designed for deploying Large Language Models (LLMs) for personal agents. It combines offloading, speculative decoding, and quantization to optimize single-user LLM deployment scenarios. With UMbreLLa, 70B-level models can achieve performance comparable to human reading speed on an RTX 4070Ti, delivering exceptional efficiency and responsiveness, especially for coding tasks. The tool supports deploying models on various GPUs and offers features like code completion and CLI/Gradio chatbots. Users can configure the LLM engine for optimal performance based on their hardware setup.
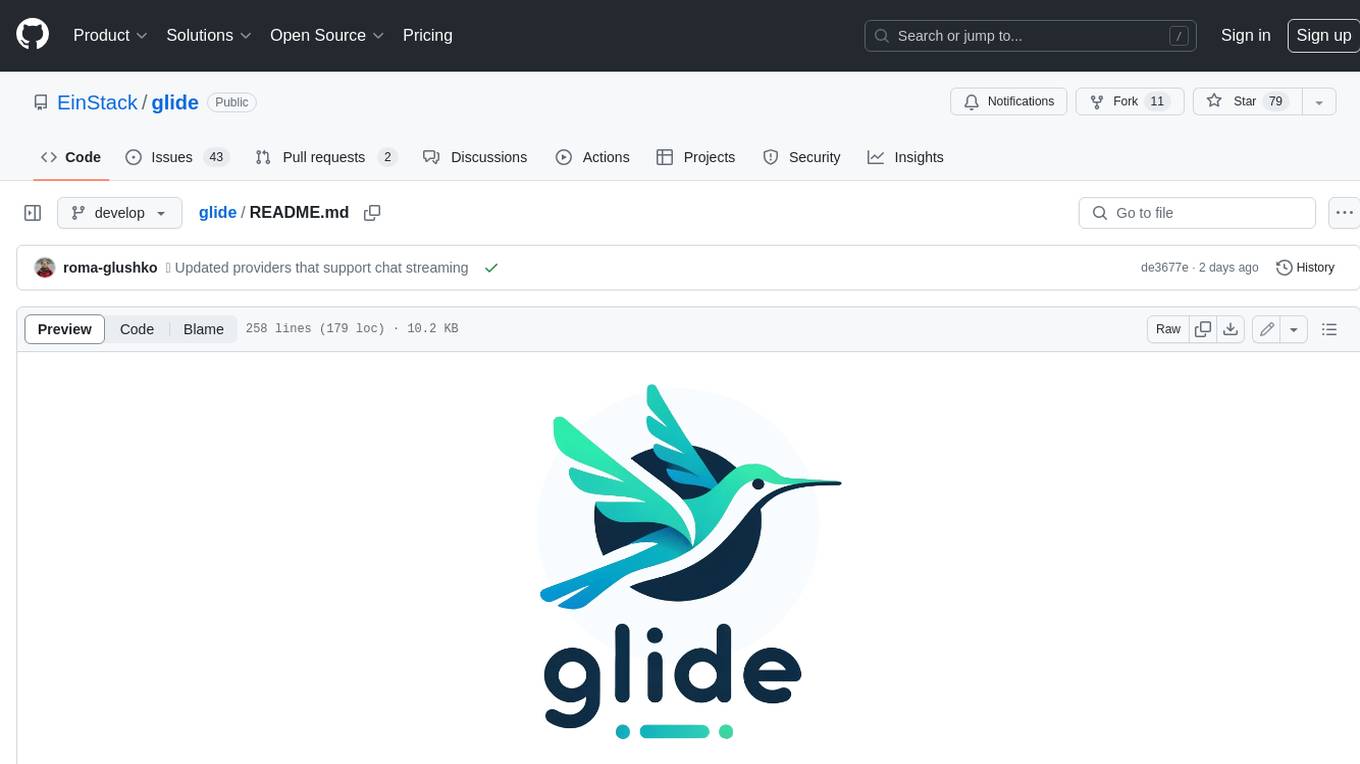
glide
Glide is a cloud-native LLM gateway that provides a unified REST API for accessing various large language models (LLMs) from different providers. It handles LLMOps tasks such as model failover, caching, key management, and more, making it easy to integrate LLMs into applications. Glide supports popular LLM providers like OpenAI, Anthropic, Azure OpenAI, AWS Bedrock (Titan), Cohere, Google Gemini, OctoML, and Ollama. It offers high availability, performance, and observability, and provides SDKs for Python and NodeJS to simplify integration.
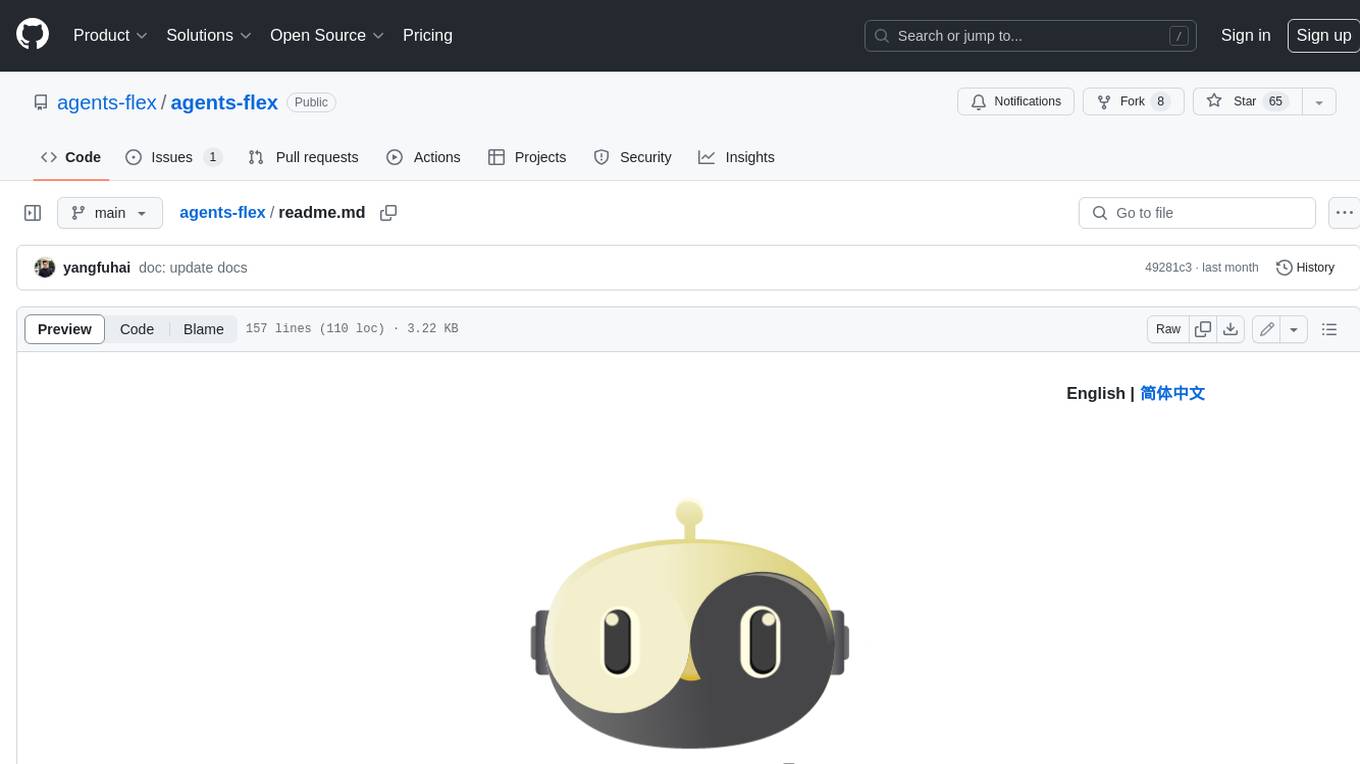
agents-flex
Agents-Flex is a LLM Application Framework like LangChain base on Java. It provides a set of tools and components for building LLM applications, including LLM Visit, Prompt and Prompt Template Loader, Function Calling Definer, Invoker and Running, Memory, Embedding, Vector Storage, Resource Loaders, Document, Splitter, Loader, Parser, LLMs Chain, and Agents Chain.
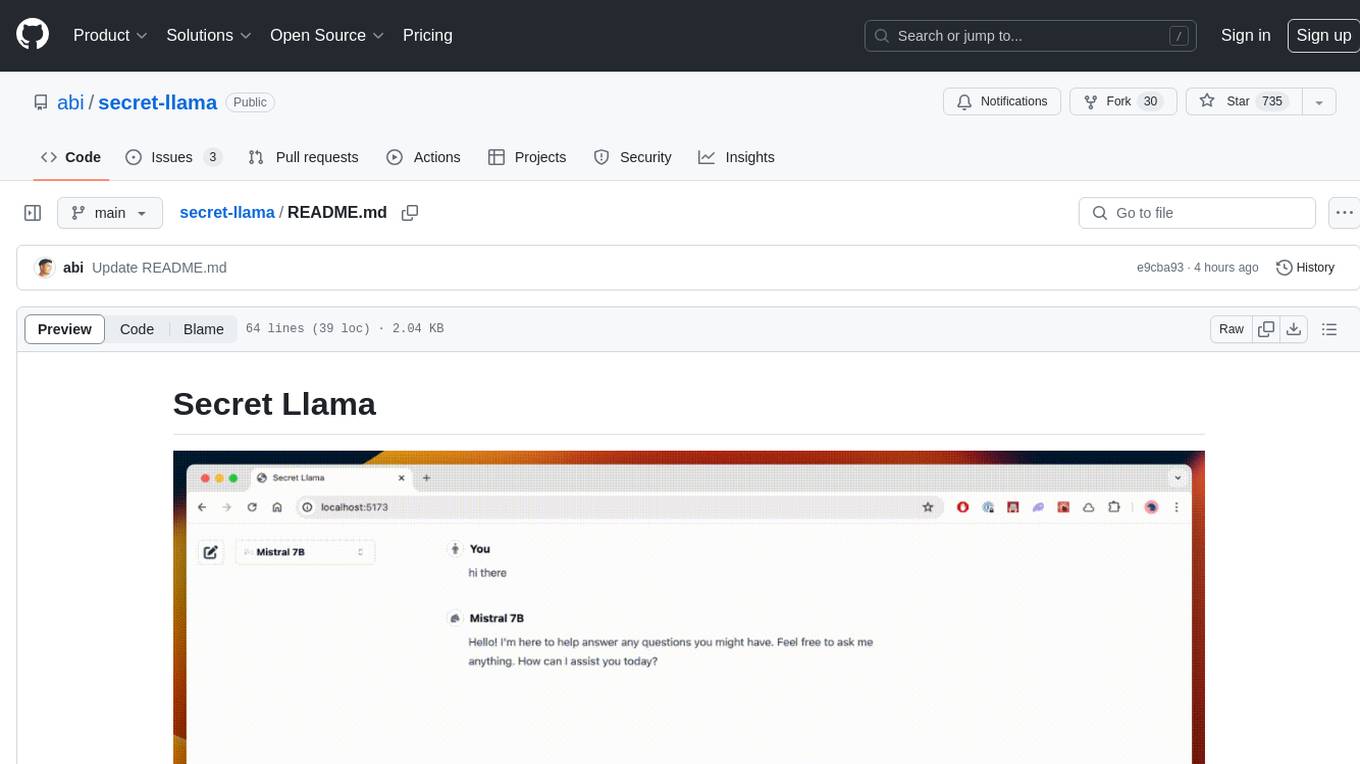
secret-llama
Entirely-in-browser, fully private LLM chatbot supporting Llama 3, Mistral and other open source models. Fully private = No conversation data ever leaves your computer. Runs in the browser = No server needed and no install needed! Works offline. Easy-to-use interface on par with ChatGPT, but for open source LLMs. System requirements include a modern browser with WebGPU support. Supported models include TinyLlama-1.1B-Chat-v0.4-q4f32_1-1k, Llama-3-8B-Instruct-q4f16_1, Phi1.5-q4f16_1-1k, and Mistral-7B-Instruct-v0.2-q4f16_1. Looking for contributors to improve the interface, support more models, speed up initial model loading time, and fix bugs.
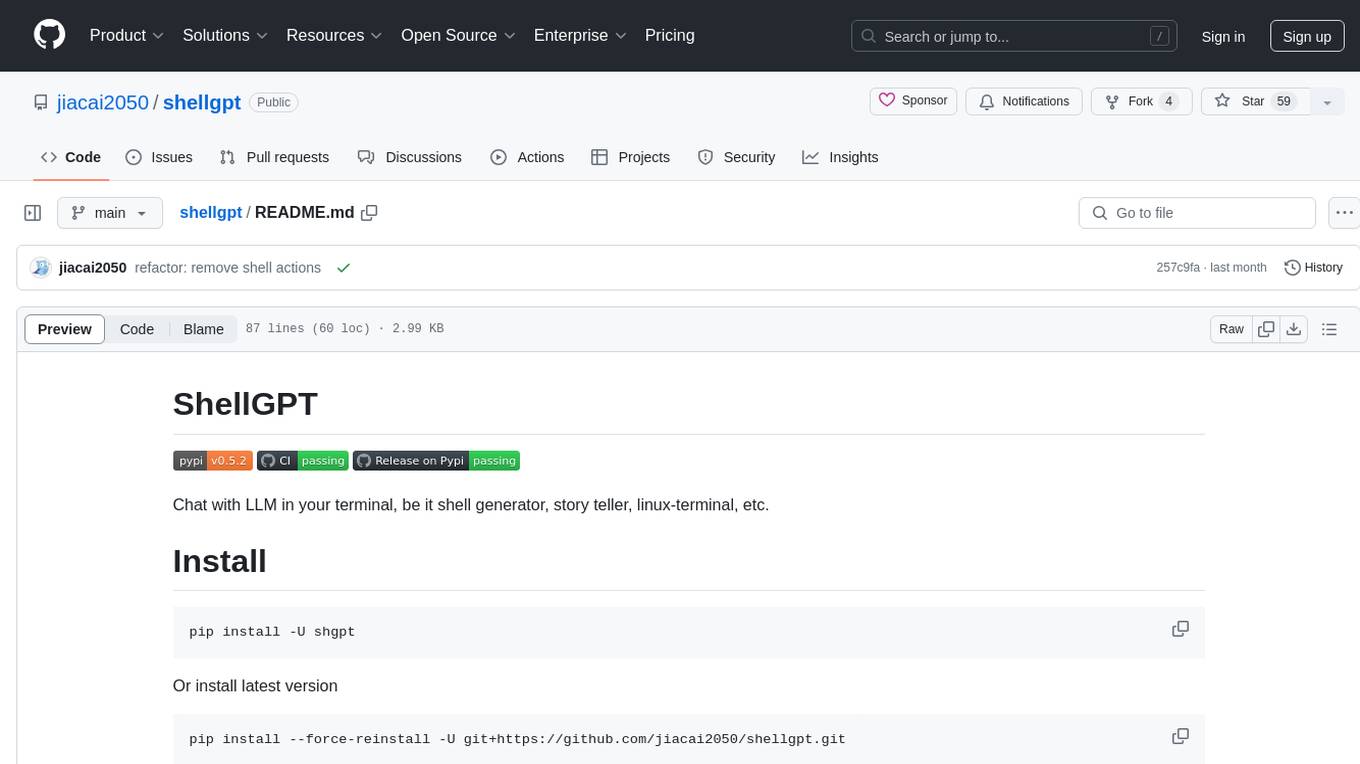
shellgpt
ShellGPT is a tool that allows users to chat with a large language model (LLM) in the terminal. It can be used for various purposes such as generating shell commands, telling stories, and interacting with Linux terminal. The tool provides different modes of usage including direct mode for asking questions, REPL mode for chatting with LLM, and TUI mode tailored for inferring shell commands. Users can customize the tool by setting up different language model backends such as Ollama or using OpenAI compatible API endpoints. Additionally, ShellGPT comes with built-in system contents for general questions, correcting typos, generating URL slugs, programming questions, shell command inference, and git commit message generation. Users can define their own content or share customized contents in the discuss section.
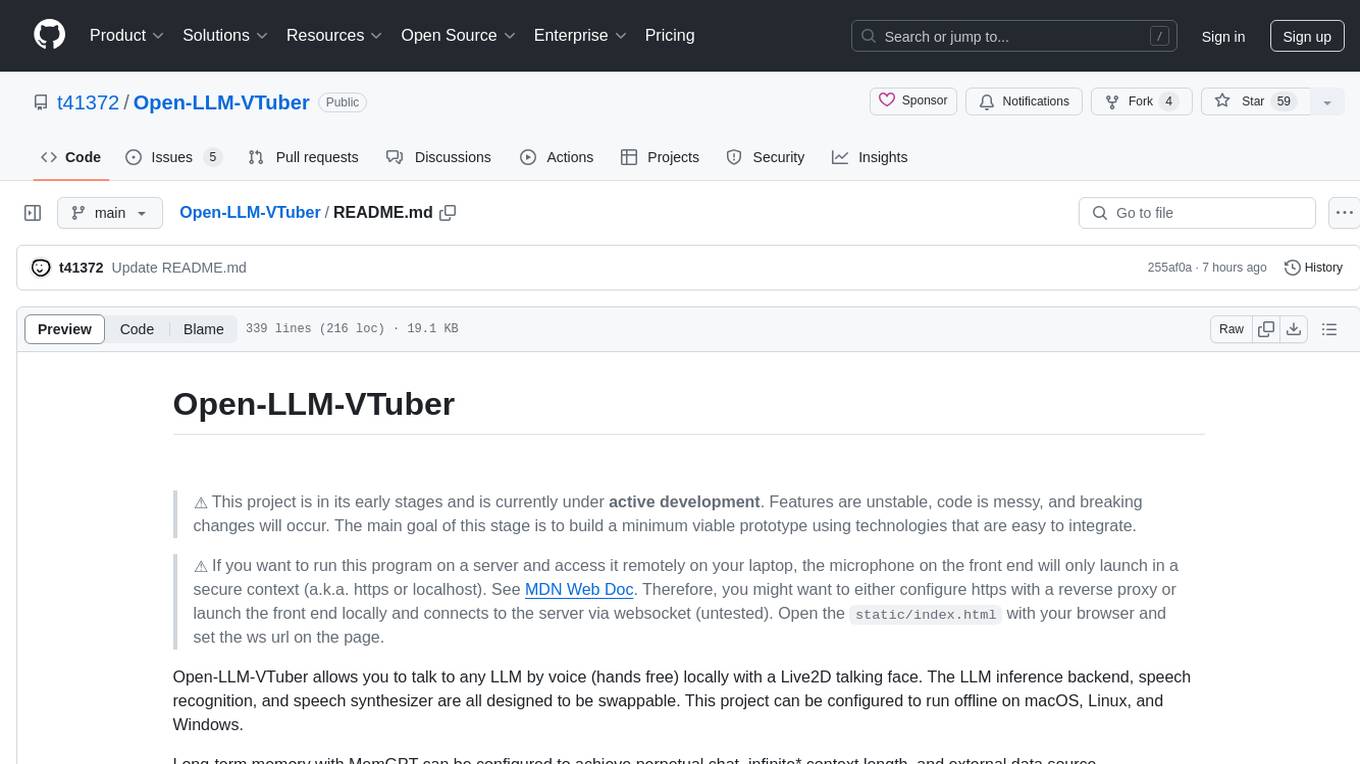
Open-LLM-VTuber
Open-LLM-VTuber is a project in early stages of development that allows users to interact with Large Language Models (LLM) using voice commands and receive responses through a Live2D talking face. The project aims to provide a minimum viable prototype for offline use on macOS, Linux, and Windows, with features like long-term memory using MemGPT, customizable LLM backends, speech recognition, and text-to-speech providers. Users can configure the project to chat with LLMs, choose different backend services, and utilize Live2D models for visual representation. The project supports perpetual chat, offline operation, and GPU acceleration on macOS, addressing limitations of existing solutions on macOS.
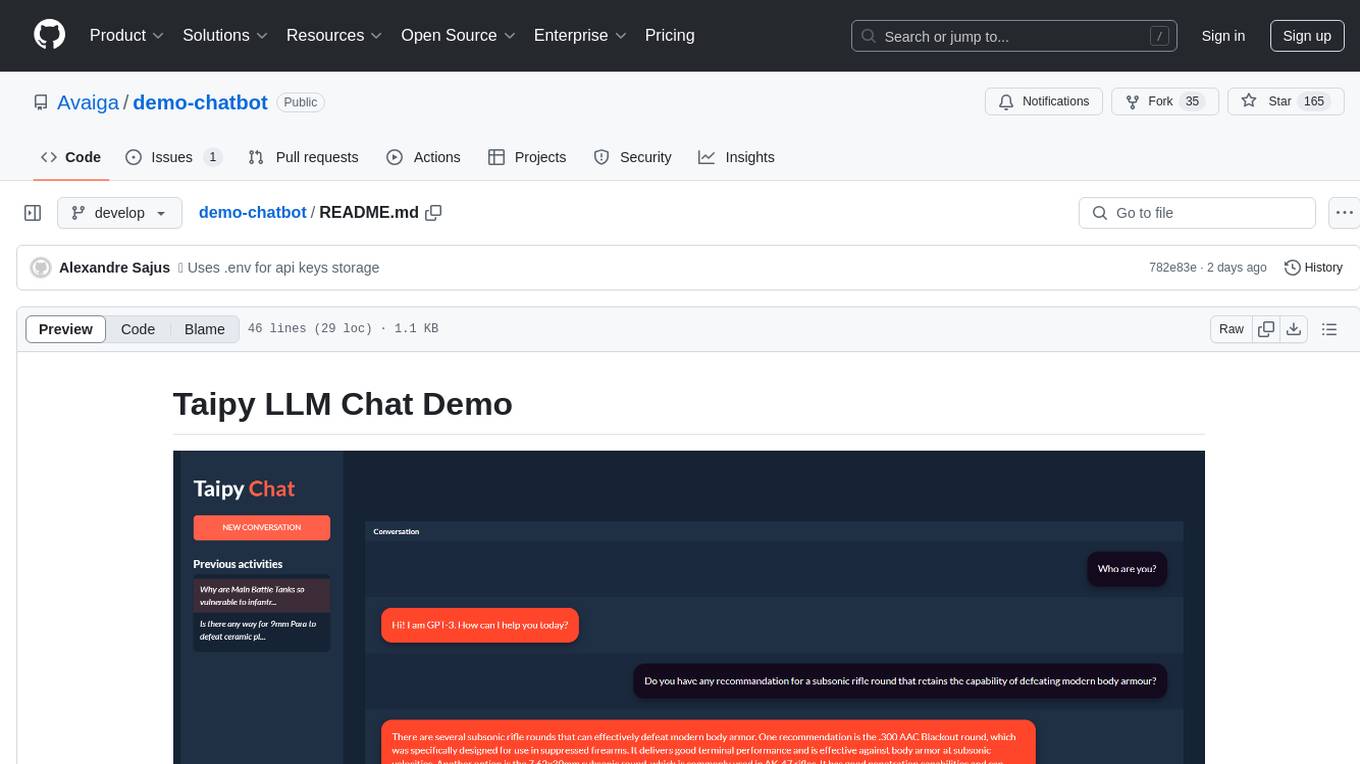
demo-chatbot
The demo-chatbot repository contains a simple app to chat with an LLM, allowing users to create any LLM Inference Web Apps using Python. The app utilizes OpenAI's GPT-4 API to generate responses to user messages, with the flexibility to switch to other APIs or models. The repository includes a tutorial in the Taipy documentation for creating the app. Users need an OpenAI account with an active API key to run the app by cloning the repository, installing dependencies, setting up the API key in a .env file, and running the main.py file.
For similar jobs
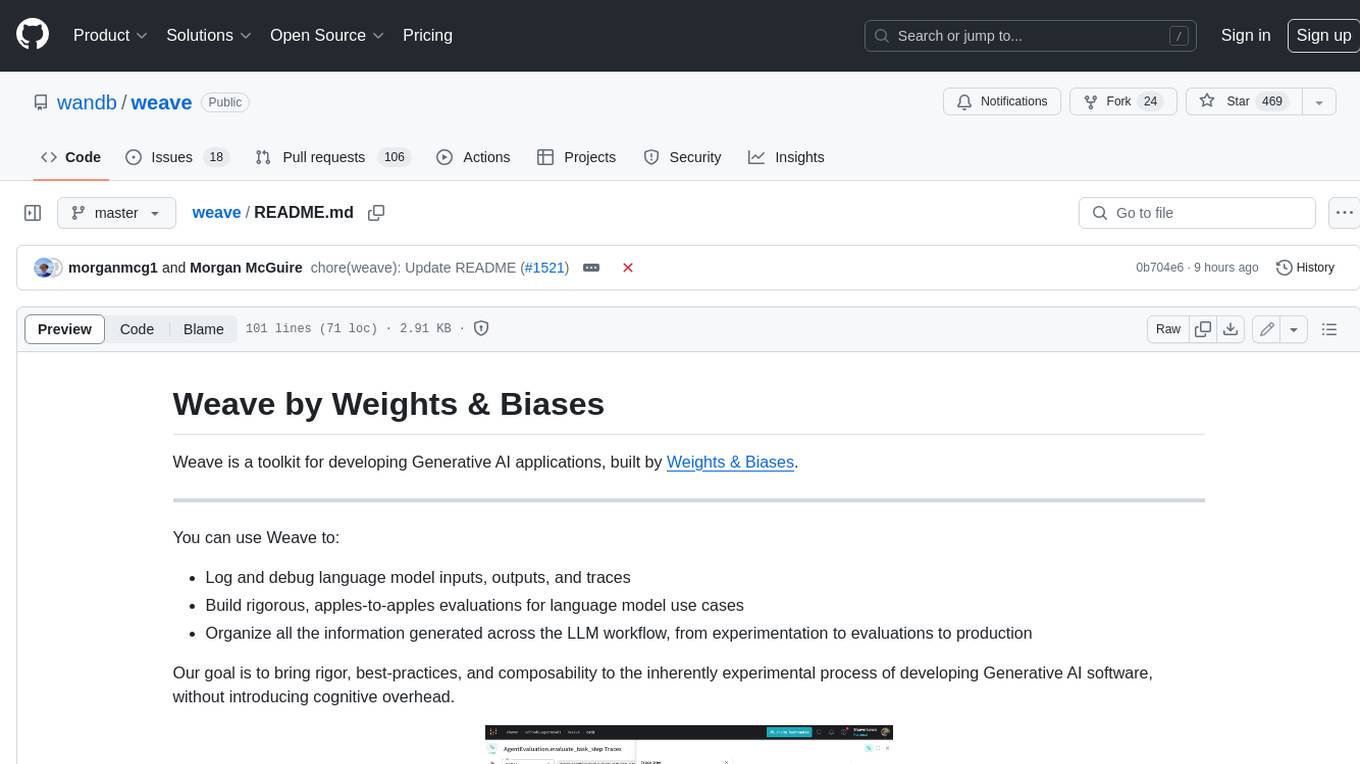
weave
Weave is a toolkit for developing Generative AI applications, built by Weights & Biases. With Weave, you can log and debug language model inputs, outputs, and traces; build rigorous, apples-to-apples evaluations for language model use cases; and organize all the information generated across the LLM workflow, from experimentation to evaluations to production. Weave aims to bring rigor, best-practices, and composability to the inherently experimental process of developing Generative AI software, without introducing cognitive overhead.
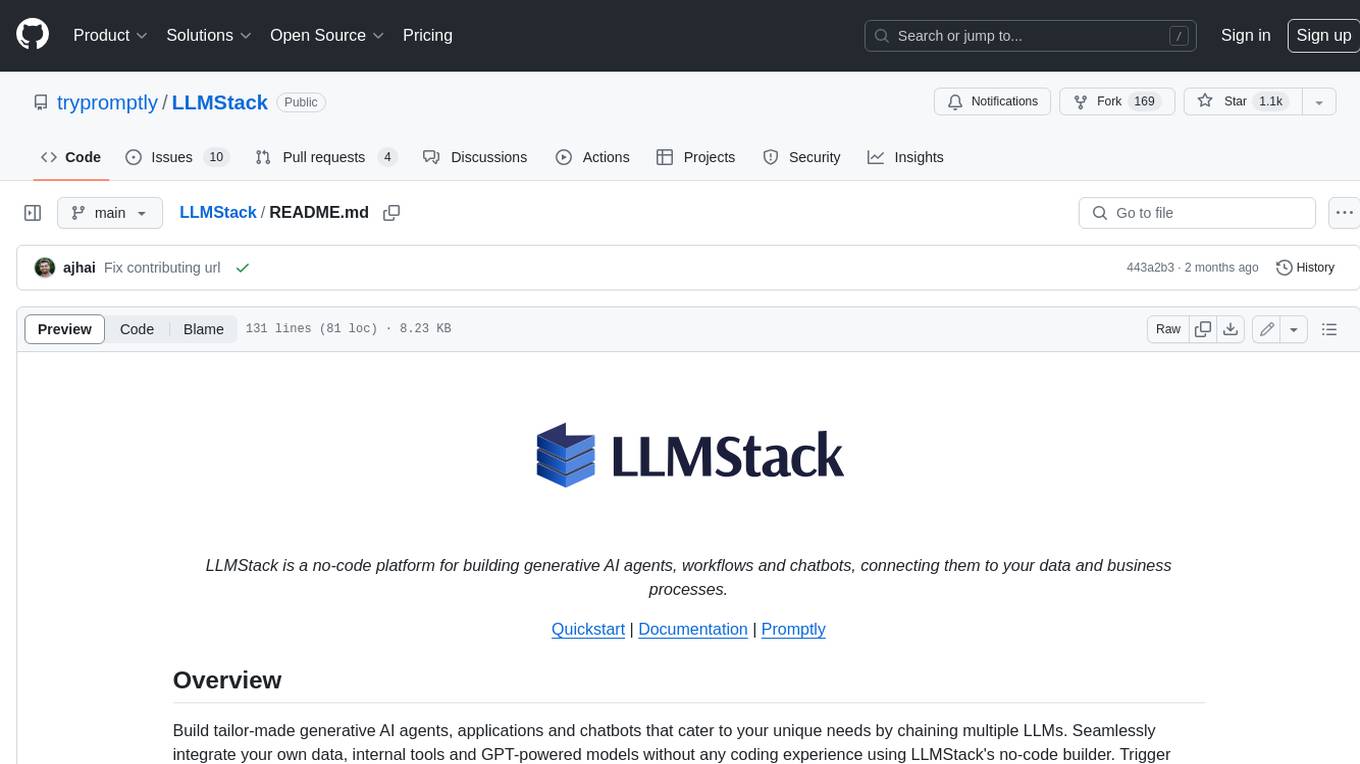
LLMStack
LLMStack is a no-code platform for building generative AI agents, workflows, and chatbots. It allows users to connect their own data, internal tools, and GPT-powered models without any coding experience. LLMStack can be deployed to the cloud or on-premise and can be accessed via HTTP API or triggered from Slack or Discord.
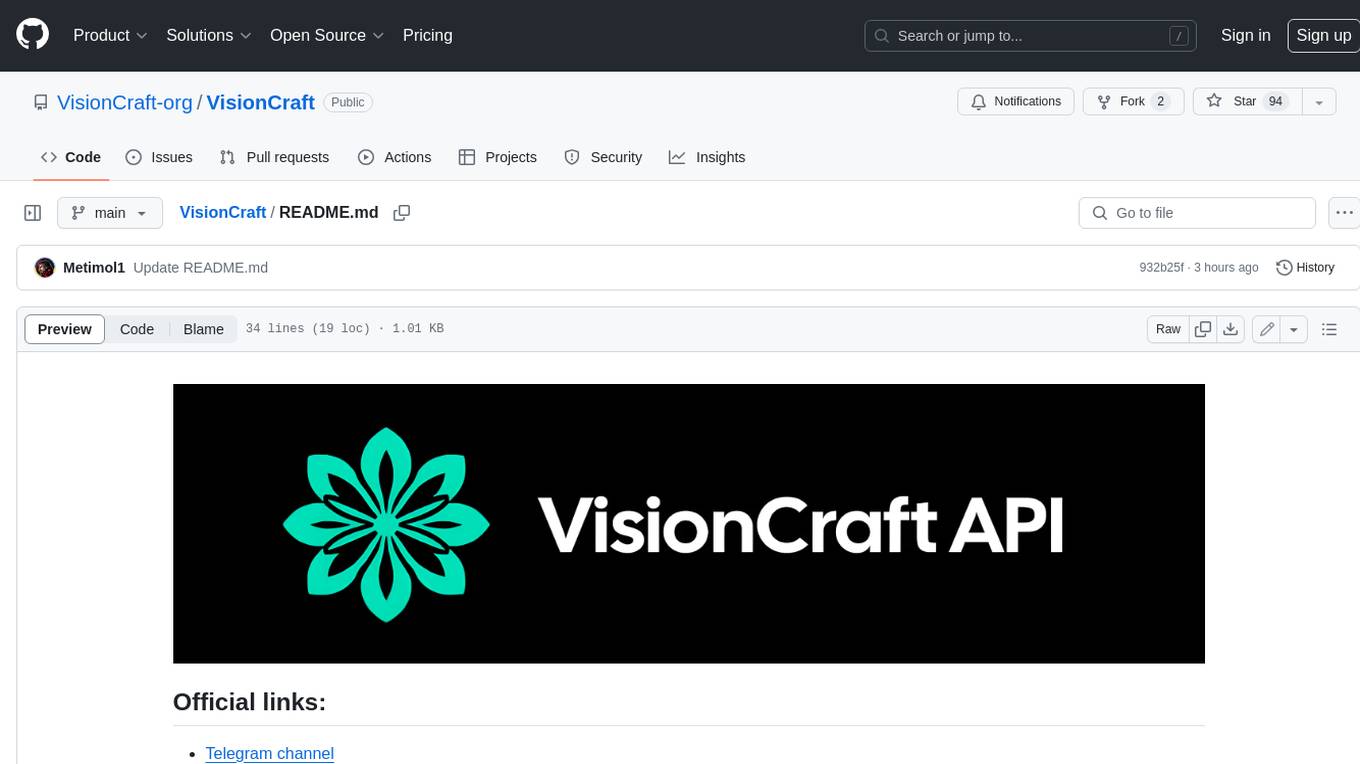
VisionCraft
The VisionCraft API is a free API for using over 100 different AI models. From images to sound.
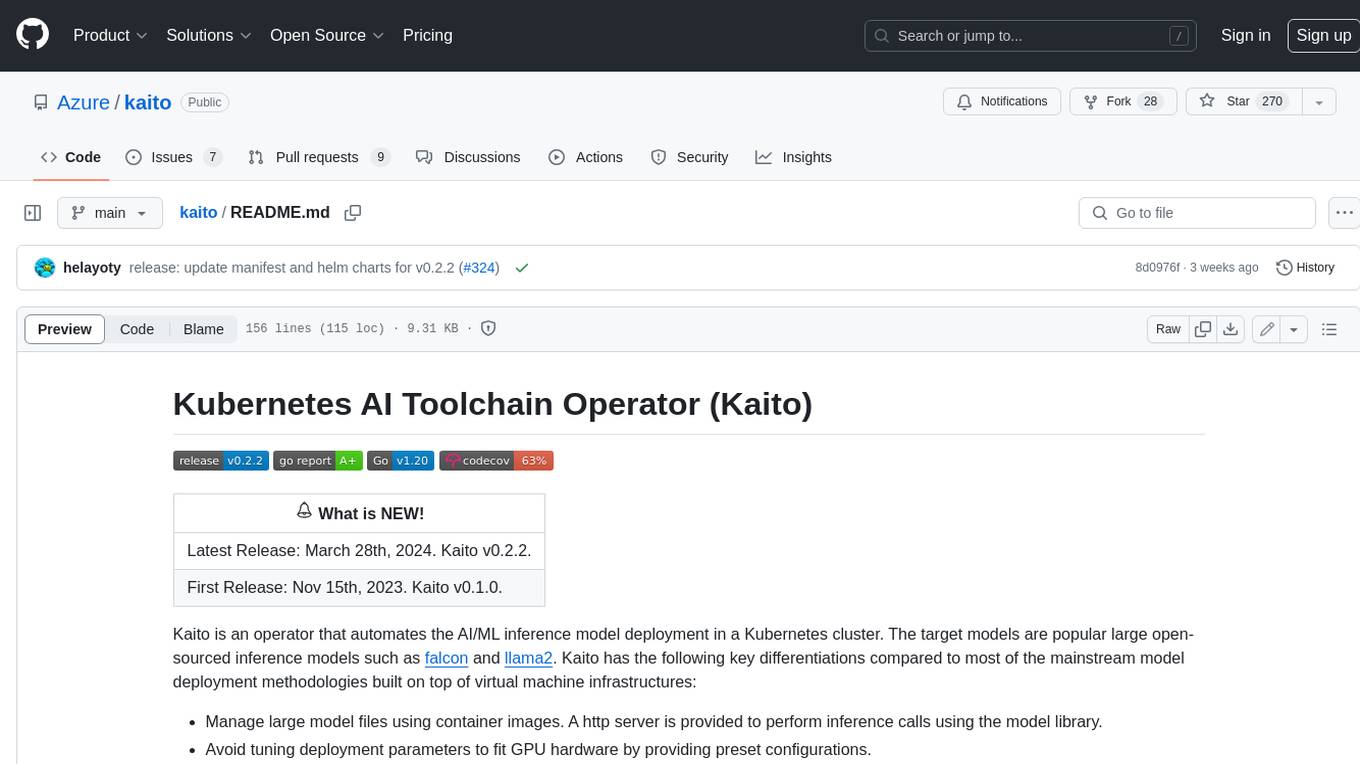
kaito
Kaito is an operator that automates the AI/ML inference model deployment in a Kubernetes cluster. It manages large model files using container images, avoids tuning deployment parameters to fit GPU hardware by providing preset configurations, auto-provisions GPU nodes based on model requirements, and hosts large model images in the public Microsoft Container Registry (MCR) if the license allows. Using Kaito, the workflow of onboarding large AI inference models in Kubernetes is largely simplified.
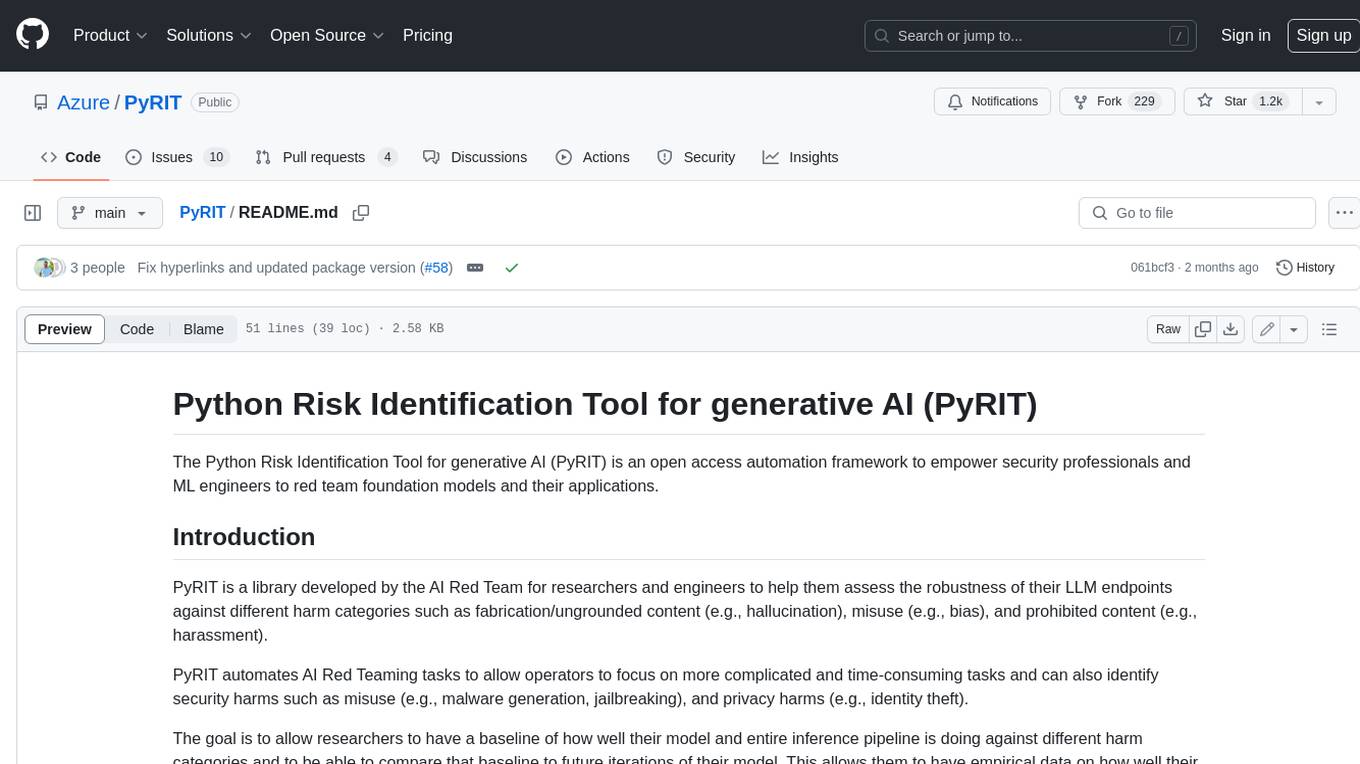
PyRIT
PyRIT is an open access automation framework designed to empower security professionals and ML engineers to red team foundation models and their applications. It automates AI Red Teaming tasks to allow operators to focus on more complicated and time-consuming tasks and can also identify security harms such as misuse (e.g., malware generation, jailbreaking), and privacy harms (e.g., identity theft). The goal is to allow researchers to have a baseline of how well their model and entire inference pipeline is doing against different harm categories and to be able to compare that baseline to future iterations of their model. This allows them to have empirical data on how well their model is doing today, and detect any degradation of performance based on future improvements.
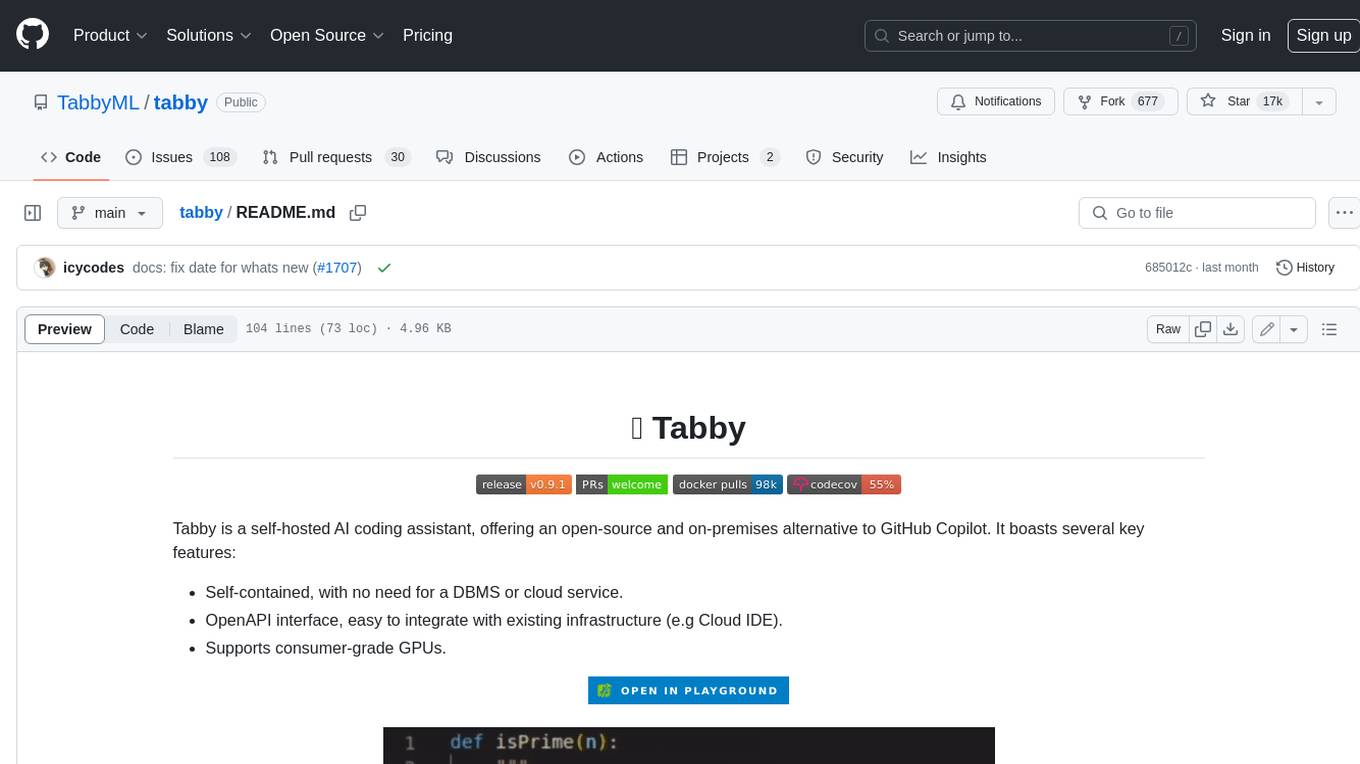
tabby
Tabby is a self-hosted AI coding assistant, offering an open-source and on-premises alternative to GitHub Copilot. It boasts several key features: * Self-contained, with no need for a DBMS or cloud service. * OpenAPI interface, easy to integrate with existing infrastructure (e.g Cloud IDE). * Supports consumer-grade GPUs.
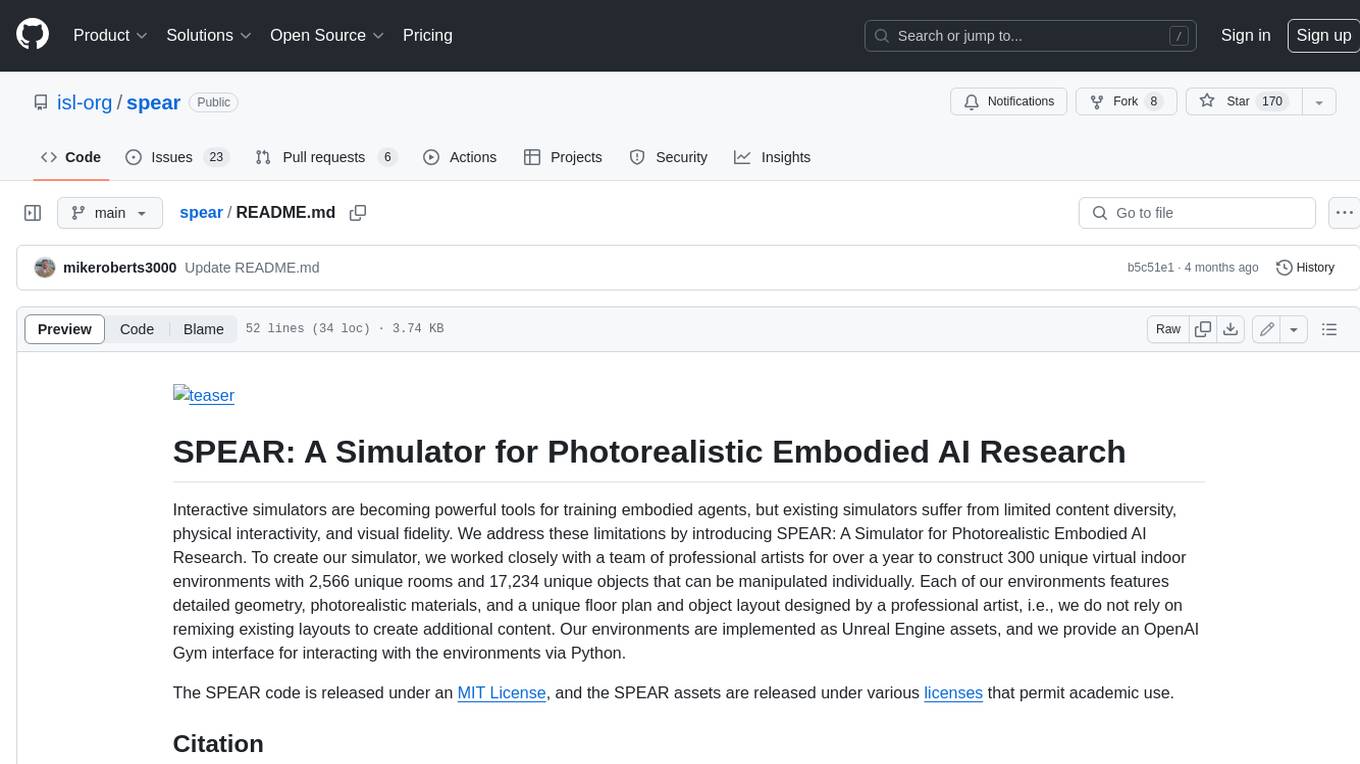
spear
SPEAR (Simulator for Photorealistic Embodied AI Research) is a powerful tool for training embodied agents. It features 300 unique virtual indoor environments with 2,566 unique rooms and 17,234 unique objects that can be manipulated individually. Each environment is designed by a professional artist and features detailed geometry, photorealistic materials, and a unique floor plan and object layout. SPEAR is implemented as Unreal Engine assets and provides an OpenAI Gym interface for interacting with the environments via Python.
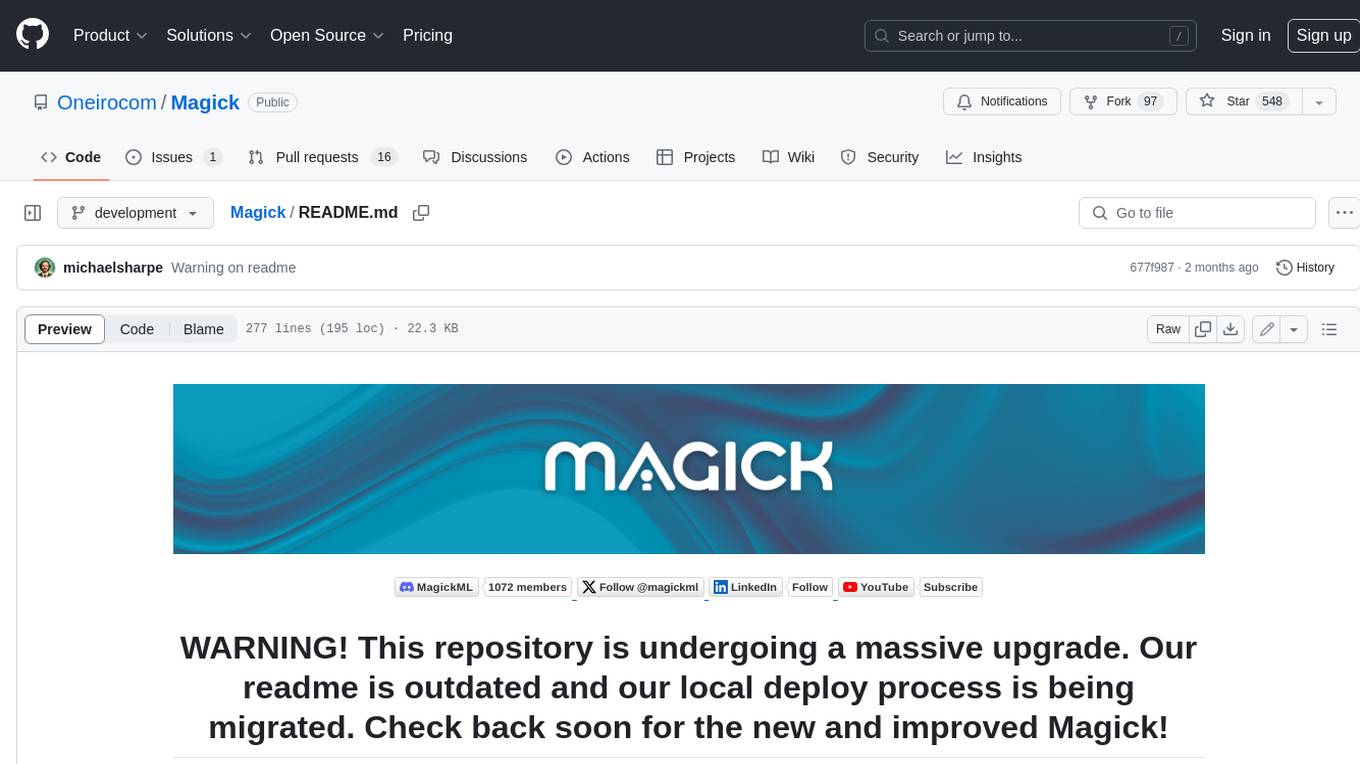
Magick
Magick is a groundbreaking visual AIDE (Artificial Intelligence Development Environment) for no-code data pipelines and multimodal agents. Magick can connect to other services and comes with nodes and templates well-suited for intelligent agents, chatbots, complex reasoning systems and realistic characters.