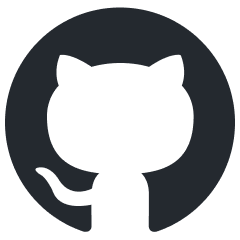
MHA2MLA
Towards Economical Inference: Enabling DeepSeek's Multi-Head Latent Attention in Any Transformer-based LLMs
Stars: 145
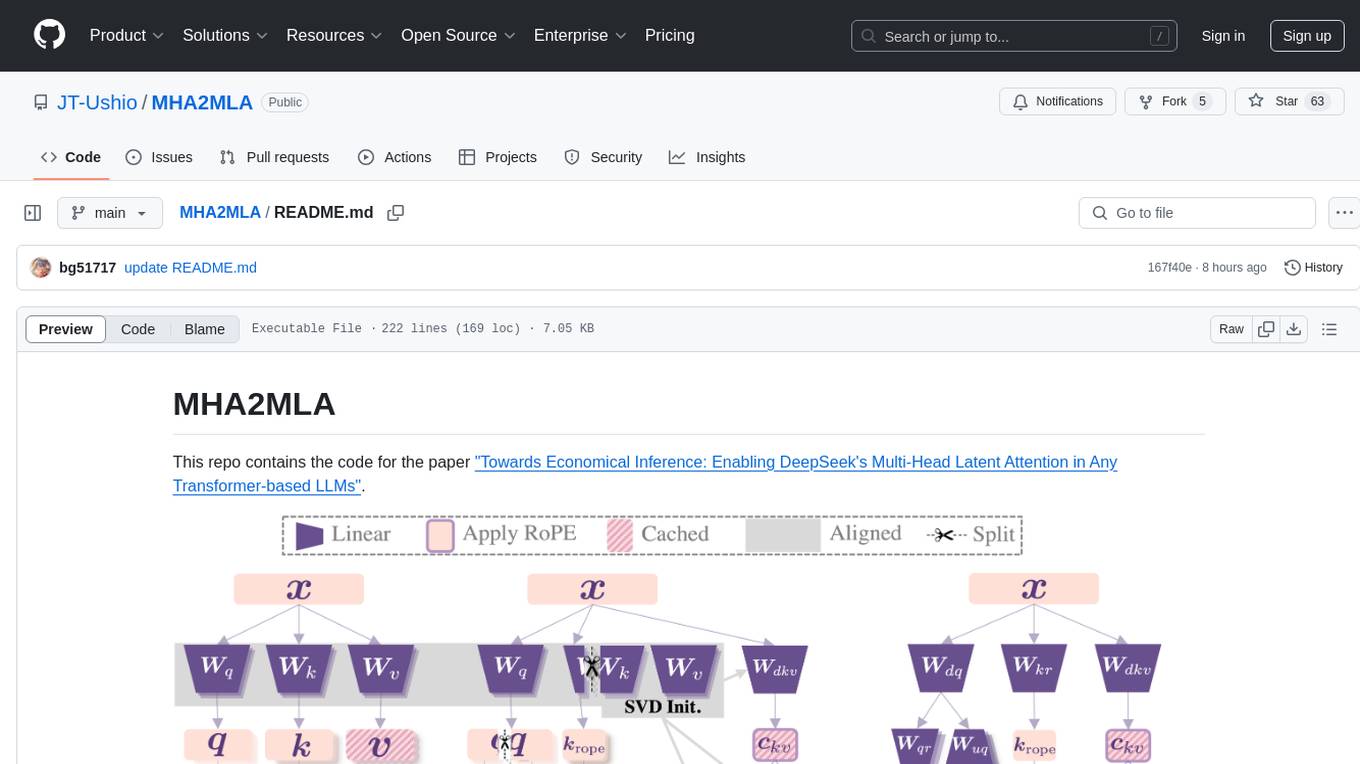
This repository contains the code for the paper 'Towards Economical Inference: Enabling DeepSeek's Multi-Head Latent Attention in Any Transformer-based LLMs'. It provides tools for fine-tuning and evaluating Llama models, converting models between different frameworks, processing datasets, and performing specific model training tasks like Partial-RoPE Fine-Tuning and Multiple-Head Latent Attention Fine-Tuning. The repository also includes commands for model evaluation using Lighteval and LongBench, along with necessary environment setup instructions.
README:
This repo contains the code for the paper "Towards Economical Inference: Enabling DeepSeek's Multi-Head Latent Attention in Any Transformer-based LLMs".
- [2025.03.12] Released the inference code implemented using PyTorch (support for FlashMLA inference requires additional development time).
- [2025.03.04] The four MLA checkpoints ($d_{kv}$=8/16/32/128) derived from
SmolLM-135M/360M/1B7
are publicly available. - [2025.03.03] The four MLA checkpoints ($d_{kv}$=16/32/64/256) derived from
Llama-2-7B
are publicly available. - [2025.02.21] The paper of MHA2MLA is publicly available: https://arxiv.org/abs/2502.14837
- [2025.02.19] Released the first version of the MHA2MLA code, providing usage code for Llama fine-tuning and evaluating.
- [ ]
Provide the code for incorporating the projection matrix and inference. - [ ] Thanks to DeepSeek for open-sourcing the FlashMLA inference framework. It’s theoretically possible to save more GPU memory usage using this framework. Let’s see how economical MHA2MLA + FlashMLA (+ KV quanto) can be!
- [x] Release the code of MHA2MLA based on HuggingFace
Transformers
- SmolLM: https://huggingface.co/blog/smollm
- Llama-2-7b-hf: https://huggingface.co/meta-llama/Llama-2-7b-hf
First download the datasets.
- smollm-corpus(fineweb-edu-dedup, cosmopedia-v2, python-edu): https://huggingface.co/datasets/HuggingFaceTB/smollm-corpus
- open-web-math: https://huggingface.co/datasets/open-web-math/open-web-math
- stackoverflow: https://huggingface.co/datasets/bigcode/stackoverflow-clean
Secondly, process the datasets according to https://github.com/huggingface/nanotron/blob/main/docs/nanoset.md.
Install pytorch and other packages.
conda create -n mla-ft python=3.11
pip install torch==2.4.0 torchvision==0.19.0
pip install -r requirements.txt
The research presented in our paper was conducted using nanotron framework. Since there are differences between
transformers
andnanotron
, hyperparameter search might be necessary. For exact reproduction of the paper's results, we recommend using nanotron for fine tuneing which refer to Our README for MHA2MLA using nanotron.
First, prepare three configuration files:
- A general configuration file referencing 135M_4GPU.yaml
- A partial-RoPE configuration file referencing rope_v4_topk4.yaml
- A SVD configuration file referencing svd_method7_rank8.yaml
The available strategies for each method are listed below:
Partial-RoPE version | Strategy |
---|---|
0 | full-RoPE |
1 | $\mathcal{S}_{\text{high}}$ |
2 | $\mathcal{S}_{\text{uniform}}$ |
4 | $\mathcal{S}_{\text{2-norm}}$ |
5 | $\mathcal{S}_{\text{low}}$ |
SVD version | Strategy |
---|---|
2 | $SVD_{split}$ |
7 | $SVD_{joint}$ |
Then, use the following command for MLA fine-tuning:
torchrun --nproc_per_node 4 \
../src/mha2mla/run_train.py \
--config_file ../configs_hf/rope/135M_4GPU.yaml \
--partial_rope_config ../configs_hf/rope/rope_v4_topk4.yaml \
--svd_config ../configs_hf/rope/svd_method7_rank8.yaml
If you want to use the partial-RoPE version 4, you should get the
qk_tensor
first. Using the following command, you can get theqk_tensor
:torchrun --nproc_per_node 1 \ ../src/mha2mla/2_norm.py \ --config_file ../configs_hf/rope/135M_4GPU.yaml \ --output_dir ./qk_tensor_hf_test.pth \ --sample_size 1024
For the MLA evaluation, you can use the following command:
accelerate launch --multi_gpu --num_processes=4 \
../src/mha2mla/eval.py --is_mla \
accelerate \
--model_args "pretrained=${model_name_or_path},revision=main,dtype=bfloat16,max_length=2048" \
--override_batch_size 48 \
--custom_tasks "../src/mha2mla/tasks.py" \
--tasks "../src/mha2mla/smollm1_base.txt" \
--output_dir "../eval_results/"
If you want to evaluate the
partial_rope
ckpt withoutlow rank approx
, you should change--is_mla
to--is_partial_rope
.
For the baseline evaluation, you can use the following command:
torchrun --nproc_per_node=4 \
../src/mha2mla/longbench.py \
--model_path ${model_name_or_path} \
--tokenizer_path ${model_name_or_path} \
--longbench True \
--lb_max_tokens 2048 \
--lb_batch_size 16 \
--output_dir /longbench/bf16 \
--dtype "bfloat16"
For the MLA model, you should add the parameter --is_mla
to the command.
If you want to use the quantized KV cache, you can use the following command:
torchrun --nproc_per_node=4 \
../src/mha2mla/longbench.py \
--model_path ${model_name_or_path} \
--tokenizer_path ${model_name_or_path} \
--longbench True \
--lb_max_tokens 2048 \
--lb_batch_size 16 \
--output_dir /longbench/${model_name_or_path}_hqq_int4 \
--dtype "bfloat16" \
--cache_implementation "quantized" \
--backend "HQQ" \
--nbits 4 \
--residual_length 128 \
- Step 1: Download the monkey patch file.
wget https://raw.githubusercontent.com/JT-Ushio/MHA2MLA/refs/heads/main/src/mha2mla/monkey_patch.py
- Step 2(Option): For MHA2MLA models using Partial-RoPE 2-nrom method, Download the qk_2-norm file.
Take
qk_tensor_1.7B.pth
as an example:
wget https://github.com/JT-Ushio/MHA2MLA/raw/refs/heads/main/utils/qk_tensor_1.7B.pth
- Step 3: Download the MHA2MLA models and run inference.
Take
fnlp/SmolLM-1B7-MLA-d_kv_16
as an example:
import torch
from transformers import AutoConfig, AutoTokenizer, LlamaForCausalLM
from monkey_patch import infer_monkey_patch
model_name = "fnlp/SmolLM-1B7-MLA-d_kv_16"
# Monkey Patch: MHA -> MLA
config = AutoConfig.from_pretrained(model_name)
if "RoPE" in config:
config.RoPE["qk_tensor_path"] = "qk_tensor_1.7B.pth" # Configuration for Specific Models
infer_monkey_patch(config.RoPE)
tokenizer = AutoTokenizer.from_pretrained(model_name, trust_remote_code=True)
model = LlamaForCausalLM.from_pretrained(model_name, config=config, torch_dtype=torch.bfloat16).cuda()
# Generate
text = "Which American-born Sinclair won the Nobel Prize for Literature in 1930?"
inputs = tokenizer(text, return_tensors="pt").to(model.device)
generation_kwargs = {"do_sample": False, "use_cache": True, "max_new_tokens": 128}
output = model.generate(**inputs, **generation_kwargs)
print(tokenizer.decode(output[0], skip_special_tokens=True))
# - Sinclair Lewis
@misc{ji2025economicalinferenceenablingdeepseeks,
title={Towards Economical Inference: Enabling DeepSeek's Multi-Head Latent Attention in Any Transformer-based LLMs},
author={Tao Ji and Bin Guo and Yuanbin Wu and Qipeng Guo and Lixing Shen and Zhan Chen and Xipeng Qiu and Qi Zhang and Tao Gui},
year={2025},
eprint={2502.14837},
archivePrefix={arXiv},
primaryClass={cs.CL},
url={https://arxiv.org/abs/2502.14837},
}
For Tasks:
Click tags to check more tools for each tasksFor Jobs:
Alternative AI tools for MHA2MLA
Similar Open Source Tools
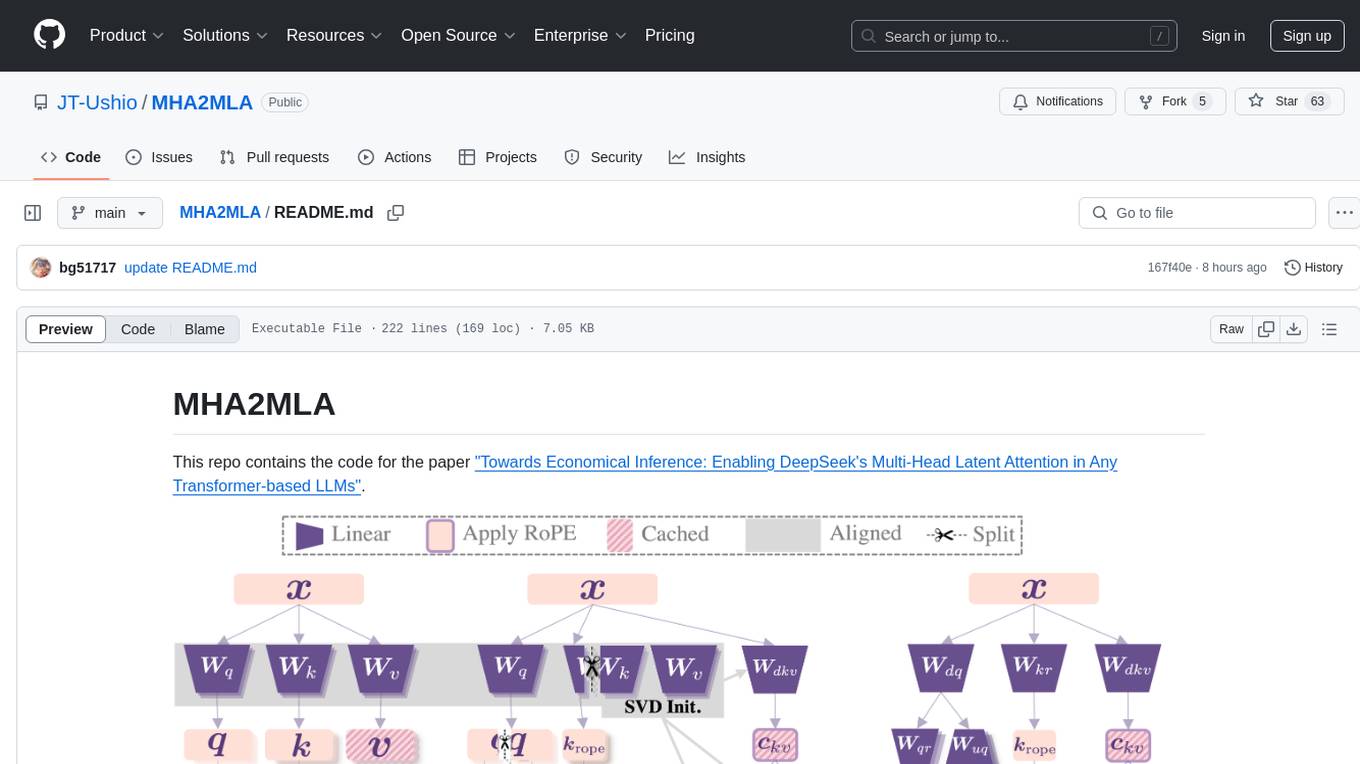
MHA2MLA
This repository contains the code for the paper 'Towards Economical Inference: Enabling DeepSeek's Multi-Head Latent Attention in Any Transformer-based LLMs'. It provides tools for fine-tuning and evaluating Llama models, converting models between different frameworks, processing datasets, and performing specific model training tasks like Partial-RoPE Fine-Tuning and Multiple-Head Latent Attention Fine-Tuning. The repository also includes commands for model evaluation using Lighteval and LongBench, along with necessary environment setup instructions.
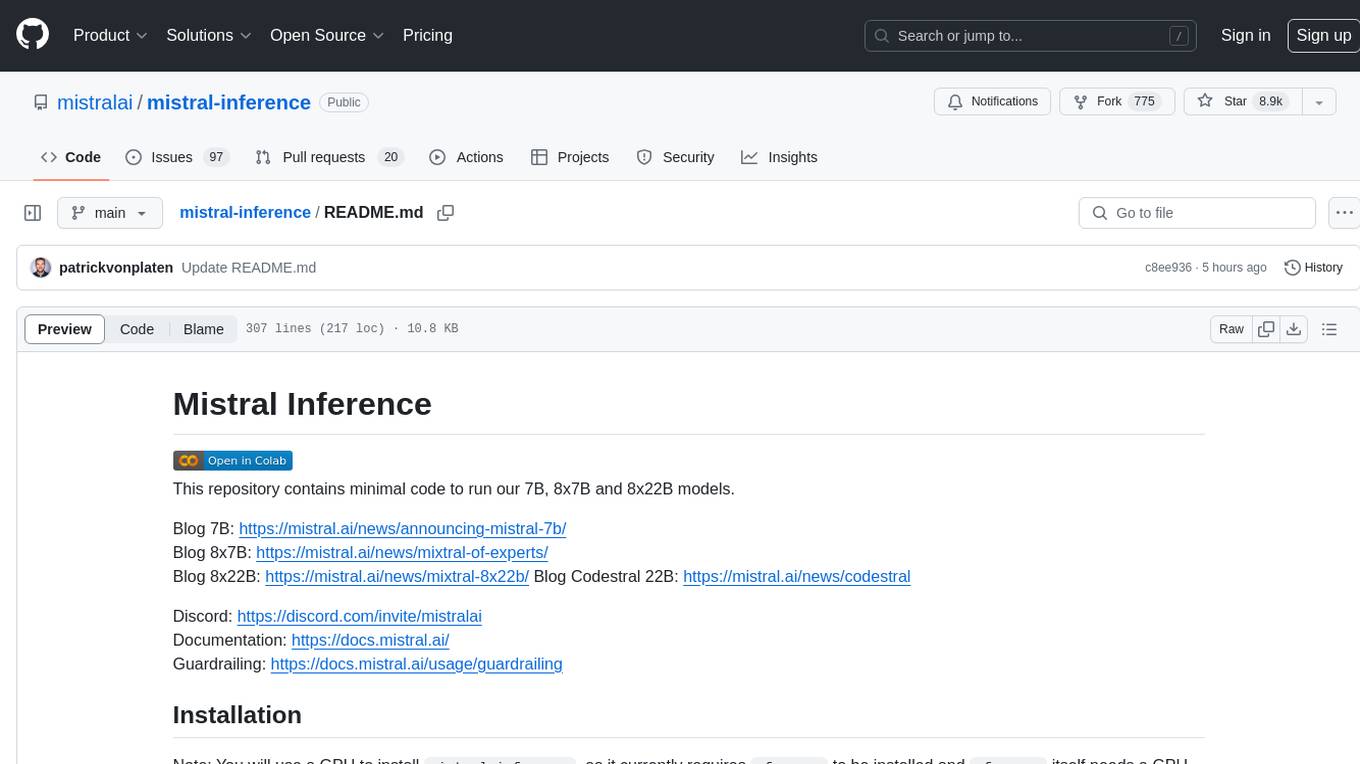
mistral-inference
Mistral Inference repository contains minimal code to run 7B, 8x7B, and 8x22B models. It provides model download links, installation instructions, and usage guidelines for running models via CLI or Python. The repository also includes information on guardrailing, model platforms, deployment, and references. Users can interact with models through commands like mistral-demo, mistral-chat, and mistral-common. Mistral AI models support function calling and chat interactions for tasks like testing models, chatting with models, and using Codestral as a coding assistant. The repository offers detailed documentation and links to blogs for further information.
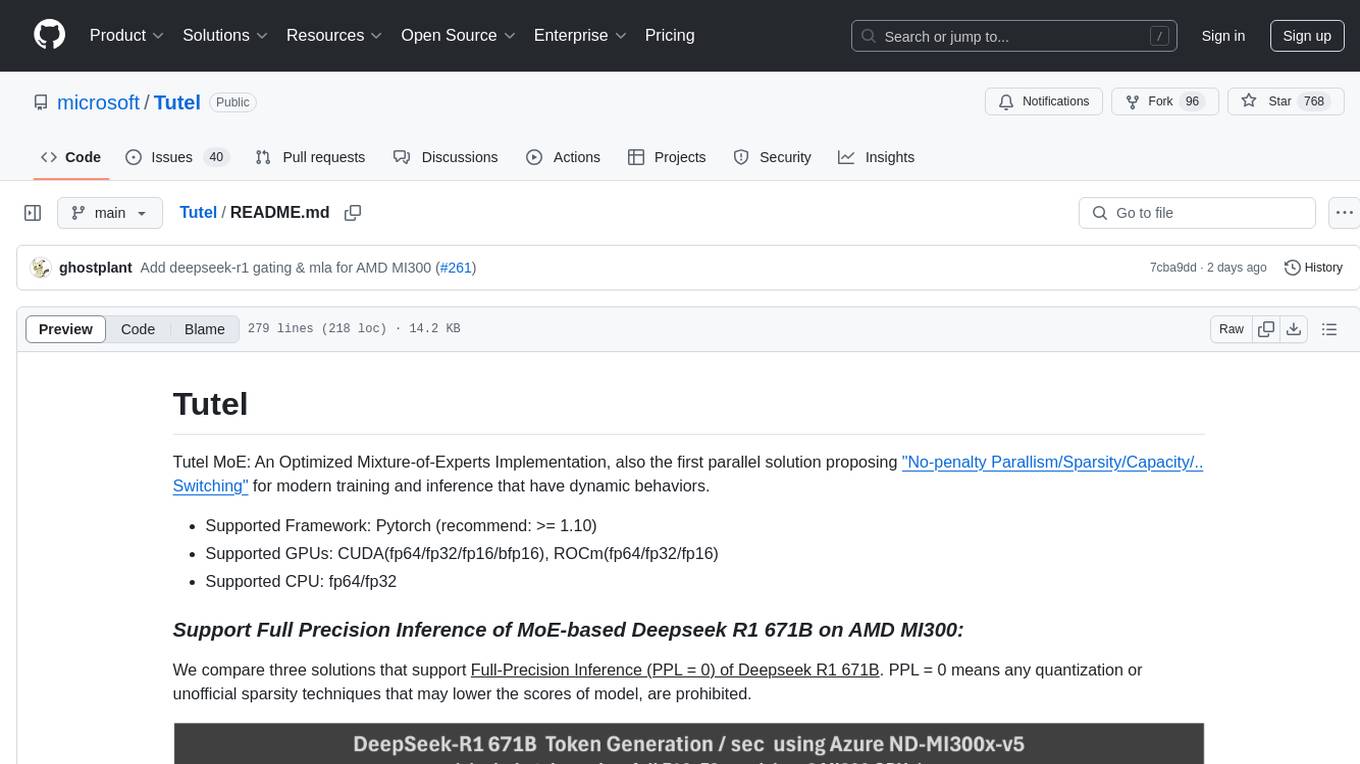
Tutel
Tutel MoE is an optimized Mixture-of-Experts implementation that offers a parallel solution with 'No-penalty Parallism/Sparsity/Capacity/Switching' for modern training and inference. It supports Pytorch framework (version >= 1.10) and various GPUs including CUDA and ROCm. The tool enables Full Precision Inference of MoE-based Deepseek R1 671B on AMD MI300. Tutel provides features like all-to-all benchmarking, tensorcore option, NCCL timeout settings, Megablocks solution, and dynamic switchable configurations. Users can run Tutel in distributed mode across multiple GPUs and machines. The tool allows for custom MoE implementations and offers detailed usage examples and reference documentation.
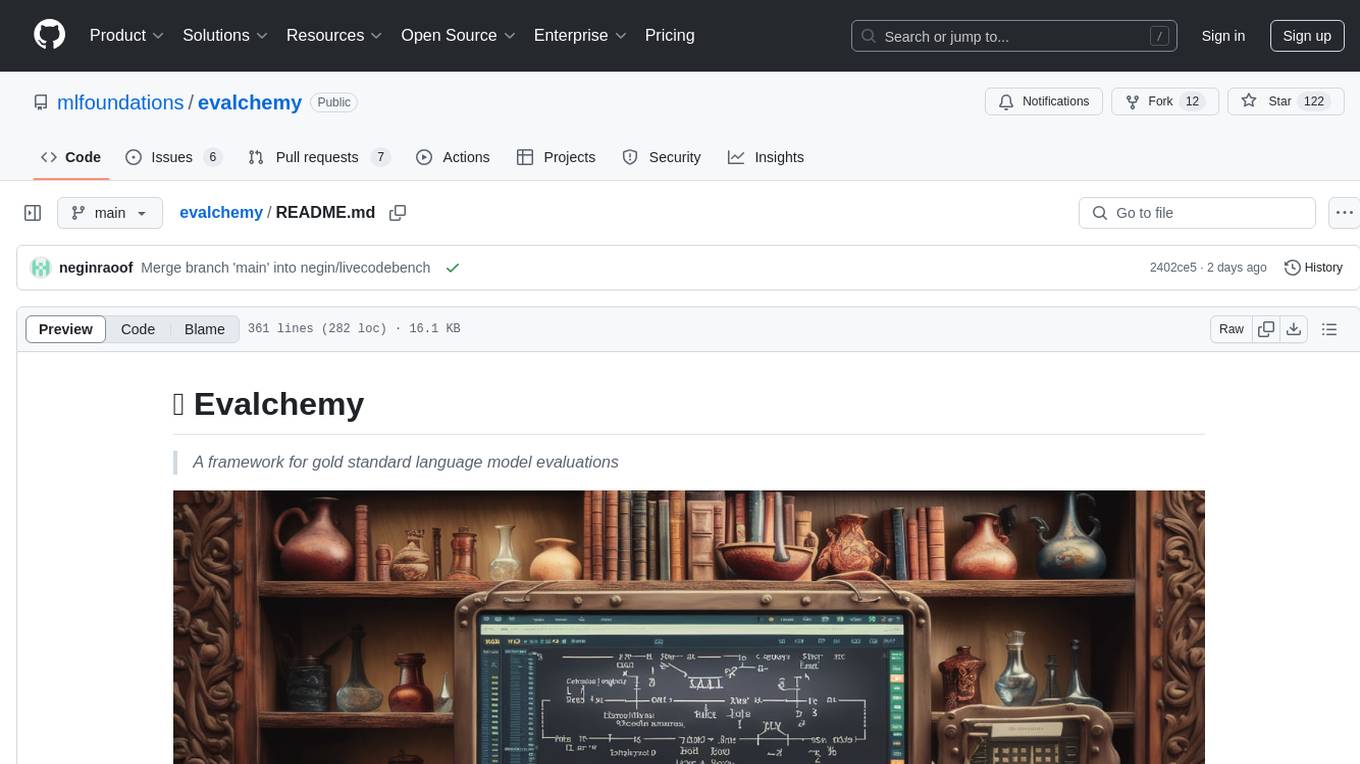
evalchemy
Evalchemy is a unified and easy-to-use toolkit for evaluating language models, focusing on post-trained models. It integrates multiple existing benchmarks such as RepoBench, AlpacaEval, and ZeroEval. Key features include unified installation, parallel evaluation, simplified usage, and results management. Users can run various benchmarks with a consistent command-line interface and track results locally or integrate with a database for systematic tracking and leaderboard submission.
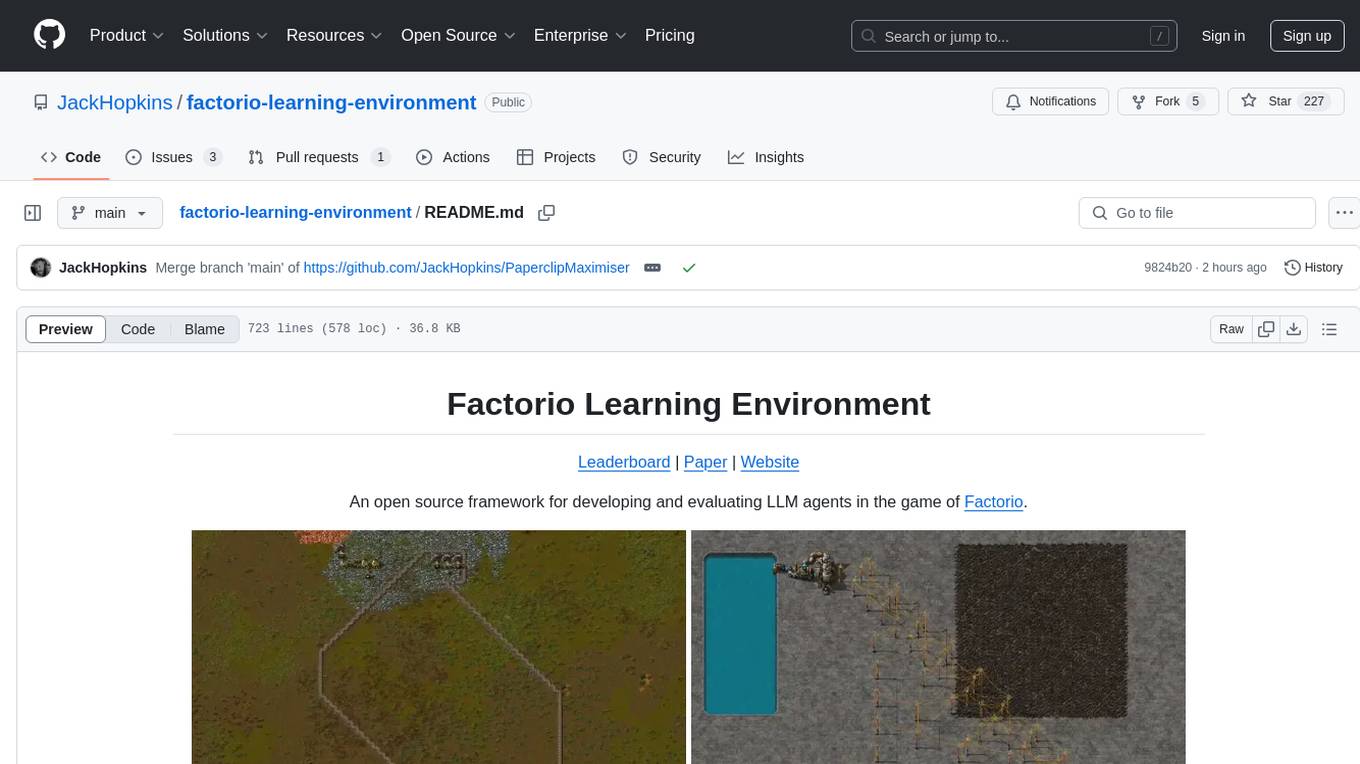
factorio-learning-environment
Factorio Learning Environment is an open source framework designed for developing and evaluating LLM agents in the game of Factorio. It provides two settings: Lab-play with structured tasks and Open-play for building large factories. Results show limitations in spatial reasoning and automation strategies. Agents interact with the environment through code synthesis, observation, action, and feedback. Tools are provided for game actions and state representation. Agents operate in episodes with observation, planning, and action execution. Tasks specify agent goals and are implemented in JSON files. The project structure includes directories for agents, environment, cluster, data, docs, eval, and more. A database is used for checkpointing agent steps. Benchmarks show performance metrics for different configurations.
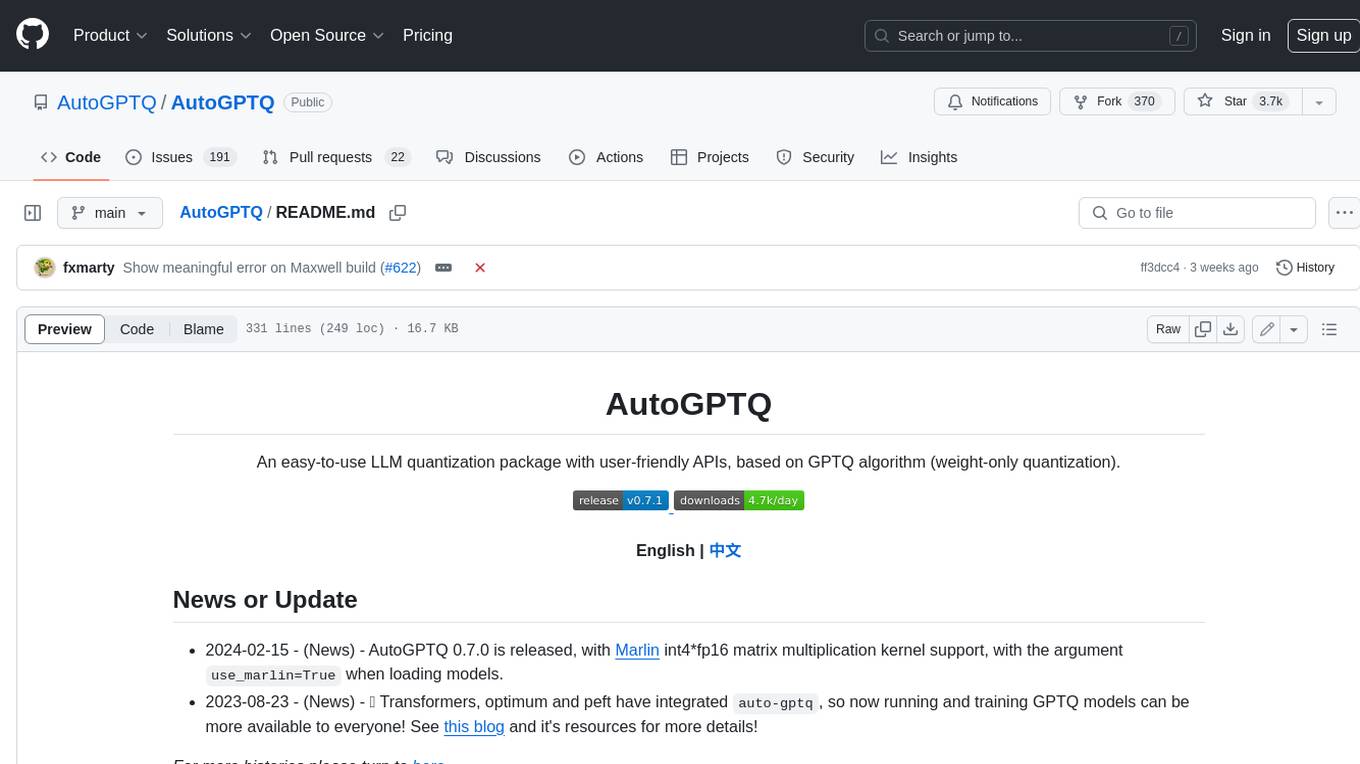
AutoGPTQ
AutoGPTQ is an easy-to-use LLM quantization package with user-friendly APIs, based on GPTQ algorithm (weight-only quantization). It provides a simple and efficient way to quantize large language models (LLMs) to reduce their size and computational cost while maintaining their performance. AutoGPTQ supports a wide range of LLM models, including GPT-2, GPT-J, OPT, and BLOOM. It also supports various evaluation tasks, such as language modeling, sequence classification, and text summarization. With AutoGPTQ, users can easily quantize their LLM models and deploy them on resource-constrained devices, such as mobile phones and embedded systems.
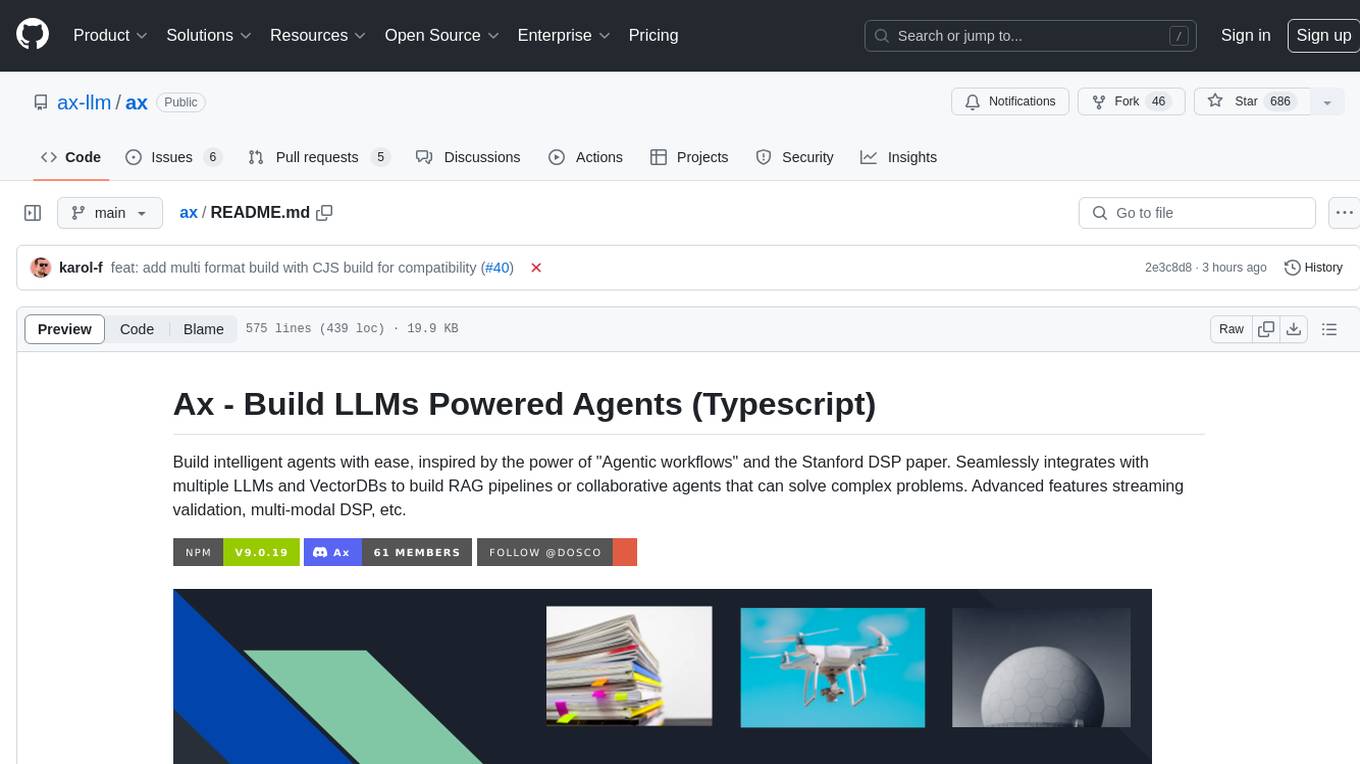
ax
Ax is a Typescript library that allows users to build intelligent agents inspired by agentic workflows and the Stanford DSP paper. It seamlessly integrates with multiple Large Language Models (LLMs) and VectorDBs to create RAG pipelines or collaborative agents capable of solving complex problems. The library offers advanced features such as streaming validation, multi-modal DSP, and automatic prompt tuning using optimizers. Users can easily convert documents of any format to text, perform smart chunking, embedding, and querying, and ensure output validation while streaming. Ax is production-ready, written in Typescript, and has zero dependencies.
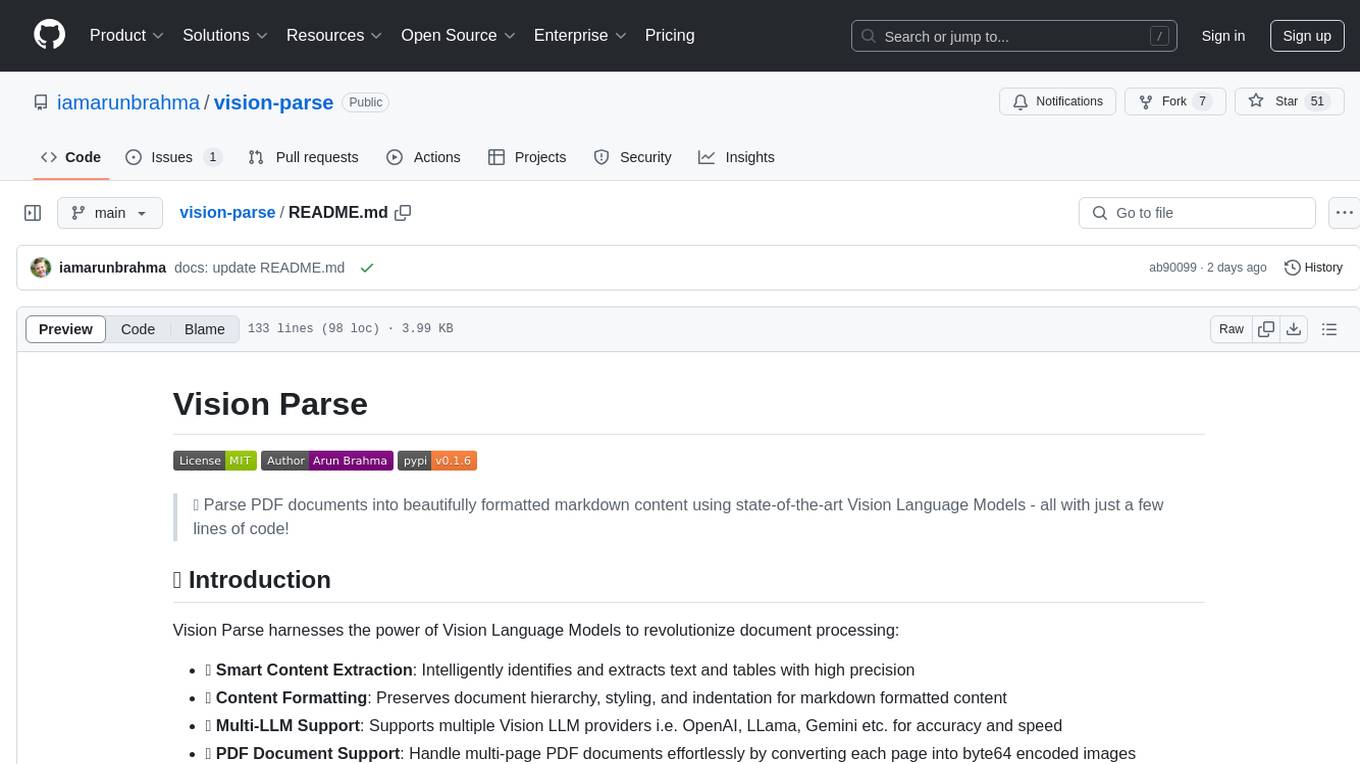
vision-parse
Vision Parse is a tool that leverages Vision Language Models to parse PDF documents into beautifully formatted markdown content. It offers smart content extraction, content formatting, multi-LLM support, PDF document support, and local model hosting using Ollama. Users can easily convert PDFs to markdown with high precision and preserve document hierarchy and styling. The tool supports multiple Vision LLM providers like OpenAI, LLama, and Gemini for accuracy and speed, making document processing efficient and effortless.
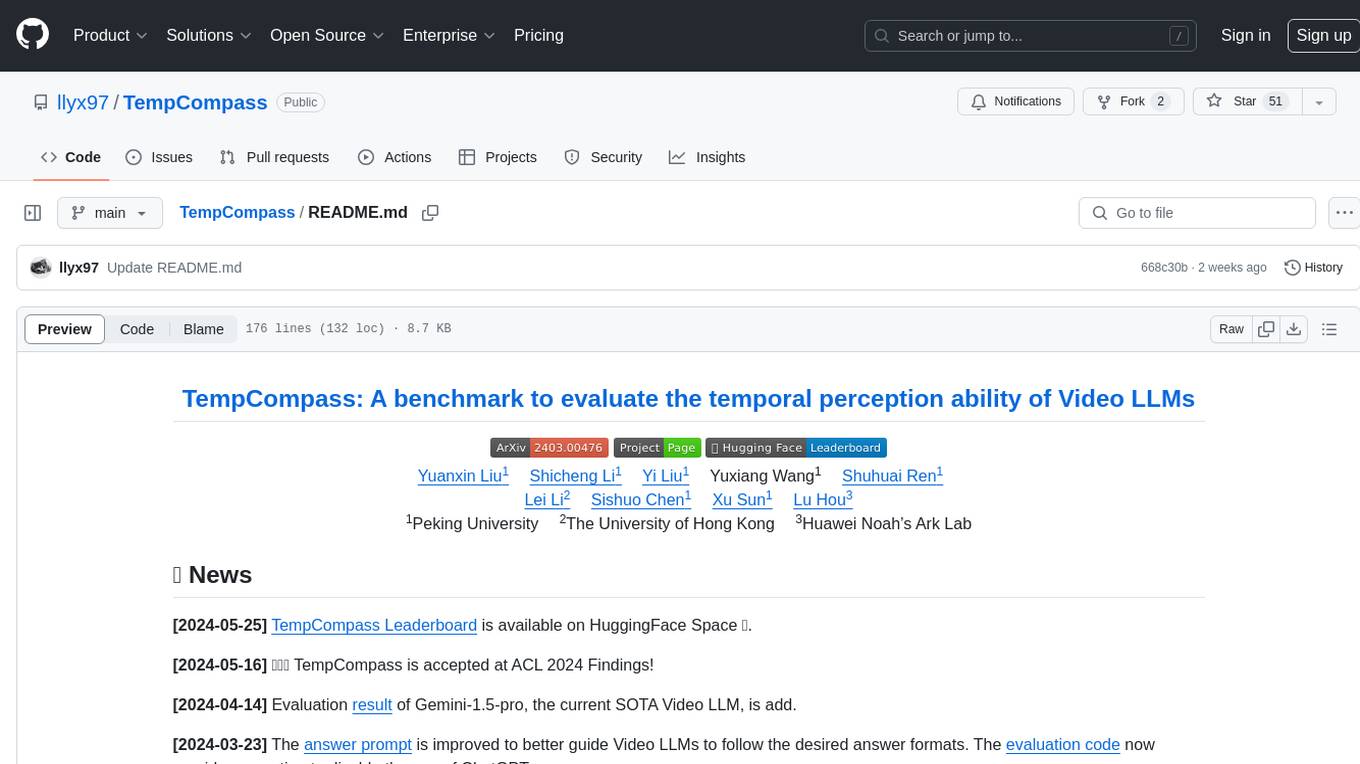
TempCompass
TempCompass is a benchmark designed to evaluate the temporal perception ability of Video LLMs. It encompasses a diverse set of temporal aspects and task formats to comprehensively assess the capability of Video LLMs in understanding videos. The benchmark includes conflicting videos to prevent models from relying on single-frame bias and language priors. Users can clone the repository, install required packages, prepare data, run inference using examples like Video-LLaVA and Gemini, and evaluate the performance of their models across different tasks such as Multi-Choice QA, Yes/No QA, Caption Matching, and Caption Generation.
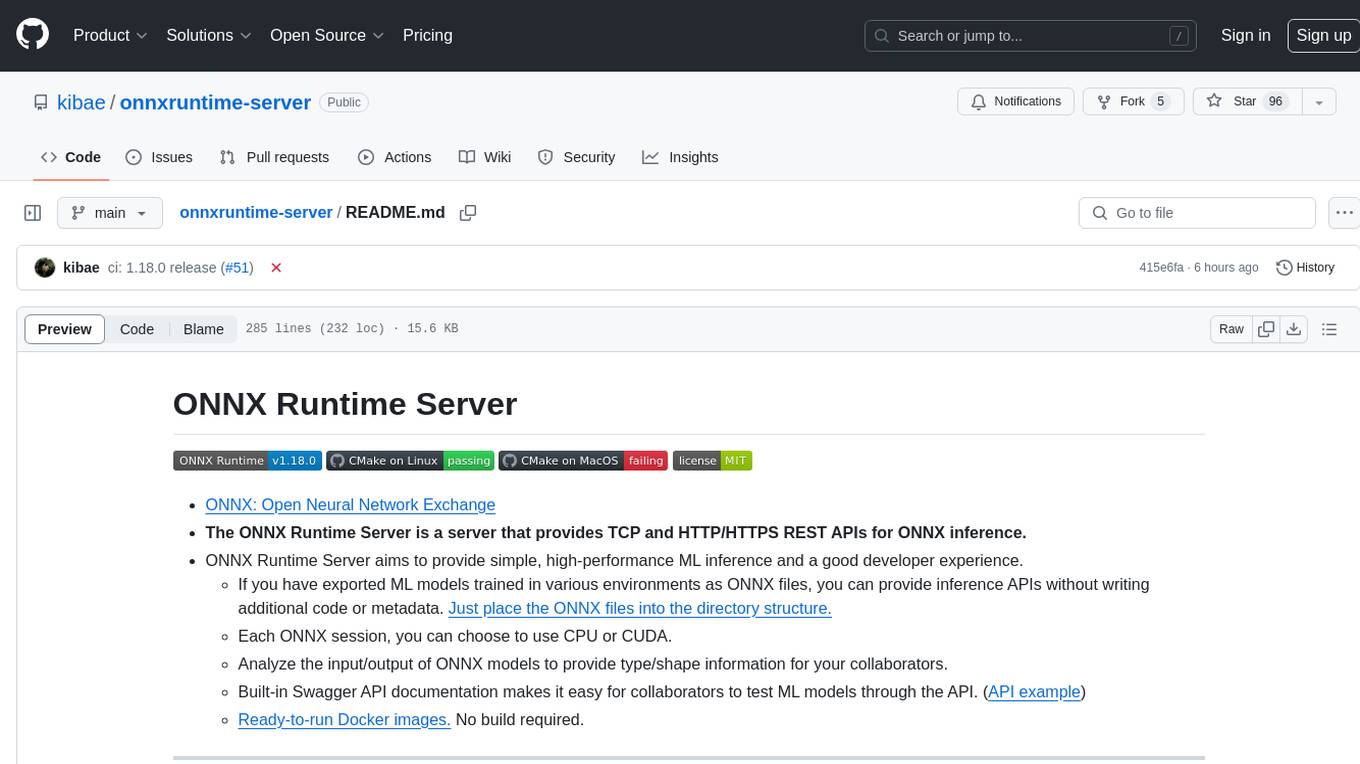
onnxruntime-server
ONNX Runtime Server is a server that provides TCP and HTTP/HTTPS REST APIs for ONNX inference. It aims to offer simple, high-performance ML inference and a good developer experience. Users can provide inference APIs for ONNX models without writing additional code by placing the models in the directory structure. Each session can choose between CPU or CUDA, analyze input/output, and provide Swagger API documentation for easy testing. Ready-to-run Docker images are available, making it convenient to deploy the server.
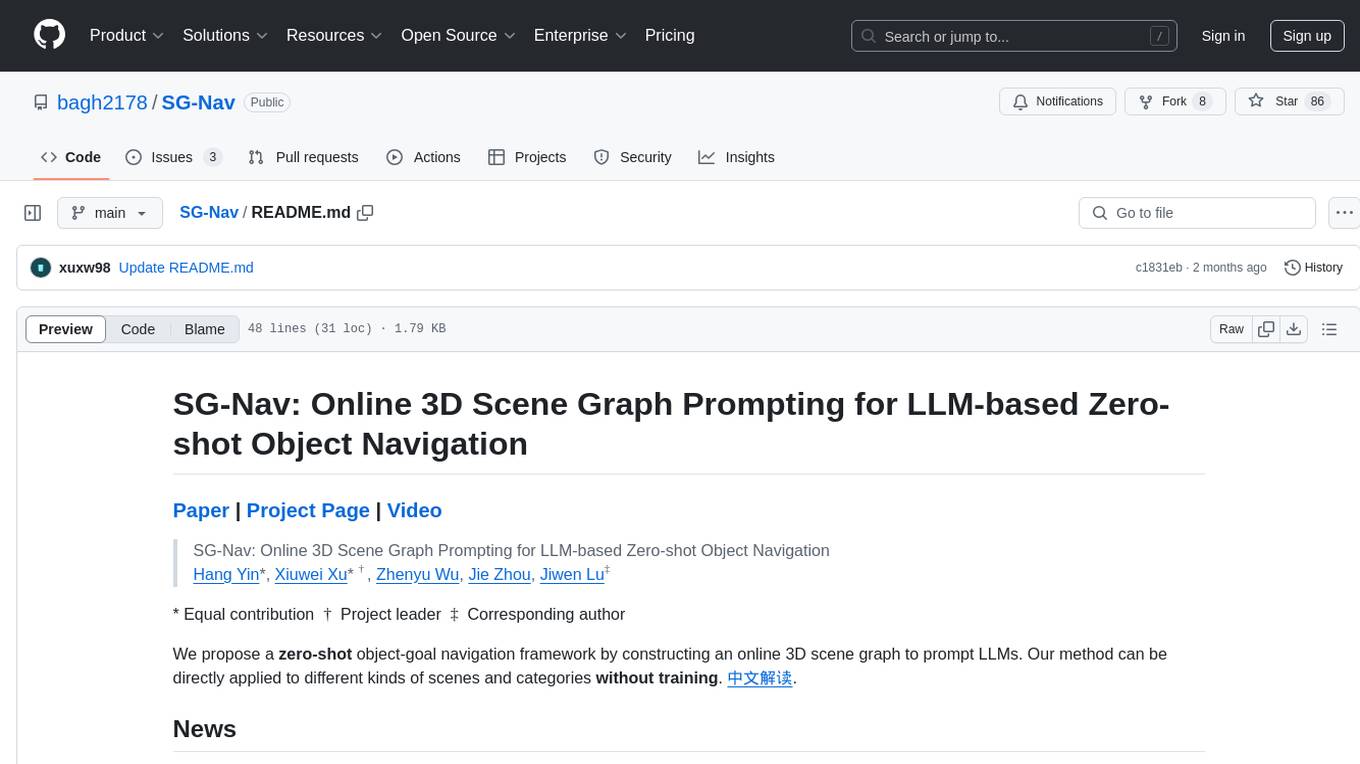
SG-Nav
SG-Nav is an online 3D scene graph prompting tool designed for LLM-based zero-shot object navigation. It proposes a framework that constructs an online 3D scene graph to prompt LLMs, allowing direct application to various scenes and categories without the need for training.
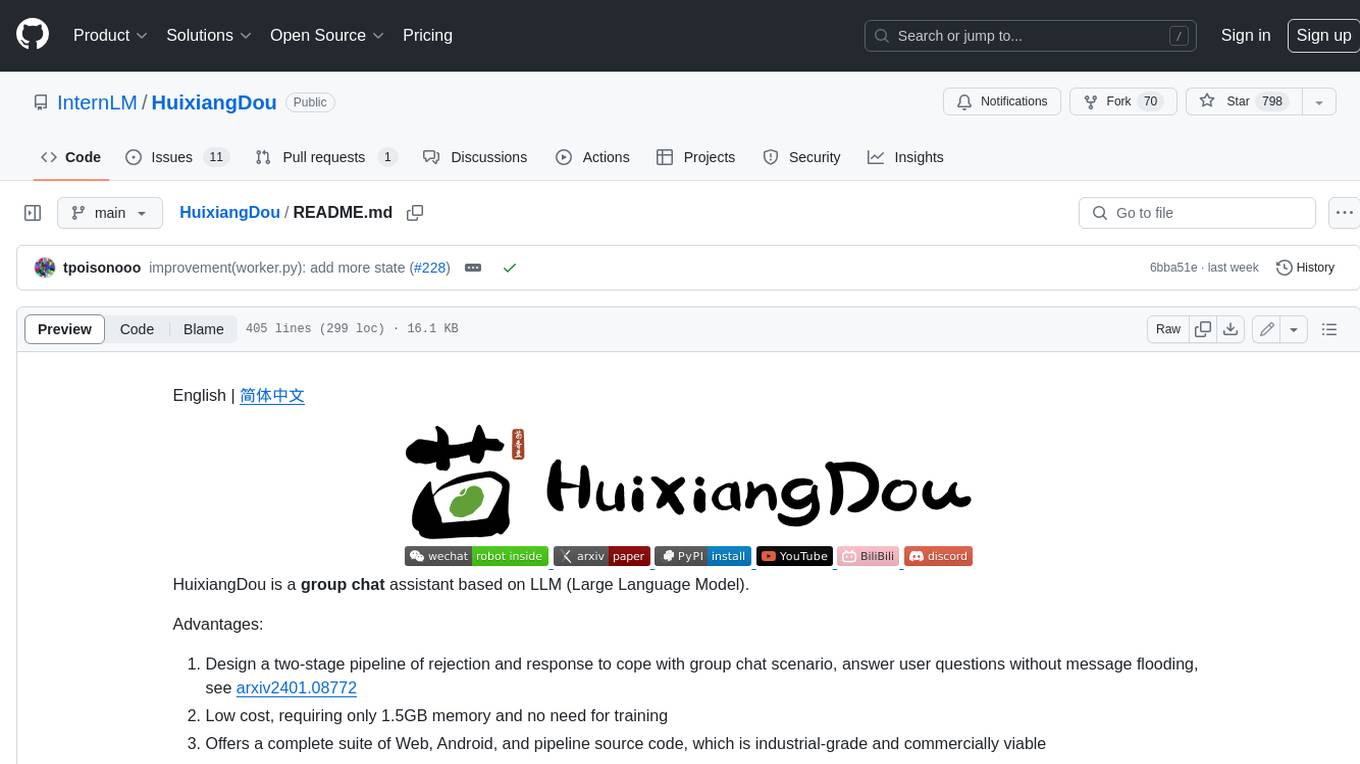
HuixiangDou
HuixiangDou is a **group chat** assistant based on LLM (Large Language Model). Advantages: 1. Design a two-stage pipeline of rejection and response to cope with group chat scenario, answer user questions without message flooding, see arxiv2401.08772 2. Low cost, requiring only 1.5GB memory and no need for training 3. Offers a complete suite of Web, Android, and pipeline source code, which is industrial-grade and commercially viable Check out the scenes in which HuixiangDou are running and join WeChat Group to try AI assistant inside. If this helps you, please give it a star ⭐
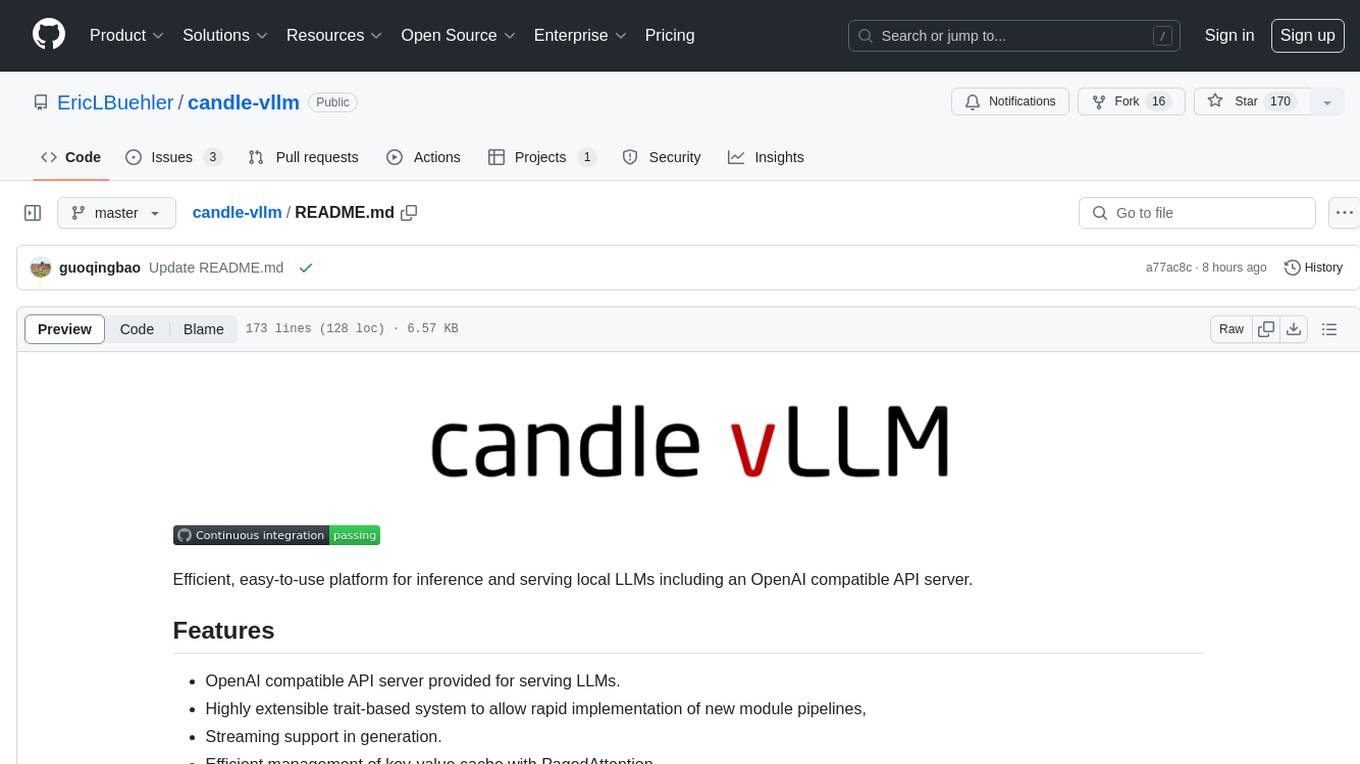
candle-vllm
Candle-vllm is an efficient and easy-to-use platform designed for inference and serving local LLMs, featuring an OpenAI compatible API server. It offers a highly extensible trait-based system for rapid implementation of new module pipelines, streaming support in generation, efficient management of key-value cache with PagedAttention, and continuous batching. The tool supports chat serving for various models and provides a seamless experience for users to interact with LLMs through different interfaces.
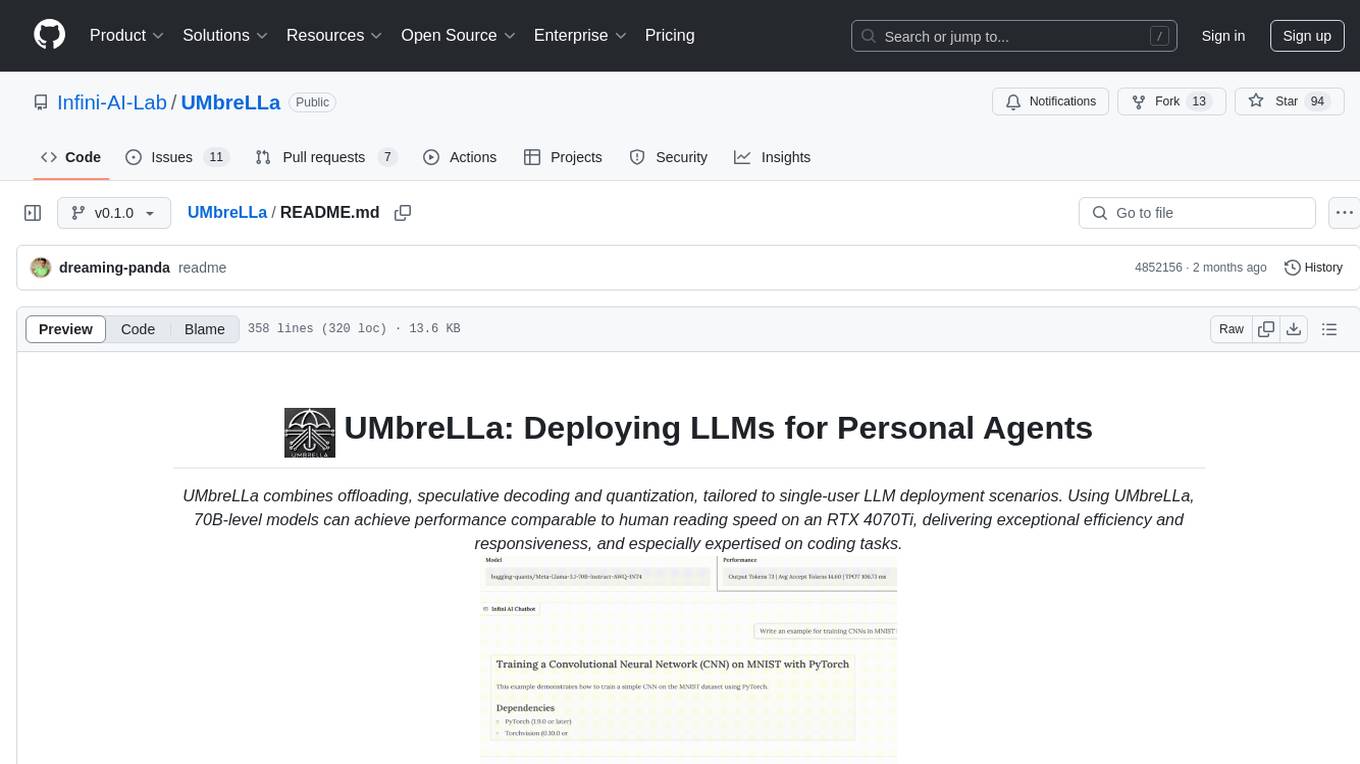
UMbreLLa
UMbreLLa is a tool designed for deploying Large Language Models (LLMs) for personal agents. It combines offloading, speculative decoding, and quantization to optimize single-user LLM deployment scenarios. With UMbreLLa, 70B-level models can achieve performance comparable to human reading speed on an RTX 4070Ti, delivering exceptional efficiency and responsiveness, especially for coding tasks. The tool supports deploying models on various GPUs and offers features like code completion and CLI/Gradio chatbots. Users can configure the LLM engine for optimal performance based on their hardware setup.
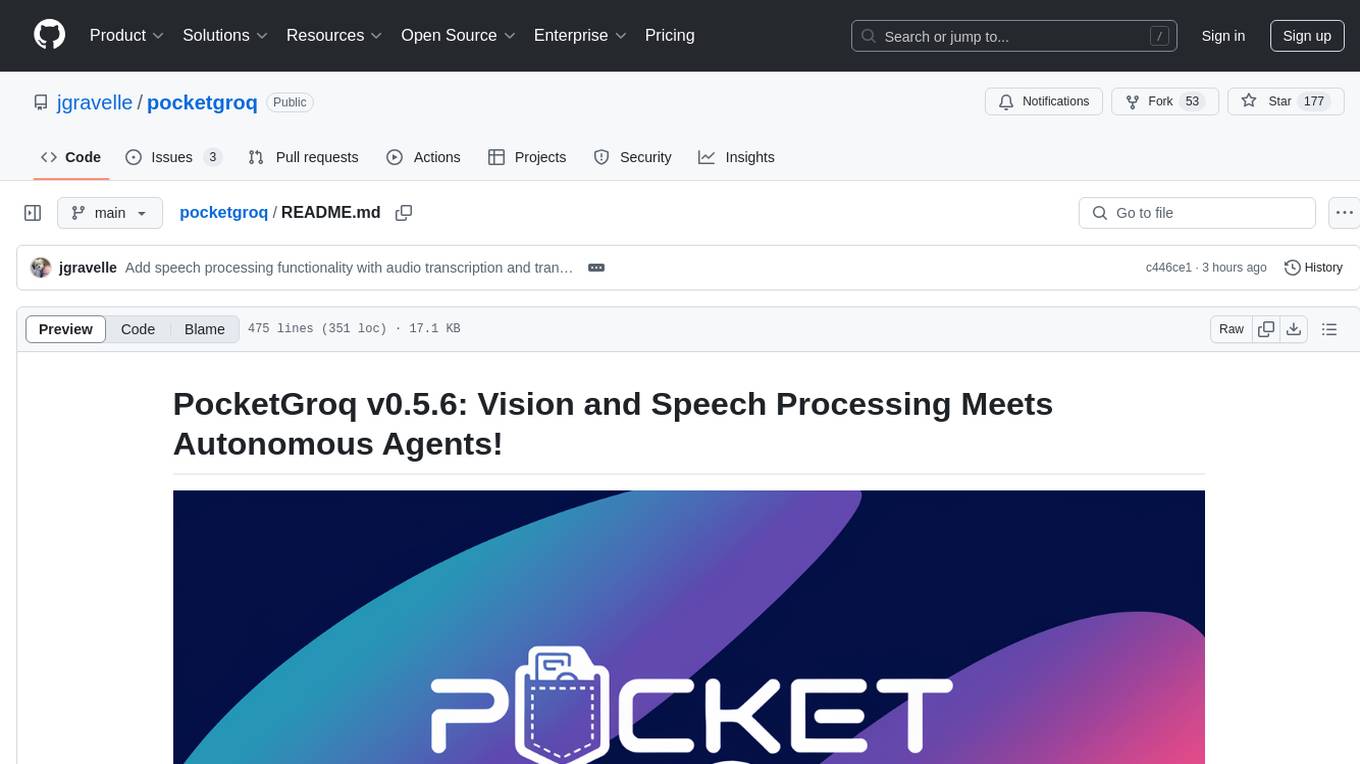
pocketgroq
PocketGroq is a tool that provides advanced functionalities for text generation, web scraping, web search, and AI response evaluation. It includes features like an Autonomous Agent for answering questions, web crawling and scraping capabilities, enhanced web search functionality, and flexible integration with Ollama server. Users can customize the agent's behavior, evaluate responses using AI, and utilize various methods for text generation, conversation management, and Chain of Thought reasoning. The tool offers comprehensive methods for different tasks, such as initializing RAG, error handling, and tool management. PocketGroq is designed to enhance development processes and enable the creation of AI-powered applications with ease.
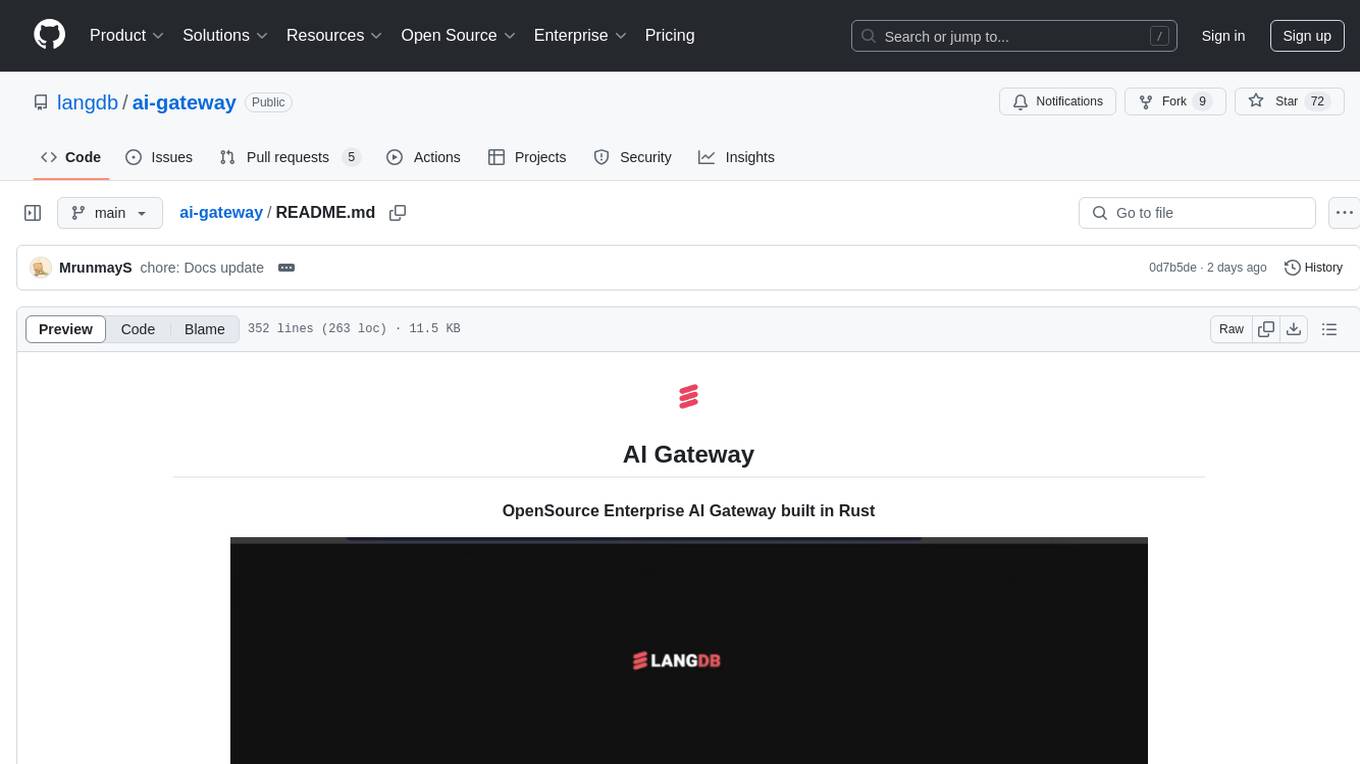
ai-gateway
LangDB AI Gateway is an open-source enterprise AI gateway built in Rust. It provides a unified interface to all LLMs using the OpenAI API format, focusing on high performance, enterprise readiness, and data control. The gateway offers features like comprehensive usage analytics, cost tracking, rate limiting, data ownership, and detailed logging. It supports various LLM providers and provides OpenAI-compatible endpoints for chat completions, model listing, embeddings generation, and image generation. Users can configure advanced settings, such as rate limiting, cost control, dynamic model routing, and observability with OpenTelemetry tracing. The gateway can be run with Docker Compose and integrated with MCP tools for server communication.
For similar tasks
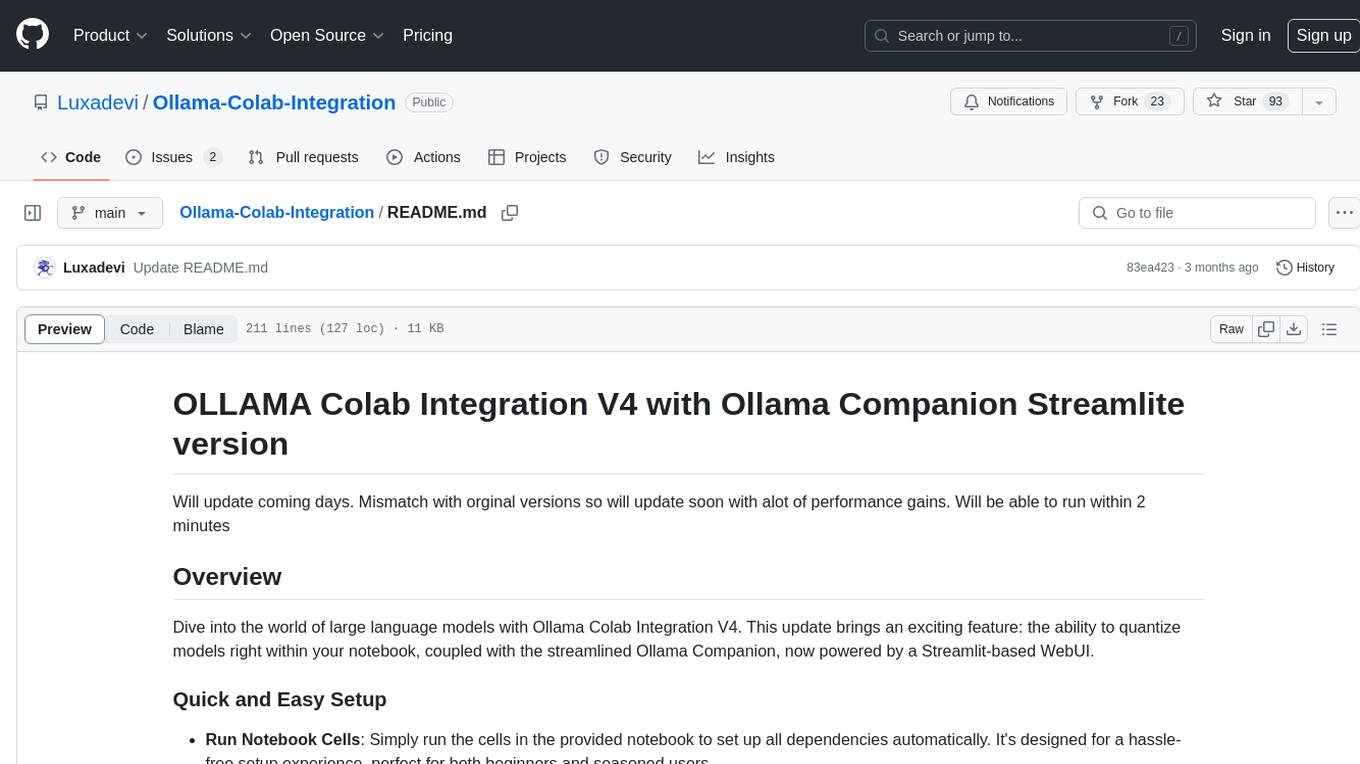
Ollama-Colab-Integration
Ollama Colab Integration V4 is a tool designed to enhance the interaction and management of large language models. It allows users to quantize models within their notebook environment, access a variety of models through a user-friendly interface, and manage public endpoints efficiently. The tool also provides features like LiteLLM proxy control, model insights, and customizable model file templating. Users can troubleshoot model loading issues, CPU fallback strategies, and manage VRAM and RAM effectively. Additionally, the tool offers functionalities for downloading model files from Hugging Face, model conversion with high precision, model quantization using Q and Kquants, and securely uploading converted models to Hugging Face.
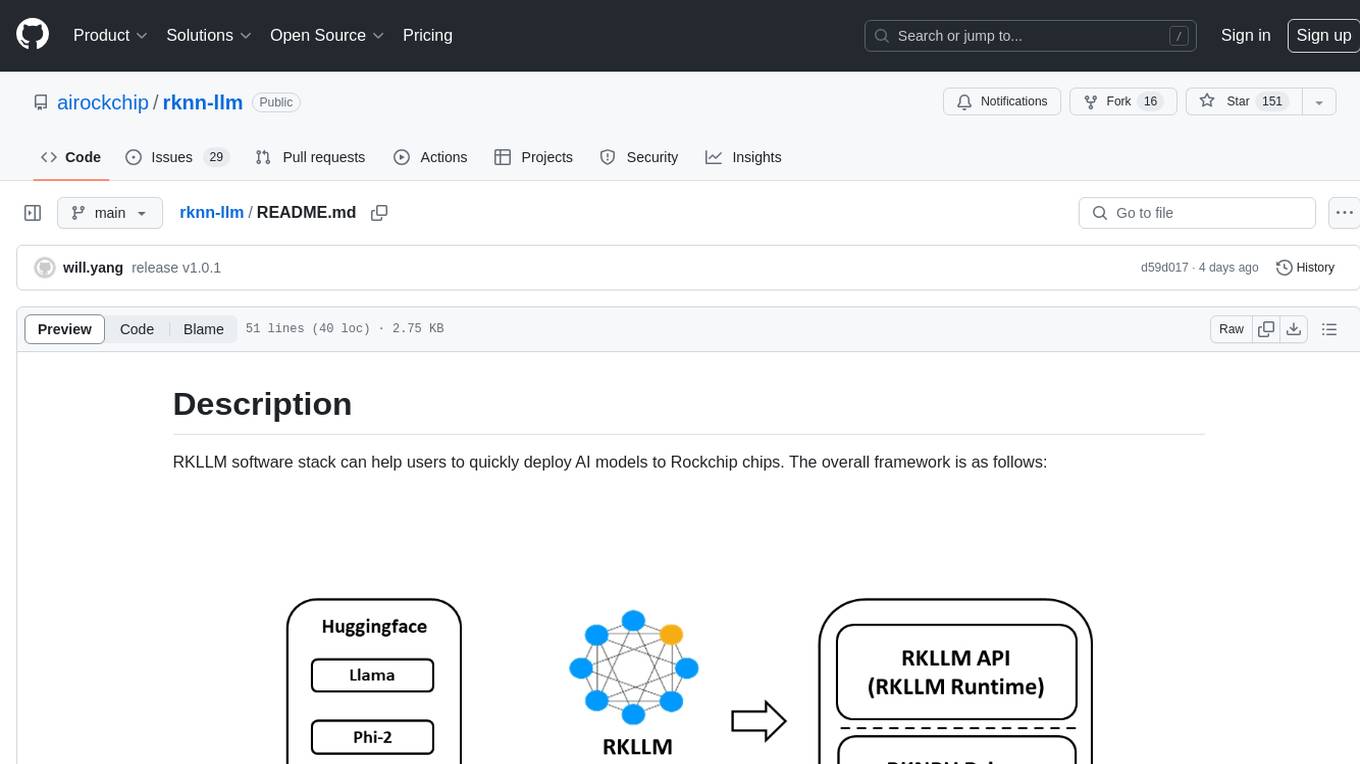
rknn-llm
RKLLM software stack is a toolkit designed to help users quickly deploy AI models to Rockchip chips. It consists of RKLLM-Toolkit for model conversion and quantization, RKLLM Runtime for deploying models on Rockchip NPU platform, and RKNPU kernel driver for hardware interaction. The toolkit supports RK3588 and RK3576 series chips and various models like TinyLLAMA, Qwen, Phi, ChatGLM3, Gemma, InternLM2, and MiniCPM. Users can download packages, docker images, examples, and docs from RKLLM_SDK. Additionally, RKNN-Toolkit2 SDK is available for deploying additional AI models.
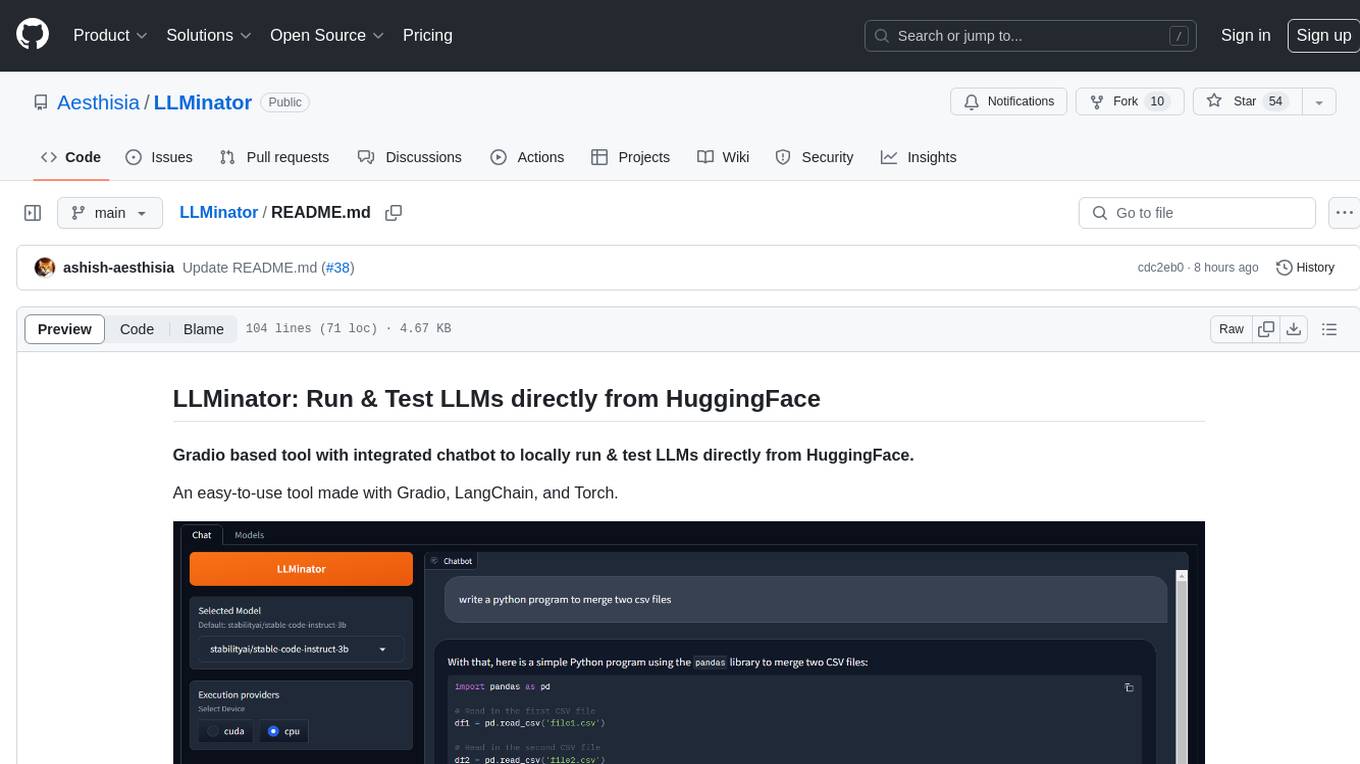
LLMinator
LLMinator is a Gradio-based tool with an integrated chatbot designed to locally run and test Language Model Models (LLMs) directly from HuggingFace. It provides an easy-to-use interface made with Gradio, LangChain, and Torch, offering features such as context-aware streaming chatbot, inbuilt code syntax highlighting, loading any LLM repo from HuggingFace, support for both CPU and CUDA modes, enabling LLM inference with llama.cpp, and model conversion capabilities.
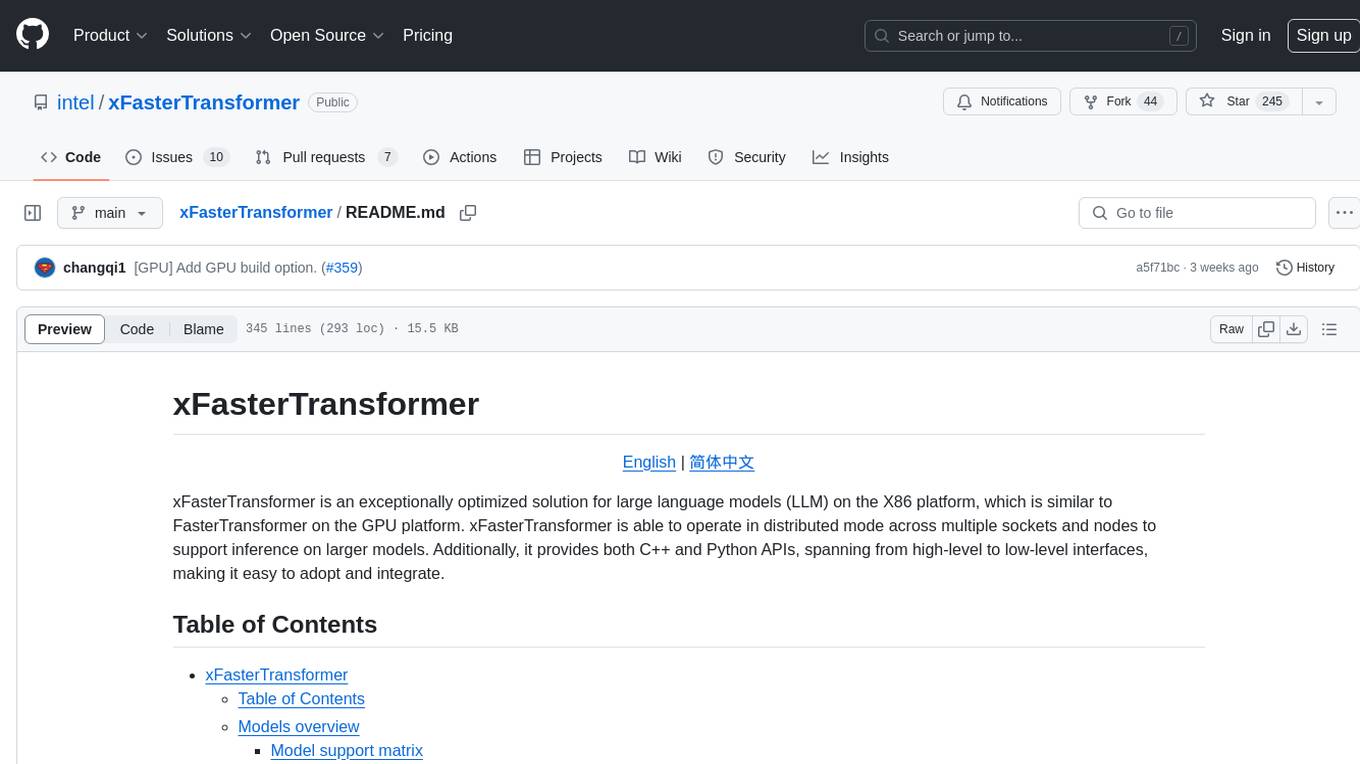
xFasterTransformer
xFasterTransformer is an optimized solution for Large Language Models (LLMs) on the X86 platform, providing high performance and scalability for inference on mainstream LLM models. It offers C++ and Python APIs for easy integration, along with example codes and benchmark scripts. Users can prepare models in a different format, convert them, and use the APIs for tasks like encoding input prompts, generating token ids, and serving inference requests. The tool supports various data types and models, and can run in single or multi-rank modes using MPI. A web demo based on Gradio is available for popular LLM models like ChatGLM and Llama2. Benchmark scripts help evaluate model inference performance quickly, and MLServer enables serving with REST and gRPC interfaces.
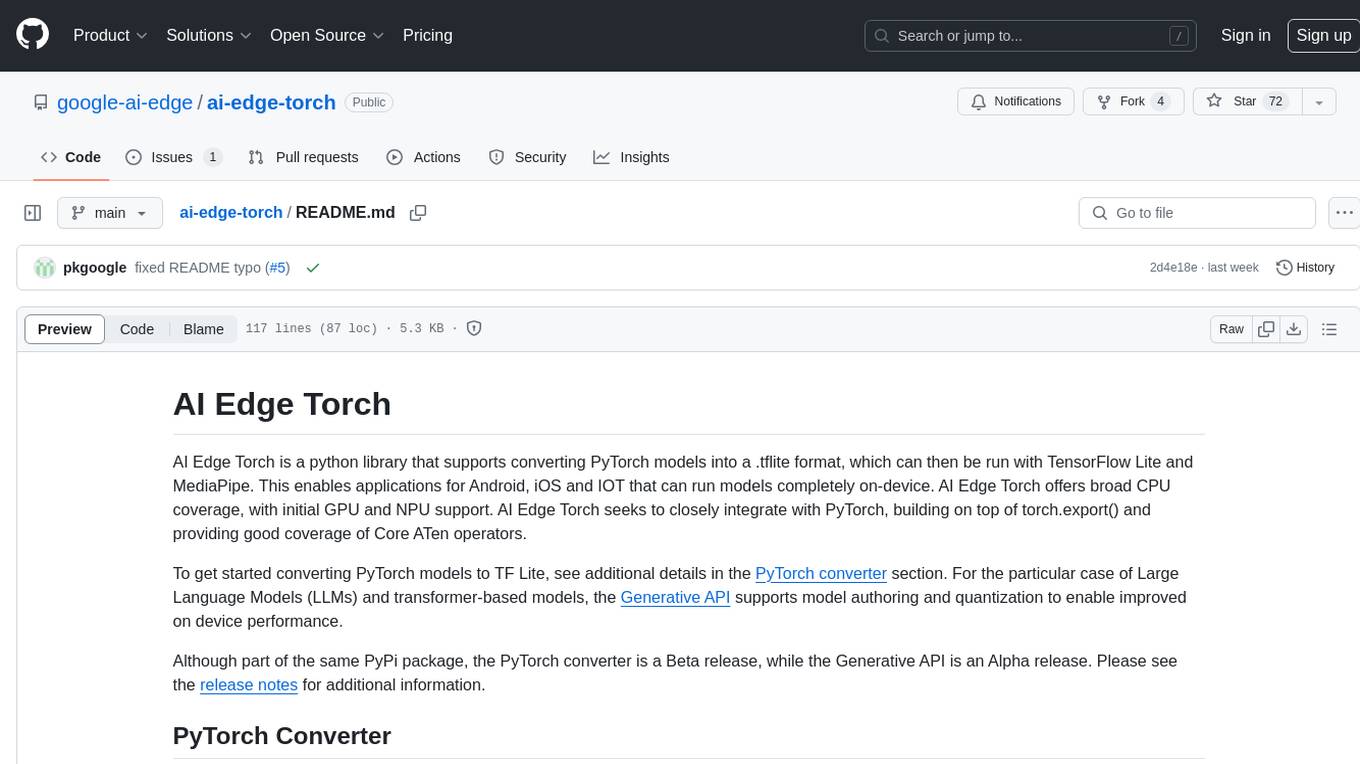
ai-edge-torch
AI Edge Torch is a Python library that supports converting PyTorch models into a .tflite format for on-device applications on Android, iOS, and IoT devices. It offers broad CPU coverage with initial GPU and NPU support, closely integrating with PyTorch and providing good coverage of Core ATen operators. The library includes a PyTorch converter for model conversion and a Generative API for authoring mobile-optimized PyTorch Transformer models, enabling easy deployment of Large Language Models (LLMs) on mobile devices.
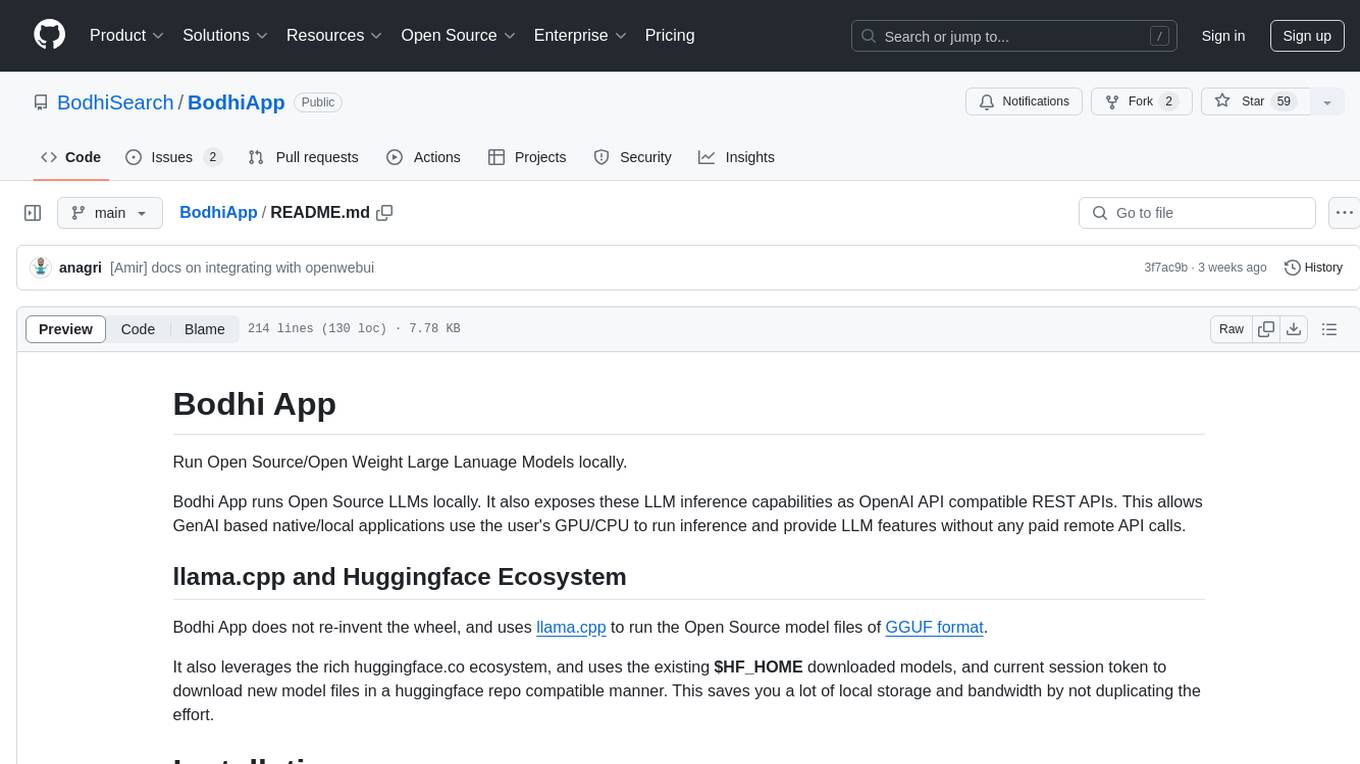
BodhiApp
Bodhi App runs Open Source Large Language Models locally, exposing LLM inference capabilities as OpenAI API compatible REST APIs. It leverages llama.cpp for GGUF format models and huggingface.co ecosystem for model downloads. Users can run fine-tuned models for chat completions, create custom aliases, and convert Huggingface models to GGUF format. The CLI offers commands for environment configuration, model management, pulling files, serving API, and more.
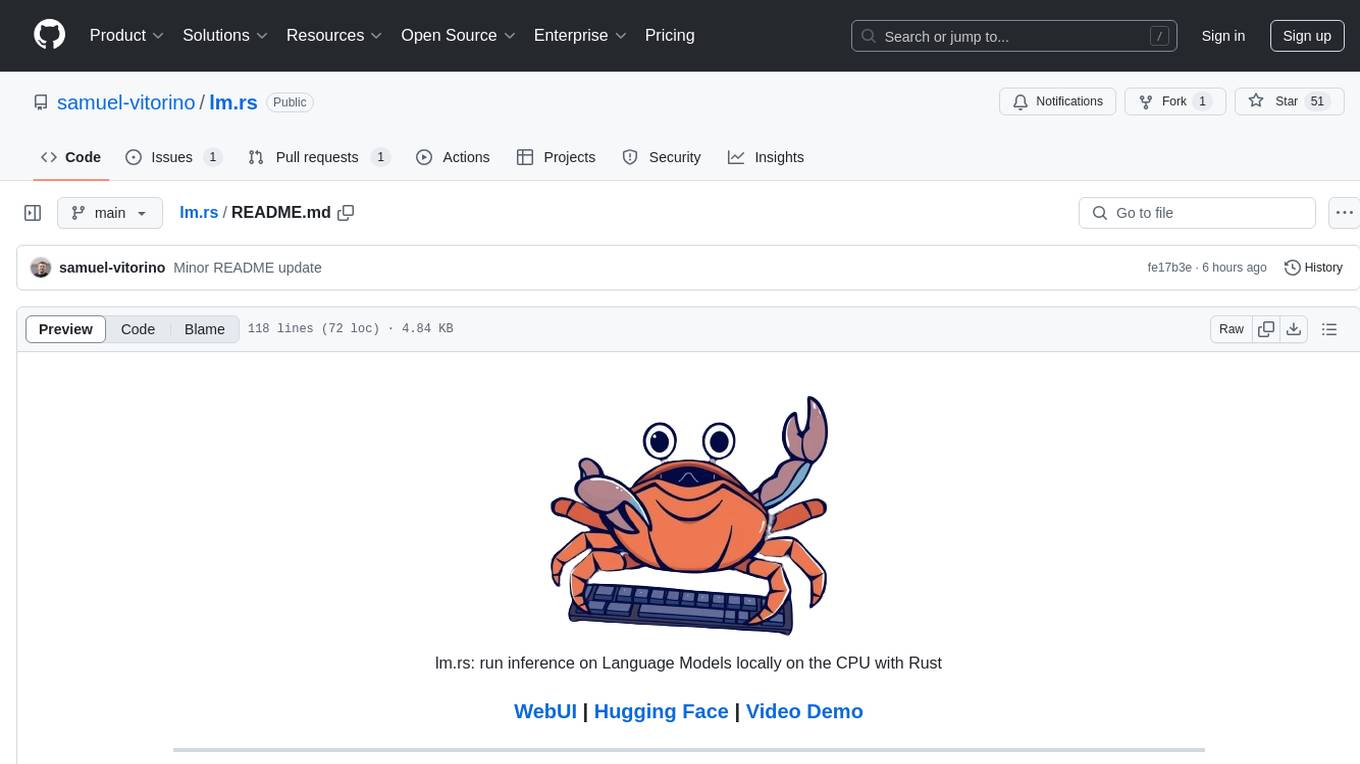
lm.rs
lm.rs is a tool that allows users to run inference on Language Models locally on the CPU using Rust. It supports LLama3.2 1B and 3B models, with a WebUI also available. The tool provides benchmarks and download links for models and tokenizers, with recommendations for quantization options. Users can convert models from Google/Meta on huggingface using provided scripts. The tool can be compiled with cargo and run with various arguments for model weights, tokenizer, temperature, and more. Additionally, a backend for the WebUI can be compiled and run to connect via the web interface.
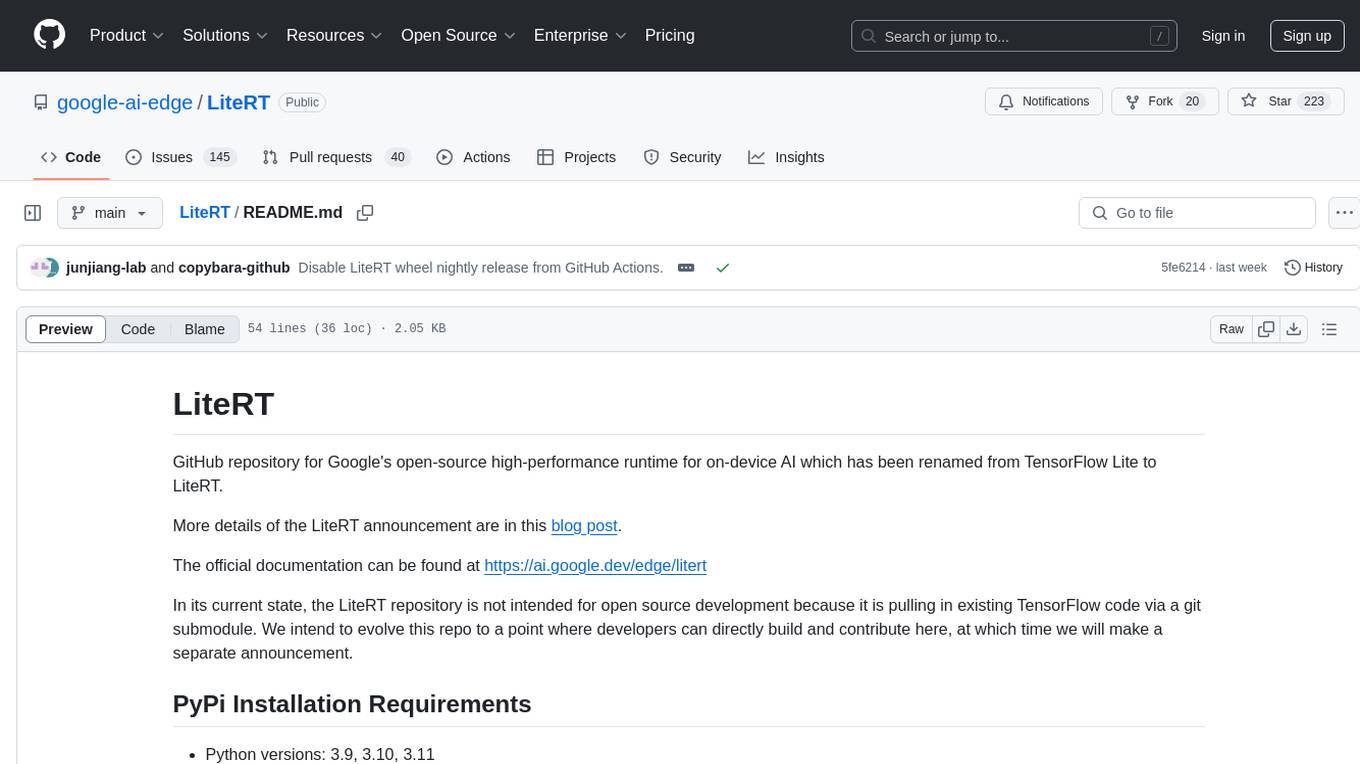
LiteRT
LiteRT is Google's open-source high-performance runtime for on-device AI, previously known as TensorFlow Lite. The repository is currently not intended for open-source development, but aims to evolve to allow direct building and contributions. LiteRT supports Python versions 3.9, 3.10, 3.11 on Linux and MacOS. It ensures compatibility with existing .tflite file extension and format, offering conversion tools and continued active development under the name LiteRT.
For similar jobs
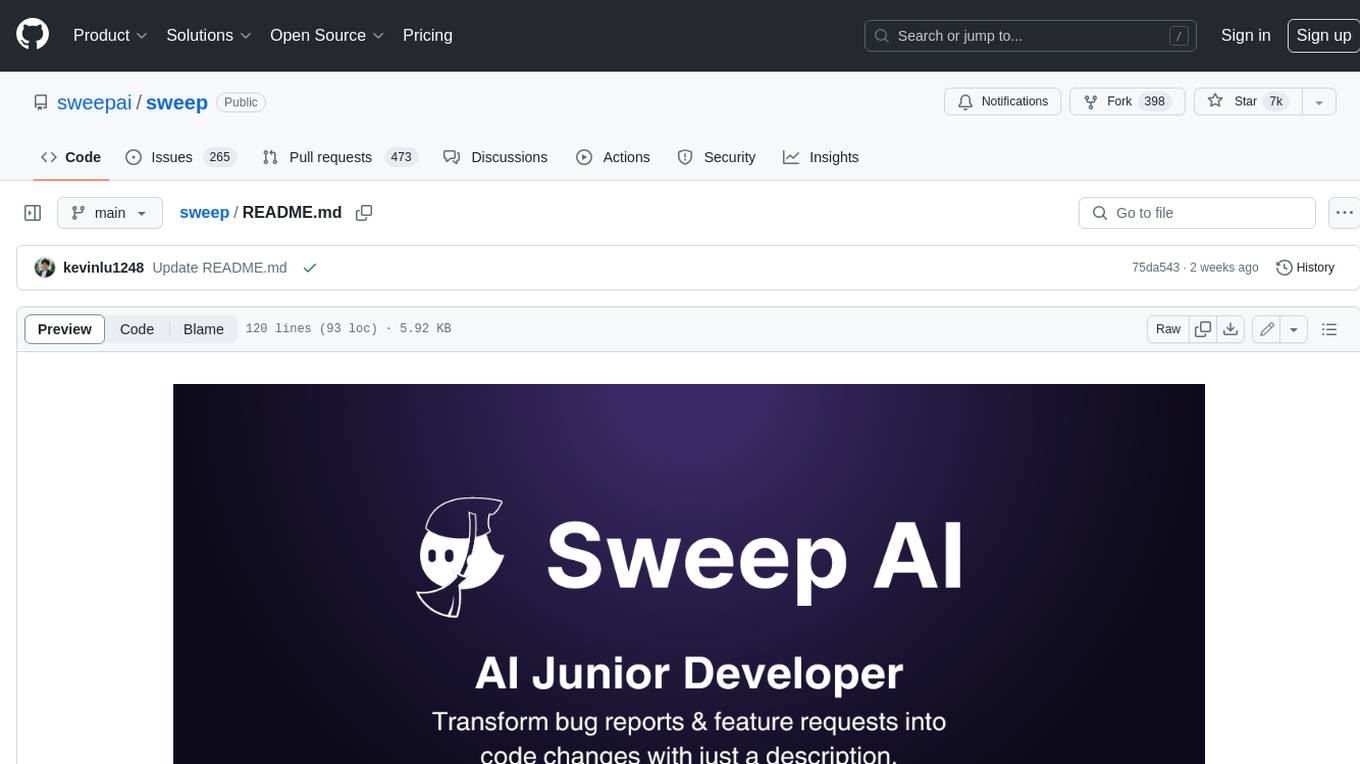
sweep
Sweep is an AI junior developer that turns bugs and feature requests into code changes. It automatically handles developer experience improvements like adding type hints and improving test coverage.
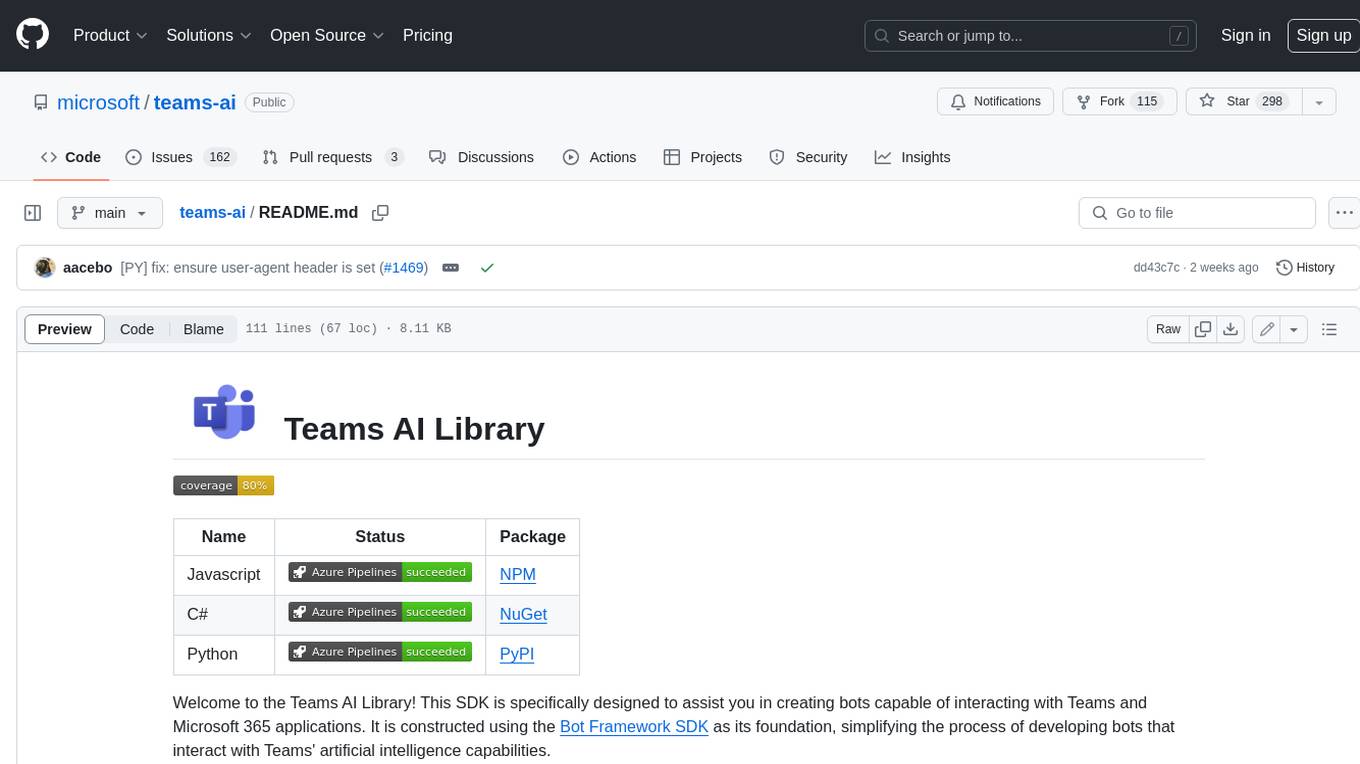
teams-ai
The Teams AI Library is a software development kit (SDK) that helps developers create bots that can interact with Teams and Microsoft 365 applications. It is built on top of the Bot Framework SDK and simplifies the process of developing bots that interact with Teams' artificial intelligence capabilities. The SDK is available for JavaScript/TypeScript, .NET, and Python.
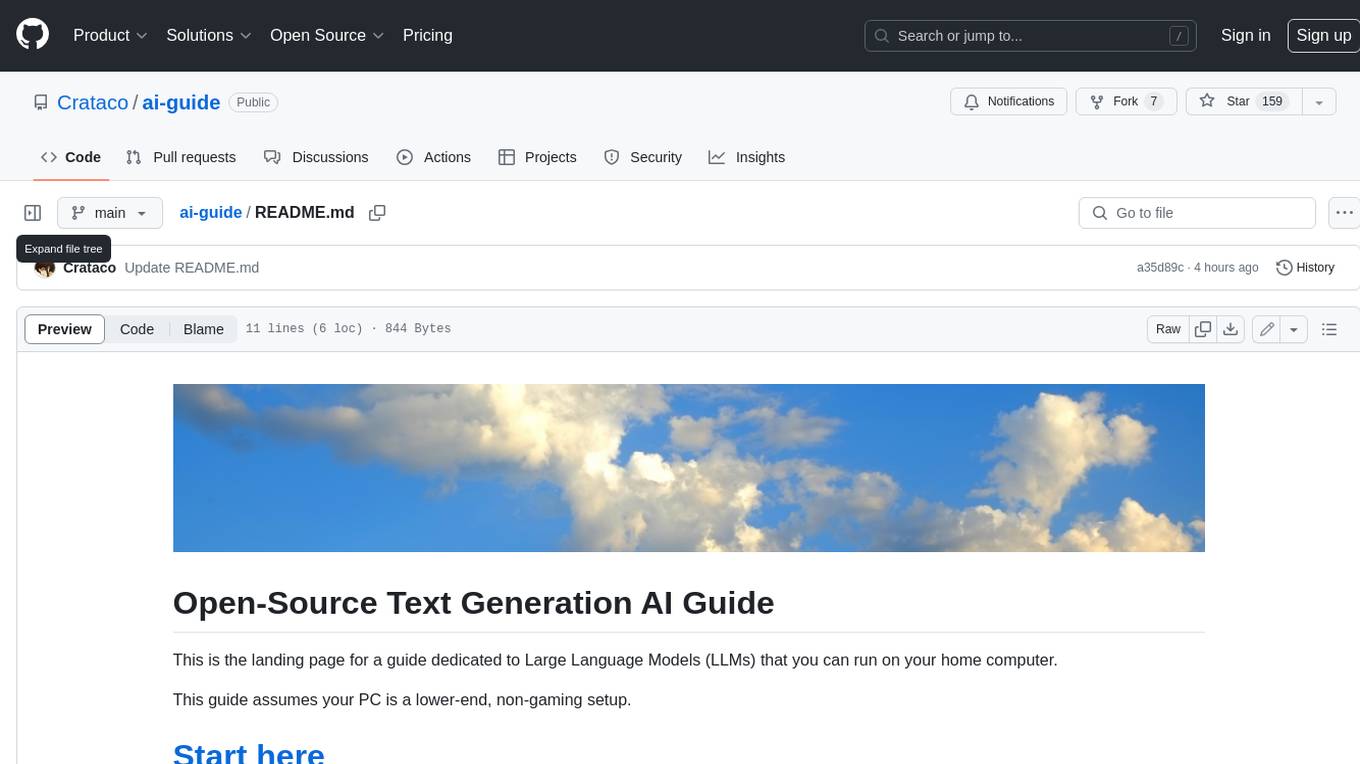
ai-guide
This guide is dedicated to Large Language Models (LLMs) that you can run on your home computer. It assumes your PC is a lower-end, non-gaming setup.
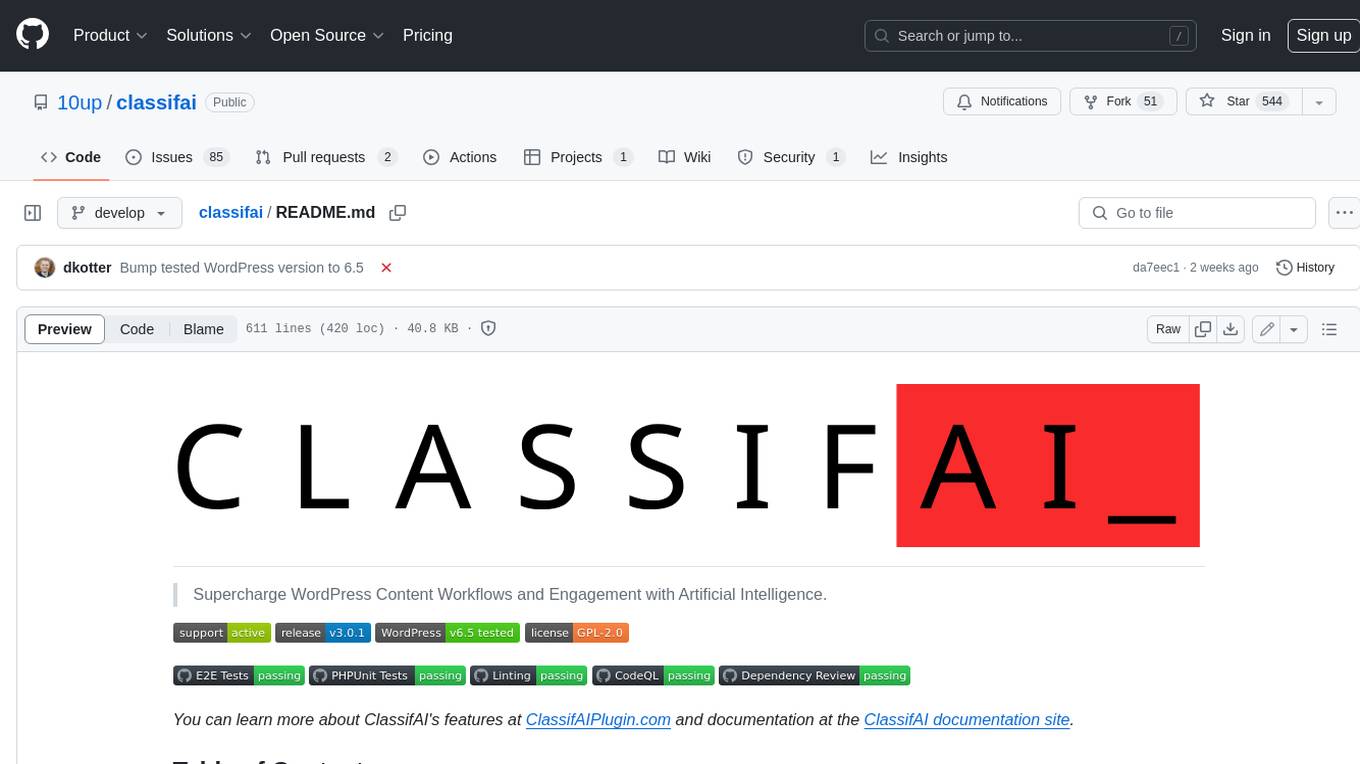
classifai
Supercharge WordPress Content Workflows and Engagement with Artificial Intelligence. Tap into leading cloud-based services like OpenAI, Microsoft Azure AI, Google Gemini and IBM Watson to augment your WordPress-powered websites. Publish content faster while improving SEO performance and increasing audience engagement. ClassifAI integrates Artificial Intelligence and Machine Learning technologies to lighten your workload and eliminate tedious tasks, giving you more time to create original content that matters.
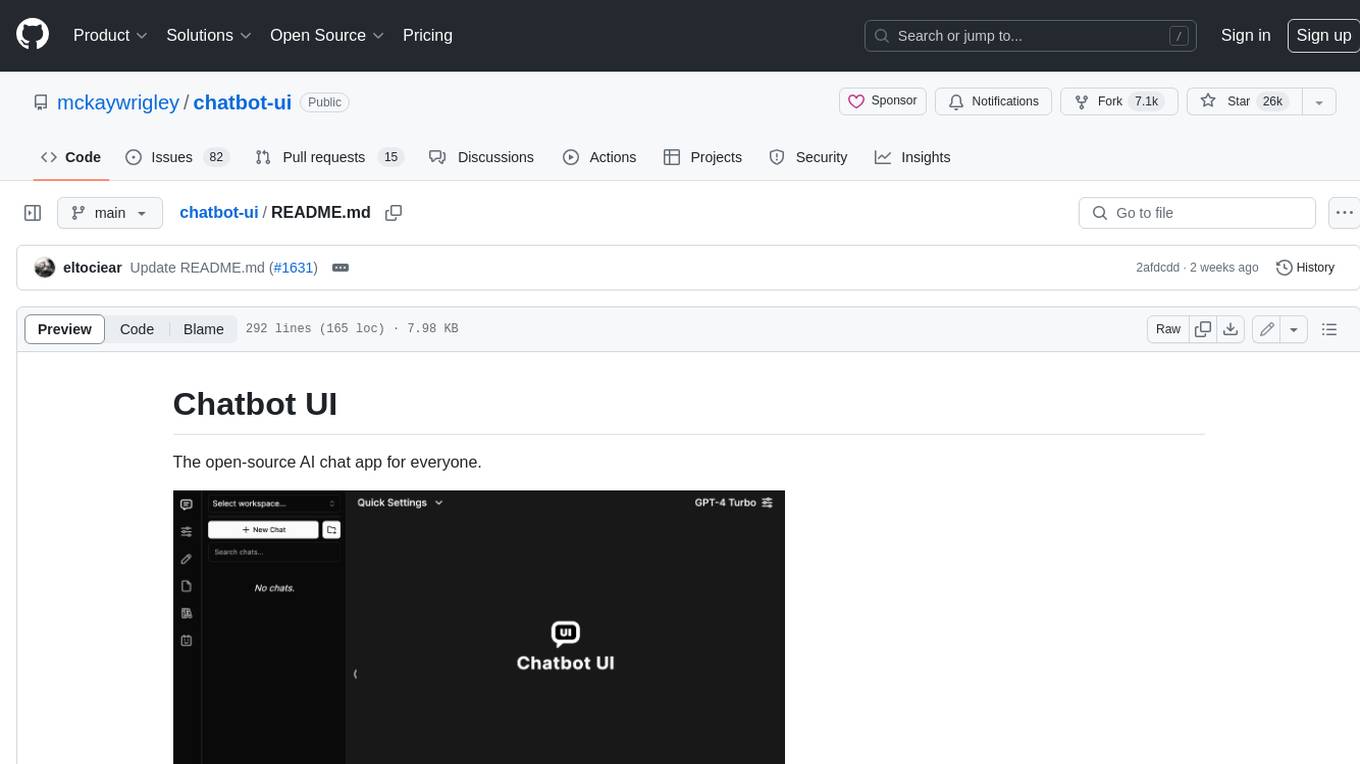
chatbot-ui
Chatbot UI is an open-source AI chat app that allows users to create and deploy their own AI chatbots. It is easy to use and can be customized to fit any need. Chatbot UI is perfect for businesses, developers, and anyone who wants to create a chatbot.
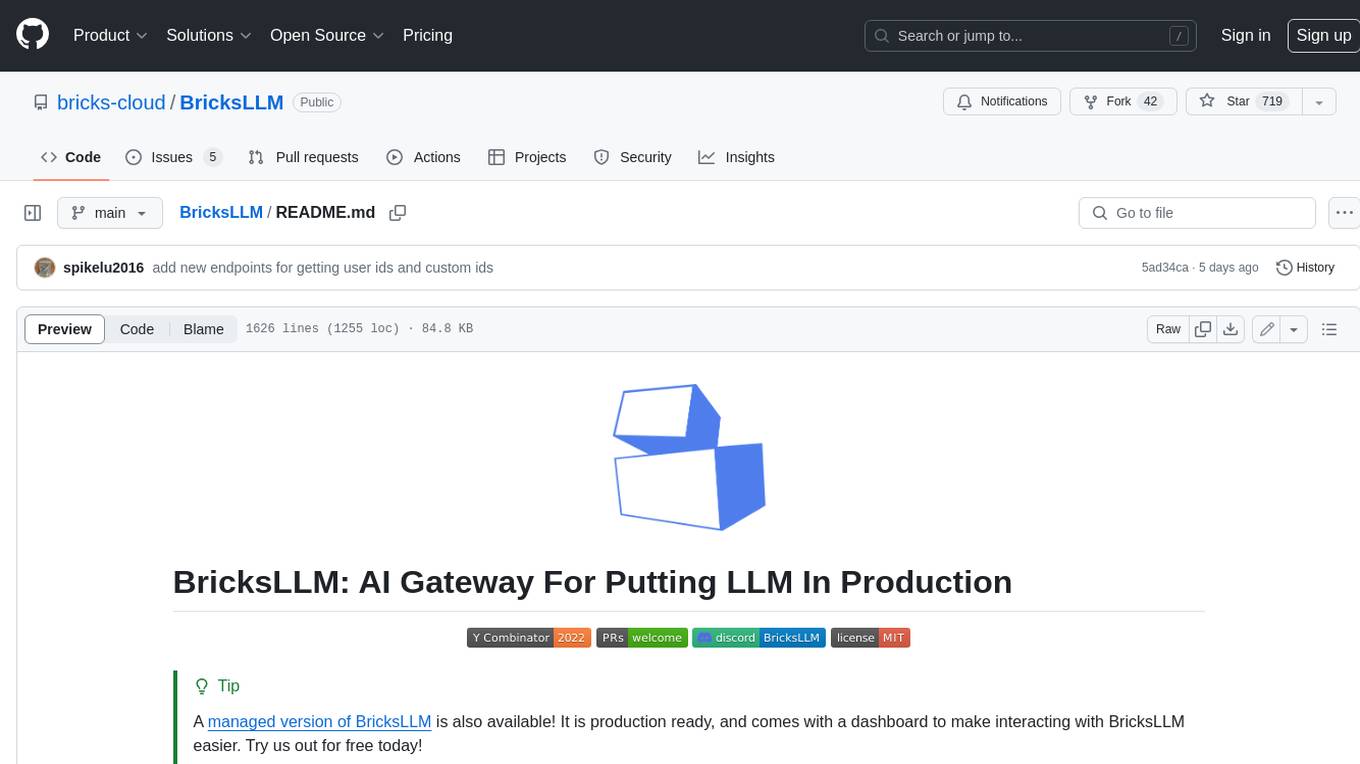
BricksLLM
BricksLLM is a cloud native AI gateway written in Go. Currently, it provides native support for OpenAI, Anthropic, Azure OpenAI and vLLM. BricksLLM aims to provide enterprise level infrastructure that can power any LLM production use cases. Here are some use cases for BricksLLM: * Set LLM usage limits for users on different pricing tiers * Track LLM usage on a per user and per organization basis * Block or redact requests containing PIIs * Improve LLM reliability with failovers, retries and caching * Distribute API keys with rate limits and cost limits for internal development/production use cases * Distribute API keys with rate limits and cost limits for students
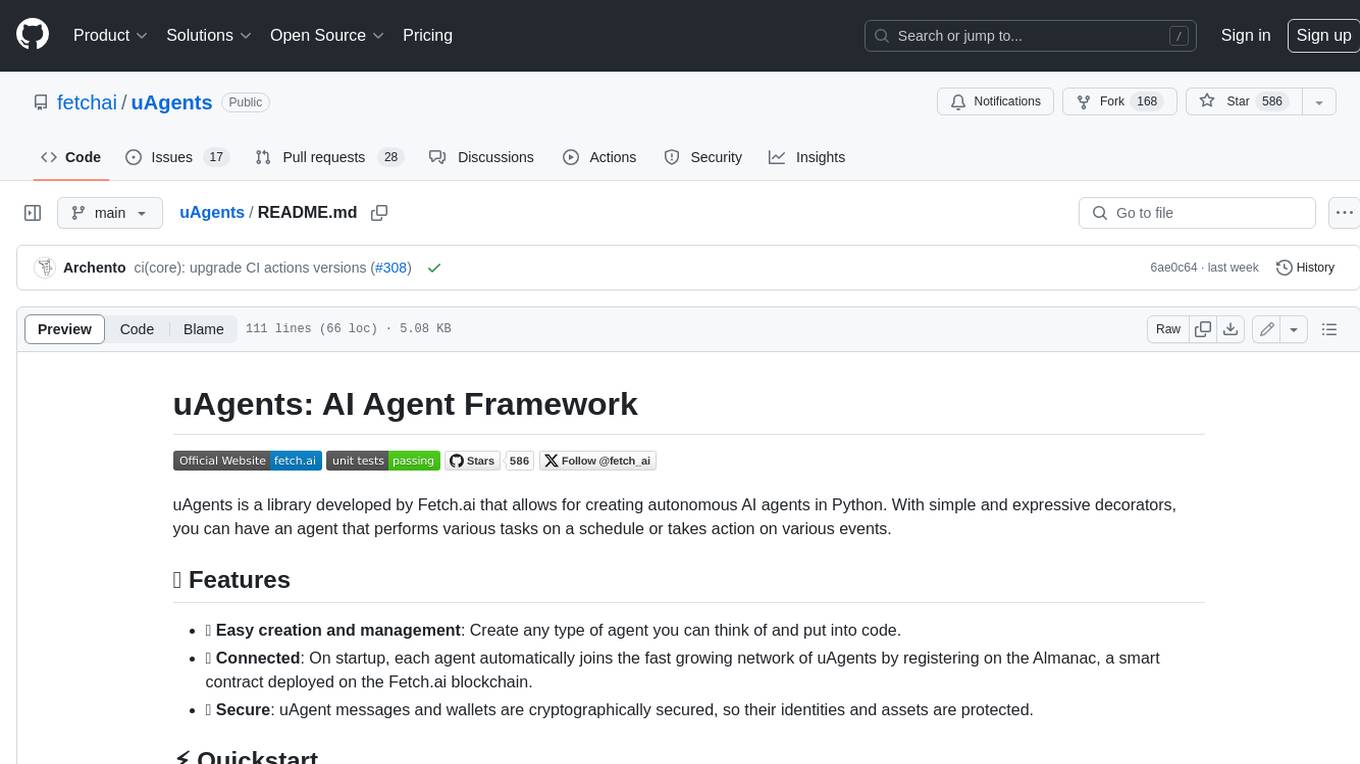
uAgents
uAgents is a Python library developed by Fetch.ai that allows for the creation of autonomous AI agents. These agents can perform various tasks on a schedule or take action on various events. uAgents are easy to create and manage, and they are connected to a fast-growing network of other uAgents. They are also secure, with cryptographically secured messages and wallets.
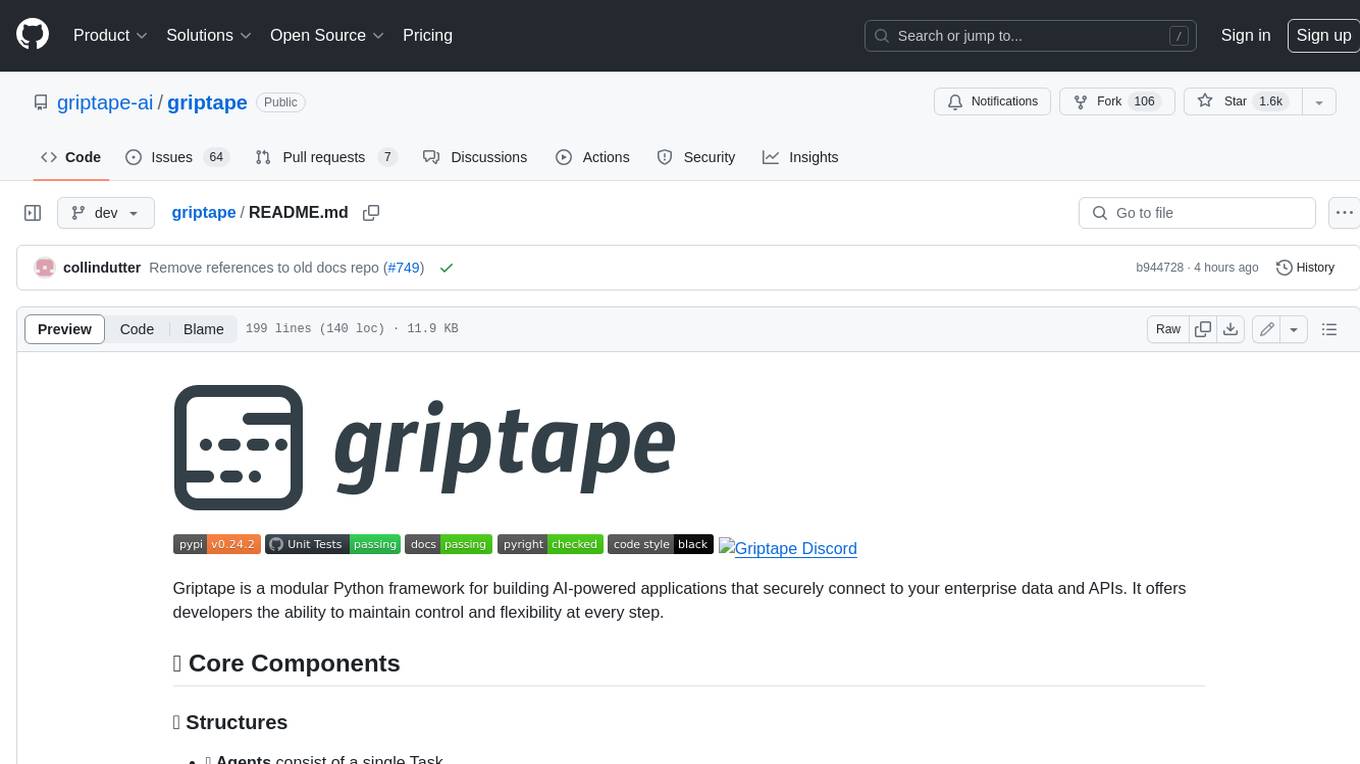
griptape
Griptape is a modular Python framework for building AI-powered applications that securely connect to your enterprise data and APIs. It offers developers the ability to maintain control and flexibility at every step. Griptape's core components include Structures (Agents, Pipelines, and Workflows), Tasks, Tools, Memory (Conversation Memory, Task Memory, and Meta Memory), Drivers (Prompt and Embedding Drivers, Vector Store Drivers, Image Generation Drivers, Image Query Drivers, SQL Drivers, Web Scraper Drivers, and Conversation Memory Drivers), Engines (Query Engines, Extraction Engines, Summary Engines, Image Generation Engines, and Image Query Engines), and additional components (Rulesets, Loaders, Artifacts, Chunkers, and Tokenizers). Griptape enables developers to create AI-powered applications with ease and efficiency.