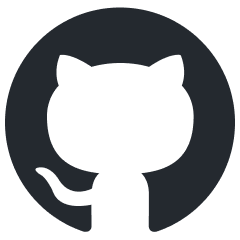
Consistency_LLM
[ICML 2024] CLLMs: Consistency Large Language Models
Stars: 293
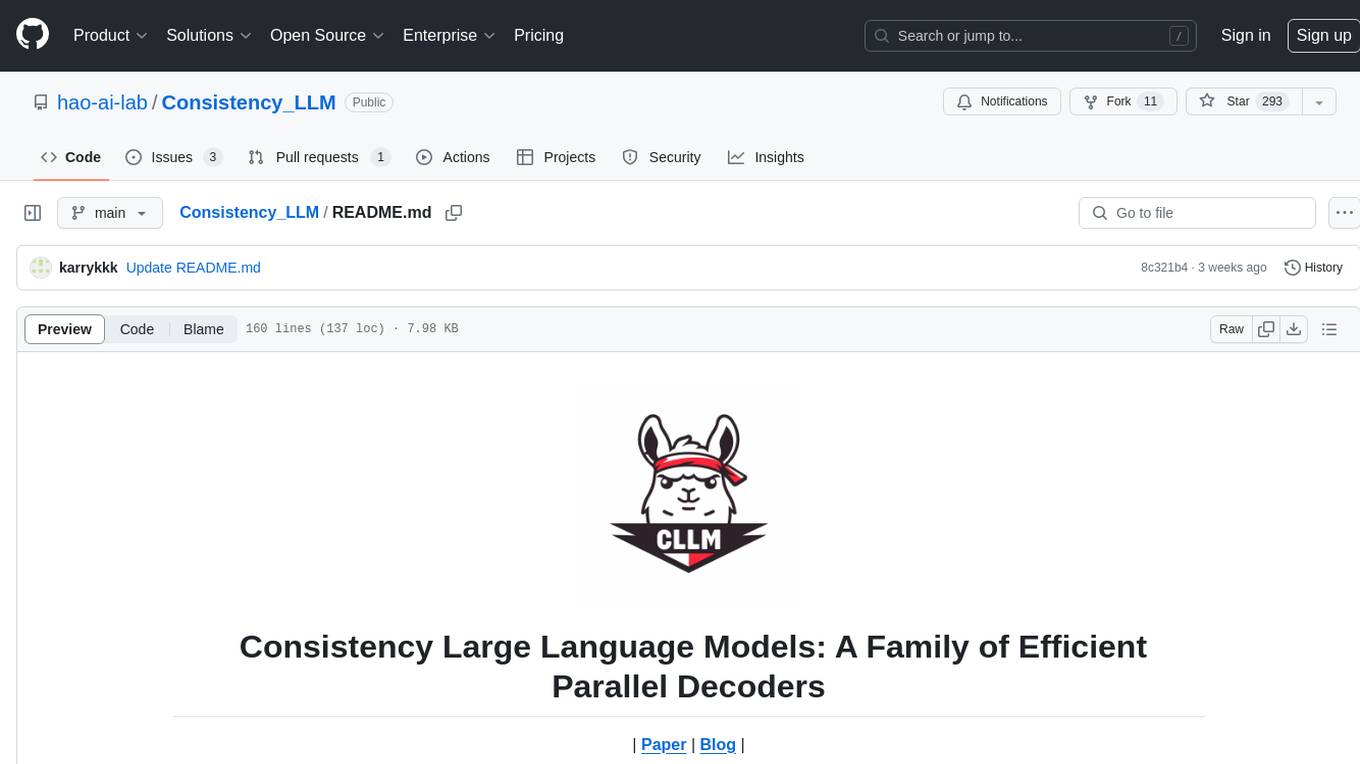
Consistency Large Language Models (CLLMs) is a family of efficient parallel decoders that reduce inference latency by efficiently decoding multiple tokens in parallel. The models are trained to perform efficient Jacobi decoding, mapping any randomly initialized token sequence to the same result as auto-regressive decoding in as few steps as possible. CLLMs have shown significant improvements in generation speed on various tasks, achieving up to 3.4 times faster generation. The tool provides a seamless integration with other techniques for efficient Large Language Model (LLM) inference, without the need for draft models or architectural modifications.
README:
Consistency large language models (CLLMs) is a new family of models capable of reducing inference latency by efficiently decoding $n$ tokens in parallel. This decoding method is called Jacobi decoding, which improves inference efficiency in comparison with conventional auto-regressive (AR) decoding. CLLMs are trained with the objective of performing efficient Jacobi decoding by mapping any randomly initialized $n$-token sequence to the same result as AR decoding in as few steps as possible.
Experiment results have demonstrated the effectiveness of CLLMs, showing $2.4\times$ to $3.4\times$ improvements in generation speed on a variety of tasks.
A demo of using CLLM to achieve significant improvements ($\sim3\times$) in generation speed to solve a basic math problem is shown below:
- [2024/3] CLLMs are integrated in FastChat!
- [2024/2] CLLM Paper now available on arXiv. CLLMs model checkpoints are released on Huggingface Hub.
Consistency Large Language Models (CLLMs) is a family of efficient parallel decoders refined from pre-trained LLMs.
Compared with existing fast decoding techniques, CLLMs achieve fast parallel decoding without the need for:
- Draft models
- Architectural modifications/auxiliary model components
This introduces a number of advantages for CLLMs:
- CLLMs don't have to deal with the complexity of obtaining 'good' draft models and managing two different models in a single system.
- CLLMs share the same architecture with target LLMs and require no additional engineering efforts when adopting the technique to different models.
- CLLMs can be integrated seamlessly with other techniques for efficient LLM inference (e.g. Lookahead Decoding) to achieve even more significant speedup.
- Environment setup:
conda create -n cllm python=3.10
conda activate cllm
- Clone this repository and build from source:
git clone [email protected]:hao-ai-lab/Consistency_LLM.git
cd Consistency_LLM
- Install dependency:
pip install -r requirements.txt
pip install flash-attn==2.4.1
Size | Dataset | Huggingface Repo |
---|---|---|
7B | ShareGPT | cllm/vicuna-7b-sharegpt-gpt4-48k |
7B | GSM8K (Math) | GAIR/Abel-7B-001 |
7B | Spider (Text-to-SQL) | cllm/deepseekcoder-7b-instruct-spider |
7B | Code-Search-Net Python | cllm/deepseekcoder_7b_codesearch_net_python |
Size | Dataset | Huggingface Repo |
---|---|---|
7B | ShareGPT | cllm/consistency-llm-7b-sharegpt48k |
7B | GSM8K (Math) | cllm/consistency-llm-7b-math |
7B | Spider (Text-to-SQL) | cllm/consistency-llm-7b-spider |
7B | Code-Search-Net Python | cllm/consistency-llm-7b-codesearchnet |
bash applications/run_chat_cllm.sh {model_path} {cllm_type}
cllm_type
can take the value of spider
, python
, gsm8k
, sharegpt
.
- Collect Jacobi trajectory:
- Method 1: Directly download Jacobi trajectory to
data/collected_jacobi_trajectory/
from our Huggingface Hub page. - Method 2 (Generate trajectory suitable to your own target model and dataset): Some raw datasets that contain additional information like database dependency or cannot be directly loaded from Huggingface Hub (for example, Spider and ShareGPT are required to be installed in
data/raw_data
). Then runscripts/generate_trajectory.sh
and the training dataset for a CLLM will be saved indata/collected_jacobi_trajectory/
.
For example, for the gsm8k dataset, run:
# max_new_tokens corresponds to the size of n_token_sequence
CUDA_VISIBLE_DEVICES=0 bash scripts/generate_trajectory.sh {filename} {model_path} {n_token_seq_size} {max_new_seq_len}
--filename: path to the raw dataset, currently supporting {data/raw_data/spider, code_search_net, data/raw_data/gsm8k_train.jsonl, data/raw_data/ShareGPT_V3_unfiltered_cleaned_split.json} \
--data_size: maximum number of prompts used to extract Jacobi trajectories \
--use_aug: use data augmentation technique \
--use_labels: add dataset's labels to the output file
- Train a CLLM:
bash scripts/train_cllm.sh {model_path} {trajectory_file} {output_path} {n_token_seq_size}
We follow the same settings in human-eval, Spider, MT-bench and GSM8K evaluate CLLMs' generation quality. An example code to evaluate CLLMs' throughput measured in tokens/s, fast-forwarded token count, stationary token count can be found in eval
folder. Take GSM8K dataset as an example:
To test the speedup, run:
CUDA_VISIBLE_DEVICES=0 bash eval/gsm8k/speedup.sh {model_path} {target_model_path} {max_new_tokens}
To test the accuracy, run:
CUDA_VISIBLE_DEVICES=0 python eval/gsm8k/acc.py --model_dir path_to_cllm --temperature 0.0 --top_p 1.0 --output_file_name 'cllm_generated_gsm8k.jsonl' \
--dev_set "gsm8k" --prompt_type math-single --max_new_tokens_for_consistency 16 --max_tokens 1024 --use_consistency_decoding
This is the official project repository for the following paper. If you find this repository helpful, Please kindly cite:
@misc{kou2024cllms,
title={CLLMs: Consistency Large Language Models},
author={Siqi Kou and Lanxiang Hu and Zhezhi He and Zhijie Deng and Hao Zhang},
year={2024},
eprint={2403.00835},
archivePrefix={arXiv},
primaryClass={cs.CL}
}
For Tasks:
Click tags to check more tools for each tasksFor Jobs:
Alternative AI tools for Consistency_LLM
Similar Open Source Tools
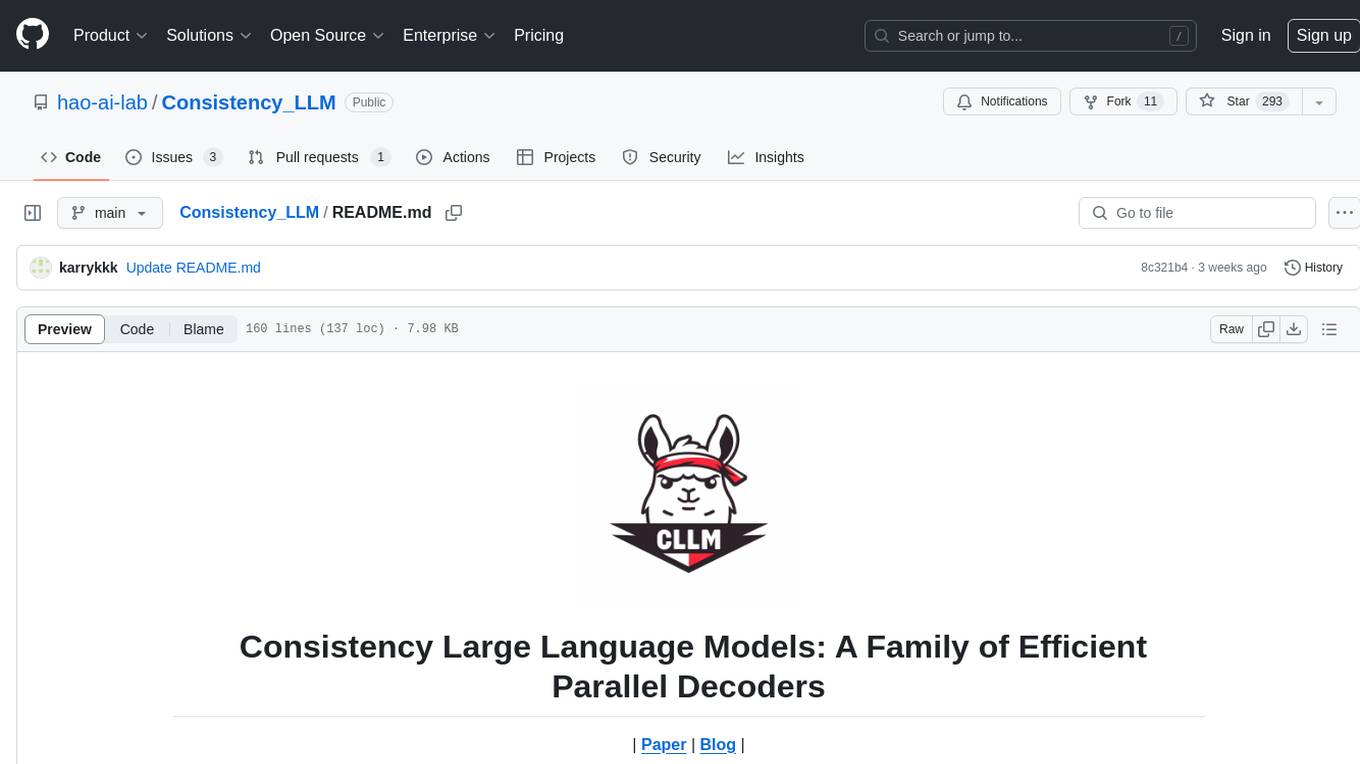
Consistency_LLM
Consistency Large Language Models (CLLMs) is a family of efficient parallel decoders that reduce inference latency by efficiently decoding multiple tokens in parallel. The models are trained to perform efficient Jacobi decoding, mapping any randomly initialized token sequence to the same result as auto-regressive decoding in as few steps as possible. CLLMs have shown significant improvements in generation speed on various tasks, achieving up to 3.4 times faster generation. The tool provides a seamless integration with other techniques for efficient Large Language Model (LLM) inference, without the need for draft models or architectural modifications.
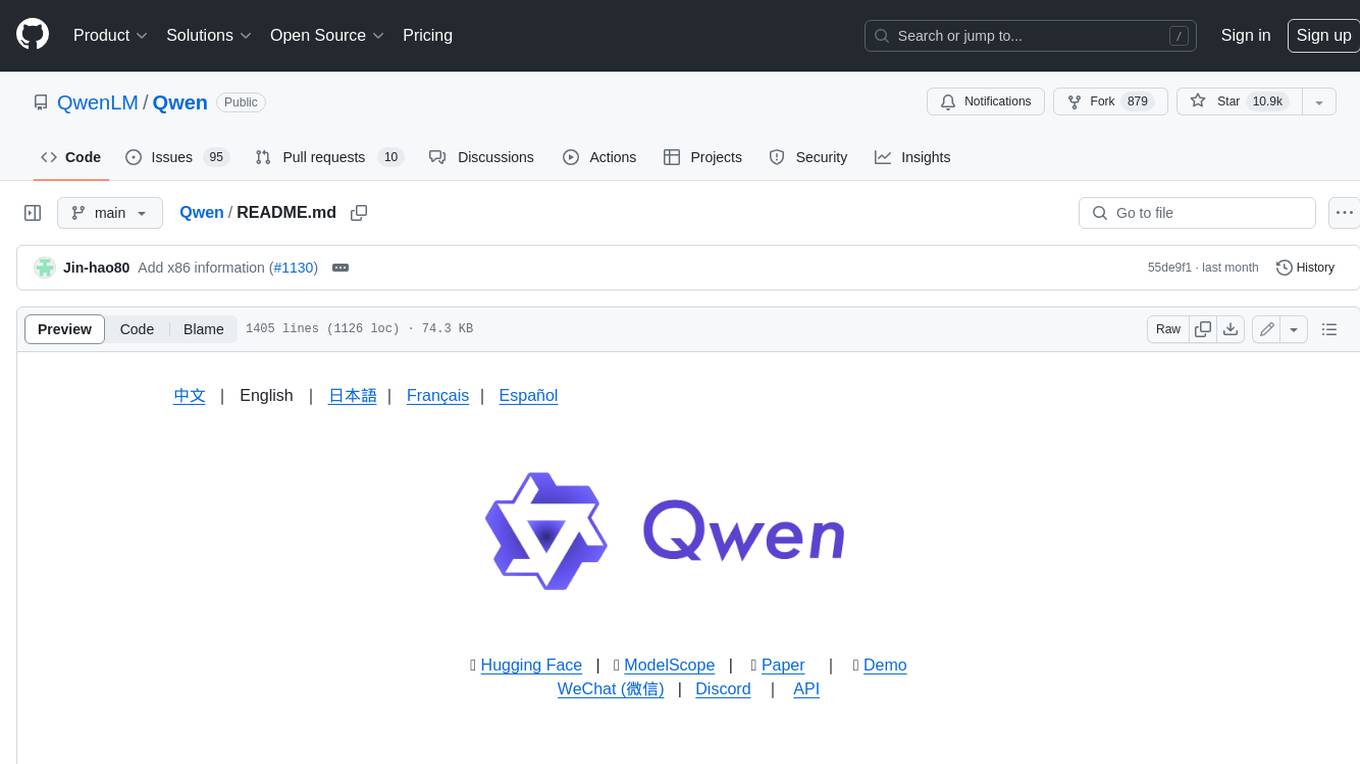
Qwen
Qwen is a series of large language models developed by Alibaba DAMO Academy. It outperforms the baseline models of similar model sizes on a series of benchmark datasets, e.g., MMLU, C-Eval, GSM8K, MATH, HumanEval, MBPP, BBH, etc., which evaluate the models’ capabilities on natural language understanding, mathematic problem solving, coding, etc. Qwen models outperform the baseline models of similar model sizes on a series of benchmark datasets, e.g., MMLU, C-Eval, GSM8K, MATH, HumanEval, MBPP, BBH, etc., which evaluate the models’ capabilities on natural language understanding, mathematic problem solving, coding, etc. Qwen-72B achieves better performance than LLaMA2-70B on all tasks and outperforms GPT-3.5 on 7 out of 10 tasks.
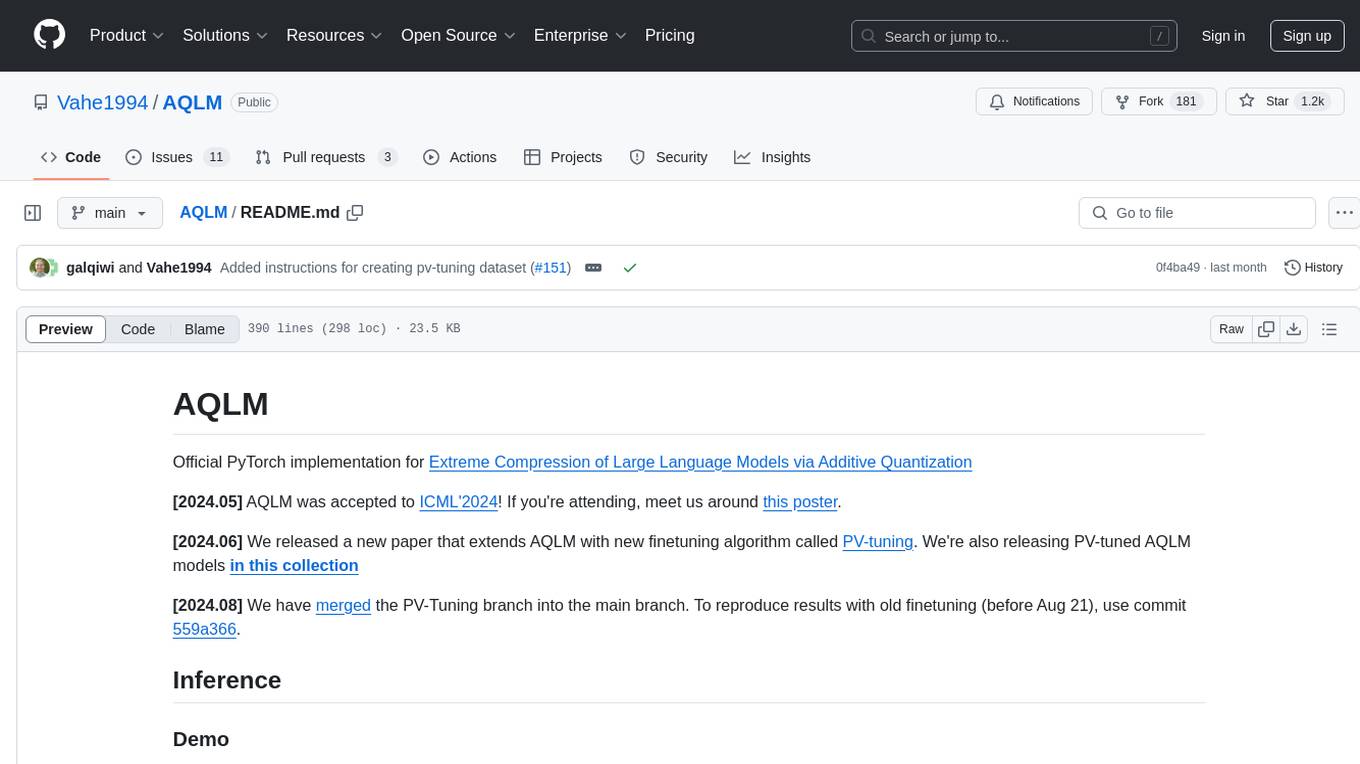
AQLM
AQLM is the official PyTorch implementation for Extreme Compression of Large Language Models via Additive Quantization. It includes prequantized AQLM models without PV-Tuning and PV-Tuned models for LLaMA, Mistral, and Mixtral families. The repository provides inference examples, model details, and quantization setups. Users can run prequantized models using Google Colab examples, work with different model families, and install the necessary inference library. The repository also offers detailed instructions for quantization, fine-tuning, and model evaluation. AQLM quantization involves calibrating models for compression, and users can improve model accuracy through finetuning. Additionally, the repository includes information on preparing models for inference and contributing guidelines.
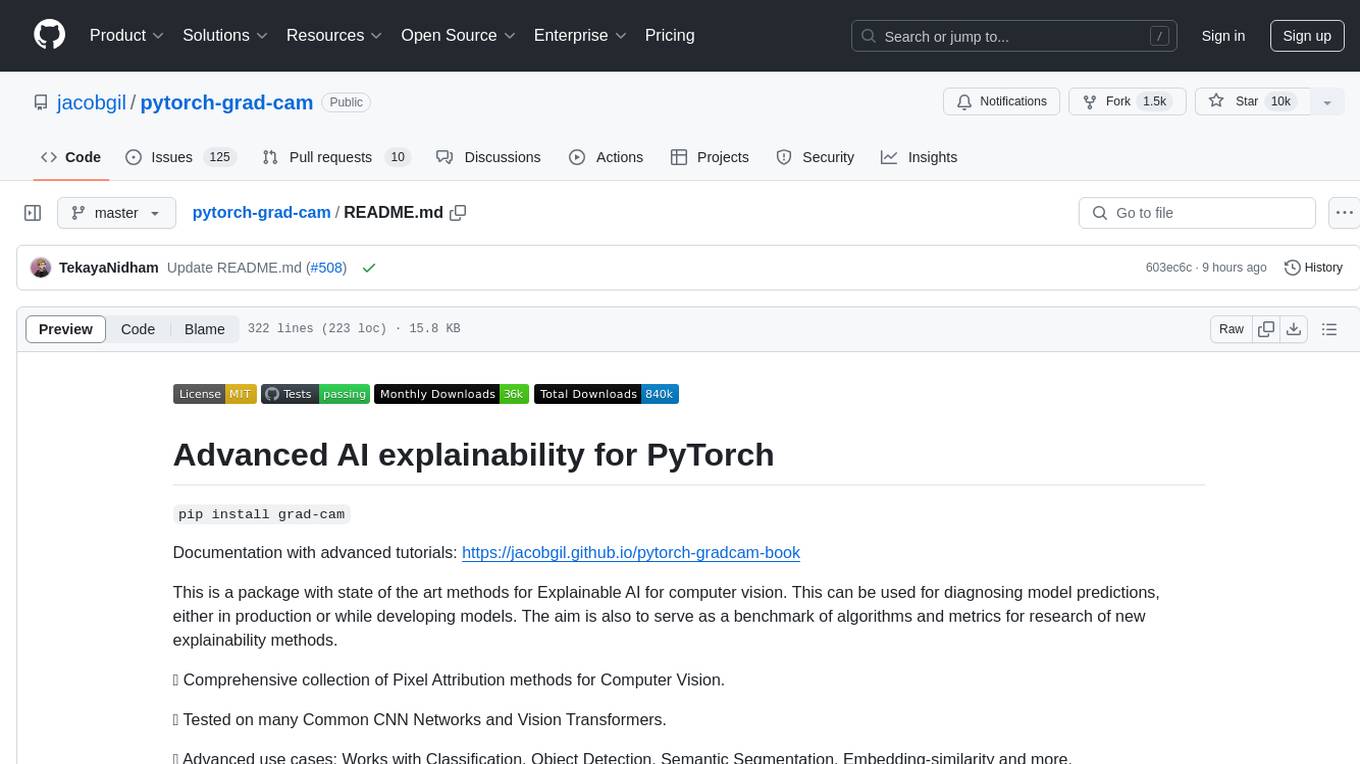
pytorch-grad-cam
This repository provides advanced AI explainability for PyTorch, offering state-of-the-art methods for Explainable AI in computer vision. It includes a comprehensive collection of Pixel Attribution methods for various tasks like Classification, Object Detection, Semantic Segmentation, and more. The package supports high performance with full batch image support and includes metrics for evaluating and tuning explanations. Users can visualize and interpret model predictions, making it suitable for both production and model development scenarios.
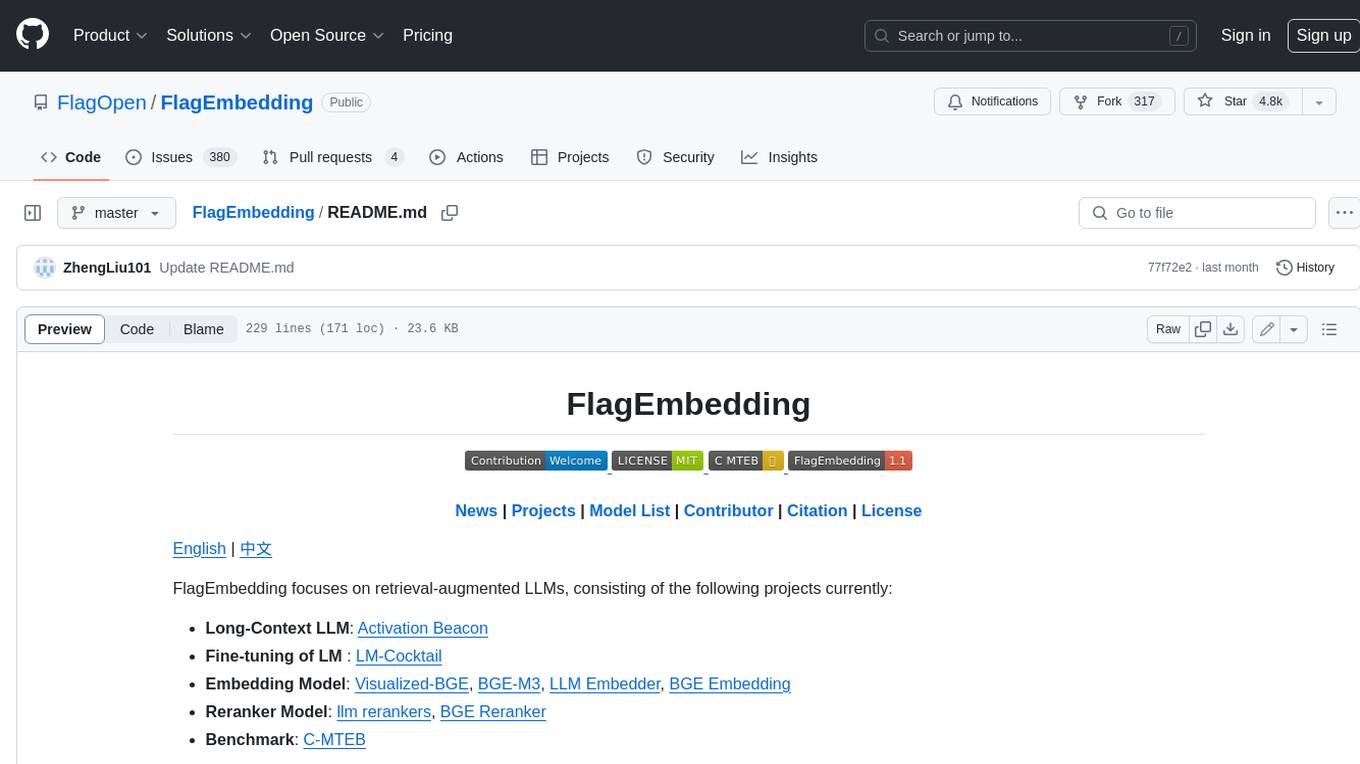
FlagEmbedding
FlagEmbedding focuses on retrieval-augmented LLMs, consisting of the following projects currently: * **Long-Context LLM** : Activation Beacon * **Fine-tuning of LM** : LM-Cocktail * **Embedding Model** : Visualized-BGE, BGE-M3, LLM Embedder, BGE Embedding * **Reranker Model** : llm rerankers, BGE Reranker * **Benchmark** : C-MTEB
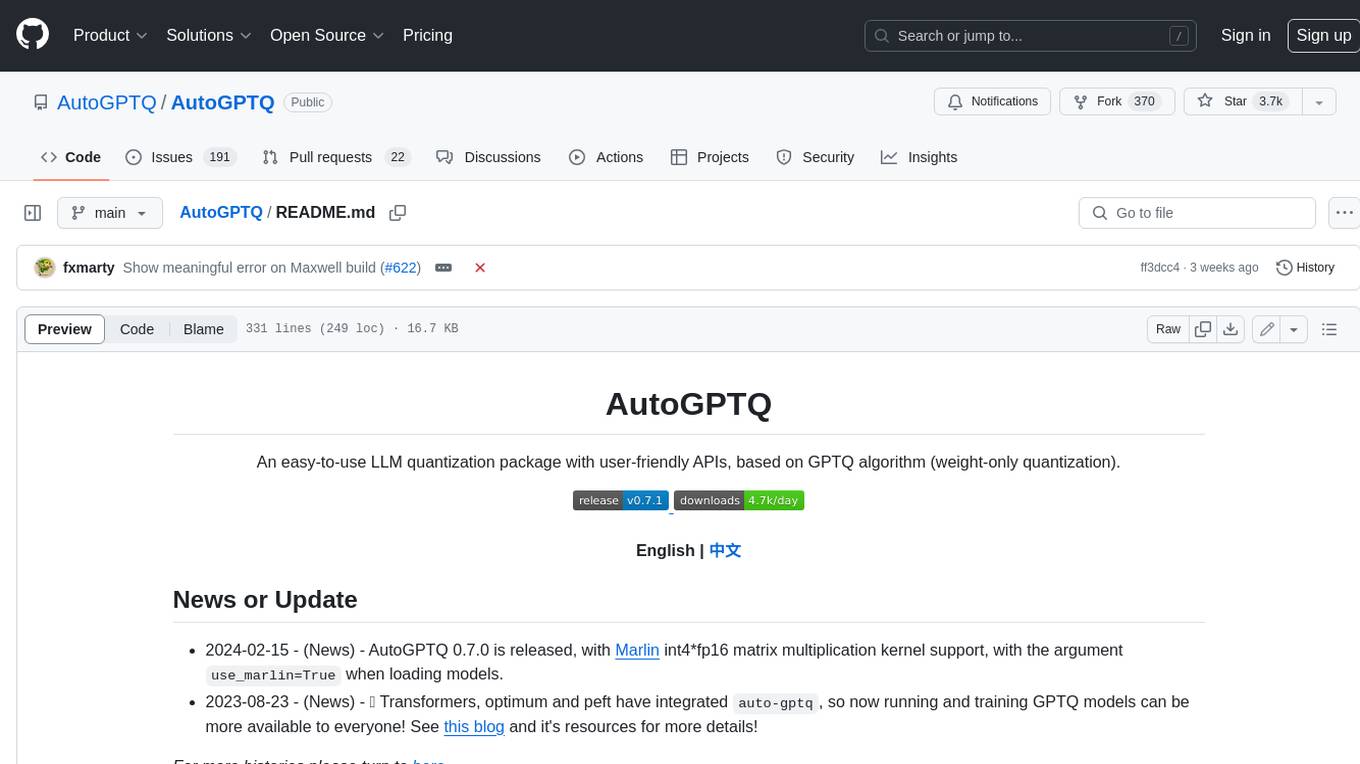
AutoGPTQ
AutoGPTQ is an easy-to-use LLM quantization package with user-friendly APIs, based on GPTQ algorithm (weight-only quantization). It provides a simple and efficient way to quantize large language models (LLMs) to reduce their size and computational cost while maintaining their performance. AutoGPTQ supports a wide range of LLM models, including GPT-2, GPT-J, OPT, and BLOOM. It also supports various evaluation tasks, such as language modeling, sequence classification, and text summarization. With AutoGPTQ, users can easily quantize their LLM models and deploy them on resource-constrained devices, such as mobile phones and embedded systems.
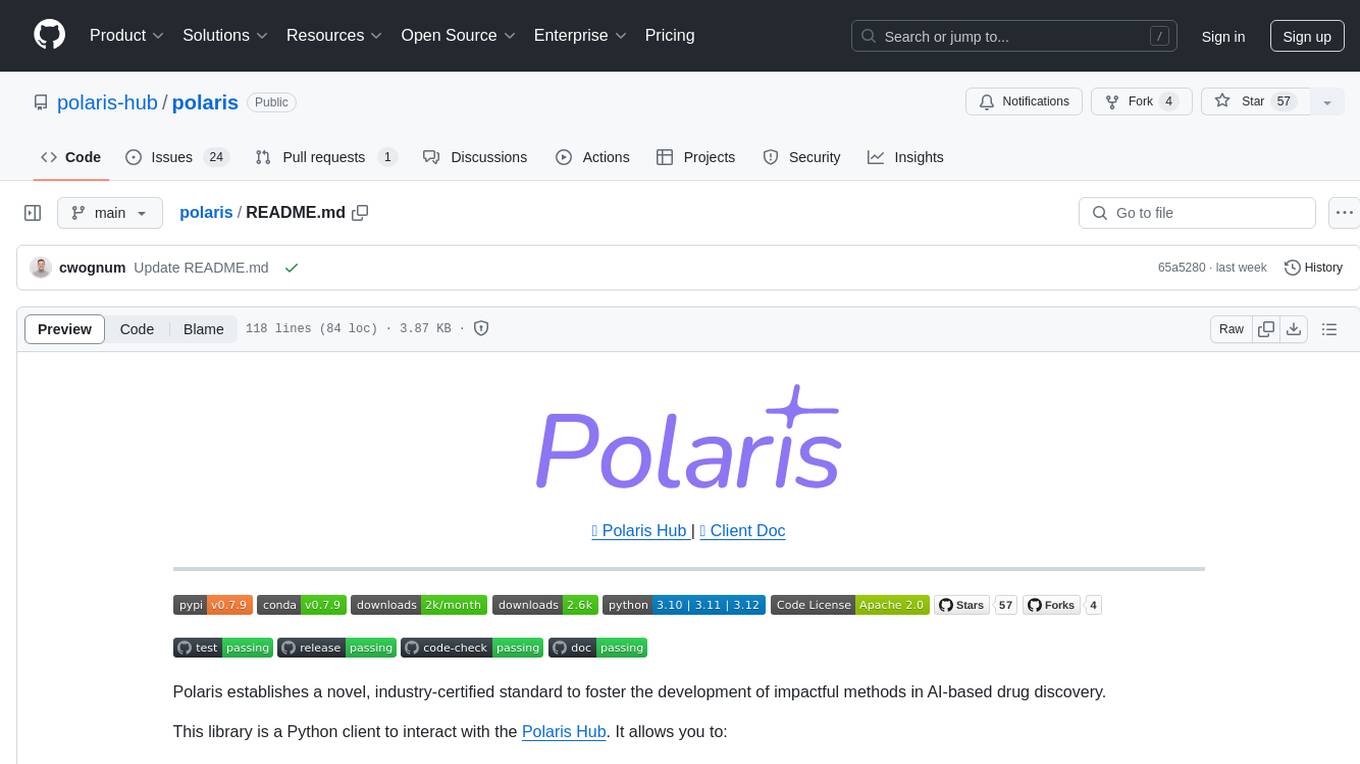
polaris
Polaris establishes a novel, industry‑certified standard to foster the development of impactful methods in AI-based drug discovery. This library is a Python client to interact with the Polaris Hub. It allows you to download Polaris datasets and benchmarks, evaluate a custom method against a Polaris benchmark, and create and upload new datasets and benchmarks.
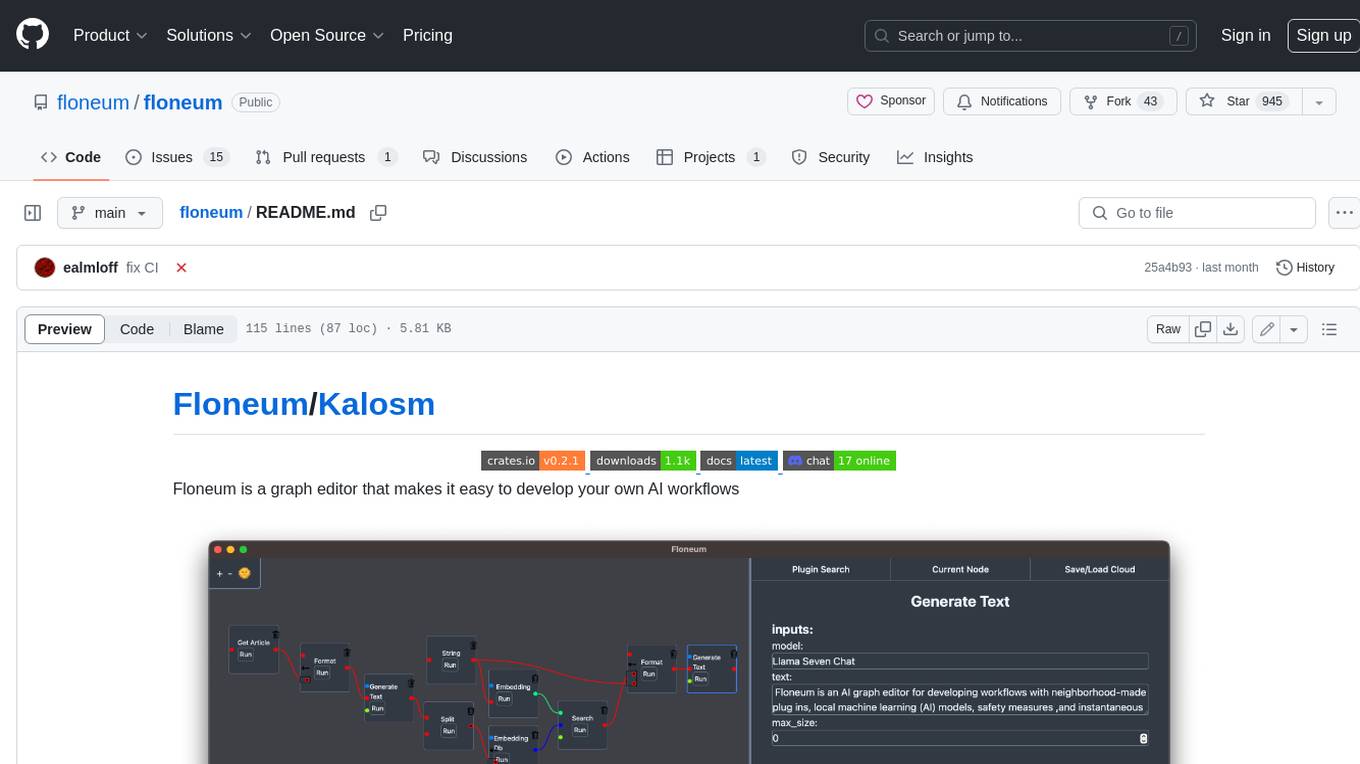
floneum
Floneum is a graph editor that makes it easy to develop your own AI workflows. It uses large language models (LLMs) to run AI models locally, without any external dependencies or even a GPU. This makes it easy to use LLMs with your own data, without worrying about privacy. Floneum also has a plugin system that allows you to improve the performance of LLMs and make them work better for your specific use case. Plugins can be used in any language that supports web assembly, and they can control the output of LLMs with a process similar to JSONformer or guidance.
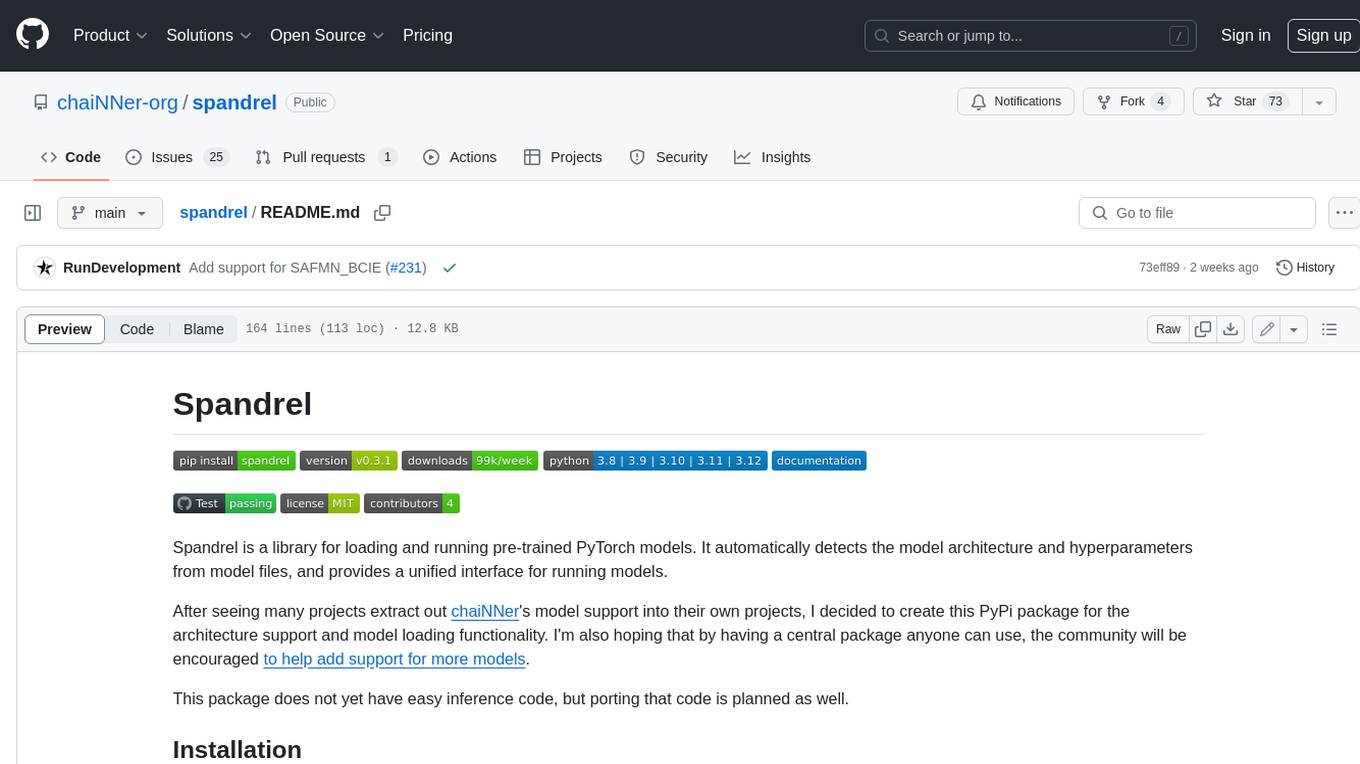
spandrel
Spandrel is a library for loading and running pre-trained PyTorch models. It automatically detects the model architecture and hyperparameters from model files, and provides a unified interface for running models.
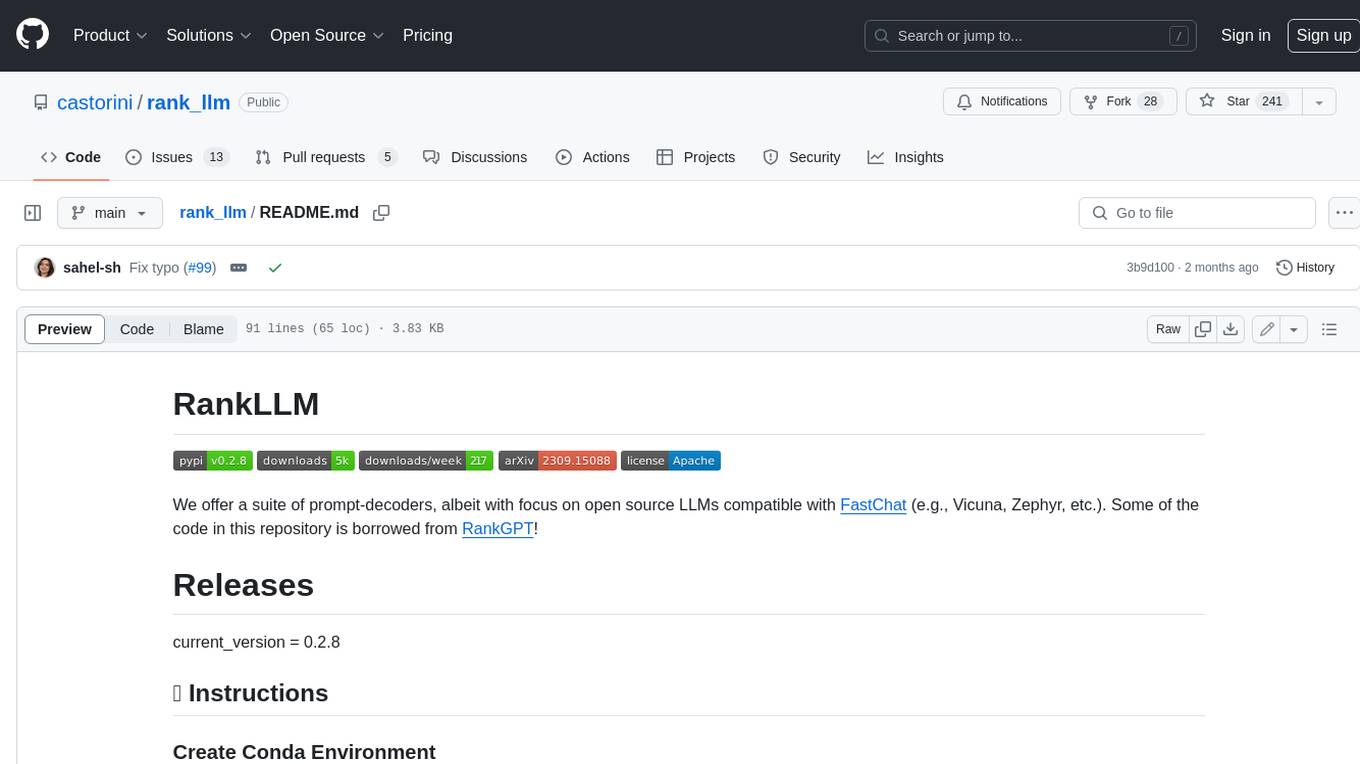
rank_llm
RankLLM is a suite of prompt-decoders compatible with open source LLMs like Vicuna and Zephyr. It allows users to create custom ranking models for various NLP tasks, such as document reranking, question answering, and summarization. The tool offers a variety of features, including the ability to fine-tune models on custom datasets, use different retrieval methods, and control the context size and variable passages. RankLLM is easy to use and can be integrated into existing NLP pipelines.
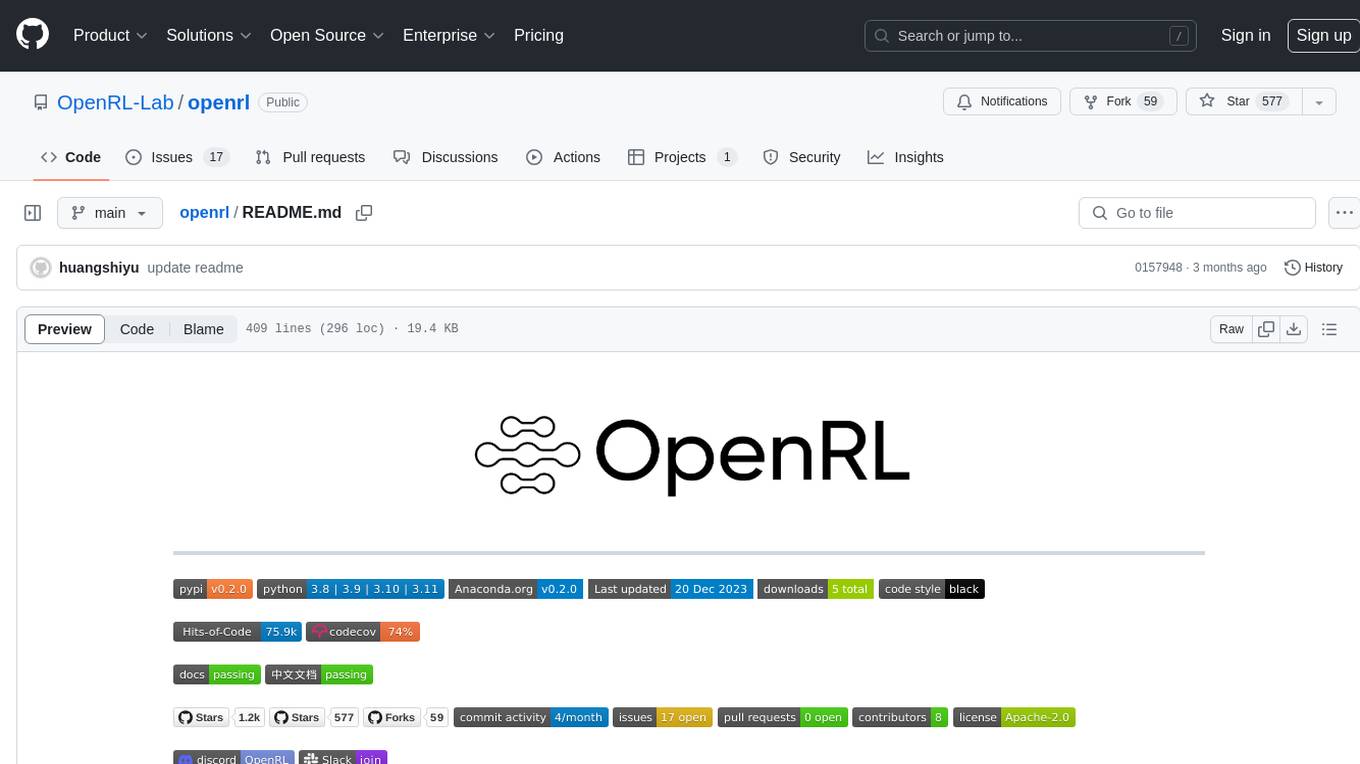
openrl
OpenRL is an open-source general reinforcement learning research framework that supports training for various tasks such as single-agent, multi-agent, offline RL, self-play, and natural language. Developed based on PyTorch, the goal of OpenRL is to provide a simple-to-use, flexible, efficient and sustainable platform for the reinforcement learning research community. It supports a universal interface for all tasks/environments, single-agent and multi-agent tasks, offline RL training with expert dataset, self-play training, reinforcement learning training for natural language tasks, DeepSpeed, Arena for evaluation, importing models and datasets from Hugging Face, user-defined environments, models, and datasets, gymnasium environments, callbacks, visualization tools, unit testing, and code coverage testing. It also supports various algorithms like PPO, DQN, SAC, and environments like Gymnasium, MuJoCo, Atari, and more.
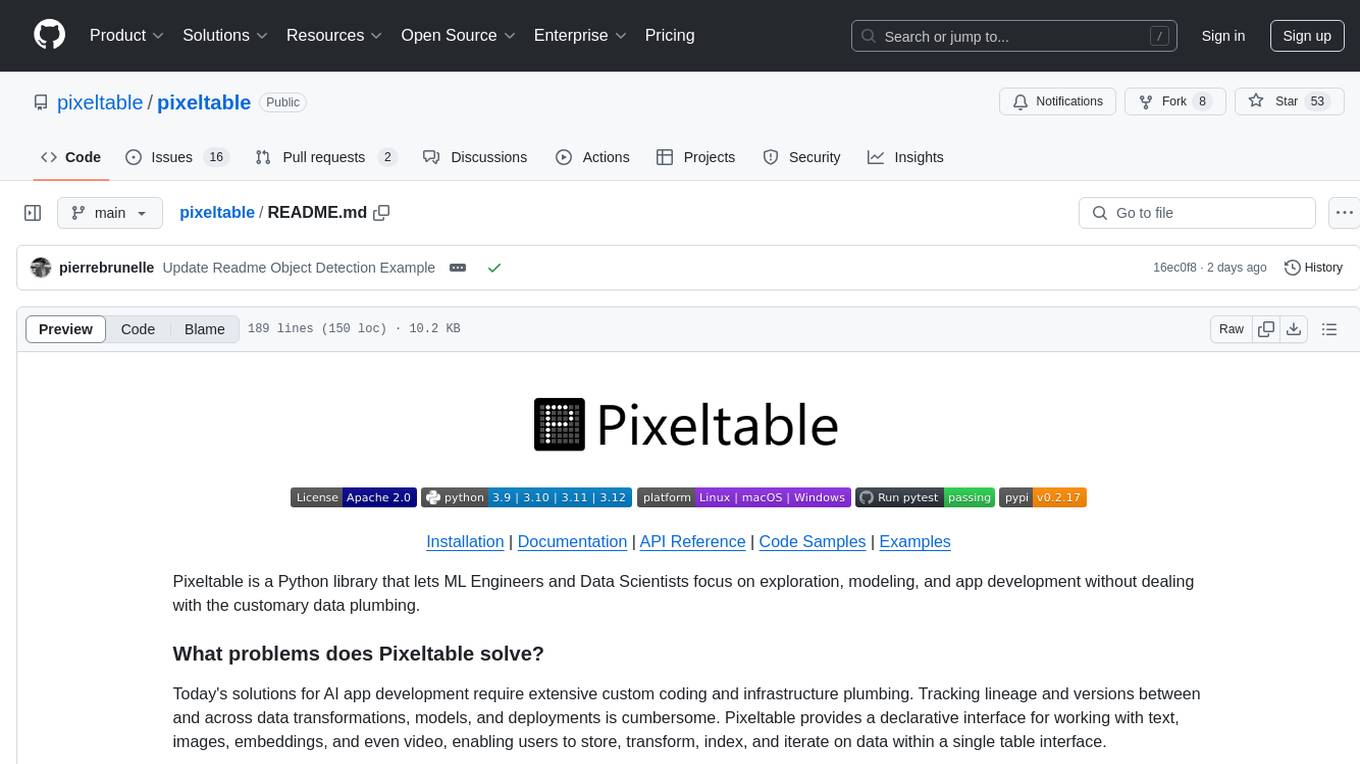
pixeltable
Pixeltable is a Python library designed for ML Engineers and Data Scientists to focus on exploration, modeling, and app development without the need to handle data plumbing. It provides a declarative interface for working with text, images, embeddings, and video, enabling users to store, transform, index, and iterate on data within a single table interface. Pixeltable is persistent, acting as a database unlike in-memory Python libraries such as Pandas. It offers features like data storage and versioning, combined data and model lineage, indexing, orchestration of multimodal workloads, incremental updates, and automatic production-ready code generation. The tool emphasizes transparency, reproducibility, cost-saving through incremental data changes, and seamless integration with existing Python code and libraries.
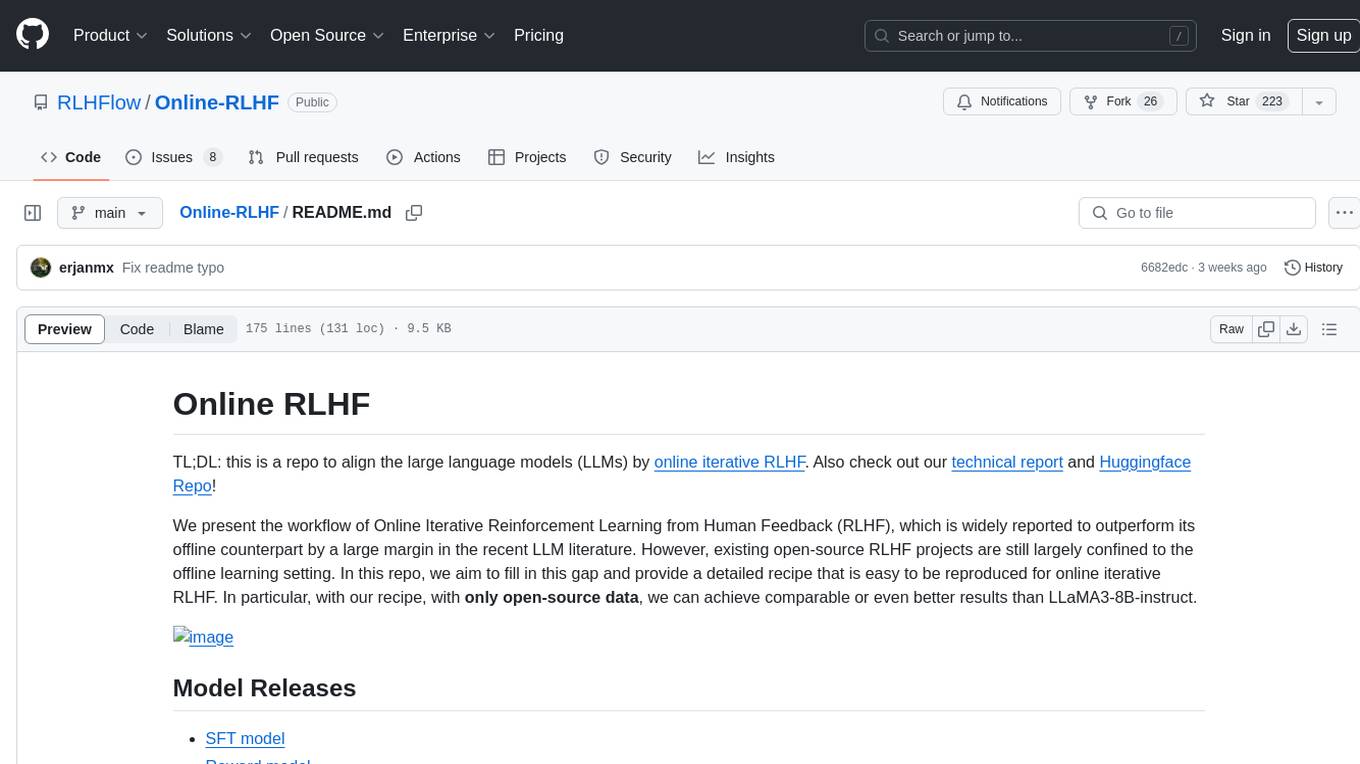
Online-RLHF
This repository, Online RLHF, focuses on aligning large language models (LLMs) through online iterative Reinforcement Learning from Human Feedback (RLHF). It aims to bridge the gap in existing open-source RLHF projects by providing a detailed recipe for online iterative RLHF. The workflow presented here has shown to outperform offline counterparts in recent LLM literature, achieving comparable or better results than LLaMA3-8B-instruct using only open-source data. The repository includes model releases for SFT, Reward model, and RLHF model, along with installation instructions for both inference and training environments. Users can follow step-by-step guidance for supervised fine-tuning, reward modeling, data generation, data annotation, and training, ultimately enabling iterative training to run automatically.
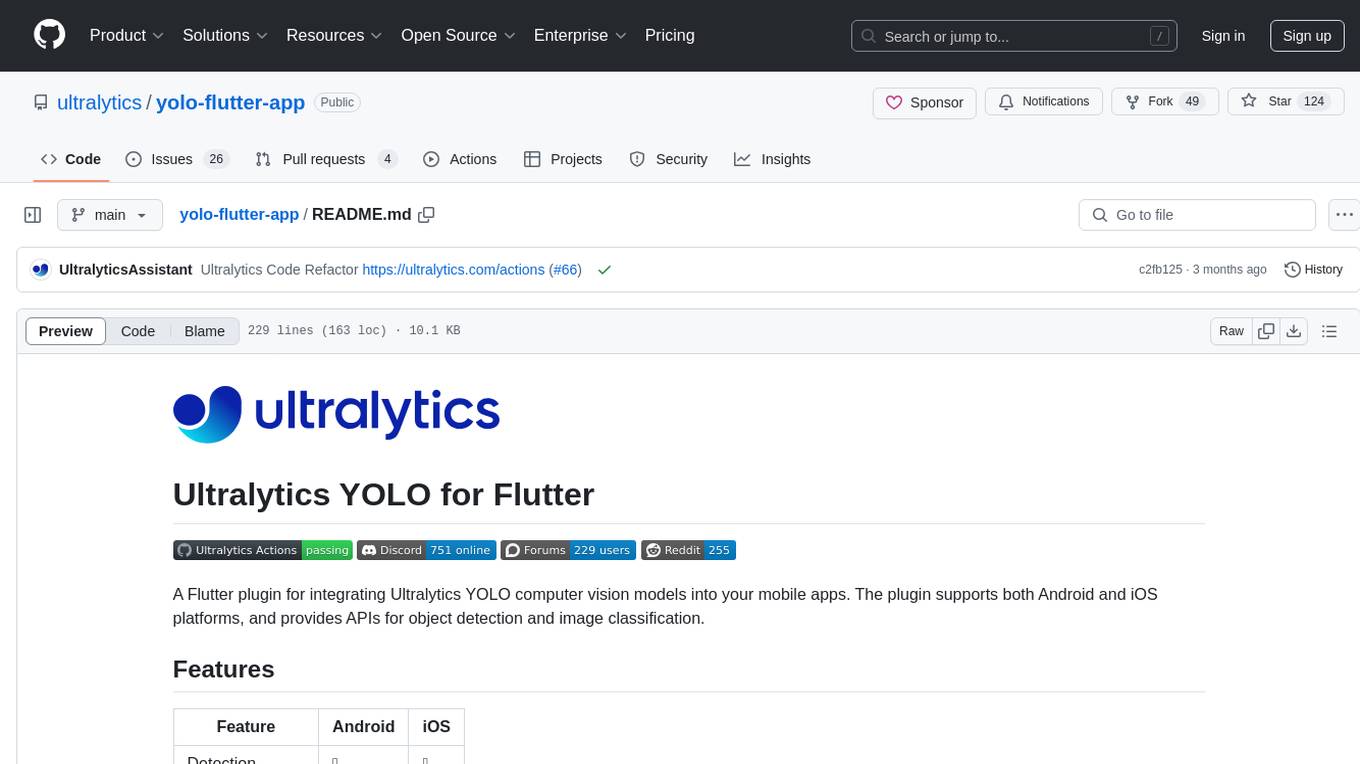
yolo-flutter-app
Ultralytics YOLO for Flutter is a Flutter plugin that allows you to integrate Ultralytics YOLO computer vision models into your mobile apps. It supports both Android and iOS platforms, providing APIs for object detection and image classification. The plugin leverages Flutter Platform Channels for seamless communication between the client and host, handling all processing natively. Before using the plugin, you need to export the required models in `.tflite` and `.mlmodel` formats. The plugin provides support for tasks like detection and classification, with specific instructions for Android and iOS platforms. It also includes features like camera preview and methods for object detection and image classification on images. Ultralytics YOLO thrives on community collaboration and offers different licensing paths for open-source and commercial use cases.
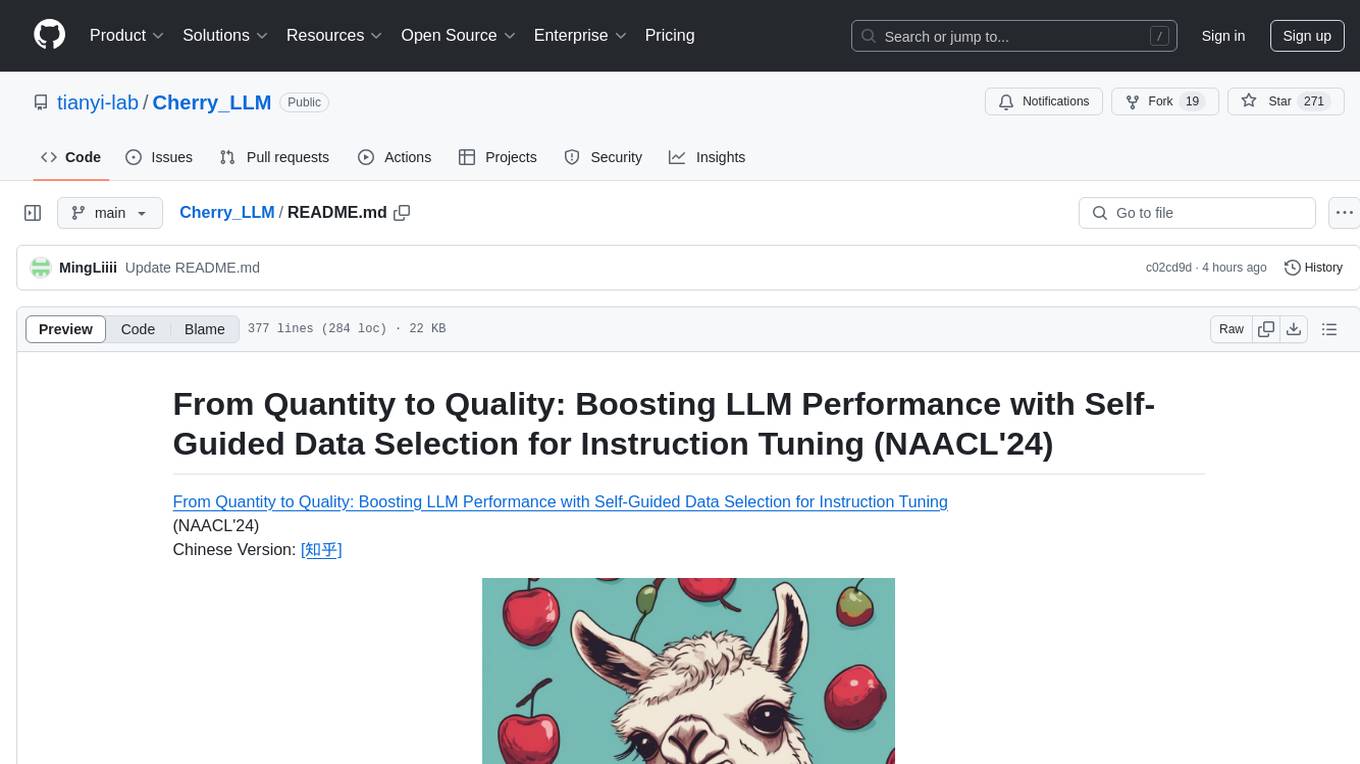
Cherry_LLM
Cherry Data Selection project introduces a self-guided methodology for LLMs to autonomously discern and select cherry samples from open-source datasets, minimizing manual curation and cost for instruction tuning. The project focuses on selecting impactful training samples ('cherry data') to enhance LLM instruction tuning by estimating instruction-following difficulty. The method involves phases like 'Learning from Brief Experience', 'Evaluating Based on Experience', and 'Retraining from Self-Guided Experience' to improve LLM performance.
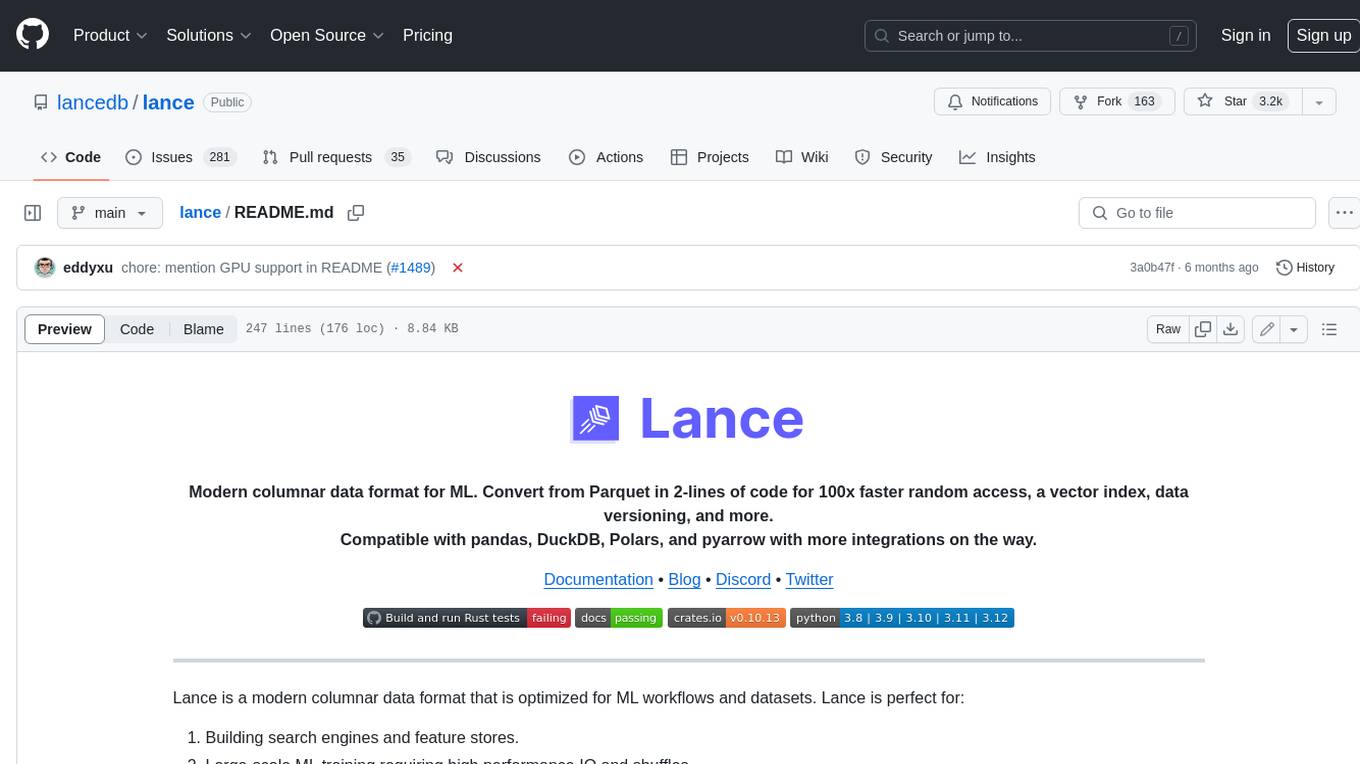
lance
Lance is a modern columnar data format optimized for ML workflows and datasets. It offers high-performance random access, vector search, zero-copy automatic versioning, and ecosystem integrations with Apache Arrow, Pandas, Polars, and DuckDB. Lance is designed to address the challenges of the ML development cycle, providing a unified data format for collection, exploration, analytics, feature engineering, training, evaluation, deployment, and monitoring. It aims to reduce data silos and streamline the ML development process.
For similar tasks
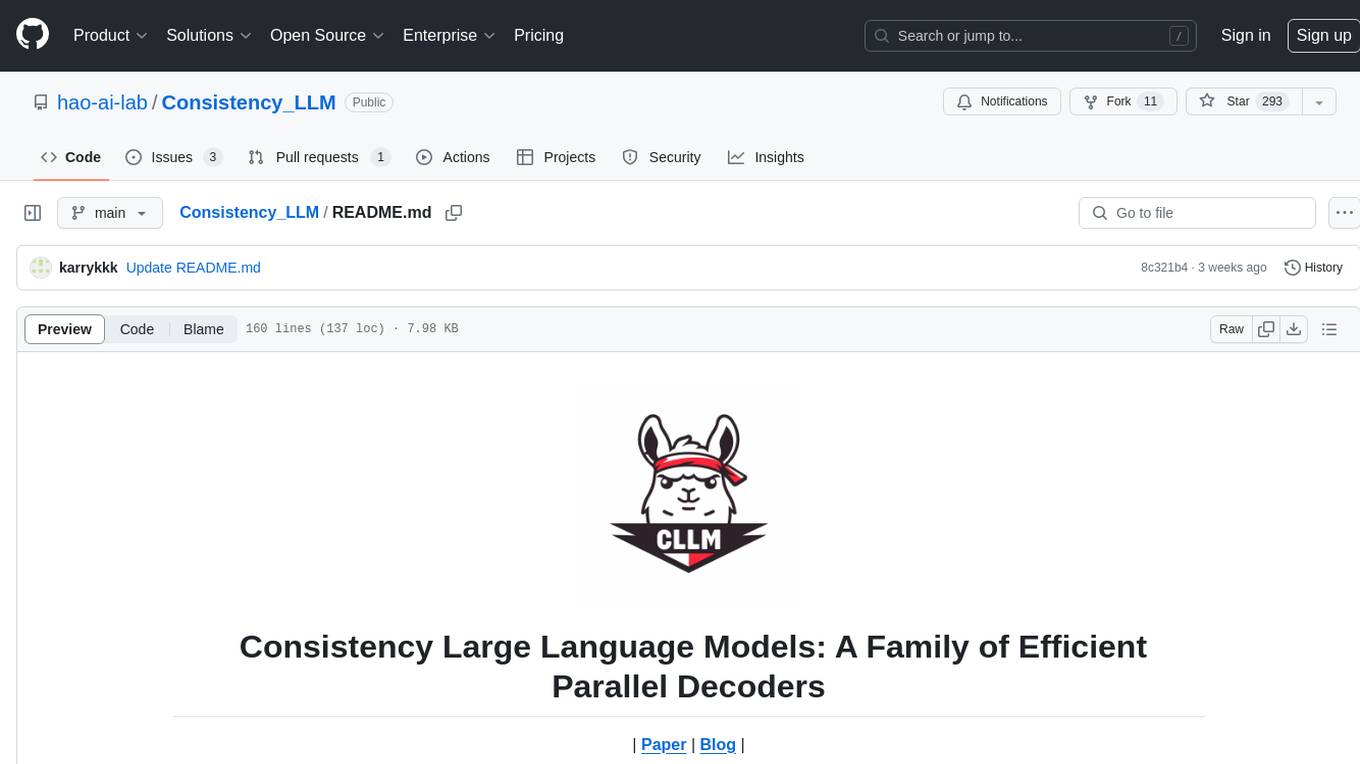
Consistency_LLM
Consistency Large Language Models (CLLMs) is a family of efficient parallel decoders that reduce inference latency by efficiently decoding multiple tokens in parallel. The models are trained to perform efficient Jacobi decoding, mapping any randomly initialized token sequence to the same result as auto-regressive decoding in as few steps as possible. CLLMs have shown significant improvements in generation speed on various tasks, achieving up to 3.4 times faster generation. The tool provides a seamless integration with other techniques for efficient Large Language Model (LLM) inference, without the need for draft models or architectural modifications.
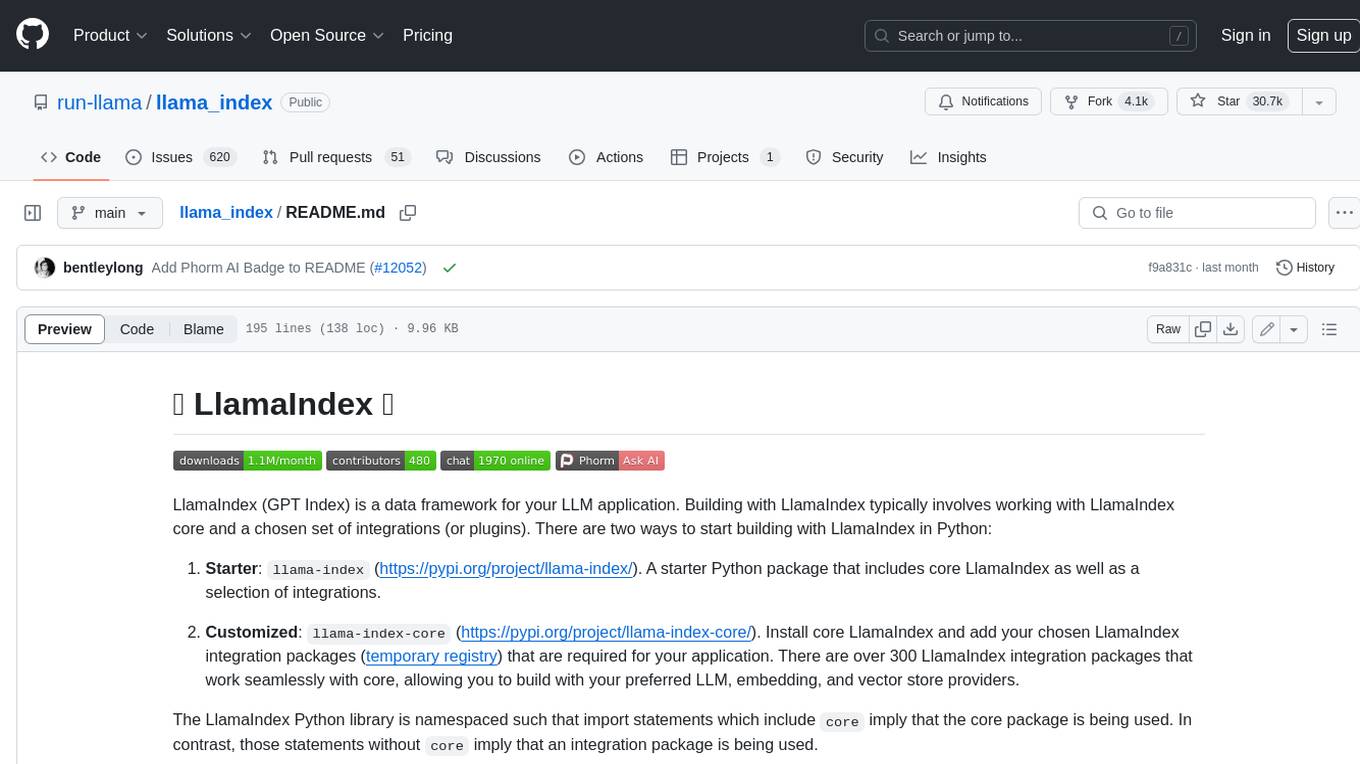
llama_index
LlamaIndex is a data framework for building LLM applications. It provides tools for ingesting, structuring, and querying data, as well as integrating with LLMs and other tools. LlamaIndex is designed to be easy to use for both beginner and advanced users, and it provides a comprehensive set of features for building LLM applications.
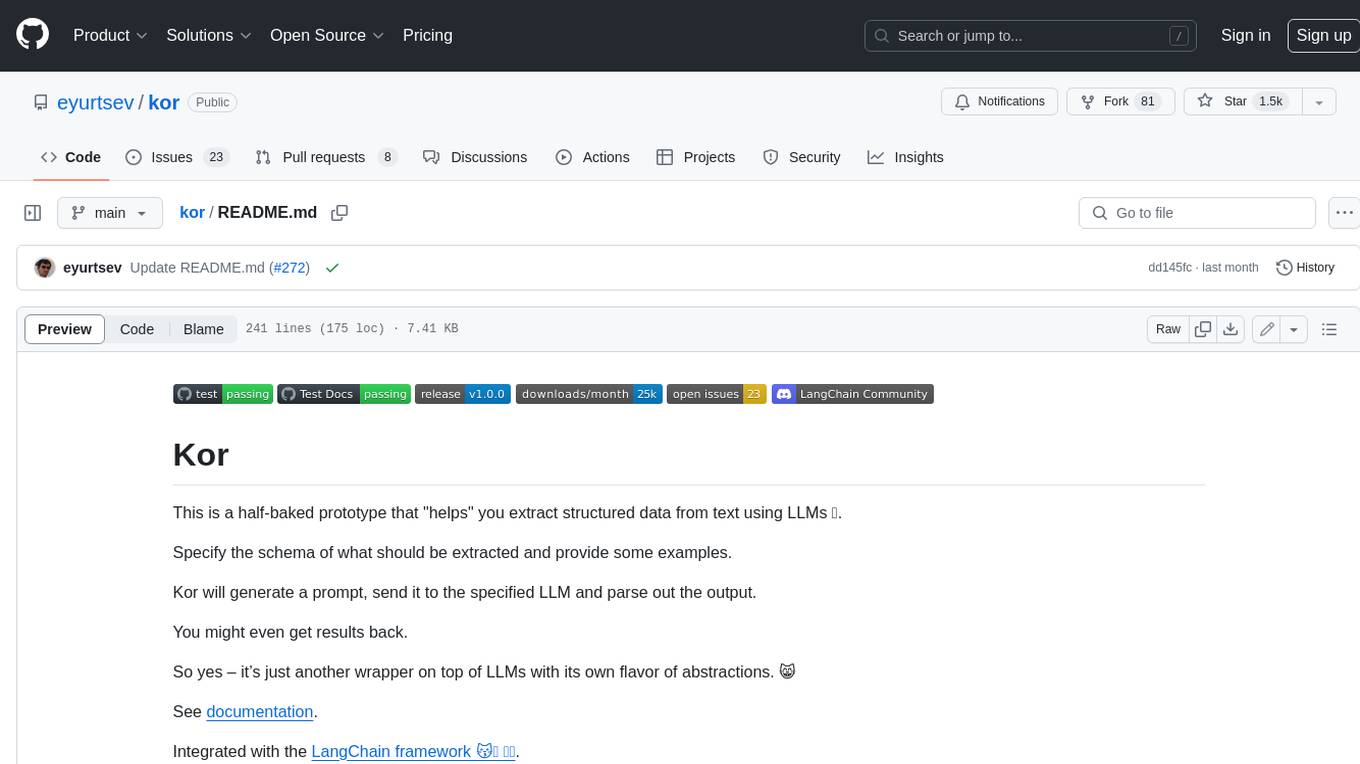
kor
Kor is a prototype tool designed to help users extract structured data from text using Language Models (LLMs). It generates prompts, sends them to specified LLMs, and parses the output. The tool works with the parsing approach and is integrated with the LangChain framework. Kor is compatible with pydantic v2 and v1, and schema is typed checked using pydantic. It is primarily used for extracting information from text based on provided reference examples and schema documentation. Kor is designed to work with all good-enough LLMs regardless of their support for function/tool calling or JSON modes.
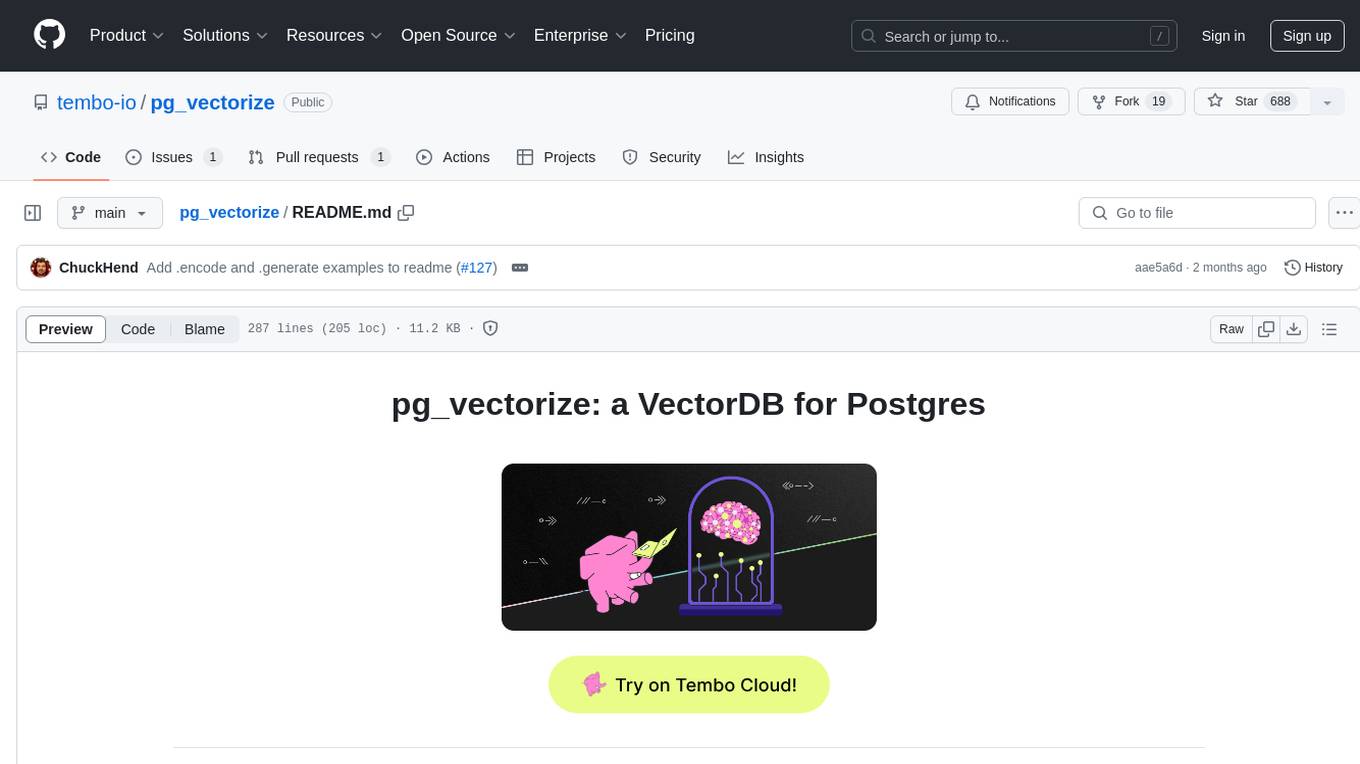
pg_vectorize
pg_vectorize is a Postgres extension that automates text to embeddings transformation, enabling vector search and LLM applications with minimal function calls. It integrates with popular LLMs, provides workflows for vector search and RAG, and automates Postgres triggers for updating embeddings. The tool is part of the VectorDB Stack on Tembo Cloud, offering high-level APIs for easy initialization and search.
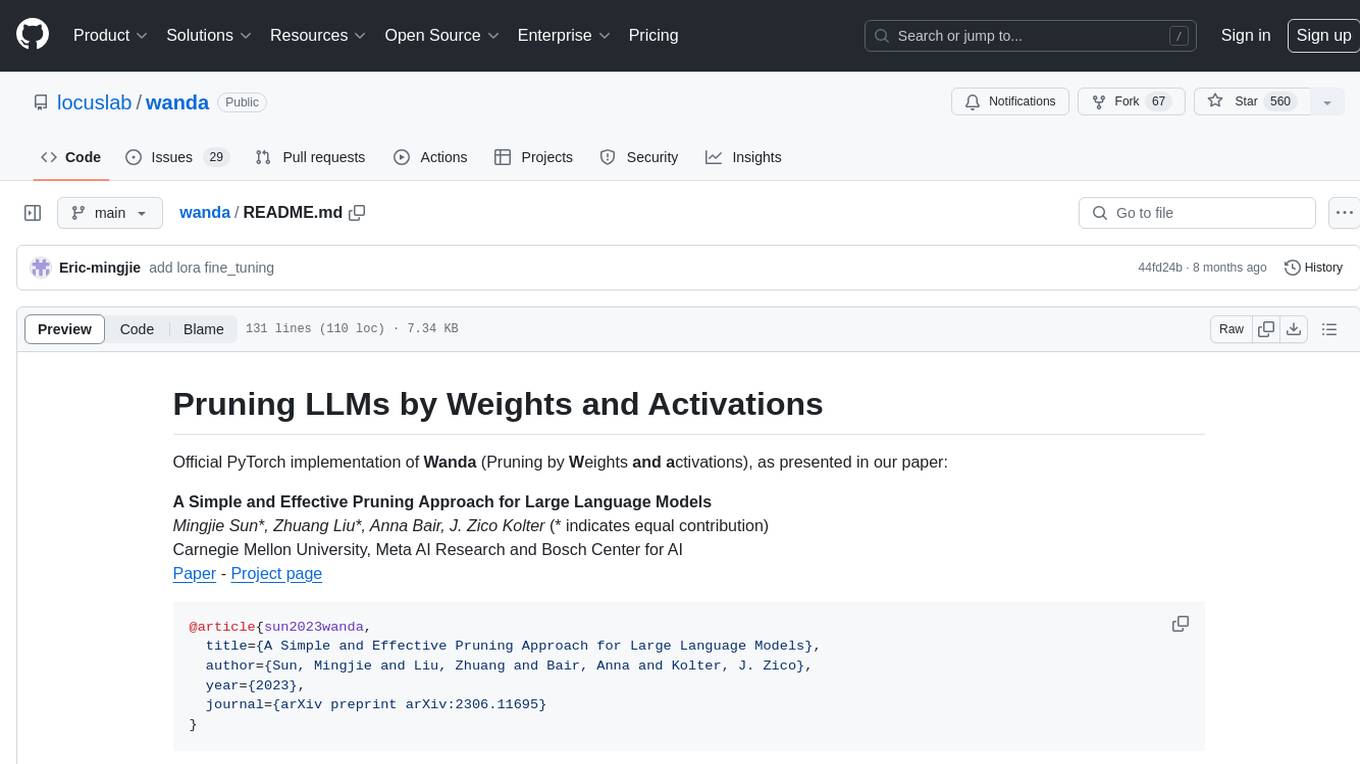
wanda
Official PyTorch implementation of Wanda (Pruning by Weights and Activations), a simple and effective pruning approach for large language models. The pruning approach removes weights on a per-output basis, by the product of weight magnitudes and input activation norms. The repository provides support for various features such as LLaMA-2, ablation study on OBS weight update, zero-shot evaluation, and speedup evaluation. Users can replicate main results from the paper using provided bash commands. The tool aims to enhance the efficiency and performance of language models through structured and unstructured sparsity techniques.
For similar jobs
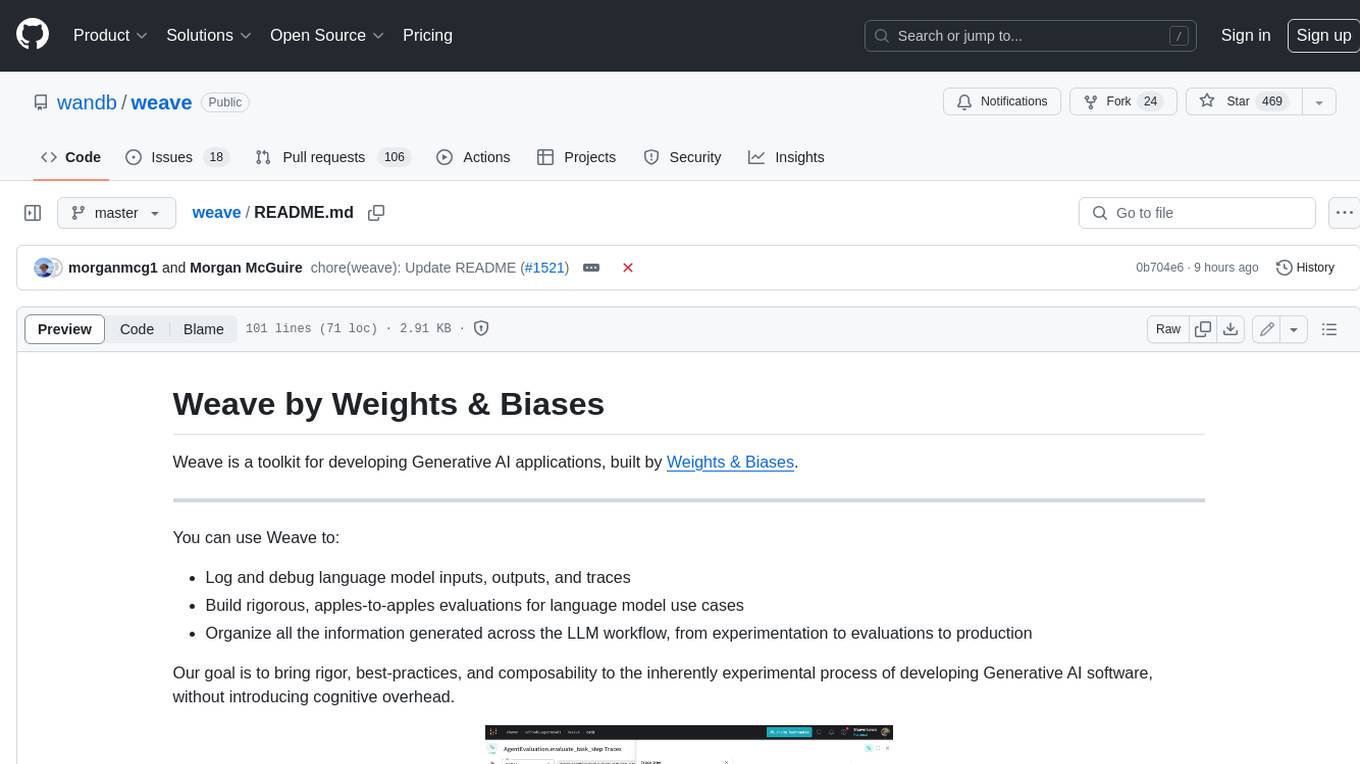
weave
Weave is a toolkit for developing Generative AI applications, built by Weights & Biases. With Weave, you can log and debug language model inputs, outputs, and traces; build rigorous, apples-to-apples evaluations for language model use cases; and organize all the information generated across the LLM workflow, from experimentation to evaluations to production. Weave aims to bring rigor, best-practices, and composability to the inherently experimental process of developing Generative AI software, without introducing cognitive overhead.
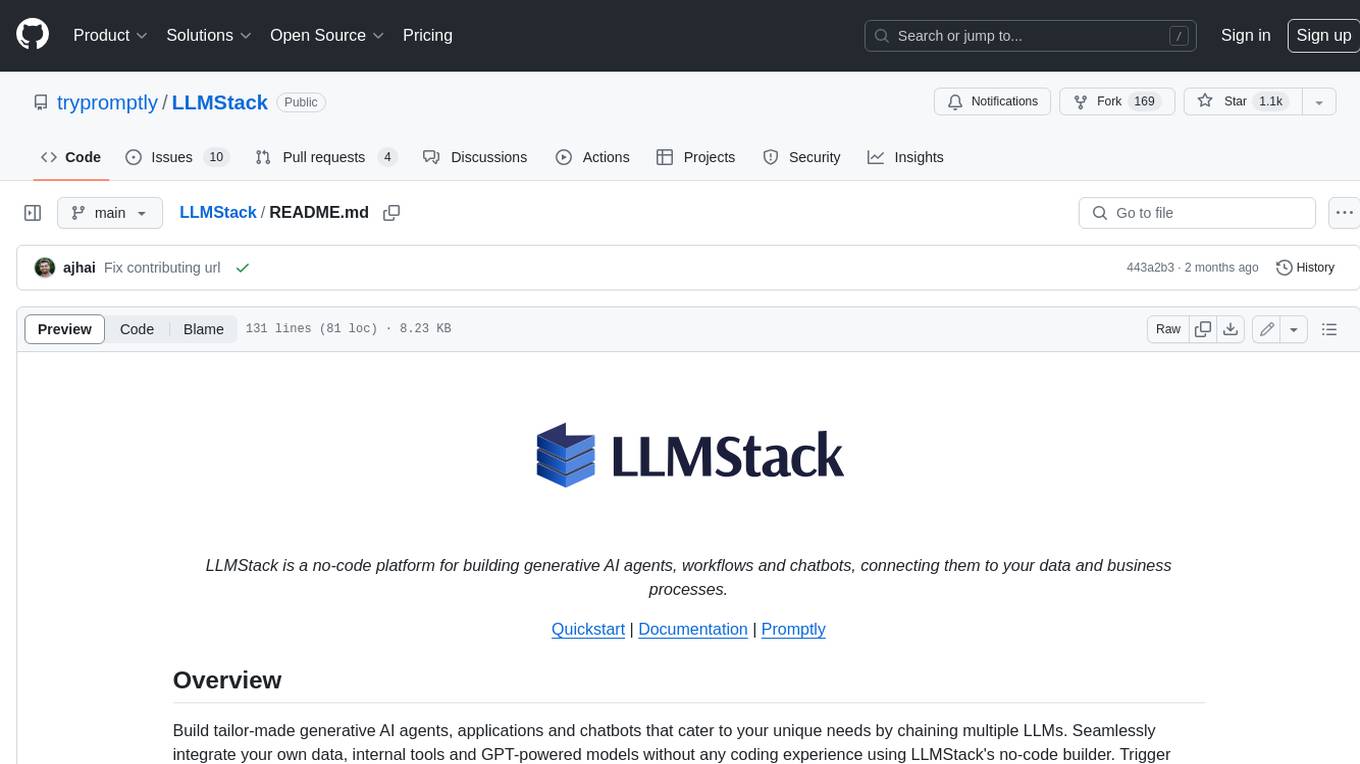
LLMStack
LLMStack is a no-code platform for building generative AI agents, workflows, and chatbots. It allows users to connect their own data, internal tools, and GPT-powered models without any coding experience. LLMStack can be deployed to the cloud or on-premise and can be accessed via HTTP API or triggered from Slack or Discord.
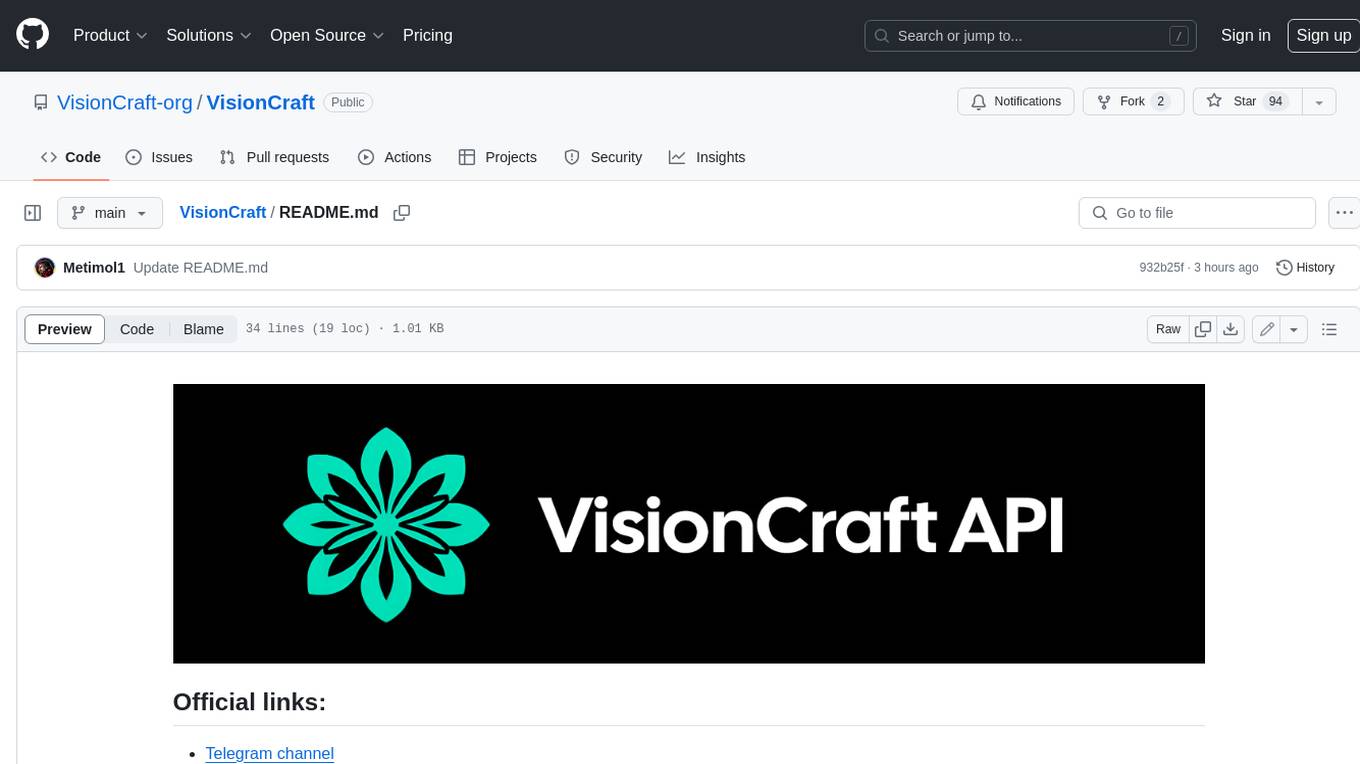
VisionCraft
The VisionCraft API is a free API for using over 100 different AI models. From images to sound.
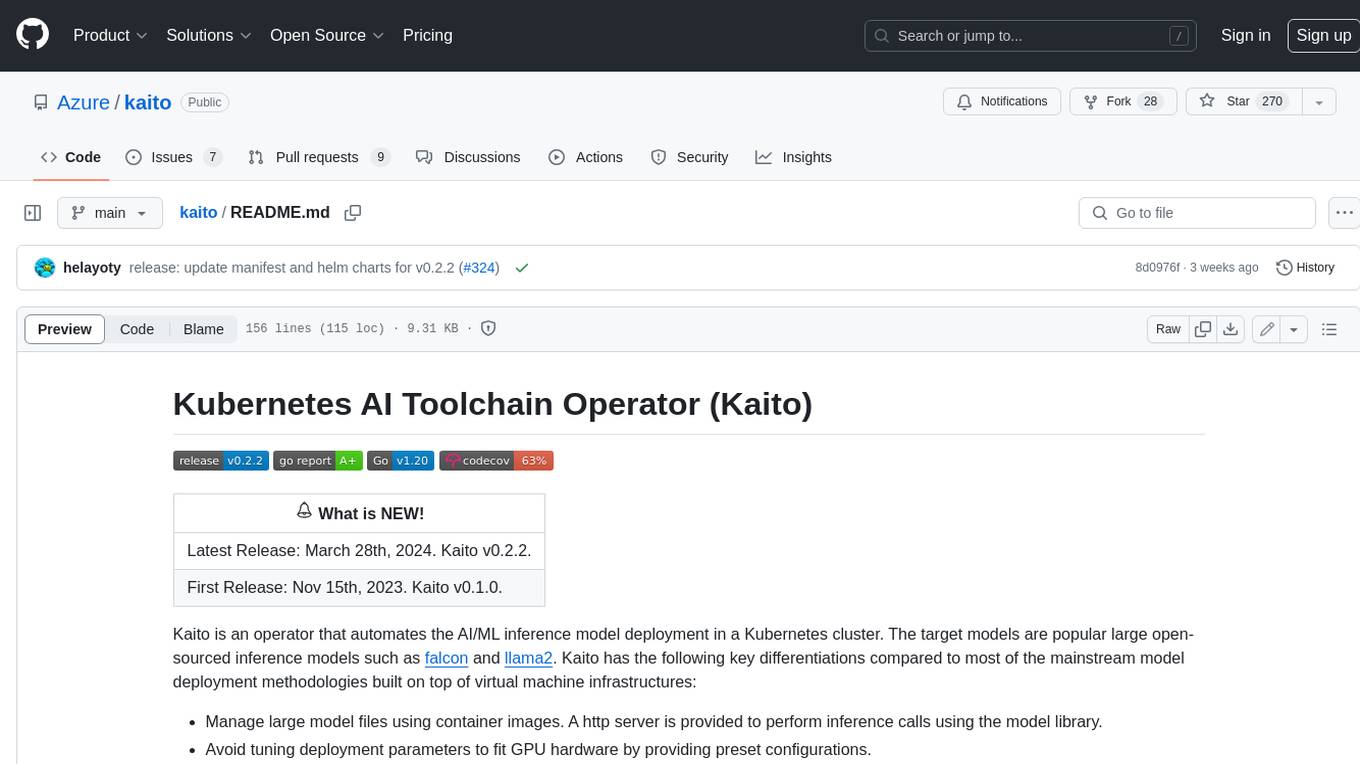
kaito
Kaito is an operator that automates the AI/ML inference model deployment in a Kubernetes cluster. It manages large model files using container images, avoids tuning deployment parameters to fit GPU hardware by providing preset configurations, auto-provisions GPU nodes based on model requirements, and hosts large model images in the public Microsoft Container Registry (MCR) if the license allows. Using Kaito, the workflow of onboarding large AI inference models in Kubernetes is largely simplified.
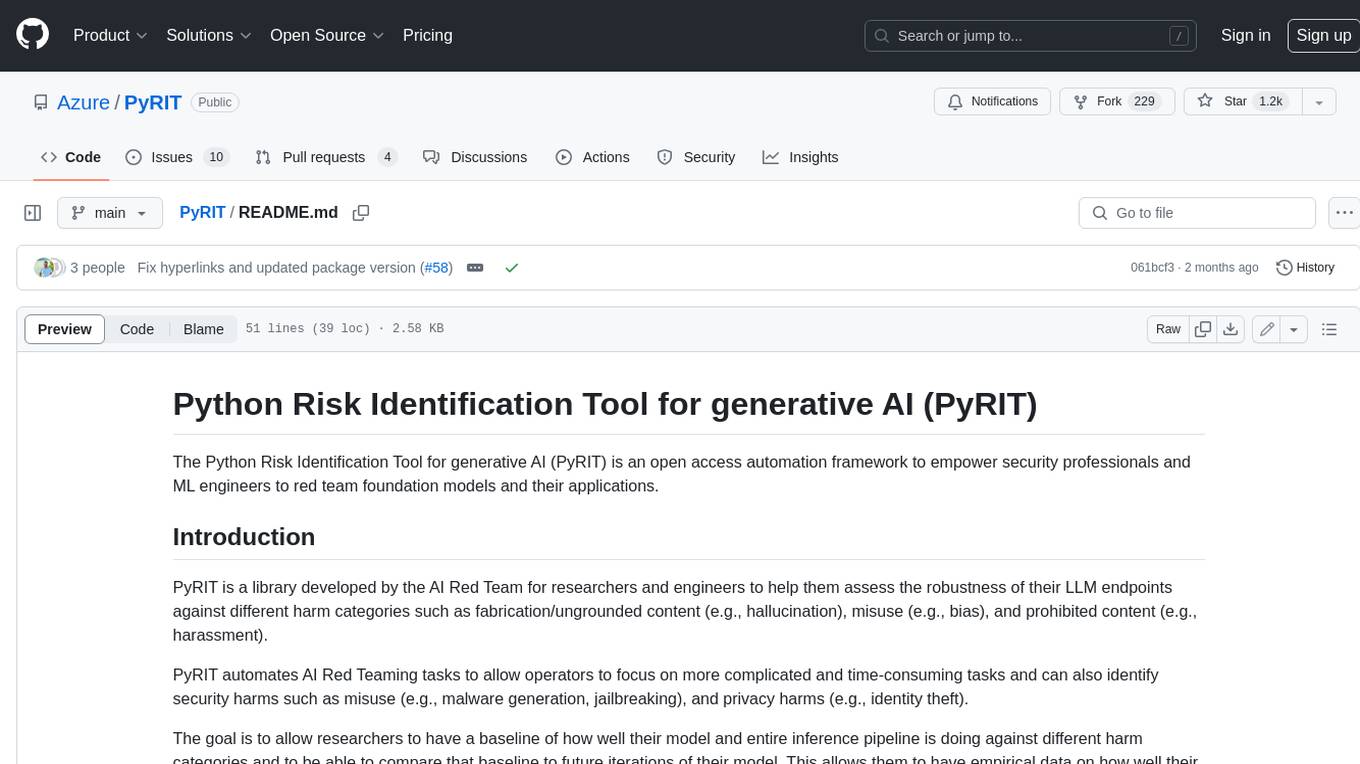
PyRIT
PyRIT is an open access automation framework designed to empower security professionals and ML engineers to red team foundation models and their applications. It automates AI Red Teaming tasks to allow operators to focus on more complicated and time-consuming tasks and can also identify security harms such as misuse (e.g., malware generation, jailbreaking), and privacy harms (e.g., identity theft). The goal is to allow researchers to have a baseline of how well their model and entire inference pipeline is doing against different harm categories and to be able to compare that baseline to future iterations of their model. This allows them to have empirical data on how well their model is doing today, and detect any degradation of performance based on future improvements.
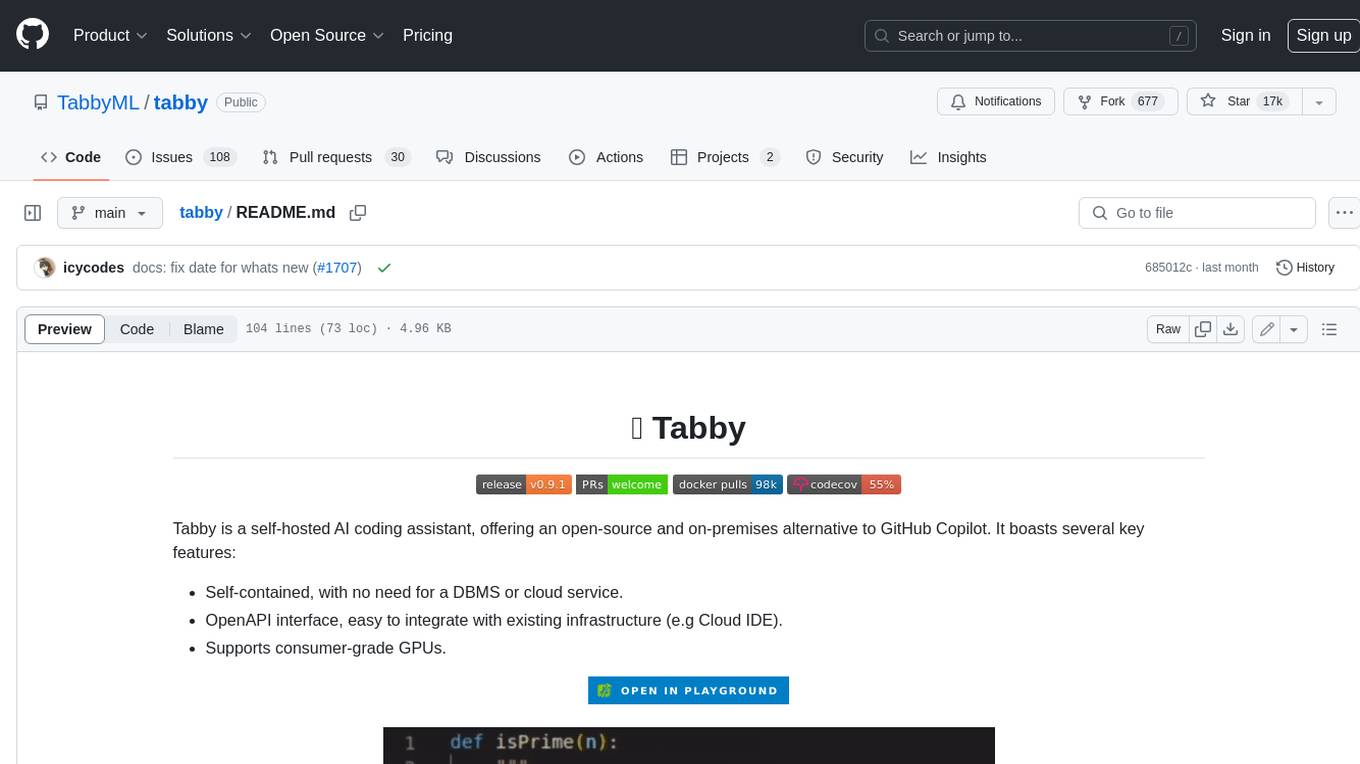
tabby
Tabby is a self-hosted AI coding assistant, offering an open-source and on-premises alternative to GitHub Copilot. It boasts several key features: * Self-contained, with no need for a DBMS or cloud service. * OpenAPI interface, easy to integrate with existing infrastructure (e.g Cloud IDE). * Supports consumer-grade GPUs.
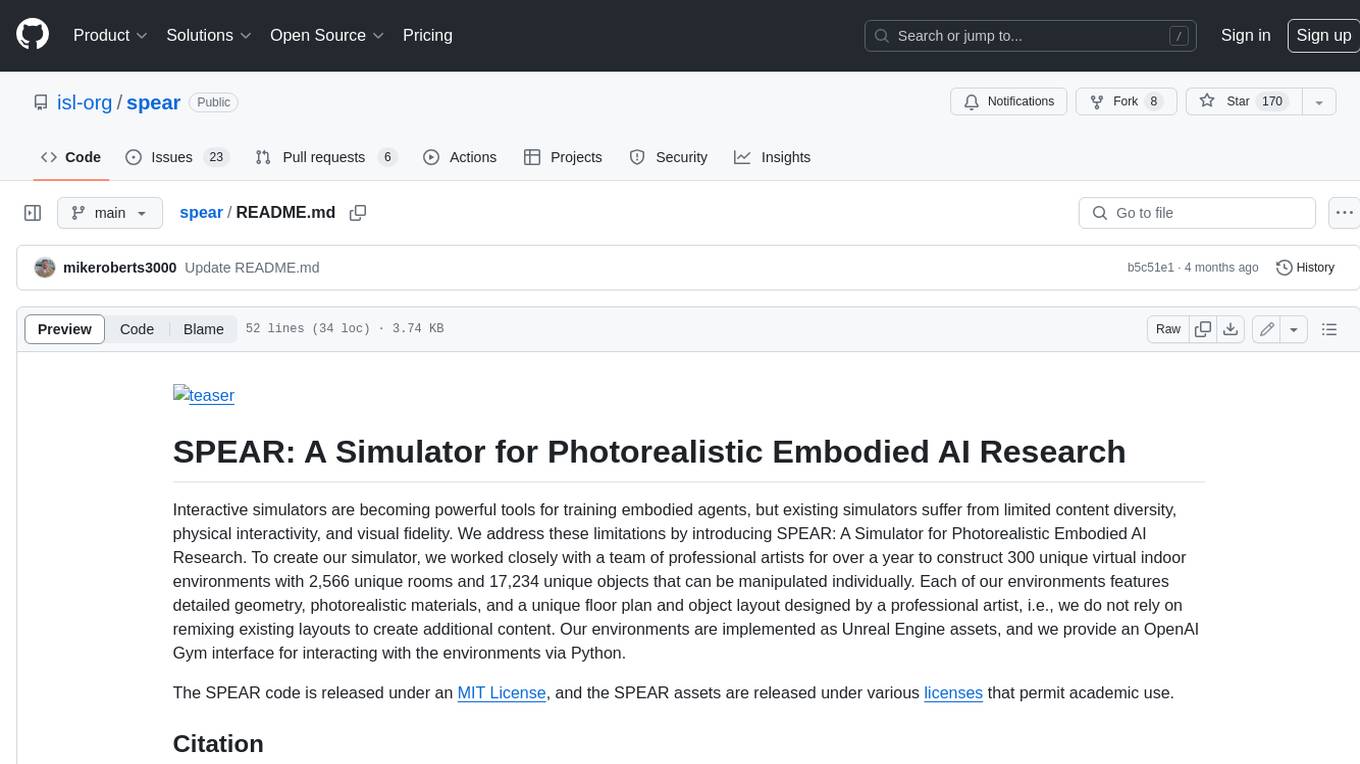
spear
SPEAR (Simulator for Photorealistic Embodied AI Research) is a powerful tool for training embodied agents. It features 300 unique virtual indoor environments with 2,566 unique rooms and 17,234 unique objects that can be manipulated individually. Each environment is designed by a professional artist and features detailed geometry, photorealistic materials, and a unique floor plan and object layout. SPEAR is implemented as Unreal Engine assets and provides an OpenAI Gym interface for interacting with the environments via Python.
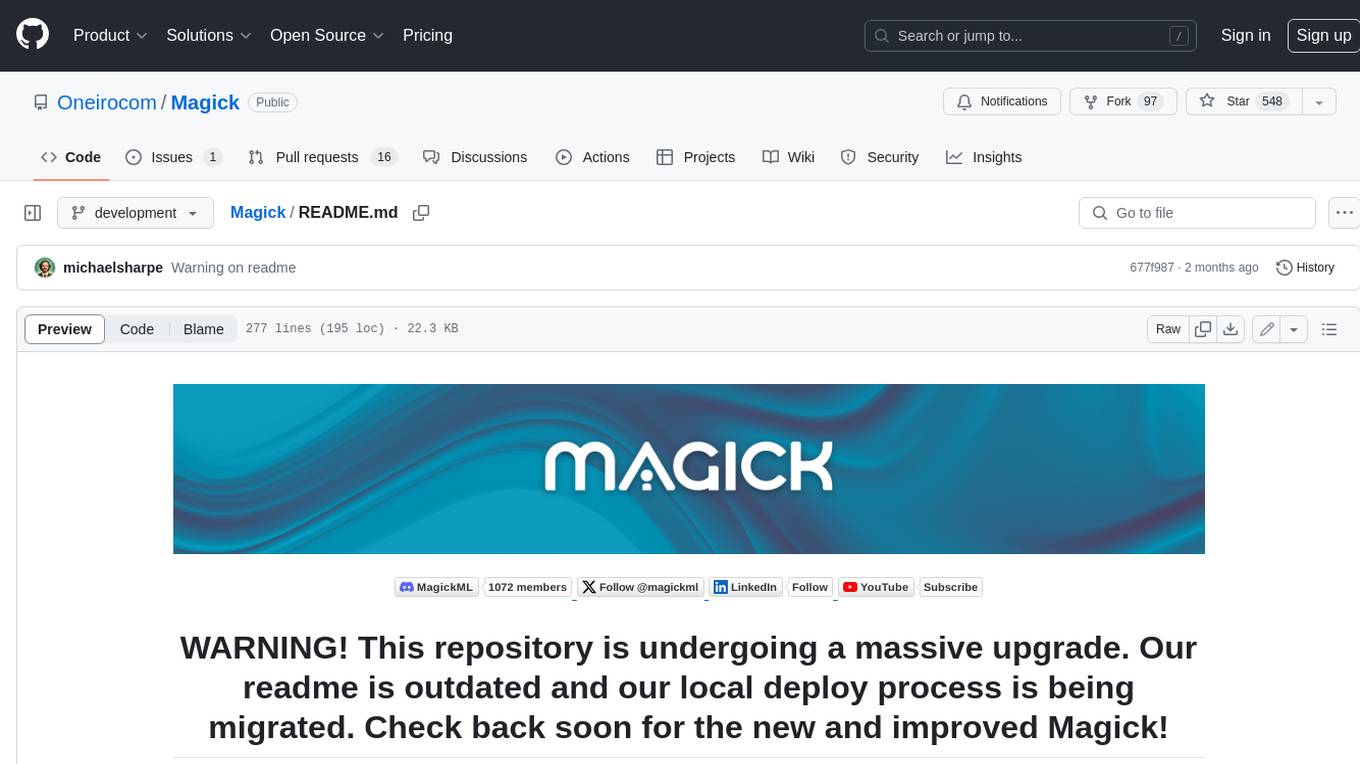
Magick
Magick is a groundbreaking visual AIDE (Artificial Intelligence Development Environment) for no-code data pipelines and multimodal agents. Magick can connect to other services and comes with nodes and templates well-suited for intelligent agents, chatbots, complex reasoning systems and realistic characters.