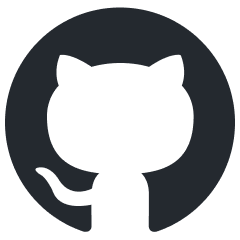
Online-RLHF
A recipe for online RLHF and online iterative DPO.
Stars: 467
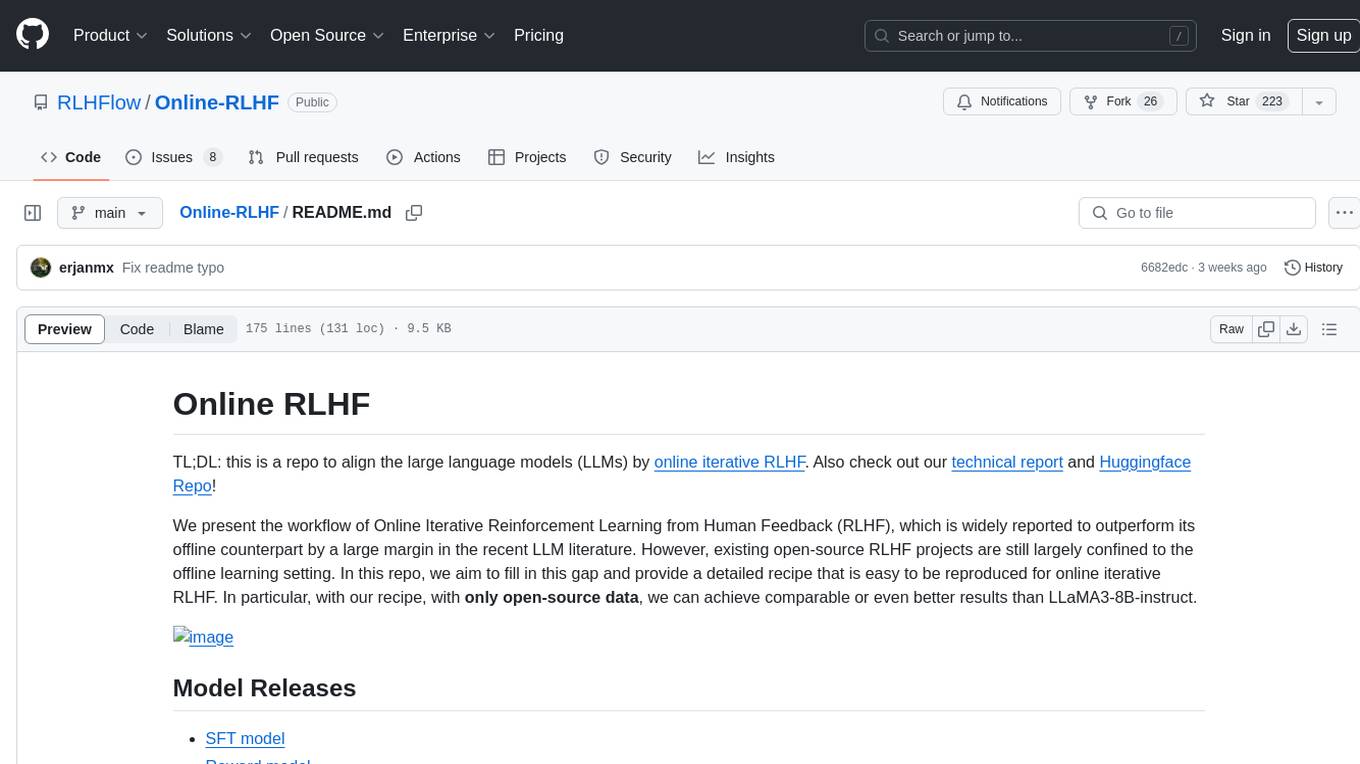
This repository, Online RLHF, focuses on aligning large language models (LLMs) through online iterative Reinforcement Learning from Human Feedback (RLHF). It aims to bridge the gap in existing open-source RLHF projects by providing a detailed recipe for online iterative RLHF. The workflow presented here has shown to outperform offline counterparts in recent LLM literature, achieving comparable or better results than LLaMA3-8B-instruct using only open-source data. The repository includes model releases for SFT, Reward model, and RLHF model, along with installation instructions for both inference and training environments. Users can follow step-by-step guidance for supervised fine-tuning, reward modeling, data generation, data annotation, and training, ultimately enabling iterative training to run automatically.
README:
TL;DL: this is a repo to align the large language models (LLMs) by online iterative RLHF. Also check out our technical report and Huggingface Repo!
We present the workflow of Online Iterative Reinforcement Learning from Human Feedback (RLHF), which is widely reported to outperform its offline counterpart by a large margin in the recent LLM literature. However, existing open-source RLHF projects are still largely confined to the offline learning setting. In this repo, we aim to fill in this gap and provide a detailed recipe that is easy to be reproduced for online iterative RLHF. In particular, with our recipe, with only open-source data, we can achieve comparable or even better results than LLaMA3-8B-instruct.
SFT Model: check more SFT checkpoints Here and dataset RLHFlow/RLHFlow-SFT-Dataset-ver2
- RLHFlow/LLaMA3-SFT: v1.0 with 1 epoch training
- RLHFlow/LLaMA3-SFT-v2: v2.0 with 2 epoch training
- RLHFlow/Llama3-SFT-v2.0-epoch1
- RLHFlow/Llama3-SFT-v2.0-epoch3
Reward Model: also check more reward models Here
- Reward model: Bradley-Terry model
- RLHFlow/pair-preference-model-LLaMA3-8B: generative pairwise preference model
- RLHFlow/ArmoRM-Llama3-8B-v0.1: multi-head reward model with mixture-of-expert aggregation
RLHF Model:
- RLHF model: trained from RLHFlow/LLaMA3-SFT
- RLHF model v2 iter1: trained from RLHFlow/LLaMA3-SFT-v2
- RLHF model v2 iter2: trained from RLHFlow/LLaMA3-SFT-v2
- RLHF model v2 iter3: trained from RLHFlow/LLaMA3-SFT-v2
Model | LC AlpacaEval | MATH | GSM8K | HumanEval | HumanEval+ | MMLU | ARC-c | Truthful QA |
---|---|---|---|---|---|---|---|---|
RLHFlow/LLaMA3-SFT | 12.47 (1146 token) | 30 | 76.9 | 0.634 | 0.561 | 0.6462 | 0.5862 | 0.5345 |
RLHFlow/LLaMA3-SFT-v2 | 12.66 (1175 token) | 41.1 | 83.4 | 0.665 | 0.616 | 0.648 | 0.5998 | 0.5393 |
RLHFlow/LLaMA3-iterative-DPO-final (v1) | 30.9 | 31.3 | 82.1 | 0.64 | 0.585 | 0.6545 | 0.628 | 0.6216 |
RLHFlow/Llama3-v2-iterative-DPO-iter1 | - | 43 | 85.3 | 0.634 | 0.585 | 0.6494 | 0.634 | 0.5955 |
RLHFlow/Llama3-v2-iterative-DPO-iter2 | - | 43.8 | 84.8 | 0.671 | 0.591 | 0.6477 | 0.651 | 0.6331 |
RLHFlow/Llama3-v2-iterative-DPO-iter3 | 31.31 (2157 token) | 44.4 | 85.3 | 0.683 | 0.622 | 0.6466 | 0.6596 | 0.6473 |
meta-llama/Meta-Llama-3-8B-Instruct | 22.9 | 26.3 | 70.2 | 0.64 | 0.567 | 0.6561 | 0.5819 | 0.5166 |
meta-llama/Llama-3.1-8B-Instruct | 20.9 | 50 | 86.5 | 0.689 | 0.622 | 0.682 | 0.558 | 0.5408 |
It is recommended to have two separate environments for inference and training, respectively.
Note that the numpy version should be numpy<2.0
. Numpy 2.0
will encounter unexpected issues!!!
SFT Environment
conda create -n sft python=3.10.9
conda activate sft
## Get axolotl for general model
git clone https://github.com/OpenAccess-AI-Collective/axolotl
cd axolotl
git checkout 55cc214c767741e83ee7b346e5e13e6c03b7b9fa
pip install -e .
# The test cuda version is 12.1, 12.2. You may need to update the torch version based on your cuda version...
# you may encounter underfined symbol error related to cuda and flash-attn and 2.1.2 can solve it ...
pip3 install torch==2.1.2 torchvision torchaudio
pip install flash-attn
# fix an error of axolotl: ModuleNotFoundError: No module named 'pynvml.nvml'; 'pynvml' is not a package
pip install nvidia-ml-py3
# also edit axolotl/src/axolotl/utils/bench.py (line 6) to: ``from pynvml import NVMLError''
## Get FastChat
git clone https://github.com/lm-sys/FastChat.git
cd FastChat
pip install -e .
git clone https://github.com/WeiXiongUST/RLHF-Reward-Modeling.git
pip install deepspeed
You also need to install wandb to record the training and log in with the huggingface accout to access Gemma.
pip install wandb
wandb login
huggingface-cli login
Inference Environment
conda create -n vllm python=3.10.9
conda activate vllm
pip install datasets
# The following code is tested for CUDA12.0-12.2, and CUDA12.6
# To develop llama-3, mistral, gemma-1, 1.1, 2, deepseek you can consider the following vllm version
pip install vllm==0.5.4
pip install accelerate==0.33.0
pip install deepspeed==0.14.5
pip install transformers==4.43.4
pip install numpy==1.26.4 #Note that the numpy version should be `numpy<2.0`. `Numpy 2.0` will encounter unexpected issues!!!
Training Environment
conda create -n rlhflow python=3.10.9
conda activate rlhflow
git clone https://github.com/huggingface/alignment-handbook.git
cd ./alignment-handbook/
git checkout 27f7dbf00663dab66ad7334afb7a1311fa251f41
pip3 install torch==2.1.2 torchvision torchaudio
python -m pip install .
pip install flash-attn==2.6.3
pip install accelerate==0.33.0
pip install huggingface-hub==0.24.7
You also need to install the wandb to record the training and login with your huggingface account so that you have access to the LLaMA3 models.
pip install wandb==0.17.7
wandb login
huggingface-cli login
We present a step-by-step guidance in this section.
We need to process the SFT data into the standard format. See RLHFlow/RLHFlow-SFT-Dataset-ver2 for an eample.
cd sft
torchrun --nproc_per_node 8 --master_port 20001 -m axolotl.cli.train llama3-8b-it.yaml
You can also modify the learning rate, batch size, output_path.. with either command or modify the ScriptArguments in the llama3-8b-it.yaml. If you encounter out-of-memory issue. Running the code with Gemma-2b-it with deepspeed stage 3 and gradient checkpoint (set in the config).
torchrun --nproc_per_node 8 --master_port 20001 -m axolotl.cli.train llama3-8b-it.yaml --deepspeed ../configs/deepspeed_stage3.json
We refer the interested readers to this repo for a detailed recipe to train the state-of-the-art open-source reward/preference models. We have trained several RMs and prepared them on the huggingface like sfairXC/FsfairX-LLaMA3-RM-v0.1, RLHFlow/pair-preference-model-LLaMA3-8B, RLHFlow/ArmoRM-Llama3-8B-v0.1, which are SOTA open-source RMs so far (2024 May).
We have prepared some prompt sets on huggingface.
- UltraFeedback RLHFlow/ultrafeedback_iter1, RLHFlow/ultrafeedback_iter2, RLHFlow/ultrafeedback_iter3
- RLHFlow/iterative-prompt-v1-iter1-20K, RLHFlow/iterative-prompt-v1-iter2-20K, RLHFlow/iterative-prompt-v1-iter3-20K ...
To accelerate data generation, we use the VLLM. We prepare two ways of using VLLM to inference for a more robust implementation, where you can try them out and choose the one that fits with your environment best. We use LLaMA3-8B as an example.
You may create a test_gen.sh file, and copy the following contents into the file and run ``bash test_gen.sh''.
# First approach: initialize 4 VLLM processes and split the prompt set to the 4 agents
# The generated samples will be stored at output_dir + local_index + ".jsonl
my_world_size=8 # how many gpu you use
infer_model=RLHFlow/LLaMA3-SFT
prompt_dir=RLHFlow/test_generation_2k
mkdir data
output_dir=./data/gen_data
conda activate vllm
CUDA_VISIBLE_DEVICES=0 python ./generation/gen_hf2.py --model_name_or_path ${infer_model} --dataset_name_or_path ${prompt_dir} --output_dir ${output_dir} --K 4 --temperature 1.0 --local_index 0 --my_world_size ${my_world_size} &
CUDA_VISIBLE_DEVICES=1 python ./generation/gen_hf2.py --model_name_or_path ${infer_model} --dataset_name_or_path ${prompt_dir} --output_dir ${output_dir} --K 4 --temperature 1.0 --local_index 1 --my_world_size ${my_world_size} &
CUDA_VISIBLE_DEVICES=2 python ./generation/gen_hf2.py --model_name_or_path ${infer_model} --dataset_name_or_path ${prompt_dir} --output_dir ${output_dir} --K 4 --temperature 1.0 --local_index 2 --my_world_size ${my_world_size} &
CUDA_VISIBLE_DEVICES=3 python ./generation/gen_hf2.py --model_name_or_path ${infer_model} --dataset_name_or_path ${prompt_dir} --output_dir ${output_dir} --K 4 --temperature 1.0 --local_index 3 --my_world_size ${my_world_size} &
CUDA_VISIBLE_DEVICES=4 python ./generation/gen_hf2.py --model_name_or_path ${infer_model} --dataset_name_or_path ${prompt_dir} --output_dir ${output_dir} --K 4 --temperature 1.0 --local_index 4 --my_world_size ${my_world_size} &
CUDA_VISIBLE_DEVICES=5 python ./generation/gen_hf2.py --model_name_or_path ${infer_model} --dataset_name_or_path ${prompt_dir} --output_dir ${output_dir} --K 4 --temperature 1.0 --local_index 5 --my_world_size ${my_world_size} &
CUDA_VISIBLE_DEVICES=6 python ./generation/gen_hf2.py --model_name_or_path ${infer_model} --dataset_name_or_path ${prompt_dir} --output_dir ${output_dir} --K 4 --temperature 1.0 --local_index 6 --my_world_size ${my_world_size} &
CUDA_VISIBLE_DEVICES=7 python ./generation/gen_hf2.py --model_name_or_path ${infer_model} --dataset_name_or_path ${prompt_dir} --output_dir ${output_dir} --K 4 --temperature 1.0 --local_index 7 --my_world_size ${my_world_size} &
# then, we merge the 8 datasets into one dataset.
wait
python ./generation/merge_data.py --base_path ${output_dir} --output_dir ./data/gen_data.json --num_datasets ${my_world_size}
We can also use API server to generate new responses.
mkdir data
conda activate vllm
# register the api server
bash ./generation/register_server.sh RLHFlow/LLaMA3-SFT
# start to generate
python ./generation/gen_hf.py --ports 8000 8001 8002 8003 8004 8005 8006 8007 --tokenizer RLHFlow/LLaMA3-SFT --dataset_name_or_path RLHFlow/test_generation_2k --output_dir ./data/gen_data.jsonl --K 4 --temperature 1.0
Then, we call the reward/preference model trained in step 2 to rank the generated responses.
accelerate launch ./annotate_data/get_rewards.py --dataset_name_or_path ./data/gen_data.jsonl --output_dir ./data/data_with_rewards.jsonl --K 4
If you encounter error ``TypeError: Got unsupported ScalarType BFloat16'', considering adjusting your transformer version.
conda activate rlhflow
accelerate launch --config_file ./configs/zero2.yaml dpo_iteration/run_dpo.py ./configs/training.yaml
If you encounter ``RuntimeError: CUDA error: invalid device ordinal, CUDA kernel errors might be asynchronously reported at some other API call'', you need to adjust num_of_process in the config file according to your GPUs.
We put everything together so that the iterative training can run automatically. Note that we set sleep 1m to wait for registering the API for inference. You may need to adjust this parameter according to your environment.
bash run_loop2.sh
The authors would like to thank the great open-source communities, including the Huggingface TRL team, the Huggingface H4 team, the Allen Institute AI RewardBench team, the Meta LLaMA team, evalplus team and Axolotl team for sharing the models, codes, and training sets.
If you find the content of this repo useful, please consider cite it as follows:
@misc{dong2024rlhf,
title={RLHF Workflow: From Reward Modeling to Online RLHF},
author={Hanze Dong and Wei Xiong and Bo Pang and Haoxiang Wang and Han Zhao and Yingbo Zhou and Nan Jiang and Doyen Sahoo and Caiming Xiong and Tong Zhang},
year={2024},
eprint={2405.07863},
archivePrefix={arXiv},
primaryClass={cs.LG}
}
@inproceedings{xiong2023iterative,
title={Iterative preference learning from human feedback: Bridging theory and practice for RLHF under KL-constraint},
author={Xiong, Wei and Dong, Hanze and Ye, Chenlu and Wang, Ziqi and Zhong, Han and Ji, Heng and Jiang, Nan and Zhang, Tong},
booktitle={ICLR 2024 Workshop on Mathematical and Empirical Understanding of Foundation Models}
}
For Tasks:
Click tags to check more tools for each tasksFor Jobs:
Alternative AI tools for Online-RLHF
Similar Open Source Tools
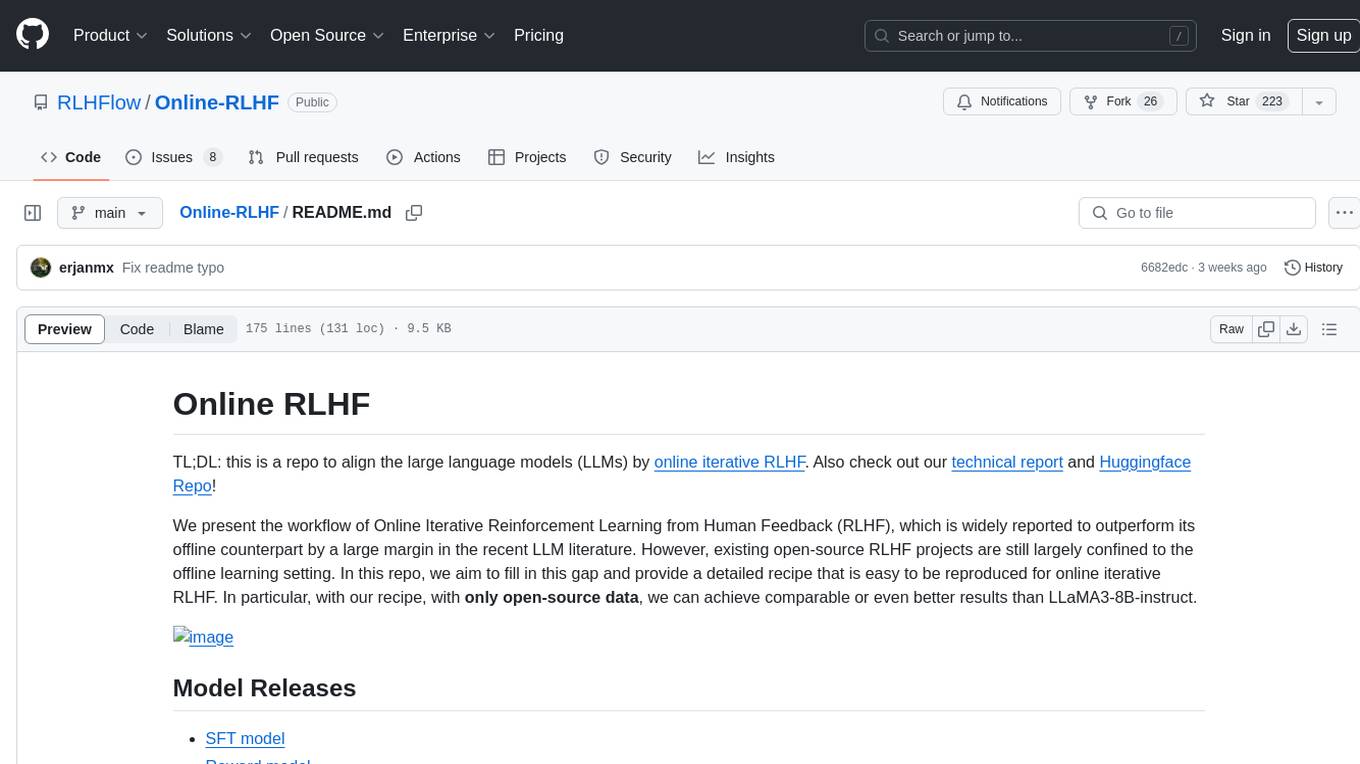
Online-RLHF
This repository, Online RLHF, focuses on aligning large language models (LLMs) through online iterative Reinforcement Learning from Human Feedback (RLHF). It aims to bridge the gap in existing open-source RLHF projects by providing a detailed recipe for online iterative RLHF. The workflow presented here has shown to outperform offline counterparts in recent LLM literature, achieving comparable or better results than LLaMA3-8B-instruct using only open-source data. The repository includes model releases for SFT, Reward model, and RLHF model, along with installation instructions for both inference and training environments. Users can follow step-by-step guidance for supervised fine-tuning, reward modeling, data generation, data annotation, and training, ultimately enabling iterative training to run automatically.
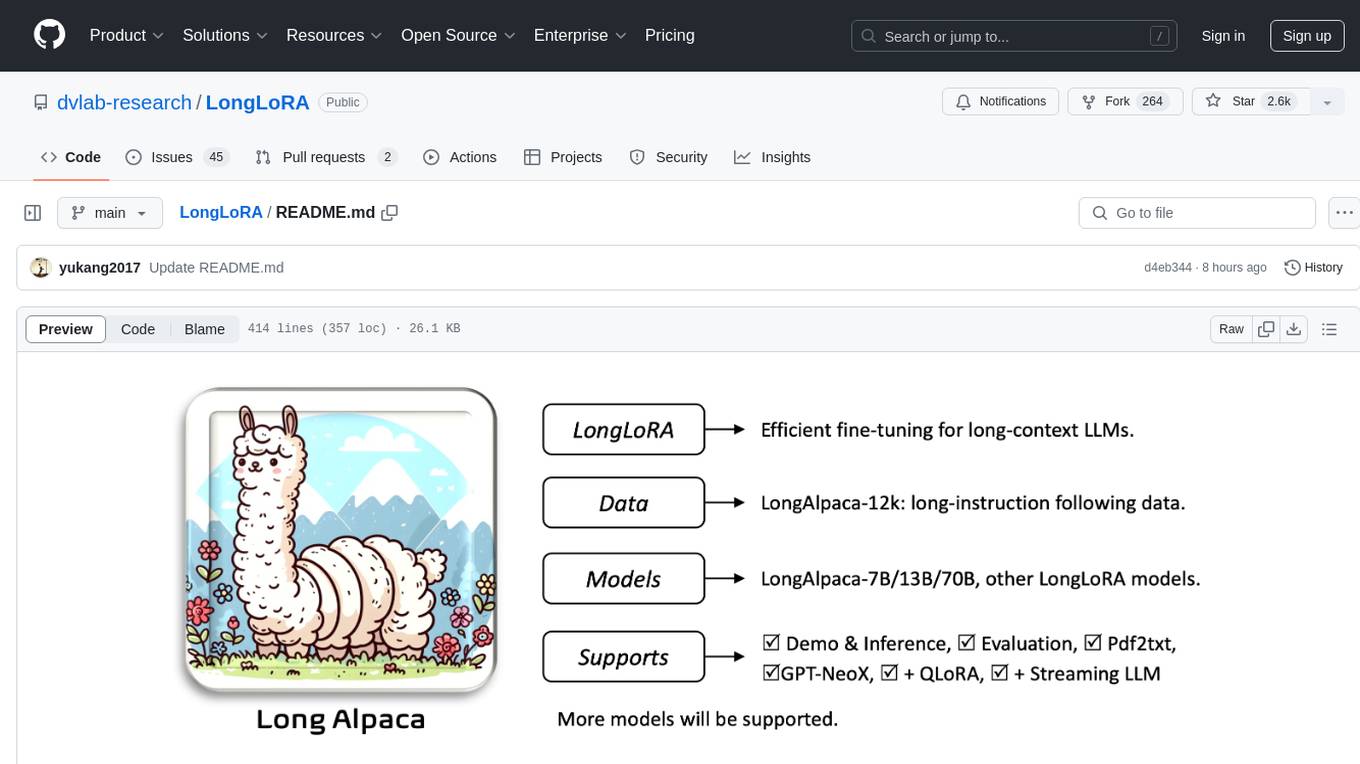
LongLoRA
LongLoRA is a tool for efficient fine-tuning of long-context large language models. It includes LongAlpaca data with long QA data collected and short QA sampled, models from 7B to 70B with context length from 8k to 100k, and support for GPTNeoX models. The tool supports supervised fine-tuning, context extension, and improved LoRA fine-tuning. It provides pre-trained weights, fine-tuning instructions, evaluation methods, local and online demos, streaming inference, and data generation via Pdf2text. LongLoRA is licensed under Apache License 2.0, while data and weights are under CC-BY-NC 4.0 License for research use only.
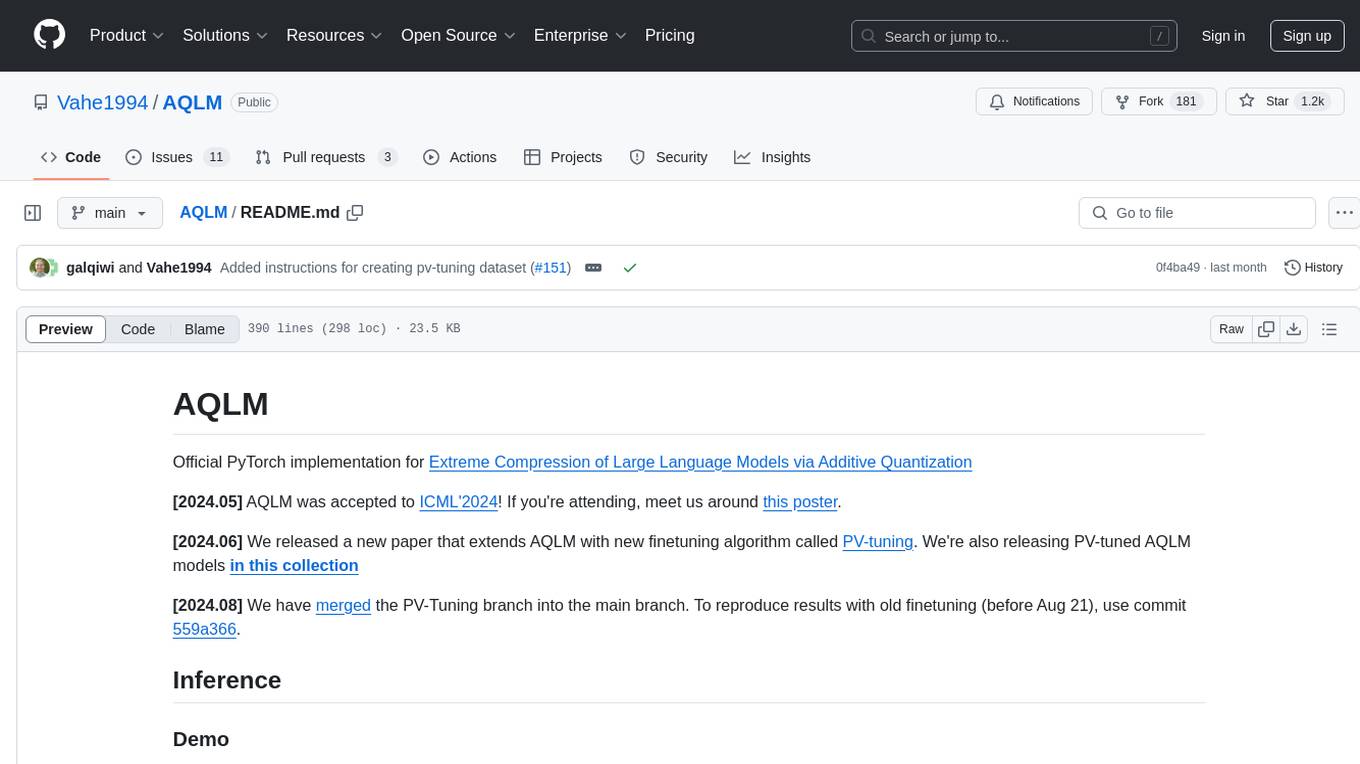
AQLM
AQLM is the official PyTorch implementation for Extreme Compression of Large Language Models via Additive Quantization. It includes prequantized AQLM models without PV-Tuning and PV-Tuned models for LLaMA, Mistral, and Mixtral families. The repository provides inference examples, model details, and quantization setups. Users can run prequantized models using Google Colab examples, work with different model families, and install the necessary inference library. The repository also offers detailed instructions for quantization, fine-tuning, and model evaluation. AQLM quantization involves calibrating models for compression, and users can improve model accuracy through finetuning. Additionally, the repository includes information on preparing models for inference and contributing guidelines.
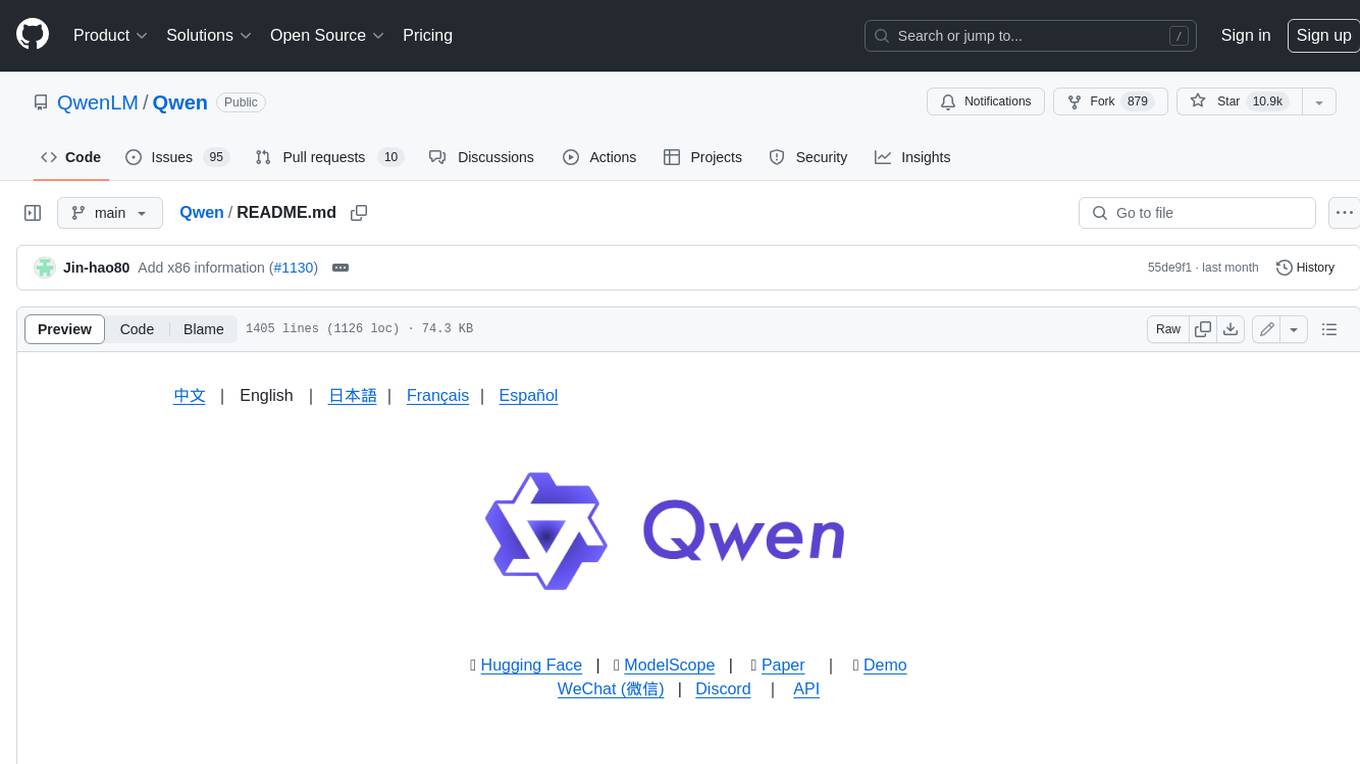
Qwen
Qwen is a series of large language models developed by Alibaba DAMO Academy. It outperforms the baseline models of similar model sizes on a series of benchmark datasets, e.g., MMLU, C-Eval, GSM8K, MATH, HumanEval, MBPP, BBH, etc., which evaluate the models’ capabilities on natural language understanding, mathematic problem solving, coding, etc. Qwen models outperform the baseline models of similar model sizes on a series of benchmark datasets, e.g., MMLU, C-Eval, GSM8K, MATH, HumanEval, MBPP, BBH, etc., which evaluate the models’ capabilities on natural language understanding, mathematic problem solving, coding, etc. Qwen-72B achieves better performance than LLaMA2-70B on all tasks and outperforms GPT-3.5 on 7 out of 10 tasks.
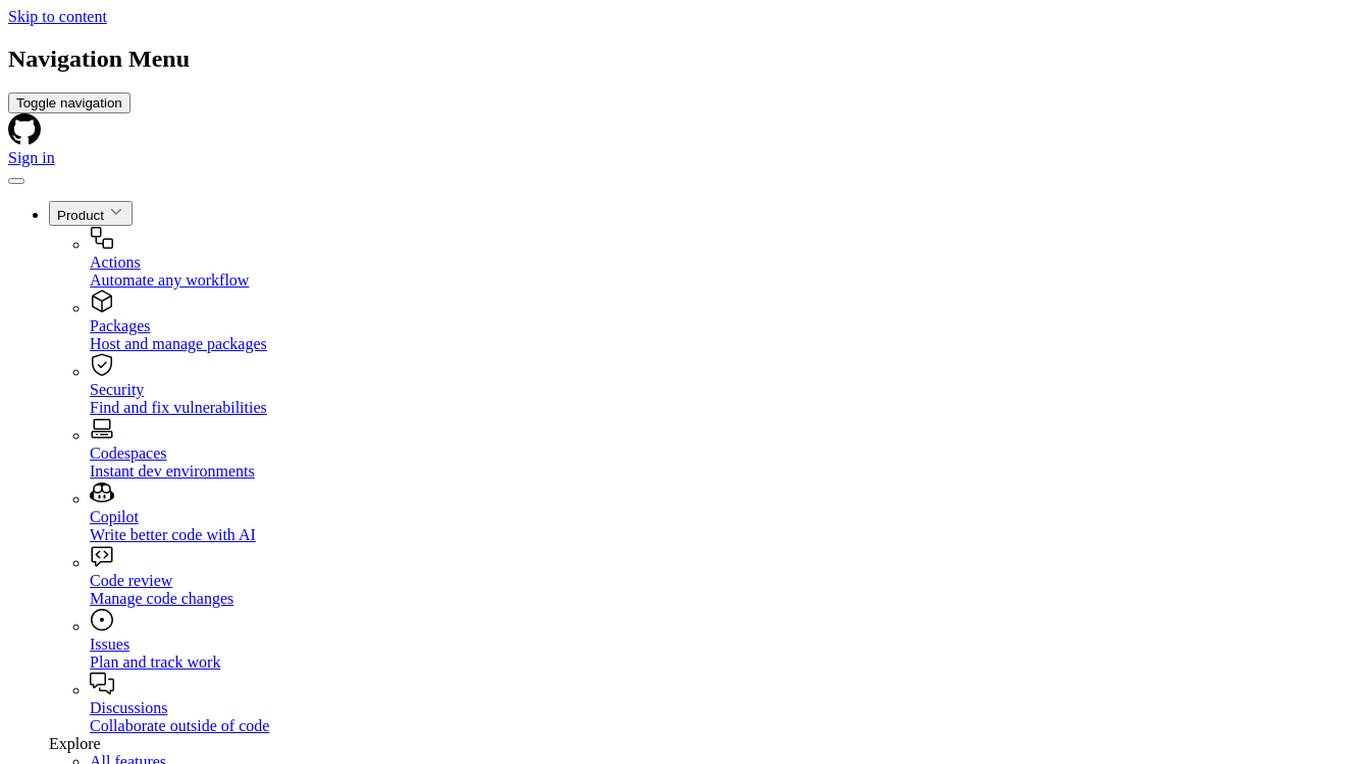
cyclops
Cyclops is a toolkit for facilitating research and deployment of ML models for healthcare. It provides a few high-level APIs namely: data - Create datasets for training, inference and evaluation. We use the popular 🤗 datasets to efficiently load and slice different modalities of data models - Use common model implementations using scikit-learn and PyTorch tasks - Use common ML task formulations such as binary classification or multi-label classification on tabular, time-series and image data evaluate - Evaluate models on clinical prediction tasks monitor - Detect dataset shift relevant for clinical use cases report - Create model report cards for clinical ML models
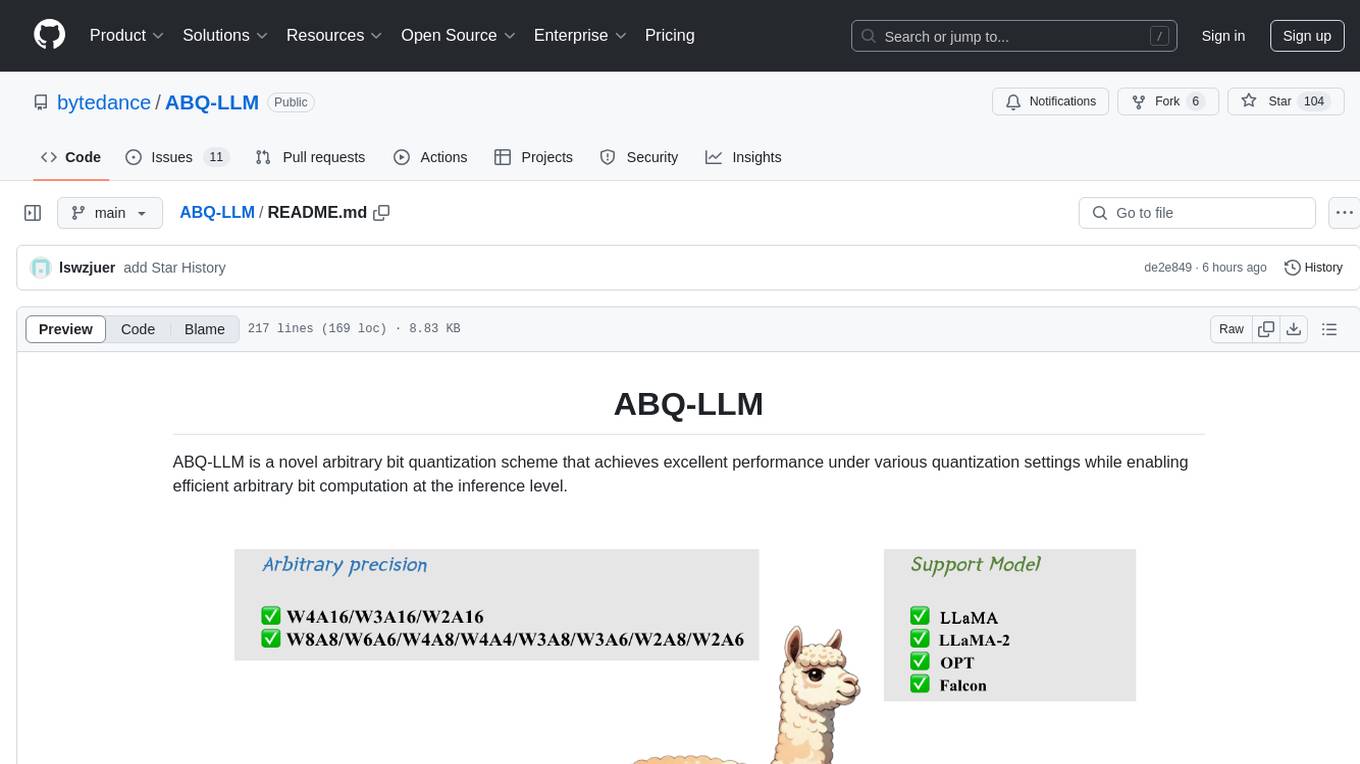
ABQ-LLM
ABQ-LLM is a novel arbitrary bit quantization scheme that achieves excellent performance under various quantization settings while enabling efficient arbitrary bit computation at the inference level. The algorithm supports precise weight-only quantization and weight-activation quantization. It provides pre-trained model weights and a set of out-of-the-box quantization operators for arbitrary bit model inference in modern architectures.
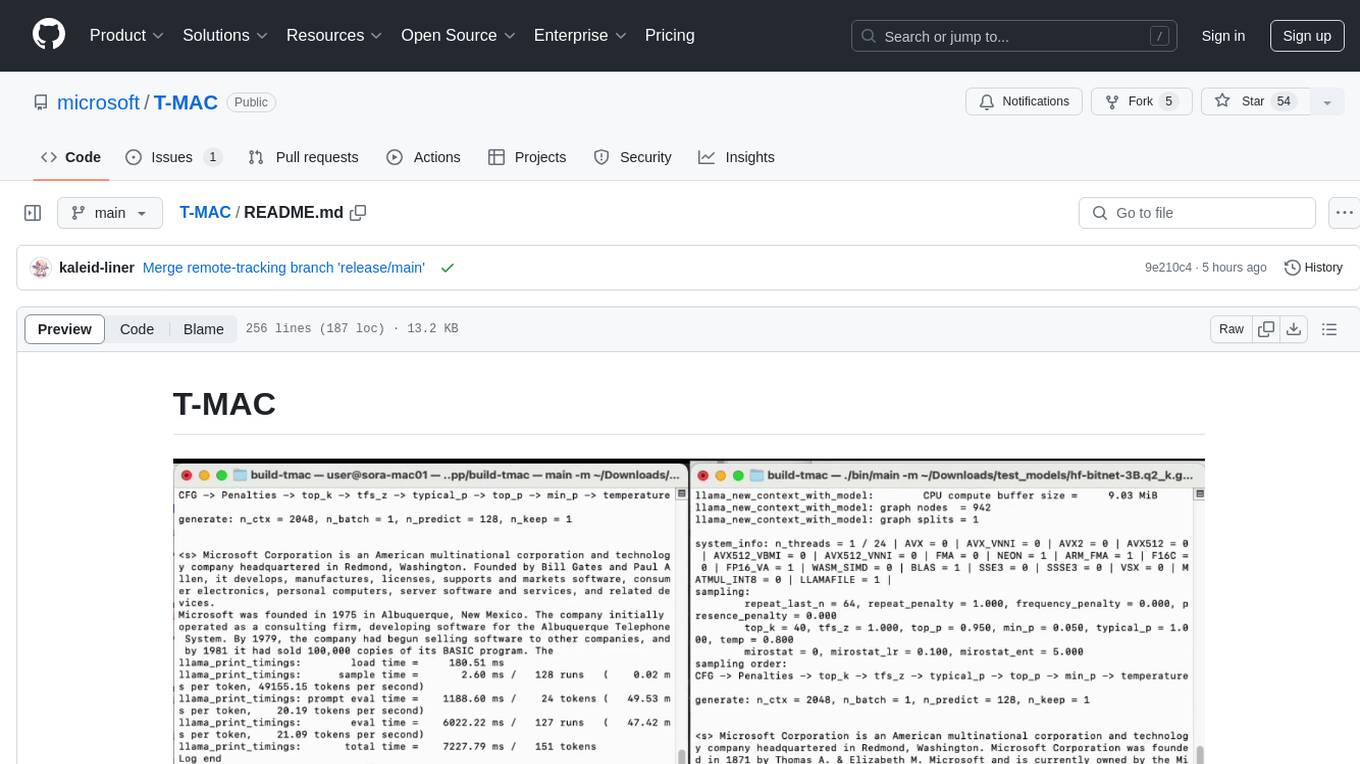
T-MAC
T-MAC is a kernel library that directly supports mixed-precision matrix multiplication without the need for dequantization by utilizing lookup tables. It aims to boost low-bit LLM inference on CPUs by offering support for various low-bit models. T-MAC achieves significant speedup compared to SOTA CPU low-bit framework (llama.cpp) and can even perform well on lower-end devices like Raspberry Pi 5. The tool demonstrates superior performance over existing low-bit GEMM kernels on CPU, reduces power consumption, and provides energy savings. It achieves comparable performance to CUDA GPU on certain tasks while delivering considerable power and energy savings. T-MAC's method involves using lookup tables to support mpGEMM and employs key techniques like precomputing partial sums, shift and accumulate operations, and utilizing tbl/pshuf instructions for fast table lookup.
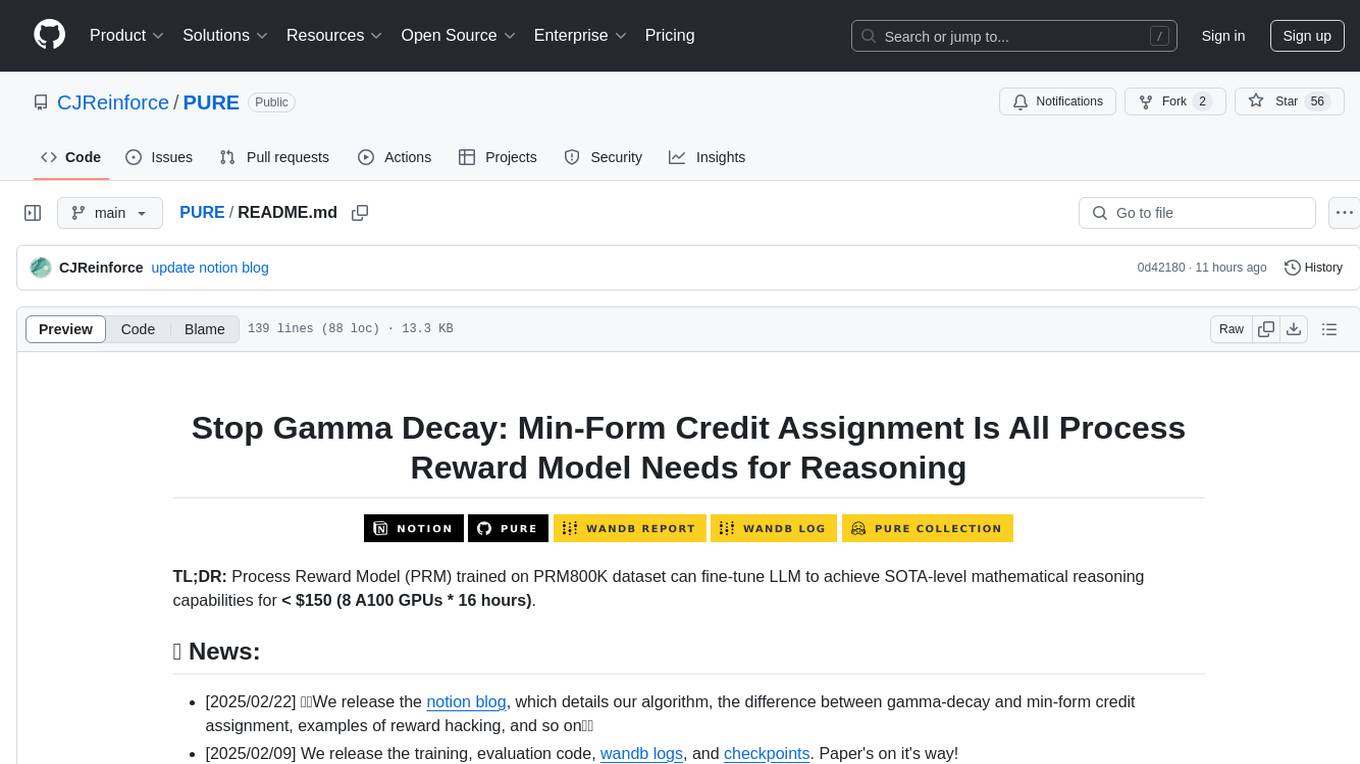
PURE
PURE (Process-sUpervised Reinforcement lEarning) is a framework that trains a Process Reward Model (PRM) on a dataset and fine-tunes a language model to achieve state-of-the-art mathematical reasoning capabilities. It uses a novel credit assignment method to calculate return and supports multiple reward types. The final model outperforms existing methods with minimal RL data or compute resources, achieving high accuracy on various benchmarks. The tool addresses reward hacking issues and aims to enhance long-range decision-making and reasoning tasks using large language models.
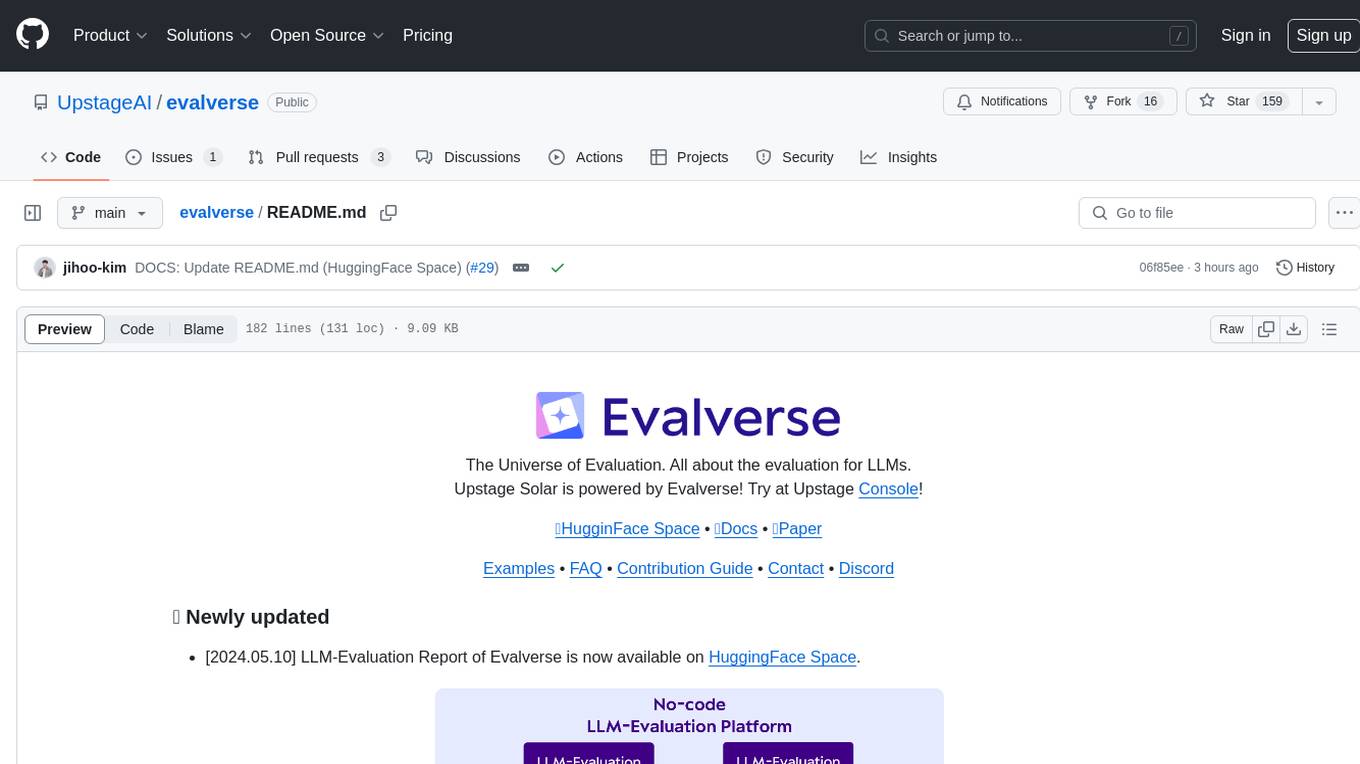
evalverse
Evalverse is an open-source project designed to support Large Language Model (LLM) evaluation needs. It provides a standardized and user-friendly solution for processing and managing LLM evaluations, catering to AI research engineers and scientists. Evalverse supports various evaluation methods, insightful reports, and no-code evaluation processes. Users can access unified evaluation with submodules, request evaluations without code via Slack bot, and obtain comprehensive reports with scores, rankings, and visuals. The tool allows for easy comparison of scores across different models and swift addition of new evaluation tools.
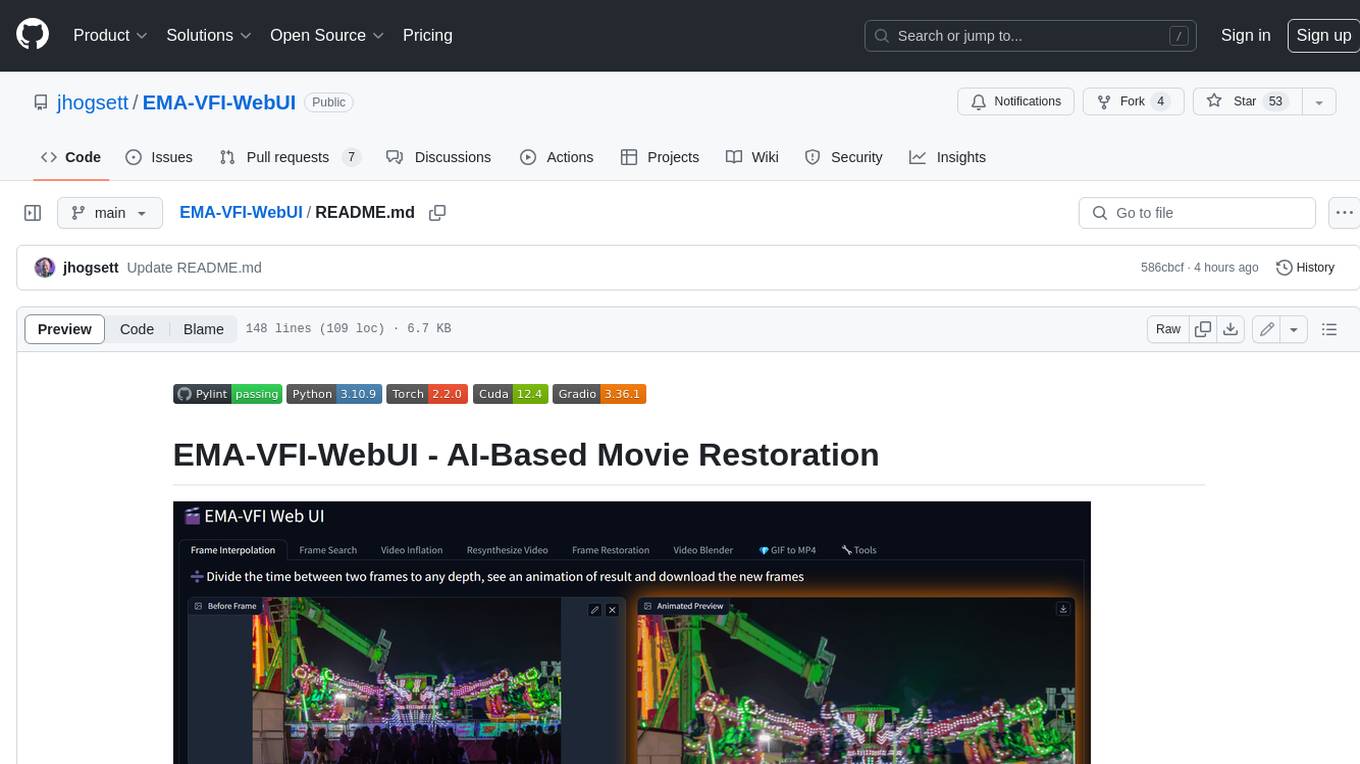
EMA-VFI-WebUI
EMA-VFI-WebUI is a web-based graphical user interface (GUI) for the EMA-VFI AI-based movie restoration tool. It provides a user-friendly interface for accessing the various features of EMA-VFI, including frame interpolation, frame search, video inflation, video resynthesis, frame restoration, video blending, file conversion, file resequencing, FPS conversion, GIF to MP4 conversion, and frame upscaling. The web UI makes it easy to use EMA-VFI's powerful features without having to deal with the command line interface.
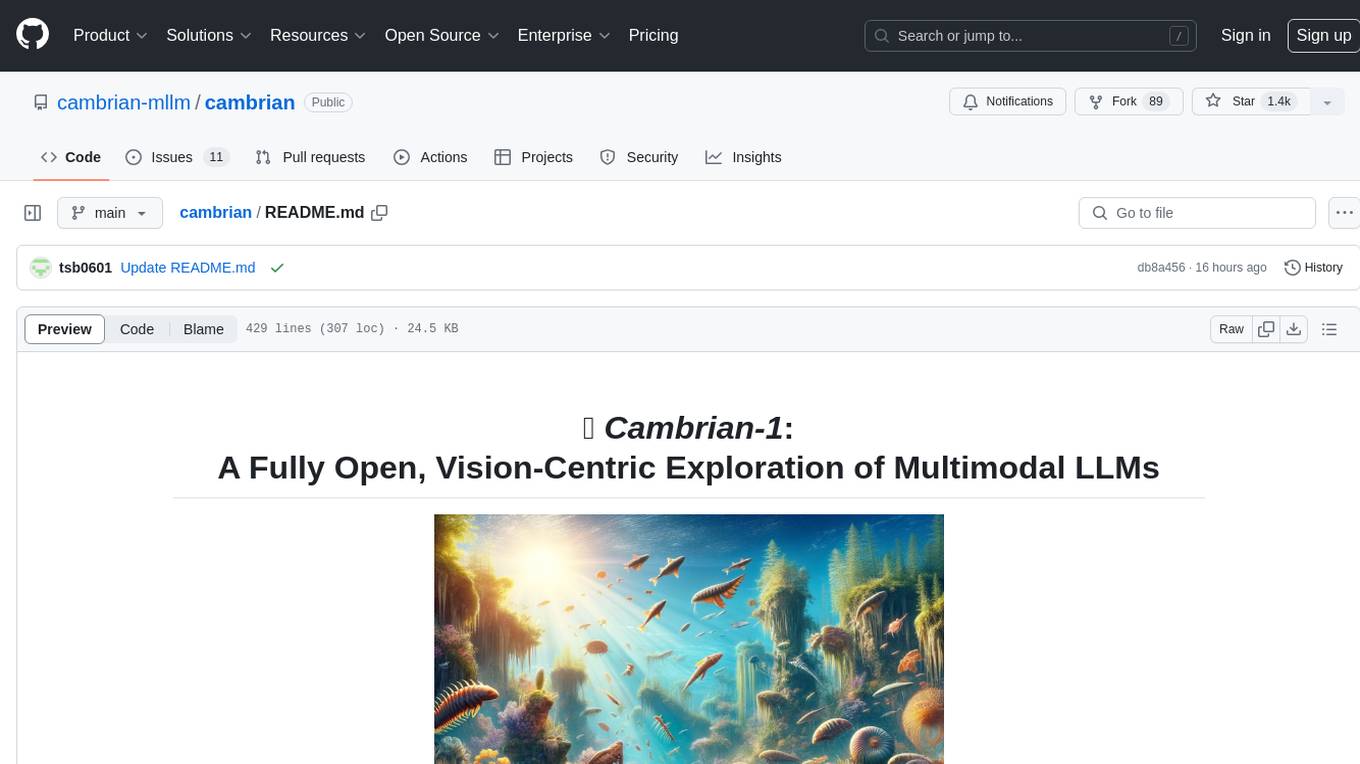
cambrian
Cambrian-1 is a fully open project focused on exploring multimodal Large Language Models (LLMs) with a vision-centric approach. It offers competitive performance across various benchmarks with models at different parameter levels. The project includes training configurations, model weights, instruction tuning data, and evaluation details. Users can interact with Cambrian-1 through a Gradio web interface for inference. The project is inspired by LLaVA and incorporates contributions from Vicuna, LLaMA, and Yi. Cambrian-1 is licensed under Apache 2.0 and utilizes datasets and checkpoints subject to their respective original licenses.
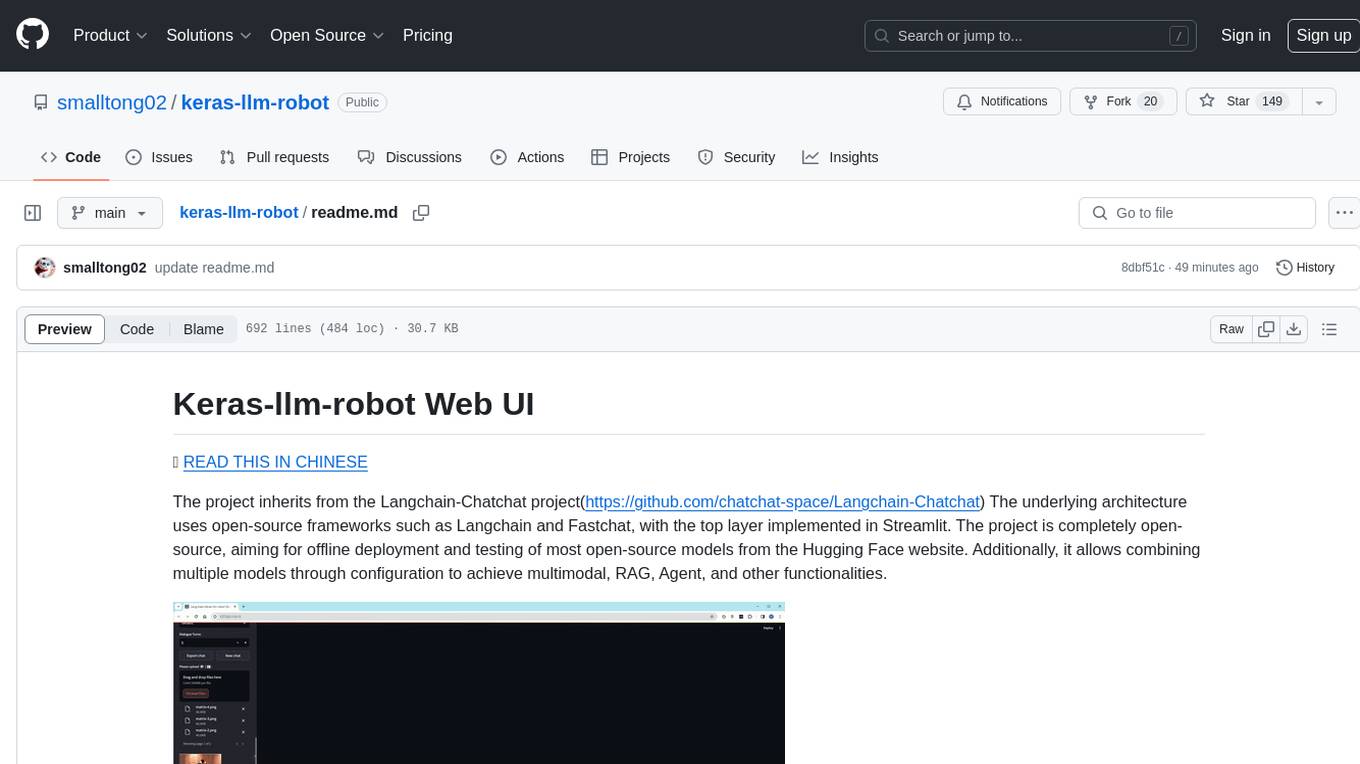
keras-llm-robot
The Keras-llm-robot Web UI project is an open-source tool designed for offline deployment and testing of various open-source models from the Hugging Face website. It allows users to combine multiple models through configuration to achieve functionalities like multimodal, RAG, Agent, and more. The project consists of three main interfaces: chat interface for language models, configuration interface for loading models, and tools & agent interface for auxiliary models. Users can interact with the language model through text, voice, and image inputs, and the tool supports features like model loading, quantization, fine-tuning, role-playing, code interpretation, speech recognition, image recognition, network search engine, and function calling.
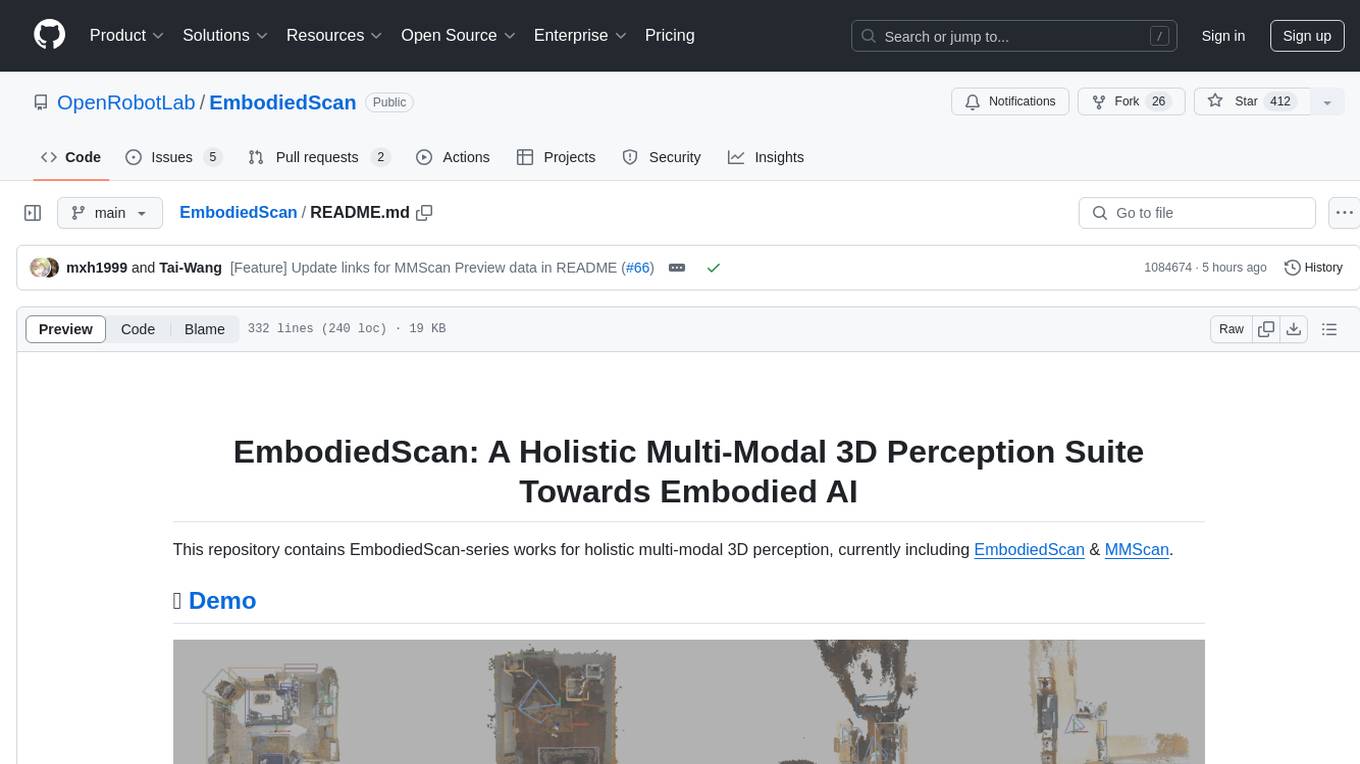
EmbodiedScan
EmbodiedScan is a holistic multi-modal 3D perception suite designed for embodied AI. It introduces a multi-modal, ego-centric 3D perception dataset and benchmark for holistic 3D scene understanding. The dataset includes over 5k scans with 1M ego-centric RGB-D views, 1M language prompts, 160k 3D-oriented boxes spanning 760 categories, and dense semantic occupancy with 80 common categories. The suite includes a baseline framework named Embodied Perceptron, capable of processing multi-modal inputs for 3D perception tasks and language-grounded tasks.
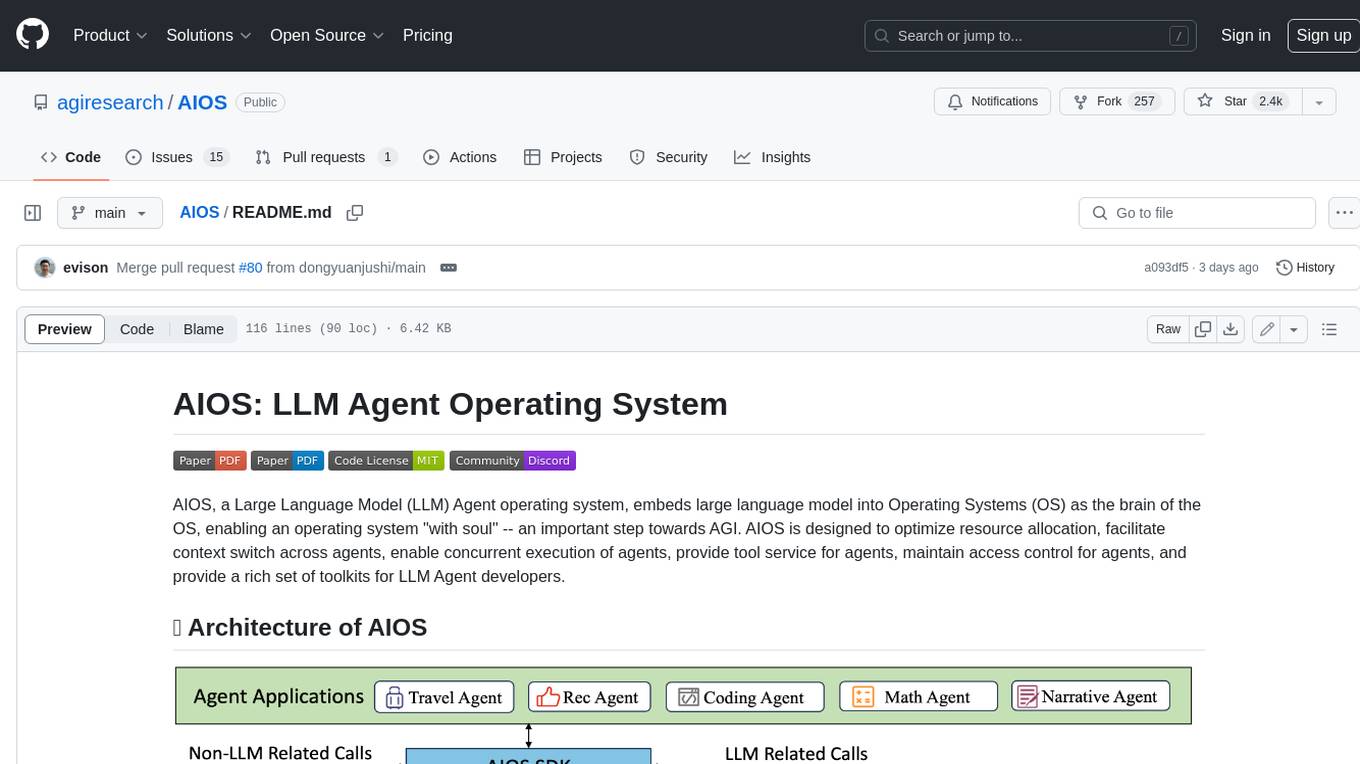
AIOS
AIOS, a Large Language Model (LLM) Agent operating system, embeds large language model into Operating Systems (OS) as the brain of the OS, enabling an operating system "with soul" -- an important step towards AGI. AIOS is designed to optimize resource allocation, facilitate context switch across agents, enable concurrent execution of agents, provide tool service for agents, maintain access control for agents, and provide a rich set of toolkits for LLM Agent developers.
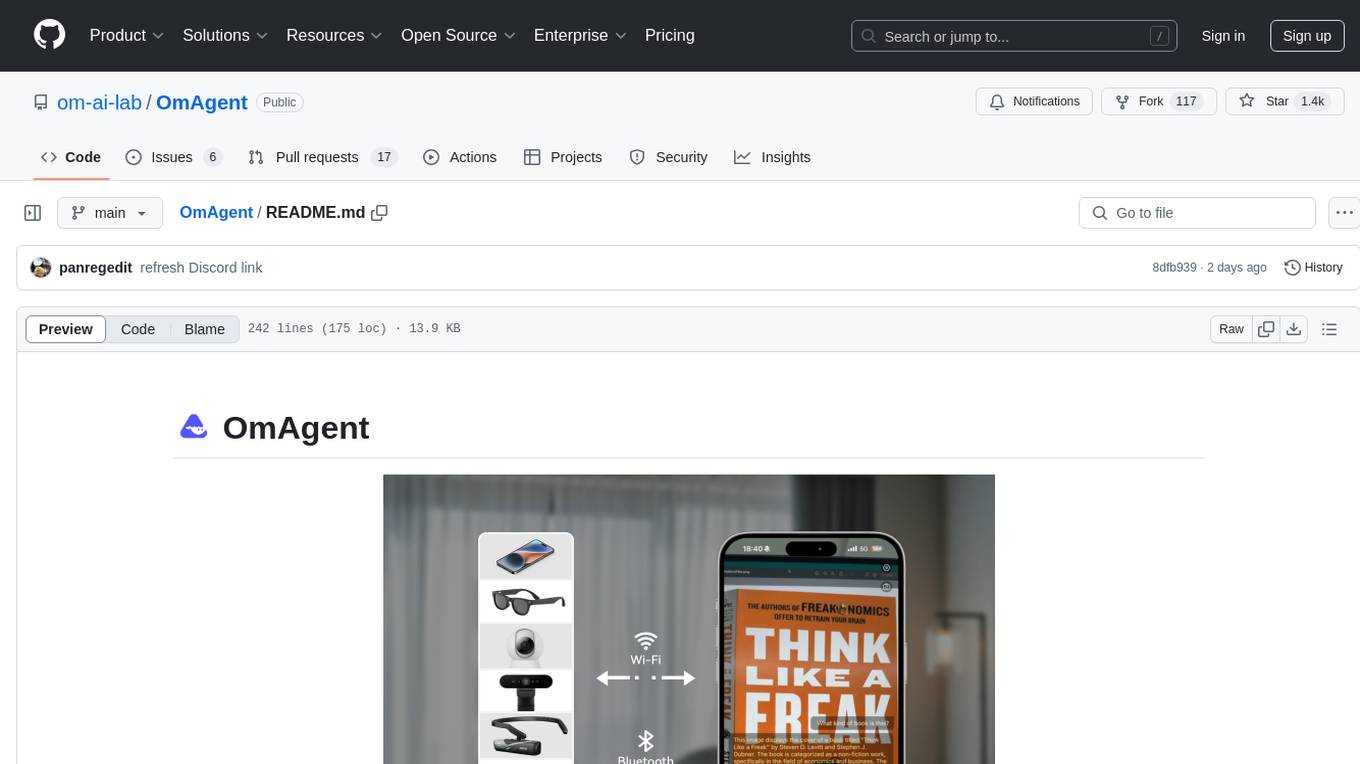
OmAgent
OmAgent is an open-source agent framework designed to streamline the development of on-device multimodal agents. It enables agents to empower various hardware devices, integrates speed-optimized SOTA multimodal models, provides SOTA multimodal agent algorithms, and focuses on optimizing the end-to-end computing pipeline for real-time user interaction experience. Key features include easy connection to diverse devices, scalability, flexibility, and workflow orchestration. The architecture emphasizes graph-based workflow orchestration, native multimodality, and device-centricity, allowing developers to create bespoke intelligent agent programs.
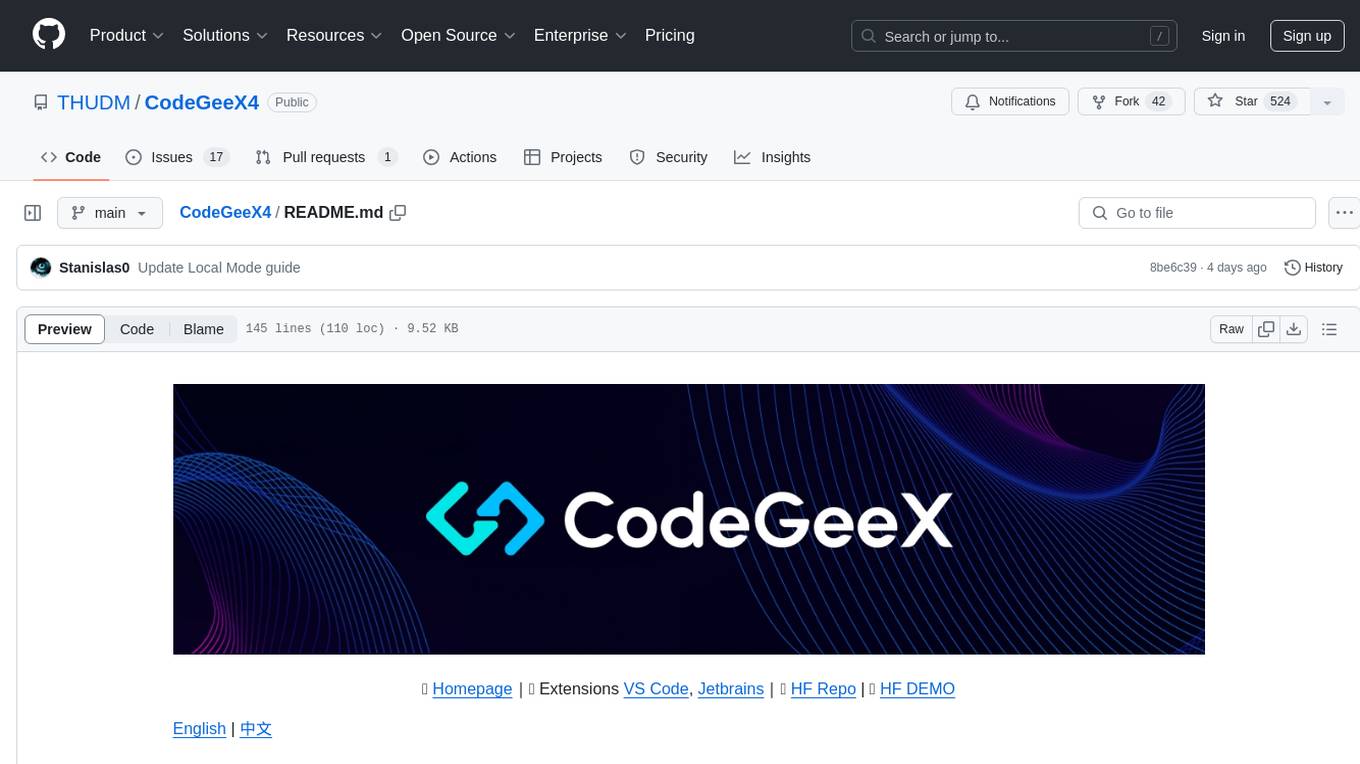
CodeGeeX4
CodeGeeX4-ALL-9B is an open-source multilingual code generation model based on GLM-4-9B, offering enhanced code generation capabilities. It supports functions like code completion, code interpreter, web search, function call, and repository-level code Q&A. The model has competitive performance on benchmarks like BigCodeBench and NaturalCodeBench, outperforming larger models in terms of speed and performance.
For similar tasks
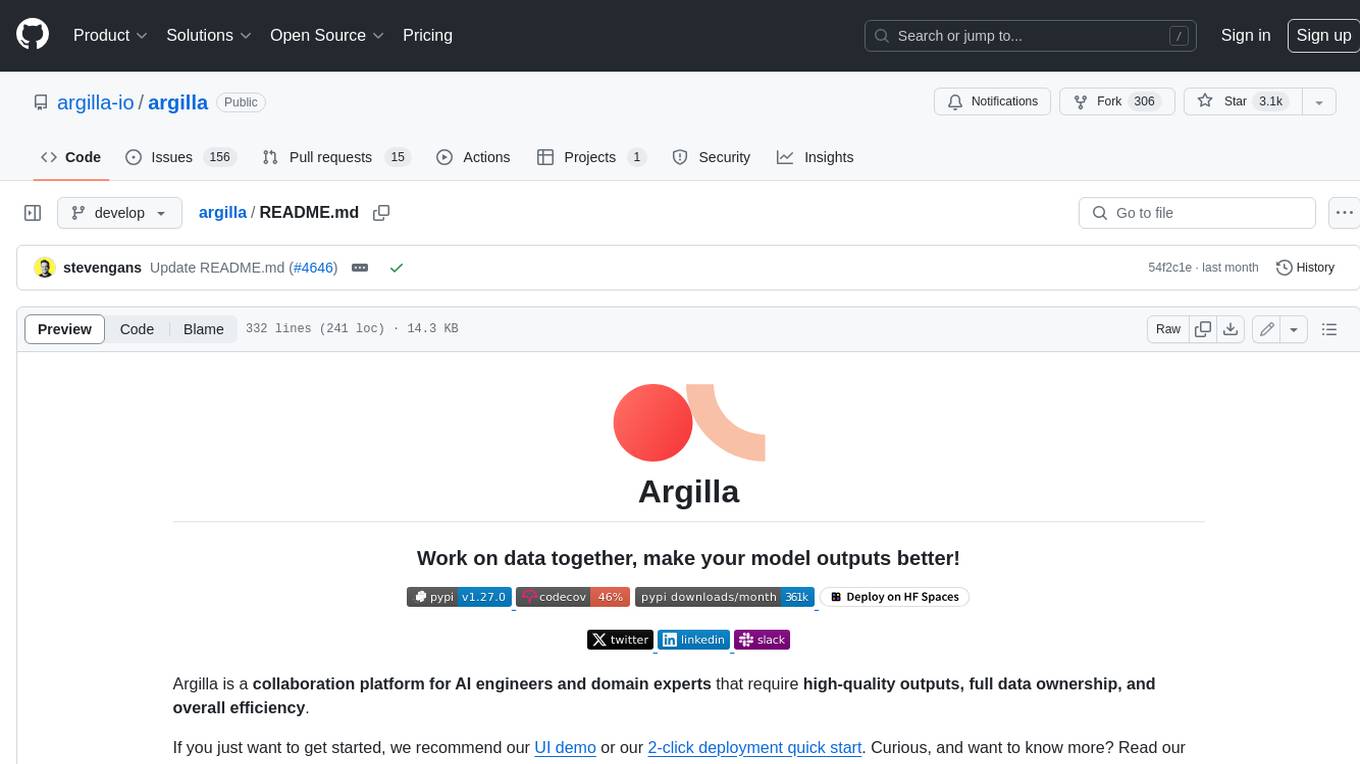
argilla
Argilla is a collaboration platform for AI engineers and domain experts that require high-quality outputs, full data ownership, and overall efficiency. It helps users improve AI output quality through data quality, take control of their data and models, and improve efficiency by quickly iterating on the right data and models. Argilla is an open-source community-driven project that provides tools for achieving and maintaining high-quality data standards, with a focus on NLP and LLMs. It is used by AI teams from companies like the Red Cross, Loris.ai, and Prolific to improve the quality and efficiency of AI projects.
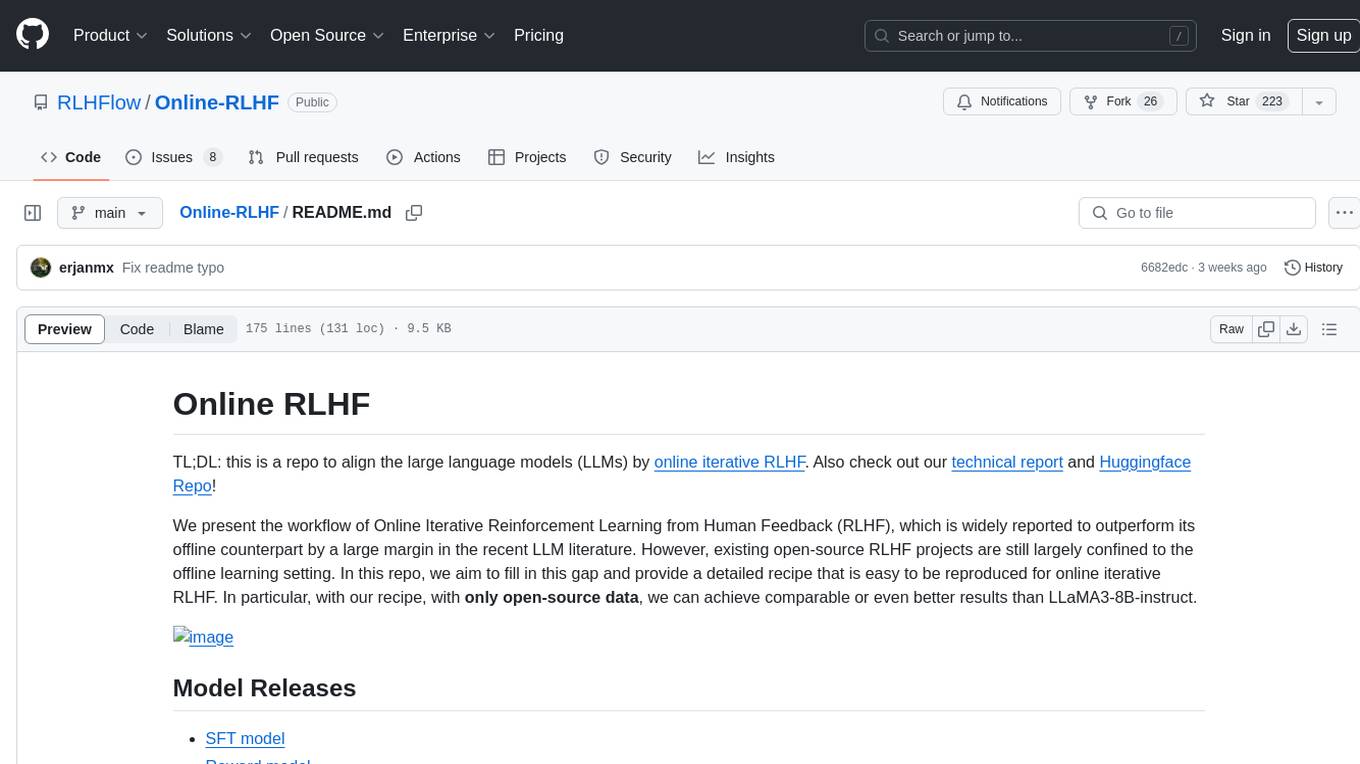
Online-RLHF
This repository, Online RLHF, focuses on aligning large language models (LLMs) through online iterative Reinforcement Learning from Human Feedback (RLHF). It aims to bridge the gap in existing open-source RLHF projects by providing a detailed recipe for online iterative RLHF. The workflow presented here has shown to outperform offline counterparts in recent LLM literature, achieving comparable or better results than LLaMA3-8B-instruct using only open-source data. The repository includes model releases for SFT, Reward model, and RLHF model, along with installation instructions for both inference and training environments. Users can follow step-by-step guidance for supervised fine-tuning, reward modeling, data generation, data annotation, and training, ultimately enabling iterative training to run automatically.
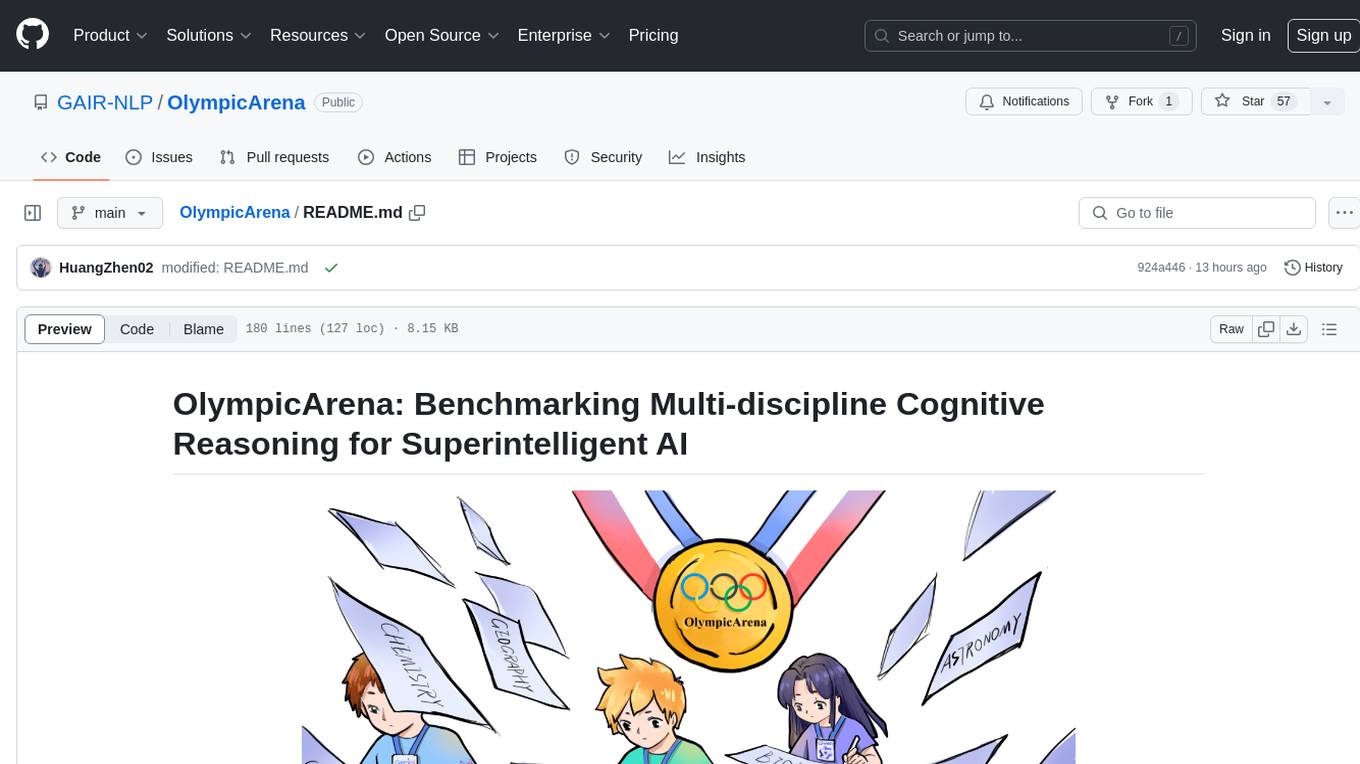
OlympicArena
OlympicArena is a comprehensive benchmark designed to evaluate advanced AI capabilities across various disciplines. It aims to push AI towards superintelligence by tackling complex challenges in science and beyond. The repository provides detailed data for different disciplines, allows users to run inference and evaluation locally, and offers a submission platform for testing models on the test set. Additionally, it includes an annotation interface and encourages users to cite their paper if they find the code or dataset helpful.
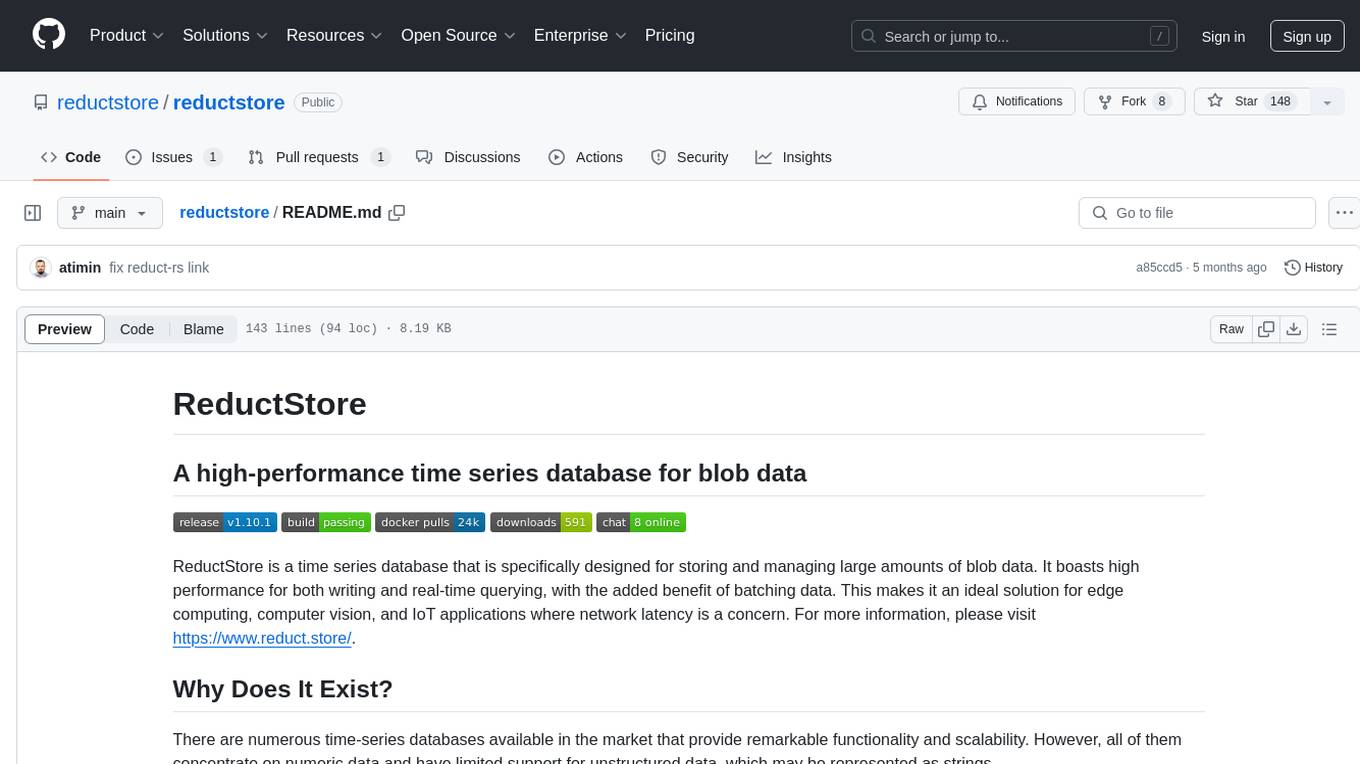
reductstore
ReductStore is a high-performance time series database designed for storing and managing large amounts of unstructured blob data. It offers features such as real-time querying, batching data, and HTTP(S) API for edge computing, computer vision, and IoT applications. The database ensures data integrity, implements retention policies, and provides efficient data access, making it a cost-effective solution for applications requiring unstructured data storage and access at specific time intervals.
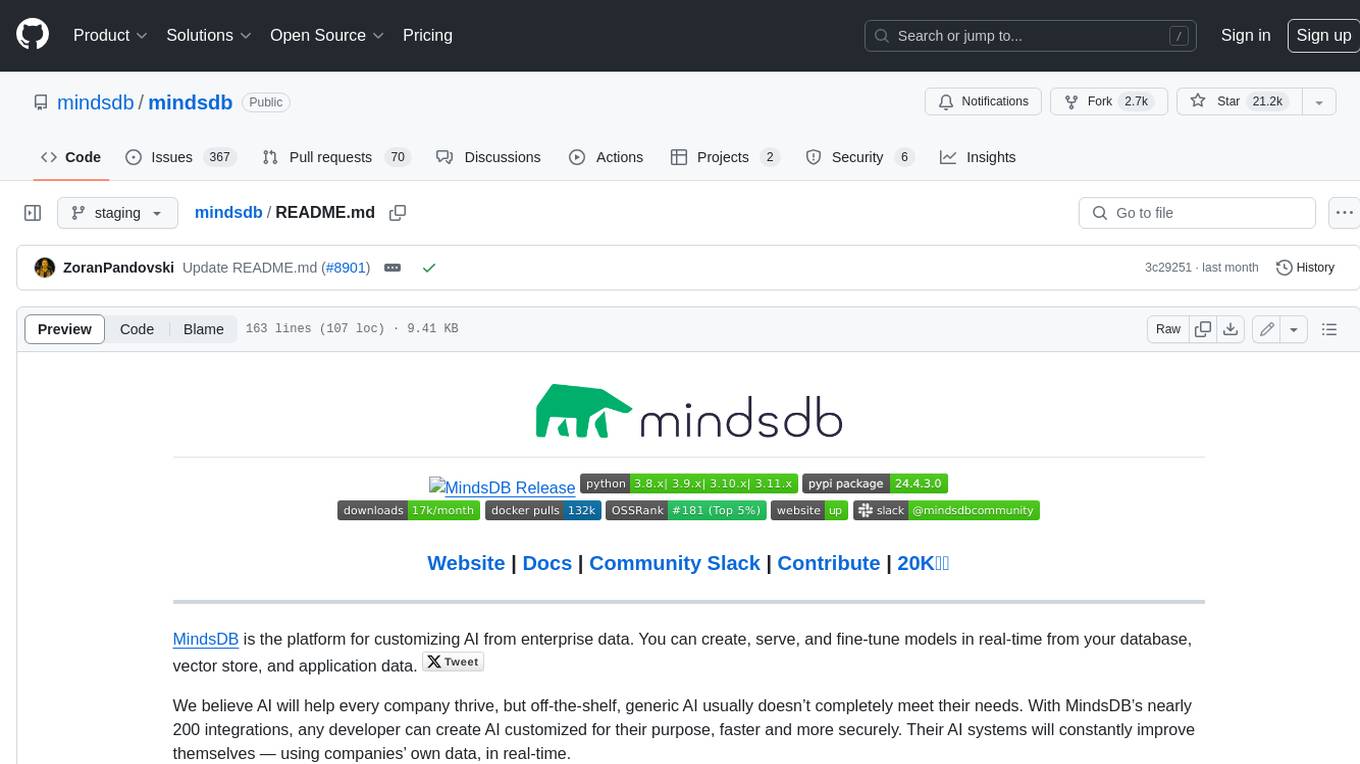
mindsdb
MindsDB is a platform for customizing AI from enterprise data. You can create, serve, and fine-tune models in real-time from your database, vector store, and application data. MindsDB "enhances" SQL syntax with AI capabilities to make it accessible for developers worldwide. With MindsDB’s nearly 200 integrations, any developer can create AI customized for their purpose, faster and more securely. Their AI systems will constantly improve themselves — using companies’ own data, in real-time.
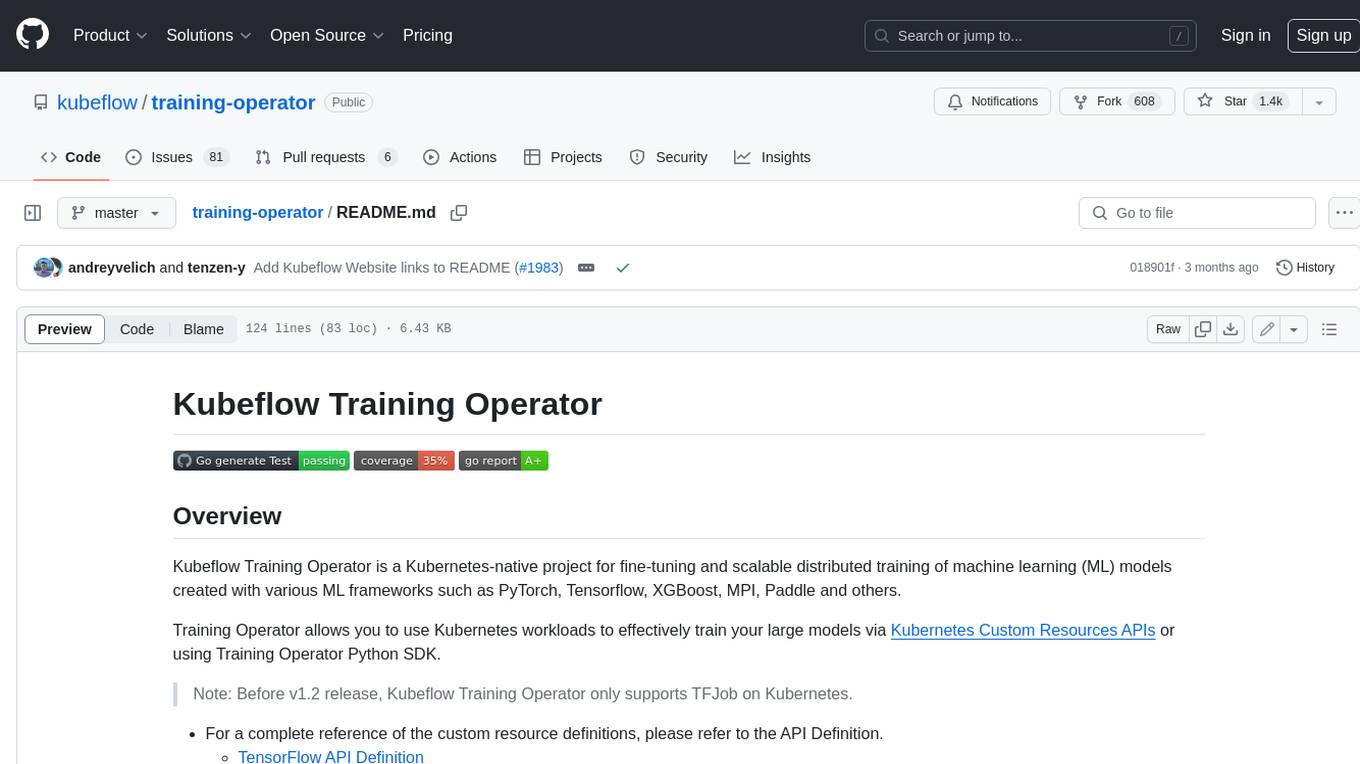
training-operator
Kubeflow Training Operator is a Kubernetes-native project for fine-tuning and scalable distributed training of machine learning (ML) models created with various ML frameworks such as PyTorch, Tensorflow, XGBoost, MPI, Paddle and others. Training Operator allows you to use Kubernetes workloads to effectively train your large models via Kubernetes Custom Resources APIs or using Training Operator Python SDK. > Note: Before v1.2 release, Kubeflow Training Operator only supports TFJob on Kubernetes. * For a complete reference of the custom resource definitions, please refer to the API Definition. * TensorFlow API Definition * PyTorch API Definition * Apache MXNet API Definition * XGBoost API Definition * MPI API Definition * PaddlePaddle API Definition * For details of all-in-one operator design, please refer to the All-in-one Kubeflow Training Operator * For details on its observability, please refer to the monitoring design doc.
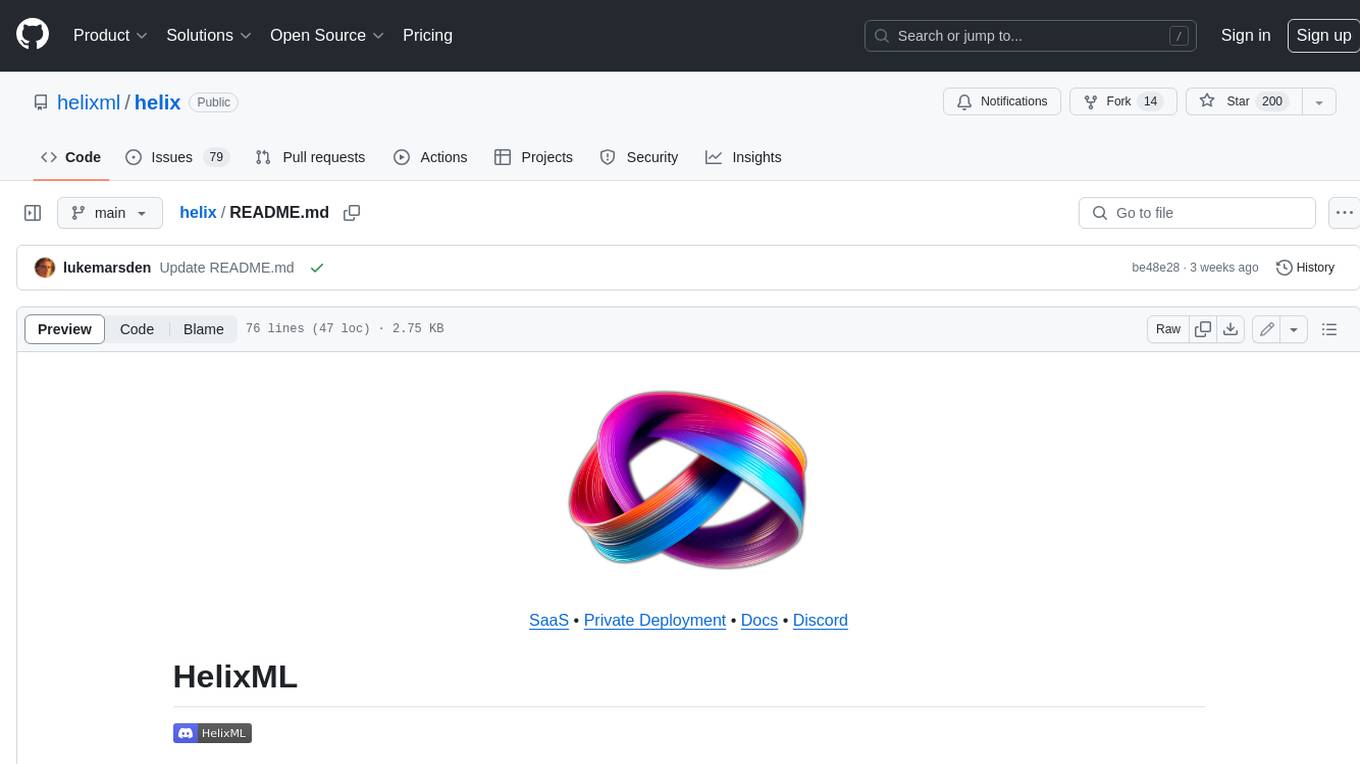
helix
HelixML is a private GenAI platform that allows users to deploy the best of open AI in their own data center or VPC while retaining complete data security and control. It includes support for fine-tuning models with drag-and-drop functionality. HelixML brings the best of open source AI to businesses in an ergonomic and scalable way, optimizing the tradeoff between GPU memory and latency.
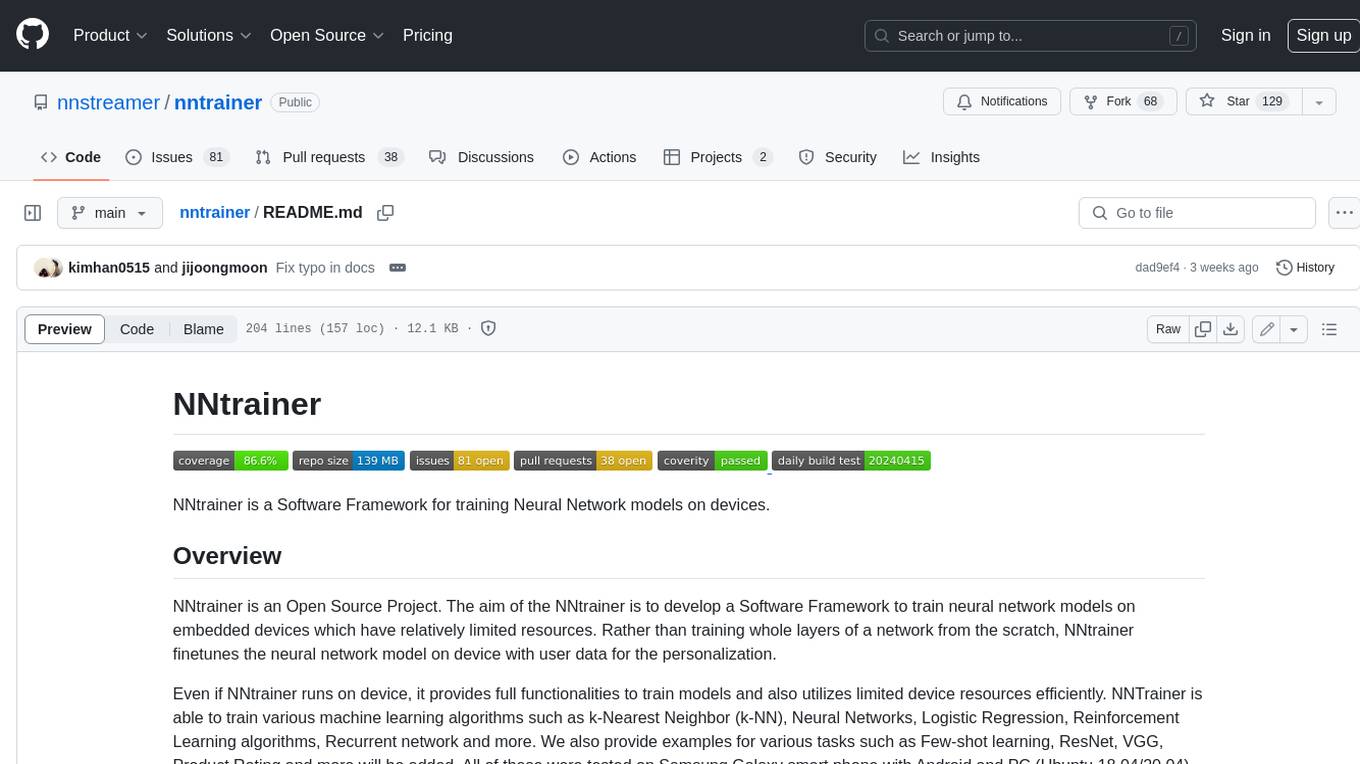
nntrainer
NNtrainer is a software framework for training neural network models on devices with limited resources. It enables on-device fine-tuning of neural networks using user data for personalization. NNtrainer supports various machine learning algorithms and provides examples for tasks such as few-shot learning, ResNet, VGG, and product rating. It is optimized for embedded devices and utilizes CBLAS and CUBLAS for accelerated calculations. NNtrainer is open source and released under the Apache License version 2.0.
For similar jobs
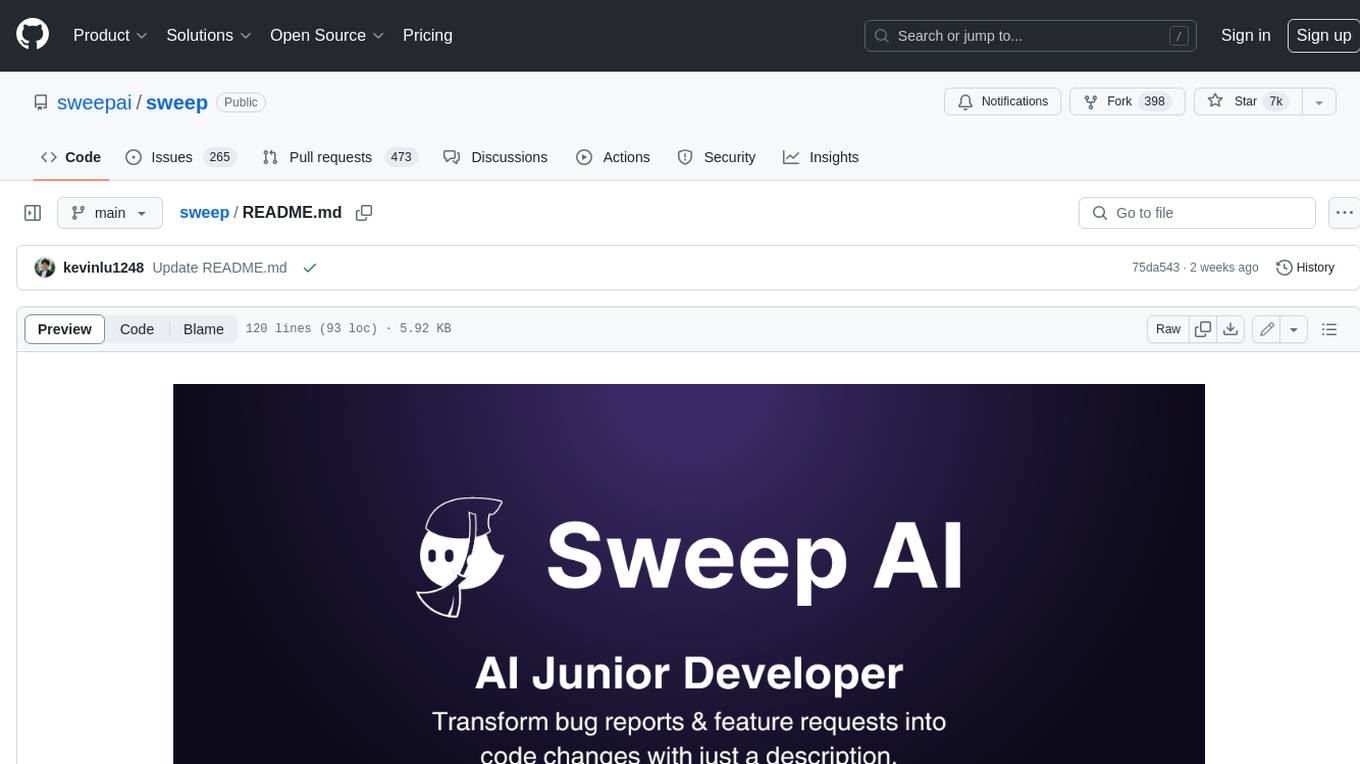
sweep
Sweep is an AI junior developer that turns bugs and feature requests into code changes. It automatically handles developer experience improvements like adding type hints and improving test coverage.
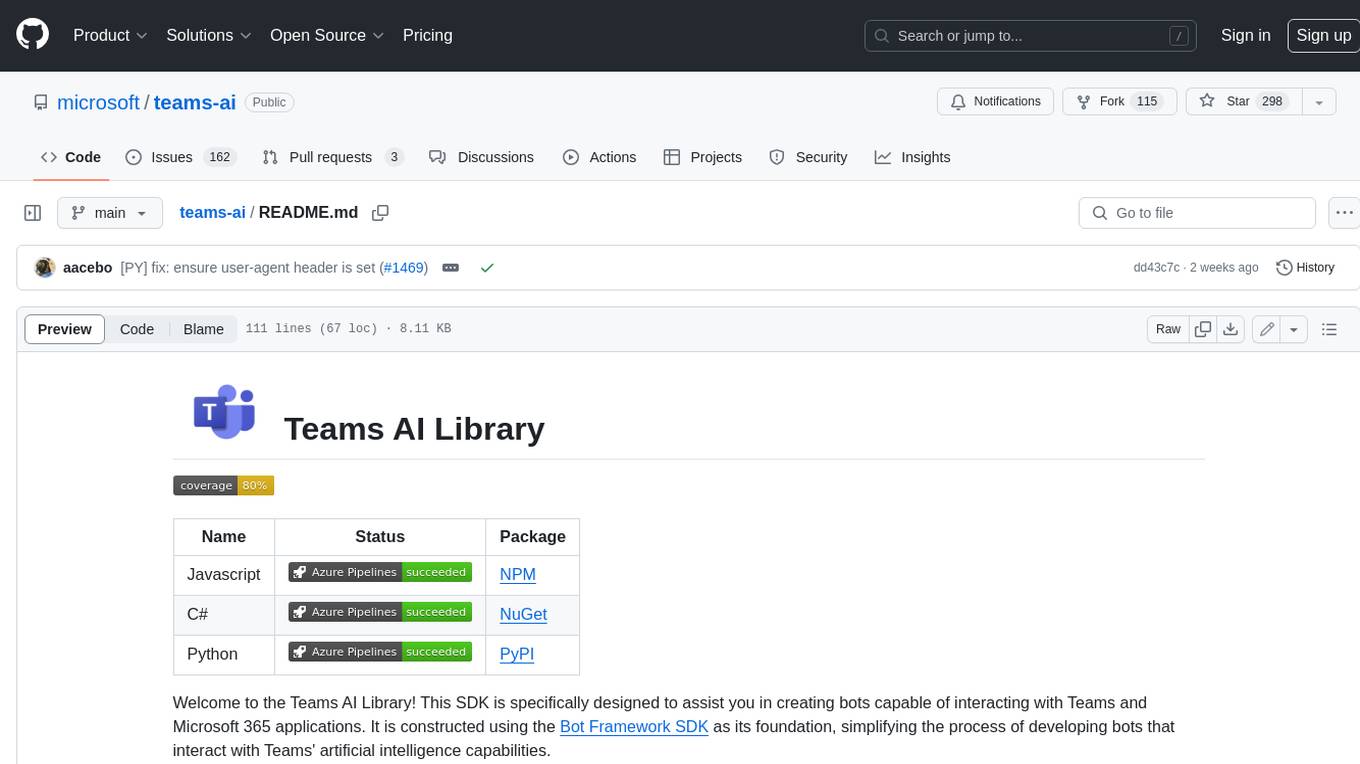
teams-ai
The Teams AI Library is a software development kit (SDK) that helps developers create bots that can interact with Teams and Microsoft 365 applications. It is built on top of the Bot Framework SDK and simplifies the process of developing bots that interact with Teams' artificial intelligence capabilities. The SDK is available for JavaScript/TypeScript, .NET, and Python.
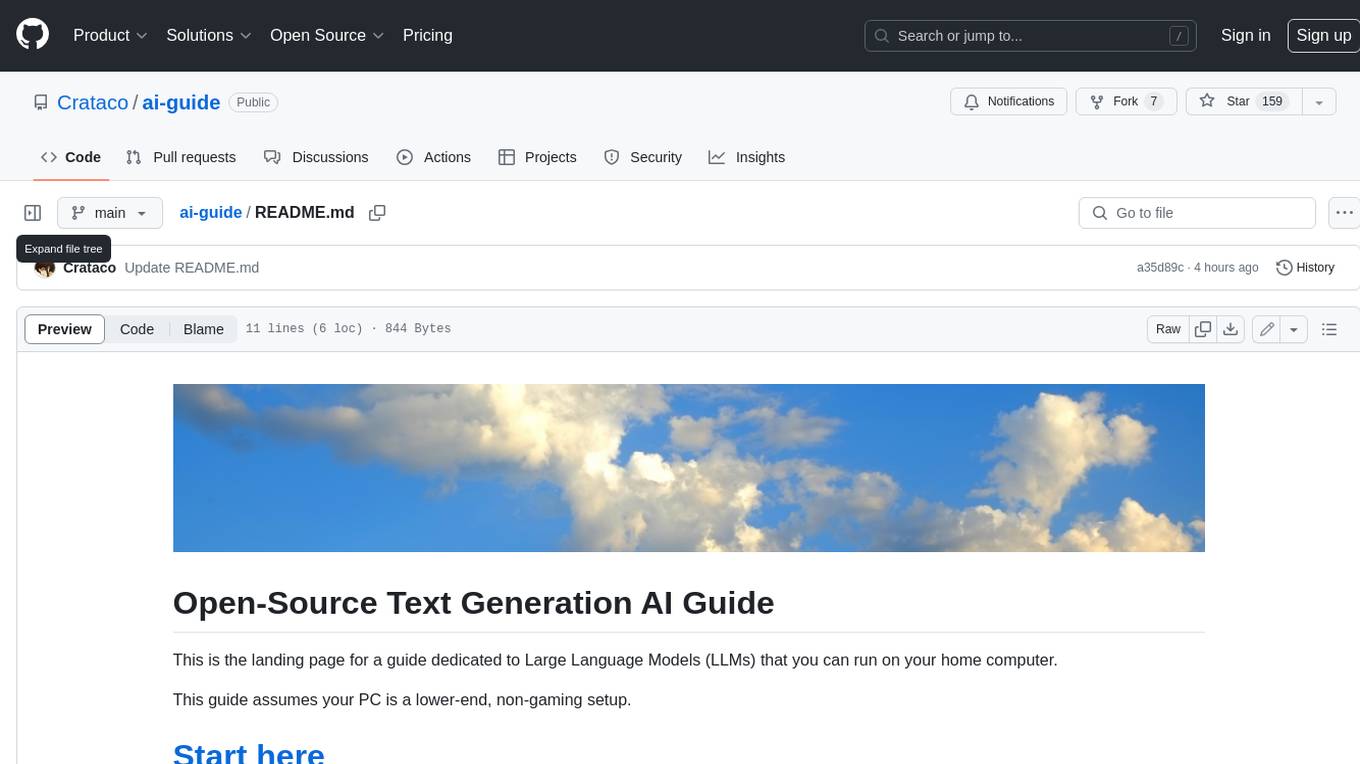
ai-guide
This guide is dedicated to Large Language Models (LLMs) that you can run on your home computer. It assumes your PC is a lower-end, non-gaming setup.
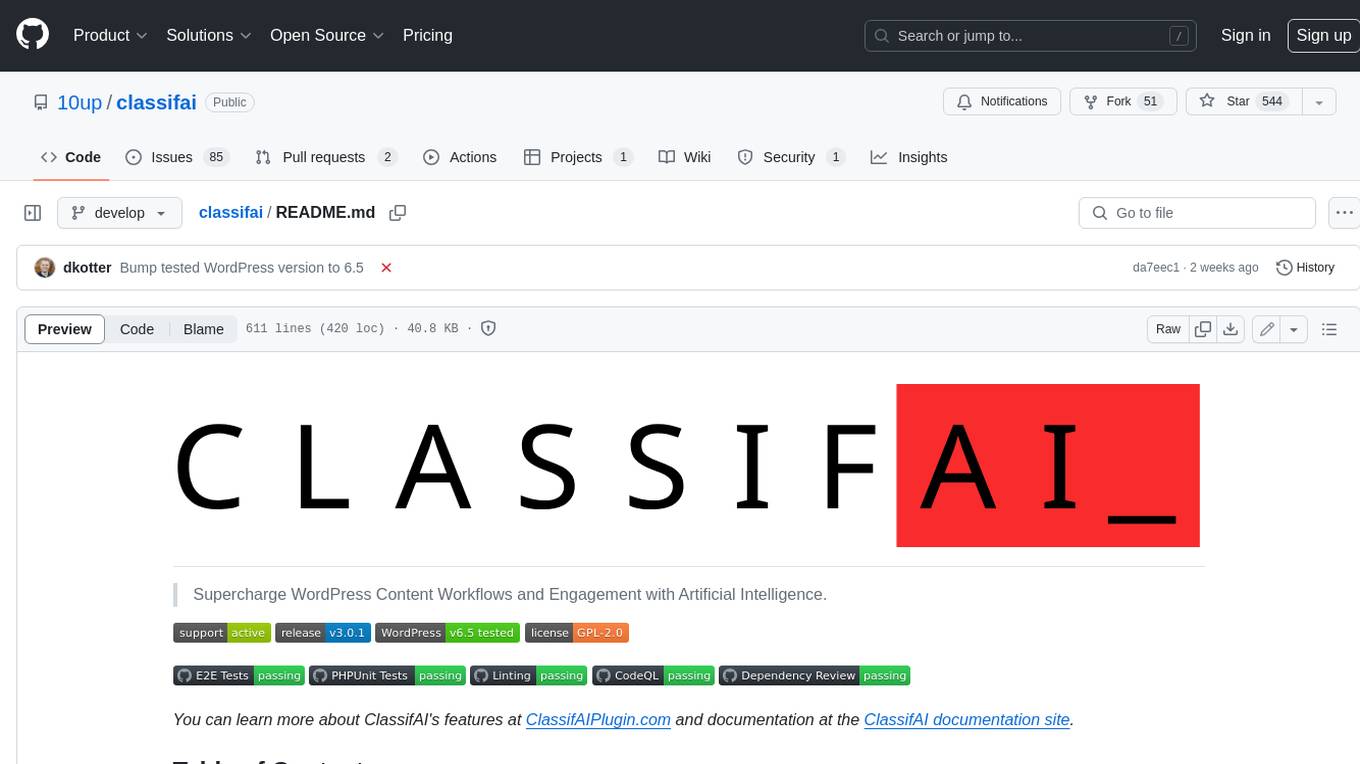
classifai
Supercharge WordPress Content Workflows and Engagement with Artificial Intelligence. Tap into leading cloud-based services like OpenAI, Microsoft Azure AI, Google Gemini and IBM Watson to augment your WordPress-powered websites. Publish content faster while improving SEO performance and increasing audience engagement. ClassifAI integrates Artificial Intelligence and Machine Learning technologies to lighten your workload and eliminate tedious tasks, giving you more time to create original content that matters.
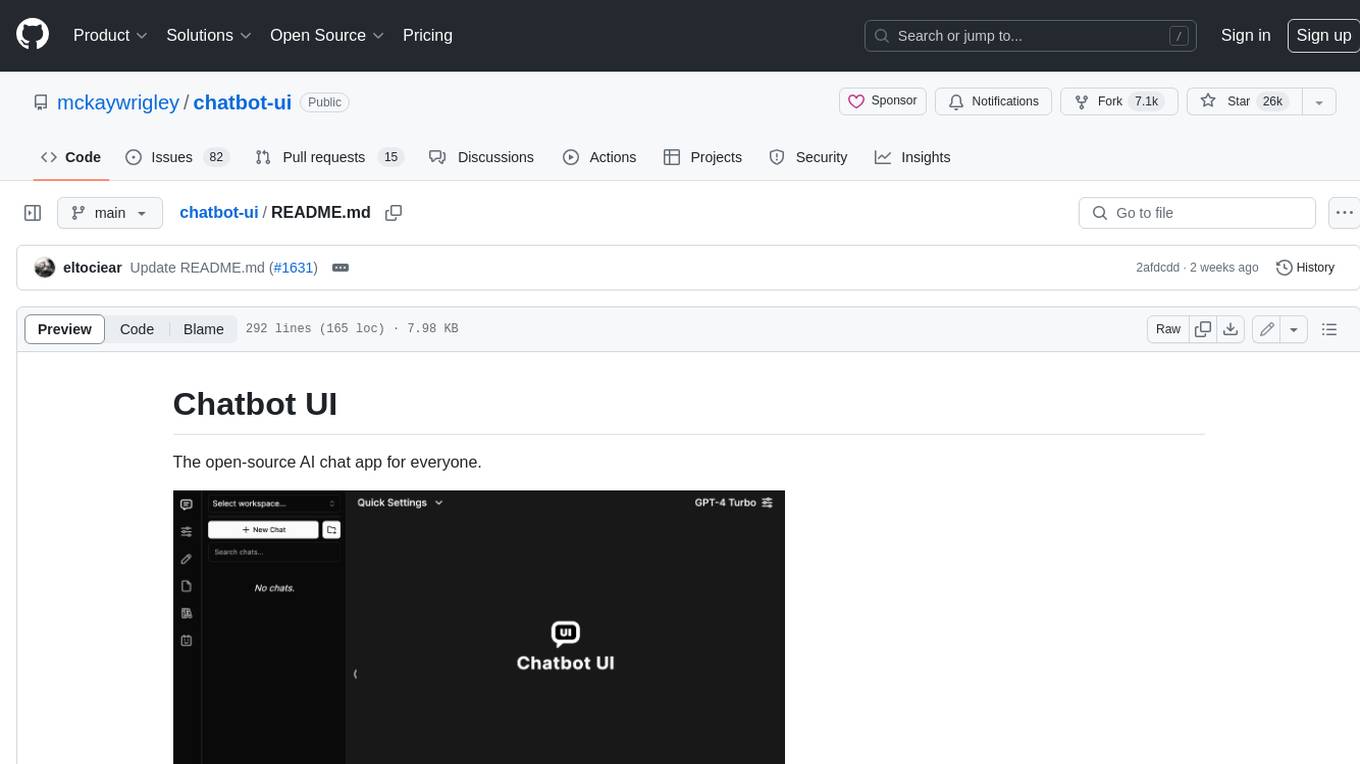
chatbot-ui
Chatbot UI is an open-source AI chat app that allows users to create and deploy their own AI chatbots. It is easy to use and can be customized to fit any need. Chatbot UI is perfect for businesses, developers, and anyone who wants to create a chatbot.
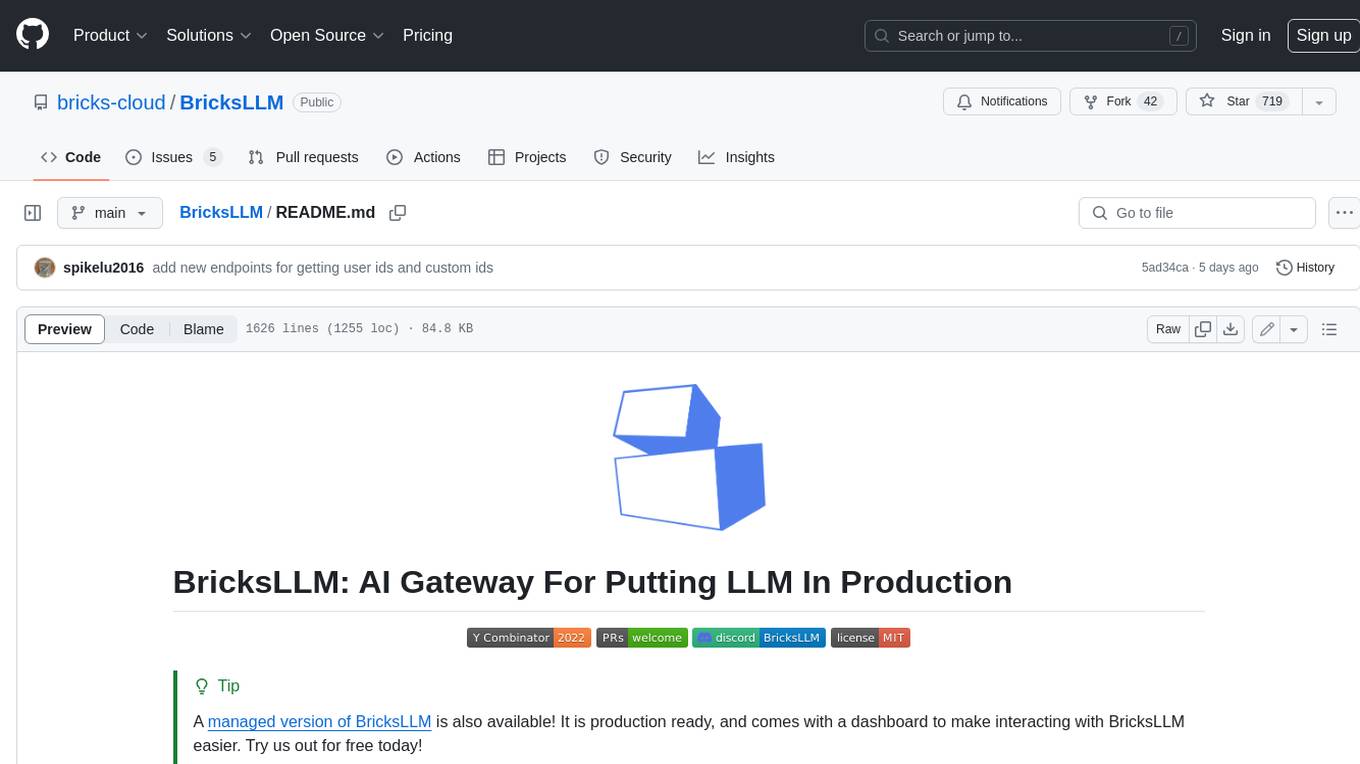
BricksLLM
BricksLLM is a cloud native AI gateway written in Go. Currently, it provides native support for OpenAI, Anthropic, Azure OpenAI and vLLM. BricksLLM aims to provide enterprise level infrastructure that can power any LLM production use cases. Here are some use cases for BricksLLM: * Set LLM usage limits for users on different pricing tiers * Track LLM usage on a per user and per organization basis * Block or redact requests containing PIIs * Improve LLM reliability with failovers, retries and caching * Distribute API keys with rate limits and cost limits for internal development/production use cases * Distribute API keys with rate limits and cost limits for students
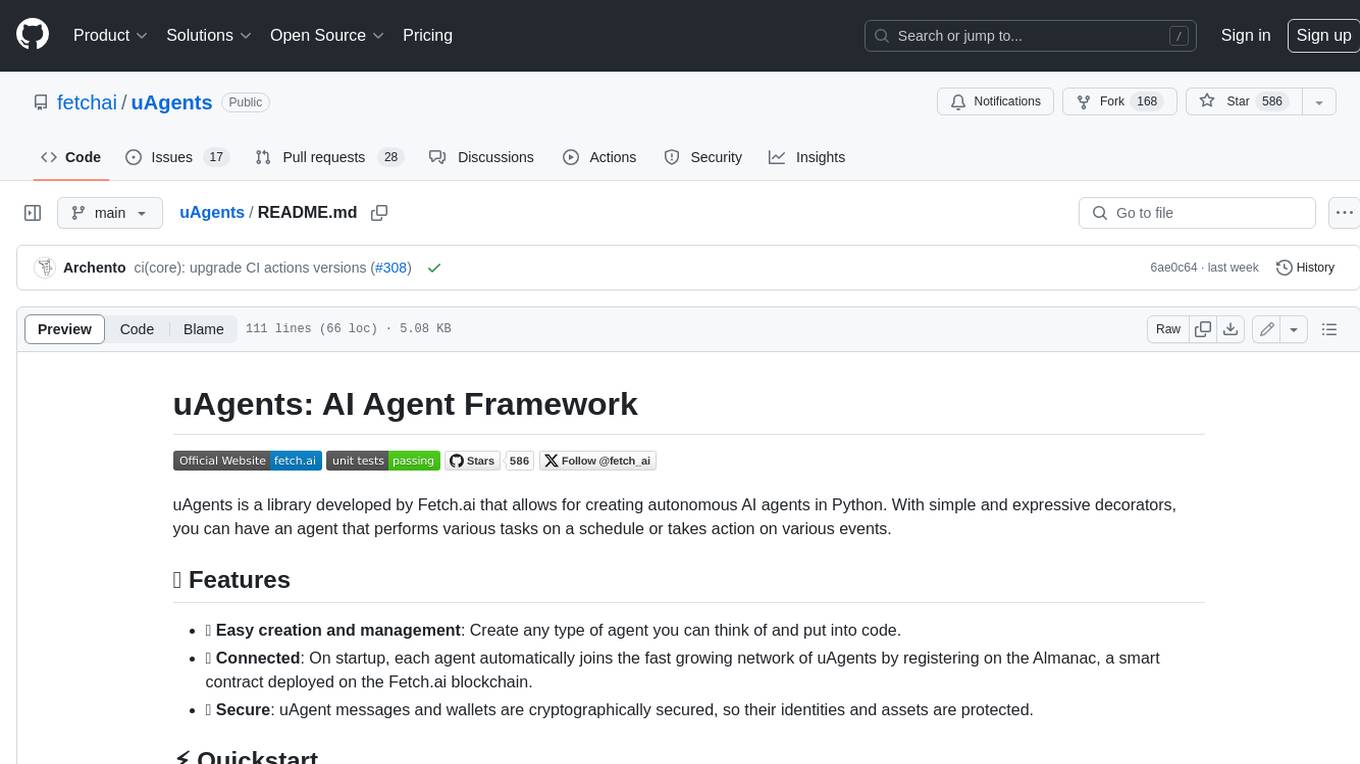
uAgents
uAgents is a Python library developed by Fetch.ai that allows for the creation of autonomous AI agents. These agents can perform various tasks on a schedule or take action on various events. uAgents are easy to create and manage, and they are connected to a fast-growing network of other uAgents. They are also secure, with cryptographically secured messages and wallets.
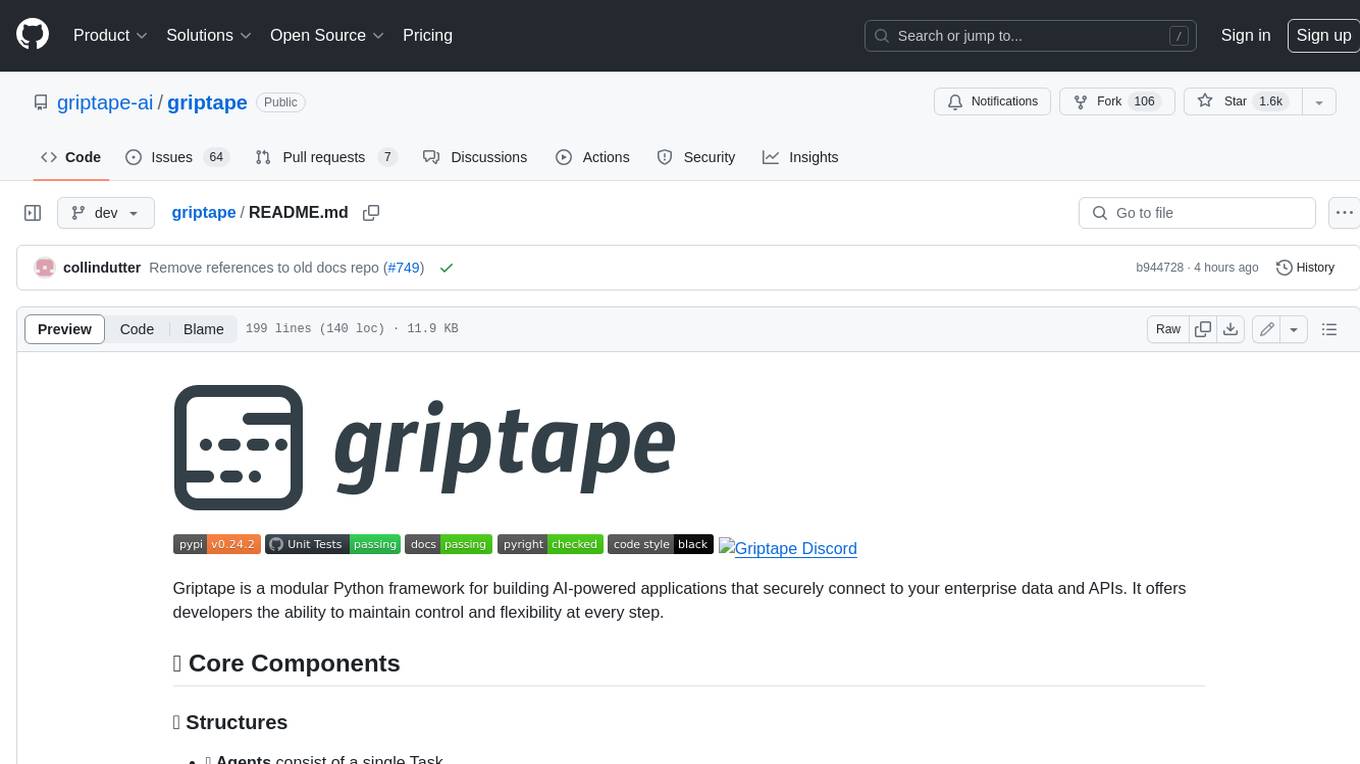
griptape
Griptape is a modular Python framework for building AI-powered applications that securely connect to your enterprise data and APIs. It offers developers the ability to maintain control and flexibility at every step. Griptape's core components include Structures (Agents, Pipelines, and Workflows), Tasks, Tools, Memory (Conversation Memory, Task Memory, and Meta Memory), Drivers (Prompt and Embedding Drivers, Vector Store Drivers, Image Generation Drivers, Image Query Drivers, SQL Drivers, Web Scraper Drivers, and Conversation Memory Drivers), Engines (Query Engines, Extraction Engines, Summary Engines, Image Generation Engines, and Image Query Engines), and additional components (Rulesets, Loaders, Artifacts, Chunkers, and Tokenizers). Griptape enables developers to create AI-powered applications with ease and efficiency.