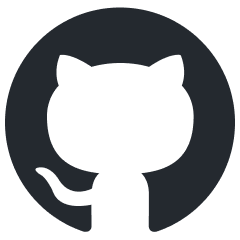
PURE
SOTA RL fine-tuning solution for advanced math reasoning of LLM
Stars: 94
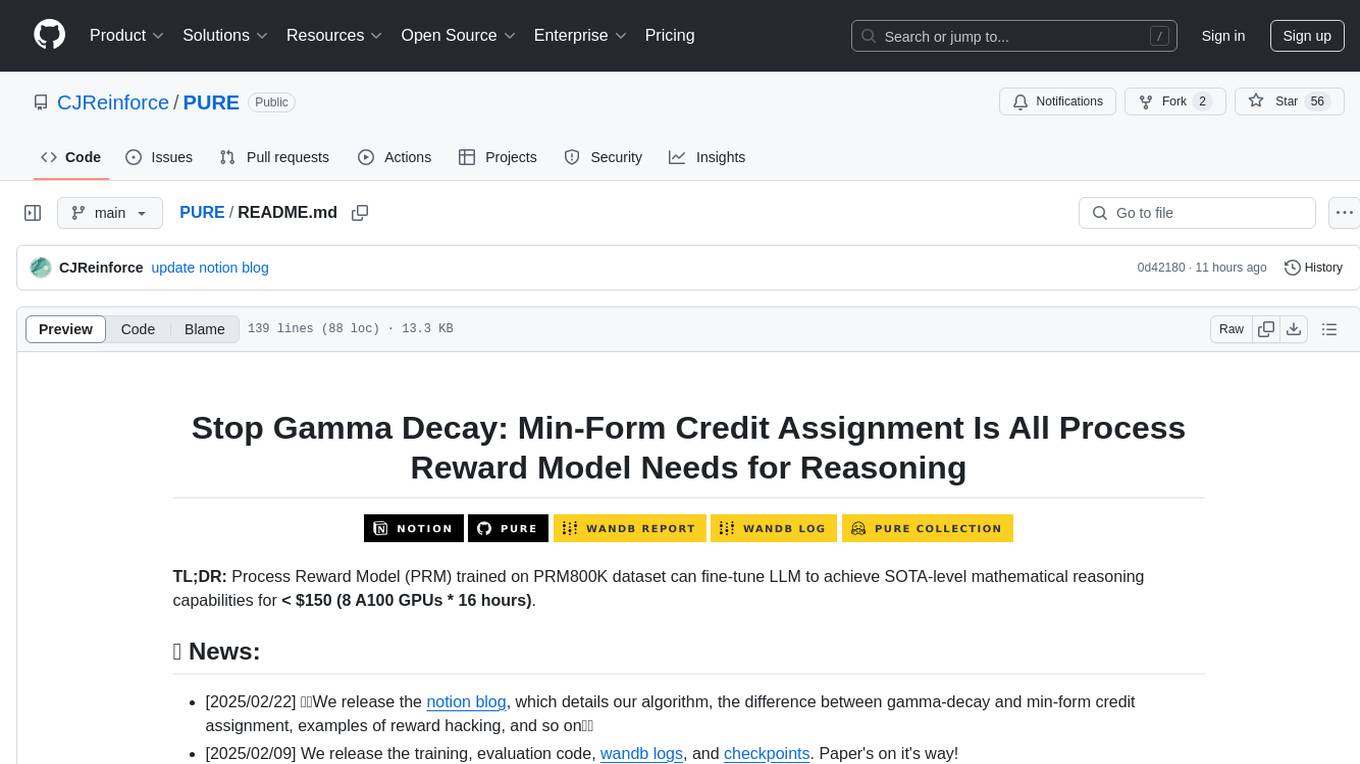
PURE (Process-sUpervised Reinforcement lEarning) is a framework that trains a Process Reward Model (PRM) on a dataset and fine-tunes a language model to achieve state-of-the-art mathematical reasoning capabilities. It uses a novel credit assignment method to calculate return and supports multiple reward types. The final model outperforms existing methods with minimal RL data or compute resources, achieving high accuracy on various benchmarks. The tool addresses reward hacking issues and aims to enhance long-range decision-making and reasoning tasks using large language models.
README:
TL;DR: Process Reward Model (PRM) trained on PRM800K dataset can fine-tune LLM to achieve SOTA-level mathematical reasoning capabilities for < $150 (8 A100 GPUs * 16 hours).
- [2025/03/24] We re-implement our algorithm based on verl. β¨β¨ Key features: (1) add ~50 additional metrics to comprehensively monitor the training process and stability, (2) add a custom wandb workerspace to monitor ~20 important metrics, (3) add curriculum learning.β¨β¨
- [2025/02/22] π₯π₯We release the notion blog, which details our algorithm, the difference between gamma-decay and min-form credit assignment, examples of reward hacking, and so onπ₯π₯
- [2025/02/09] We release the training, evaluation code, wandb, and checkpoints. Paper's on it's way!
This month, we saw a huge boost in LLM reasoning power from the verifiable reward (VR)-based Reinforcement learning fine-tuning (ReFT). Previous work has encountered challenges and made unsuccessful attempts in exploring PRM, so we wonder: How far can PRM actually take us? How does it stack up against VR-based methods in reasoning performance, training costs?
To answer these questions, we present PURE (Process-sUpervised Reinforcement lEarning). Employing Qwen2.5-Math-7B as the base model, we train a PRM using PRM800K dataset, and then fine-tune another Qwen2.5-Math-7B model using only 8K MATH prompts, process rewards from the PRM, and optional verifiable rewards. For the RL algorithm, we use the PPO loss with an RLOO advantage estimator. We improve credit assignment by using a weighted sum of the process rewards, $\sum_t \text{softmax}(-\text{PR}_t/T)\cdot\text{PR}_t$ which approximates ${\min}_t \text{PR}_t$ when $T\rightarrow 0$, instead of the usual gamma decay sum $\sum_t \gamma^t \cdot \text{PR}_t$ to calculate return. Our framework supports multiple reward types: only process reward (PURE-PRM), only verifiable reward (PURE-VR) which is the Deepseek-R1-zero's setting, or a mix of both (PURE-PRM+VR), as shown in the following table.
π The final model achieves pass@1 accuracy of 82.6% on MATH500, 82.5% on AMC, and 53.3% on average across 5 benchmarks, beating Qwen2.5-math-7B-instruct, PRIME, and SimpleRL with just either <1/50th RL data or 1/5th of the compute resources.
All results are in pass@1 accuracy
AIME 2024 | MATH 500 | AMC | Minerva Math | OlympiadBench | Avg. | |
---|---|---|---|---|---|---|
Qwen2.5-Math-7B-Base | 13.3 | 71.8 | 47.5 | 29.8 | 35.1 | 39.5 |
Qwen-2.5-Math-7B-Instruct | 16.7 | 83.2 | 52.5 | 37.5 | 41.3 | 46.2 |
Eurus-2-7B-PRIME | 26.7 | 79.2 | 57.8 | 38.6 | 42.1 | 48.9 |
Qwen2.5-7B-SimpleRL-Zero | 33.3 | 77.2 | 62.5 | 33.5 | 37.6 | 48.8 |
Qwen2.5-7B-PURE-PRM+VR* | 20.0 | 82.6 | 82.5 | 37.1 | 44.1 | 53.3 |
Qwen2.5-7B-PURE-PRM | 16.7 | 81.8 | 60.0 | 38.2 | 44.7 | 49.3 |
Qwen2.5-7B-PURE-VR | 23.3 | 79.4 | 60.0 | 36.8 | 41.8 | 48.3 |
*The SOTA model was trained using 8K MATH problems, of which only ~800 gave ground-truth final answers that could be used to calculate VRs.
Note: Eurus-2-7B-PRIME, and SimpleRL-Zero are also based on Qwen-2.5-Math-7B.
We implement our algorithm on two frameworks, OpenRLHF and verl, in 2 different branches respectively. If you are new to our project, we recommend using verl version.
Please follow OpenRLHF's guidance to configure required environments. Then run pip install -r requirements.txt
.
Please refer to the official installation guidance of verl.
We train the PRM in 2 stages using TRL and a preprocessed PRM800K dataset. In the first stage, we freeze the LLM and only train the last score layer (MLP) with 1e-4 learning rate rate for 3 epochs. In the second stage, we unfreeze the LLM and fine-tune all parameters with 1e-6 learning rate for 1 epoch. The resultant PRM is released through HuggingFace.
cd PRM
# stage 1
bash train_stage_1.sh
# stage 2
bash train_stage_2.sh
Switch to the openrlhf branch. Run the following command. It uses Ray+vLLM for rollout acceleration, with the first 4 GPUs allocated for the actor, initial actor (reference model), and PRM. The remaining GPUs are used for the vLLM engines. This setup works with 5 to 8 GPUsβjust adjust the number of vLLM engines in the script accordingly.
bash examples/scripts/train_pure.sh
Switch to the verl branch. Modify the actor_rollout_ref.model.path
, trainer.default_local_dir
in the config file. Then start training:
python -m verl.trainer.main_ppo
The hybrid engine of verl allows for higher gpu utilization compared to the openrlhf version.
We use Qwen Math's codebase for evaluation (i.e., pass@1 accuracy). For fairness considerations, we completely prohibited solving problems by calling code, following SimpleRL. Please follow the /eval
instructions for evaluation.
- [x] re-implementation on verl
- [ ] paper with more discussions and evaluations
- [ ] attempts to mitigate reward hacking for PRM (Online PURE)
If you find our code useful, we would appreciate it if you could cite our work:
@misc{cheng2025pure,
title={Stop Gamma Decay: Min-Form Credit Assignment Is All Process Reward Model Needs for Reasoning},
author={Jie Cheng and Lijun Li and Gang Xiong and Jing Shao and Yisheng Lv and Fei-Yue Wang},
year={2025},
howpublished={\url{https://tungsten-ink-510.notion.site/Stop-Gamma-Decay-Min-Form-Credit-Assignment-Is-All-Process-Reward-Model-Needs-for-Reasoning-19fcb6ed0184804eb07fd310b38af155?pvs=4}},
note={Notion Blog}
year={2025}
}
We implement our RL algorithm based on OpenRLHF and verl. We thank the developers of OpenRLHF and the author of SimpleRL for discussion! In addition, we also refer to TRL, PRIME's code and hyperparameter values to varying degrees. Thank them for their wonderful work!
For Tasks:
Click tags to check more tools for each tasksFor Jobs:
Alternative AI tools for PURE
Similar Open Source Tools
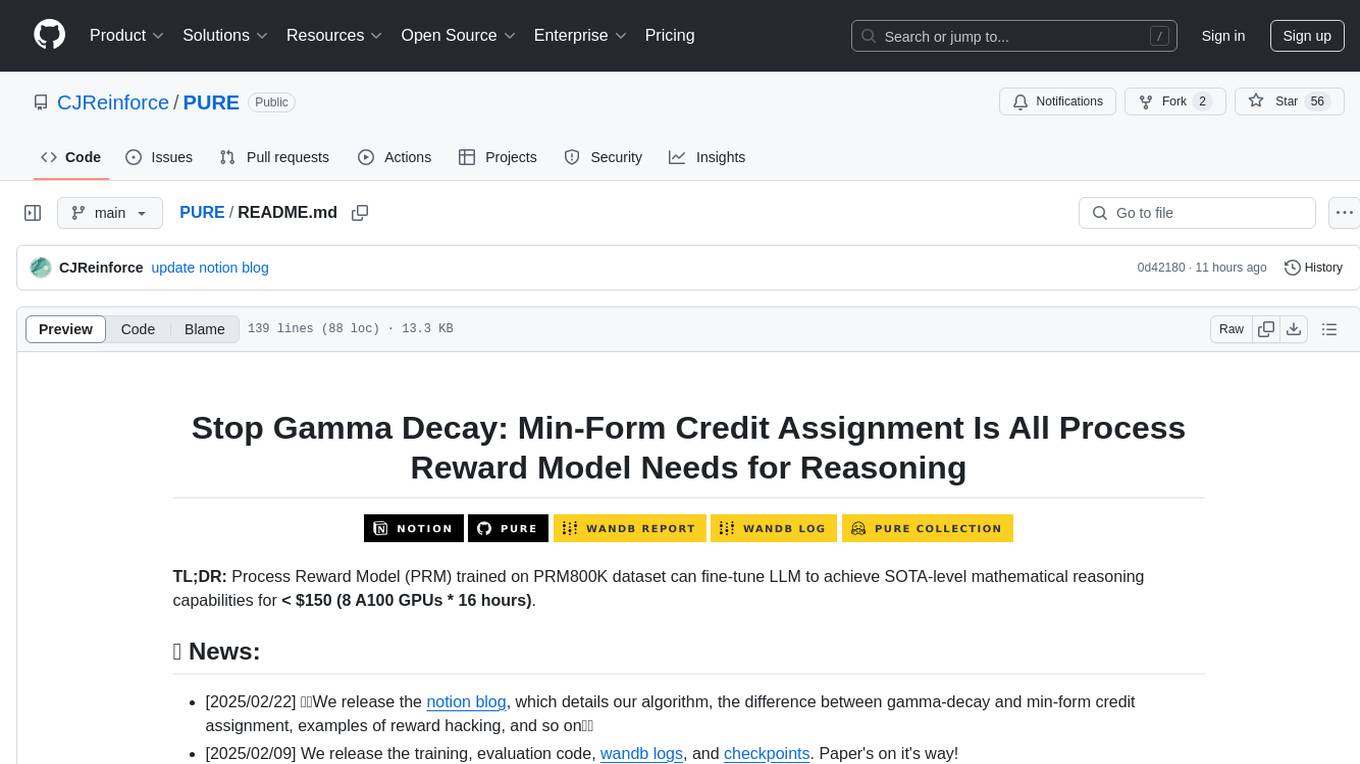
PURE
PURE (Process-sUpervised Reinforcement lEarning) is a framework that trains a Process Reward Model (PRM) on a dataset and fine-tunes a language model to achieve state-of-the-art mathematical reasoning capabilities. It uses a novel credit assignment method to calculate return and supports multiple reward types. The final model outperforms existing methods with minimal RL data or compute resources, achieving high accuracy on various benchmarks. The tool addresses reward hacking issues and aims to enhance long-range decision-making and reasoning tasks using large language models.
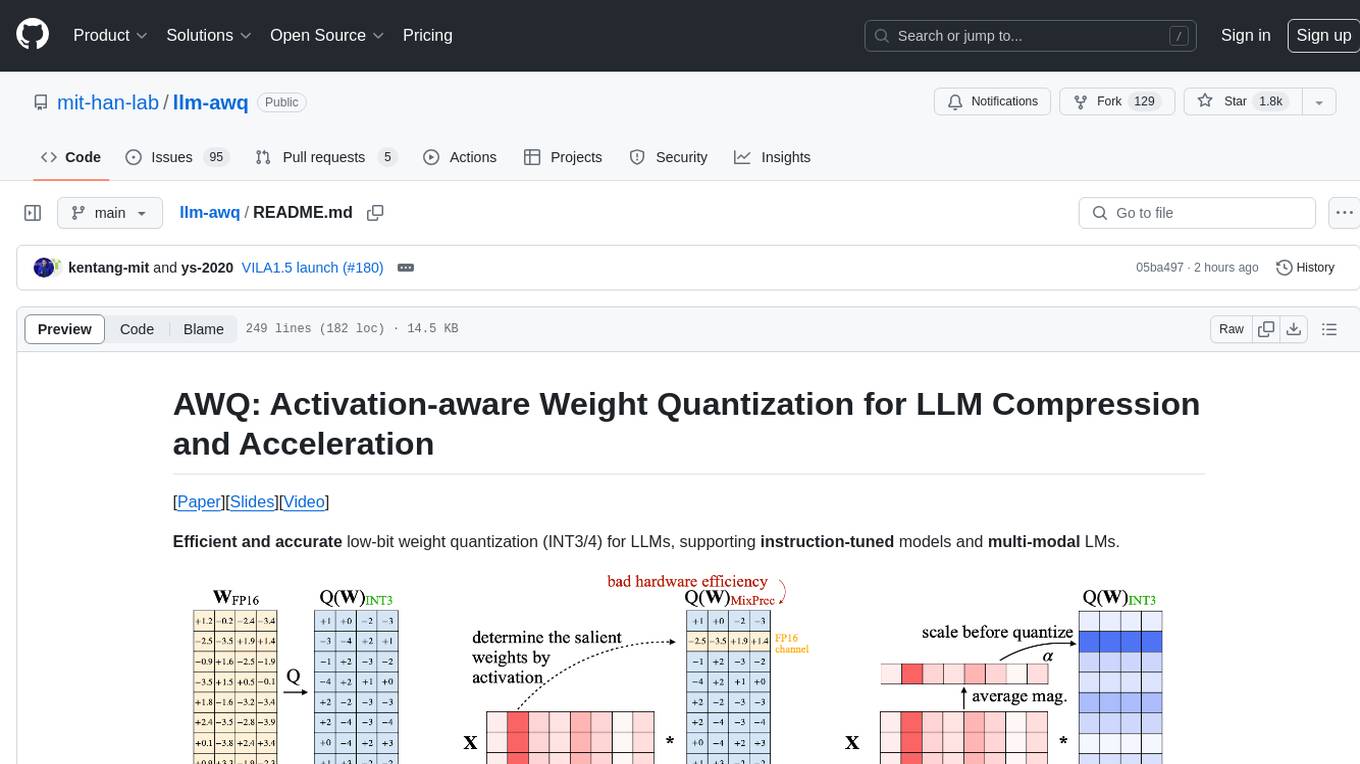
llm-awq
AWQ (Activation-aware Weight Quantization) is a tool designed for efficient and accurate low-bit weight quantization (INT3/4) for Large Language Models (LLMs). It supports instruction-tuned models and multi-modal LMs, providing features such as AWQ search for accurate quantization, pre-computed AWQ model zoo for various LLMs, memory-efficient 4-bit linear in PyTorch, and efficient CUDA kernel implementation for fast inference. The tool enables users to run large models on resource-constrained edge platforms, delivering more efficient responses with LLM/VLM chatbots through 4-bit inference.
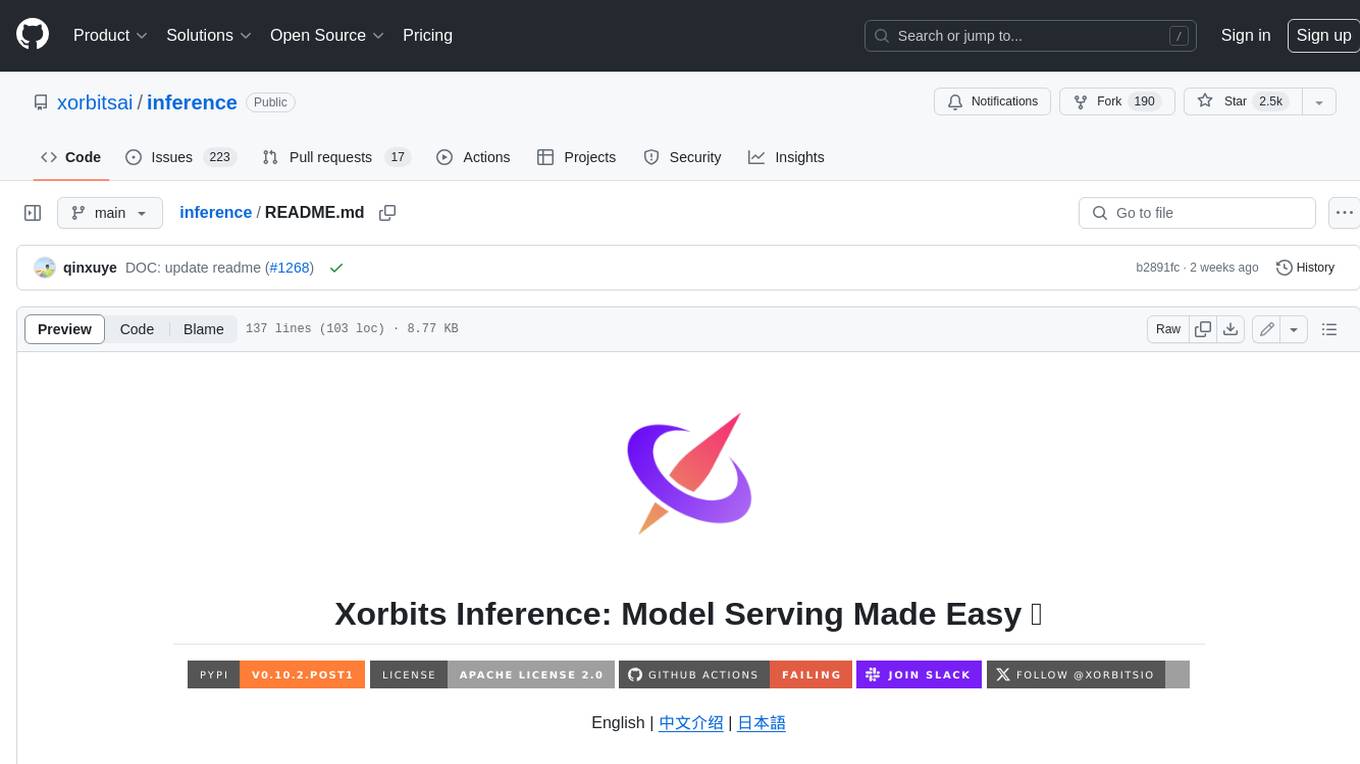
inference
Xorbits Inference (Xinference) is a powerful and versatile library designed to serve language, speech recognition, and multimodal models. With Xorbits Inference, you can effortlessly deploy and serve your or state-of-the-art built-in models using just a single command. Whether you are a researcher, developer, or data scientist, Xorbits Inference empowers you to unleash the full potential of cutting-edge AI models.
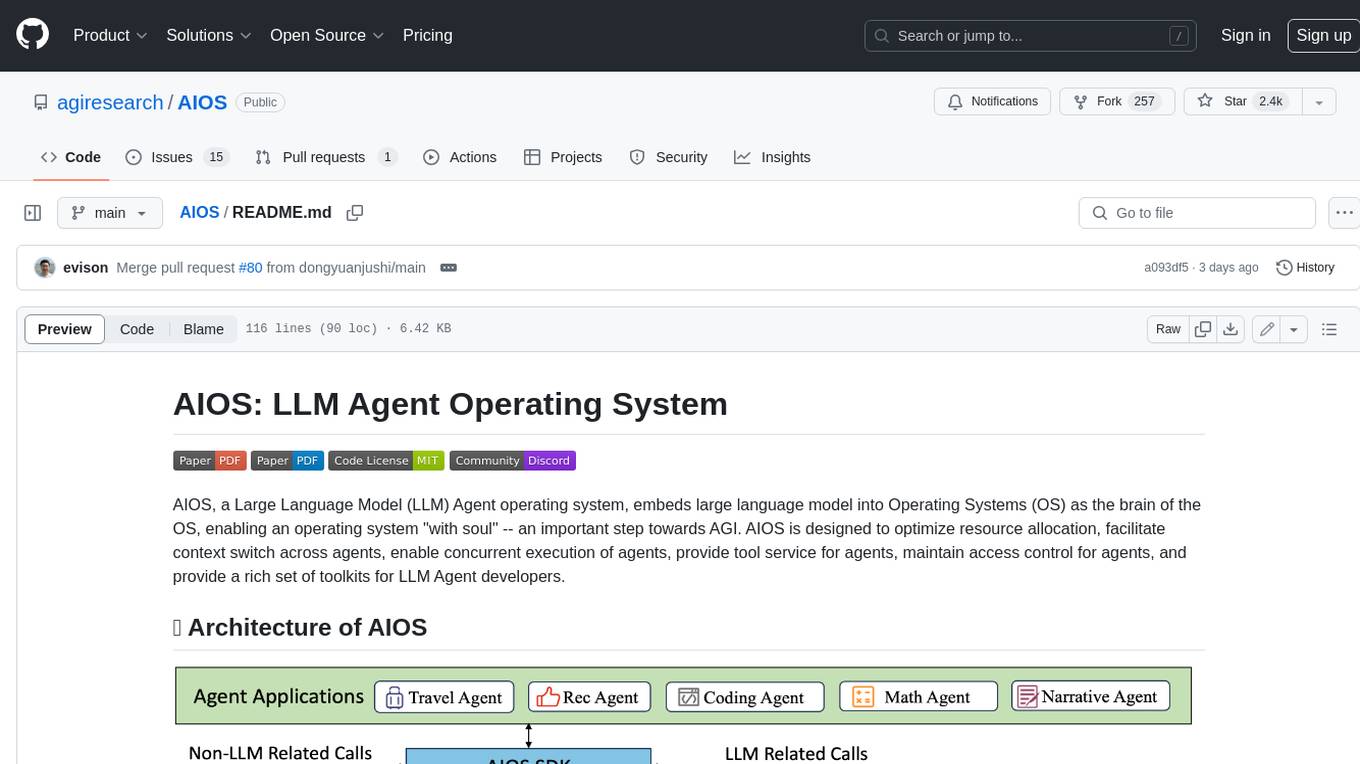
AIOS
AIOS, a Large Language Model (LLM) Agent operating system, embeds large language model into Operating Systems (OS) as the brain of the OS, enabling an operating system "with soul" -- an important step towards AGI. AIOS is designed to optimize resource allocation, facilitate context switch across agents, enable concurrent execution of agents, provide tool service for agents, maintain access control for agents, and provide a rich set of toolkits for LLM Agent developers.
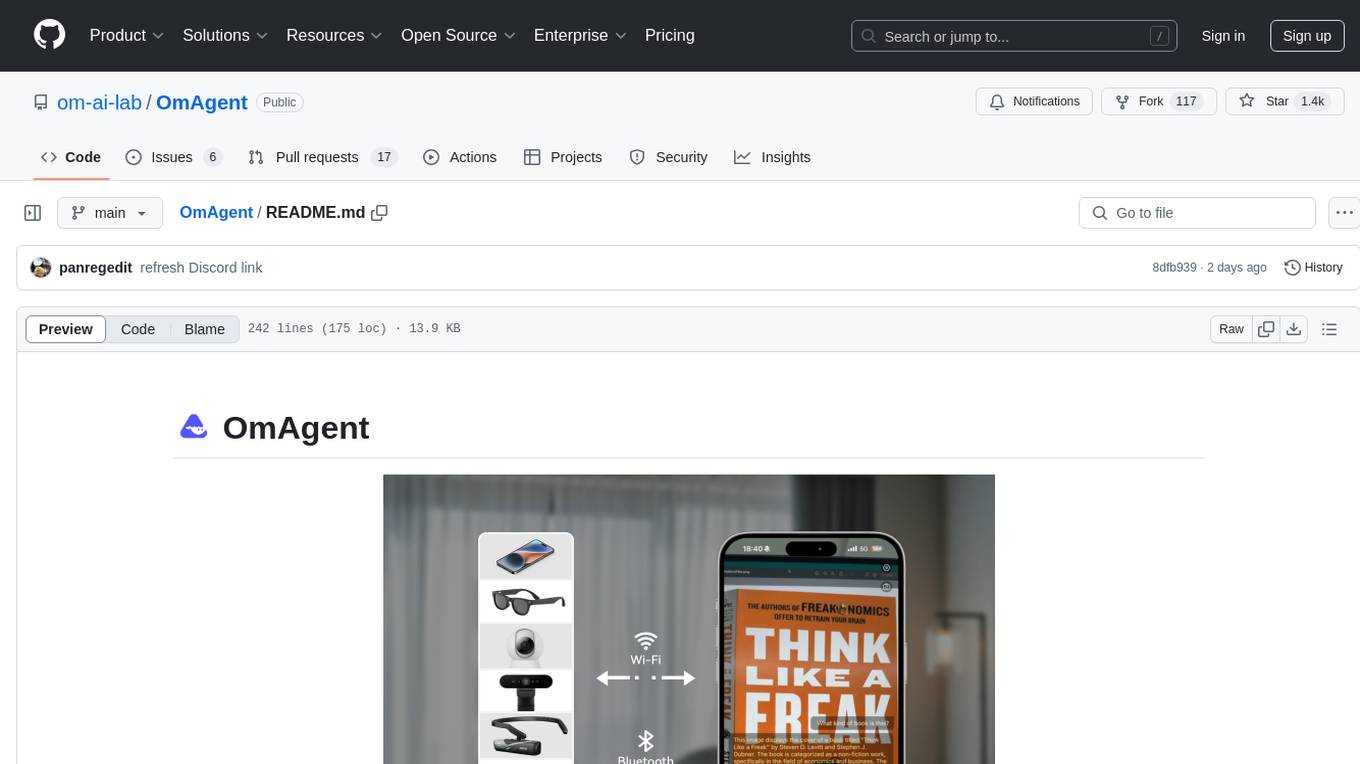
OmAgent
OmAgent is an open-source agent framework designed to streamline the development of on-device multimodal agents. It enables agents to empower various hardware devices, integrates speed-optimized SOTA multimodal models, provides SOTA multimodal agent algorithms, and focuses on optimizing the end-to-end computing pipeline for real-time user interaction experience. Key features include easy connection to diverse devices, scalability, flexibility, and workflow orchestration. The architecture emphasizes graph-based workflow orchestration, native multimodality, and device-centricity, allowing developers to create bespoke intelligent agent programs.
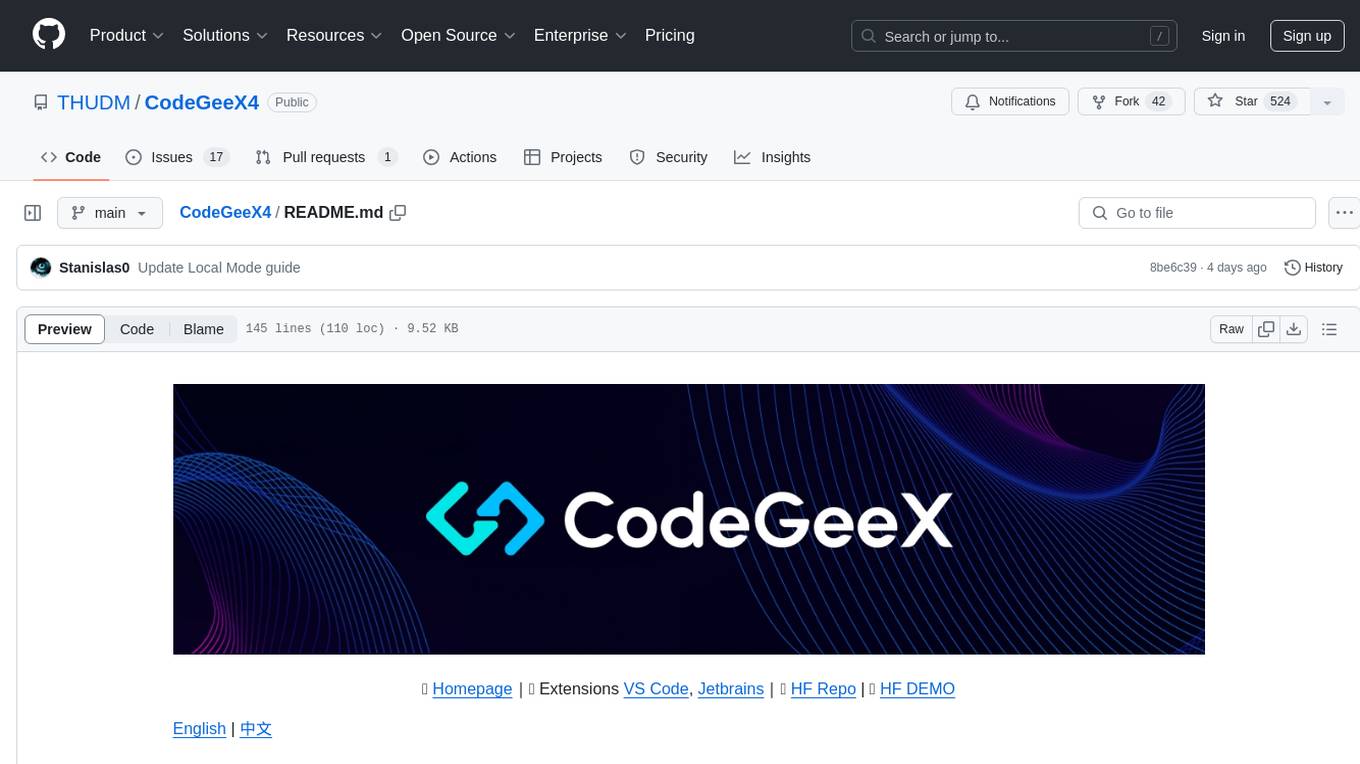
CodeGeeX4
CodeGeeX4-ALL-9B is an open-source multilingual code generation model based on GLM-4-9B, offering enhanced code generation capabilities. It supports functions like code completion, code interpreter, web search, function call, and repository-level code Q&A. The model has competitive performance on benchmarks like BigCodeBench and NaturalCodeBench, outperforming larger models in terms of speed and performance.
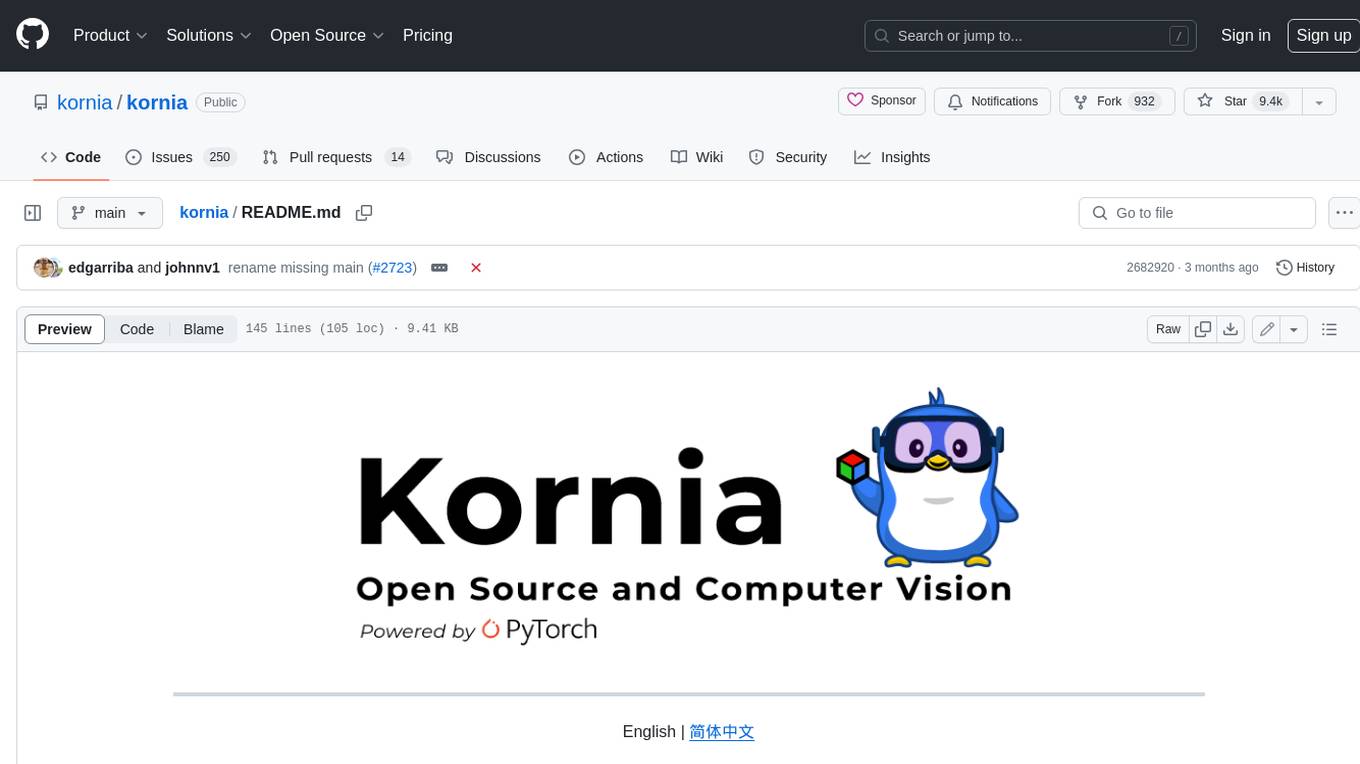
kornia
Kornia is a differentiable computer vision library for PyTorch. It consists of a set of routines and differentiable modules to solve generic computer vision problems. At its core, the package uses PyTorch as its main backend both for efficiency and to take advantage of the reverse-mode auto-differentiation to define and compute the gradient of complex functions.
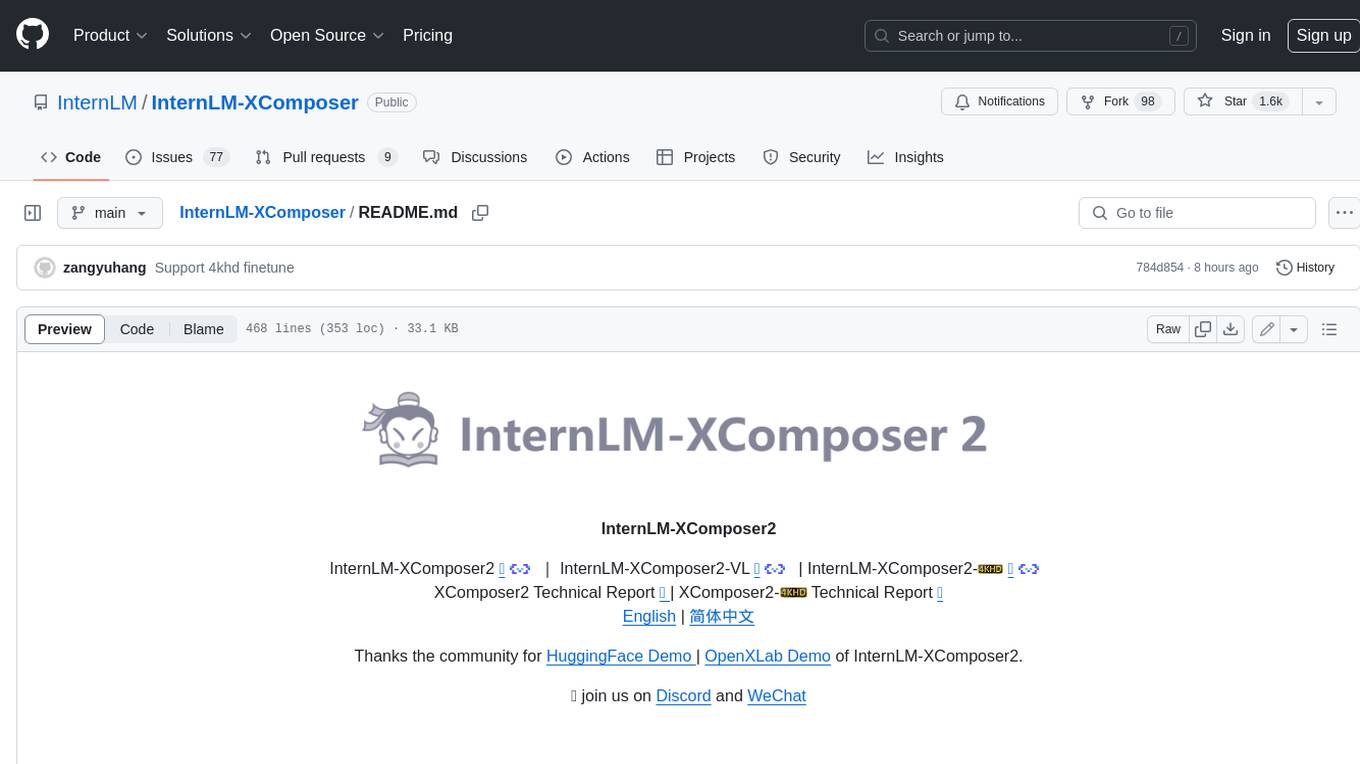
InternLM-XComposer
InternLM-XComposer2 is a groundbreaking vision-language large model (VLLM) based on InternLM2-7B excelling in free-form text-image composition and comprehension. It boasts several amazing capabilities and applications: * **Free-form Interleaved Text-Image Composition** : InternLM-XComposer2 can effortlessly generate coherent and contextual articles with interleaved images following diverse inputs like outlines, detailed text requirements and reference images, enabling highly customizable content creation. * **Accurate Vision-language Problem-solving** : InternLM-XComposer2 accurately handles diverse and challenging vision-language Q&A tasks based on free-form instructions, excelling in recognition, perception, detailed captioning, visual reasoning, and more. * **Awesome performance** : InternLM-XComposer2 based on InternLM2-7B not only significantly outperforms existing open-source multimodal models in 13 benchmarks but also **matches or even surpasses GPT-4V and Gemini Pro in 6 benchmarks** We release InternLM-XComposer2 series in three versions: * **InternLM-XComposer2-4KHD-7B** π€: The high-resolution multi-task trained VLLM model with InternLM-7B as the initialization of the LLM for _High-resolution understanding_ , _VL benchmarks_ and _AI assistant_. * **InternLM-XComposer2-VL-7B** π€ : The multi-task trained VLLM model with InternLM-7B as the initialization of the LLM for _VL benchmarks_ and _AI assistant_. **It ranks as the most powerful vision-language model based on 7B-parameter level LLMs, leading across 13 benchmarks.** * **InternLM-XComposer2-VL-1.8B** π€ : A lightweight version of InternLM-XComposer2-VL based on InternLM-1.8B. * **InternLM-XComposer2-7B** π€: The further instruction tuned VLLM for _Interleaved Text-Image Composition_ with free-form inputs. Please refer to Technical Report and 4KHD Technical Reportfor more details.
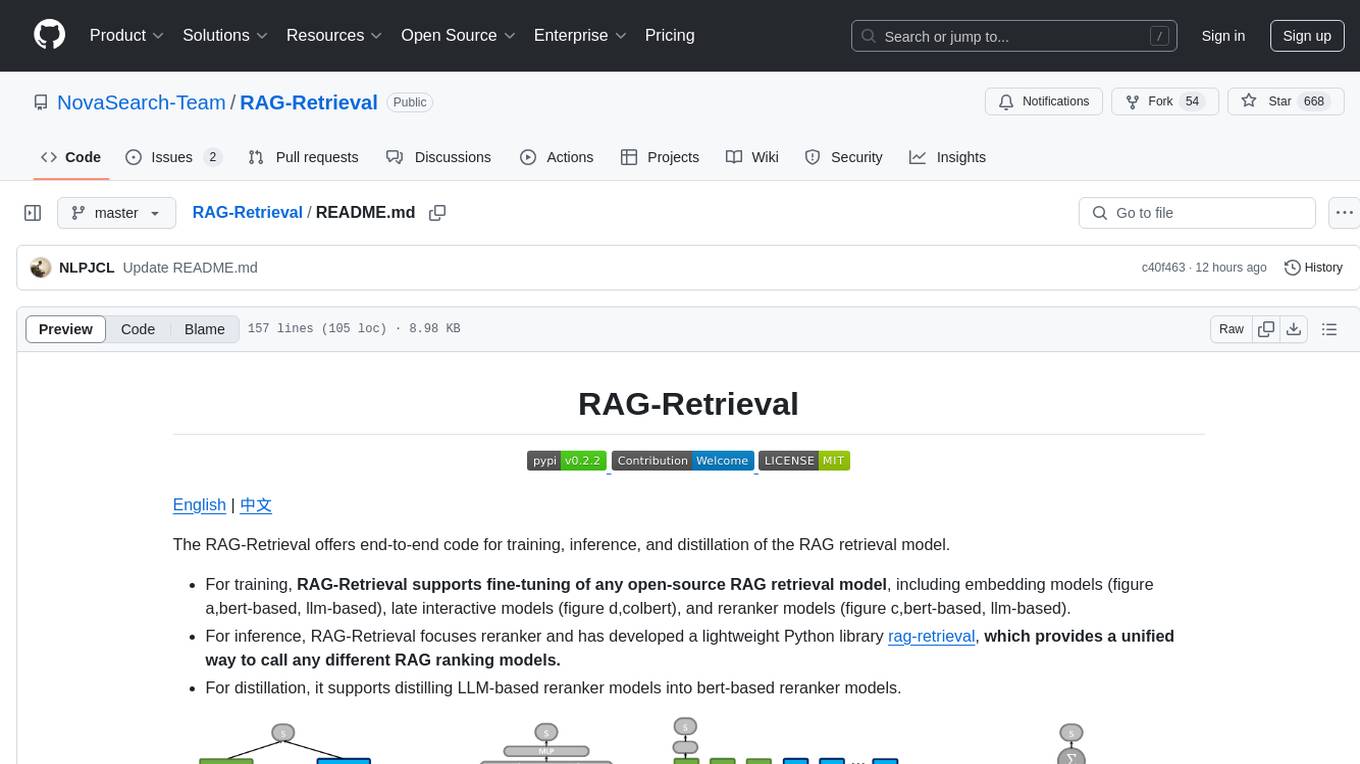
RAG-Retrieval
RAG-Retrieval is an end-to-end code repository that provides training, inference, and distillation capabilities for the RAG retrieval model. It supports fine-tuning of various open-source RAG retrieval models, including embedding models, late interactive models, and reranker models. The repository offers a lightweight Python library for calling different RAG ranking models and allows distillation of LLM-based reranker models into bert-based reranker models. It includes features such as support for end-to-end fine-tuning, distillation of large models, advanced algorithms like MRL, multi-GPU training strategy, and a simple code structure for easy modifications.
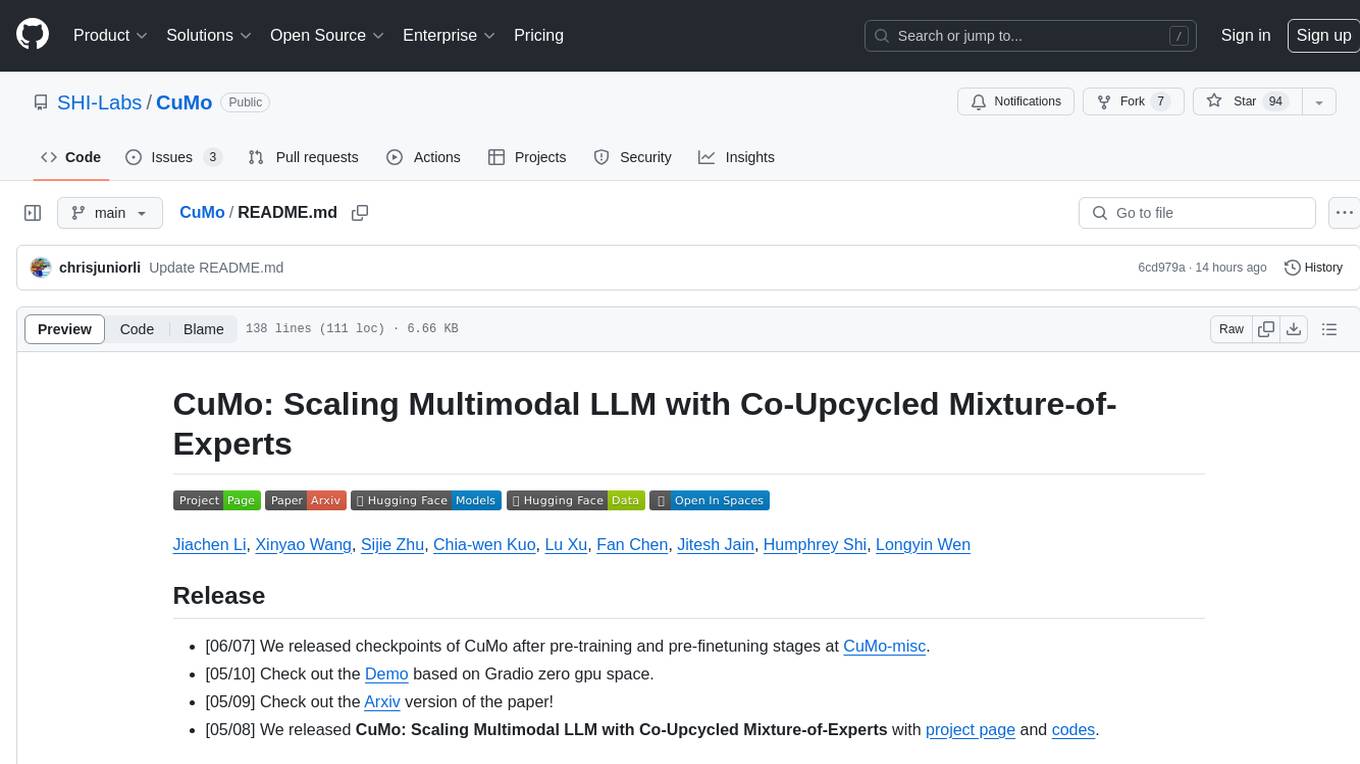
CuMo
CuMo is a project focused on scaling multimodal Large Language Models (LLMs) with Co-Upcycled Mixture-of-Experts. It introduces CuMo, which incorporates Co-upcycled Top-K sparsely-gated Mixture-of-experts blocks into the vision encoder and the MLP connector, enhancing the capabilities of multimodal LLMs. The project adopts a three-stage training approach with auxiliary losses to stabilize the training process and maintain a balanced loading of experts. CuMo achieves comparable performance to other state-of-the-art multimodal LLMs on various Visual Question Answering (VQA) and visual-instruction-following benchmarks.
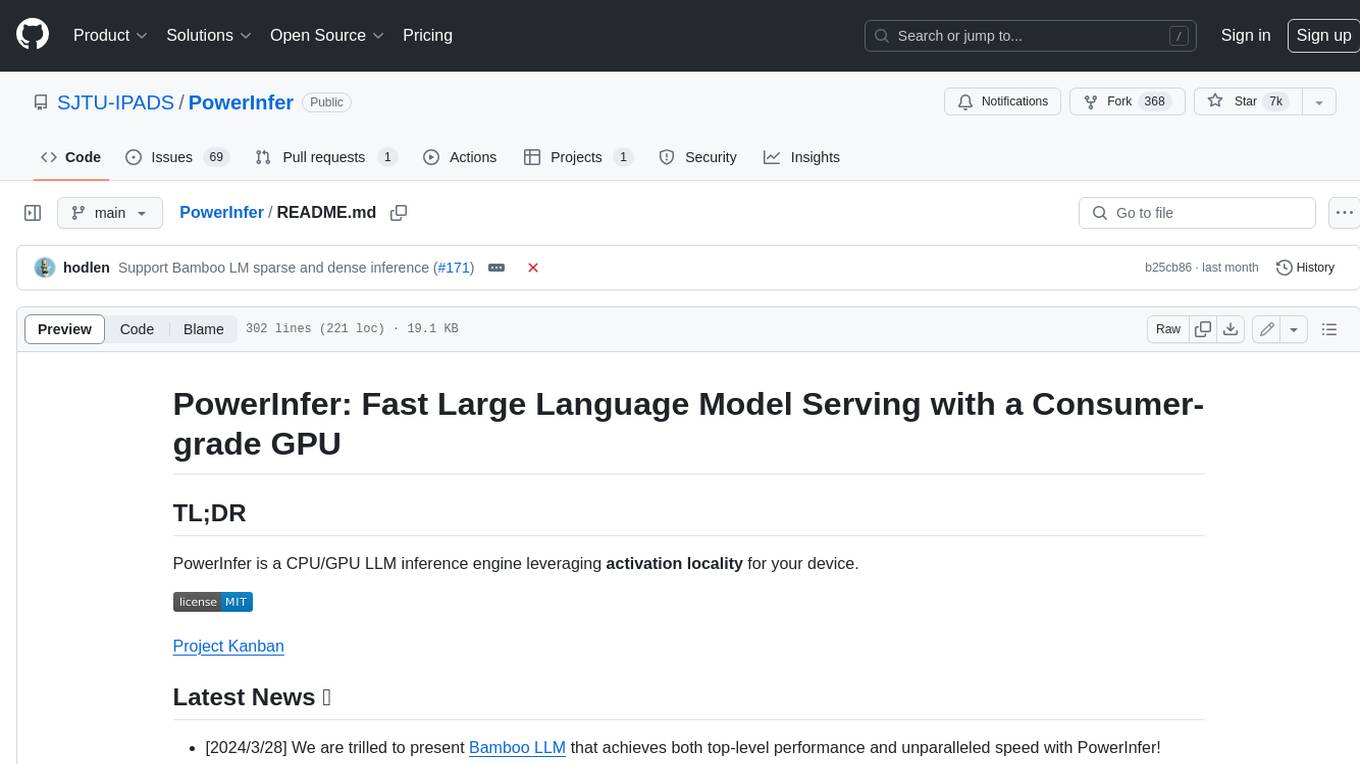
PowerInfer
PowerInfer is a high-speed Large Language Model (LLM) inference engine designed for local deployment on consumer-grade hardware, leveraging activation locality to optimize efficiency. It features a locality-centric design, hybrid CPU/GPU utilization, easy integration with popular ReLU-sparse models, and support for various platforms. PowerInfer achieves high speed with lower resource demands and is flexible for easy deployment and compatibility with existing models like Falcon-40B, Llama2 family, ProSparse Llama2 family, and Bamboo-7B.
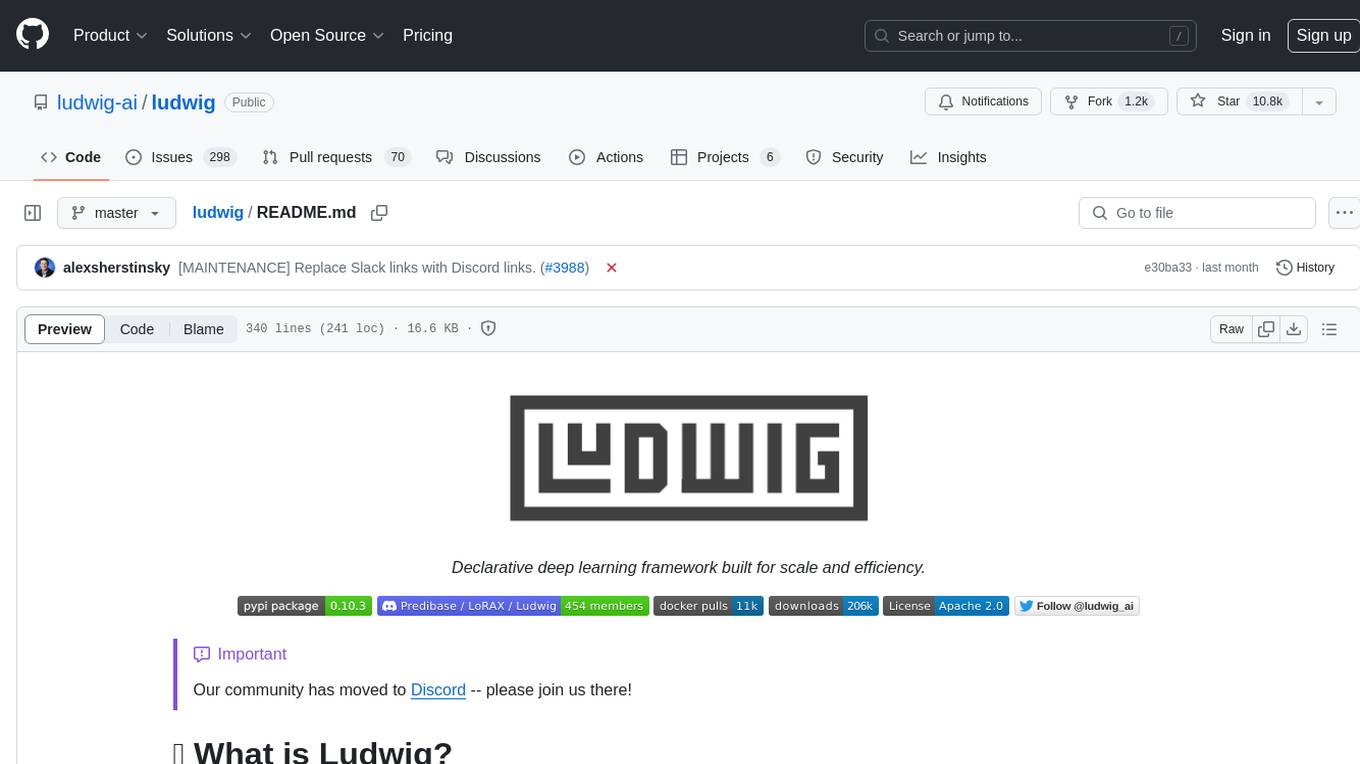
ludwig
Ludwig is a declarative deep learning framework designed for scale and efficiency. It is a low-code framework that allows users to build custom AI models like LLMs and other deep neural networks with ease. Ludwig offers features such as optimized scale and efficiency, expert level control, modularity, and extensibility. It is engineered for production with prebuilt Docker containers, support for running with Ray on Kubernetes, and the ability to export models to Torchscript and Triton. Ludwig is hosted by the Linux Foundation AI & Data.
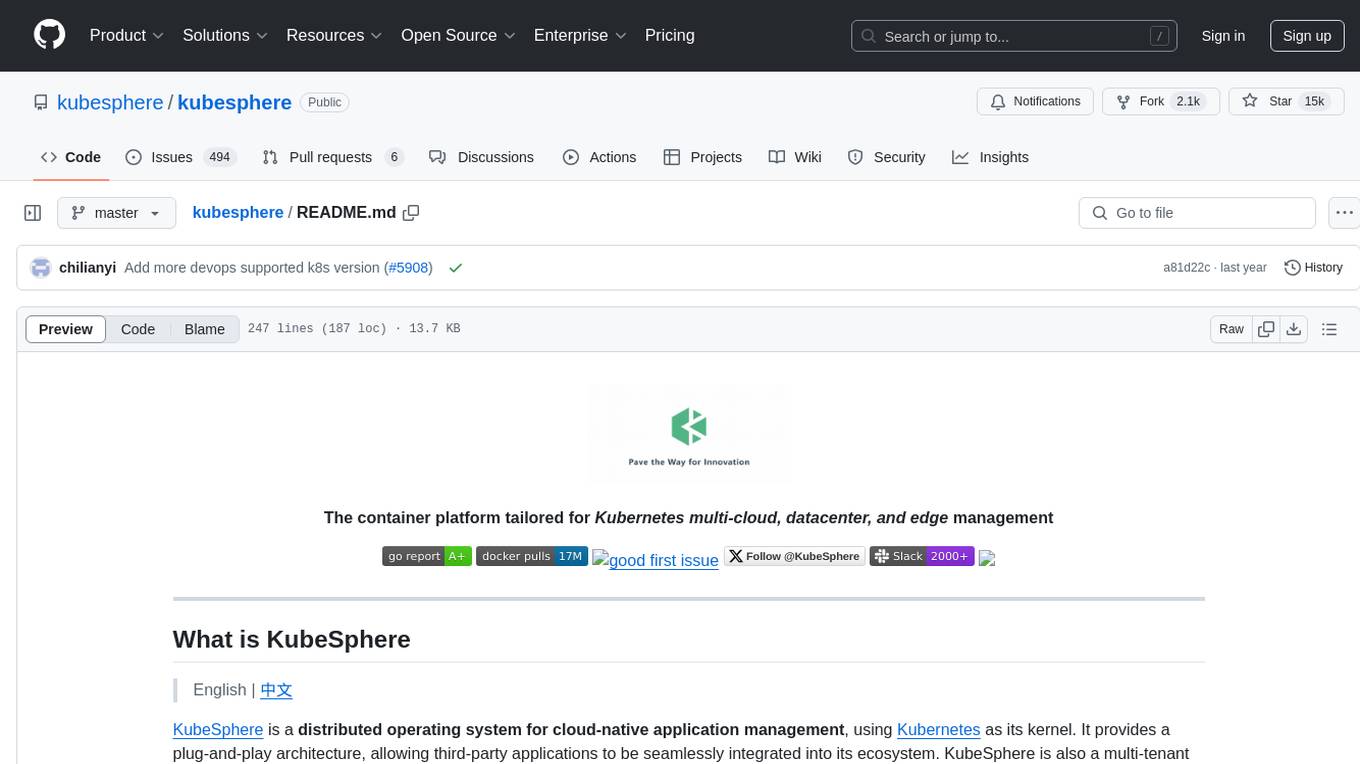
kubesphere
KubeSphere is a distributed operating system for cloud-native application management, using Kubernetes as its kernel. It provides a plug-and-play architecture, allowing third-party applications to be seamlessly integrated into its ecosystem. KubeSphere is also a multi-tenant container platform with full-stack automated IT operation and streamlined DevOps workflows. It provides developer-friendly wizard web UI, helping enterprises to build out a more robust and feature-rich platform, which includes most common functionalities needed for enterprise Kubernetes strategy.
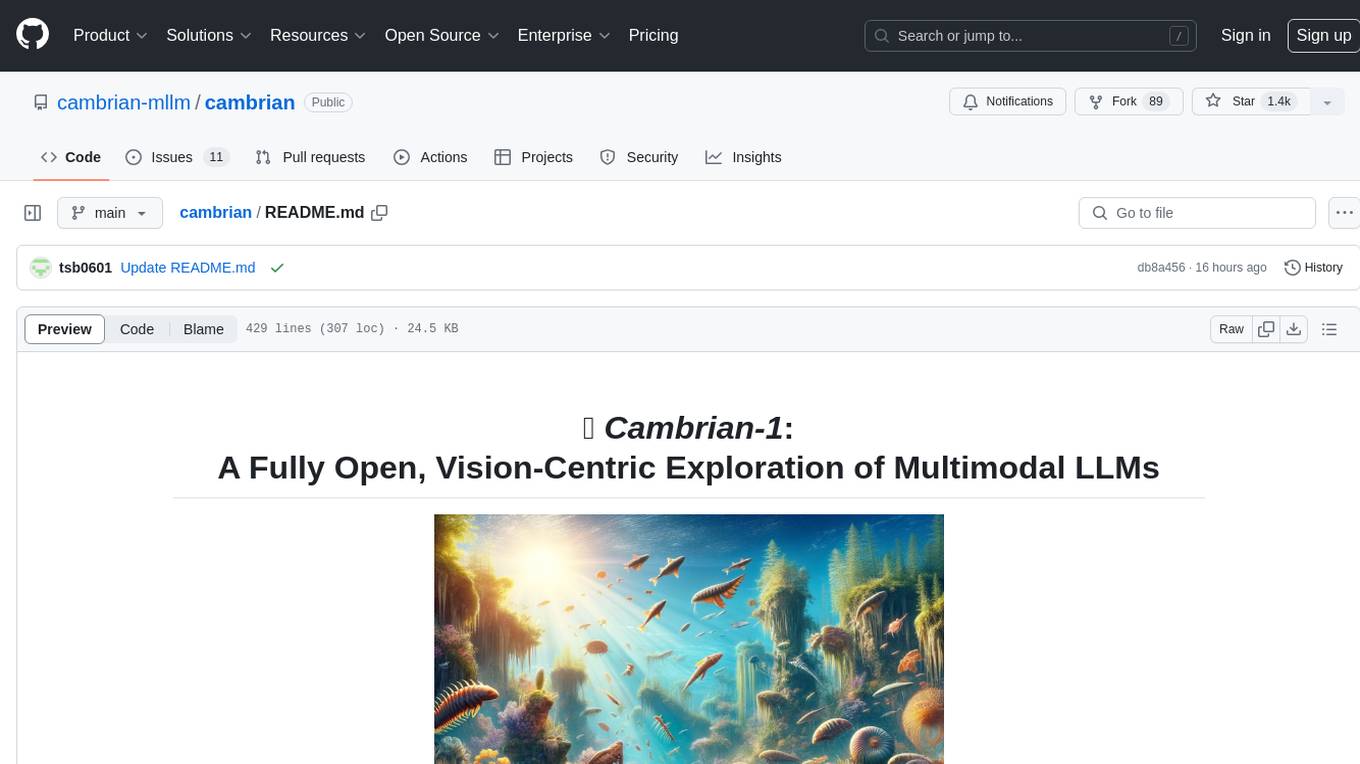
cambrian
Cambrian-1 is a fully open project focused on exploring multimodal Large Language Models (LLMs) with a vision-centric approach. It offers competitive performance across various benchmarks with models at different parameter levels. The project includes training configurations, model weights, instruction tuning data, and evaluation details. Users can interact with Cambrian-1 through a Gradio web interface for inference. The project is inspired by LLaVA and incorporates contributions from Vicuna, LLaMA, and Yi. Cambrian-1 is licensed under Apache 2.0 and utilizes datasets and checkpoints subject to their respective original licenses.
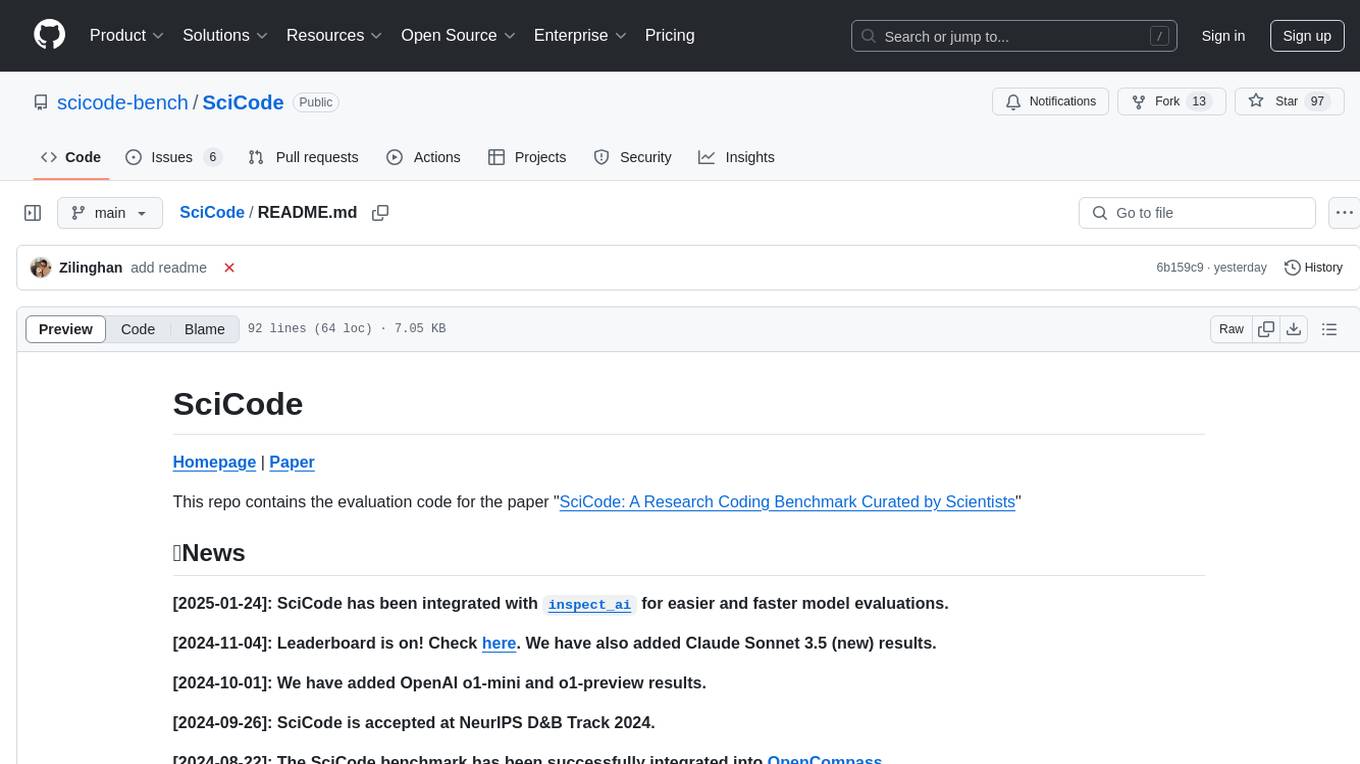
SciCode
SciCode is a challenging benchmark designed to evaluate the capabilities of language models (LMs) in generating code for solving realistic scientific research problems. It contains 338 subproblems decomposed from 80 challenging main problems across 16 subdomains from 6 domains. The benchmark offers optional descriptions specifying useful scientific background information and scientist-annotated gold-standard solutions and test cases for evaluation. SciCode demonstrates a realistic workflow of identifying critical science concepts and facts and transforming them into computation and simulation code, aiming to help showcase LLMs' progress towards assisting scientists and contribute to the future building and evaluation of scientific AI.
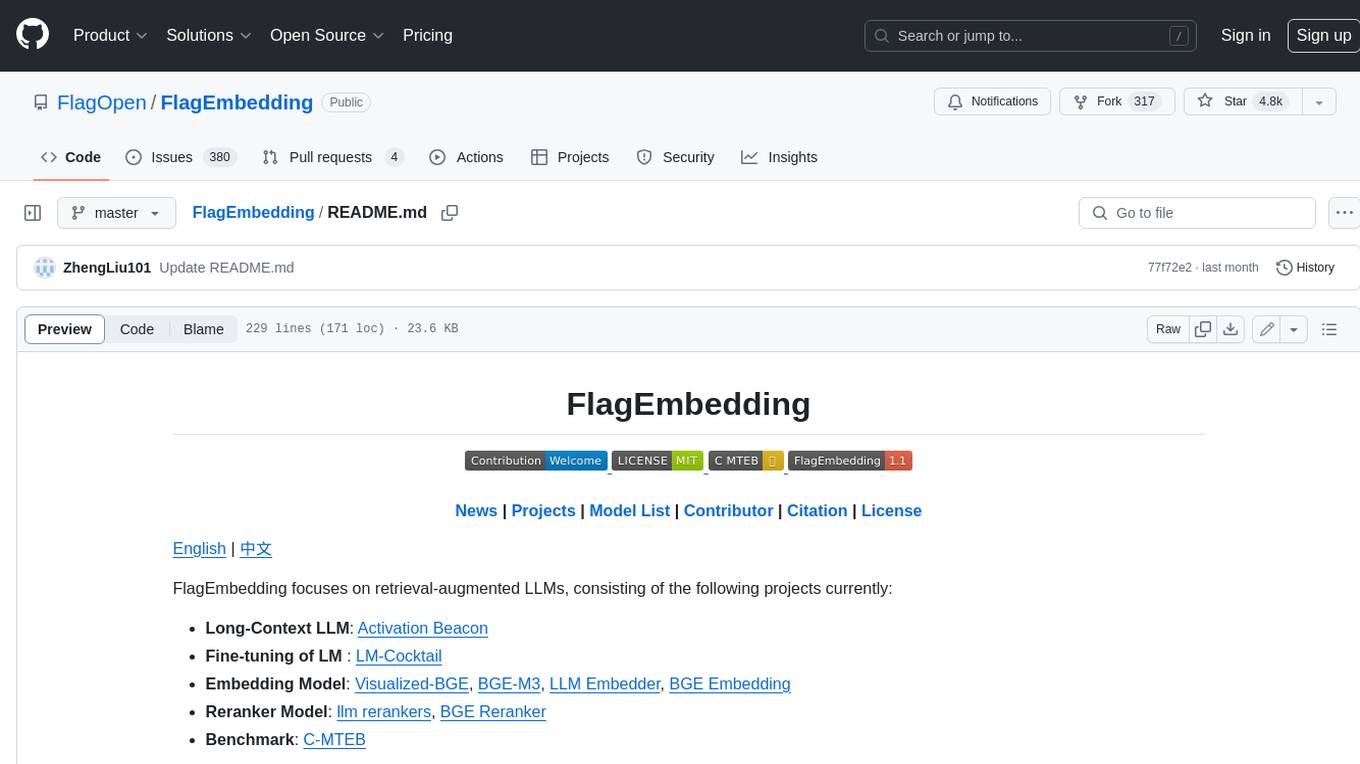
FlagEmbedding
FlagEmbedding focuses on retrieval-augmented LLMs, consisting of the following projects currently: * **Long-Context LLM** : Activation Beacon * **Fine-tuning of LM** : LM-Cocktail * **Embedding Model** : Visualized-BGE, BGE-M3, LLM Embedder, BGE Embedding * **Reranker Model** : llm rerankers, BGE Reranker * **Benchmark** : C-MTEB
For similar tasks
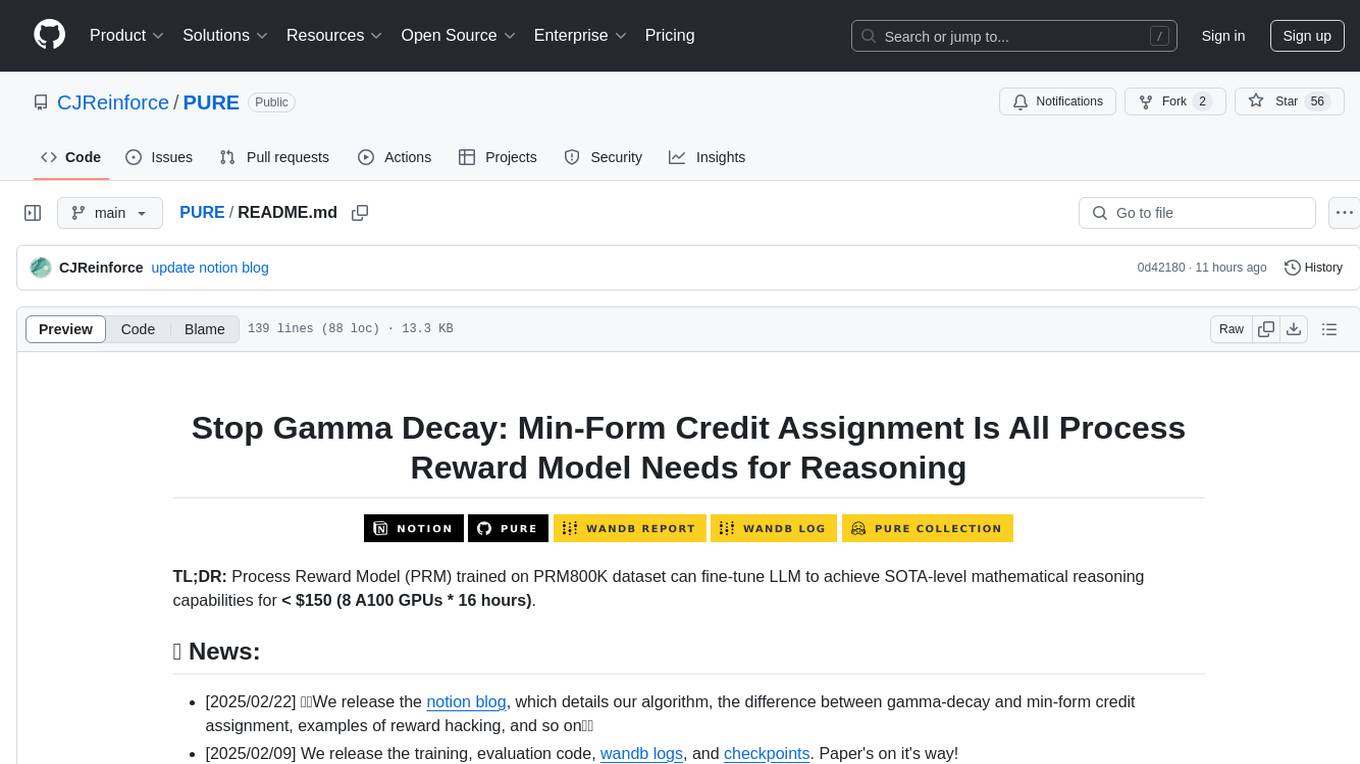
PURE
PURE (Process-sUpervised Reinforcement lEarning) is a framework that trains a Process Reward Model (PRM) on a dataset and fine-tunes a language model to achieve state-of-the-art mathematical reasoning capabilities. It uses a novel credit assignment method to calculate return and supports multiple reward types. The final model outperforms existing methods with minimal RL data or compute resources, achieving high accuracy on various benchmarks. The tool addresses reward hacking issues and aims to enhance long-range decision-making and reasoning tasks using large language models.
For similar jobs
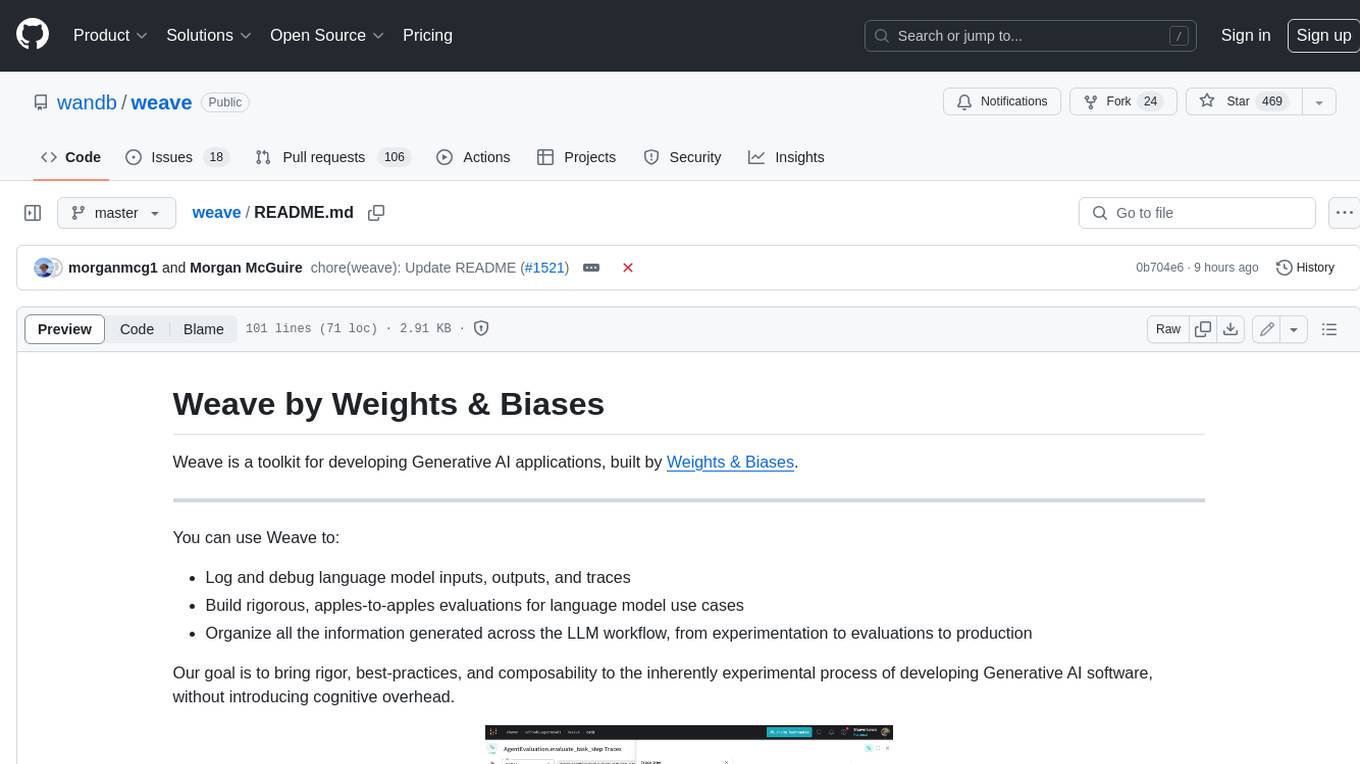
weave
Weave is a toolkit for developing Generative AI applications, built by Weights & Biases. With Weave, you can log and debug language model inputs, outputs, and traces; build rigorous, apples-to-apples evaluations for language model use cases; and organize all the information generated across the LLM workflow, from experimentation to evaluations to production. Weave aims to bring rigor, best-practices, and composability to the inherently experimental process of developing Generative AI software, without introducing cognitive overhead.
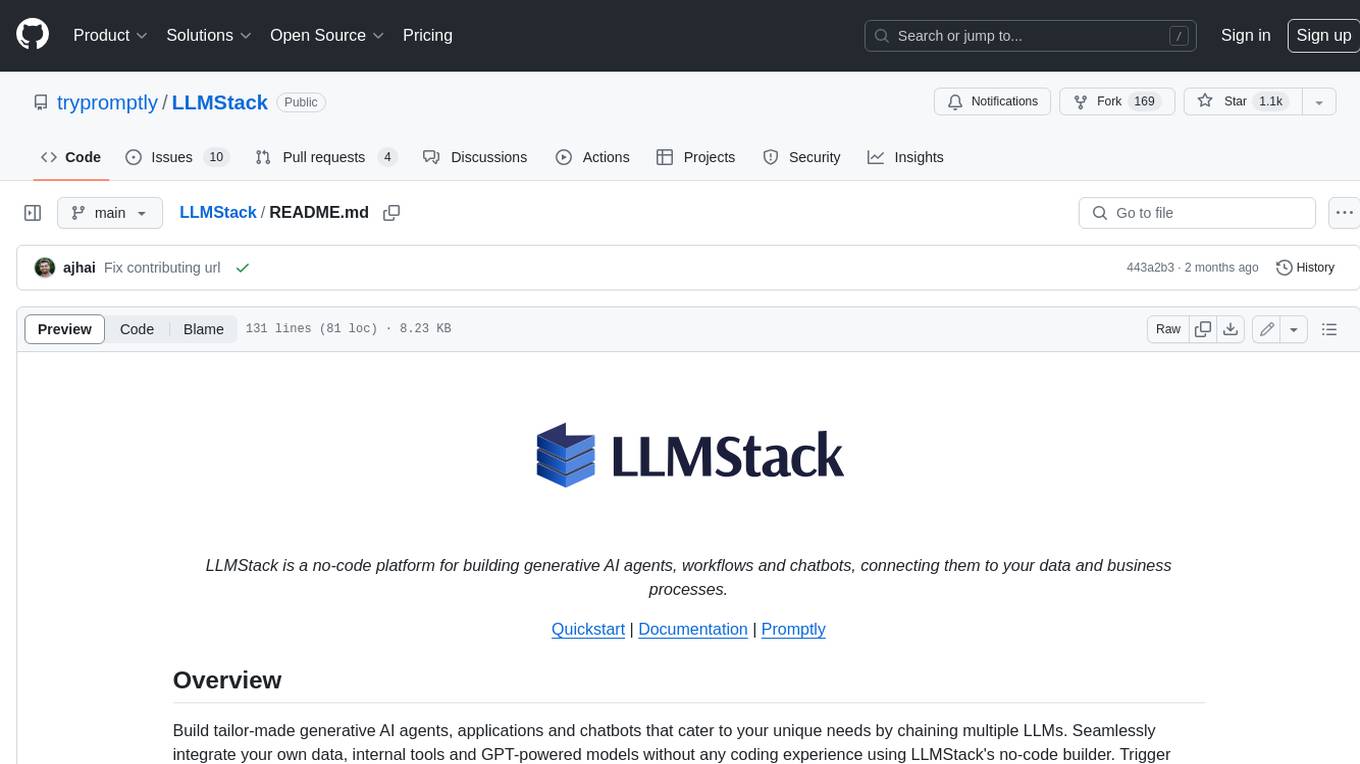
LLMStack
LLMStack is a no-code platform for building generative AI agents, workflows, and chatbots. It allows users to connect their own data, internal tools, and GPT-powered models without any coding experience. LLMStack can be deployed to the cloud or on-premise and can be accessed via HTTP API or triggered from Slack or Discord.
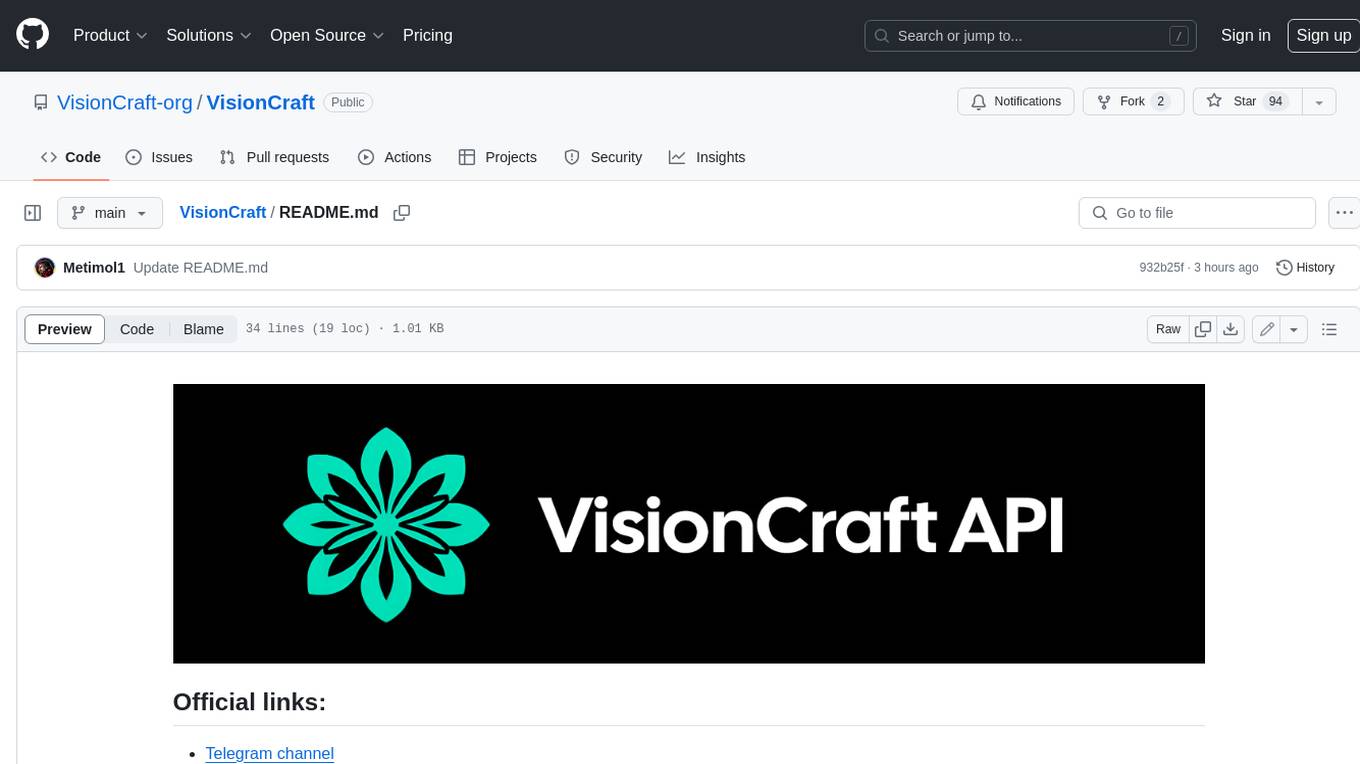
VisionCraft
The VisionCraft API is a free API for using over 100 different AI models. From images to sound.
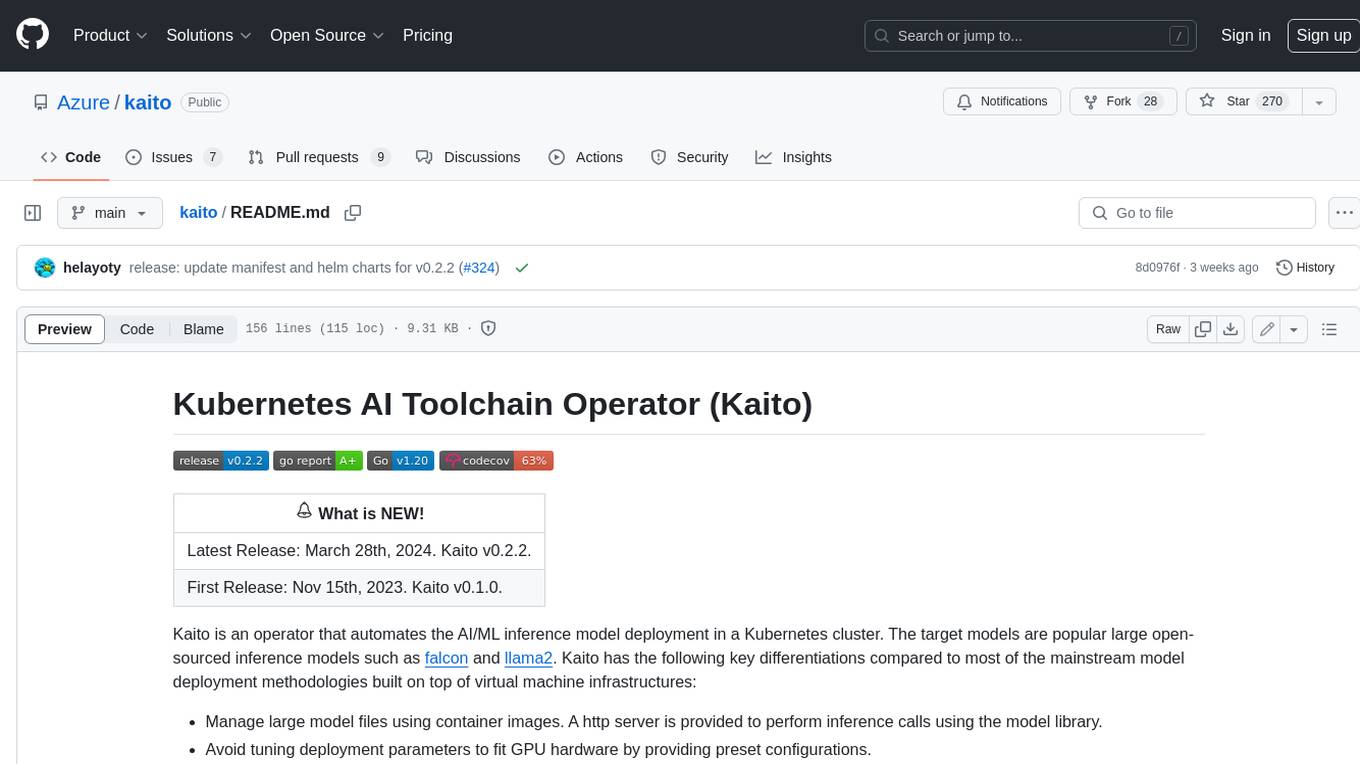
kaito
Kaito is an operator that automates the AI/ML inference model deployment in a Kubernetes cluster. It manages large model files using container images, avoids tuning deployment parameters to fit GPU hardware by providing preset configurations, auto-provisions GPU nodes based on model requirements, and hosts large model images in the public Microsoft Container Registry (MCR) if the license allows. Using Kaito, the workflow of onboarding large AI inference models in Kubernetes is largely simplified.
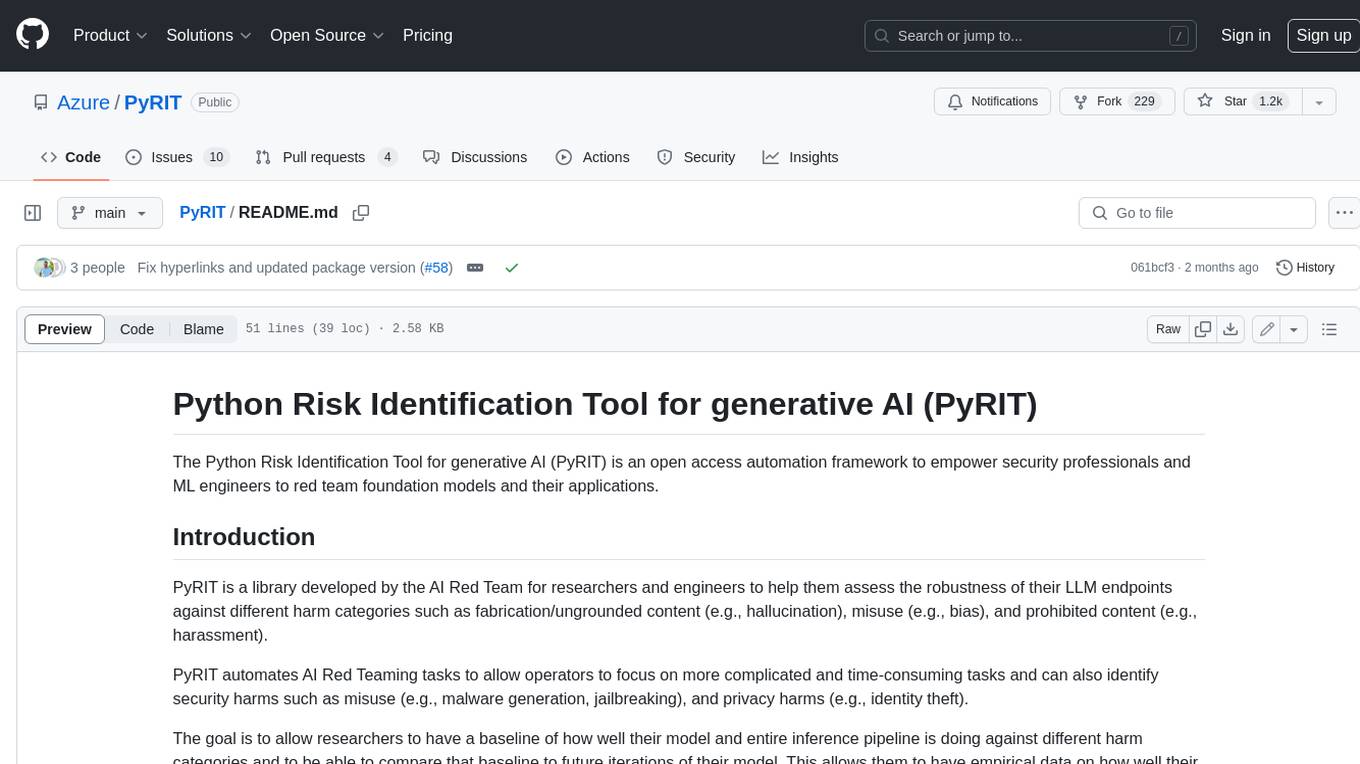
PyRIT
PyRIT is an open access automation framework designed to empower security professionals and ML engineers to red team foundation models and their applications. It automates AI Red Teaming tasks to allow operators to focus on more complicated and time-consuming tasks and can also identify security harms such as misuse (e.g., malware generation, jailbreaking), and privacy harms (e.g., identity theft). The goal is to allow researchers to have a baseline of how well their model and entire inference pipeline is doing against different harm categories and to be able to compare that baseline to future iterations of their model. This allows them to have empirical data on how well their model is doing today, and detect any degradation of performance based on future improvements.
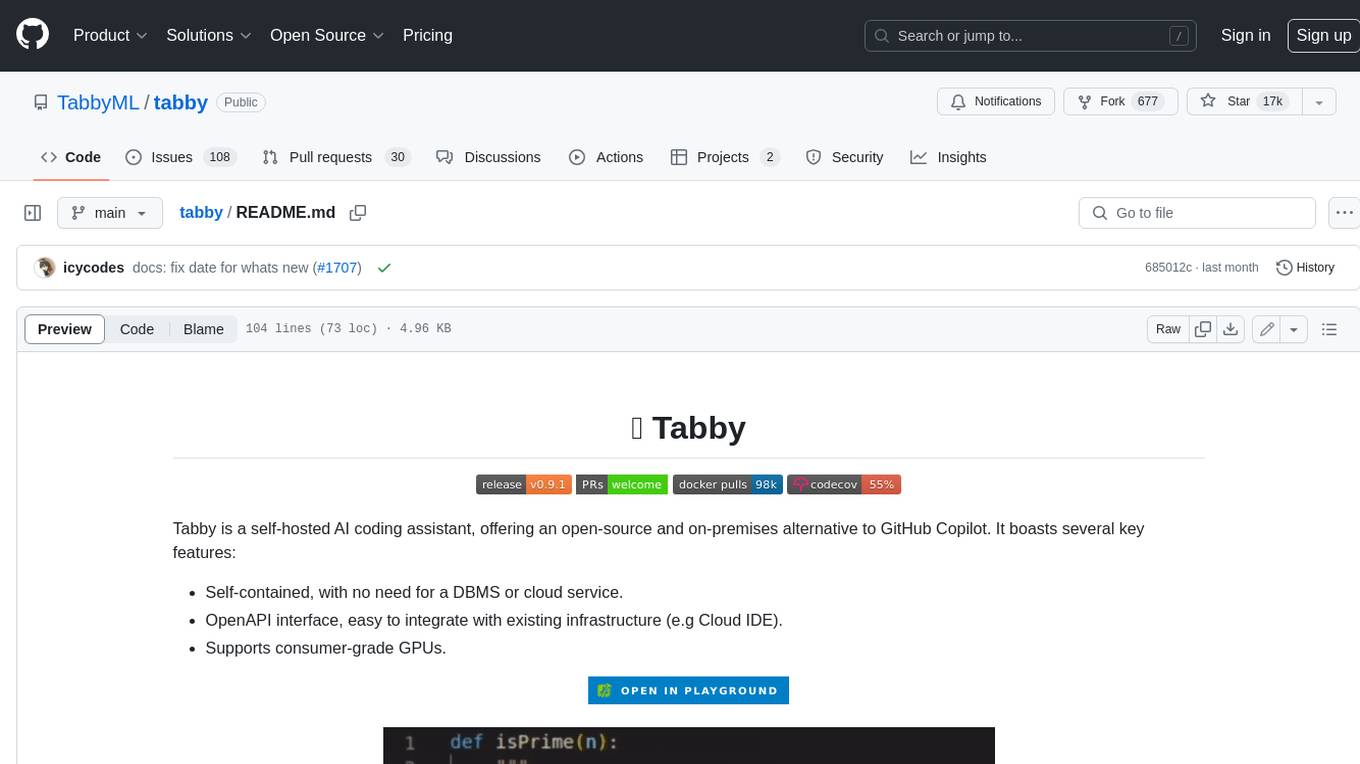
tabby
Tabby is a self-hosted AI coding assistant, offering an open-source and on-premises alternative to GitHub Copilot. It boasts several key features: * Self-contained, with no need for a DBMS or cloud service. * OpenAPI interface, easy to integrate with existing infrastructure (e.g Cloud IDE). * Supports consumer-grade GPUs.
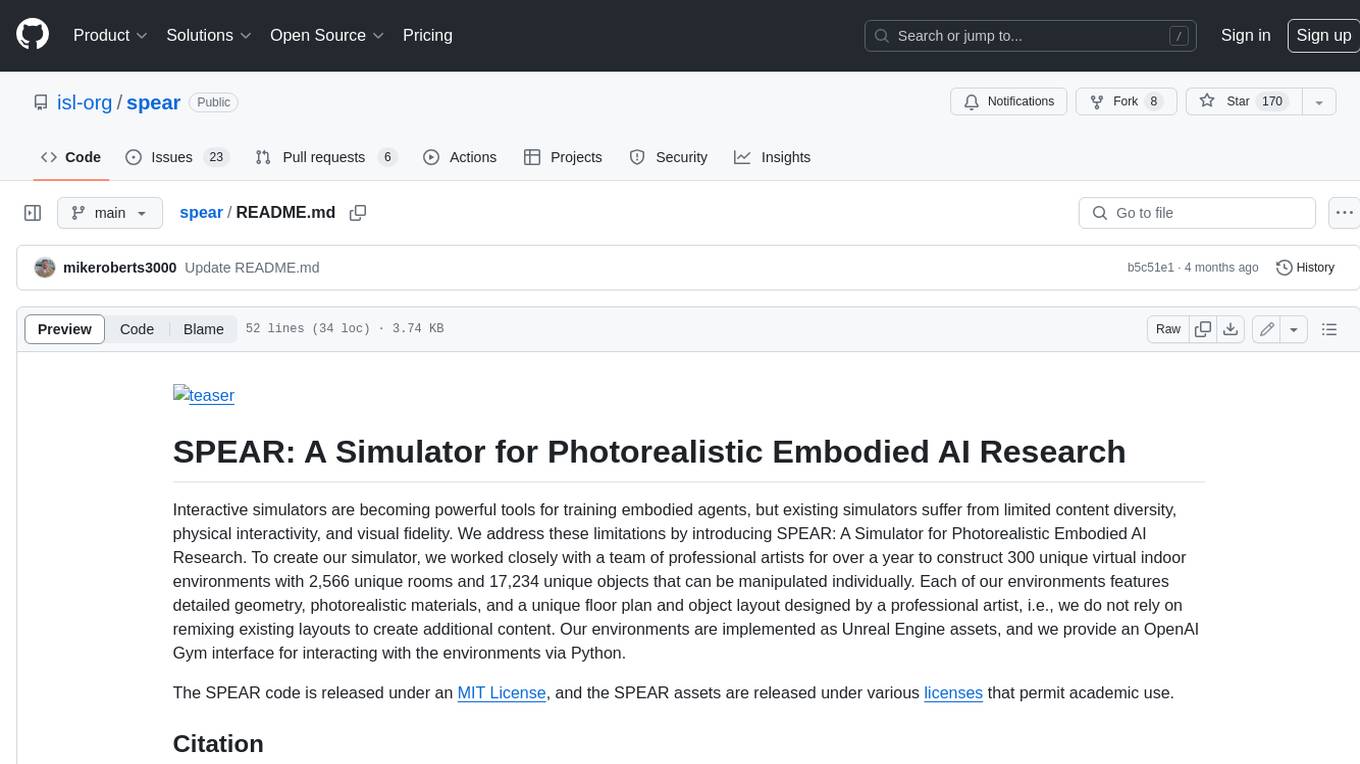
spear
SPEAR (Simulator for Photorealistic Embodied AI Research) is a powerful tool for training embodied agents. It features 300 unique virtual indoor environments with 2,566 unique rooms and 17,234 unique objects that can be manipulated individually. Each environment is designed by a professional artist and features detailed geometry, photorealistic materials, and a unique floor plan and object layout. SPEAR is implemented as Unreal Engine assets and provides an OpenAI Gym interface for interacting with the environments via Python.
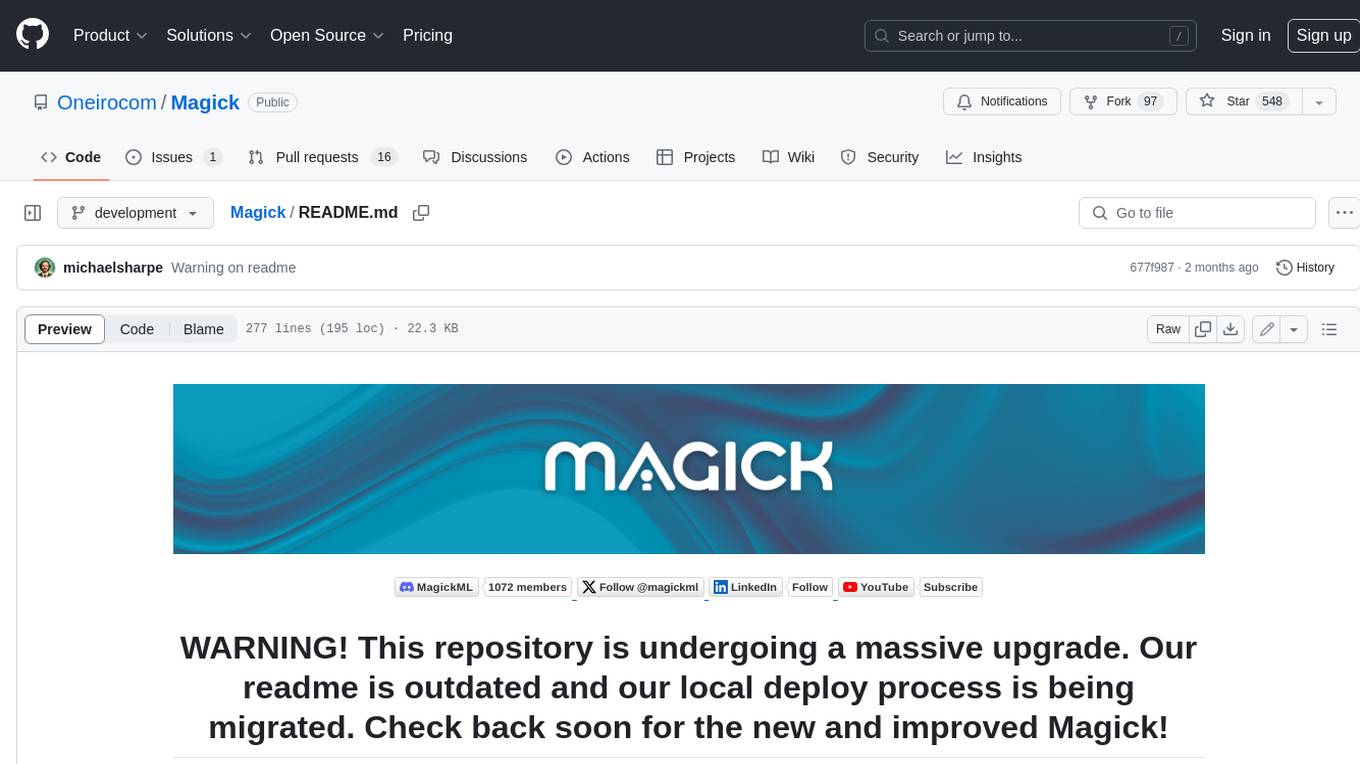
Magick
Magick is a groundbreaking visual AIDE (Artificial Intelligence Development Environment) for no-code data pipelines and multimodal agents. Magick can connect to other services and comes with nodes and templates well-suited for intelligent agents, chatbots, complex reasoning systems and realistic characters.