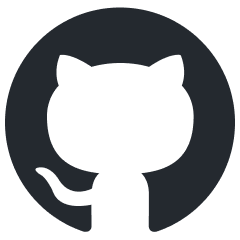
AIOS
AIOS: LLM Agent Operating System
Stars: 3320
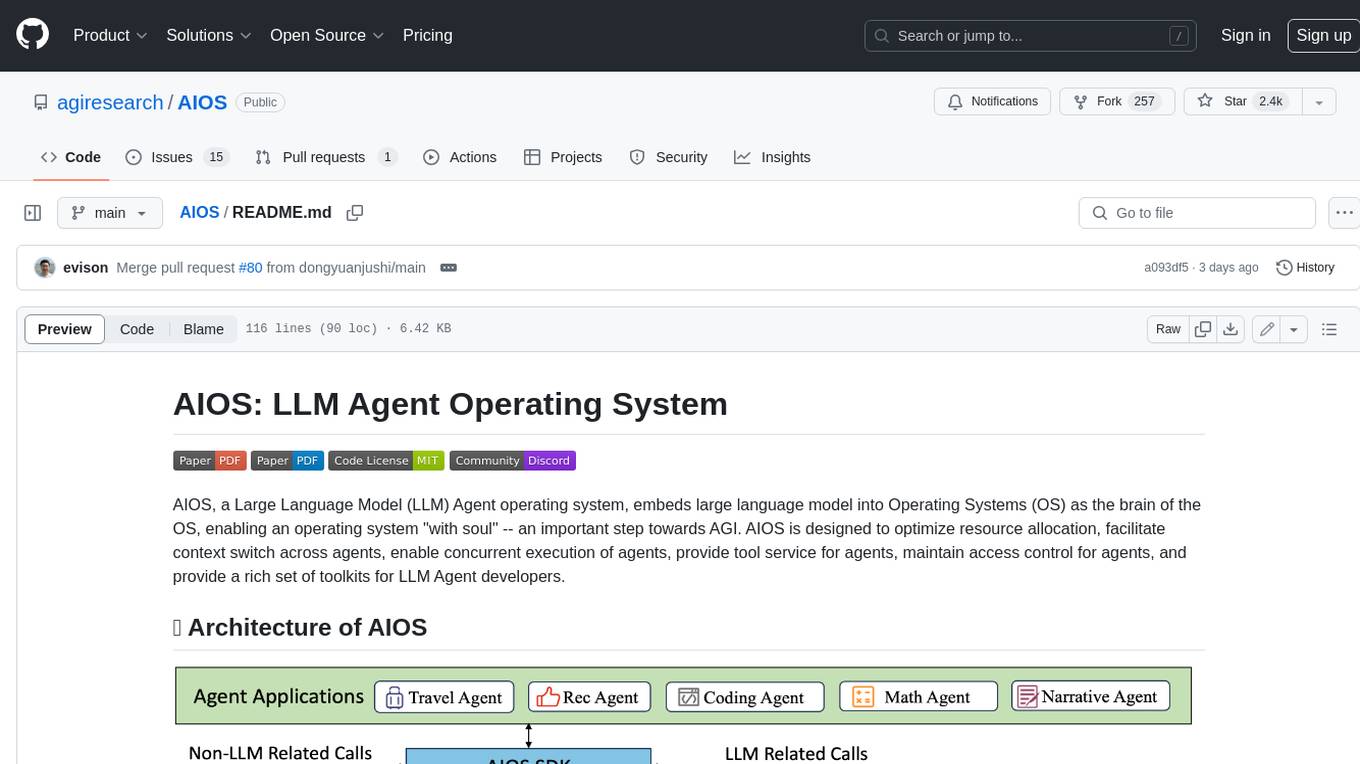
AIOS, a Large Language Model (LLM) Agent operating system, embeds large language model into Operating Systems (OS) as the brain of the OS, enabling an operating system "with soul" -- an important step towards AGI. AIOS is designed to optimize resource allocation, facilitate context switch across agents, enable concurrent execution of agents, provide tool service for agents, maintain access control for agents, and provide a rich set of toolkits for LLM Agent developers.
README:
The goal of AIOS is to build a large language model (LLM) agent operating system, which intends to embed large language model into the operating system as the brain of the OS. AIOS is designed to address problems (e.g., scheduling, context switch, memory management, etc.) during the development and deployment of LLM-based agents, for a better ecosystem among agent developers and users.
AIOS provides the LLM kernel as an abstraction on top of the OS kernel. The kernel facilitates the installation, execution and usage of agents. Furthermore, the AIOS SDK facilitates the development and deployment of agents.
- [2024-09-01] π₯ AIOS supports multiple agent creation frameworks (e.g., ReAct, Reflexion, OpenAGI, AutoGen, Open Interpreter, MetaGPT). Agents created by these frameworks can onboard AIOS. Onboarding guidelines can be found at the Doc.
- [2024-07-10] π AIOS documentation template is up: Code and Website.
- [2024-06-20] π₯ Function calling for open-sourced LLMs (native huggingface, vllm, ollama) is supported.
- [2024-05-20] π More agents with ChatGPT-based tool calling are added (i.e., MathAgent, RecAgent, TravelAgent, AcademicAgent and CreationAgent), their profiles and workflows can be found in OpenAGI.
- [2024-05-13] π οΈ Local models (diffusion models) as tools from HuggingFace are integrated.
- [2024-05-01] π οΈ The agent creation in AIOS is refactored, which can be found in our OpenAGI package.
- [2024-04-05] π οΈ AIOS currently supports external tool callings (google search, wolframalpha, rapid API, etc).
- [2024-04-02] π€ AIOS Discord Community is up. Welcome to join the community for discussions, brainstorming, development, or just random chats! For how to contribute to AIOS, please see CONTRIBUTE.
-
[2024-03-25]
βοΈ Our paper AIOS: LLM Agent Operating System is released! - [2023-12-06] π After several months of working, our perspective paper LLM as OS, Agents as Apps: Envisioning AIOS, Agents and the AIOS-Agent Ecosystem is officially released.
Please see our ongoing documentation for more information.
Git clone AIOS
git clone https://github.com/agiresearch/AIOS.git
cd AIOS
Create venv environment (recommended)
python -m venv venv
source venv/bin/activate
or create conda environment
conda create -n venv python=3.10 # For Python 3.10
conda create -n venv python=3.11 # For Python 3.11
conda activate venv
If you have GPU environments, you can install the dependencies using
pip install -r requirements-cuda.txt
or else you can install the dependencies using
pip install -r requirements.txt
[!TIP]
For the config of LLM endpoints, multiple API keys may be required to set up. Here we provide the .env.example to for easier configuration of these API keys, you can just copy .env.example as .env and set up the required keys based on your needs.
Note: Please use launch.py
for the WebUI, or agent_repl.py
for the TUI.
You need to get your OpenAI API key from https://platform.openai.com/api-keys. Then set up your OpenAI API key as an environment variable
export OPENAI_API_KEY=<YOUR_OPENAI_API_KEY>
Then run main.py with the models provided by OpenAI API
python main.py --llm_name gpt-3.5-turbo # use gpt-3.5-turbo for example
You need to get your Gemini API key from https://ai.google.dev/gemini-api
export GEMINI_API_KEY=<YOUR_GEMINI_API_KEY>
Then run main.py with the models provided by OpenAI API
python main.py --llm_name gemini-1.5-flash # use gemini-1.5-flash for example
If you want to use open-sourced models provided by huggingface, here we provide three options:
- Use with ollama
- Use with native huggingface models
- Use with vllm
You need to download ollama from from https://ollama.com/.
Then you need to start the ollama server either from ollama app
or using the following command in the terminal
ollama serve
To use models provided by ollama, you need to pull the available models from https://ollama.com/library
ollama pull llama3:8b # use llama3:8b for example
ollama can support CPU-only environment, so if you do not have CUDA environment
You can run aios with ollama models by
python main.py --llm_name ollama/llama3:8b --use_backend ollama # use ollama/llama3:8b for example
However, if you have the GPU environment, you can also pass GPU-related parameters to speed up using the following command
python main.py --llm_name ollama/llama3:8b --use_backend ollama --max_gpu_memory '{"0": "24GB"}' --eval_device "cuda:0" --max_new_tokens 256
Some of the huggingface models require authentification, if you want to use all of the models you need to set up your authentification token in https://huggingface.co/settings/tokens and set up it as an environment variable using the following command
export HF_AUTH_TOKENS=<YOUR_TOKEN_ID>
You can run with the
python main.py --llm_name meta-llama/Meta-Llama-3-8B-Instruct --max_gpu_memory '{"0": "24GB"}' --eval_device "cuda:0" --max_new_tokens 256
By default, huggingface will download the models in the ~/.cache
directory.
If you want to designate the download directory, you can set up it using the following command
export HF_HOME=<YOUR_HF_HOME>
If you want to speed up the inference of huggingface models, you can use vllm as the backend.
[!NOTE]
It is important to note that vllm currently only supports linux and GPU-enabled environment. So if you do not have the environment, you need to choose other options.
Considering that vllm itself does not support passing designated GPU ids, you need to either setup the environment variable,
export CUDA_VISIBLE_DEVICES="0" # replace with your designated gpu ids
Then run the command
python main.py --llm_name meta-llama/Meta-Llama-3-8B-Instruct --use_backend vllm --max_gpu_memory '{"0": "24GB"}' --eval_device "cuda:0" --max_new_tokens 256
or you can pass the CUDA_VISIBLE_DEVICES
as the prefix
CUDA_VISIBLE_DEVICES=0 python main.py --llm_name meta-llama/Meta-Llama-3-8B-Instruct --use_backend vllm --max_gpu_memory '{"0": "24GB"}' --eval_device "cuda:0" --max_new_tokens 256
- Supported versions: Python 3.9 - 3.11
- Supported versions: LTS support ONLY
Run the launch.py to start both the frontend and backend
python launch.py
which should open up https://localhost:3000
(if it doesn't, navigate to that on your browser)
Interact with all agents by using the @
to tag an agent.
@article{mei2024aios,
title={AIOS: LLM Agent Operating System},
author={Mei, Kai and Li, Zelong and Xu, Shuyuan and Ye, Ruosong and Ge, Yingqiang and Zhang, Yongfeng}
journal={arXiv:2403.16971},
year={2024}
}
@article{ge2023llm,
title={LLM as OS, Agents as Apps: Envisioning AIOS, Agents and the AIOS-Agent Ecosystem},
author={Ge, Yingqiang and Ren, Yujie and Hua, Wenyue and Xu, Shuyuan and Tan, Juntao and Zhang, Yongfeng},
journal={arXiv:2312.03815},
year={2023}
}
For how to contribute, see CONTRIBUTE. If you would like to contribute to the codebase, issues or pull requests are always welcome!
If you would like to join the community, ask questions, chat with fellows, learn about or propose new features, and participate in future developments, join our Discord Community!
For issues related to AIOS development, we encourage submitting issues, pull requests, or initiating discussions in AIOS Discord Channel. For other issues please feel free to contact AIOS Foundation ([email protected]).
For Tasks:
Click tags to check more tools for each tasksFor Jobs:
Alternative AI tools for AIOS
Similar Open Source Tools
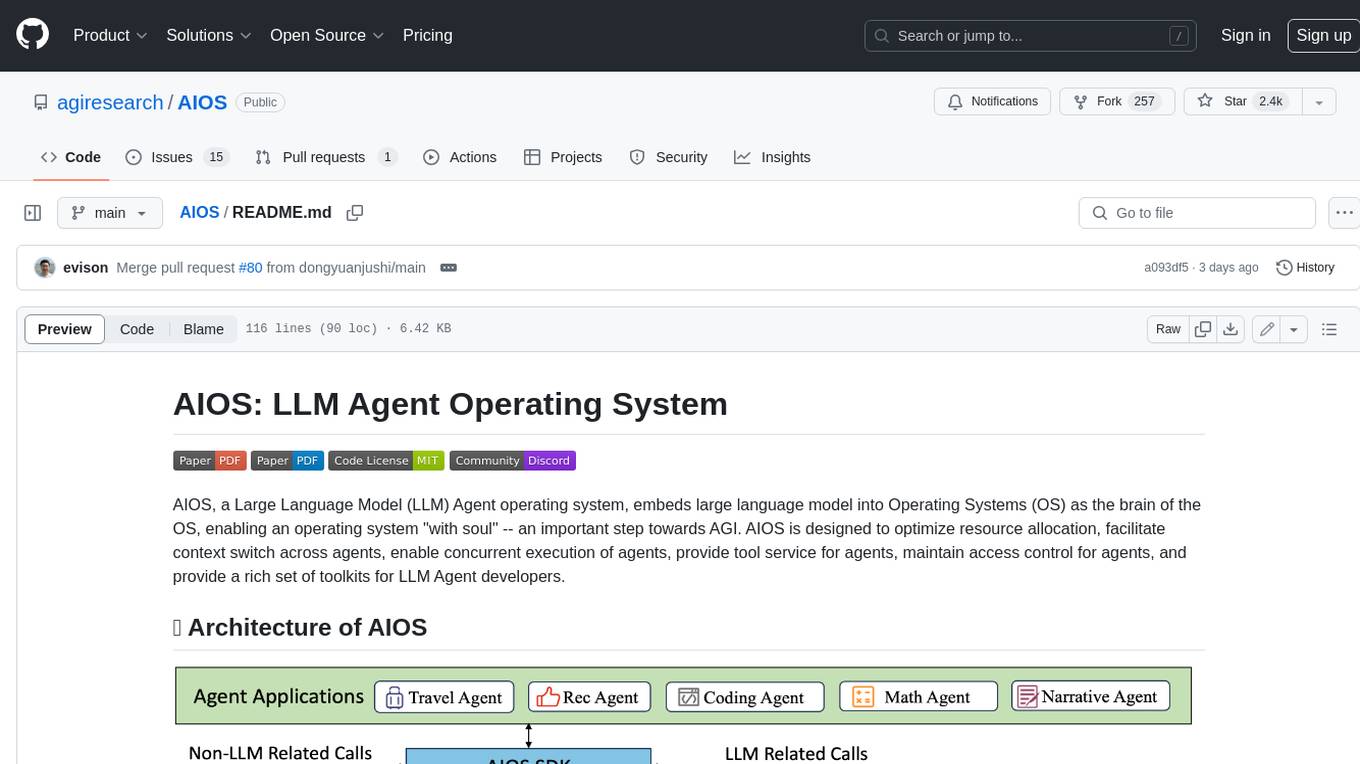
AIOS
AIOS, a Large Language Model (LLM) Agent operating system, embeds large language model into Operating Systems (OS) as the brain of the OS, enabling an operating system "with soul" -- an important step towards AGI. AIOS is designed to optimize resource allocation, facilitate context switch across agents, enable concurrent execution of agents, provide tool service for agents, maintain access control for agents, and provide a rich set of toolkits for LLM Agent developers.
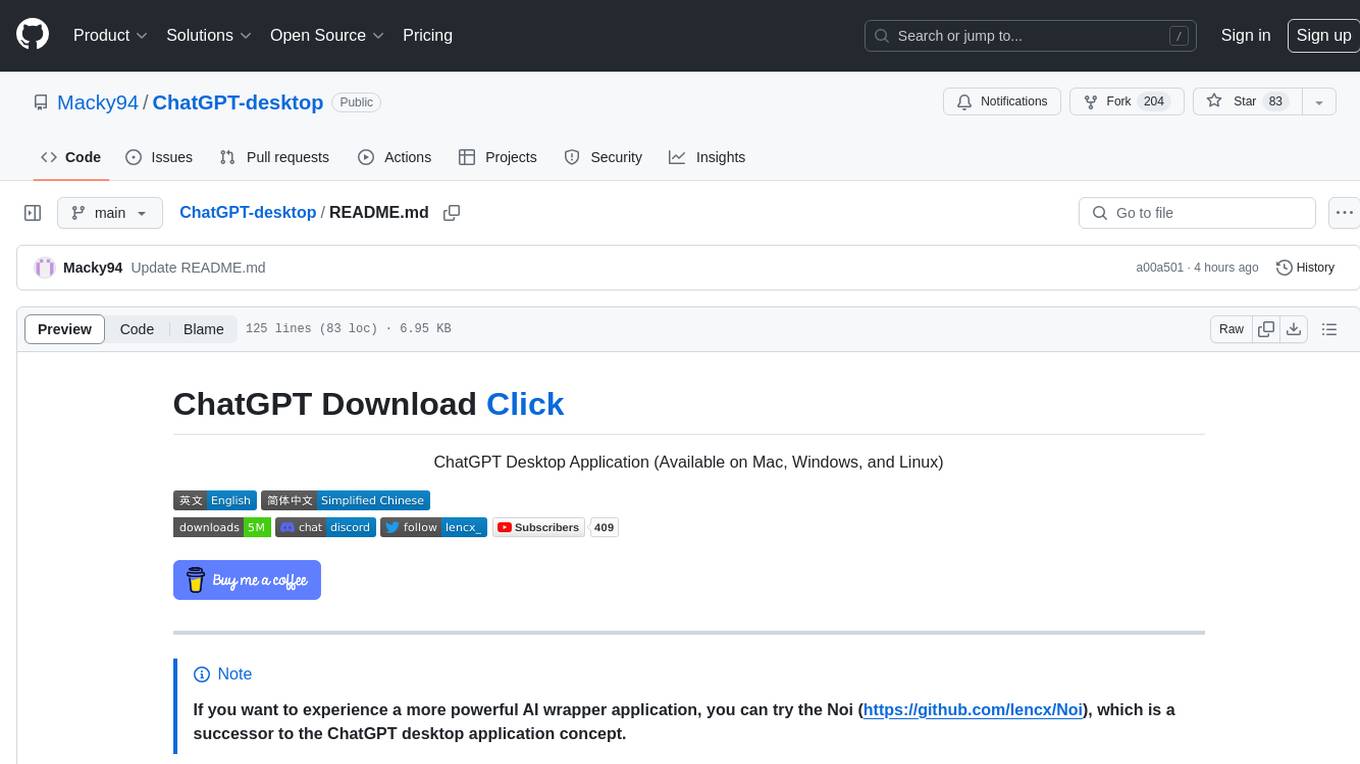
ChatGPT-desktop
ChatGPT Desktop Application is a multi-platform tool that provides a powerful AI wrapper for generating text. It offers features like text-to-speech, exporting chat history in various formats, automatic application upgrades, system tray hover window, support for slash commands, customization of global shortcuts, and pop-up search. The application is built using Tauri and aims to enhance user experience by simplifying text generation tasks. It is available for Mac, Windows, and Linux, and is designed for personal learning and research purposes.
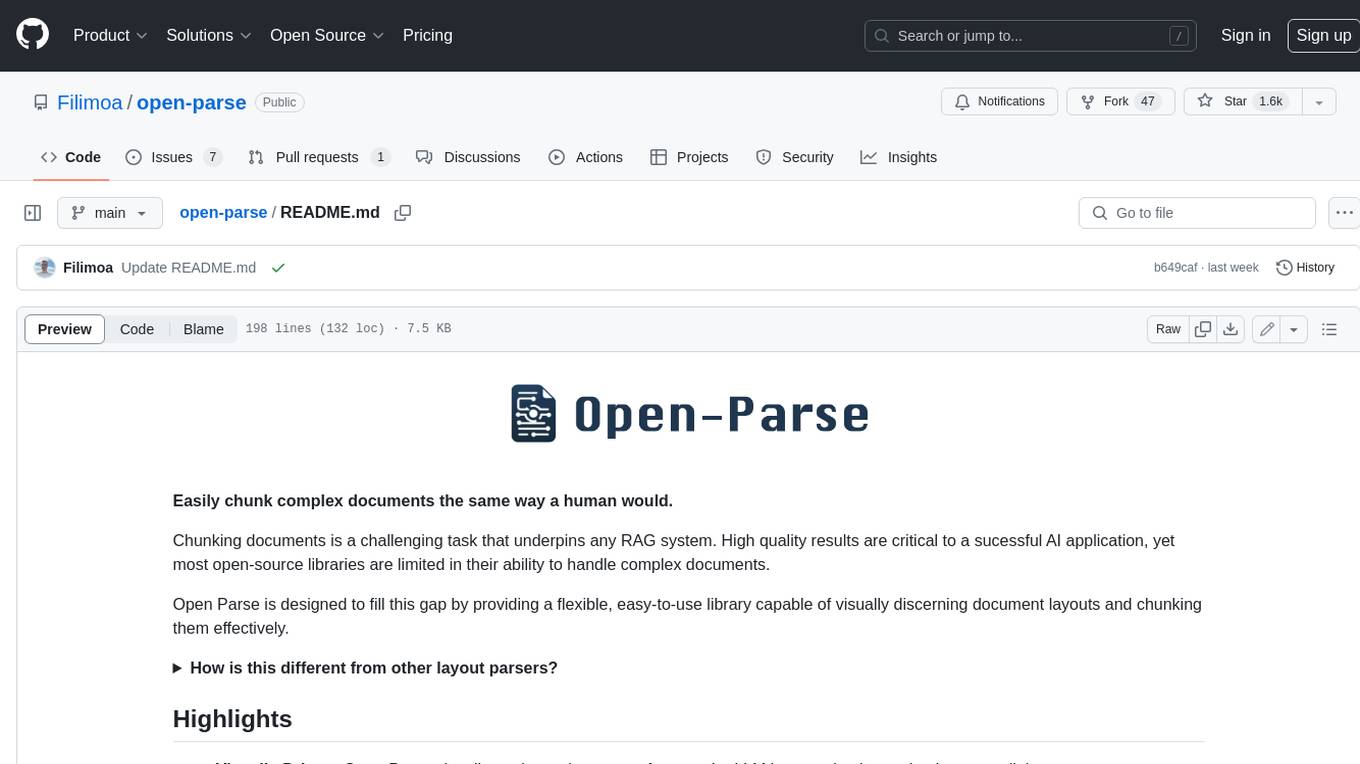
open-parse
Open Parse is a Python library for visually discerning document layouts and chunking them effectively. It is designed to fill the gap in open-source libraries for handling complex documents. Unlike text splitting, which converts a file to raw text and slices it up, Open Parse visually analyzes documents for superior LLM input. It also supports basic markdown for parsing headings, bold, and italics, and has high-precision table support, extracting tables into clean Markdown formats with accuracy that surpasses traditional tools. Open Parse is extensible, allowing users to easily implement their own post-processing steps. It is also intuitive, with great editor support and completion everywhere, making it easy to use and learn.
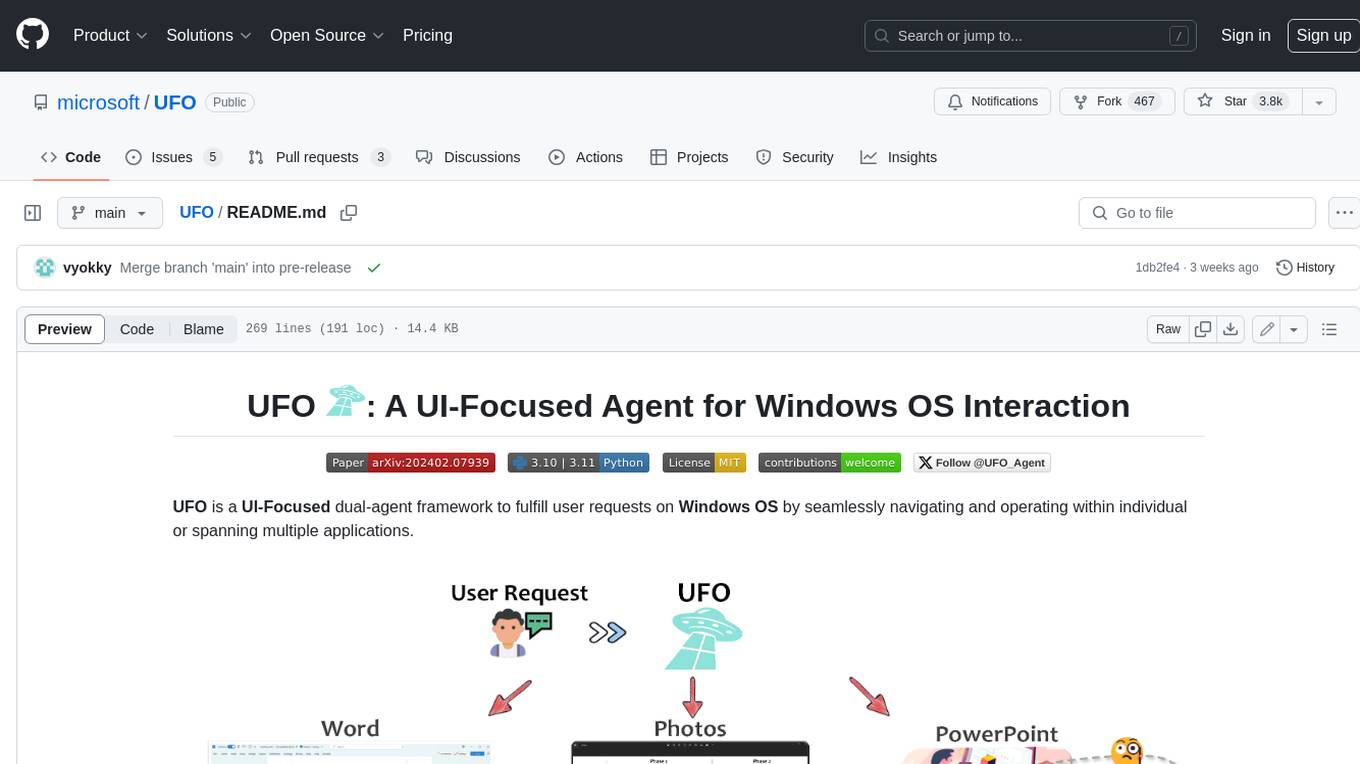
UFO
UFO is a UI-focused dual-agent framework to fulfill user requests on Windows OS by seamlessly navigating and operating within individual or spanning multiple applications.
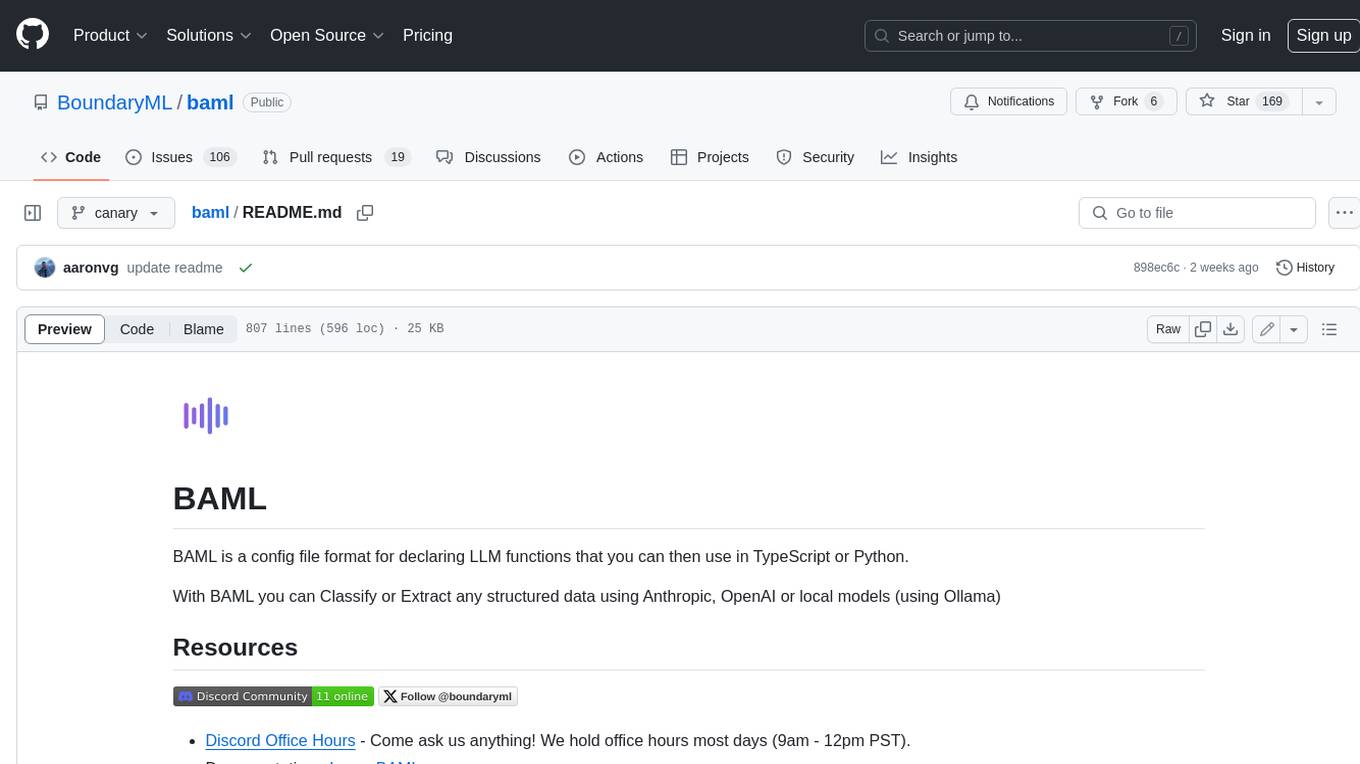
baml
BAML is a config file format for declaring LLM functions that you can then use in TypeScript or Python. With BAML you can Classify or Extract any structured data using Anthropic, OpenAI or local models (using Ollama) ## Resources  [Discord Community](https://discord.gg/boundaryml)  [Follow us on Twitter](https://twitter.com/boundaryml) * Discord Office Hours - Come ask us anything! We hold office hours most days (9am - 12pm PST). * Documentation - Learn BAML * Documentation - BAML Syntax Reference * Documentation - Prompt engineering tips * Boundary Studio - Observability and more #### Starter projects * BAML + NextJS 14 * BAML + FastAPI + Streaming ## Motivation Calling LLMs in your code is frustrating: * your code uses types everywhere: classes, enums, and arrays * but LLMs speak English, not types BAML makes calling LLMs easy by taking a type-first approach that lives fully in your codebase: 1. Define what your LLM output type is in a .baml file, with rich syntax to describe any field (even enum values) 2. Declare your prompt in the .baml config using those types 3. Add additional LLM config like retries or redundancy 4. Transpile the .baml files to a callable Python or TS function with a type-safe interface. (VSCode extension does this for you automatically). We were inspired by similar patterns for type safety: protobuf and OpenAPI for RPCs, Prisma and SQLAlchemy for databases. BAML guarantees type safety for LLMs and comes with tools to give you a great developer experience:  Jump to BAML code or how Flexible Parsing works without additional LLM calls. | BAML Tooling | Capabilities | | ----------------------------------------------------------------------------------------- | ---------------------------------------------------------------------------------------------------------------------------------------------------------------------------------------------------------------------------------------------------------------------------------------------------------------------------------- | | BAML Compiler install | Transpiles BAML code to a native Python / Typescript library (you only need it for development, never for releases) Works on Mac, Windows, Linux  | | VSCode Extension install | Syntax highlighting for BAML files Real-time prompt preview Testing UI | | Boundary Studio open (not open source) | Type-safe observability Labeling |
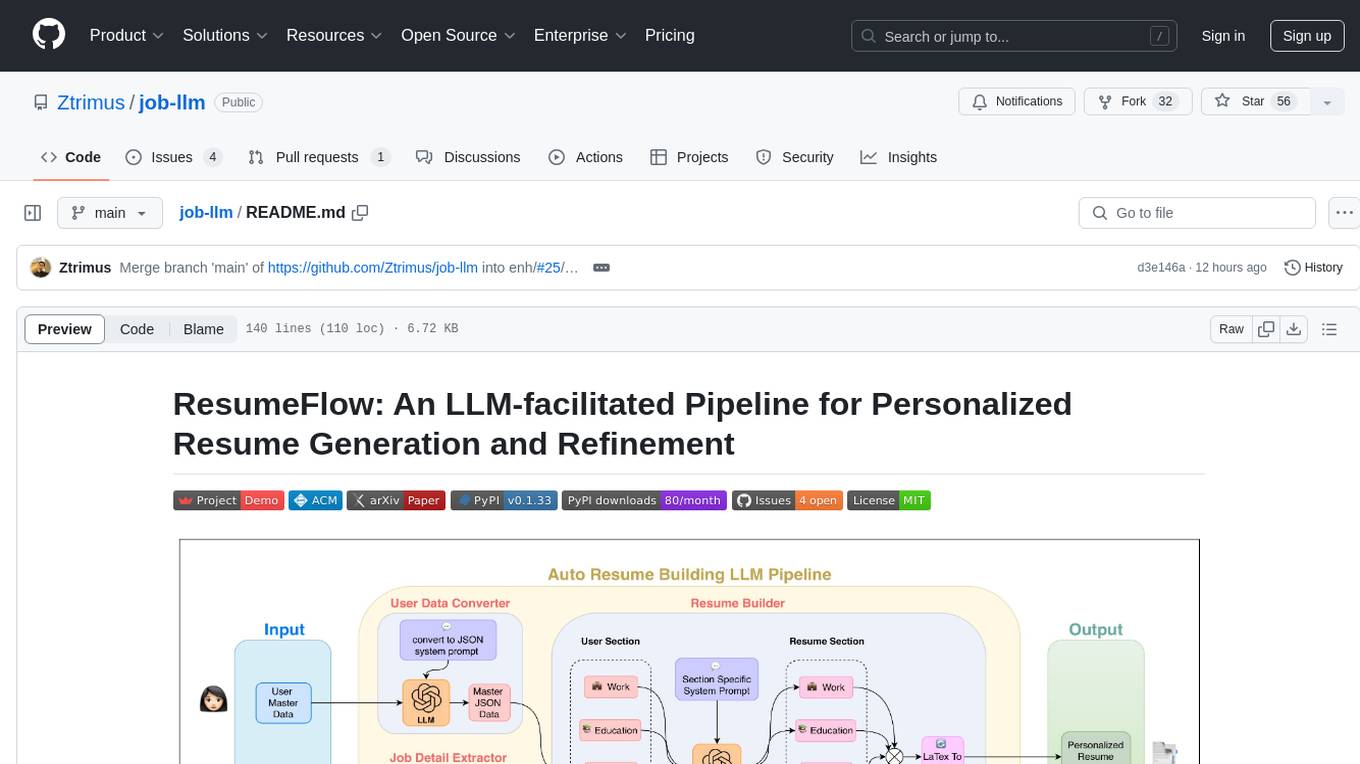
job-llm
ResumeFlow is an automated system utilizing Large Language Models (LLMs) to streamline the job application process. It aims to reduce human effort in various steps of job hunting by integrating LLM technology. Users can access ResumeFlow as a web tool, install it as a Python package, or download the source code. The project focuses on leveraging LLMs to automate tasks such as resume generation and refinement, making job applications smoother and more efficient.
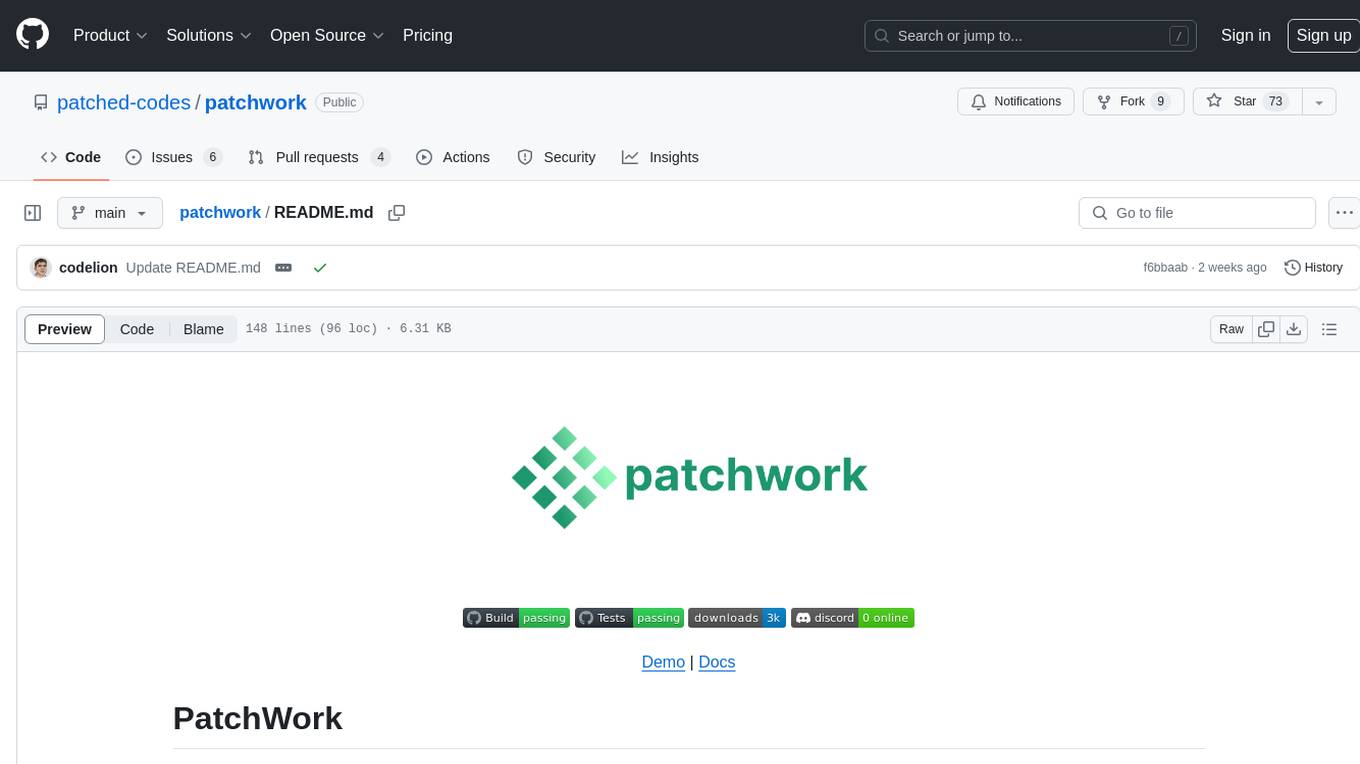
patchwork
PatchWork is an open-source framework designed for automating development tasks using large language models. It enables users to automate workflows such as PR reviews, bug fixing, security patching, and more through a self-hosted CLI agent and preferred LLMs. The framework consists of reusable atomic actions called Steps, customizable LLM prompts known as Prompt Templates, and LLM-assisted automations called Patchflows. Users can run Patchflows locally in their CLI/IDE or as part of CI/CD pipelines. PatchWork offers predefined patchflows like AutoFix, PRReview, GenerateREADME, DependencyUpgrade, and ResolveIssue, with the flexibility to create custom patchflows. Prompt templates are used to pass queries to LLMs and can be customized. Contributions to new patchflows, steps, and the core framework are encouraged, with chat assistants available to aid in the process. The roadmap includes expanding the patchflow library, introducing a debugger and validation module, supporting large-scale code embeddings, parallelization, fine-tuned models, and an open-source GUI. PatchWork is licensed under AGPL-3.0 terms, while custom patchflows and steps can be shared using the Apache-2.0 licensed patchwork template repository.
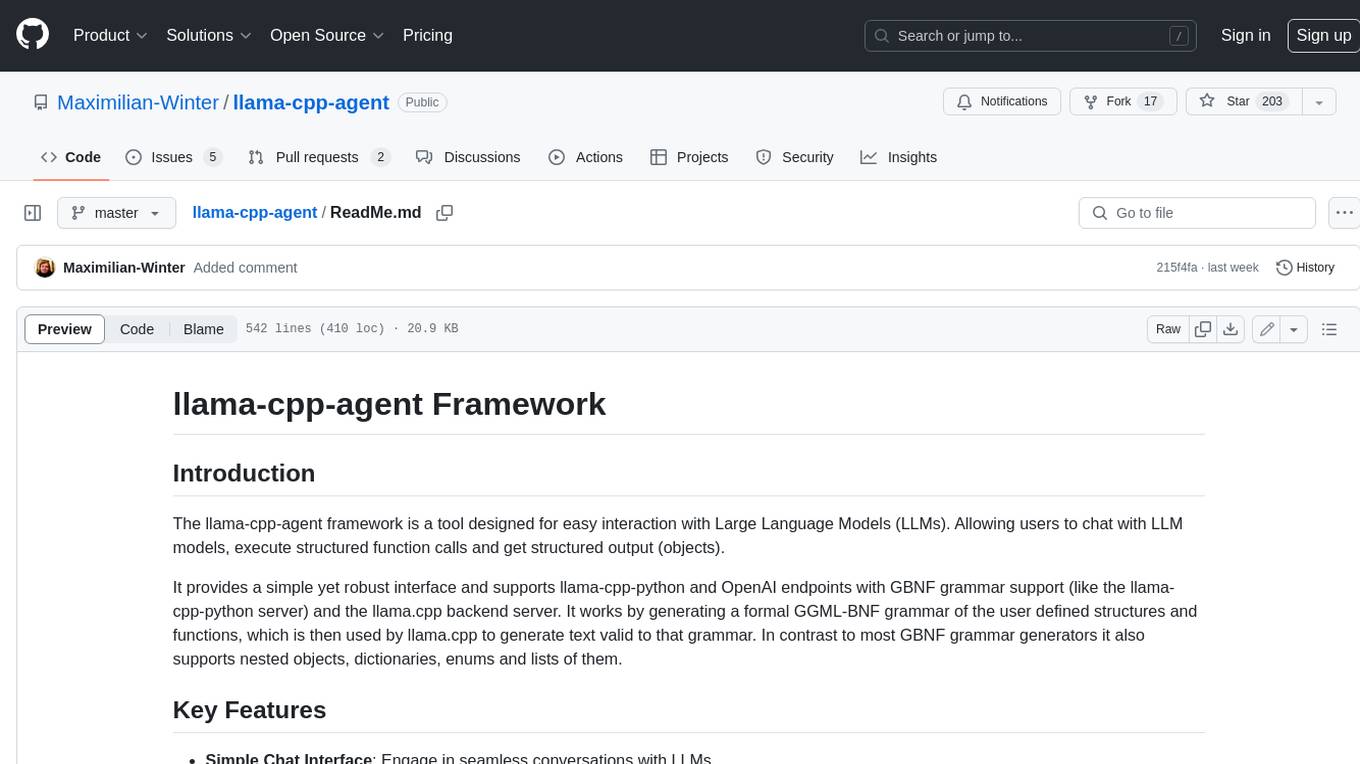
llama-cpp-agent
The llama-cpp-agent framework is a tool designed for easy interaction with Large Language Models (LLMs). Allowing users to chat with LLM models, execute structured function calls and get structured output (objects). It provides a simple yet robust interface and supports llama-cpp-python and OpenAI endpoints with GBNF grammar support (like the llama-cpp-python server) and the llama.cpp backend server. It works by generating a formal GGML-BNF grammar of the user defined structures and functions, which is then used by llama.cpp to generate text valid to that grammar. In contrast to most GBNF grammar generators it also supports nested objects, dictionaries, enums and lists of them.
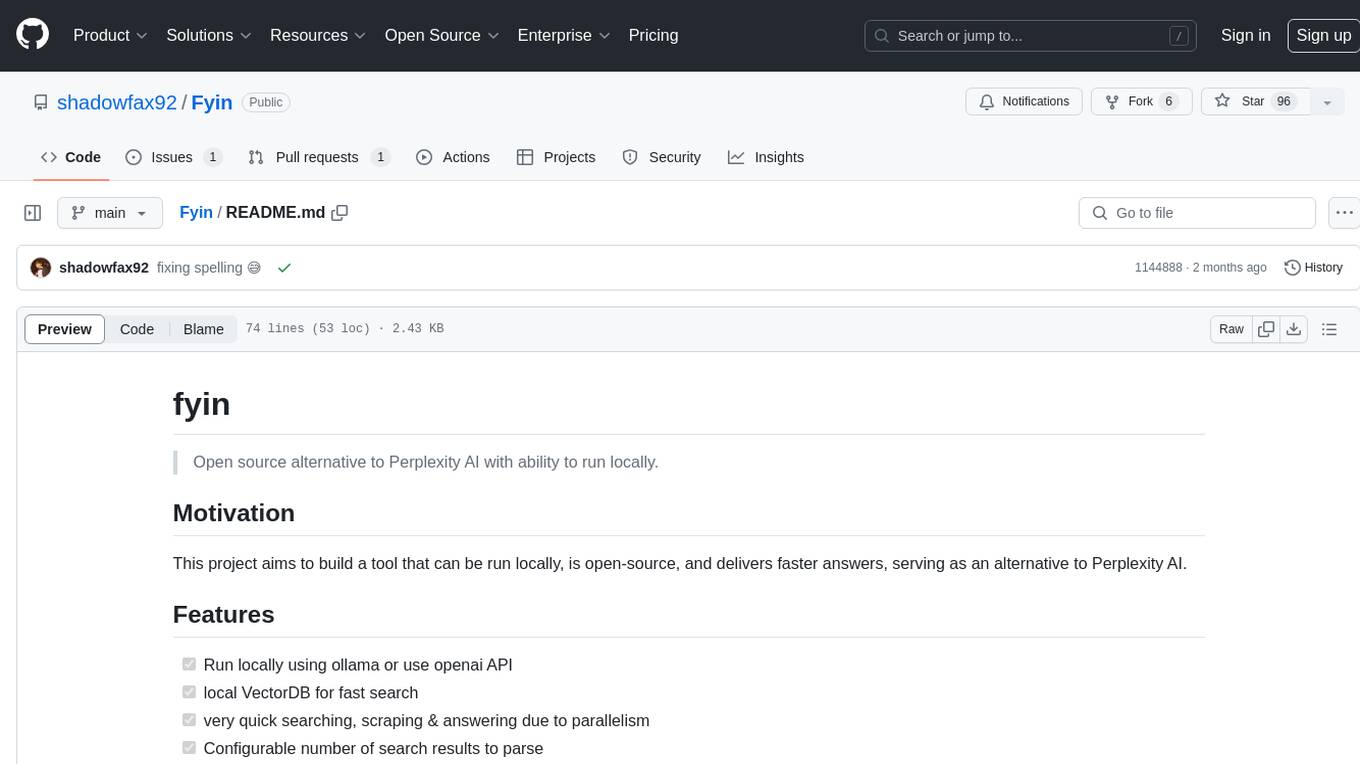
Fyin
Fyin is an open-source tool that serves as an alternative to Perplexity AI, allowing users to run it locally for faster answers. It features the ability to run locally using ollama or OpenAI API, a local VectorDB for fast search, quick searching, scraping & answering due to parallelism, configurable number of search results to parse, and local scraping of websites. The tool aims to provide a more efficient and customizable solution for obtaining answers through search and scraping functionalities.
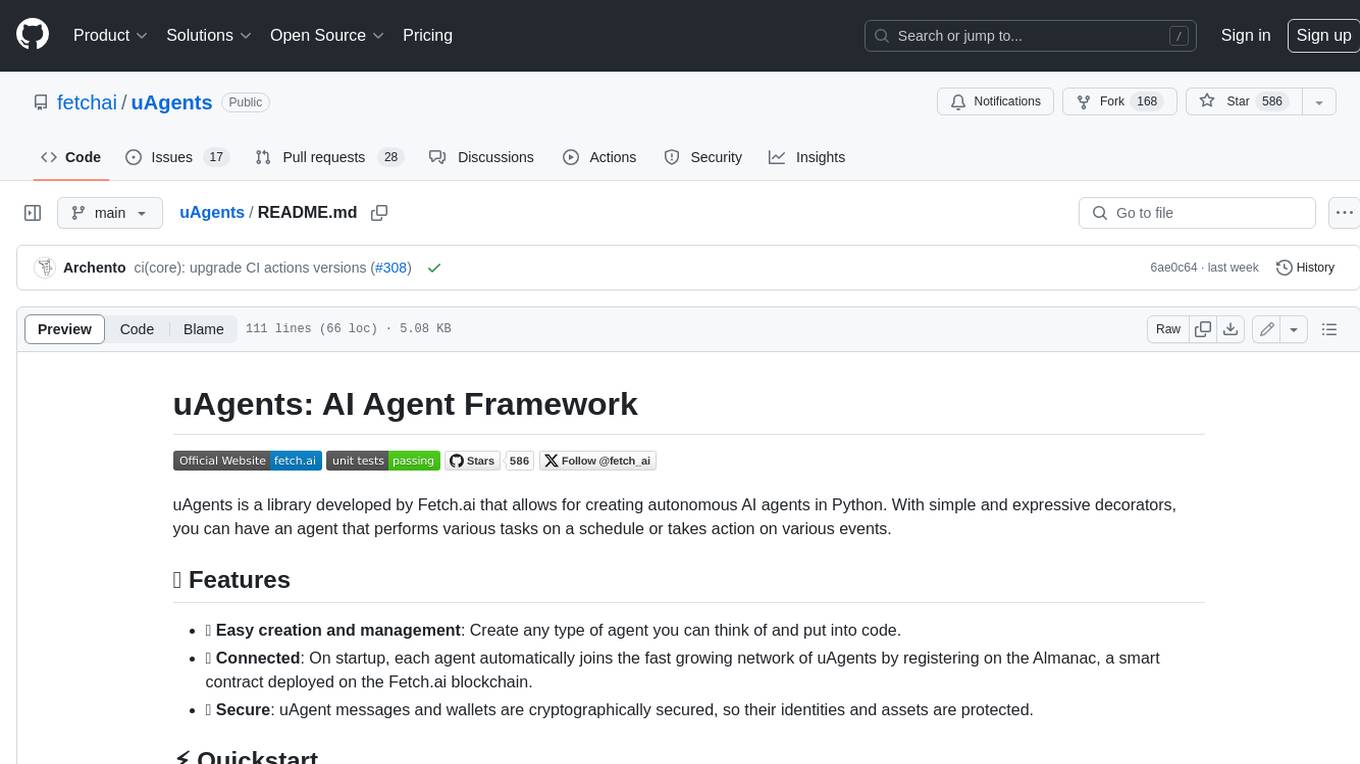
uAgents
uAgents is a Python library developed by Fetch.ai that allows for the creation of autonomous AI agents. These agents can perform various tasks on a schedule or take action on various events. uAgents are easy to create and manage, and they are connected to a fast-growing network of other uAgents. They are also secure, with cryptographically secured messages and wallets.
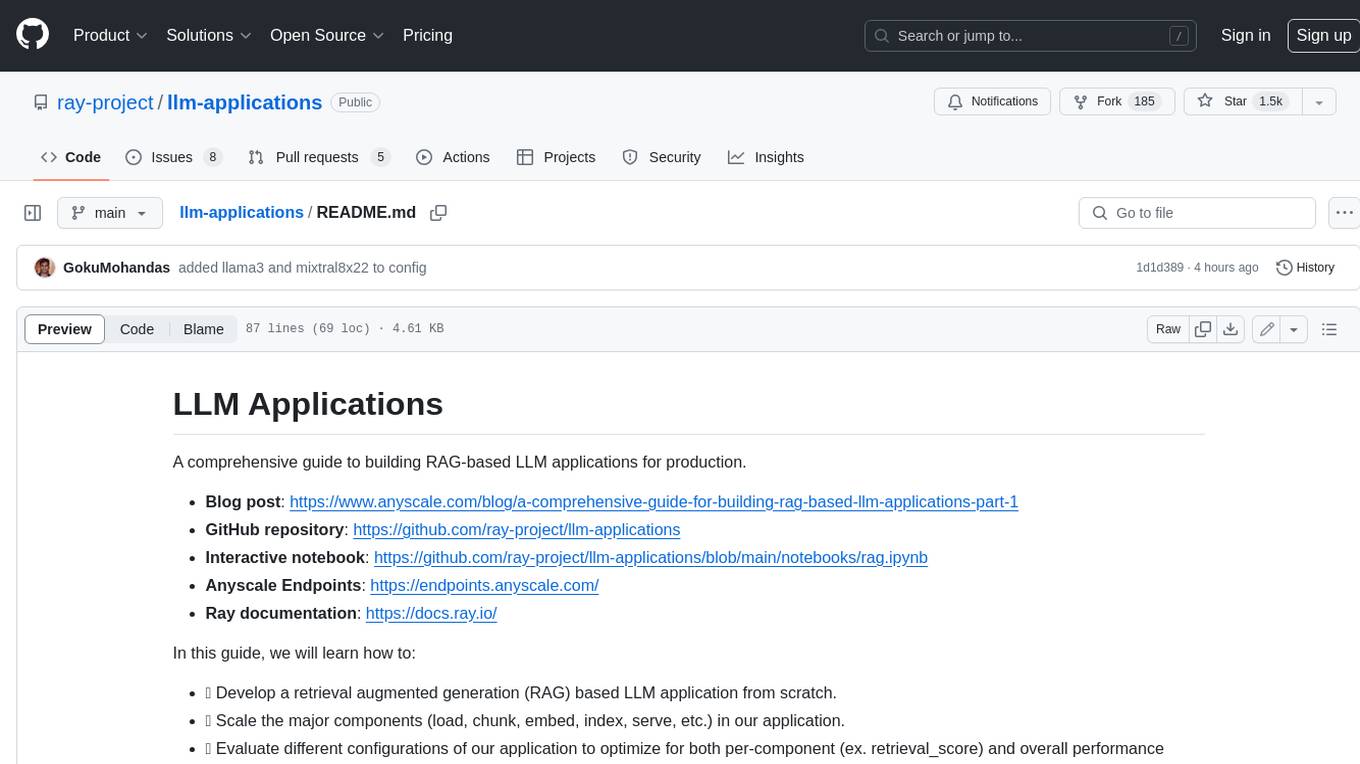
llm-applications
A comprehensive guide to building Retrieval Augmented Generation (RAG)-based LLM applications for production. This guide covers developing a RAG-based LLM application from scratch, scaling the major components, evaluating different configurations, implementing LLM hybrid routing, serving the application in a highly scalable and available manner, and sharing the impacts LLM applications have had on products.
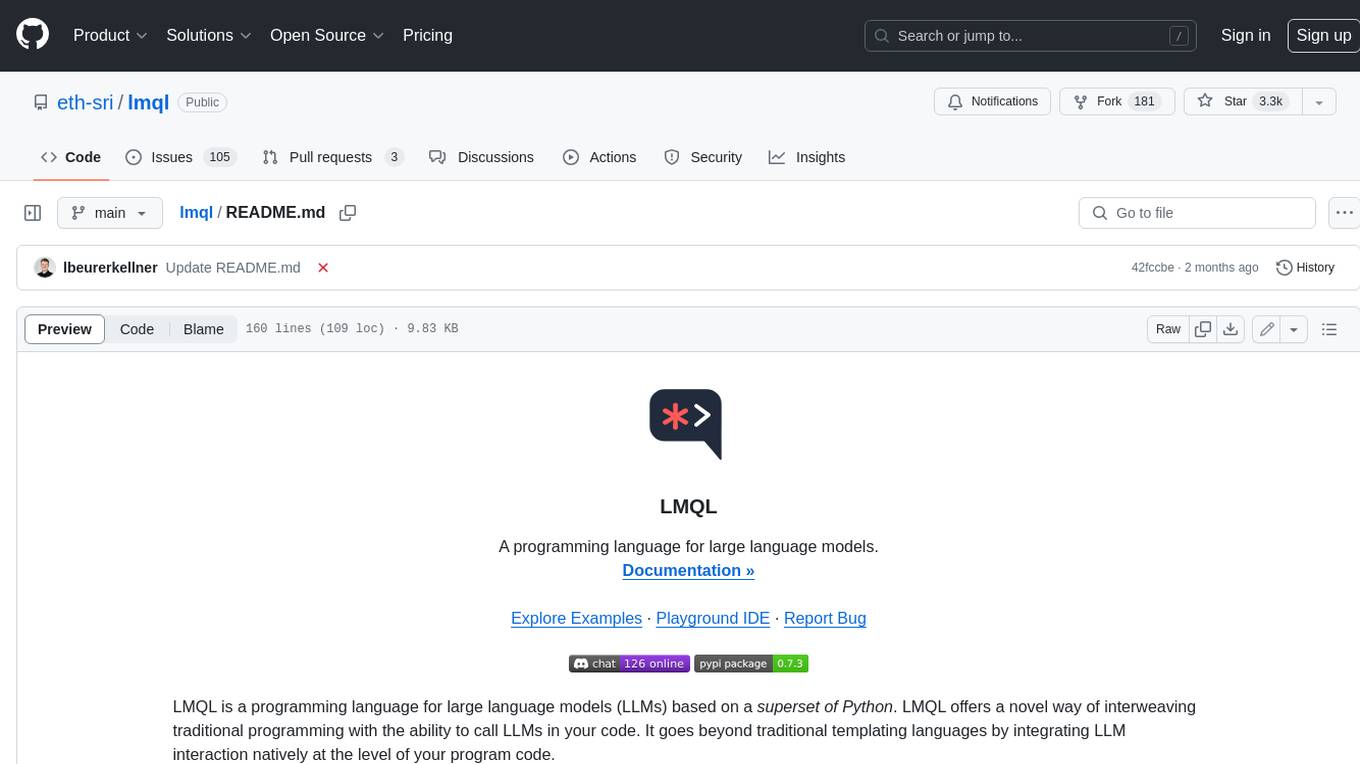
lmql
LMQL is a programming language designed for large language models (LLMs) that offers a unique way of integrating traditional programming with LLM interaction. It allows users to write programs that combine algorithmic logic with LLM calls, enabling model reasoning capabilities within the context of the program. LMQL provides features such as Python syntax integration, rich control-flow options, advanced decoding techniques, powerful constraints via logit masking, runtime optimization, sync and async API support, multi-model compatibility, and extensive applications like JSON decoding and interactive chat interfaces. The tool also offers library integration, flexible tooling, and output streaming options for easy model output handling.
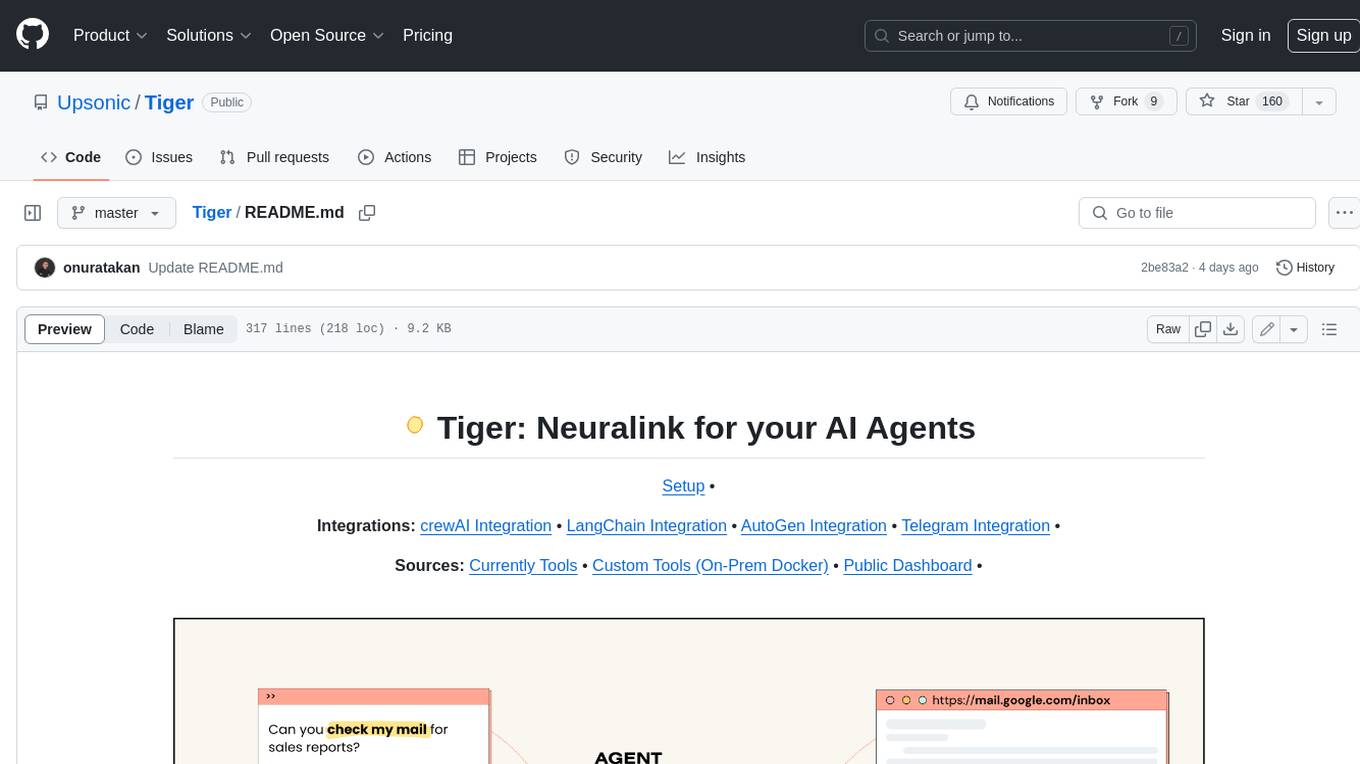
Tiger
Tiger is a community-driven project developing a reusable and integrated tool ecosystem for LLM Agent Revolution. It utilizes Upsonic for isolated tool storage, profiling, and automatic document generation. With Tiger, you can create a customized environment for your agents or leverage the robust and publicly maintained Tiger curated by the community itself.
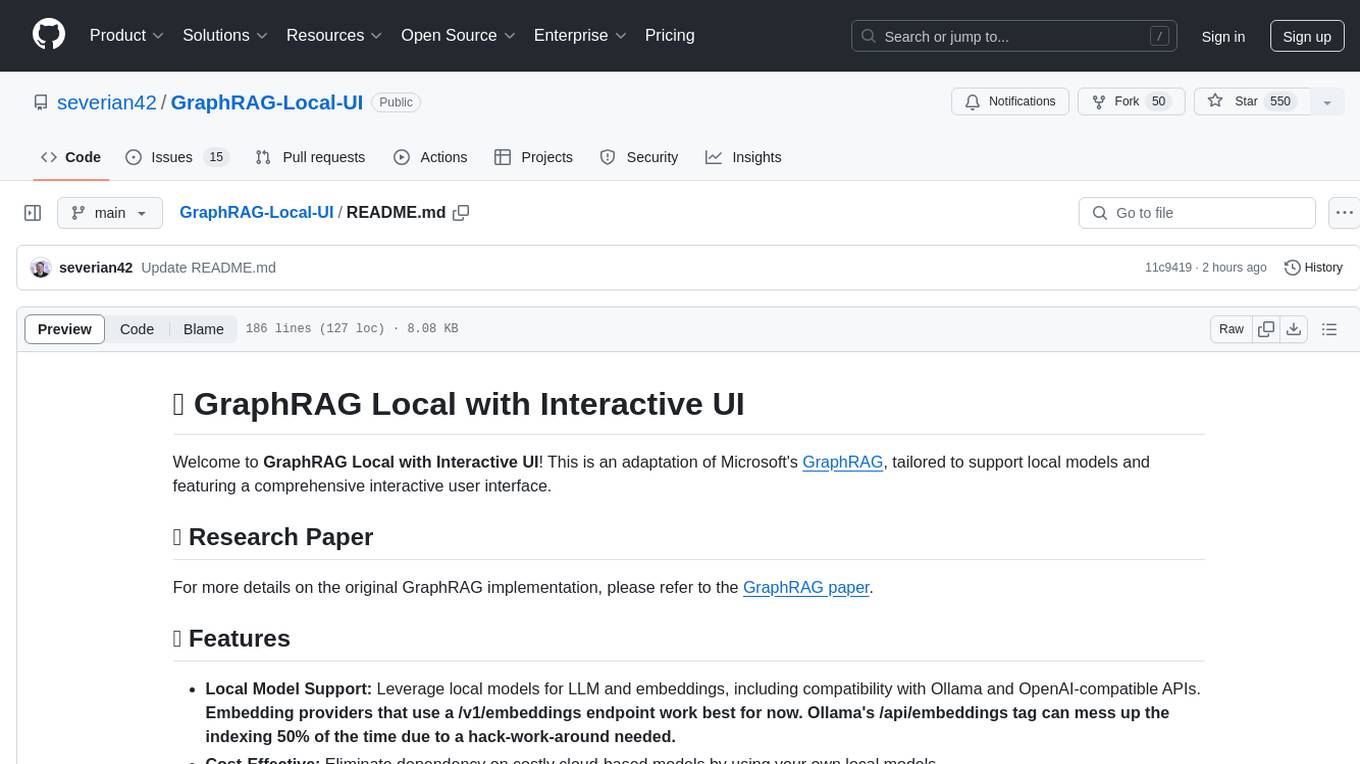
GraphRAG-Local-UI
GraphRAG Local with Interactive UI is an adaptation of Microsoft's GraphRAG, tailored to support local models and featuring a comprehensive interactive user interface. It allows users to leverage local models for LLM and embeddings, visualize knowledge graphs in 2D or 3D, manage files, settings, and queries, and explore indexing outputs. The tool aims to be cost-effective by eliminating dependency on costly cloud-based models and offers flexible querying options for global, local, and direct chat queries.
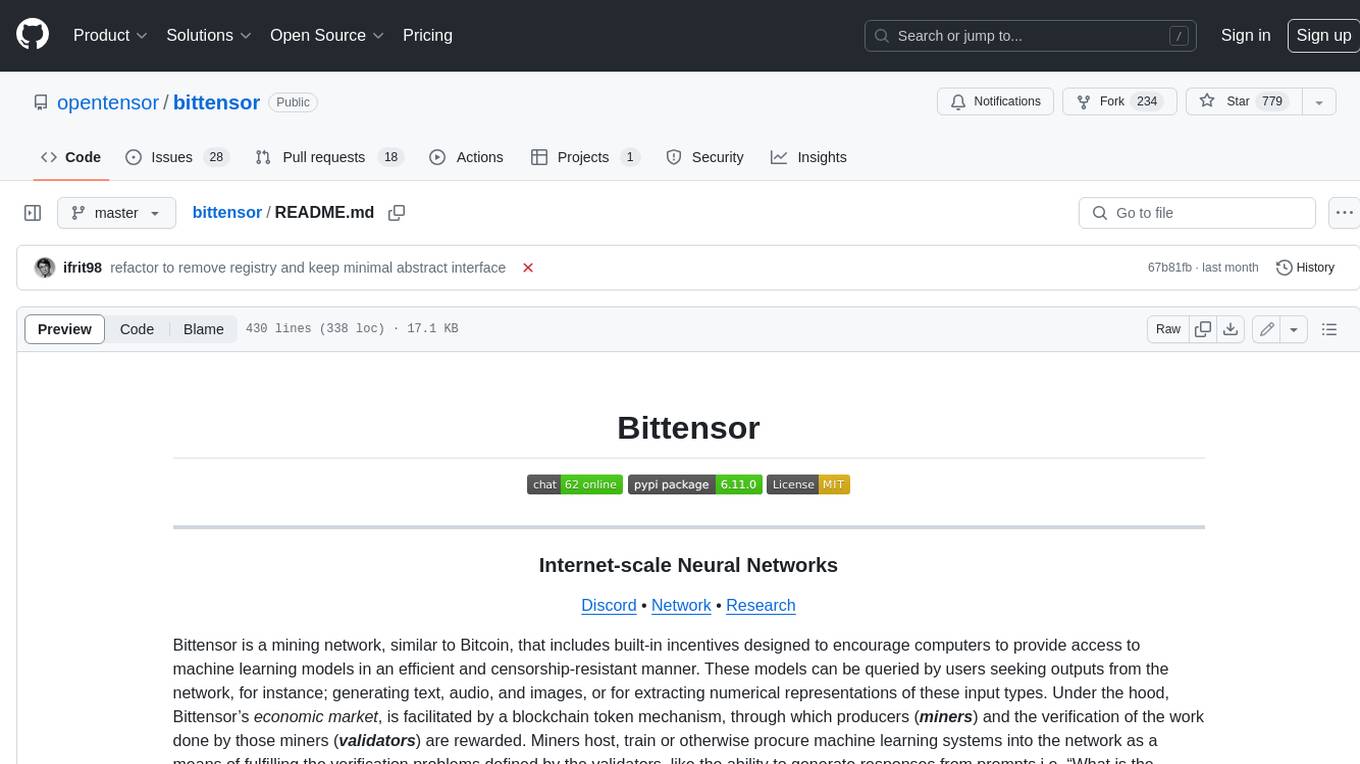
bittensor
Bittensor is an internet-scale neural network that incentivizes computers to provide access to machine learning models in a decentralized and censorship-resistant manner. It operates through a token-based mechanism where miners host, train, and procure machine learning systems to fulfill verification problems defined by validators. The network rewards miners and validators for their contributions, ensuring continuous improvement in knowledge output. Bittensor allows anyone to participate, extract value, and govern the network without centralized control. It supports tasks such as generating text, audio, images, and extracting numerical representations.
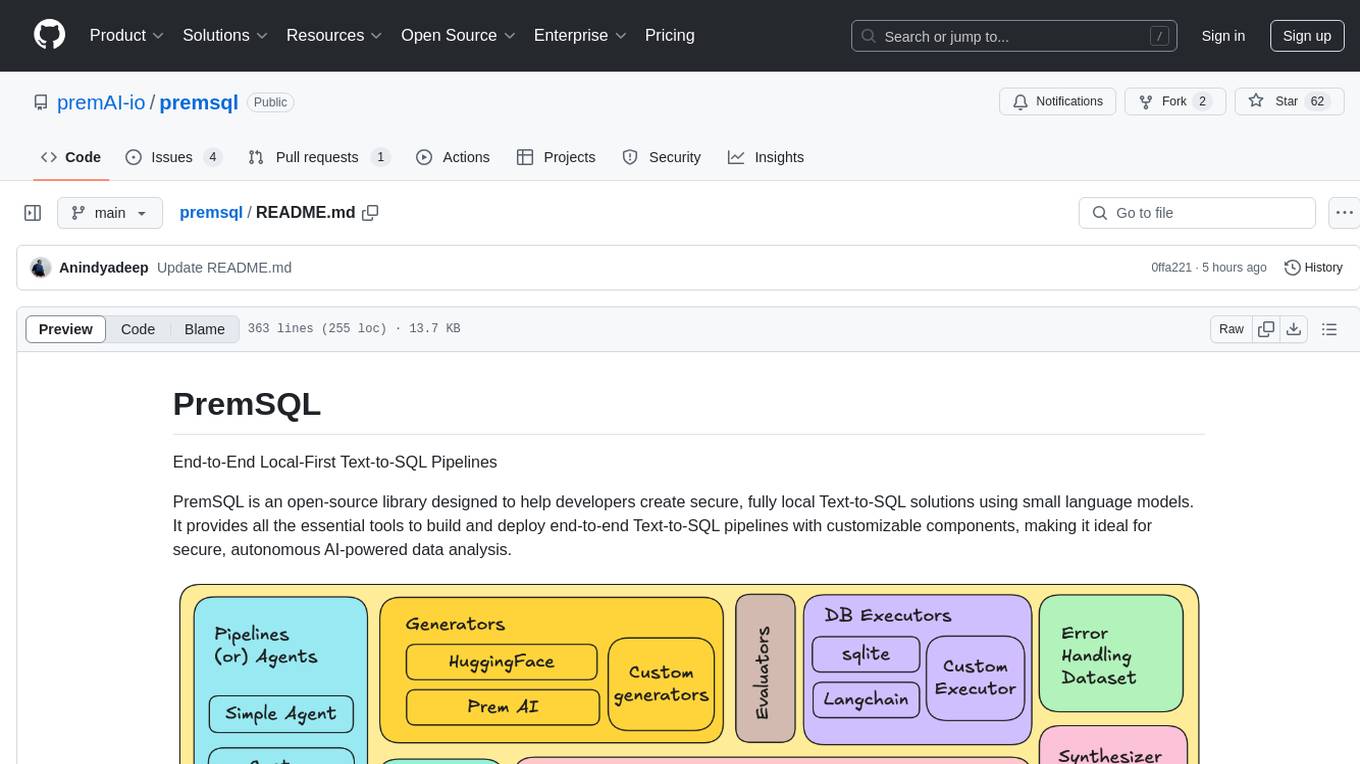
premsql
PremSQL is an open-source library designed to help developers create secure, fully local Text-to-SQL solutions using small language models. It provides essential tools for building and deploying end-to-end Text-to-SQL pipelines with customizable components, ideal for secure, autonomous AI-powered data analysis. The library offers features like Local-First approach, Customizable Datasets, Robust Executors and Evaluators, Advanced Generators, Error Handling and Self-Correction, Fine-Tuning Support, and End-to-End Pipelines. Users can fine-tune models, generate SQL queries from natural language inputs, handle errors, and evaluate model performance against predefined metrics. PremSQL is extendible for customization and private data usage.
For similar tasks
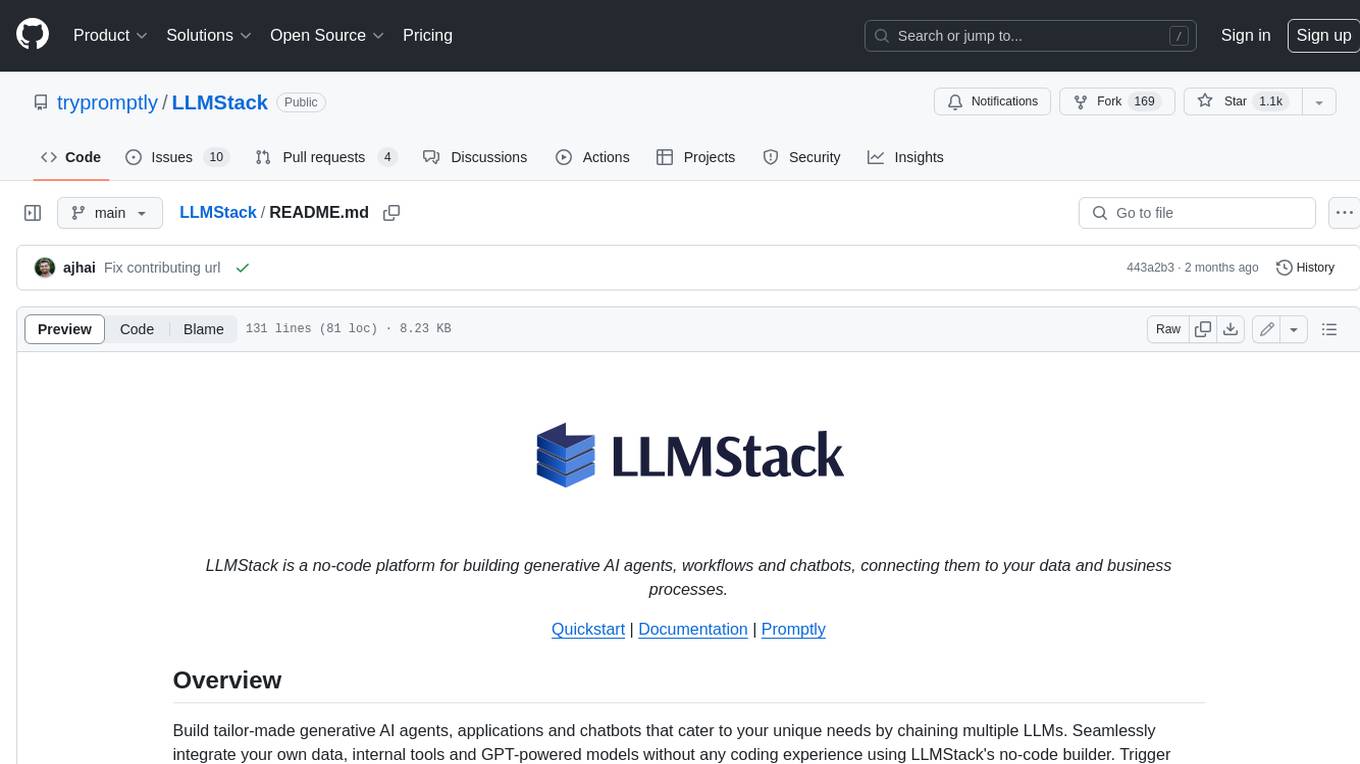
LLMStack
LLMStack is a no-code platform for building generative AI agents, workflows, and chatbots. It allows users to connect their own data, internal tools, and GPT-powered models without any coding experience. LLMStack can be deployed to the cloud or on-premise and can be accessed via HTTP API or triggered from Slack or Discord.
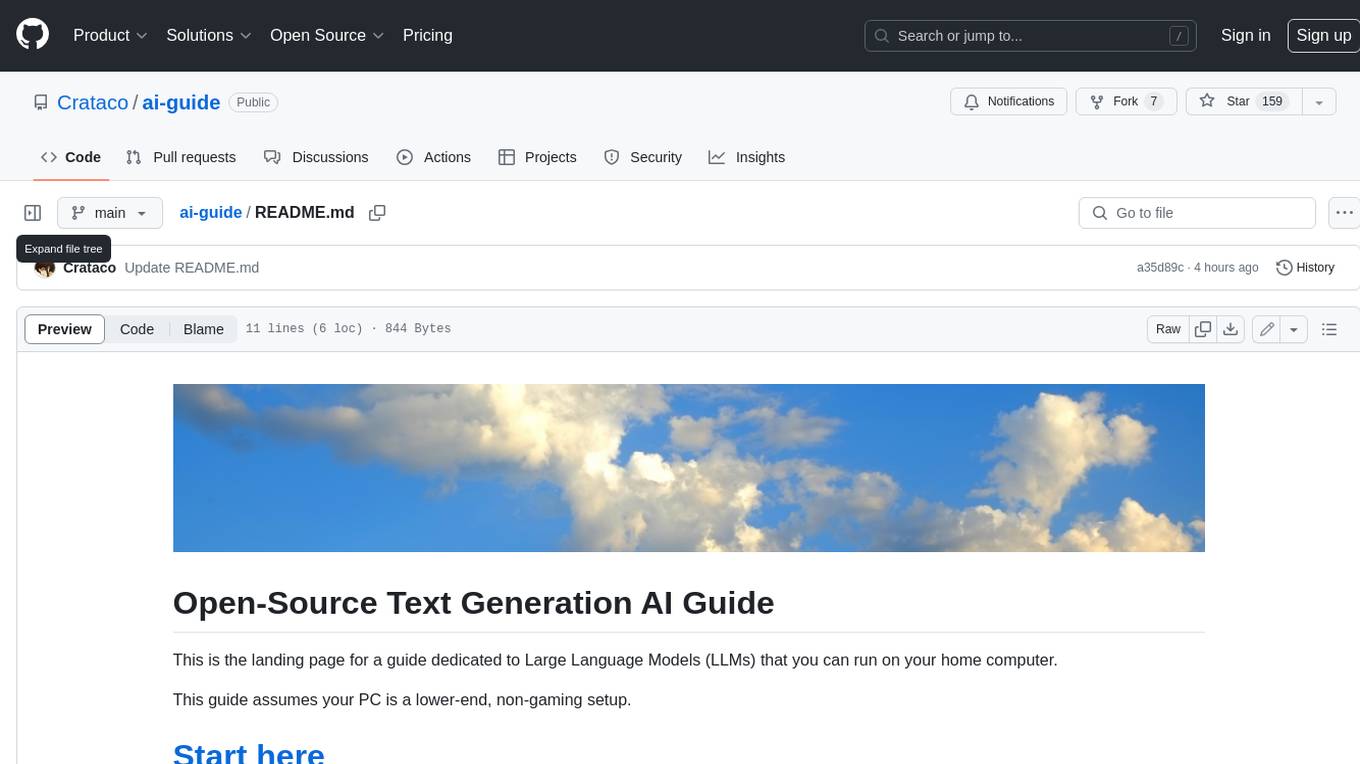
ai-guide
This guide is dedicated to Large Language Models (LLMs) that you can run on your home computer. It assumes your PC is a lower-end, non-gaming setup.
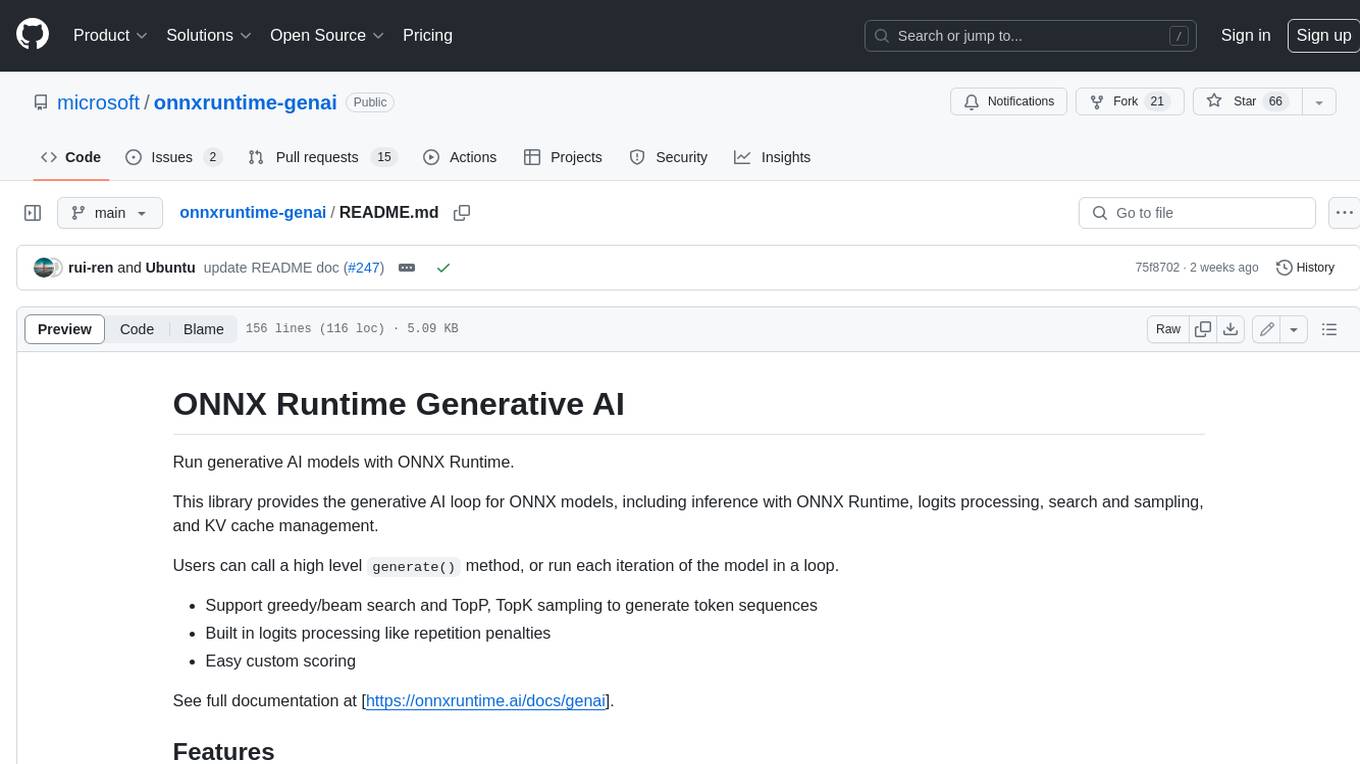
onnxruntime-genai
ONNX Runtime Generative AI is a library that provides the generative AI loop for ONNX models, including inference with ONNX Runtime, logits processing, search and sampling, and KV cache management. Users can call a high level `generate()` method, or run each iteration of the model in a loop. It supports greedy/beam search and TopP, TopK sampling to generate token sequences, has built in logits processing like repetition penalties, and allows for easy custom scoring.
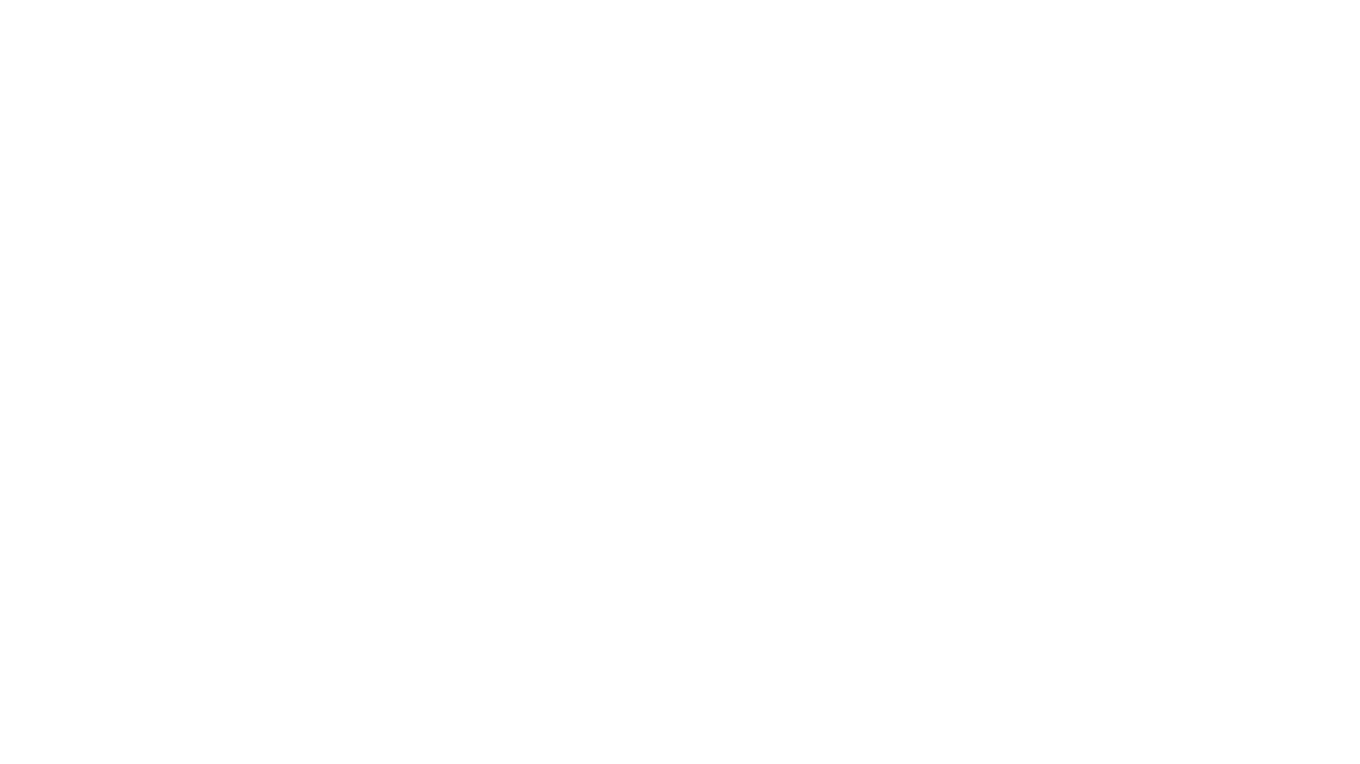
jupyter-ai
Jupyter AI connects generative AI with Jupyter notebooks. It provides a user-friendly and powerful way to explore generative AI models in notebooks and improve your productivity in JupyterLab and the Jupyter Notebook. Specifically, Jupyter AI offers: * An `%%ai` magic that turns the Jupyter notebook into a reproducible generative AI playground. This works anywhere the IPython kernel runs (JupyterLab, Jupyter Notebook, Google Colab, Kaggle, VSCode, etc.). * A native chat UI in JupyterLab that enables you to work with generative AI as a conversational assistant. * Support for a wide range of generative model providers, including AI21, Anthropic, AWS, Cohere, Gemini, Hugging Face, NVIDIA, and OpenAI. * Local model support through GPT4All, enabling use of generative AI models on consumer grade machines with ease and privacy.
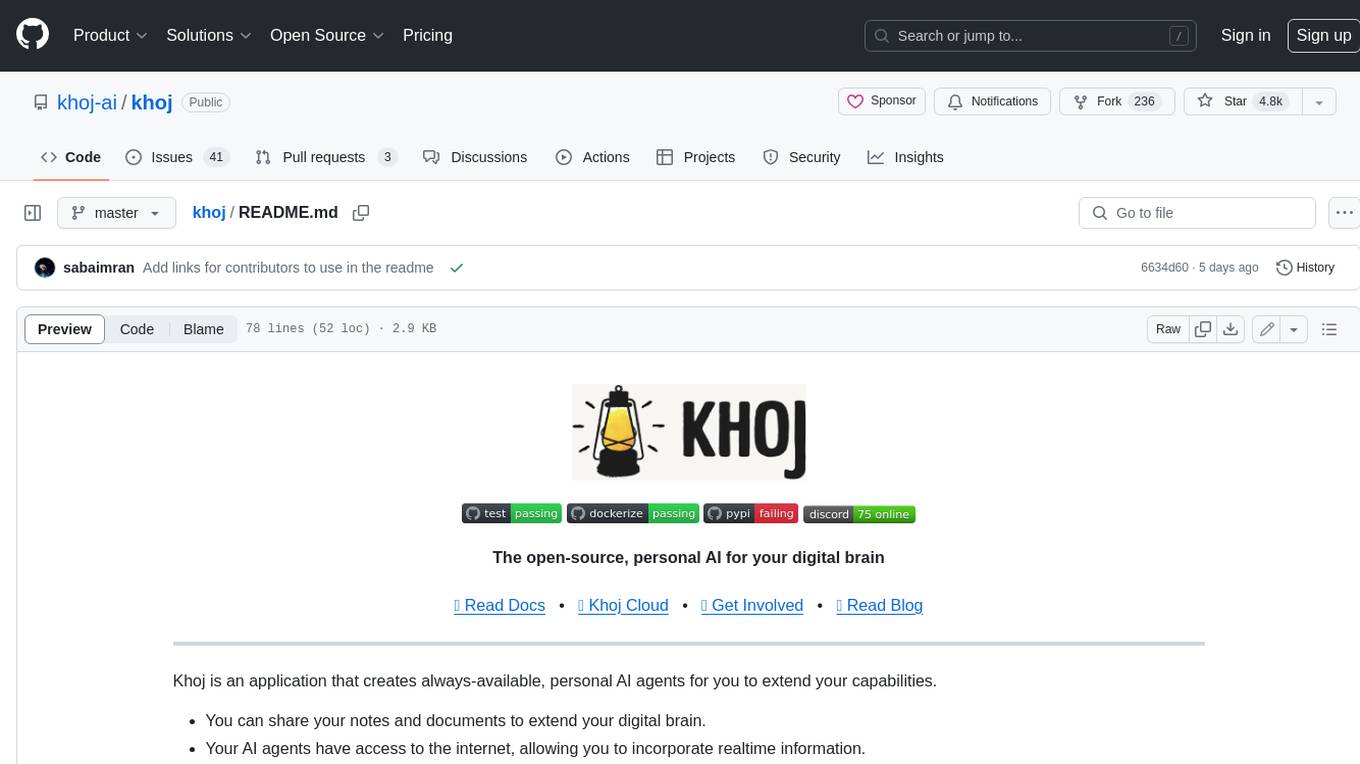
khoj
Khoj is an open-source, personal AI assistant that extends your capabilities by creating always-available AI agents. You can share your notes and documents to extend your digital brain, and your AI agents have access to the internet, allowing you to incorporate real-time information. Khoj is accessible on Desktop, Emacs, Obsidian, Web, and Whatsapp, and you can share PDF, markdown, org-mode, notion files, and GitHub repositories. You'll get fast, accurate semantic search on top of your docs, and your agents can create deeply personal images and understand your speech. Khoj is self-hostable and always will be.
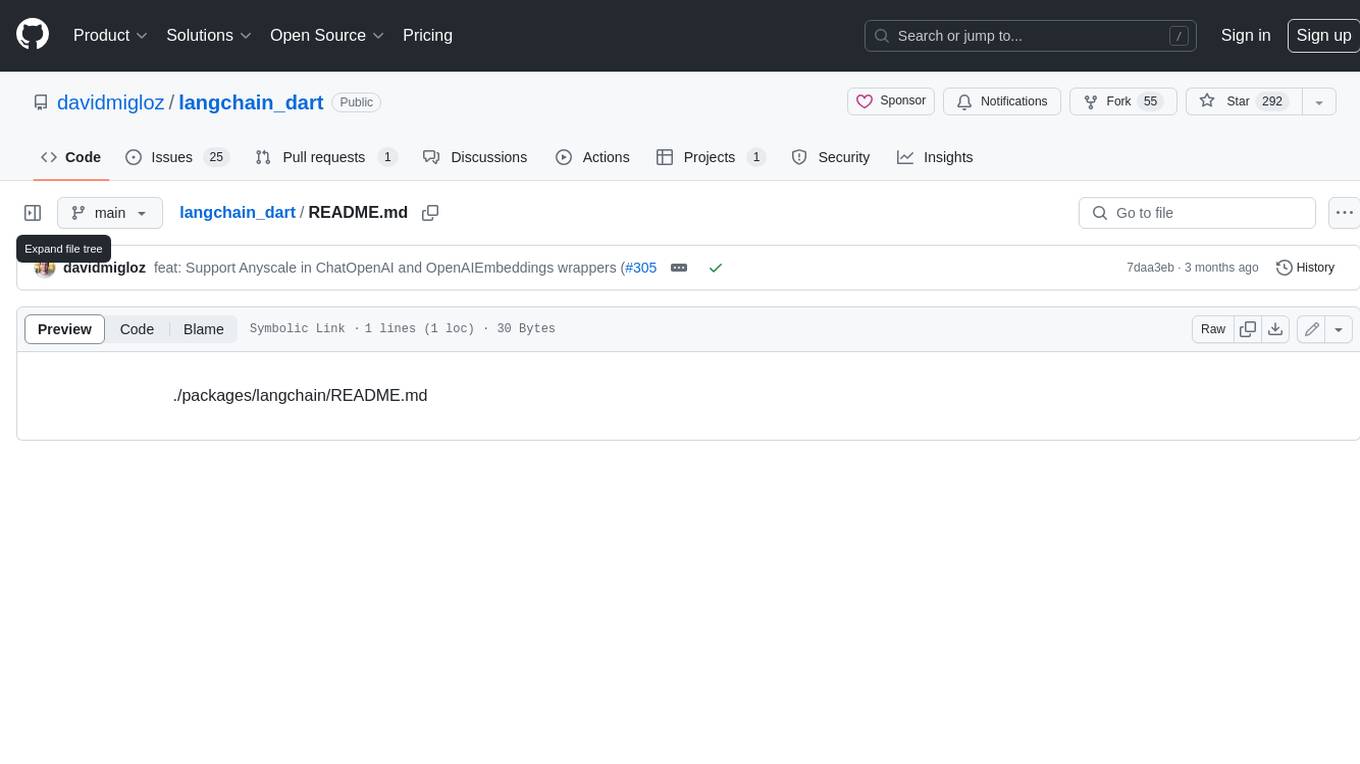
langchain_dart
LangChain.dart is a Dart port of the popular LangChain Python framework created by Harrison Chase. LangChain provides a set of ready-to-use components for working with language models and a standard interface for chaining them together to formulate more advanced use cases (e.g. chatbots, Q&A with RAG, agents, summarization, extraction, etc.). The components can be grouped into a few core modules: * **Model I/O:** LangChain offers a unified API for interacting with various LLM providers (e.g. OpenAI, Google, Mistral, Ollama, etc.), allowing developers to switch between them with ease. Additionally, it provides tools for managing model inputs (prompt templates and example selectors) and parsing the resulting model outputs (output parsers). * **Retrieval:** assists in loading user data (via document loaders), transforming it (with text splitters), extracting its meaning (using embedding models), storing (in vector stores) and retrieving it (through retrievers) so that it can be used to ground the model's responses (i.e. Retrieval-Augmented Generation or RAG). * **Agents:** "bots" that leverage LLMs to make informed decisions about which available tools (such as web search, calculators, database lookup, etc.) to use to accomplish the designated task. The different components can be composed together using the LangChain Expression Language (LCEL).
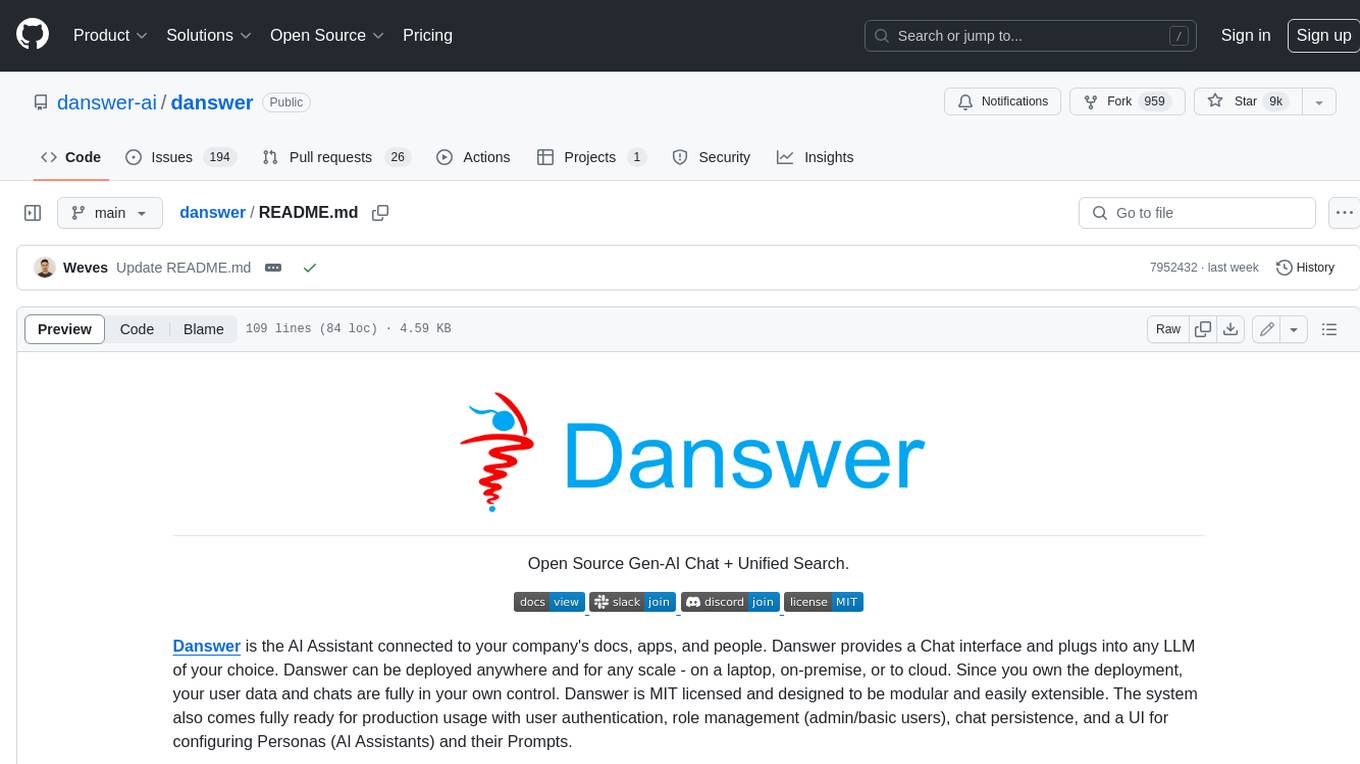
danswer
Danswer is an open-source Gen-AI Chat and Unified Search tool that connects to your company's docs, apps, and people. It provides a Chat interface and plugs into any LLM of your choice. Danswer can be deployed anywhere and for any scale - on a laptop, on-premise, or to cloud. Since you own the deployment, your user data and chats are fully in your own control. Danswer is MIT licensed and designed to be modular and easily extensible. The system also comes fully ready for production usage with user authentication, role management (admin/basic users), chat persistence, and a UI for configuring Personas (AI Assistants) and their Prompts. Danswer also serves as a Unified Search across all common workplace tools such as Slack, Google Drive, Confluence, etc. By combining LLMs and team specific knowledge, Danswer becomes a subject matter expert for the team. Imagine ChatGPT if it had access to your team's unique knowledge! It enables questions such as "A customer wants feature X, is this already supported?" or "Where's the pull request for feature Y?"
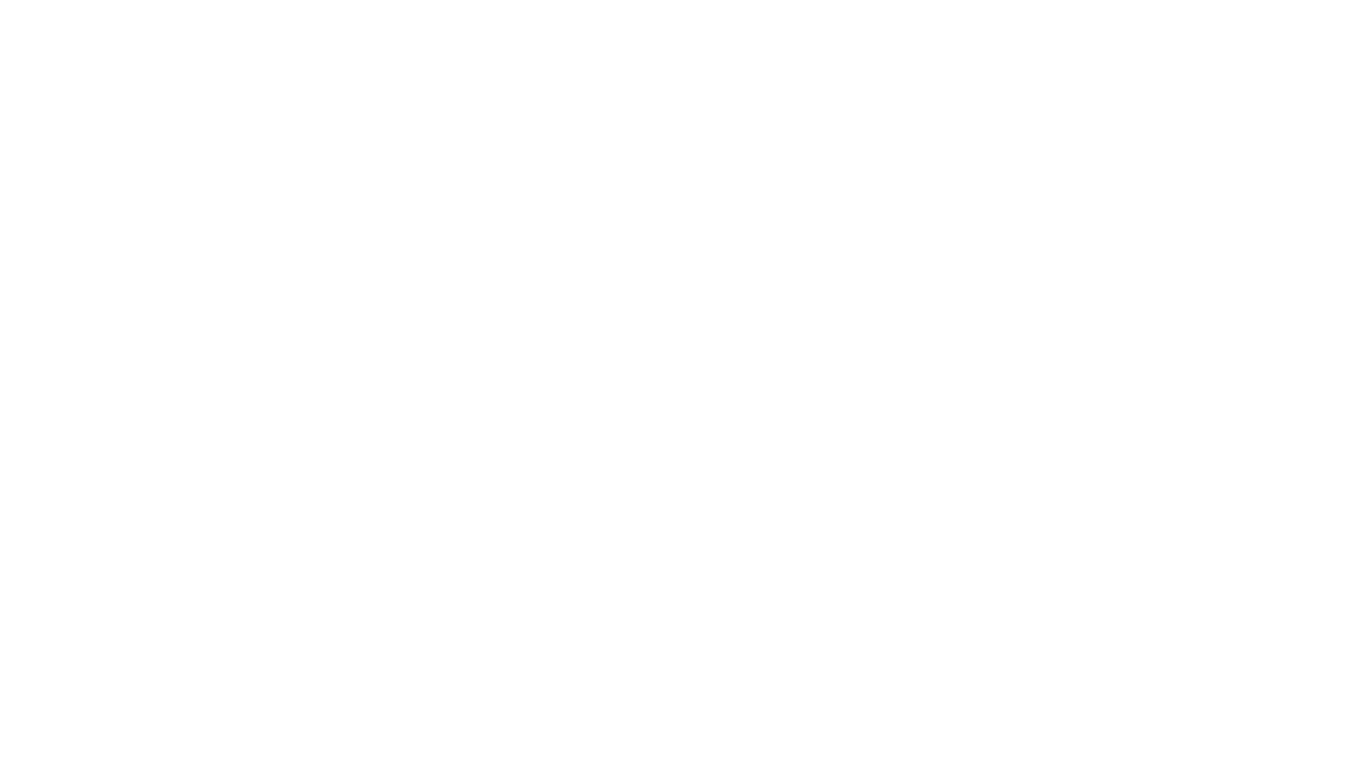
infinity
Infinity is an AI-native database designed for LLM applications, providing incredibly fast full-text and vector search capabilities. It supports a wide range of data types, including vectors, full-text, and structured data, and offers a fused search feature that combines multiple embeddings and full text. Infinity is easy to use, with an intuitive Python API and a single-binary architecture that simplifies deployment. It achieves high performance, with 0.1 milliseconds query latency on million-scale vector datasets and up to 15K QPS.
For similar jobs
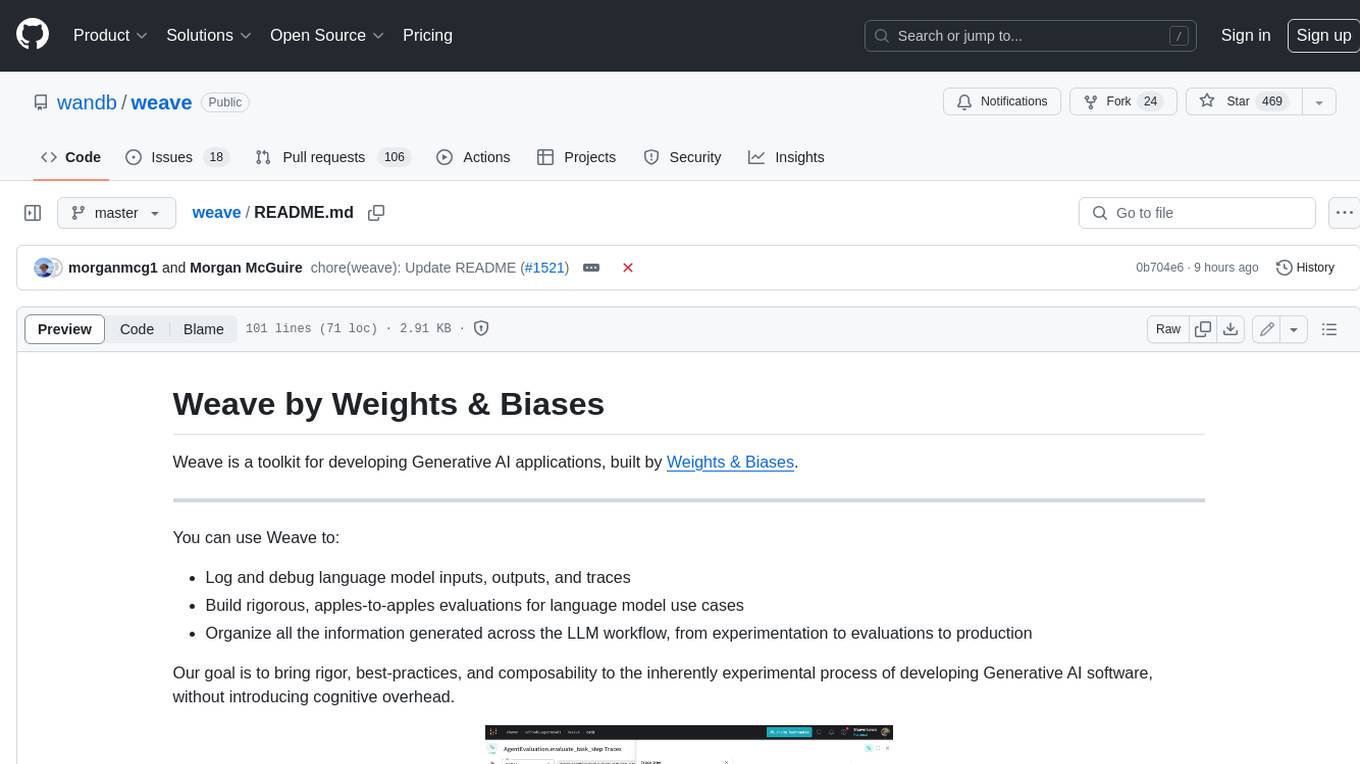
weave
Weave is a toolkit for developing Generative AI applications, built by Weights & Biases. With Weave, you can log and debug language model inputs, outputs, and traces; build rigorous, apples-to-apples evaluations for language model use cases; and organize all the information generated across the LLM workflow, from experimentation to evaluations to production. Weave aims to bring rigor, best-practices, and composability to the inherently experimental process of developing Generative AI software, without introducing cognitive overhead.
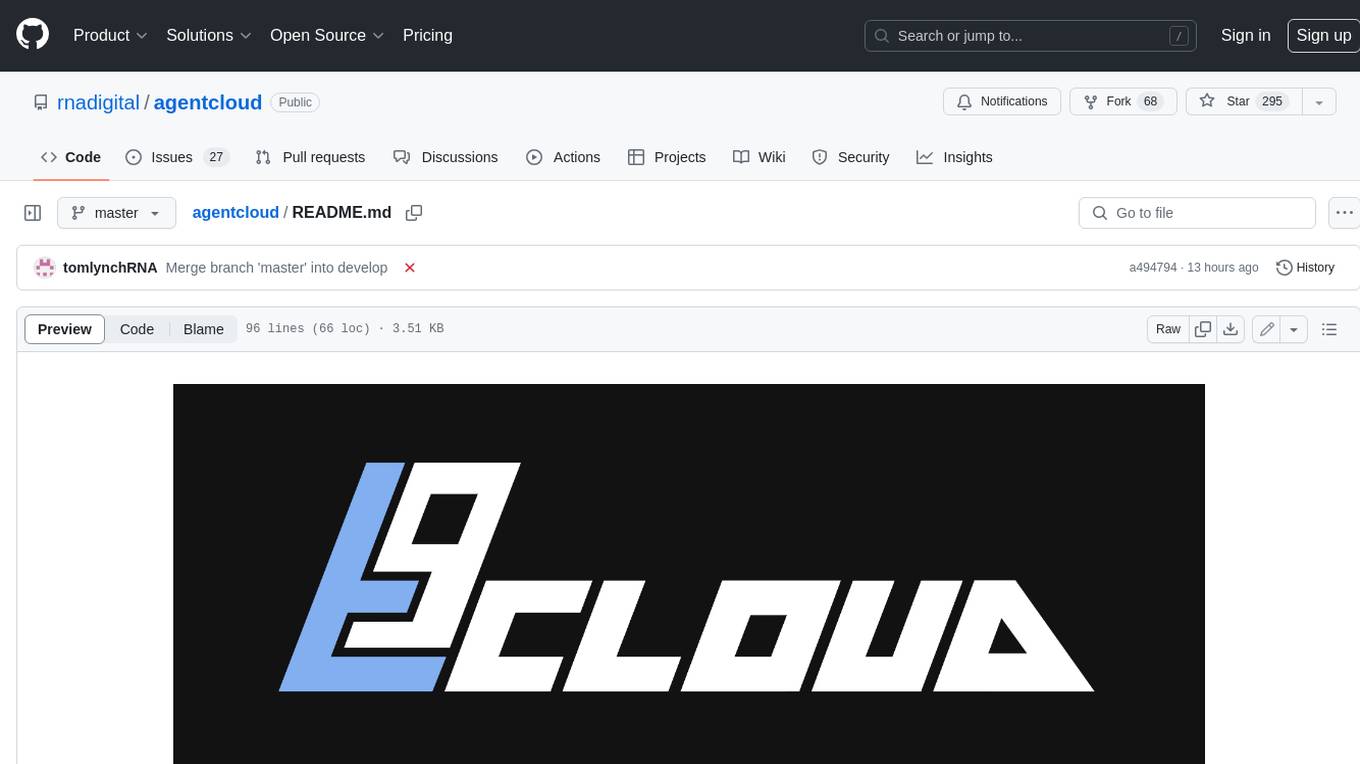
agentcloud
AgentCloud is an open-source platform that enables companies to build and deploy private LLM chat apps, empowering teams to securely interact with their data. It comprises three main components: Agent Backend, Webapp, and Vector Proxy. To run this project locally, clone the repository, install Docker, and start the services. The project is licensed under the GNU Affero General Public License, version 3 only. Contributions and feedback are welcome from the community.
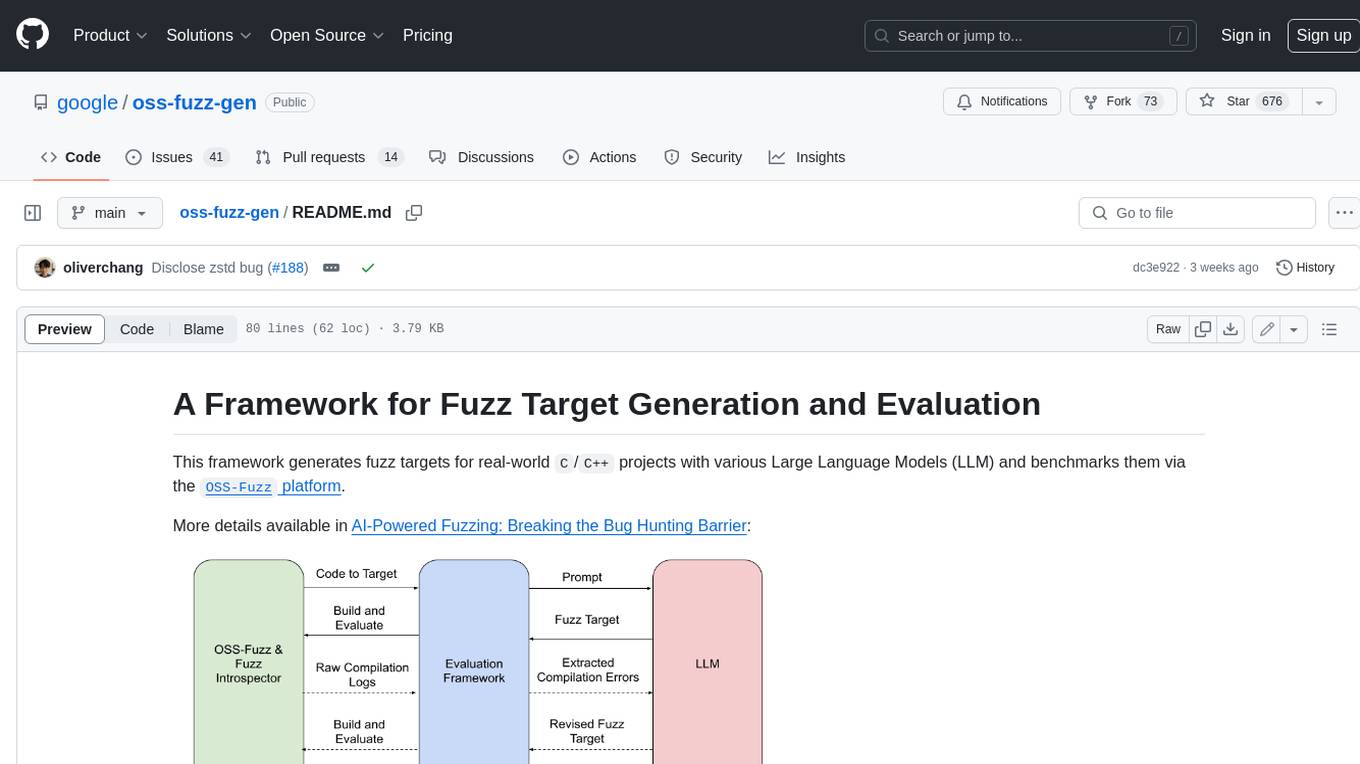
oss-fuzz-gen
This framework generates fuzz targets for real-world `C`/`C++` projects with various Large Language Models (LLM) and benchmarks them via the `OSS-Fuzz` platform. It manages to successfully leverage LLMs to generate valid fuzz targets (which generate non-zero coverage increase) for 160 C/C++ projects. The maximum line coverage increase is 29% from the existing human-written targets.
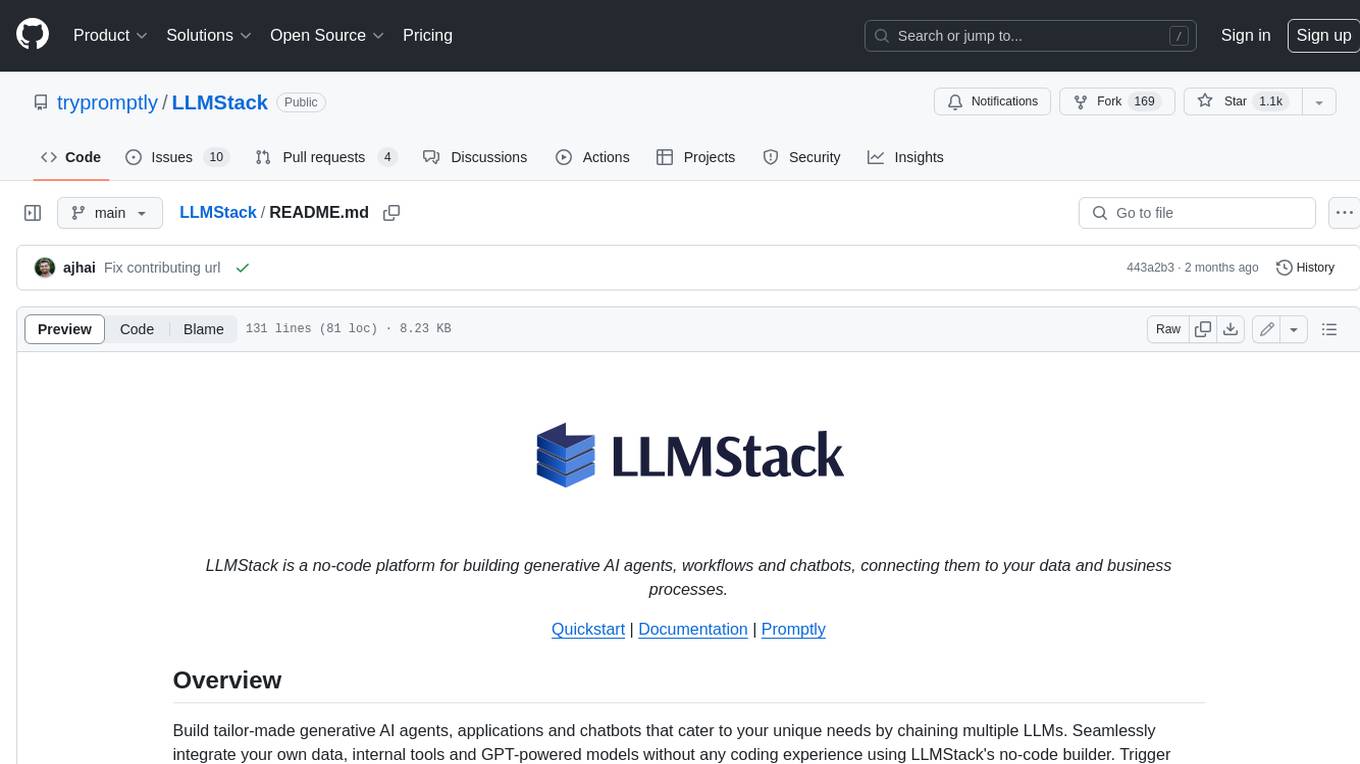
LLMStack
LLMStack is a no-code platform for building generative AI agents, workflows, and chatbots. It allows users to connect their own data, internal tools, and GPT-powered models without any coding experience. LLMStack can be deployed to the cloud or on-premise and can be accessed via HTTP API or triggered from Slack or Discord.
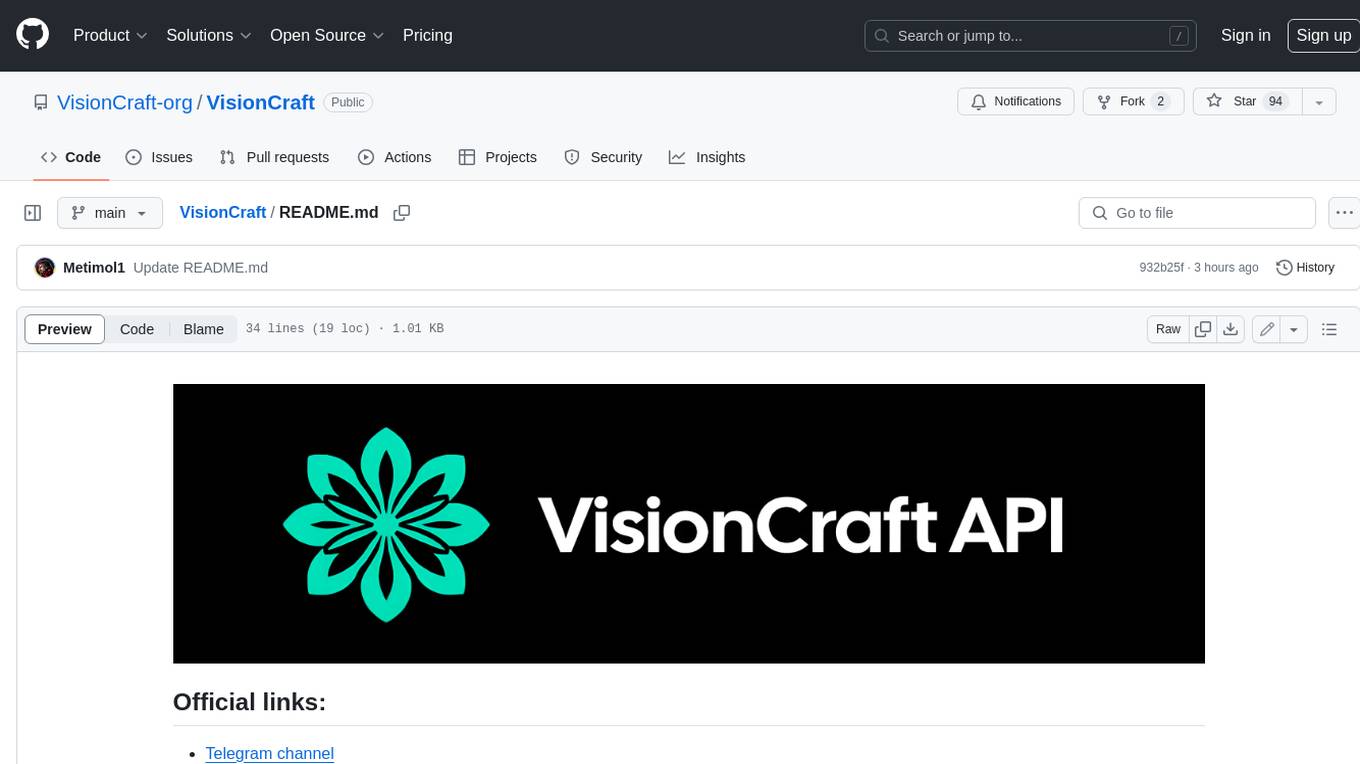
VisionCraft
The VisionCraft API is a free API for using over 100 different AI models. From images to sound.
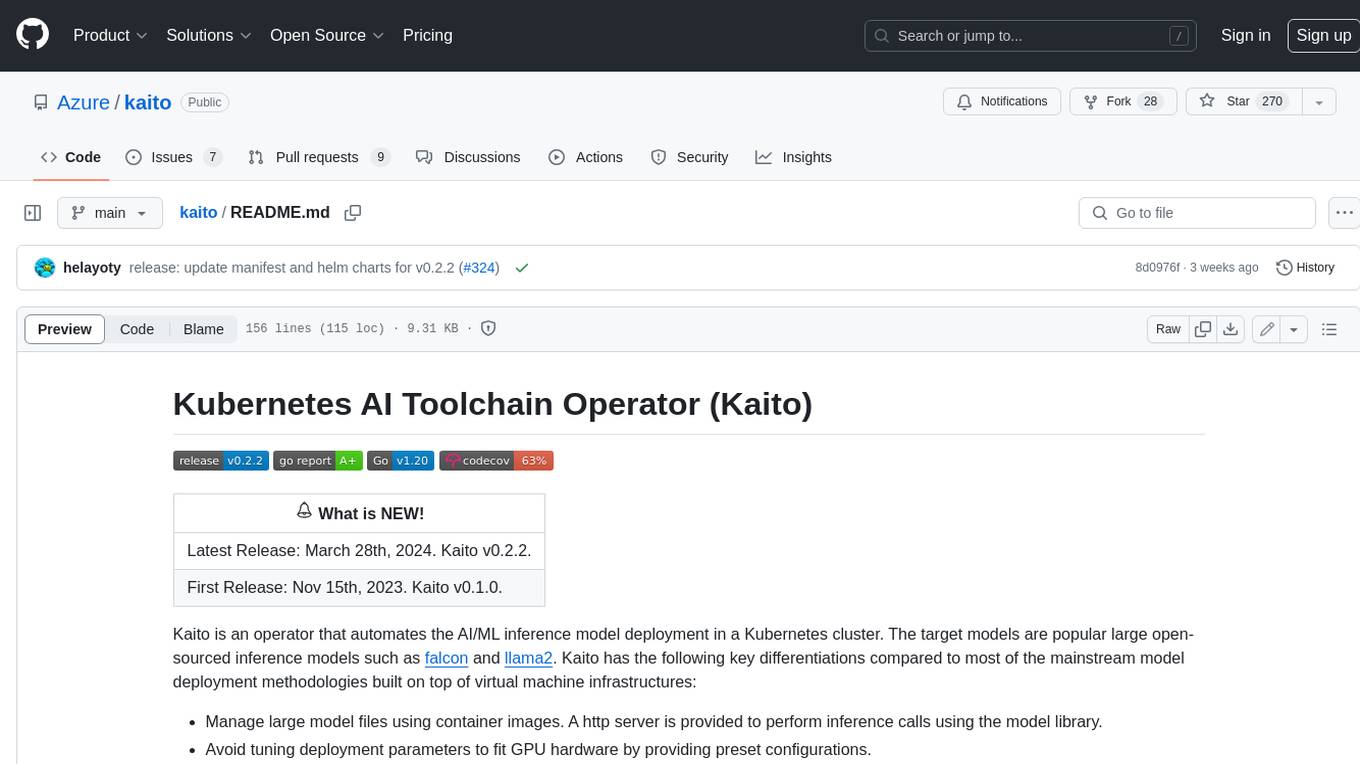
kaito
Kaito is an operator that automates the AI/ML inference model deployment in a Kubernetes cluster. It manages large model files using container images, avoids tuning deployment parameters to fit GPU hardware by providing preset configurations, auto-provisions GPU nodes based on model requirements, and hosts large model images in the public Microsoft Container Registry (MCR) if the license allows. Using Kaito, the workflow of onboarding large AI inference models in Kubernetes is largely simplified.
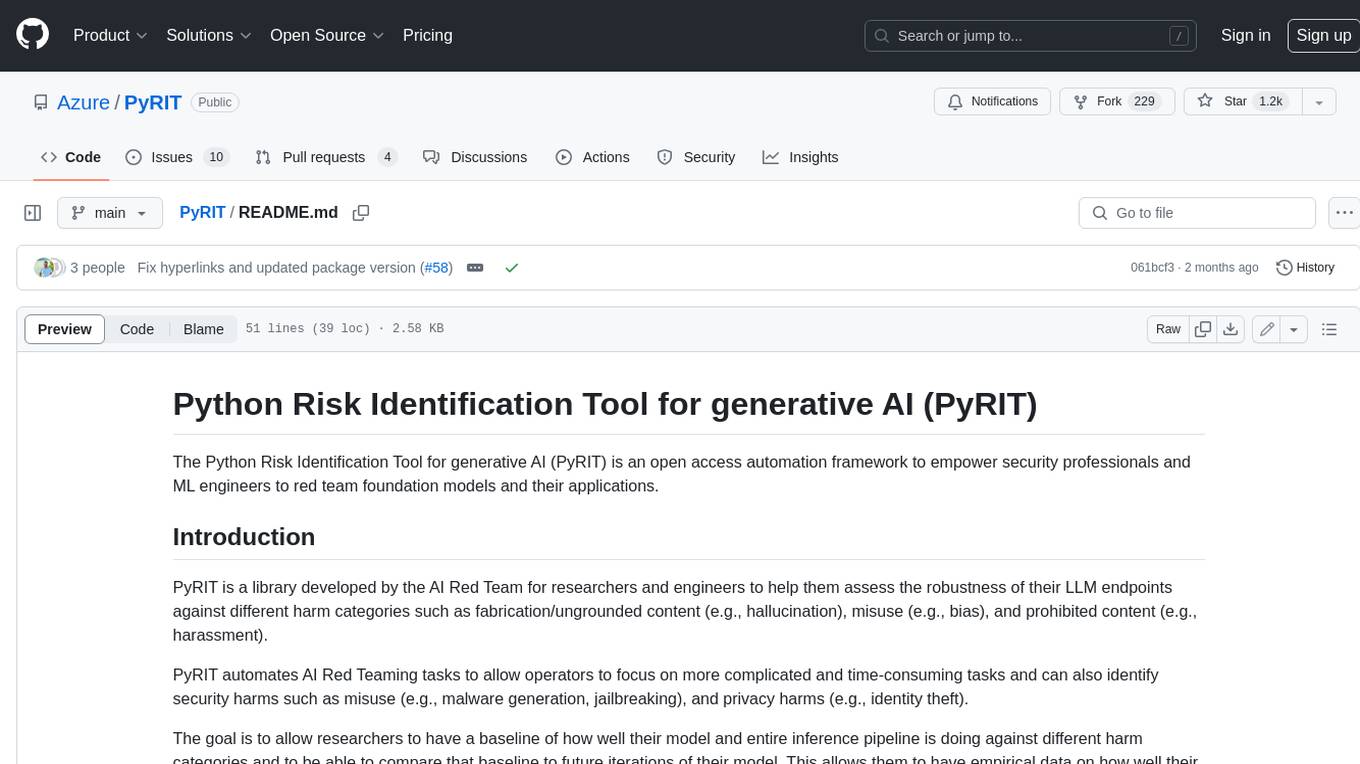
PyRIT
PyRIT is an open access automation framework designed to empower security professionals and ML engineers to red team foundation models and their applications. It automates AI Red Teaming tasks to allow operators to focus on more complicated and time-consuming tasks and can also identify security harms such as misuse (e.g., malware generation, jailbreaking), and privacy harms (e.g., identity theft). The goal is to allow researchers to have a baseline of how well their model and entire inference pipeline is doing against different harm categories and to be able to compare that baseline to future iterations of their model. This allows them to have empirical data on how well their model is doing today, and detect any degradation of performance based on future improvements.
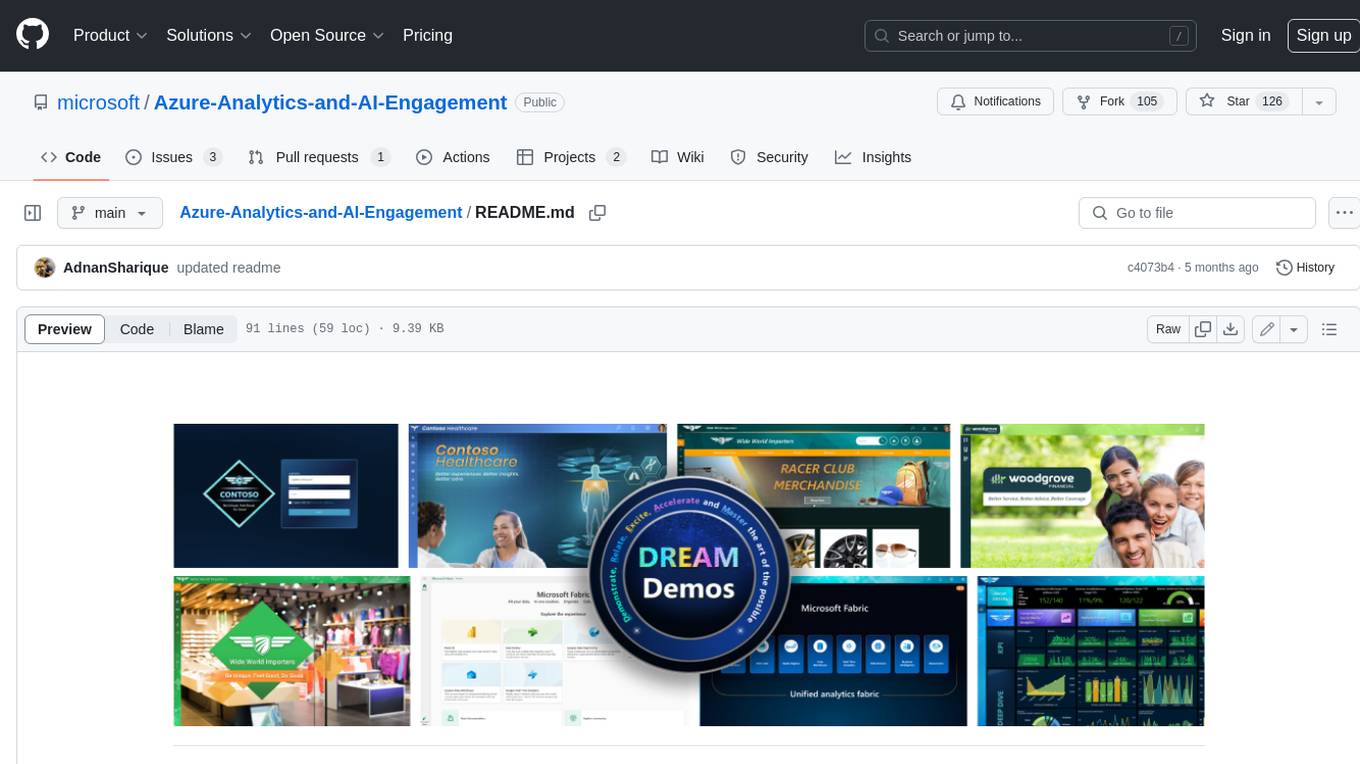
Azure-Analytics-and-AI-Engagement
The Azure-Analytics-and-AI-Engagement repository provides packaged Industry Scenario DREAM Demos with ARM templates (Containing a demo web application, Power BI reports, Synapse resources, AML Notebooks etc.) that can be deployed in a customerβs subscription using the CAPE tool within a matter of few hours. Partners can also deploy DREAM Demos in their own subscriptions using DPoC.