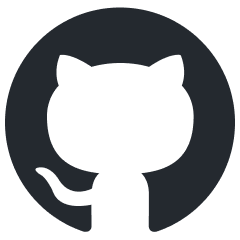
BentoML
The easiest way to serve AI apps and models - Build Model Inference APIs, Job queues, LLM apps, Multi-model pipelines, and more!
Stars: 7262
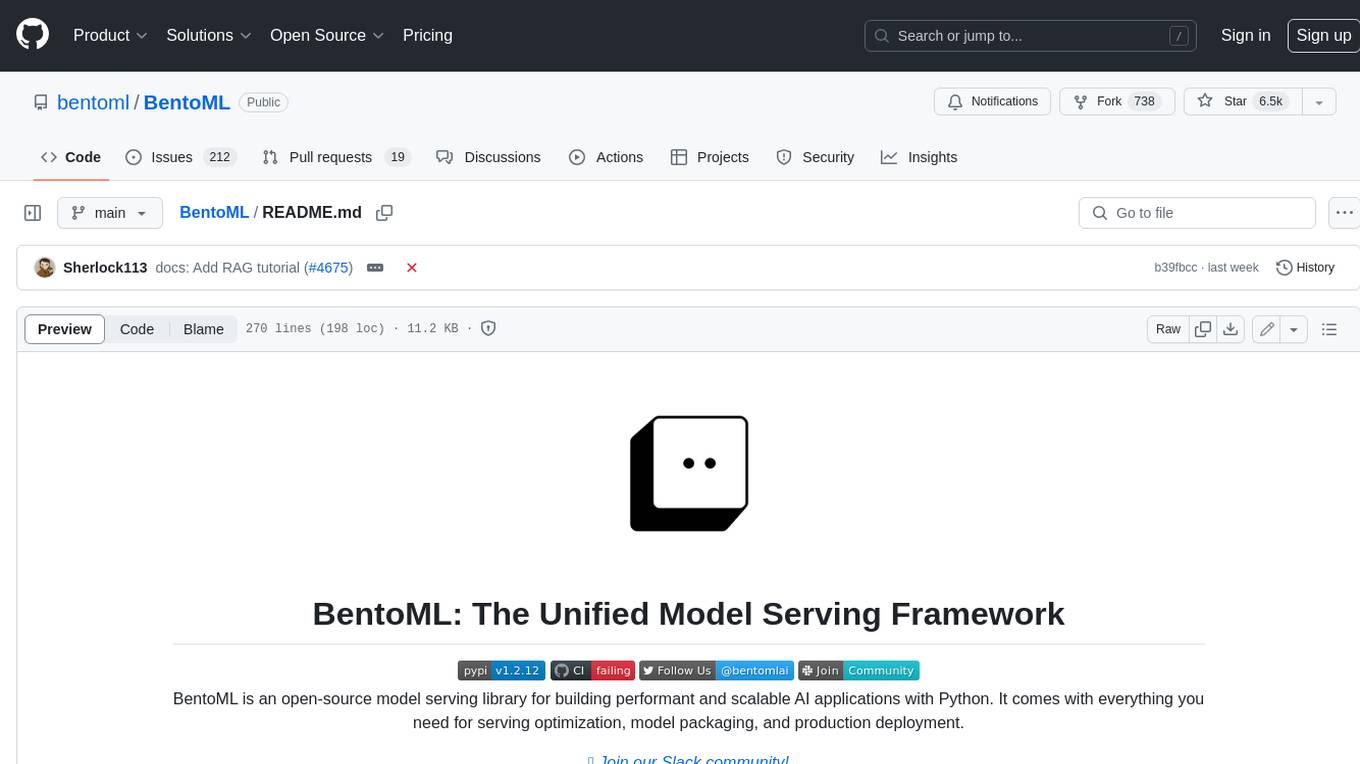
BentoML is an open-source model serving library for building performant and scalable AI applications with Python. It comes with everything you need for serving optimization, model packaging, and production deployment.
README:
🍱 Build model inference APIs and multi-model serving systems with any open-source or custom AI models. 👉 Join our Slack community!
BentoML is a Python library for building online serving systems optimized for AI apps and model inference.
- 🍱 Easily build APIs for Any AI/ML Model. Turn any model inference script into a REST API server with just a few lines of code and standard Python type hints.
- 🐳 Docker Containers made simple. No more dependency hell! Manage your environments, dependencies and model versions with a simple config file. BentoML automatically generates Docker images, ensures reproducibility, and simplifies how you deploy to different environments.
- 🧭 Maximize CPU/GPU utilization. Build high performance inference APIs leveraging built-in serving optimization features like dynamic batching, model parallelism, multi-stage pipeline and multi-model inference-graph orchestration.
- 👩💻 Fully customizable. Easily implement your own APIs or task queues, with custom business logic, model inference and multi-model composition. Supports any ML framework, modality, and inference runtime.
- 🚀 Ready for Production. Develop, run and debug locally. Seamlessly deploy to production with Docker containers or BentoCloud.
Install BentoML:
# Requires Python≥3.9
pip install -U bentoml
Define APIs in a service.py
file.
from __future__ import annotations
import bentoml
@bentoml.service(
resources={"cpu": "4"}
)
class Summarization:
def __init__(self) -> None:
import torch
from transformers import pipeline
device = "cuda" if torch.cuda.is_available() else "cpu"
self.pipeline = pipeline('summarization', device=device)
@bentoml.api(batchable=True)
def summarize(self, texts: list[str]) -> list[str]:
results = self.pipeline(texts)
return [item['summary_text'] for item in results]
Run the service code locally (serving at http://localhost:3000 by default):
pip install torch transformers # additional dependencies for local run
bentoml serve service.py:Summarization
Now you can run inference from your browser at http://localhost:3000 or with a Python script:
import bentoml
with bentoml.SyncHTTPClient('http://localhost:3000') as client:
summarized_text: str = client.summarize([bentoml.__doc__])[0]
print(f"Result: {summarized_text}")
To deploy your BentoML Service code, first create a bentofile.yaml
file to define its dependencies and environments. Find the full list of bentofile options here.
service: 'service:Summarization' # Entry service import path
include:
- '*.py' # Include all .py files in current directory
python:
packages: # Python dependencies to include
- torch
- transformers
docker:
python_version: "3.11"
Then, choose one of the following ways for deployment:
🐳 Docker Container
Run bentoml build
to package necessary code, models, dependency configs into a Bento - the standardized deployable artifact in BentoML:
bentoml build
Ensure Docker is running. Generate a Docker container image for deployment:
bentoml containerize summarization:latest
Run the generated image:
docker run --rm -p 3000:3000 summarization:latest
☁️ BentoCloud
BentoCloud provides compute infrastructure for rapid and reliable GenAI adoption. It helps speed up your BentoML development process leveraging cloud compute resources, and simplify how you deploy, scale and operate BentoML in production.
Sign up for BentoCloud for personal access; for enterprise use cases, contact our team.
# After signup, run the following command to create an API token:
bentoml cloud login
# Deploy from current directory:
bentoml deploy .
For detailed explanations, read the Hello World example.
- LLMs: Llama 3.2, Mixtral, Solar, and Mistral.
- Image Generation: Stable Diffusion 3 Medium, Stable Video Diffusion, Stable Diffusion XL Turbo, ControlNet, and LCM LoRAs.
- Embeddings: SentenceTransformers and ColPali
- Audio: ChatTTS, XTTS, WhisperX, Bark
- Computer Vision: YOLO and ResNet
- Advanced examples: Function calling, LangGraph, CrewAI
Check out the full list for more sample code and usage.
- Model composition
- Workers and model parallelization
- Adaptive batching
- GPU inference
- Distributed serving systems
- Concurrency and autoscaling
- Model loading and Model Store
- Observability
- BentoCloud deployment
See Documentation for more tutorials and guides.
Get involved and join our Community Slack 💬, where thousands of AI/ML engineers help each other, contribute to the project, and talk about building AI products.
To report a bug or suggest a feature request, use GitHub Issues.
There are many ways to contribute to the project:
- Report bugs and "Thumbs up" on issues that are relevant to you.
- Investigate issues and review other developers' pull requests.
- Contribute code or documentation to the project by submitting a GitHub pull request.
- Check out the Contributing Guide and Development Guide to learn more.
- Share your feedback and discuss roadmap plans in the
#bentoml-contributors
channel here.
Thanks to all of our amazing contributors!
The BentoML framework collects anonymous usage data that helps our community improve the product. Only BentoML's internal API calls are being reported. This excludes any sensitive information, such as user code, model data, model names, or stack traces. Here's the code used for usage tracking. You can opt-out of usage tracking by the --do-not-track
CLI option:
bentoml [command] --do-not-track
Or by setting the environment variable:
export BENTOML_DO_NOT_TRACK=True
For Tasks:
Click tags to check more tools for each tasksFor Jobs:
Alternative AI tools for BentoML
Similar Open Source Tools
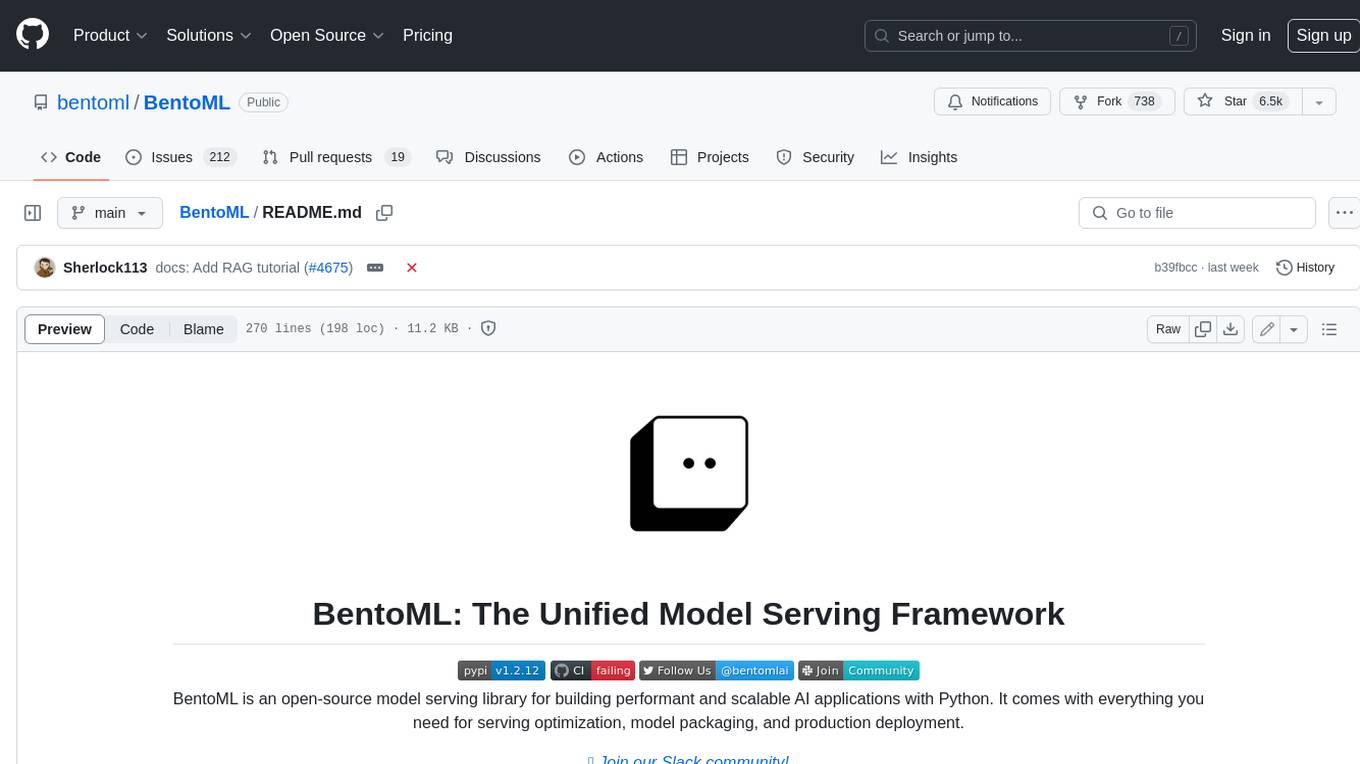
BentoML
BentoML is an open-source model serving library for building performant and scalable AI applications with Python. It comes with everything you need for serving optimization, model packaging, and production deployment.
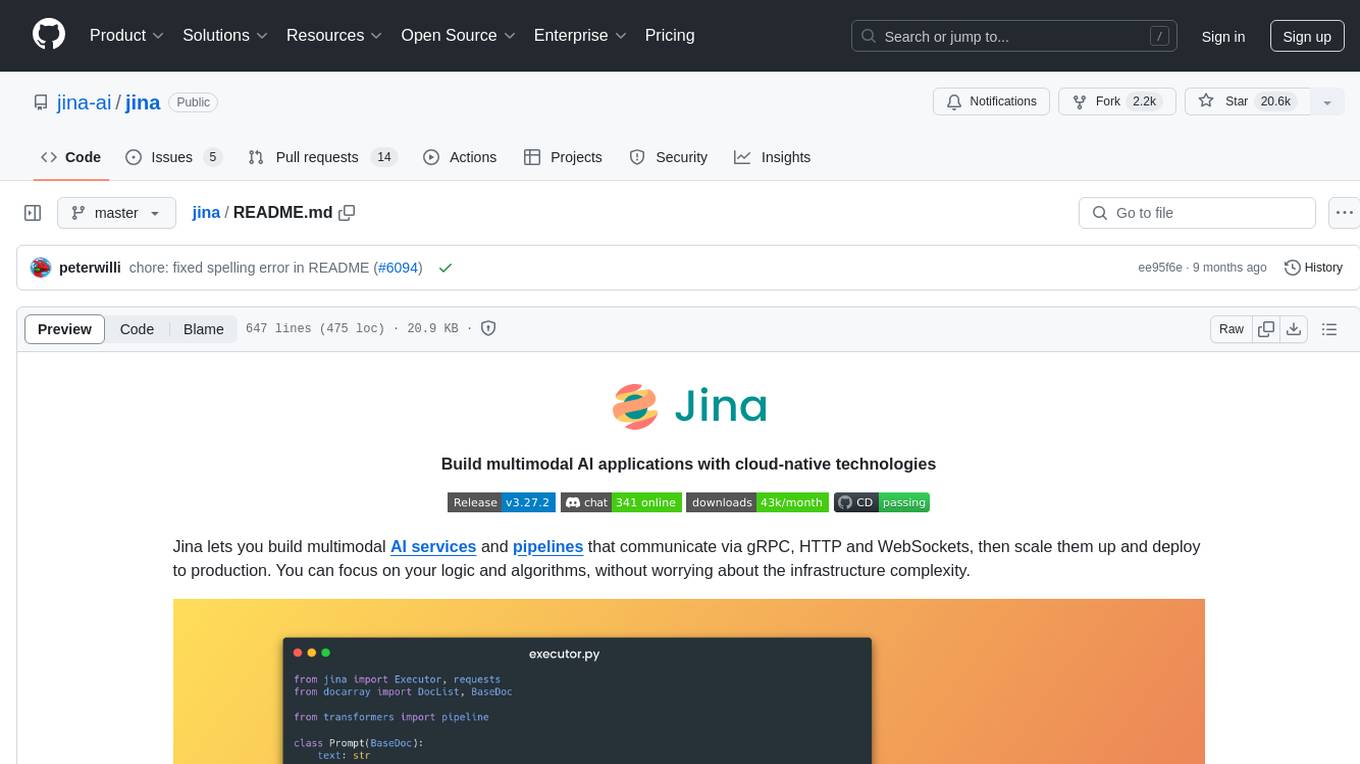
jina
Jina is a tool that allows users to build multimodal AI services and pipelines using cloud-native technologies. It provides a Pythonic experience for serving ML models and transitioning from local deployment to advanced orchestration frameworks like Docker-Compose, Kubernetes, or Jina AI Cloud. Users can build and serve models for any data type and deep learning framework, design high-performance services with easy scaling, serve LLM models while streaming their output, integrate with Docker containers via Executor Hub, and host on CPU/GPU using Jina AI Cloud. Jina also offers advanced orchestration and scaling capabilities, a smooth transition to the cloud, and easy scalability and concurrency features for applications. Users can deploy to their own cloud or system with Kubernetes and Docker Compose integration, and even deploy to JCloud for autoscaling and monitoring.
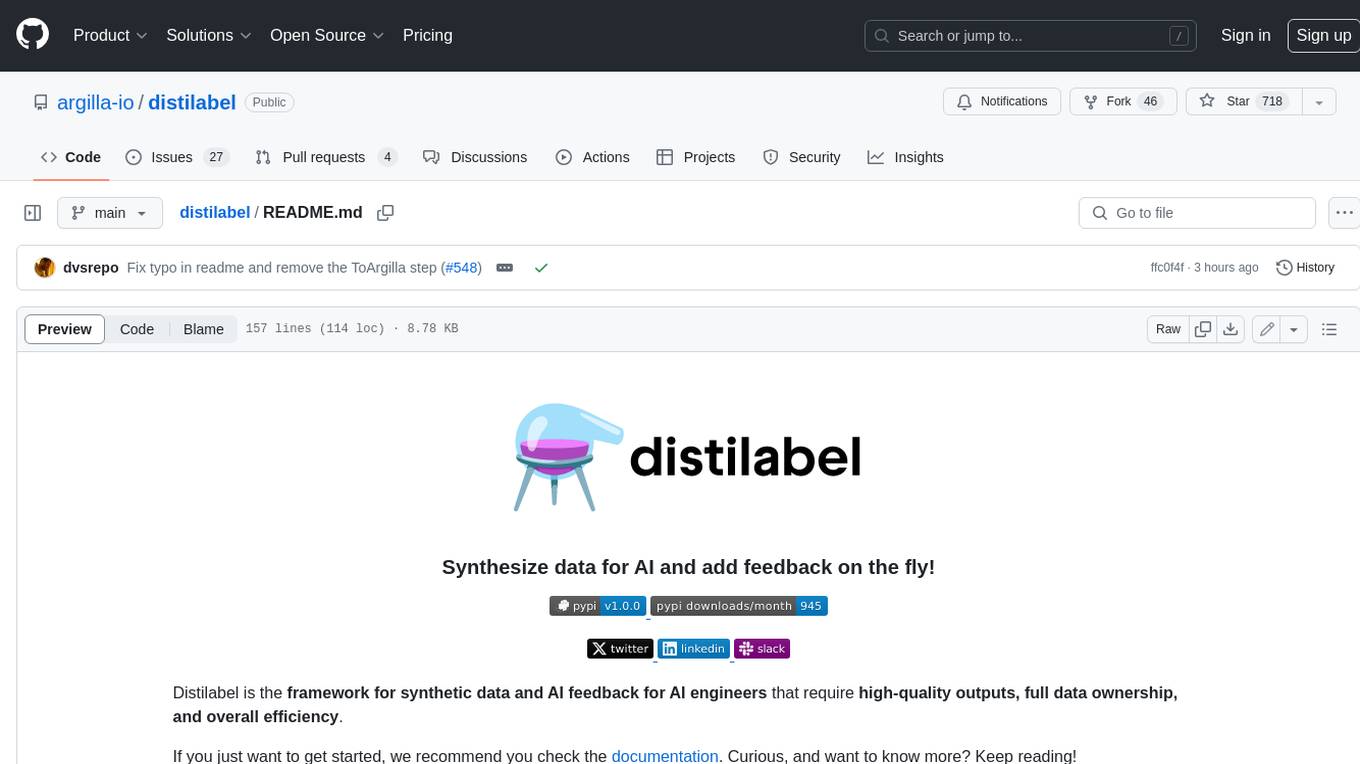
distilabel
Distilabel is a framework for synthetic data and AI feedback for AI engineers that require high-quality outputs, full data ownership, and overall efficiency. It helps you synthesize data and provide AI feedback to improve the quality of your AI models. With Distilabel, you can: * **Synthesize data:** Generate synthetic data to train your AI models. This can help you to overcome the challenges of data scarcity and bias. * **Provide AI feedback:** Get feedback from AI models on your data. This can help you to identify errors and improve the quality of your data. * **Improve your AI output quality:** By using Distilabel to synthesize data and provide AI feedback, you can improve the quality of your AI models and get better results.
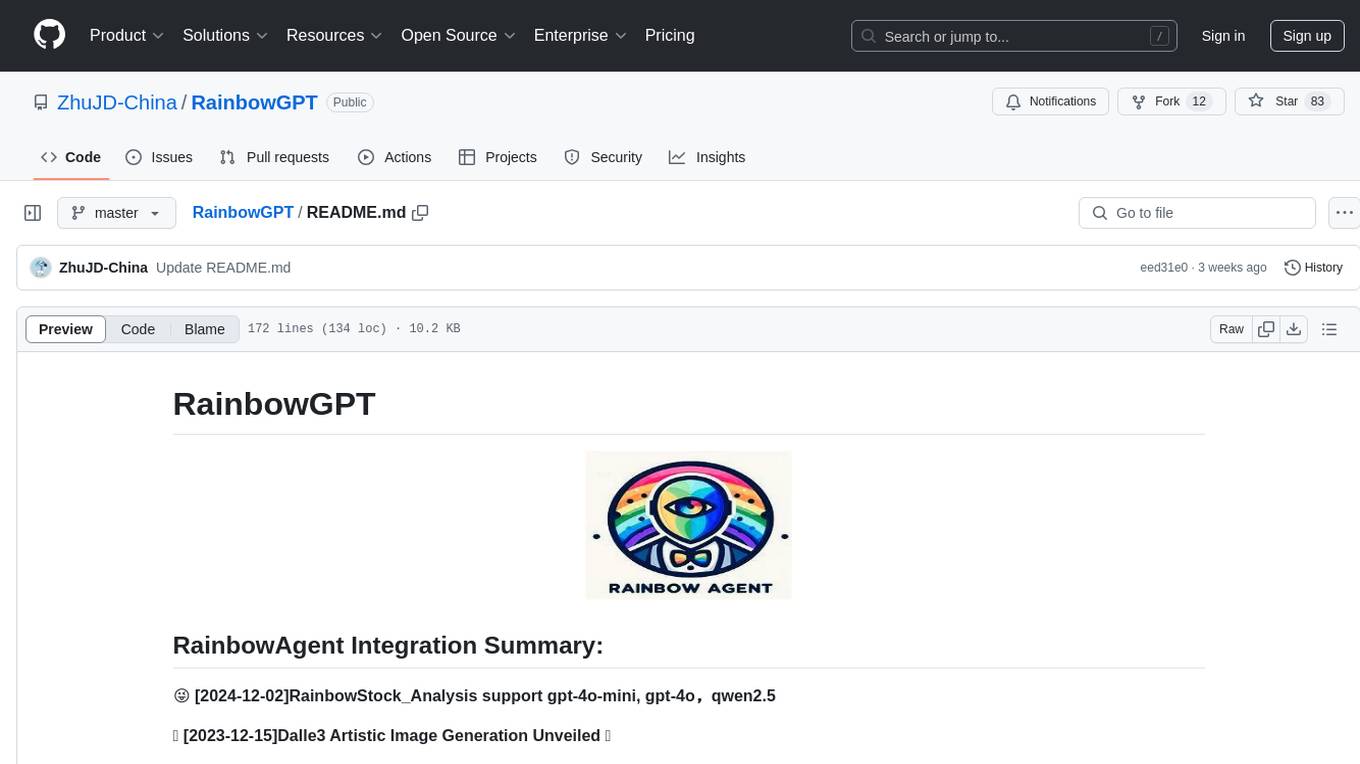
RainbowGPT
RainbowGPT is a versatile tool that offers a range of functionalities, including Stock Analysis for financial decision-making, MySQL Management for database navigation, and integration of AI technologies like GPT-4 and ChatGlm3. It provides a user-friendly interface suitable for all skill levels, ensuring seamless information flow and continuous expansion of emerging technologies. The tool enhances adaptability, creativity, and insight, making it a valuable asset for various projects and tasks.
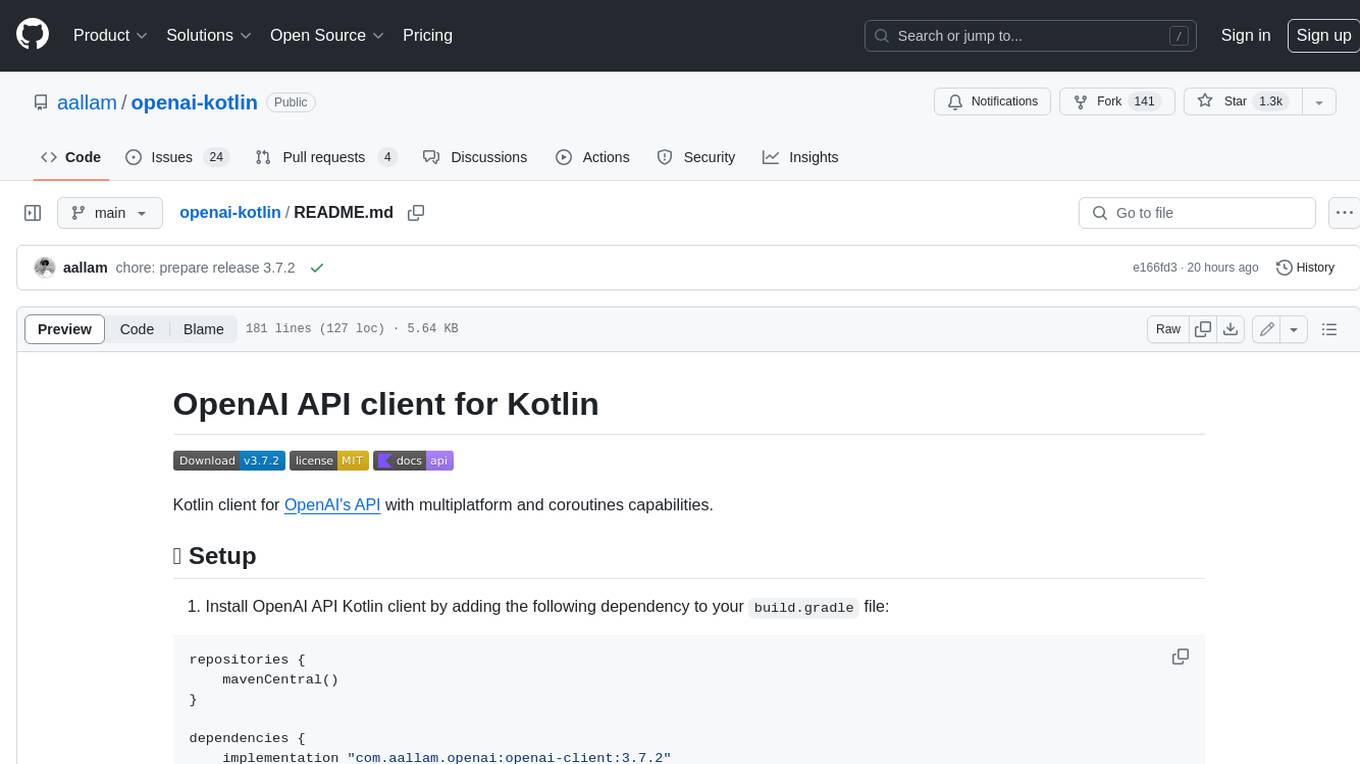
openai-kotlin
OpenAI Kotlin API client is a Kotlin client for OpenAI's API with multiplatform and coroutines capabilities. It allows users to interact with OpenAI's API using Kotlin programming language. The client supports various features such as models, chat, images, embeddings, files, fine-tuning, moderations, audio, assistants, threads, messages, and runs. It also provides guides on getting started, chat & function call, file source guide, and assistants. Sample apps are available for reference, and troubleshooting guides are provided for common issues. The project is open-source and licensed under the MIT license, allowing contributions from the community.
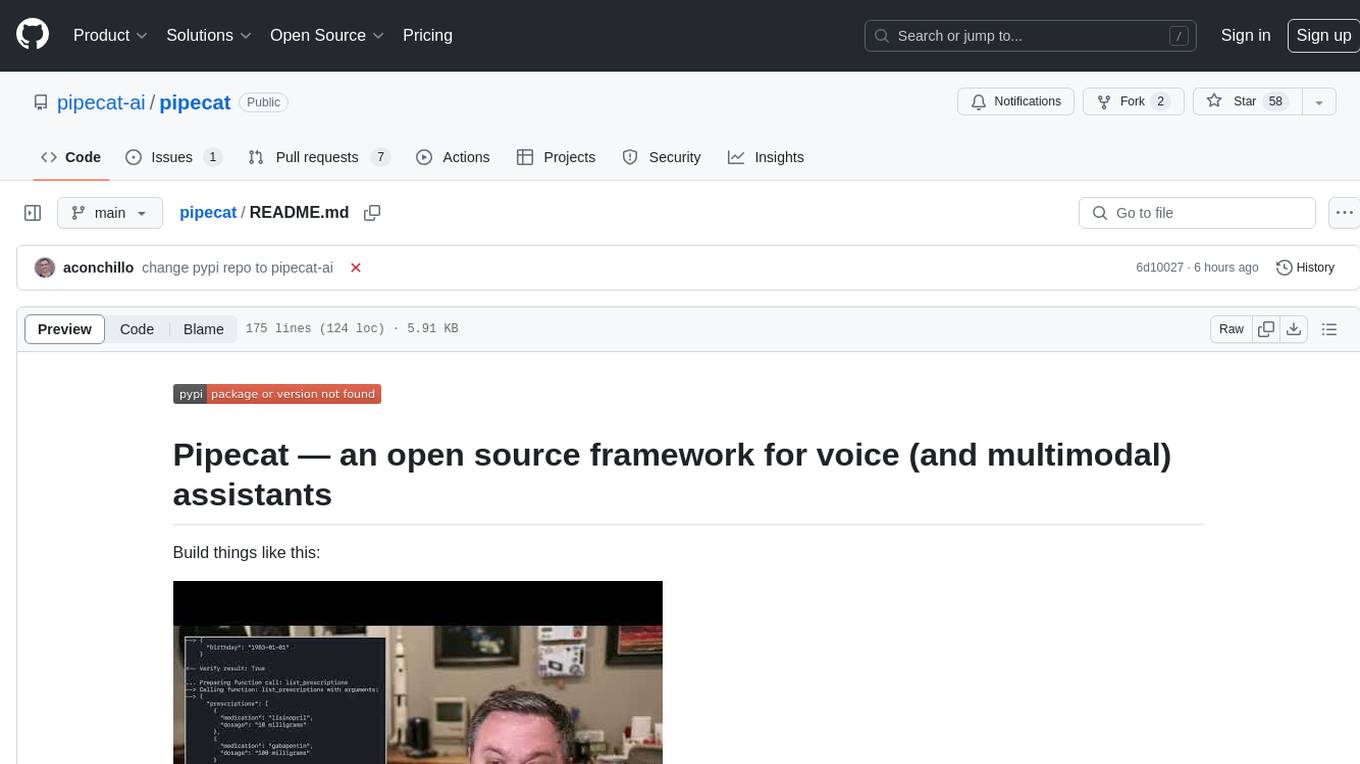
pipecat
Pipecat is an open-source framework designed for building generative AI voice bots and multimodal assistants. It provides code building blocks for interacting with AI services, creating low-latency data pipelines, and transporting audio, video, and events over the Internet. Pipecat supports various AI services like speech-to-text, text-to-speech, image generation, and vision models. Users can implement new services and contribute to the framework. Pipecat aims to simplify the development of applications like personal coaches, meeting assistants, customer support bots, and more by providing a complete framework for integrating AI services.
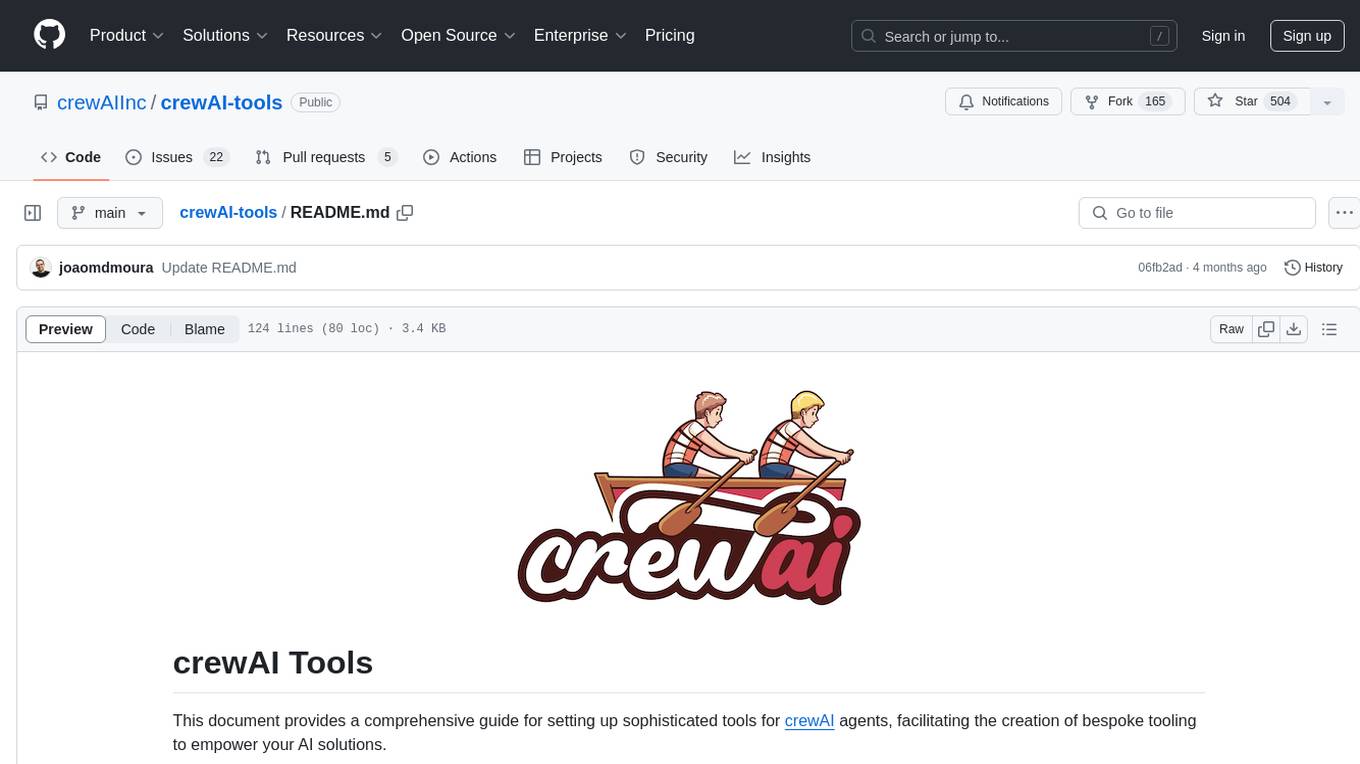
crewAI-tools
This repository provides a guide for setting up tools for crewAI agents to enhance functionality. It offers steps to equip agents with ready-to-use tools and create custom ones. Tools are expected to return strings for generating responses. Users can create tools by subclassing BaseTool or using the tool decorator. Contributions are welcome to enrich the toolset, and guidelines are provided for contributing. The development setup includes installing dependencies, activating virtual environment, setting up pre-commit hooks, running tests, static type checking, packaging, and local installation. The goal is to empower AI solutions through advanced tooling.
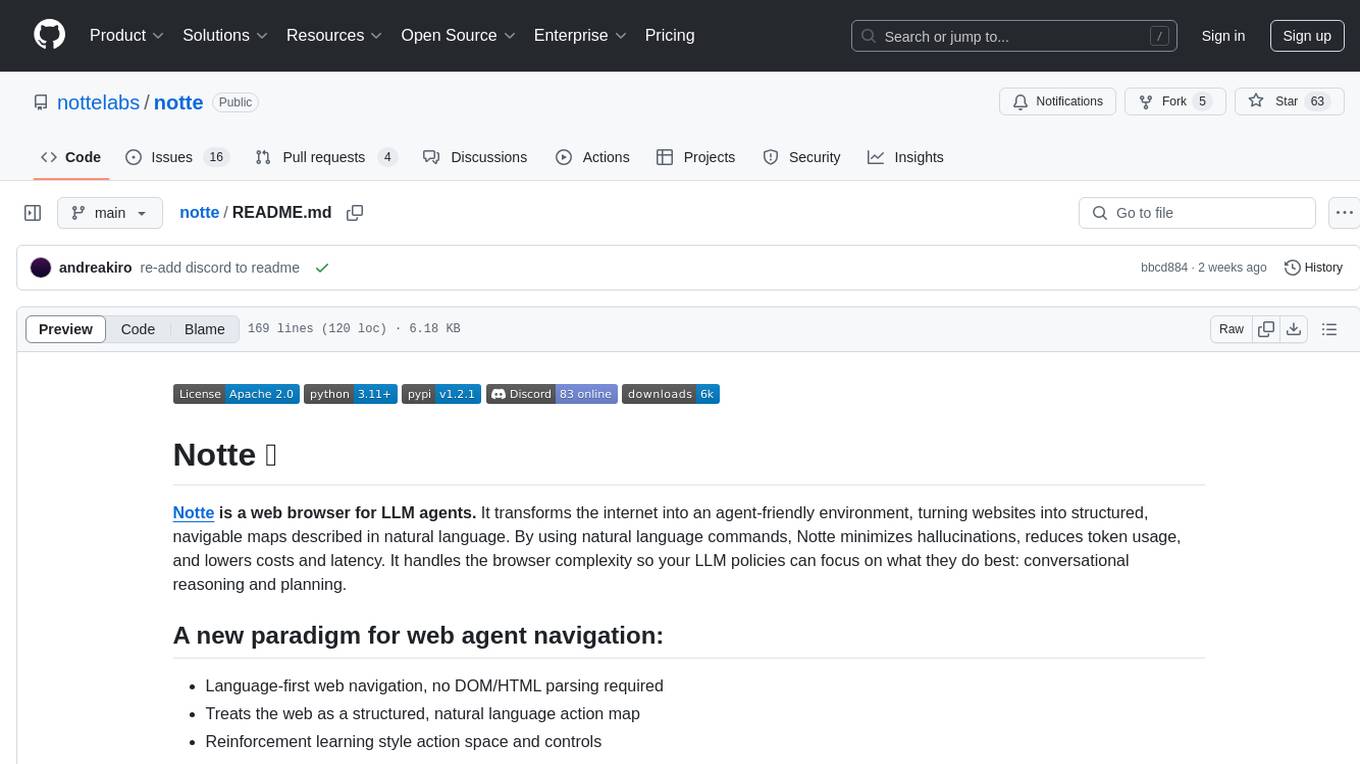
notte
Notte is a web browser designed specifically for LLM agents, providing a language-first web navigation experience without the need for DOM/HTML parsing. It transforms websites into structured, navigable maps described in natural language, enabling users to interact with the web using natural language commands. By simplifying browser complexity, Notte allows LLM policies to focus on conversational reasoning and planning, reducing token usage, costs, and latency. The tool supports various language model providers and offers a reinforcement learning style action space and controls for full navigation control.
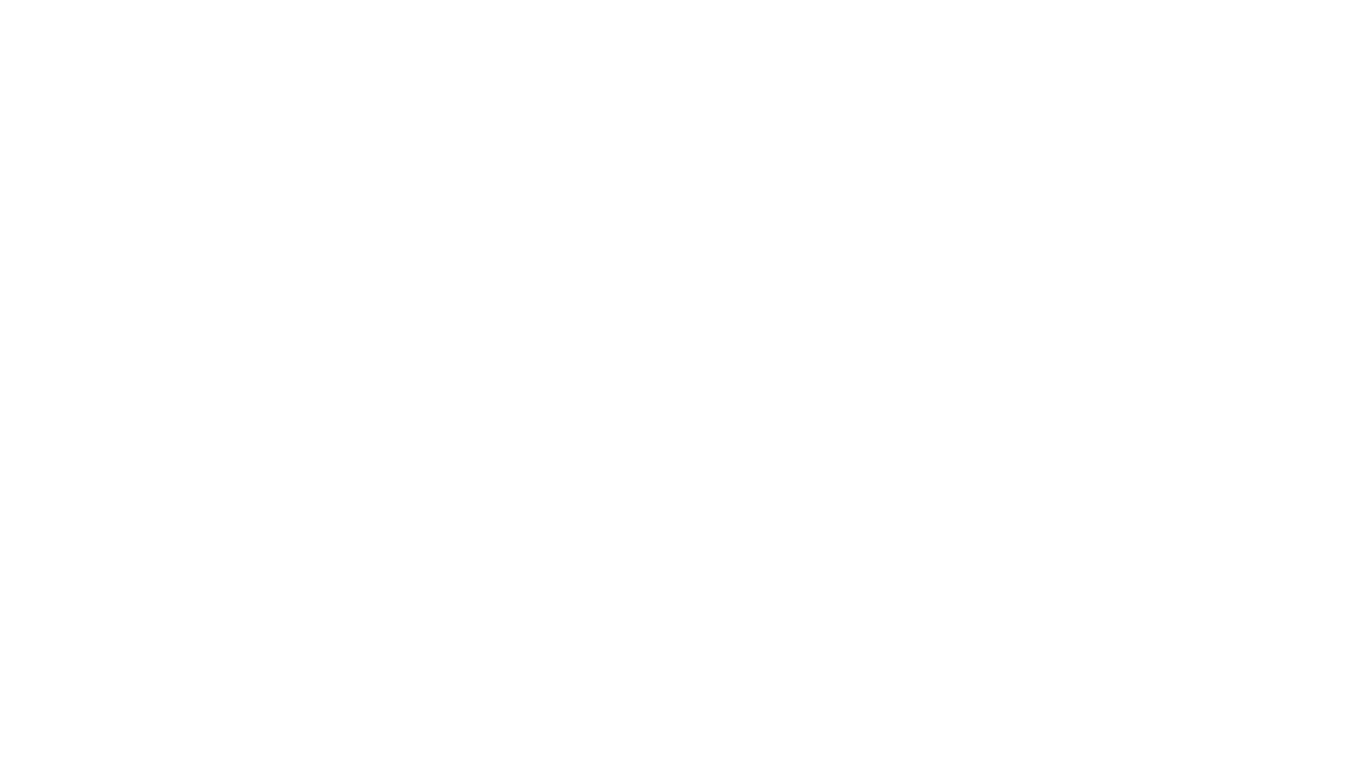
orama-core
OramaCore is a database designed for AI projects, answer engines, copilots, and search functionalities. It offers features such as a full-text search engine, vector database, LLM interface, and various utilities. The tool is currently under active development and not recommended for production use due to potential API changes. OramaCore aims to provide a comprehensive solution for managing data and enabling advanced AI capabilities in projects.
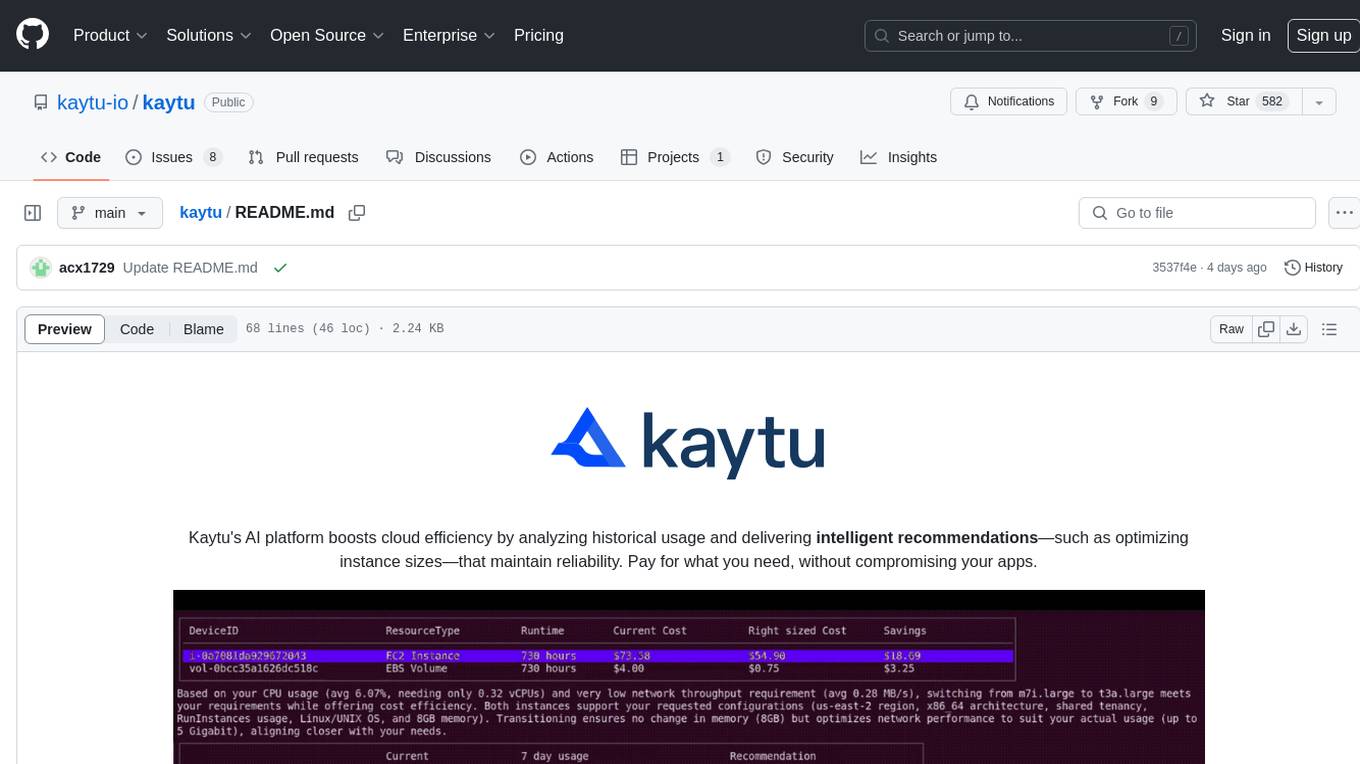
kaytu
Kaytu is an AI platform that enhances cloud efficiency by analyzing historical usage data and providing intelligent recommendations for optimizing instance sizes. Users can pay for only what they need without compromising the performance of their applications. The platform is easy to use with a one-line command, allows customization for specific requirements, and ensures security by extracting metrics from the client side. Kaytu is open-source and supports AWS services, with plans to expand to GCP, Azure, GPU optimization, and observability data from Prometheus in the future.
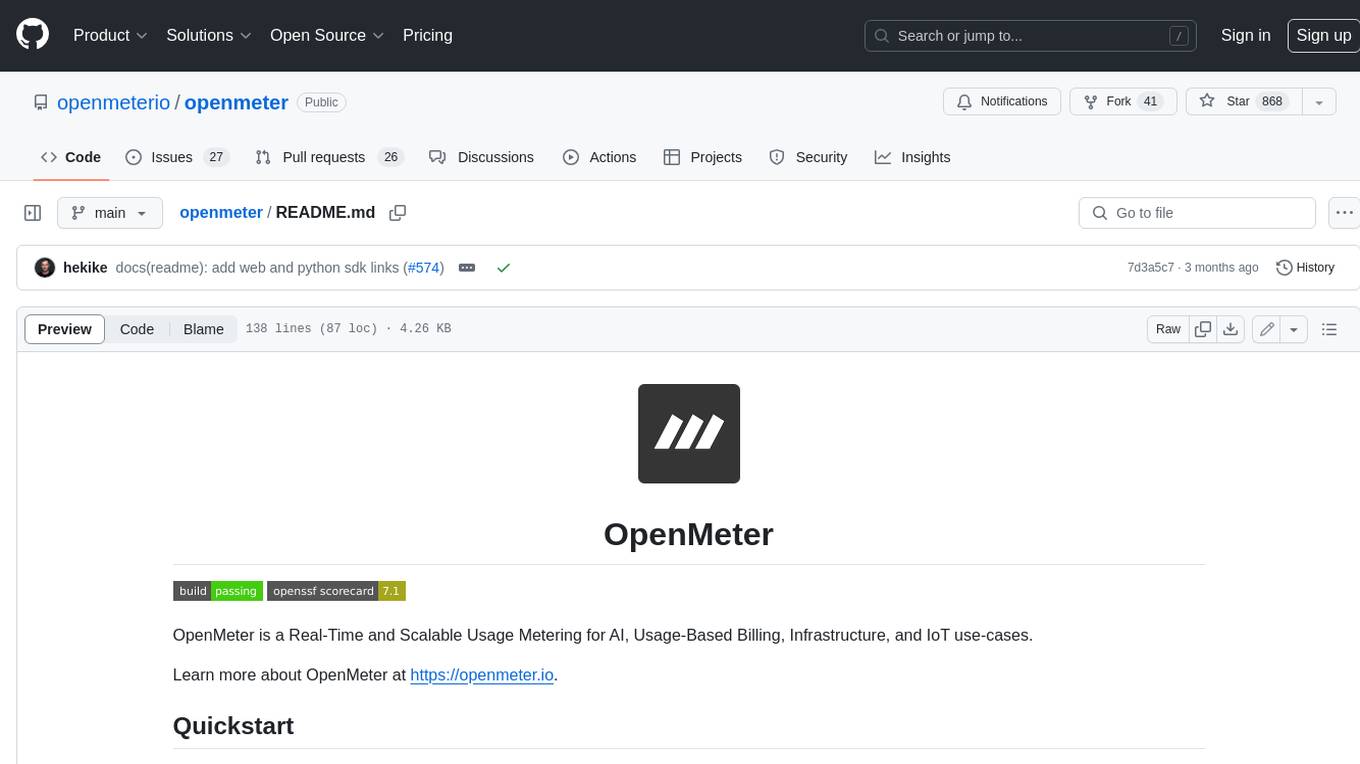
openmeter
OpenMeter is a real-time and scalable usage metering tool for AI, usage-based billing, infrastructure, and IoT use cases. It provides a REST API for integrations and offers client SDKs in Node.js, Python, Go, and Web. OpenMeter is licensed under the Apache 2.0 License.
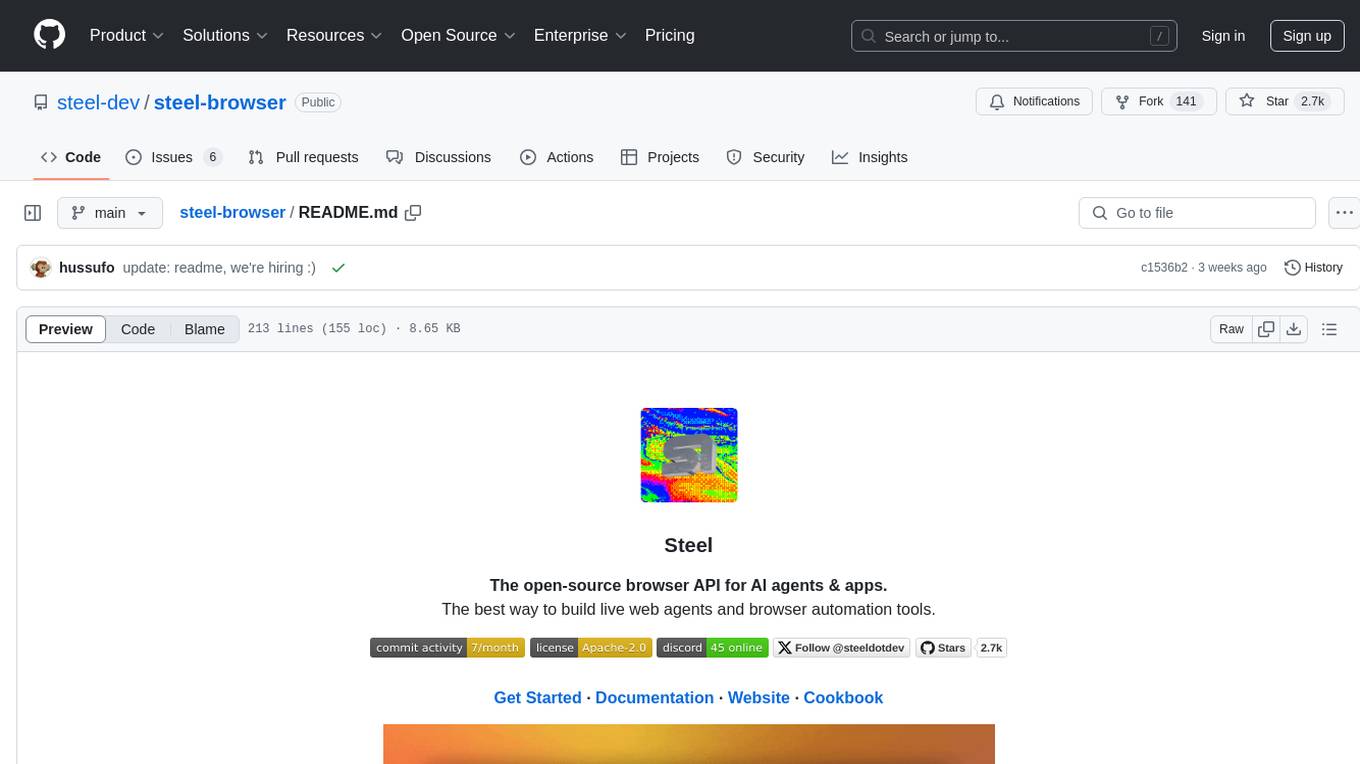
steel-browser
Steel is an open-source browser API designed for AI agents and applications, simplifying the process of building live web agents and browser automation tools. It serves as a core building block for a production-ready, containerized browser sandbox with features like stealth capabilities, text-to-markdown session management, UI for session viewing/debugging, and full browser control through popular automation frameworks. Steel allows users to control, run, and manage a production-ready browser environment via a REST API, offering features such as full browser control, session management, proxy support, extension support, debugging tools, anti-detection mechanisms, resource management, and various browser tools. It aims to streamline complex browsing tasks programmatically, enabling users to focus on their AI applications while Steel handles the underlying complexity.
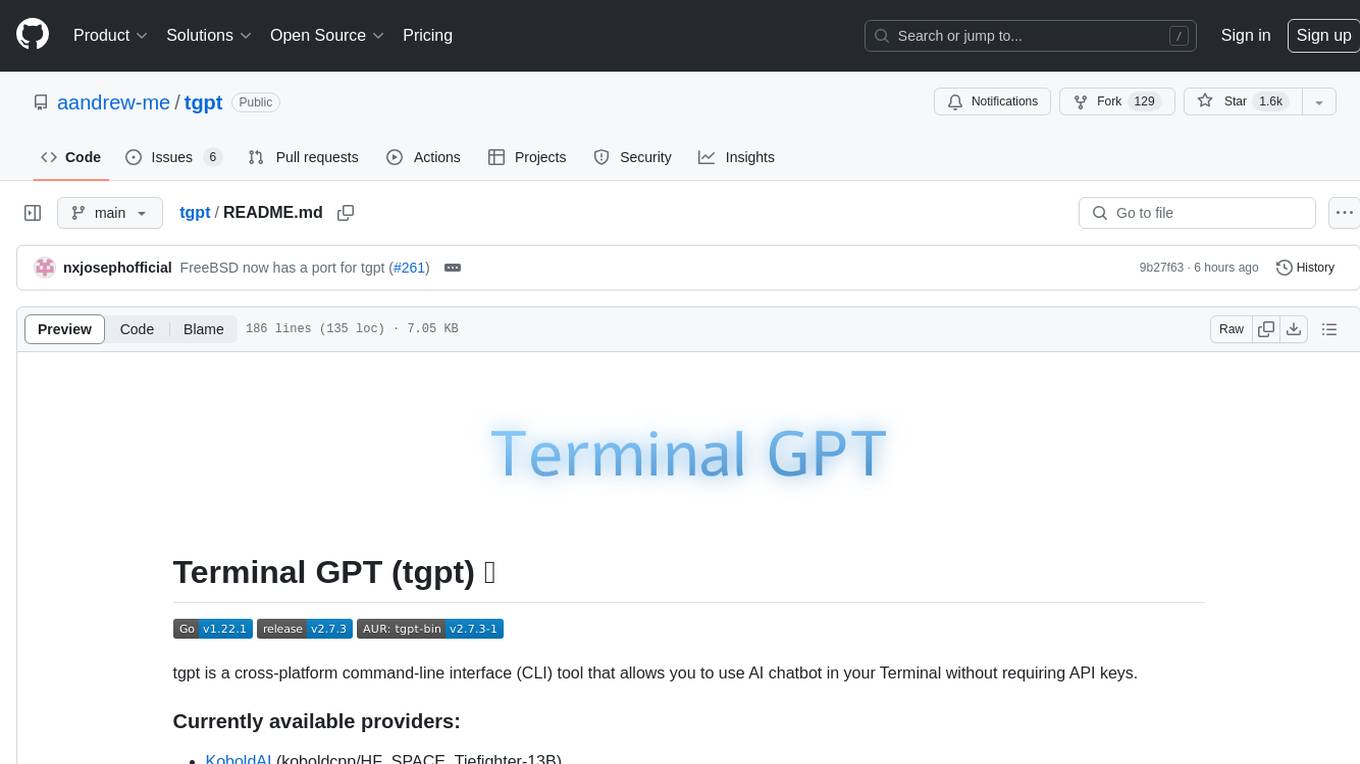
tgpt
tgpt is a cross-platform command-line interface (CLI) tool that allows users to interact with AI chatbots in the Terminal without needing API keys. It supports various AI providers such as KoboldAI, Phind, Llama2, Blackbox AI, and OpenAI. Users can generate text, code, and images using different flags and options. The tool can be installed on GNU/Linux, MacOS, FreeBSD, and Windows systems. It also supports proxy configurations and provides options for updating and uninstalling the tool.
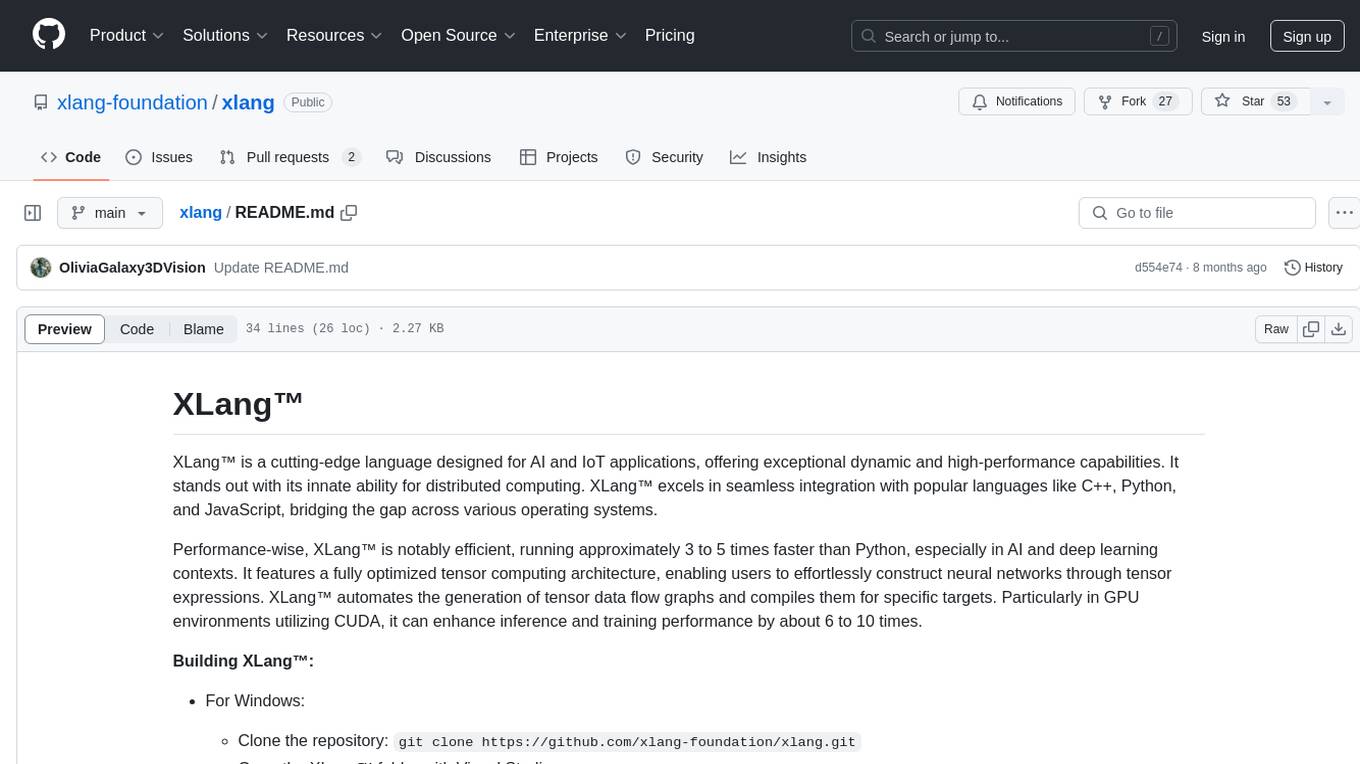
xlang
XLang™ is a cutting-edge language designed for AI and IoT applications, offering exceptional dynamic and high-performance capabilities. It excels in distributed computing and seamless integration with popular languages like C++, Python, and JavaScript. Notably efficient, running 3 to 5 times faster than Python in AI and deep learning contexts. Features optimized tensor computing architecture for constructing neural networks through tensor expressions. Automates tensor data flow graph generation and compilation for specific targets, enhancing GPU performance by 6 to 10 times in CUDA environments.
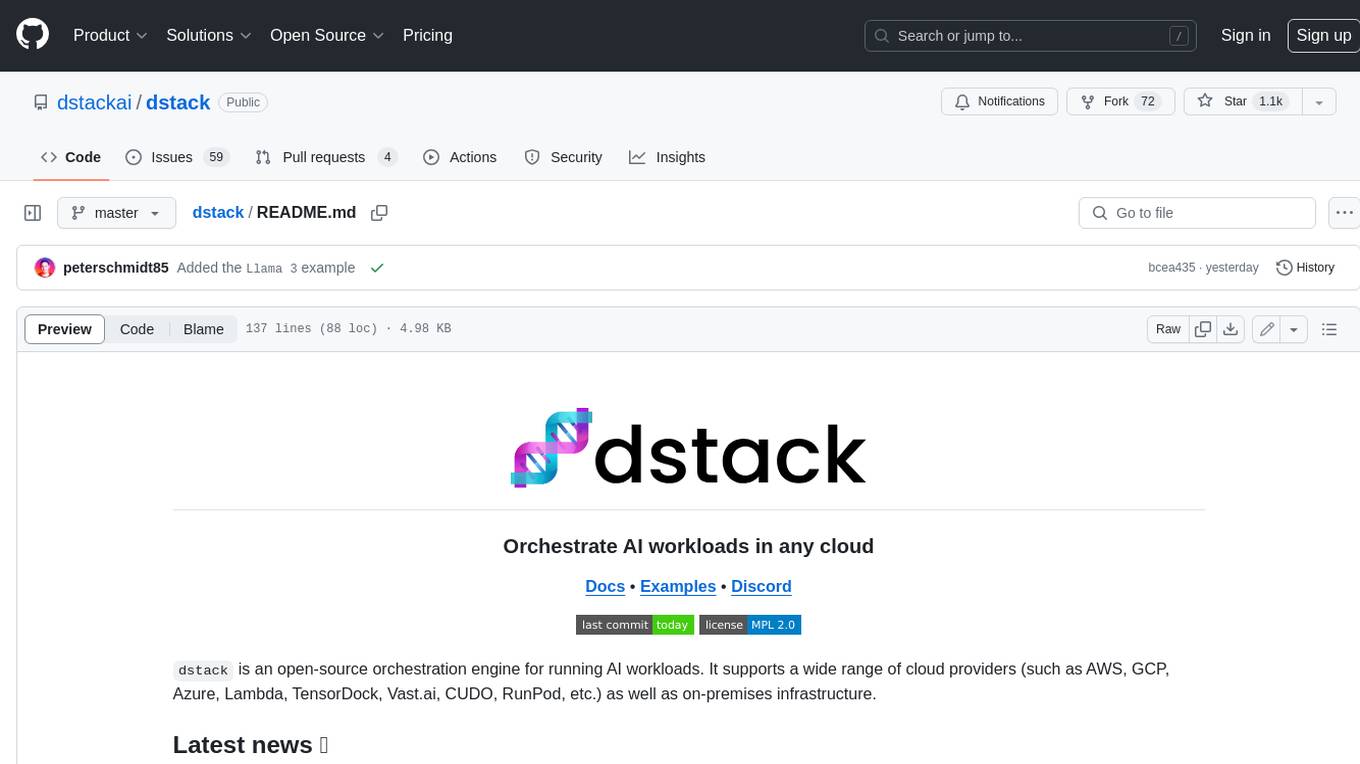
dstack
Dstack is an open-source orchestration engine for running AI workloads in any cloud. It supports a wide range of cloud providers (such as AWS, GCP, Azure, Lambda, TensorDock, Vast.ai, CUDO, RunPod, etc.) as well as on-premises infrastructure. With Dstack, you can easily set up and manage dev environments, tasks, services, and pools for your AI workloads.
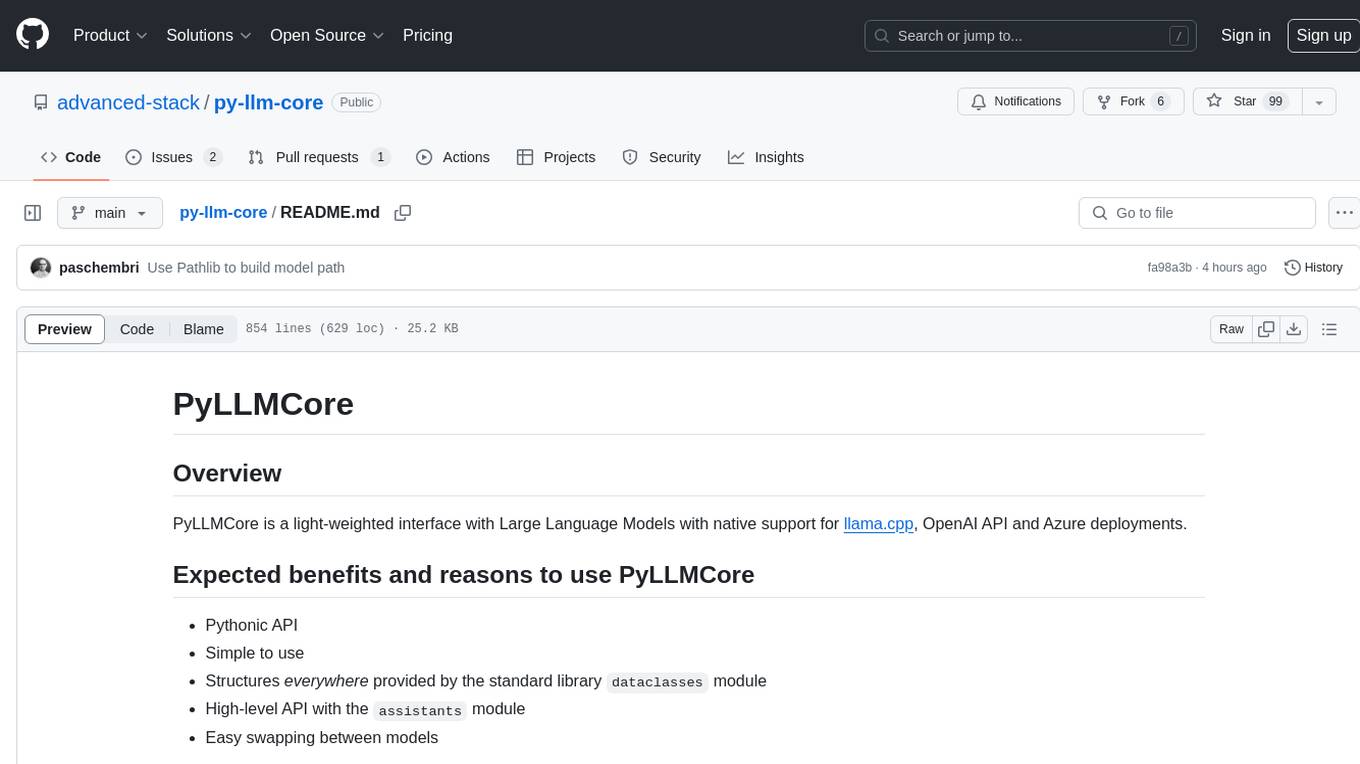
py-llm-core
PyLLMCore is a light-weighted interface with Large Language Models with native support for llama.cpp, OpenAI API, and Azure deployments. It offers a Pythonic API that is simple to use, with structures provided by the standard library dataclasses module. The high-level API includes the assistants module for easy swapping between models. PyLLMCore supports various models including those compatible with llama.cpp, OpenAI, and Azure APIs. It covers use cases such as parsing, summarizing, question answering, hallucinations reduction, context size management, and tokenizing. The tool allows users to interact with language models for tasks like parsing text, summarizing content, answering questions, reducing hallucinations, managing context size, and tokenizing text.
For similar tasks
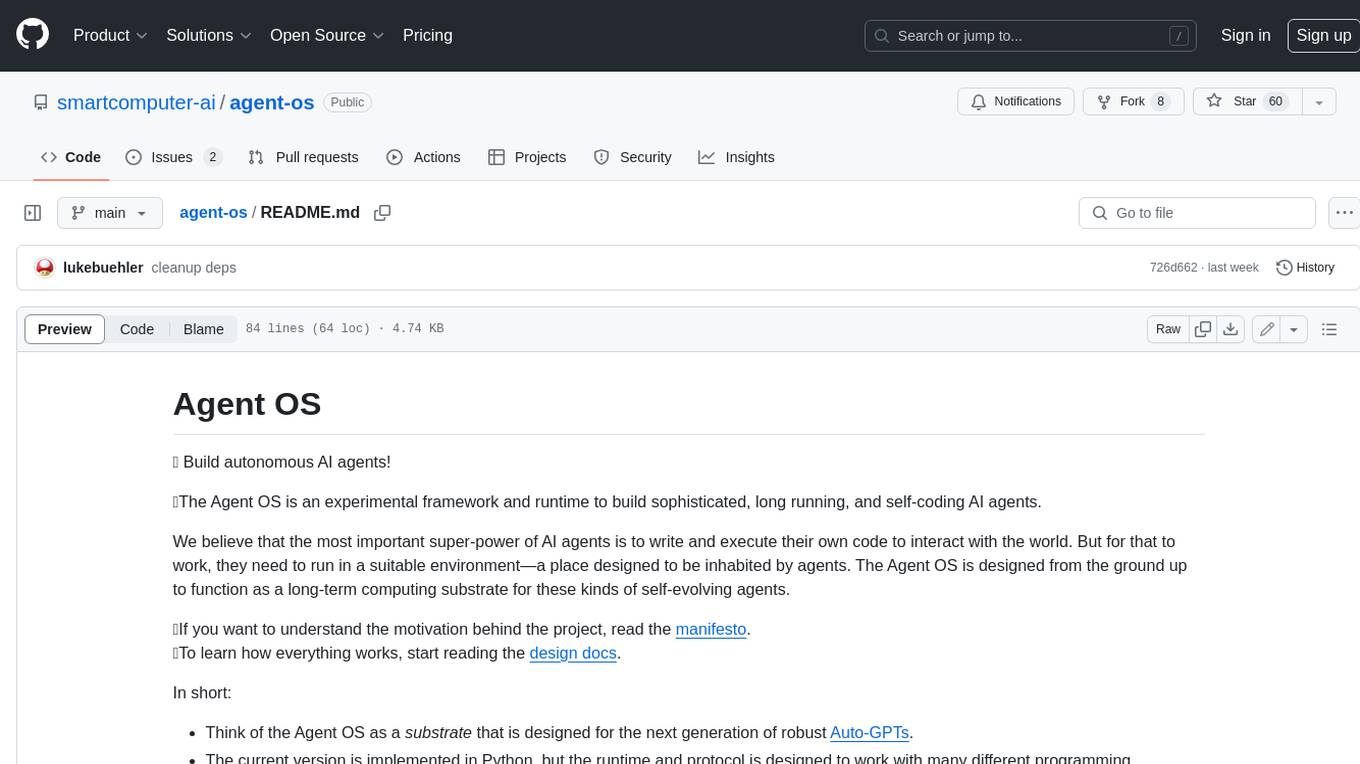
agent-os
The Agent OS is an experimental framework and runtime to build sophisticated, long running, and self-coding AI agents. We believe that the most important super-power of AI agents is to write and execute their own code to interact with the world. But for that to work, they need to run in a suitable environment—a place designed to be inhabited by agents. The Agent OS is designed from the ground up to function as a long-term computing substrate for these kinds of self-evolving agents.
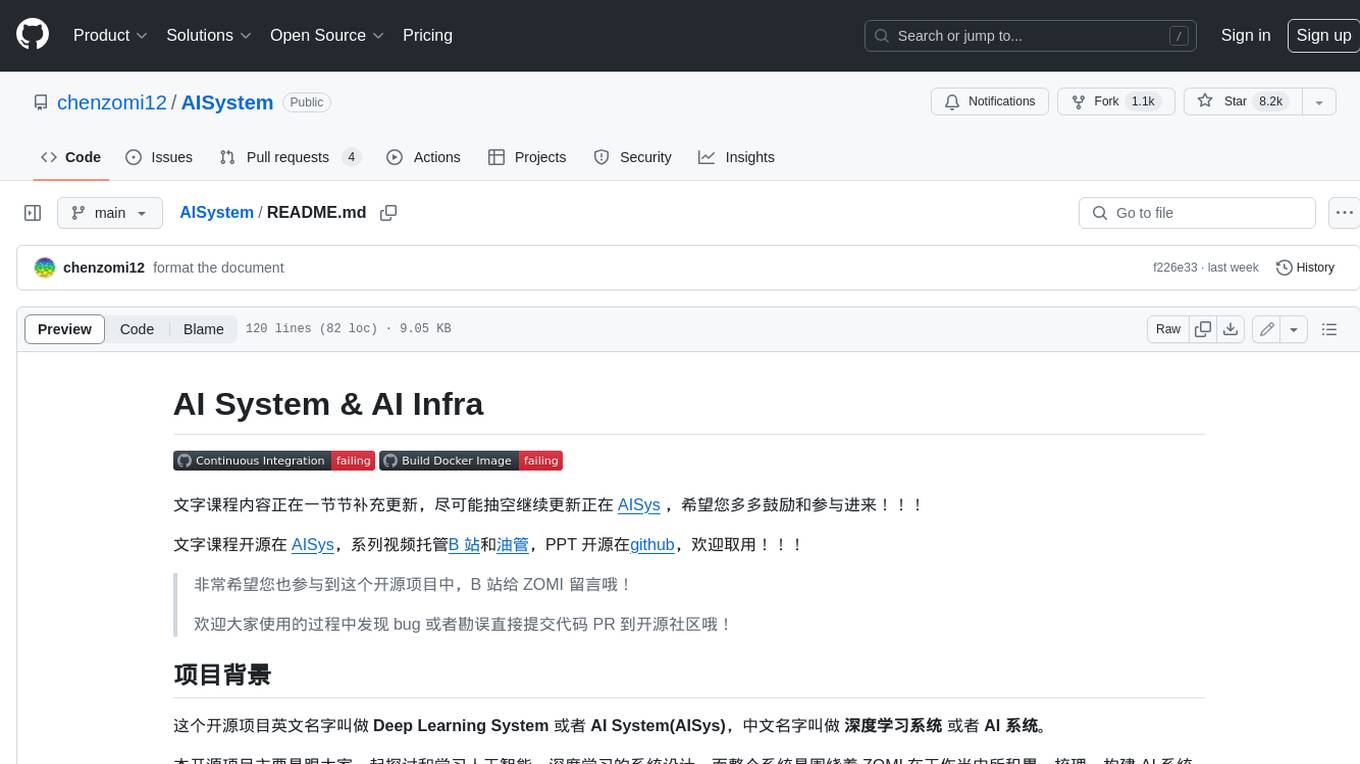
AISystem
This open-source project, also known as **Deep Learning System** or **AI System (AISys)**, aims to explore and learn about the system design of artificial intelligence and deep learning. The project is centered around the full-stack content of AI systems that ZOMI has accumulated,整理, and built during his work. The goal is to collaborate with all friends who are interested in AI open-source projects to jointly promote learning and discussion.
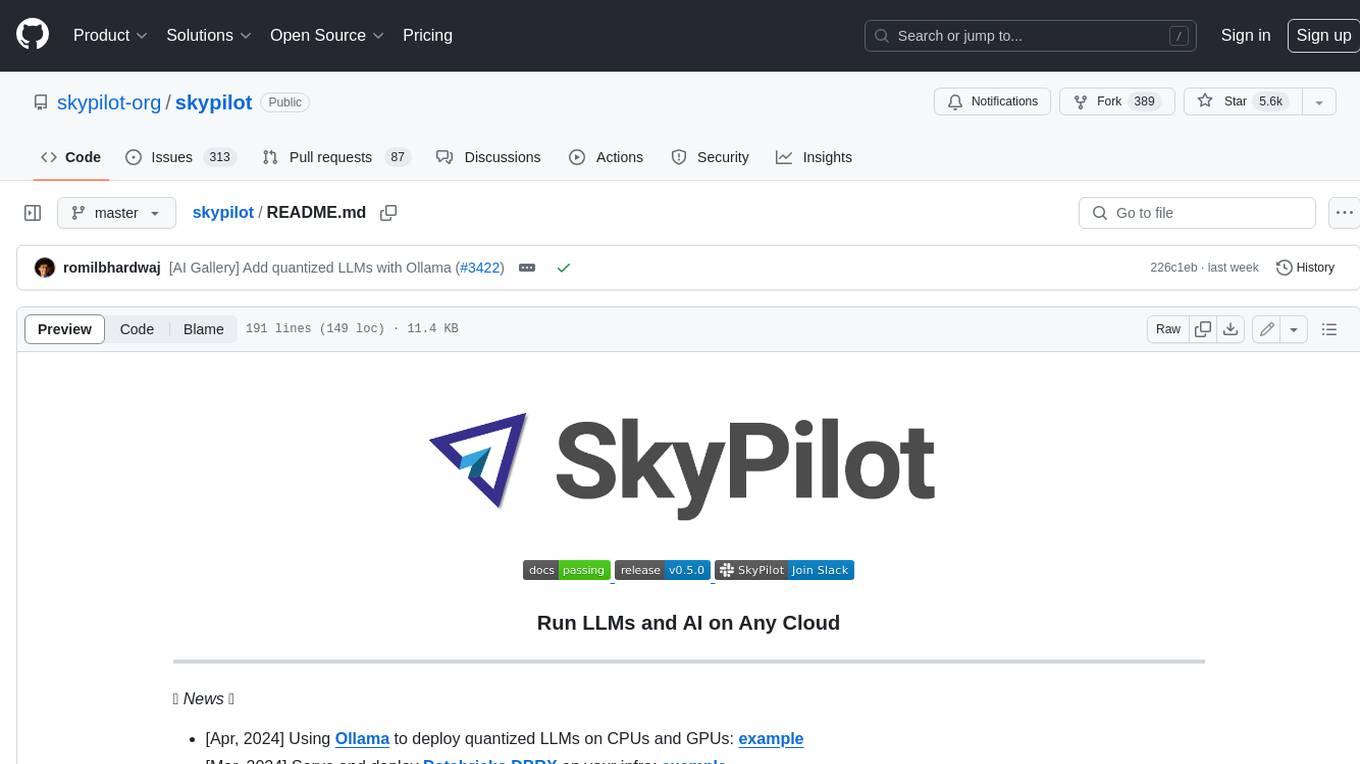
skypilot
SkyPilot is a framework for running LLMs, AI, and batch jobs on any cloud, offering maximum cost savings, highest GPU availability, and managed execution. SkyPilot abstracts away cloud infra burdens: - Launch jobs & clusters on any cloud - Easy scale-out: queue and run many jobs, automatically managed - Easy access to object stores (S3, GCS, R2) SkyPilot maximizes GPU availability for your jobs: * Provision in all zones/regions/clouds you have access to (the _Sky_), with automatic failover SkyPilot cuts your cloud costs: * Managed Spot: 3-6x cost savings using spot VMs, with auto-recovery from preemptions * Optimizer: 2x cost savings by auto-picking the cheapest VM/zone/region/cloud * Autostop: hands-free cleanup of idle clusters SkyPilot supports your existing GPU, TPU, and CPU workloads, with no code changes.
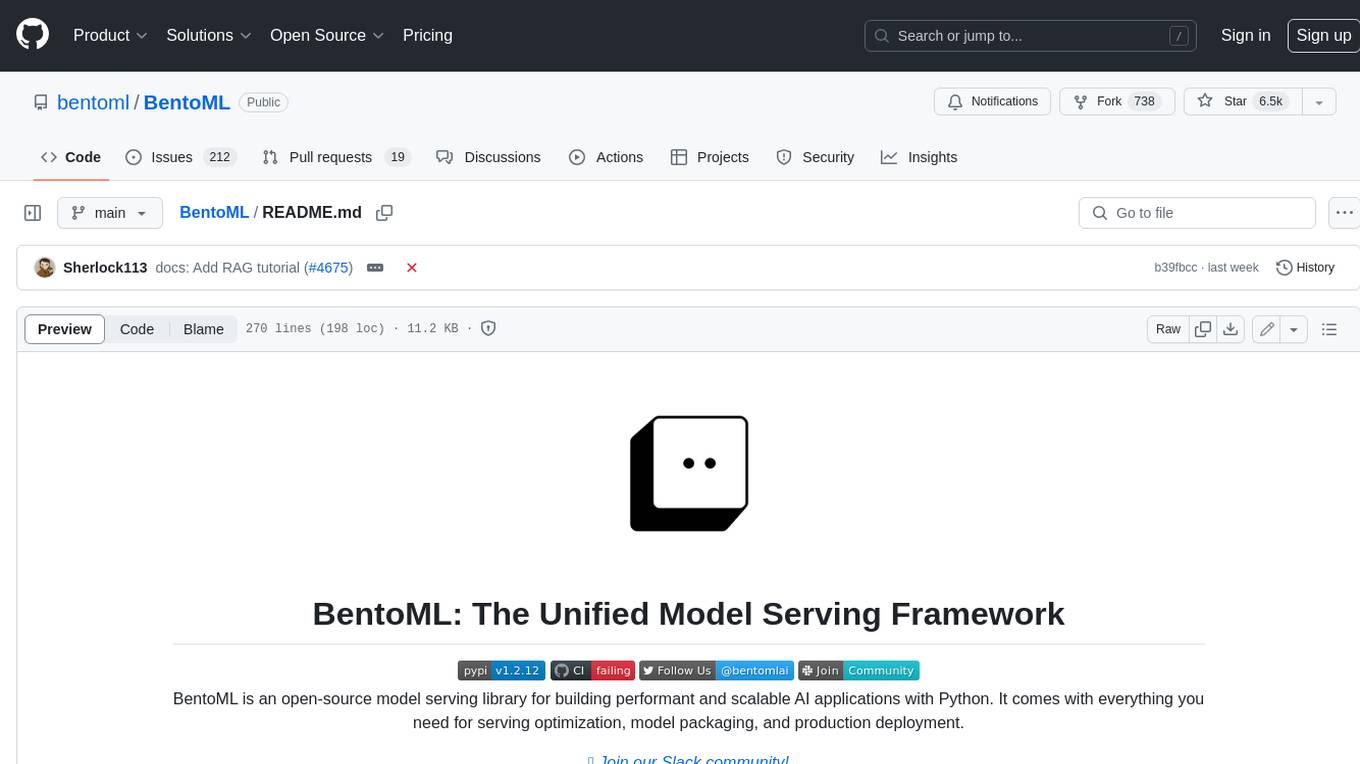
BentoML
BentoML is an open-source model serving library for building performant and scalable AI applications with Python. It comes with everything you need for serving optimization, model packaging, and production deployment.
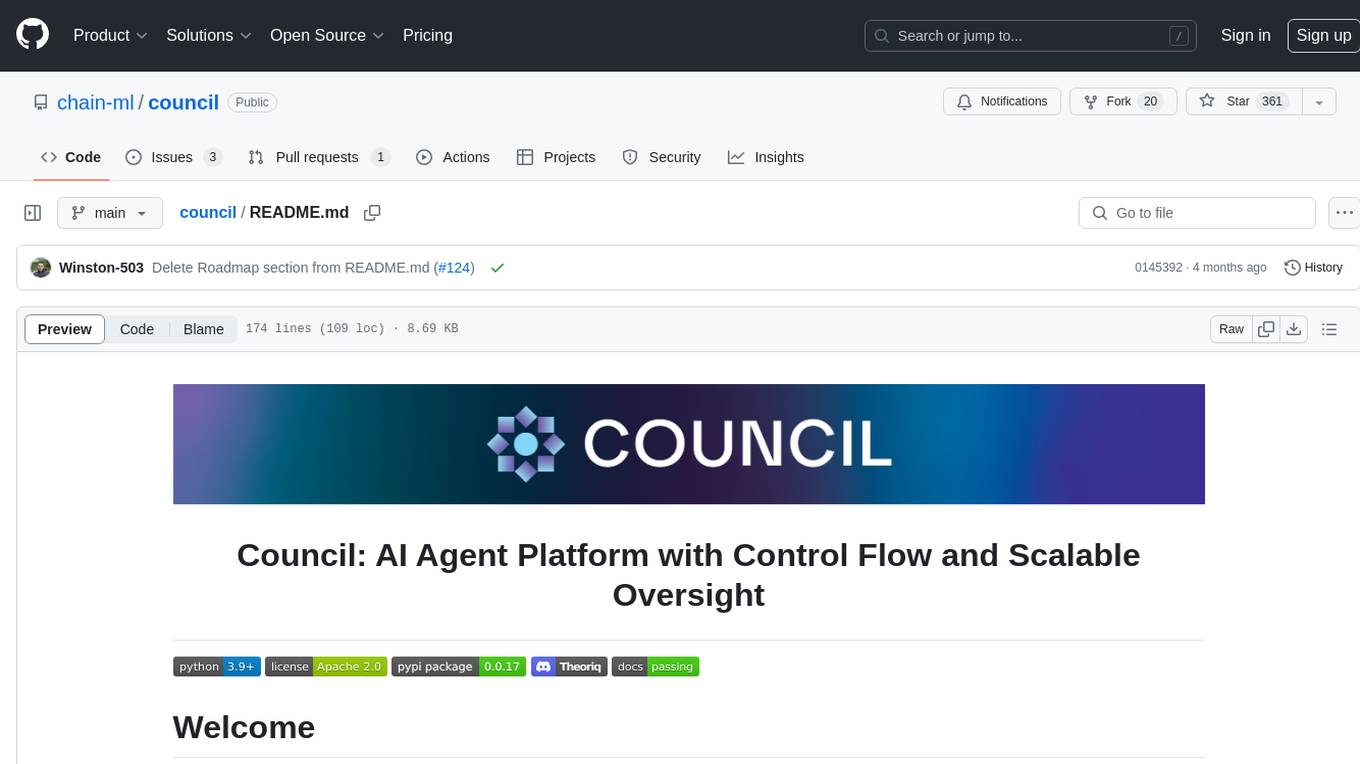
council
Council is an open-source platform designed for the rapid development and deployment of customized generative AI applications using teams of agents. It extends the LLM tool ecosystem by providing advanced control flow and scalable oversight for AI agents. Users can create sophisticated agents with predictable behavior by leveraging Council's powerful approach to control flow using Controllers, Filters, Evaluators, and Budgets. The framework allows for automated routing between agents, comparing, evaluating, and selecting the best results for a task. Council aims to facilitate packaging and deploying agents at scale on multiple platforms while enabling enterprise-grade monitoring and quality control.
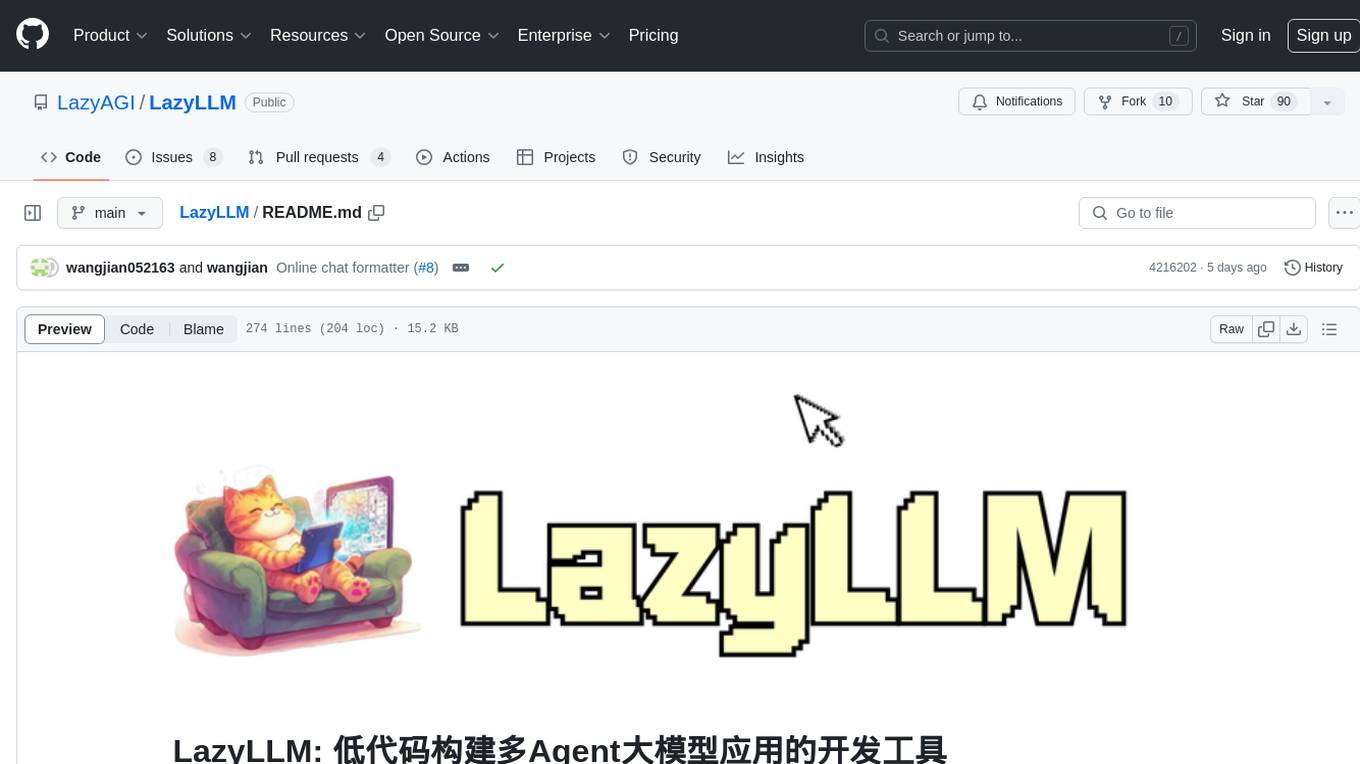
LazyLLM
LazyLLM is a low-code development tool for building complex AI applications with multiple agents. It assists developers in building AI applications at a low cost and continuously optimizing their performance. The tool provides a convenient workflow for application development and offers standard processes and tools for various stages of application development. Users can quickly prototype applications with LazyLLM, analyze bad cases with scenario task data, and iteratively optimize key components to enhance the overall application performance. LazyLLM aims to simplify the AI application development process and provide flexibility for both beginners and experts to create high-quality applications.
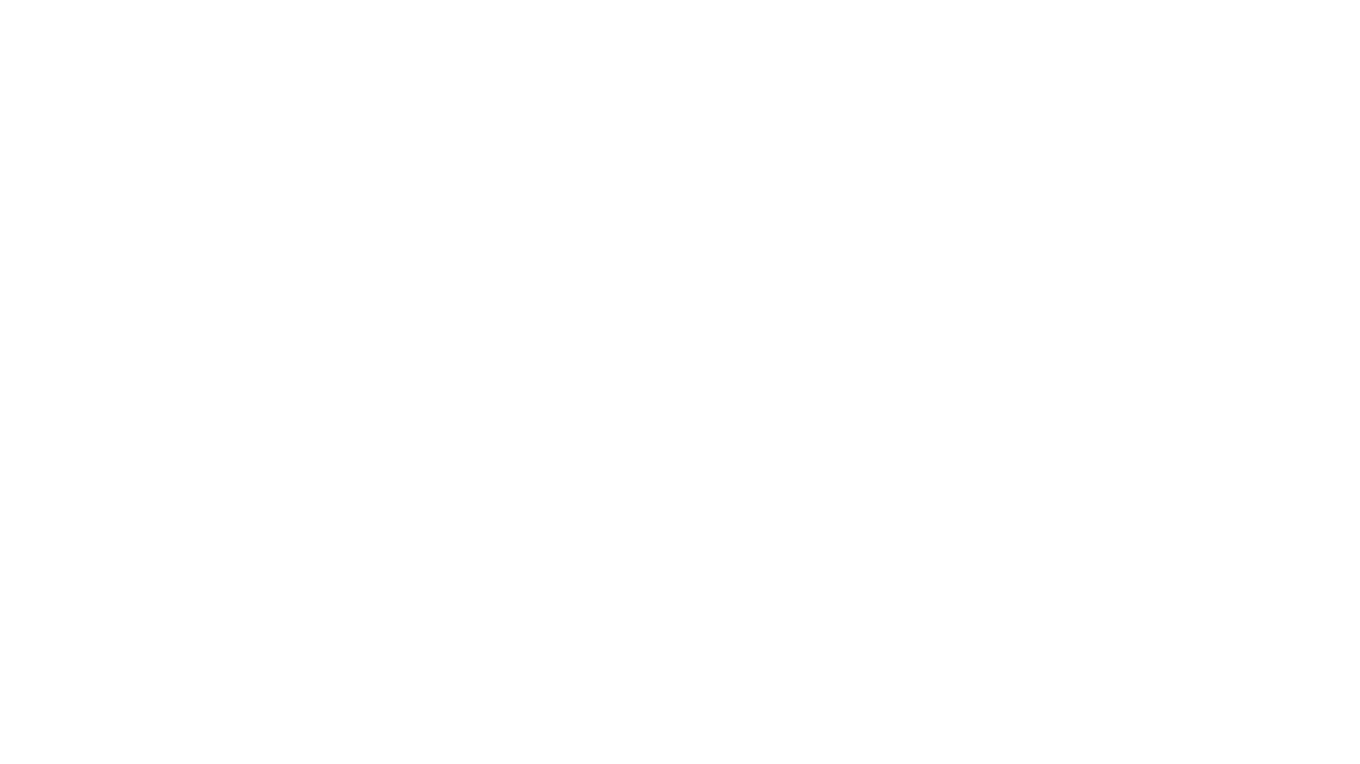
spring-ai-alibaba
Spring AI Alibaba is an AI application framework for Java developers that seamlessly integrates with Alibaba Cloud QWen LLM services and cloud-native infrastructures. It provides features like support for various AI models, high-level AI agent abstraction, function calling, and RAG support. The framework aims to simplify the development, evaluation, deployment, and observability of AI native Java applications. It offers open-source framework and ecosystem integrations to support features like prompt template management, event-driven AI applications, and more.
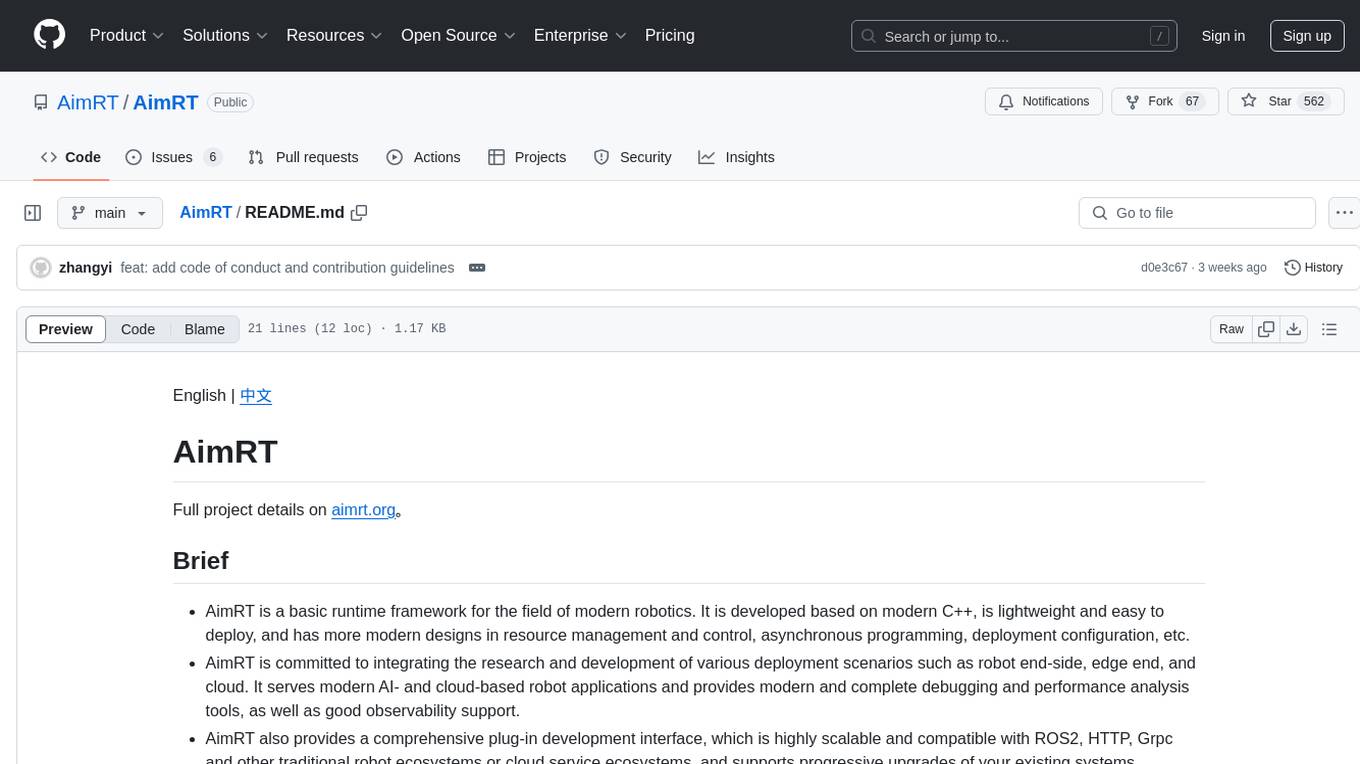
AimRT
AimRT is a basic runtime framework for modern robotics, developed in modern C++ with lightweight and easy deployment. It integrates research and development for robot applications in various deployment scenarios, providing debugging tools and observability support. AimRT offers a plug-in development interface compatible with ROS2, HTTP, Grpc, and other ecosystems for progressive system upgrades.
For similar jobs
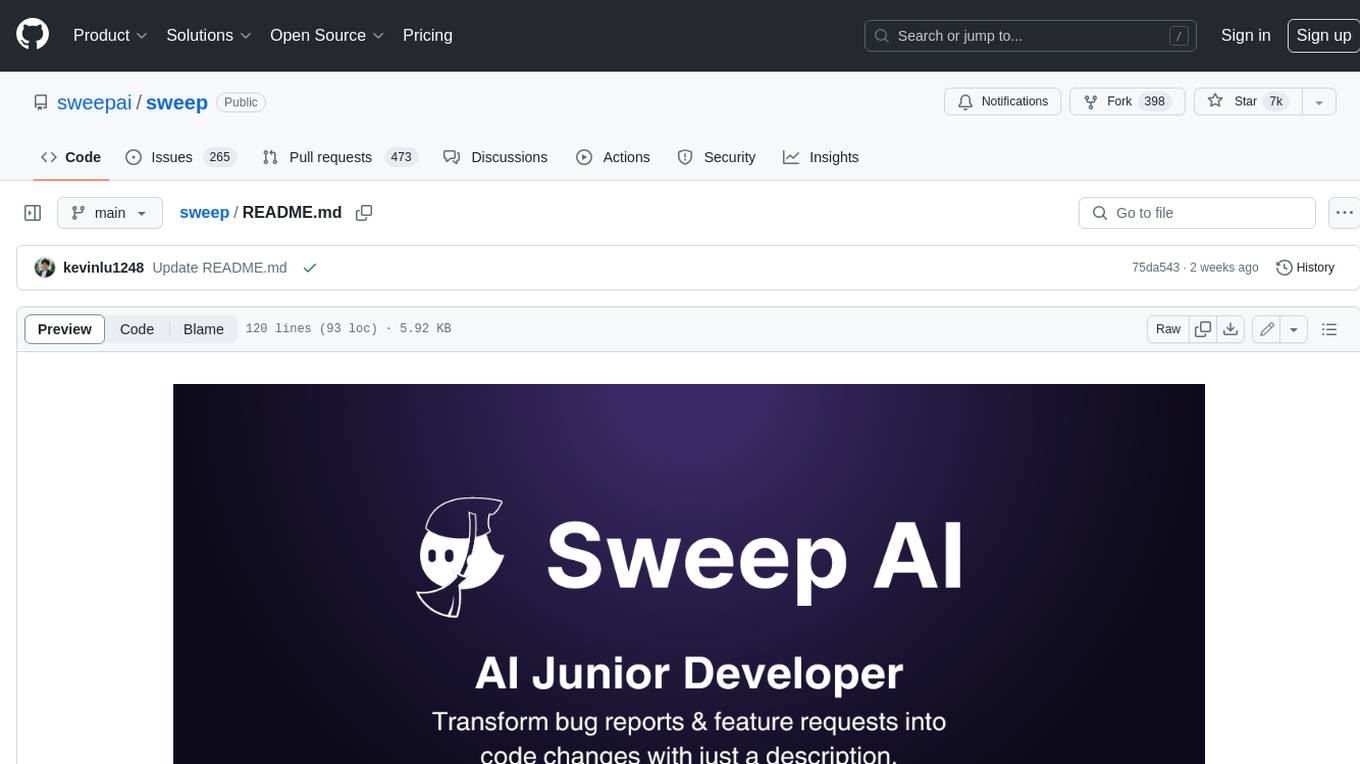
sweep
Sweep is an AI junior developer that turns bugs and feature requests into code changes. It automatically handles developer experience improvements like adding type hints and improving test coverage.
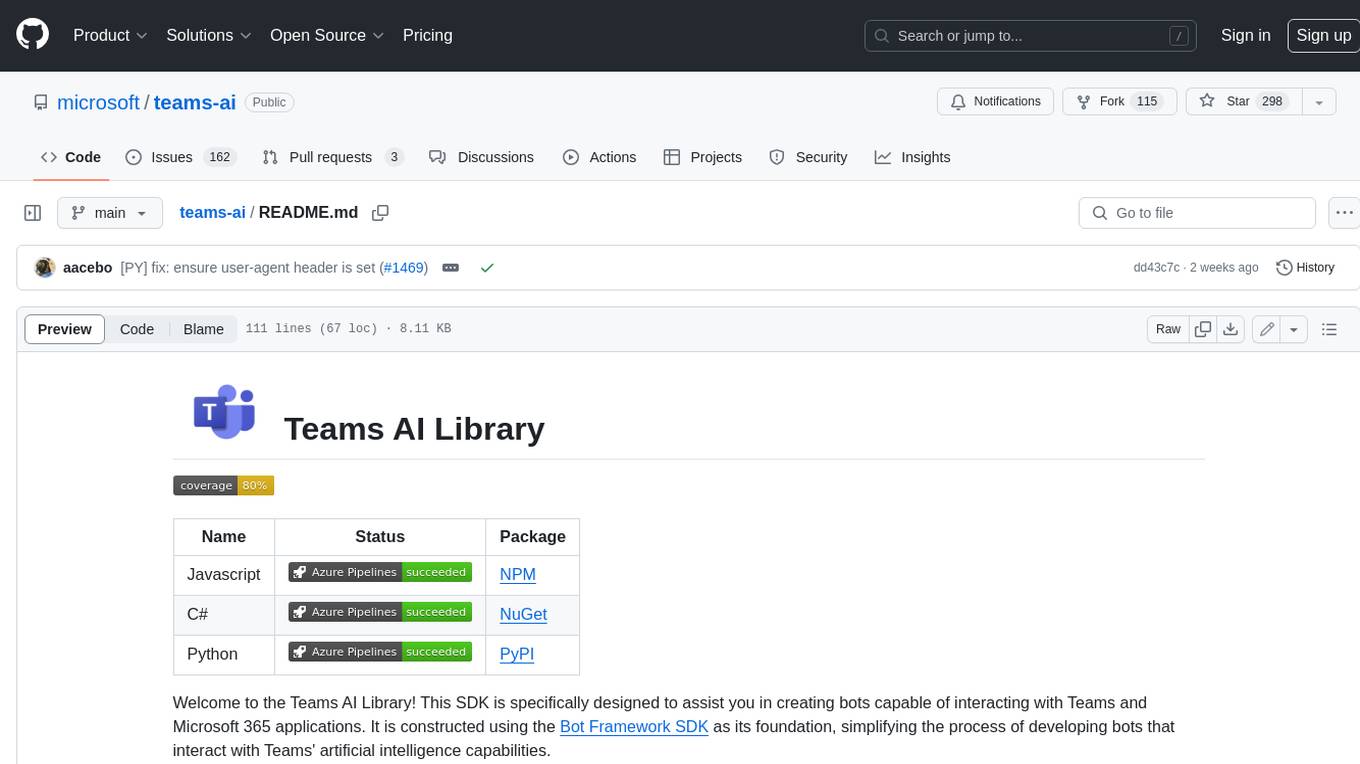
teams-ai
The Teams AI Library is a software development kit (SDK) that helps developers create bots that can interact with Teams and Microsoft 365 applications. It is built on top of the Bot Framework SDK and simplifies the process of developing bots that interact with Teams' artificial intelligence capabilities. The SDK is available for JavaScript/TypeScript, .NET, and Python.
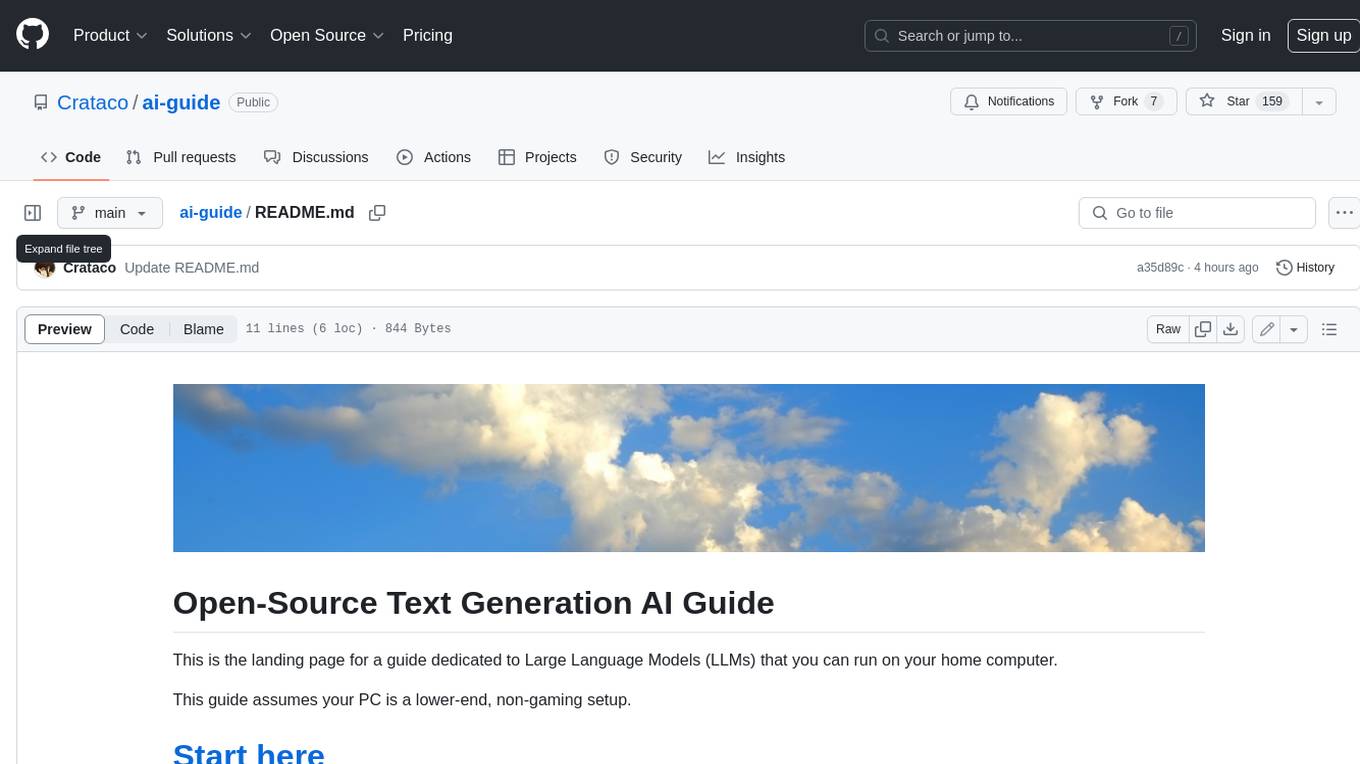
ai-guide
This guide is dedicated to Large Language Models (LLMs) that you can run on your home computer. It assumes your PC is a lower-end, non-gaming setup.
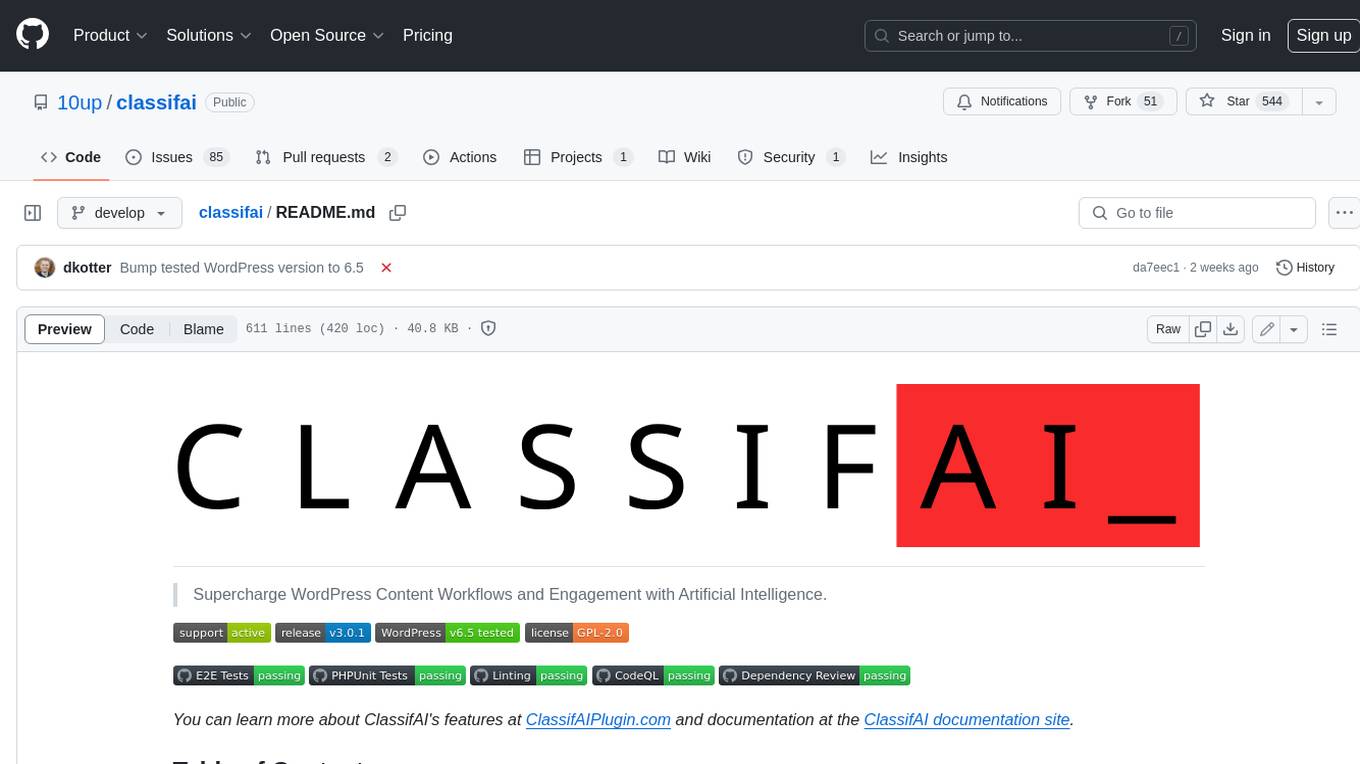
classifai
Supercharge WordPress Content Workflows and Engagement with Artificial Intelligence. Tap into leading cloud-based services like OpenAI, Microsoft Azure AI, Google Gemini and IBM Watson to augment your WordPress-powered websites. Publish content faster while improving SEO performance and increasing audience engagement. ClassifAI integrates Artificial Intelligence and Machine Learning technologies to lighten your workload and eliminate tedious tasks, giving you more time to create original content that matters.
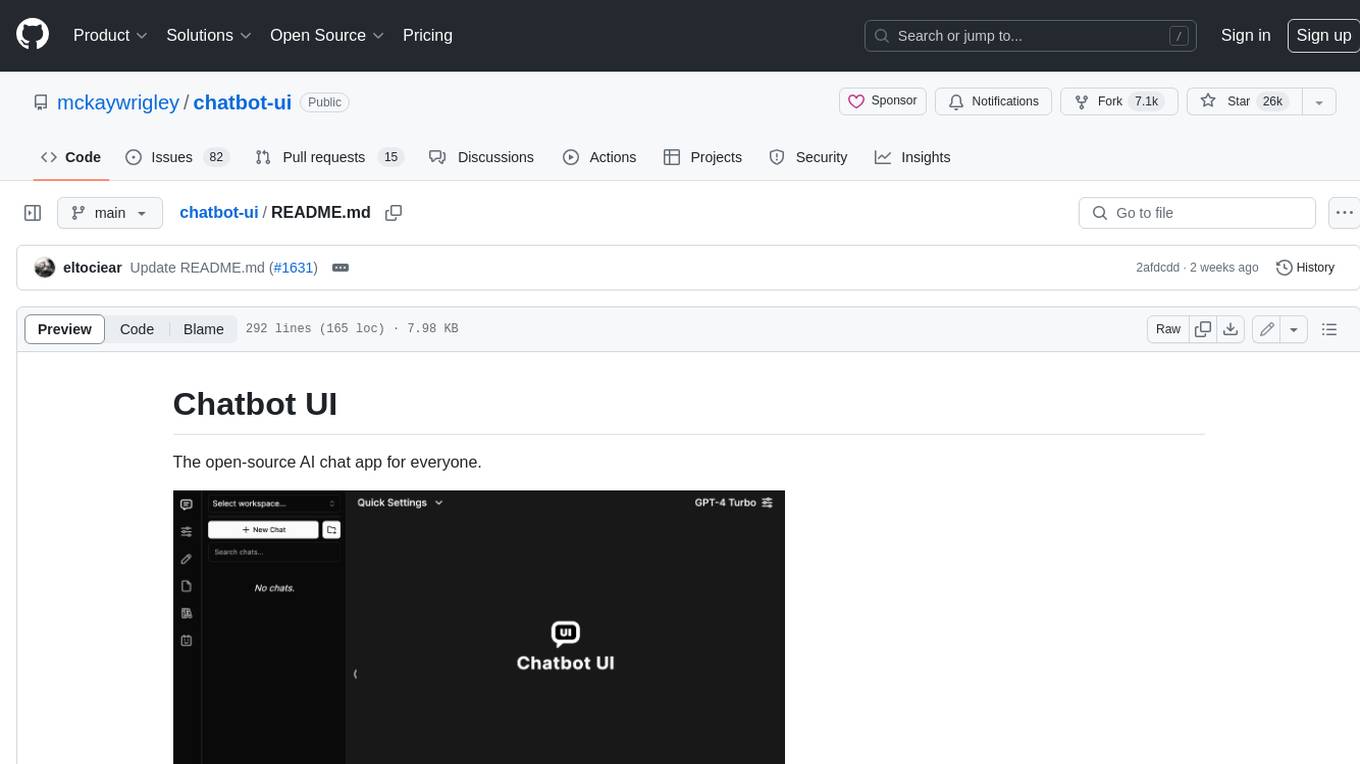
chatbot-ui
Chatbot UI is an open-source AI chat app that allows users to create and deploy their own AI chatbots. It is easy to use and can be customized to fit any need. Chatbot UI is perfect for businesses, developers, and anyone who wants to create a chatbot.
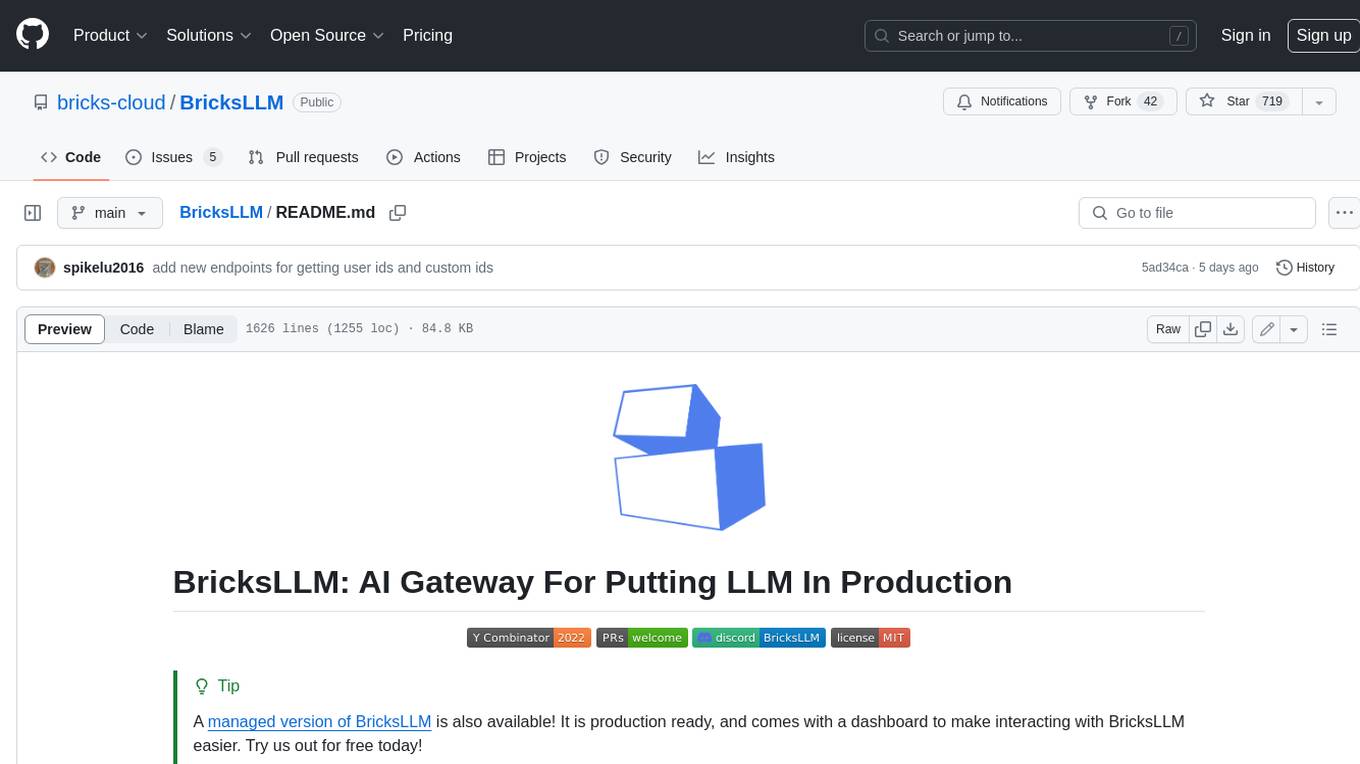
BricksLLM
BricksLLM is a cloud native AI gateway written in Go. Currently, it provides native support for OpenAI, Anthropic, Azure OpenAI and vLLM. BricksLLM aims to provide enterprise level infrastructure that can power any LLM production use cases. Here are some use cases for BricksLLM: * Set LLM usage limits for users on different pricing tiers * Track LLM usage on a per user and per organization basis * Block or redact requests containing PIIs * Improve LLM reliability with failovers, retries and caching * Distribute API keys with rate limits and cost limits for internal development/production use cases * Distribute API keys with rate limits and cost limits for students
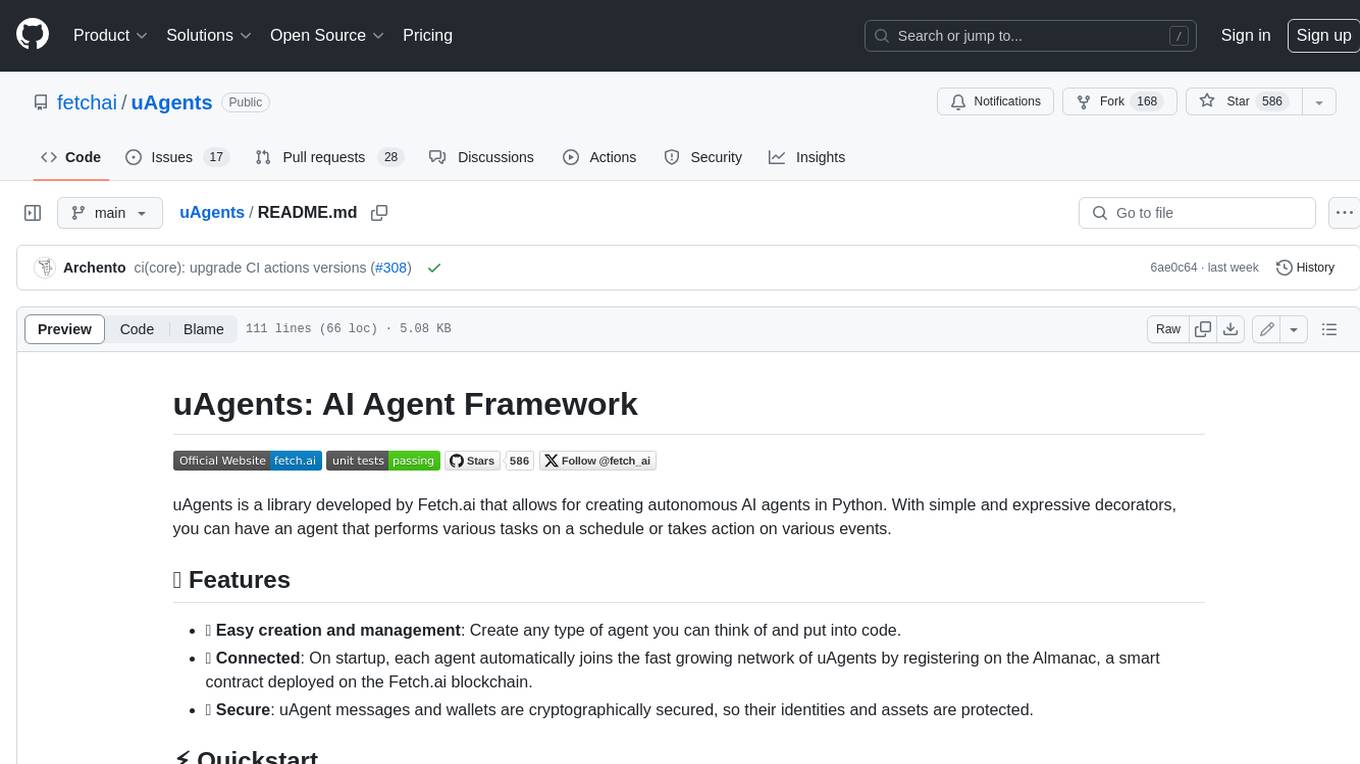
uAgents
uAgents is a Python library developed by Fetch.ai that allows for the creation of autonomous AI agents. These agents can perform various tasks on a schedule or take action on various events. uAgents are easy to create and manage, and they are connected to a fast-growing network of other uAgents. They are also secure, with cryptographically secured messages and wallets.
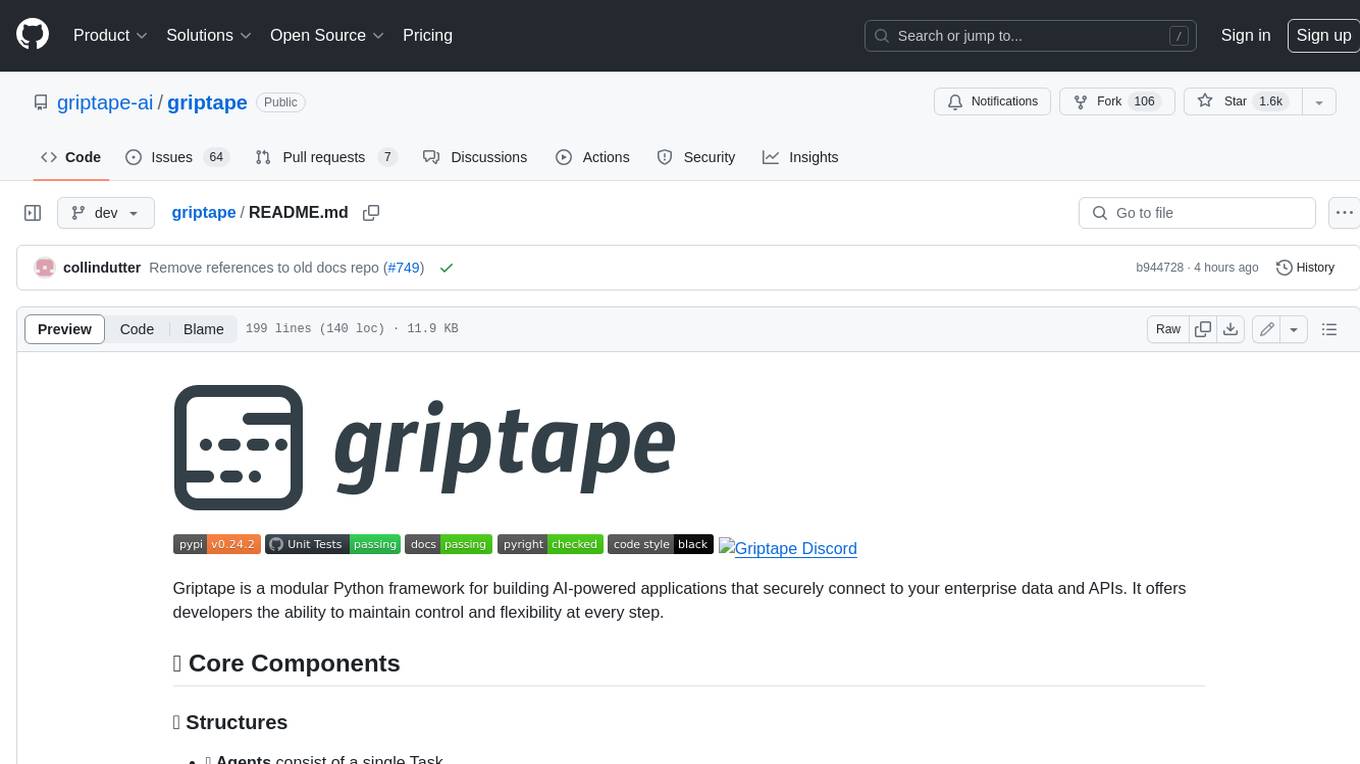
griptape
Griptape is a modular Python framework for building AI-powered applications that securely connect to your enterprise data and APIs. It offers developers the ability to maintain control and flexibility at every step. Griptape's core components include Structures (Agents, Pipelines, and Workflows), Tasks, Tools, Memory (Conversation Memory, Task Memory, and Meta Memory), Drivers (Prompt and Embedding Drivers, Vector Store Drivers, Image Generation Drivers, Image Query Drivers, SQL Drivers, Web Scraper Drivers, and Conversation Memory Drivers), Engines (Query Engines, Extraction Engines, Summary Engines, Image Generation Engines, and Image Query Engines), and additional components (Rulesets, Loaders, Artifacts, Chunkers, and Tokenizers). Griptape enables developers to create AI-powered applications with ease and efficiency.