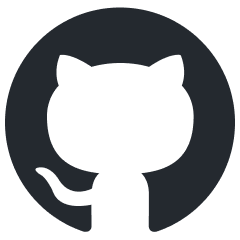
LazyLLM
Easiest and laziest way for building multi-agent LLMs applications.
Stars: 1143
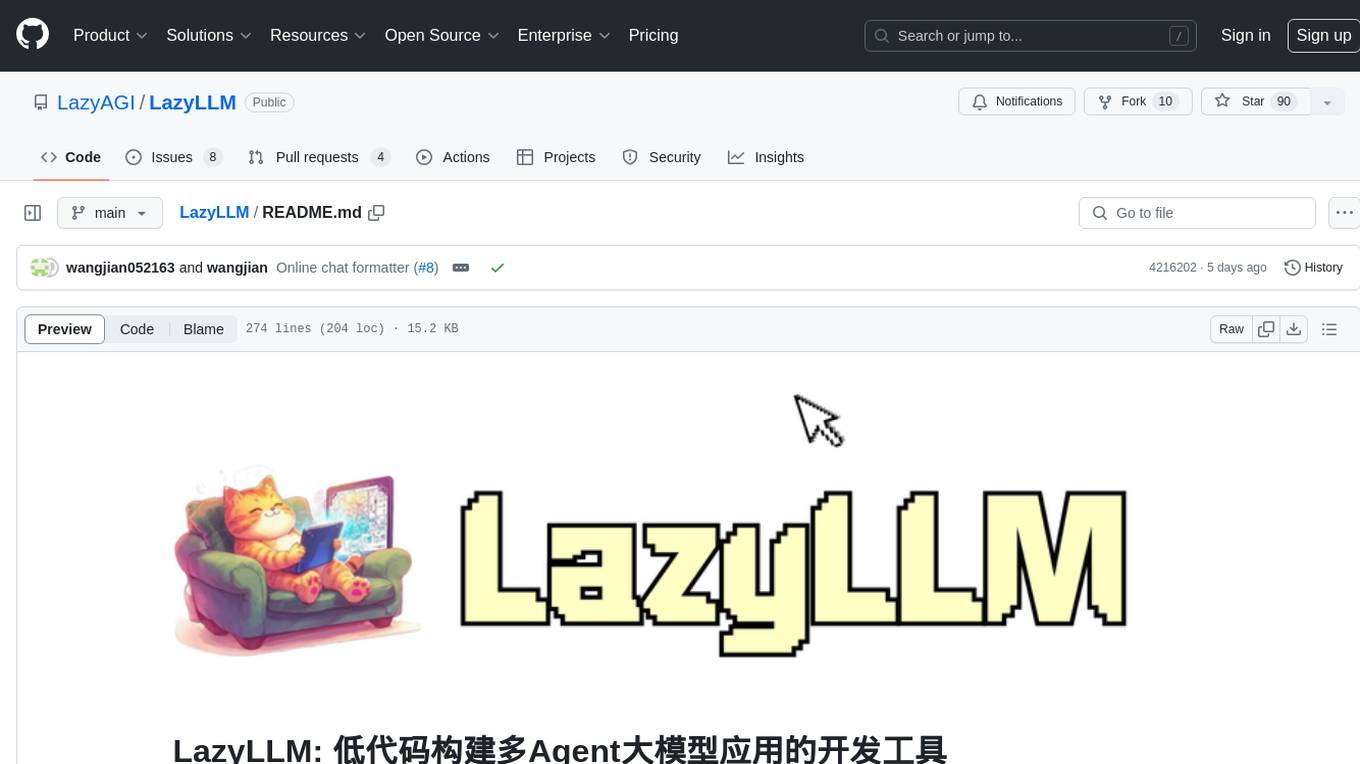
LazyLLM is a low-code development tool for building complex AI applications with multiple agents. It assists developers in building AI applications at a low cost and continuously optimizing their performance. The tool provides a convenient workflow for application development and offers standard processes and tools for various stages of application development. Users can quickly prototype applications with LazyLLM, analyze bad cases with scenario task data, and iteratively optimize key components to enhance the overall application performance. LazyLLM aims to simplify the AI application development process and provide flexibility for both beginners and experts to create high-quality applications.
README:
LazyLLM is a low-code development tool for building multi-agent LLMs(large language models) applications. It assists developers in creating complex AI applications at very low costs and enables continuous iterative optimization. LazyLLM offers a convenient workflow for application building and provides numerous standard processes and tools for various stages of the application development process.
The AI application development process based on LazyLLM follows the prototype building -> data feedback -> iterative optimization workflow. This means you can quickly build a prototype application using LazyLLM, then analyze bad cases using task-specific data, and subsequently iterate on algorithms and fine-tune models at critical stages of the application to gradually enhance the overall performance.
Tutorials: https://docs.lazyllm.ai/
Scan the QR code below with WeChat to join the group chat
Convenient AI Application Assembly Process: Even if you are not familiar with large models, you can still easily assemble AI applications with multiple agents using our built-in data flow and functional modules, just like Lego building.
One-Click Deployment of Complex Applications: We offer the capability to deploy all modules with a single click. Specifically, during the POC (Proof of Concept) phase, LazyLLM simplifies the deployment process of multi-agent applications through a lightweight gateway mechanism, solving the problem of sequentially starting each submodule service (such as LLM, Embedding, etc.) and configuring URLs, making the entire process smoother and more efficient. In the application release phase, LazyLLM provides the ability to package images with one click, making it easy to utilize Kubernetes' gateway, load balancing, and fault tolerance capabilities.
Cross-Platform Compatibility: Switch IaaS platforms with one click without modifying code, compatible with bare-metal servers, development machines, Slurm clusters, public clouds, etc. This allows developed applications to be seamlessly migrated to other IaaS platforms, greatly reducing the workload of code modification.
Support for Grid Search Parameter Optimization: Automatically try different base models, retrieval strategies, and fine-tuning parameters based on user configurations to evaluate and optimize applications. This makes hyperparameter tuning efficient without requiring extensive intrusive modifications to application code, helping users quickly find the best configuration.
Efficient Model Fine-Tuning: Support fine-tuning models within applications to continuously improve application performance. Automatically select the best fine-tuning framework and model splitting strategy based on the fine-tuning scenario. This not only simplifies the maintenance of model iterations but also allows algorithm researchers to focus more on algorithm and data iteration, without handling tedious engineering tasks.
LazyLLM can be used to build common artificial intelligence applications. Here are some examples.
This is a simple example of a chat bot.
# set environment variable: LAZYLLM_OPENAI_API_KEY=xx
# or you can make a config file(~/.lazyllm/config.json) and add openai_api_key=xx
import lazyllm
chat = lazyllm.OnlineChatModule()
lazyllm.WebModule(chat).start().wait()
If you want to use a locally deployed model, please ensure you have installed at least one inference framework (lightllm or vllm), and then use the following code
import lazyllm
# Model will be downloaded automatically if you have an internet connection.
chat = lazyllm.TrainableModule('internlm2-chat-7b')
lazyllm.WebModule(chat, port=23466).start().wait()
If you installed lazyllm
using pip
and ensured that the bin
directory of your Python environment is in your $PATH
, you can quickly start a chatbot by executing lazyllm run chatbot
. If you want to use a local model, you need to specify the model name with the --model
parameter. For example, you can start a chatbot based on a local model by using lazyllm run chatbot --model=internlm2-chat-7b
.
This is an advanced bot example with multimodality and intent recognition.
click to look up prompts and imports
from lazyllm import TrainableModule, WebModule, deploy, pipeline
from lazyllm.tools import IntentClassifier
painter_prompt = 'Now you are a master of drawing prompts, capable of converting any Chinese content entered by the user into English drawing prompts. In this task, you need to convert any input content into English drawing prompts, and you can enrich and expand the prompt content.'
musician_prompt = 'Now you are a master of music composition prompts, capable of converting any Chinese content entered by the user into English music composition prompts. In this task, you need to convert any input content into English music composition prompts, and you can enrich and expand the prompt content.'
base = TrainableModule('internlm2-chat-7b')
with IntentClassifier(base) as ic:
ic.case['Chat', base]
ic.case['Speech Recognition', TrainableModule('SenseVoiceSmall')]
ic.case['Image QA', TrainableModule('Mini-InternVL-Chat-2B-V1-5').deploy_method(deploy.LMDeploy)]
ic.case['Drawing', pipeline(base.share().prompt(painter_prompt), TrainableModule('stable-diffusion-3-medium'))]
ic.case['Generate Music', pipeline(base.share().prompt(musician_prompt), TrainableModule('musicgen-small'))]
ic.case['Text to Speech', TrainableModule('ChatTTS')]
WebModule(ic, history=[base], audio=True, port=8847).start().wait()
click to look up prompts and imports
import os
import lazyllm
from lazyllm import pipeline, parallel, bind, SentenceSplitter, Document, Retriever, Reranker
prompt = 'You will play the role of an AI Q&A assistant and complete a dialogue task. In this task, you need to provide your answer based on the given context and question.'
Here is an online deployment example:
documents = Document(dataset_path="your data path", embed=lazyllm.OnlineEmbeddingModule(), manager=False)
documents.create_node_group(name="sentences", transform=SentenceSplitter, chunk_size=1024, chunk_overlap=100)
with pipeline() as ppl:
with parallel().sum as ppl.prl:
prl.retriever1 = Retriever(documents, group_name="sentences", similarity="cosine", topk=3)
prl.retriever2 = Retriever(documents, "CoarseChunk", "bm25_chinese", 0.003, topk=3)
ppl.reranker = Reranker("ModuleReranker", model="bge-reranker-large", topk=1) | bind(query=ppl.input)
ppl.formatter = (lambda nodes, query: dict(context_str="".join([node.get_content() for node in nodes]), query=query)) | bind(query=ppl.input)
ppl.llm = lazyllm.OnlineChatModule(stream=False).prompt(lazyllm.ChatPrompter(prompt, extro_keys=["context_str"]))
lazyllm.WebModule(ppl, port=23466).start().wait()
Here is an example of a local deployment:
documents = Document(dataset_path='/file/to/yourpath', embed=lazyllm.TrainableModule('bge-large-zh-v1.5'))
documents.create_node_group(name="sentences", transform=SentenceSplitter, chunk_size=1024, chunk_overlap=100)
with pipeline() as ppl:
with parallel().sum as ppl.prl:
prl.retriever1 = Retriever(documents, group_name="sentences", similarity="cosine", topk=3)
prl.retriever2 = Retriever(documents, "CoarseChunk", "bm25_chinese", 0.003, topk=3)
ppl.reranker = Reranker("ModuleReranker", model="bge-reranker-large", topk=1) | bind(query=ppl.input)
ppl.formatter = (lambda nodes, query: dict(context_str="".join([node.get_content() for node in nodes]), query=query)) | bind(query=ppl.input)
ppl.llm = lazyllm.TrainableModule("internlm2-chat-7b").prompt(lazyllm.ChatPrompter(prompt, extro_keys=["context_str"]))
lazyllm.WebModule(ppl, port=23456).start().wait()
https://github.com/LazyAGI/LazyLLM/assets/12124621/77267adc-6e40-47b8-96a8-895df165b0ce
If you installed lazyllm
using pip
and ensured that the bin
directory of your Python environment is in your $PATH
, you can quickly start a retrieval-augmented bot by executing lazyllm run rag --documents=/file/to/yourpath
. If you want to use a local model, you need to specify the model name with the --model
parameter. For example, you can start a retrieval-augmented bot based on a local model by using lazyllm run rag --documents=/file/to/yourpath --model=internlm2-chat-7b
.
click to look up prompts and imports
import lazyllm
from lazyllm import pipeline, warp, bind
from lazyllm.components.formatter import JsonFormatter
toc_prompt="""
You are now an intelligent assistant. Your task is to understand the user's input and convert the outline into a list of nested dictionaries. Each dictionary contains a `title` and a `describe`, where the `title` should clearly indicate the level using Markdown format, and the `describe` is a description and writing guide for that section.
Please generate the corresponding list of nested dictionaries based on the following user input:
Example output:
[
{
"title": "# Level 1 Title",
"describe": "Please provide a detailed description of the content under this title, offering background information and core viewpoints."
},
{
"title": "## Level 2 Title",
"describe": "Please provide a detailed description of the content under this title, giving specific details and examples to support the viewpoints of the Level 1 title."
},
{
"title": "### Level 3 Title",
"describe": "Please provide a detailed description of the content under this title, deeply analyzing and providing more details and data support."
}
]
User input is as follows:
"""
completion_prompt="""
You are now an intelligent assistant. Your task is to receive a dictionary containing `title` and `describe`, and expand the writing according to the guidance in `describe`.
Input example:
{
"title": "# Level 1 Title",
"describe": "This is the description for writing."
}
Output:
This is the expanded content for writing.
Receive as follows:
"""
writer_prompt = {"system": completion_prompt, "user": '{"title": {title}, "describe": {describe}}'}
Here is an online deployment example:
with pipeline() as ppl:
ppl.outline_writer = lazyllm.OnlineChatModule(stream=False).formatter(JsonFormatter()).prompt(toc_prompt)
ppl.story_generater = warp(lazyllm.OnlineChatModule(stream=False).prompt(writer_prompt))
ppl.synthesizer = (lambda *storys, outlines: "\n".join([f"{o['title']}\n{s}" for s, o in zip(storys, outlines)])) | bind(outlines=ppl.output('outline_writer'))
lazyllm.WebModule(ppl, port=23466).start().wait()
Here is an example of a local deployment:
with pipeline() as ppl:
ppl.outline_writer = lazyllm.TrainableModule('internlm2-chat-7b').formatter(JsonFormatter()).prompt(toc_prompt)
ppl.story_generater = warp(ppl.outline_writer.share(prompt=writer_prompt).formatter())
ppl.synthesizer = (lambda *storys, outlines: "\n".join([f"{o['title']}\n{s}" for s, o in zip(storys, outlines)])) | bind(outlines=ppl.output('outline_writer'))
lazyllm.WebModule(ppl, port=23466).start().wait()
click to look up prompts and imports
import lazyllm
from lazyllm import pipeline
prompt = 'You are a drawing prompt word master who can convert any Chinese content entered by the user into English drawing prompt words. In this task, you need to convert any input content into English drawing prompt words, and you can enrich and expand the prompt word content.'
with pipeline() as ppl:
ppl.llm = lazyllm.TrainableModule('internlm2-chat-7b').prompt(lazyllm.ChatPrompter(prompt))
ppl.sd3 = lazyllm.TrainableModule('stable-diffusion-3-medium')
lazyllm.WebModule(ppl, port=23466).start().wait()
- Application Building: Defines workflows such as pipeline, parallel, diverter, if, switch, and loop. Developers can quickly build multi-agent AI applications based on any functions and modules. Supports one-click deployment for assembled multi-agent applications, and also supports partial or complete updates to the applications.
- Platform-independent: Consistent user experience across different computing platforms. Currently compatible with various platforms such as bare metal, Slurm, SenseCore, etc.
-
Supports fine-tuning and inference for large models:
- Offline (local) model services:
- Supports fine-tuning frameworks: collie, peft
- Supports inference frameworks: lightllm, vllm
- Supports automatically selecting the most suitable framework and model parameters (such as micro-bs, tp, zero, etc.) based on user scenarios..
- Online services:
- Supports fine-tuning services: GPT, SenseNova, Tongyi Qianwen
- Supports inference services: GPT, SenseNova, Kimi, Zhipu, Tongyi Qianwen
- Supports embedding inference services: OpenAI, SenseNova, GLM, Tongyi Qianwen
- Support developers to use local services and online services uniformly.
- Offline (local) model services:
- Supports common RAG (Retrieval-Augmented Generation) components: Document, Parser, Retriever, Reranker, etc.
- Supports basic webs: such as chat interface and document management interface, etc.
git clone [email protected]:LazyAGI/LazyLLM.git
cd LazyLLM
pip install -r requirements.txt
pip install -r requirements.full.txt
is used when you want to finetune, deploy or build your rag application.
Only install lazyllm and necessary dependencies, you can use:
pip3 install lazyllm
Install lazyllm and all dependencies, you can use:
pip3 install lazyllm
lazyllm install full
The design philosophy of LazyLLM stems from a deep understanding of the current limitations of large models in production environments. We recognize that at this stage, large models cannot yet fully solve all practical problems end-to-end. Therefore, the AI application development process based on LazyLLM emphasizes "rapid prototyping, bad-case analysis using scenario-specific data, algorithmic experimentation, and model fine-tuning on key aspects to improve the overall application performance." LazyLLM handles the tedious engineering work involved in this process, offering convenient interfaces that allow users to focus on enhancing algorithmic effectiveness and creating outstanding AI applications.
The goal of LazyLLM is to free algorithm researchers and developers from the complexities of engineering implementations, allowing them to concentrate on what they do best: algorithms and data, and solving real-world problems. Whether you are a beginner or an experienced expert, We hope LazyLLM can provide you with some assistance. For novice developers, LazyLLM thoroughly simplifies the AI application development process. They no longer need to worry about how to schedule tasks on different IaaS platforms, understand the details of API service construction, choose frameworks or split models during fine-tuning, or master any web development knowledge. With pre-built components and simple integration operations, novice developers can easily create tools with production value. For seasoned experts, LazyLLM offers a high degree of flexibility. Each module supports customization and extension, enabling users to seamlessly integrate their own algorithms and state-of-the-art production tools to build more powerful applications.
To prevent you from being bogged down by the implementation details of dependent auxiliary tools, LazyLLM strives to ensure a consistent user experience across similar modules. For instance, we have established a set of Prompt rules that provide a uniform usage method for both online models (such as ChatGPT, SenseNova, Kimi, ChatGLM, etc.) and local models. This consistency allows you to easily and flexibly switch between local and online models in your applications.
Unlike most frameworks on the market, LazyLLM carefully selects and integrates 2-3 tools that we believe are the most advantageous at each stage. This not only simplifies the user’s decision-making process but also ensures that users can build the most productive applications at the lowest cost. We do not pursue the quantity of tools or models, but focus on quality and practical effectiveness, committed to providing the optimal solutions. LazyLLM aims to provide a quick, efficient, and low-threshold path for AI application development, freeing developers' creativity, and promoting the adoption and popularization of AI technology in real-world production.
Finally, LazyLLM is a user-centric tool. If you have any ideas or feedback, feel free to leave us a message. We will do our best to address your concerns and ensure that LazyLLM provides you with the convenience you need.
A Component is the smallest execution unit in LazyLLM; it can be either a function or a bash command. Components have three typical capabilities:
- Cross-platform execution using a launcher, allowing seamless user experience:
- EmptyLauncher: Runs locally, supporting development machines, bare metal, etc.
- RemoteLauncher: Schedules execution on compute nodes, supporting Slurm, SenseCore, etc.
- Implements a registration mechanism for grouping and quickly locating methods. Supports registration of functions and bash commands. Here is an example:
import lazyllm
lazyllm.component_register.new_group('demo')
@lazyllm.component_register('demo')
def test(input):
return f'input is {input}'
@lazyllm.component_register.cmd('demo')
def test_cmd(input):
return f'echo input is {input}'
# >>> lazyllm.demo.test()(1)
# 'input is 1'
# >>> lazyllm.demo.test_cmd(launcher=launchers.slurm)(2)
# Command: srun -p pat_rd -N 1 --job-name=xf488db3 -n1 bash -c 'echo input is 2'
Modules are the top-level components in LazyLLM, equipped with four key capabilities: training, deployment, inference, and evaluation. Each module can choose to implement some or all of these capabilities, and each capability can be composed of one or more components. As shown in the table below, we have built-in some basic modules for everyone to use.
Function | Training/Fine-tuning | Deployment | Inference | Evaluation | |
---|---|---|---|---|---|
ActionModule | Can wrap functions, modules, flows, etc., into a Module | Supports training/fine-tuning of its Submodules through ActionModule | Supports deployment of its Submodules through ActionModule | ✅ | ✅ |
UrlModule | Wraps any URL into a Module to access external services | ❌ | ❌ | ✅ | ✅ |
ServerModule | Wraps any function, flow, or Module into an API service | ❌ | ✅ | ✅ | ✅ |
TrainableModule | Trainable Module, all supported models are TrainableModules | ✅ | ✅ | ✅ | ✅ |
WebModule | Launches a multi-round dialogue interface service | ❌ | ✅ | ❌ | ✅ |
OnlineChatModule | Integrates online model fine-tuning and inference services | ✅ | ✅ | ✅ | ✅ |
OnlineEmbeddingModule | Integrates online Embedding model inference services | ❌ | ✅ | ✅ | ✅ |
Flow in LazyLLM defines the data stream, describing how data is passed from one callable object to another. You can use Flow to intuitively and efficiently organize and manage data flow. Based on various predefined Flows, we can easily build and manage complex applications using Modules, Components, Flows, or any callable objects. The Flows currently implemented in LazyLLM include Pipeline, Parallel, Diverter, Warp, IFS, Loop, etc., which can cover almost all application scenarios. Building applications with Flow offers the following advantages:
- You can easily combine, add, and replace various modules and components; the design of Flow makes adding new features simple and facilitates collaboration between different modules and even projects.
- Through a standardized interface and data flow mechanism, Flow reduces the repetitive work developers face when handling data transfer and transformation. Developers can focus more on core business logic, thus improving overall development efficiency.
- Some Flows support asynchronous processing and parallel execution, significantly enhancing response speed and system performance when dealing with large-scale data or complex tasks.
For Tasks:
Click tags to check more tools for each tasksFor Jobs:
Alternative AI tools for LazyLLM
Similar Open Source Tools
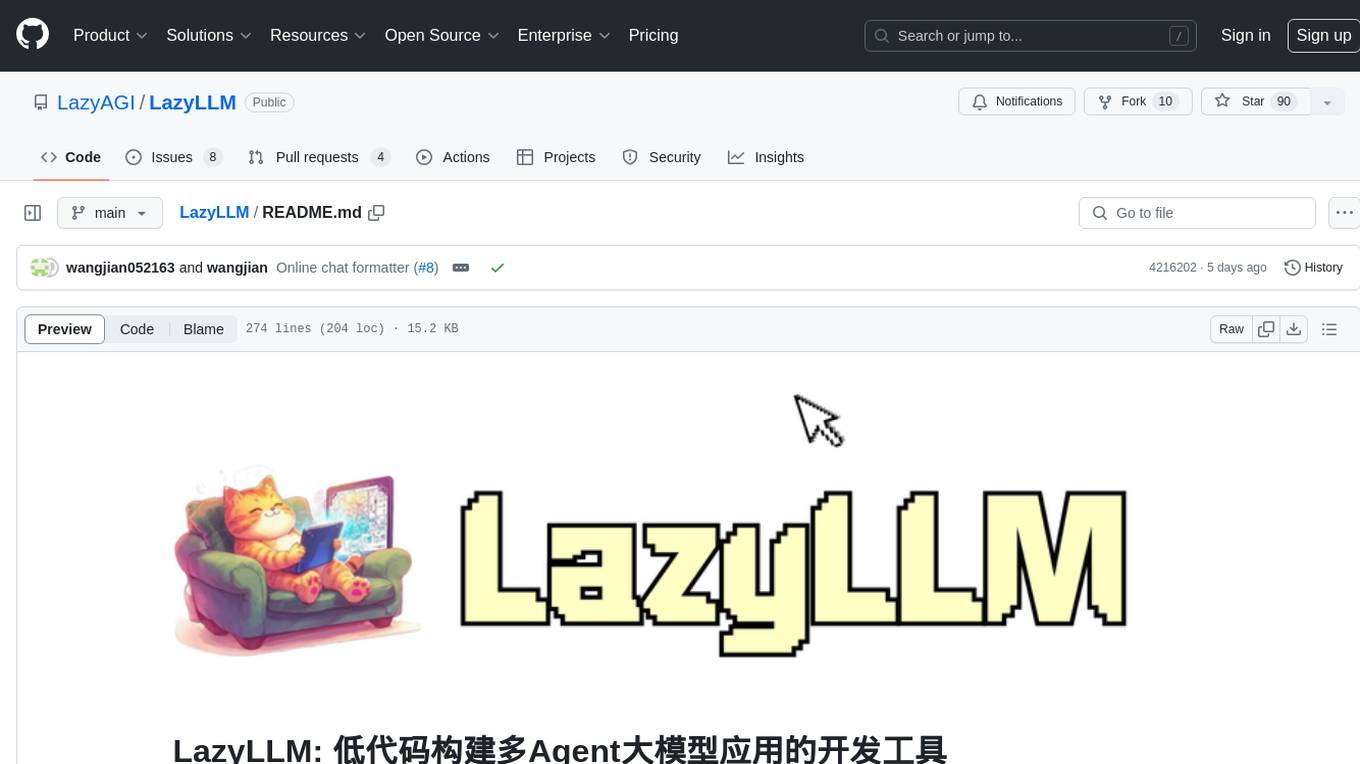
LazyLLM
LazyLLM is a low-code development tool for building complex AI applications with multiple agents. It assists developers in building AI applications at a low cost and continuously optimizing their performance. The tool provides a convenient workflow for application development and offers standard processes and tools for various stages of application development. Users can quickly prototype applications with LazyLLM, analyze bad cases with scenario task data, and iteratively optimize key components to enhance the overall application performance. LazyLLM aims to simplify the AI application development process and provide flexibility for both beginners and experts to create high-quality applications.
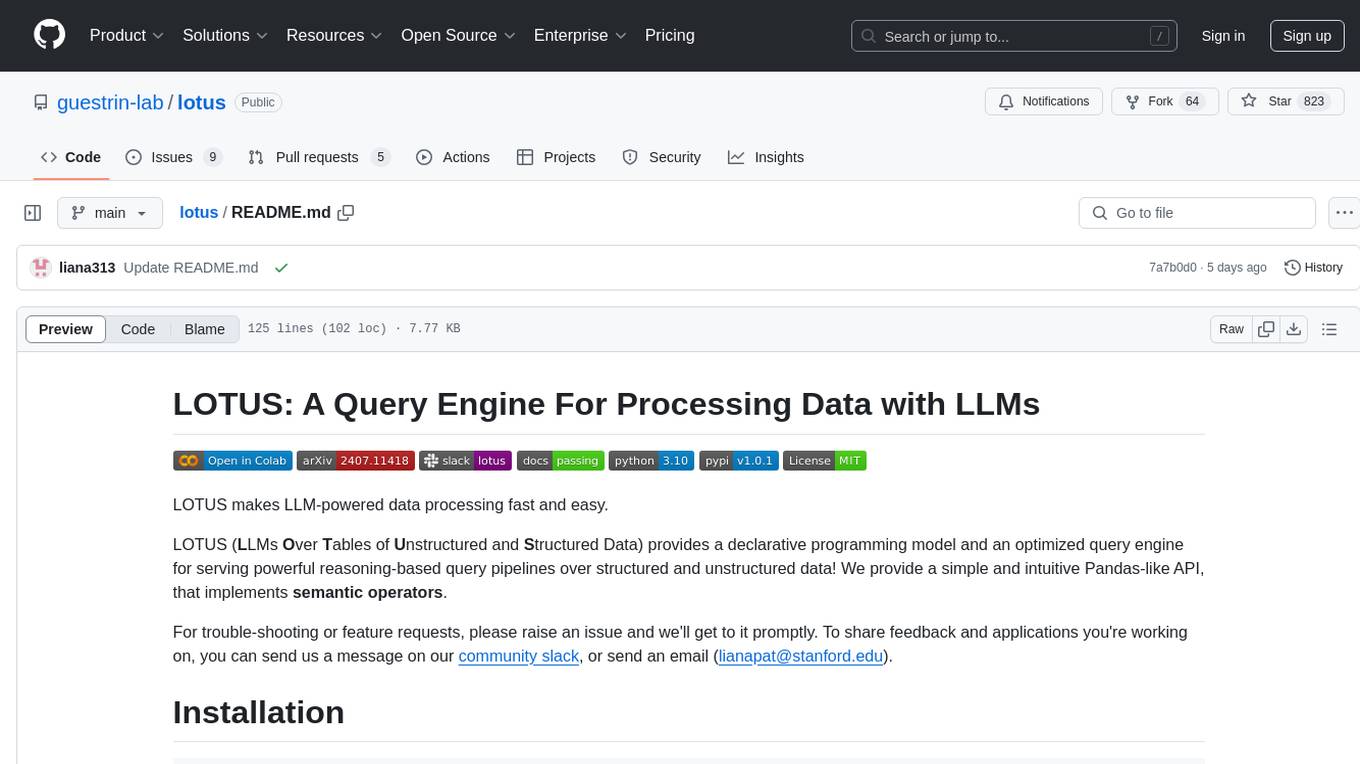
lotus
LOTUS (LLMs Over Tables of Unstructured and Structured Data) is a query engine that provides a declarative programming model and an optimized query engine for reasoning-based query pipelines over structured and unstructured data. It offers a simple and intuitive Pandas-like API with semantic operators for fast and easy LLM-powered data processing. The tool implements a semantic operator programming model, allowing users to write AI-based pipelines with high-level logic and leaving the rest of the work to the query engine. LOTUS supports various semantic operators like sem_map, sem_filter, sem_extract, sem_agg, sem_topk, sem_join, sem_sim_join, and sem_search, enabling users to perform tasks like mapping records, filtering data, aggregating records, and more. The tool also supports different model classes such as LM, RM, and Reranker for language modeling, retrieval, and reranking tasks respectively.
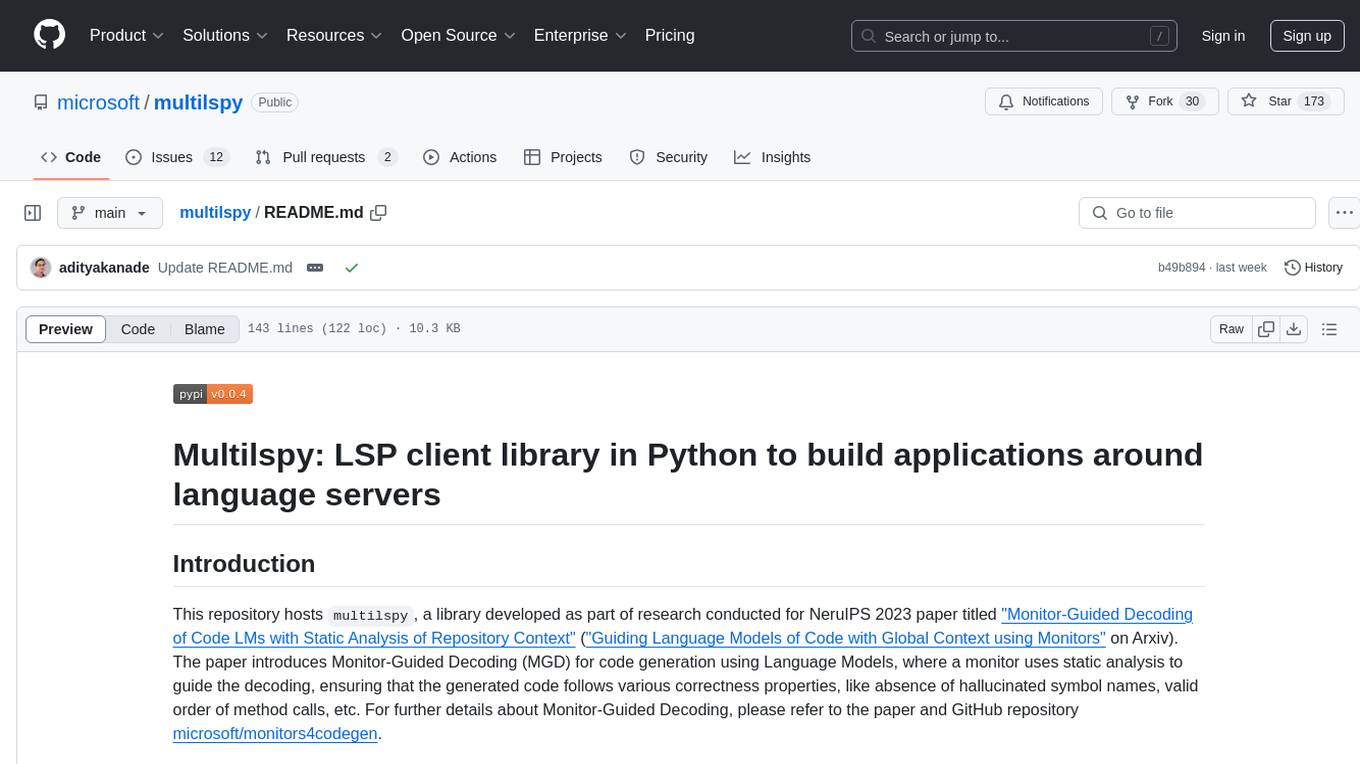
multilspy
Multilspy is a Python library developed for research purposes to facilitate the creation of language server clients for querying and obtaining results of static analyses from various language servers. It simplifies the process by handling server setup, communication, and configuration parameters, providing a common interface for different languages. The library supports features like finding function/class definitions, callers, completions, hover information, and document symbols. It is designed to work with AI systems like Large Language Models (LLMs) for tasks such as Monitor-Guided Decoding to ensure code generation correctness and boost compilability.
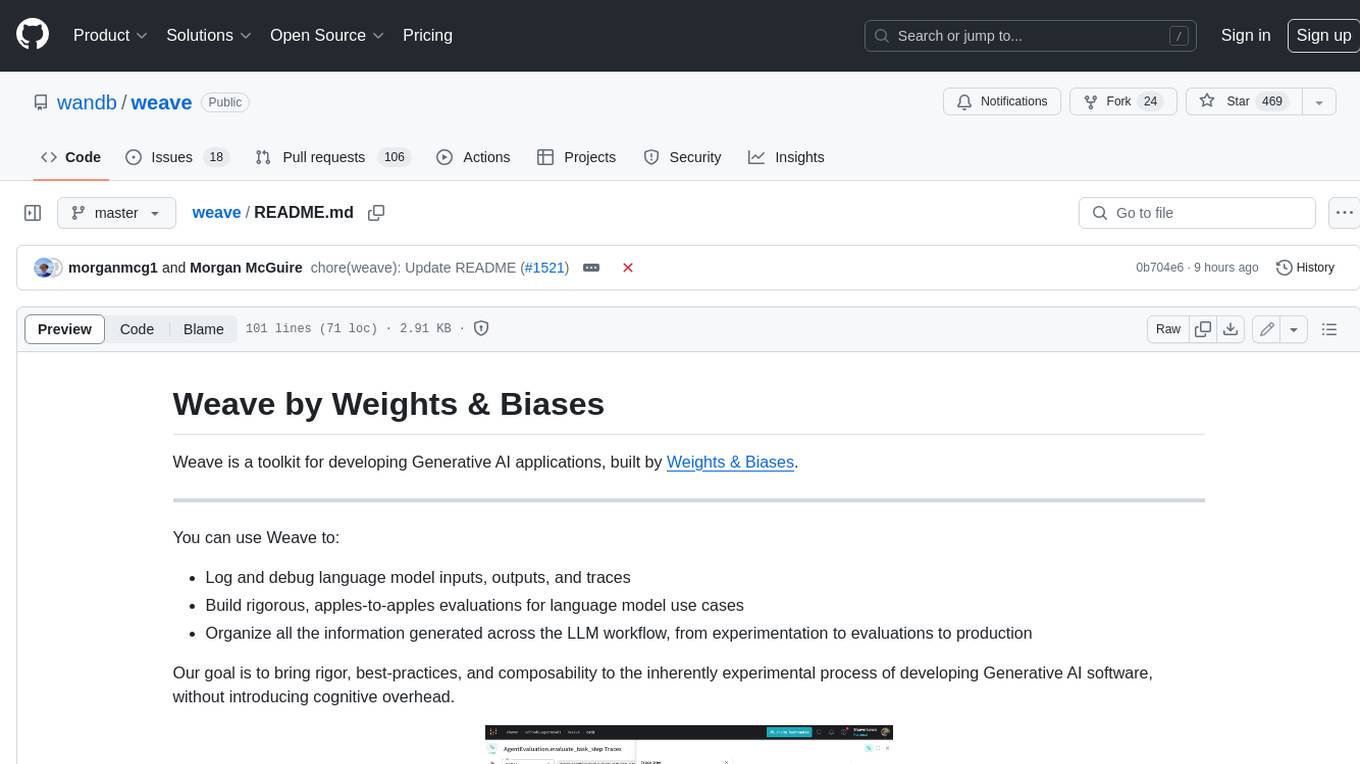
weave
Weave is a toolkit for developing Generative AI applications, built by Weights & Biases. With Weave, you can log and debug language model inputs, outputs, and traces; build rigorous, apples-to-apples evaluations for language model use cases; and organize all the information generated across the LLM workflow, from experimentation to evaluations to production. Weave aims to bring rigor, best-practices, and composability to the inherently experimental process of developing Generative AI software, without introducing cognitive overhead.
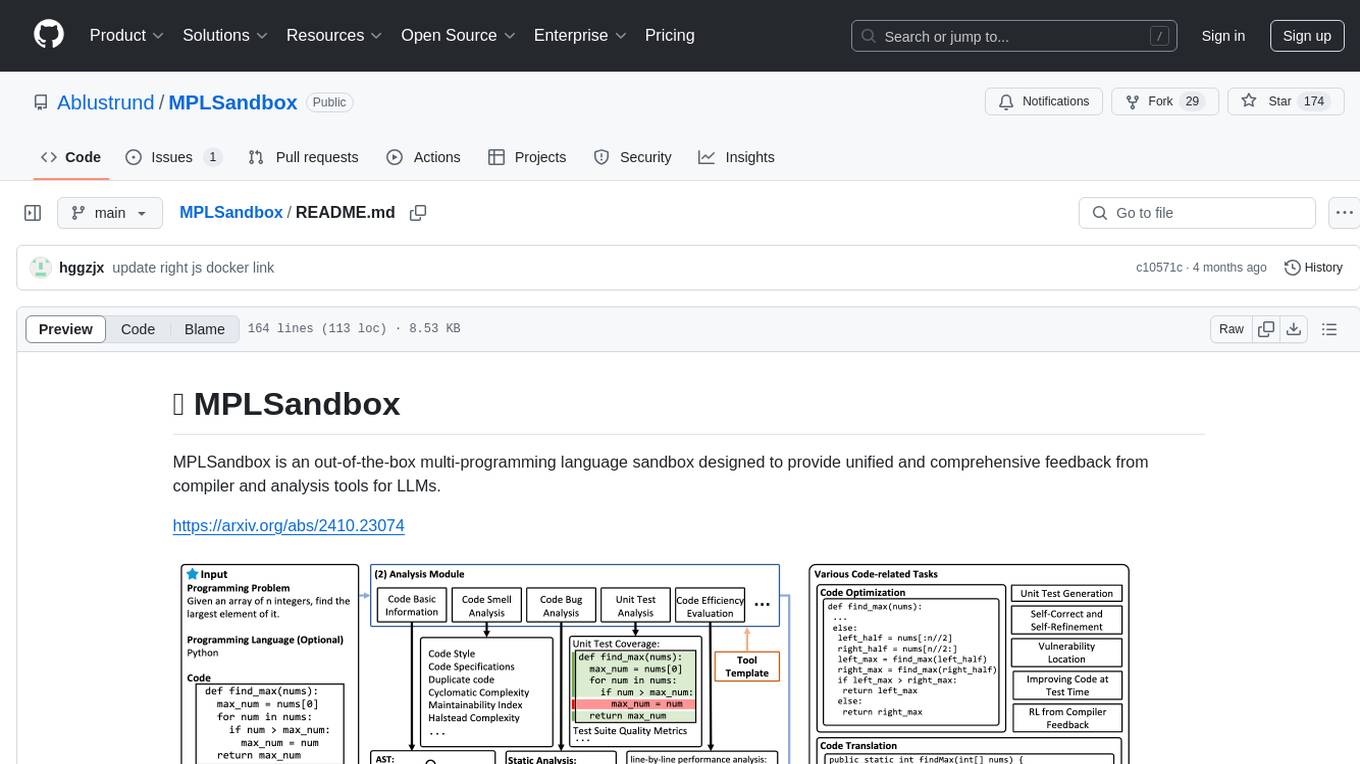
MPLSandbox
MPLSandbox is an out-of-the-box multi-programming language sandbox designed to provide unified and comprehensive feedback from compiler and analysis tools for LLMs. It simplifies code analysis for researchers and can be seamlessly integrated into LLM training and application processes to enhance performance in a range of code-related tasks. The sandbox environment ensures safe code execution, the code analysis module offers comprehensive analysis reports, and the information integration module combines compilation feedback and analysis results for complex code-related tasks.
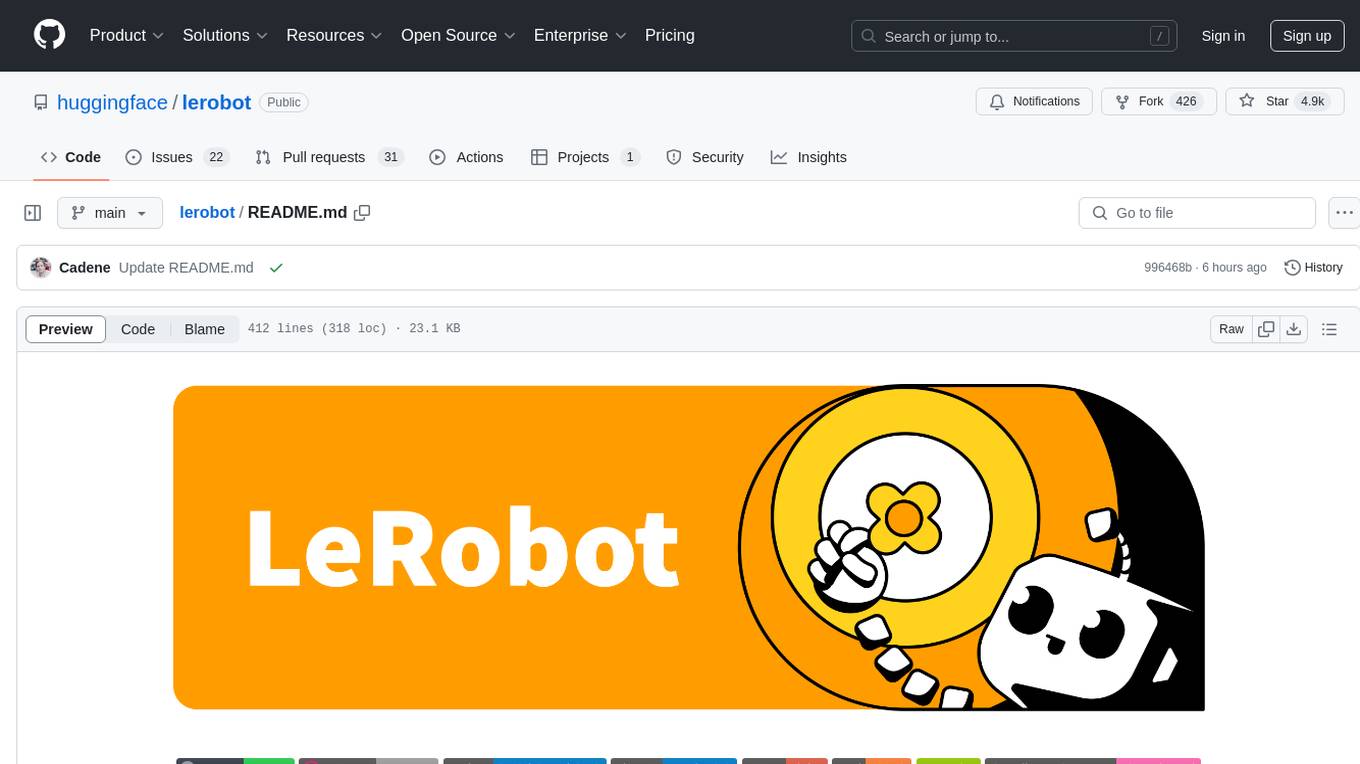
lerobot
LeRobot is a state-of-the-art AI library for real-world robotics in PyTorch. It aims to provide models, datasets, and tools to lower the barrier to entry to robotics, focusing on imitation learning and reinforcement learning. LeRobot offers pretrained models, datasets with human-collected demonstrations, and simulation environments. It plans to support real-world robotics on affordable and capable robots. The library hosts pretrained models and datasets on the Hugging Face community page.
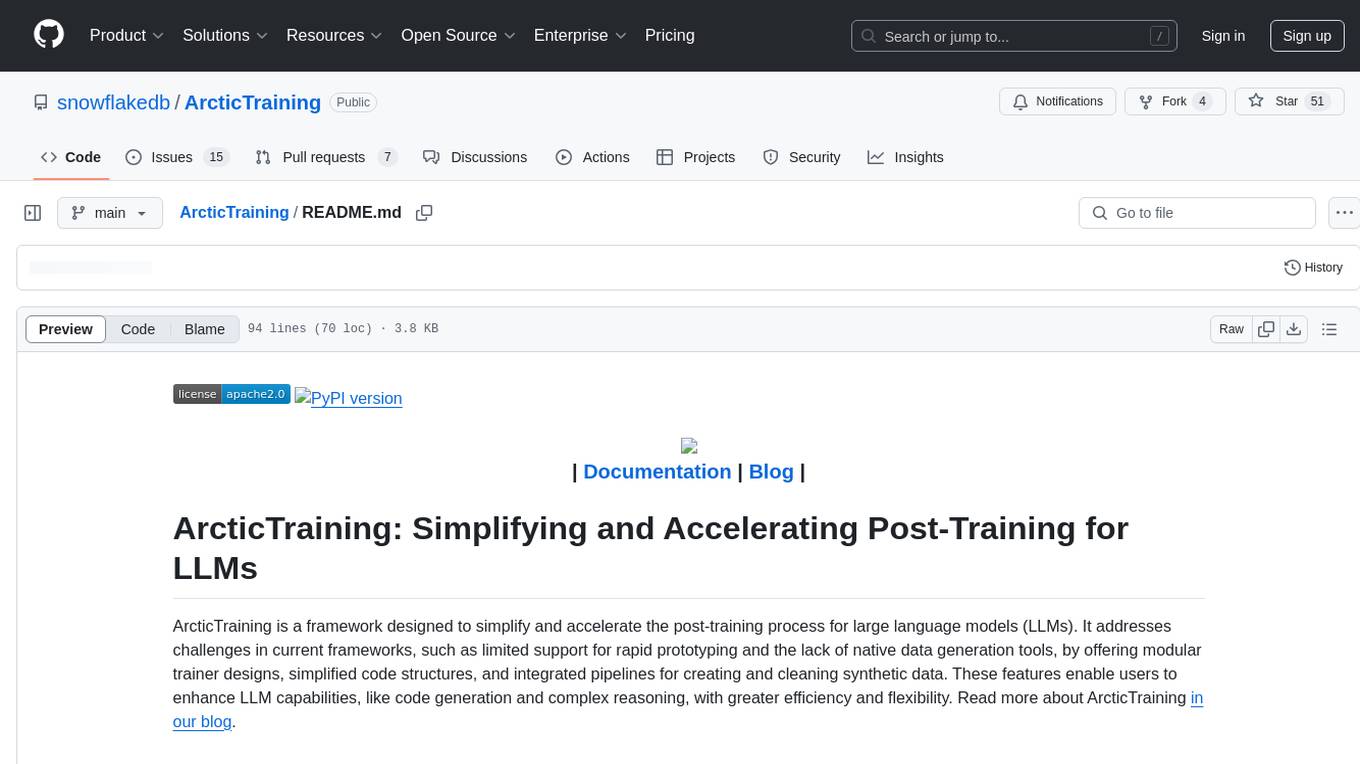
ArcticTraining
ArcticTraining is a framework designed to simplify and accelerate the post-training process for large language models (LLMs). It offers modular trainer designs, simplified code structures, and integrated pipelines for creating and cleaning synthetic data, enabling users to enhance LLM capabilities like code generation and complex reasoning with greater efficiency and flexibility.
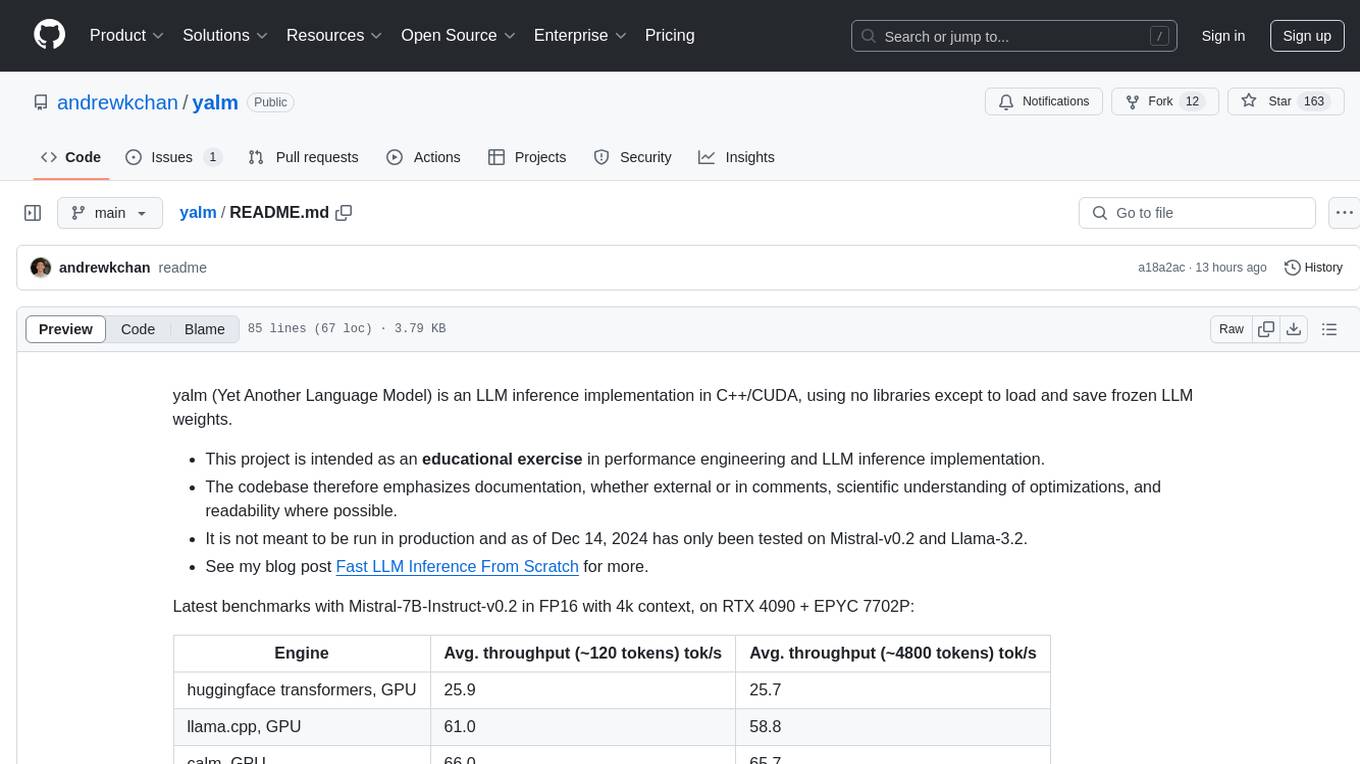
yalm
Yalm (Yet Another Language Model) is an LLM inference implementation in C++/CUDA, emphasizing performance engineering, documentation, scientific optimizations, and readability. It is not for production use and has been tested on Mistral-v0.2 and Llama-3.2. Requires C++20-compatible compiler, CUDA toolkit, and LLM safetensor weights in huggingface format converted to .yalm file.
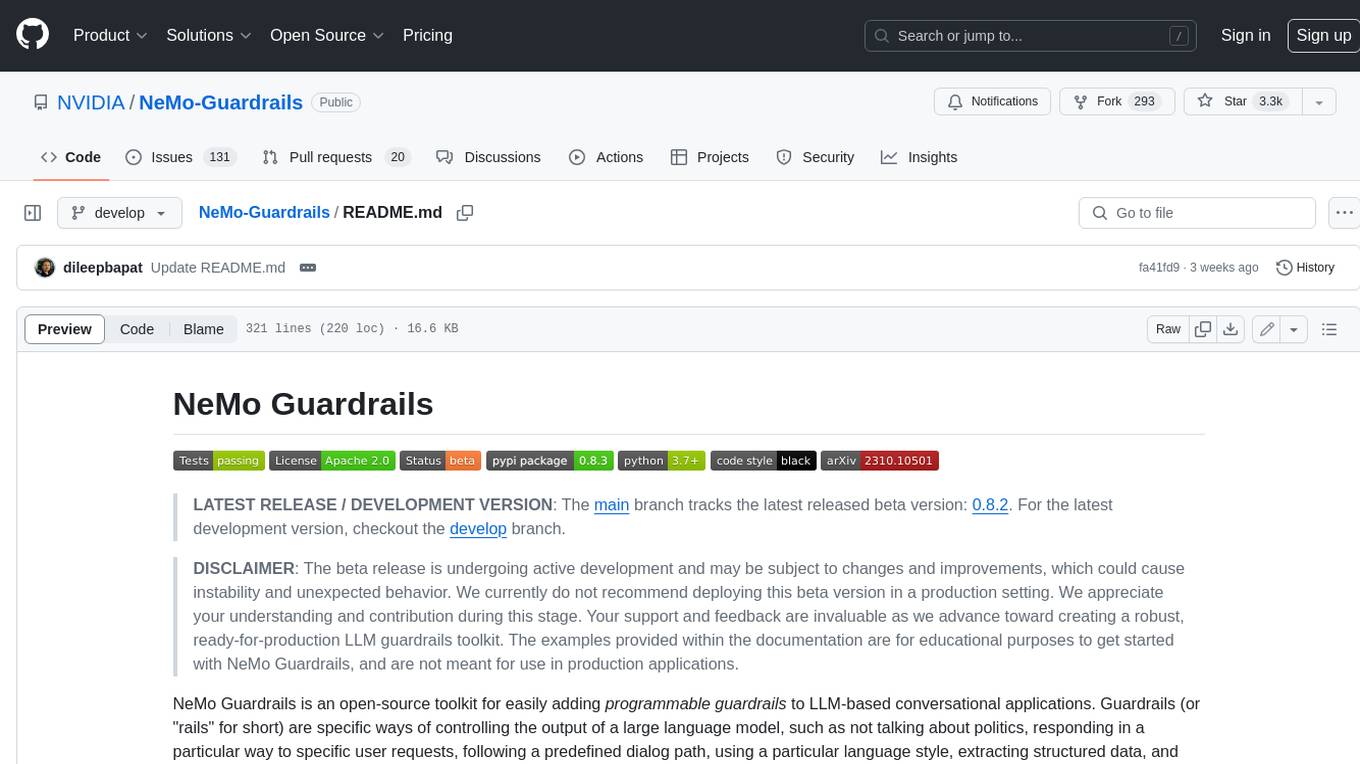
NeMo-Guardrails
NeMo Guardrails is an open-source toolkit for easily adding _programmable guardrails_ to LLM-based conversational applications. Guardrails (or "rails" for short) are specific ways of controlling the output of a large language model, such as not talking about politics, responding in a particular way to specific user requests, following a predefined dialog path, using a particular language style, extracting structured data, and more.
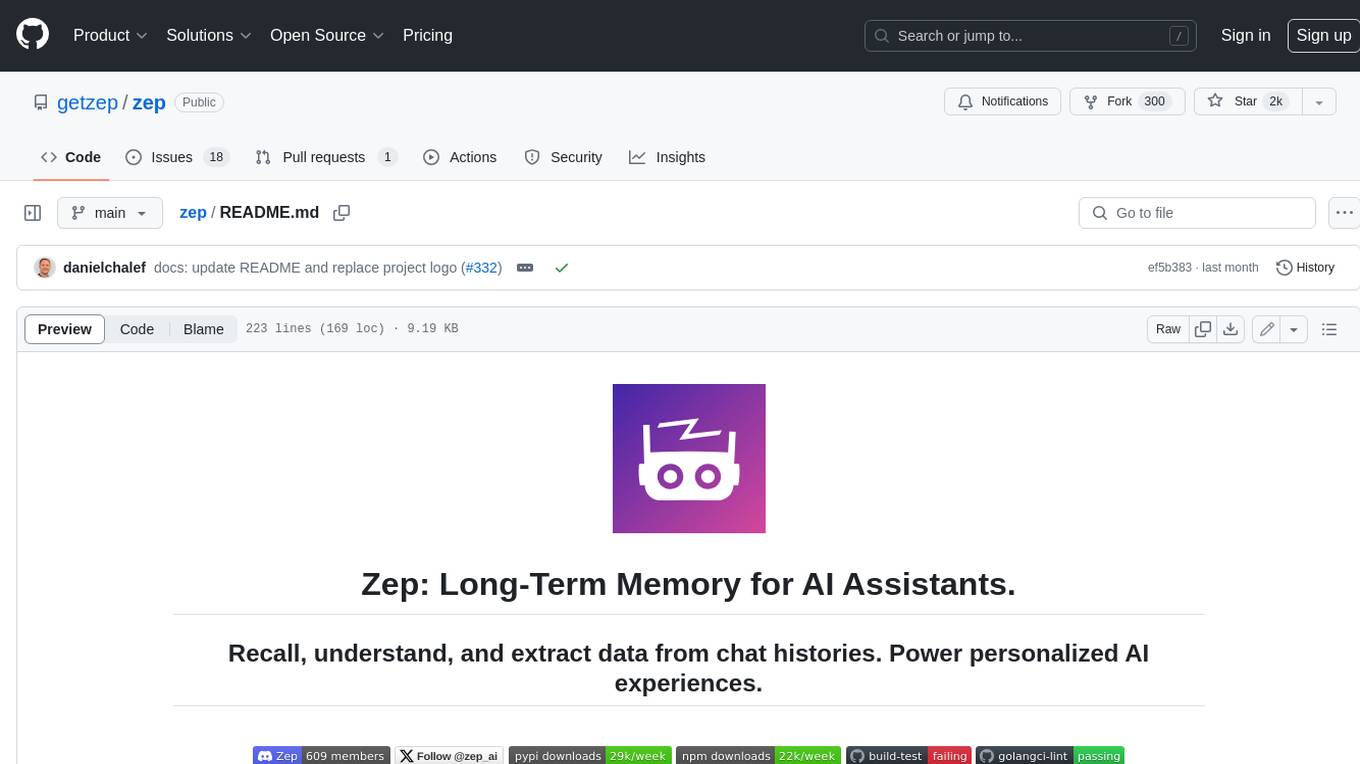
zep
Zep is a long-term memory service for AI Assistant apps. With Zep, you can provide AI assistants with the ability to recall past conversations, no matter how distant, while also reducing hallucinations, latency, and cost. Zep persists and recalls chat histories, and automatically generates summaries and other artifacts from these chat histories. It also embeds messages and summaries, enabling you to search Zep for relevant context from past conversations. Zep does all of this asyncronously, ensuring these operations don't impact your user's chat experience. Data is persisted to database, allowing you to scale out when growth demands. Zep also provides a simple, easy to use abstraction for document vector search called Document Collections. This is designed to complement Zep's core memory features, but is not designed to be a general purpose vector database. Zep allows you to be more intentional about constructing your prompt: 1. automatically adding a few recent messages, with the number customized for your app; 2. a summary of recent conversations prior to the messages above; 3. and/or contextually relevant summaries or messages surfaced from the entire chat session. 4. and/or relevant Business data from Zep Document Collections.
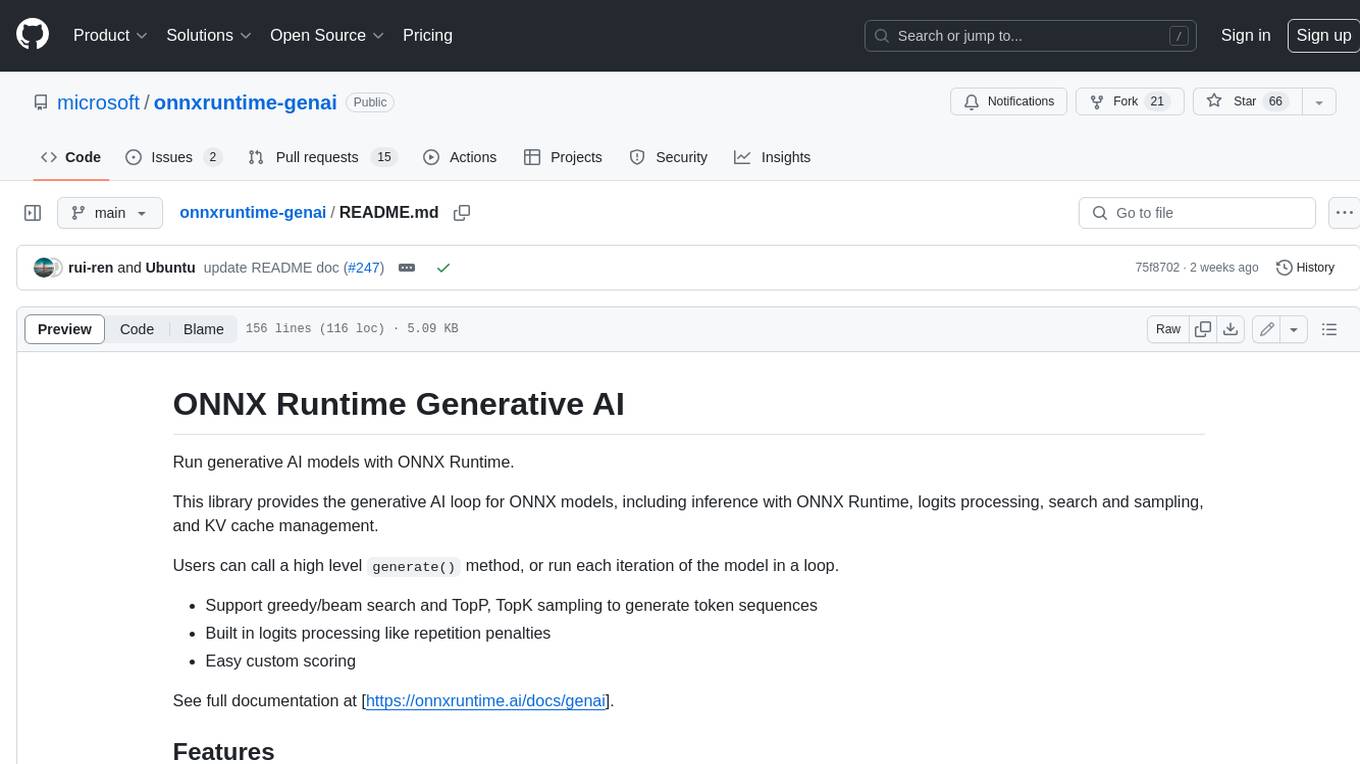
onnxruntime-genai
ONNX Runtime Generative AI is a library that provides the generative AI loop for ONNX models, including inference with ONNX Runtime, logits processing, search and sampling, and KV cache management. Users can call a high level `generate()` method, or run each iteration of the model in a loop. It supports greedy/beam search and TopP, TopK sampling to generate token sequences, has built in logits processing like repetition penalties, and allows for easy custom scoring.
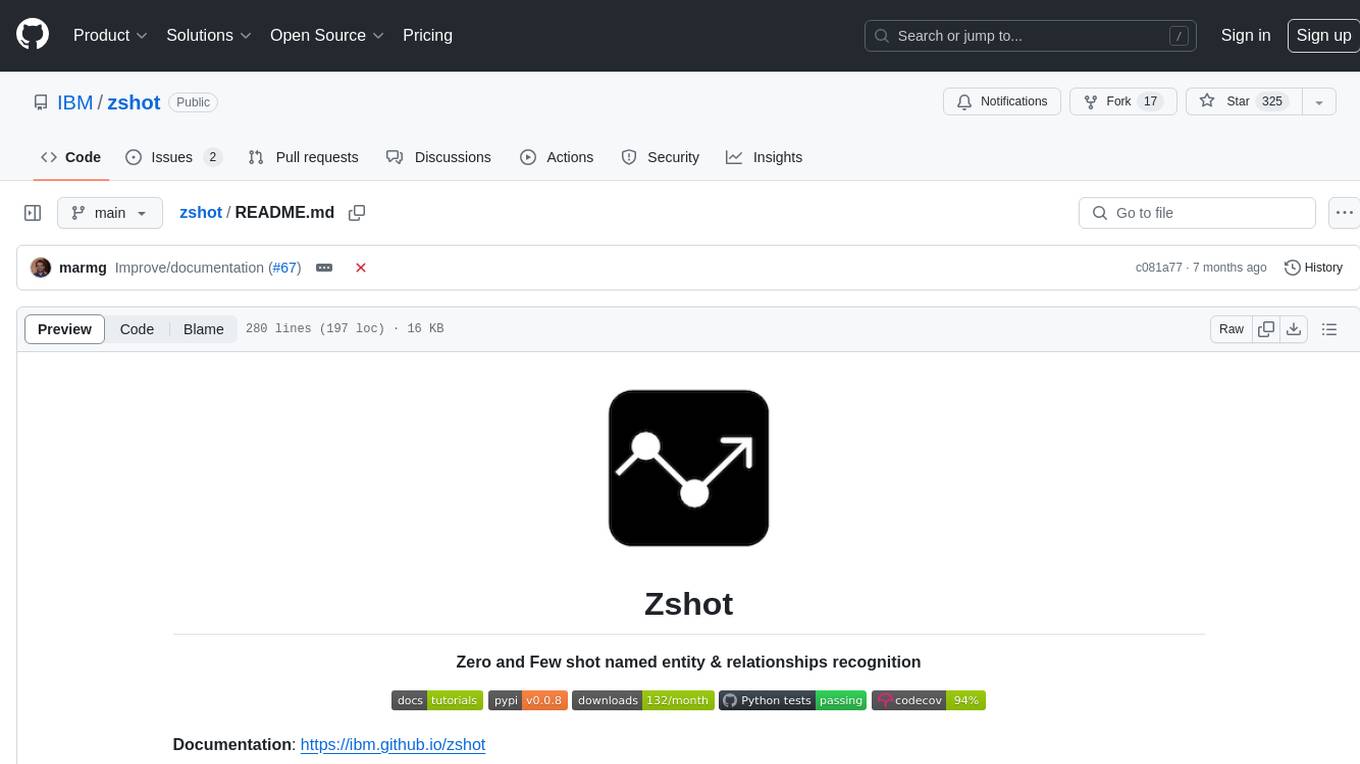
zshot
Zshot is a highly customizable framework for performing Zero and Few shot named entity and relationships recognition. It can be used for mentions extraction, wikification, zero and few shot named entity recognition, zero and few shot named relationship recognition, and visualization of zero-shot NER and RE extraction. The framework consists of two main components: the mentions extractor and the linker. There are multiple mentions extractors and linkers available, each serving a specific purpose. Zshot also includes a relations extractor and a knowledge extractor for extracting relations among entities and performing entity classification. The tool requires Python 3.6+ and dependencies like spacy, torch, transformers, evaluate, and datasets for evaluation over datasets like OntoNotes. Optional dependencies include flair and blink for additional functionalities. Zshot provides examples, tutorials, and evaluation methods to assess the performance of the components.
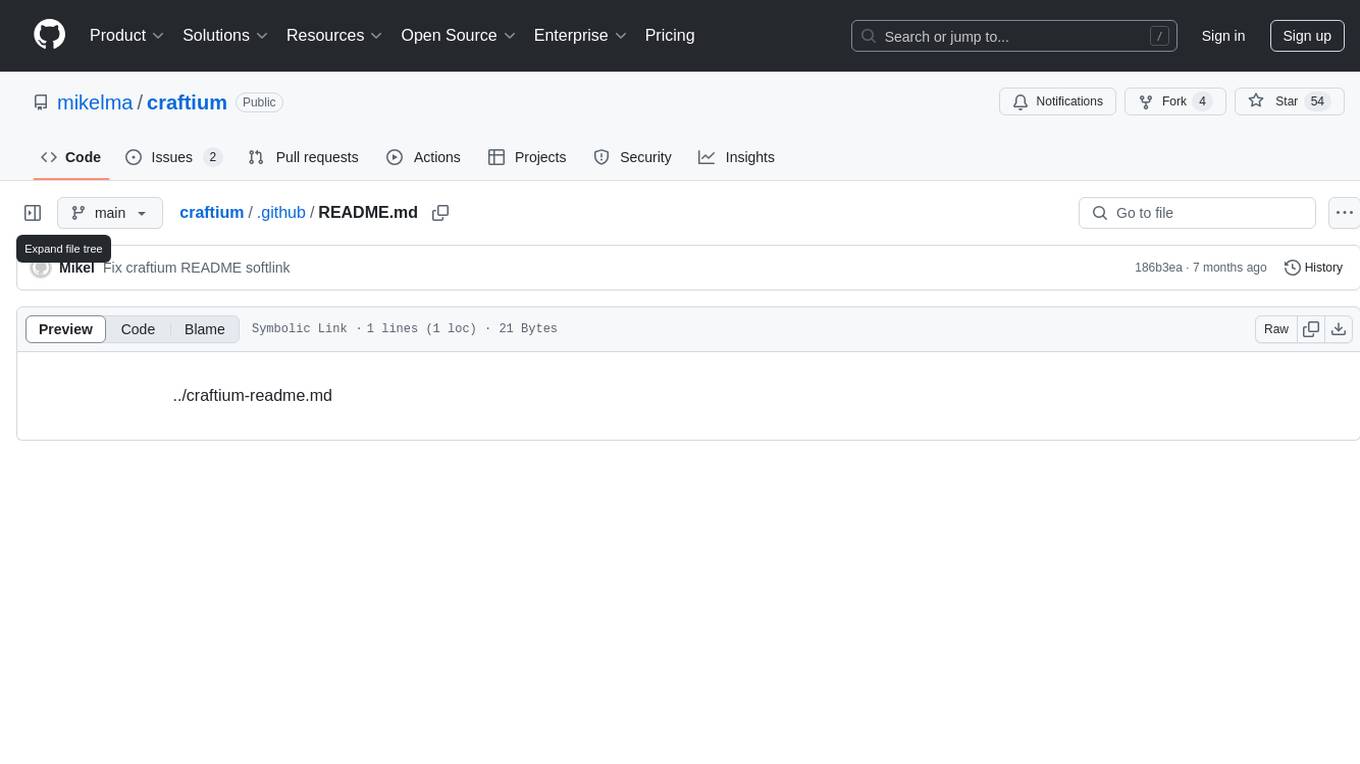
craftium
Craftium is an open-source platform based on the Minetest voxel game engine and the Gymnasium and PettingZoo APIs, designed for creating fast, rich, and diverse single and multi-agent environments. It allows for connecting to Craftium's Python process, executing actions as keyboard and mouse controls, extending the Lua API for creating RL environments and tasks, and supporting client/server synchronization for slow agents. Craftium is fully extensible, extensively documented, modern RL API compatible, fully open source, and eliminates the need for Java. It offers a variety of environments for research and development in reinforcement learning.
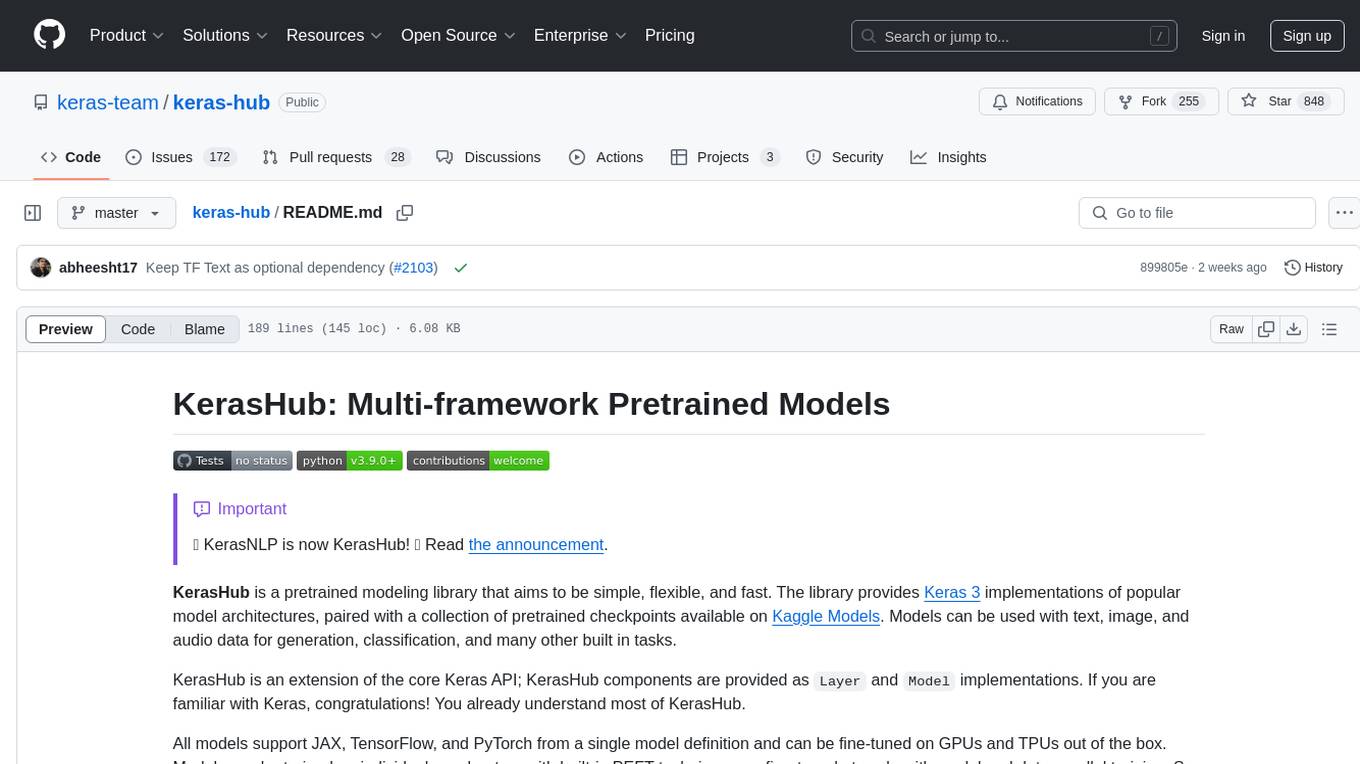
keras-hub
KerasHub is a pretrained modeling library that provides Keras 3 implementations of popular model architectures with pretrained checkpoints. It supports text, image, and audio data for generation, classification, and other tasks. Models are compatible with JAX, TensorFlow, and PyTorch, and can be fine-tuned on GPUs and TPUs. KerasHub components are provided as Layer and Model implementations, extending the core Keras API.
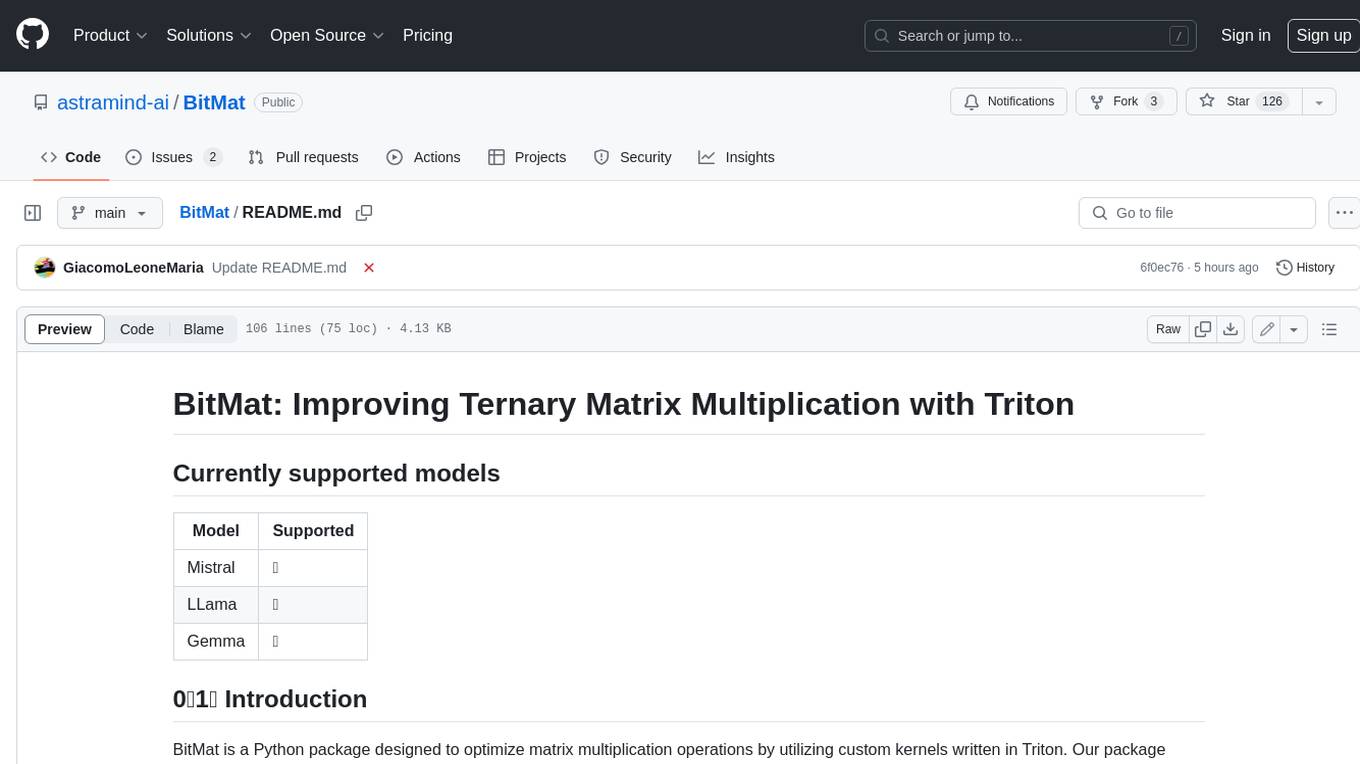
BitMat
BitMat is a Python package designed to optimize matrix multiplication operations by utilizing custom kernels written in Triton. It leverages the principles outlined in the "1bit-LLM Era" paper, specifically utilizing packed int8 data to enhance computational efficiency and performance in deep learning and numerical computing tasks.
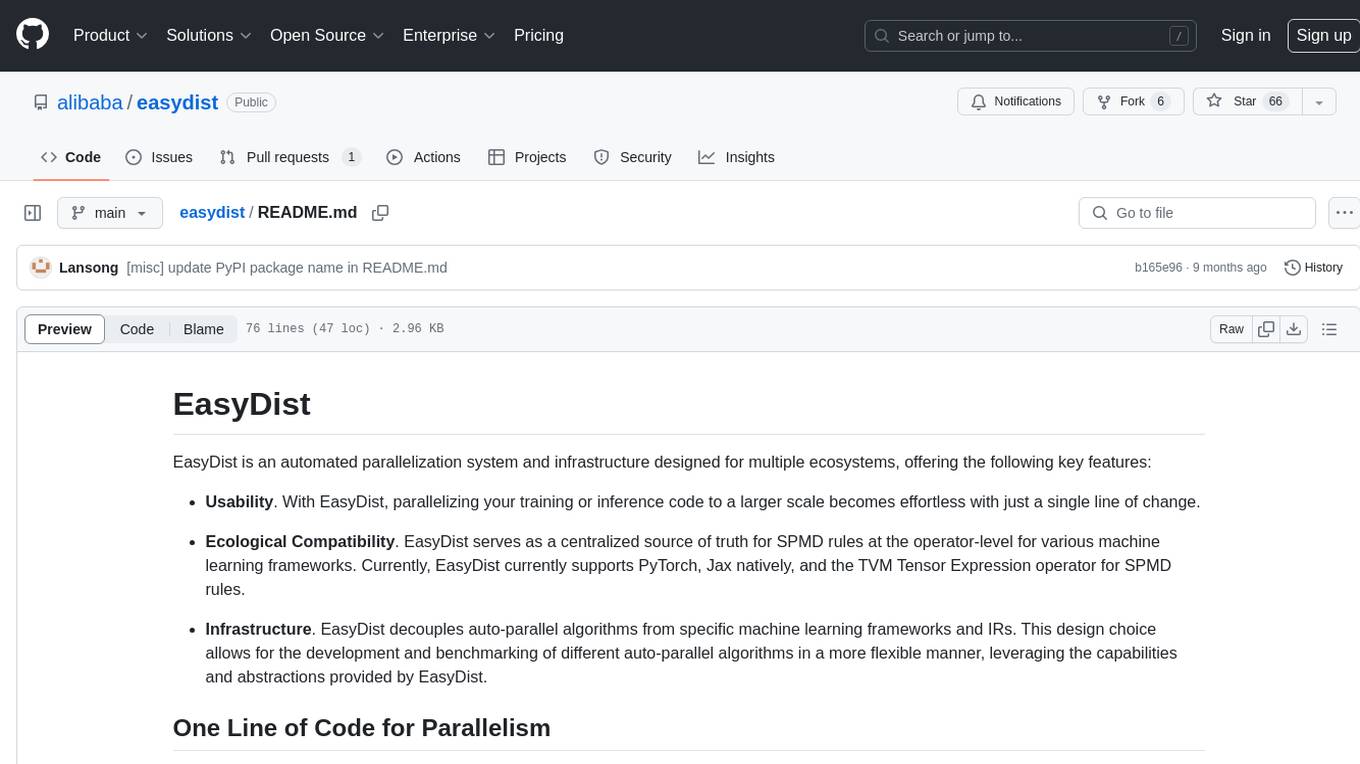
easydist
EasyDist is an automated parallelization system and infrastructure designed for multiple ecosystems. It offers usability by making parallelizing training or inference code effortless with just a single line of change. It ensures ecological compatibility by serving as a centralized source of truth for SPMD rules at the operator-level for various machine learning frameworks. EasyDist decouples auto-parallel algorithms from specific frameworks and IRs, allowing for the development and benchmarking of different auto-parallel algorithms in a flexible manner. The architecture includes MetaOp, MetaIR, and the ShardCombine Algorithm for SPMD sharding rules without manual annotations.
For similar tasks
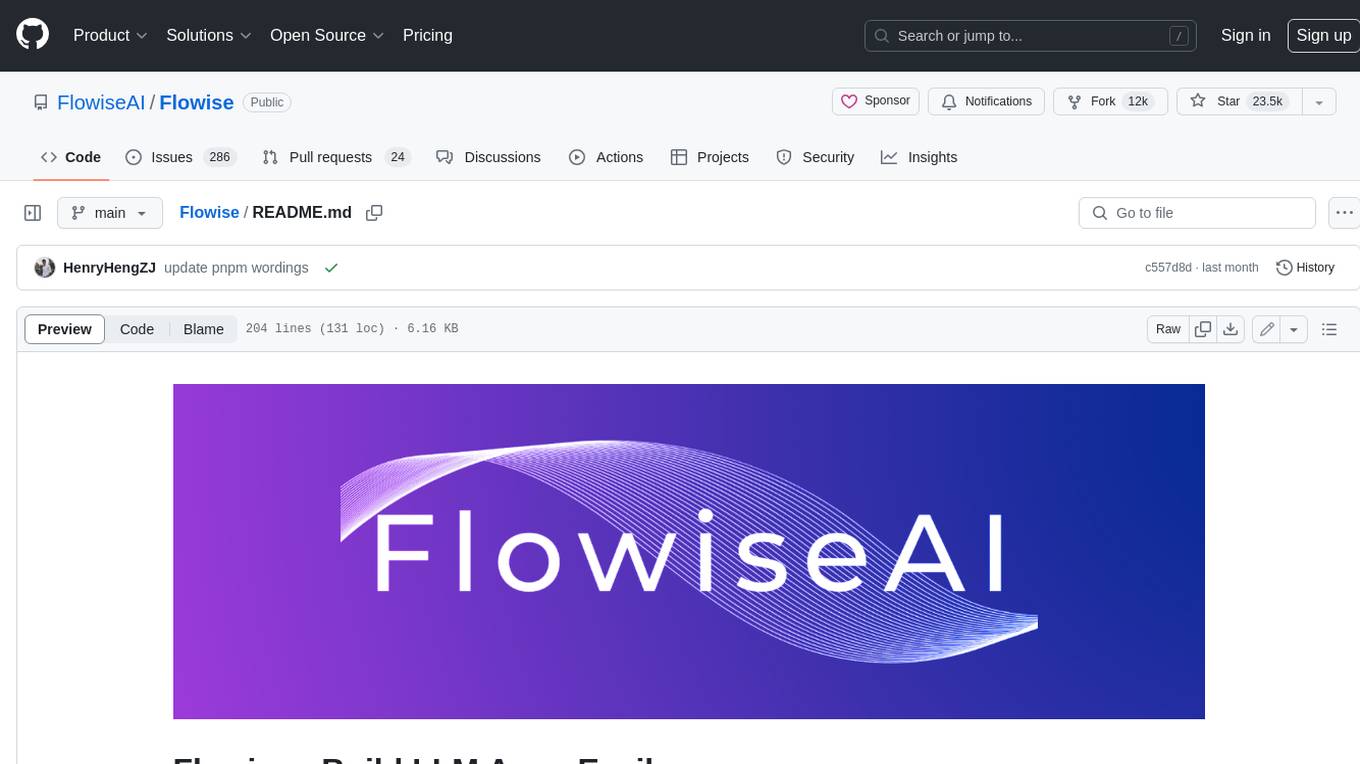
Flowise
Flowise is a tool that allows users to build customized LLM flows with a drag-and-drop UI. It is open-source and self-hostable, and it supports various deployments, including AWS, Azure, Digital Ocean, GCP, Railway, Render, HuggingFace Spaces, Elestio, Sealos, and RepoCloud. Flowise has three different modules in a single mono repository: server, ui, and components. The server module is a Node backend that serves API logics, the ui module is a React frontend, and the components module contains third-party node integrations. Flowise supports different environment variables to configure your instance, and you can specify these variables in the .env file inside the packages/server folder.
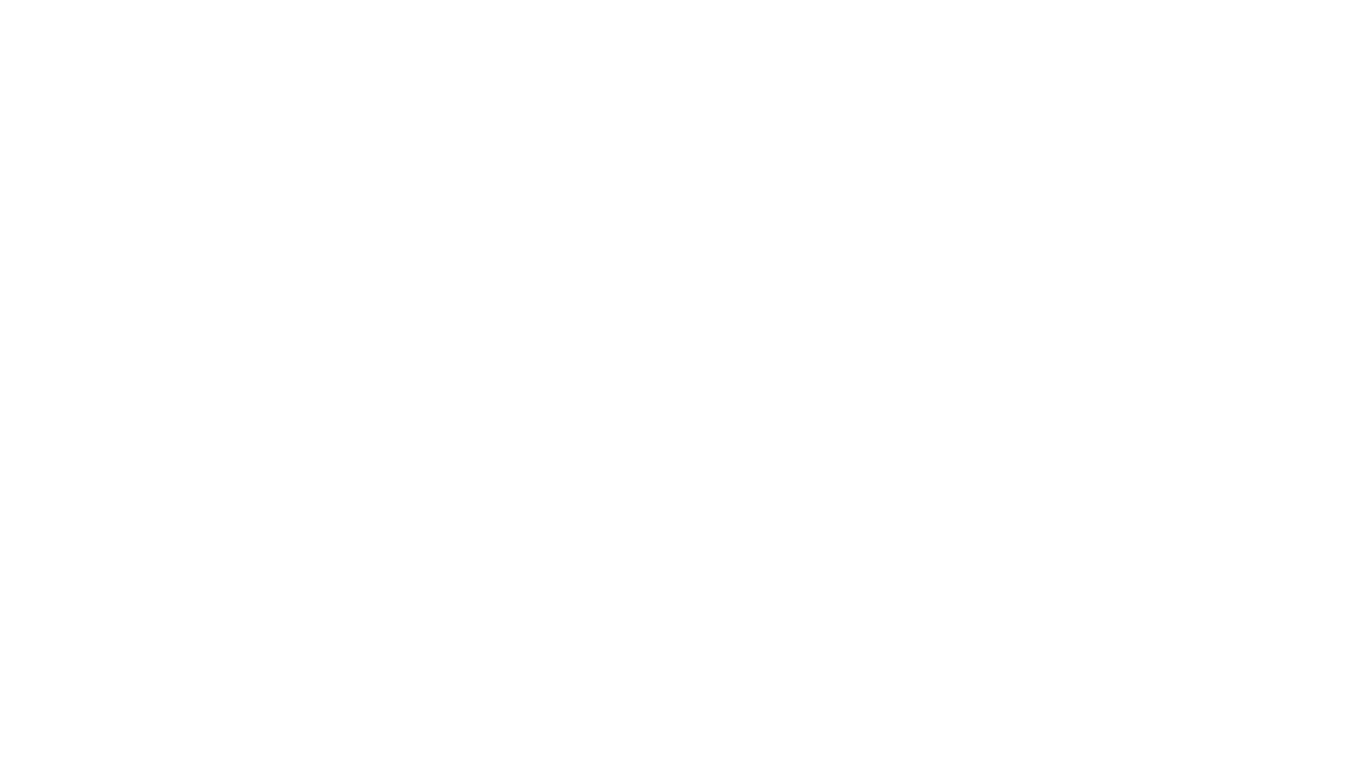
nlux
nlux is an open-source Javascript and React JS library that makes it super simple to integrate powerful large language models (LLMs) like ChatGPT into your web app or website. With just a few lines of code, you can add conversational AI capabilities and interact with your favourite LLM.
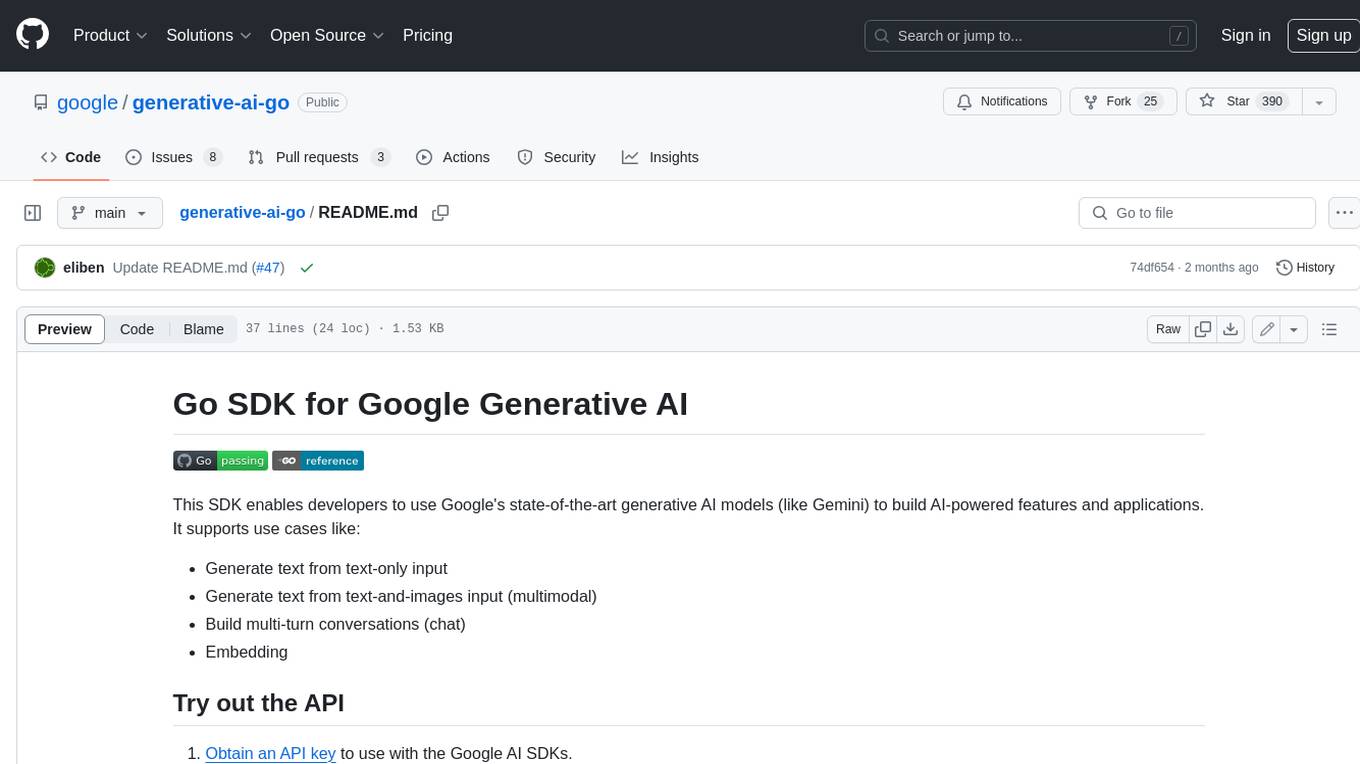
generative-ai-go
The Google AI Go SDK enables developers to use Google's state-of-the-art generative AI models (like Gemini) to build AI-powered features and applications. It supports use cases like generating text from text-only input, generating text from text-and-images input (multimodal), building multi-turn conversations (chat), and embedding.
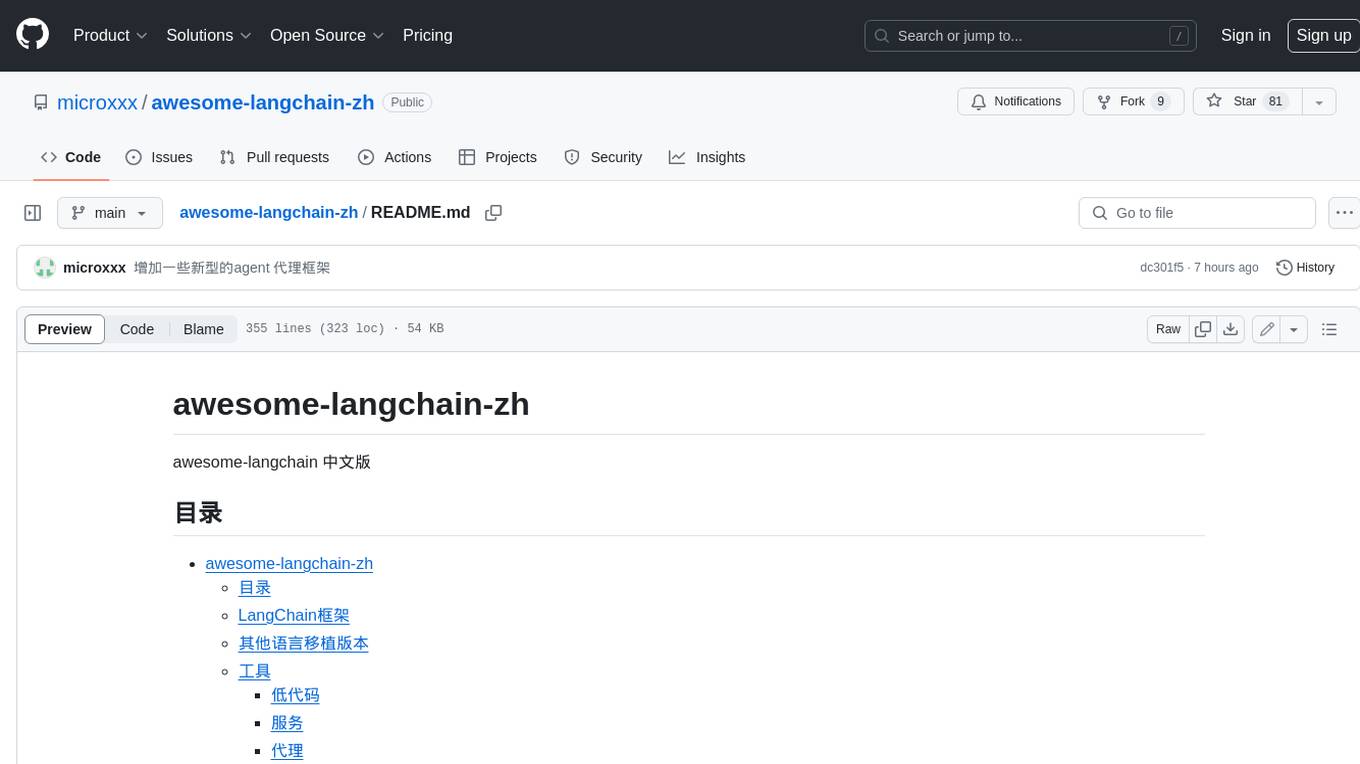
awesome-langchain-zh
The awesome-langchain-zh repository is a collection of resources related to LangChain, a framework for building AI applications using large language models (LLMs). The repository includes sections on the LangChain framework itself, other language ports of LangChain, tools for low-code development, services, agents, templates, platforms, open-source projects related to knowledge management and chatbots, as well as learning resources such as notebooks, videos, and articles. It also covers other LLM frameworks and provides additional resources for exploring and working with LLMs. The repository serves as a comprehensive guide for developers and AI enthusiasts interested in leveraging LangChain and LLMs for various applications.
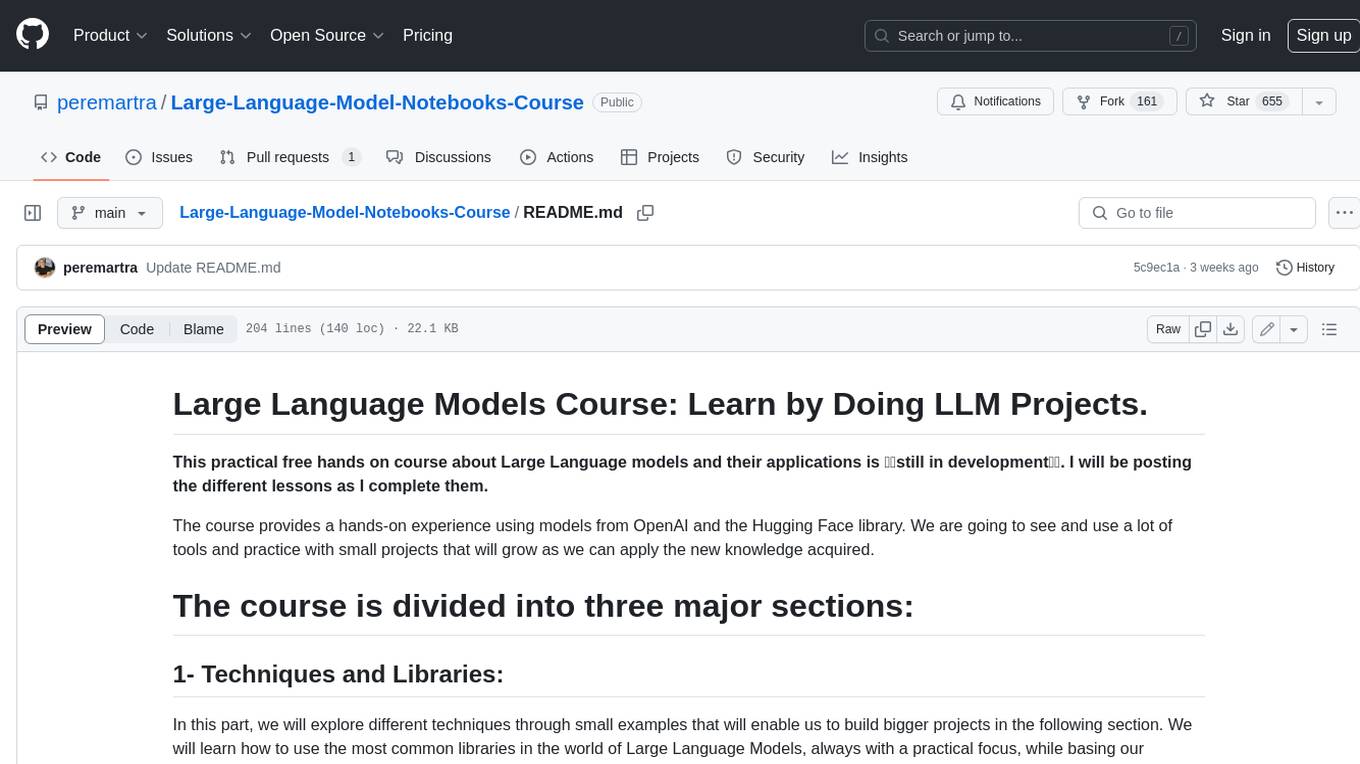
Large-Language-Model-Notebooks-Course
This practical free hands-on course focuses on Large Language models and their applications, providing a hands-on experience using models from OpenAI and the Hugging Face library. The course is divided into three major sections: Techniques and Libraries, Projects, and Enterprise Solutions. It covers topics such as Chatbots, Code Generation, Vector databases, LangChain, Fine Tuning, PEFT Fine Tuning, Soft Prompt tuning, LoRA, QLoRA, Evaluate Models, Knowledge Distillation, and more. Each section contains chapters with lessons supported by notebooks and articles. The course aims to help users build projects and explore enterprise solutions using Large Language Models.
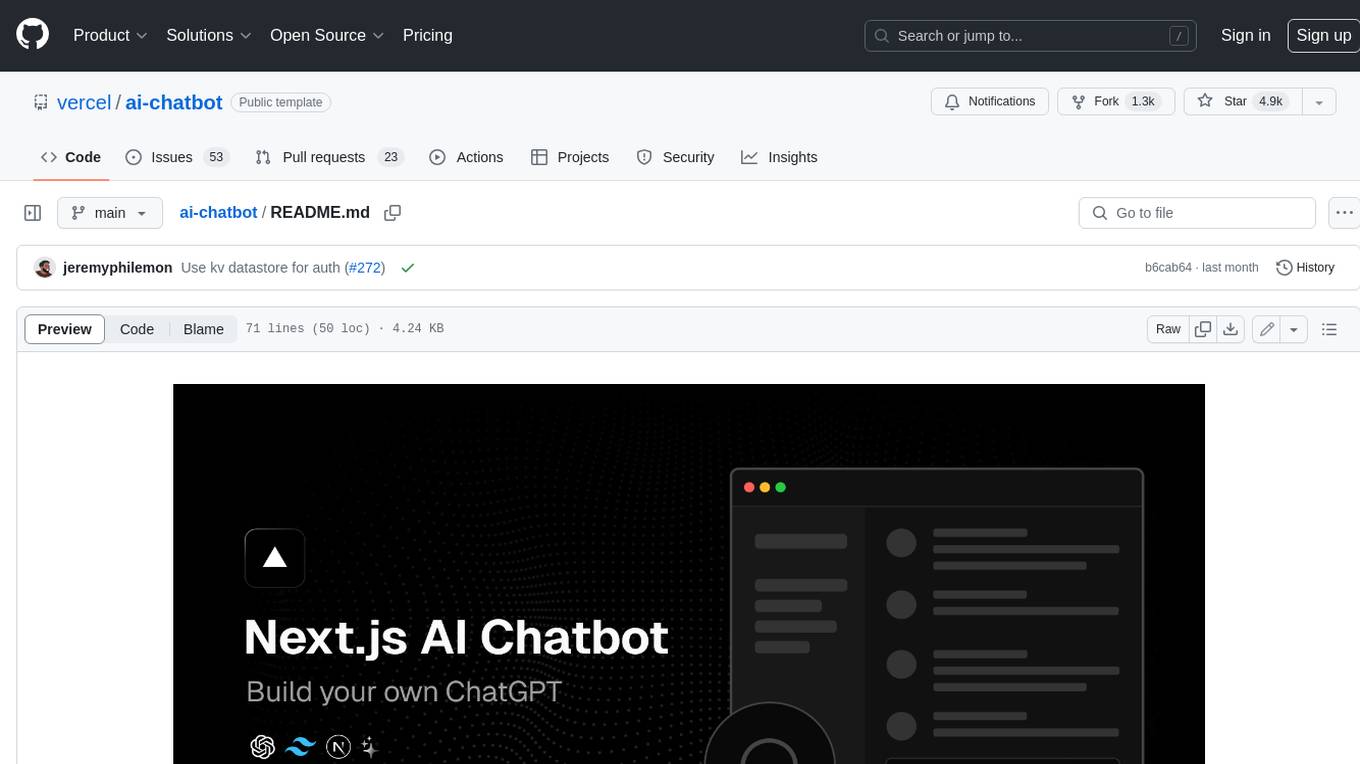
ai-chatbot
Next.js AI Chatbot is an open-source app template for building AI chatbots using Next.js, Vercel AI SDK, OpenAI, and Vercel KV. It includes features like Next.js App Router, React Server Components, Vercel AI SDK for streaming chat UI, support for various AI models, Tailwind CSS styling, Radix UI for headless components, chat history management, rate limiting, session storage with Vercel KV, and authentication with NextAuth.js. The template allows easy deployment to Vercel and customization of AI model providers.
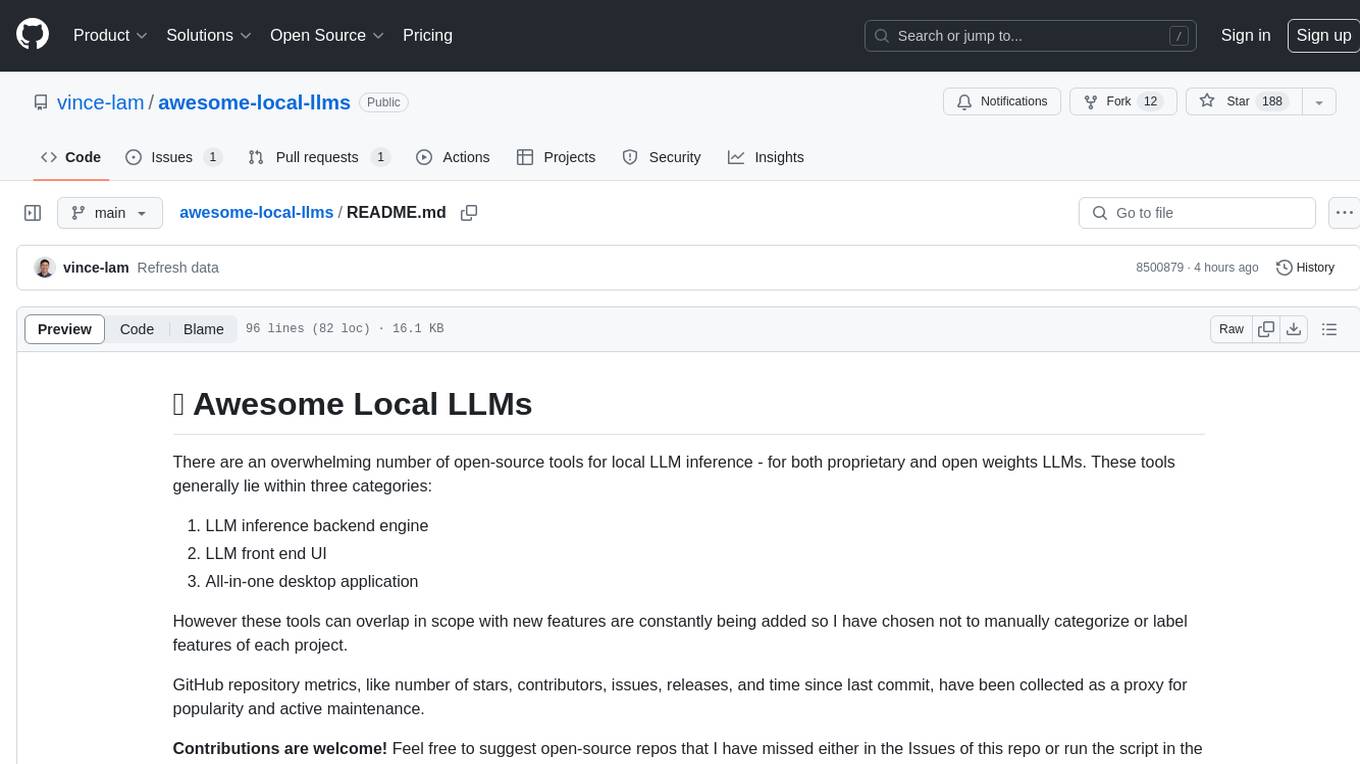
awesome-local-llms
The 'awesome-local-llms' repository is a curated list of open-source tools for local Large Language Model (LLM) inference, covering both proprietary and open weights LLMs. The repository categorizes these tools into LLM inference backend engines, LLM front end UIs, and all-in-one desktop applications. It collects GitHub repository metrics as proxies for popularity and active maintenance. Contributions are encouraged, and users can suggest additional open-source repositories through the Issues section or by running a provided script to update the README and make a pull request. The repository aims to provide a comprehensive resource for exploring and utilizing local LLM tools.
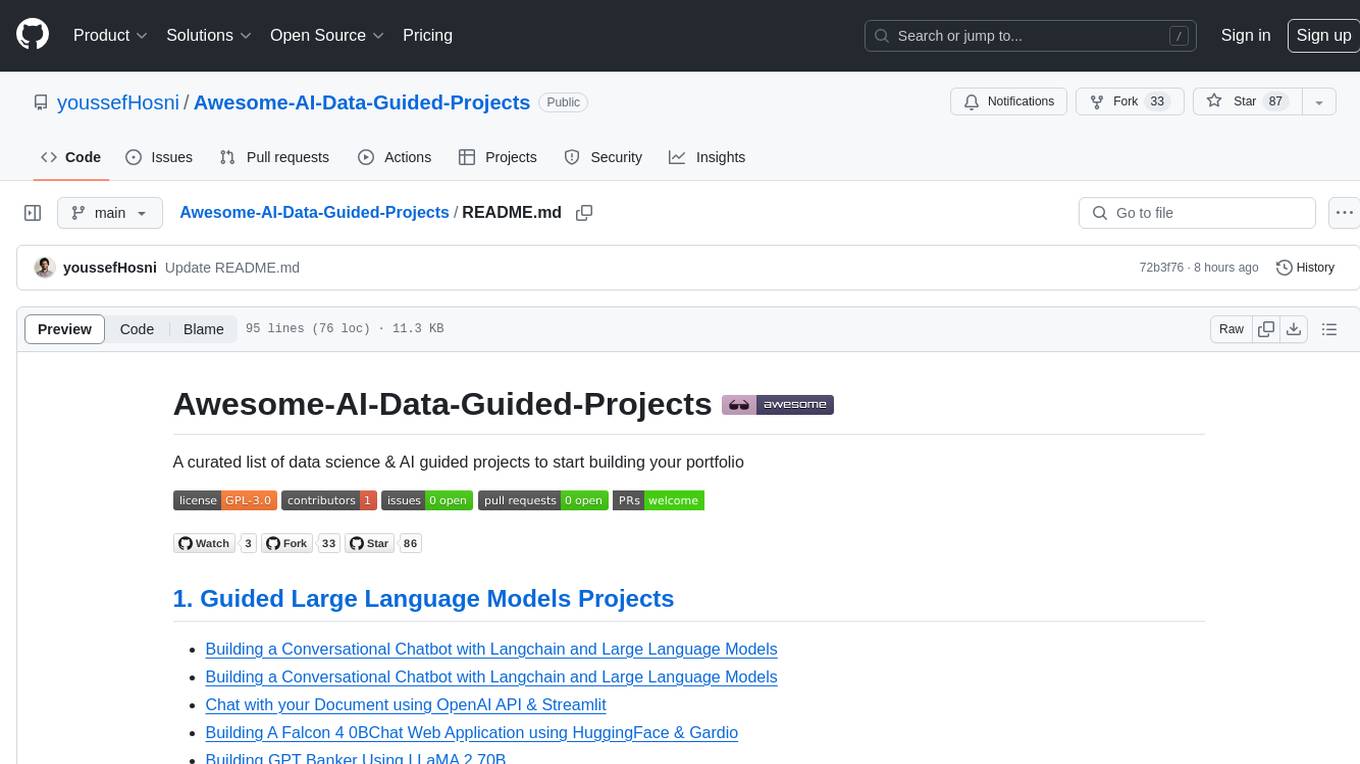
Awesome-AI-Data-Guided-Projects
A curated list of data science & AI guided projects to start building your portfolio. The repository contains guided projects covering various topics such as large language models, time series analysis, computer vision, natural language processing (NLP), and data science. Each project provides detailed instructions on how to implement specific tasks using different tools and technologies.
For similar jobs
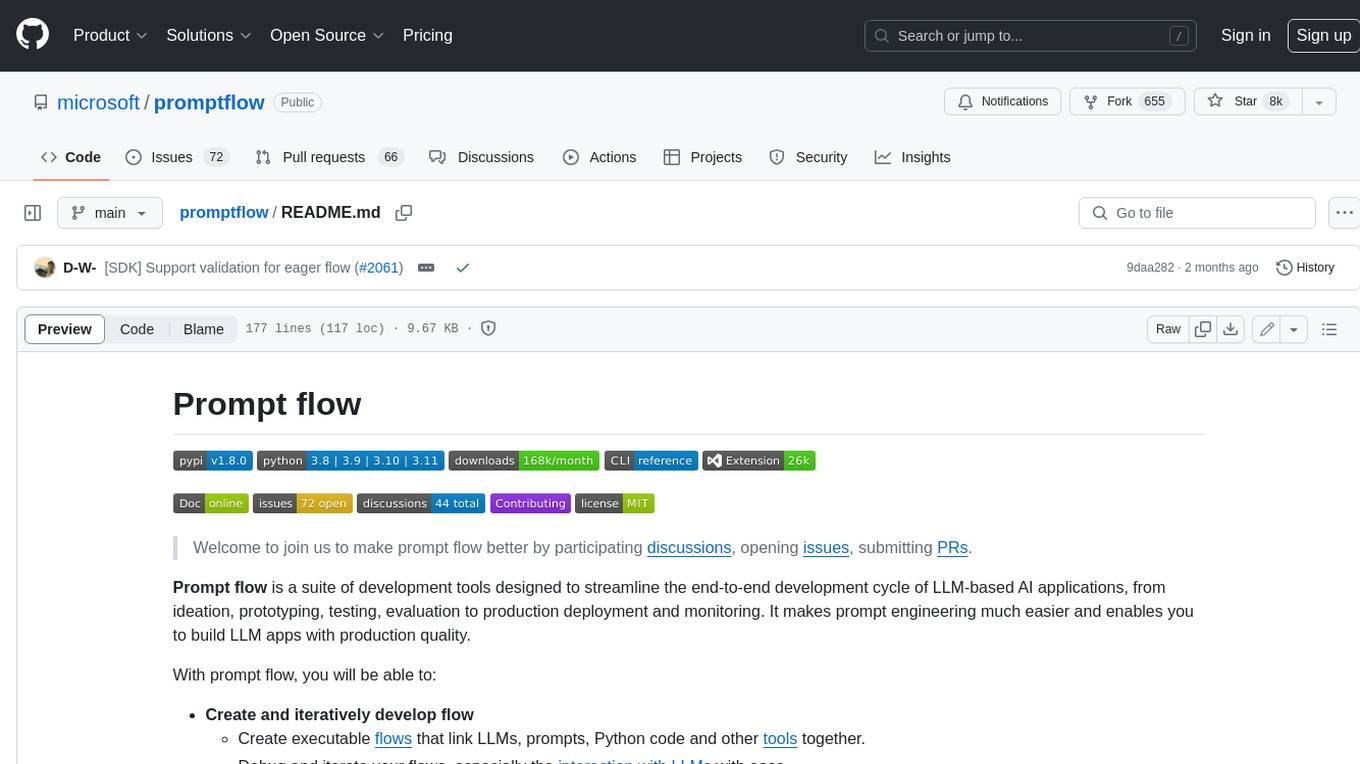
promptflow
**Prompt flow** is a suite of development tools designed to streamline the end-to-end development cycle of LLM-based AI applications, from ideation, prototyping, testing, evaluation to production deployment and monitoring. It makes prompt engineering much easier and enables you to build LLM apps with production quality.
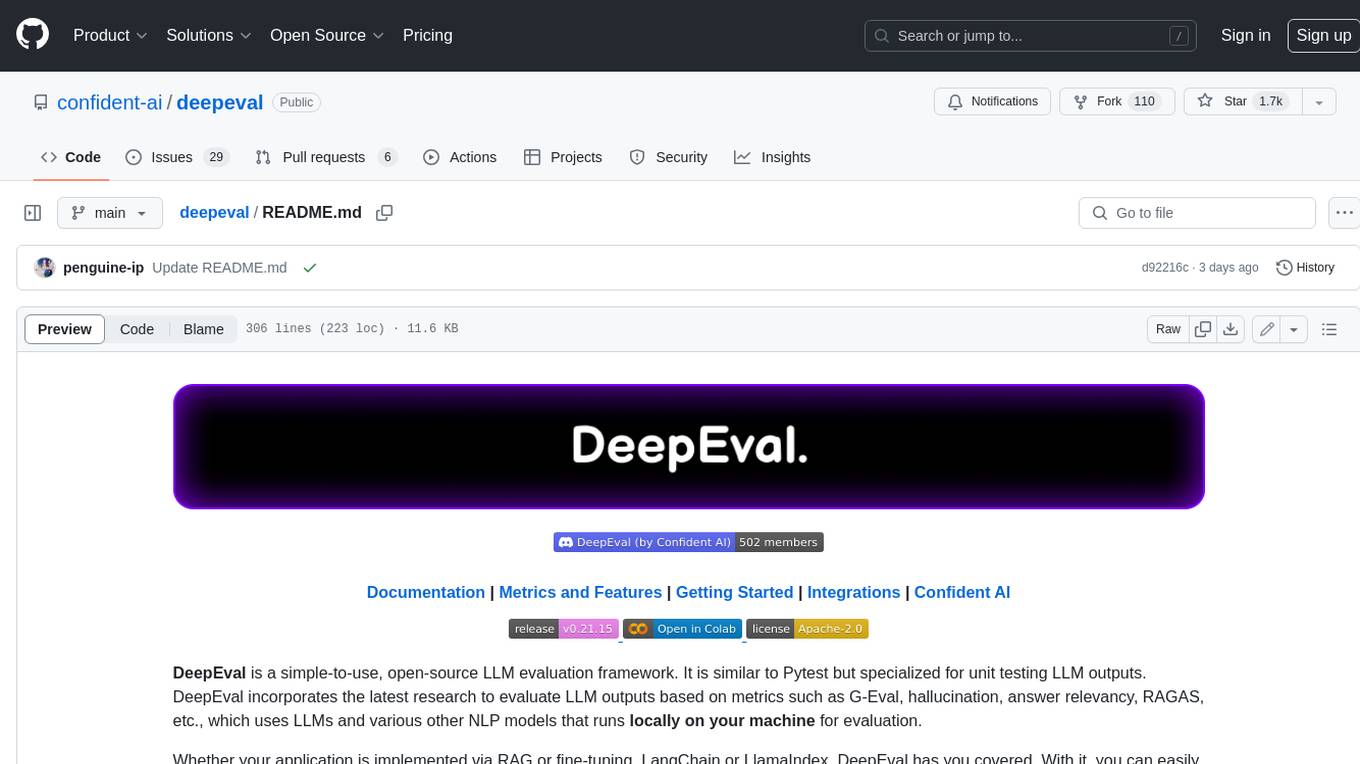
deepeval
DeepEval is a simple-to-use, open-source LLM evaluation framework specialized for unit testing LLM outputs. It incorporates various metrics such as G-Eval, hallucination, answer relevancy, RAGAS, etc., and runs locally on your machine for evaluation. It provides a wide range of ready-to-use evaluation metrics, allows for creating custom metrics, integrates with any CI/CD environment, and enables benchmarking LLMs on popular benchmarks. DeepEval is designed for evaluating RAG and fine-tuning applications, helping users optimize hyperparameters, prevent prompt drifting, and transition from OpenAI to hosting their own Llama2 with confidence.
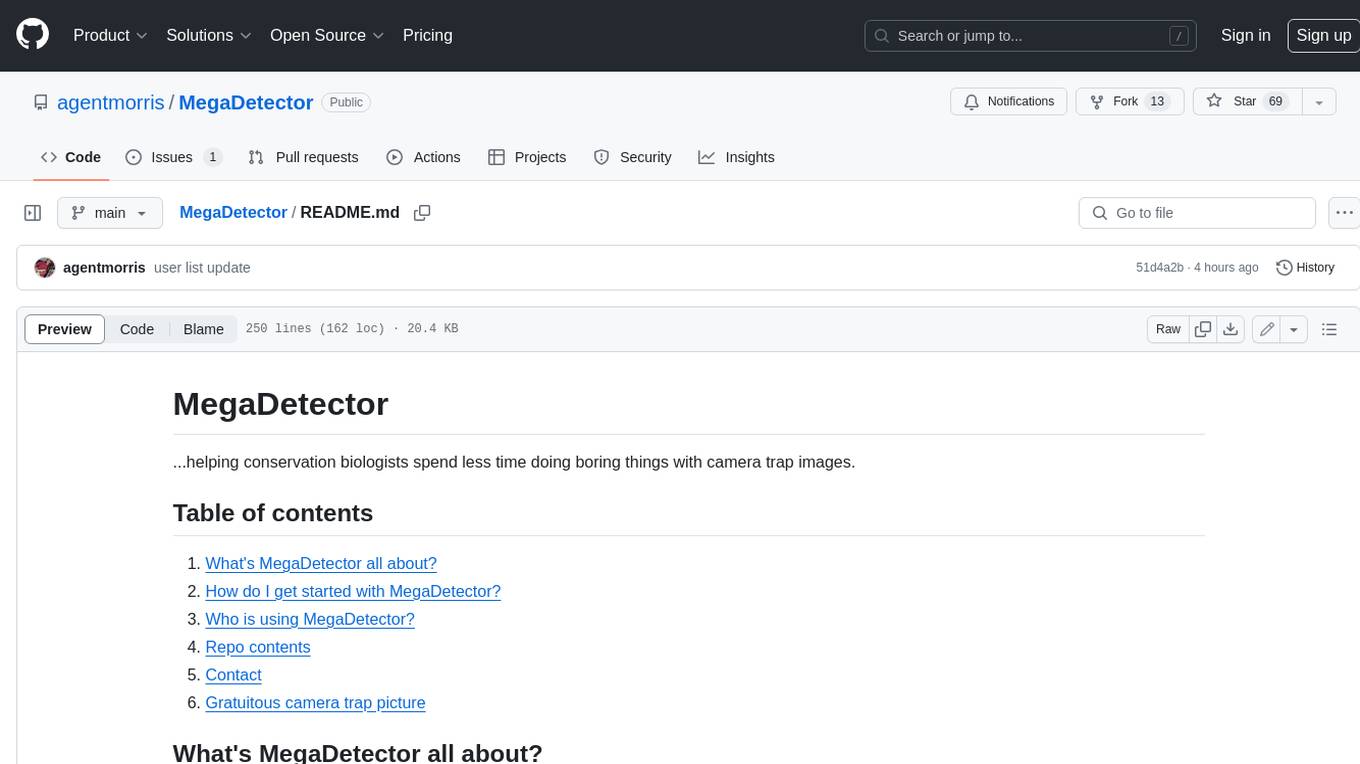
MegaDetector
MegaDetector is an AI model that identifies animals, people, and vehicles in camera trap images (which also makes it useful for eliminating blank images). This model is trained on several million images from a variety of ecosystems. MegaDetector is just one of many tools that aims to make conservation biologists more efficient with AI. If you want to learn about other ways to use AI to accelerate camera trap workflows, check out our of the field, affectionately titled "Everything I know about machine learning and camera traps".
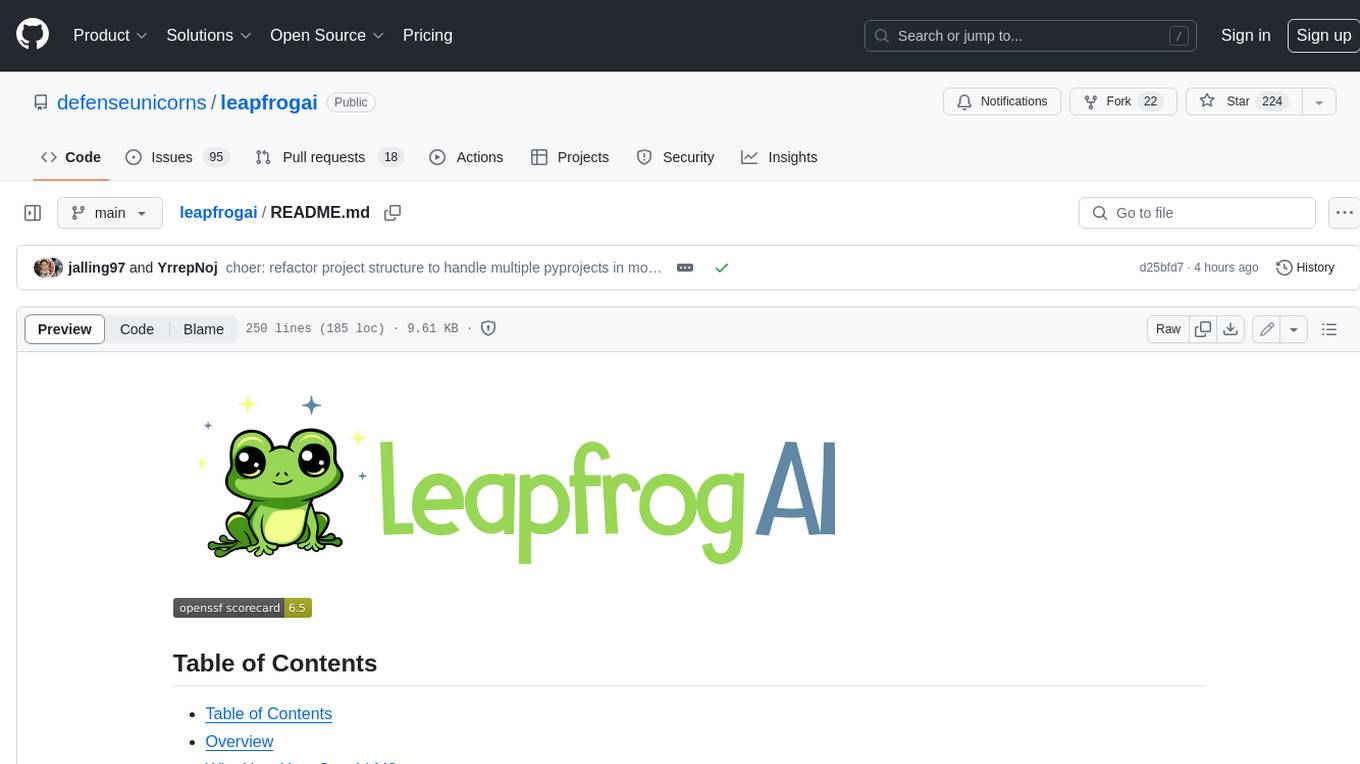
leapfrogai
LeapfrogAI is a self-hosted AI platform designed to be deployed in air-gapped resource-constrained environments. It brings sophisticated AI solutions to these environments by hosting all the necessary components of an AI stack, including vector databases, model backends, API, and UI. LeapfrogAI's API closely matches that of OpenAI, allowing tools built for OpenAI/ChatGPT to function seamlessly with a LeapfrogAI backend. It provides several backends for various use cases, including llama-cpp-python, whisper, text-embeddings, and vllm. LeapfrogAI leverages Chainguard's apko to harden base python images, ensuring the latest supported Python versions are used by the other components of the stack. The LeapfrogAI SDK provides a standard set of protobuffs and python utilities for implementing backends and gRPC. LeapfrogAI offers UI options for common use-cases like chat, summarization, and transcription. It can be deployed and run locally via UDS and Kubernetes, built out using Zarf packages. LeapfrogAI is supported by a community of users and contributors, including Defense Unicorns, Beast Code, Chainguard, Exovera, Hypergiant, Pulze, SOSi, United States Navy, United States Air Force, and United States Space Force.
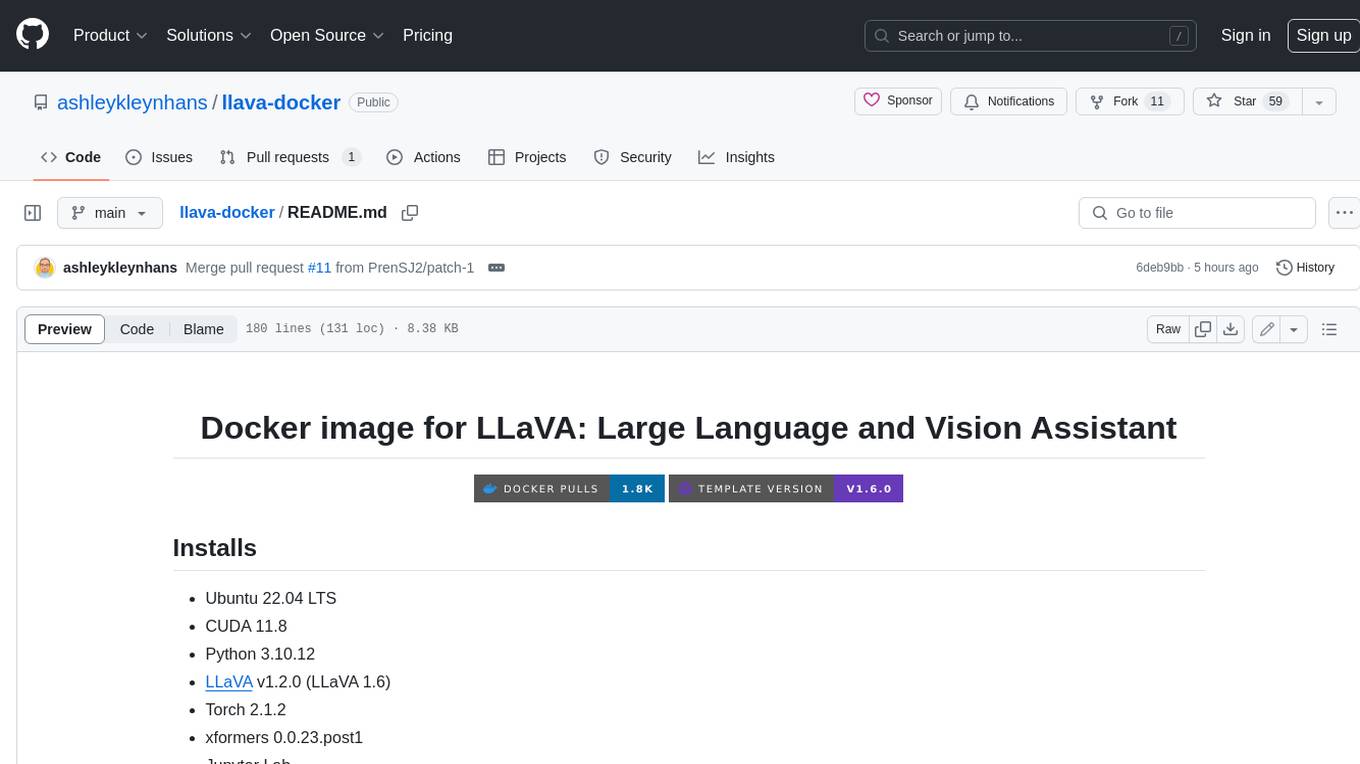
llava-docker
This Docker image for LLaVA (Large Language and Vision Assistant) provides a convenient way to run LLaVA locally or on RunPod. LLaVA is a powerful AI tool that combines natural language processing and computer vision capabilities. With this Docker image, you can easily access LLaVA's functionalities for various tasks, including image captioning, visual question answering, text summarization, and more. The image comes pre-installed with LLaVA v1.2.0, Torch 2.1.2, xformers 0.0.23.post1, and other necessary dependencies. You can customize the model used by setting the MODEL environment variable. The image also includes a Jupyter Lab environment for interactive development and exploration. Overall, this Docker image offers a comprehensive and user-friendly platform for leveraging LLaVA's capabilities.
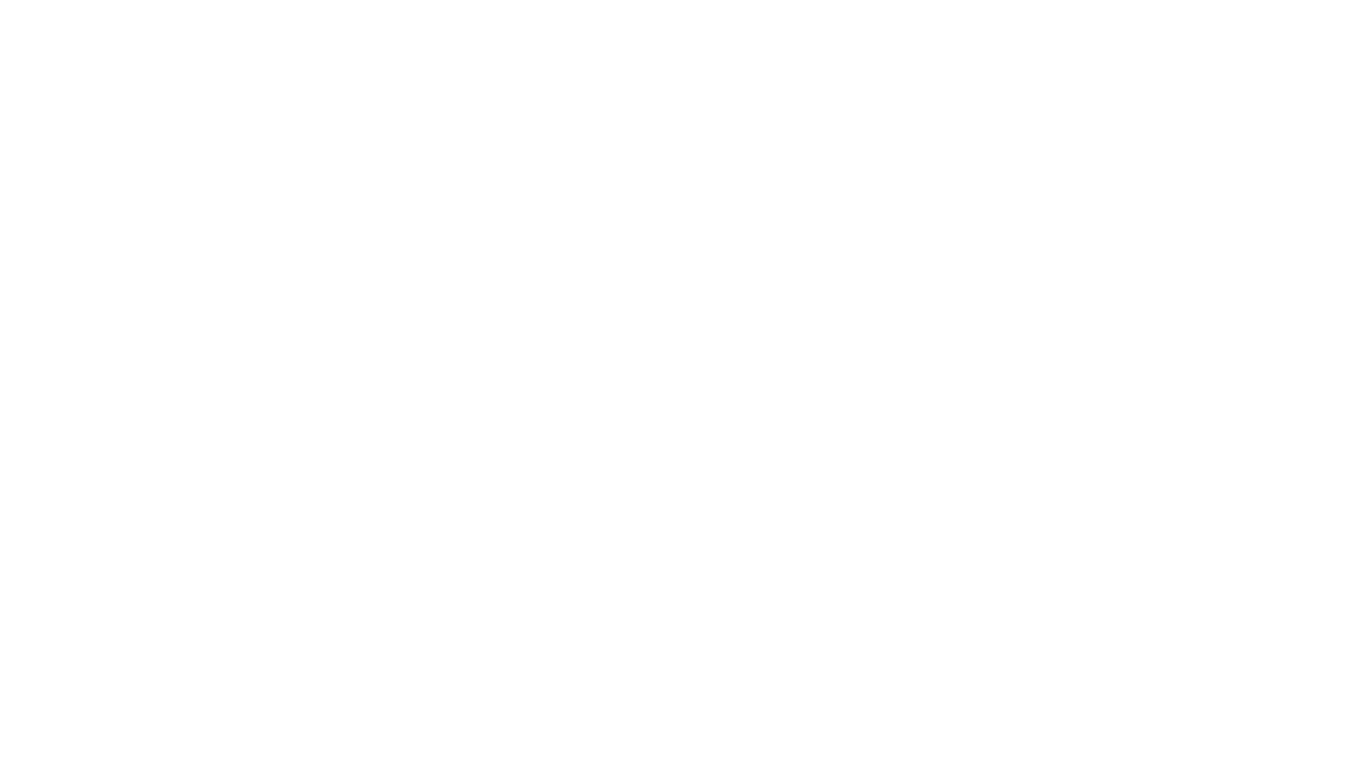
carrot
The 'carrot' repository on GitHub provides a list of free and user-friendly ChatGPT mirror sites for easy access. The repository includes sponsored sites offering various GPT models and services. Users can find and share sites, report errors, and access stable and recommended sites for ChatGPT usage. The repository also includes a detailed list of ChatGPT sites, their features, and accessibility options, making it a valuable resource for ChatGPT users seeking free and unlimited GPT services.
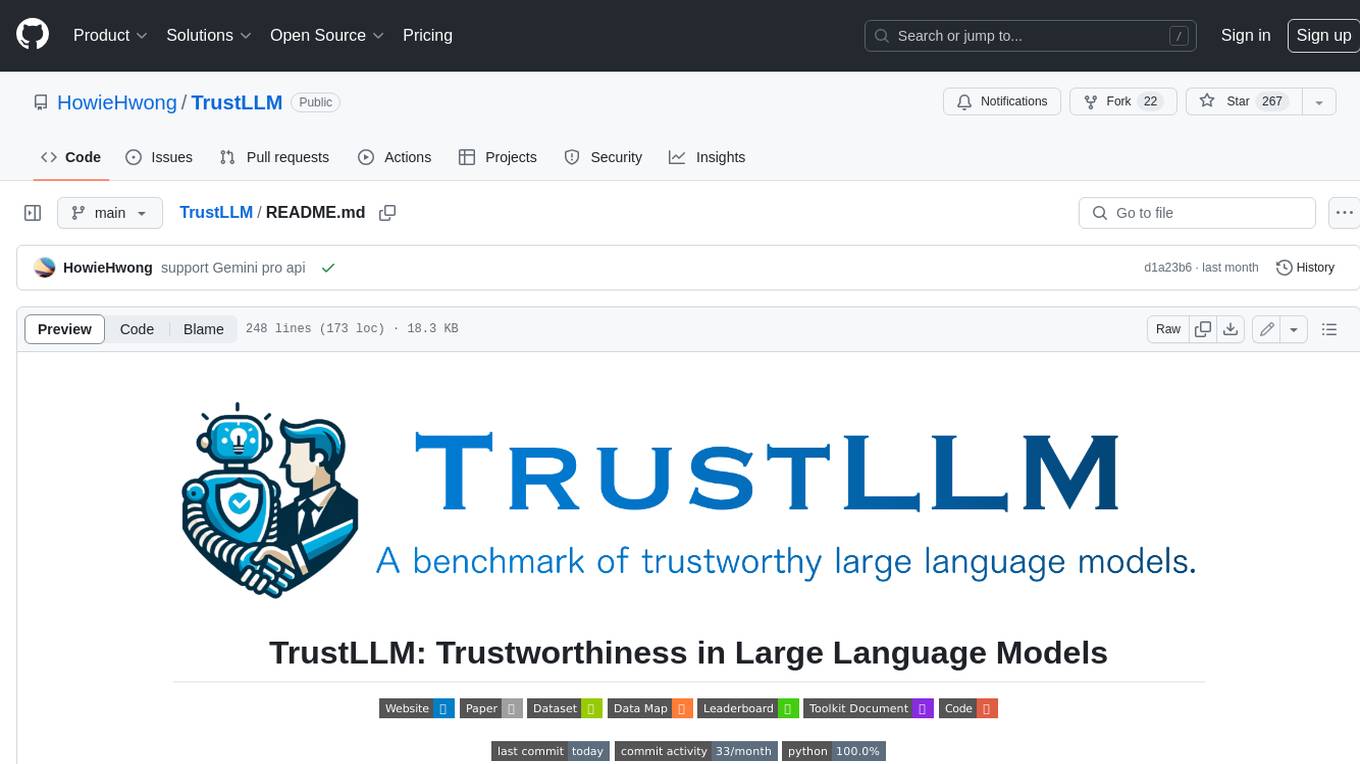
TrustLLM
TrustLLM is a comprehensive study of trustworthiness in LLMs, including principles for different dimensions of trustworthiness, established benchmark, evaluation, and analysis of trustworthiness for mainstream LLMs, and discussion of open challenges and future directions. Specifically, we first propose a set of principles for trustworthy LLMs that span eight different dimensions. Based on these principles, we further establish a benchmark across six dimensions including truthfulness, safety, fairness, robustness, privacy, and machine ethics. We then present a study evaluating 16 mainstream LLMs in TrustLLM, consisting of over 30 datasets. The document explains how to use the trustllm python package to help you assess the performance of your LLM in trustworthiness more quickly. For more details about TrustLLM, please refer to project website.
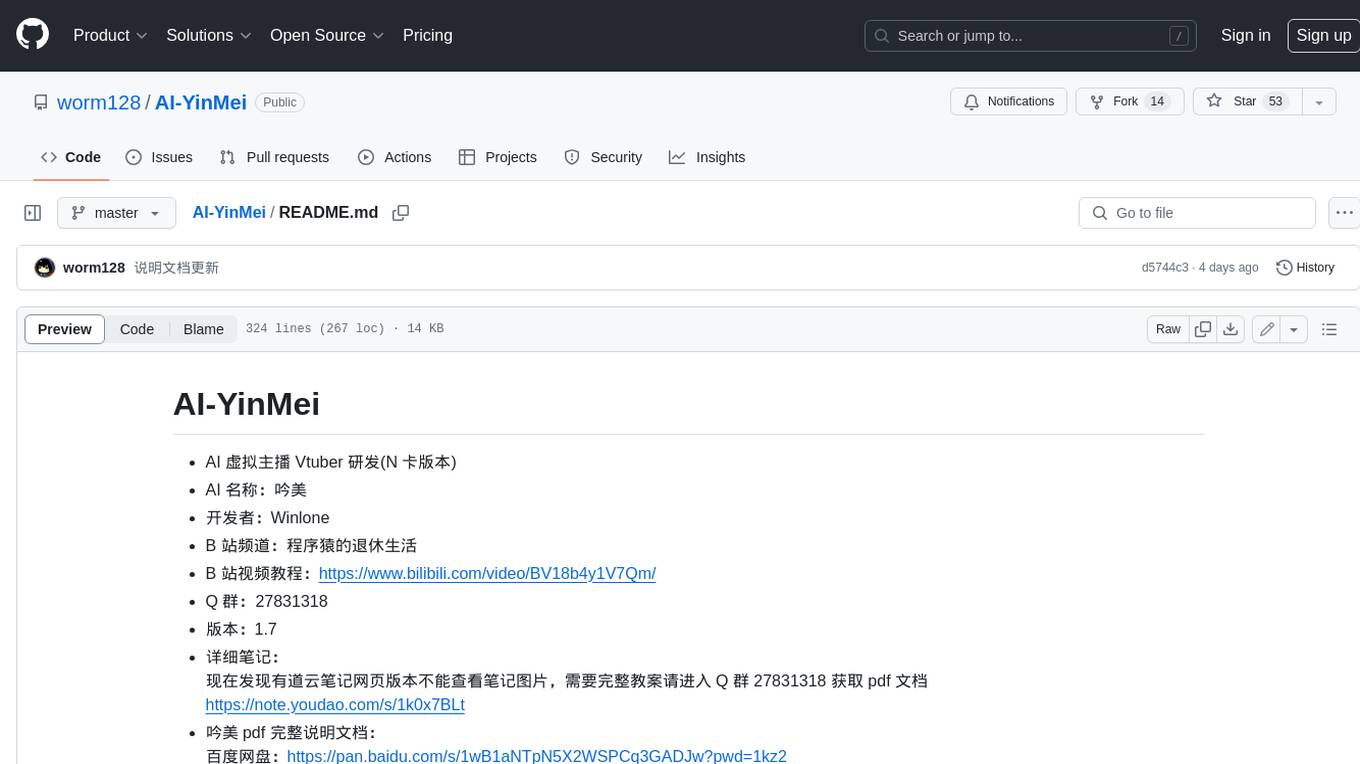
AI-YinMei
AI-YinMei is an AI virtual anchor Vtuber development tool (N card version). It supports fastgpt knowledge base chat dialogue, a complete set of solutions for LLM large language models: [fastgpt] + [one-api] + [Xinference], supports docking bilibili live broadcast barrage reply and entering live broadcast welcome speech, supports Microsoft edge-tts speech synthesis, supports Bert-VITS2 speech synthesis, supports GPT-SoVITS speech synthesis, supports expression control Vtuber Studio, supports painting stable-diffusion-webui output OBS live broadcast room, supports painting picture pornography public-NSFW-y-distinguish, supports search and image search service duckduckgo (requires magic Internet access), supports image search service Baidu image search (no magic Internet access), supports AI reply chat box [html plug-in], supports AI singing Auto-Convert-Music, supports playlist [html plug-in], supports dancing function, supports expression video playback, supports head touching action, supports gift smashing action, supports singing automatic start dancing function, chat and singing automatic cycle swing action, supports multi scene switching, background music switching, day and night automatic switching scene, supports open singing and painting, let AI automatically judge the content.