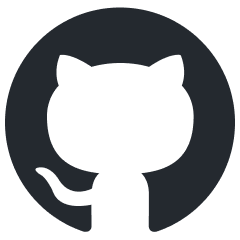
Large-Language-Model-Notebooks-Course
Practical course about Large Language Models.
Stars: 1215
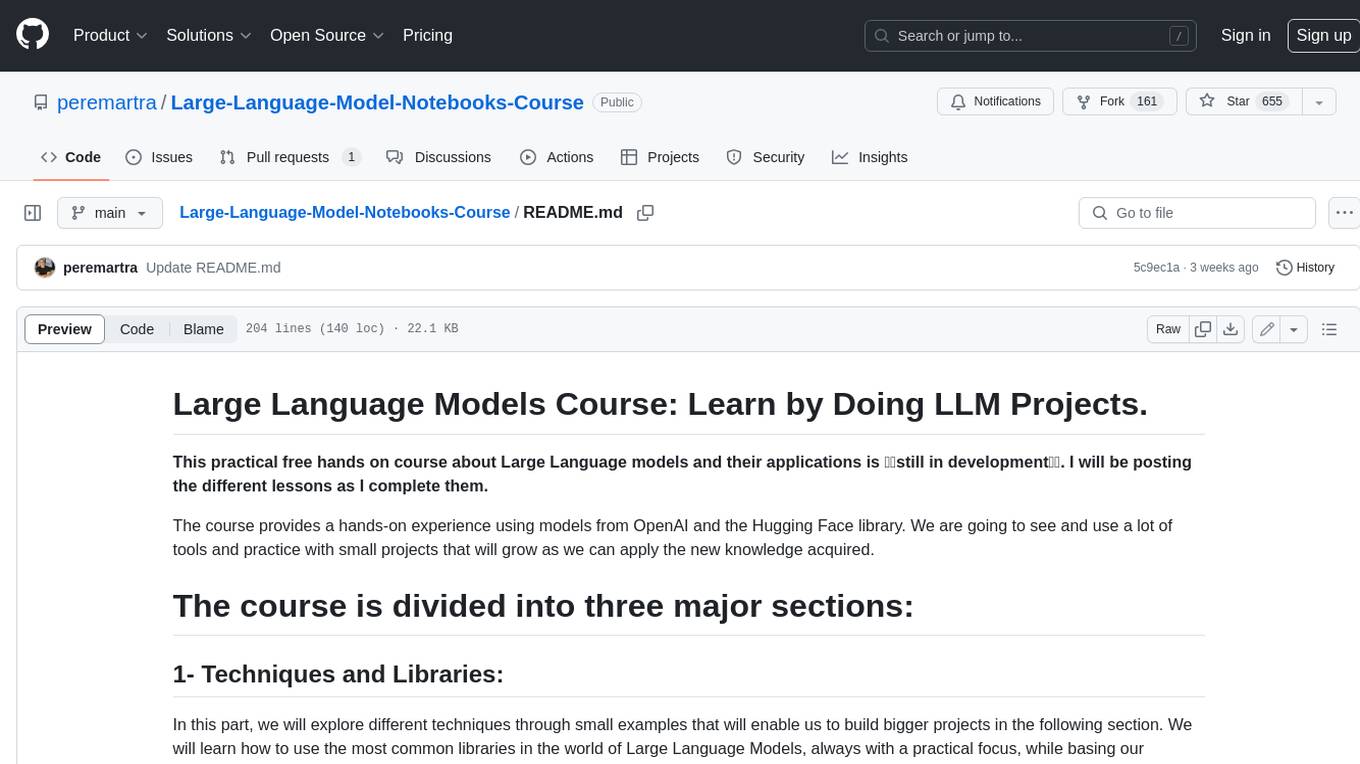
This practical free hands-on course focuses on Large Language models and their applications, providing a hands-on experience using models from OpenAI and the Hugging Face library. The course is divided into three major sections: Techniques and Libraries, Projects, and Enterprise Solutions. It covers topics such as Chatbots, Code Generation, Vector databases, LangChain, Fine Tuning, PEFT Fine Tuning, Soft Prompt tuning, LoRA, QLoRA, Evaluate Models, Knowledge Distillation, and more. Each section contains chapters with lessons supported by notebooks and articles. The course aims to help users build projects and explore enterprise solutions using Large Language Models.
README:
![]() |
This is the unofficial repository for the book: Large Language Models: Apply and Implement Strategies for Large Language Models (Apress). The book is based on the content of this repository, but the notebooks are being updated, and I am incorporating new examples and chapters. If you are looking for the official repository for the book, with the original notebooks, you should visit the Apress repository, where you can find all the notebooks in their original format as they appear in the book. Buy it at: [Amazon] [Springer] |
Please note that the course on GitHub does not contain all the information that is in the book.
This practical free hands on course about Large Language models and their applications is ๐ท๐ผin permanent development๐ท๐ผ. I will be posting the different lessons and samples as I complete them.
The course provides a hands-on experience using models from OpenAI and the Hugging Face library. We are going to see and use a lot of tools and practice with small projects that will grow as we can apply the new knowledge acquired.
In this part, we will explore different techniques through small examples that will enable us to build bigger projects in the following section. We will learn how to use the most common libraries in the world of Large Language Models, always with a practical focus, while basing our approach on published papers.Some of the topics and technologies covered in this section include: Chatbots, Code Generation, OpenAI API, Hugging Face, Vector databases, LangChain, Fine Tuning, PEFT Fine Tuning, Soft Prompt tuning, LoRA, QLoRA, Evaluate Models, Knowledge Distillation.
We will create projects, explaining design decisions. Each project may have more than one possible implementation, as often there is not just one perfect solution. In this section, we will also delve into LLMOps-related topics, although it is not the primary focus of the course. Large Language Models are not a standalone solution. In large corporate environments, they are just one piece of the puzzle. We will explore how to structure solutions capable of transforming organizations with thousands of employees, and how Large Language Models play a main role in these new solutions. Under each section you can find different chapters, that are formed by different lessons. The title of the lesson is a link to the lesson page, where you can found all the notebooks and articles of the lesson.Each Lesson is conformed by notebooks and articles. The notebooks contain sufficient information for understanding the code within them, the article provides more detailed explanations about the code and the topic covered.
My advice is to have the article open alongside the notebook and follow along. Many of the articles offer small tips on variations that you can introduce to the notebooks. I recommend following them to enhance clarity of the concepts.
Most of the notebooks are hosted on Colab, while a few are on Kaggle. Kaggle provides more memory in the free version compared to Colab, but I find that copying and sharing notebooks is simpler in Colab, and not everyone has a Kaggle account.
Some of the notebooks require more memory than what the free version of Colab provides. As we are working with large language models, this is a common situation that will recur if you continue working with them. You can run the notebooks in your own environment or opt for the Pro version of Colab.
Each notebook is supported with a Medium article where the code is explained in detail.
In this first section of the course, we will learn to work with the OpenAI API by creating two small projects. We'll delve into OpenAI's roles and how to provide the necessary instructions to the model through the prompt to make it behave as we desire.
The first project is a restaurant chatbot where the model will take customer orders. Building upon this project, we will construct an SQL statement generator. Here, we'll attempt to create a secure prompt that only accepts SQL creation commands and nothing else.
We will be utilizing OpenAI GPT-3.5 and Panel to develop a straightforward Chatbot tailored for a fast food restaurant. During the course, we will explore the fundamentals of prompt engineering, including understanding the various OpenAI roles, manipulating temperature settings, and how to avoid Prompt Injections.
article panel / article gradio | Notebook panel / notebook gradio |
---|
Following the same framework utilized in the previous article to create the ChatBot, we made a few modifications to develop a Natural Language to SQL translator. In this case, the Model needs to be provided with the table structures, and adjustments were made to the prompt to ensure smooth functionality and avoid any potential malfunctions. With these modifications in place, the translator is capable of converting natural language queries into SQL queries. @fmquaglia has created a notebook using DBML to describe the tables that by far is a better aproach than the original.
Article / Article Gradio | Notebook / Notebook Gradio / Notebook DBML |
---|
We will explore prompt engineering techniques to improve the results we obtain from Models. Like how to format the answer and obtain a structured response using Few Shot Samples.
Article | Notebook |
---|
A brief introduction to Vector Databases, a technology that will accompany us in many lessons throughout the course. We will work on an example of Retrieval Augmented Generation using information from various news datasets stored in ChromaDB.
If there's one aspect gaining importance in the world of large language models, it's exploring how to leverage proprietary information with them. In this lesson, we explore a possible solution that involves storing information in a vector database, ChromaDB in our case, and using it to create enriched prompts.
Article | Notebook |
---|
We enhanced the RAG system by introducing a semantic cache layer capable of determining if a similar question has been asked before. If affirmative, it retrieves information from a cache system created with Faiss instead of accessing the Vector Database.
The inspiration and base code of the semantic cache present in this notebook exist thanks to the course: https://maven.com/boring-bot/advanced-llm/1/home from Hamza Farooq.
Article | Notebook |
---|---|
WIP | Notebook |
LangChain has been one of the libraries in the universe of large language models that has contributed the most to this revolution. It allows us to chain calls to Models and other systems, allowing us to build applications based on large language models. In the course, we will use it several times, creating increasingly complex projects.
In this lesson, we used LangChain to enhance the notebook from the previous lesson, where we used data from two datasets to create an enriched prompt. This time, with the help of LangChain, we built a pipeline that is responsible for retrieving data from the vector database and passing it to the Language Model. The notebook is set up to work with two different datasets and two different models. One of the models is trained for Text Generation, while the other is trained for Text2Text Generation.
Article | Notebook |
---|
We will create a comment response system using a two-model pipeline built with LangChain. In this setup, the second model will be responsible for moderating the responses generated by the first model.
One effective way to prevent our system from generating unwanted responses is by using a second model that has no direct interaction with users to handle response generation.
This approach can reduce the risk of undesired responses generated by the first model in response to the user's entry.
I will create separate notebooks for this task. One will involve models from OpenAI, and the others will utilize open-source models provided by Hugging Face. The results obtained in the three notebooks are very different. The system works much better with the OpenAI, and LLAMA2 models.
Article | Notebook |
---|---|
OpenAI article | OpenAI notebook |
Llama2-7B Article | Llama2-7B Notebook |
No Article | GPT-J Notebook |
Agents are one of the most powerful tools in the world of Large Language Models. The agent is capable of interpreting the user's request and using the tools and libraries at its disposal until it achieves the expected result.
With LangChain Agents, we are going to create in just a few lines one of the simplest yet incredibly powerful agents. The agent will act as a Data Analyst Assistant and help us in analyzing data contained in any Excel file. It will be able to identify trends, use models, make forecasts. In summary, we are going to create a simple agent that we can use in our daily work to analyze our data.
Article | Notebook |
---|
In this example, two technologies seen previously are combined: agents and vector databases. Medical information is stored in ChromaDB, and a LangChain Agent is created, which will fetch it only when necessary to create an enriched prompt that will be sent to the model to answer the user's question.
In other words, a RAG system is created to assist a Medical ChatBot.
Attention!!! Use it only as an example. Nobody should take the boot's recommendations as those of a real doctor. I disclaim all responsibility for the use that may be given to the ChatBot. I have built it only as an example of different technologies.
Article | Notebook |
---|
The metrics used to measure the performance of Large Language Models are quite different from the ones we've been using in more traditional models. We're shifting away from metrics like Accuracy, F1 score, or recall, and moving towards metrics like BLEU, ROUGE, or METEOR.
These metrics are tailored to the specific task assigned to the language model.
In this section, we'll explore examples of several of these metrics and how to use them to determine whether one model is superior to another for a given task. We'll delve into practical scenarios where these metrics help us make informed decisions about the performance of different models.
Bleu is one of the first Metrics stablished to evaluate the quality of translations. In the notebook we compare the quality of a translation made by google with other from an Open Source Model from Hugging Face.
Article WIP | Notebook |
---|
We will explore the usage of the ROUGE metric to measure the quality of summaries generated by a language model. We are going to use two T5 models, one of them being the t5-Base model and the other a t5-base fine-tuned specifically designed for creating summaries.
Article | Notebook |
---|
In this initial example, you can observe how to use LangSmith to monitor the traffic between the various components that make up the Agent. The agent is a RAG system that utilizes a vectorial database to construct an enriched prompt and pass it to the model. LangSmith captures both the use of the Agent's tools and the decisions made by the model, providing information at all times about the sent/received data, consumed tokens, the duration of the query, and all of this in a truly user-friendly environment.
Article | Notebook |
---|
Previously in the notebook, Rouge Metrics: Evaluating Summaries, we learned how to use ROUGE to evaluate which summary best approximated the one created by a human. This time, we will use embedding distance and LangSmith to verify which model produces summaries more similar to the reference ones.
Article | Notebook |
---|
We take the agent that functions as a medical assistant and incorporate Giskard to evaluate if its responses are correct. In this way, not only the model's response is evaluated, but also the information retrieval in the vector database. Giskard is a solution that allows evaluating a complete RAG solution.
Article WIP | Notebook |
---|
In the FineTuning & Optimization section, we will explore different techniques such as Prompt Fine Tuning or LoRA, and we will use the Hugging Face PEFT library to efficiently fine-tune Large Language Models. We will explore techniques like quantization to reduce the weight of the Models.
In this notebook, two models are trained using Prompt Tuning from the PEFT library. This technique not only allows us to train by modifying the weights of very few parameters but also enables us to have different specialized models loaded in memory that use the same foundational model.
Prompt tuning is an additive technique, and the weights of the pre-trained model are not modified. The weights that we modify in this case are those of virtual tokens that we add to the prompt.
Article | Notebook |
---|
After a brief explanation of how the fine-tuning technique LoRA works, we will fine-tune a model from the Bloom family to teach it to construct prompts that can be used to instruct large language models.
Article | Notebook |
---|
We are going to see a brief introduction to quantization, used to reduce the size of big Large Language Models. With quantization, you can load big models reducing the memory resources needed. It also applies to the fine-tuning process, you can fine-tune the model in a single GPU without consuming all the resources. After the brief explanation we see an example about how is possible to fine-tune a Bloom 7B Model ina a t4 16GB GPU on Google Colab.
Article | Notebook |
---|
In this straightforward initial project, we are going to develop a SQL generator from natural language. We'll begin by creating the prompt to implement two solutions: one using OpenAI models running on Azure, and the other with an open-source model from Hugging Face.
Article | Notebook |
---|---|
Create a NL2SQL prompt for OpenAI | Prompt creation for OpenAI |
WIP | Prompt creation for defog/SQLCoder |
Inference Azure Configuration. | Using Azure Inference Point |
In this small project we will create a new model aligning a microsoft-phi-3-model with DPO and then publish it to Hugging Face.
Article | Notebook |
---|---|
WIP | Aligning with DPO a phi3-3 model. |
In this initial solution, we design an architecture for an NL2SQL system capable of operating on a large database. The system is intended to be used with two or three different models. In fact, we use three models in the example.
It's an architecture that enables a fast project kickoff, providing service for only a few tables in the database, allowing us to add more tables at our pace.
In this solution, we explore the transformative power of embeddings and large language models (LLMs) in customer risk assessment and product recommendation in the financial industry. We'll be altering the format in which we store customer information, and consequently, we'll also be changing how this information travels within the systems, achieving important advantages.
Please, if you find any problems, open an issue . I will do my best to fix it as soon as possible, and give you credit.
If you'd like to make a contribution or suggest a topic, please don't hesitate to start a Discussion. I'd be delighted to receive any opinions or advice.
Don't be shy, share the course on your social networks with your friends. Connect with me on LinkedIn or Twitter and feel free to share anything you'd like or ask any questions you may have.
Give a Star โญ๏ธ to the repository. It helps me a lot, and encourages me to continue adding lessons. It's a nice way to support free Open Source courses like this one.
Large Language Models Are State-of-the-Art Evaluators of Translation Quality. Evaluating LLMs with LLMs.
ReAct: Synergizing Reasoning and Acting in Language Models. LangChain & Agents Section. Medical Assistant Sample.
The Power of Scale for Parameter-Efficient Prompt Tuning. Fine Tuning & Optimization Section. Prompt Tuning Sample.
LoRA: Low-Rank Adaptation of Large Language Models. Fine Tuning & Optimization Section. LoRA Fine-Tuning Sample.
QLoRA: Efficient Finetuning of Quantized LLMs. Fine Tuning & Optimization Section. QLoRA Fine-Tuning Sample.
How to Prompt LLMs for Text-to-SQL: A Study in Zero-shot, Single-domain, and Cross-domain Settings. Project. Natural Language to SQL.
For Tasks:
Click tags to check more tools for each tasksFor Jobs:
Alternative AI tools for Large-Language-Model-Notebooks-Course
Similar Open Source Tools
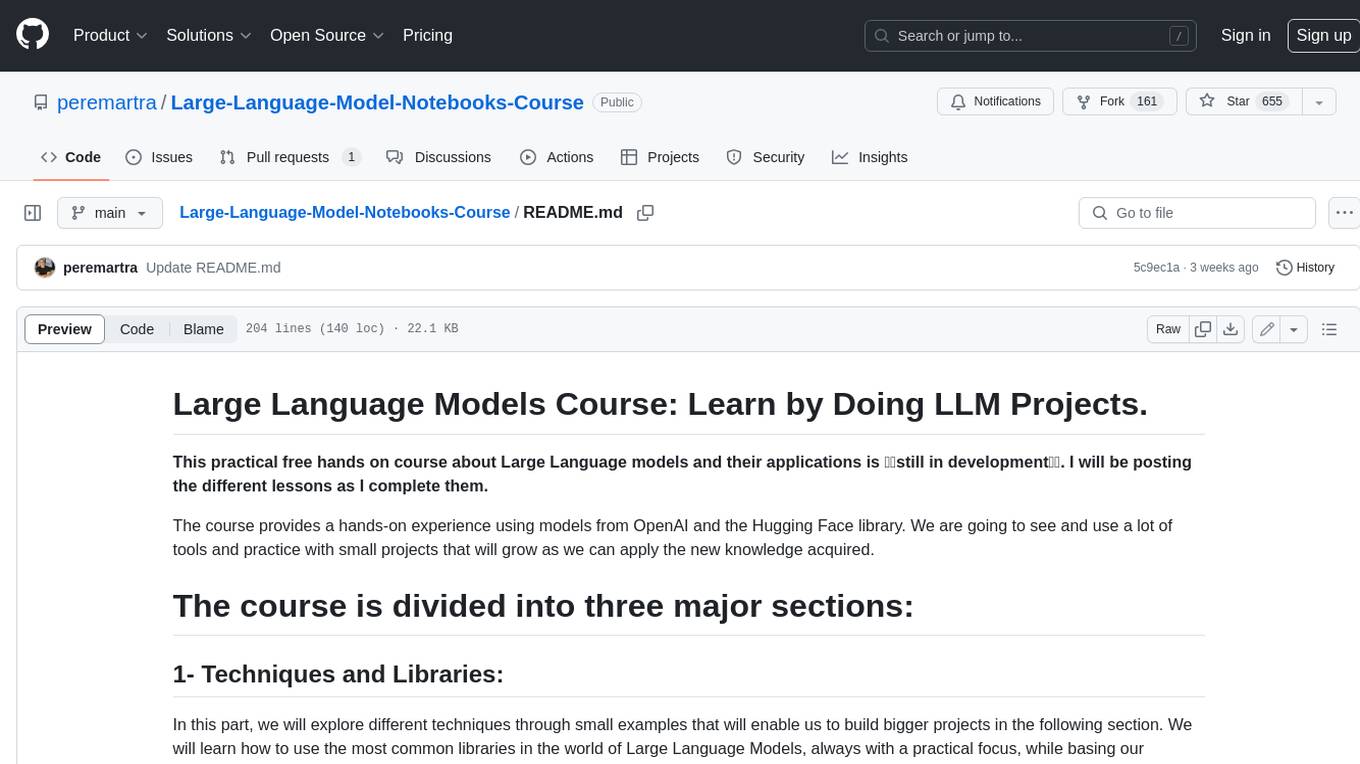
Large-Language-Model-Notebooks-Course
This practical free hands-on course focuses on Large Language models and their applications, providing a hands-on experience using models from OpenAI and the Hugging Face library. The course is divided into three major sections: Techniques and Libraries, Projects, and Enterprise Solutions. It covers topics such as Chatbots, Code Generation, Vector databases, LangChain, Fine Tuning, PEFT Fine Tuning, Soft Prompt tuning, LoRA, QLoRA, Evaluate Models, Knowledge Distillation, and more. Each section contains chapters with lessons supported by notebooks and articles. The course aims to help users build projects and explore enterprise solutions using Large Language Models.
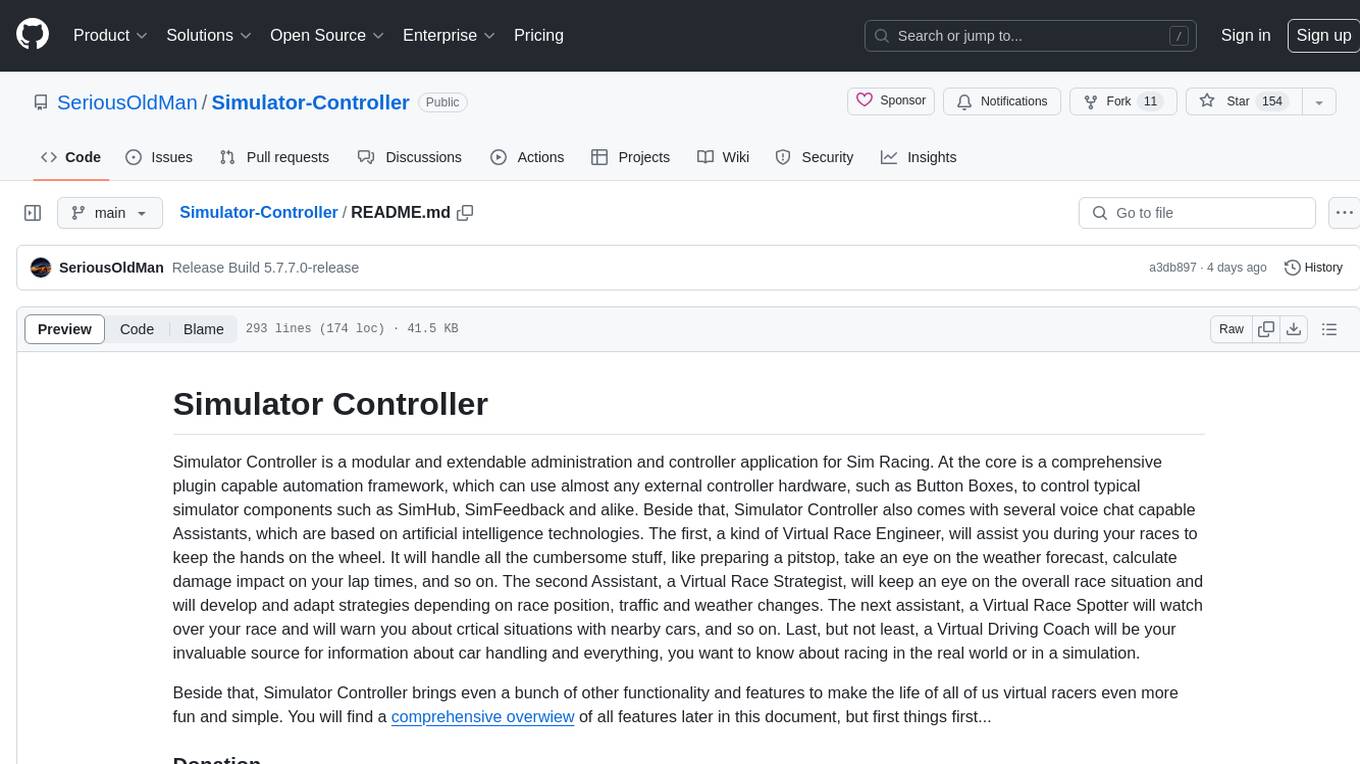
Simulator-Controller
Simulator Controller is a modular administration and controller application for Sim Racing, featuring a comprehensive plugin automation framework for external controller hardware. It includes voice chat capable Assistants like Virtual Race Engineer, Race Strategist, Race Spotter, and Driving Coach. The tool offers features for setup, strategy development, monitoring races, and more. Developed in AutoHotkey, it supports various simulation games and integrates with third-party applications for enhanced functionality.
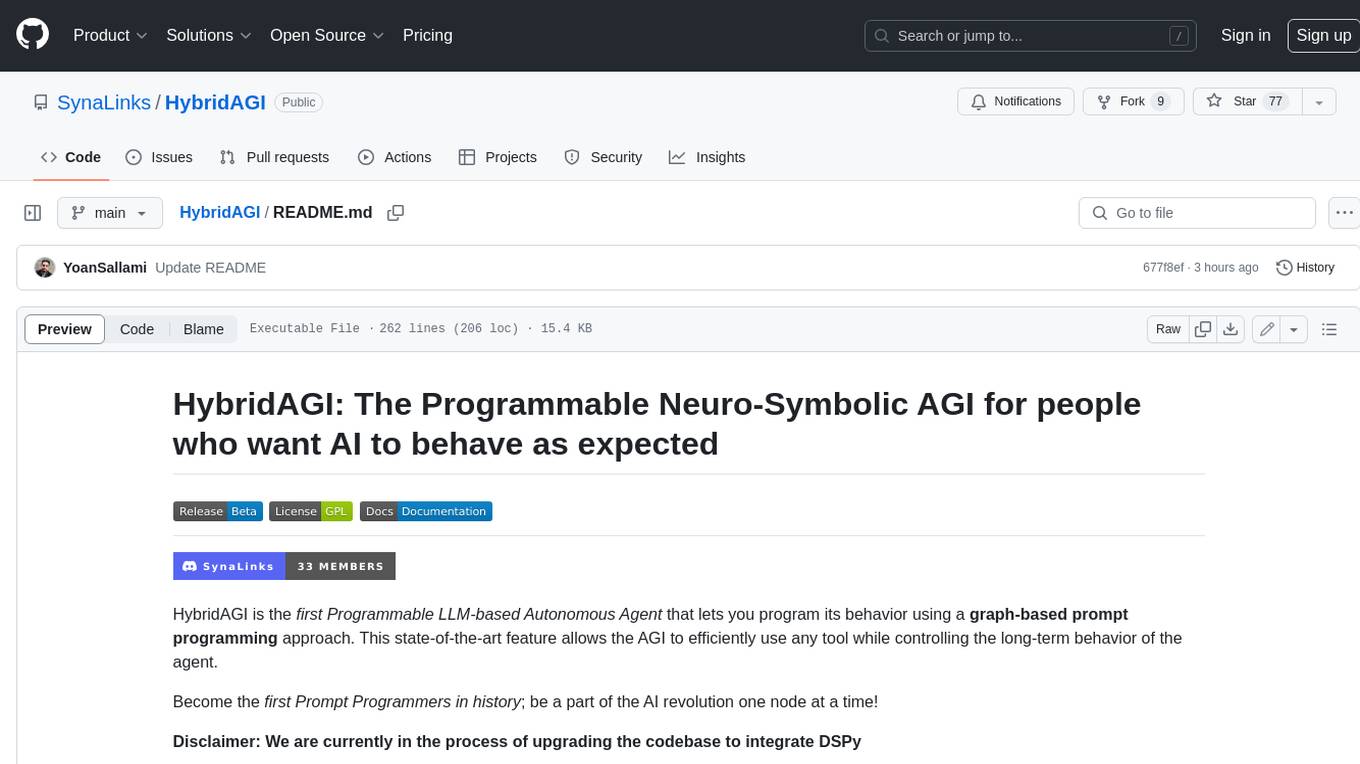
HybridAGI
HybridAGI is the first Programmable LLM-based Autonomous Agent that lets you program its behavior using a **graph-based prompt programming** approach. This state-of-the-art feature allows the AGI to efficiently use any tool while controlling the long-term behavior of the agent. Become the _first Prompt Programmers in history_ ; be a part of the AI revolution one node at a time! **Disclaimer: We are currently in the process of upgrading the codebase to integrate DSPy**
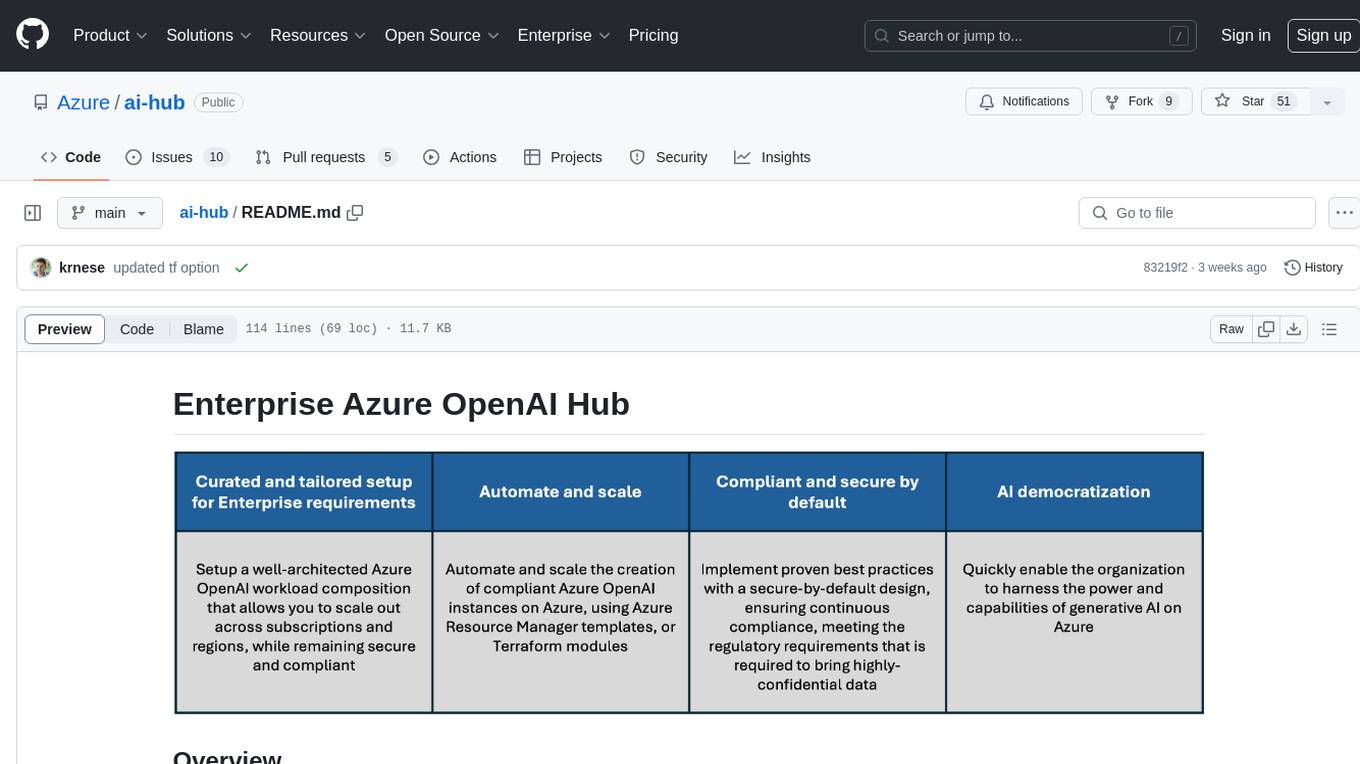
ai-hub
The Enterprise Azure OpenAI Hub is a comprehensive repository designed to guide users through the world of Generative AI on the Azure platform. It offers a structured learning experience to accelerate the transition from concept to production in an Enterprise context. The hub empowers users to explore various use cases with Azure services, ensuring security and compliance. It provides real-world examples and playbooks for practical insights into solving complex problems and developing cutting-edge AI solutions. The repository also serves as a library of proven patterns, aligning with industry standards and promoting best practices for secure and compliant AI development.
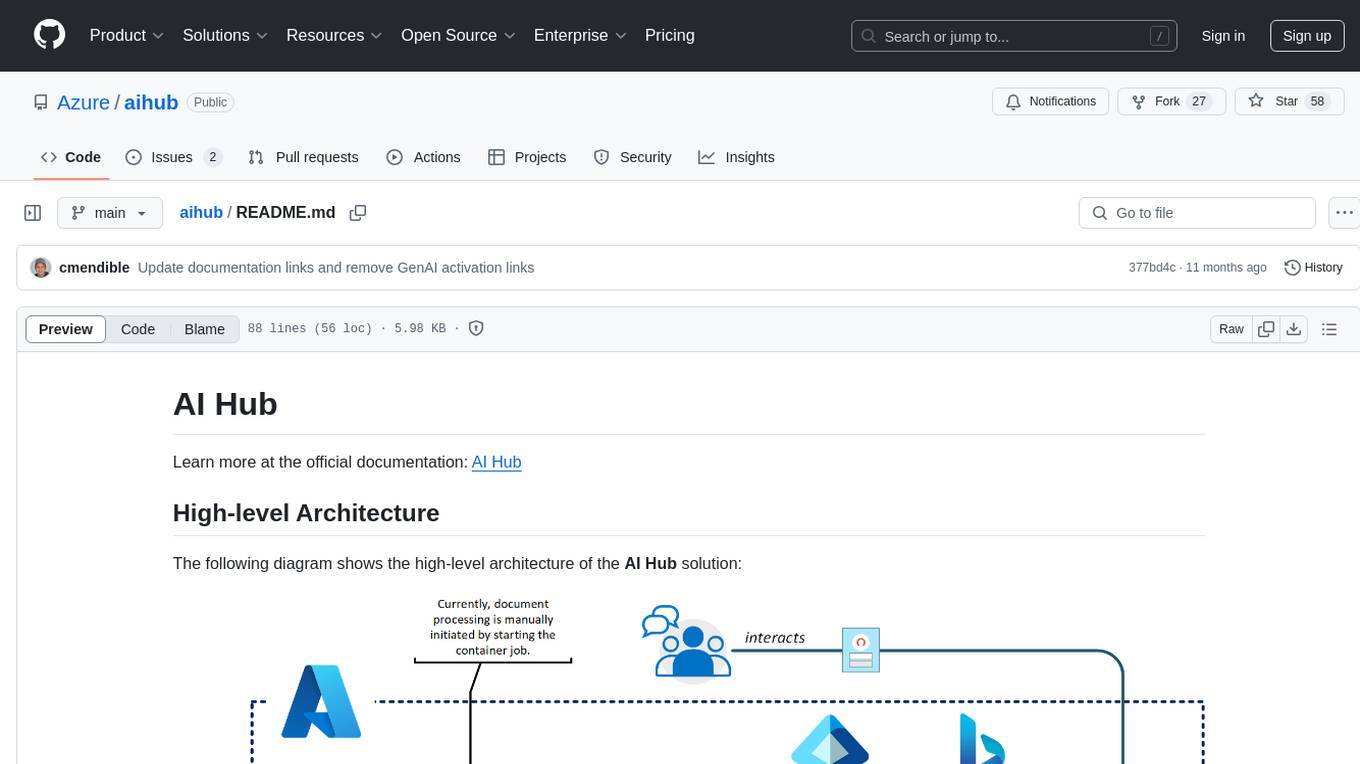
aihub
AI Hub is a comprehensive solution that leverages artificial intelligence and cloud computing to provide functionalities such as document search and retrieval, call center analytics, image analysis, brand reputation analysis, form analysis, document comparison, and content safety moderation. It integrates various Azure services like Cognitive Search, ChatGPT, Azure Vision Services, and Azure Document Intelligence to offer scalable, extensible, and secure AI-powered capabilities for different use cases and scenarios.
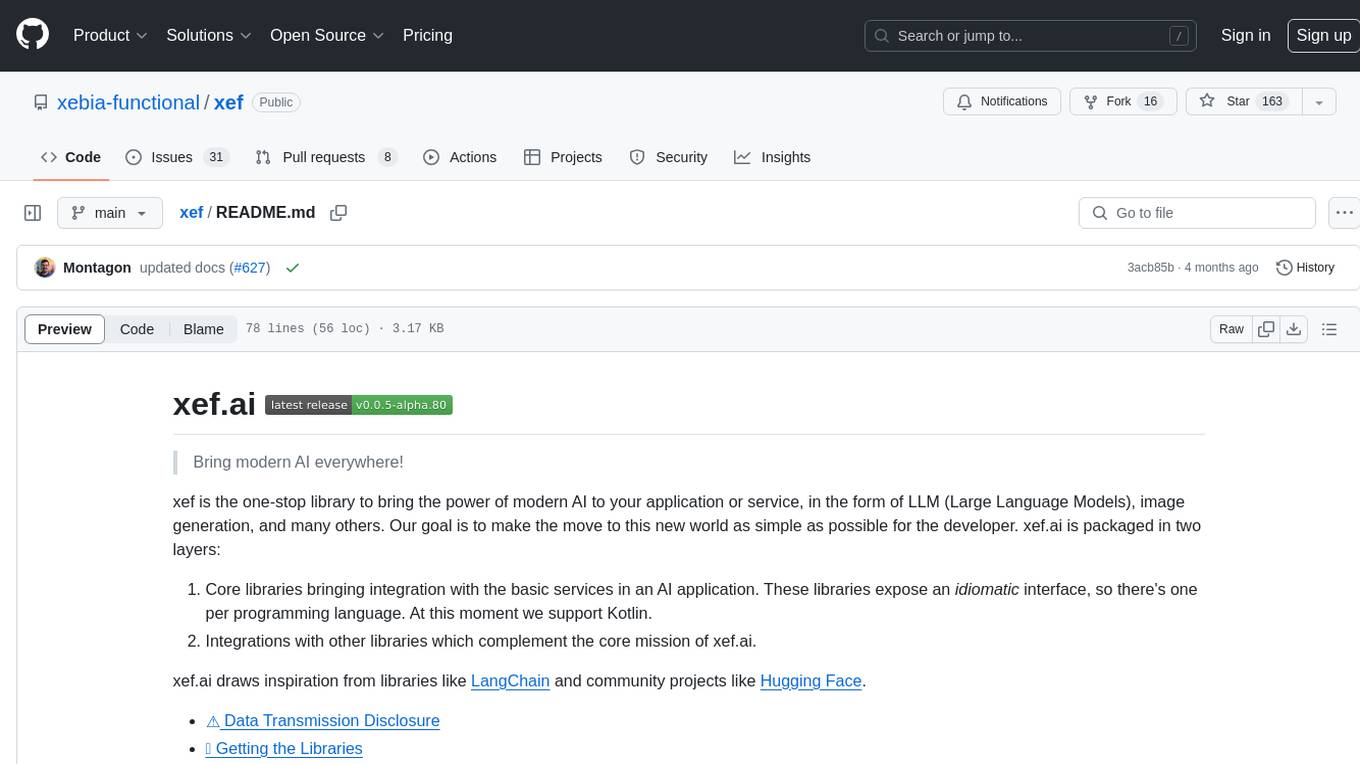
xef
xef.ai is a one-stop library designed to bring the power of modern AI to applications and services. It offers integration with Large Language Models (LLM), image generation, and other AI services. The library is packaged in two layers: core libraries for basic AI services integration and integrations with other libraries. xef.ai aims to simplify the transition to modern AI for developers by providing an idiomatic interface, currently supporting Kotlin. Inspired by LangChain and Hugging Face, xef.ai may transmit source code and user input data to third-party services, so users should review privacy policies and take precautions. Libraries are available in Maven Central under the `com.xebia` group, with `xef-core` as the core library. Developers can add these libraries to their projects and explore examples to understand usage.
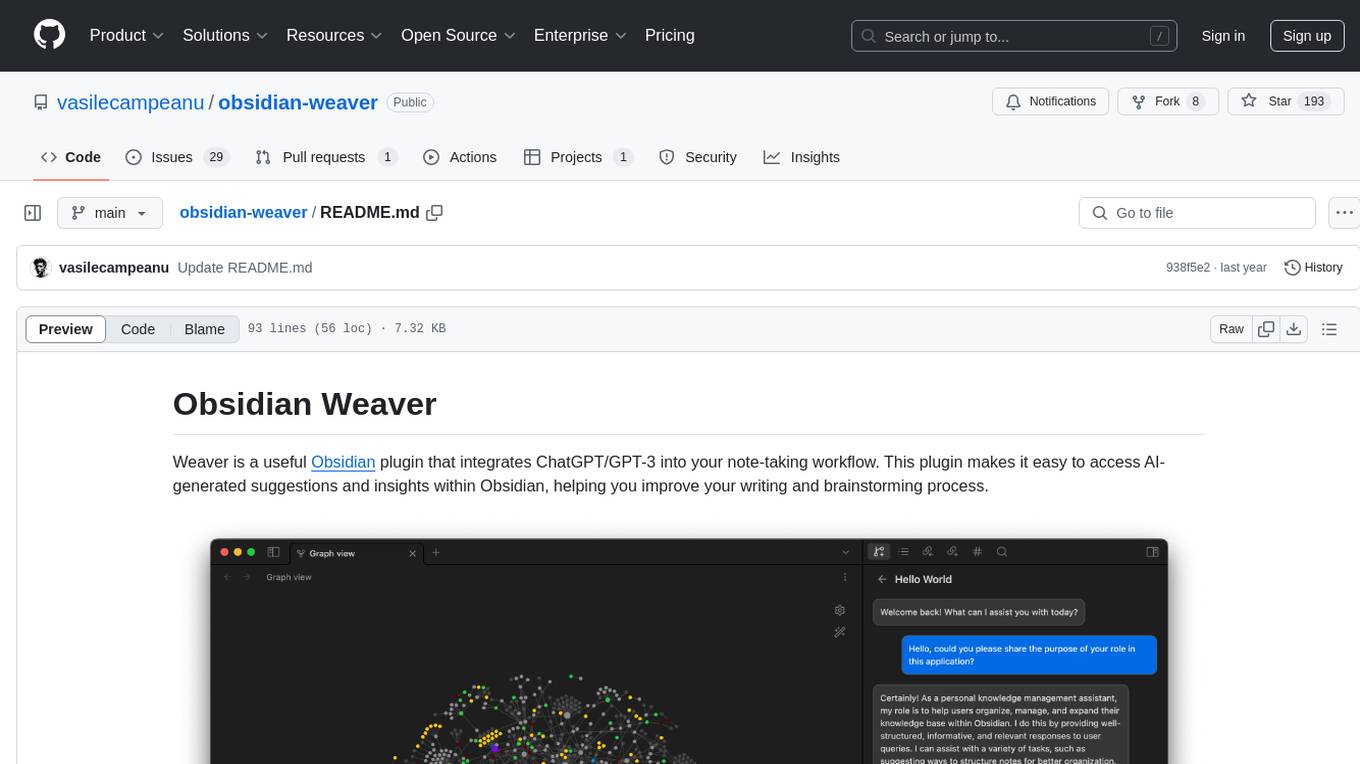
obsidian-weaver
Obsidian Weaver is a plugin that integrates ChatGPT/GPT-3 into the note-taking workflow of Obsidian. It allows users to easily access AI-generated suggestions and insights within Obsidian, enhancing the writing and brainstorming process. The plugin respects Obsidian's philosophy of storing notes locally, ensuring data security and privacy. Weaver offers features like creating new chat sessions with the AI assistant and receiving instant responses, all within the Obsidian environment. It provides a seamless integration with Obsidian's interface, making the writing process efficient and helping users stay focused. The plugin is constantly being improved with new features and updates to enhance the note-taking experience.
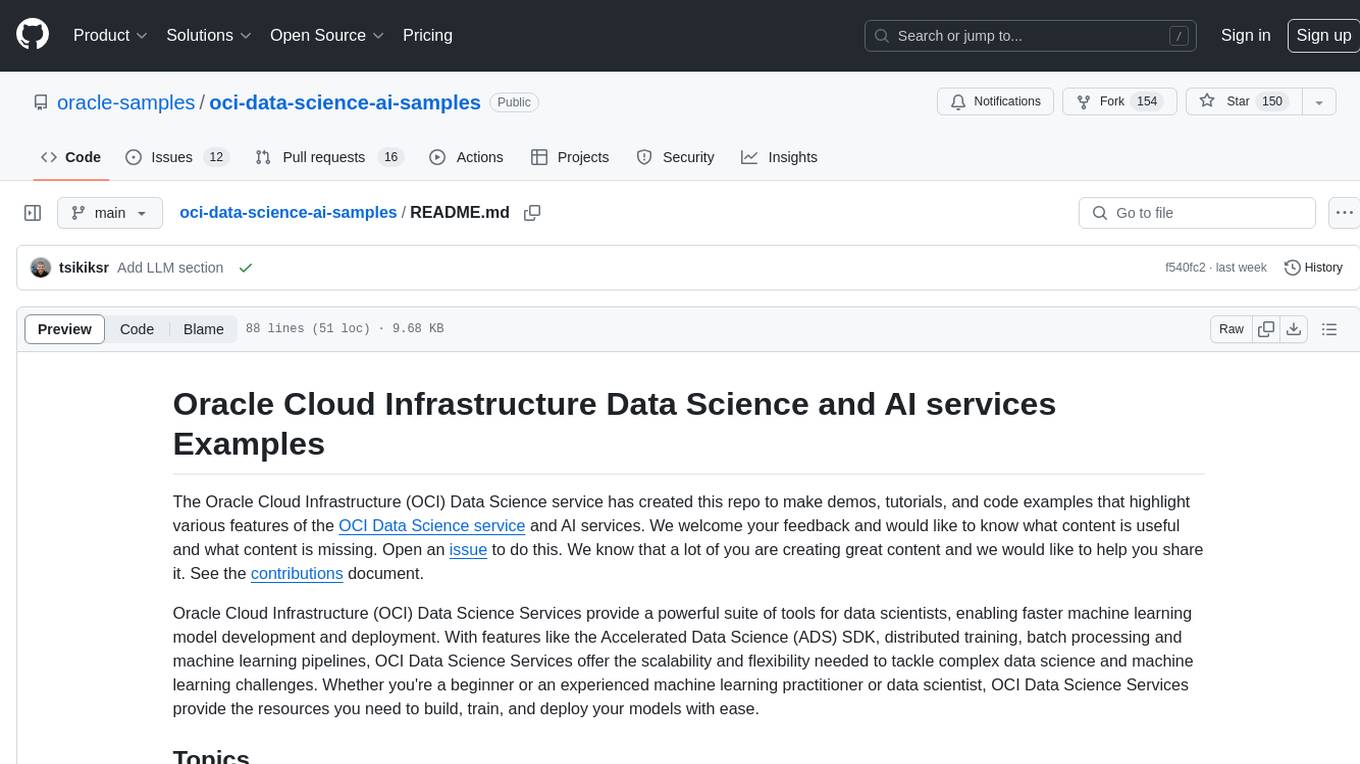
oci-data-science-ai-samples
The Oracle Cloud Infrastructure Data Science and AI services Examples repository provides demos, tutorials, and code examples showcasing various features of the OCI Data Science service and AI services. It offers tools for data scientists to develop and deploy machine learning models efficiently, with features like Accelerated Data Science SDK, distributed training, batch processing, and machine learning pipelines. Whether you're a beginner or an experienced practitioner, OCI Data Science Services provide the resources needed to build, train, and deploy models easily.
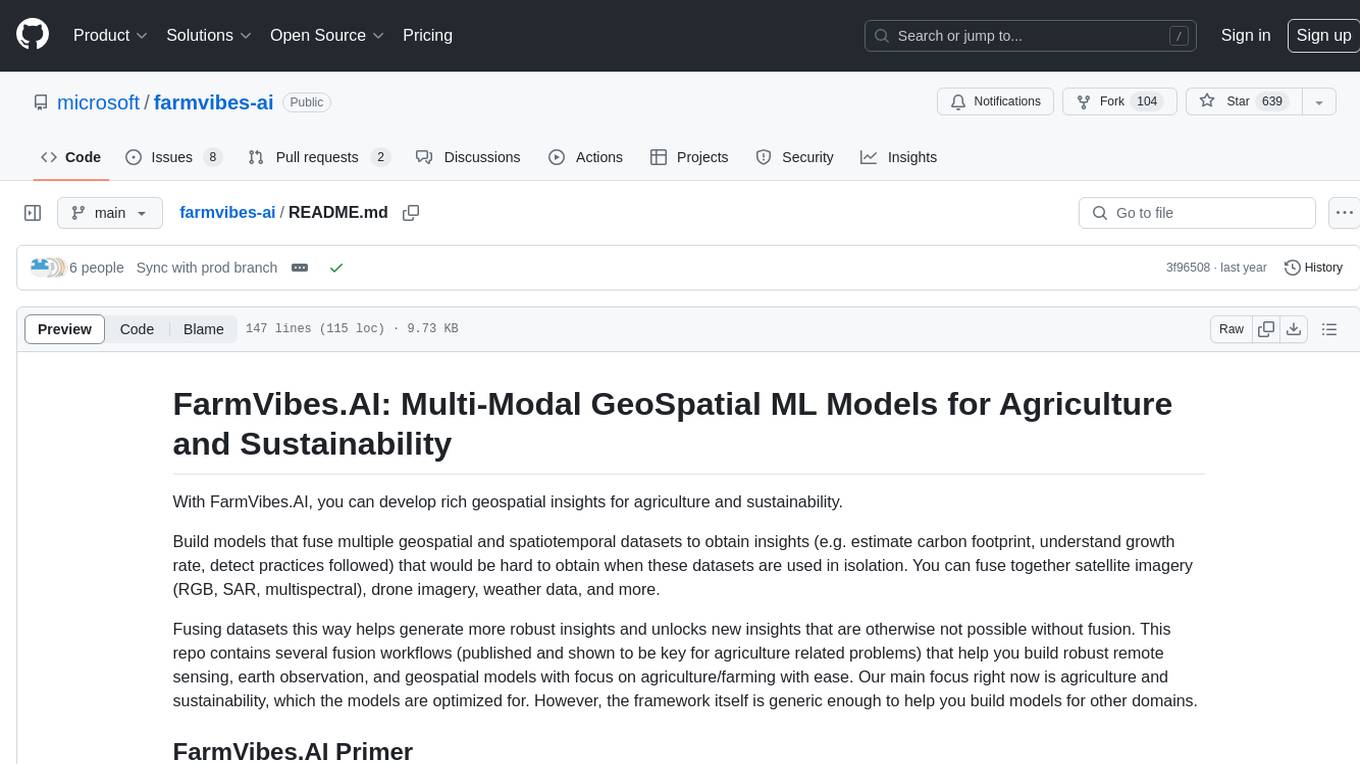
farmvibes-ai
FarmVibes.AI is a repository focused on developing multi-modal geospatial machine learning models for agriculture and sustainability. It enables users to fuse various geospatial and spatiotemporal datasets, such as satellite imagery, drone imagery, and weather data, to generate robust insights for agriculture-related problems. The repository provides fusion workflows, data preparation tools, model training notebooks, and an inference engine to facilitate the creation of geospatial models tailored for agriculture and farming. Users can interact with the tools via a local cluster, REST API, or a Python client, and the repository includes documentation and notebook examples to guide users in utilizing FarmVibes.AI for tasks like harvest date detection, climate impact estimation, micro climate prediction, and crop identification.
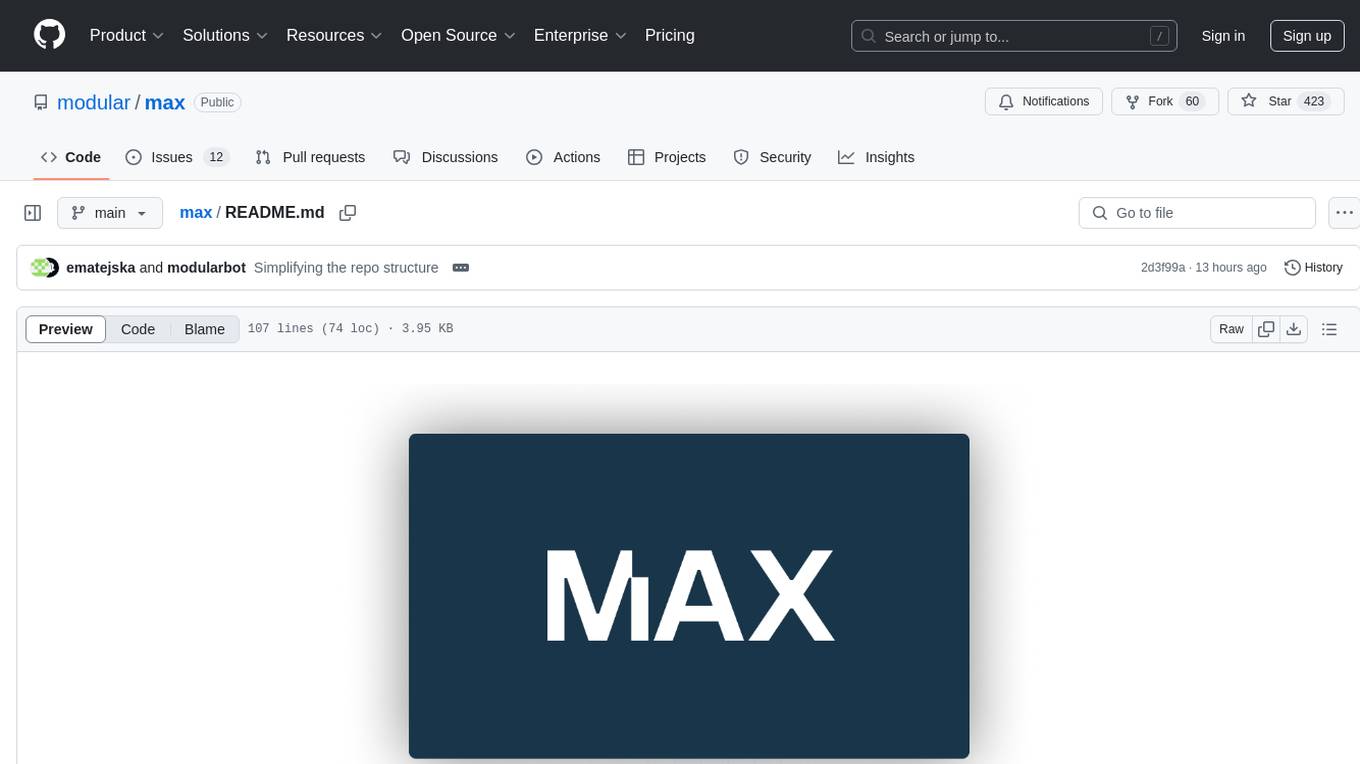
max
The Modular Accelerated Xecution (MAX) platform is an integrated suite of AI libraries, tools, and technologies that unifies commonly fragmented AI deployment workflows. MAX accelerates time to market for the latest innovations by giving AI developers a single toolchain that unlocks full programmability, unparalleled performance, and seamless hardware portability.
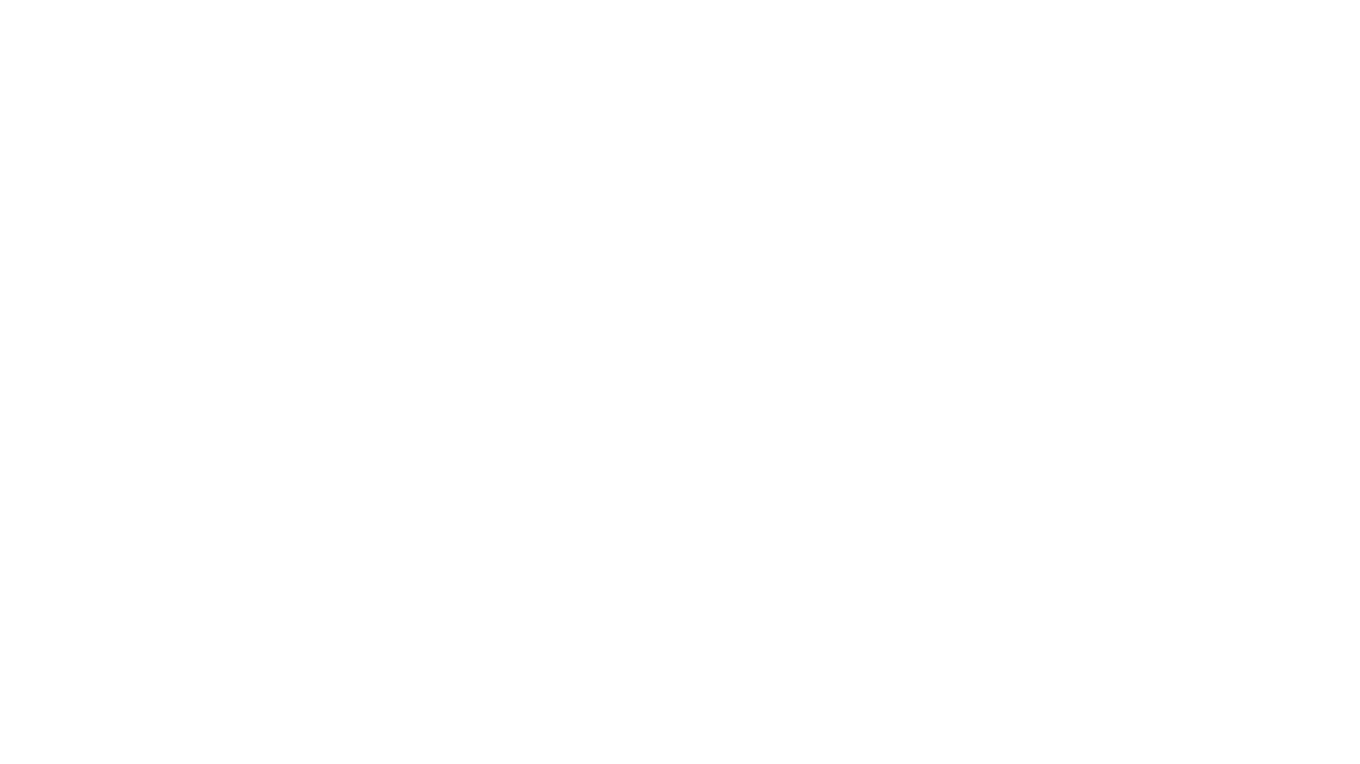
NaLLM
The NaLLM project repository explores the synergies between Neo4j and Large Language Models (LLMs) through three primary use cases: Natural Language Interface to a Knowledge Graph, Creating a Knowledge Graph from Unstructured Data, and Generating a Report using static and LLM data. The repository contains backend and frontend code organized for easy navigation. It includes blog posts, a demo database, instructions for running demos, and guidelines for contributing. The project aims to showcase the potential of Neo4j and LLMs in various applications.
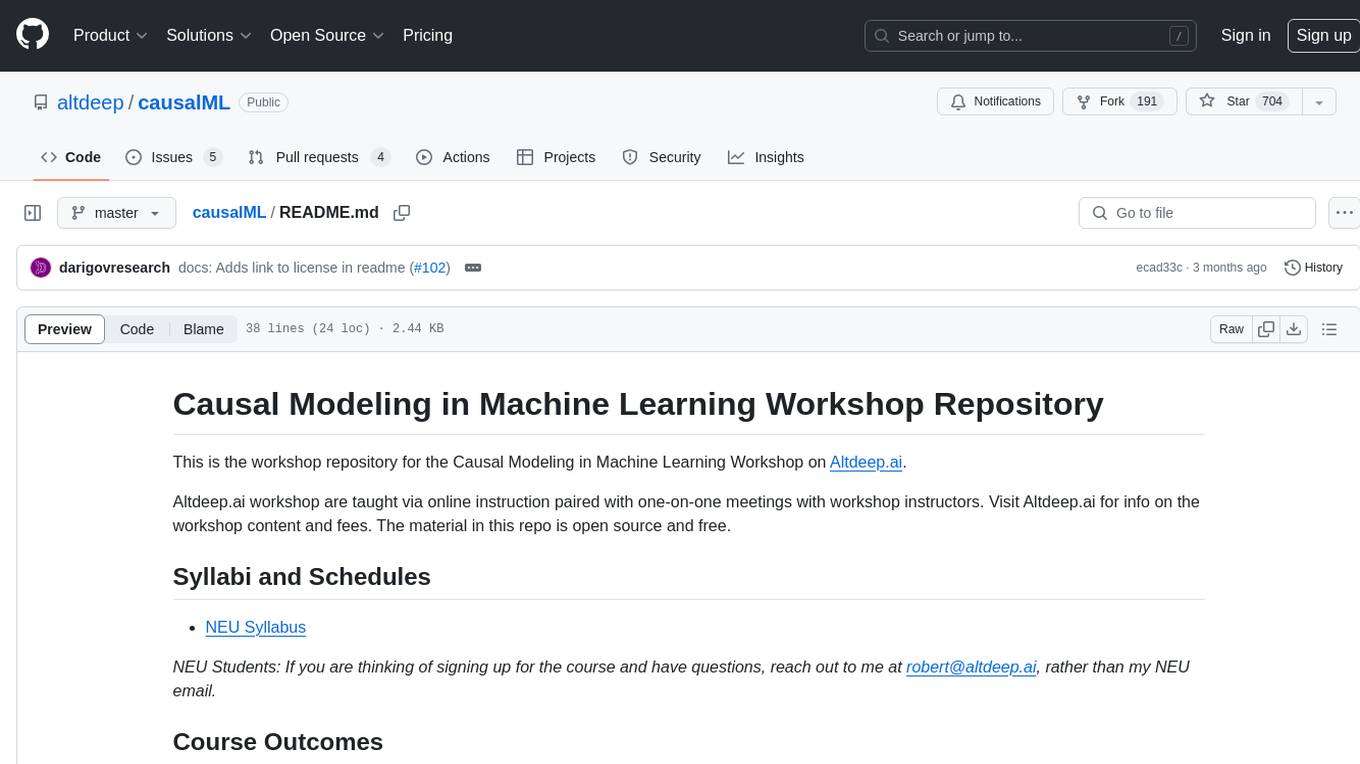
causalML
This repository is the workshop repository for the Causal Modeling in Machine Learning Workshop on Altdeep.ai. The material is open source and free. The course covers causality in model-based machine learning, Bayesian modeling, interventions, counterfactual reasoning, and deep causal latent variable models. It aims to equip learners with the ability to build causal reasoning algorithms into decision-making systems in data science and machine learning teams within top-tier technology organizations.
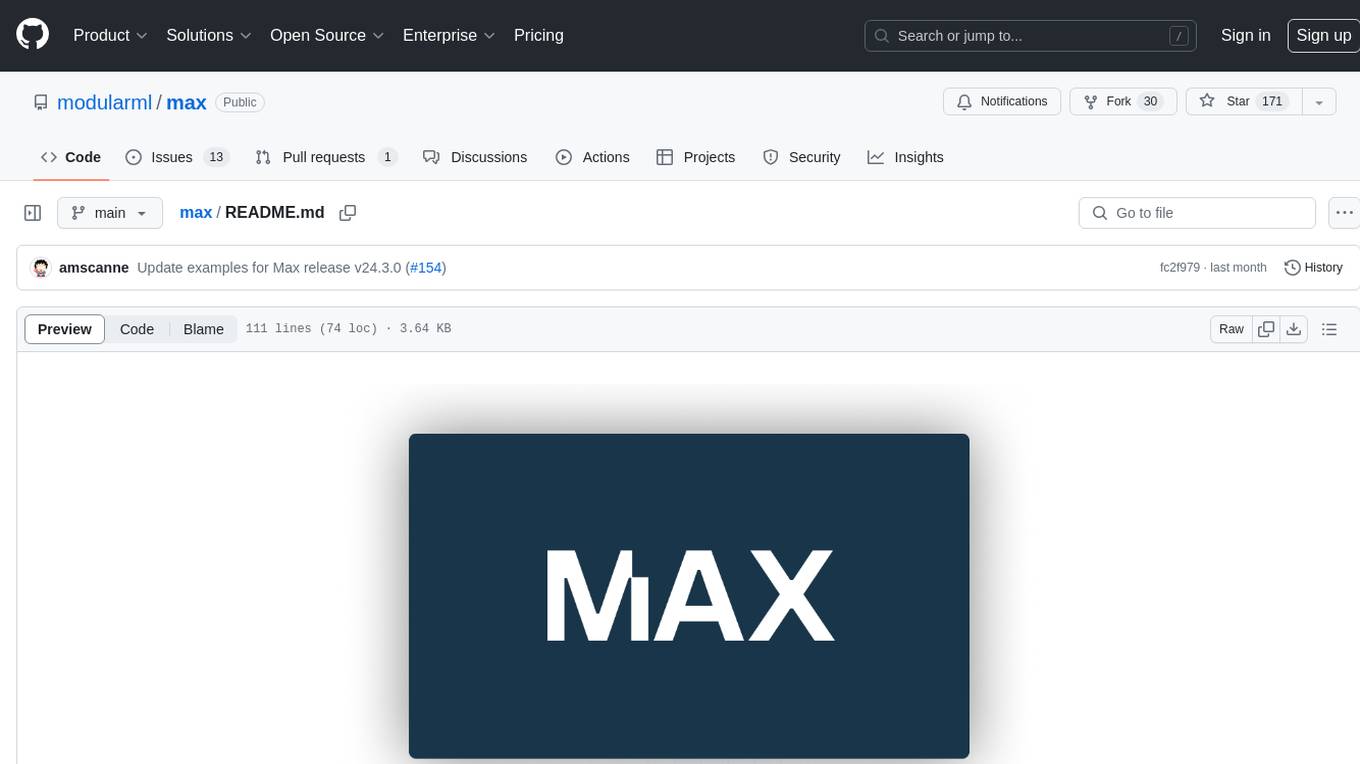
max
The Modular Accelerated Xecution (MAX) platform is an integrated suite of AI libraries, tools, and technologies that unifies commonly fragmented AI deployment workflows. MAX accelerates time to market for the latest innovations by giving AI developers a single toolchain that unlocks full programmability, unparalleled performance, and seamless hardware portability.
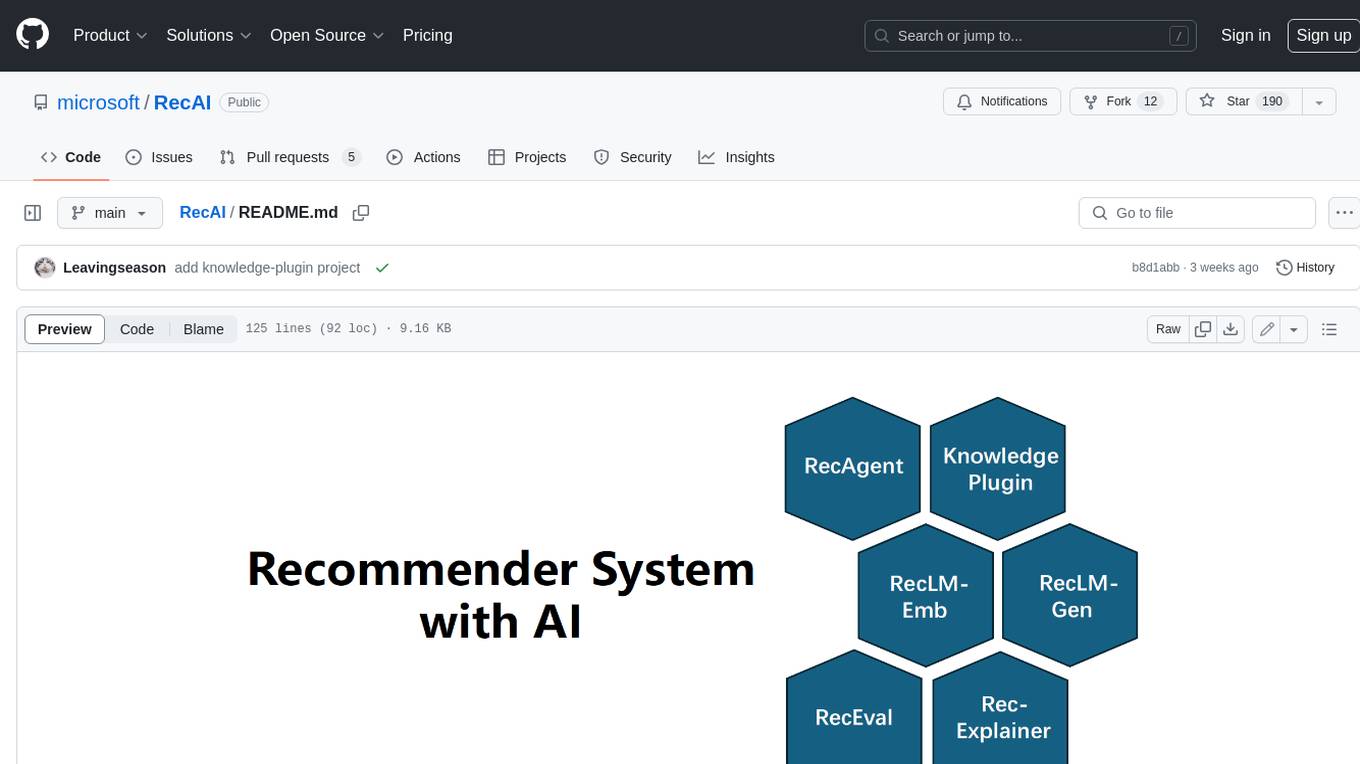
RecAI
RecAI is a project that explores the integration of Large Language Models (LLMs) into recommender systems, addressing the challenges of interactivity, explainability, and controllability. It aims to bridge the gap between general-purpose LLMs and domain-specific recommender systems, providing a holistic perspective on the practical requirements of LLM4Rec. The project investigates various techniques, including Recommender AI agents, selective knowledge injection, fine-tuning language models, evaluation, and LLMs as model explainers, to create more sophisticated, interactive, and user-centric recommender systems.
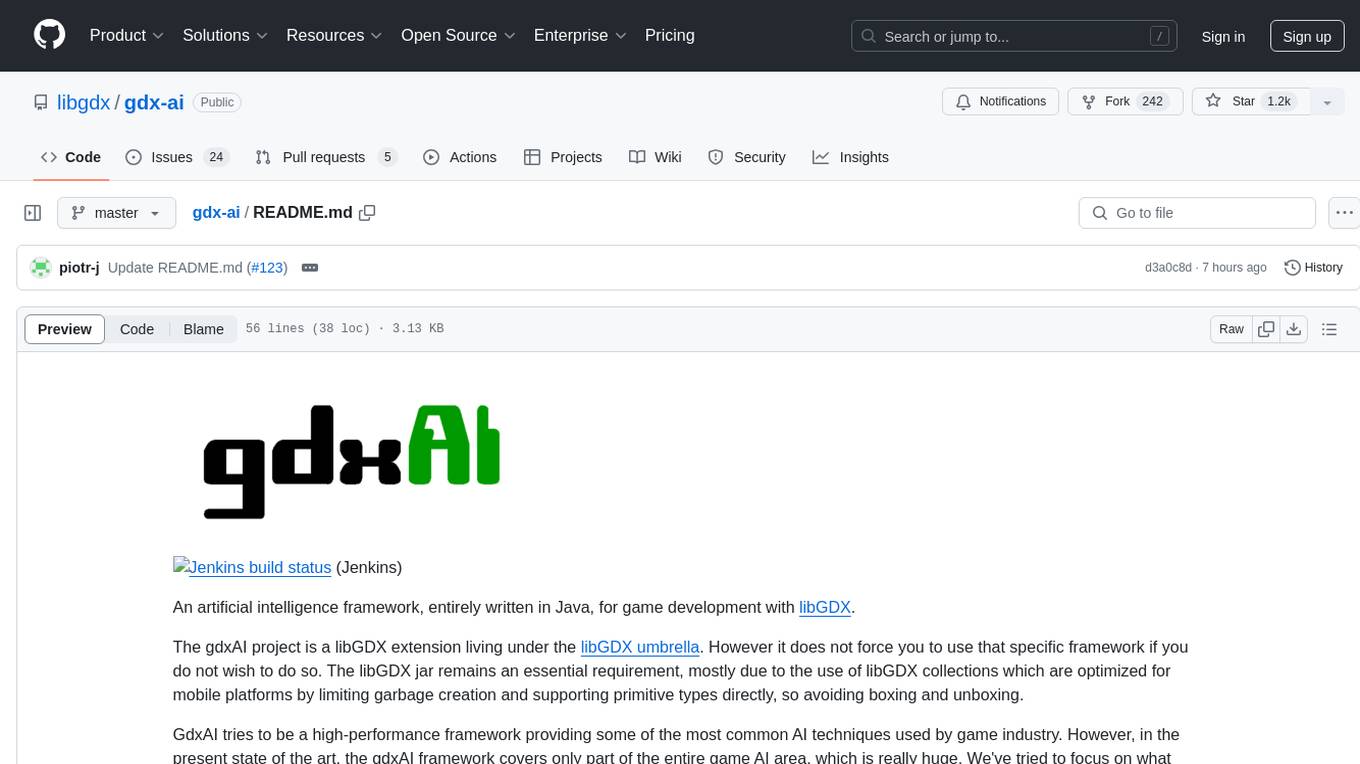
gdx-ai
An artificial intelligence framework entirely written in Java for game development with libGDX. It is a high-performance framework providing common AI techniques used in the game industry, covering movement AI, pathfinding, decision making, and infrastructure. The framework is designed to be used with libGDX but can be used independently. Current features include steering behaviors, formation motion, A* pathfinding, hierarchical pathfinding, behavior trees, state machine, message handling, and scheduling.
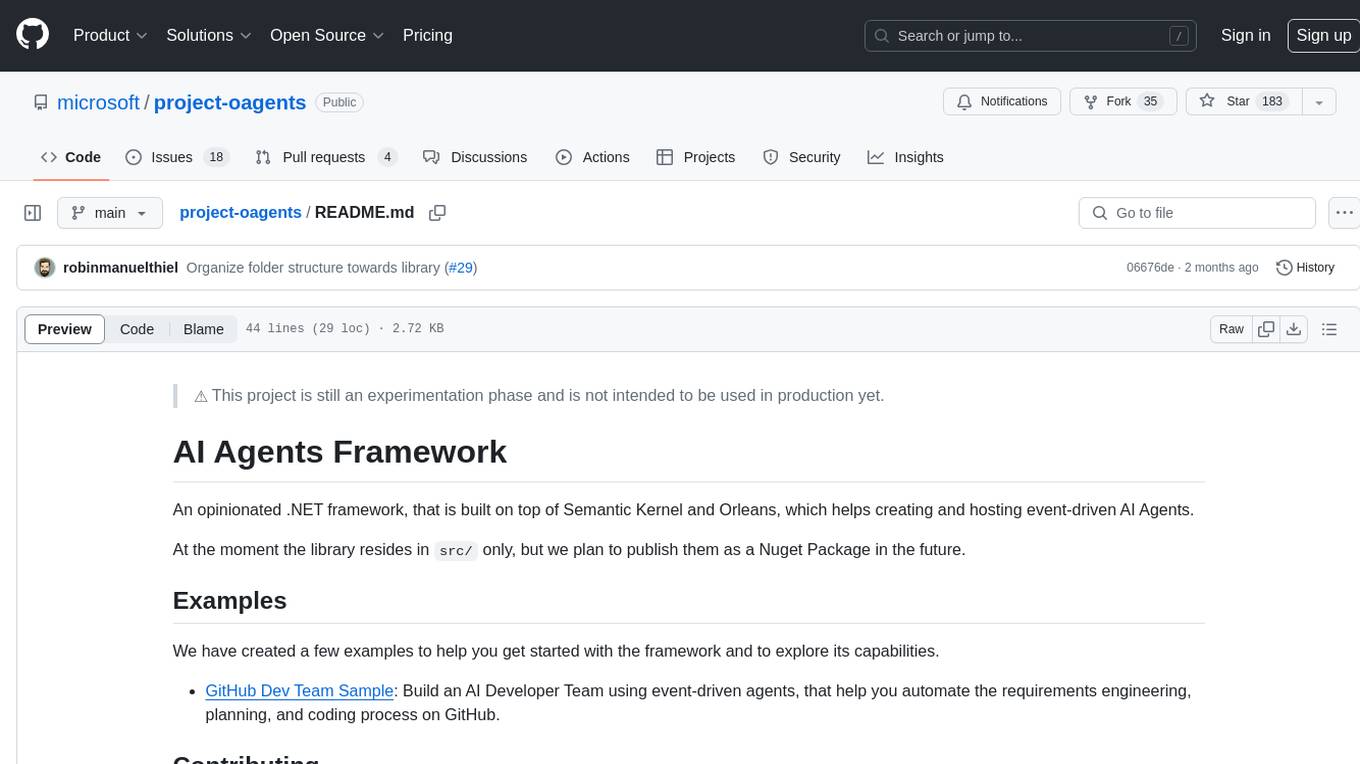
project-oagents
AI Agents Framework is a .NET framework built on Semantic Kernel and Orleans for creating and hosting event-driven AI Agents. It is currently in an experimental phase and not recommended for production use. The framework aims to automate requirements engineering, planning, and coding processes using event-driven agents.
For similar tasks
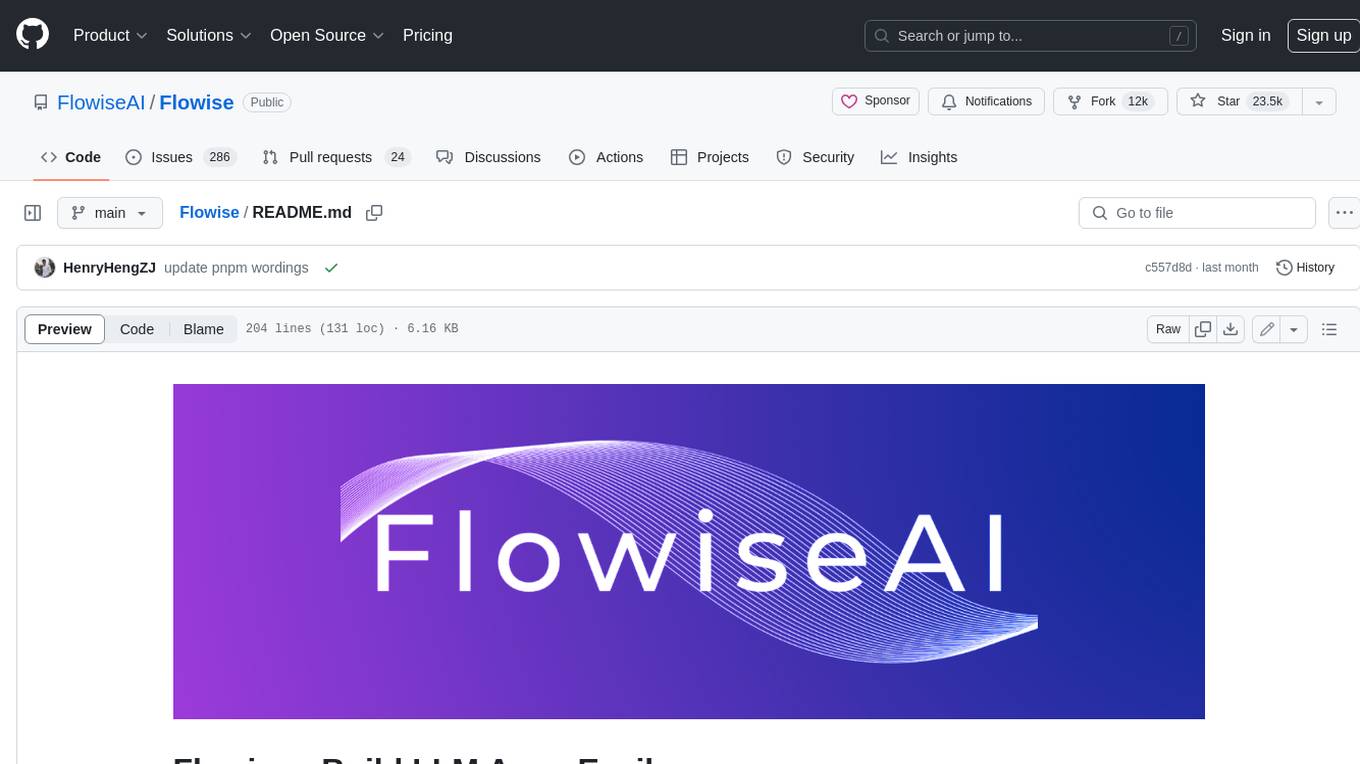
Flowise
Flowise is a tool that allows users to build customized LLM flows with a drag-and-drop UI. It is open-source and self-hostable, and it supports various deployments, including AWS, Azure, Digital Ocean, GCP, Railway, Render, HuggingFace Spaces, Elestio, Sealos, and RepoCloud. Flowise has three different modules in a single mono repository: server, ui, and components. The server module is a Node backend that serves API logics, the ui module is a React frontend, and the components module contains third-party node integrations. Flowise supports different environment variables to configure your instance, and you can specify these variables in the .env file inside the packages/server folder.
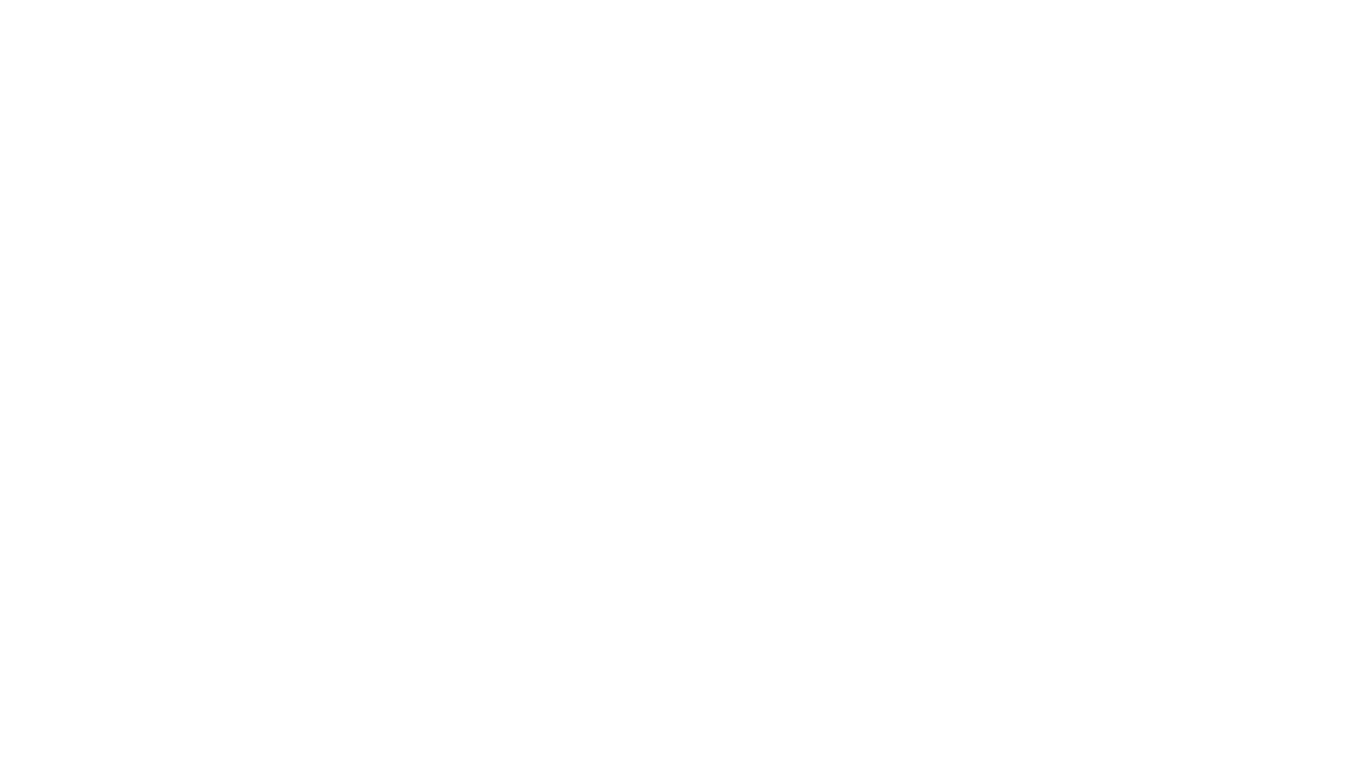
nlux
nlux is an open-source Javascript and React JS library that makes it super simple to integrate powerful large language models (LLMs) like ChatGPT into your web app or website. With just a few lines of code, you can add conversational AI capabilities and interact with your favourite LLM.
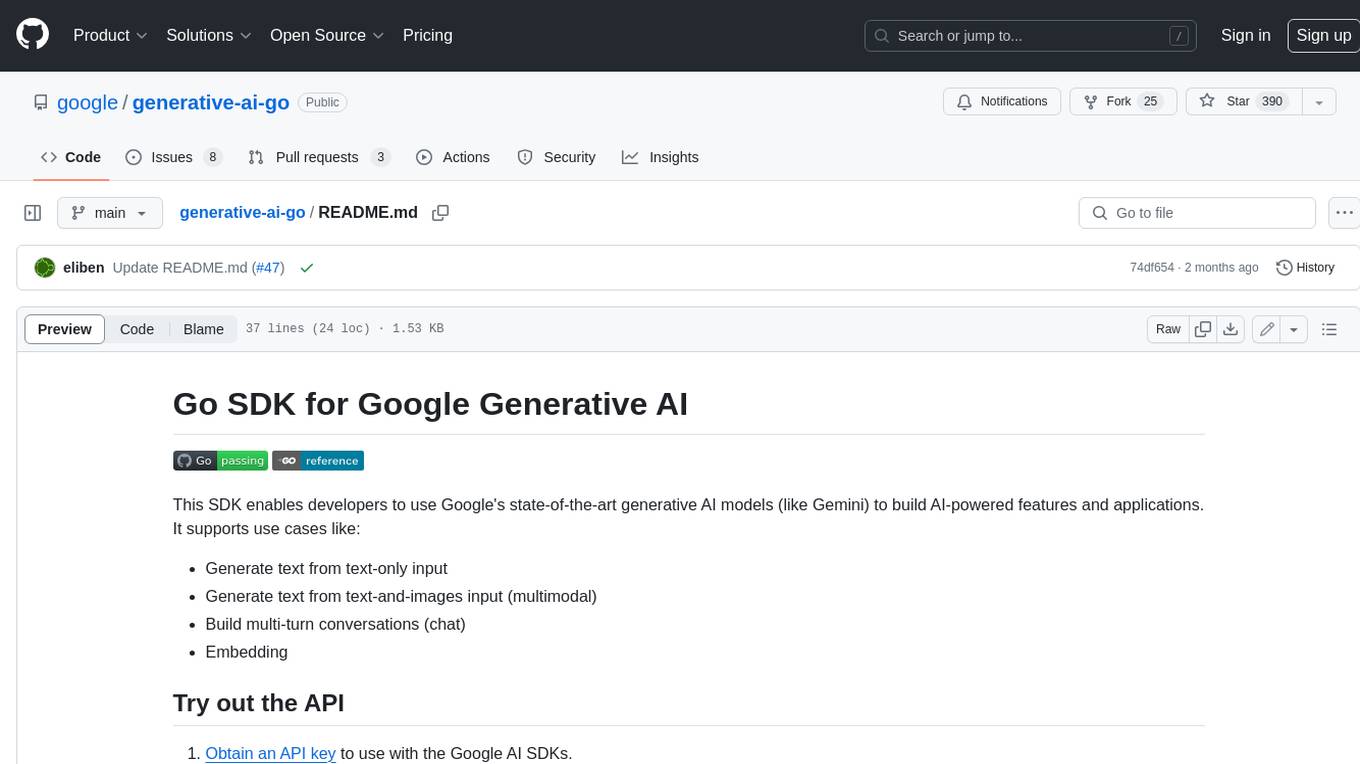
generative-ai-go
The Google AI Go SDK enables developers to use Google's state-of-the-art generative AI models (like Gemini) to build AI-powered features and applications. It supports use cases like generating text from text-only input, generating text from text-and-images input (multimodal), building multi-turn conversations (chat), and embedding.
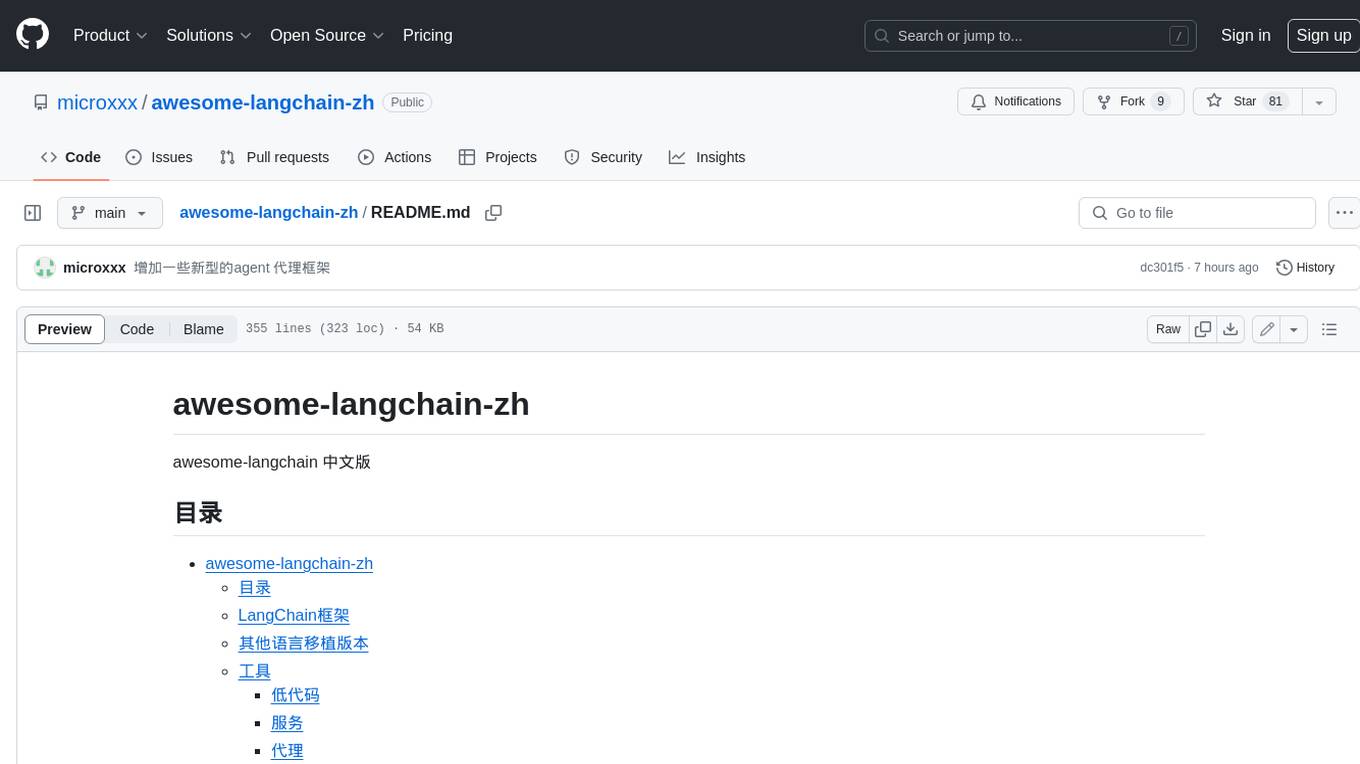
awesome-langchain-zh
The awesome-langchain-zh repository is a collection of resources related to LangChain, a framework for building AI applications using large language models (LLMs). The repository includes sections on the LangChain framework itself, other language ports of LangChain, tools for low-code development, services, agents, templates, platforms, open-source projects related to knowledge management and chatbots, as well as learning resources such as notebooks, videos, and articles. It also covers other LLM frameworks and provides additional resources for exploring and working with LLMs. The repository serves as a comprehensive guide for developers and AI enthusiasts interested in leveraging LangChain and LLMs for various applications.
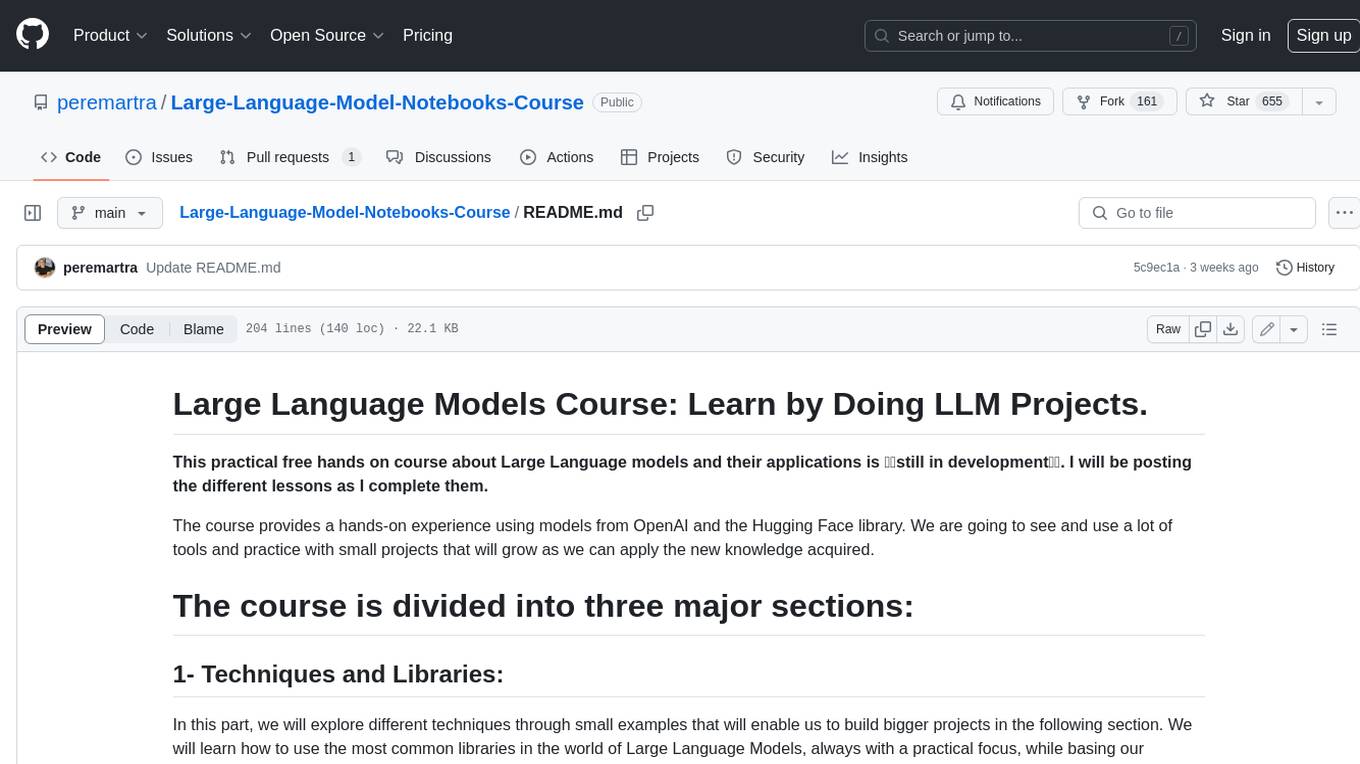
Large-Language-Model-Notebooks-Course
This practical free hands-on course focuses on Large Language models and their applications, providing a hands-on experience using models from OpenAI and the Hugging Face library. The course is divided into three major sections: Techniques and Libraries, Projects, and Enterprise Solutions. It covers topics such as Chatbots, Code Generation, Vector databases, LangChain, Fine Tuning, PEFT Fine Tuning, Soft Prompt tuning, LoRA, QLoRA, Evaluate Models, Knowledge Distillation, and more. Each section contains chapters with lessons supported by notebooks and articles. The course aims to help users build projects and explore enterprise solutions using Large Language Models.
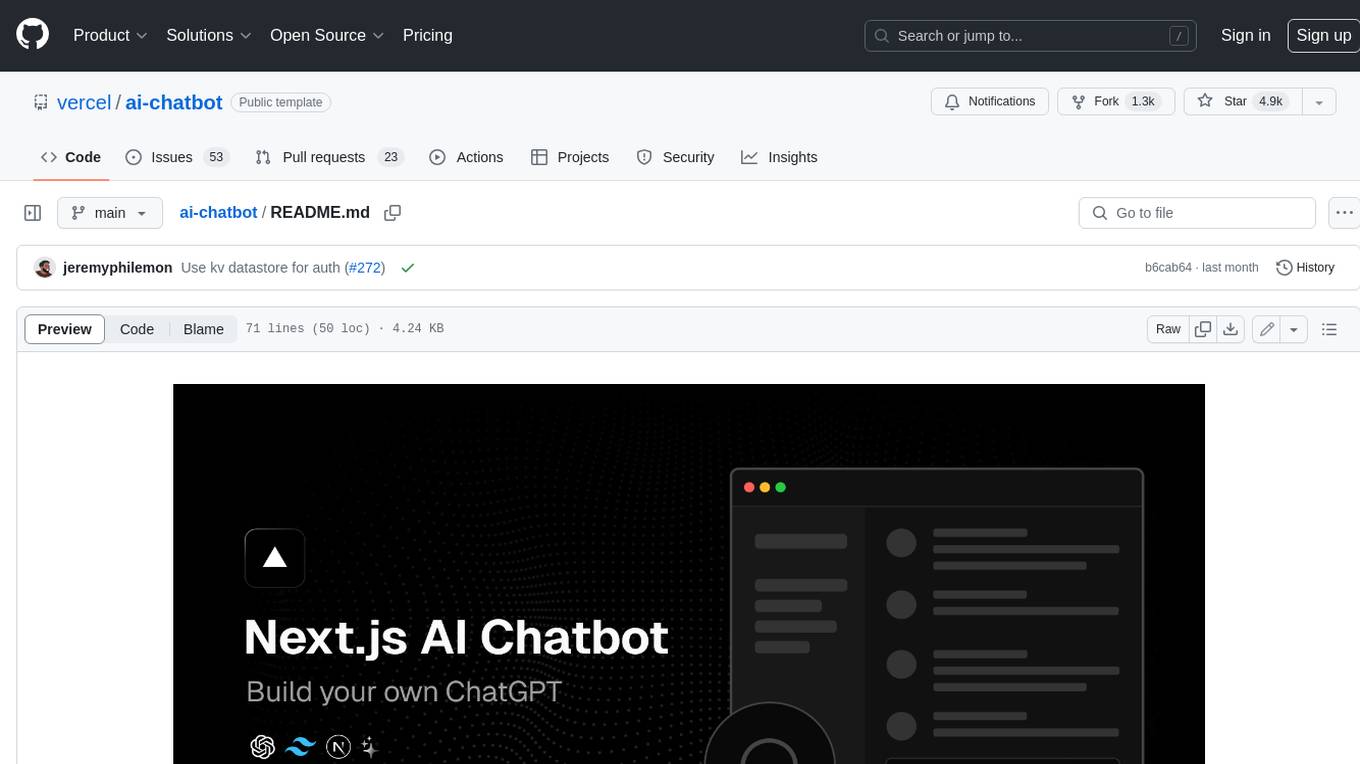
ai-chatbot
Next.js AI Chatbot is an open-source app template for building AI chatbots using Next.js, Vercel AI SDK, OpenAI, and Vercel KV. It includes features like Next.js App Router, React Server Components, Vercel AI SDK for streaming chat UI, support for various AI models, Tailwind CSS styling, Radix UI for headless components, chat history management, rate limiting, session storage with Vercel KV, and authentication with NextAuth.js. The template allows easy deployment to Vercel and customization of AI model providers.
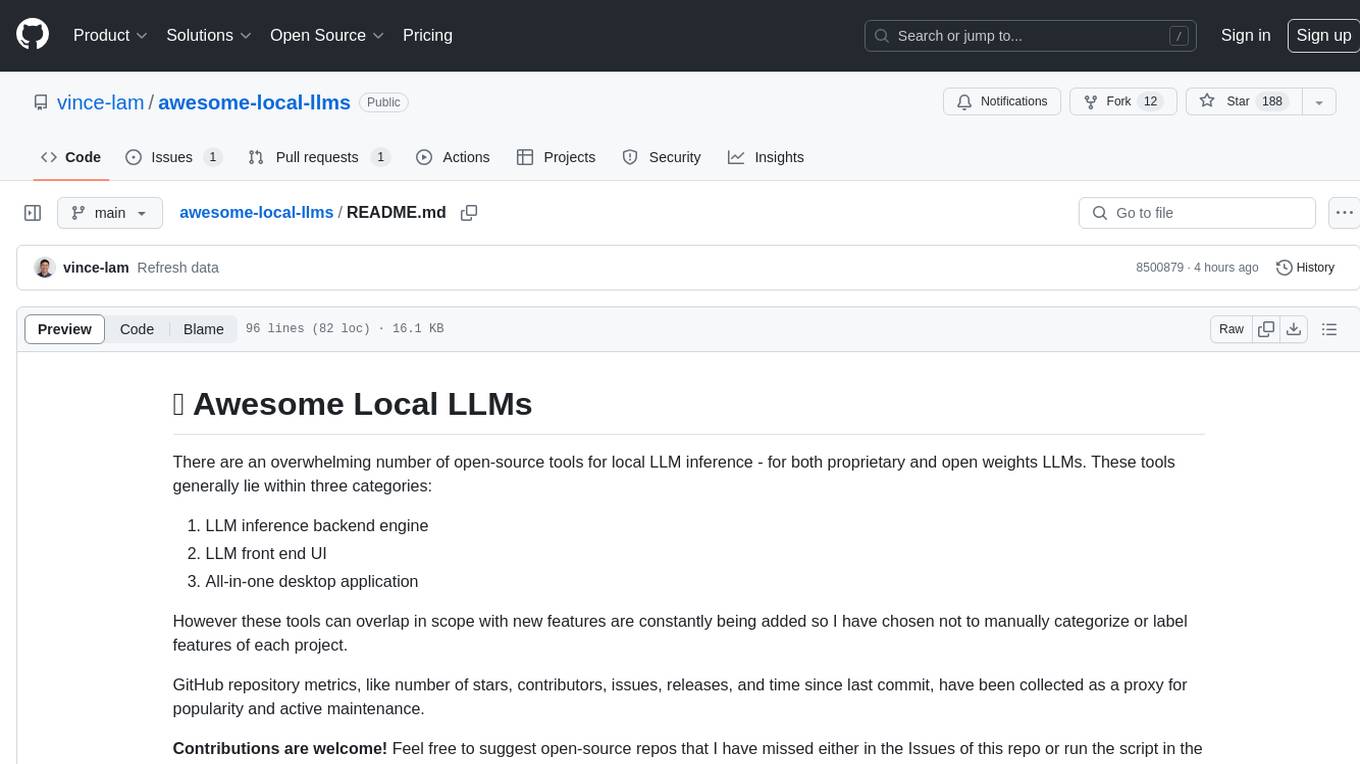
awesome-local-llms
The 'awesome-local-llms' repository is a curated list of open-source tools for local Large Language Model (LLM) inference, covering both proprietary and open weights LLMs. The repository categorizes these tools into LLM inference backend engines, LLM front end UIs, and all-in-one desktop applications. It collects GitHub repository metrics as proxies for popularity and active maintenance. Contributions are encouraged, and users can suggest additional open-source repositories through the Issues section or by running a provided script to update the README and make a pull request. The repository aims to provide a comprehensive resource for exploring and utilizing local LLM tools.
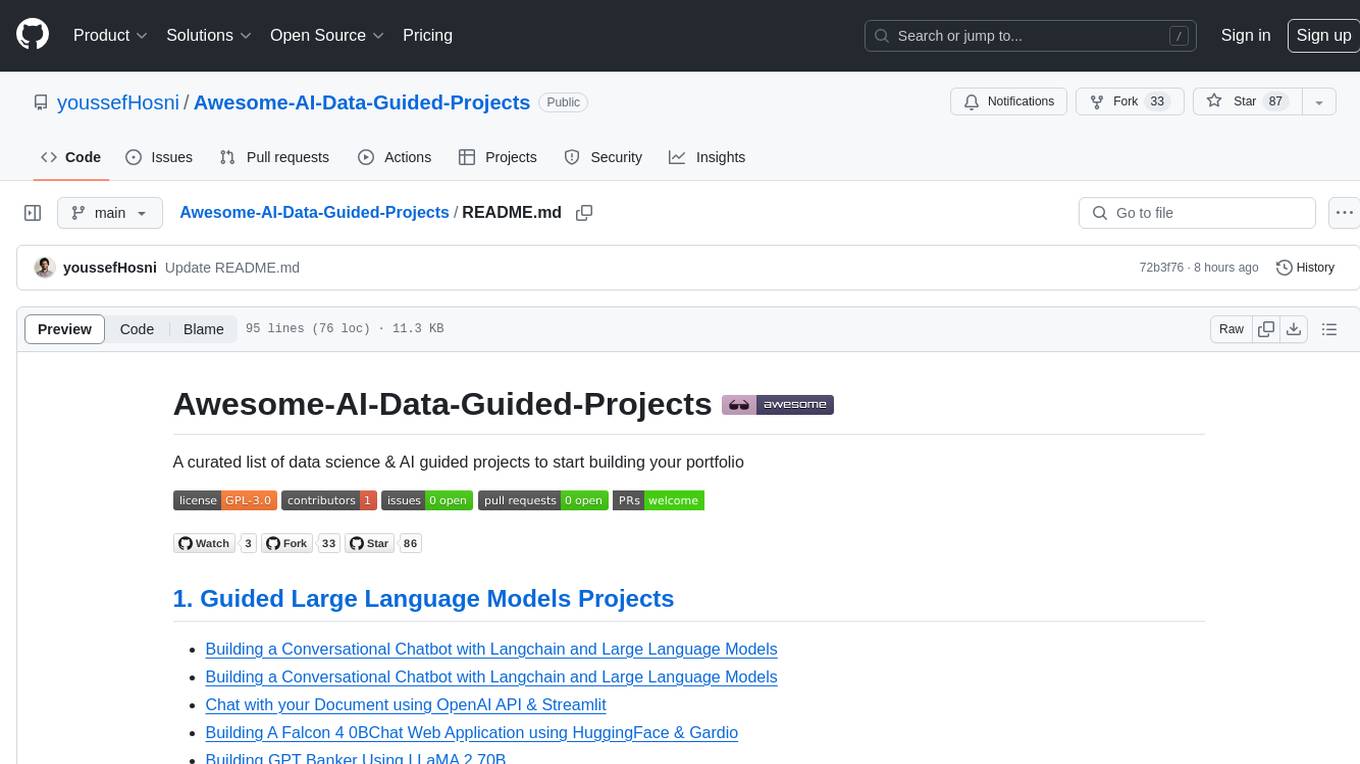
Awesome-AI-Data-Guided-Projects
A curated list of data science & AI guided projects to start building your portfolio. The repository contains guided projects covering various topics such as large language models, time series analysis, computer vision, natural language processing (NLP), and data science. Each project provides detailed instructions on how to implement specific tasks using different tools and technologies.
For similar jobs
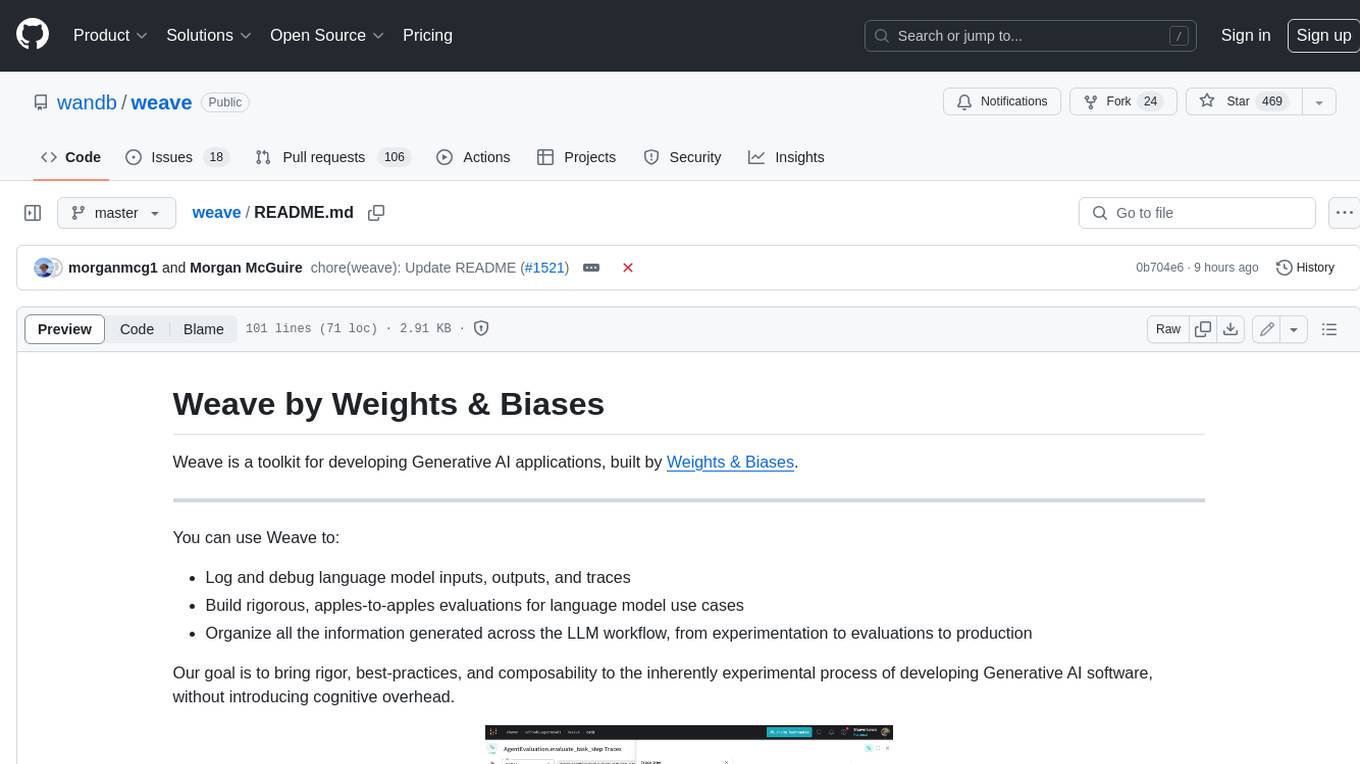
weave
Weave is a toolkit for developing Generative AI applications, built by Weights & Biases. With Weave, you can log and debug language model inputs, outputs, and traces; build rigorous, apples-to-apples evaluations for language model use cases; and organize all the information generated across the LLM workflow, from experimentation to evaluations to production. Weave aims to bring rigor, best-practices, and composability to the inherently experimental process of developing Generative AI software, without introducing cognitive overhead.
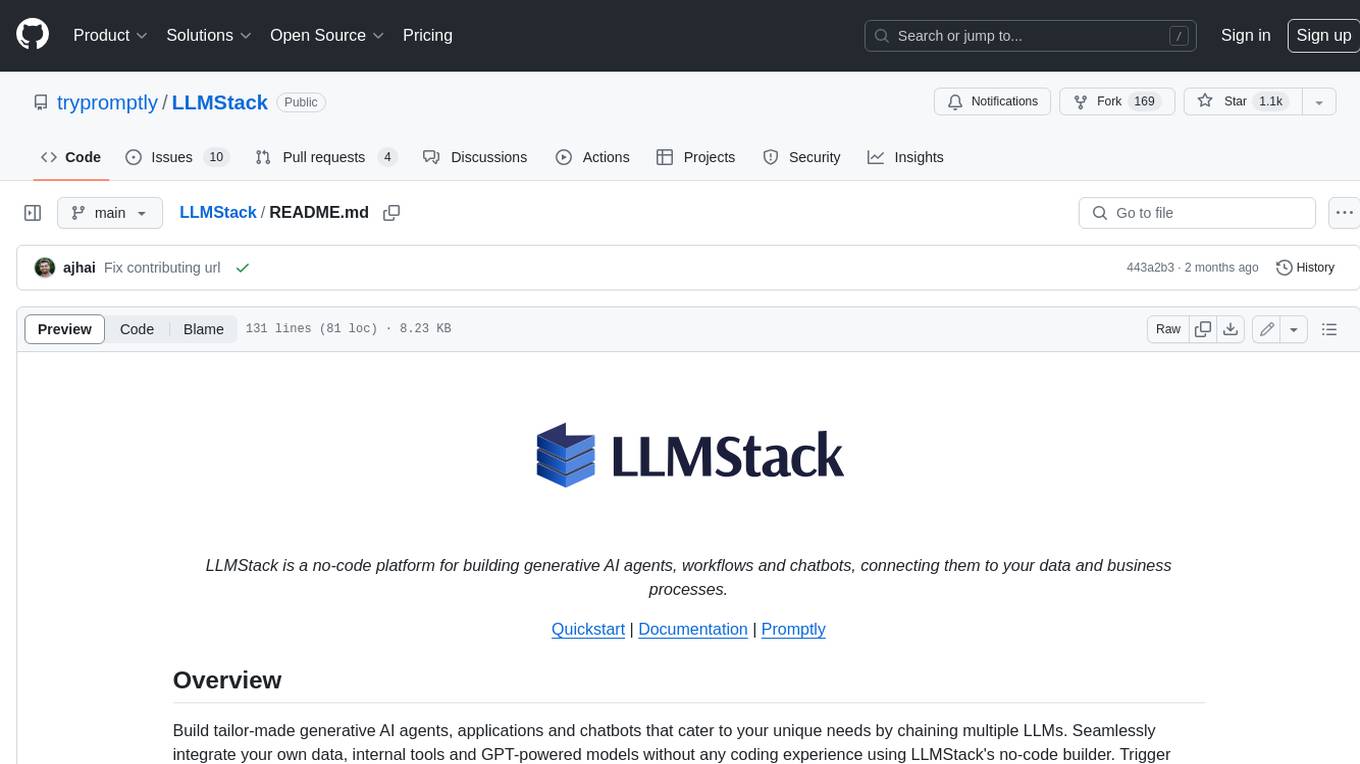
LLMStack
LLMStack is a no-code platform for building generative AI agents, workflows, and chatbots. It allows users to connect their own data, internal tools, and GPT-powered models without any coding experience. LLMStack can be deployed to the cloud or on-premise and can be accessed via HTTP API or triggered from Slack or Discord.
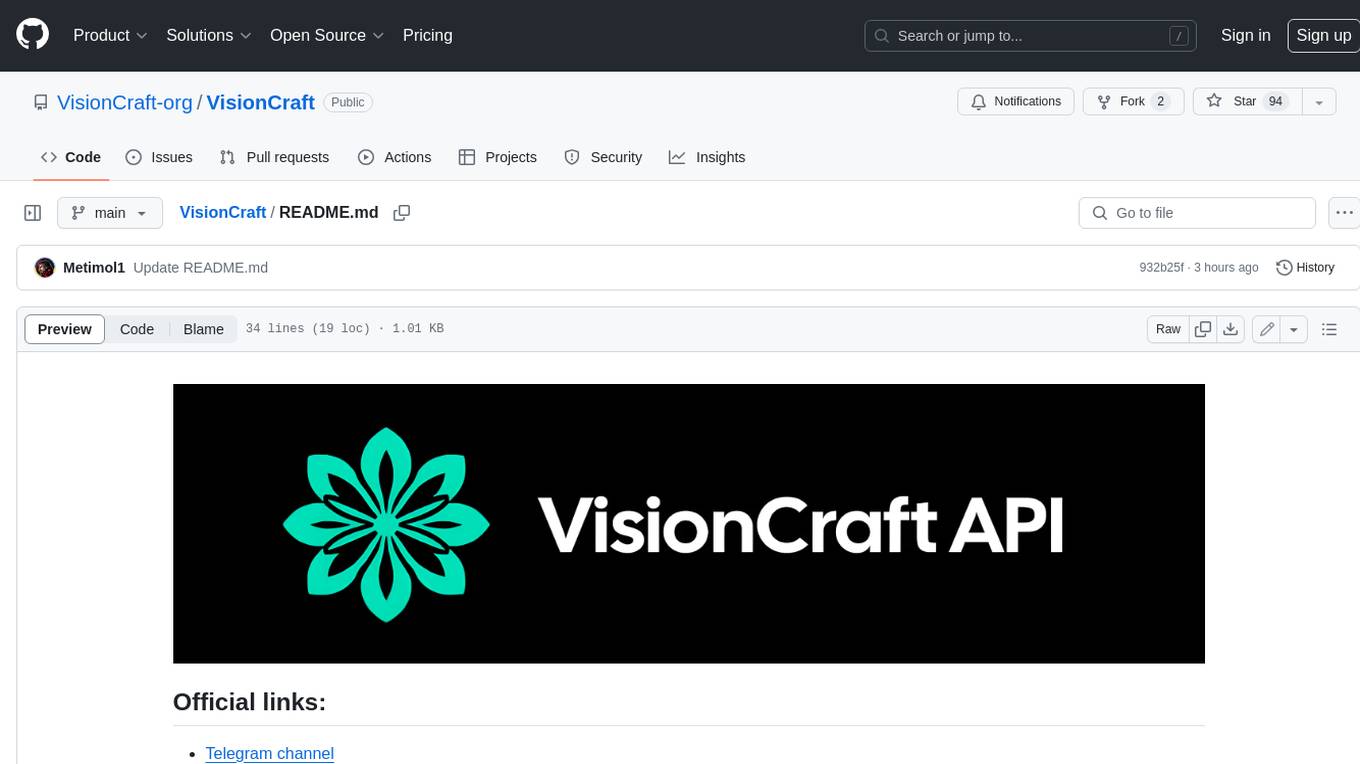
VisionCraft
The VisionCraft API is a free API for using over 100 different AI models. From images to sound.
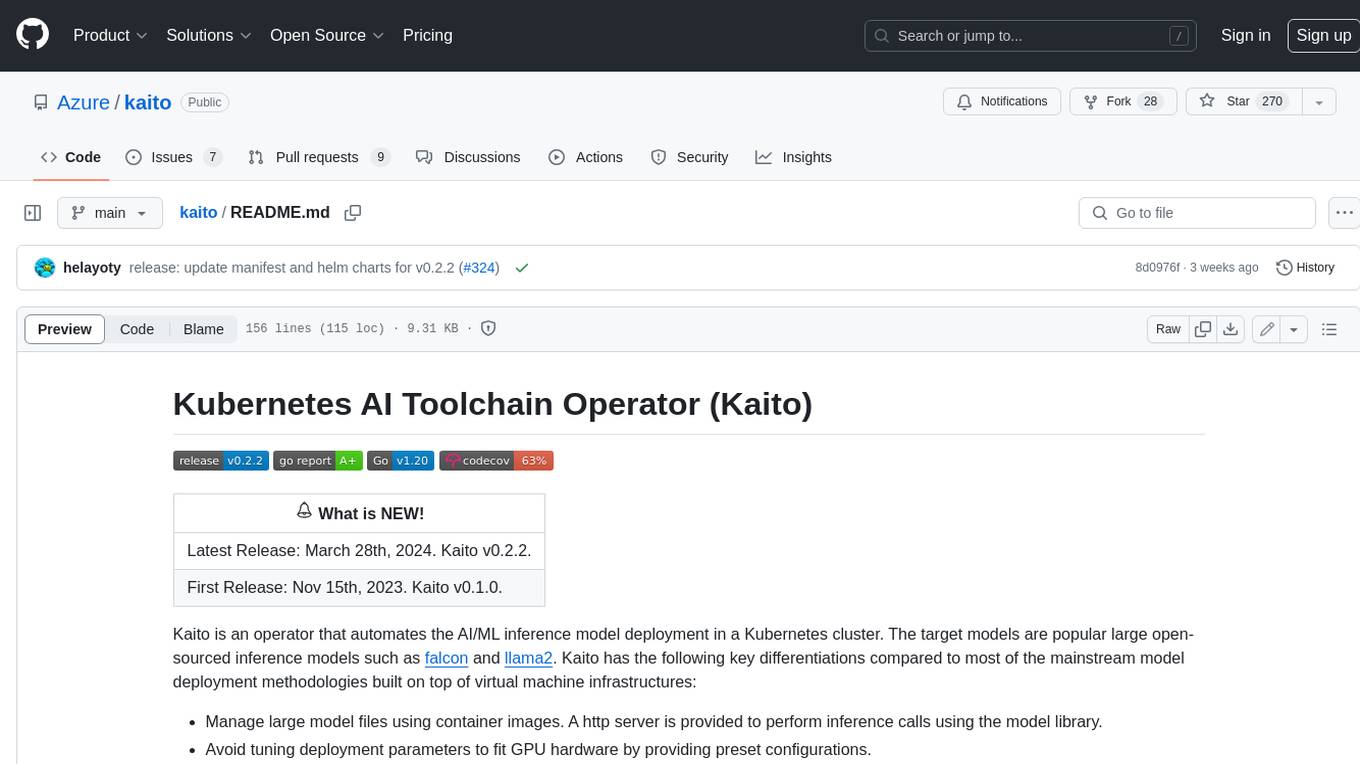
kaito
Kaito is an operator that automates the AI/ML inference model deployment in a Kubernetes cluster. It manages large model files using container images, avoids tuning deployment parameters to fit GPU hardware by providing preset configurations, auto-provisions GPU nodes based on model requirements, and hosts large model images in the public Microsoft Container Registry (MCR) if the license allows. Using Kaito, the workflow of onboarding large AI inference models in Kubernetes is largely simplified.
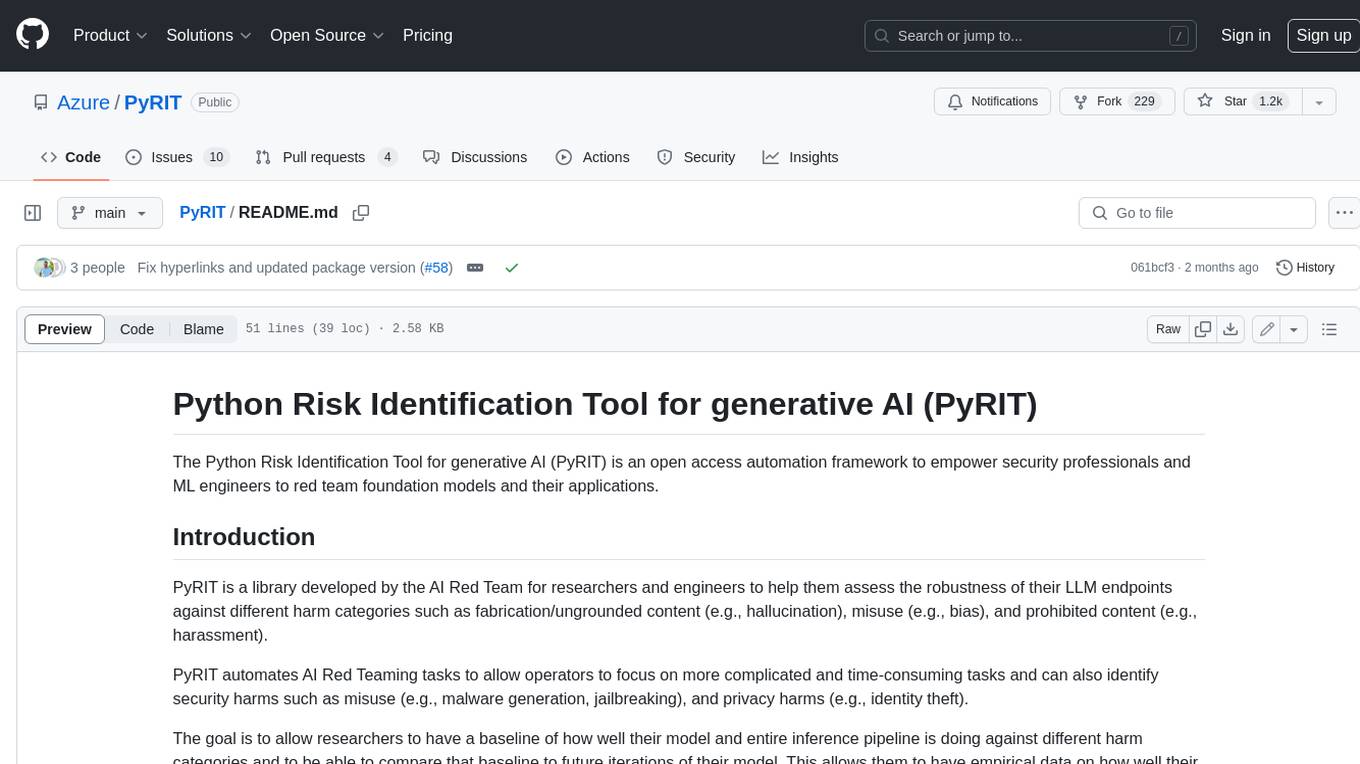
PyRIT
PyRIT is an open access automation framework designed to empower security professionals and ML engineers to red team foundation models and their applications. It automates AI Red Teaming tasks to allow operators to focus on more complicated and time-consuming tasks and can also identify security harms such as misuse (e.g., malware generation, jailbreaking), and privacy harms (e.g., identity theft). The goal is to allow researchers to have a baseline of how well their model and entire inference pipeline is doing against different harm categories and to be able to compare that baseline to future iterations of their model. This allows them to have empirical data on how well their model is doing today, and detect any degradation of performance based on future improvements.
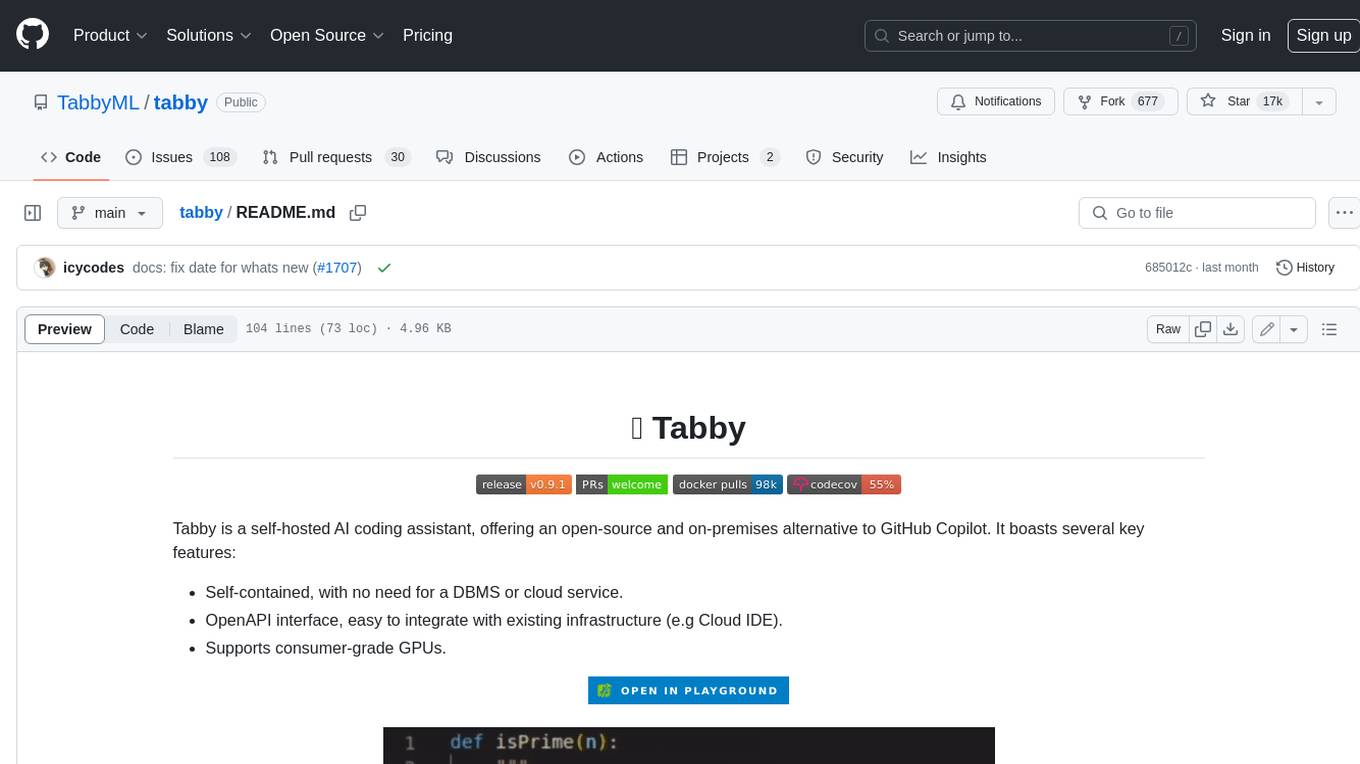
tabby
Tabby is a self-hosted AI coding assistant, offering an open-source and on-premises alternative to GitHub Copilot. It boasts several key features: * Self-contained, with no need for a DBMS or cloud service. * OpenAPI interface, easy to integrate with existing infrastructure (e.g Cloud IDE). * Supports consumer-grade GPUs.
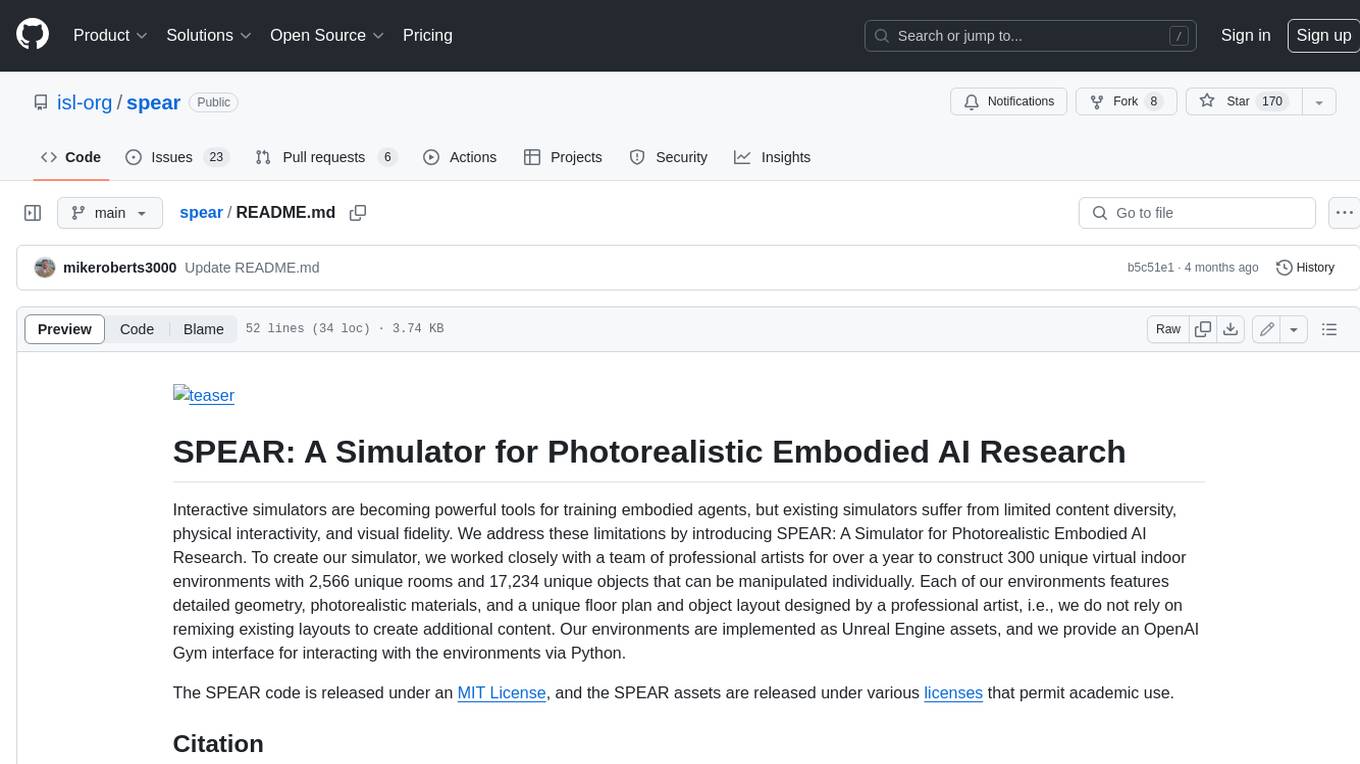
spear
SPEAR (Simulator for Photorealistic Embodied AI Research) is a powerful tool for training embodied agents. It features 300 unique virtual indoor environments with 2,566 unique rooms and 17,234 unique objects that can be manipulated individually. Each environment is designed by a professional artist and features detailed geometry, photorealistic materials, and a unique floor plan and object layout. SPEAR is implemented as Unreal Engine assets and provides an OpenAI Gym interface for interacting with the environments via Python.
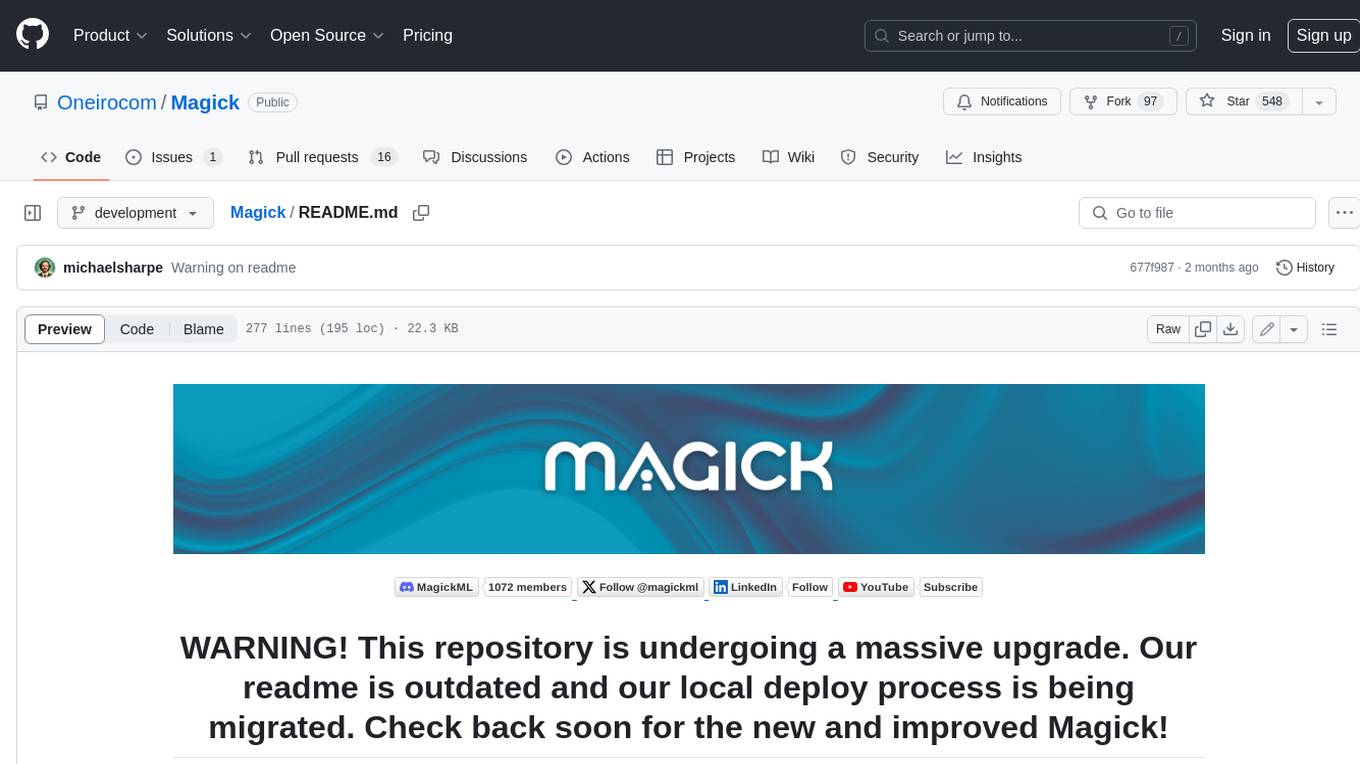
Magick
Magick is a groundbreaking visual AIDE (Artificial Intelligence Development Environment) for no-code data pipelines and multimodal agents. Magick can connect to other services and comes with nodes and templates well-suited for intelligent agents, chatbots, complex reasoning systems and realistic characters.