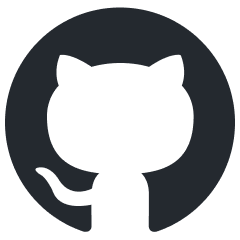
BentoVLLM
Self-host LLMs with vLLM and BentoML
Stars: 62
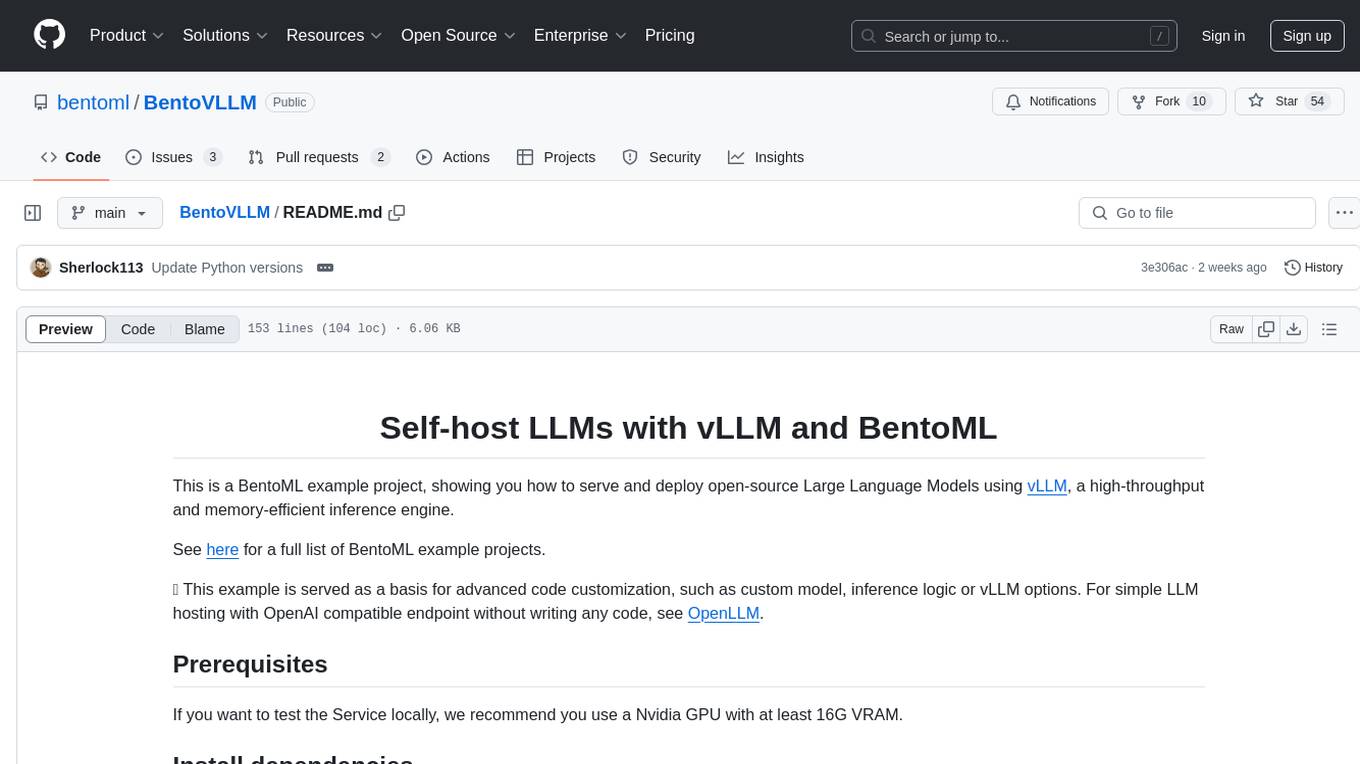
BentoVLLM is an example project demonstrating how to serve and deploy open-source Large Language Models using vLLM, a high-throughput and memory-efficient inference engine. It provides a basis for advanced code customization, such as custom models, inference logic, or vLLM options. The project allows for simple LLM hosting with OpenAI compatible endpoints without the need to write any code. Users can interact with the server using Swagger UI or other methods, and the service can be deployed to BentoCloud for better management and scalability. Additionally, the repository includes integration examples for different LLM models and tools.
README:
This is a BentoML example project, showing you how to serve and deploy open-source Large Language Models using vLLM, a high-throughput and memory-efficient inference engine.
See here for a full list of BentoML example projects.
đź’ˇ This example is served as a basis for advanced code customization, such as custom model, inference logic or vLLM options. For simple LLM hosting with OpenAI compatible endpoint without writing any code, see OpenLLM.
If you want to test the Service locally, we recommend you use a Nvidia GPU with at least 16G VRAM.
git clone https://github.com/bentoml/BentoVLLM.git
cd BentoVLLM/mistral-7b-instruct
# Recommend Python 3.11
pip install -r requirements.txt && pip install -f -U "pydantic>=2.0"
We have defined a BentoML Service in service.py
. Run bentoml serve
 in your project directory to start the Service.
$ bentoml serve .
2024-01-18T07:51:30+0800 [INFO] [cli] Starting production HTTP BentoServer from "service:VLLM" listening on http://localhost:3000 (Press CTRL+C to quit)
INFO 01-18 07:51:40 model_runner.py:501] Capturing the model for CUDA graphs. This may lead to unexpected consequences if the model is not static. To run the model in eager mode, set 'enforce_eager=True' or use '--enforce-eager' in the CLI.
INFO 01-18 07:51:40 model_runner.py:505] CUDA graphs can take additional 1~3 GiB memory per GPU. If you are running out of memory, consider decreasing `gpu_memory_utilization` or enforcing eager mode.
INFO 01-18 07:51:46 model_runner.py:547] Graph capturing finished in 6 secs.
The server is now active at http://localhost:3000. You can interact with it using the Swagger UI or in other different ways.
CURL
curl -X 'POST' \
'http://localhost:3000/generate' \
-H 'accept: text/event-stream' \
-H 'Content-Type: application/json' \
-d '{
"prompt": "Explain superconductors like I'\''m five years old",
"tokens": null
}'
Python client
import bentoml
with bentoml.SyncHTTPClient("http://localhost:3000") as client:
response_generator = client.generate(
prompt="Explain superconductors like I'm five years old",
tokens=None
)
for response in response_generator:
print(response)
OpenAI-compatible endpoints
This Service uses the @openai_endpoints
decorator to set up OpenAI-compatible endpoints (chat/completions
and completions
). This means your client can interact with the backend Service (in this case, the VLLM class) as if they were communicating directly with OpenAI's API. This utility does not affect your BentoML Service code, and you can use it for other LLMs as well.
from openai import OpenAI
client = OpenAI(base_url='http://localhost:3000/v1', api_key='na')
# Use the following func to get the available models
client.models.list()
chat_completion = client.chat.completions.create(
model="mistralai/Mistral-7B-Instruct-v0.2",
messages=[
{
"role": "user",
"content": "Explain superconductors like I'm five years old"
}
],
stream=True,
)
for chunk in chat_completion:
# Extract and print the content of the model's reply
print(chunk.choices[0].delta.content or "", end="")
Note: If your Service is deployed with protected endpoints on BentoCloud, you need to set the environment variable OPENAI_API_KEY
to your BentoCloud API key first.
export OPENAI_API_KEY={YOUR_BENTOCLOUD_API_TOKEN}
You can then use the following line to replace the client in the above code snippet. Refer to Obtain the endpoint URL to retrieve the endpoint URL.
client = OpenAI(base_url='your_bentocloud_deployment_endpoint_url/v1')
For detailed explanations of the Service code, see vLLM inference.
After the Service is ready, you can deploy the application to BentoCloud for better management and scalability. Sign up if you haven't got a BentoCloud account.
Make sure you have logged in to BentoCloud, then run the following command to deploy it.
bentoml deploy .
Once the application is up and running on BentoCloud, you can access it via the exposed URL.
Note: For custom deployment in your own infrastructure, use BentoML to generate an OCI-compliant image.
Besides the mistral-7b-instruct model, we have examples for other models in subdirectories of this repository. Below is a list of these models and links to the example subdirectories.
- gemma-7b-it
- Llama-2-7b-chat-hf
- Llama-3-8b-instruct
- Llama-3-70b-instruct with AWQ quantization
- Mistral-7B-Instruct-v0.2
- Mixtral-8x7B-Instruct-v0.1 with gptq quantization
- Outlines integration
- SOLAR-10.7B-v1.0
- Every model directory contains codes to add OpenAI compatible endpoints to the BentoML service.
- outlines-integration/ contains the code to integrate with outlines for structured generation.
For Tasks:
Click tags to check more tools for each tasksFor Jobs:
Alternative AI tools for BentoVLLM
Similar Open Source Tools
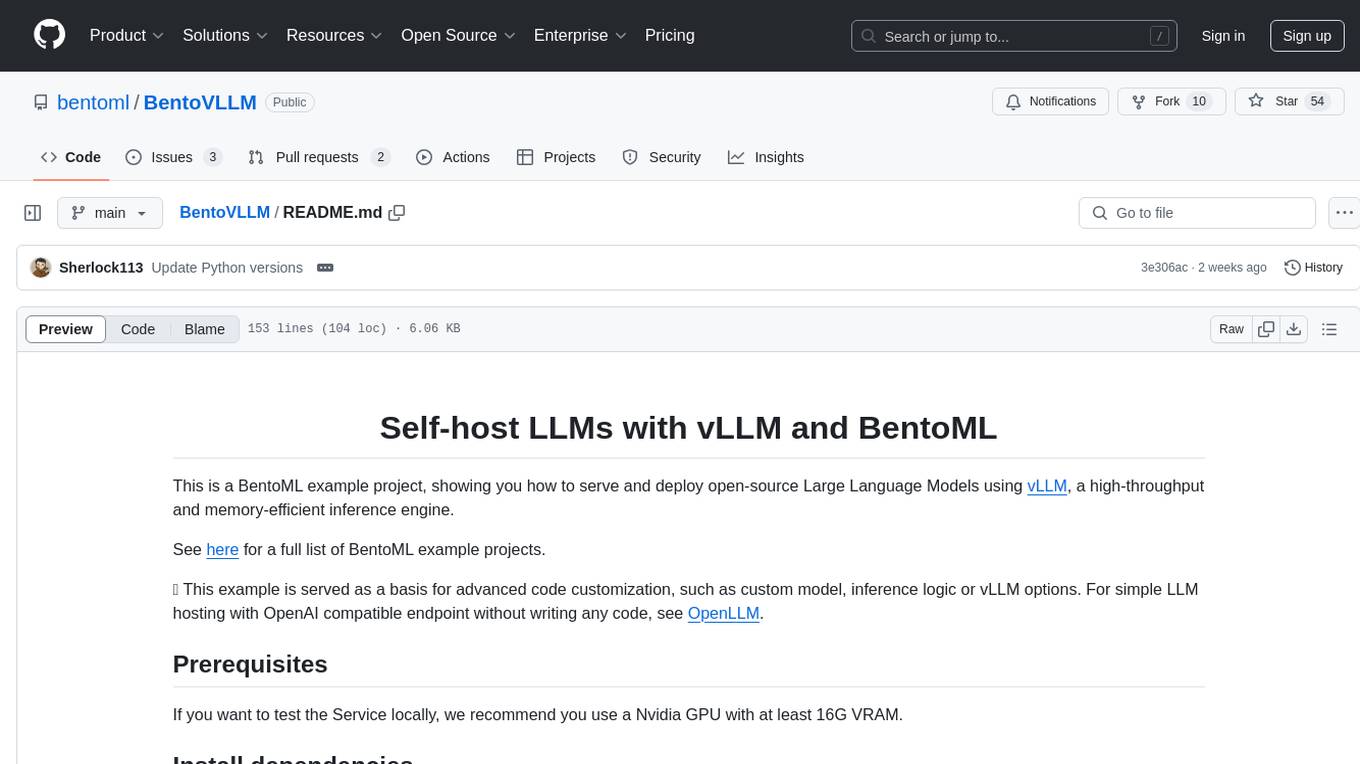
BentoVLLM
BentoVLLM is an example project demonstrating how to serve and deploy open-source Large Language Models using vLLM, a high-throughput and memory-efficient inference engine. It provides a basis for advanced code customization, such as custom models, inference logic, or vLLM options. The project allows for simple LLM hosting with OpenAI compatible endpoints without the need to write any code. Users can interact with the server using Swagger UI or other methods, and the service can be deployed to BentoCloud for better management and scalability. Additionally, the repository includes integration examples for different LLM models and tools.
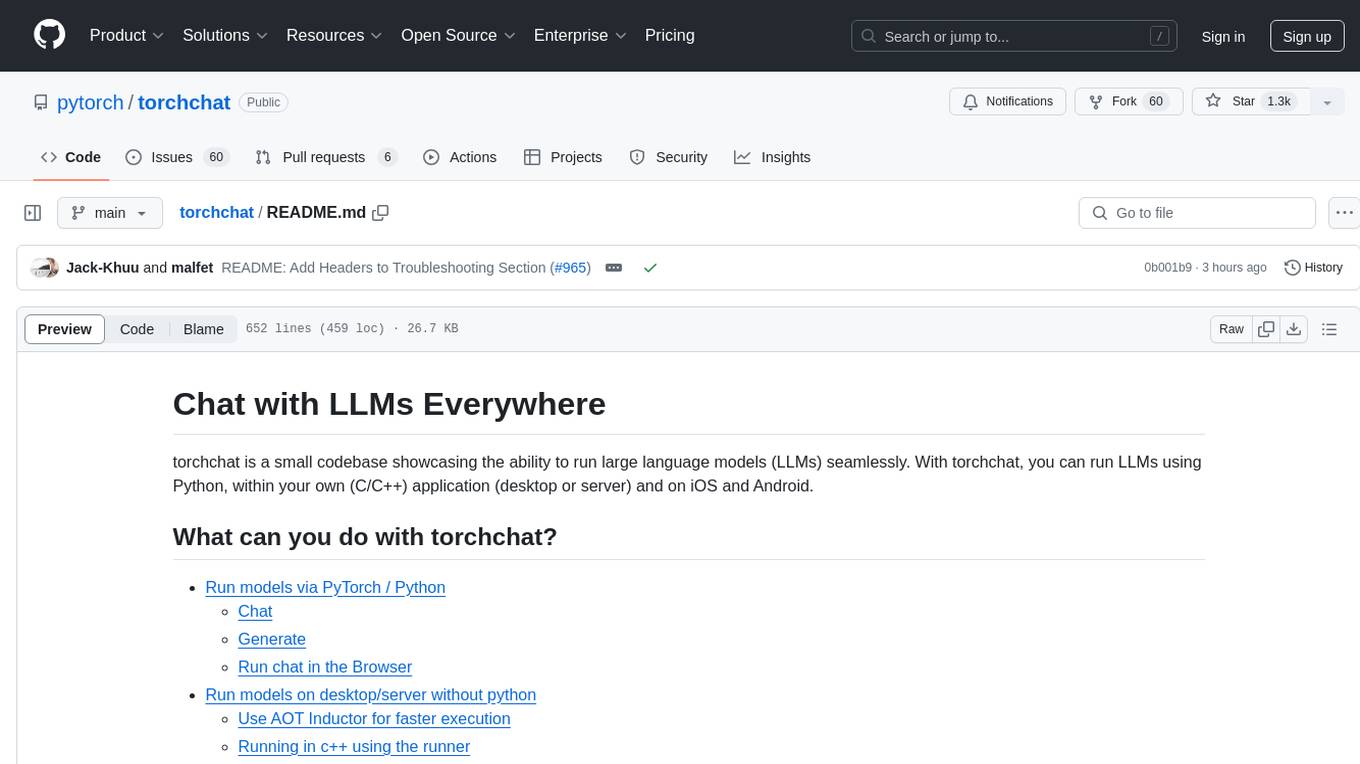
torchchat
torchchat is a codebase showcasing the ability to run large language models (LLMs) seamlessly. It allows running LLMs using Python in various environments such as desktop, server, iOS, and Android. The tool supports running models via PyTorch, chatting, generating text, running chat in the browser, and running models on desktop/server without Python. It also provides features like AOT Inductor for faster execution, running in C++ using the runner, and deploying and running on iOS and Android. The tool supports popular hardware and OS including Linux, Mac OS, Android, and iOS, with various data types and execution modes available.
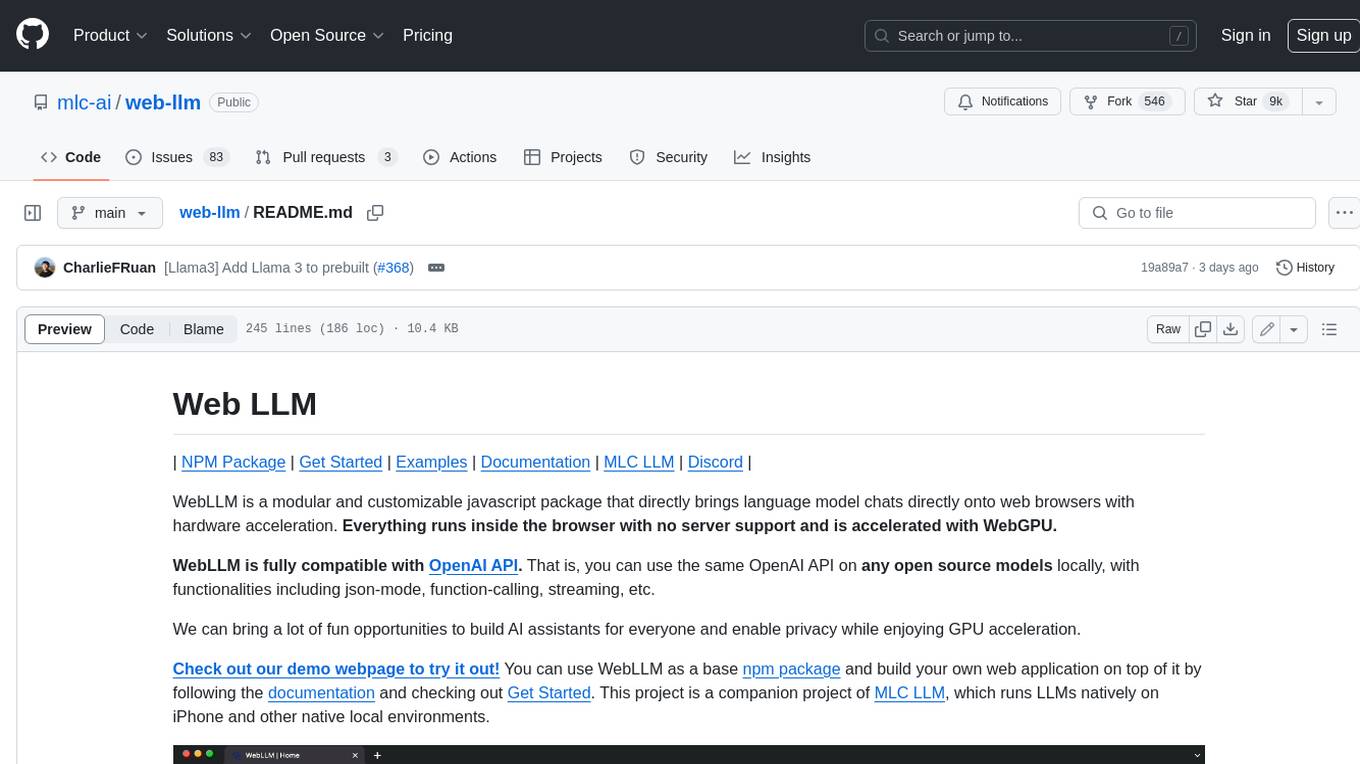
web-llm
WebLLM is a modular and customizable javascript package that directly brings language model chats directly onto web browsers with hardware acceleration. Everything runs inside the browser with no server support and is accelerated with WebGPU. WebLLM is fully compatible with OpenAI API. That is, you can use the same OpenAI API on any open source models locally, with functionalities including json-mode, function-calling, streaming, etc. We can bring a lot of fun opportunities to build AI assistants for everyone and enable privacy while enjoying GPU acceleration.
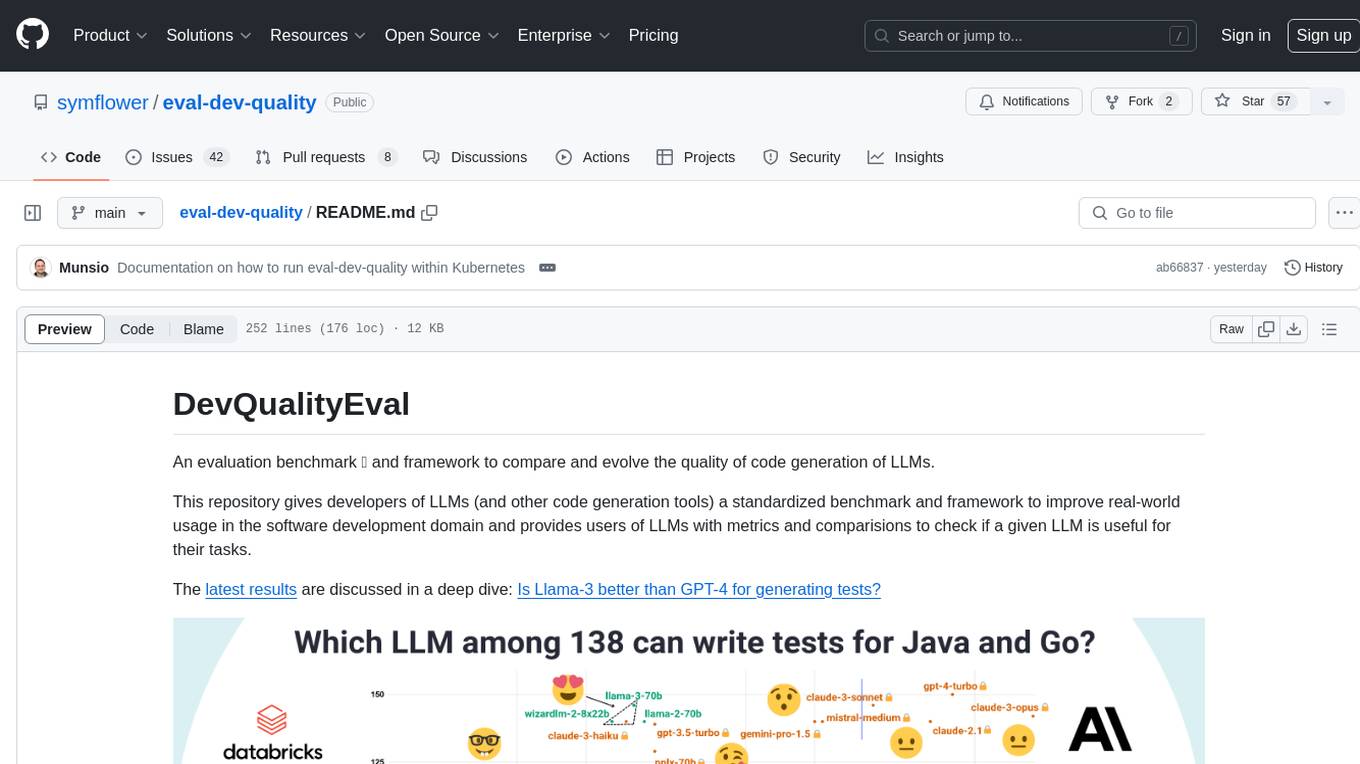
eval-dev-quality
DevQualityEval is an evaluation benchmark and framework designed to compare and improve the quality of code generation of Language Model Models (LLMs). It provides developers with a standardized benchmark to enhance real-world usage in software development and offers users metrics and comparisons to assess the usefulness of LLMs for their tasks. The tool evaluates LLMs' performance in solving software development tasks and measures the quality of their results through a point-based system. Users can run specific tasks, such as test generation, across different programming languages to evaluate LLMs' language understanding and code generation capabilities.
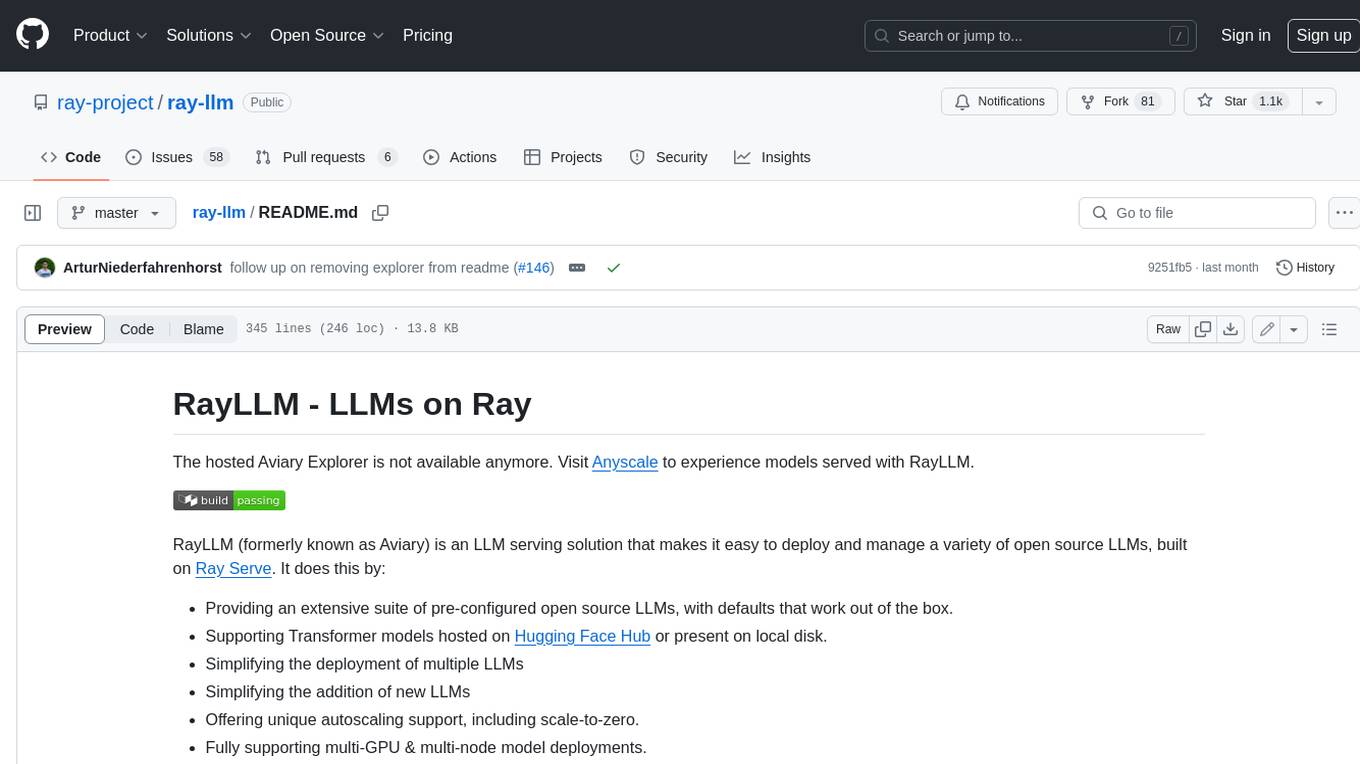
ray-llm
RayLLM (formerly known as Aviary) is an LLM serving solution that makes it easy to deploy and manage a variety of open source LLMs, built on Ray Serve. It provides an extensive suite of pre-configured open source LLMs, with defaults that work out of the box. RayLLM supports Transformer models hosted on Hugging Face Hub or present on local disk. It simplifies the deployment of multiple LLMs, the addition of new LLMs, and offers unique autoscaling support, including scale-to-zero. RayLLM fully supports multi-GPU & multi-node model deployments and offers high performance features like continuous batching, quantization and streaming. It provides a REST API that is similar to OpenAI's to make it easy to migrate and cross test them. RayLLM supports multiple LLM backends out of the box, including vLLM and TensorRT-LLM.
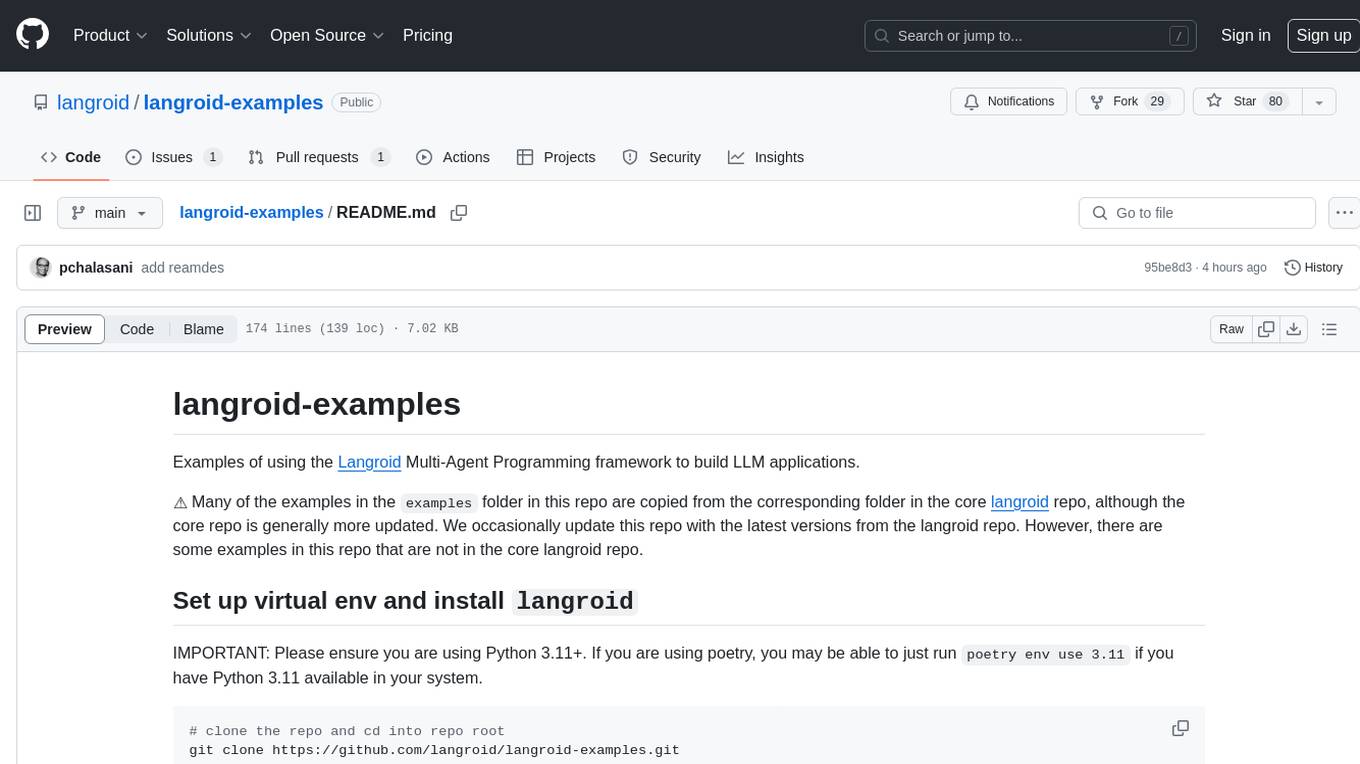
langroid-examples
Langroid-examples is a repository containing examples of using the Langroid Multi-Agent Programming framework to build LLM applications. It provides a collection of scripts and instructions for setting up the environment, working with local LLMs, using OpenAI LLMs, and running various examples. The repository also includes optional setup instructions for integrating with Qdrant, Redis, Momento, GitHub, and Google Custom Search API. Users can explore different scenarios and functionalities of Langroid through the provided examples and documentation.
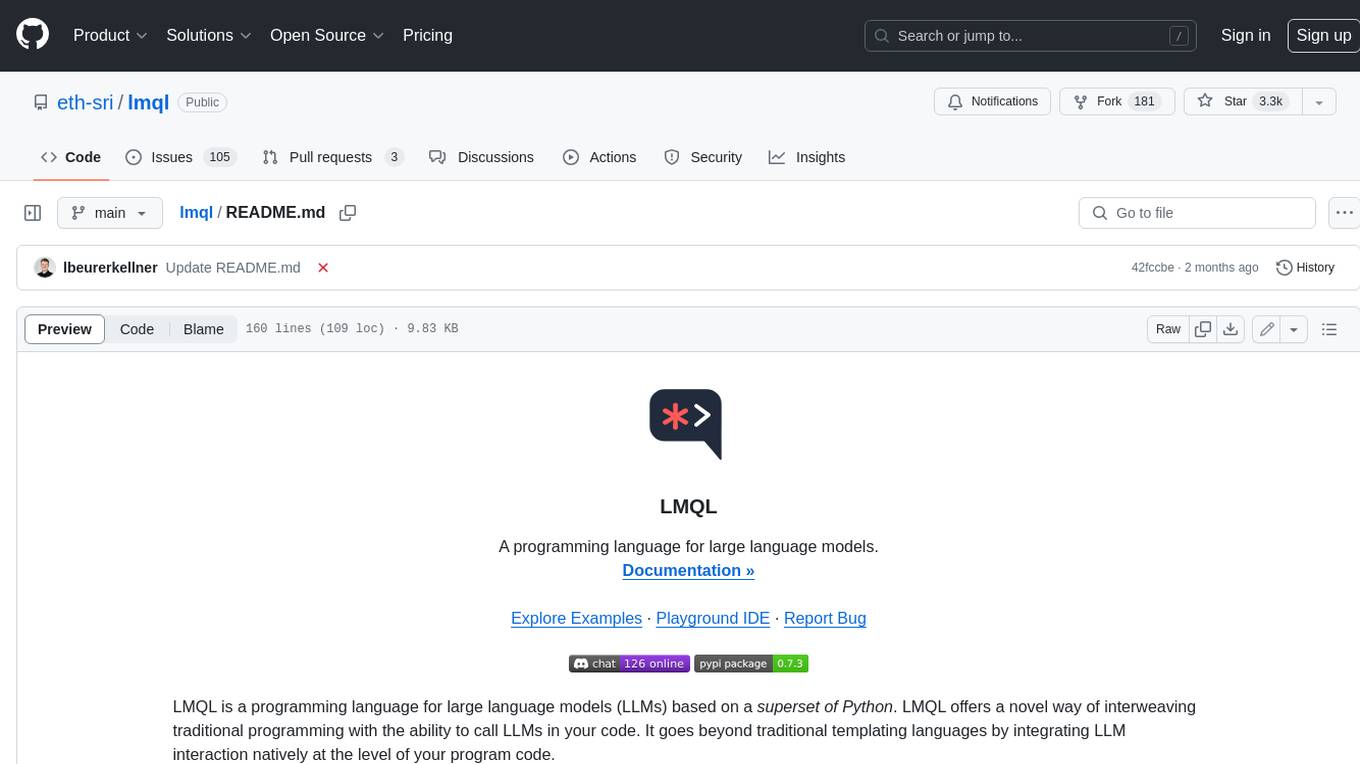
lmql
LMQL is a programming language designed for large language models (LLMs) that offers a unique way of integrating traditional programming with LLM interaction. It allows users to write programs that combine algorithmic logic with LLM calls, enabling model reasoning capabilities within the context of the program. LMQL provides features such as Python syntax integration, rich control-flow options, advanced decoding techniques, powerful constraints via logit masking, runtime optimization, sync and async API support, multi-model compatibility, and extensive applications like JSON decoding and interactive chat interfaces. The tool also offers library integration, flexible tooling, and output streaming options for easy model output handling.
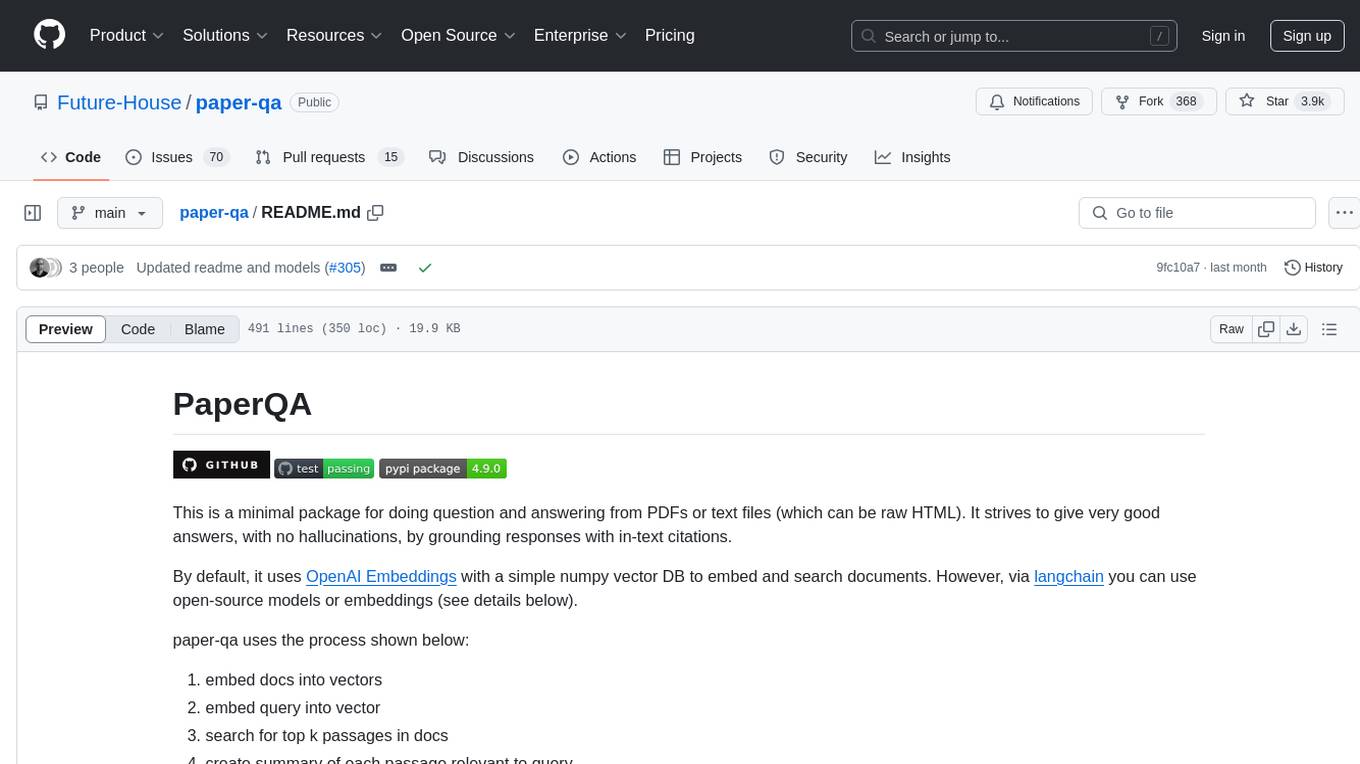
paper-qa
PaperQA is a minimal package for question and answering from PDFs or text files, providing very good answers with in-text citations. It uses OpenAI Embeddings to embed and search documents, and includes a process of embedding docs, queries, searching for top passages, creating summaries, using an LLM to re-score and select relevant summaries, putting summaries into prompt, and generating answers. The tool can be used to answer specific questions related to scientific research by leveraging citations and relevant passages from documents.
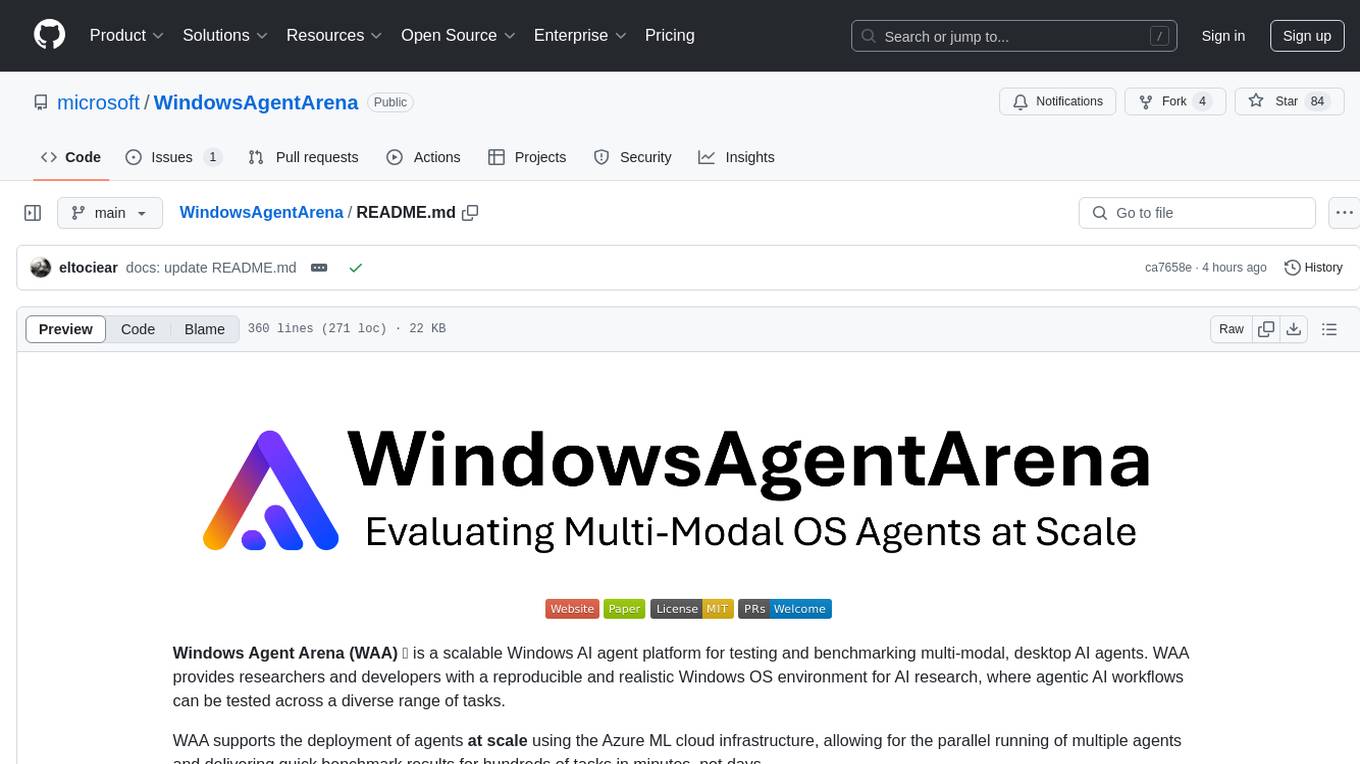
WindowsAgentArena
Windows Agent Arena (WAA) is a scalable Windows AI agent platform designed for testing and benchmarking multi-modal, desktop AI agents. It provides researchers and developers with a reproducible and realistic Windows OS environment for AI research, enabling testing of agentic AI workflows across various tasks. WAA supports deploying agents at scale using Azure ML cloud infrastructure, allowing parallel running of multiple agents and delivering quick benchmark results for hundreds of tasks in minutes.
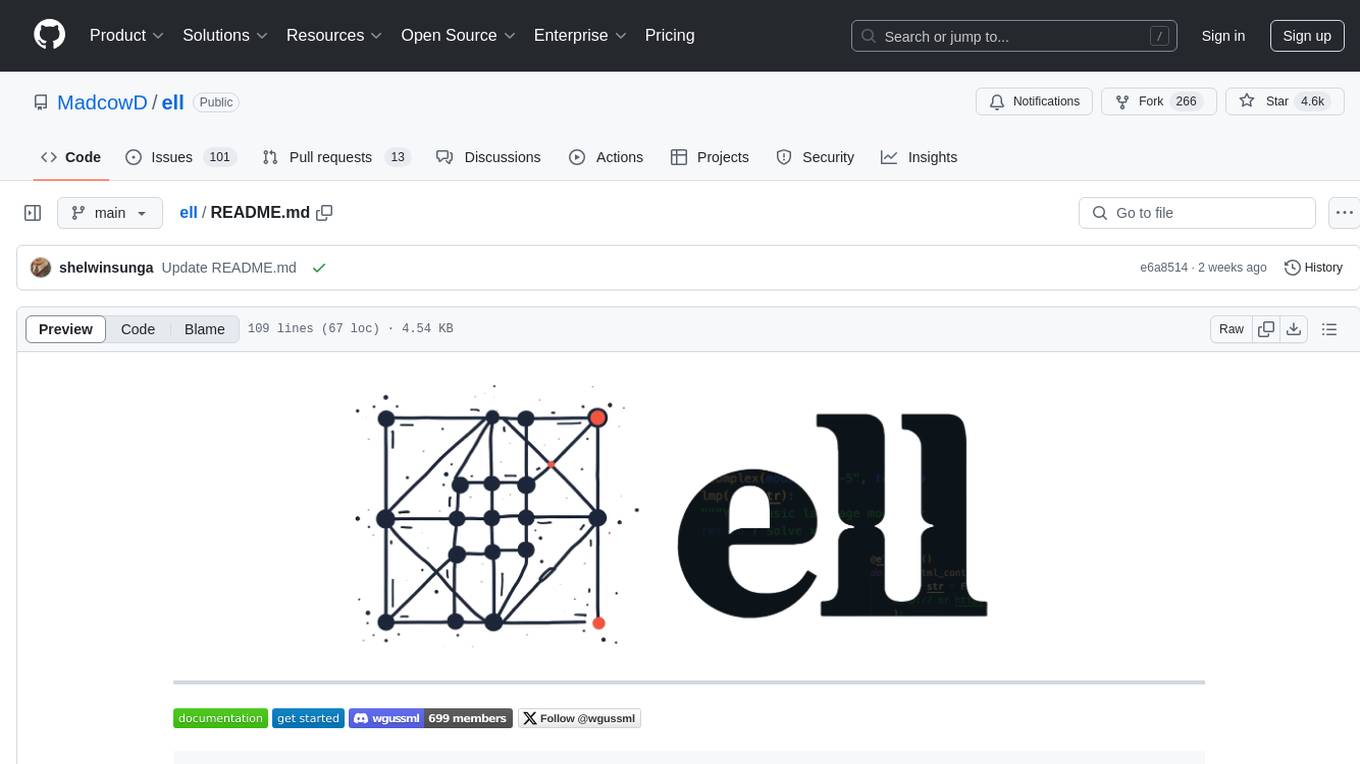
ell
ell is a lightweight, functional prompt engineering framework that treats prompts as programs rather than strings. It provides tools for prompt versioning, monitoring, and visualization, as well as support for multimodal inputs and outputs. The framework aims to simplify the process of prompt engineering for language models.
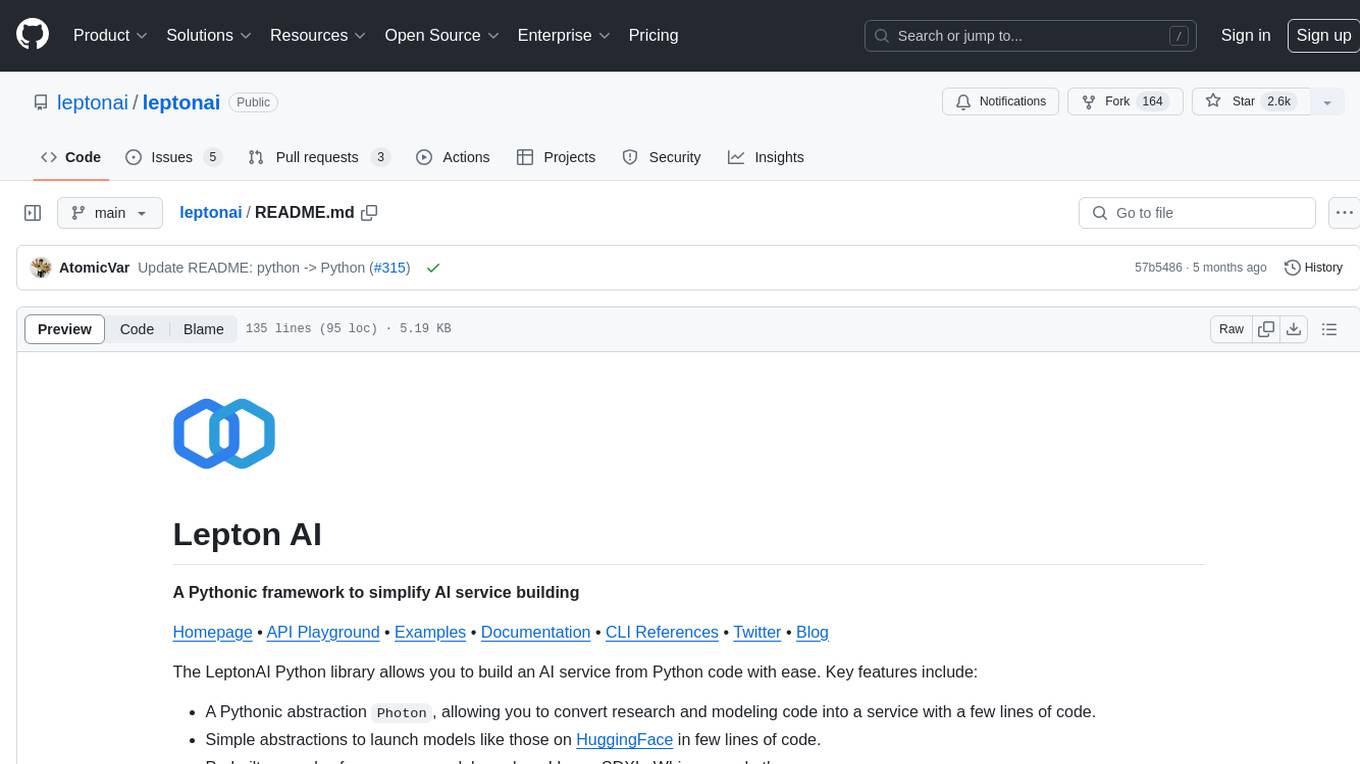
leptonai
A Pythonic framework to simplify AI service building. The LeptonAI Python library allows you to build an AI service from Python code with ease. Key features include a Pythonic abstraction Photon, simple abstractions to launch models like those on HuggingFace, prebuilt examples for common models, AI tailored batteries, a client to automatically call your service like native Python functions, and Pythonic configuration specs to be readily shipped in a cloud environment.
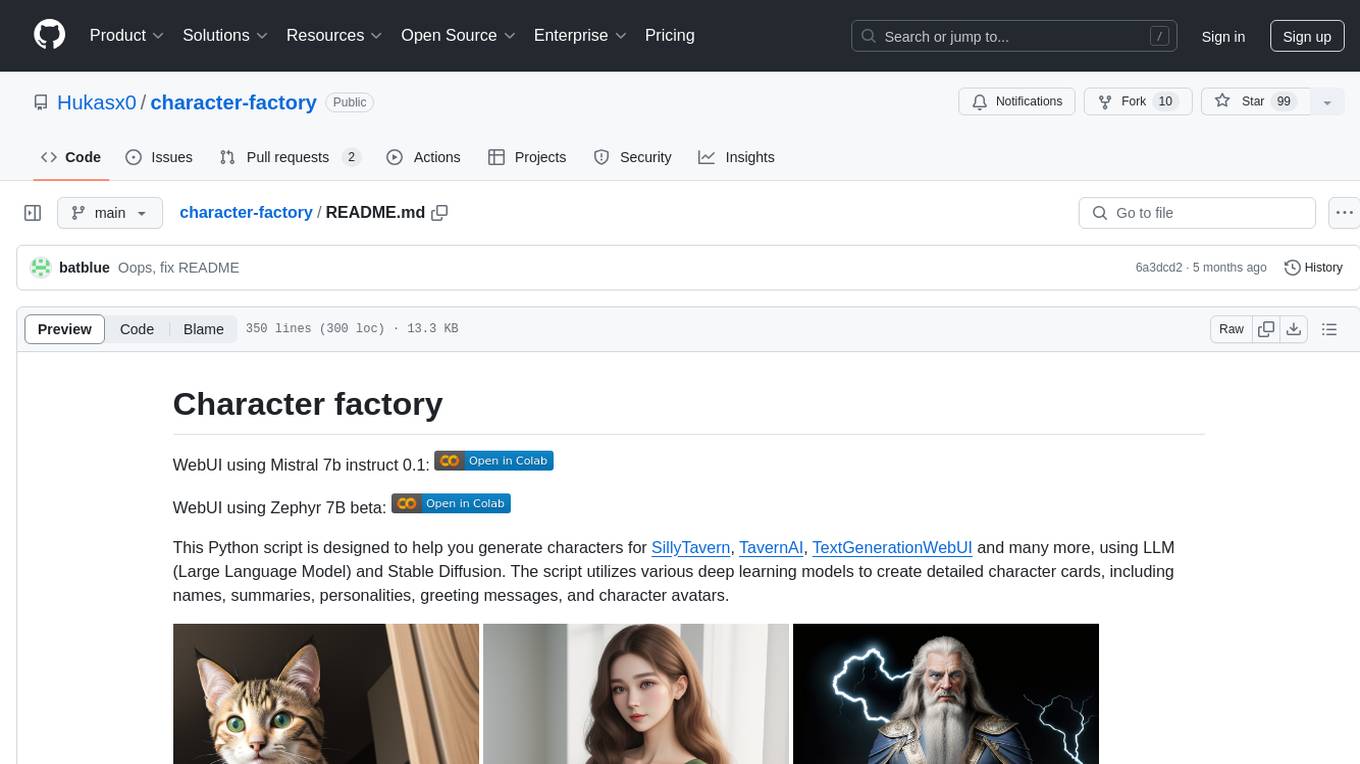
character-factory
Character Factory is a Python script designed to generate detailed character cards for SillyTavern, TavernAI, TextGenerationWebUI, and more using Large Language Model (LLM) and Stable Diffusion. It streamlines character generation by leveraging deep learning models to create names, summaries, personalities, greeting messages, and avatars for characters. The tool provides an easy way to create unique and imaginative characters for storytelling, chatting, and other purposes.
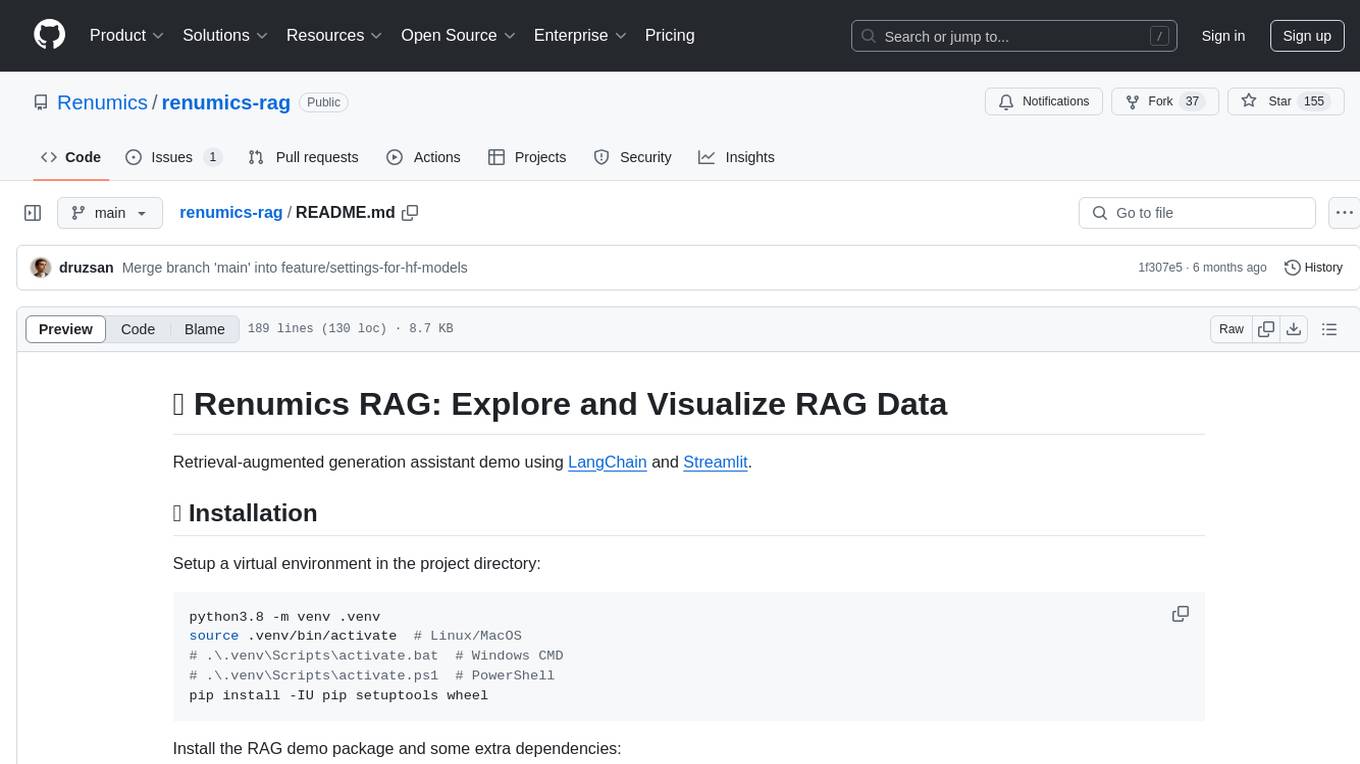
renumics-rag
Renumics RAG is a retrieval-augmented generation assistant demo that utilizes LangChain and Streamlit. It provides a tool for indexing documents and answering questions based on the indexed data. Users can explore and visualize RAG data, configure OpenAI and Hugging Face models, and interactively explore questions and document snippets. The tool supports GPU and CPU setups, offers a command-line interface for retrieving and answering questions, and includes a web application for easy access. It also allows users to customize retrieval settings, embeddings models, and database creation. Renumics RAG is designed to enhance the question-answering process by leveraging indexed documents and providing detailed answers with sources.
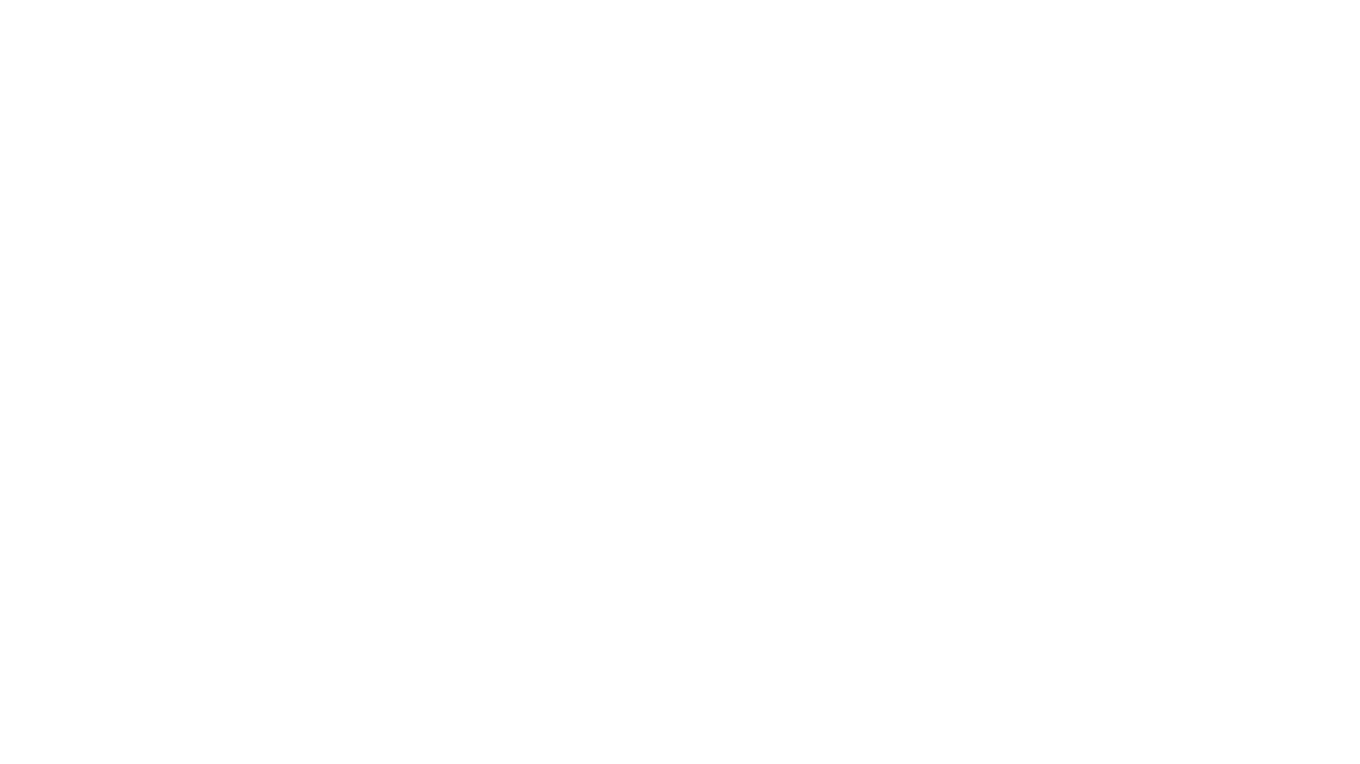
reader
Reader is a tool that converts any URL to an LLM-friendly input with a simple prefix `https://r.jina.ai/`. It improves the output for your agent and RAG systems at no cost. Reader supports image reading, captioning all images at the specified URL and adding `Image [idx]: [caption]` as an alt tag. This enables downstream LLMs to interact with the images in reasoning, summarizing, etc. Reader offers a streaming mode, useful when the standard mode provides an incomplete result. In streaming mode, Reader waits a bit longer until the page is fully rendered, providing more complete information. Reader also supports a JSON mode, which contains three fields: `url`, `title`, and `content`. Reader is backed by Jina AI and licensed under Apache-2.0.
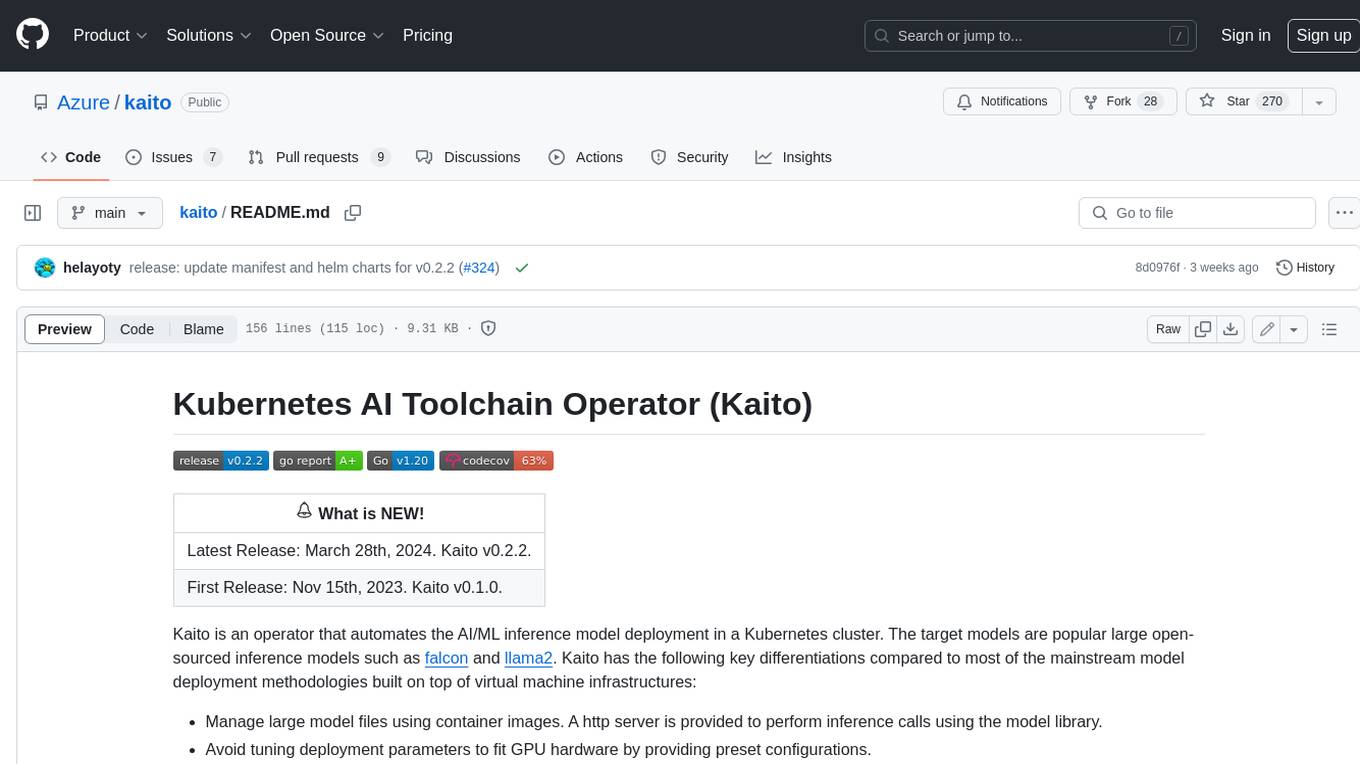
kaito
Kaito is an operator that automates the AI/ML inference model deployment in a Kubernetes cluster. It manages large model files using container images, avoids tuning deployment parameters to fit GPU hardware by providing preset configurations, auto-provisions GPU nodes based on model requirements, and hosts large model images in the public Microsoft Container Registry (MCR) if the license allows. Using Kaito, the workflow of onboarding large AI inference models in Kubernetes is largely simplified.
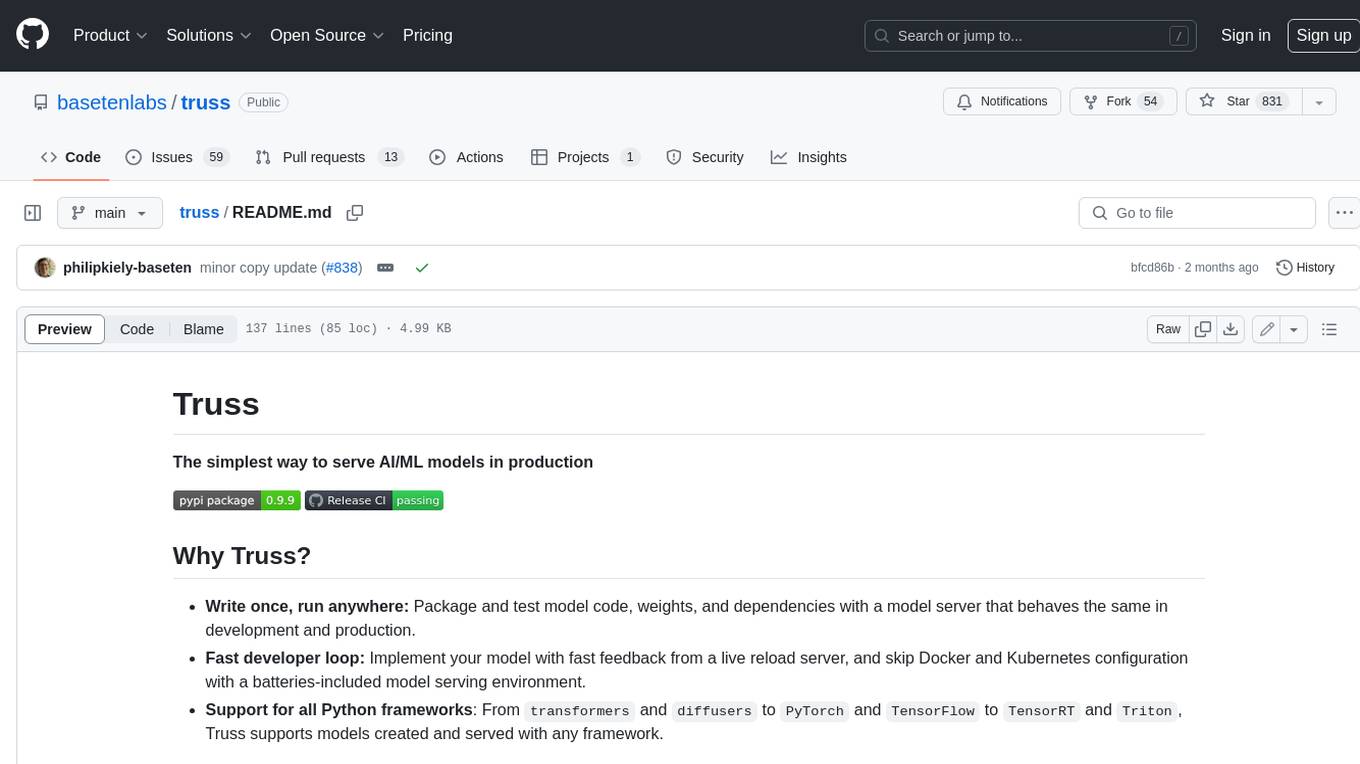
truss
Truss is a tool that simplifies the process of serving AI/ML models in production. It provides a consistent and easy-to-use interface for packaging, testing, and deploying models, regardless of the framework they were created with. Truss also includes a live reload server for fast feedback during development, and a batteries-included model serving environment that eliminates the need for Docker and Kubernetes configuration.
For similar tasks
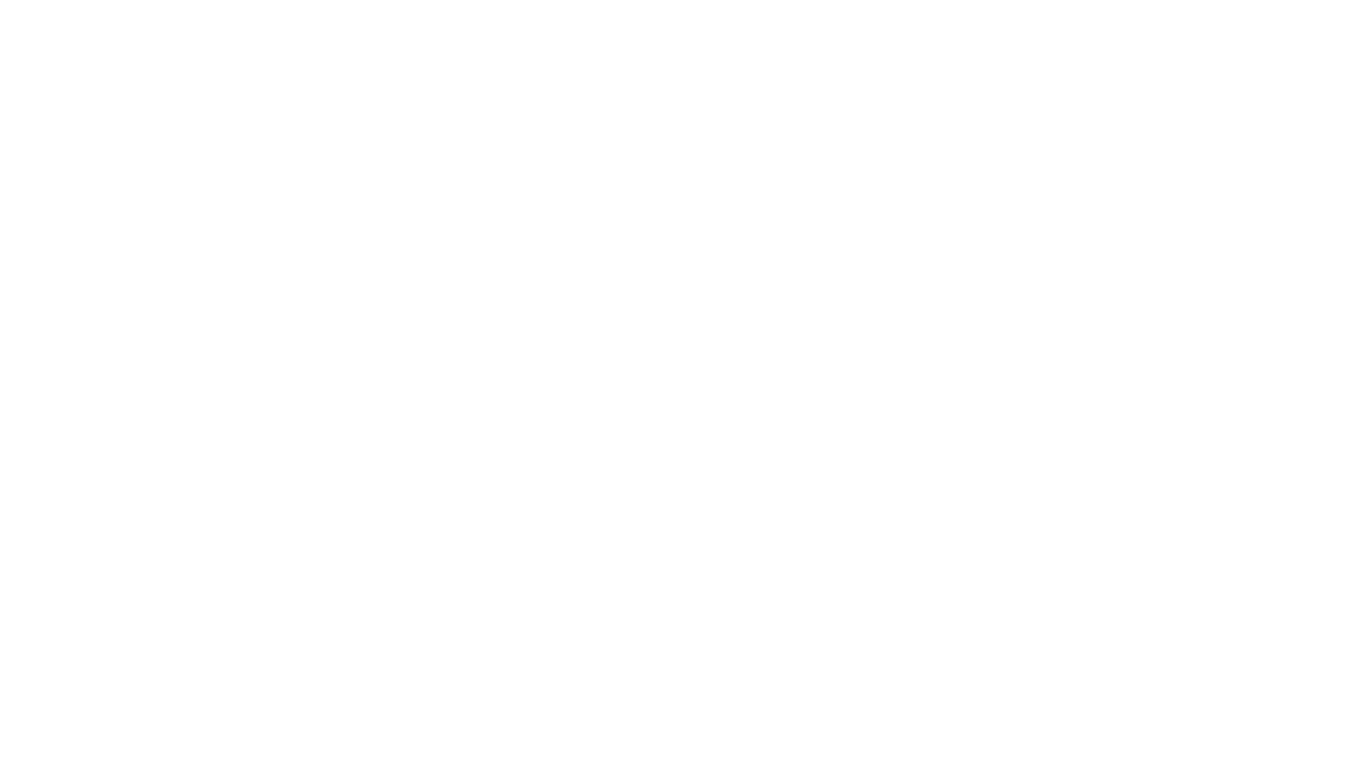
cria
Cria is a Python library designed for running Large Language Models with minimal configuration. It provides an easy and concise way to interact with LLMs, offering advanced features such as custom models, streams, message history management, and running multiple models in parallel. Cria simplifies the process of using LLMs by providing a straightforward API that requires only a few lines of code to get started. It also handles model installation automatically, making it efficient and user-friendly for various natural language processing tasks.
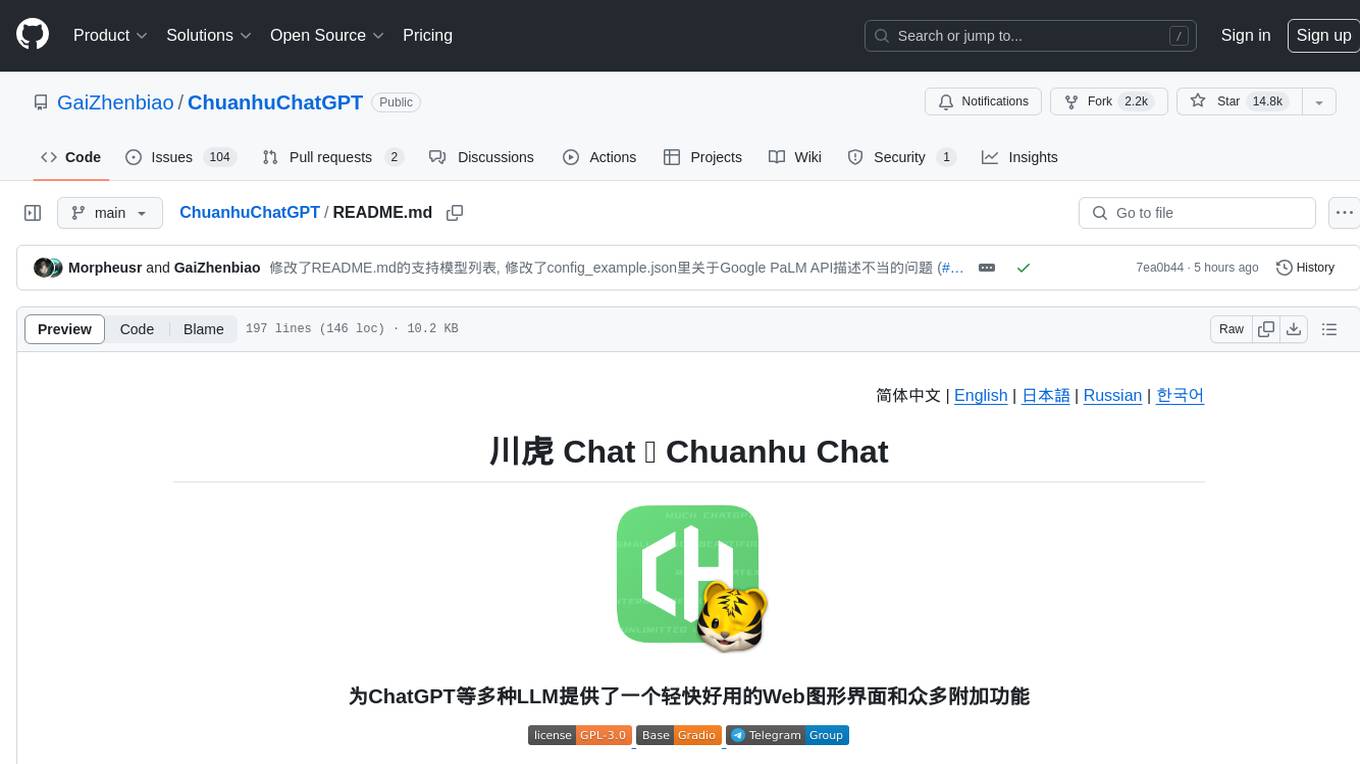
ChuanhuChatGPT
Chuanhu Chat is a user-friendly web graphical interface that provides various additional features for ChatGPT and other language models. It supports GPT-4, file-based question answering, local deployment of language models, online search, agent assistant, and fine-tuning. The tool offers a range of functionalities including auto-solving questions, online searching with network support, knowledge base for quick reading, local deployment of language models, GPT 3.5 fine-tuning, and custom model integration. It also features system prompts for effective role-playing, basic conversation capabilities with options to regenerate or delete dialogues, conversation history management with auto-saving and search functionalities, and a visually appealing user experience with themes, dark mode, LaTeX rendering, and PWA application support.
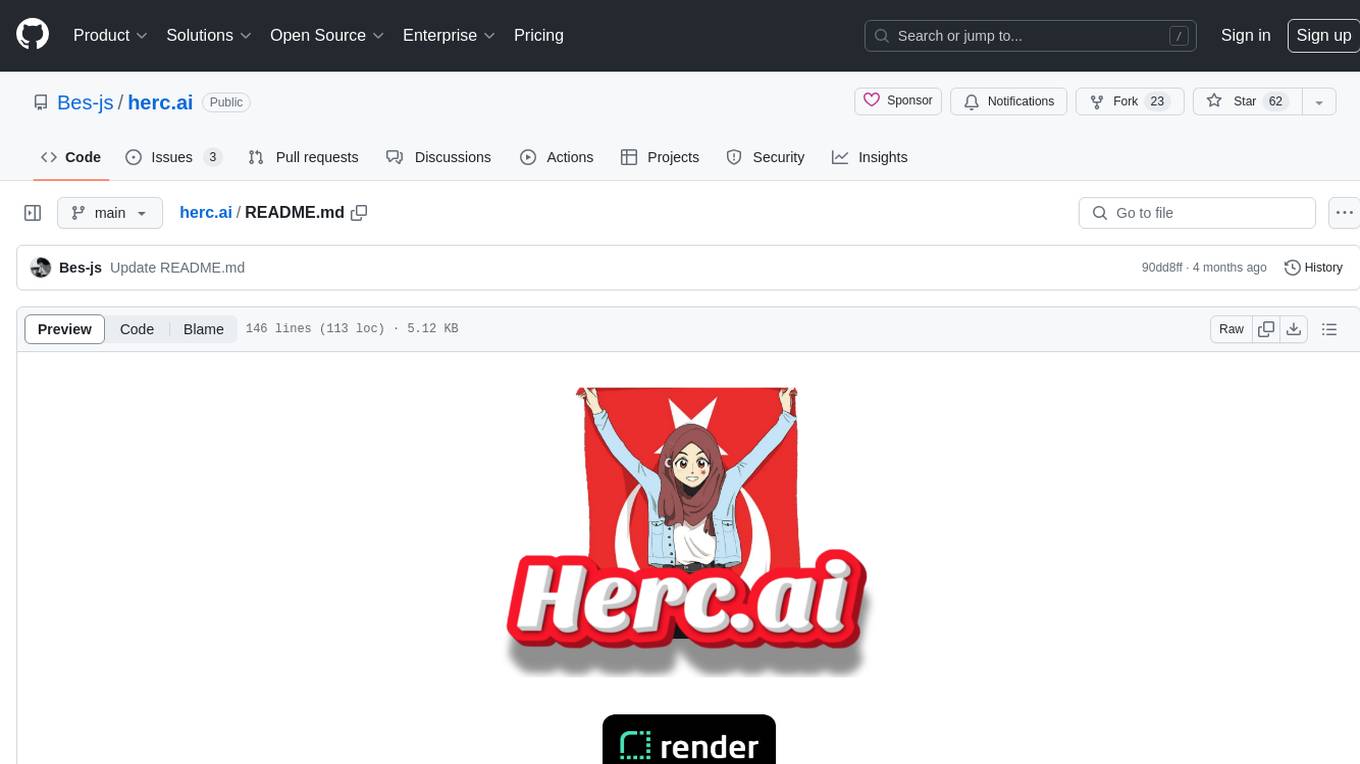
herc.ai
Herc.ai is a powerful library for interacting with the Herc.ai API. It offers free access to users and supports all languages. Users can benefit from Herc.ai's features unlimitedly with a one-time subscription and API key. The tool provides functionalities for question answering and text-to-image generation, with support for various models and customization options. Herc.ai can be easily integrated into CLI, CommonJS, TypeScript, and supports beta models for advanced usage. Developed by FiveSoBes and Luppux Development.
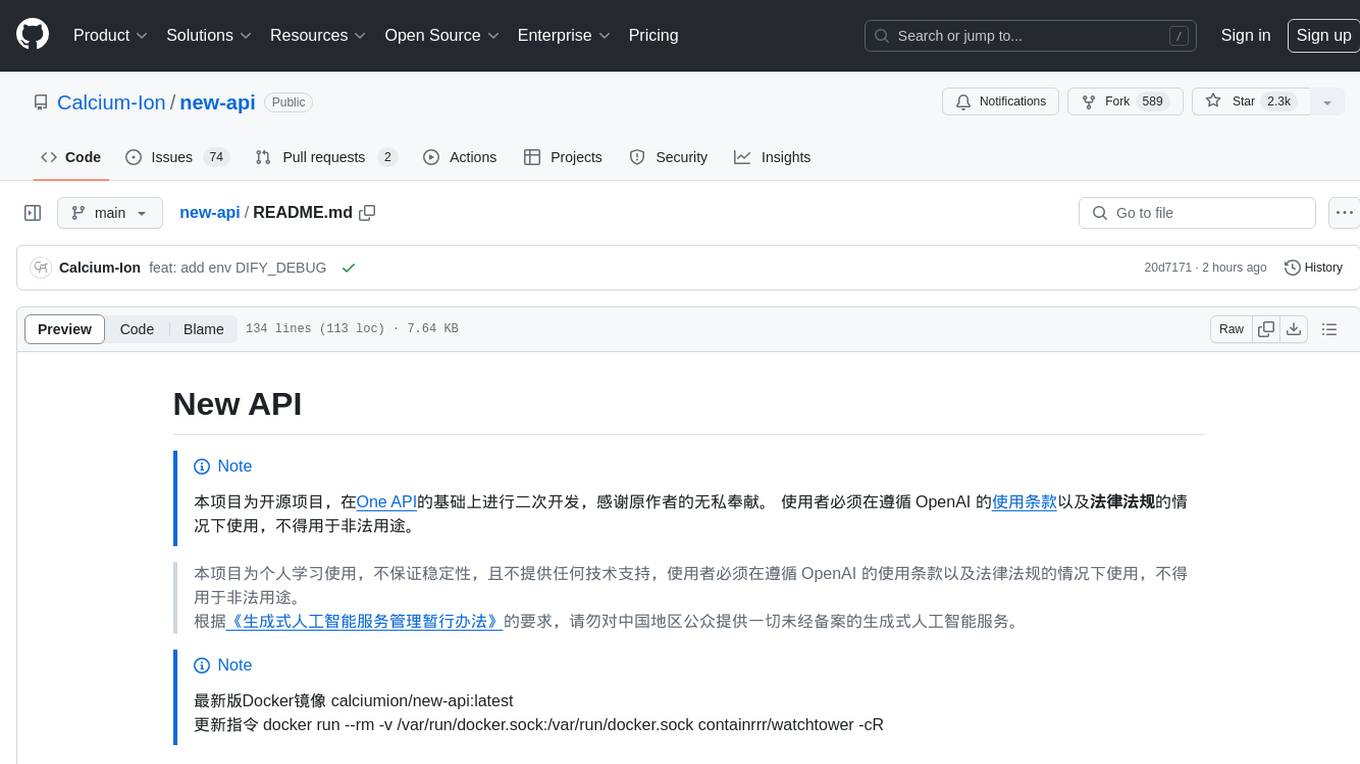
new-api
New API is an open-source project based on One API with additional features and improvements. It offers a new UI interface, supports Midjourney-Proxy(Plus) interface, online recharge functionality, model-based charging, channel weight randomization, data dashboard, token-controlled models, Telegram authorization login, Suno API support, Rerank model integration, and various third-party models. Users can customize models, retry channels, and configure caching settings. The deployment can be done using Docker with SQLite or MySQL databases. The project provides documentation for Midjourney and Suno interfaces, and it is suitable for AI enthusiasts and developers looking to enhance AI capabilities.
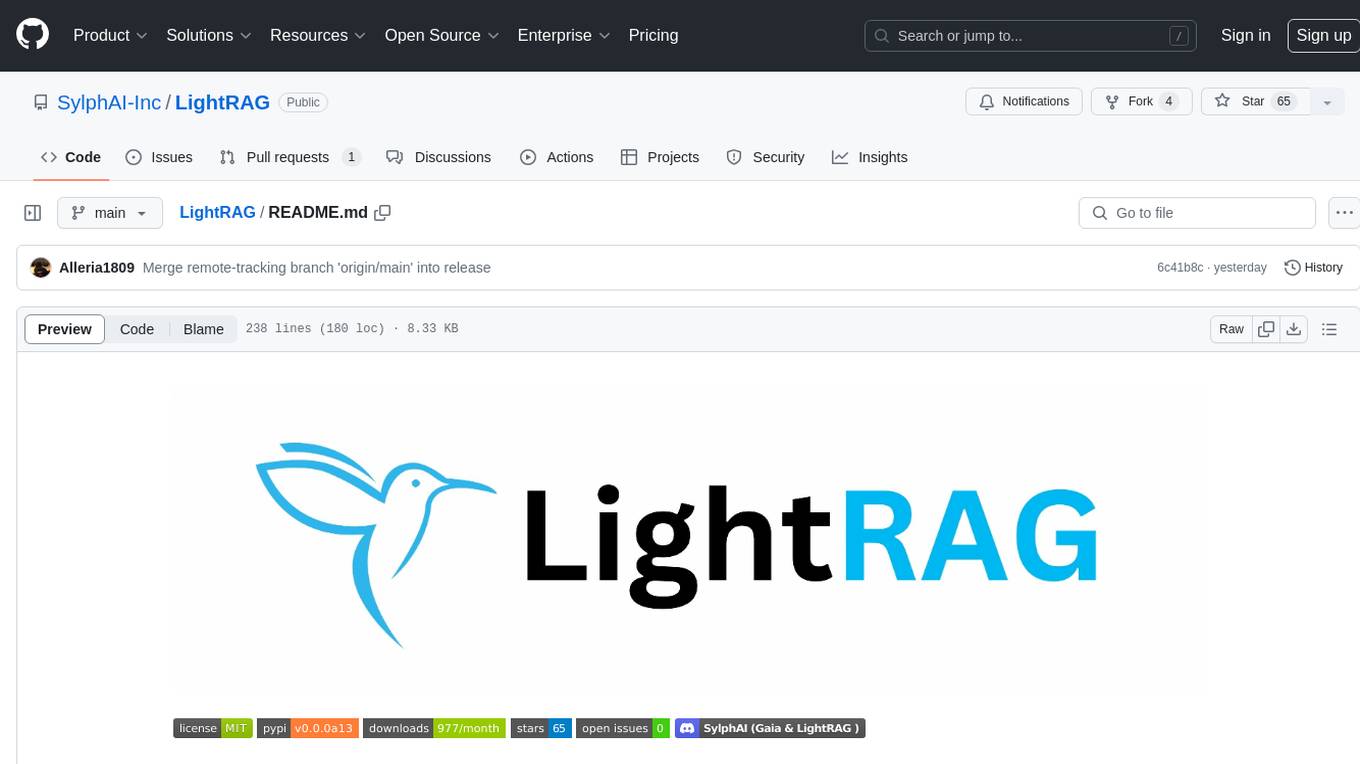
LightRAG
LightRAG is a PyTorch library designed for building and optimizing Retriever-Agent-Generator (RAG) pipelines. It follows principles of simplicity, quality, and optimization, offering developers maximum customizability with minimal abstraction. The library includes components for model interaction, output parsing, and structured data generation. LightRAG facilitates tasks like providing explanations and examples for concepts through a question-answering pipeline.
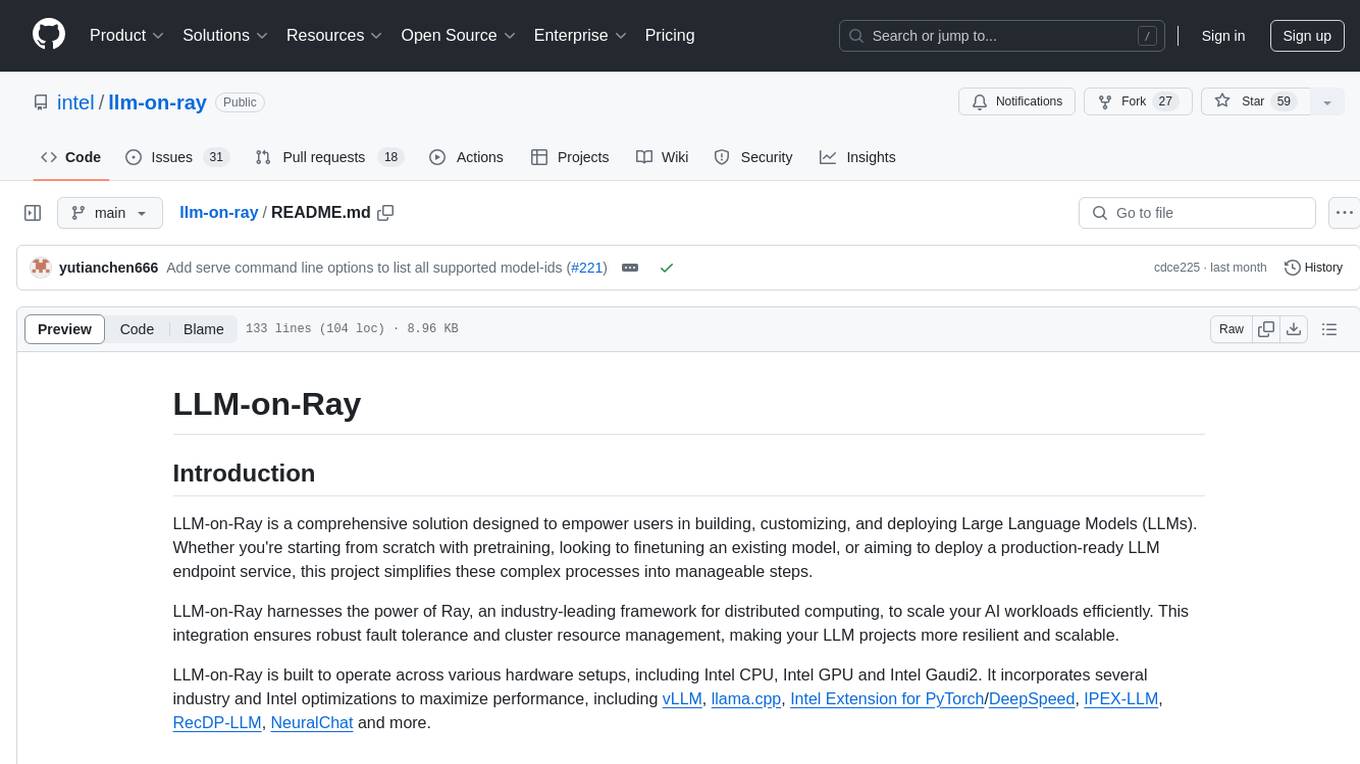
llm-on-ray
LLM-on-Ray is a comprehensive solution for building, customizing, and deploying Large Language Models (LLMs). It simplifies complex processes into manageable steps by leveraging the power of Ray for distributed computing. The tool supports pretraining, finetuning, and serving LLMs across various hardware setups, incorporating industry and Intel optimizations for performance. It offers modular workflows with intuitive configurations, robust fault tolerance, and scalability. Additionally, it provides an Interactive Web UI for enhanced usability, including a chatbot application for testing and refining models.
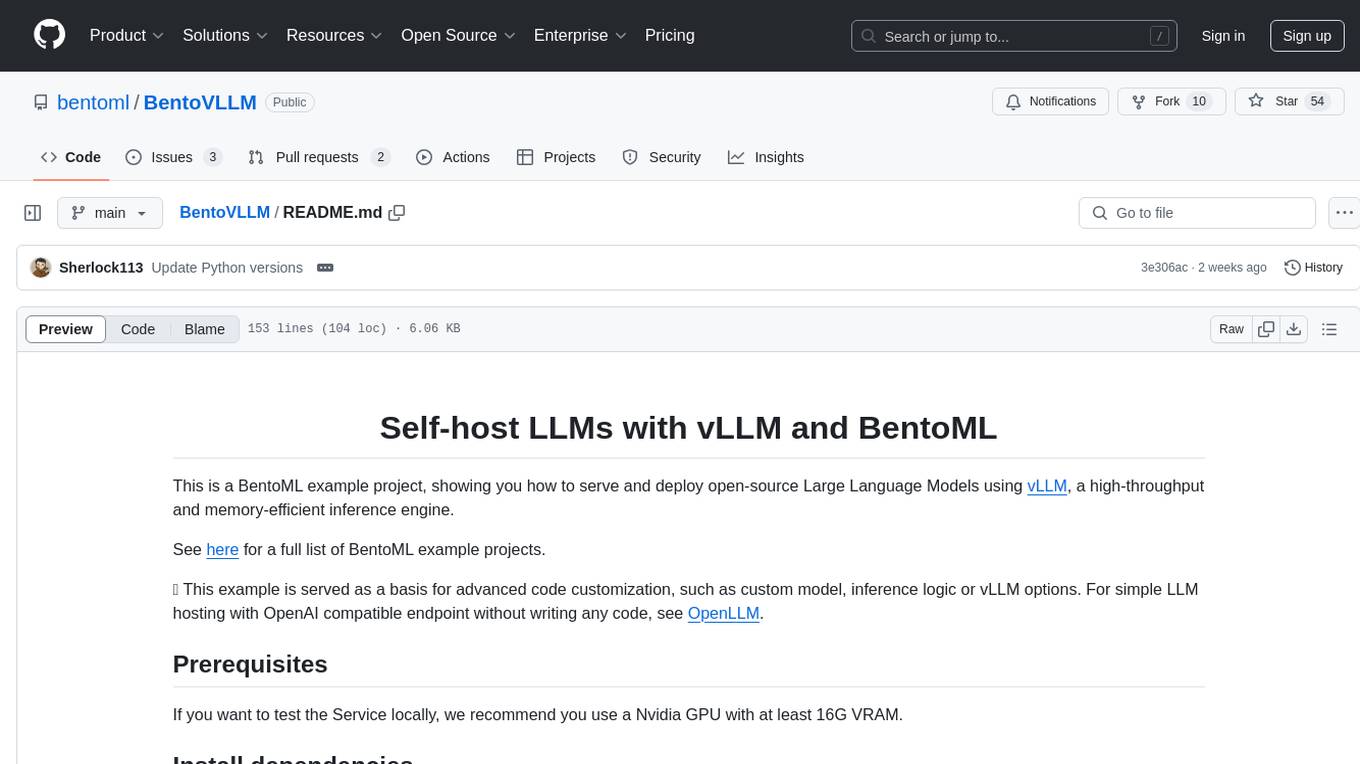
BentoVLLM
BentoVLLM is an example project demonstrating how to serve and deploy open-source Large Language Models using vLLM, a high-throughput and memory-efficient inference engine. It provides a basis for advanced code customization, such as custom models, inference logic, or vLLM options. The project allows for simple LLM hosting with OpenAI compatible endpoints without the need to write any code. Users can interact with the server using Swagger UI or other methods, and the service can be deployed to BentoCloud for better management and scalability. Additionally, the repository includes integration examples for different LLM models and tools.
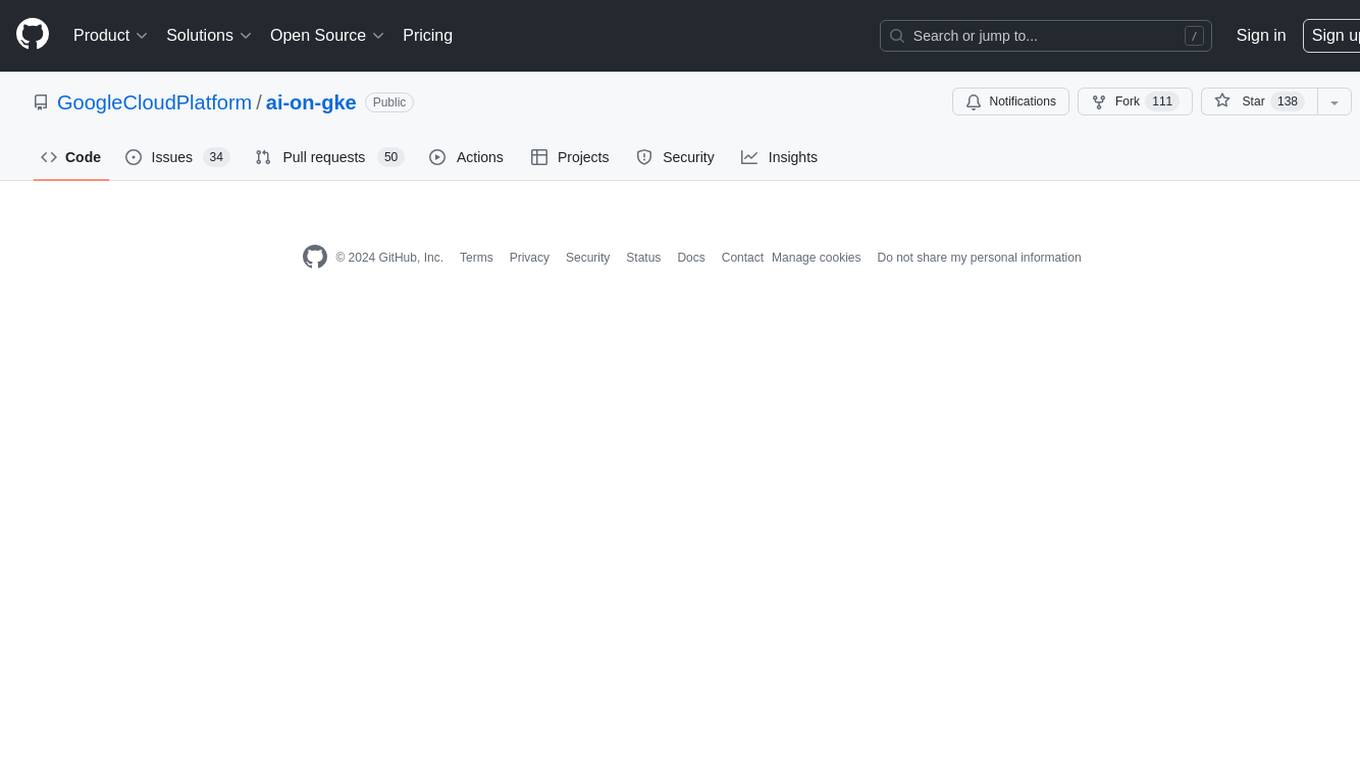
ai-on-gke
This repository contains assets related to AI/ML workloads on Google Kubernetes Engine (GKE). Run optimized AI/ML workloads with Google Kubernetes Engine (GKE) platform orchestration capabilities. A robust AI/ML platform considers the following layers: Infrastructure orchestration that support GPUs and TPUs for training and serving workloads at scale Flexible integration with distributed computing and data processing frameworks Support for multiple teams on the same infrastructure to maximize utilization of resources
For similar jobs
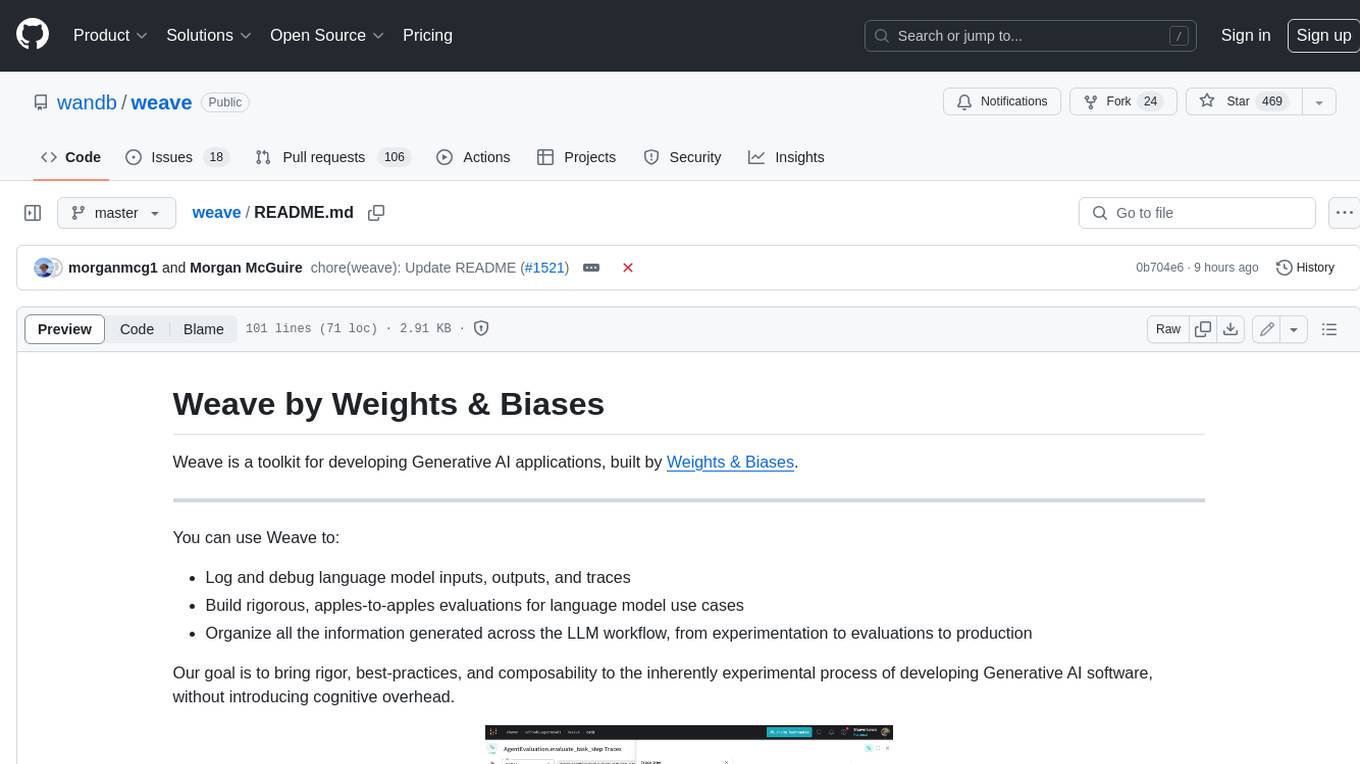
weave
Weave is a toolkit for developing Generative AI applications, built by Weights & Biases. With Weave, you can log and debug language model inputs, outputs, and traces; build rigorous, apples-to-apples evaluations for language model use cases; and organize all the information generated across the LLM workflow, from experimentation to evaluations to production. Weave aims to bring rigor, best-practices, and composability to the inherently experimental process of developing Generative AI software, without introducing cognitive overhead.
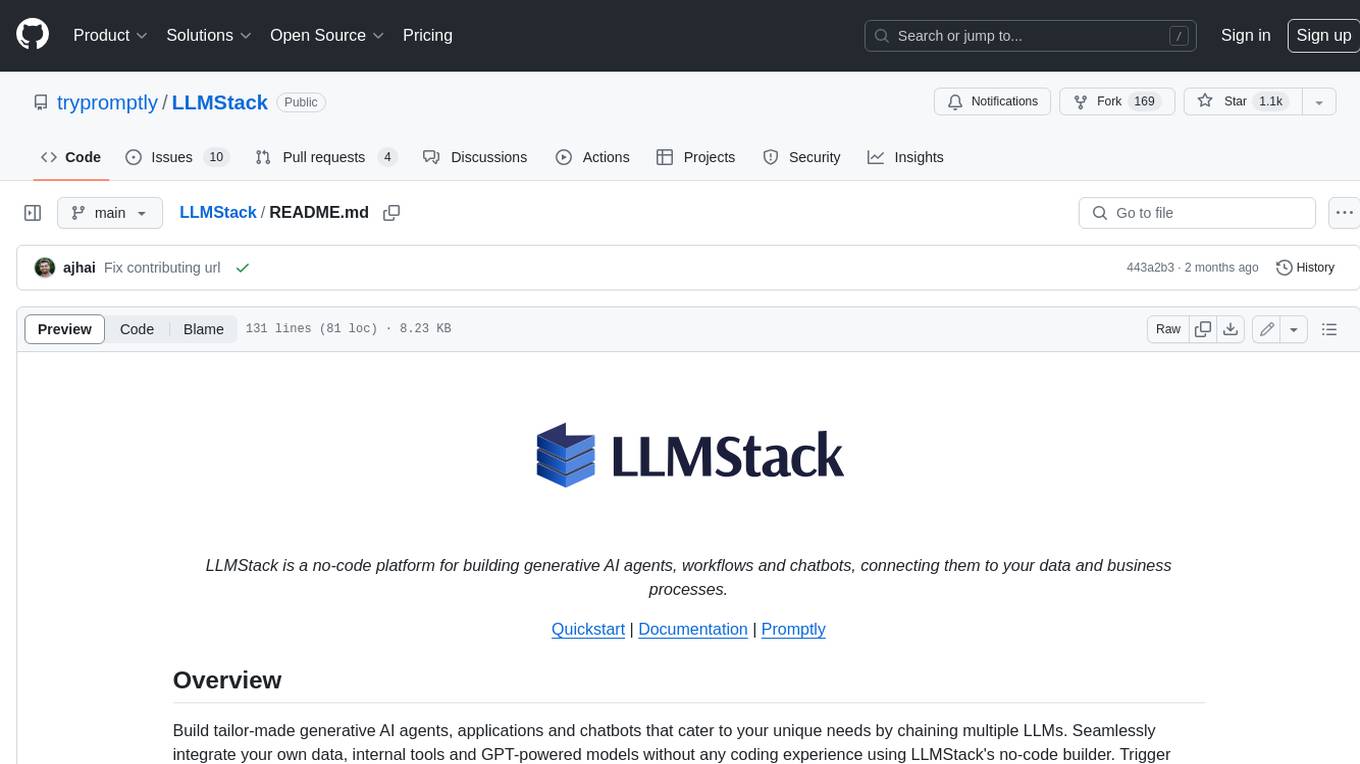
LLMStack
LLMStack is a no-code platform for building generative AI agents, workflows, and chatbots. It allows users to connect their own data, internal tools, and GPT-powered models without any coding experience. LLMStack can be deployed to the cloud or on-premise and can be accessed via HTTP API or triggered from Slack or Discord.
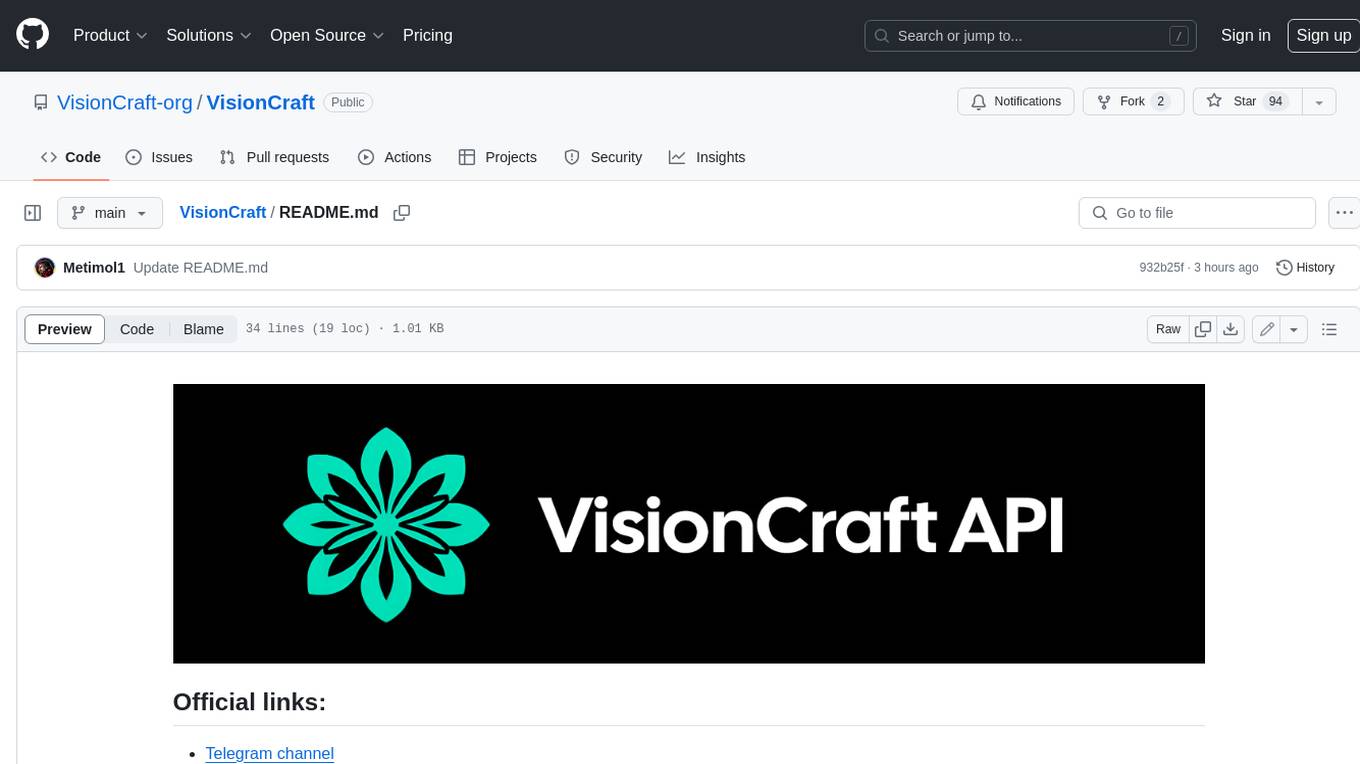
VisionCraft
The VisionCraft API is a free API for using over 100 different AI models. From images to sound.
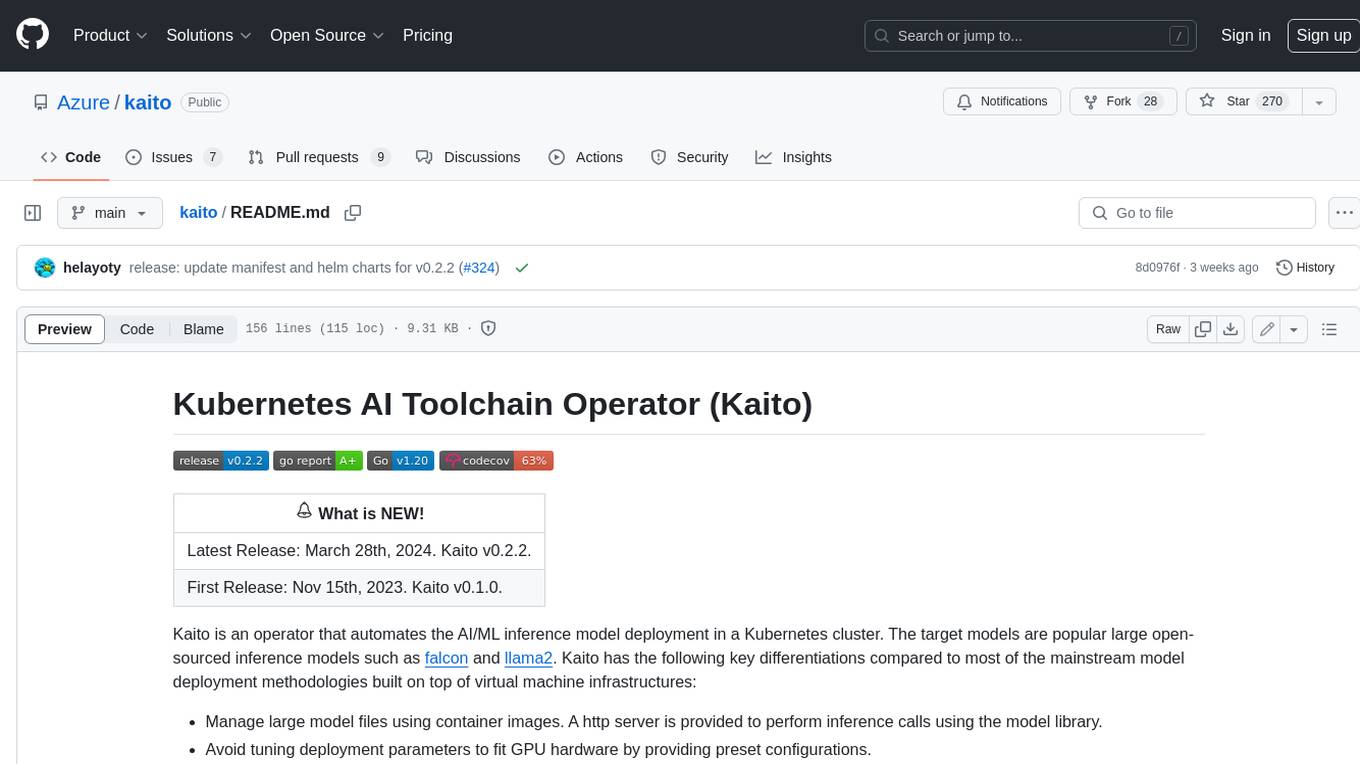
kaito
Kaito is an operator that automates the AI/ML inference model deployment in a Kubernetes cluster. It manages large model files using container images, avoids tuning deployment parameters to fit GPU hardware by providing preset configurations, auto-provisions GPU nodes based on model requirements, and hosts large model images in the public Microsoft Container Registry (MCR) if the license allows. Using Kaito, the workflow of onboarding large AI inference models in Kubernetes is largely simplified.
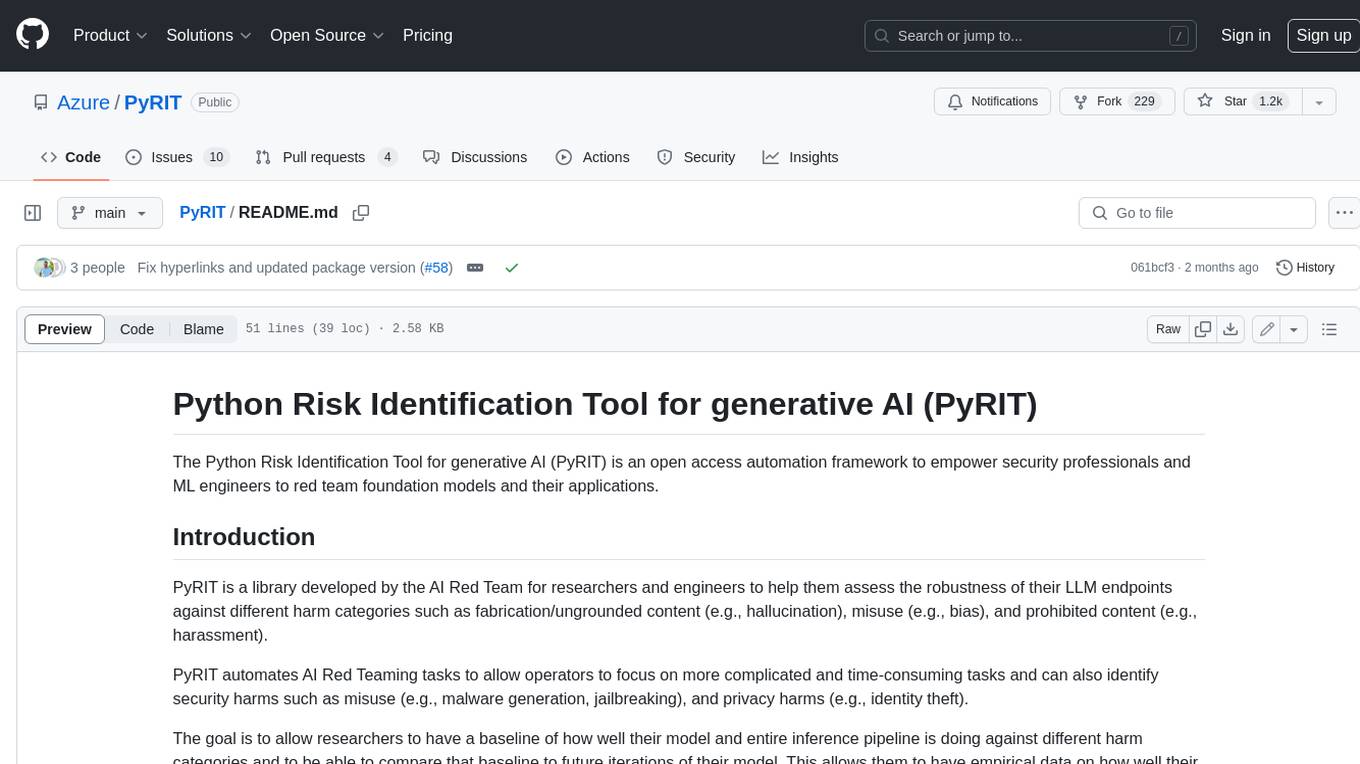
PyRIT
PyRIT is an open access automation framework designed to empower security professionals and ML engineers to red team foundation models and their applications. It automates AI Red Teaming tasks to allow operators to focus on more complicated and time-consuming tasks and can also identify security harms such as misuse (e.g., malware generation, jailbreaking), and privacy harms (e.g., identity theft). The goal is to allow researchers to have a baseline of how well their model and entire inference pipeline is doing against different harm categories and to be able to compare that baseline to future iterations of their model. This allows them to have empirical data on how well their model is doing today, and detect any degradation of performance based on future improvements.
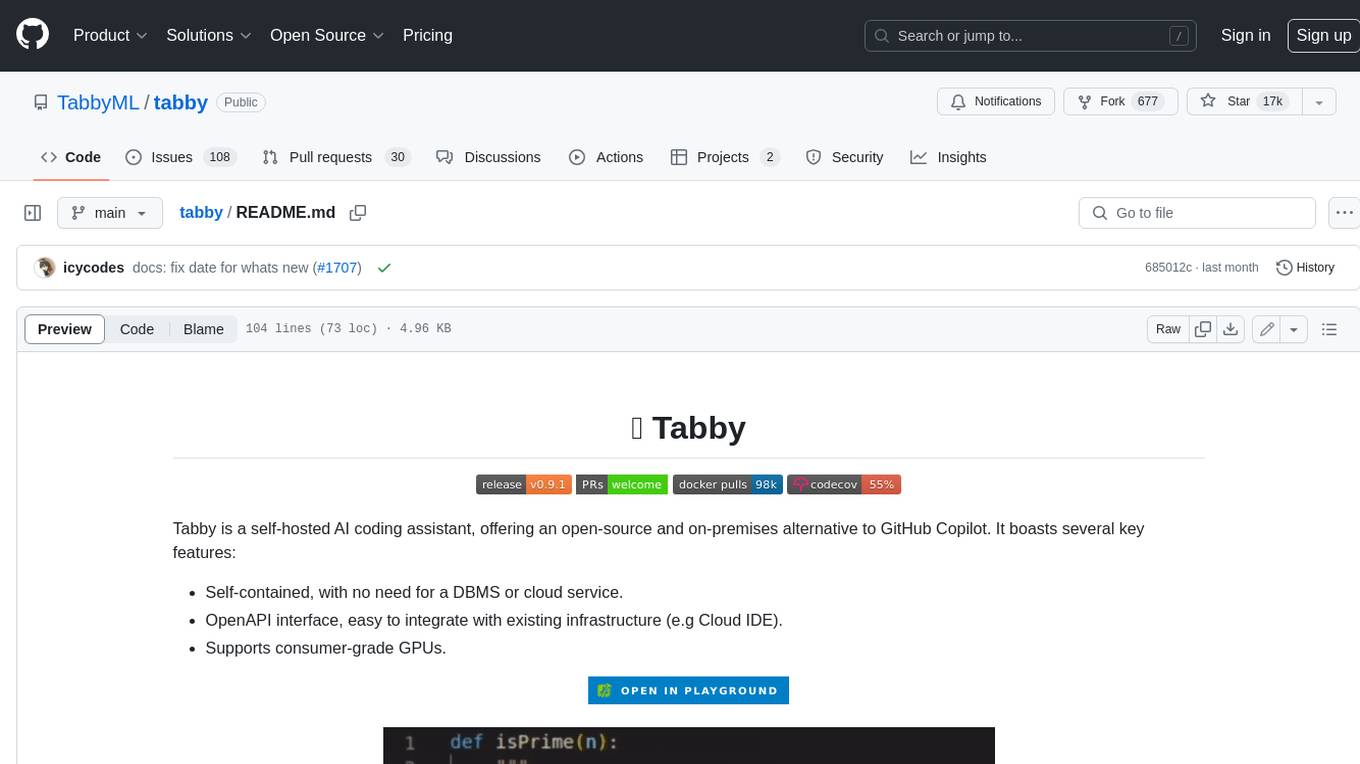
tabby
Tabby is a self-hosted AI coding assistant, offering an open-source and on-premises alternative to GitHub Copilot. It boasts several key features: * Self-contained, with no need for a DBMS or cloud service. * OpenAPI interface, easy to integrate with existing infrastructure (e.g Cloud IDE). * Supports consumer-grade GPUs.
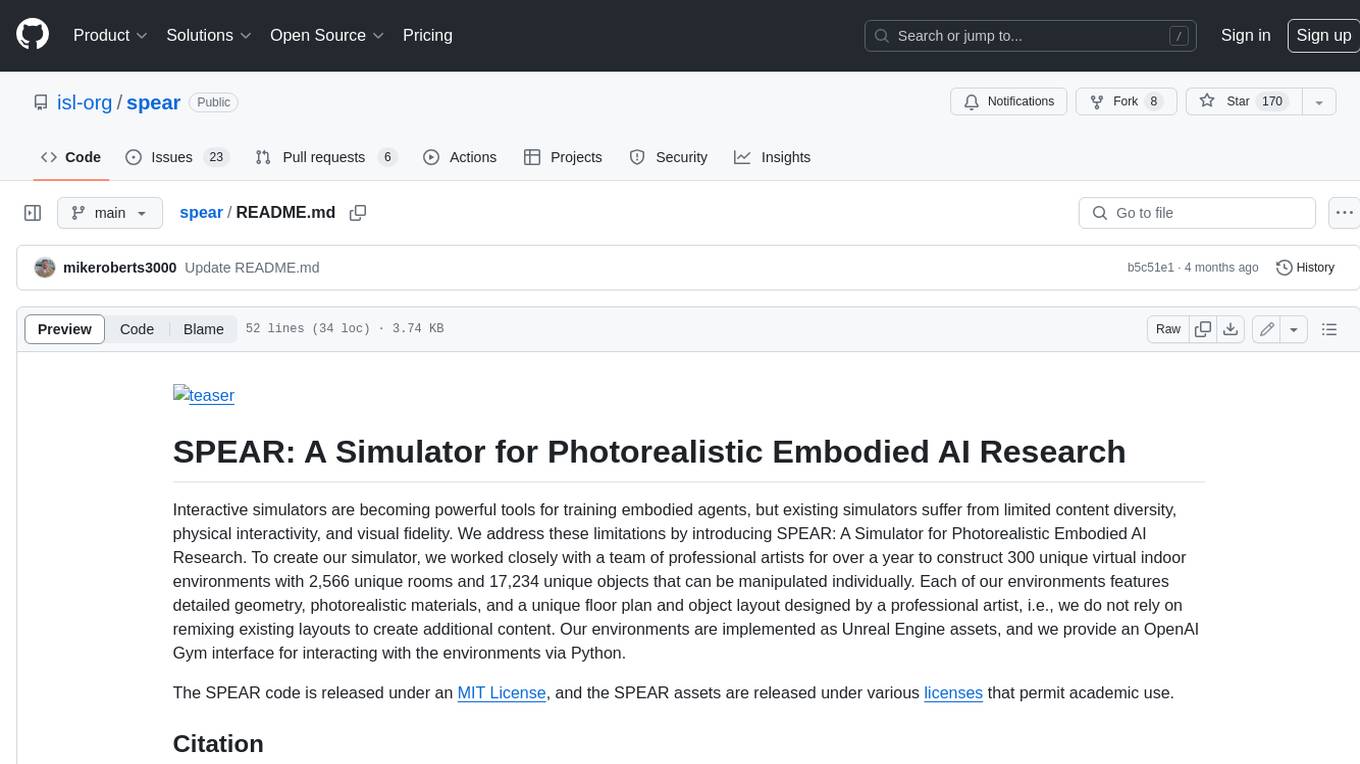
spear
SPEAR (Simulator for Photorealistic Embodied AI Research) is a powerful tool for training embodied agents. It features 300 unique virtual indoor environments with 2,566 unique rooms and 17,234 unique objects that can be manipulated individually. Each environment is designed by a professional artist and features detailed geometry, photorealistic materials, and a unique floor plan and object layout. SPEAR is implemented as Unreal Engine assets and provides an OpenAI Gym interface for interacting with the environments via Python.
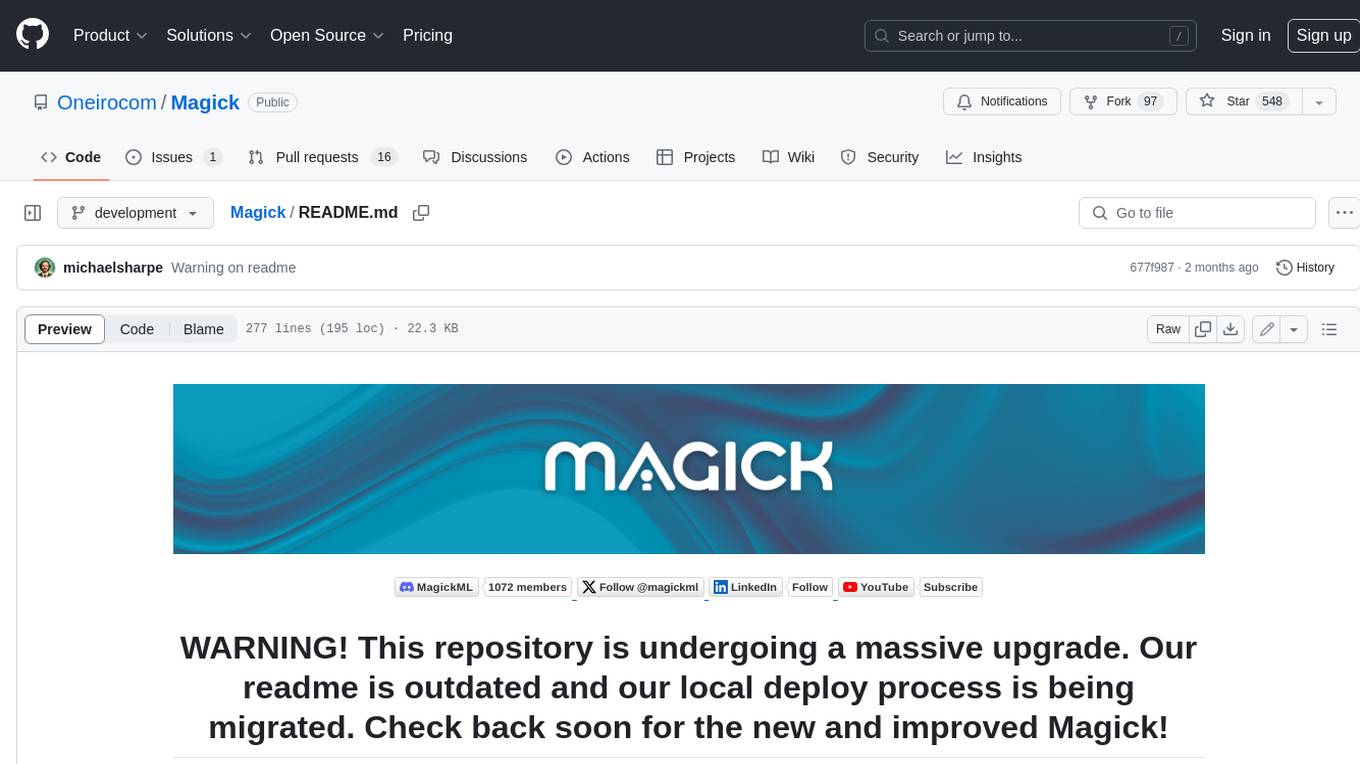
Magick
Magick is a groundbreaking visual AIDE (Artificial Intelligence Development Environment) for no-code data pipelines and multimodal agents. Magick can connect to other services and comes with nodes and templates well-suited for intelligent agents, chatbots, complex reasoning systems and realistic characters.