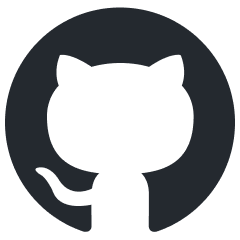
abliteration
Make abliterated models with transformers, easy and fast
Stars: 64
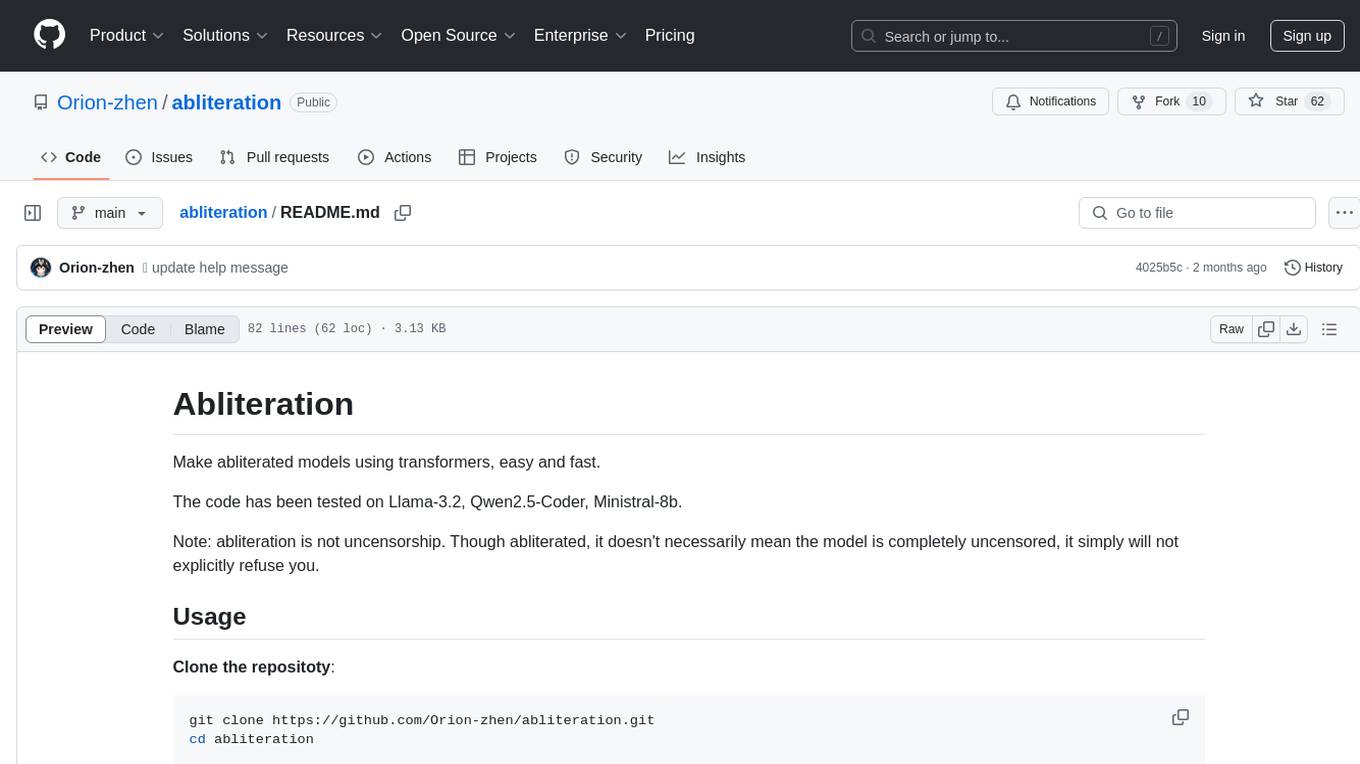
Abliteration is a tool that allows users to create abliterated models using transformers quickly and easily. It is not a tool for uncensorship, but rather for making models that will not explicitly refuse users. Users can clone the repository, install dependencies, and make abliterations using the provided commands. The tool supports adjusting parameters for stubborn models and offers various options for customization. Abliteration can be used for creating modified models for specific tasks or topics.
README:
Make abliterated models using transformers, easy and fast.
There exist some directions that make LLMs to refuse users' input. Abliteration is a technique that can calculate the most significant refusal directions with harmful and harmless prompts, and then remove them from the model. This is a crude, proof-of-concept implementation to remove refusals from an LLM model without using TransformerLens.
The code has been tested on Llama-3.2, Qwen2.5-Coder, Ministral-8b.
VRAM/RAM requirements: This repository has been making efforts to reduce VRAM usage. You can abliterate whatever model you want, as long as it fits in your VRAM. Loading model in 4-bit precision using bitsandbytes is recommended for large models if you have limited VRAM. However, I always assume that you have enough memory to load the bf16 model.
[!NOTE] Abliteration is not uncensorment. Though abliterated, it doesn't necessarily mean the model is completely uncensored, it simply will not explicitly refuse you, theoretically.
git clone https://github.com/Orion-zhen/abliteration.git && cd abliteration
pip install -r requirements.txt
python abliterate.py -m <path_to_your_model> -o <output_dir>
python chat.py -m <path_to_your_abliterated_model>
python compare.py -a <model_a> -b <model_b>
- Abliterate Llama-3.2:
python abliterate.py -m meta-llama/Llama-3.2-3B-Instruct -o llama3.2-3b-abliterated
- Load model in 4-bit precision using bitsandbytes:
python abliterate.py -m meta-llama/Llama-3.2-3B-Instruct -o llama3.2-3b-abliterated --load-in-4bit
- Compare your abliterated model with the original model:
python compare.py -a meta-llama/Llama-3.2-3B-Instruct -b llama3.2-3b-abliterated
- Compare in 4-bit precision using bitsandbytes:
python compare.py -a meta-llama/Llama-3.2-3B-Instruct -b llama3.2-3b-abliterated --load-in-4bit
[!NOTE] If you use
--load-in-4bit
or--load-in-8bit
, then I will assume you are lack of VRAM, and the final appliance step will be performed with CPU and memory. Please make sure you have enough memory to load the bf16 model.
Now your model will be abliterated and saved to <output_dir>
. Once it finishes, you can immediately chat with your abliterated model in the terminal. For Chinese models, you can use --deccp
to abliterate it from certain topics.
This repository now supports .json
config file. This file should contain a dict
of config key value pairs. For example:
{
"model": "/absolute/path/to/your/model",
"output": "/output/dir",
"data-harmful": "/absolute/path/to/harmful-prompts.txt",
"scale-factor": 114,
"load-in-4bit": true
}
python abliterate.py -c config.json
Loading config file will overwrite command line arguments.
You can use your own prompts to abliterate your model. Supported file formats are .txt
, .parquet
and .json
. Detailed formats are listed below:
-
.txt
: Each line of the file is a prompt -
.parquet
: A parquet file with columntext
-
.json
: A json file with list of strings
Then load your own prompts using --data-harmful
and --data-harmless
arguments:
python abliterate.py -m <path_to_your_model> -o <output_dir> --data-harmful /path/to/my/harmful.txt --data-harmless /path/to/my/harmless.txt
You can use --scale-factor
to control the abliteration strength. A scale factor larger then 1 will impose stronger removal of refusals, while a negative scale factor will encourage refusal. You can try to increase the scale factor to see if it helps.
python abliterate.py -m <path_to_your_model> -o <output_dir> --scale-factor 1.5
You can output the refusals to a file using --output-refusals
argument:
python abliterate.py -m <path_to_your_model> -o <output_dir> --output-refusals refusals.bin
And load the refusals back using --load-refusals
argument:
python abliterate.py -m <path_to_your_model> --input-refusals refusals.bin -o <output_dir>
If --input-refusal
is provided, the script will not compute refusal directions again.
By default, abliteration will be applied to o_proj
and down_proj
. You can add more targets by modifying the code below, as long as it won't mess up the model:
# utils/apply.py, apply_abliteration()
lm_model.layers[layer_idx].self_attn.o_proj.weight = modify_tensor(
lm_model.layers[layer_idx].self_attn.o_proj.weight.data,
refusal_dir,
scale_factor,
)
lm_model.layers[layer_idx].mlp.down_proj.weight = modify_tensor(
lm_model.layers[layer_idx].mlp.down_proj.weight.data,
refusal_dir,
scale_factor,
)
Available targets can be found in transformers model architectures and mergekit model architectures.
This repository provides a bunch of parameters to optimize. To get the best results, you can try the following steps:
- Carefully choose your prompts. Prompts in this repository is a general example, you can use your own prompts to get better results.
- Adjust parameters. The script provides various parameters to control the abliteration progress. You can try different values to see if it helps.
- Change the targets. You can modify the code to abliterate other targets, as long as it won't mess up the model.
- If you have limited VRAM, try
--load-in-4bit
or--load-in-8bit
to load the model in 4-bit or 8-bit precision.
Use --help
to see all available arguments:
python abliterate.py --help
For Tasks:
Click tags to check more tools for each tasksFor Jobs:
Alternative AI tools for abliteration
Similar Open Source Tools
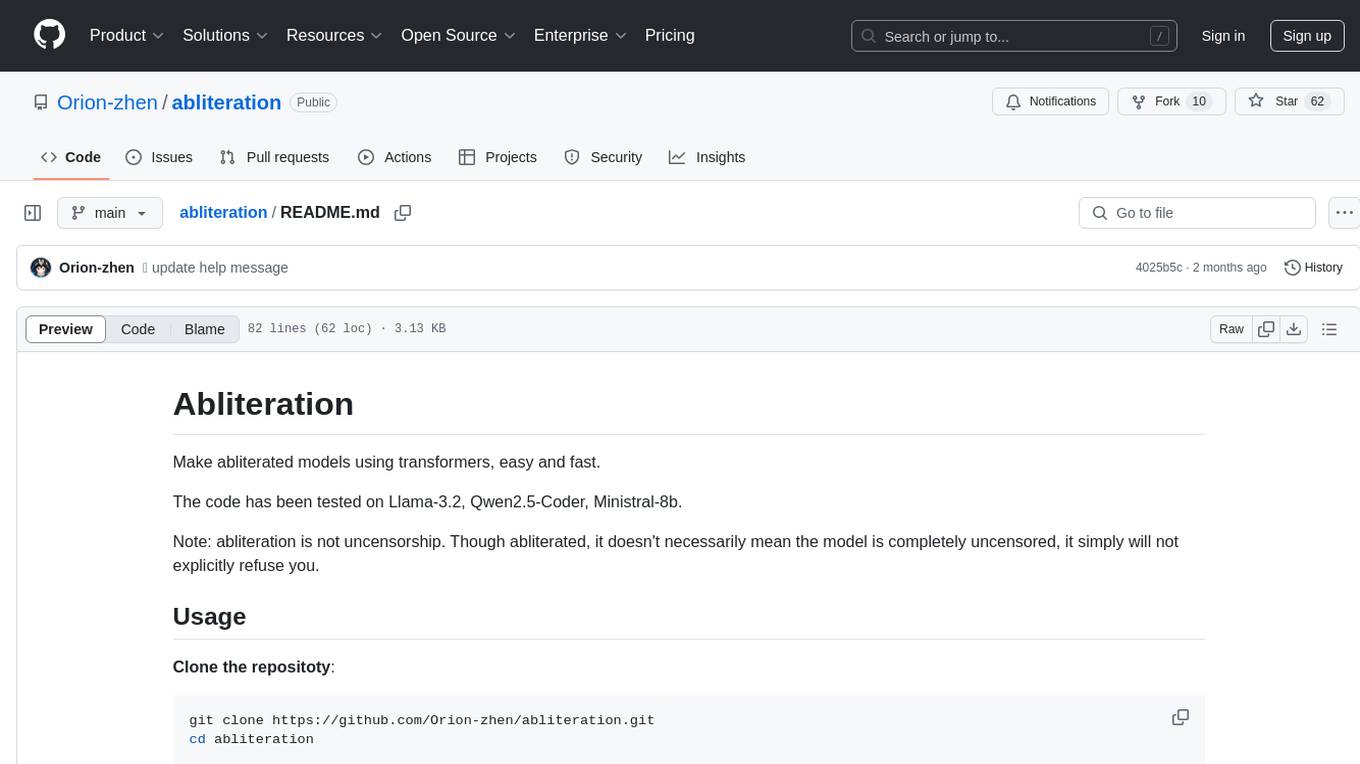
abliteration
Abliteration is a tool that allows users to create abliterated models using transformers quickly and easily. It is not a tool for uncensorship, but rather for making models that will not explicitly refuse users. Users can clone the repository, install dependencies, and make abliterations using the provided commands. The tool supports adjusting parameters for stubborn models and offers various options for customization. Abliteration can be used for creating modified models for specific tasks or topics.
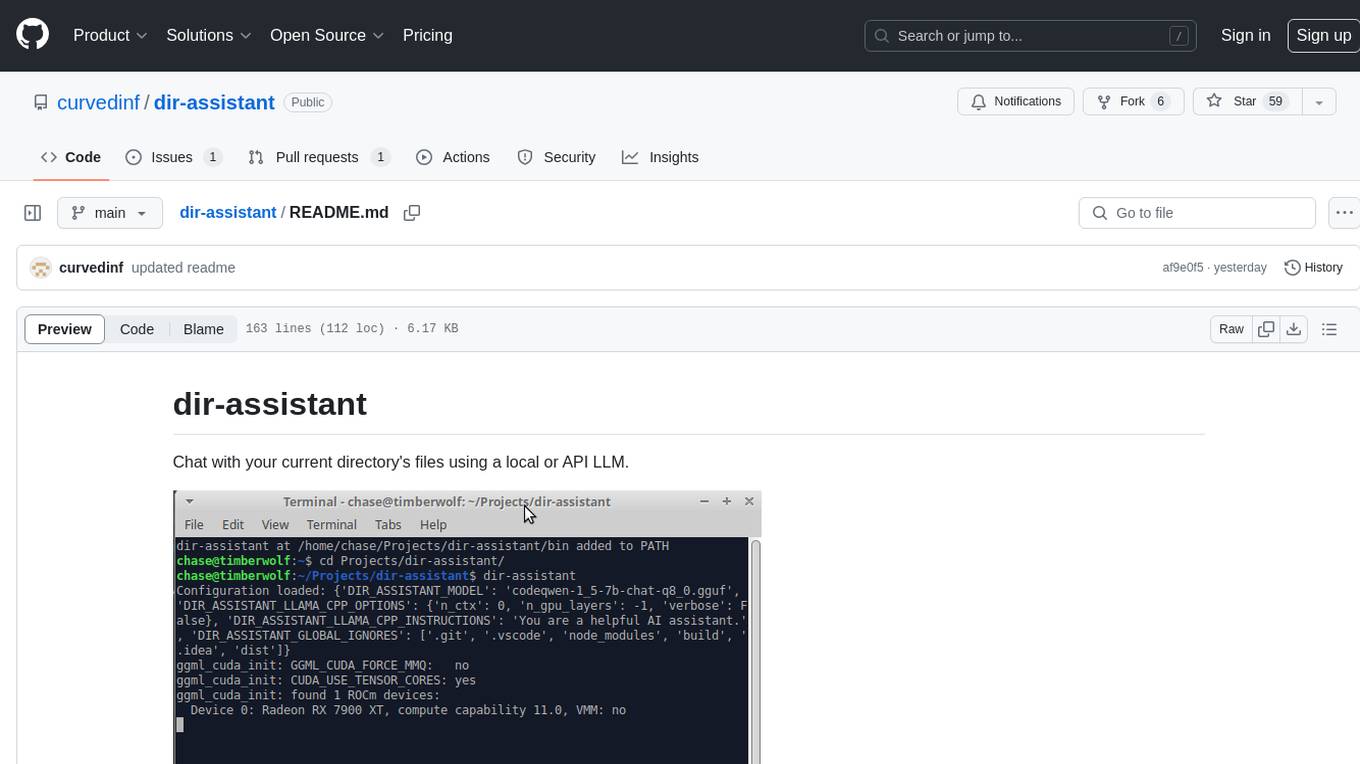
dir-assistant
Dir-assistant is a tool that allows users to interact with their current directory's files using local or API Language Models (LLMs). It supports various platforms and provides API support for major LLM APIs. Users can configure and customize their local LLMs and API LLMs using the tool. Dir-assistant also supports model downloads and configurations for efficient usage. It is designed to enhance file interaction and retrieval using advanced language models.
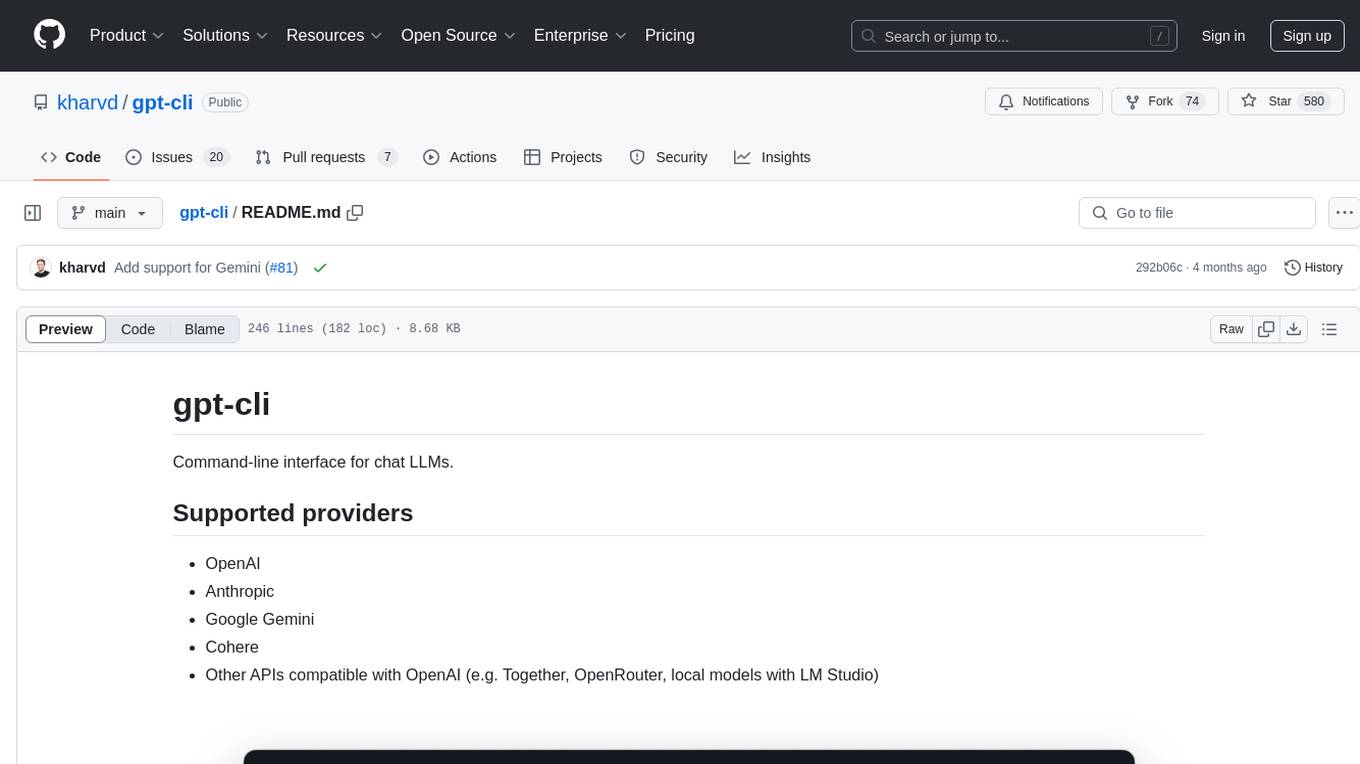
gpt-cli
gpt-cli is a command-line interface tool for interacting with various chat language models like ChatGPT, Claude, and others. It supports model customization, usage tracking, keyboard shortcuts, multi-line input, markdown support, predefined messages, and multiple assistants. Users can easily switch between different assistants, define custom assistants, and configure model parameters and API keys in a YAML file for easy customization and management.
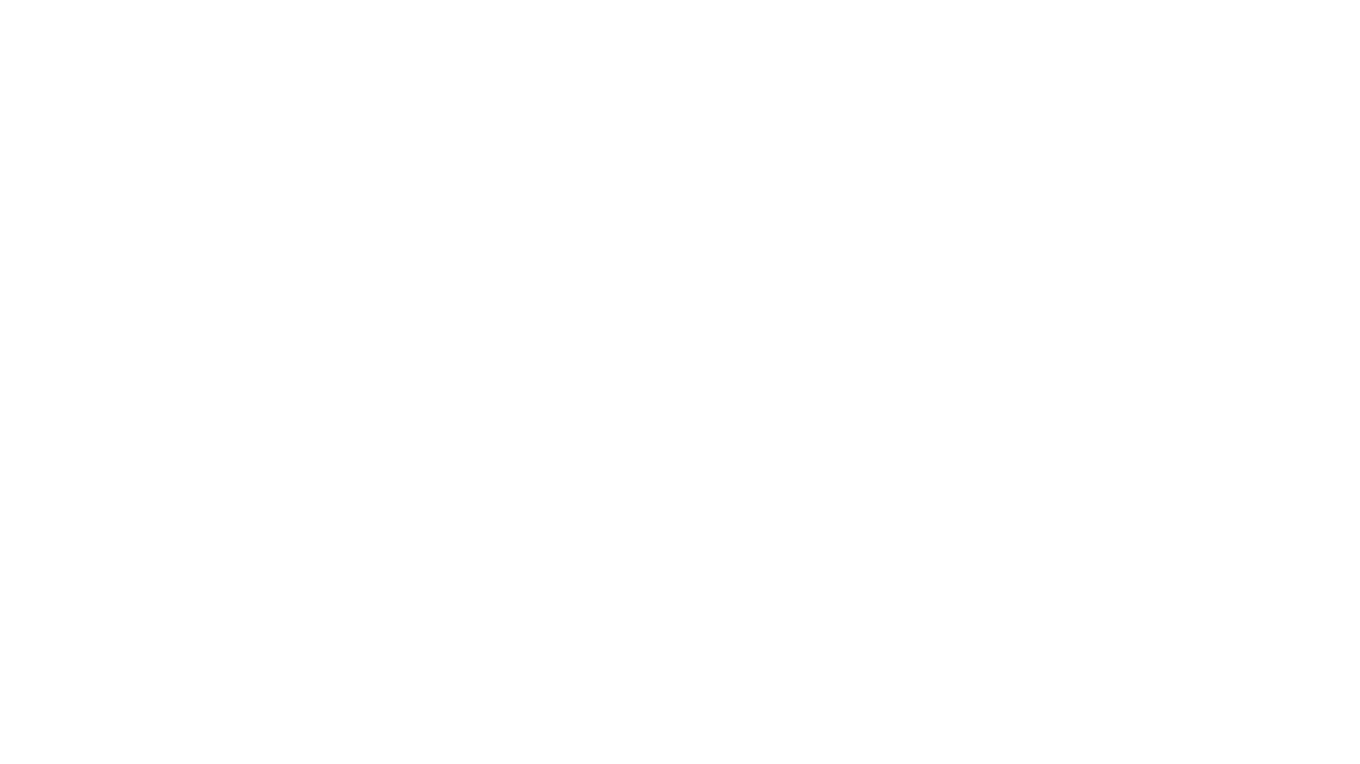
reader
Reader is a tool that converts any URL to an LLM-friendly input with a simple prefix `https://r.jina.ai/`. It improves the output for your agent and RAG systems at no cost. Reader supports image reading, captioning all images at the specified URL and adding `Image [idx]: [caption]` as an alt tag. This enables downstream LLMs to interact with the images in reasoning, summarizing, etc. Reader offers a streaming mode, useful when the standard mode provides an incomplete result. In streaming mode, Reader waits a bit longer until the page is fully rendered, providing more complete information. Reader also supports a JSON mode, which contains three fields: `url`, `title`, and `content`. Reader is backed by Jina AI and licensed under Apache-2.0.
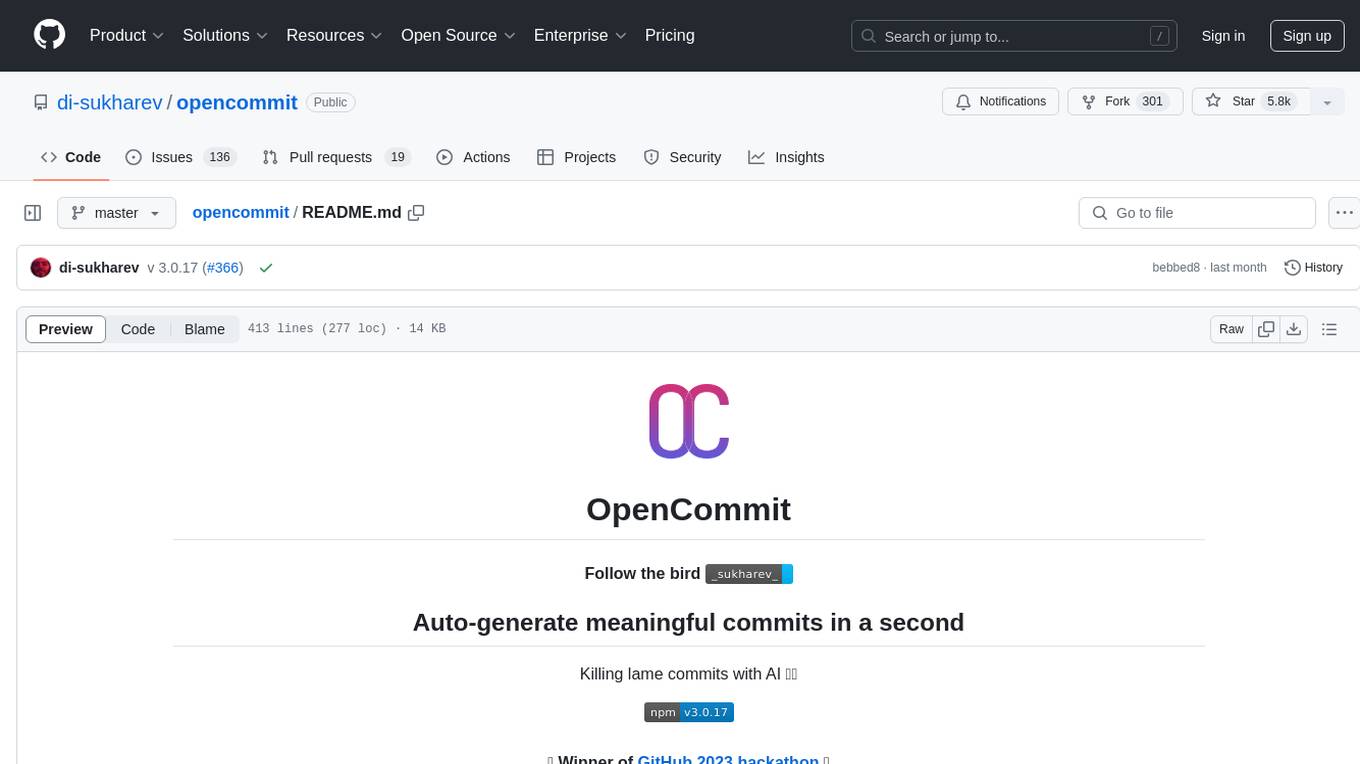
opencommit
OpenCommit is a tool that auto-generates meaningful commits using AI, allowing users to quickly create commit messages for their staged changes. It provides a CLI interface for easy usage and supports customization of commit descriptions, emojis, and AI models. Users can configure local and global settings, switch between different AI providers, and set up Git hooks for integration with IDE Source Control. Additionally, OpenCommit can be used as a GitHub Action to automatically improve commit messages on push events, ensuring all commits are meaningful and not generic. Payments for OpenAI API requests are handled by the user, with the tool storing API keys locally.
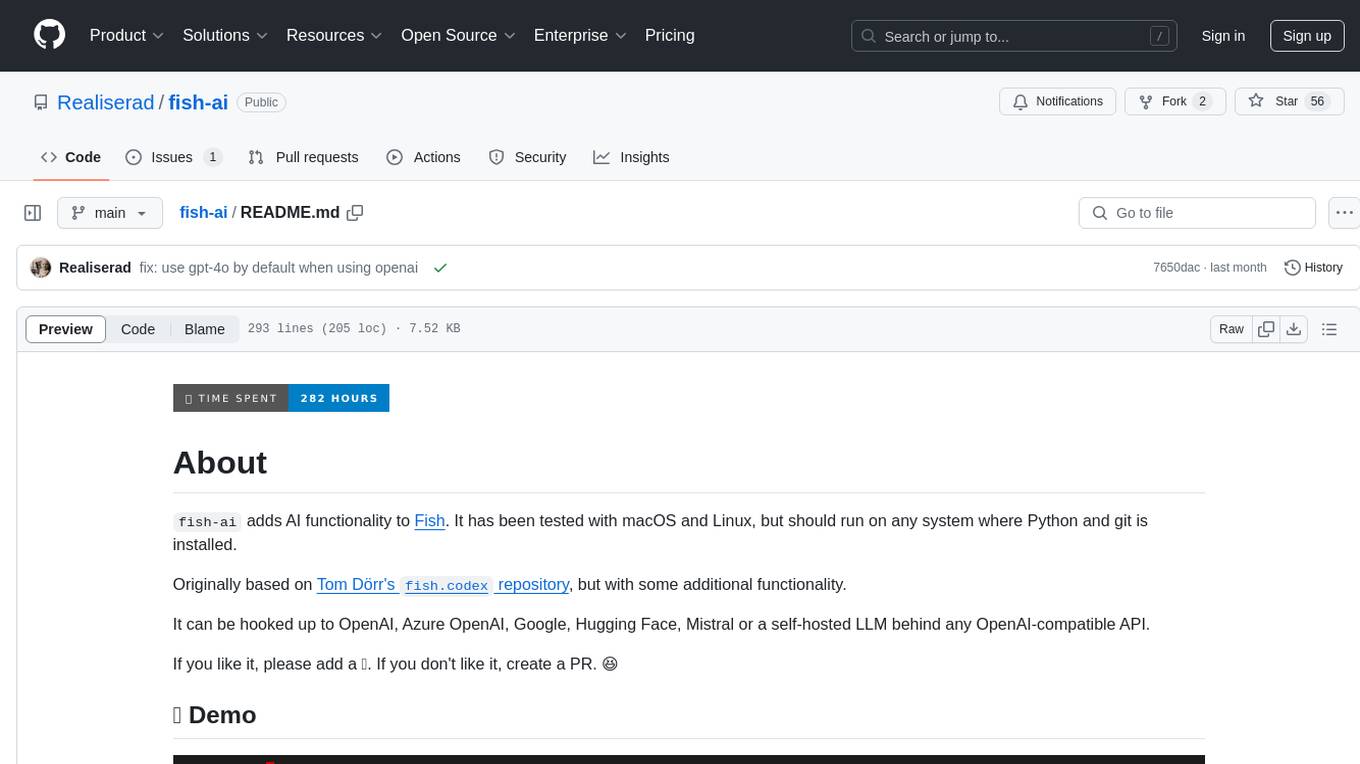
fish-ai
fish-ai is a tool that adds AI functionality to Fish shell. It can be integrated with various AI providers like OpenAI, Azure OpenAI, Google, Hugging Face, Mistral, or a self-hosted LLM. Users can transform comments into commands, autocomplete commands, and suggest fixes. The tool allows customization through configuration files and supports switching between contexts. Data privacy is maintained by redacting sensitive information before submission to the AI models. Development features include debug logging, testing, and creating releases.
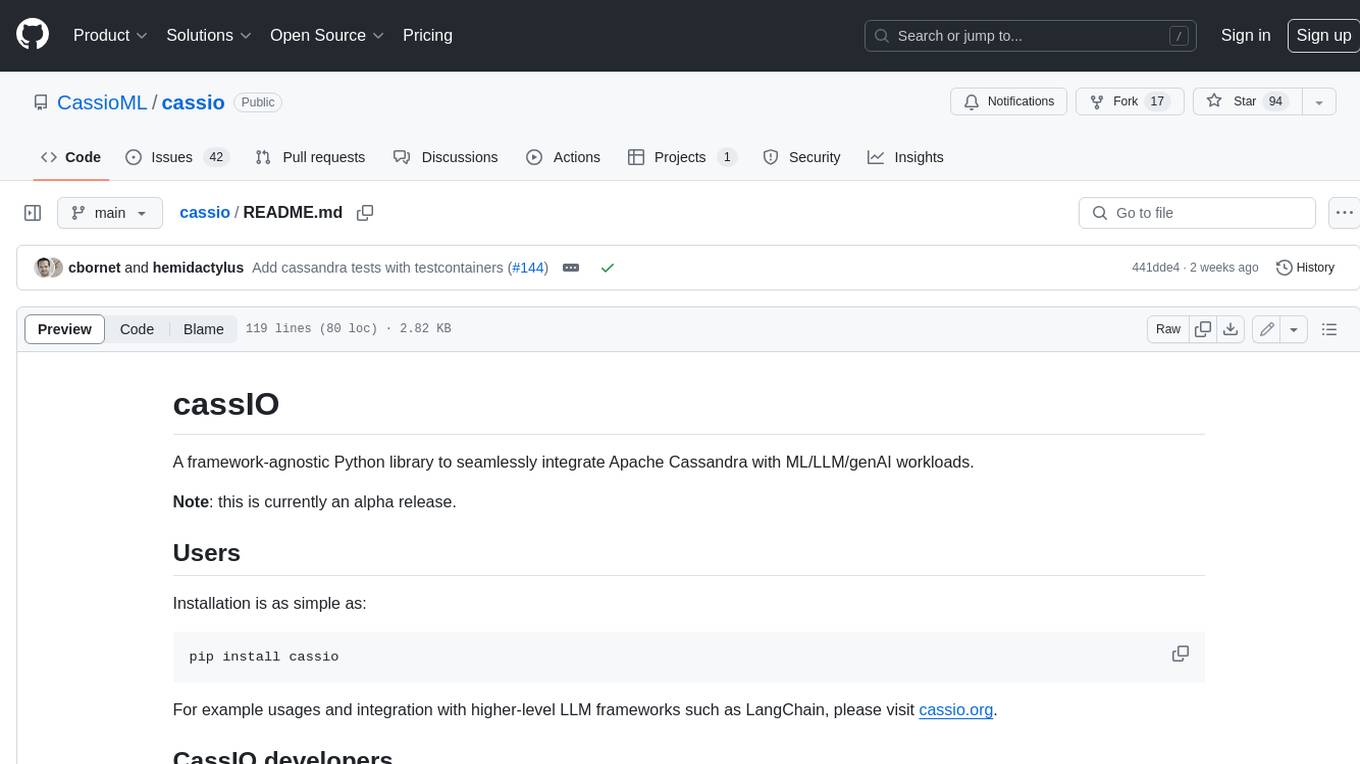
cassio
cassIO is a framework-agnostic Python library that seamlessly integrates Apache Cassandra with ML/LLM/genAI workloads. It provides an easy-to-use interface for developers to connect their Cassandra databases to machine learning models, allowing them to perform complex data analysis and AI-powered tasks directly on their Cassandra data. cassIO is designed to be flexible and extensible, making it suitable for a wide range of use cases, from data exploration and visualization to predictive modeling and natural language processing.
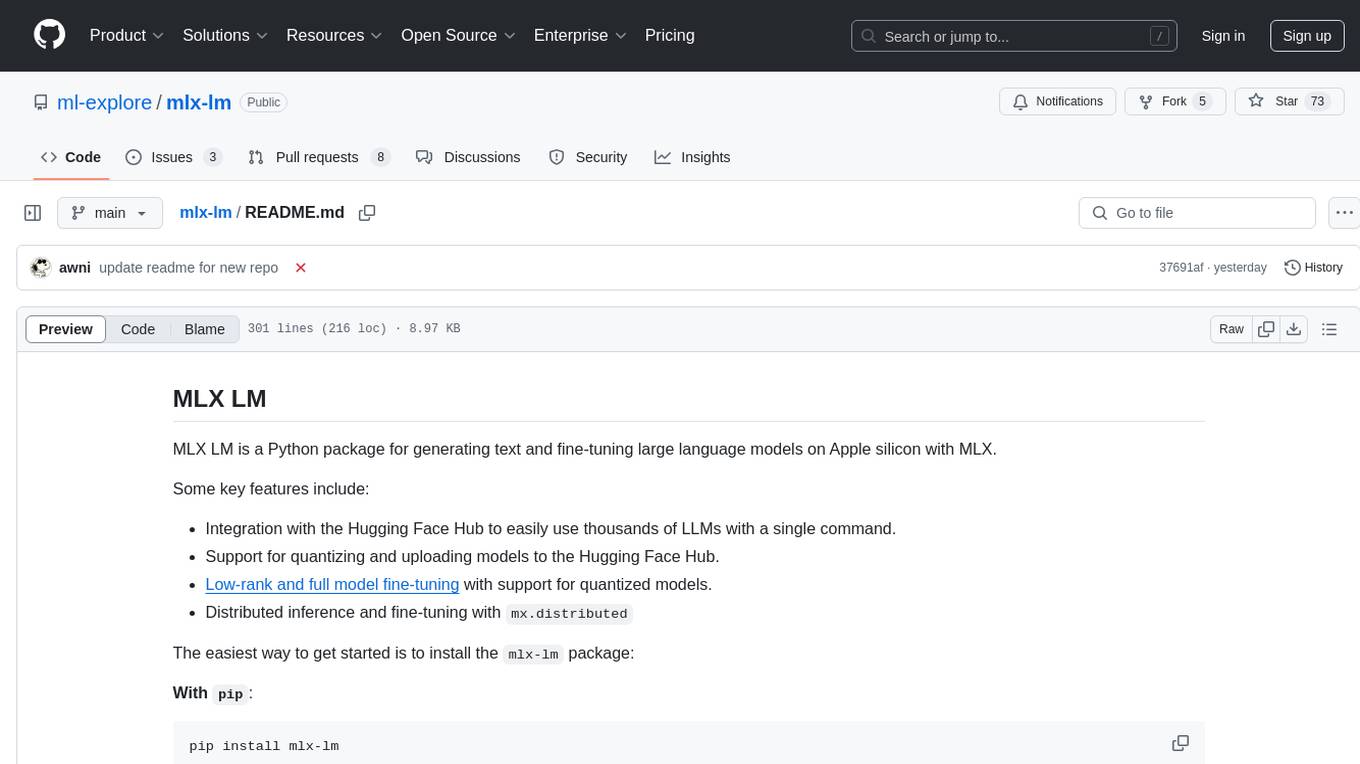
mlx-lm
MLX LM is a Python package designed for generating text and fine-tuning large language models on Apple silicon using MLX. It offers integration with the Hugging Face Hub for easy access to thousands of LLMs, support for quantizing and uploading models to the Hub, low-rank and full model fine-tuning capabilities, and distributed inference and fine-tuning with `mx.distributed`. Users can interact with the package through command line options or the Python API, enabling tasks such as text generation, chatting with language models, model conversion, streaming generation, and sampling. MLX LM supports various Hugging Face models and provides tools for efficient scaling to long prompts and generations, including a rotating key-value cache and prompt caching. It requires macOS 15.0 or higher for optimal performance.
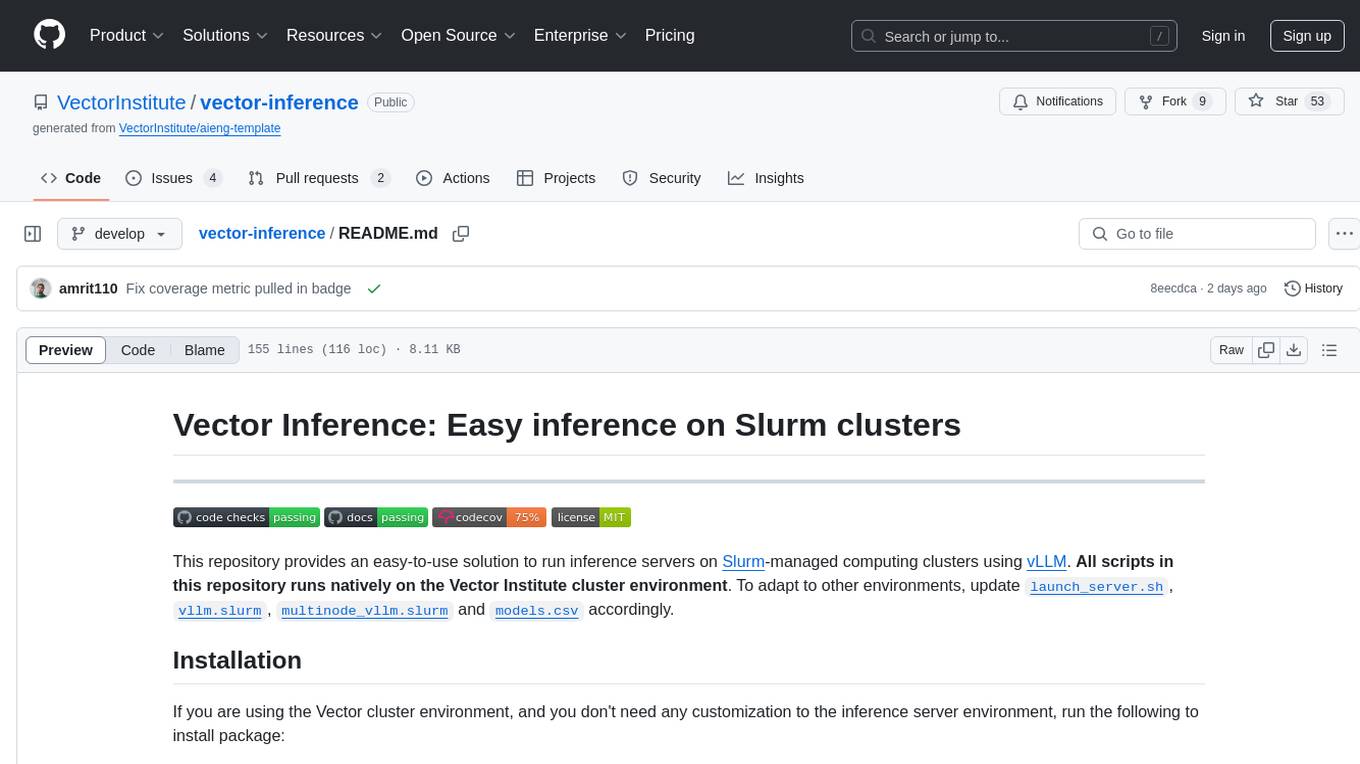
vector-inference
This repository provides an easy-to-use solution for running inference servers on Slurm-managed computing clusters using vLLM. All scripts in this repository run natively on the Vector Institute cluster environment. Users can deploy models as Slurm jobs, check server status and performance metrics, and shut down models. The repository also supports launching custom models with specific configurations. Additionally, users can send inference requests and set up an SSH tunnel to run inference from a local device.
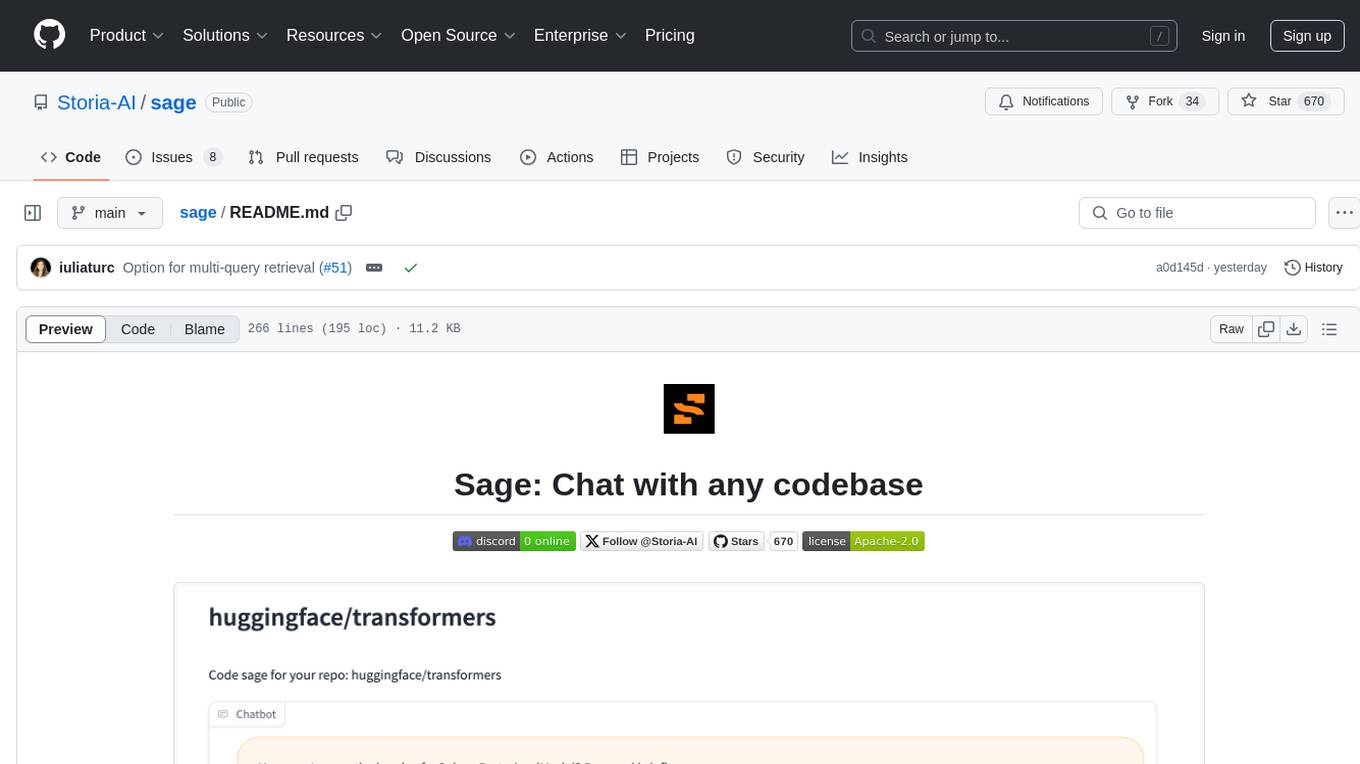
sage
Sage is a tool that allows users to chat with any codebase, providing a chat interface for code understanding and integration. It simplifies the process of learning how a codebase works by offering heavily documented answers sourced directly from the code. Users can set up Sage locally or on the cloud with minimal effort. The tool is designed to be easily customizable, allowing users to swap components of the pipeline and improve the algorithms powering code understanding and generation.
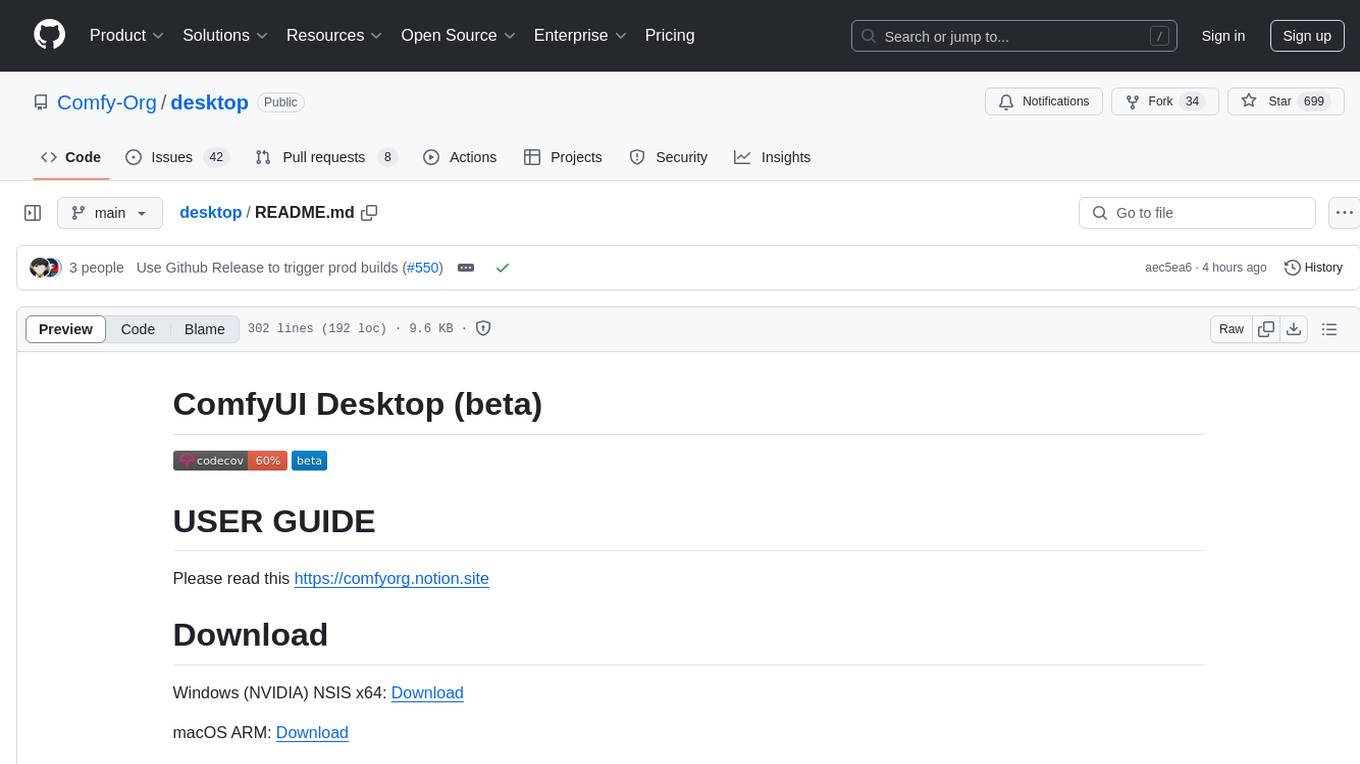
desktop
ComfyUI Desktop is a packaged desktop application that allows users to easily use ComfyUI with bundled features like ComfyUI source code, ComfyUI-Manager, and uv. It automatically installs necessary Python dependencies and updates with stable releases. The app comes with Electron, Chromium binaries, and node modules. Users can store ComfyUI files in a specified location and manage model paths. The tool requires Python 3.12+ and Visual Studio with Desktop C++ workload for Windows. It uses nvm to manage node versions and yarn as the package manager. Users can install ComfyUI and dependencies using comfy-cli, download uv, and build/launch the code. Troubleshooting steps include rebuilding modules and installing missing libraries. The tool supports debugging in VSCode and provides utility scripts for cleanup. Crash reports can be sent to help debug issues, but no personal data is included.
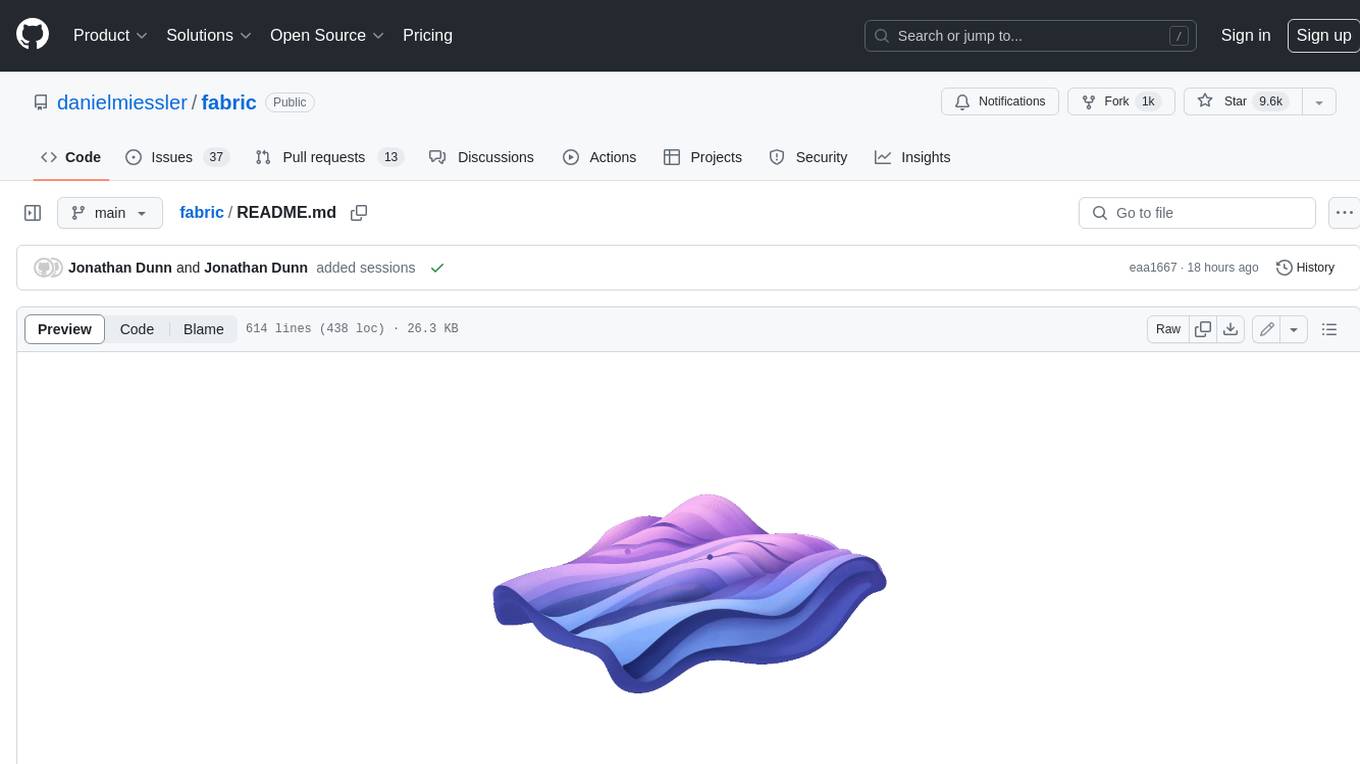
fabric
Fabric is an open-source framework for augmenting humans using AI. It provides a structured approach to breaking down problems into individual components and applying AI to them one at a time. Fabric includes a collection of pre-defined Patterns (prompts) that can be used for a variety of tasks, such as extracting the most interesting parts of YouTube videos and podcasts, writing essays, summarizing academic papers, creating AI art prompts, and more. Users can also create their own custom Patterns. Fabric is designed to be easy to use, with a command-line interface and a variety of helper apps. It is also extensible, allowing users to integrate it with their own AI applications and infrastructure.
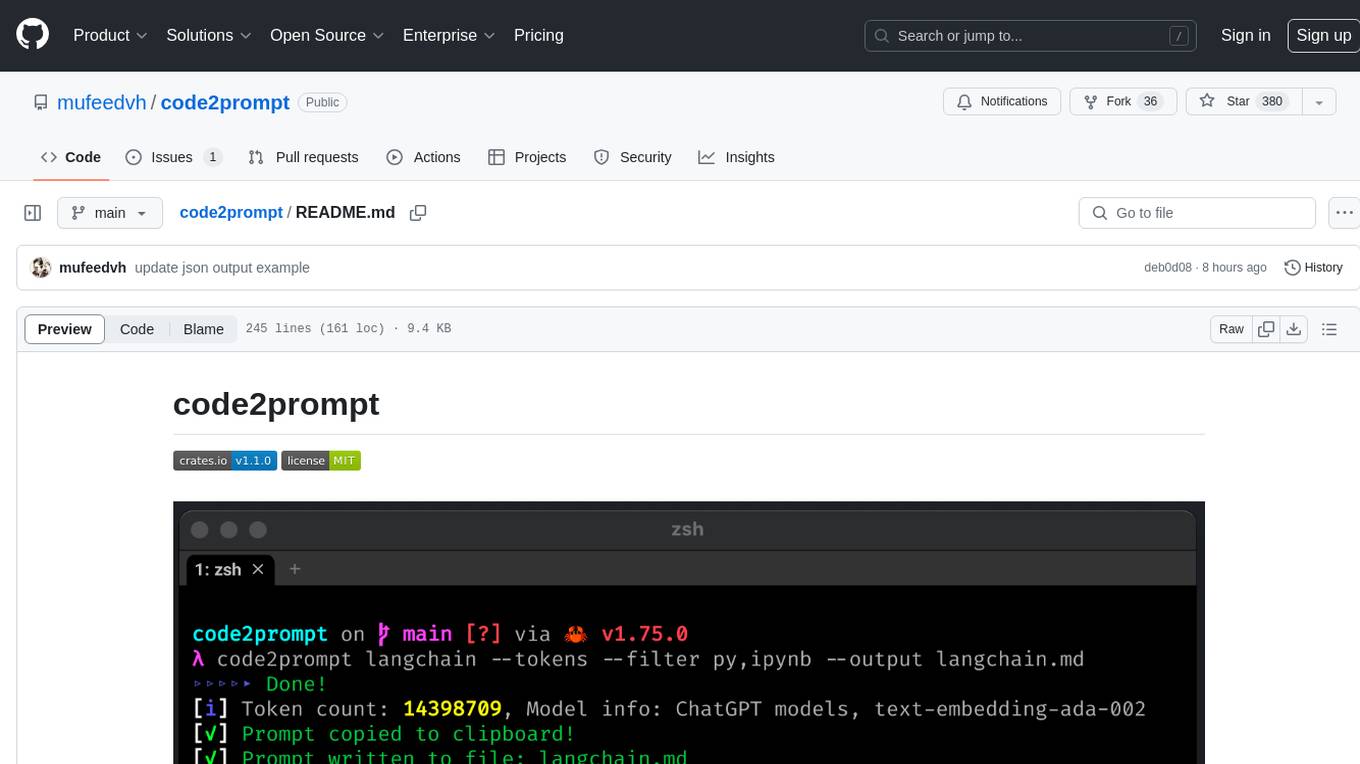
code2prompt
code2prompt is a command-line tool that converts your codebase into a single LLM prompt with a source tree, prompt templating, and token counting. It automates generating LLM prompts from codebases of any size, customizing prompt generation with Handlebars templates, respecting .gitignore, filtering and excluding files using glob patterns, displaying token count, including Git diff output, copying prompt to clipboard, saving prompt to an output file, excluding files and folders, adding line numbers to source code blocks, and more. It helps streamline the process of creating LLM prompts for code analysis, generation, and other tasks.
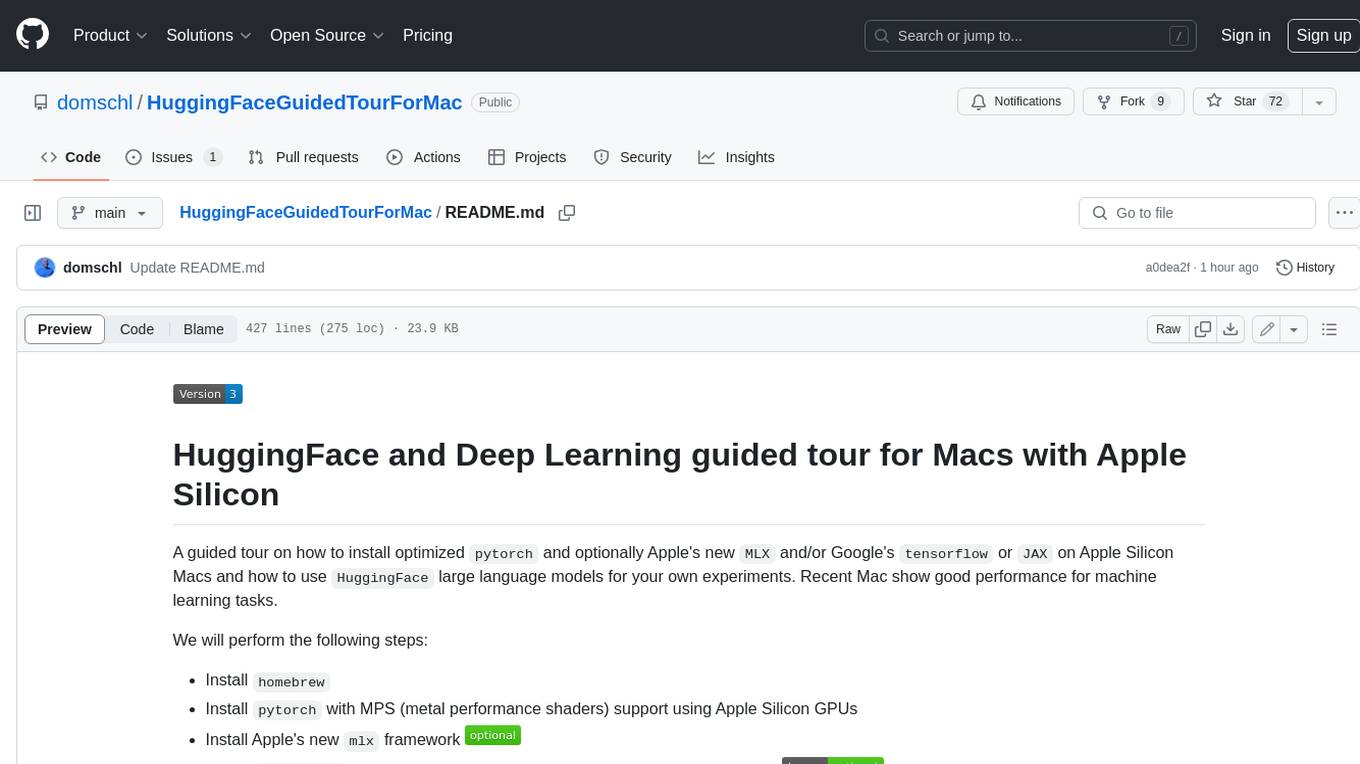
HuggingFaceGuidedTourForMac
HuggingFaceGuidedTourForMac is a guided tour on how to install optimized pytorch and optionally Apple's new MLX, JAX, and TensorFlow on Apple Silicon Macs. The repository provides steps to install homebrew, pytorch with MPS support, MLX, JAX, TensorFlow, and Jupyter lab. It also includes instructions on running large language models using HuggingFace transformers. The repository aims to help users set up their Macs for deep learning experiments with optimized performance.
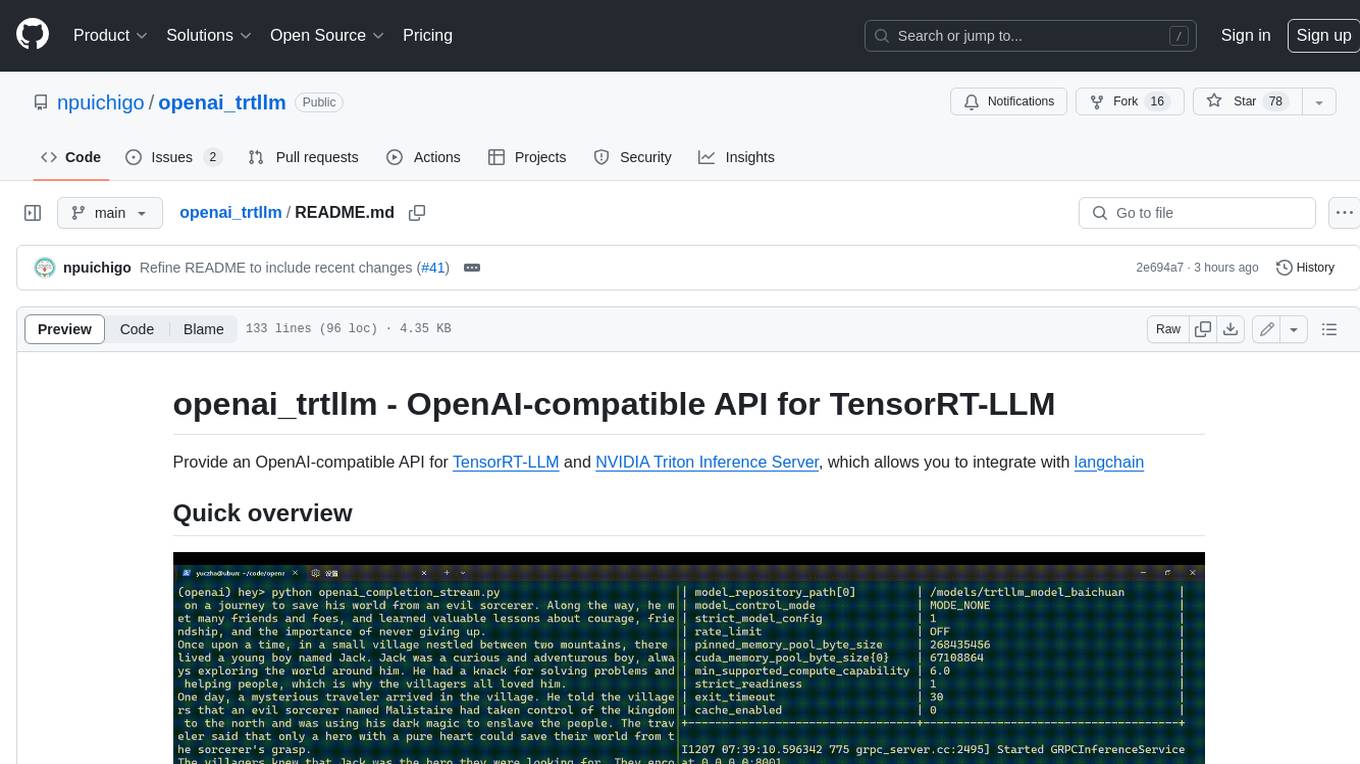
openai_trtllm
OpenAI-compatible API for TensorRT-LLM and NVIDIA Triton Inference Server, which allows you to integrate with langchain
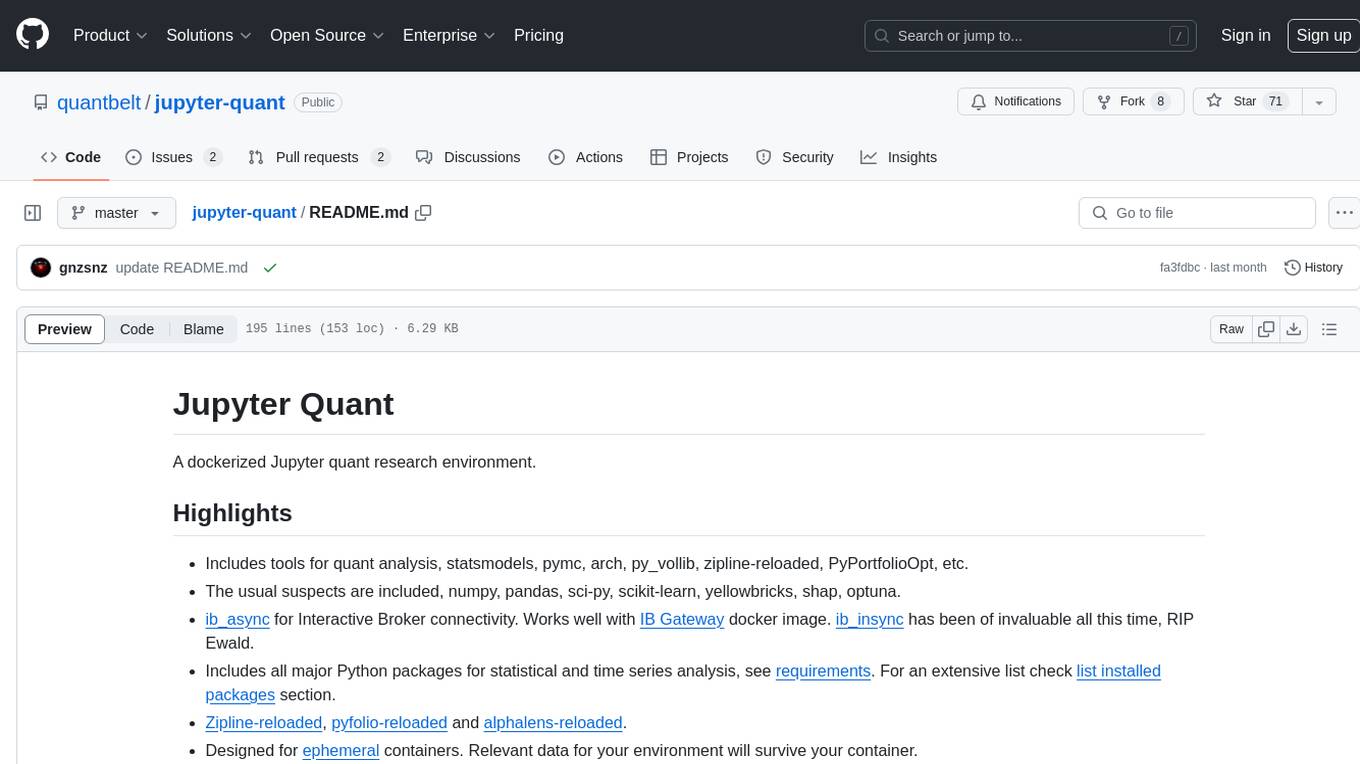
jupyter-quant
Jupyter Quant is a dockerized environment tailored for quantitative research, equipped with essential tools like statsmodels, pymc, arch, py_vollib, zipline-reloaded, PyPortfolioOpt, numpy, pandas, sci-py, scikit-learn, yellowbricks, shap, optuna, and more. It provides Interactive Broker connectivity via ib_async and includes major Python packages for statistical and time series analysis. The image is optimized for size, includes jedi language server, jupyterlab-lsp, and common command line utilities. Users can install new packages with sudo, leverage apt cache, and bring their own dot files and SSH keys. The tool is designed for ephemeral containers, ensuring data persistence and flexibility for quantitative analysis tasks.
For similar tasks
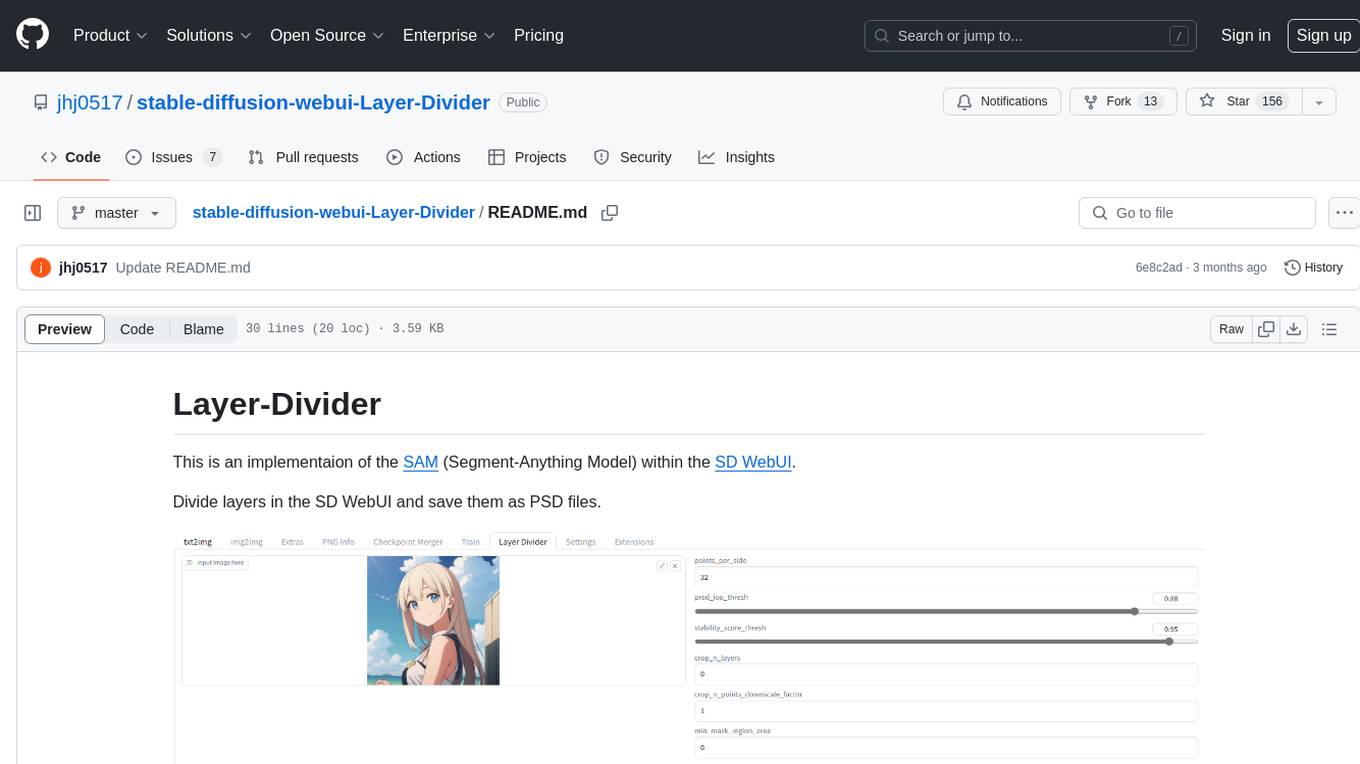
stable-diffusion-webui-Layer-Divider
This repository contains an implementation of the Segment-Anything Model (SAM) within the SD WebUI. It allows users to divide layers in the SD WebUI and save them as PSD files. Users can adjust parameters, click 'Generate', and view the output below. A PSD file will be saved in the designated folder. The tool provides various parameters for customization, such as points_per_side, pred_iou_thresh, stability_score_thresh, crops_n_layers, crop_n_points_downscale_factor, and min_mask_region_area.
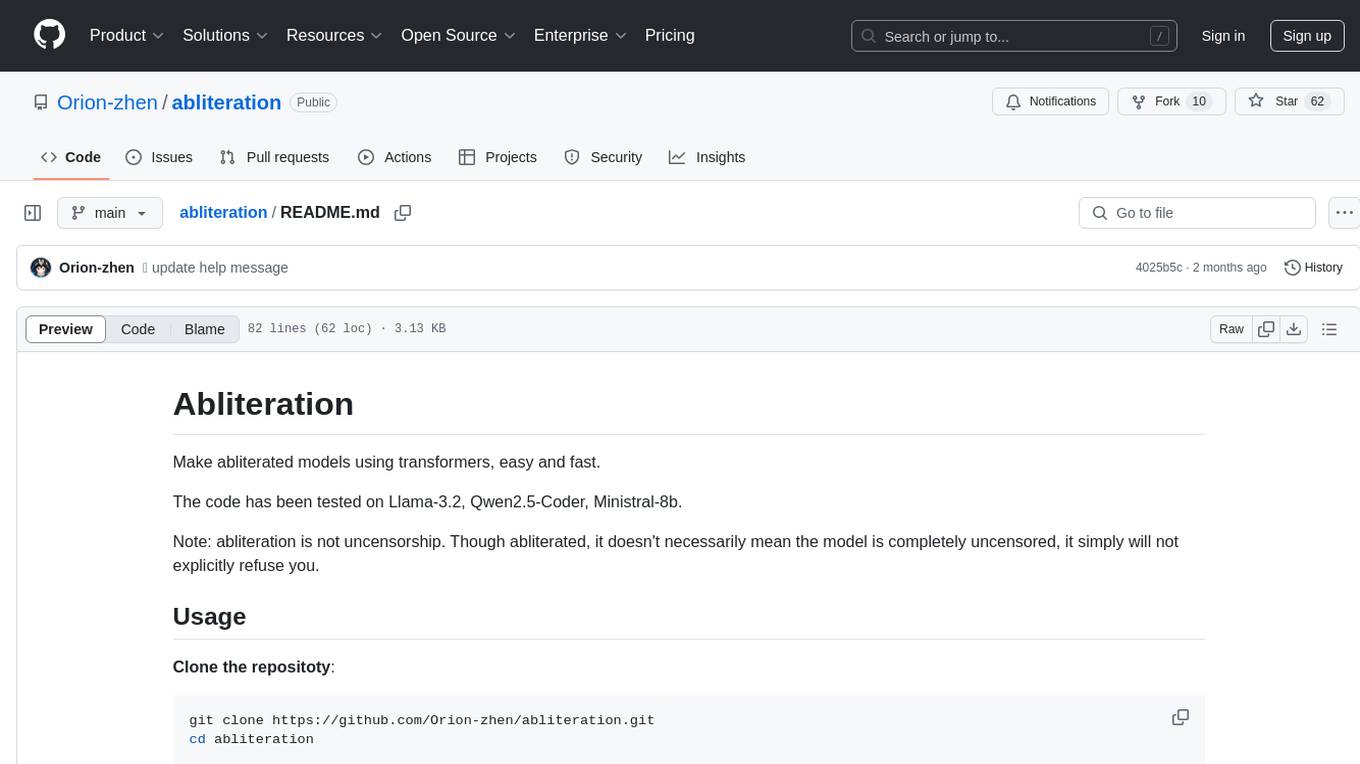
abliteration
Abliteration is a tool that allows users to create abliterated models using transformers quickly and easily. It is not a tool for uncensorship, but rather for making models that will not explicitly refuse users. Users can clone the repository, install dependencies, and make abliterations using the provided commands. The tool supports adjusting parameters for stubborn models and offers various options for customization. Abliteration can be used for creating modified models for specific tasks or topics.
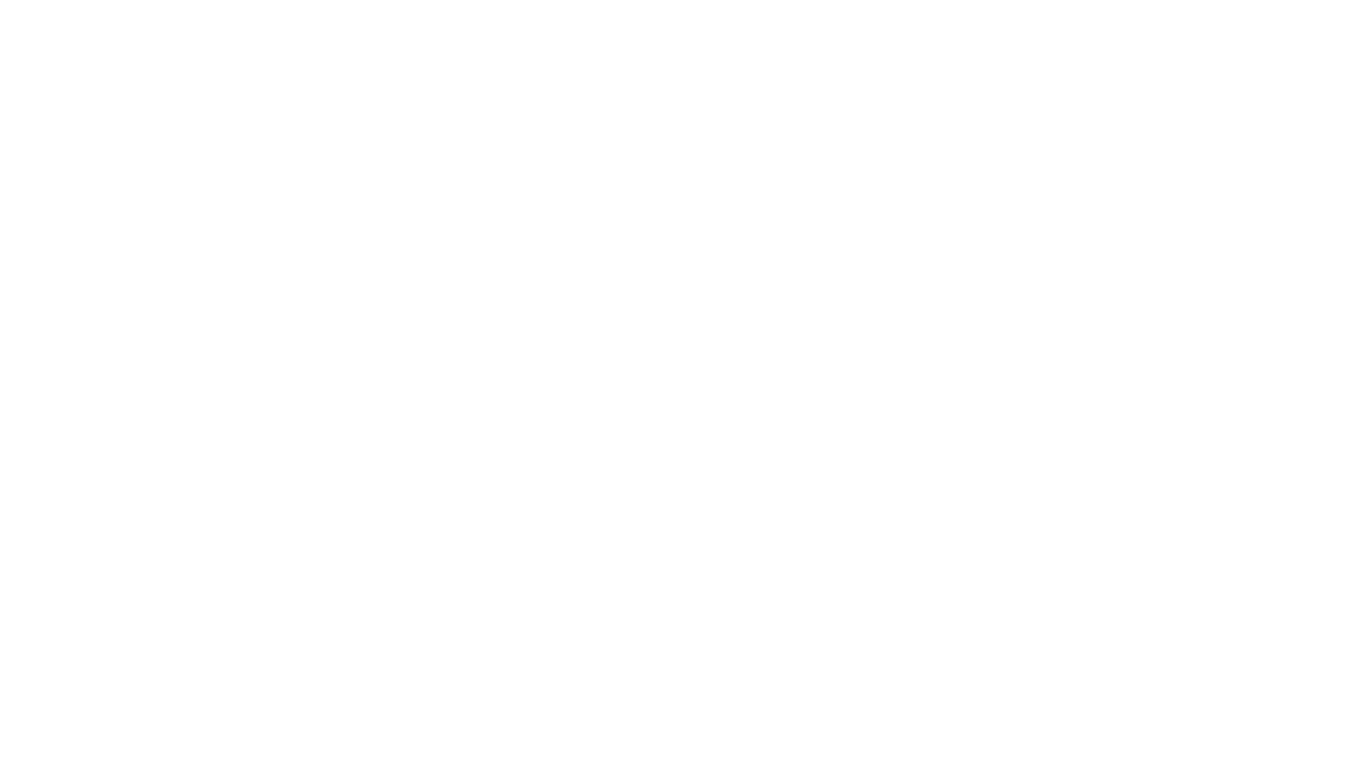
cria
Cria is a Python library designed for running Large Language Models with minimal configuration. It provides an easy and concise way to interact with LLMs, offering advanced features such as custom models, streams, message history management, and running multiple models in parallel. Cria simplifies the process of using LLMs by providing a straightforward API that requires only a few lines of code to get started. It also handles model installation automatically, making it efficient and user-friendly for various natural language processing tasks.
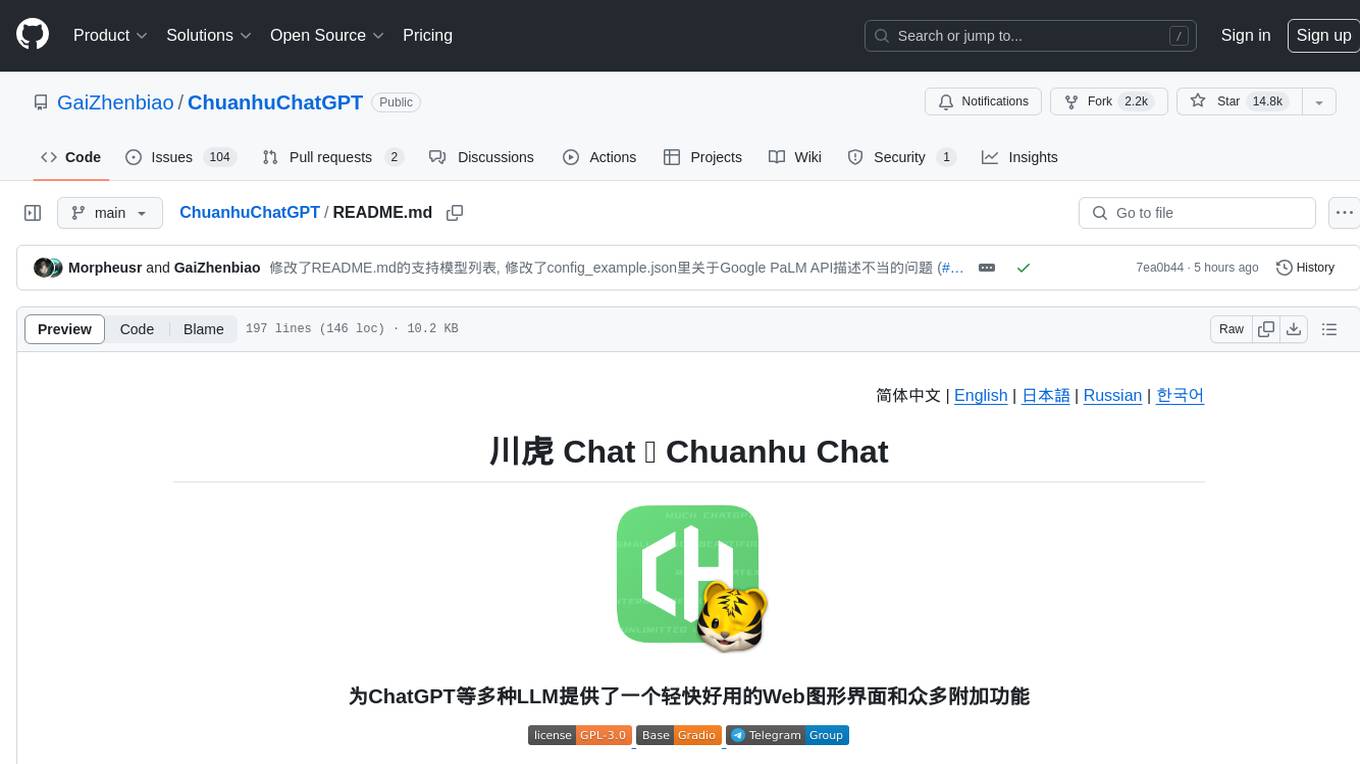
ChuanhuChatGPT
Chuanhu Chat is a user-friendly web graphical interface that provides various additional features for ChatGPT and other language models. It supports GPT-4, file-based question answering, local deployment of language models, online search, agent assistant, and fine-tuning. The tool offers a range of functionalities including auto-solving questions, online searching with network support, knowledge base for quick reading, local deployment of language models, GPT 3.5 fine-tuning, and custom model integration. It also features system prompts for effective role-playing, basic conversation capabilities with options to regenerate or delete dialogues, conversation history management with auto-saving and search functionalities, and a visually appealing user experience with themes, dark mode, LaTeX rendering, and PWA application support.
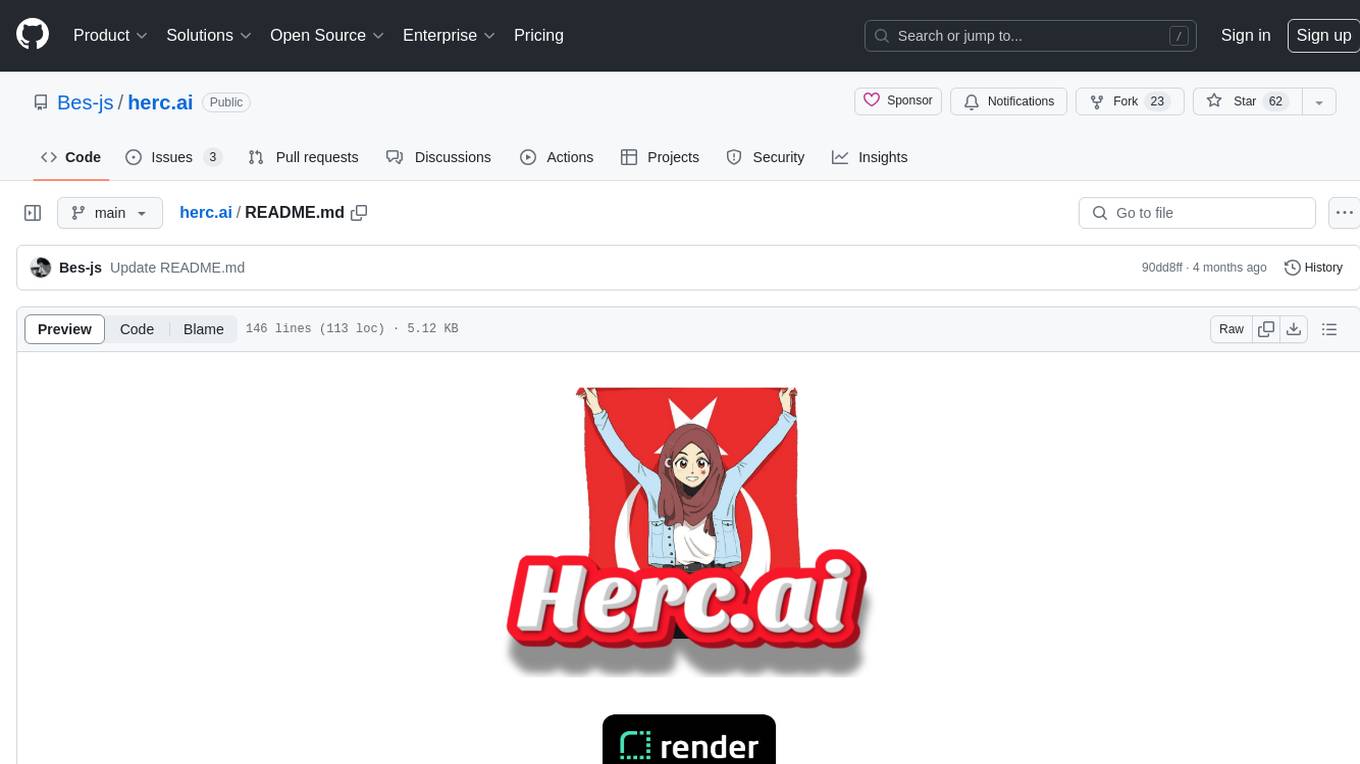
herc.ai
Herc.ai is a powerful library for interacting with the Herc.ai API. It offers free access to users and supports all languages. Users can benefit from Herc.ai's features unlimitedly with a one-time subscription and API key. The tool provides functionalities for question answering and text-to-image generation, with support for various models and customization options. Herc.ai can be easily integrated into CLI, CommonJS, TypeScript, and supports beta models for advanced usage. Developed by FiveSoBes and Luppux Development.
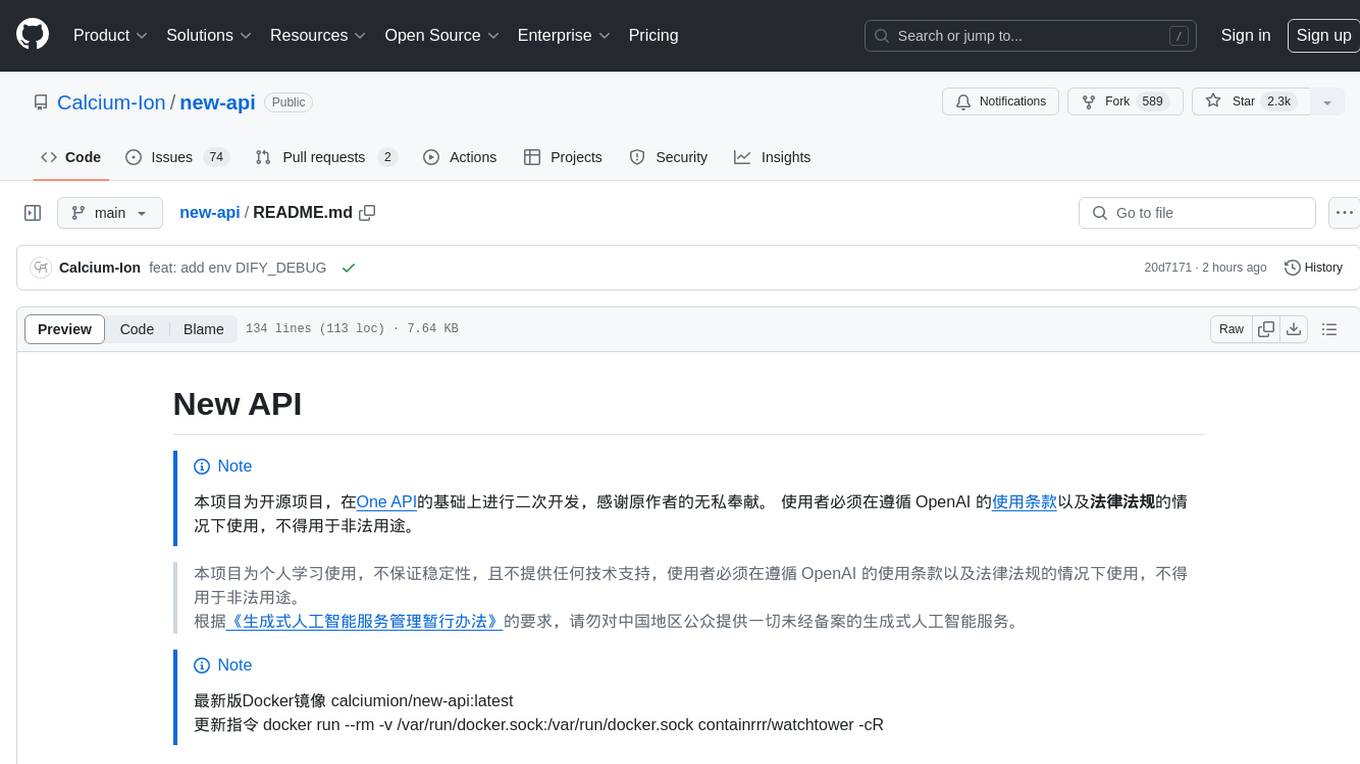
new-api
New API is an open-source project based on One API with additional features and improvements. It offers a new UI interface, supports Midjourney-Proxy(Plus) interface, online recharge functionality, model-based charging, channel weight randomization, data dashboard, token-controlled models, Telegram authorization login, Suno API support, Rerank model integration, and various third-party models. Users can customize models, retry channels, and configure caching settings. The deployment can be done using Docker with SQLite or MySQL databases. The project provides documentation for Midjourney and Suno interfaces, and it is suitable for AI enthusiasts and developers looking to enhance AI capabilities.
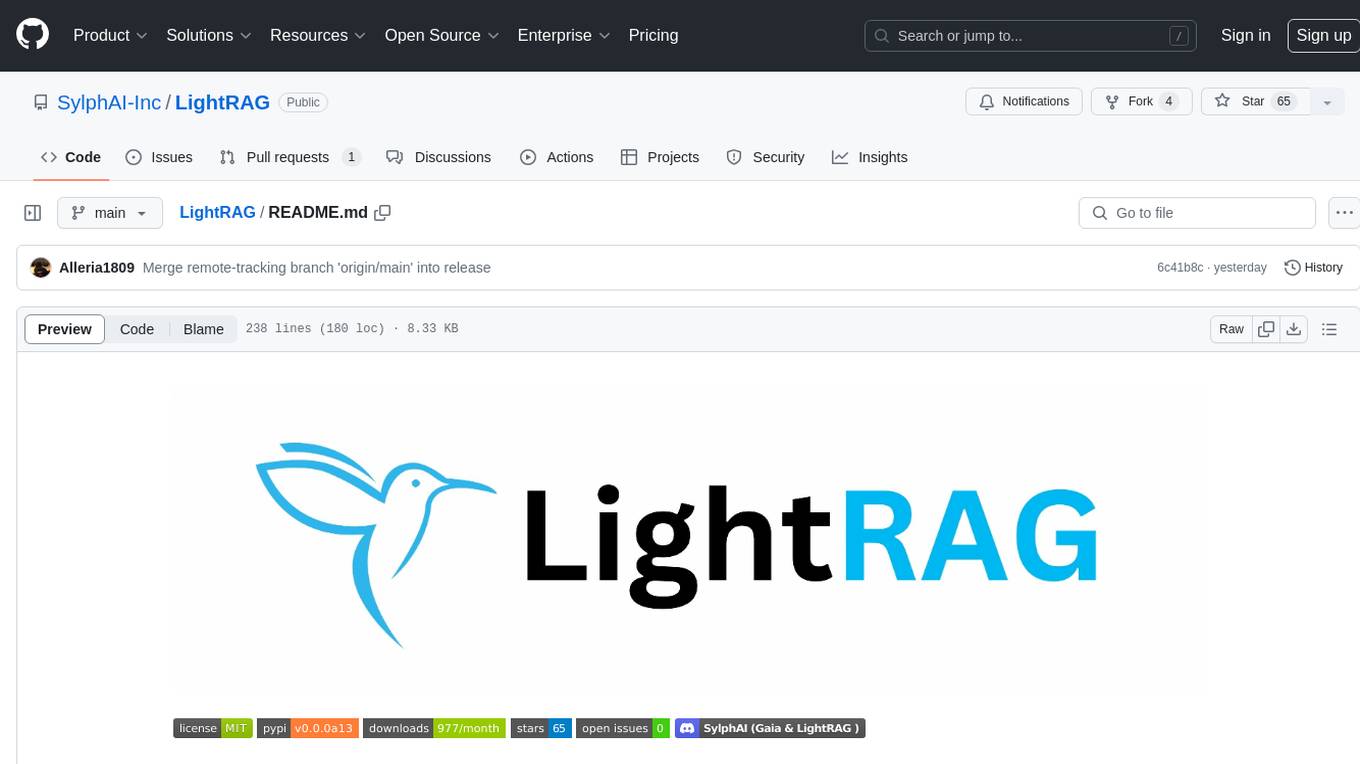
LightRAG
LightRAG is a PyTorch library designed for building and optimizing Retriever-Agent-Generator (RAG) pipelines. It follows principles of simplicity, quality, and optimization, offering developers maximum customizability with minimal abstraction. The library includes components for model interaction, output parsing, and structured data generation. LightRAG facilitates tasks like providing explanations and examples for concepts through a question-answering pipeline.
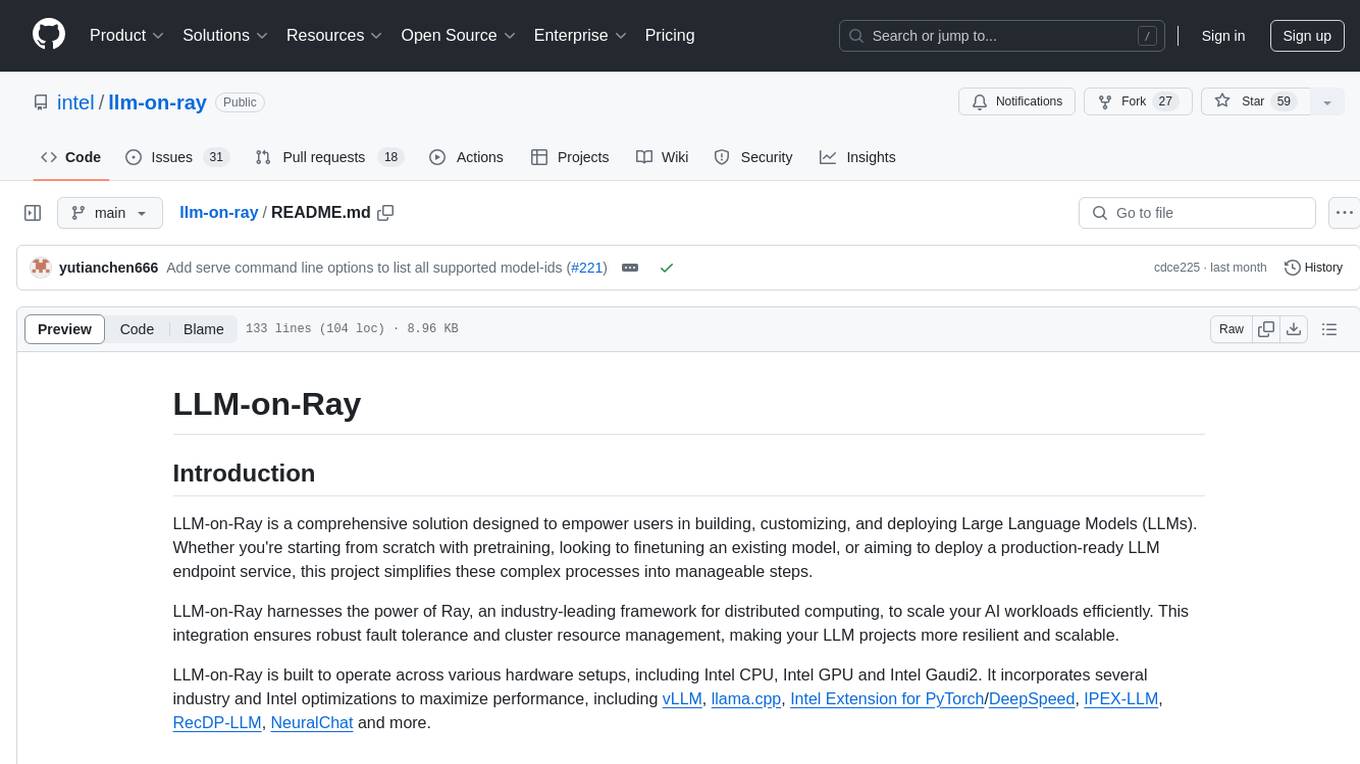
llm-on-ray
LLM-on-Ray is a comprehensive solution for building, customizing, and deploying Large Language Models (LLMs). It simplifies complex processes into manageable steps by leveraging the power of Ray for distributed computing. The tool supports pretraining, finetuning, and serving LLMs across various hardware setups, incorporating industry and Intel optimizations for performance. It offers modular workflows with intuitive configurations, robust fault tolerance, and scalability. Additionally, it provides an Interactive Web UI for enhanced usability, including a chatbot application for testing and refining models.
For similar jobs
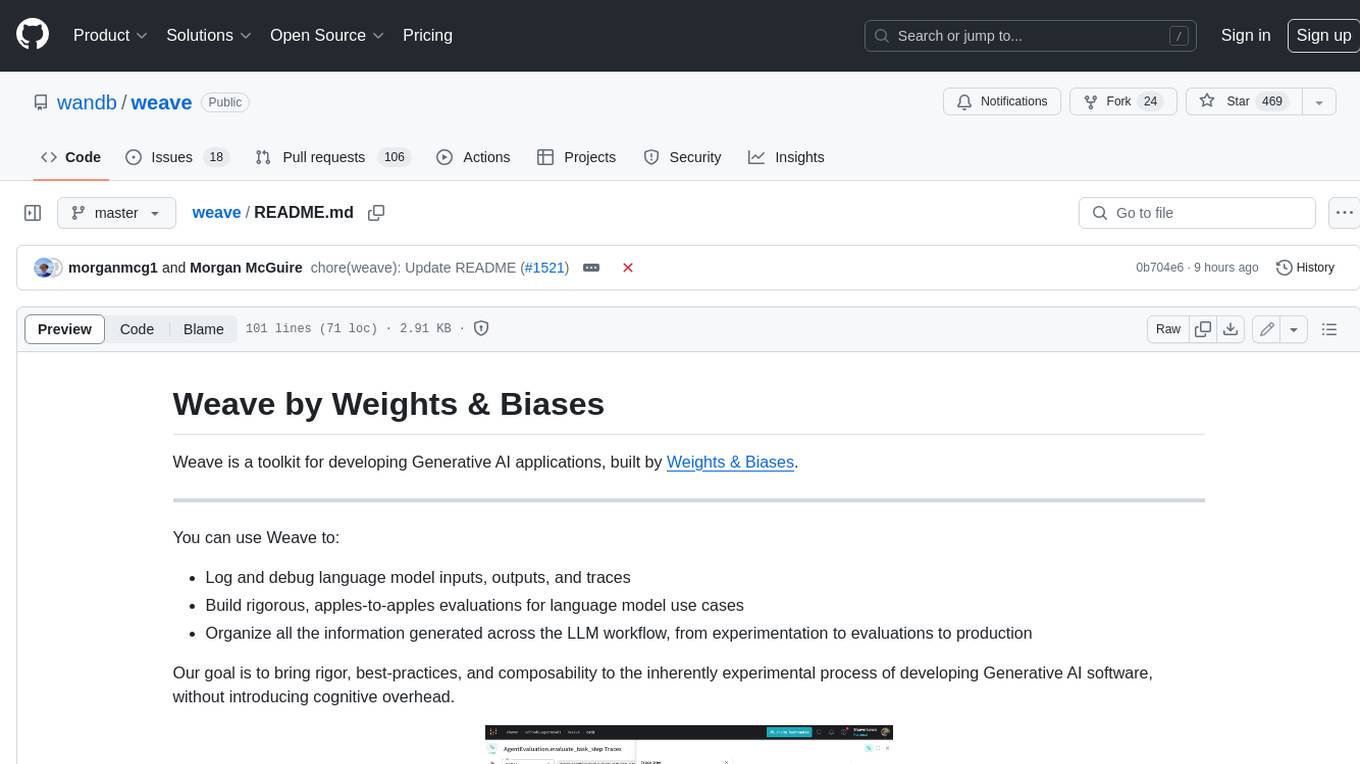
weave
Weave is a toolkit for developing Generative AI applications, built by Weights & Biases. With Weave, you can log and debug language model inputs, outputs, and traces; build rigorous, apples-to-apples evaluations for language model use cases; and organize all the information generated across the LLM workflow, from experimentation to evaluations to production. Weave aims to bring rigor, best-practices, and composability to the inherently experimental process of developing Generative AI software, without introducing cognitive overhead.
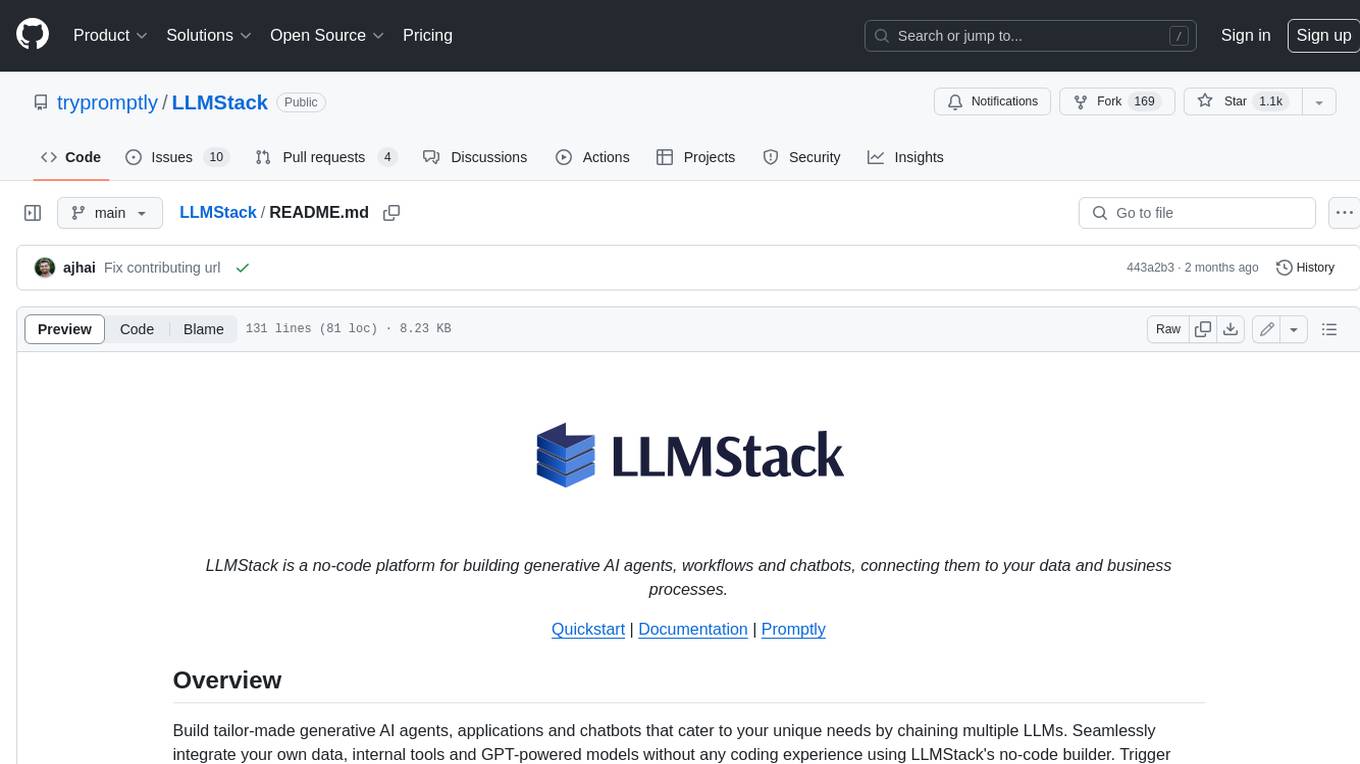
LLMStack
LLMStack is a no-code platform for building generative AI agents, workflows, and chatbots. It allows users to connect their own data, internal tools, and GPT-powered models without any coding experience. LLMStack can be deployed to the cloud or on-premise and can be accessed via HTTP API or triggered from Slack or Discord.
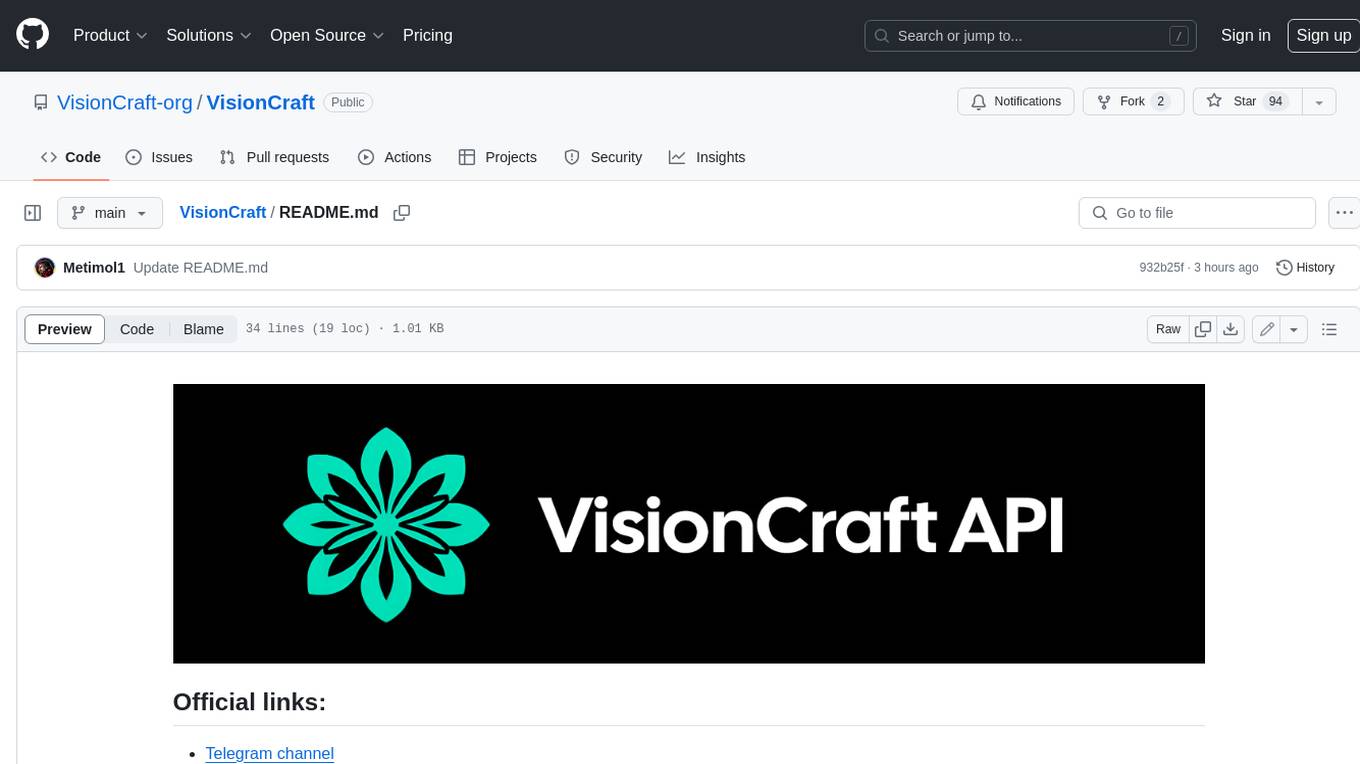
VisionCraft
The VisionCraft API is a free API for using over 100 different AI models. From images to sound.
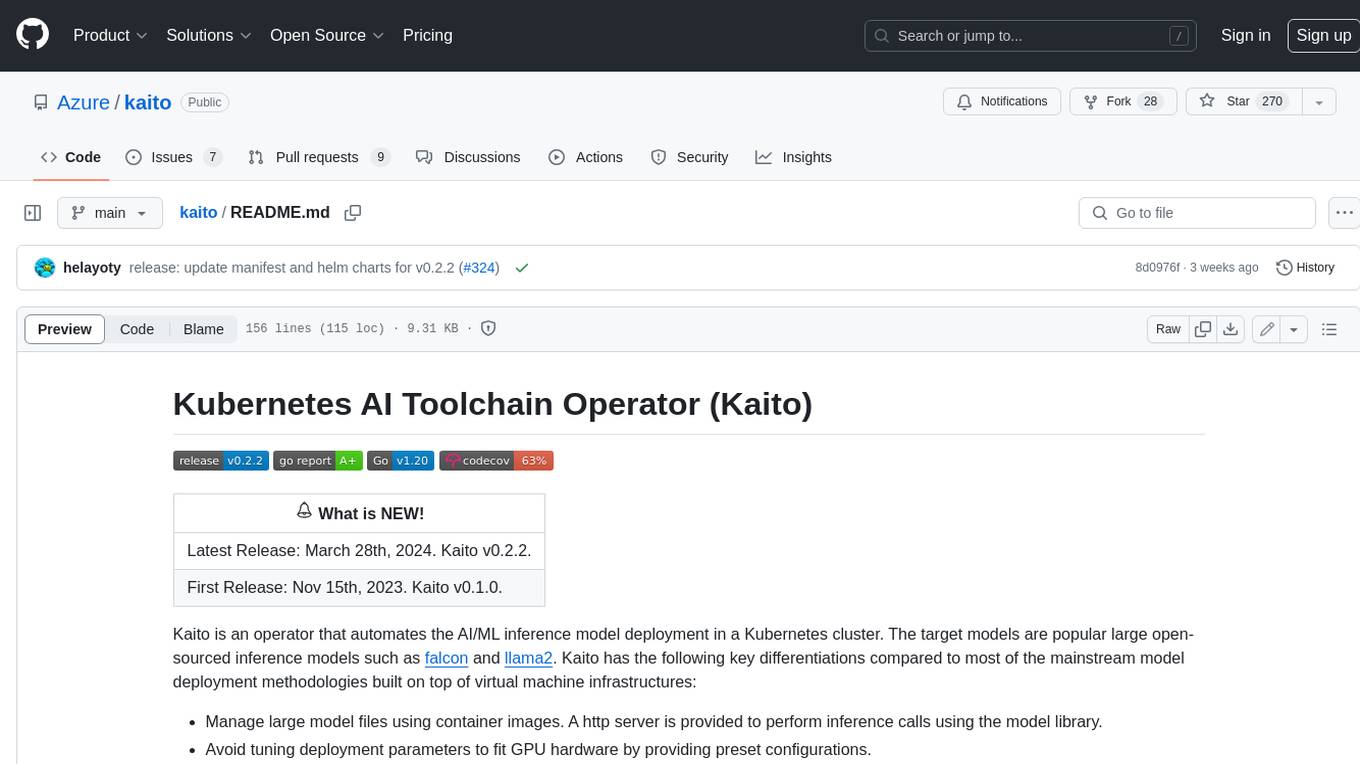
kaito
Kaito is an operator that automates the AI/ML inference model deployment in a Kubernetes cluster. It manages large model files using container images, avoids tuning deployment parameters to fit GPU hardware by providing preset configurations, auto-provisions GPU nodes based on model requirements, and hosts large model images in the public Microsoft Container Registry (MCR) if the license allows. Using Kaito, the workflow of onboarding large AI inference models in Kubernetes is largely simplified.
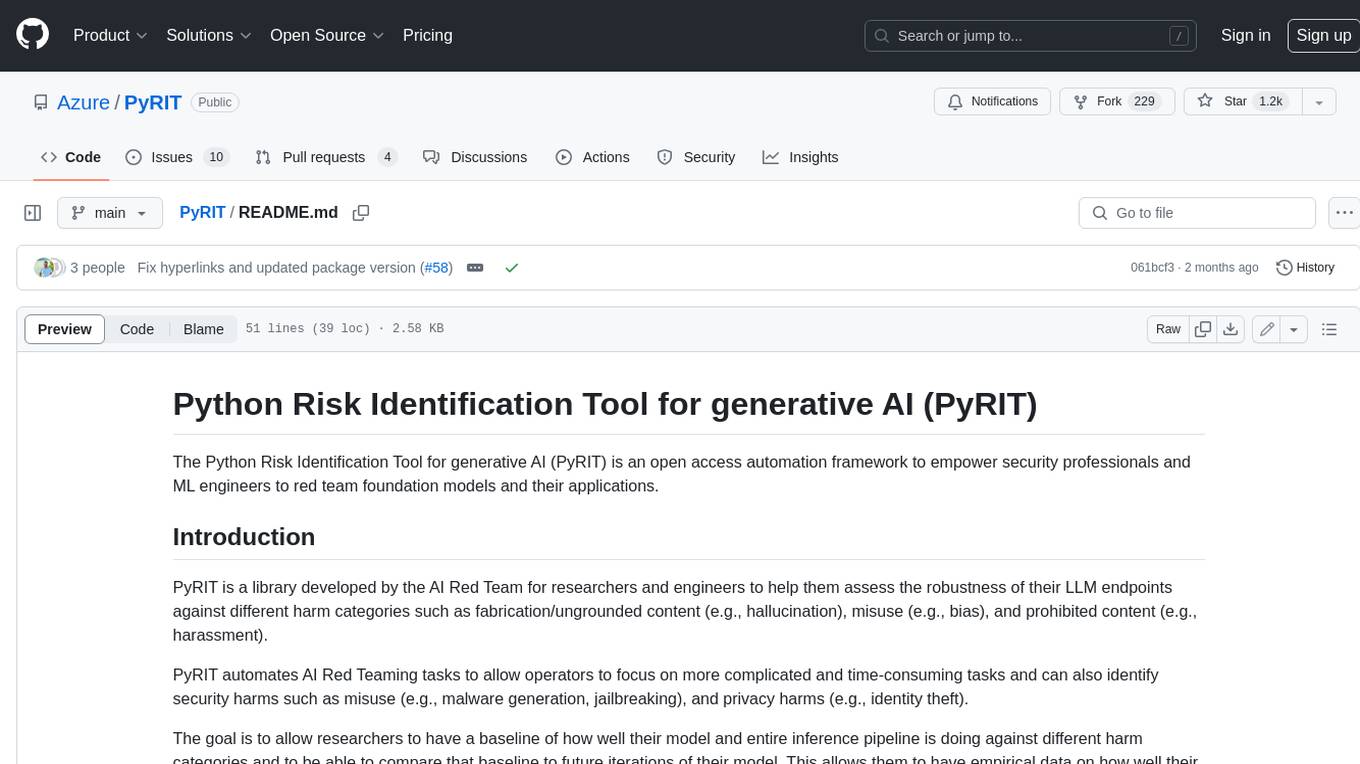
PyRIT
PyRIT is an open access automation framework designed to empower security professionals and ML engineers to red team foundation models and their applications. It automates AI Red Teaming tasks to allow operators to focus on more complicated and time-consuming tasks and can also identify security harms such as misuse (e.g., malware generation, jailbreaking), and privacy harms (e.g., identity theft). The goal is to allow researchers to have a baseline of how well their model and entire inference pipeline is doing against different harm categories and to be able to compare that baseline to future iterations of their model. This allows them to have empirical data on how well their model is doing today, and detect any degradation of performance based on future improvements.
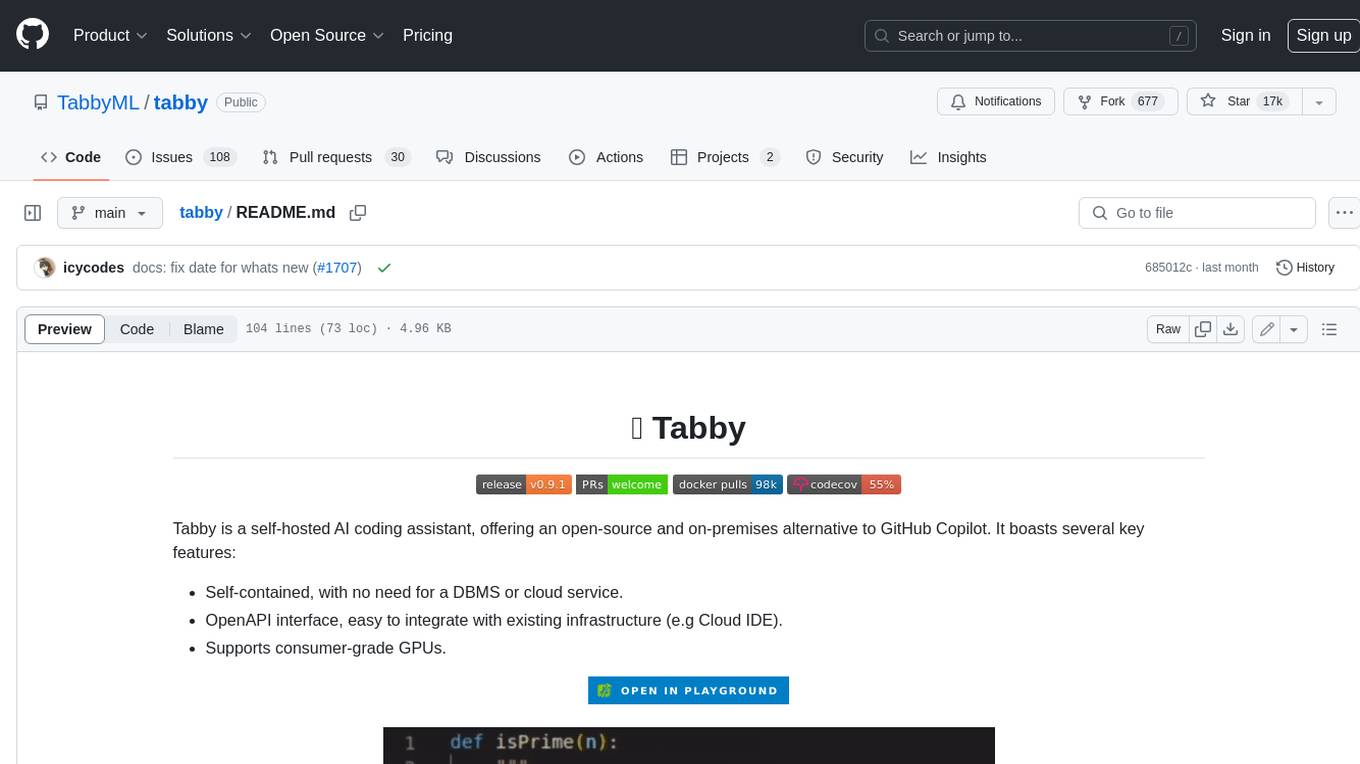
tabby
Tabby is a self-hosted AI coding assistant, offering an open-source and on-premises alternative to GitHub Copilot. It boasts several key features: * Self-contained, with no need for a DBMS or cloud service. * OpenAPI interface, easy to integrate with existing infrastructure (e.g Cloud IDE). * Supports consumer-grade GPUs.
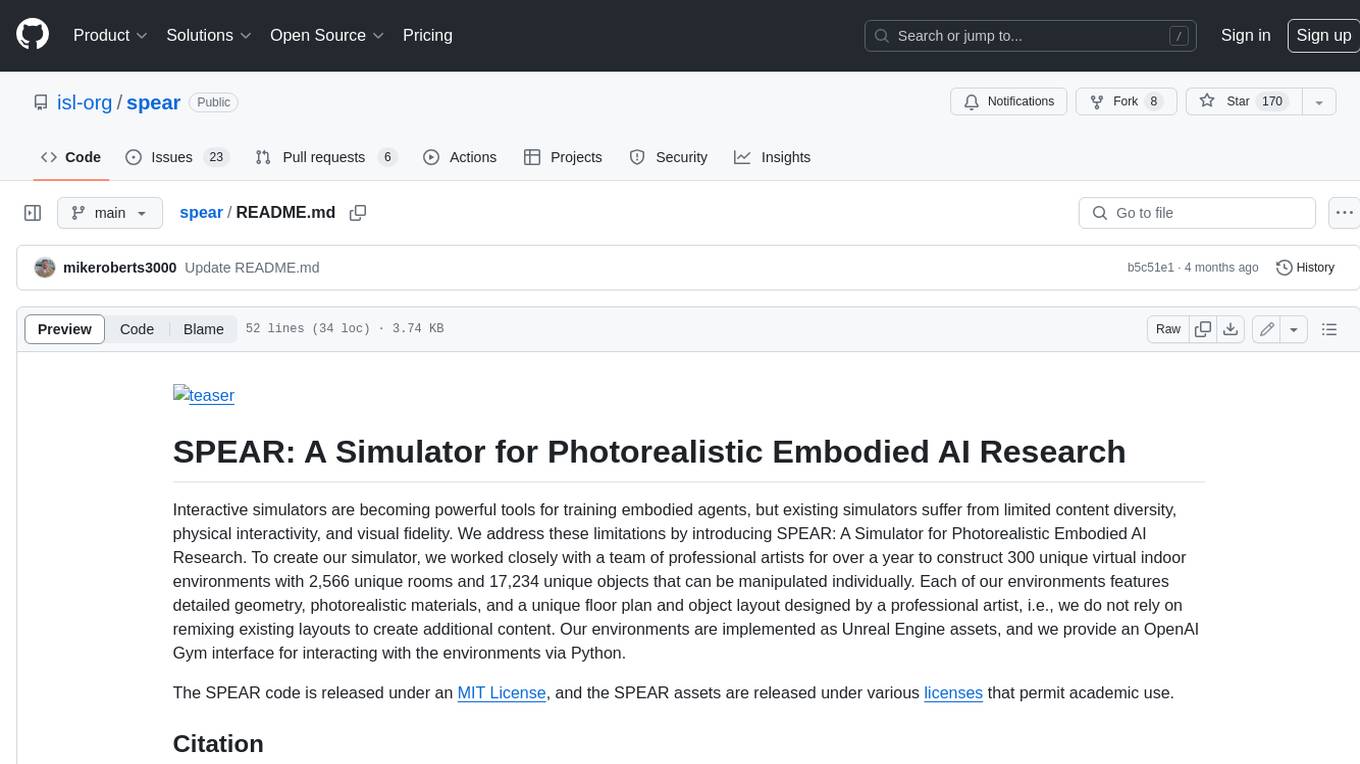
spear
SPEAR (Simulator for Photorealistic Embodied AI Research) is a powerful tool for training embodied agents. It features 300 unique virtual indoor environments with 2,566 unique rooms and 17,234 unique objects that can be manipulated individually. Each environment is designed by a professional artist and features detailed geometry, photorealistic materials, and a unique floor plan and object layout. SPEAR is implemented as Unreal Engine assets and provides an OpenAI Gym interface for interacting with the environments via Python.
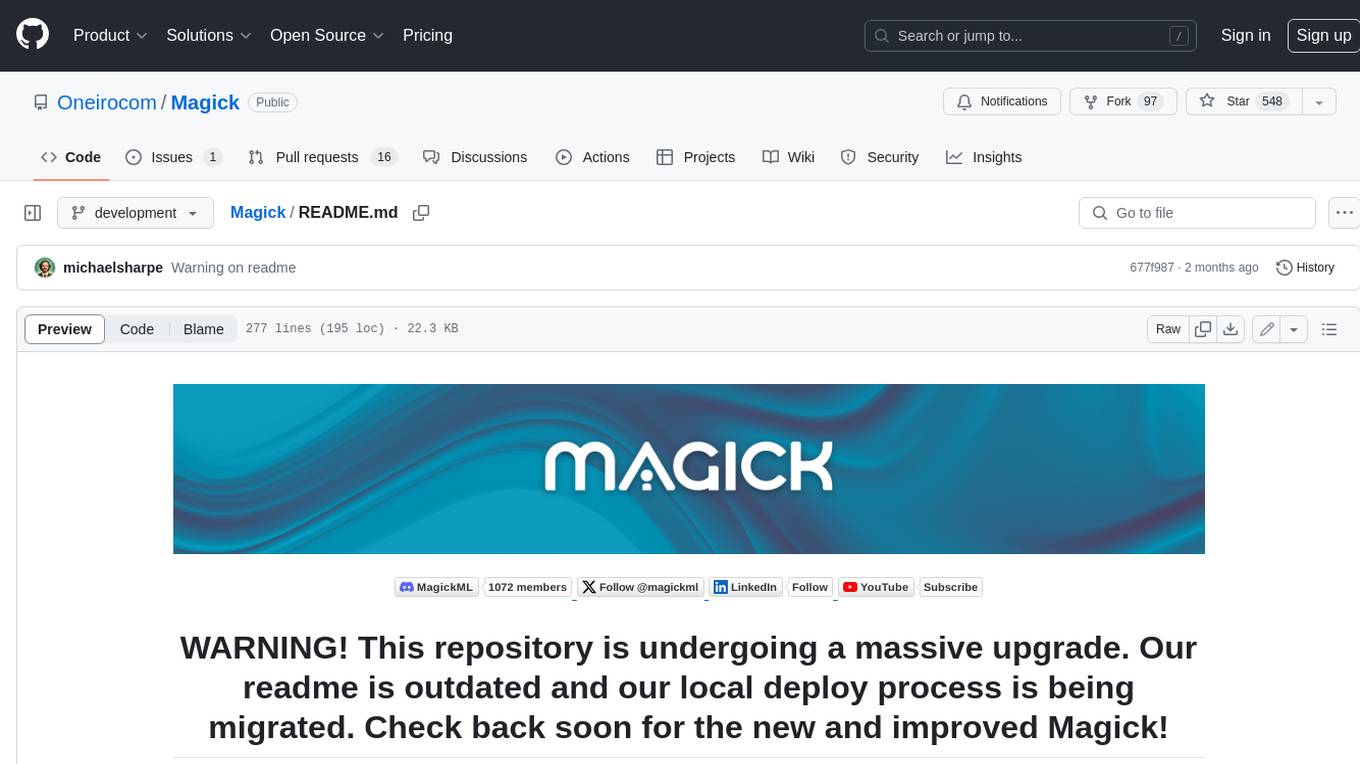
Magick
Magick is a groundbreaking visual AIDE (Artificial Intelligence Development Environment) for no-code data pipelines and multimodal agents. Magick can connect to other services and comes with nodes and templates well-suited for intelligent agents, chatbots, complex reasoning systems and realistic characters.