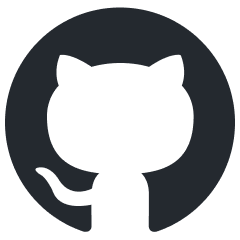
cria
Run LLMs locally with as little friction as possible.
Stars: 105
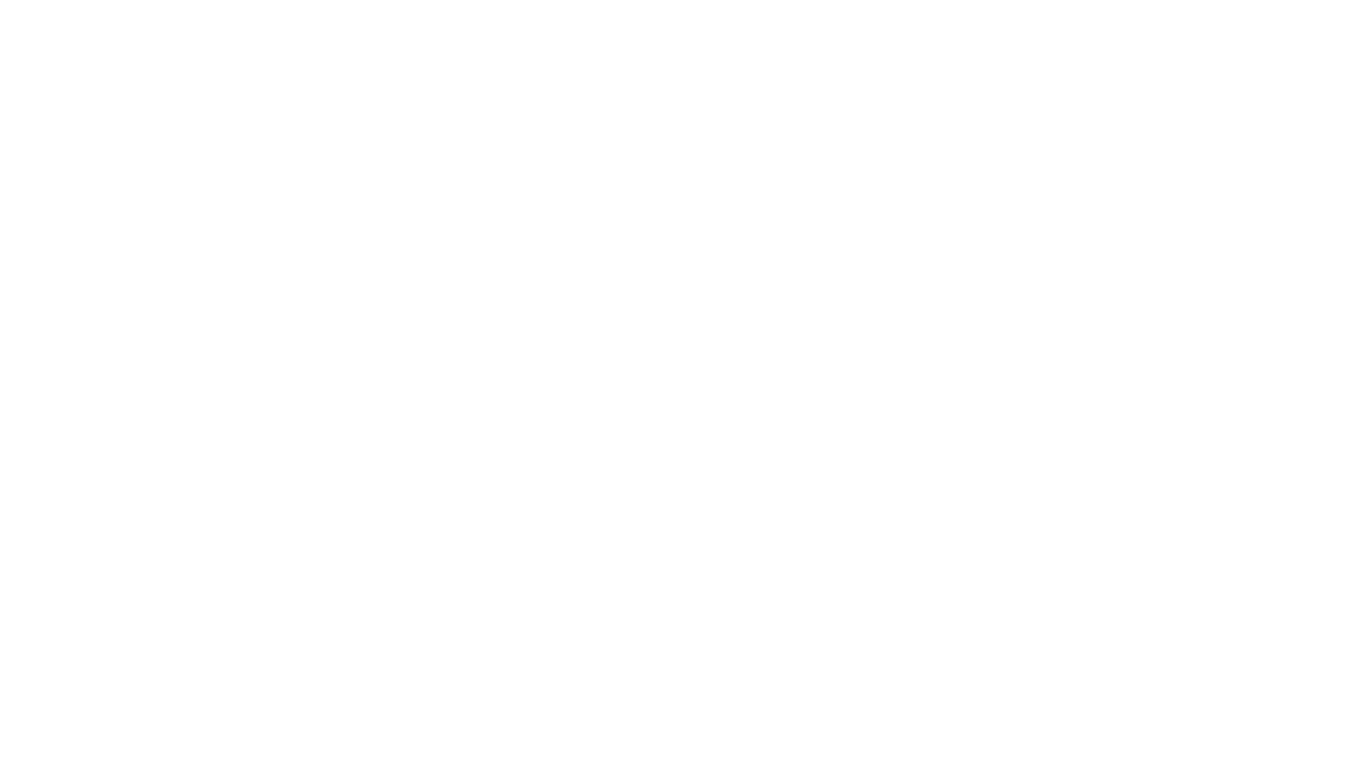
Cria is a Python library designed for running Large Language Models with minimal configuration. It provides an easy and concise way to interact with LLMs, offering advanced features such as custom models, streams, message history management, and running multiple models in parallel. Cria simplifies the process of using LLMs by providing a straightforward API that requires only a few lines of code to get started. It also handles model installation automatically, making it efficient and user-friendly for various natural language processing tasks.
README:
Cria, use Python to run LLMs with as little friction as possible.
Cria is a library for programmatically running Large Language Models through Python. Cria is built so you need as little configuration as possible — even with more advanced features.
- Easy: No configuration is required out of the box. Getting started takes just five lines of code.
- Concise: Write less code to save time and avoid duplication.
- Local: Free and unobstructed by rate limits, running LLMs requires no internet connection.
-
Efficient: Use advanced features with your own
ollama
instance, or a subprocess.
Running Cria is easy. After installation, you need just five lines of code — no configurations, no manual downloads, no API keys, and no servers to worry about.
import cria
ai = cria.Cria()
prompt = "Who is the CEO of OpenAI?"
for chunk in ai.chat(prompt):
print(chunk, end="")
>>> The CEO of OpenAI is Sam Altman!
or, you can run this more configurable example.
import cria
with cria.Model() as ai:
prompt = "Who is the CEO of OpenAI?"
response = ai.chat(prompt, stream=False)
print(response)
>>> The CEO of OpenAI is Sam Altman!
[!WARNING] If no model is configured, Cria automatically installs and runs the default model:
llama3:8b
(4.7GB).
-
Cria uses
ollama
, to install it, run the following.curl -fsSL https://ollama.com/install.sh | sh
-
Install Cria with
pip
.pip install cria
To run other LLMs, pass them into your ai
variable.
import cria
ai = cria.Cria("llama2")
prompt = "Who is the CEO of OpenAI?"
for chunk in ai.chat(prompt):
print(chunk, end="") # The CEO of OpenAI is Sam Altman. He co-founded OpenAI in 2015 with...
You can find available models here.
Streams are used by default in Cria, but you can turn them off by passing in a boolean for the stream
parameter.
prompt = "Who is the CEO of OpenAI?"
response = ai.chat(prompt, stream=False)
print(response) # The CEO of OpenAI is Sam Altman!
By default, models are closed when you exit the Python program, but closing them manually is a best practice.
ai.close()
You can also use with
statements to close models automatically (recommended).
Message history is automatically saved in Cria, so asking follow-up questions is easy.
prompt = "Who is the CEO of OpenAI?"
response = ai.chat(prompt, stream=False)
print(response) # The CEO of OpenAI is Sam Altman.
prompt = "Tell me more about him."
response = ai.chat(prompt, stream=False)
print(response) # Sam Altman is an American entrepreneur and technologist who serves as the CEO of OpenAI...
You can reset message history by running the clear
method.
prompt = "Who is the CEO of OpenAI?"
response = ai.chat(prompt, stream=False)
print(response) # Sam Altman is an American entrepreneur and technologist who serves as the CEO of OpenAI...
ai.clear()
prompt = "Tell me more about him."
response = ai.chat(prompt, stream=False)
print(response) # I apologize, but I don't have any information about "him" because the conversation just started...
You can also create a custom message history, and pass in your own context.
context = "Our AI system employed a hybrid approach combining reinforcement learning and generative adversarial networks (GANs) to optimize the decision-making..."
messages = [
{"role": "system", "content": "You are a technical documentation writer"},
{"role": "user", "content": context},
]
prompt = "Write some documentation using the text I gave you."
for chunk in ai.chat(messages=messages, prompt=prompt):
print(chunk, end="") # AI System Optimization: Hybrid Approach Combining Reinforcement Learning and...
In the example, instructions are given to the LLM as the system
. Then, extra context is given as the user
. Finally, the prompt is entered (as a user
). You can use any mixture of roles to specify the LLM to your liking.
The available roles for messages are:
-
user
- Pass prompts as the user. -
system
- Give instructions as the system. -
assistant
- Act as the AI assistant yourself, and give the LLM lines.
The prompt parameter will always be appended to messages under the user
role, to override this, you can choose to pass in nothing for prompt
.
If you are streaming messages with Cria, you can interrupt the prompt mid way.
response = ""
max_token_length = 5
prompt = "Who is the CEO of OpenAI?"
for i, chunk in enumerate(ai.chat(prompt)):
if i >= max_token_length:
ai.stop()
response += chunk
print(response) # The CEO of OpenAI is
response = ""
max_token_length = 5
prompt = "Who is the CEO of OpenAI?"
for i, chunk in enumerate(ai.generate(prompt)):
if i >= max_token_length:
ai.stop()
response += chunk
print(response) # The CEO of OpenAI is
In the examples, after the AI generates five tokens (units of text that are usually a couple of characters long), text generation is stopped via the stop
method. After stop
is called, you can safely break
out of the for
loop.
By default, Cria automatically saves responses in message history, even if the stream is interrupted. To prevent this behaviour though, you can pass in the allow_interruption
boolean.
ai = cria.Cria(allow_interruption=False)
response = ""
max_token_length = 5
prompt = "Who is the CEO of OpenAI?"
for i, chunk in enumerate(ai.chat(prompt)):
if i >= max_token_length:
ai.stop()
break
print(chunk, end="") # The CEO of OpenAI is
prompt = "Tell me more about him."
for chunk in ai.chat(prompt):
print(chunk, end="") # I apologize, but I don't have any information about "him" because the conversation just started...
If you are running multiple models or parallel conversations, the Model
class is also available. This is recommended for most use cases.
import cria
ai = cria.Model()
prompt = "Who is the CEO of OpenAI?"
response = ai.chat(prompt, stream=False)
print(response) # The CEO of OpenAI is Sam Altman.
All methods that apply to the Cria
class also apply to Model
.
Multiple models can be run through a with
statement. This automatically closes them after use.
import cria
prompt = "Who is the CEO of OpenAI?"
with cria.Model("llama3") as ai:
response = ai.chat(prompt, stream=False)
print(response) # OpenAI's CEO is Sam Altman, who also...
with cria.Model("llama2") as ai:
response = ai.chat(prompt, stream=False)
print(response) # The CEO of OpenAI is Sam Altman.
Or, models can be run traditionally.
import cria
prompt = "Who is the CEO of OpenAI?"
llama3 = cria.Model("llama3")
response = llama3.chat(prompt, stream=False)
print(response) # OpenAI's CEO is Sam Altman, who also...
llama2 = cria.Model("llama2")
response = llama2.chat(prompt, stream=False)
print(response) # The CEO of OpenAI is Sam Altman.
# Not required, but best practice.
llama3.close()
llama2.close()
Cria also has a generate
method.
prompt = "Who is the CEO of OpenAI?"
for chunk in ai.generate(prompt):
print(chunk, end="") # The CEO of OpenAI (Open-source Artificial Intelligence) is Sam Altman.
promt = "Tell me more about him."
response = ai.generate(prompt, stream=False)
print(response) # I apologize, but I think there may have been some confusion earlier. As this...
When you run cria.Cria()
, an ollama
instance will start up if one is not already running. When the program exits, this instance will terminate.
However, if you want to save resources by not exiting ollama
, either run your own ollama
instance in another terminal, or run a managed subprocess.
ollama serve
prompt = "Who is the CEO of OpenAI?"
with cria.Model() as ai:
response = ai.generate("Who is the CEO of OpenAI?", stream=False)
print(response)
# If it is the first time you start the program, ollama will start automatically
# If it is the second time (or subsequent times) you run the program, ollama will already be running
ai = cria.Cria(standalone=True, close_on_exit=False)
prompt = "Who is the CEO of OpenAI?"
with cria.Model("llama2") as llama2:
response = llama2.generate("Who is the CEO of OpenAI?", stream=False)
print(response)
with cria.Model("llama3") as llama3:
response = llama3.generate("Who is the CEO of OpenAI?", stream=False)
print(response)
quit()
# Despite exiting, olama will keep running, and be used the next time this program starts.
To format the output of the LLM, pass in the format keyword.
ai = cria.Cria()
prompt = "Return a JSON array of AI companies."
response = ai.chat(prompt, stream=False, format="json")
print(response) # ["OpenAI", "Anthropic", "Meta", "Google", "Cohere", ...].
The current supported formats are:
- JSON
If you have a feature request, feel free to make an issue!
Contributions are highly appreciated.
For Tasks:
Click tags to check more tools for each tasksFor Jobs:
Alternative AI tools for cria
Similar Open Source Tools
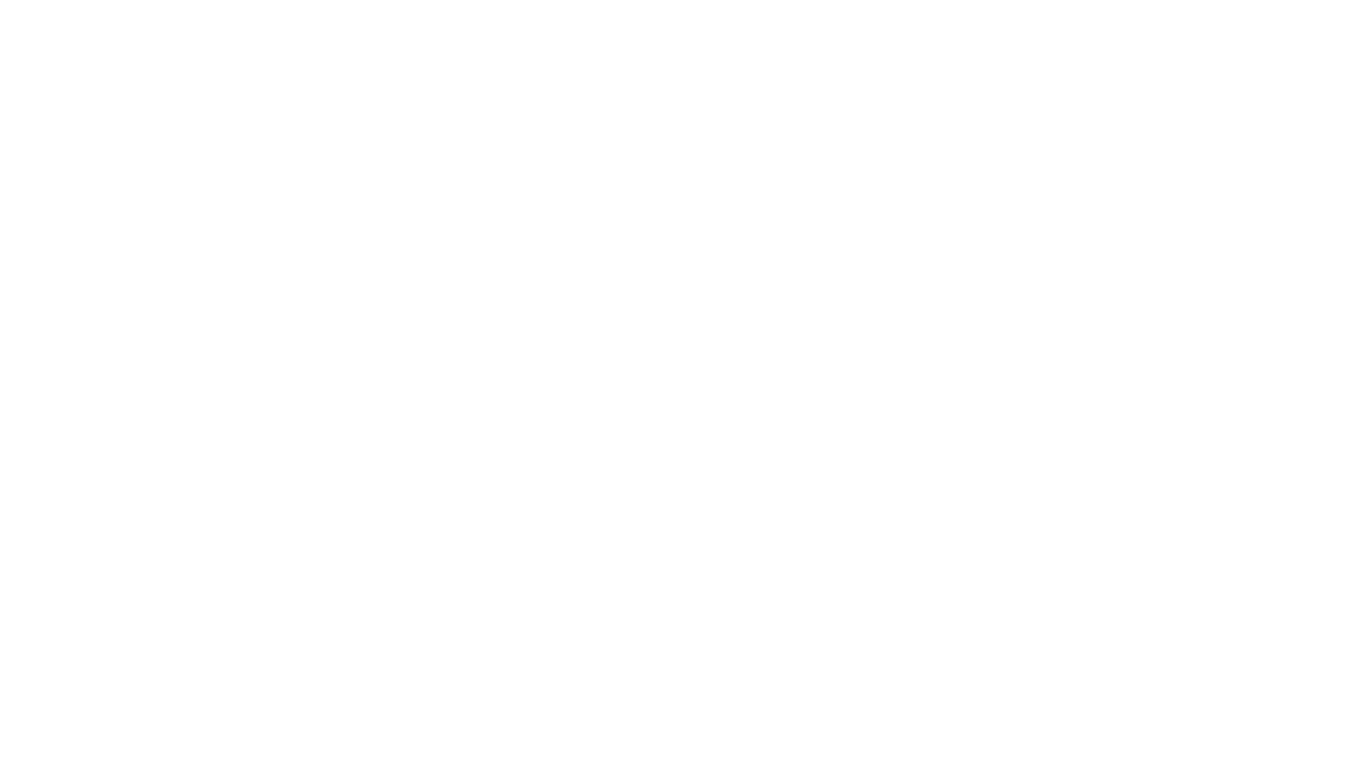
cria
Cria is a Python library designed for running Large Language Models with minimal configuration. It provides an easy and concise way to interact with LLMs, offering advanced features such as custom models, streams, message history management, and running multiple models in parallel. Cria simplifies the process of using LLMs by providing a straightforward API that requires only a few lines of code to get started. It also handles model installation automatically, making it efficient and user-friendly for various natural language processing tasks.
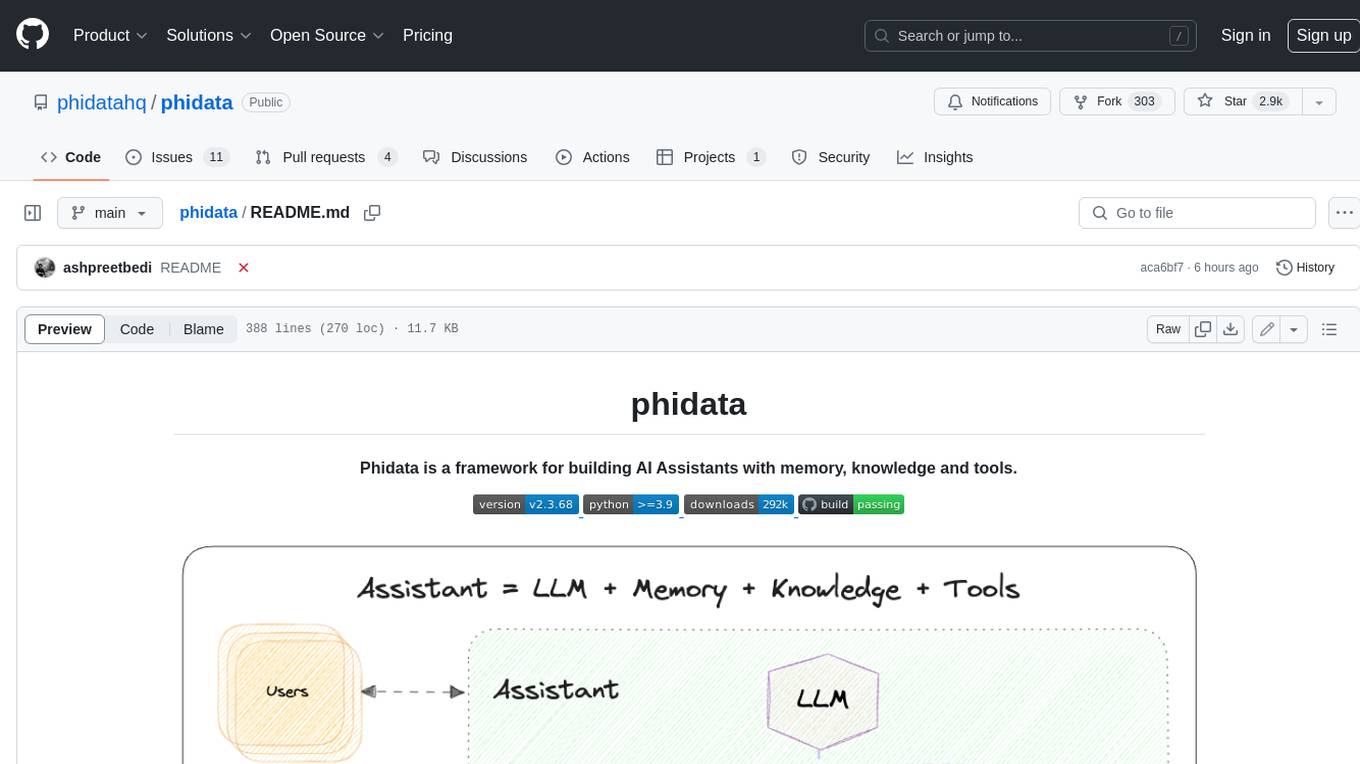
phidata
Phidata is a framework for building AI Assistants with memory, knowledge, and tools. It enables LLMs to have long-term conversations by storing chat history in a database, provides them with business context by storing information in a vector database, and enables them to take actions like pulling data from an API, sending emails, or querying a database. Memory and knowledge make LLMs smarter, while tools make them autonomous.
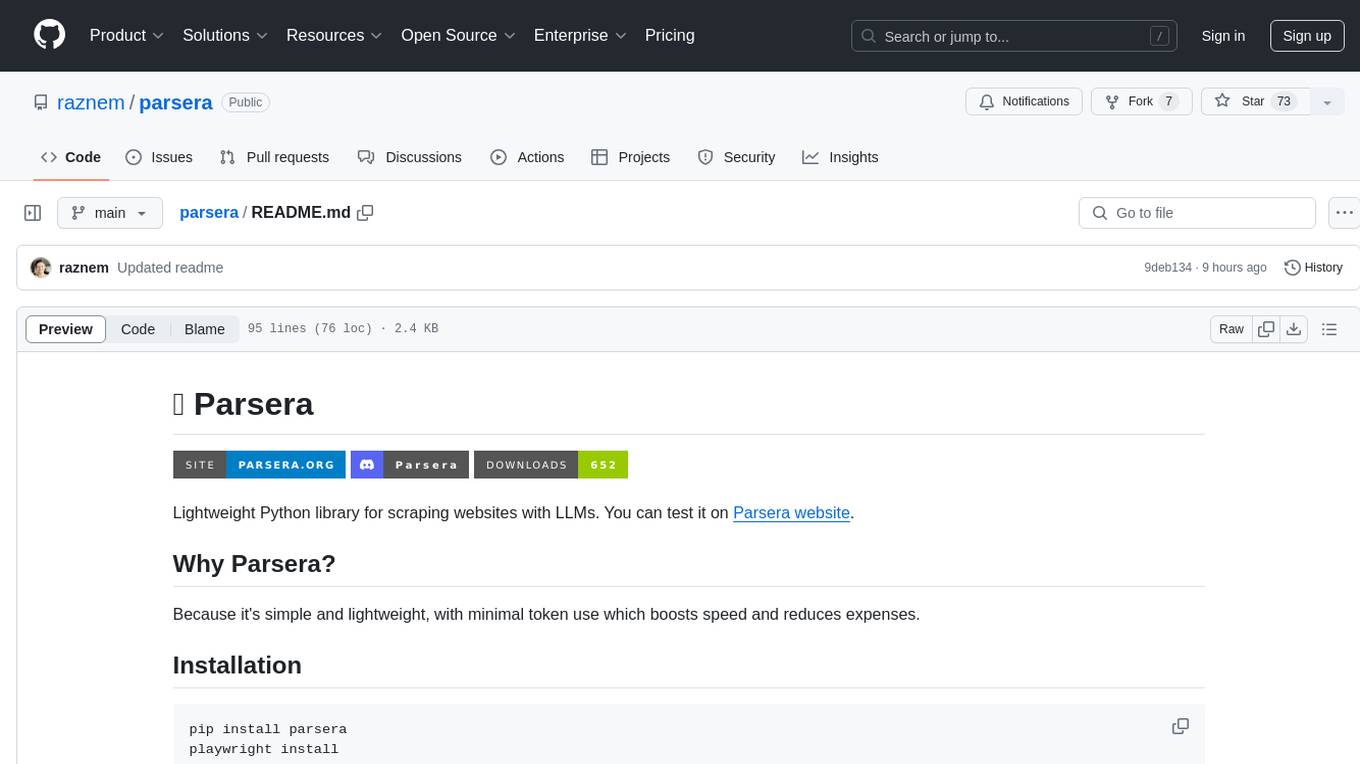
parsera
Parsera is a lightweight Python library designed for scraping websites using LLMs. It offers simplicity and efficiency by minimizing token usage, enhancing speed, and reducing costs. Users can easily set up and run the tool to extract specific elements from web pages, generating JSON output with relevant data. Additionally, Parsera supports integration with various chat models, such as Azure, expanding its functionality and customization options for web scraping tasks.
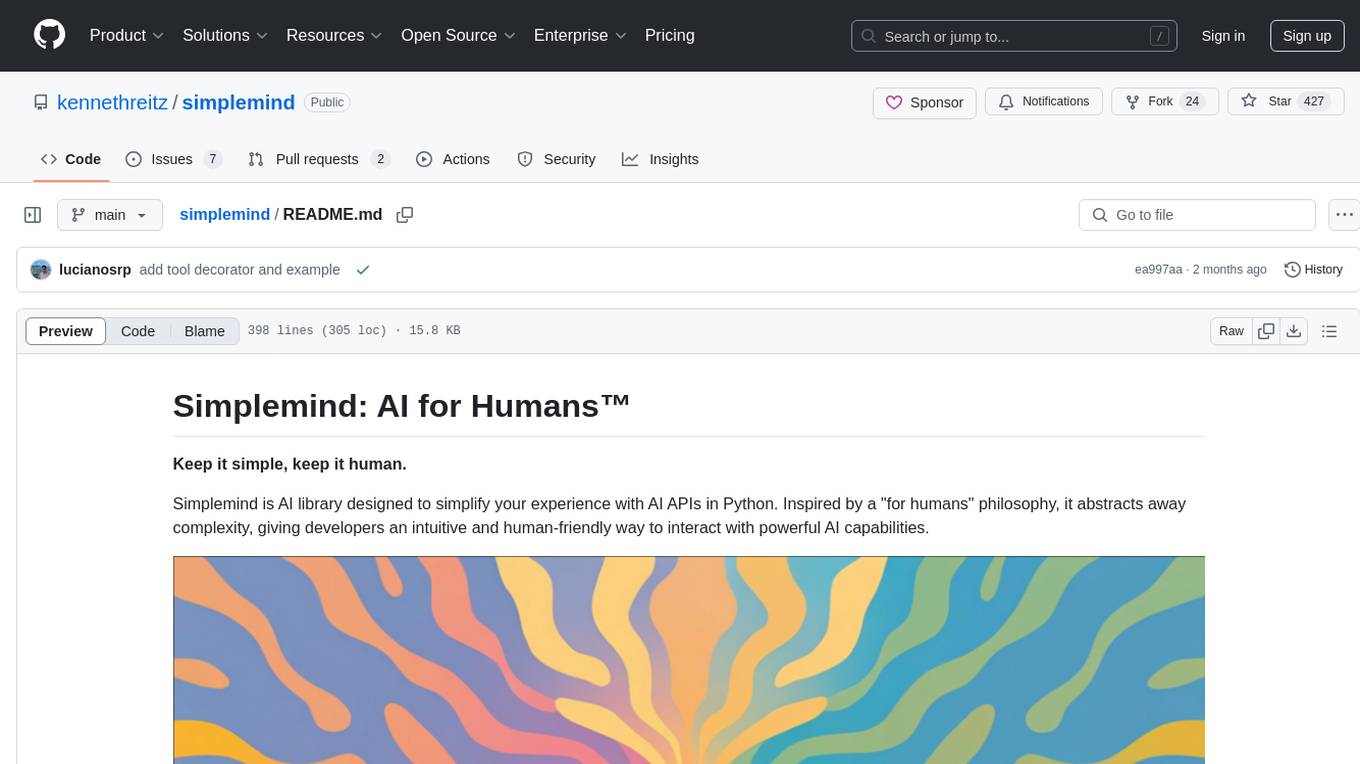
simplemind
Simplemind is an AI library designed to simplify the experience with AI APIs in Python. It provides easy-to-use AI tools with a human-centered design and minimal configuration. Users can tap into powerful AI capabilities through simple interfaces, without needing to be experts. The library supports various APIs from different providers/models and offers features like text completion, streaming text, structured data handling, conversational AI, tool calling, and logging. Simplemind aims to make AI models accessible to all by abstracting away complexity and prioritizing readability and usability.
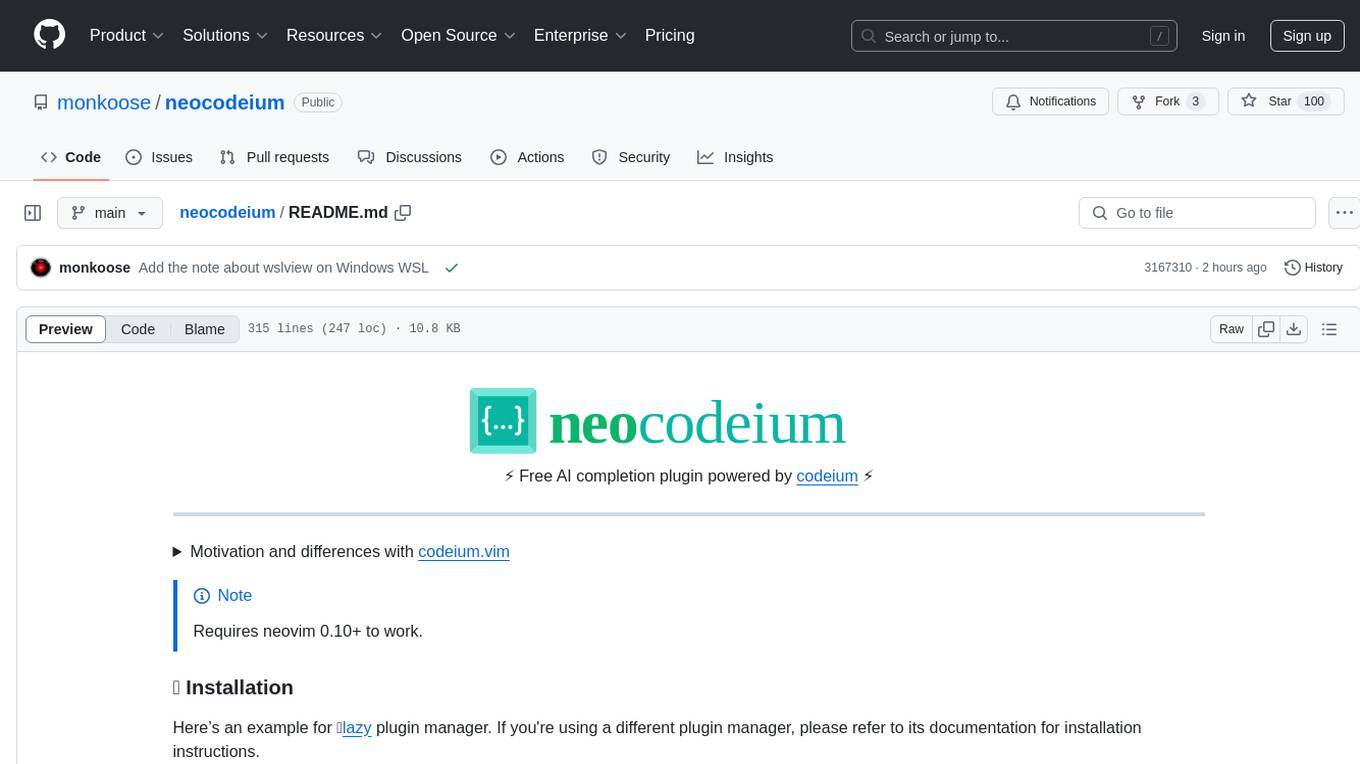
neocodeium
NeoCodeium is a free AI completion plugin powered by Codeium, designed for Neovim users. It aims to provide a smoother experience by eliminating flickering suggestions and allowing for repeatable completions using the `.` key. The plugin offers performance improvements through cache techniques, displays suggestion count labels, and supports Lua scripting. Users can customize keymaps, manage suggestions, and interact with the AI chat feature. NeoCodeium enhances code completion in Neovim, making it a valuable tool for developers seeking efficient coding assistance.
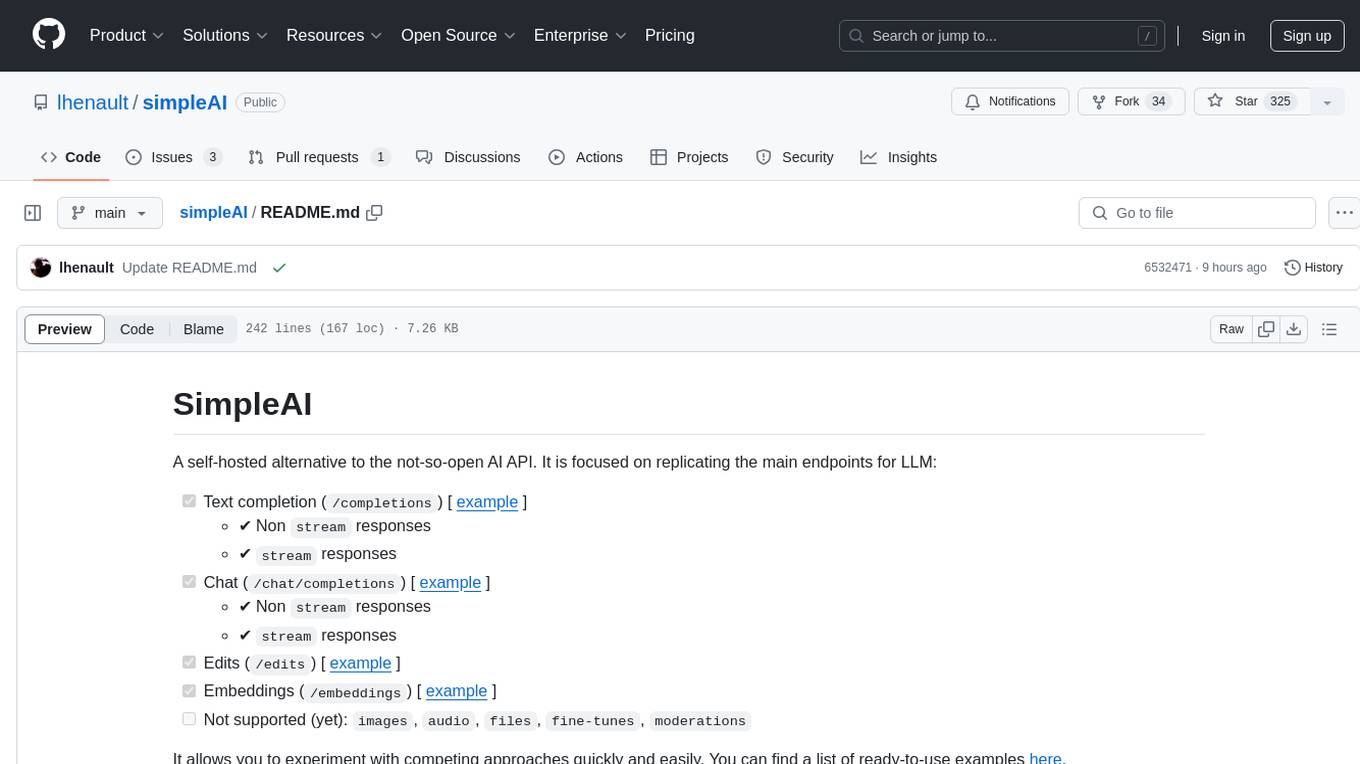
simpleAI
SimpleAI is a self-hosted alternative to the not-so-open AI API, focused on replicating main endpoints for LLM such as text completion, chat, edits, and embeddings. It allows quick experimentation with different models, creating benchmarks, and handling specific use cases without relying on external services. Users can integrate and declare models through gRPC, query endpoints using Swagger UI or API, and resolve common issues like CORS with FastAPI middleware. The project is open for contributions and welcomes PRs, issues, documentation, and more.
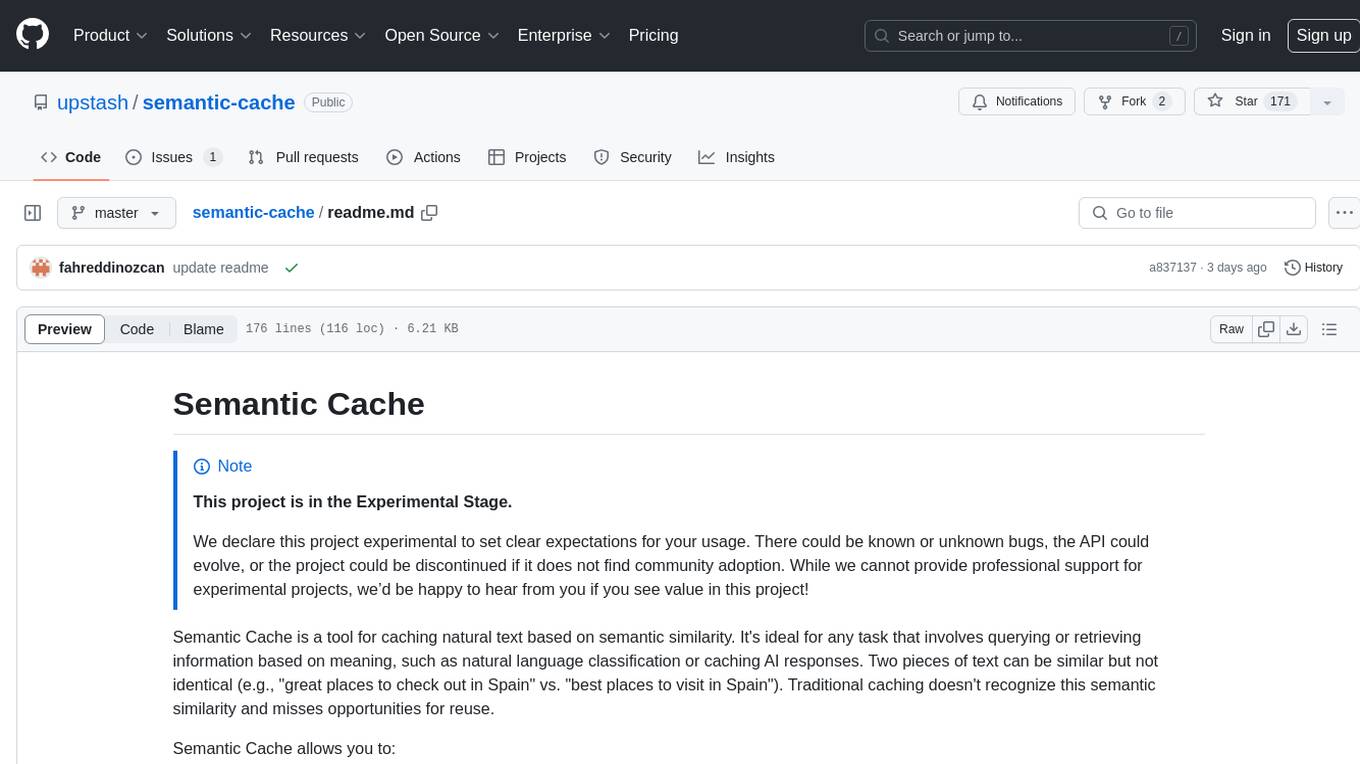
semantic-cache
Semantic Cache is a tool for caching natural text based on semantic similarity. It allows for classifying text into categories, caching AI responses, and reducing API latency by responding to similar queries with cached values. The tool stores cache entries by meaning, handles synonyms, supports multiple languages, understands complex queries, and offers easy integration with Node.js applications. Users can set a custom proximity threshold for filtering results. The tool is ideal for tasks involving querying or retrieving information based on meaning, such as natural language classification or caching AI responses.
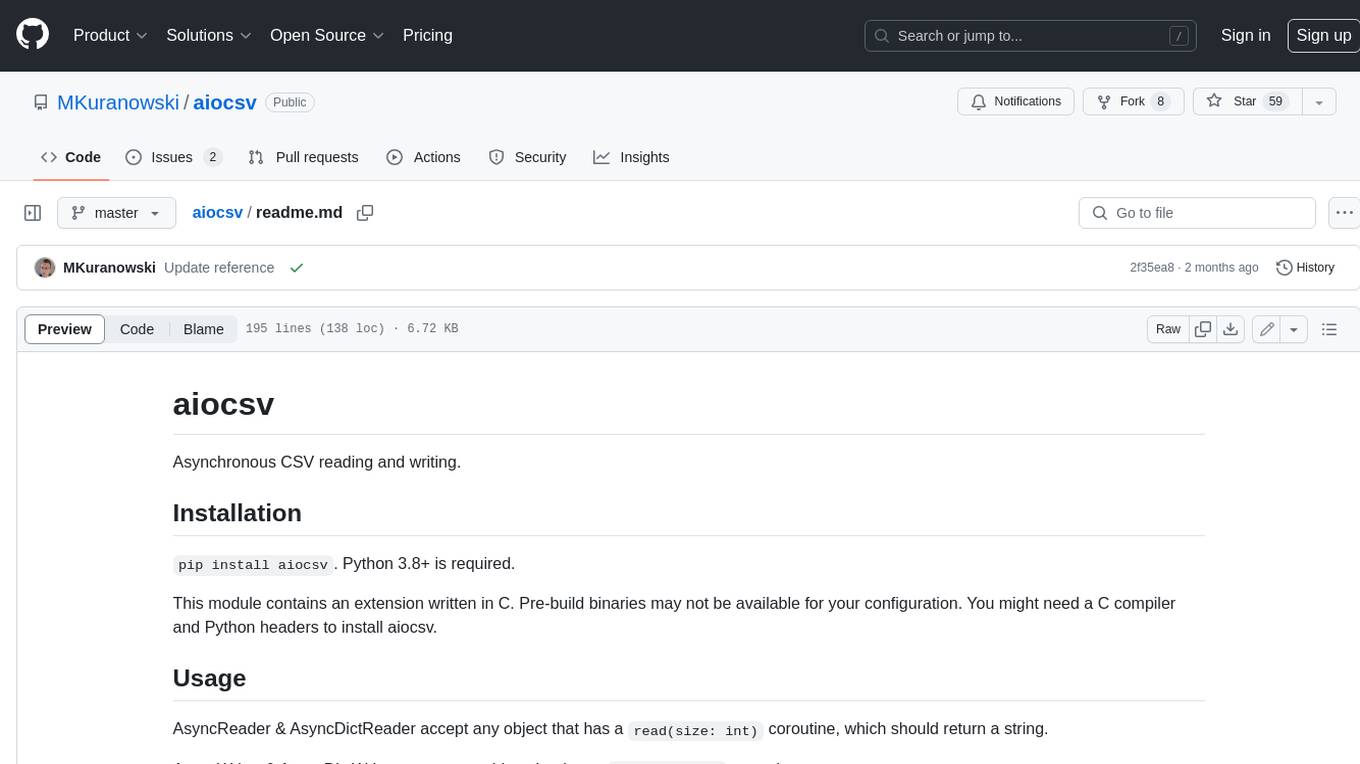
aiocsv
aiocsv is a Python module that provides asynchronous CSV reading and writing. It is designed to be a drop-in replacement for the Python's builtin csv module, but with the added benefit of being able to read and write CSV files asynchronously. This makes it ideal for use in applications that need to process large CSV files efficiently.
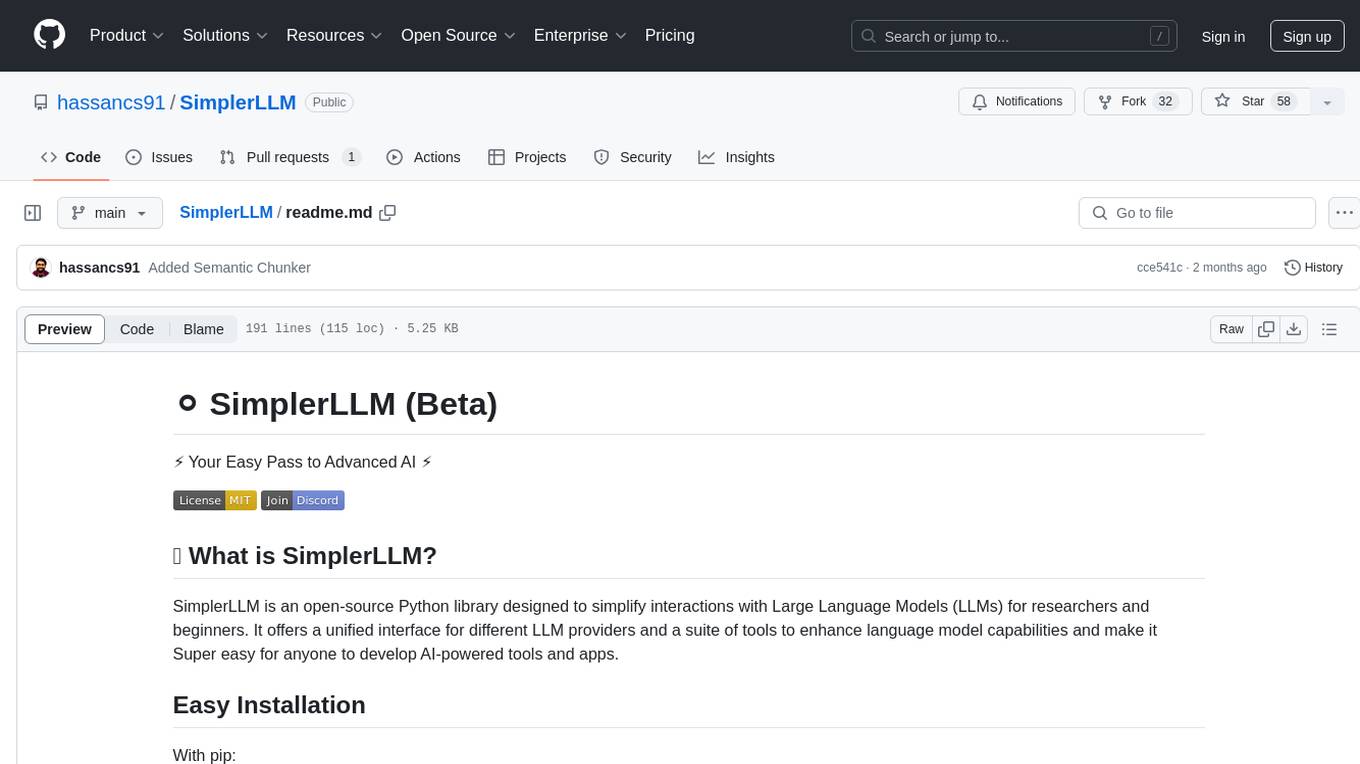
SimplerLLM
SimplerLLM is an open-source Python library that simplifies interactions with Large Language Models (LLMs) for researchers and beginners. It provides a unified interface for different LLM providers, tools for enhancing language model capabilities, and easy development of AI-powered tools and apps. The library offers features like unified LLM interface, generic text loader, RapidAPI connector, SERP integration, prompt template builder, and more. Users can easily set up environment variables, create LLM instances, use tools like SERP, generic text loader, calling RapidAPI APIs, and prompt template builder. Additionally, the library includes chunking functions to split texts into manageable chunks based on different criteria. Future updates will bring more tools, interactions with local LLMs, prompt optimization, response evaluation, GPT Trainer, document chunker, advanced document loader, integration with more providers, Simple RAG with SimplerVectors, integration with vector databases, agent builder, and LLM server.
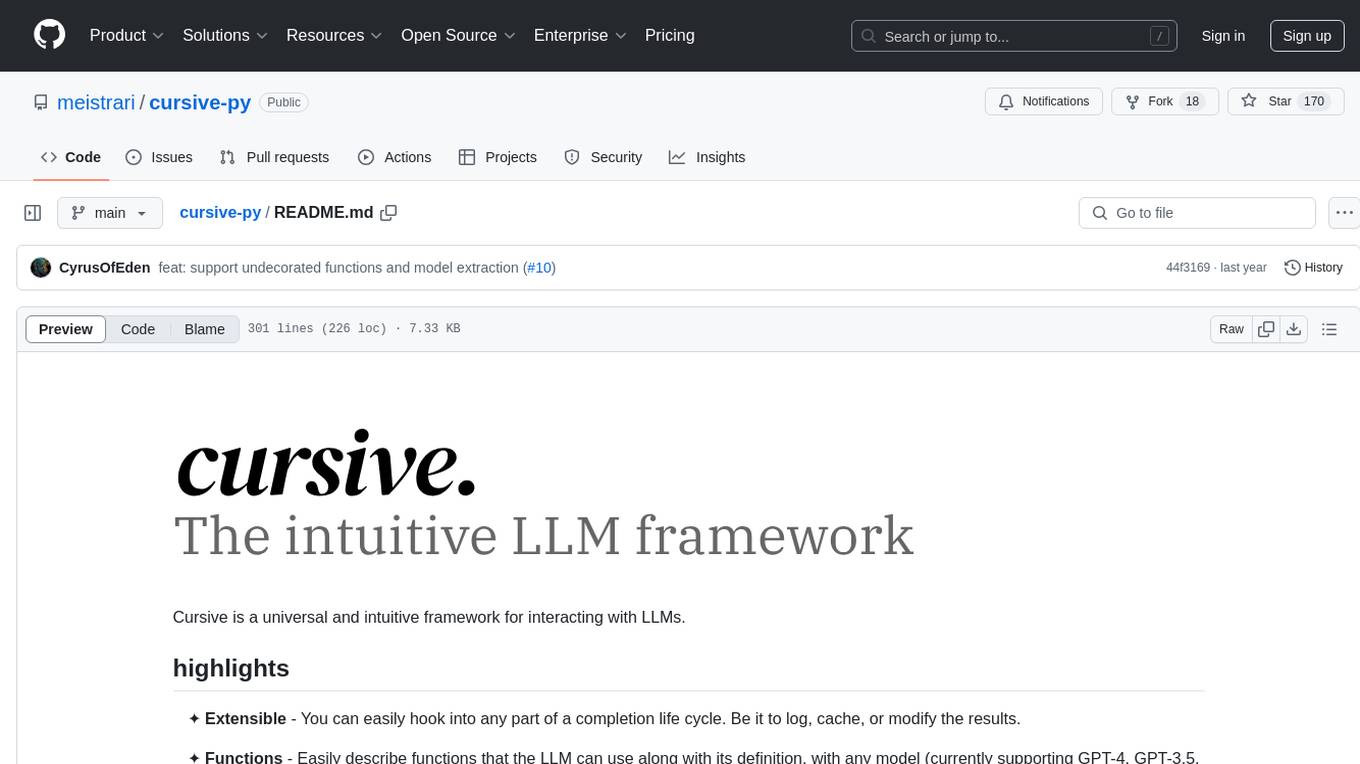
cursive-py
Cursive is a universal and intuitive framework for interacting with LLMs. It is extensible, allowing users to hook into any part of a completion life cycle. Users can easily describe functions that LLMs can use with any supported model. Cursive aims to bridge capabilities between different models, providing a single interface for users to choose any model. It comes with built-in token usage and costs calculations, automatic retry, and model expanding features. Users can define and describe functions, generate Pydantic BaseModels, hook into completion life cycle, create embeddings, and configure retry and model expanding behavior. Cursive supports various models from OpenAI, Anthropic, OpenRouter, Cohere, and Replicate, with options to pass API keys for authentication.
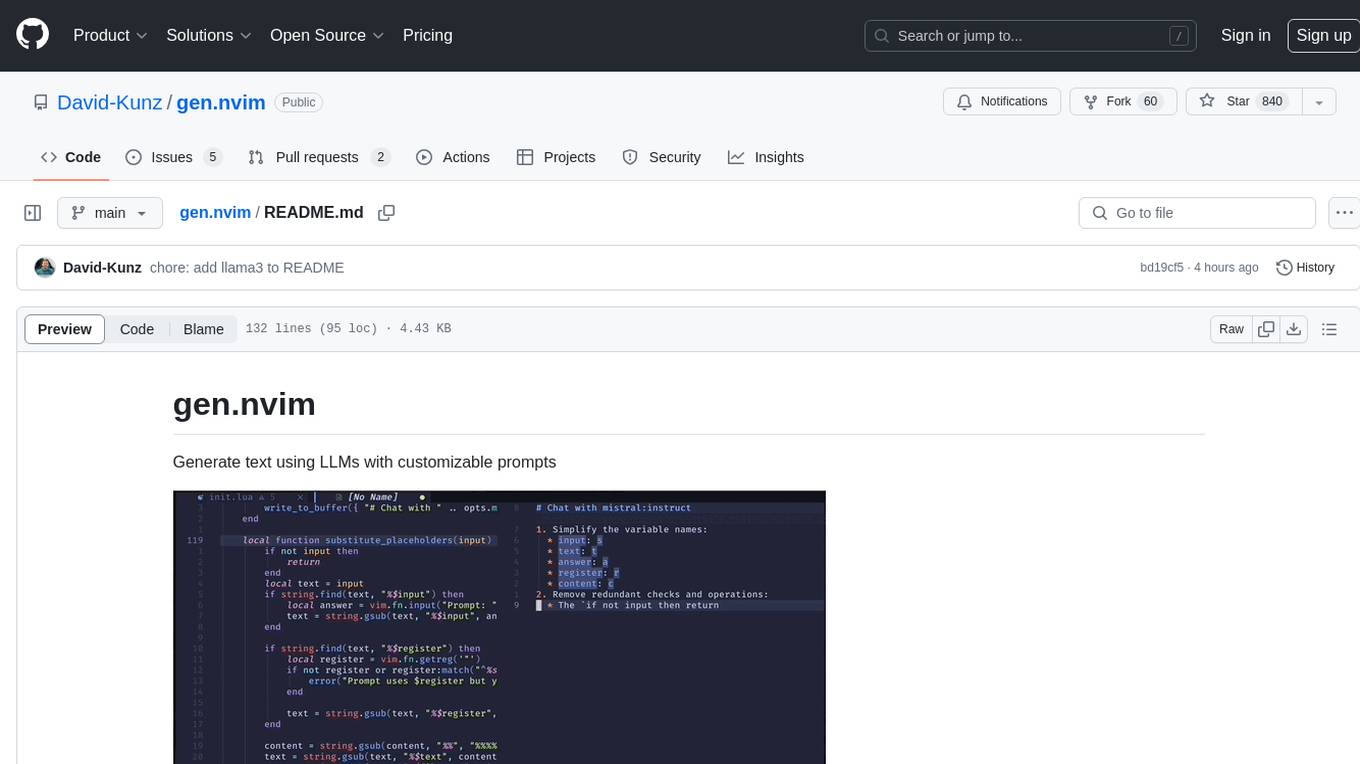
gen.nvim
gen.nvim is a tool that allows users to generate text using Language Models (LLMs) with customizable prompts. It requires Ollama with models like `llama3`, `mistral`, or `zephyr`, along with Curl for installation. Users can use the `Gen` command to generate text based on predefined or custom prompts. The tool provides key maps for easy invocation and allows for follow-up questions during conversations. Additionally, users can select a model from a list of installed models and customize prompts as needed.
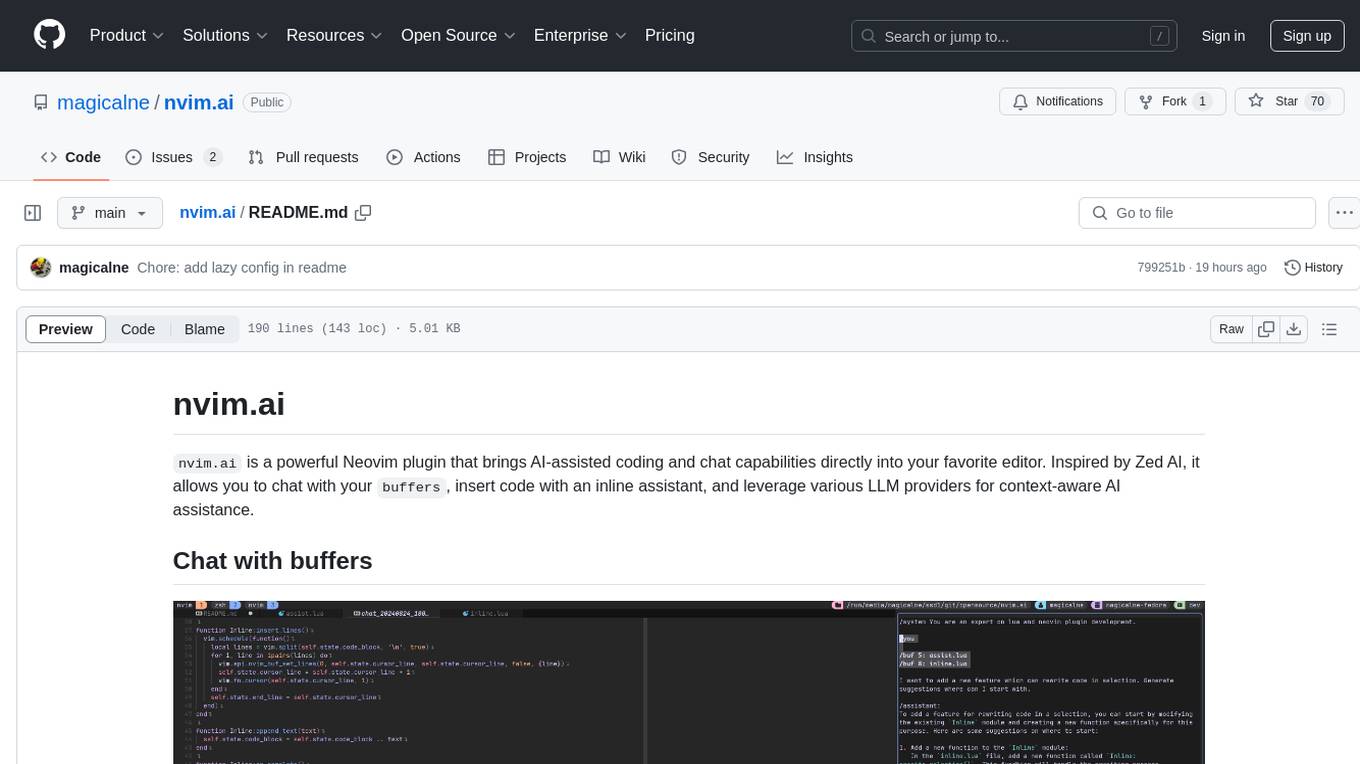
nvim.ai
nvim.ai is a powerful Neovim plugin that enables AI-assisted coding and chat capabilities within the editor. Users can chat with buffers, insert code with an inline assistant, and utilize various LLM providers for context-aware AI assistance. The plugin supports features like interacting with AI about code and documents, receiving relevant help based on current work, code insertion, code rewriting (Work in Progress), and integration with multiple LLM providers. Users can configure the plugin, add API keys to dotfiles, and integrate with nvim-cmp for command autocompletion. Keymaps are available for chat and inline assist functionalities. The chat dialog allows parsing content with keywords and supports roles like /system, /you, and /assistant. Context-aware assistance can be accessed through inline assist by inserting code blocks anywhere in the file.
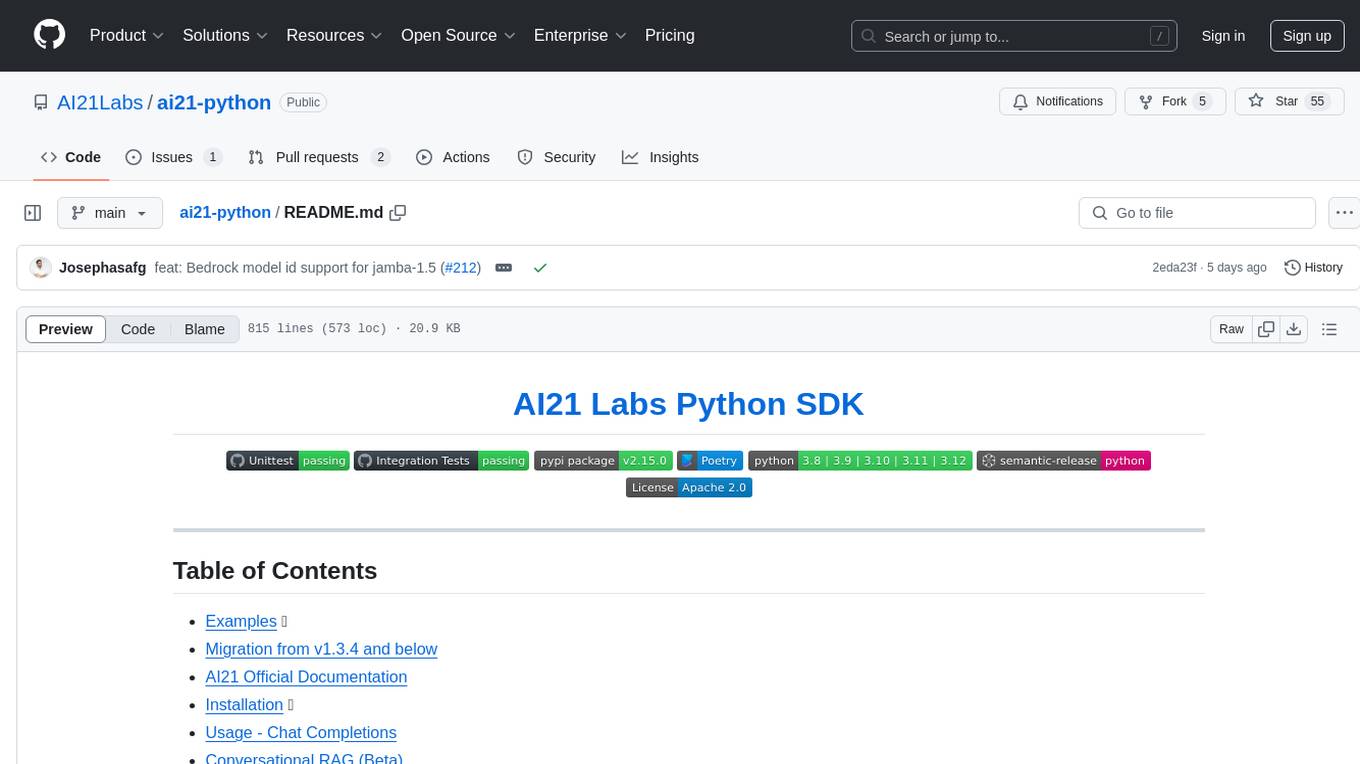
ai21-python
The AI21 Labs Python SDK is a comprehensive tool for interacting with the AI21 API. It provides functionalities for chat completions, conversational RAG, token counting, error handling, and support for various cloud providers like AWS, Azure, and Vertex. The SDK offers both synchronous and asynchronous usage, along with detailed examples and documentation. Users can quickly get started with the SDK to leverage AI21's powerful models for various natural language processing tasks.
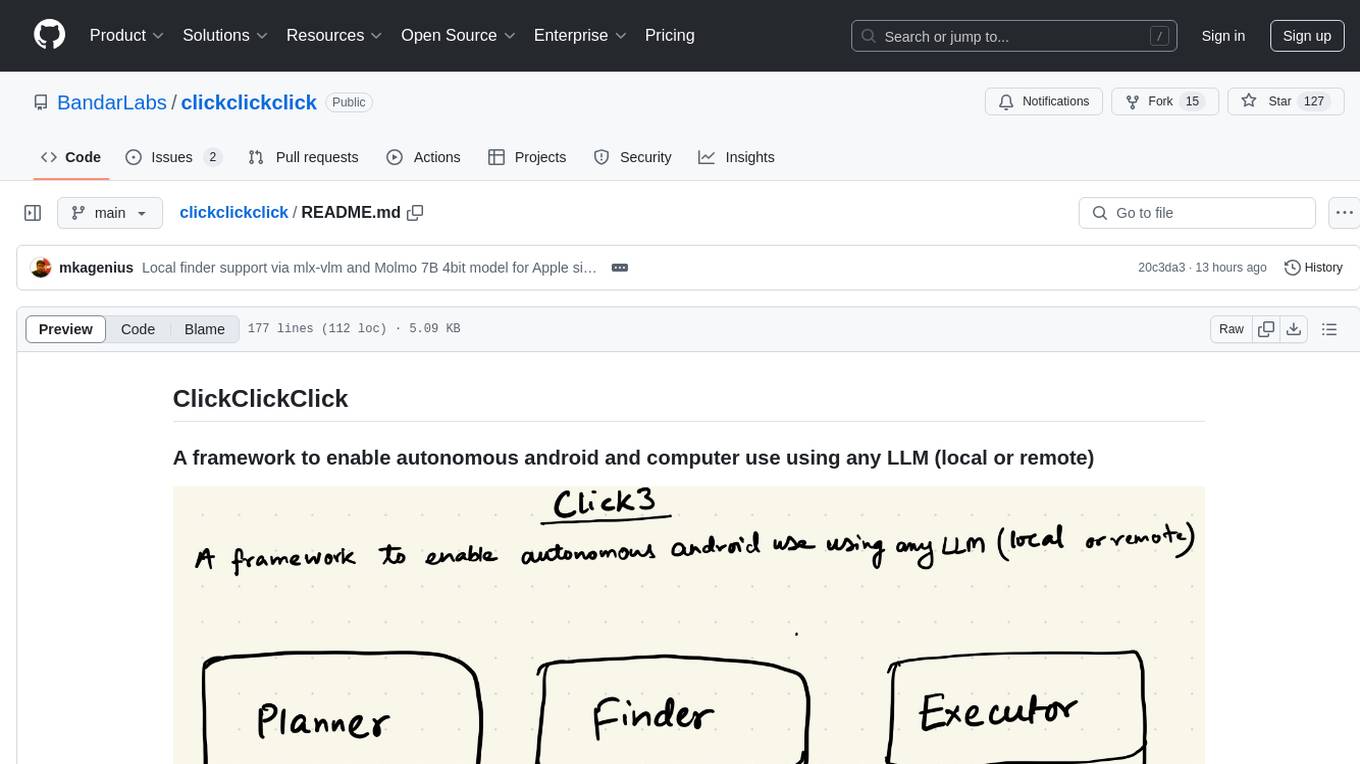
clickclickclick
ClickClickClick is a framework designed to enable autonomous Android and computer use using various LLM models, both locally and remotely. It supports tasks such as drafting emails, opening browsers, and starting games, with current support for local models via Ollama, Gemini, and GPT 4o. The tool is highly experimental and evolving, with the best results achieved using specific model combinations. Users need prerequisites like `adb` installation and USB debugging enabled on Android phones. The tool can be installed via cloning the repository, setting up a virtual environment, and installing dependencies. It can be used as a CLI tool or script, allowing users to configure planner and finder models for different tasks. Additionally, it can be used as an API to execute tasks based on provided prompts, platform, and models.
For similar tasks
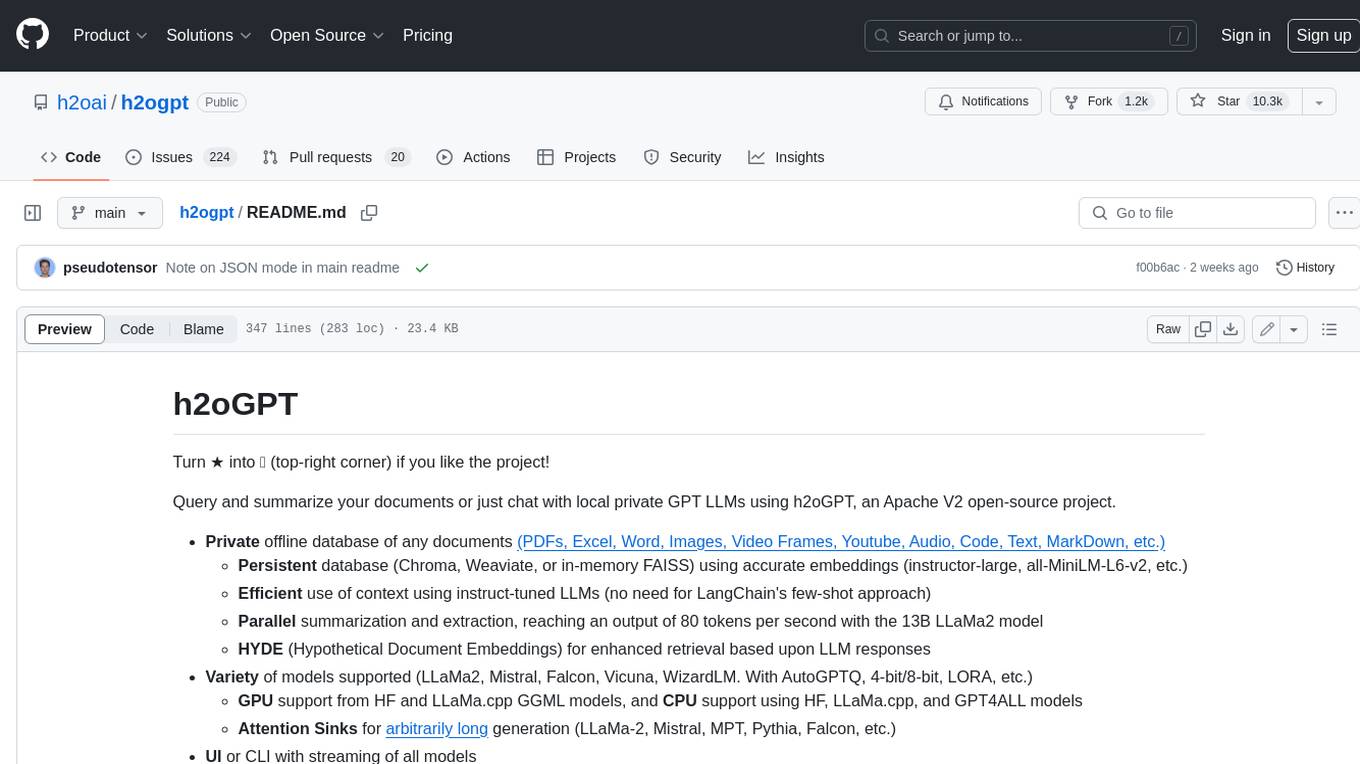
h2ogpt
h2oGPT is an Apache V2 open-source project that allows users to query and summarize documents or chat with local private GPT LLMs. It features a private offline database of any documents (PDFs, Excel, Word, Images, Video Frames, Youtube, Audio, Code, Text, MarkDown, etc.), a persistent database (Chroma, Weaviate, or in-memory FAISS) using accurate embeddings (instructor-large, all-MiniLM-L6-v2, etc.), and efficient use of context using instruct-tuned LLMs (no need for LangChain's few-shot approach). h2oGPT also offers parallel summarization and extraction, reaching an output of 80 tokens per second with the 13B LLaMa2 model, HYDE (Hypothetical Document Embeddings) for enhanced retrieval based upon LLM responses, a variety of models supported (LLaMa2, Mistral, Falcon, Vicuna, WizardLM. With AutoGPTQ, 4-bit/8-bit, LORA, etc.), GPU support from HF and LLaMa.cpp GGML models, and CPU support using HF, LLaMa.cpp, and GPT4ALL models. Additionally, h2oGPT provides Attention Sinks for arbitrarily long generation (LLaMa-2, Mistral, MPT, Pythia, Falcon, etc.), a UI or CLI with streaming of all models, the ability to upload and view documents through the UI (control multiple collaborative or personal collections), Vision Models LLaVa, Claude-3, Gemini-Pro-Vision, GPT-4-Vision, Image Generation Stable Diffusion (sdxl-turbo, sdxl) and PlaygroundAI (playv2), Voice STT using Whisper with streaming audio conversion, Voice TTS using MIT-Licensed Microsoft Speech T5 with multiple voices and Streaming audio conversion, Voice TTS using MPL2-Licensed TTS including Voice Cloning and Streaming audio conversion, AI Assistant Voice Control Mode for hands-free control of h2oGPT chat, Bake-off UI mode against many models at the same time, Easy Download of model artifacts and control over models like LLaMa.cpp through the UI, Authentication in the UI by user/password via Native or Google OAuth, State Preservation in the UI by user/password, Linux, Docker, macOS, and Windows support, Easy Windows Installer for Windows 10 64-bit (CPU/CUDA), Easy macOS Installer for macOS (CPU/M1/M2), Inference Servers support (oLLaMa, HF TGI server, vLLM, Gradio, ExLLaMa, Replicate, OpenAI, Azure OpenAI, Anthropic), OpenAI-compliant, Server Proxy API (h2oGPT acts as drop-in-replacement to OpenAI server), Python client API (to talk to Gradio server), JSON Mode with any model via code block extraction. Also supports MistralAI JSON mode, Claude-3 via function calling with strict Schema, OpenAI via JSON mode, and vLLM via guided_json with strict Schema, Web-Search integration with Chat and Document Q/A, Agents for Search, Document Q/A, Python Code, CSV frames (Experimental, best with OpenAI currently), Evaluate performance using reward models, and Quality maintained with over 1000 unit and integration tests taking over 4 GPU-hours.
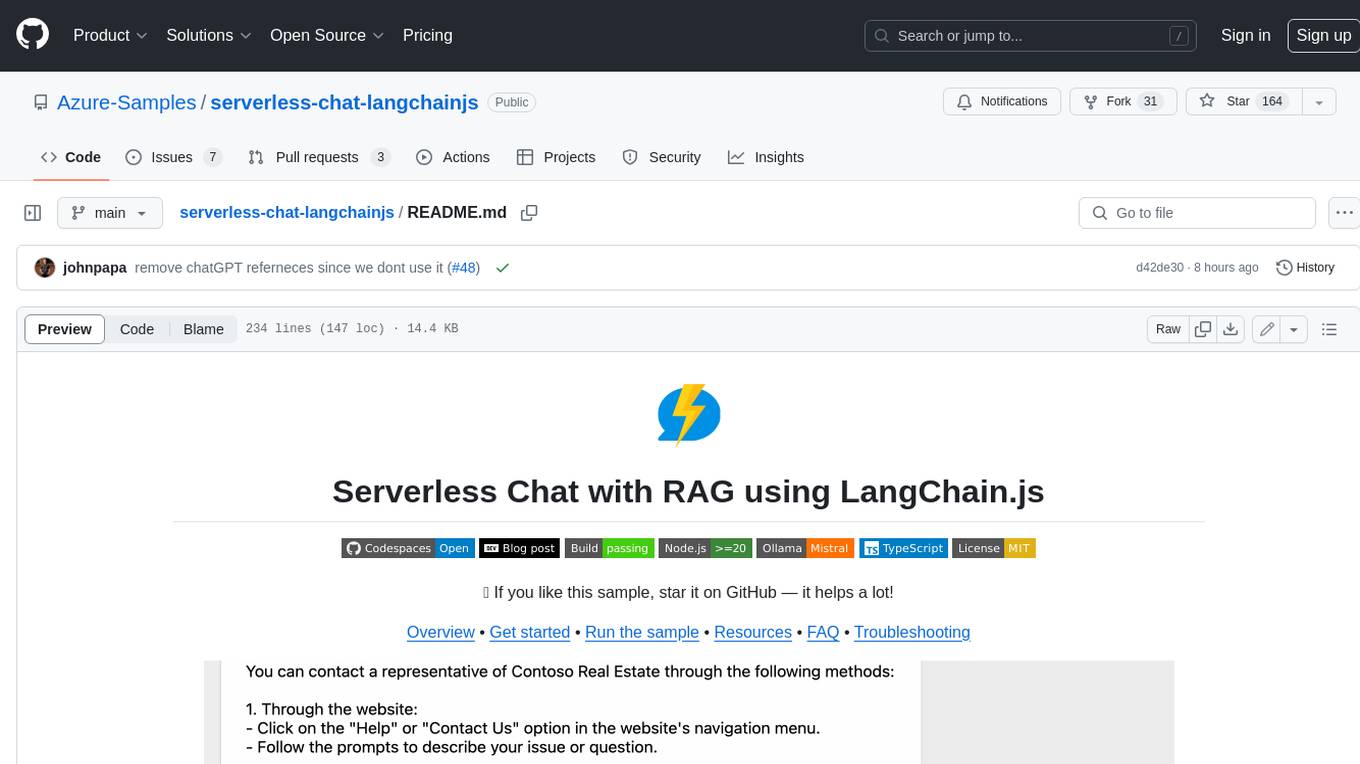
serverless-chat-langchainjs
This sample shows how to build a serverless chat experience with Retrieval-Augmented Generation using LangChain.js and Azure. The application is hosted on Azure Static Web Apps and Azure Functions, with Azure Cosmos DB for MongoDB vCore as the vector database. You can use it as a starting point for building more complex AI applications.
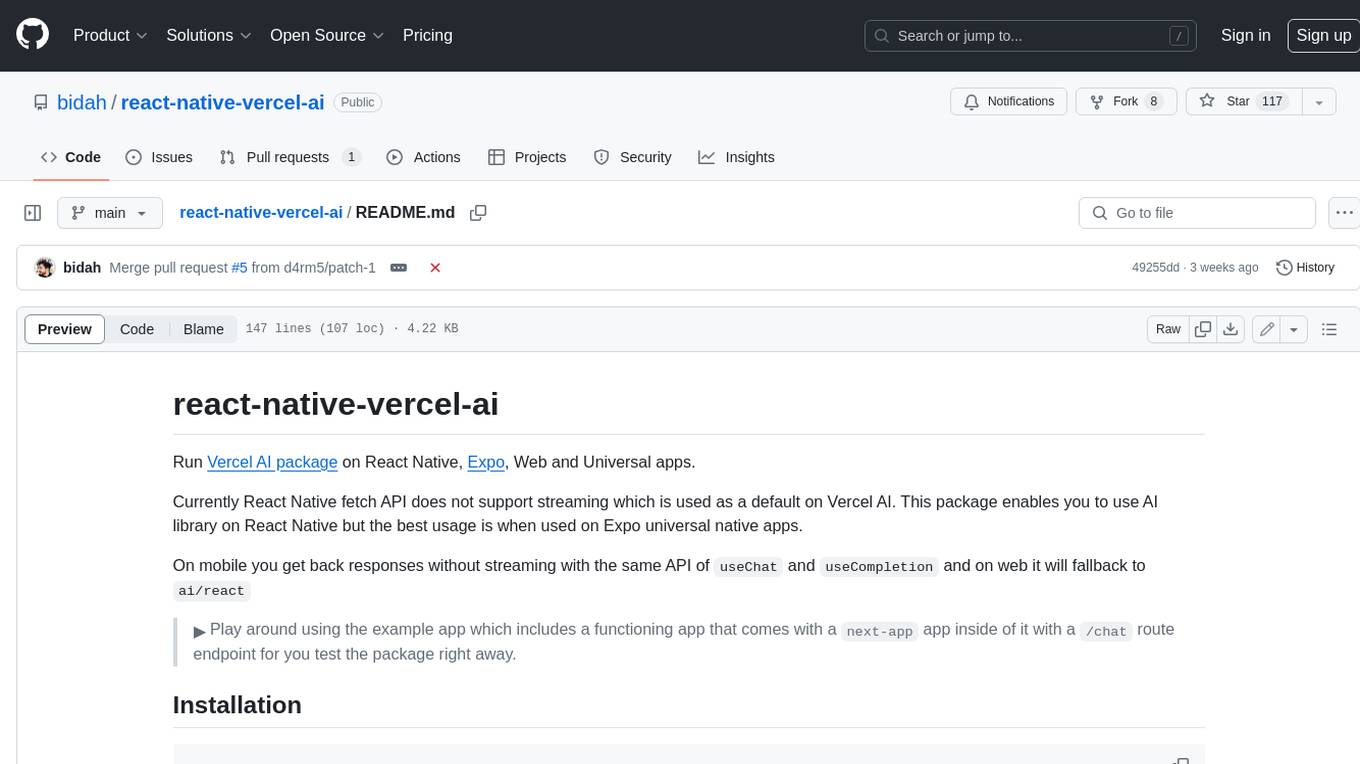
react-native-vercel-ai
Run Vercel AI package on React Native, Expo, Web and Universal apps. Currently React Native fetch API does not support streaming which is used as a default on Vercel AI. This package enables you to use AI library on React Native but the best usage is when used on Expo universal native apps. On mobile you get back responses without streaming with the same API of `useChat` and `useCompletion` and on web it will fallback to `ai/react`
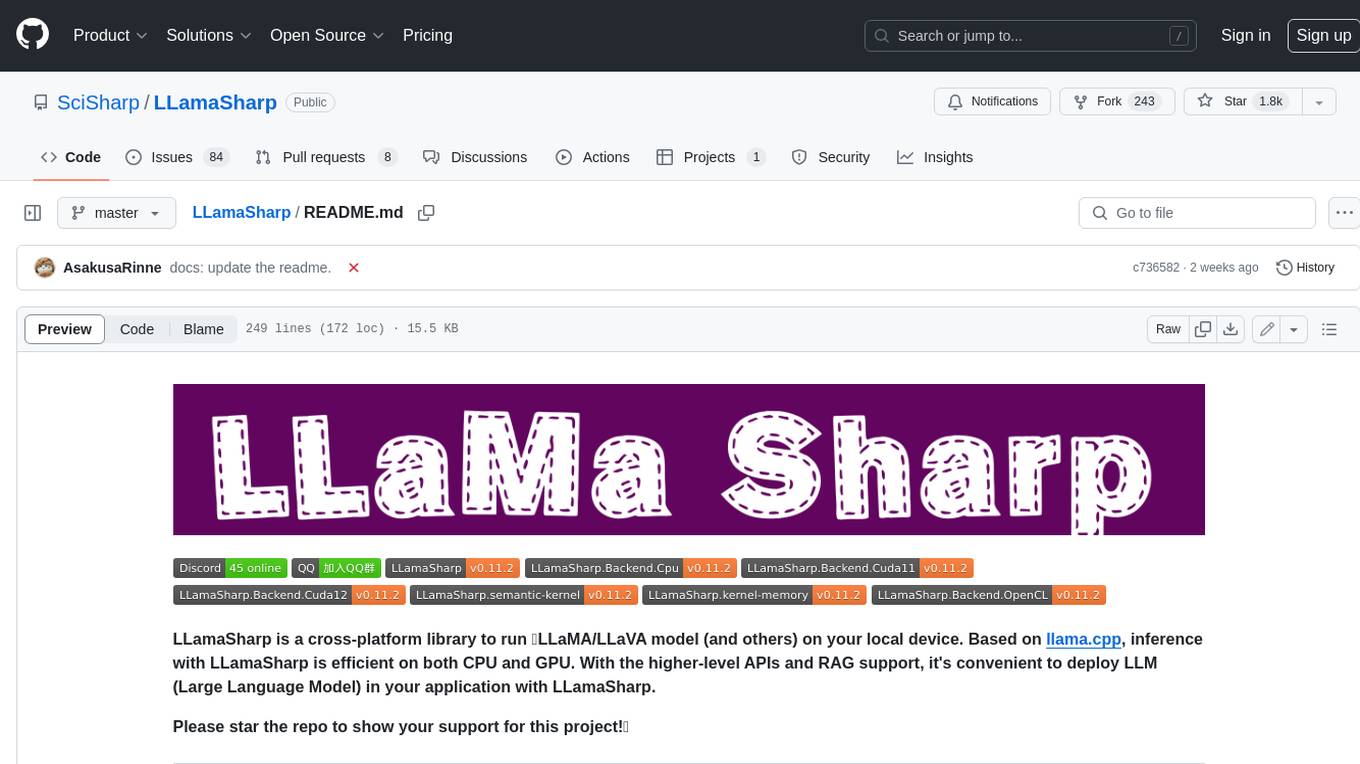
LLamaSharp
LLamaSharp is a cross-platform library to run 🦙LLaMA/LLaVA model (and others) on your local device. Based on llama.cpp, inference with LLamaSharp is efficient on both CPU and GPU. With the higher-level APIs and RAG support, it's convenient to deploy LLM (Large Language Model) in your application with LLamaSharp.
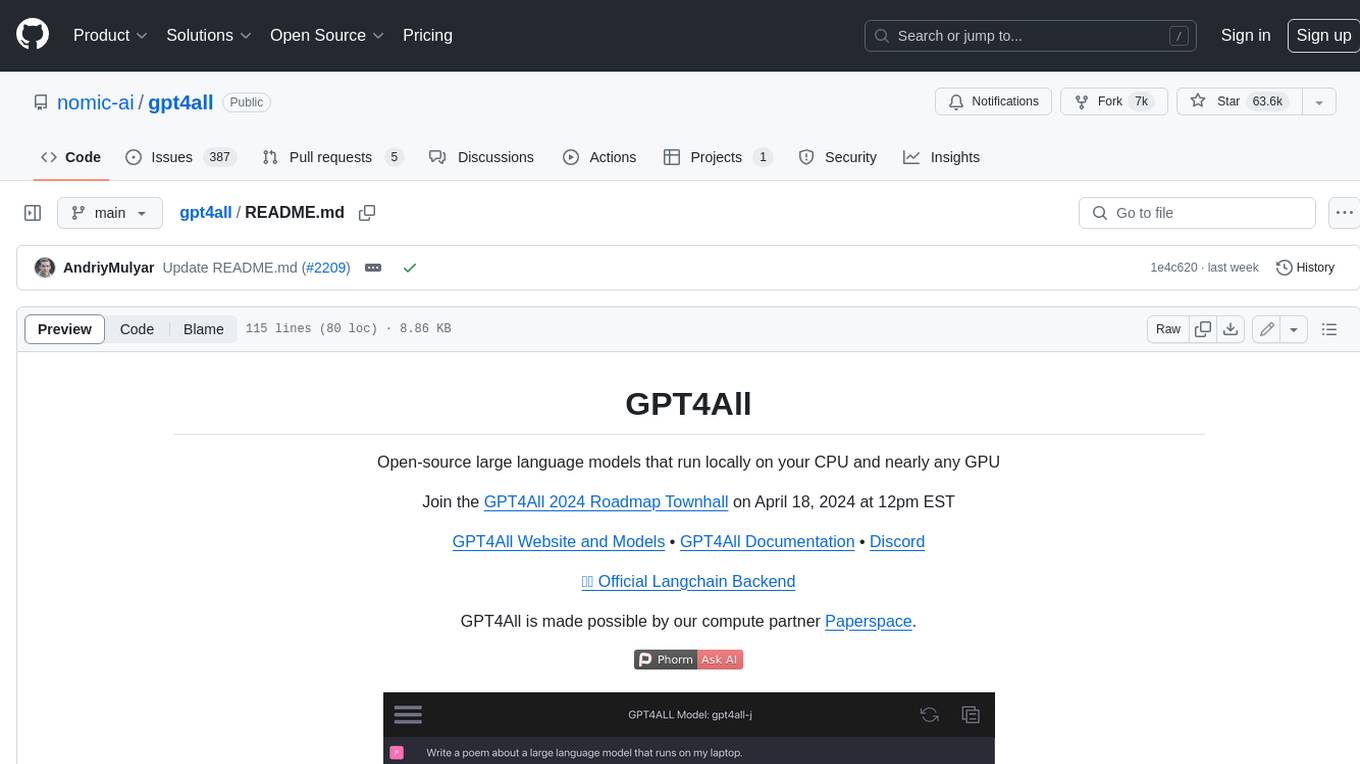
gpt4all
GPT4All is an ecosystem to run powerful and customized large language models that work locally on consumer grade CPUs and any GPU. Note that your CPU needs to support AVX or AVX2 instructions. Learn more in the documentation. A GPT4All model is a 3GB - 8GB file that you can download and plug into the GPT4All open-source ecosystem software. Nomic AI supports and maintains this software ecosystem to enforce quality and security alongside spearheading the effort to allow any person or enterprise to easily train and deploy their own on-edge large language models.
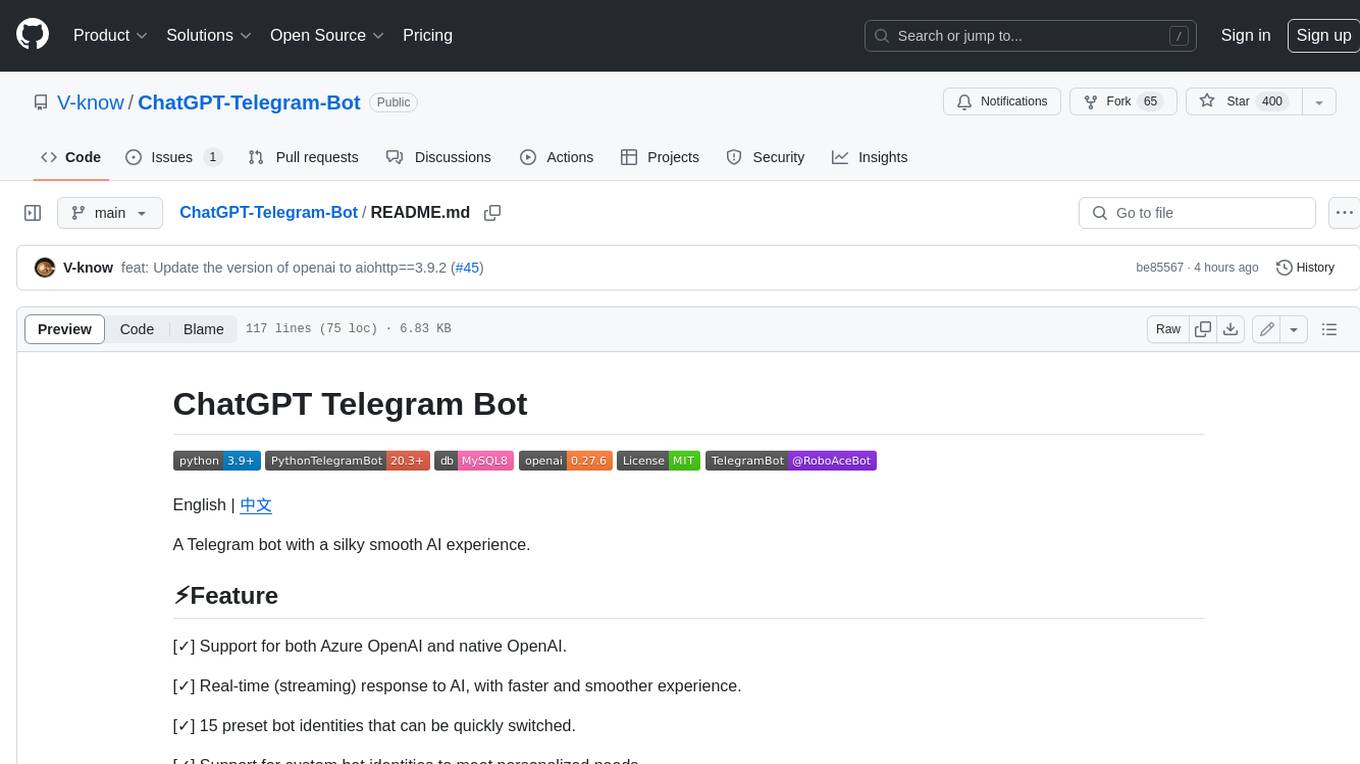
ChatGPT-Telegram-Bot
ChatGPT Telegram Bot is a Telegram bot that provides a smooth AI experience. It supports both Azure OpenAI and native OpenAI, and offers real-time (streaming) response to AI, with a faster and smoother experience. The bot also has 15 preset bot identities that can be quickly switched, and supports custom bot identities to meet personalized needs. Additionally, it supports clearing the contents of the chat with a single click, and restarting the conversation at any time. The bot also supports native Telegram bot button support, making it easy and intuitive to implement required functions. User level division is also supported, with different levels enjoying different single session token numbers, context numbers, and session frequencies. The bot supports English and Chinese on UI, and is containerized for easy deployment.
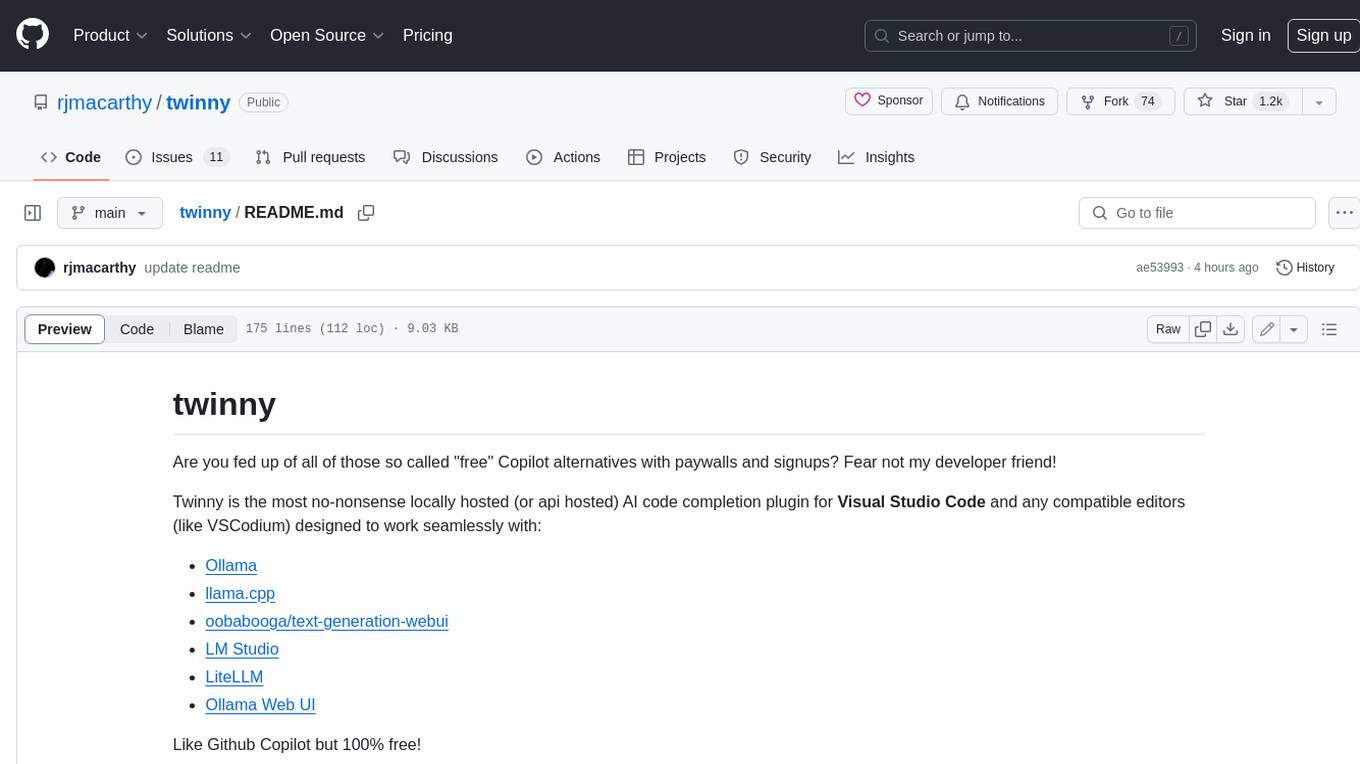
twinny
Twinny is a free and open-source AI code completion plugin for Visual Studio Code and compatible editors. It integrates with various tools and frameworks, including Ollama, llama.cpp, oobabooga/text-generation-webui, LM Studio, LiteLLM, and Open WebUI. Twinny offers features such as fill-in-the-middle code completion, chat with AI about your code, customizable API endpoints, and support for single or multiline fill-in-middle completions. It is easy to install via the Visual Studio Code extensions marketplace and provides a range of customization options. Twinny supports both online and offline operation and conforms to the OpenAI API standard.
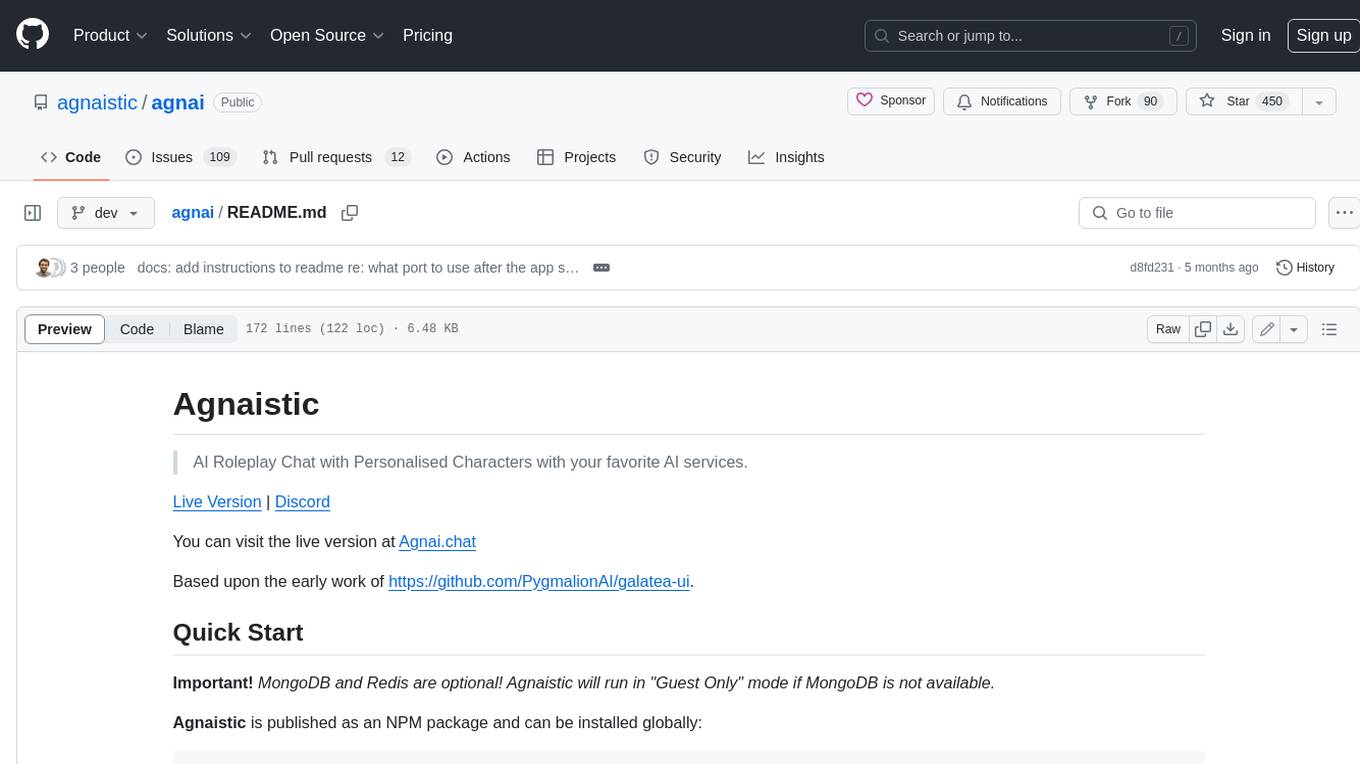
agnai
Agnaistic is an AI roleplay chat tool that allows users to interact with personalized characters using their favorite AI services. It supports multiple AI services, persona schema formats, and features such as group conversations, user authentication, and memory/lore books. Agnaistic can be self-hosted or run using Docker, and it provides a range of customization options through its settings.json file. The tool is designed to be user-friendly and accessible, making it suitable for both casual users and developers.
For similar jobs
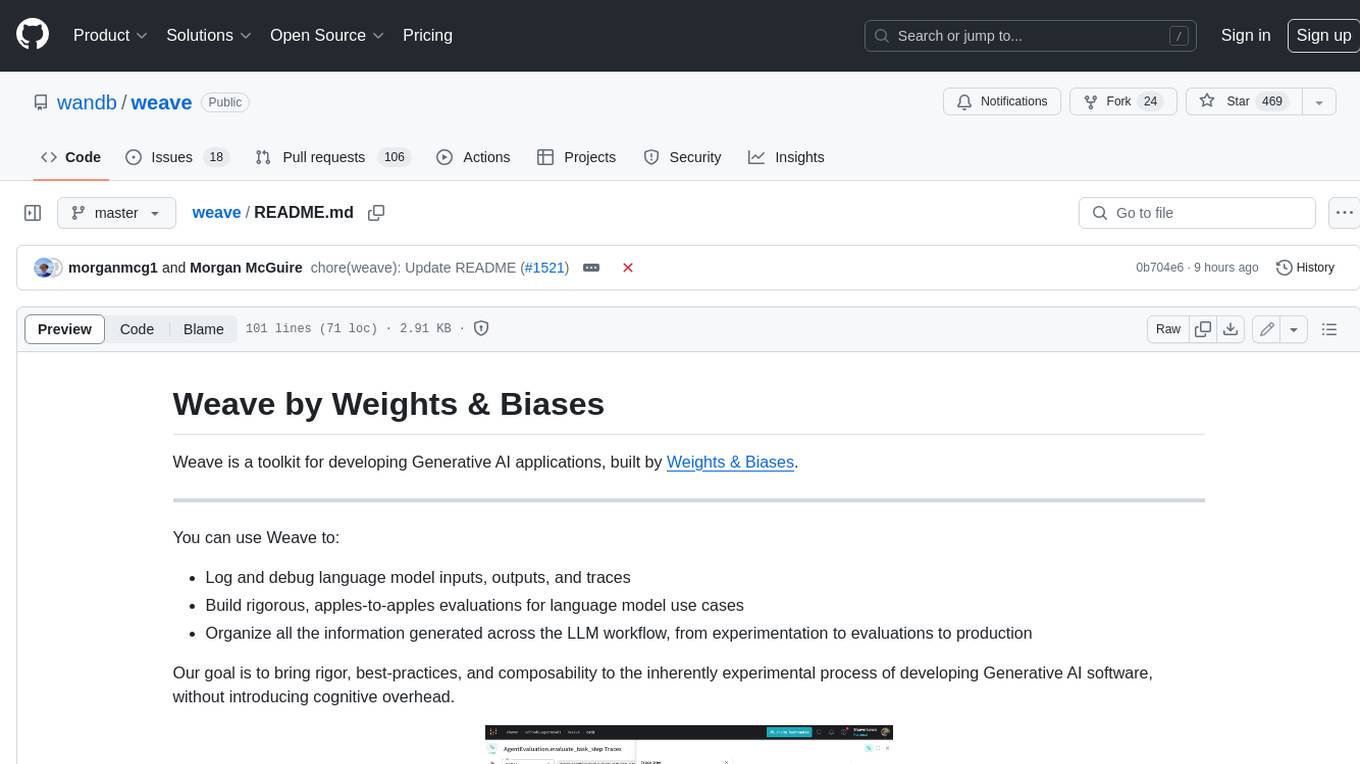
weave
Weave is a toolkit for developing Generative AI applications, built by Weights & Biases. With Weave, you can log and debug language model inputs, outputs, and traces; build rigorous, apples-to-apples evaluations for language model use cases; and organize all the information generated across the LLM workflow, from experimentation to evaluations to production. Weave aims to bring rigor, best-practices, and composability to the inherently experimental process of developing Generative AI software, without introducing cognitive overhead.
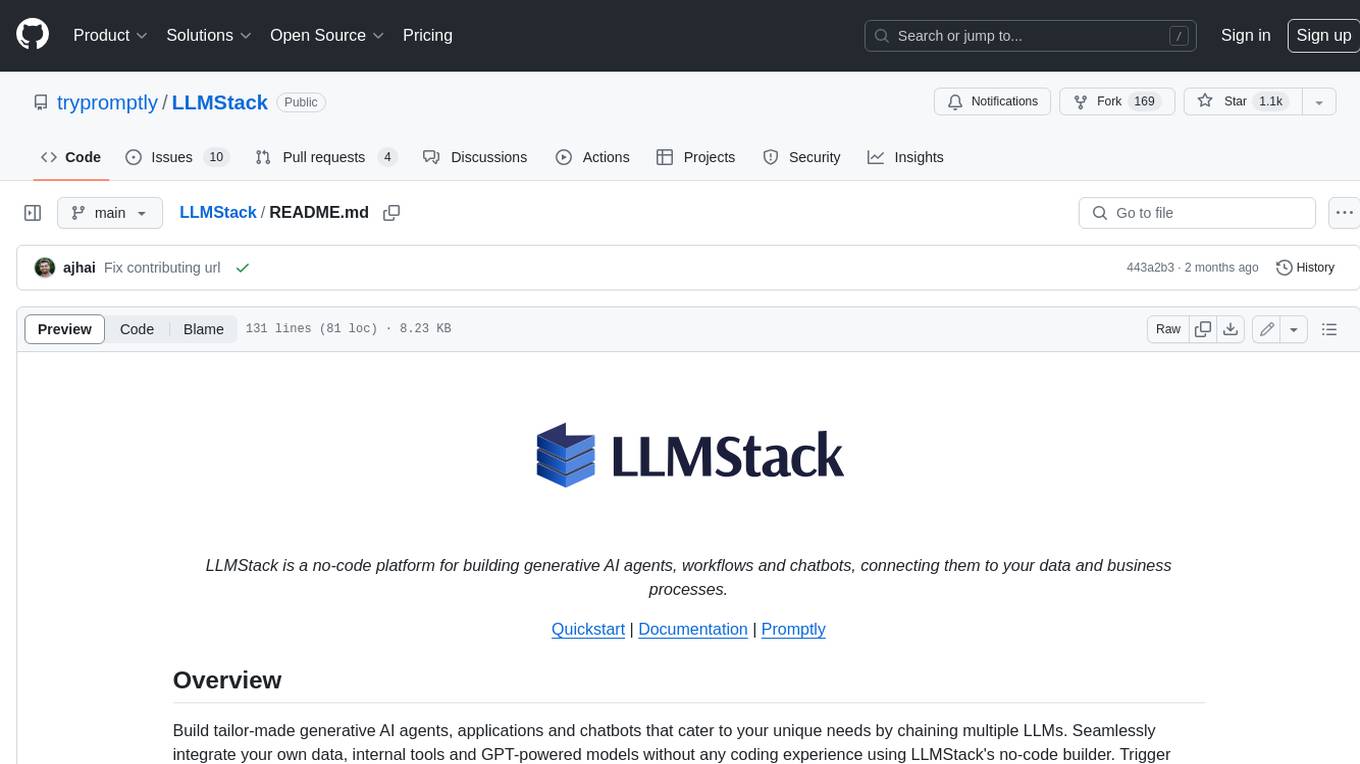
LLMStack
LLMStack is a no-code platform for building generative AI agents, workflows, and chatbots. It allows users to connect their own data, internal tools, and GPT-powered models without any coding experience. LLMStack can be deployed to the cloud or on-premise and can be accessed via HTTP API or triggered from Slack or Discord.
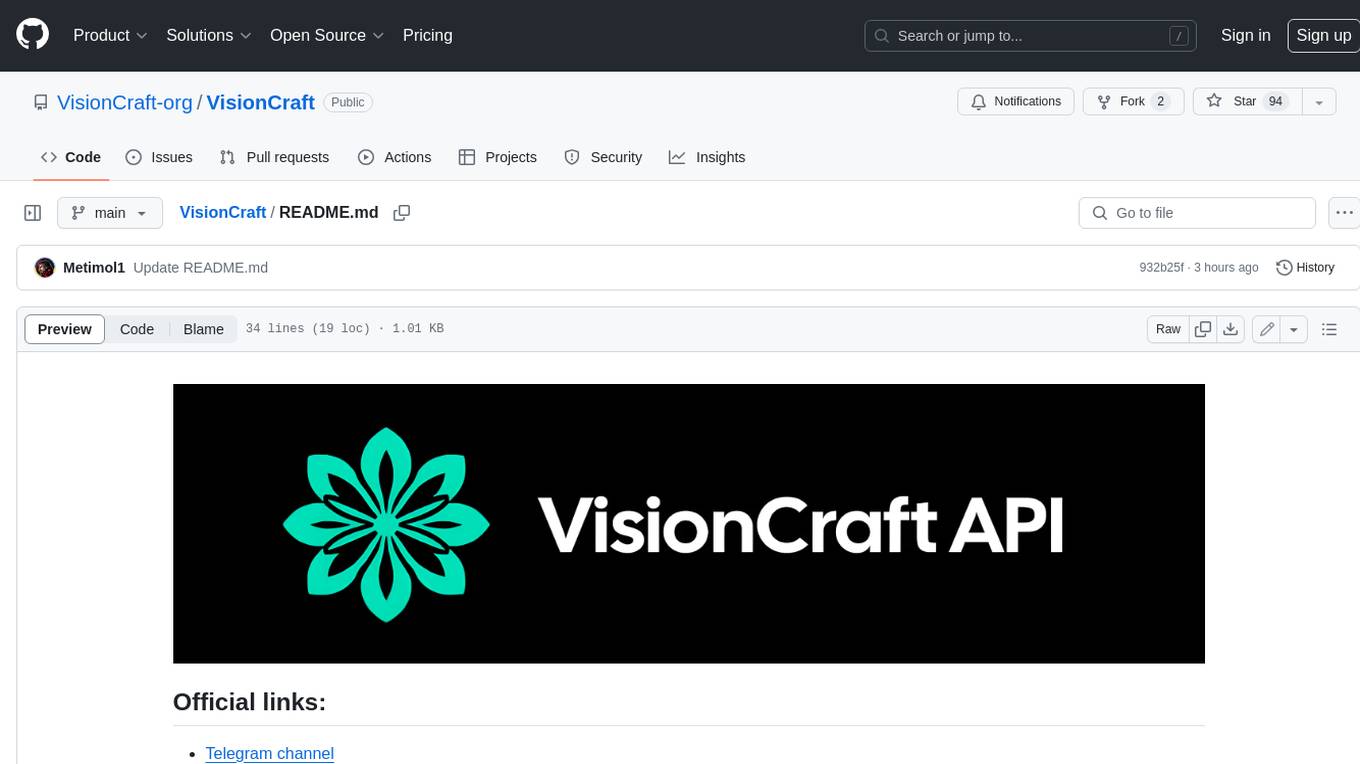
VisionCraft
The VisionCraft API is a free API for using over 100 different AI models. From images to sound.
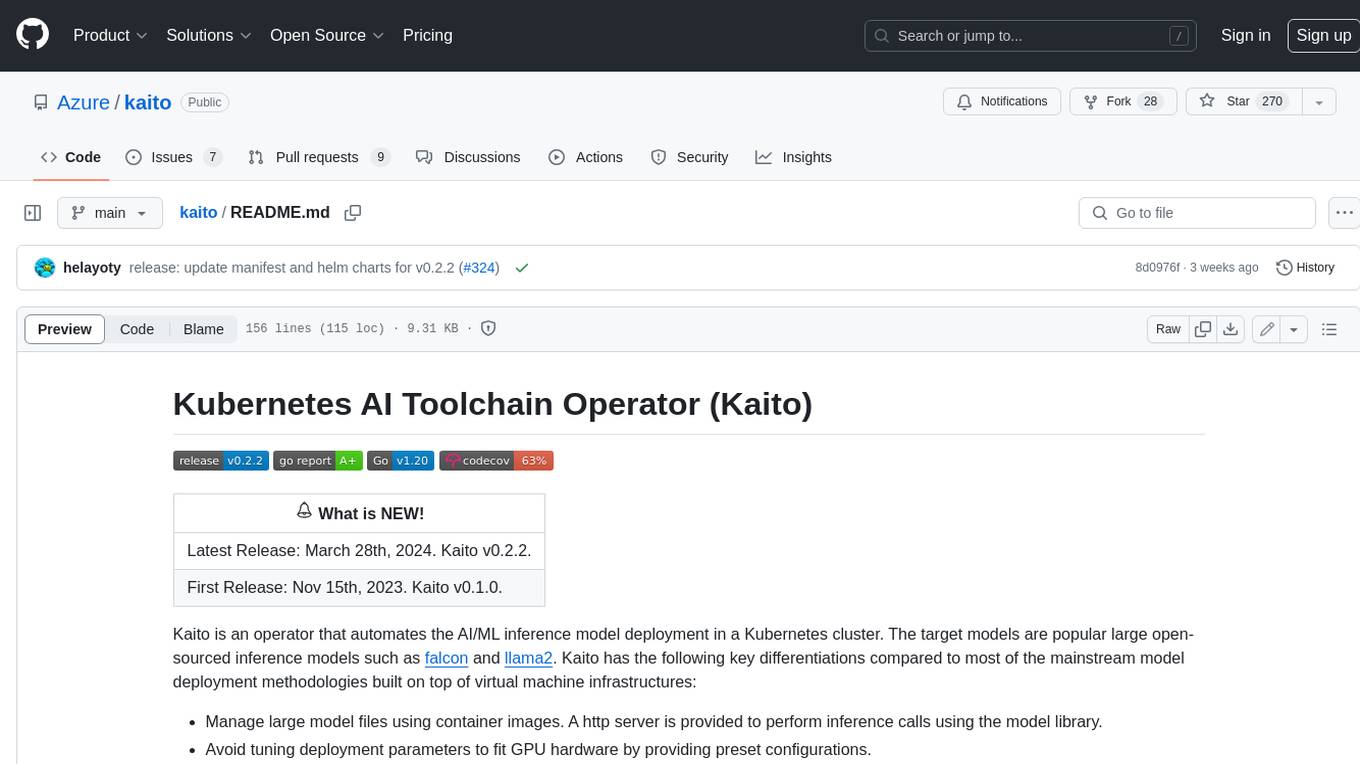
kaito
Kaito is an operator that automates the AI/ML inference model deployment in a Kubernetes cluster. It manages large model files using container images, avoids tuning deployment parameters to fit GPU hardware by providing preset configurations, auto-provisions GPU nodes based on model requirements, and hosts large model images in the public Microsoft Container Registry (MCR) if the license allows. Using Kaito, the workflow of onboarding large AI inference models in Kubernetes is largely simplified.
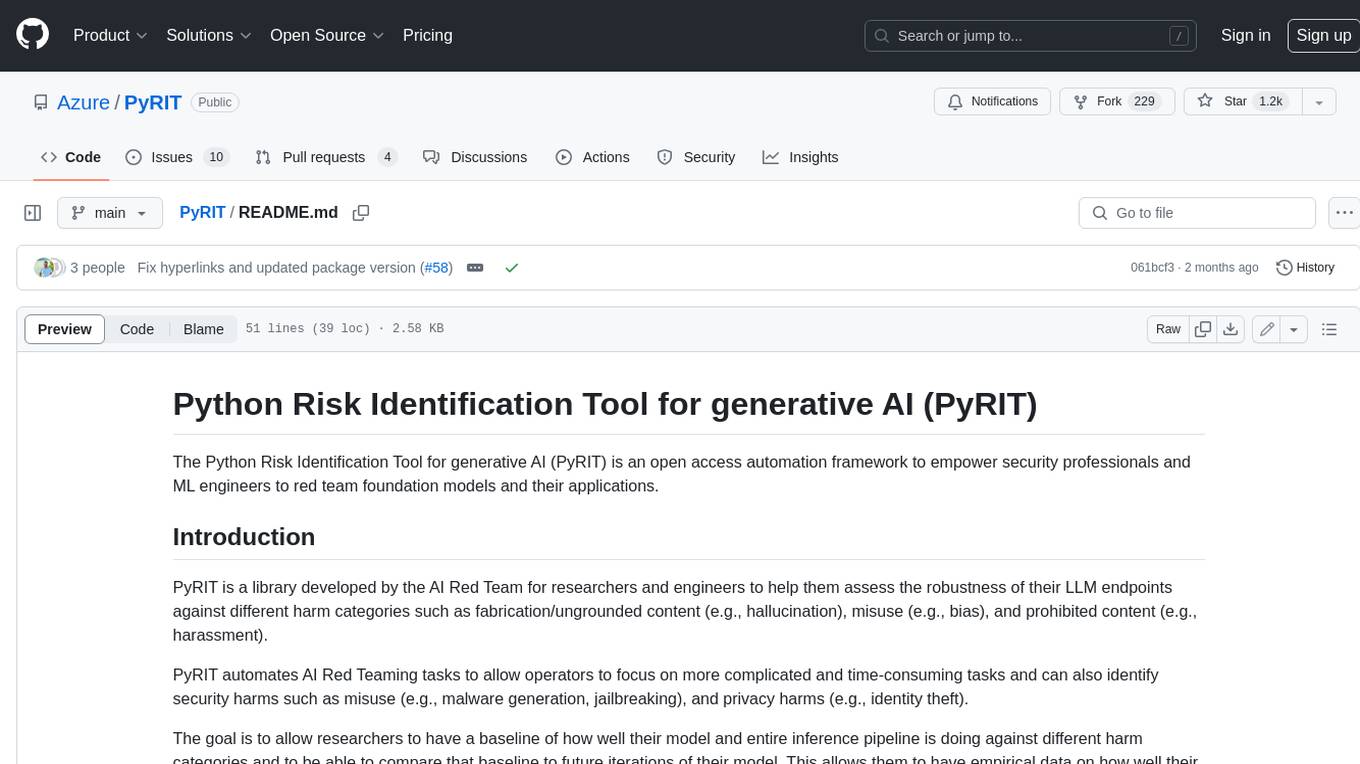
PyRIT
PyRIT is an open access automation framework designed to empower security professionals and ML engineers to red team foundation models and their applications. It automates AI Red Teaming tasks to allow operators to focus on more complicated and time-consuming tasks and can also identify security harms such as misuse (e.g., malware generation, jailbreaking), and privacy harms (e.g., identity theft). The goal is to allow researchers to have a baseline of how well their model and entire inference pipeline is doing against different harm categories and to be able to compare that baseline to future iterations of their model. This allows them to have empirical data on how well their model is doing today, and detect any degradation of performance based on future improvements.
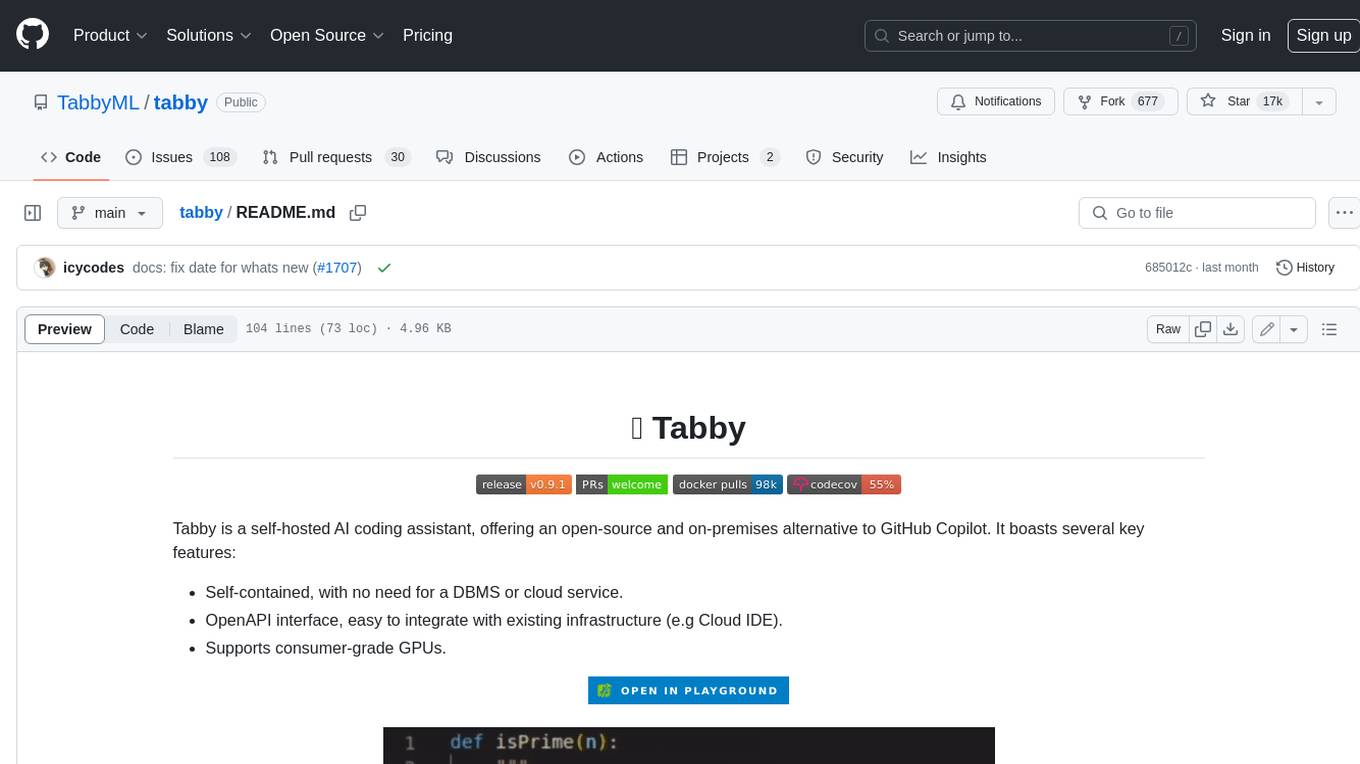
tabby
Tabby is a self-hosted AI coding assistant, offering an open-source and on-premises alternative to GitHub Copilot. It boasts several key features: * Self-contained, with no need for a DBMS or cloud service. * OpenAPI interface, easy to integrate with existing infrastructure (e.g Cloud IDE). * Supports consumer-grade GPUs.
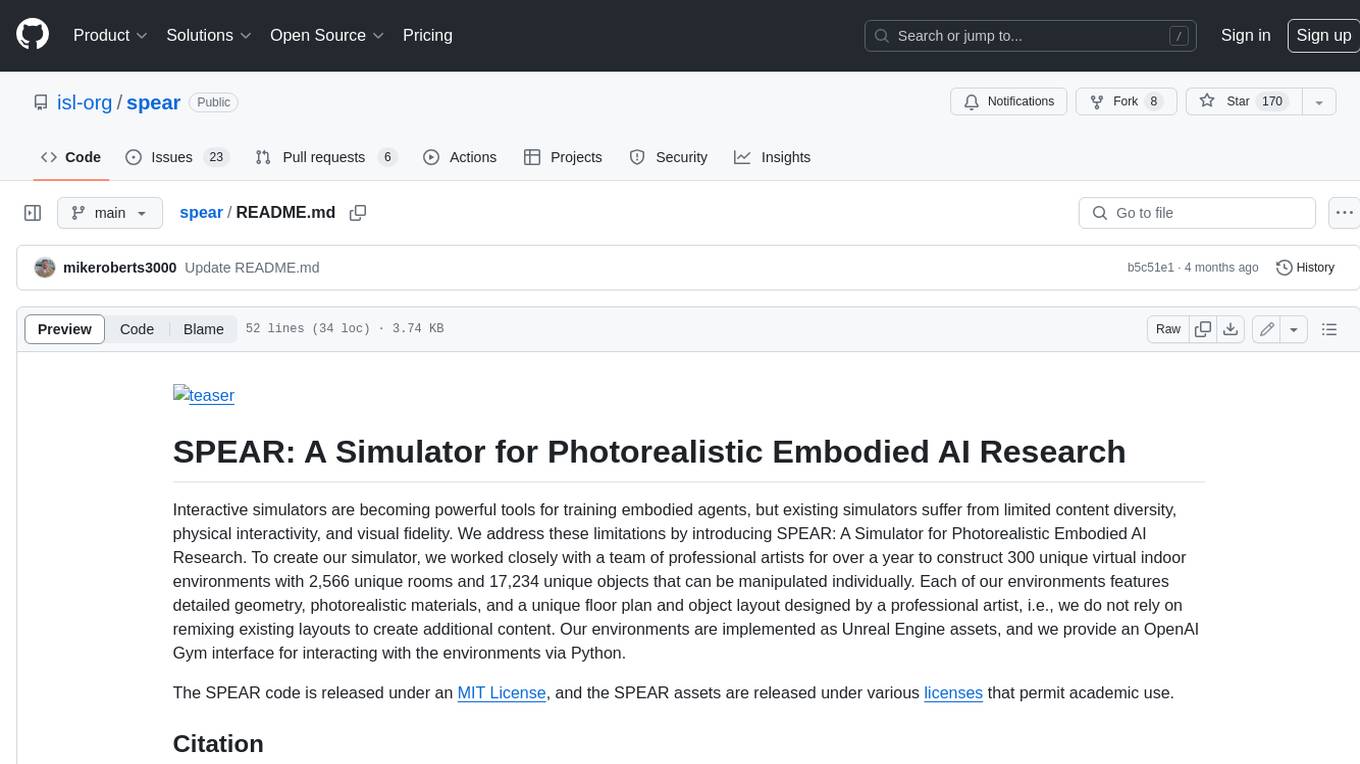
spear
SPEAR (Simulator for Photorealistic Embodied AI Research) is a powerful tool for training embodied agents. It features 300 unique virtual indoor environments with 2,566 unique rooms and 17,234 unique objects that can be manipulated individually. Each environment is designed by a professional artist and features detailed geometry, photorealistic materials, and a unique floor plan and object layout. SPEAR is implemented as Unreal Engine assets and provides an OpenAI Gym interface for interacting with the environments via Python.
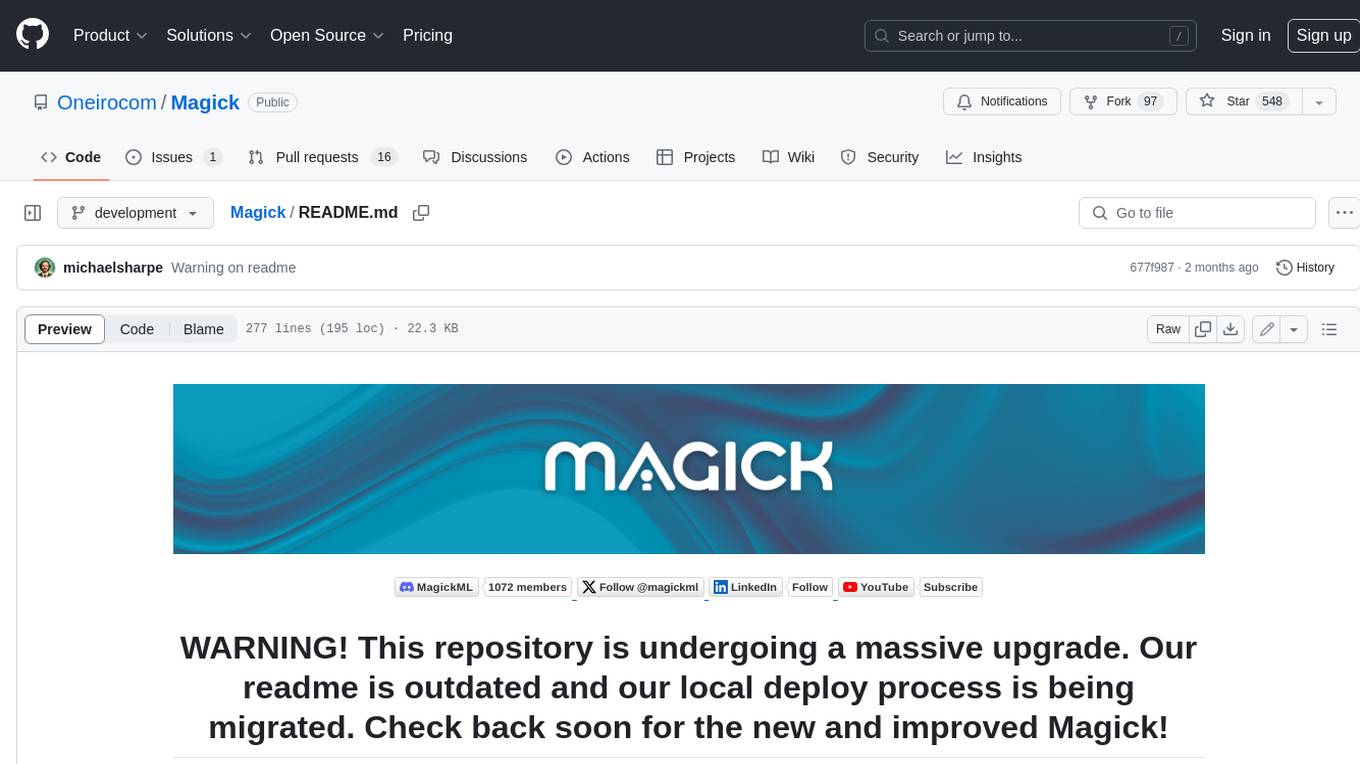
Magick
Magick is a groundbreaking visual AIDE (Artificial Intelligence Development Environment) for no-code data pipelines and multimodal agents. Magick can connect to other services and comes with nodes and templates well-suited for intelligent agents, chatbots, complex reasoning systems and realistic characters.