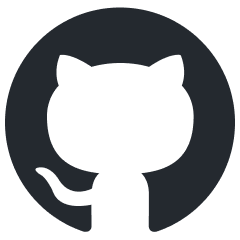
MiniAgents
An async-first framework for building multi-agent AI systems with an innovative approach to parallelism, so you can focus on creating intelligent agents, not on managing the concurrency of your flows.
Stars: 93
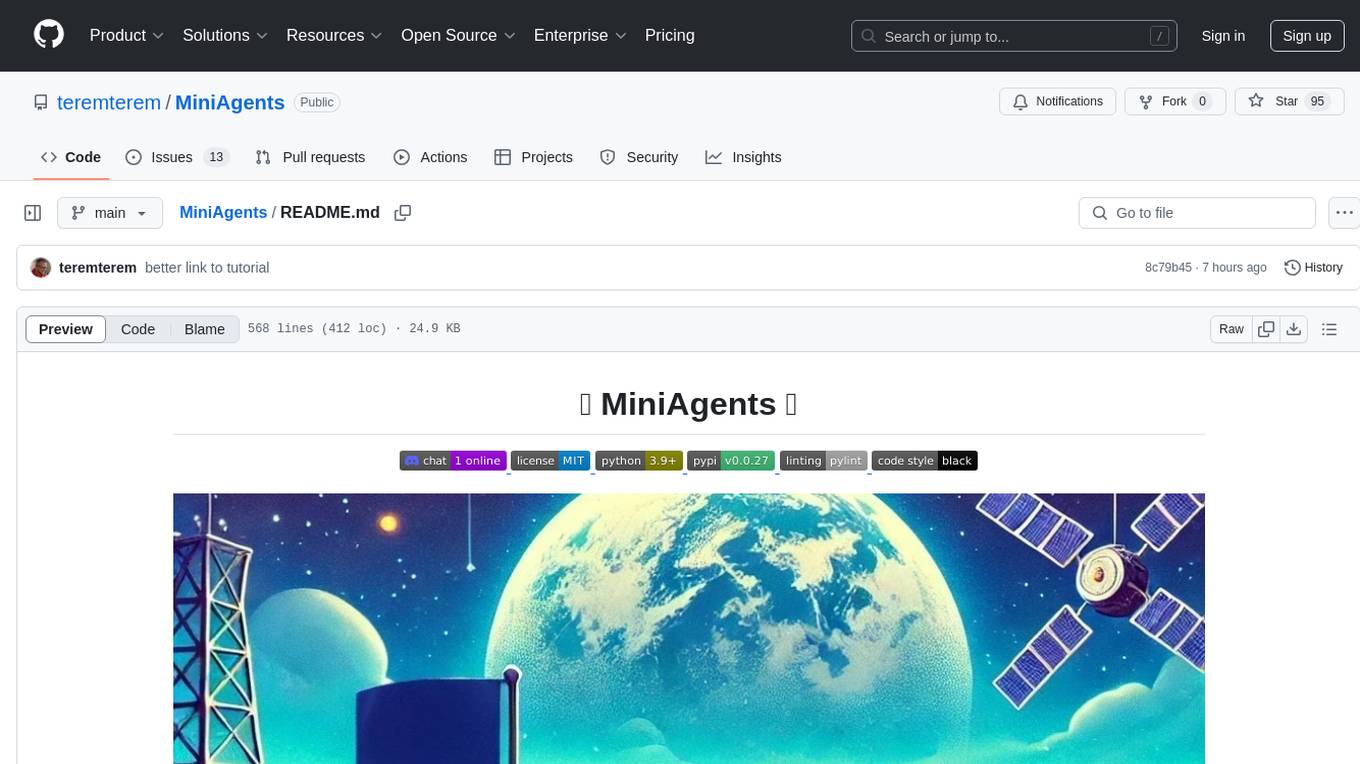
README:
MiniAgents is an open-source Python framework that takes the complexity out of building multi-agent AI systems. With its innovative approach to parallelism and async-first design, you can focus on creating intelligent agents in an easy to follow procedural fashion while the framework handles the concurrency challenges for you.
Built on top of asyncio, MiniAgents provides a robust foundation for LLM-based applications with immutable, Pydantic-based messages and seamless asynchronous token and message streaming between agents.
pip install -U miniagents
We STRONGLY RECOMMEND checking this tutorial before you proceed with the README:
The above step-by-step tutorial teaches you how to build a practical multi-agent web research system that can break down complex questions, run parallel searches, and synthesize comprehensive answers.
Following that tutorial first will make the rest of this README easier to understand!
Here's a simple example of how to define an agent:
from miniagents import miniagent, InteractionContext, MiniAgents
@miniagent
async def my_agent(ctx: InteractionContext) -> None:
async for msg_promise in ctx.message_promises:
ctx.reply(f"You said: {await msg_promise}")
async def main() -> None:
async for msg_promise in my_agent.trigger(["Hello", "World"]):
print(await msg_promise)
if __name__ == "__main__":
MiniAgents().run(main())
This script will print the following lines to the console:
You said: Hello
You said: World
Despite agents running in completely detached asyncio tasks, MiniAgents ensures proper exception propagation from callee agents to caller agents. When an exception occurs in a callee agent, it's captured and propagated through the promises of response message sequences. These exceptions are re-raised when those sequences are iterated over or awaited in any of the caller agents, ensuring that errors are not silently swallowed and can be properly handled.
Here's a simple example showing exception propagation:
from miniagents import miniagent, InteractionContext, MiniAgents
@miniagent
async def faulty_agent(ctx: InteractionContext) -> None:
# This agent will raise an exception
raise ValueError("Something went wrong in callee agent")
@miniagent
async def caller_agent(ctx: InteractionContext) -> None:
# The exception from faulty_agent WILL NOT propagate here
faulty_response_promises = faulty_agent.trigger("Hello")
try:
# The exception from faulty_agent WILL propagate here
async for msg_promise in faulty_response_promises:
await msg_promise
except ValueError as e:
ctx.reply(f"Exception while iterating over response: {e}")
async def main() -> None:
async for msg_promise in caller_agent.trigger("Start"):
print(await msg_promise)
if __name__ == "__main__":
MiniAgents().run(main())
Output:
Exception while iterating over response: Something went wrong in callee agent
MiniAgents provides built-in support for OpenAI and Anthropic language models with possibility to add other integrations.
pip install -U openai
and set your OpenAI API key in the OPENAI_API_KEY
environment variable before running the example below.
from miniagents import MiniAgents
from miniagents.ext.llms import OpenAIAgent
# NOTE: "Forking" an agent is a convenient way of creating a new agent instance
# with the specified configuration. Alternatively, you could pass the `model`
# parameter to `OpenAIAgent.trigger()` directly everytime you talk to the
# agent.
gpt_4o_agent = OpenAIAgent.fork(model="gpt-4o-mini")
async def main() -> None:
reply_sequence = gpt_4o_agent.trigger(
"Hello, how are you?",
system="You are a helpful assistant.",
max_tokens=50,
temperature=0.7,
)
async for msg_promise in reply_sequence:
async for token in msg_promise:
print(token, end="", flush=True)
# MINOR: Let's separate messages with a double newline (even though in
# this particular case we are actually going to receive only one
# message).
print("\n")
if __name__ == "__main__":
MiniAgents().run(main())
Even though OpenAI models return a single assistant response, the OpenAIAgent.trigger()
method is still designed to return a sequence of multiple message promises. This generalizes to arbitrary agents, making agents in the MiniAgents framework easily interchangeable (agents in this framework support sending and receiving zero or more messages).
You can read agent responses token-by-token as shown above regardless of whether the agent is streaming token by token or returning full messages. The complete message content will just be returned as a single "token" in the latter case.
The dialog_loop
agent is a pre-packaged agent that implements a dialog loop between a user agent and an assistant agent. Here is how you can use it to set up an interaction between a user and your agent (can be bare LLM agent, like OpenAIAgent
or AnthropicAgent
, can also be a custom agent that you define yourself - a more complex agent that uses LLM agents under the hood but also introduces more complex behavior, i.e. Retrieval Augmented Generation etc.):
pip install -U openai
and set your OpenAI API key in the OPENAI_API_KEY
environment variable before running the example below.
from miniagents import MiniAgents
from miniagents.ext import (
dialog_loop,
console_user_agent,
MarkdownHistoryAgent,
)
from miniagents.ext.llms import SystemMessage, OpenAIAgent
async def main() -> None:
dialog_loop.trigger(
SystemMessage(
"Your job is to improve the styling and grammar of the sentences "
"that the user throws at you. Leave the sentences unchanged if "
"they seem fine."
),
user_agent=console_user_agent.fork(
# Write chat history to a markdown file (`CHAT.md` in the current
# working directory by default, fork `MarkdownHistoryAgent` if
# you want to customize the filepath to write to).
history_agent=MarkdownHistoryAgent
),
assistant_agent=OpenAIAgent.fork(
model="gpt-4o-mini",
max_tokens=1000,
),
)
if __name__ == "__main__":
MiniAgents(
# Log LLM prompts and responses to `llm_logs/` folder in the current
# working directory. These logs will have a form of time-stamped
# markdown files - single file per single prompt-response pair.
llm_logger_agent=True
).run(main())
Here is what the interaction might look like if you run this script:
YOU ARE NOW IN A CHAT WITH AN AI ASSISTANT
Press Enter to send your message.
Press Ctrl+Space to insert a newline.
Press Ctrl+C (or type "exit") to quit the conversation.
USER: hi
OPENAI_AGENT: Hello! The greeting "hi" is casual and perfectly acceptable.
It's grammatically correct and doesn't require any changes. If you wanted
to use a more formal greeting, you could consider "Hello," "Good morning/afternoon/evening,"
or "Greetings."
USER: got it, thanks!
OPENAI_AGENT: You're welcome! "Got it, thanks!" is a concise and clear expression
of understanding and gratitude. It's perfectly fine for casual communication.
A slightly more formal alternative could be "I understand. Thank you!"
Here is how you can implement a dialog loop between an agent and a user from ground up yourself (for simplicity there is no history agent in this example - check out in_memory_history_agent
and how it is used if you want to know how to implement your own history agent too):
from miniagents import miniagent, InteractionContext, MiniAgents
from miniagents.ext import agent_loop, AWAIT
@miniagent
async def user_agent(ctx: InteractionContext) -> None:
async for msg_promise in ctx.message_promises:
print("ASSISTANT: ", end="", flush=True)
async for token in msg_promise:
print(token, end="", flush=True)
print()
ctx.reply(input("USER: "))
@miniagent
async def assistant_agent(ctx: InteractionContext) -> None:
# Turn a sequence of message promises into a single message promise (if
# there had been multiple messages in the sequence they would have had
# been separated by double newlines - this is how `as_single_promise()`
# works by default).
aggregated_message = await ctx.message_promises.as_single_promise()
ctx.reply(f'You said "{aggregated_message}"')
async def main() -> None:
agent_loop.trigger(agents=[user_agent, AWAIT, assistant_agent])
if __name__ == "__main__":
MiniAgents().run(main())
Output:
USER: hi
ASSISTANT: You said "hi"
USER: nice!
ASSISTANT: You said "nice!"
USER: bye
ASSISTANT: You said "bye"
Remember, with this framework the agents pass promises of message sequences between each other, not the already-resolved message sequences themselves! Even when you pass concrete messages in your code, they are still wrapped into promises of message sequences behind the scenes (while your async agent code is just sent to be processed in the background).
For this reason, the presence of AWAIT
sentinel in the agent chain in the example above is important. Without it the agent_loop
would have kept scheduling more and more interactions between the agents of the chain without ever taking a break to catch up with their processing.
AWAIT
forces the agent_loop
to stop and await
for the complete sequence of replies from the agent right before AWAIT
prior to scheduling (triggering) the execution of the agent right after AWAIT
, thus allowing it to catch up with the asynchronous processing of agents and their responses in the background.
-
miniagents.ext.llms
-
OpenAIAgent
: Connects to OpenAI models like GPT-4o, GPT-4o-mini, etc. Supports all OpenAI API parameters and handles token streaming seamlessly. -
AnthropicAgent
: Similar to OpenAIAgent but for Anthropic's Claude models.
-
-
miniagents.ext
-
console_input_agent
: Prompts the user for input via the console with support for multi-line input. -
console_output_agent
: Echoes messages to the console token by token, which is useful when the response is streamed from an LLM (if response messages are delivered all at once instead, this agent will also just print them all at once). -
file_output_agent
: Writes messages to a specified file, useful for saving responses from other agents. -
user_agent
: A user agent that echoes messages from the agent that called it, then reads the user input and returns the user input as its response. This agent is an aggregation of theconsole_output_agent
andconsole_input_agent
(these two agents can be substituted with other agents of similar functionality, however). -
agent_loop
: Creates an infinite loop of interactions between the specified agents. It's designed for ongoing conversations or continuous processing, particularly useful for chat interfaces where agents need to take turns indefinitely (or unless stopped withKeyboardInterrupt
). -
dialog_loop
: A special case ofagent_loop
designed for conversation between a user and an assistant, with optional chat history tracking. -
agent_chain
: Executes a sequence of agents in order, where each agent processes the output of the previous agent. This creates a pipeline of processing steps, with messages flowing from one agent to the next in a specified sequence. -
in_memory_history_agent
: Keeps track of conversation history in memory, enabling context-aware interactions without external storage. -
MarkdownHistoryAgent
: Keeps track of conversation history in a markdown file, allowing to resume a conversation from the same point even if the app is restarted. -
markdown_llm_logger_agent
: Logs LLM interactions (prompts and responses) in markdown format, useful for debugging and auditing purposes (look forllm_logger_agent=True
of theMiniAgents()
context manager in one of the code examples above).
-
Feel free to explore the source code in the miniagents.ext
package to see how various agents are implemented and get inspiration for building your own!
Let's consider an example that consists of two dummy agents and an aggregator agent that aggregates the responses from the two dummy agents (and also adds some messages of its own):
import asyncio
from miniagents.miniagents import (
MiniAgents,
miniagent,
InteractionContext,
Message,
)
@miniagent
async def agent1(ctx: InteractionContext) -> None:
print("Agent 1 started")
ctx.reply("*** MESSAGE #1 from Agent 1 ***")
print("Agent 1 still working")
ctx.reply("*** MESSAGE #2 from Agent 1 ***")
print("Agent 1 finished")
@miniagent
async def agent2(ctx: InteractionContext) -> None:
print("Agent 2 started")
ctx.reply("*** MESSAGE from Agent 2 ***")
print("Agent 2 finished")
@miniagent
async def aggregator_agent(ctx: InteractionContext) -> None:
print("Aggregator started")
ctx.reply(
[
"*** AGGREGATOR MESSAGE #1 ***",
agent1.trigger(),
agent2.trigger(),
]
)
print("Aggregator still working")
ctx.reply("*** AGGREGATOR MESSAGE #2 ***")
print("Aggregator finished")
async def main() -> None:
print("TRIGGERING AGGREGATOR")
msg_promises = aggregator_agent.trigger()
print("TRIGGERING AGGREGATOR DONE\n")
print("SLEEPING FOR ONE SECOND")
# This is when the agents will actually start processing (in fact, any
# other kind of task switch would have had the same effect).
await asyncio.sleep(1)
print("SLEEPING DONE\n")
print("PREPARING TO GET MESSAGES FROM AGGREGATOR")
async for msg_promise in msg_promises:
# MessagePromises always resolve into Message objects (or subclasses),
# even if the agent was replying with bare strings
message: Message = await msg_promise
print(message)
# You can safely `await` again. Concrete messages (and tokens, if there was
# token streaming) are cached inside the promises. Message sequences (as
# well as token sequences) are "replayable".
print("TOTAL NUMBER OF MESSAGES FROM AGGREGATOR:", len(await msg_promises))
if __name__ == "__main__":
MiniAgents().run(main())
This script will print the following lines to the console:
TRIGGERING AGGREGATOR
TRIGGERING AGGREGATOR DONE
SLEEPING FOR ONE SECOND
Aggregator started
Aggregator still working
Aggregator finished
Agent 1 started
Agent 1 still working
Agent 1 finished
Agent 2 started
Agent 2 finished
SLEEPING DONE
PREPARING TO GET MESSAGES FROM AGGREGATOR
*** AGGREGATOR MESSAGE #1 ***
*** MESSAGE #1 from Agent 1 ***
*** MESSAGE #2 from Agent 1 ***
*** MESSAGE from Agent 2 ***
*** AGGREGATOR MESSAGE #2 ***
TOTAL NUMBER OF MESSAGES FROM AGGREGATOR: 5
None of the agent functions start executing upon any of the calls to the trigger()
method. Instead, in all cases the trigger()
method immediately returns with promises to "talk to the agent(s)" (promises of sequences of promises of response messages, to be very precise - see MessageSequencePromise
and MessagePromise
classes for details).
As long as the global start_soon
setting is set to True
(which is the default - see the source code of PromisingContext
, the parent class of MiniAgents
context manager for details), the actual agent functions will start processing at the earliest task switch (the behaviour of asyncio.create_task()
, which is used under the hood). In this example it is going to be await asyncio.sleep(1)
inside the main()
function, but if this sleep()
wasn't there, it would have happened upon the first iteration of the async for
loop which is the next place where a task switch happens.
๐ช EXERCISE FOR READER: Add another await asyncio.sleep(1)
right before print("Aggregator finished")
in the aggregator_agent
function and then try to predict how the output will change. After that, run the modified script and check if your prediction was correct.
start_soon
to False
for individual agent calls if you need to prevent them from starting any earlier than the first time their response sequence promise is awaited for: some_agent.trigger(request_messages_if_any, start_soon=False)
. However, setting it to False
for the whole system globally is not recommended because it can lead to deadlocks.
Here's a simple example demonstrating how to use agent_call = some_agent.initiate_call()
and then do agent_call.send_message()
two times before calling agent_call.reply_sequence()
(instead of all-in-one some_agent.trigger()
):
from miniagents import miniagent, InteractionContext, MiniAgents
@miniagent
async def output_agent(ctx: InteractionContext) -> None:
async for msg_promise in ctx.message_promises:
ctx.reply(f"Echo: {await msg_promise}")
async def main() -> None:
agent_call = output_agent.initiate_call()
agent_call.send_message("Hello")
agent_call.send_message("World")
reply_sequence = agent_call.reply_sequence()
async for msg_promise in reply_sequence:
print(await msg_promise)
if __name__ == "__main__":
MiniAgents().run(main())
This will output:
Echo: Hello
Echo: World
There are three ways to use the MiniAgents()
context:
-
Calling its
run()
method with your main function as a parameter (themain()
function in this example should be defined asasync
):MiniAgents().run(main())
-
Using it as an async context manager:
async with MiniAgents(): ... # your async code that works with agents goes here
-
Directly calling its
activate()
(and, potentially,afinalize()
at the end) methods:mini_agents = MiniAgents() mini_agents.activate() try: ... # your async code that works with agents goes here finally: await mini_agents.afinalize()
The third way might be ideal for web applications and other cases when there is no single function that you can encapsulate with the MiniAgents()
context manager (or it is unclear what such function would be). You just do mini_agents.activate()
somewhere upon the init of the server and forget about it.
from miniagents.ext.llms import UserMessage, SystemMessage, AssistantMessage
user_message = UserMessage("Hello!")
system_message = SystemMessage("System message")
assistant_message = AssistantMessage("Assistant message")
The difference between these message types is in the default values of the role
field of the message:
-
UserMessage
hasrole="user"
by default -
SystemMessage
hasrole="system"
by default -
AssistantMessage
hasrole="assistant"
by default
You can create custom message types by subclassing Message
.
from miniagents.messages import Message
class CustomMessage(Message):
custom_field: str
message = CustomMessage("Hello", custom_field="Custom Value")
print(message.content) # Output: Hello
print(message.custom_field) # Output: Custom Value
For some more examples, check out the examples directory.
There are two main features of MiniAgents the idea of which motivated the creation of this framework:
- It is very easy to throw bare strings, messages, message promises, collections, and sequences of messages and message promises (as well as the promises of the sequences themselves) all together into an agent reply (see
MessageType
). This entire hierarchical structure will be asynchronously resolved in the background into a flat and uniform sequence of message promises (it will be automatically "flattened" in the background). - By default, agents work in so called
start_soon
mode, which is different from the usual way coroutines work where you need to actively await on them or iterate over them (in case of asynchronous generators). Instart_soon
mode, every agent, after it was invoked, actively seeks every opportunity to proceed its processing in the background when async tasks switch.
The second feature combines this start_soon
approach with regular async/await and async generators by using so called streamed promises (see StreamedPromise
and Promise
classes) which were designed to be "replayable".
The design choice for immutable messages was made specifically to enable this kind of highly parallelized agent execution. Since Generative AI applications are inherently IO-bound (with models typically hosted externally), immutable messages eliminate concerns about concurrent state mutations. This approach allows multiple agents to process messages simultaneously without risk of race conditions or data corruption, maximizing throughput in distributed LLM workflows.
MiniAgents provides a way to persist messages as they are resolved from promises using the @MiniAgents().on_persist_message
decorator. This allows you to implement custom logic for storing or logging messages.
Additionally, messages (as well as any other Pydantic models derived from Frozen
) have a hash_key
property. This property calculates the sha256 hash of the content of the message and is used as the id of the Messages
(or any other Frozen
model), much like there are commit hashes in git.
Here's a simple example of how to use the on_persist_message
decorator:
from miniagents import MiniAgents, Message
mini_agents = MiniAgents()
@mini_agents.on_persist_message
async def persist_message(_, message: Message) -> None:
print(f"Persisting message with hash key: {message.hash_key}")
# Here you could save the message to a database or log it to a file
Here are some of the core concepts in the MiniAgents framework:
-
MiniAgent
: A wrapper around an async function (or a whole class withasync def __call__()
method) that defines an agent's behavior. Created using the@miniagent
decorator. -
InteractionContext
: Passed to each agent function, provides access to incoming messages and allows sending replies. -
Message
: Represents a message exchanged between agents. Can contain content, metadata, and nested messages. Immutable once created. -
MessagePromise
: A promise of a message that can be streamed token by token. -
MessageSequencePromise
: A promise of a sequence of message promises. -
Frozen
: An immutable Pydantic model with a git-style hash key calculated from its JSON representation. The base class forMessage
.
More underlying concepts (you will rarely need to use them directly, if at all):
-
StreamedPromise
: A promise that can be resolved piece by piece, allowing for streaming. The base class forMessagePromise
andMessageSequencePromise
. -
Promise
: Represents a value that may not be available yet, but will be resolved in the future. The base class forStreamedPromise
.
Join our Discord community to get help with your projects. We welcome questions, feature suggestions, and contributions!
MiniAgents is released under the MIT License.
Happy coding with MiniAgents! ๐
For Tasks:
Click tags to check more tools for each tasksFor Jobs:
Alternative AI tools for MiniAgents
Similar Open Source Tools
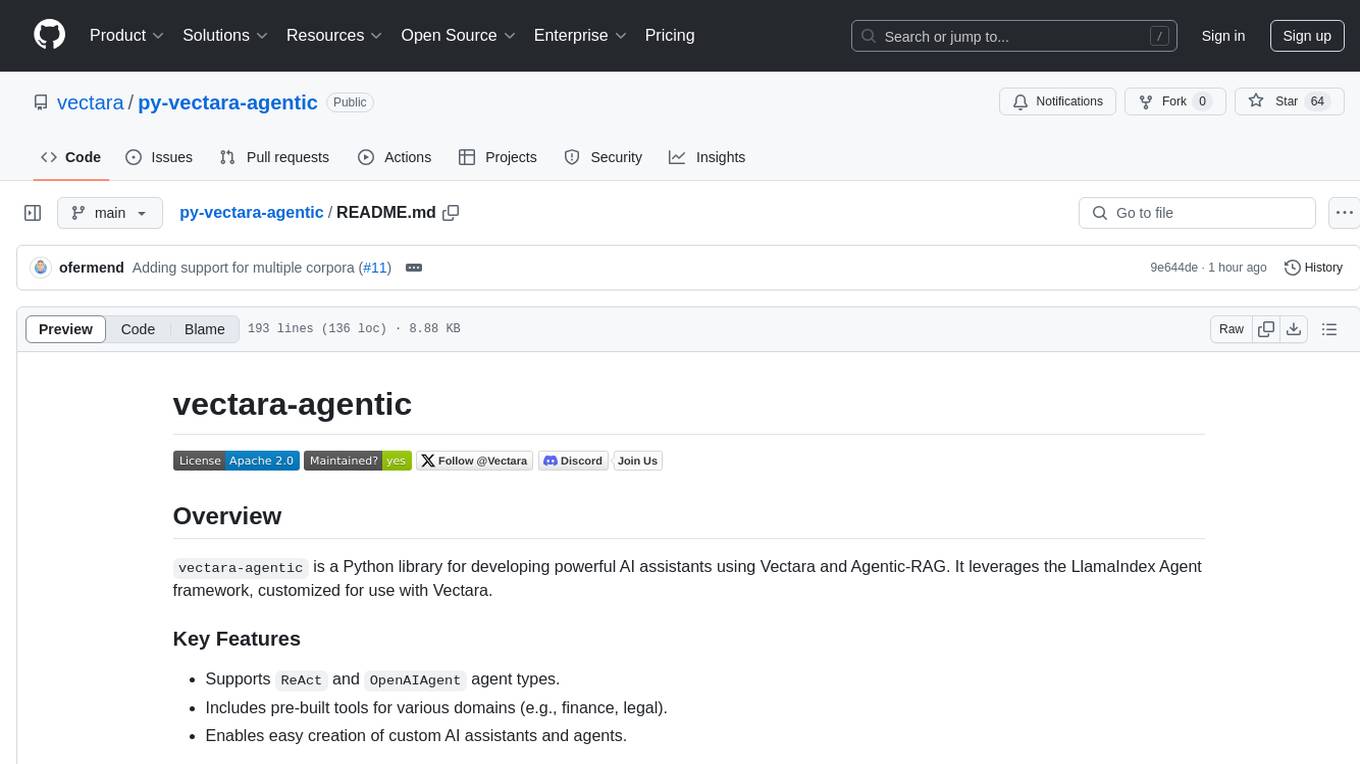
py-vectara-agentic
The `vectara-agentic` Python library is designed for developing powerful AI assistants using Vectara and Agentic-RAG. It supports various agent types, includes pre-built tools for domains like finance and legal, and enables easy creation of custom AI assistants and agents. The library provides tools for summarizing text, rephrasing text, legal tasks like summarizing legal text and critiquing as a judge, financial tasks like analyzing balance sheets and income statements, and database tools for inspecting and querying databases. It also supports observability via LlamaIndex and Arize Phoenix integration.
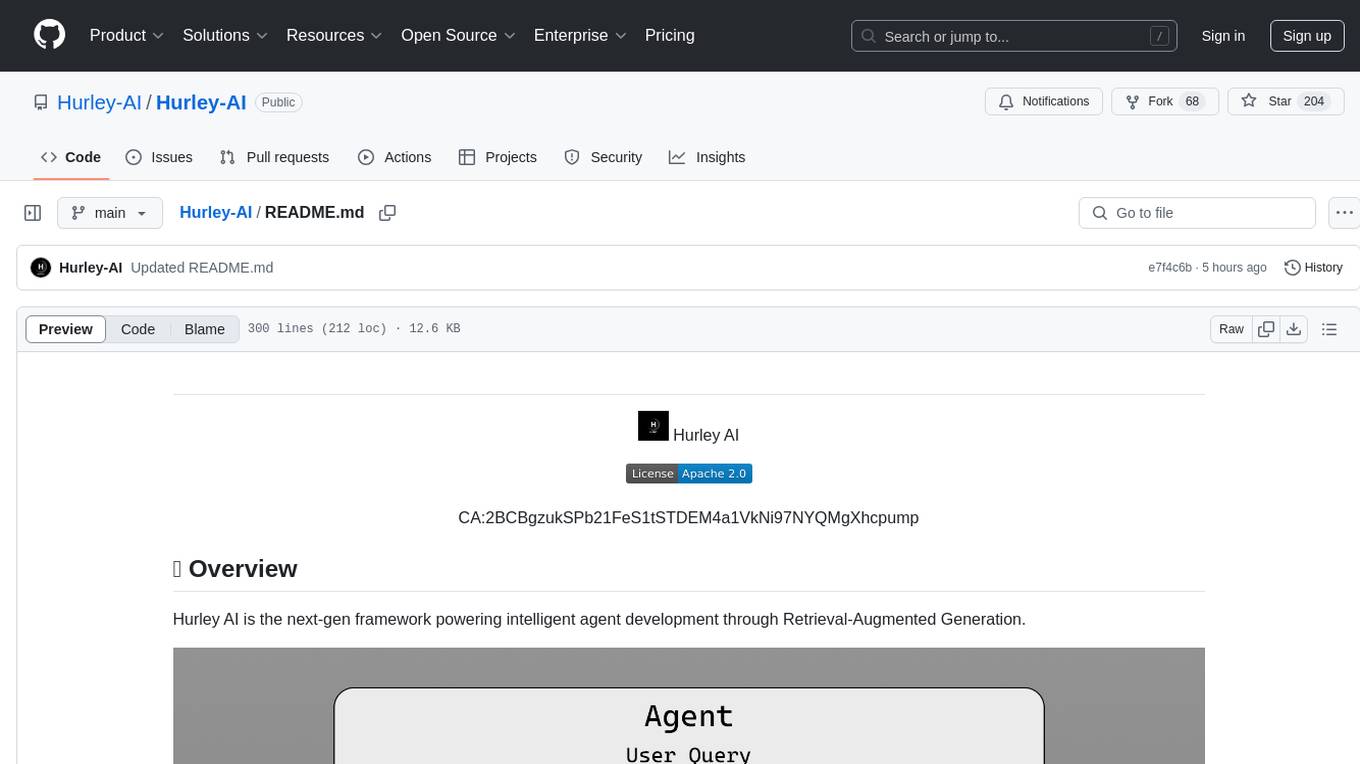
Hurley-AI
Hurley AI is a next-gen framework for developing intelligent agents through Retrieval-Augmented Generation. It enables easy creation of custom AI assistants and agents, supports various agent types, and includes pre-built tools for domains like finance and legal. Hurley AI integrates with LLM inference services and provides observability with Arize Phoenix. Users can create Hurley RAG tools with a single line of code and customize agents with specific instructions. The tool also offers various helper functions to connect with Hurley RAG and search tools, along with pre-built tools for tasks like summarizing text, rephrasing text, understanding memecoins, and querying databases.
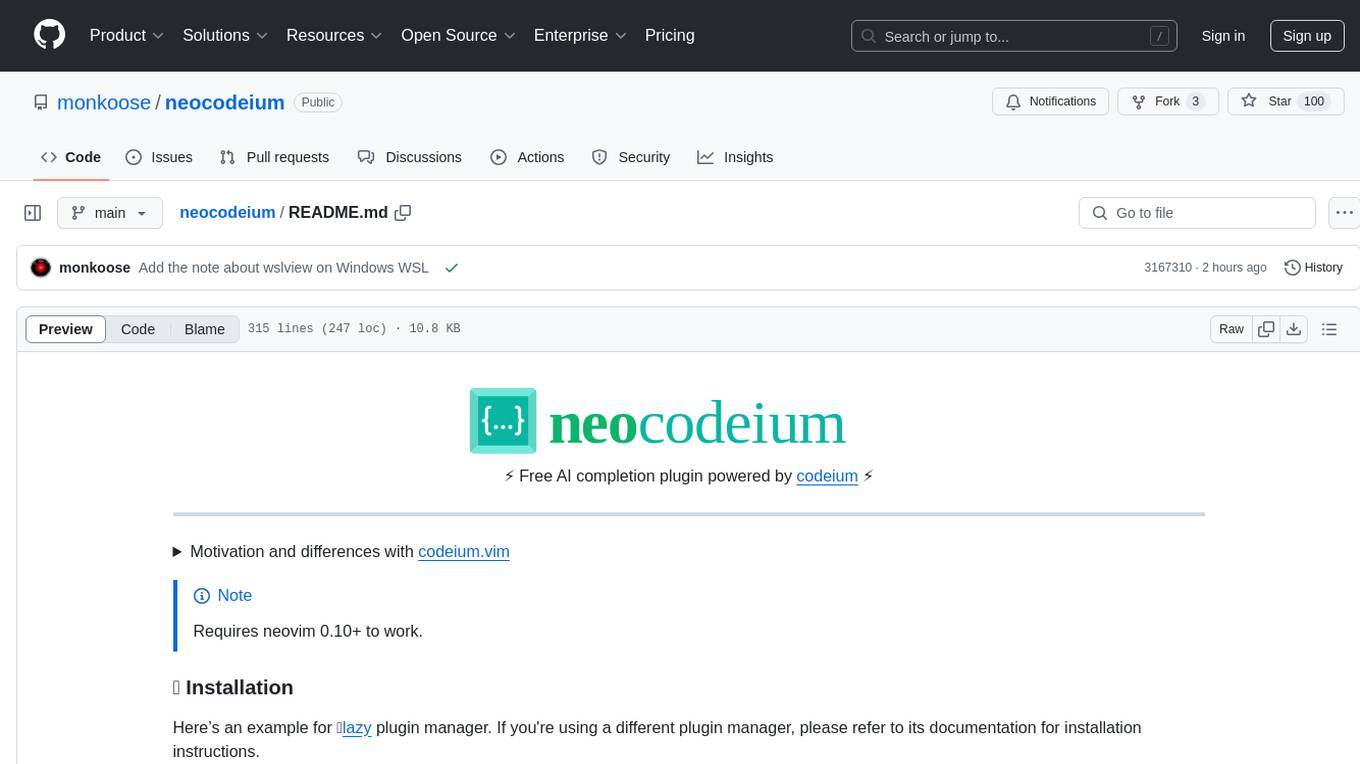
neocodeium
NeoCodeium is a free AI completion plugin powered by Codeium, designed for Neovim users. It aims to provide a smoother experience by eliminating flickering suggestions and allowing for repeatable completions using the `.` key. The plugin offers performance improvements through cache techniques, displays suggestion count labels, and supports Lua scripting. Users can customize keymaps, manage suggestions, and interact with the AI chat feature. NeoCodeium enhances code completion in Neovim, making it a valuable tool for developers seeking efficient coding assistance.
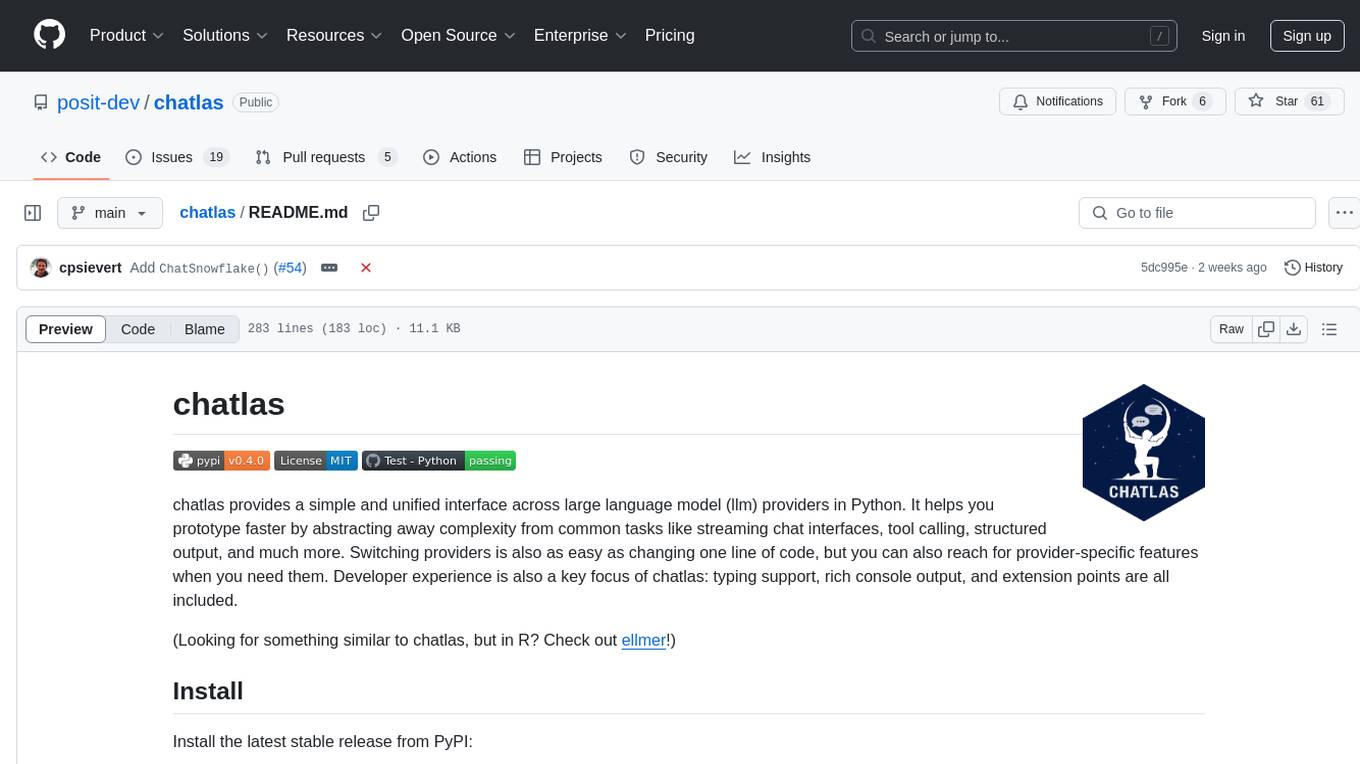
chatlas
Chatlas is a Python tool that provides a simple and unified interface across various large language model providers. It helps users prototype faster by abstracting complexity from tasks like streaming chat interfaces, tool calling, and structured output. Users can easily switch providers by changing one line of code and access provider-specific features when needed. Chatlas focuses on developer experience with typing support, rich console output, and extension points.
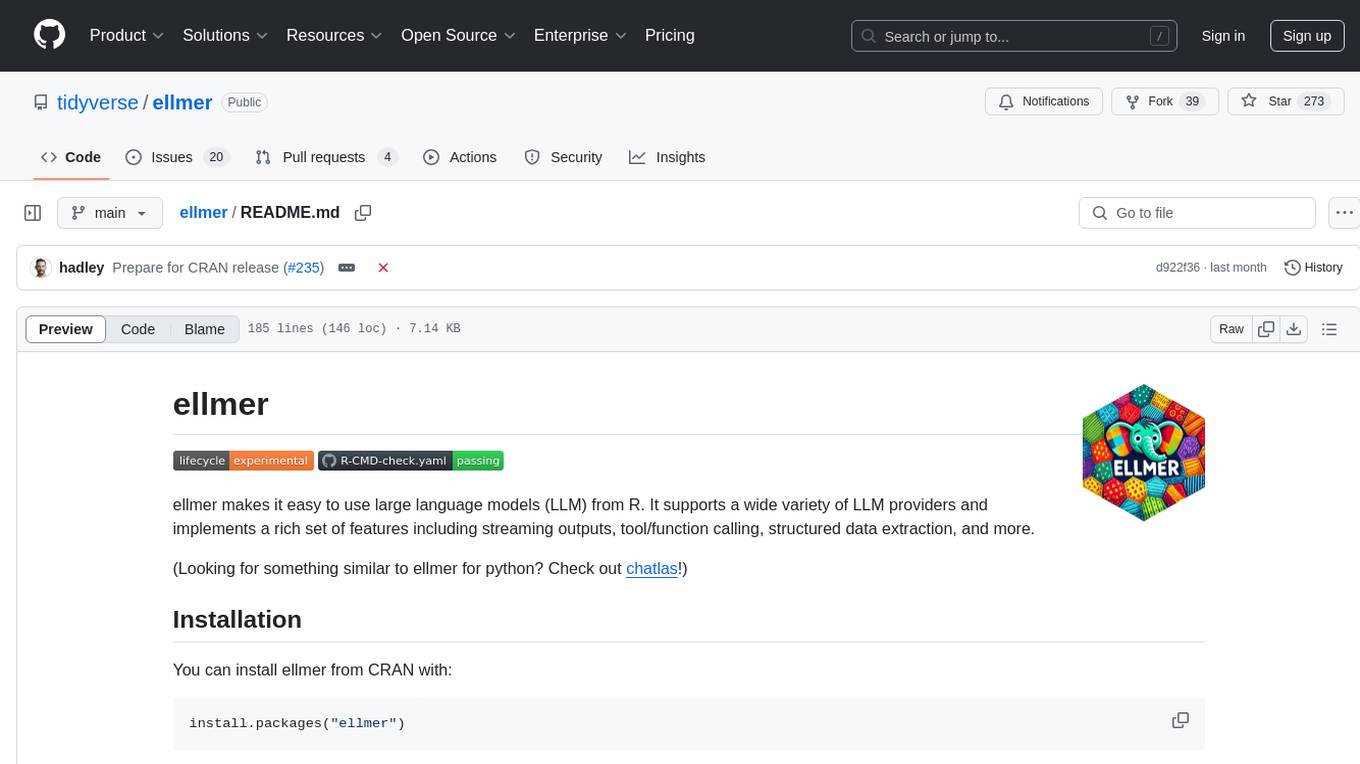
ellmer
ellmer is a tool that facilitates the use of large language models (LLM) from R. It supports various LLM providers and offers features such as streaming outputs, tool/function calling, and structured data extraction. Users can interact with ellmer in different ways, including interactive chat console, interactive method call, and programmatic chat. The tool provides support for multiple model providers and offers recommendations for different use cases, such as exploration or organizational use.
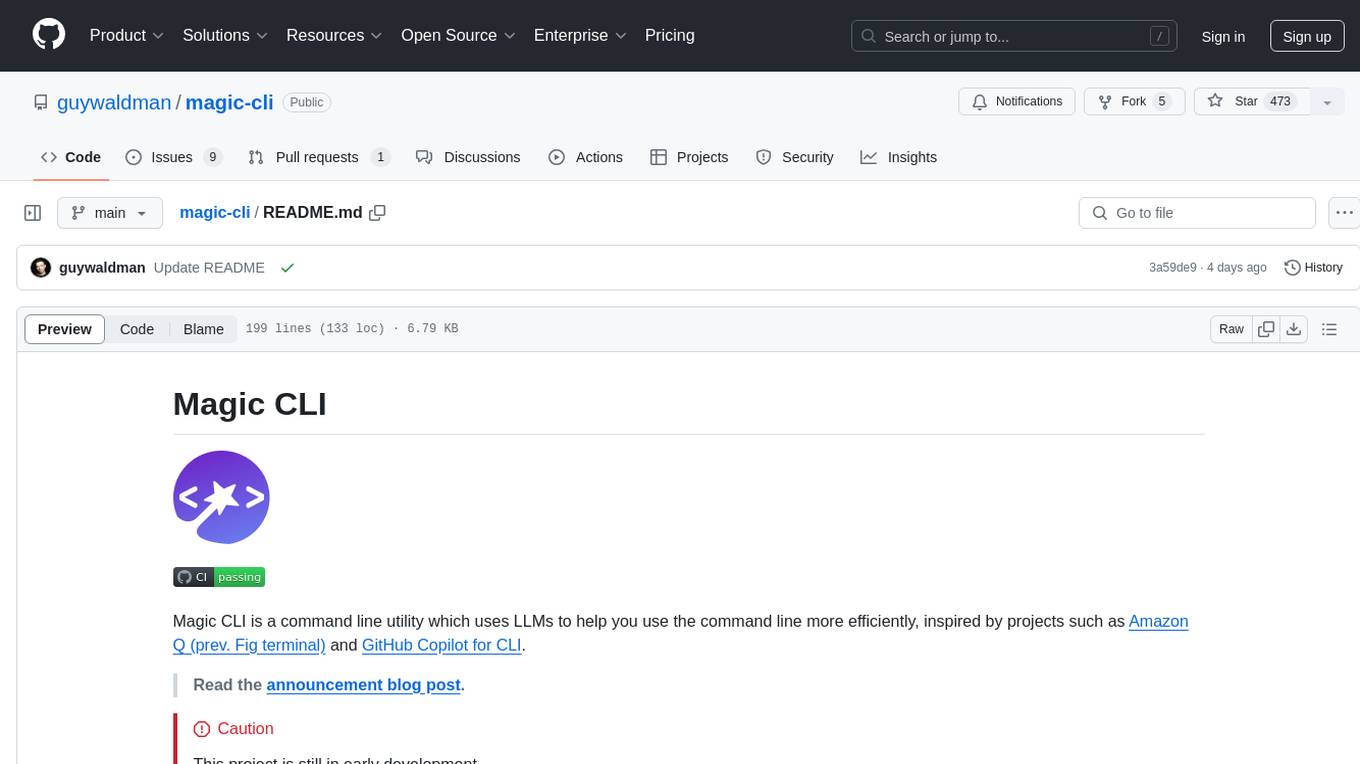
magic-cli
Magic CLI is a command line utility that leverages Large Language Models (LLMs) to enhance command line efficiency. It is inspired by projects like Amazon Q and GitHub Copilot for CLI. The tool allows users to suggest commands, search across command history, and generate commands for specific tasks using local or remote LLM providers. Magic CLI also provides configuration options for LLM selection and response generation. The project is still in early development, so users should expect breaking changes and bugs.
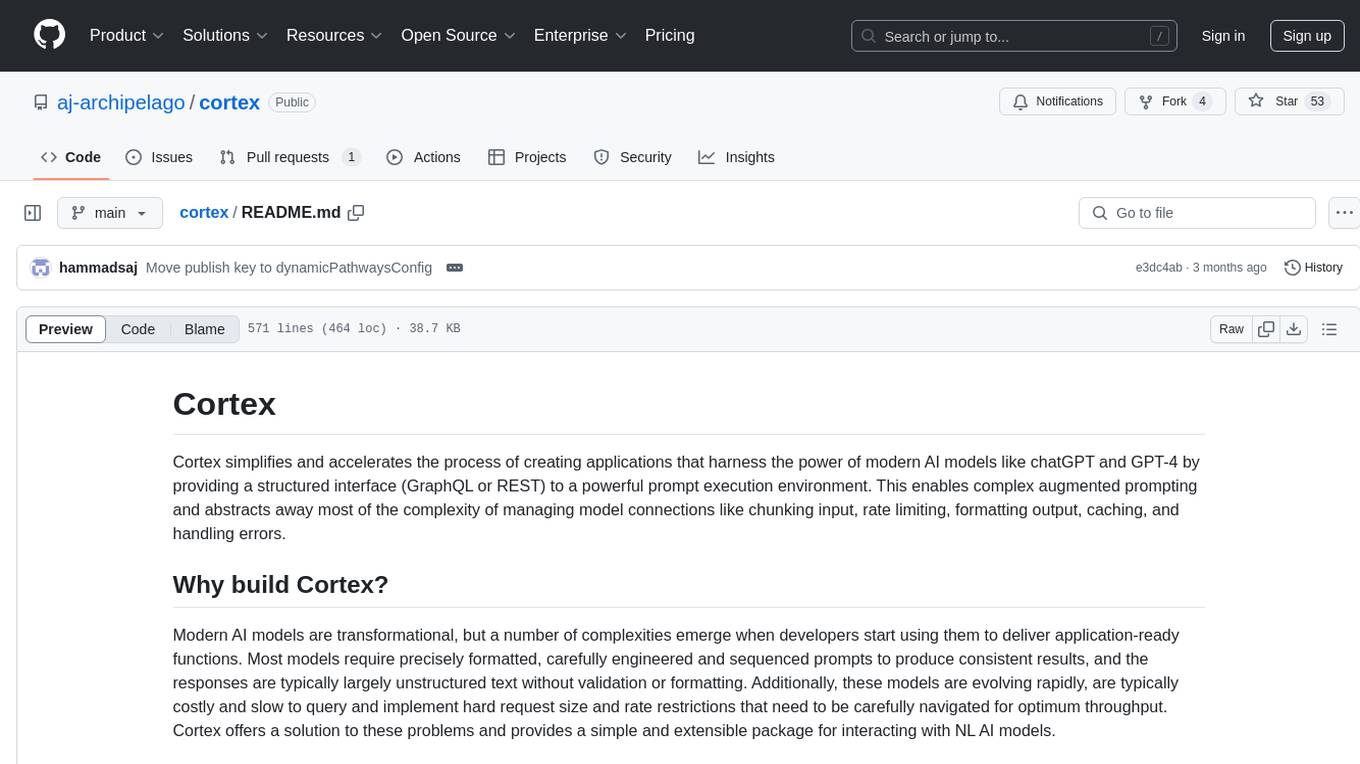
cortex
Cortex is a tool that simplifies and accelerates the process of creating applications utilizing modern AI models like chatGPT and GPT-4. It provides a structured interface (GraphQL or REST) to a prompt execution environment, enabling complex augmented prompting and abstracting away model connection complexities like input chunking, rate limiting, output formatting, caching, and error handling. Cortex offers a solution to challenges faced when using AI models, providing a simple package for interacting with NL AI models.
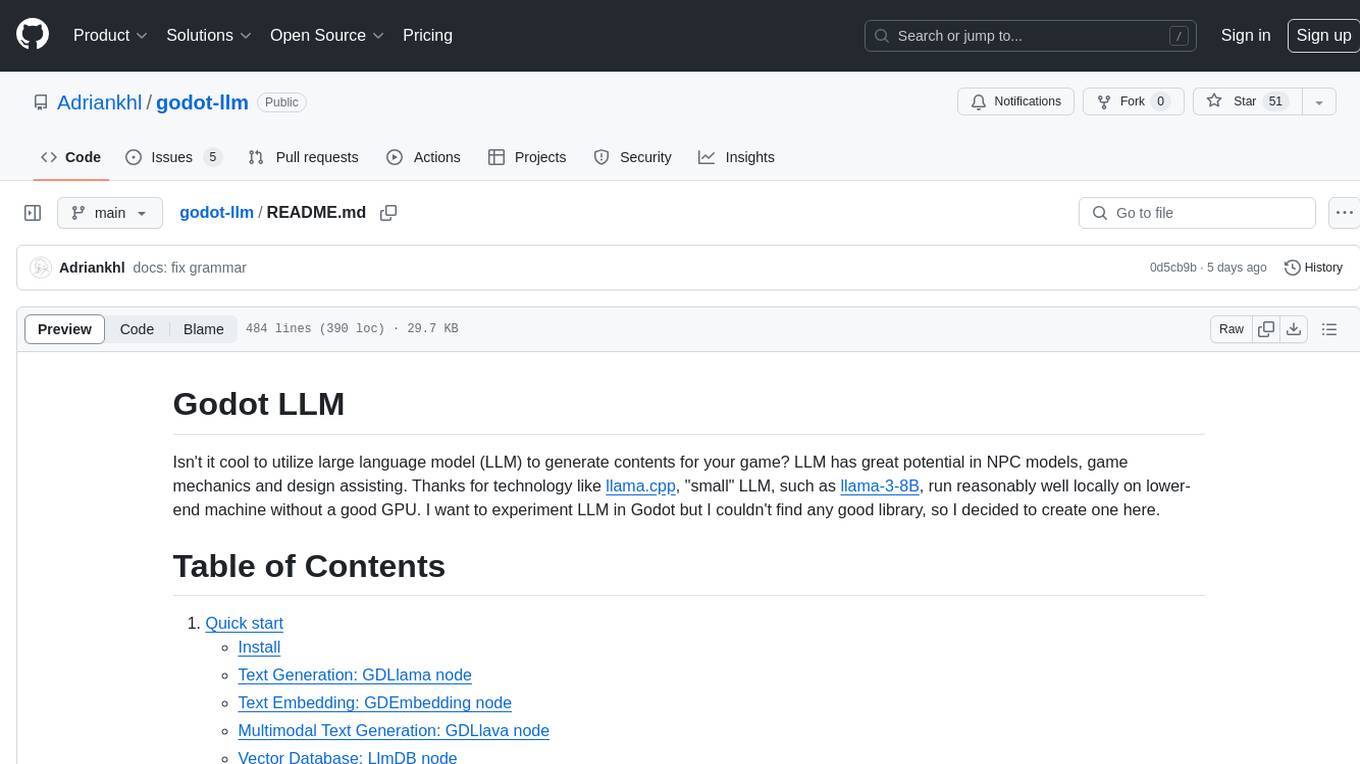
godot-llm
Godot LLM is a plugin that enables the utilization of large language models (LLM) for generating content in games. It provides functionality for text generation, text embedding, multimodal text generation, and vector database management within the Godot game engine. The plugin supports features like Retrieval Augmented Generation (RAG) and integrates llama.cpp-based functionalities for text generation, embedding, and multimodal capabilities. It offers support for various platforms and allows users to experiment with LLM models in their game development projects.
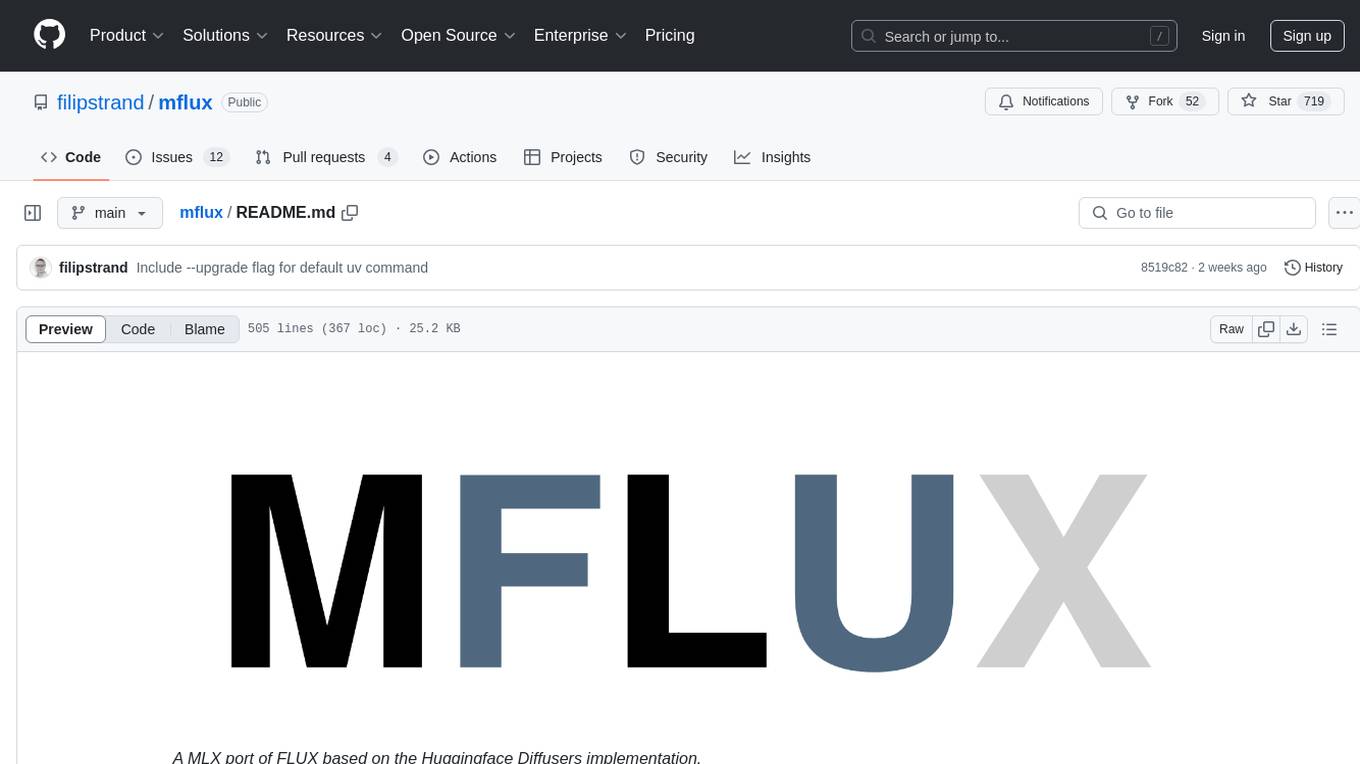
mflux
MFLUX is a line-by-line port of the FLUX implementation in the Huggingface Diffusers library to Apple MLX. It aims to run powerful FLUX models from Black Forest Labs locally on Mac machines. The codebase is minimal and explicit, prioritizing readability over generality and performance. Models are implemented from scratch in MLX, with tokenizers from the Huggingface Transformers library. Dependencies include Numpy and Pillow for image post-processing. Installation can be done using `uv tool` or classic virtual environment setup. Command-line arguments allow for image generation with specified models, prompts, and optional parameters. Quantization options for speed and memory reduction are available. LoRA adapters can be loaded for fine-tuning image generation. Controlnet support provides more control over image generation with reference images. Current limitations include generating images one by one, lack of support for negative prompts, and some LoRA adapters not working.
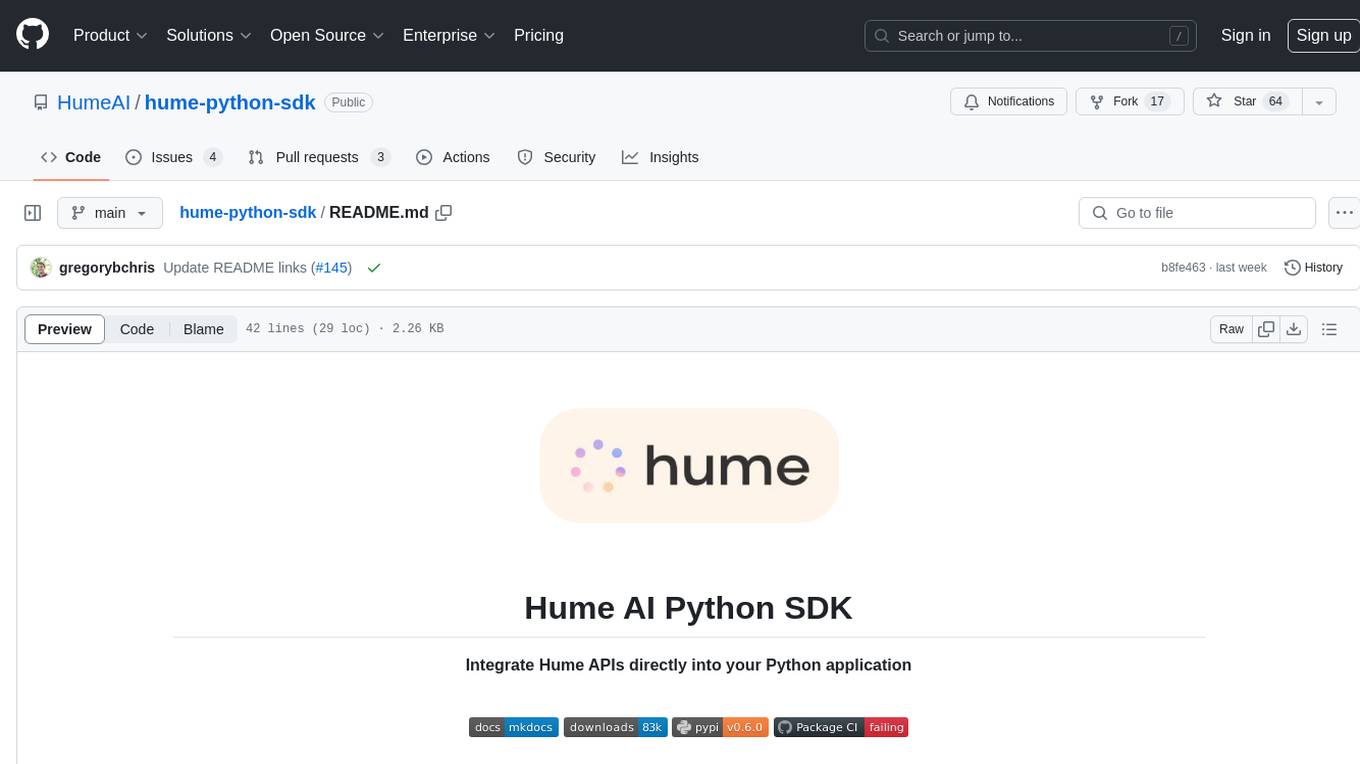
hume-python-sdk
The Hume AI Python SDK allows users to integrate Hume APIs directly into their Python applications. Users can access complete documentation, quickstart guides, and example notebooks to get started. The SDK is designed to provide support for Hume's expressive communication platform built on scientific research. Users are encouraged to create an account at beta.hume.ai and stay updated on changes through Discord. The SDK may undergo breaking changes to improve tooling and ensure reliable releases in the future.
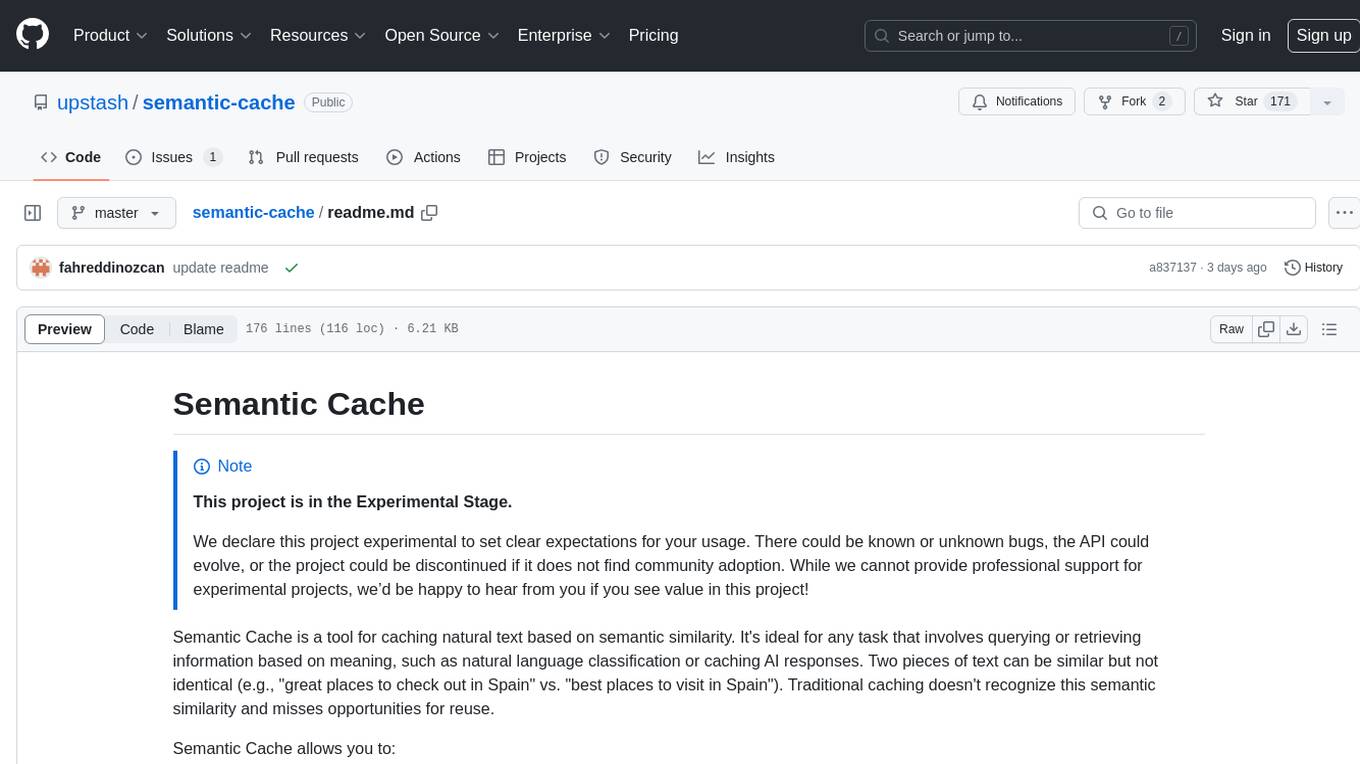
semantic-cache
Semantic Cache is a tool for caching natural text based on semantic similarity. It allows for classifying text into categories, caching AI responses, and reducing API latency by responding to similar queries with cached values. The tool stores cache entries by meaning, handles synonyms, supports multiple languages, understands complex queries, and offers easy integration with Node.js applications. Users can set a custom proximity threshold for filtering results. The tool is ideal for tasks involving querying or retrieving information based on meaning, such as natural language classification or caching AI responses.
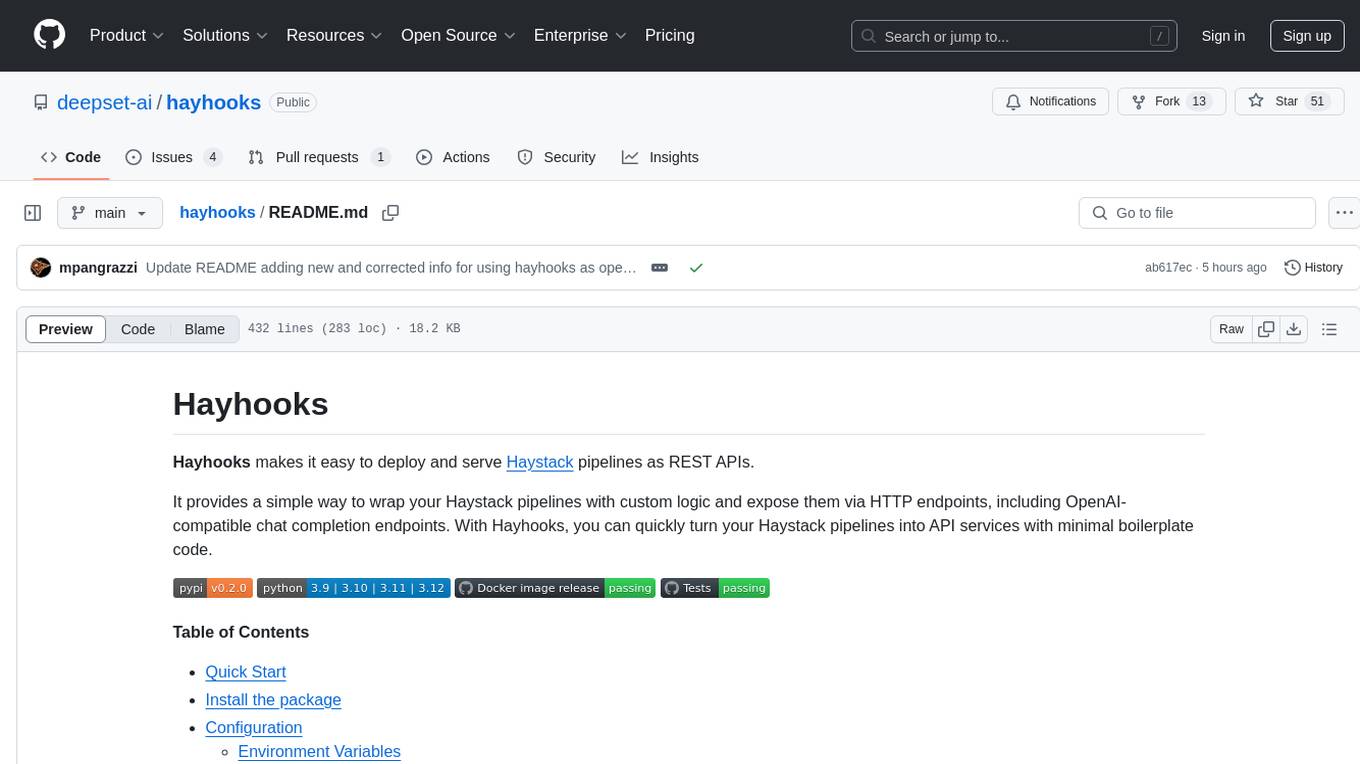
hayhooks
Hayhooks is a tool that simplifies the deployment and serving of Haystack pipelines as REST APIs. It allows users to wrap their pipelines with custom logic and expose them via HTTP endpoints, including OpenAI-compatible chat completion endpoints. With Hayhooks, users can easily convert their Haystack pipelines into API services with minimal boilerplate code.
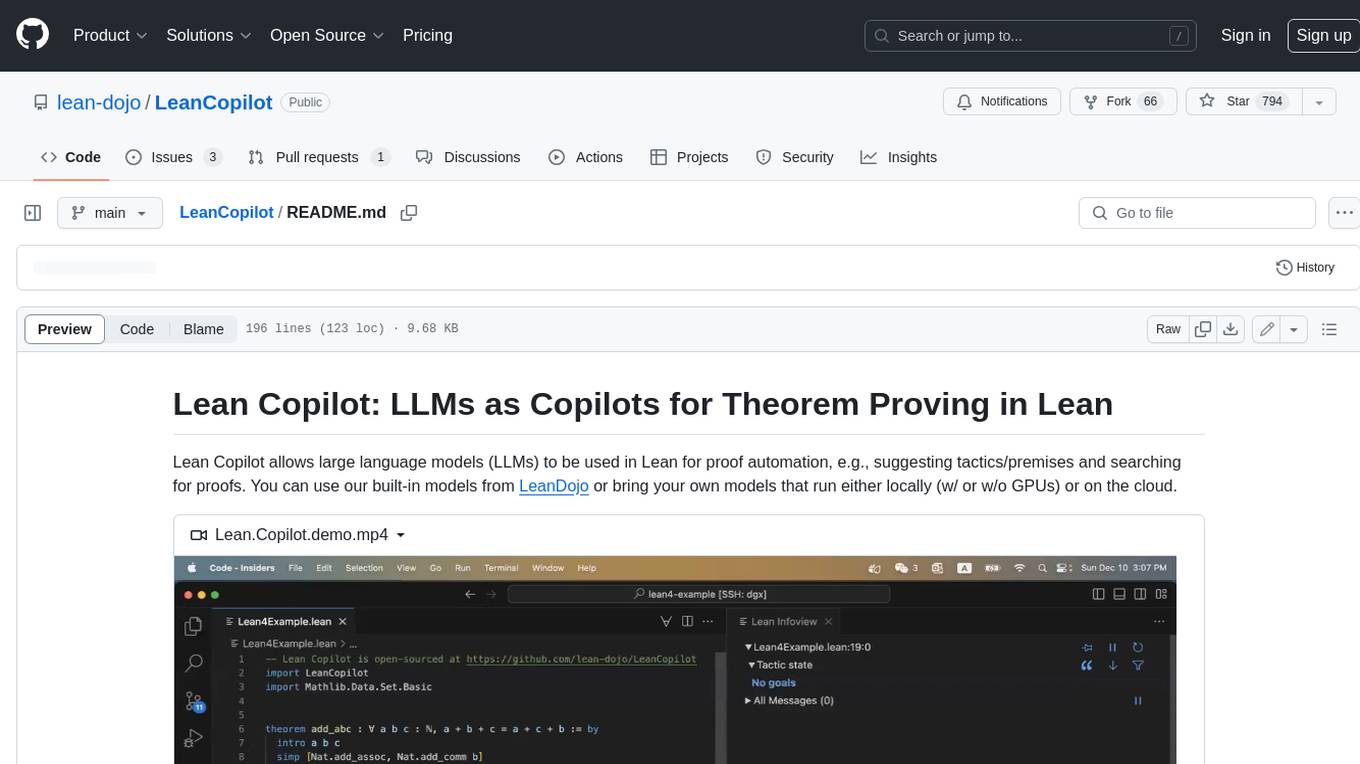
LeanCopilot
Lean Copilot is a tool that enables the use of large language models (LLMs) in Lean for proof automation. It provides features such as suggesting tactics/premises, searching for proofs, and running inference of LLMs. Users can utilize built-in models from LeanDojo or bring their own models to run locally or on the cloud. The tool supports platforms like Linux, macOS, and Windows WSL, with optional CUDA and cuDNN for GPU acceleration. Advanced users can customize behavior using Tactic APIs and Model APIs. Lean Copilot also allows users to bring their own models through ExternalGenerator or ExternalEncoder. The tool comes with caveats such as occasional crashes and issues with premise selection and proof search. Users can get in touch through GitHub Discussions for questions, bug reports, feature requests, and suggestions. The tool is designed to enhance theorem proving in Lean using LLMs.
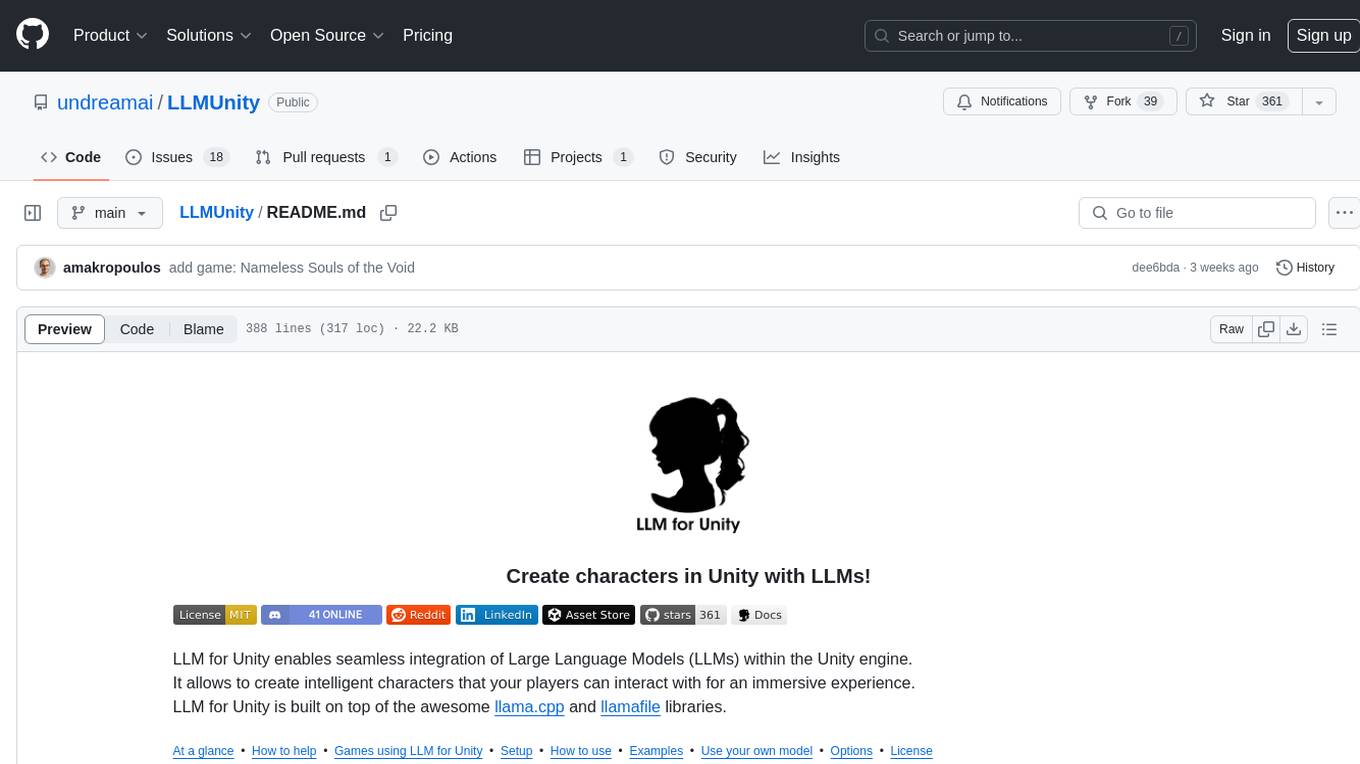
LLMUnity
LLM for Unity enables seamless integration of Large Language Models (LLMs) within the Unity engine, allowing users to create intelligent characters for immersive player interactions. The tool supports major LLM models, runs locally without internet access, offers fast inference on CPU and GPU, and is easy to set up with a single line of code. It is free for both personal and commercial use, tested on Unity 2021 LTS, 2022 LTS, and 2023. Users can build multiple AI characters efficiently, use remote servers for processing, and customize model settings for text generation.