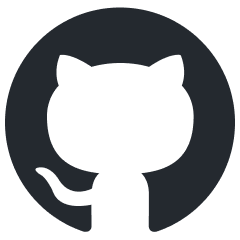
ai2-scholarqa-lib
Repo housing the open sourced code for the ai2 scholar qa app and also the corresponding library
Stars: 142
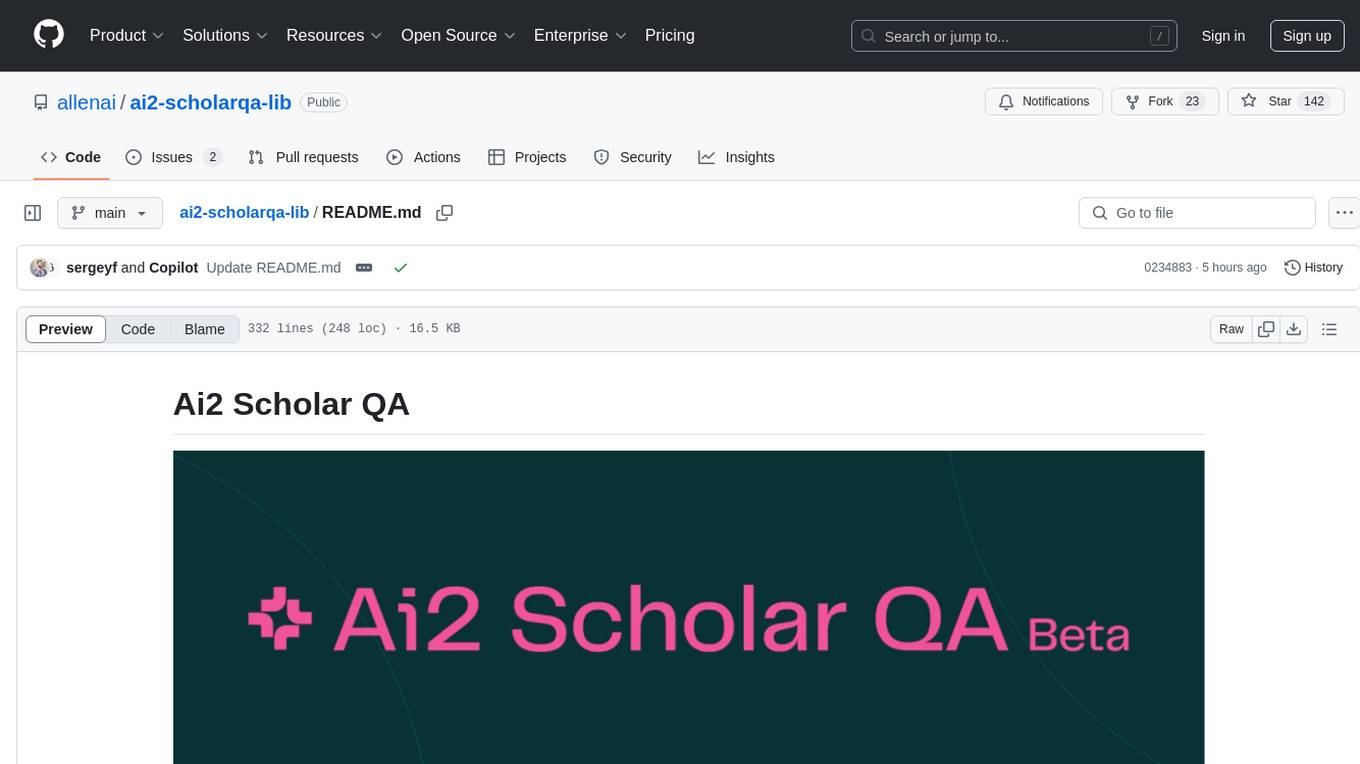
README:
This repo houses the code for the live demo and can be run as local docker containers or embedded into another application as a python package.
Ai2 Scholar QA is a system for answering scientific queries and literature review by gathering evidence from multiple documents across our corpus and synthesizing an organized report with evidence for each claim. As a RAG based architecture, Ai2 Scholar QA has a retrieval component and a three step generator pipeline.
-
The retrieval component consists of two sub-components:
i. Retriever - Based on the user query, relevant evidence passages are fetched using the Semantic Scholar public api's snippet/search end point which looks up an index of open source papers. Further, we also use the api's keyword search to suppliement the results from the index with paper abstracts. The user query is preprocessed to extract entities for filtering the papers and re-writing the query as needed. Prompt
ii. Reranker - The results from the retriever are then reranked with mixedbread-ai/mxbai-rerank-large-v1 and top k results are retained and aggregated at the paper-level to combine all the passages from a single paper.
These components are encapsulated in the PaperFinder class.
-
The generation pipeline comprises of three steps:
i. Quote Extraction - The user query along with the aggregated passages from the retrieval component are sent to an LLM (Claude Sonnet 3.5 default) to extract exact quotes relevant to answer the query. Prompt
ii. Planning and Clustering - The llm is then prompted to generate an organization of the output report with sections headings and format of the section. The quotes from step (i) are clustered and assigned to each heading. Prompt
iii. Summary Generation - Each section is generated based on the quotes assigned to that section and all the prior text generated in the report. Prompt
These steps are encapsulated in the MultiStepQAPipeline class. For some sections, we also generate literature review tables that compare and contrast all papers referenced in that section. We generate these tables using the pipeline proposed by the ArxivDIGESTables paper, which is available here.
Both the PaperFinder and MultiStepQAPipeline are in turn members of ScholarQA, which is the main class powering our system.
For more info please refer to our blogpost.
Environment Variables
Ai2 Scholar QA requires Semantic Scholar api and LLMs for its core functionality of retrieval and generation. So please ensure to create a .env
file in the root directory with the following environment variables:
export S2_API_KEY=
export ANTHROPIC_API_KEY=
export OPENAI_API_KEY=
S2_API_KEY
: Used to retrieve the relevant paper passages , keyword search results and associated metadata via the Semantic Scholar public api.
ANTHROPIC_API_KEY
: Ai2 Scholar QA uses Anthropic's Claude 3.5 Sonnet as the primary LLM for generation, but any model served by litellm should work. Please configure the corresponding api key here.
OPENAI_API_KEY
: OpenAI's GPT 4o is configured as the fallback llm.
Note: We also use OpenAI's text moderation api to validate and filter harmful queries. If you don't have access to an OpenAI api key, this feature will be disabled.
If you use Modal to serve your models, please configure MODAL_TOKEN
and MODAL_TOKEN_SECRET
here as well.
Please refer to default.json for the default runtime config.
{
"logs": {
"log_dir": "logs",
"llm_cache_dir": "llm_cache",
"event_trace_loc": "scholarqa_traces",
"tracing_mode": "local"
},
"run_config": {
"retrieval_service": "public_api",
"retriever_args": {
"n_retrieval": 256,
"n_keyword_srch": 20
},
"reranker_service": "modal",
"reranker_args": {
"app_name": "ai2-scholar-qa",
"api_name": "inference_api",
"batch_size": 256,
"gen_options": {}
},
"paper_finder_args": {
"n_rerank": 50,
"context_threshold": 0.5
},
"pipeline_args": {
"validate": true,
"llm": "anthropic/claude-3-5-sonnet-20241022",
"decomposer_llm": "anthropic/claude-3-5-sonnet-20241022"
}
}
}
The config is used to populate the AppConfig instance:
Logging
class LogsConfig(BaseModel):
log_dir: str = Field(default="logs", description="Directory to store logs, event traces and litellm cache")
llm_cache_dir: str = Field(default="llm_cache", description="Sub directory to cache llm calls")
event_trace_loc: str = Field(default="scholarqa_traces", description="Sub directory to store event traces"
"OR the GCS bucket name")
tracing_mode: Literal["local", "gcs"] = Field(default="local",
description="Mode to store event traces (local or gcs)")
Note:
i. Event Traces are json documents containing a trace of the entire pipeline i.e. the results of retrieval, reranking, each step of the qa pipeline and associated costs, if any.
ii. llm_cache_dir is used to initialize the local disk cache for caching llm calls via litellm.
iii. The traces are stored locally in {log_dir}/{event_trace_loc}
by
default. They can also be persisted in a Google Cloud Storage (GCS)
bucket. Please set the tracing_mode="gcs"
and event_trace_loc=<GCS bucket name>
here and the export GOOGLE_APPLICATION_CREDENTIALS=<Service Account Key json file path>
in .env
.
iv. By default, the working directory is ./api
, so the log_dir
will be created inside it as a sub-directory unless the config is modified.
You can also activate Langsmith based log traces if you have an api key configured. Please add the following environment variables:
LANGCHAIN_API_KEY
LANGCHAIN_TRACING_V2
LANGCHAIN_ENDPOINT
LANGCHAIN_PROJECT
Pipeline
class RunConfig(BaseModel):
retrieval_service: str = Field(default="public_api", description="Service to use for paper retrieval")
retriever_args: dict = Field(default=None, description="Arguments for the retrieval service")
reranker_service: str = Field(default="modal", description="Service to use for paper reranking")
reranker_args: dict = Field(default=None, description="Arguments for the reranker service")
paper_finder_args: dict = Field(default=None, description="Arguments for the paper finder service")
pipeline_args: dict = Field(default=None, description="Arguments for the Scholar QA pipeline service")
Note:
i. *(retrieval, reranker)_service
can be used to indicate the type
of retrieval/reranker you want to instantiate. Ai2 Scholar QA uses the
FullTextRetriever
and ModalReranker
respectively, which are chosen based on the
default public_api
and modal
keywords. To choose a
SentenceTransformers reranker, replace modal
with cross_encoder
or
biencoder
or define your own types.
ii. *(retriever, reranker, paper_finder, pipeline)_args
are used to
initialize the corresponding instances of the pipeline components. eg.
retriever = FullTextRetriever(**run_config.retriever_args)
. You
can initialize multiple runs and customize your pipeline.
iii. If the reranker_args
are not defined, the app resorts to using only the retrieval service.
The web app initializes 4 docker containers - one each for the API, GUI, nginx proxy and sonar with their own Dockerfile. The api container config can also be used to declare environment variables -
api:
build: ./api
volumes:
- ./api:/api
- ./secret:/secret
environment:
# This ensures that errors are printed as they occur, which
# makes debugging easier.
- PYTHONUNBUFFERED=1
- LOG_LEVEL=INFO
- CONFIG_PATH=run_configs/default.json
ports:
- 8000:8000
env_file:
- .env
environment.CONFIG_PATH
indicates the path of the application configuration json file.
env_file
indicates the path of the file with environment variables.
Please refer to DOCKER.md for more info on setting up the docker app.
i. Clone the repo
git clone [email protected]:allenai/ai2-scholarqa-lib.git
cd ai2-scholarqa-lib
ii. Run docker-compose
docker compose up --build
The docker compose command takes a while to run the first time to install torch and related dependencies. You can get the verbose output with the following command:
docker compose build --progress plain
https://github.com/user-attachments/assets/7d6761d6-1e95-4dac-9aeb-a5a898a89fbe
https://github.com/user-attachments/assets/baed8710-2161-4fbf-b713-3a2dcf46ac61
https://github.com/user-attachments/assets/f9a1b39f-36c8-41c4-a0ac-10046ded0593
The Ai2 Scholar QA UI is powered by an async api at the back end in app.py which is run from dev.sh.
i. The query_corpusqa
end point is first called with the query
, and a uuid as the user_id
, adn it returns a task_id
.
ii. Subsequently, the query_corpusqa
is then polled to get the updated status of the async task until the task status is not COMPLETED
conda create -n scholarqa python=3.11.3
conda activate scholarqa
pip install ai2-scholar-qa
#to use sentence transformer models as re-ranker
pip install 'ai2-scholar-qa[all]'
Both the webapp and the api are powered by the same pipeline represented by the ScholarQA class. The pipeline consists of a retrieval component, the PaperFinder
which consists of a retriever and maybe a reranker and a 3 step generator component MultiStepQAPipeline
. Each component is extensible and can be replaced by custom instances/classes as required.
Sample usage
from scholarqa.rag.reranker.modal_engine import ModalReranker
from scholarqa.rag.retrieval import PaperFinderWithReranker
from scholarqa.rag.retriever_base import FullTextRetriever
from scholarqa import ScholarQA
from scholarqa.llms.constants import CLAUDE_35_SONNET
retriever = FullTextRetriever(n_retrieval=256, n_keyword_srch=20)
reranker = ModalReranker(app_name=<modal_app_name>, api_name=<modal_api_name>, batch_size=256, gen_options=dict())
paper_finder = PaperFinderWithReranker(retriever, reranker, n_rerank=50, context_threshold=0.5)
#For wrapper class with MultiStepQAPipeline integrated
scholar_qa = ScholarQA(paper_finder=paper_finder, llm_model=CLAUDE_35_SONNET) #llm_model can be any litellm model
print(scholar_qa.answer_query("Which is the 9th planet in our solar system?"))
#Custom MultiStepQAPipeline class/steps
from scholarqa.rag.multi_step_qa_pipeline import MultiStepQAPipeline
mqa_pipeline = MultiStepQAPipeline(llm_model=CLAUDE_35_SONNET)
per_paper_summaries, completion_results = mqa_pipeline.step_select_quotes(query, scored_df, sys_prompt)
plan_json = mqa_pipeline.step_clustering(query, per_paper_summaries, sys_prompt)
response = list(generate_iterative_summary(query, per_paper_summaries, plan_json, sys_prompt))
-
The api end points in app.py can be extended with a fastapi APIRouter in another script. eg.
custom_app.py
from fastapi import APIRouter, FastAPI from scholarqa.app import create_app as create_app_base def create_app() -> FastAPI: app = create_app_base() custom_router = APIRouter() @custom_router.post("/custom") def custom_endpt(): pass app.include_router(custom_router) return app.py
To run
custom_app.py
, simply replacescholarqa.app:create_app
in dev.sh with<package>.custom_app:create_app
-
To extend the existing ScholarQA functionality in a new class you can either create a sub class of ScholarQA or a new class altogether. Either way,
lazy_load_scholarqa
in app.py should be reimplemented in the new api script to ensure the correct class is initialized. -
The components of the pipeline are individually extensible. We have the following abstract classes that can be extended to achieve desired customization for retrieval:
and the MultiStepQAPipeline can be extended/modified as needed for generation.
-
If you would prefer to serve your models via modal, please refer to MODAL.md for more info and sample code that we used to deploy the reranker model in the live demo.
For Tasks:
Click tags to check more tools for each tasksFor Jobs:
Alternative AI tools for ai2-scholarqa-lib
Similar Open Source Tools
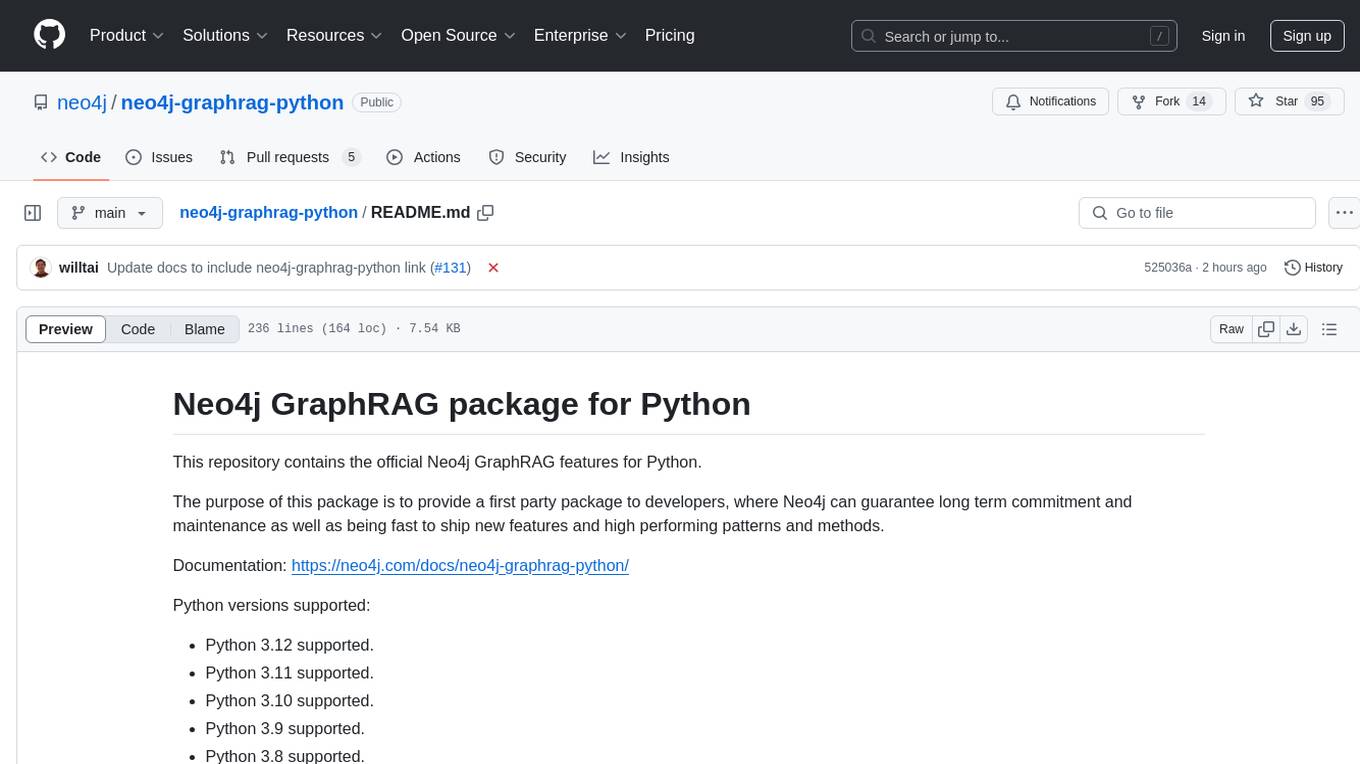
neo4j-graphrag-python
The Neo4j GraphRAG package for Python is an official repository that provides features for creating and managing vector indexes in Neo4j databases. It aims to offer developers a reliable package with long-term commitment, maintenance, and fast feature updates. The package supports various Python versions and includes functionalities for creating vector indexes, populating them, and performing similarity searches. It also provides guidelines for installation, examples, and development processes such as installing dependencies, making changes, and running tests.
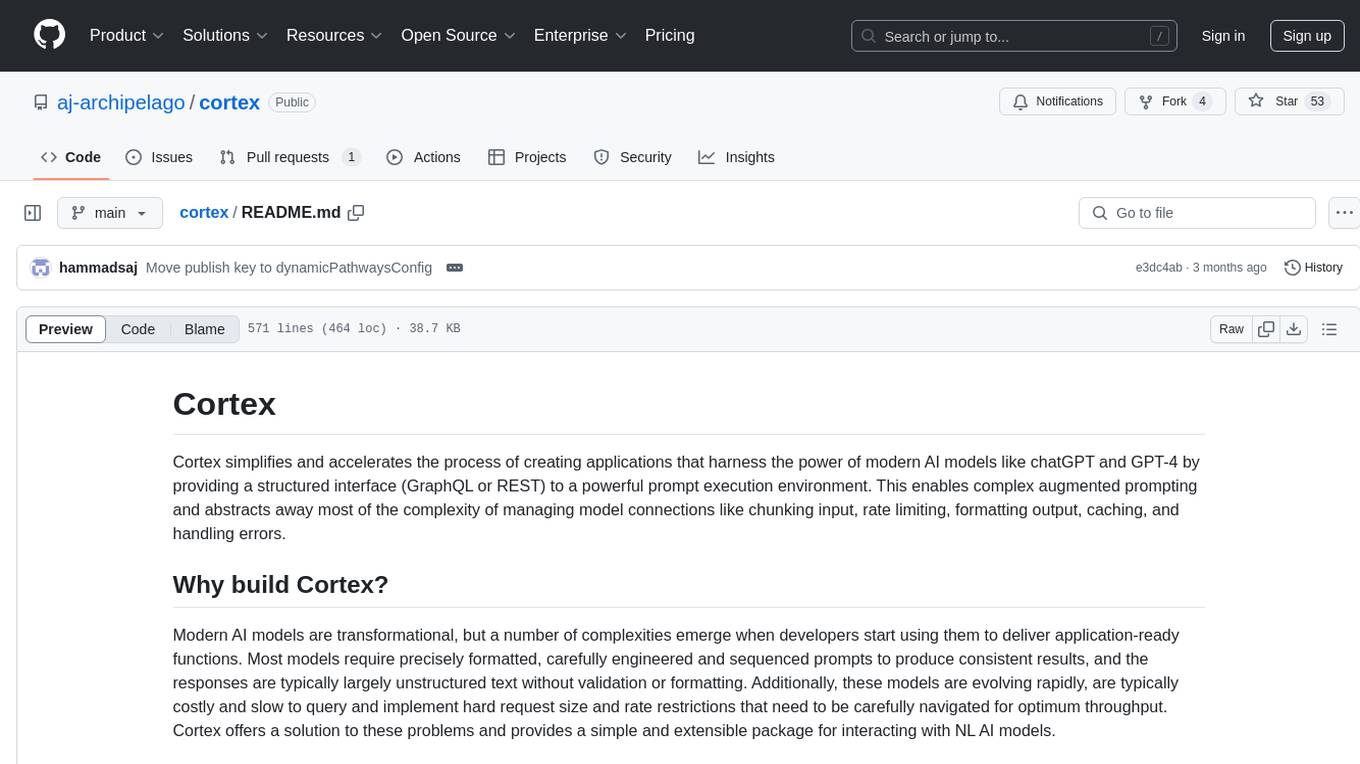
cortex
Cortex is a tool that simplifies and accelerates the process of creating applications utilizing modern AI models like chatGPT and GPT-4. It provides a structured interface (GraphQL or REST) to a prompt execution environment, enabling complex augmented prompting and abstracting away model connection complexities like input chunking, rate limiting, output formatting, caching, and error handling. Cortex offers a solution to challenges faced when using AI models, providing a simple package for interacting with NL AI models.
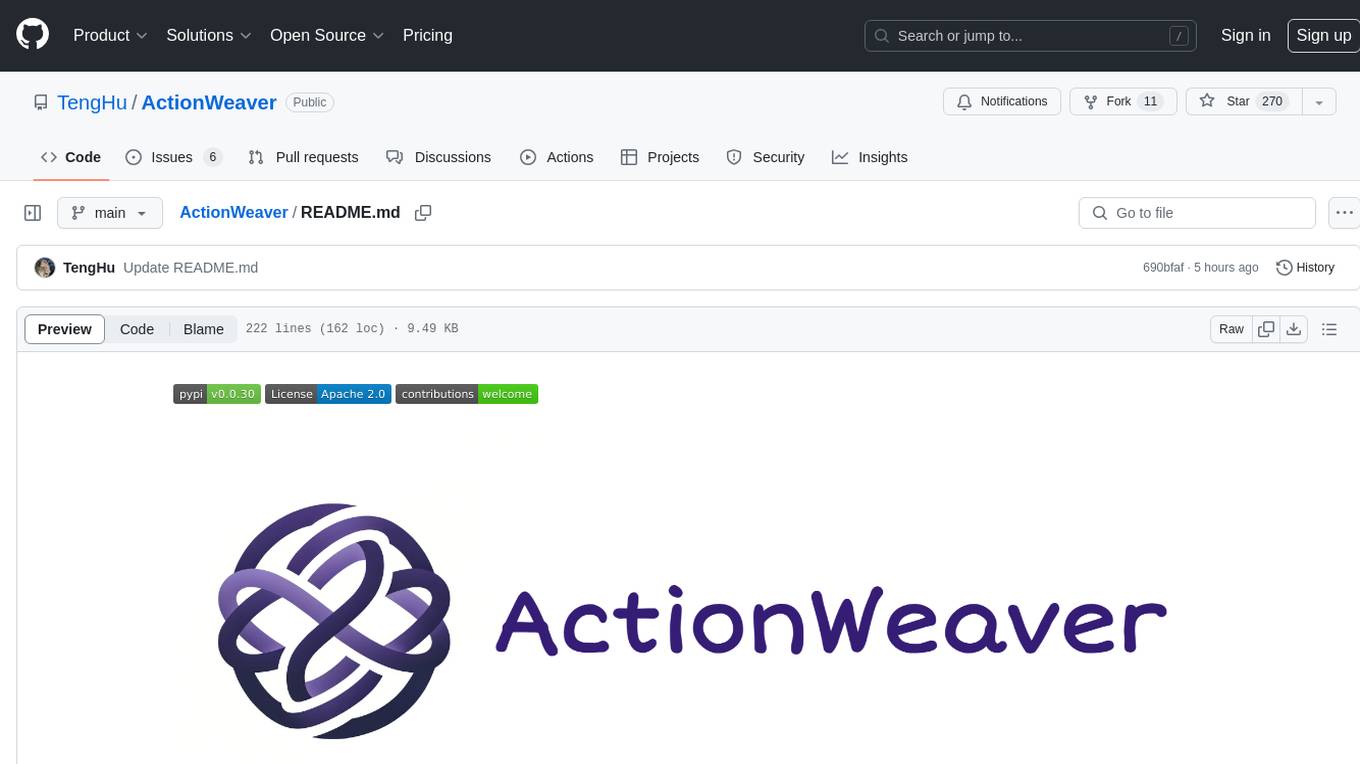
ActionWeaver
ActionWeaver is an AI application framework designed for simplicity, relying on OpenAI and Pydantic. It supports both OpenAI API and Azure OpenAI service. The framework allows for function calling as a core feature, extensibility to integrate any Python code, function orchestration for building complex call hierarchies, and telemetry and observability integration. Users can easily install ActionWeaver using pip and leverage its capabilities to create, invoke, and orchestrate actions with the language model. The framework also provides structured extraction using Pydantic models and allows for exception handling customization. Contributions to the project are welcome, and users are encouraged to cite ActionWeaver if found useful.
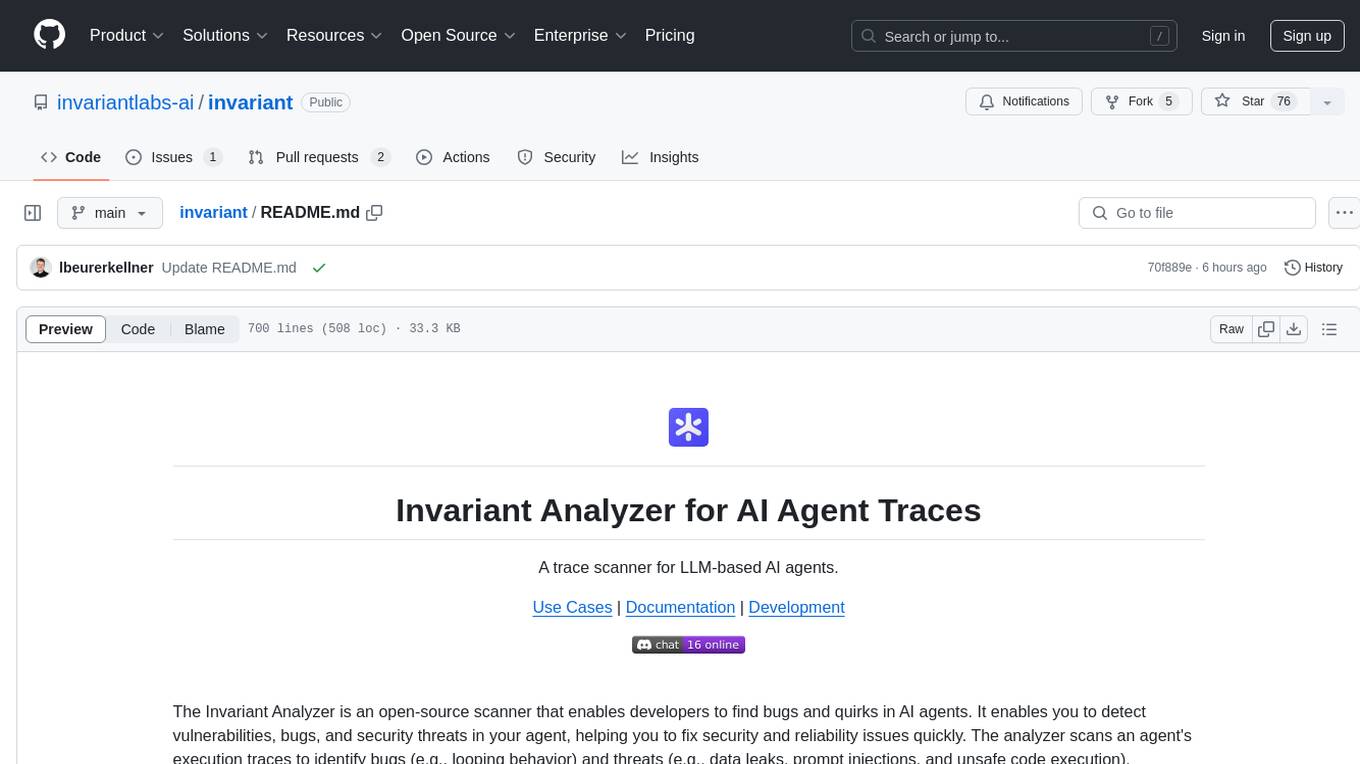
invariant
Invariant Analyzer is an open-source scanner designed for LLM-based AI agents to find bugs, vulnerabilities, and security threats. It scans agent execution traces to identify issues like looping behavior, data leaks, prompt injections, and unsafe code execution. The tool offers a library of built-in checkers, an expressive policy language, data flow analysis, real-time monitoring, and extensible architecture for custom checkers. It helps developers debug AI agents, scan for security violations, and prevent security issues and data breaches during runtime. The analyzer leverages deep contextual understanding and a purpose-built rule matching engine for security policy enforcement.
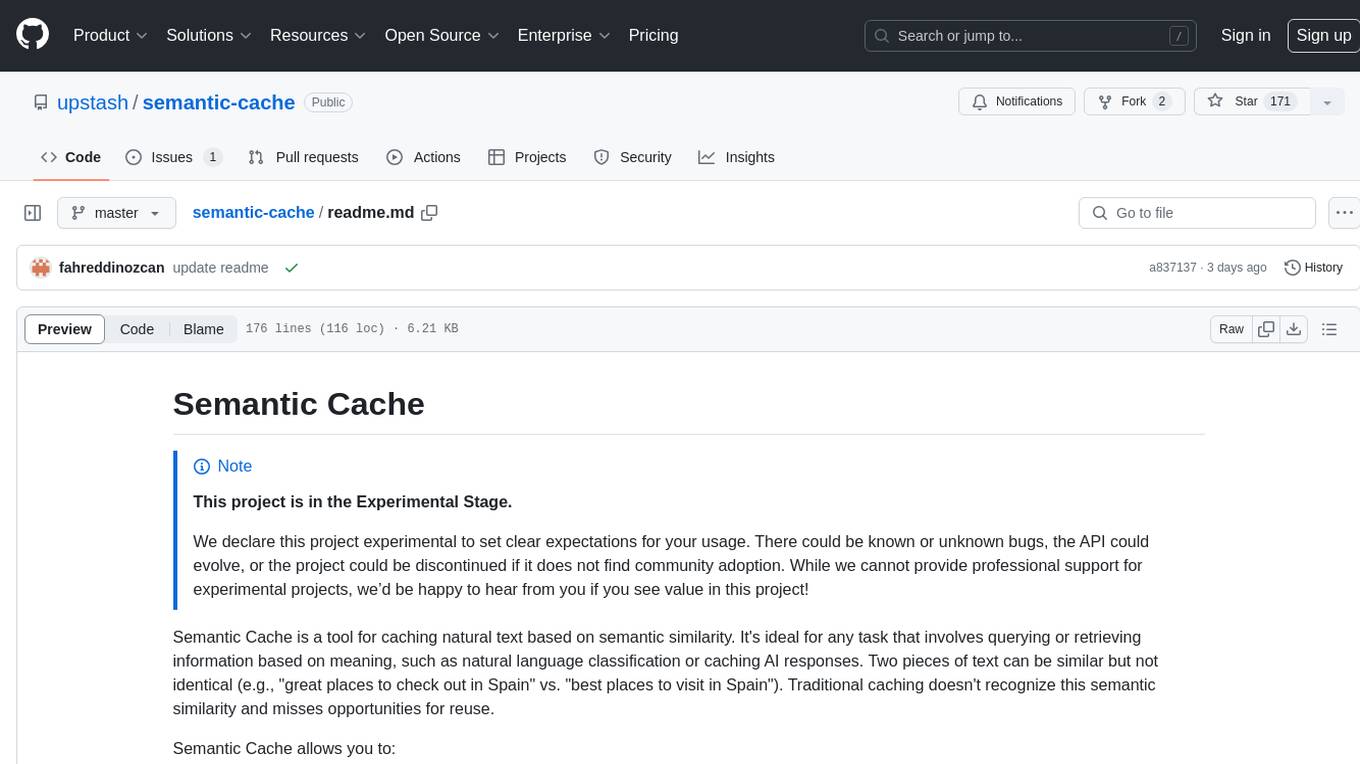
semantic-cache
Semantic Cache is a tool for caching natural text based on semantic similarity. It allows for classifying text into categories, caching AI responses, and reducing API latency by responding to similar queries with cached values. The tool stores cache entries by meaning, handles synonyms, supports multiple languages, understands complex queries, and offers easy integration with Node.js applications. Users can set a custom proximity threshold for filtering results. The tool is ideal for tasks involving querying or retrieving information based on meaning, such as natural language classification or caching AI responses.
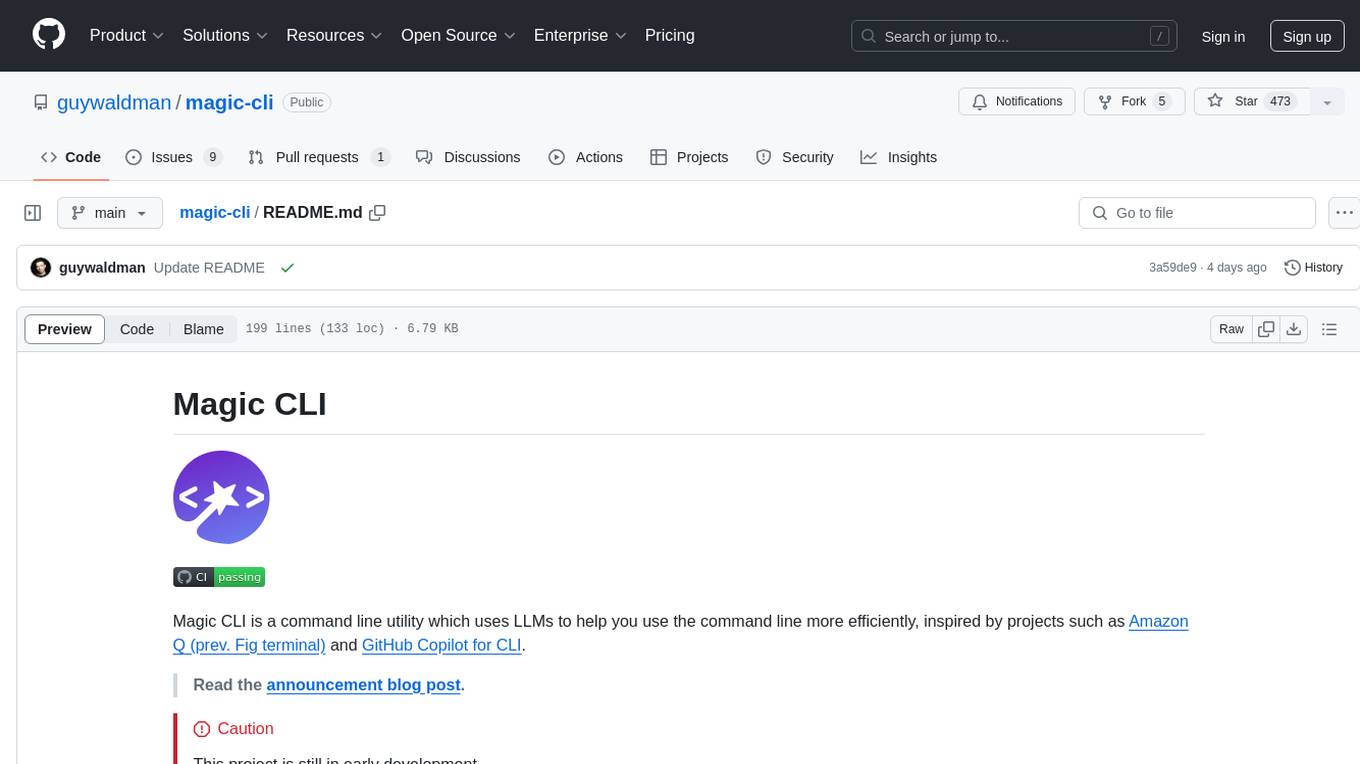
magic-cli
Magic CLI is a command line utility that leverages Large Language Models (LLMs) to enhance command line efficiency. It is inspired by projects like Amazon Q and GitHub Copilot for CLI. The tool allows users to suggest commands, search across command history, and generate commands for specific tasks using local or remote LLM providers. Magic CLI also provides configuration options for LLM selection and response generation. The project is still in early development, so users should expect breaking changes and bugs.
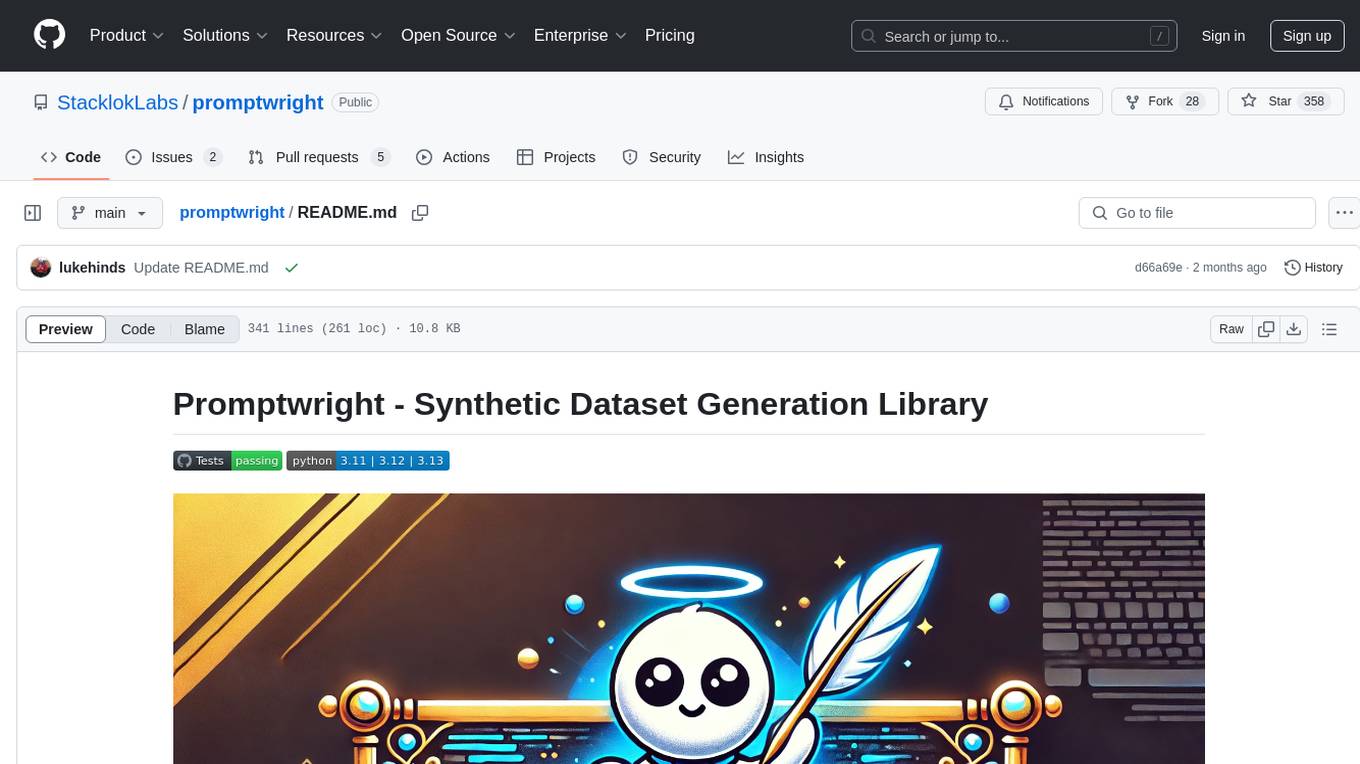
promptwright
Promptwright is a Python library designed for generating large synthetic datasets using a local LLM and various LLM service providers. It offers flexible interfaces for generating prompt-led synthetic datasets. The library supports multiple providers, configurable instructions and prompts, YAML configuration for tasks, command line interface for running tasks, push to Hugging Face Hub for dataset upload, and system message control. Users can define generation tasks using YAML configuration or Python code. Promptwright integrates with LiteLLM to interface with LLM providers and supports automatic dataset upload to Hugging Face Hub.
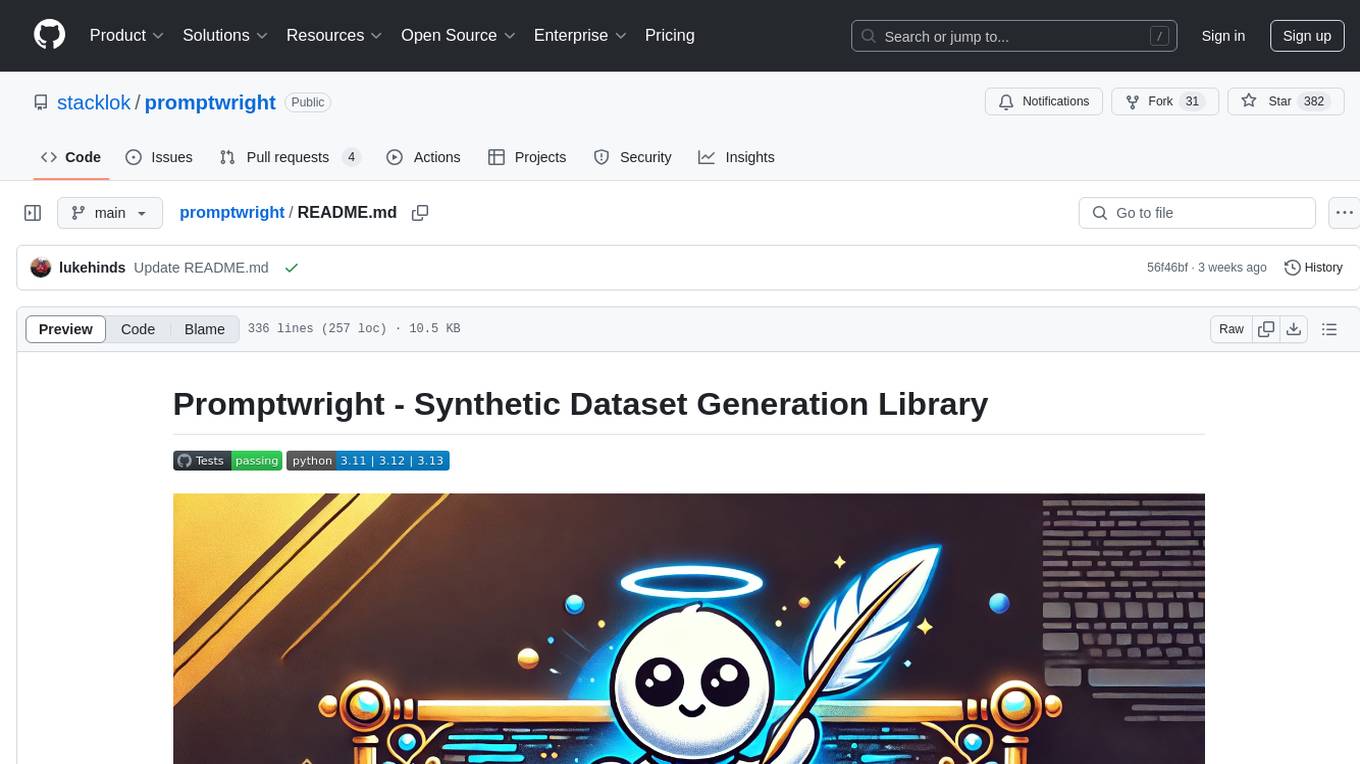
promptwright
Promptwright is a Python library designed for generating large synthetic datasets using local LLM and various LLM service providers. It offers flexible interfaces for generating prompt-led synthetic datasets. The library supports multiple providers, configurable instructions and prompts, YAML configuration, command line interface, push to Hugging Face Hub, and system message control. Users can define generation tasks using YAML configuration files or programmatically using Python code. Promptwright integrates with LiteLLM for LLM providers and supports automatic dataset upload to Hugging Face Hub. The library is not responsible for the content generated by models and advises users to review the data before using it in production environments.
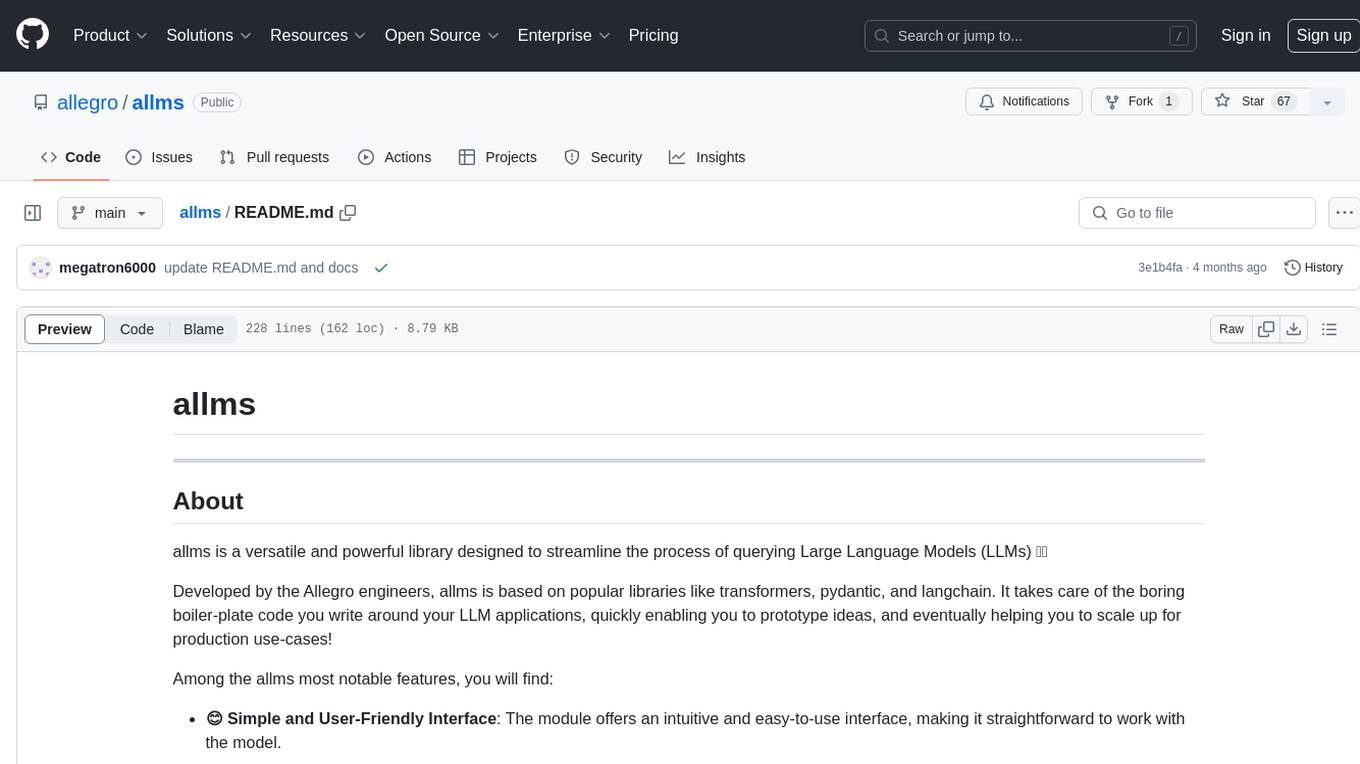
allms
allms is a versatile and powerful library designed to streamline the process of querying Large Language Models (LLMs). Developed by Allegro engineers, it simplifies working with LLM applications by providing a user-friendly interface, asynchronous querying, automatic retrying mechanism, error handling, and output parsing. It supports various LLM families hosted on different platforms like OpenAI, Google, Azure, and GCP. The library offers features for configuring endpoint credentials, batch querying with symbolic variables, and forcing structured output format. It also provides documentation, quickstart guides, and instructions for local development, testing, updating documentation, and making new releases.
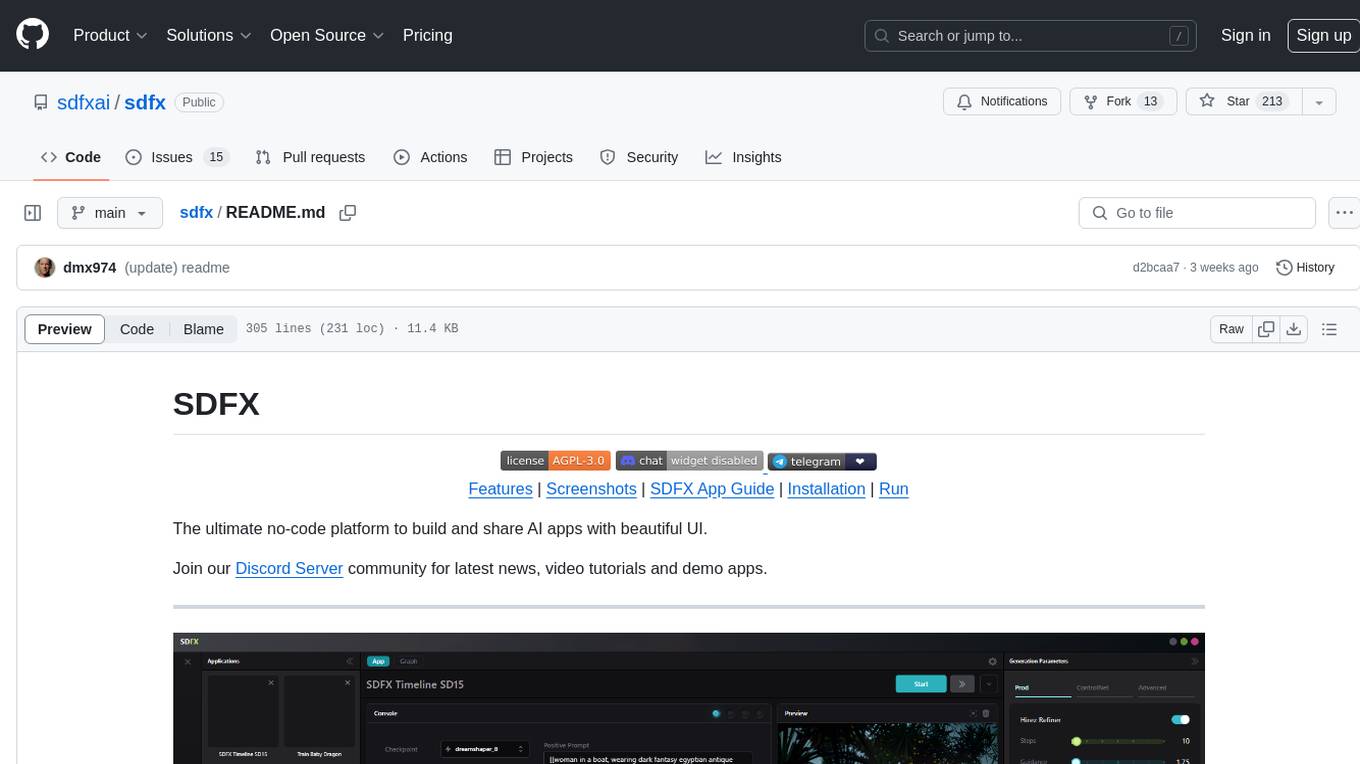
sdfx
SDFX is the ultimate no-code platform for building and sharing AI apps with beautiful UI. It enables the creation of user-friendly interfaces for complex workflows by combining Comfy workflow with a UI. The tool is designed to merge the benefits of form-based UI and graph-node based UI, allowing users to create intricate graphs with a high-level UI overlay. SDFX is fully compatible with ComfyUI, abstracting the need for installing ComfyUI. It offers features like animated graph navigation, node bookmarks, UI debugger, custom nodes manager, app and template export, image and mask editor, and more. The tool compiles as a native app or web app, making it easy to maintain and add new features.
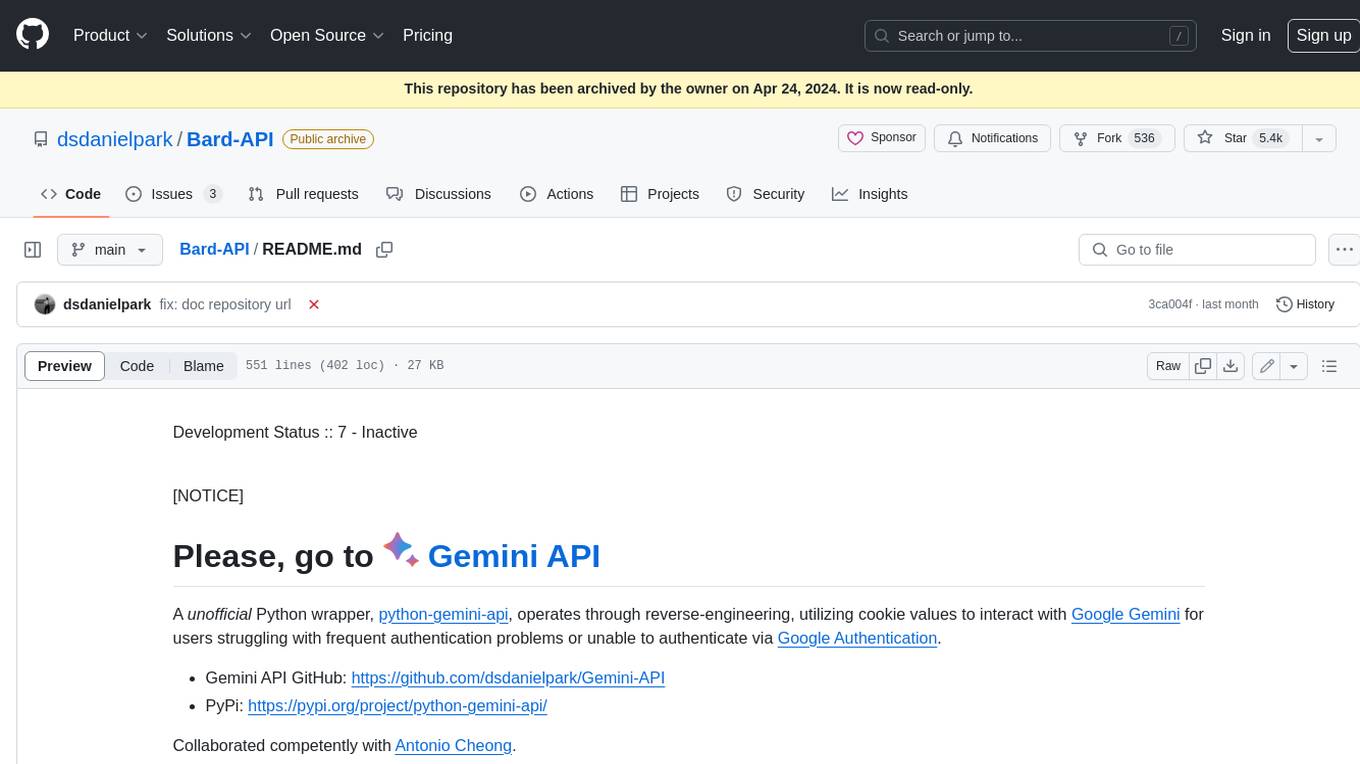
Bard-API
The Bard API is a Python package that returns responses from Google Bard through the value of a cookie. It is an unofficial API that operates through reverse-engineering, utilizing cookie values to interact with Google Bard for users struggling with frequent authentication problems or unable to authenticate via Google Authentication. The Bard API is not a free service, but rather a tool provided to assist developers with testing certain functionalities due to the delayed development and release of Google Bard's API. It has been designed with a lightweight structure that can easily adapt to the emergence of an official API. Therefore, using it for any other purposes is strongly discouraged. If you have access to a reliable official PaLM-2 API or Google Generative AI API, replace the provided response with the corresponding official code. Check out https://github.com/dsdanielpark/Bard-API/issues/262.
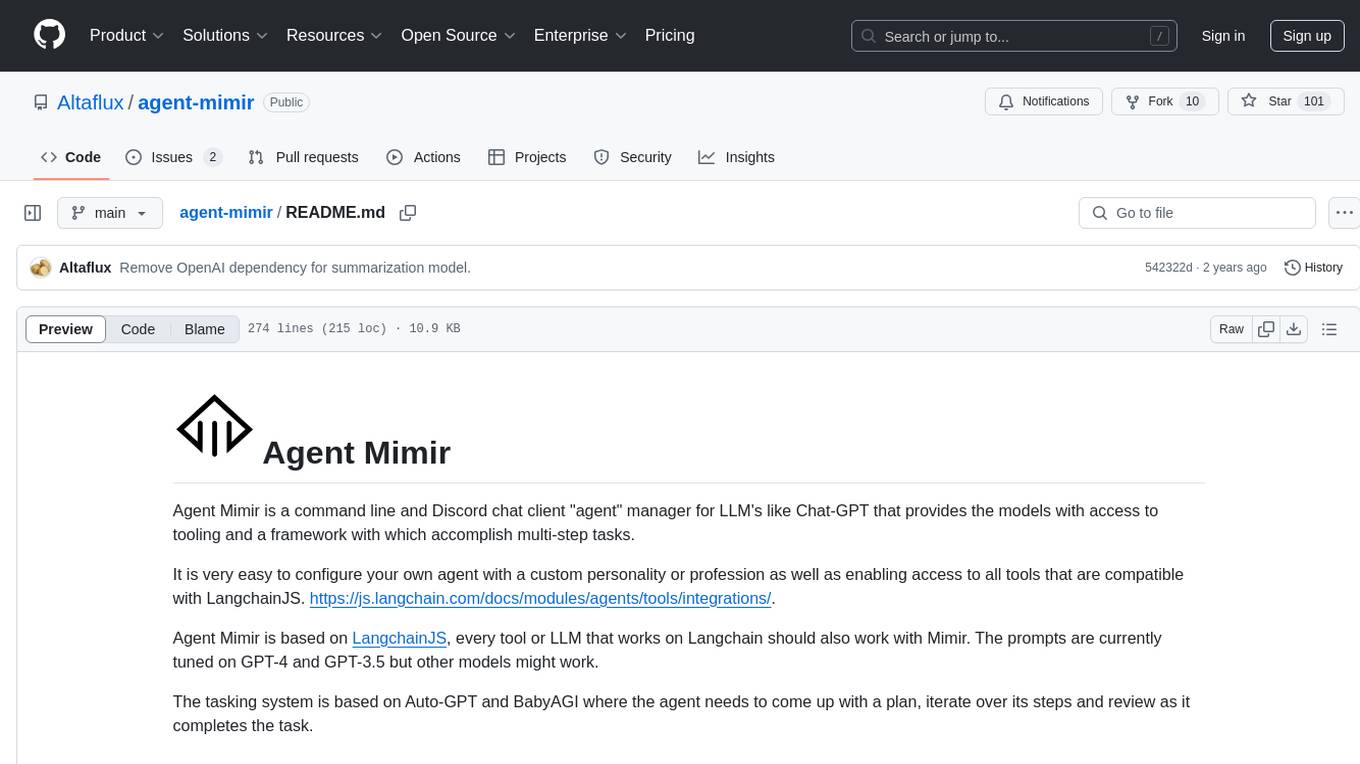
agent-mimir
Agent Mimir is a command line and Discord chat client 'agent' manager for LLM's like Chat-GPT that provides the models with access to tooling and a framework with which accomplish multi-step tasks. It is easy to configure your own agent with a custom personality or profession as well as enabling access to all tools that are compatible with LangchainJS. Agent Mimir is based on LangchainJS, every tool or LLM that works on Langchain should also work with Mimir. The tasking system is based on Auto-GPT and BabyAGI where the agent needs to come up with a plan, iterate over its steps and review as it completes the task.
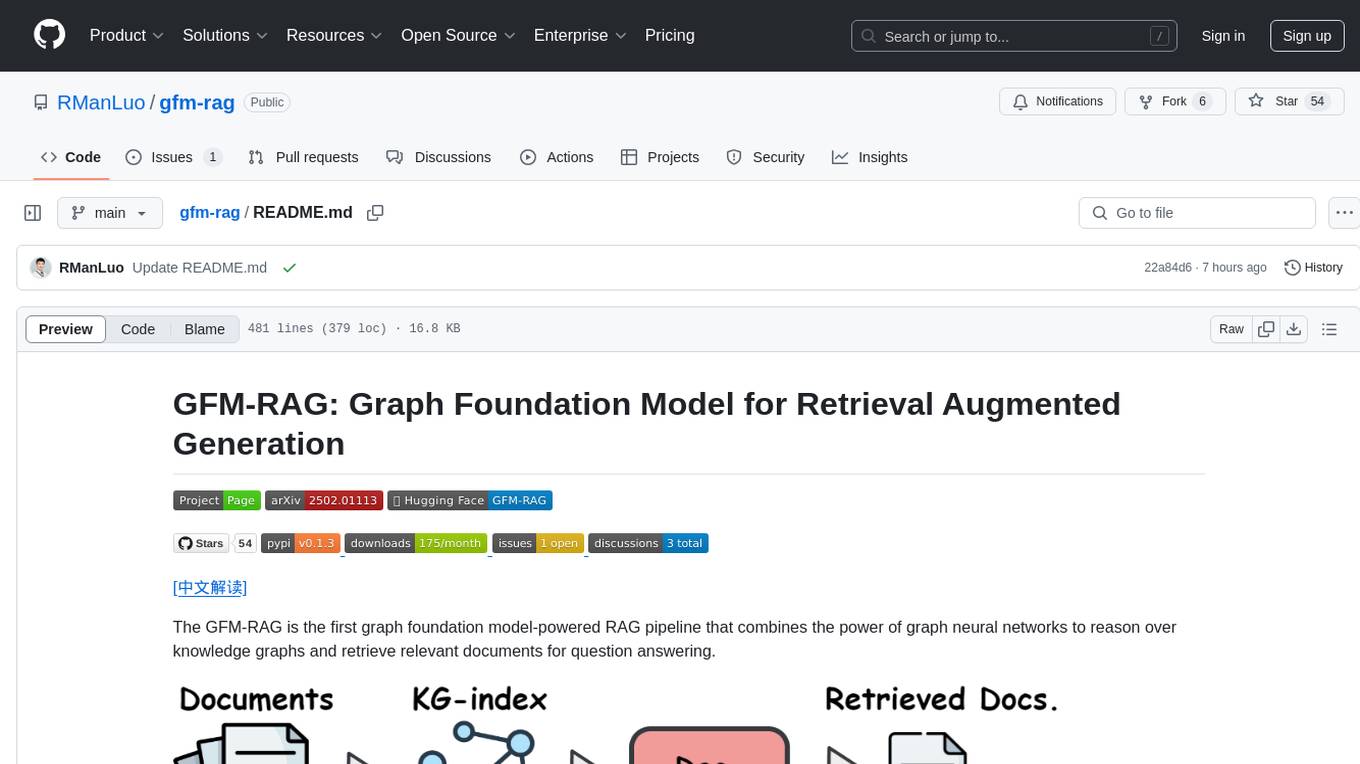
gfm-rag
The GFM-RAG is a graph foundation model-powered pipeline that combines graph neural networks to reason over knowledge graphs and retrieve relevant documents for question answering. It features a knowledge graph index, efficiency in multi-hop reasoning, generalizability to unseen datasets, transferability for fine-tuning, compatibility with agent-based frameworks, and interpretability of reasoning paths. The tool can be used for conducting retrieval and question answering tasks using pre-trained models or fine-tuning on custom datasets.
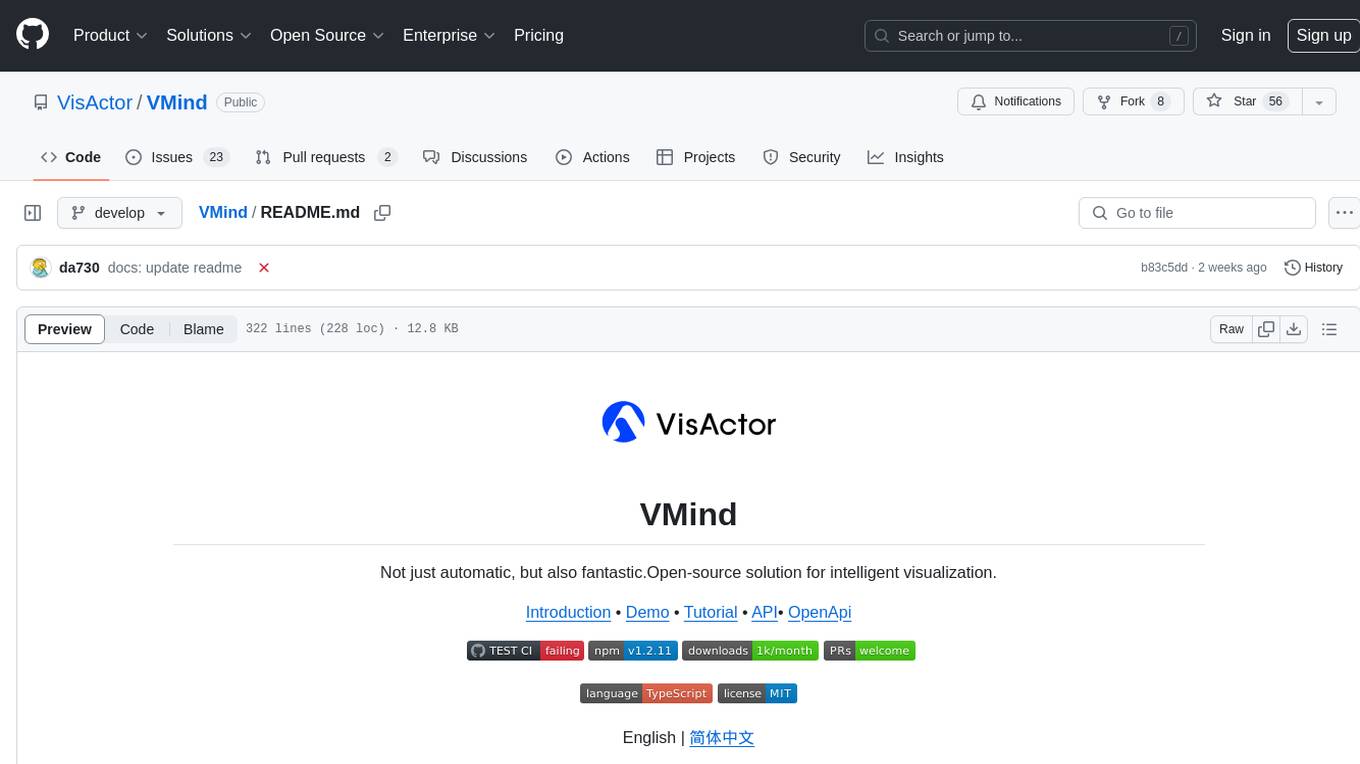
VMind
VMind is an open-source solution for intelligent visualization, providing an intelligent chart component based on LLM by VisActor. It allows users to create chart narrative works with natural language interaction, edit charts through dialogue, and export narratives as videos or GIFs. The tool is easy to use, scalable, supports various chart types, and offers one-click export functionality. Users can customize chart styles, specify themes, and aggregate data using LLM models. VMind aims to enhance efficiency in creating data visualization works through dialogue-based editing and natural language interaction.
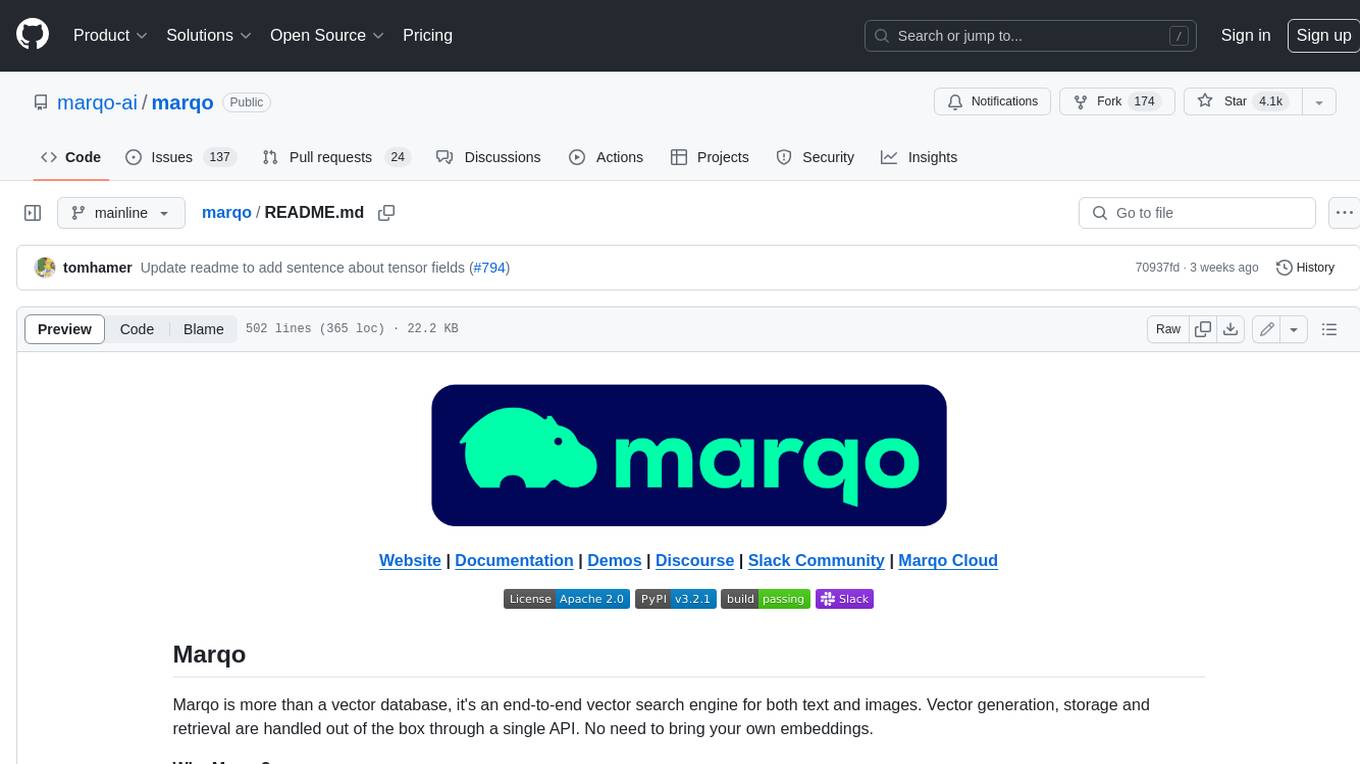
marqo
Marqo is more than a vector database, it's an end-to-end vector search engine for both text and images. Vector generation, storage and retrieval are handled out of the box through a single API. No need to bring your own embeddings.