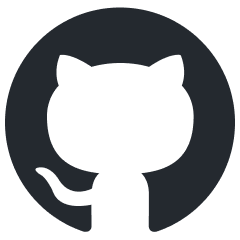
ActionWeaver
Make function calling with LLM easier
Stars: 296
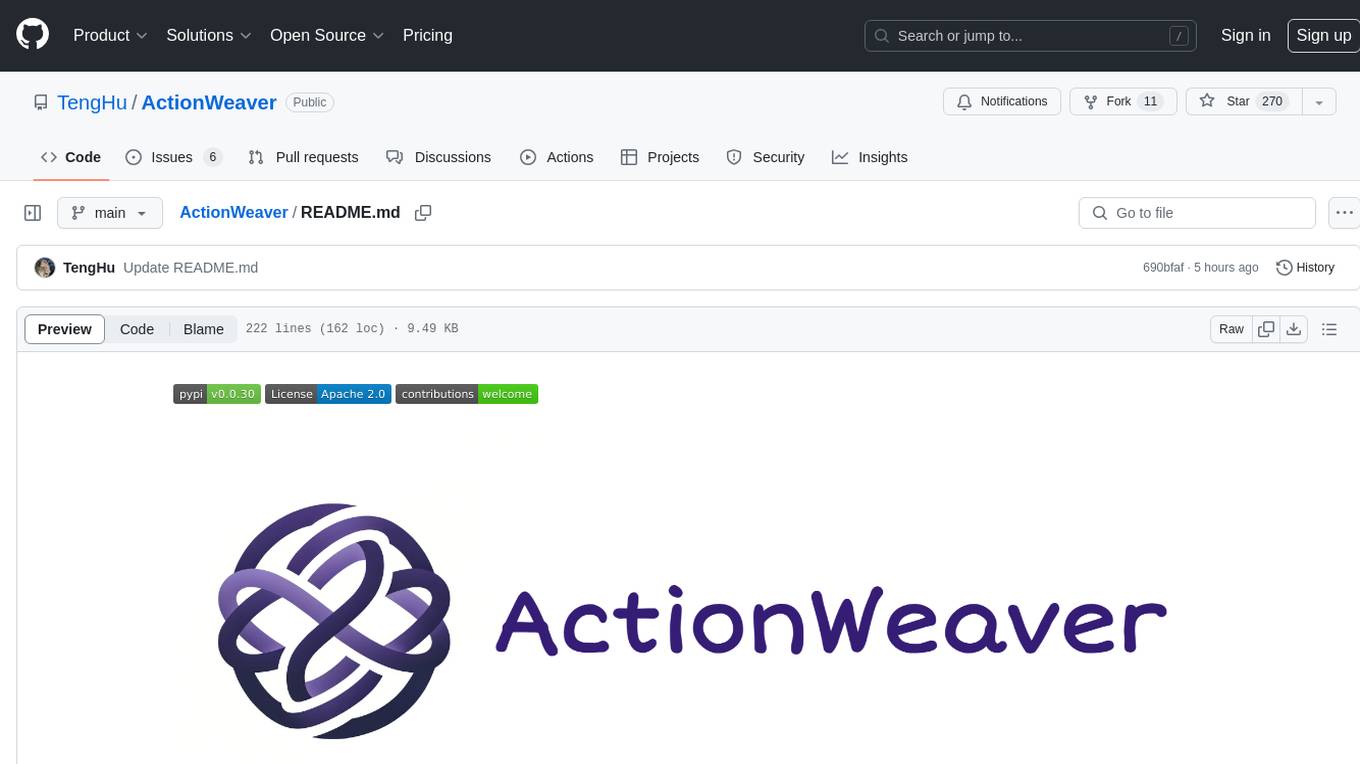
ActionWeaver is an AI application framework designed for simplicity, relying on OpenAI and Pydantic. It supports both OpenAI API and Azure OpenAI service. The framework allows for function calling as a core feature, extensibility to integrate any Python code, function orchestration for building complex call hierarchies, and telemetry and observability integration. Users can easily install ActionWeaver using pip and leverage its capabilities to create, invoke, and orchestrate actions with the language model. The framework also provides structured extraction using Pydantic models and allows for exception handling customization. Contributions to the project are welcome, and users are encouraged to cite ActionWeaver if found useful.
README:
🪡 AI application framework that makes function calling with LLM easier🪡
- Designed for simplicity, only relying on OpenAI and Pydantic.
- Supporting both OpenAI API and Azure OpenAI service!
Explore Our Cookbooks For Tutorials & Examples!
NOTEBOOKS TO GET STARTED:
- QuickStart
- Using Pydantic for Structured Data Parsing and Validation
- Function Validation with Pydantic and Exception Handler
- Built Traceable Action with LangSmith Tracing
- Action Orchestration
- Stateful Agent
ActionWeaver strives to be the most reliable, user-friendly, high-speed, and cost-effective function-calling framework for AI engineers.
Features:
- Function Calling as First-Class Citizen: Put function-calling at the core of the framework.
- Extensibility: Integrate ANY Python code into your agent's toolbox with a single line of code, or combining tools from other ecosystems like LangChain or Llama Index.
- Function Orchestration: Build complex orchestration of function callings. including intricate hierarchies or chains.
- Telemetry and Observability: Easy integration with platforms like LangSmith to build tracable application. Also take a look at this link to see how ActionWeaver uses structured logging.
You can install ActionWeaver using pip:
pip install actionweaver
Use the LATEST OpenAI API that supports parallel function calling !
from actionweaver.llms import wrap
from openai import OpenAI
openai_client = wrap(OpenAI())
or using Azure OpenAI service
import os
from openai import AzureOpenAI
azure_client = wrap(AzureOpenAI(
azure_endpoint = os.getenv("AZURE_OPENAI_ENDPOINT"),
api_key=os.getenv("AZURE_OPENAI_KEY"),
api_version="2023-10-01-preview"
))
The ActionWeaver wrapped client will manage the function calling loop, which includes passing function descriptions, executing functions with arguments returned by llm, and handling exceptions.
This client will expose a create
API built upon the original chat.completions.create
API. The enhanced create
API will retain all original arguments and include additional parameters such as:
-
action
: providing available actions to LLM. -
orch
: orchestrating actions throughout the function calling loop. -
exception_handler
: an object guiding the function calling loop on how to handle exceptions. These arguments will be demonstrated in the subsequent sections.
These additional arguments are optional, and there's always the fallback option to access the original OpenAI client via openai_client.client
.
Note: An
action
represents a tool that can be used by LLM. Each action comprises two main elements: a Pydantic model that is auto-generated to facilitate prompting, and a conventional Python function.
Developers can attach ANY Python function as an action with a simple decorator. In the following example, we introduce action GetCurrentTime
, and then proceed to use the OpenAI API to invoke it.
ActionWeaver utilizes the decorated method's signature and docstring as a description, passing them along to OpenAI's function API. The Action decorator is also highly adaptable and can be combined with other decorators, provided that the original signature is preserved.
from actionweaver import action
@action(name="GetCurrentTime")
def get_current_time() -> str:
"""
Use this for getting the current time in the specified time zone.
:return: A string representing the current time in the specified time zone.
"""
import datetime
current_time = datetime.datetime.now()
return f"The current time is {current_time}"
# Ask LLM what time is it
response = openai_client.create(
model="gpt-4o",
messages=[{"role": "user", "content": "what time is it"}],
actions = [get_current_time]
)
Take a look what is passing to OpenAI API
get_current_weather.get_function_details()
"""
{'name': 'GetWeather',
'description': 'Get the current weather in a given location',
'parameters': {'properties': {'location': {'title': 'Location'},
'unit': {'default': 'fahrenheit', 'title': 'Unit'}},
'required': ['location'],
'title': 'Get_Current_Weather',
'type': 'object'}}
"""
You can also compel the language model to execute the action by calling the invoke
method of an action. Its arguments includes the ActionWeaver-wrapped client and other arguments passed to the create API.
get_current_time.invoke(openai_client, messages=[{"role": "user", "content": "what time is it"}], model="gpt-3.5-turbo", stream=False, force=True)
You can create a Pydantic model to define the structural data you want to extract, create an action using action_from_model
and then force the language model to extract structured data from information in the prompt.
from pydantic import BaseModel
from actionweaver.actions.factories.pydantic_model_to_action import action_from_model
class User(BaseModel):
name: str
age: int
action_from_model(User, stop=True).invoke(client, messages=[{"role": "user", "content": "Tom is 31 years old"}], model="gpt-3.5-turbo", stream=False, force=False)
Note: The
stop
property of an action, with a default value of False, determines whether the function calling loop will immediately return the action's result instead of passing it to LLM if set to True.
Note: You can simultaneously pass actions generated from both functions and Pydantic models.
ActionWeaver enables the design of hierarchies and chains of actions by passing in orch
argument. orch
is a mapping from actions as keys to values including
- a list of actions: if the key action is invoked, LLM will proceed to choose an action from the provided list, or respond with a text message.
- an action: after key action is invoked, LLM will invoke the value action.
- None: after key action is invoked, LLM will respond with a text message.
For example, let's say we have actions a1, a2, a3.
client.create(
[
{"role": "user", "content": "message"}
],
actions=[a1, a2, a3], # First, LLM respond with either a1, a2 or a3, or text without action
# Define the orchestration logic for actions:
orch={
a1.name: [a2, a3], # If a1 is invoked, the next response will be either a2, a3 or a text response.
a2.name: a3, # If a2 is invoked, the next action will be a3
a3.name: [a4] # If a3 is invoked, the next response will be a4 or a text response.
a4.name: None # If a4 is invoked, the next response will guarantee to be a text message
}
)
Users can provide a specific implementation of ExceptionHandler, where the handle_exception
method is invoked upon encountering an exception. The info
parameter encapsulates contextual details such as messages and API responses within a dictionary.
The handle_exception
method dictates the course of action for the function calling loop, returning either:
-
Return
: providing immediate content back to the user -
Continue
: instructing the loop to proceed.
from actionweaver.llms import ExceptionAction, ChatLoopInfo, Continue, Return
class ExceptionHandler(ABC):
"""Base class for exception handlers.
This class provides a framework for handling exceptions within the function calling loop.
"""
@abstractmethod
def handle_exception(self, e: Exception, info: ChatLoopInfo) -> ExceptionAction:
pass
Take a look at this example for details.
Contributions in the form of bug fixes, new features, documentation improvements, and pull requests are VERY welcomed.
If you find ActionWeaver useful, please consider citing the project:
@software{Teng_Hu_ActionWeaver_2024,
author = {Teng Hu},
license = {Apache-2.0},
month = Aug,
title = {ActionWeaver: Application Framework for LLMs},
url = {https://github.com/TengHu/ActionWeaver},
year = {2024}
}
For Tasks:
Click tags to check more tools for each tasksFor Jobs:
Alternative AI tools for ActionWeaver
Similar Open Source Tools
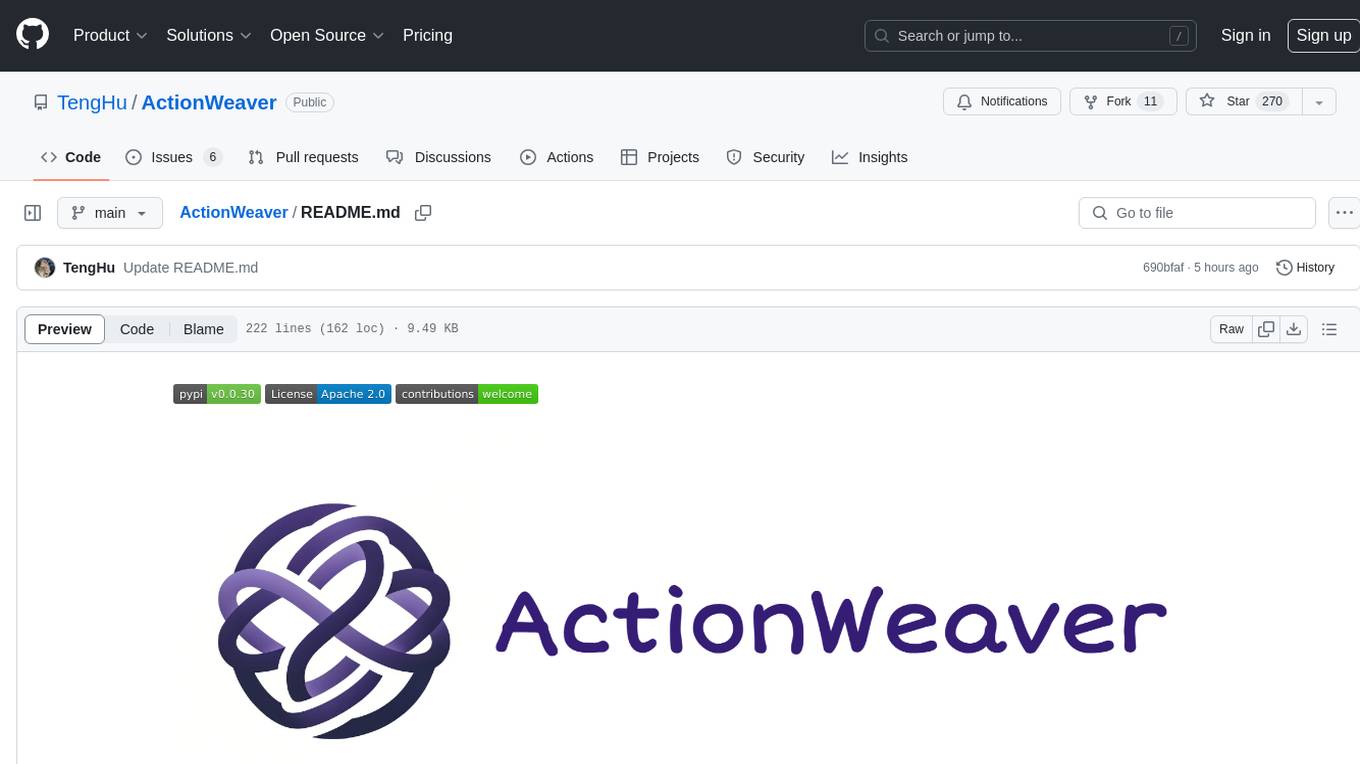
ActionWeaver
ActionWeaver is an AI application framework designed for simplicity, relying on OpenAI and Pydantic. It supports both OpenAI API and Azure OpenAI service. The framework allows for function calling as a core feature, extensibility to integrate any Python code, function orchestration for building complex call hierarchies, and telemetry and observability integration. Users can easily install ActionWeaver using pip and leverage its capabilities to create, invoke, and orchestrate actions with the language model. The framework also provides structured extraction using Pydantic models and allows for exception handling customization. Contributions to the project are welcome, and users are encouraged to cite ActionWeaver if found useful.
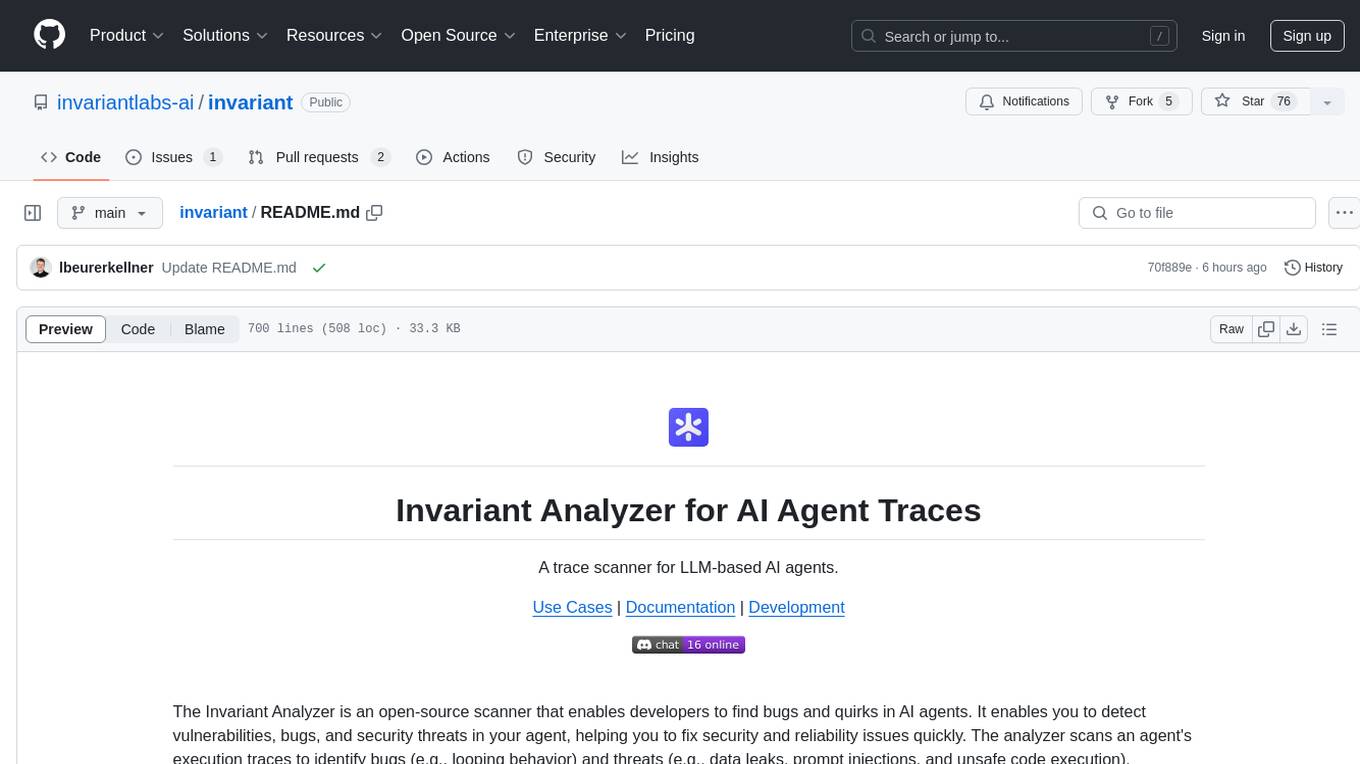
invariant
Invariant Analyzer is an open-source scanner designed for LLM-based AI agents to find bugs, vulnerabilities, and security threats. It scans agent execution traces to identify issues like looping behavior, data leaks, prompt injections, and unsafe code execution. The tool offers a library of built-in checkers, an expressive policy language, data flow analysis, real-time monitoring, and extensible architecture for custom checkers. It helps developers debug AI agents, scan for security violations, and prevent security issues and data breaches during runtime. The analyzer leverages deep contextual understanding and a purpose-built rule matching engine for security policy enforcement.
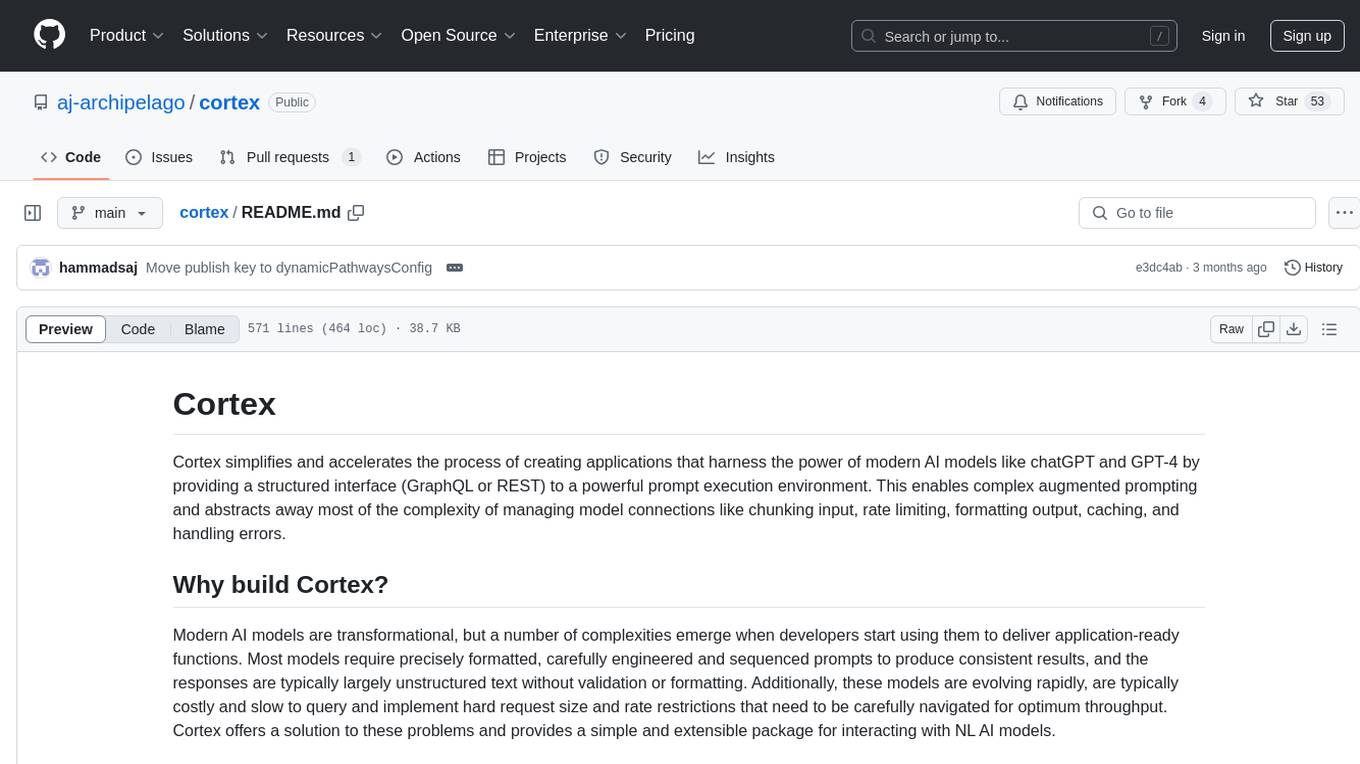
cortex
Cortex is a tool that simplifies and accelerates the process of creating applications utilizing modern AI models like chatGPT and GPT-4. It provides a structured interface (GraphQL or REST) to a prompt execution environment, enabling complex augmented prompting and abstracting away model connection complexities like input chunking, rate limiting, output formatting, caching, and error handling. Cortex offers a solution to challenges faced when using AI models, providing a simple package for interacting with NL AI models.
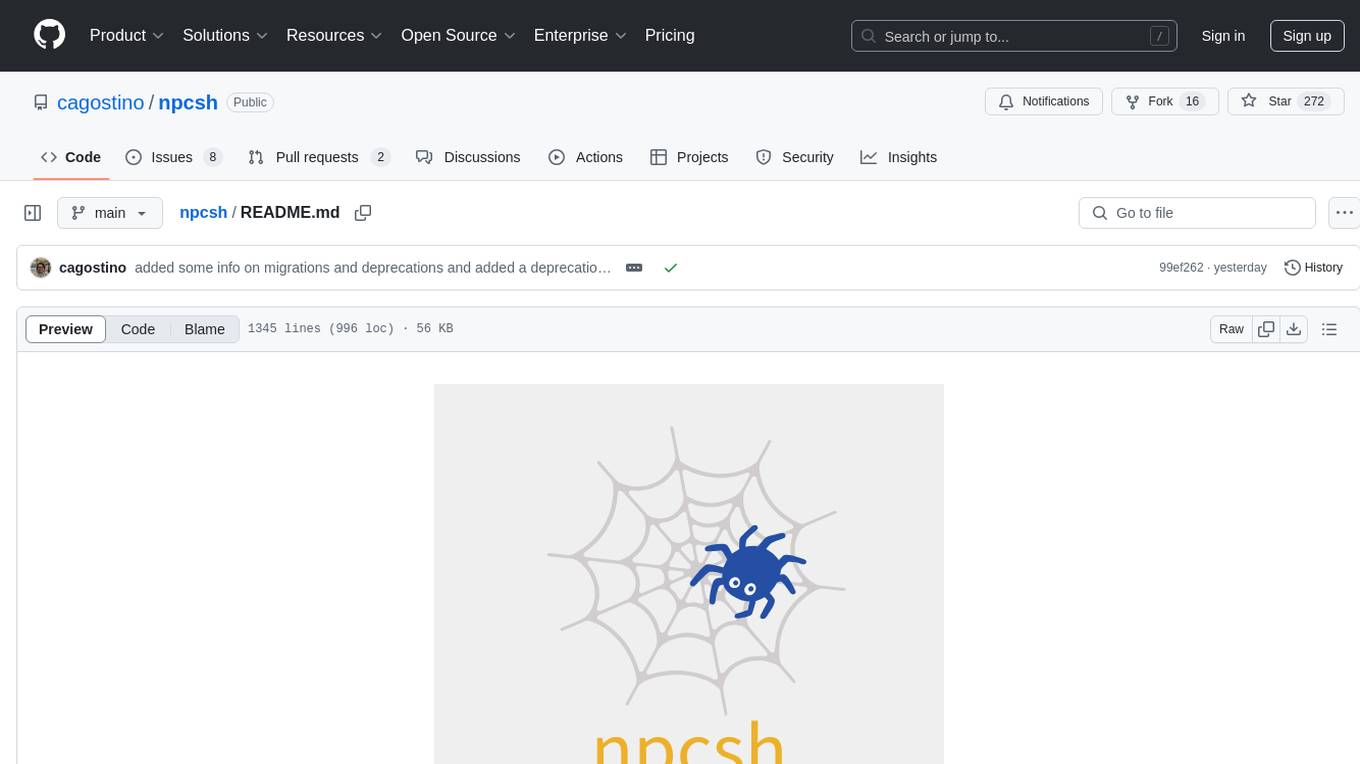
npcsh
`npcsh` is a python-based command-line tool designed to integrate Large Language Models (LLMs) and Agents into one's daily workflow by making them available and easily configurable through the command line shell. It leverages the power of LLMs to understand natural language commands and questions, execute tasks, answer queries, and provide relevant information from local files and the web. Users can also build their own tools and call them like macros from the shell. `npcsh` allows users to take advantage of agents (i.e. NPCs) through a managed system, tailoring NPCs to specific tasks and workflows. The tool is extensible with Python, providing useful functions for interacting with LLMs, including explicit coverage for popular providers like ollama, anthropic, openai, gemini, deepseek, and openai-like providers. Users can set up a flask server to expose their NPC team for use as a backend service, run SQL models defined in their project, execute assembly lines, and verify the integrity of their NPC team's interrelations. Users can execute bash commands directly, use favorite command-line tools like VIM, Emacs, ipython, sqlite3, git, pipe the output of these commands to LLMs, or pass LLM results to bash commands.
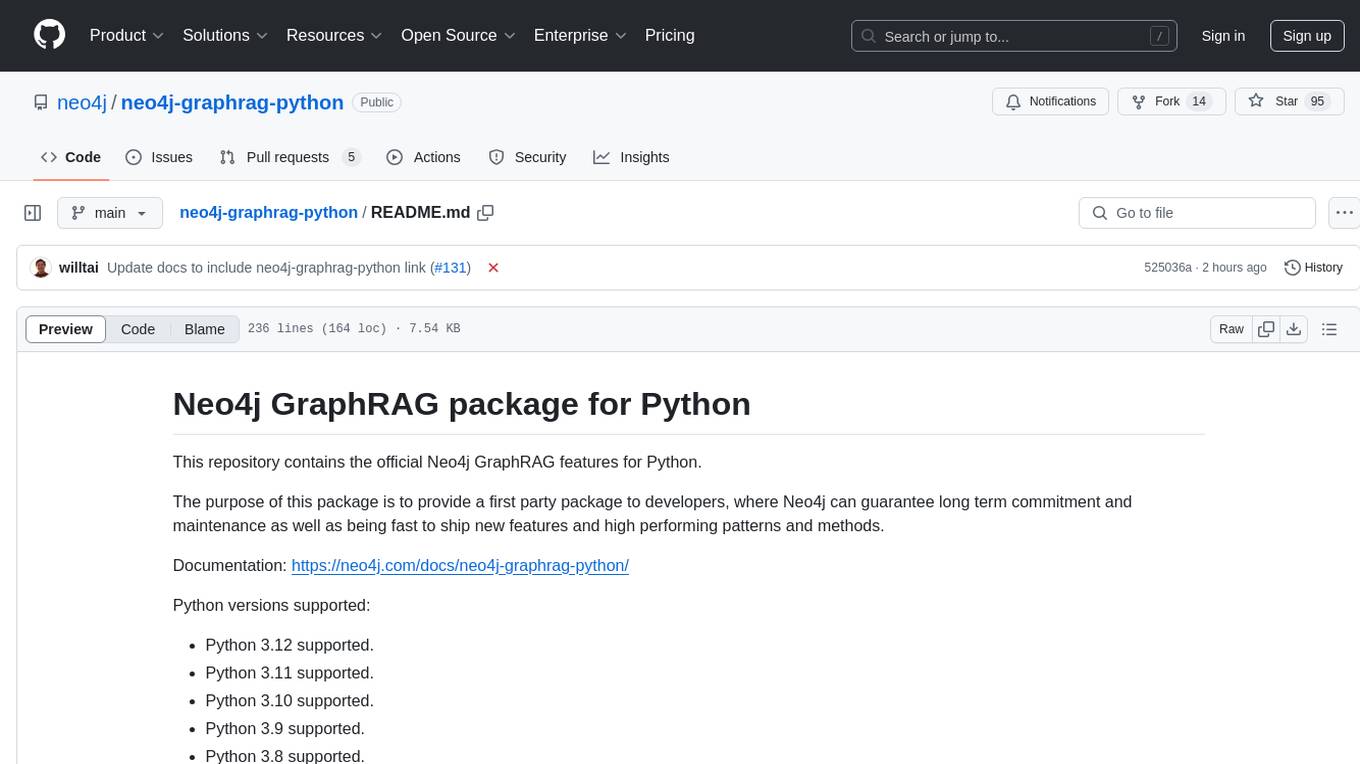
neo4j-graphrag-python
The Neo4j GraphRAG package for Python is an official repository that provides features for creating and managing vector indexes in Neo4j databases. It aims to offer developers a reliable package with long-term commitment, maintenance, and fast feature updates. The package supports various Python versions and includes functionalities for creating vector indexes, populating them, and performing similarity searches. It also provides guidelines for installation, examples, and development processes such as installing dependencies, making changes, and running tests.
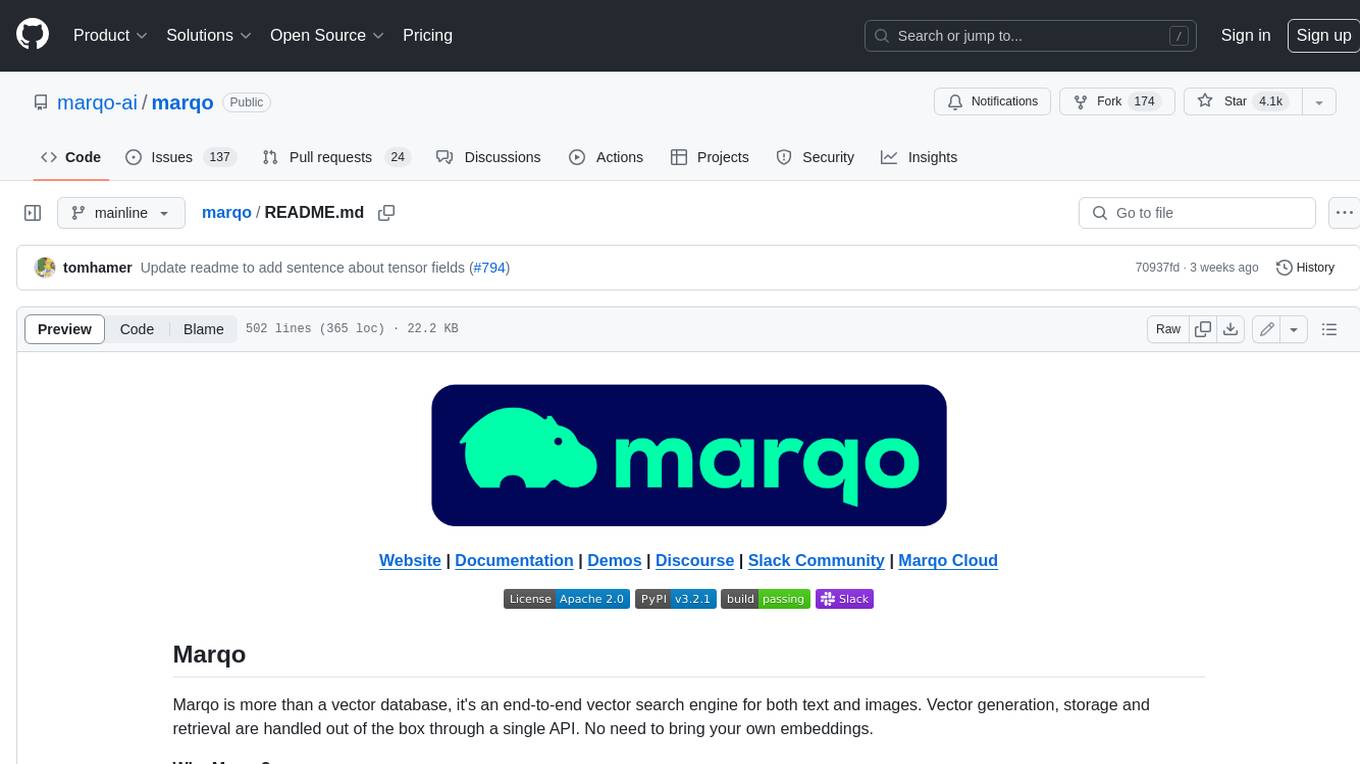
marqo
Marqo is more than a vector database, it's an end-to-end vector search engine for both text and images. Vector generation, storage and retrieval are handled out of the box through a single API. No need to bring your own embeddings.
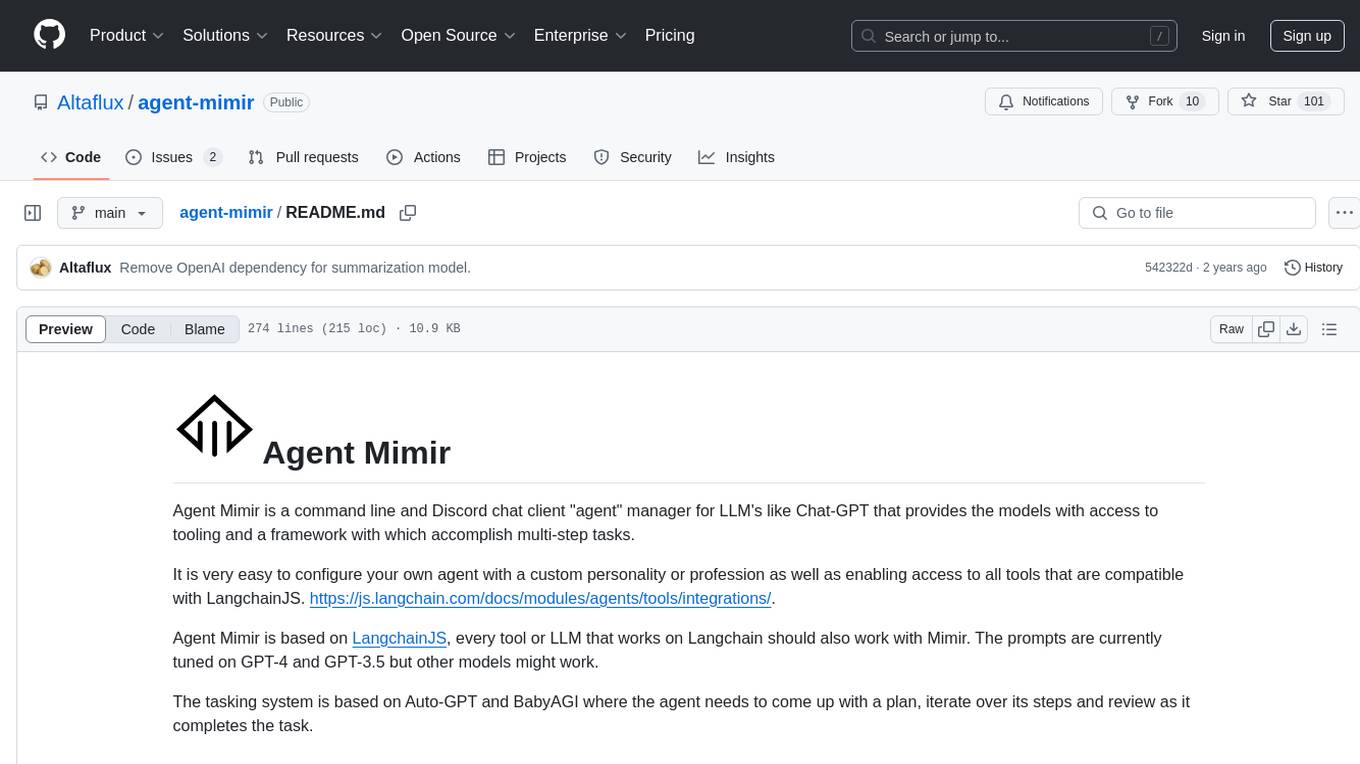
agent-mimir
Agent Mimir is a command line and Discord chat client 'agent' manager for LLM's like Chat-GPT that provides the models with access to tooling and a framework with which accomplish multi-step tasks. It is easy to configure your own agent with a custom personality or profession as well as enabling access to all tools that are compatible with LangchainJS. Agent Mimir is based on LangchainJS, every tool or LLM that works on Langchain should also work with Mimir. The tasking system is based on Auto-GPT and BabyAGI where the agent needs to come up with a plan, iterate over its steps and review as it completes the task.
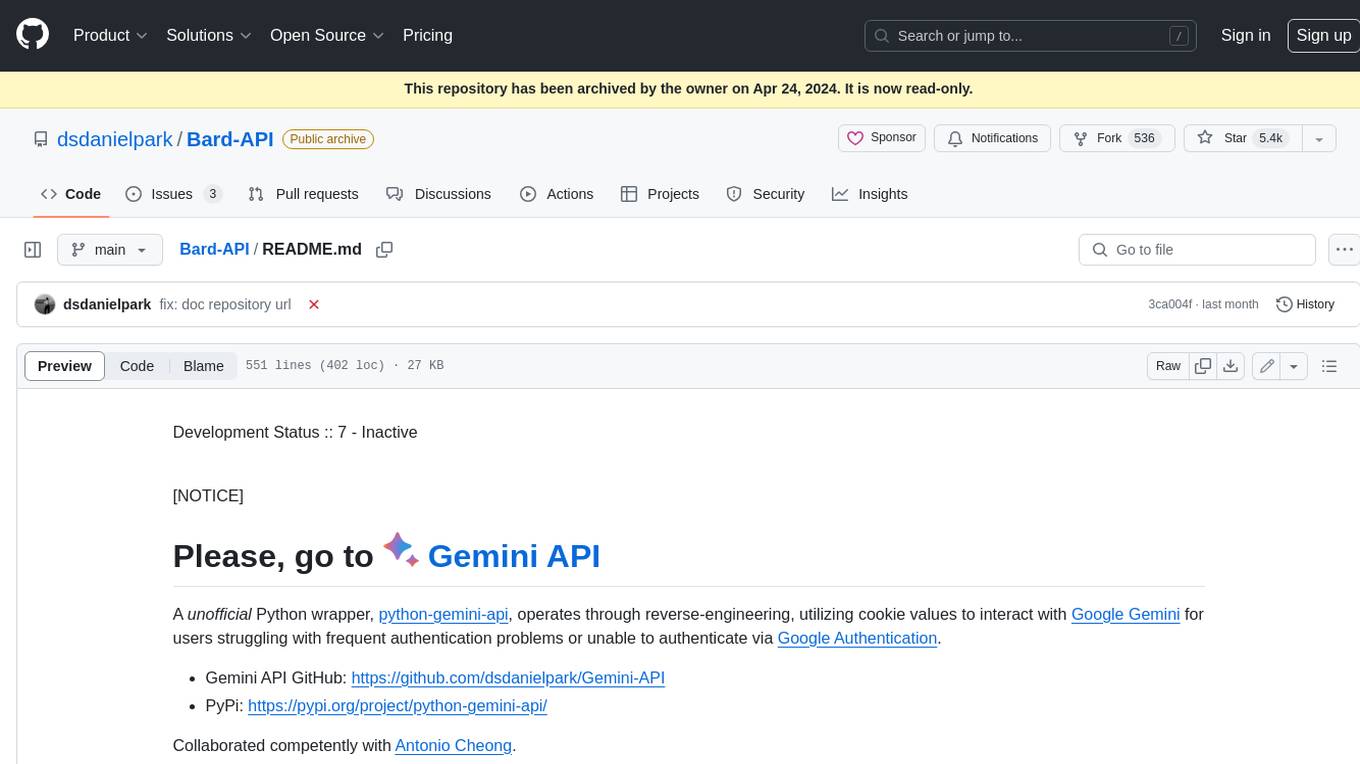
Bard-API
The Bard API is a Python package that returns responses from Google Bard through the value of a cookie. It is an unofficial API that operates through reverse-engineering, utilizing cookie values to interact with Google Bard for users struggling with frequent authentication problems or unable to authenticate via Google Authentication. The Bard API is not a free service, but rather a tool provided to assist developers with testing certain functionalities due to the delayed development and release of Google Bard's API. It has been designed with a lightweight structure that can easily adapt to the emergence of an official API. Therefore, using it for any other purposes is strongly discouraged. If you have access to a reliable official PaLM-2 API or Google Generative AI API, replace the provided response with the corresponding official code. Check out https://github.com/dsdanielpark/Bard-API/issues/262.
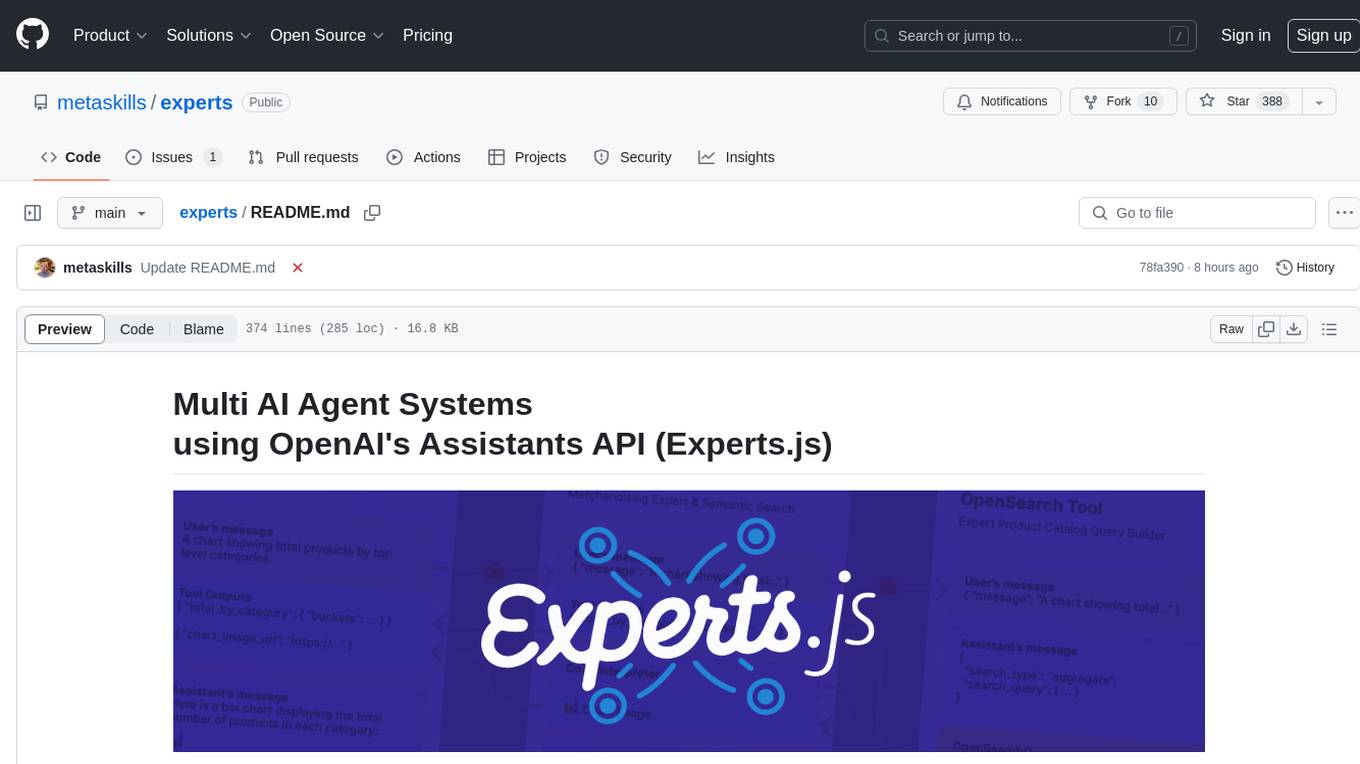
experts
Experts.js is a tool that simplifies the creation and deployment of OpenAI's Assistants, allowing users to link them together as Tools to create a Panel of Experts system with expanded memory and attention to detail. It leverages the new Assistants API from OpenAI, which offers advanced features such as referencing attached files & images as knowledge sources, supporting instructions up to 256,000 characters, integrating with 128 tools, and utilizing the Vector Store API for efficient file search. Experts.js introduces Assistants as Tools, enabling the creation of Multi AI Agent Systems where each Tool is an LLM-backed Assistant that can take on specialized roles or fulfill complex tasks.
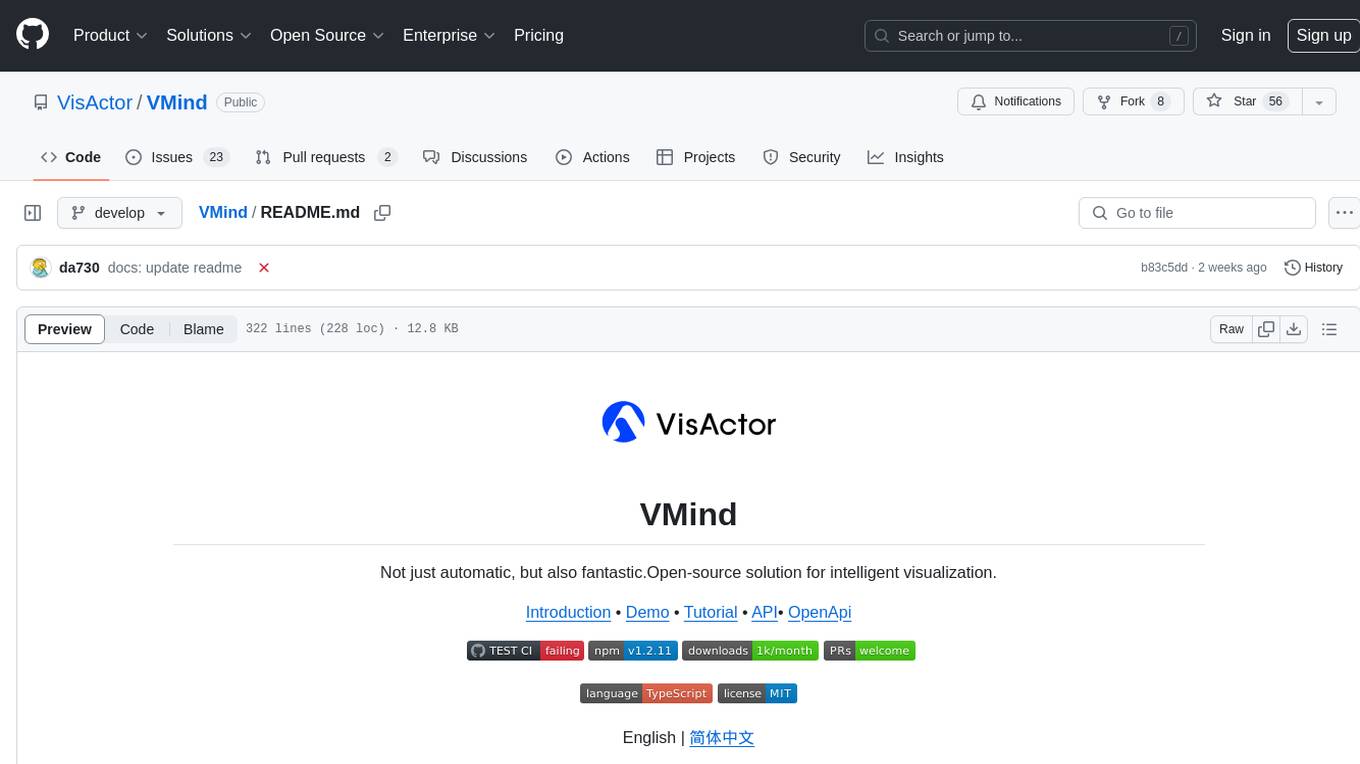
VMind
VMind is an open-source solution for intelligent visualization, providing an intelligent chart component based on LLM by VisActor. It allows users to create chart narrative works with natural language interaction, edit charts through dialogue, and export narratives as videos or GIFs. The tool is easy to use, scalable, supports various chart types, and offers one-click export functionality. Users can customize chart styles, specify themes, and aggregate data using LLM models. VMind aims to enhance efficiency in creating data visualization works through dialogue-based editing and natural language interaction.
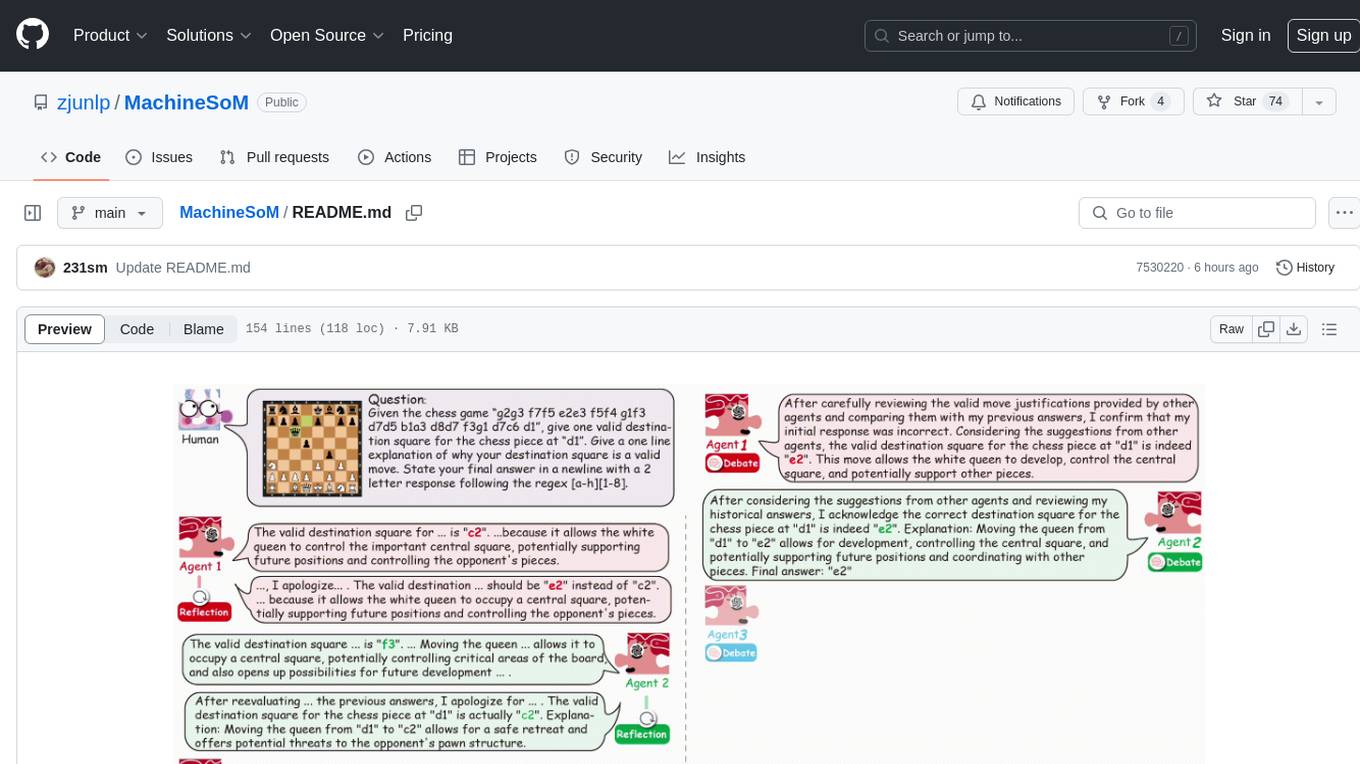
MachineSoM
MachineSoM is a code repository for the paper 'Exploring Collaboration Mechanisms for LLM Agents: A Social Psychology View'. It focuses on the emergence of intelligence from collaborative and communicative computational modules, enabling effective completion of complex tasks. The repository includes code for societies of LLM agents with different traits, collaboration processes such as debate and self-reflection, and interaction strategies for determining when and with whom to interact. It provides a coding framework compatible with various inference services like Replicate, OpenAI, Dashscope, and Anyscale, supporting models like Qwen and GPT. Users can run experiments, evaluate results, and draw figures based on the paper's content, with available datasets for MMLU, Math, and Chess Move Validity.
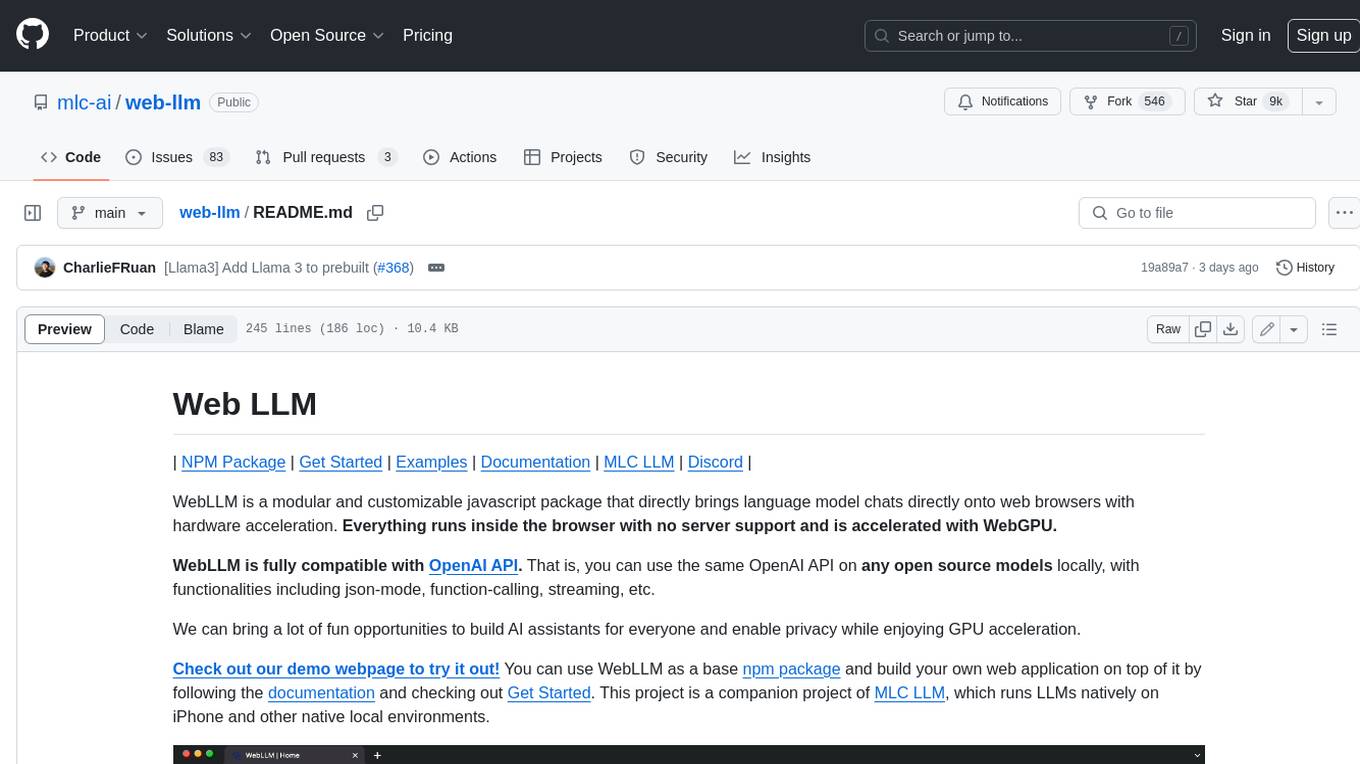
web-llm
WebLLM is a modular and customizable javascript package that directly brings language model chats directly onto web browsers with hardware acceleration. Everything runs inside the browser with no server support and is accelerated with WebGPU. WebLLM is fully compatible with OpenAI API. That is, you can use the same OpenAI API on any open source models locally, with functionalities including json-mode, function-calling, streaming, etc. We can bring a lot of fun opportunities to build AI assistants for everyone and enable privacy while enjoying GPU acceleration.
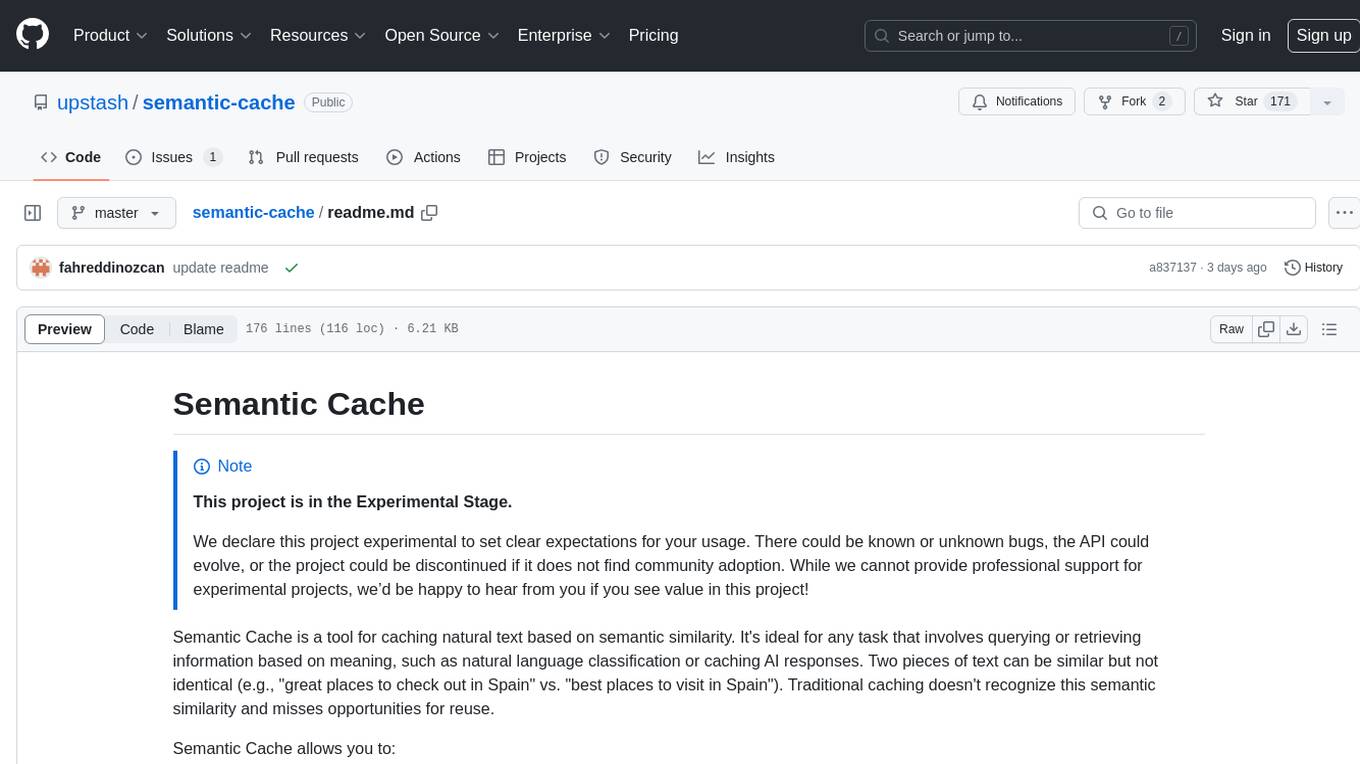
semantic-cache
Semantic Cache is a tool for caching natural text based on semantic similarity. It allows for classifying text into categories, caching AI responses, and reducing API latency by responding to similar queries with cached values. The tool stores cache entries by meaning, handles synonyms, supports multiple languages, understands complex queries, and offers easy integration with Node.js applications. Users can set a custom proximity threshold for filtering results. The tool is ideal for tasks involving querying or retrieving information based on meaning, such as natural language classification or caching AI responses.
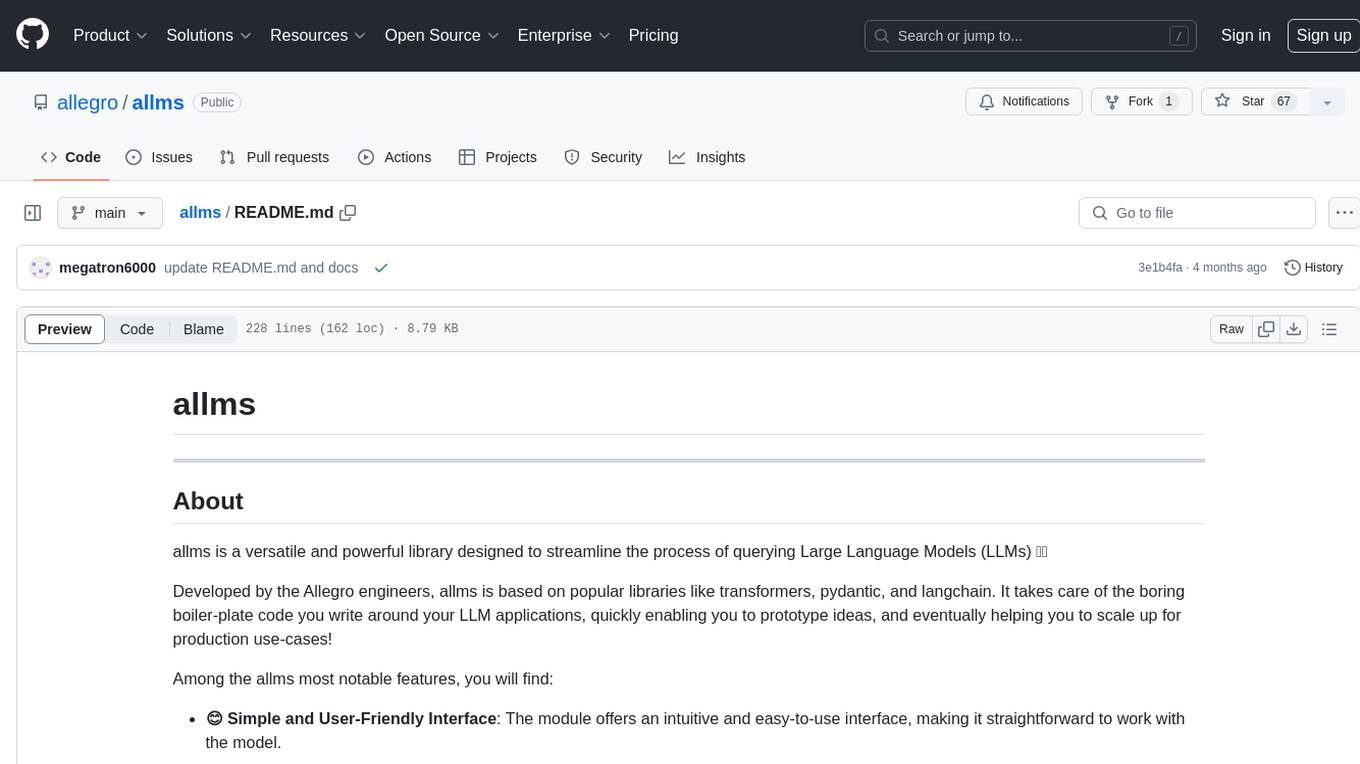
allms
allms is a versatile and powerful library designed to streamline the process of querying Large Language Models (LLMs). Developed by Allegro engineers, it simplifies working with LLM applications by providing a user-friendly interface, asynchronous querying, automatic retrying mechanism, error handling, and output parsing. It supports various LLM families hosted on different platforms like OpenAI, Google, Azure, and GCP. The library offers features for configuring endpoint credentials, batch querying with symbolic variables, and forcing structured output format. It also provides documentation, quickstart guides, and instructions for local development, testing, updating documentation, and making new releases.
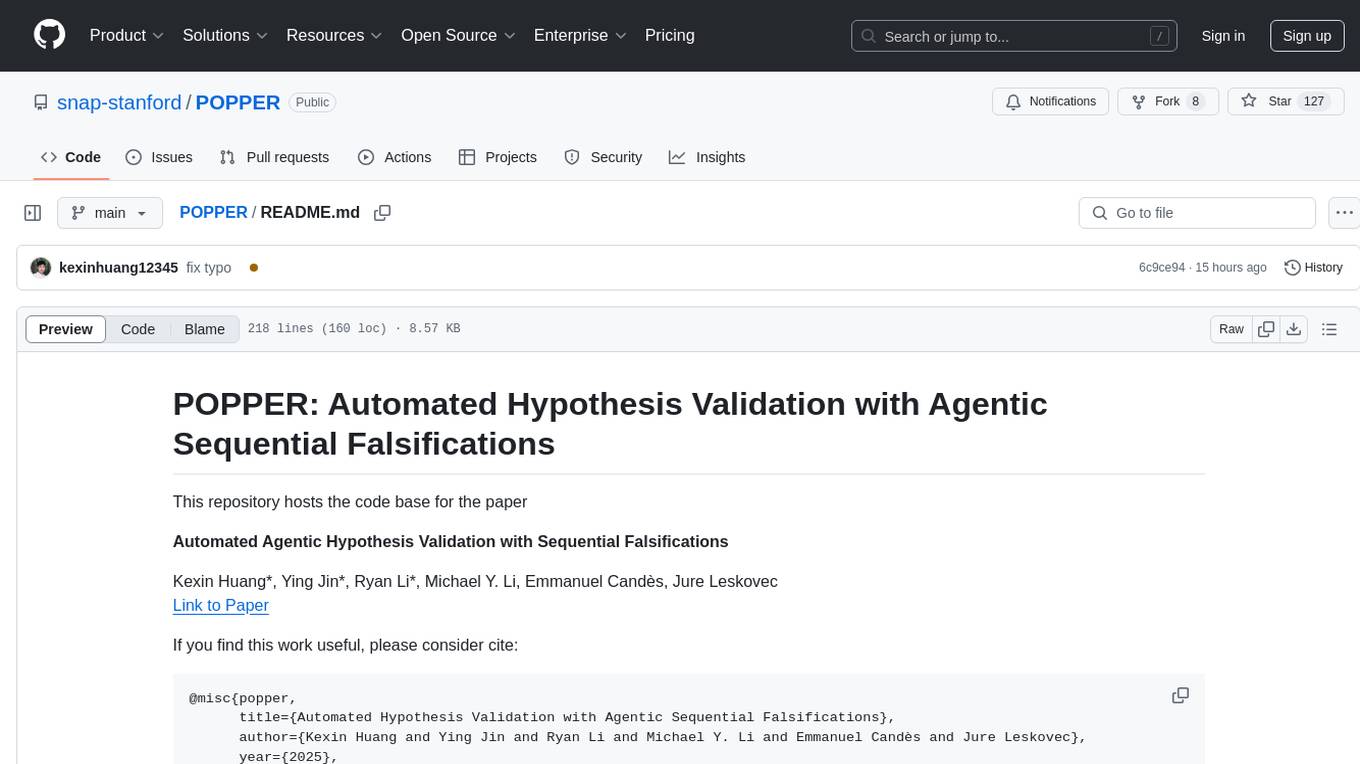
POPPER
Popper is an agentic framework for automated validation of free-form hypotheses using Large Language Models (LLMs). It follows Karl Popper's principle of falsification and designs falsification experiments to validate hypotheses. Popper ensures strict Type-I error control and actively gathers evidence from diverse observations. It delivers robust error control, high power, and scalability across various domains like biology, economics, and sociology. Compared to human scientists, Popper achieves comparable performance in validating complex biological hypotheses while reducing time by 10 folds, providing a scalable, rigorous solution for hypothesis validation.
For similar tasks
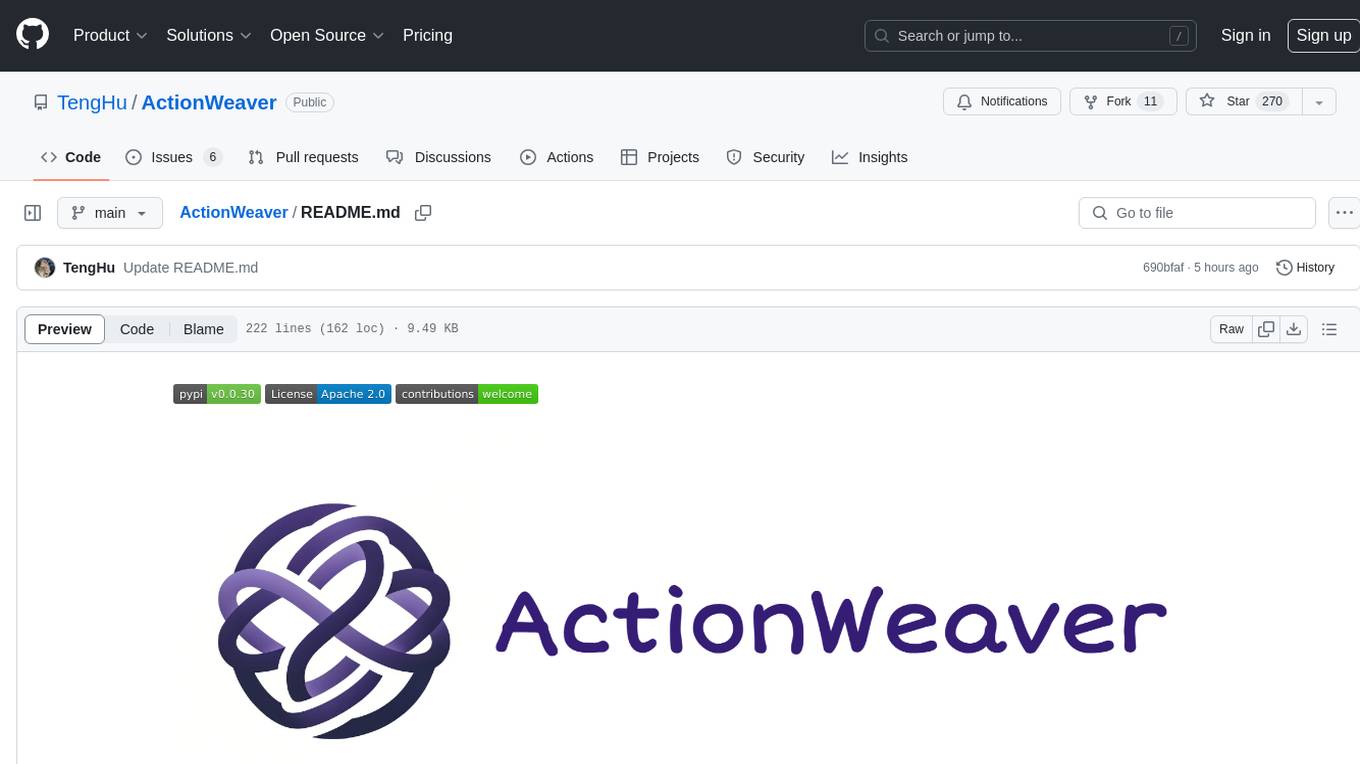
ActionWeaver
ActionWeaver is an AI application framework designed for simplicity, relying on OpenAI and Pydantic. It supports both OpenAI API and Azure OpenAI service. The framework allows for function calling as a core feature, extensibility to integrate any Python code, function orchestration for building complex call hierarchies, and telemetry and observability integration. Users can easily install ActionWeaver using pip and leverage its capabilities to create, invoke, and orchestrate actions with the language model. The framework also provides structured extraction using Pydantic models and allows for exception handling customization. Contributions to the project are welcome, and users are encouraged to cite ActionWeaver if found useful.
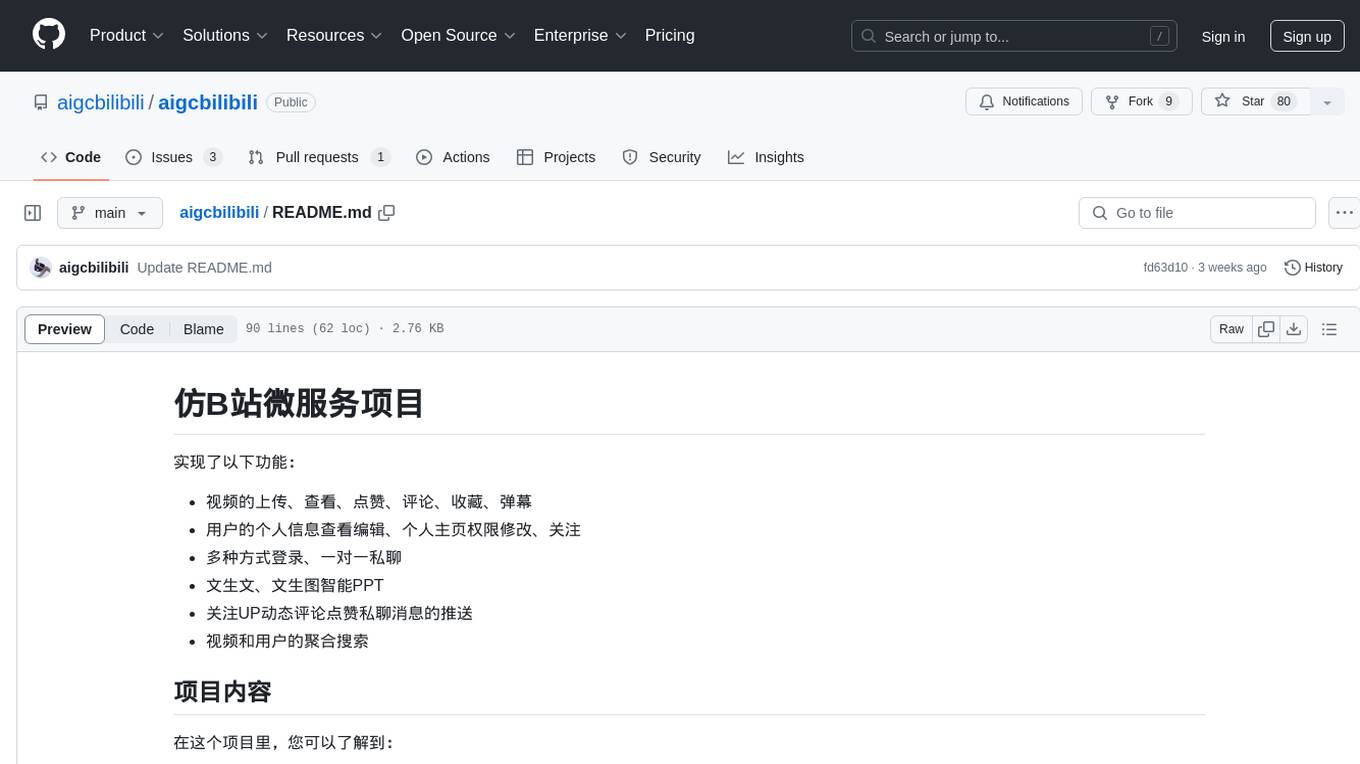
aigcbilibili
Aigcbilibili is a project that mimics the microservices of Bilibili, providing functionalities such as video uploading, viewing, liking, commenting, collecting, danmaku, user profile management, login methods, private messaging, intelligent PPT generation, dynamic updates, search aggregation, database operations with MyBatis-Plus, file management with MinIO, asynchronous tasks with CompletableFuture, JSON handling with FastJson, Gson, and Jackson, exception handling, logging management, file transfer, configuration management with Nacos, routing management with Gateway, authentication and authorization with Security + JWT, multiple login methods, caching with Redis, messaging with RocketMQ, search engine integration with Elasticsearch, data synchronization with XXL-Job, Redis, RocketMQ, Elasticsearch, cache implementation, real-time communication with WebSocket, distributed tracing with Sleuth + Zipkin, documentation and monitoring with Swagger, Druid, and intelligent content generation with Xunfei Xinghuo.
For similar jobs
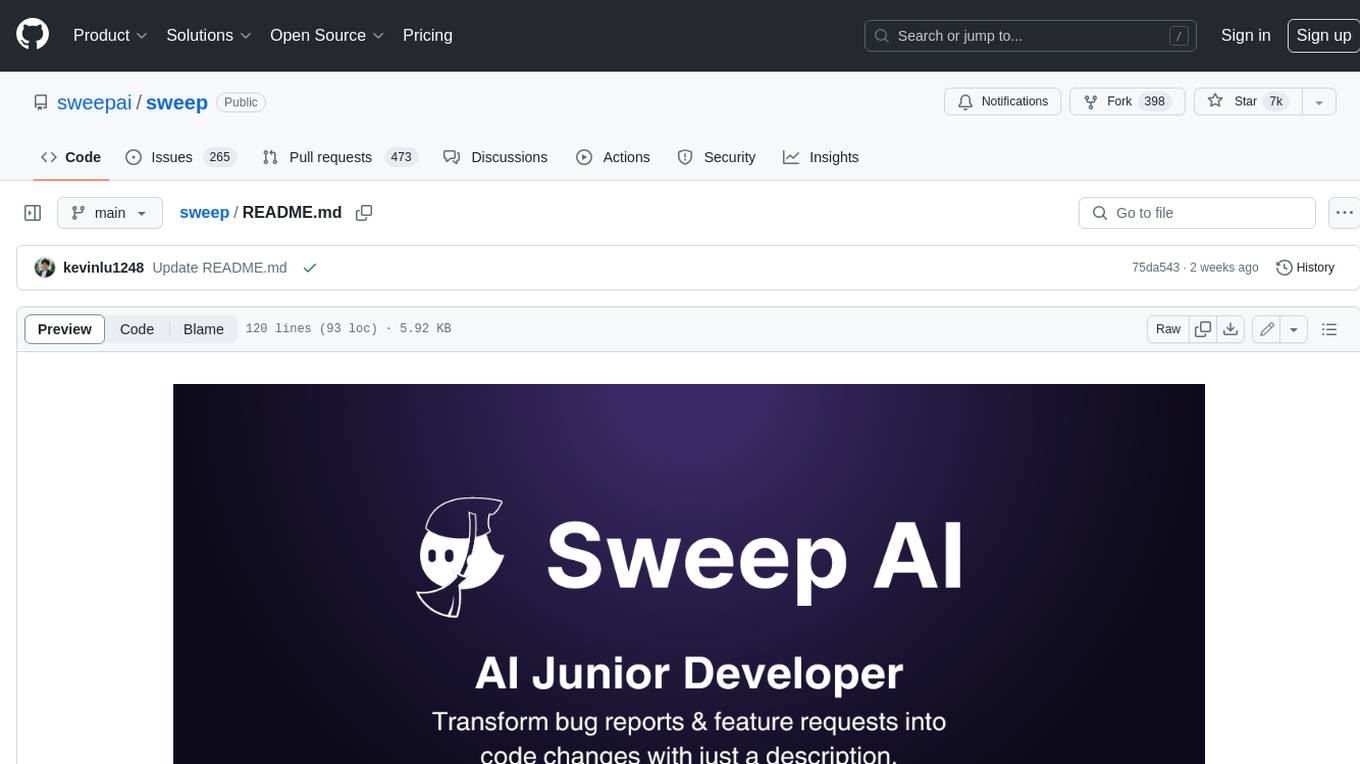
sweep
Sweep is an AI junior developer that turns bugs and feature requests into code changes. It automatically handles developer experience improvements like adding type hints and improving test coverage.
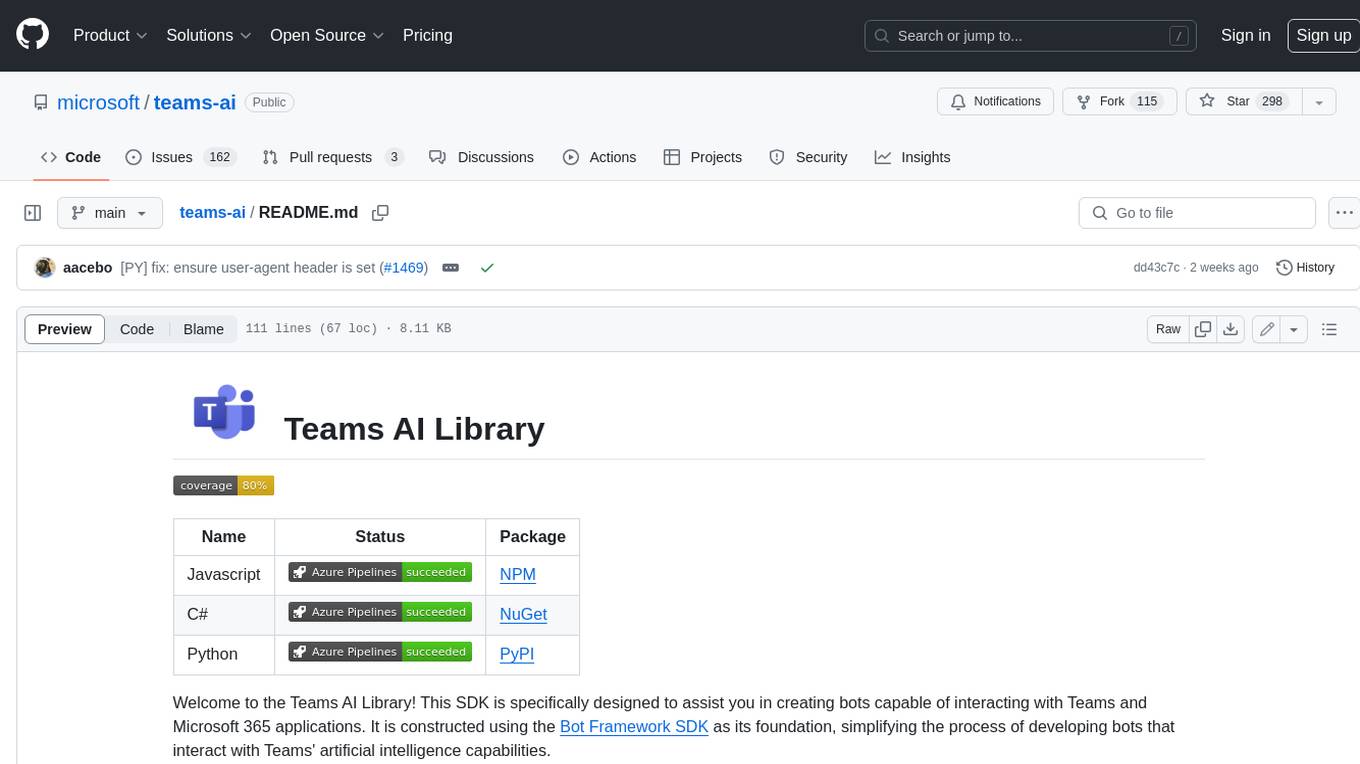
teams-ai
The Teams AI Library is a software development kit (SDK) that helps developers create bots that can interact with Teams and Microsoft 365 applications. It is built on top of the Bot Framework SDK and simplifies the process of developing bots that interact with Teams' artificial intelligence capabilities. The SDK is available for JavaScript/TypeScript, .NET, and Python.
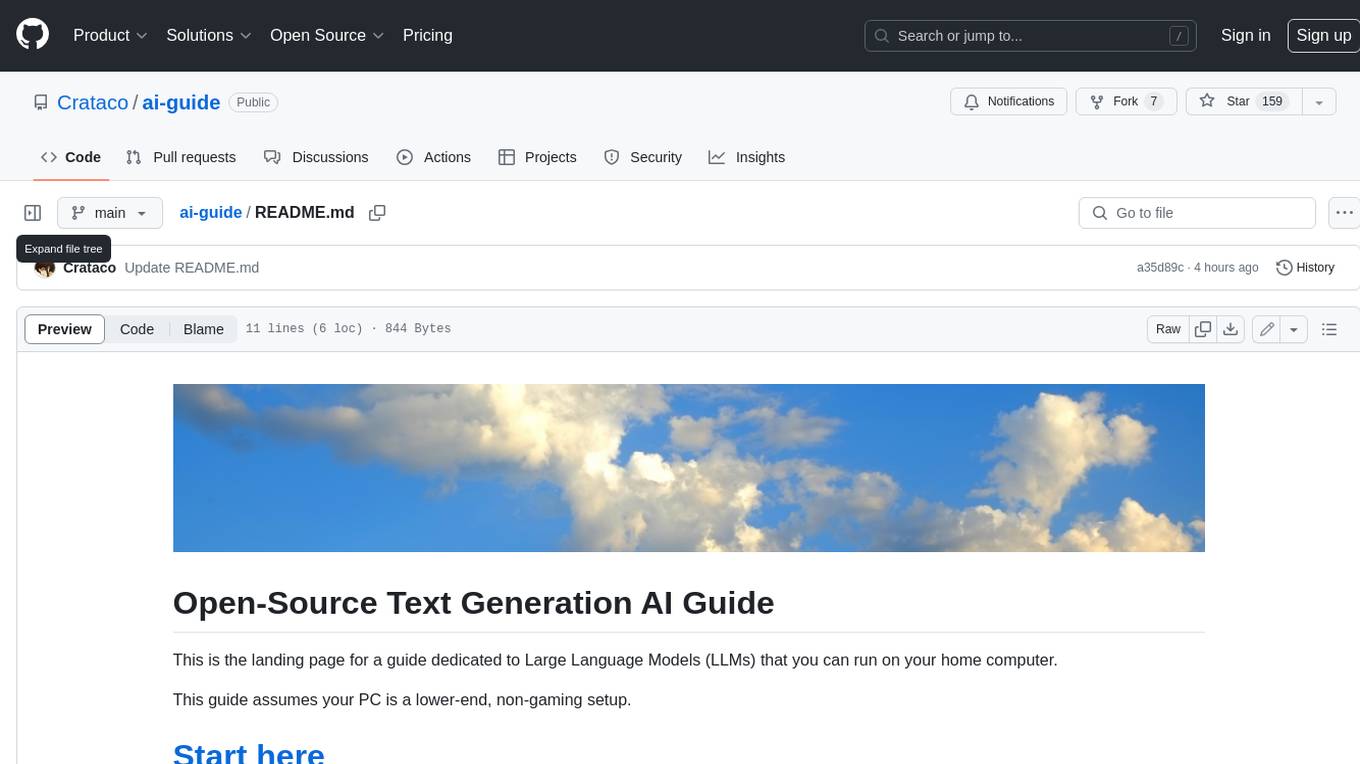
ai-guide
This guide is dedicated to Large Language Models (LLMs) that you can run on your home computer. It assumes your PC is a lower-end, non-gaming setup.
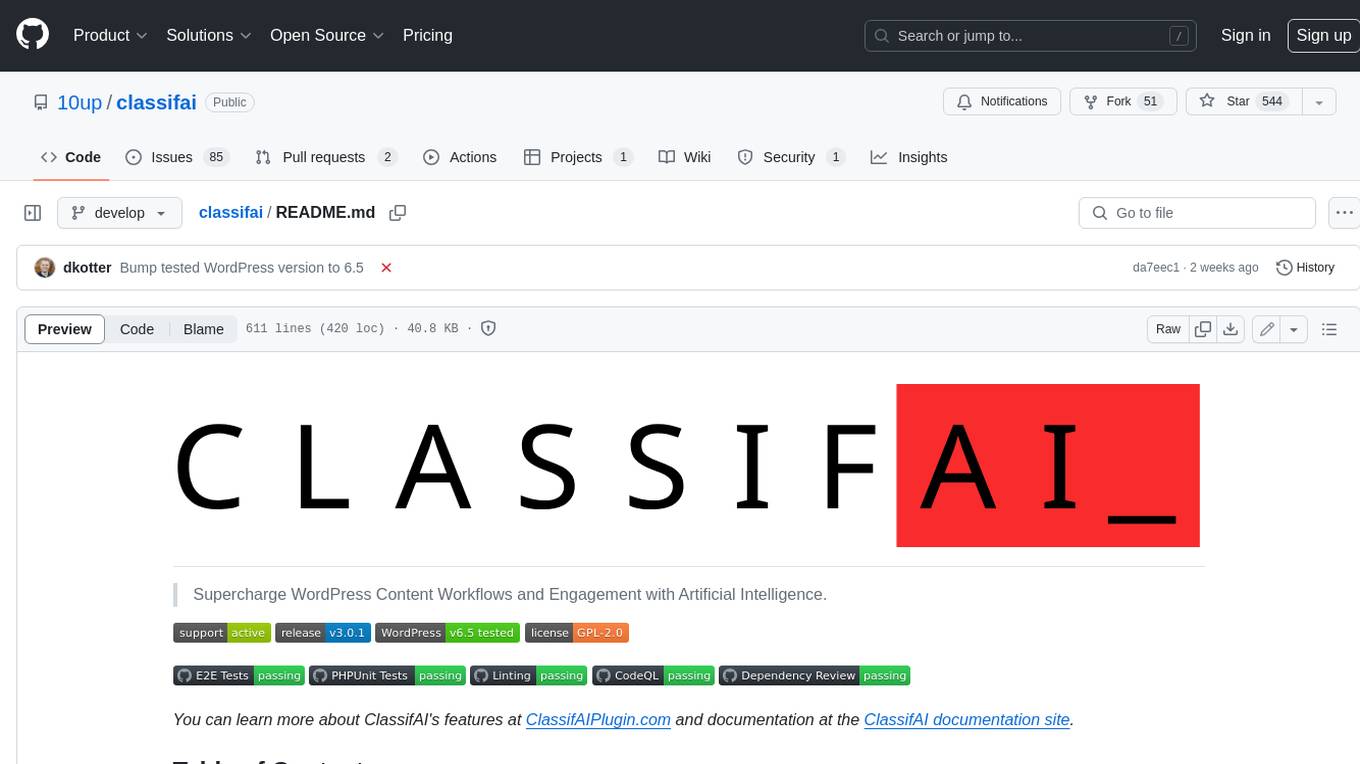
classifai
Supercharge WordPress Content Workflows and Engagement with Artificial Intelligence. Tap into leading cloud-based services like OpenAI, Microsoft Azure AI, Google Gemini and IBM Watson to augment your WordPress-powered websites. Publish content faster while improving SEO performance and increasing audience engagement. ClassifAI integrates Artificial Intelligence and Machine Learning technologies to lighten your workload and eliminate tedious tasks, giving you more time to create original content that matters.
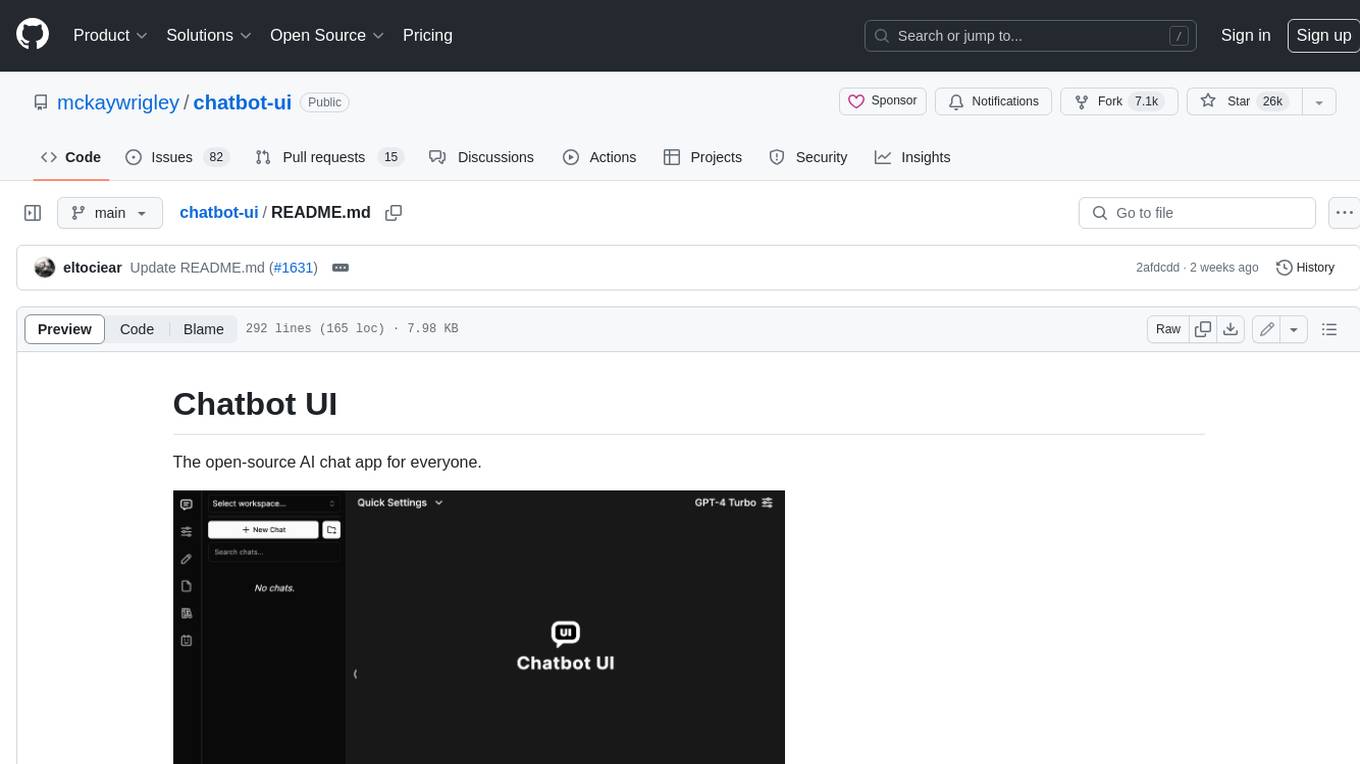
chatbot-ui
Chatbot UI is an open-source AI chat app that allows users to create and deploy their own AI chatbots. It is easy to use and can be customized to fit any need. Chatbot UI is perfect for businesses, developers, and anyone who wants to create a chatbot.
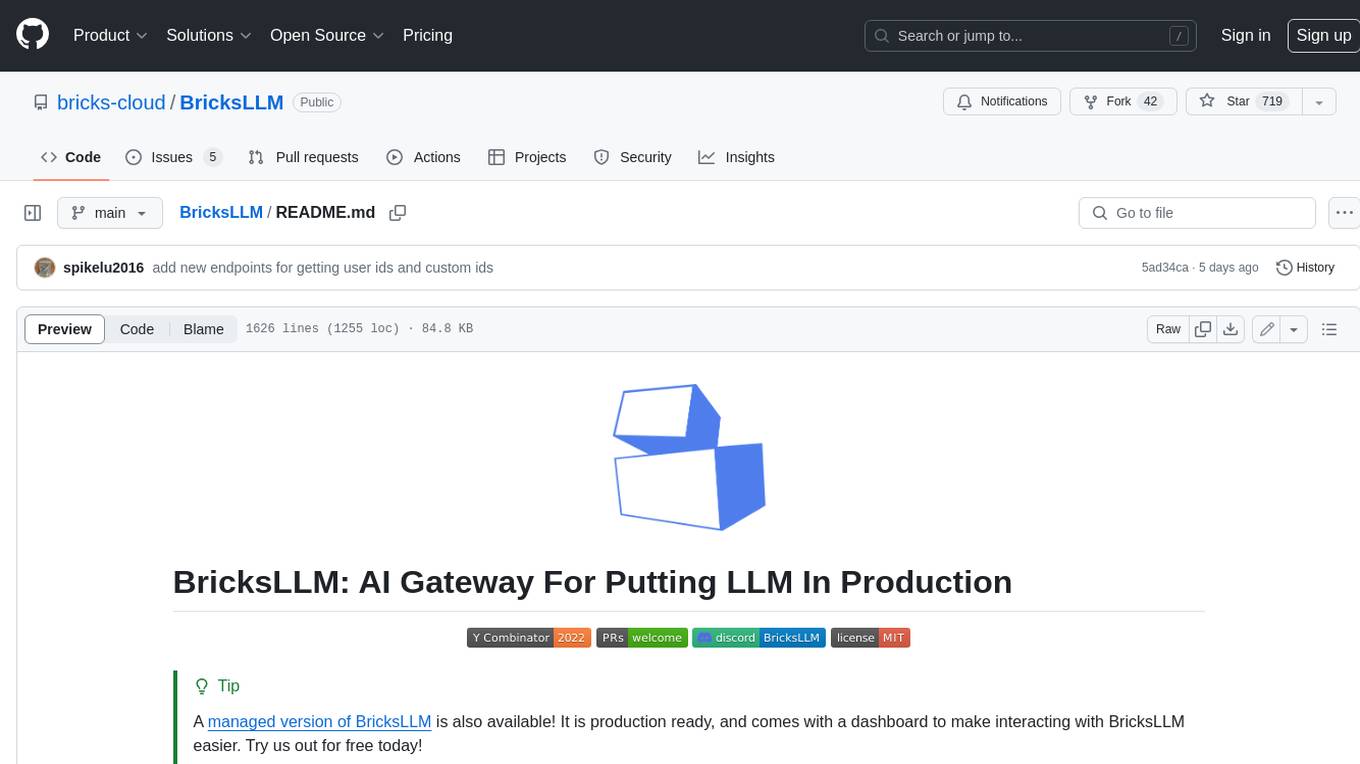
BricksLLM
BricksLLM is a cloud native AI gateway written in Go. Currently, it provides native support for OpenAI, Anthropic, Azure OpenAI and vLLM. BricksLLM aims to provide enterprise level infrastructure that can power any LLM production use cases. Here are some use cases for BricksLLM: * Set LLM usage limits for users on different pricing tiers * Track LLM usage on a per user and per organization basis * Block or redact requests containing PIIs * Improve LLM reliability with failovers, retries and caching * Distribute API keys with rate limits and cost limits for internal development/production use cases * Distribute API keys with rate limits and cost limits for students
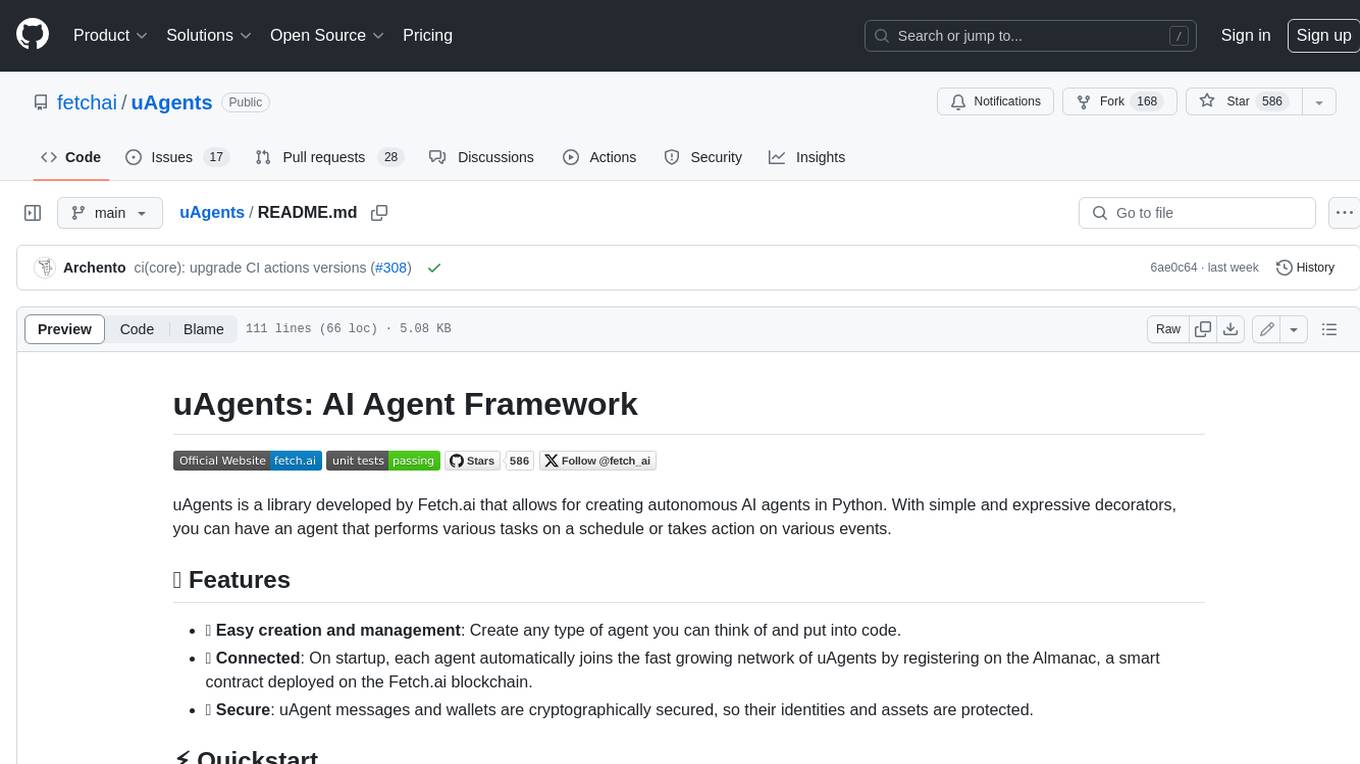
uAgents
uAgents is a Python library developed by Fetch.ai that allows for the creation of autonomous AI agents. These agents can perform various tasks on a schedule or take action on various events. uAgents are easy to create and manage, and they are connected to a fast-growing network of other uAgents. They are also secure, with cryptographically secured messages and wallets.
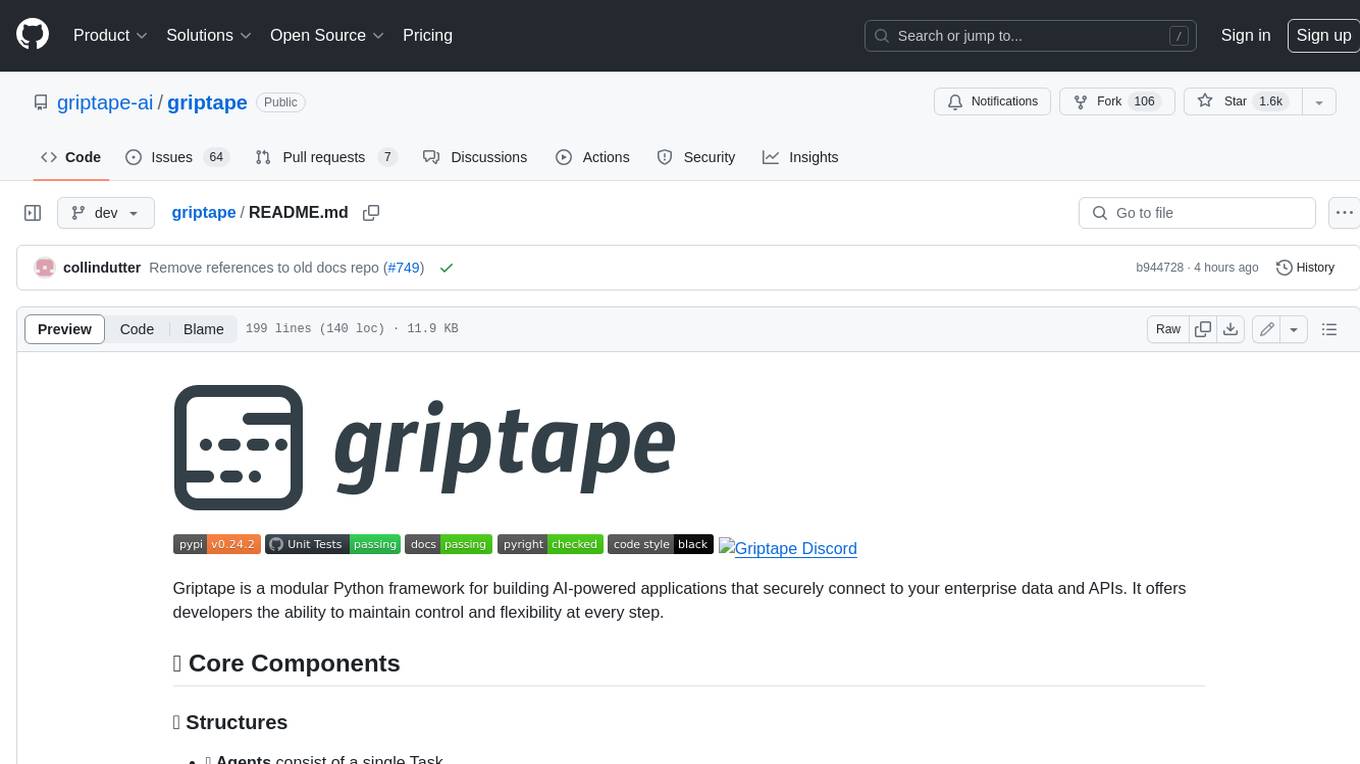
griptape
Griptape is a modular Python framework for building AI-powered applications that securely connect to your enterprise data and APIs. It offers developers the ability to maintain control and flexibility at every step. Griptape's core components include Structures (Agents, Pipelines, and Workflows), Tasks, Tools, Memory (Conversation Memory, Task Memory, and Meta Memory), Drivers (Prompt and Embedding Drivers, Vector Store Drivers, Image Generation Drivers, Image Query Drivers, SQL Drivers, Web Scraper Drivers, and Conversation Memory Drivers), Engines (Query Engines, Extraction Engines, Summary Engines, Image Generation Engines, and Image Query Engines), and additional components (Rulesets, Loaders, Artifacts, Chunkers, and Tokenizers). Griptape enables developers to create AI-powered applications with ease and efficiency.