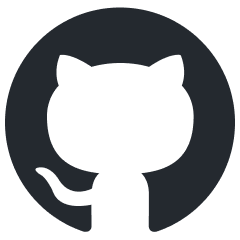
neo4j-graphrag-python
Neo4j GraphRAG for Python
Stars: 162
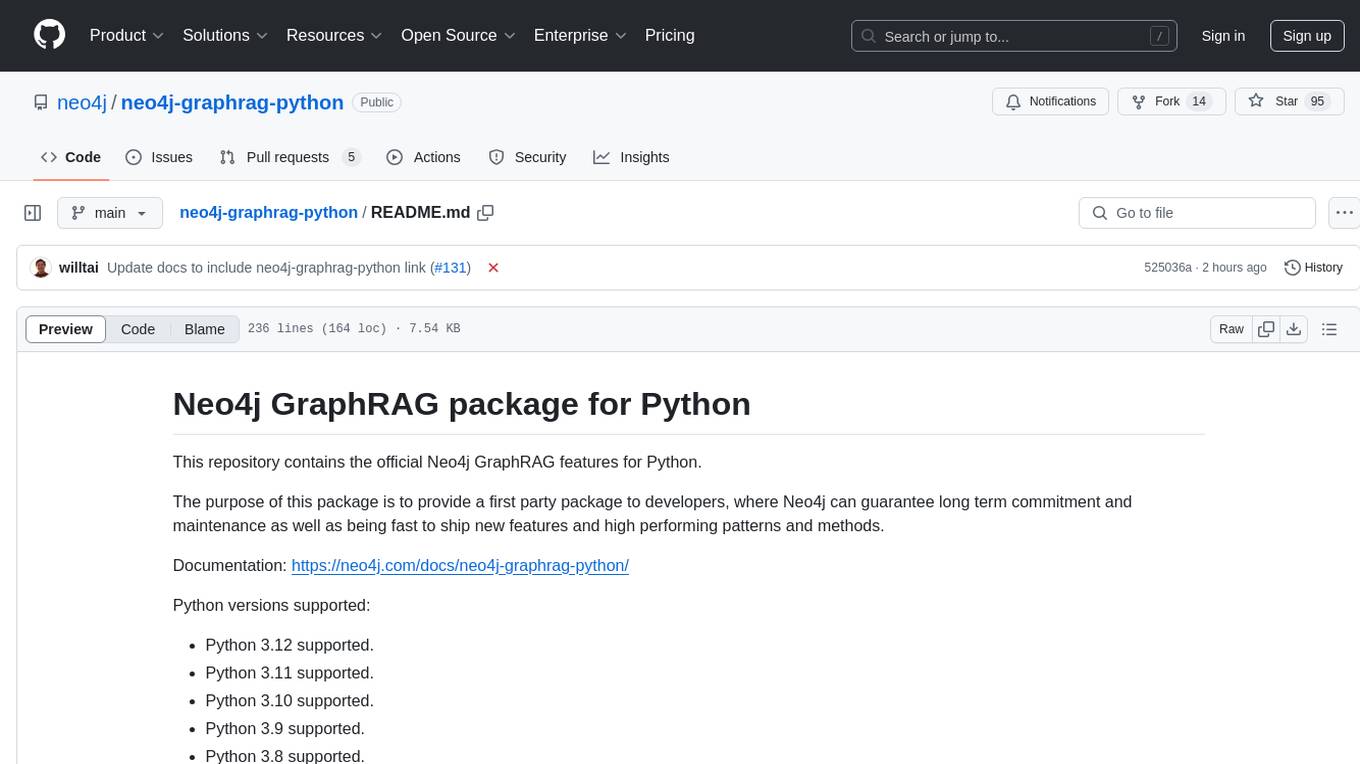
The Neo4j GraphRAG package for Python is an official repository that provides features for creating and managing vector indexes in Neo4j databases. It aims to offer developers a reliable package with long-term commitment, maintenance, and fast feature updates. The package supports various Python versions and includes functionalities for creating vector indexes, populating them, and performing similarity searches. It also provides guidelines for installation, examples, and development processes such as installing dependencies, making changes, and running tests.
README:
The official Neo4j GraphRAG package for Python enables developers to build graph retrieval augmented generation (GraphRAG) applications using the power of Neo4j and Python. As a first-party library, it offers a robust, feature-rich, and high-performance solution, with the added assurance of long-term support and maintenance directly from Neo4j.
Documentation can be found here
Version | Supported? |
---|---|
3.12 | โ |
3.11 | โ |
3.10 | โ |
3.9 | โ |
3.8 | โ |
To install the latest stable version, run:
pip install neo4j-graphrag
pygraphviz
is used for visualizing pipelines.
Installation instructions can be found here.
The scripts below demonstrate how to get started with the package and make use of its key features.
To run these examples, ensure that you have a Neo4j instance up and running and update the NEO4J_URI
, NEO4J_USERNAME
, and NEO4J_PASSWORD
variables in each script with the details of your Neo4j instance.
For the examples, make sure to export your OpenAI key as an environment variable named OPENAI_API_KEY
.
Additional examples are available in the examples
folder.
NOTE: The APOC core library must be installed in your Neo4j instance in order to use this feature
This package offers two methods for constructing a knowledge graph.
The Pipeline
class provides extensive customization options, making it ideal for advanced use cases.
See the examples/pipeline
folder for examples of how to use this class.
For a more streamlined approach, the SimpleKGPipeline
class offers a simplified abstraction layer over the Pipeline
, making it easier to build knowledge graphs.
Both classes support working directly with text and PDFs.
import asyncio
from neo4j import GraphDatabase
from neo4j_graphrag.embeddings import OpenAIEmbeddings
from neo4j_graphrag.experimental.pipeline.kg_builder import SimpleKGPipeline
from neo4j_graphrag.llm.openai_llm import OpenAILLM
NEO4J_URI = "neo4j://localhost:7687"
NEO4J_USERNAME = "neo4j"
NEO4J_PASSWORD = "password"
# Connect to the Neo4j database
driver = GraphDatabase.driver(NEO4J_URI, auth=(NEO4J_USERNAME, NEO4J_PASSWORD))
# List the entities and relations the LLM should look for in the text
entities = ["Person", "House", "Planet"]
relations = ["PARENT_OF", "HEIR_OF", "RULES"]
potential_schema = [
("Person", "PARENT_OF", "Person"),
("Person", "HEIR_OF", "House"),
("House", "RULES", "Planet"),
]
# Create an Embedder object
embedder = OpenAIEmbeddings(model="text-embedding-3-large")
# Instantiate the LLM
llm = OpenAILLM(
model_name="gpt-4o",
model_params={
"max_tokens": 2000,
"response_format": {"type": "json_object"},
"temperature": 0,
},
)
# Instantiate the SimpleKGPipeline
kg_builder = SimpleKGPipeline(
llm=llm,
driver=driver,
embedder=embedder,
entities=entities,
relations=relations,
on_error="IGNORE",
from_pdf=False,
)
# Run the pipeline on a piece of text
text = (
"The son of Duke Leto Atreides and the Lady Jessica, Paul is the heir of House "
"Atreides, an aristocratic family that rules the planet Caladan."
)
asyncio.run(kg_builder.run_async(text=text))
driver.close()
Example knowledge graph created using the above script:
When creating a vector index, make sure you match the number of dimensions in the index with the number of dimensions your embeddings have.
from neo4j import GraphDatabase
from neo4j_graphrag.indexes import create_vector_index
NEO4J_URI = "neo4j://localhost:7687"
NEO4J_USERNAME = "neo4j"
NEO4J_PASSWORD = "password"
INDEX_NAME = "vector-index-name"
# Connect to the Neo4j database
driver = GraphDatabase.driver(NEO4J_URI, auth=(NEO4J_USERNAME, NEO4J_PASSWORD))
# Create the index
create_vector_index(
driver,
INDEX_NAME,
label="Chunk",
embedding_property="embedding",
dimensions=3072,
similarity_fn="euclidean",
)
driver.close()
This example demonstrates one method for upserting data in your Neo4j database. It's important to note that there are alternative approaches, such as using the Neo4j Python driver.
Ensure that your vector index is created prior to executing this example.
from neo4j import GraphDatabase
from neo4j_graphrag.embeddings import OpenAIEmbeddings
from neo4j_graphrag.indexes import upsert_vector
NEO4J_URI = "neo4j://localhost:7687"
NEO4J_USERNAME = "neo4j"
NEO4J_PASSWORD = "password"
# Connect to the Neo4j database
driver = GraphDatabase.driver(NEO4J_URI, auth=(NEO4J_USERNAME, NEO4J_PASSWORD))
# Create an Embedder object
embedder = OpenAIEmbeddings(model="text-embedding-3-large")
# Generate an embedding for some text
text = (
"The son of Duke Leto Atreides and the Lady Jessica, Paul is the heir of House "
"Atreides, an aristocratic family that rules the planet Caladan."
)
vector = embedder.embed_query(text)
# Upsert the vector
upsert_vector(
driver,
node_id=0,
embedding_property="embedding",
vector=vector,
)
driver.close()
Please note that when querying a Neo4j vector index approximate nearest neighbor search is used, which may not always deliver exact results. For more information, refer to the Neo4j documentation on limitations and issues of vector indexes.
In the example below, we perform a simple vector search using a retriever that conducts a similarity search over the vector-index-name
vector index.
This library provides more retrievers beyond just the VectorRetriever
.
See the examples
folder for examples of how to use these retrievers.
Before running this example, make sure your vector index has been created and populated.
from neo4j import GraphDatabase
from neo4j_graphrag.embeddings import OpenAIEmbeddings
from neo4j_graphrag.generation import GraphRAG
from neo4j_graphrag.llm import OpenAILLM
from neo4j_graphrag.retrievers import VectorRetriever
NEO4J_URI = "neo4j://localhost:7687"
NEO4J_USERNAME = "neo4j"
NEO4J_PASSWORD = "password"
INDEX_NAME = "vector-index-name"
# Connect to the Neo4j database
driver = GraphDatabase.driver(NEO4J_URI, auth=(NEO4J_USERNAME, NEO4J_PASSWORD))
# Create an Embedder object
embedder = OpenAIEmbeddings(model="text-embedding-3-large")
# Initialize the retriever
retriever = VectorRetriever(driver, INDEX_NAME, embedder)
# Instantiate the LLM
llm = OpenAILLM(model_name="gpt-4o", model_params={"temperature": 0})
# Instantiate the RAG pipeline
rag = GraphRAG(retriever=retriever, llm=llm)
# Query the graph
query_text = "Who is Paul Atreides?"
response = rag.search(query_text=query_text, retriever_config={"top_k": 5})
print(response.answer)
driver.close()
You must sign the contributors license agreement in order to make contributions to this project.
Our Python dependencies are managed using Poetry. If Poetry is not yet installed on your system, you can follow the instructions here to set it up. To begin development on this project, start by cloning the repository and then install all necessary dependencies, including the development dependencies, with the following command:
poetry install --with dev
If you have a bug to report or feature to request, first search to see if an issue already exists. If a related issue doesn't exist, please raise a new issue using the issue form.
If you're a Neo4j Enterprise customer, you can also reach out to Customer Support.
If you don't have a bug to report or feature request, but you need a hand with the library; community support is available via Neo4j Online Community and/or Discord.
- Fork the repository.
- Install Python and Poetry.
- Create a working branch from
main
and start with your changes!
Our codebase follows strict formatting and linting standards using Ruff for code quality checks and Mypy for type checking. Before contributing, ensure that all code is properly formatted, free of linting issues, and includes accurate type annotations.
Adherence to these standards is required for contributions to be accepted.
We recommend setting up pre-commit to automate code quality checks. This ensures your changes meet our guidelines before committing.
-
Install pre-commit by following the installation guide.
-
Set up the pre-commit hooks by running:
pre-commit install
-
To manually check if a file meets the quality requirements, run:
pre-commit run --file path/to/file
When you're finished with your changes, create a pull request (PR) using the following workflow.
- Ensure you have formatted and linted your code.
- Ensure that you have signed the CLA.
- Ensure that the base of your PR is set to
main
. - Don't forget to link your PR to an issue if you are solving one.
- Check the checkbox to allow maintainer edits so that maintainers can make any necessary tweaks and update your branch for merge.
- Reviewers may ask for changes to be made before a PR can be merged, either using suggested changes or normal pull request comments. You can apply suggested changes directly through the UI. Any other changes can be made in your fork and committed to the PR branch.
- As you update your PR and apply changes, mark each conversation as resolved.
- Update the
CHANGELOG.md
if you have made significant changes to the project, these include:- Major changes:
- New features
- Bug fixes with high impact
- Breaking changes
- Minor changes:
- Documentation improvements
- Code refactoring without functional impact
- Minor bug fixes
- Major changes:
- Keep
CHANGELOG.md
changes brief and focus on the most important changes.
- You can automatically generate a changelog suggestion for your PR by commenting on it using CodiumAI:
@CodiumAI-Agent /update_changelog
- Edit the suggestion if necessary and update the appropriate subsection in the
CHANGELOG.md
file under 'Next'. - Commit the changes.
Install the project dependencies then run the following command to run the unit tests locally:
poetry run pytest tests/unit
To execute end-to-end (e2e) tests, you need the following services to be running locally:
- neo4j
- weaviate
- weaviate-text2vec-transformers
The simplest way to set these up is by using Docker Compose:
docker compose -f tests/e2e/docker-compose.yml up
(tip: If you encounter any caching issues within the databases, you can completely remove them by running docker compose -f tests/e2e/docker-compose.yml down
)
Once all the services are running, execute the following command to run the e2e tests:
poetry run pytest tests/e2e
For Tasks:
Click tags to check more tools for each tasksFor Jobs:
Alternative AI tools for neo4j-graphrag-python
Similar Open Source Tools
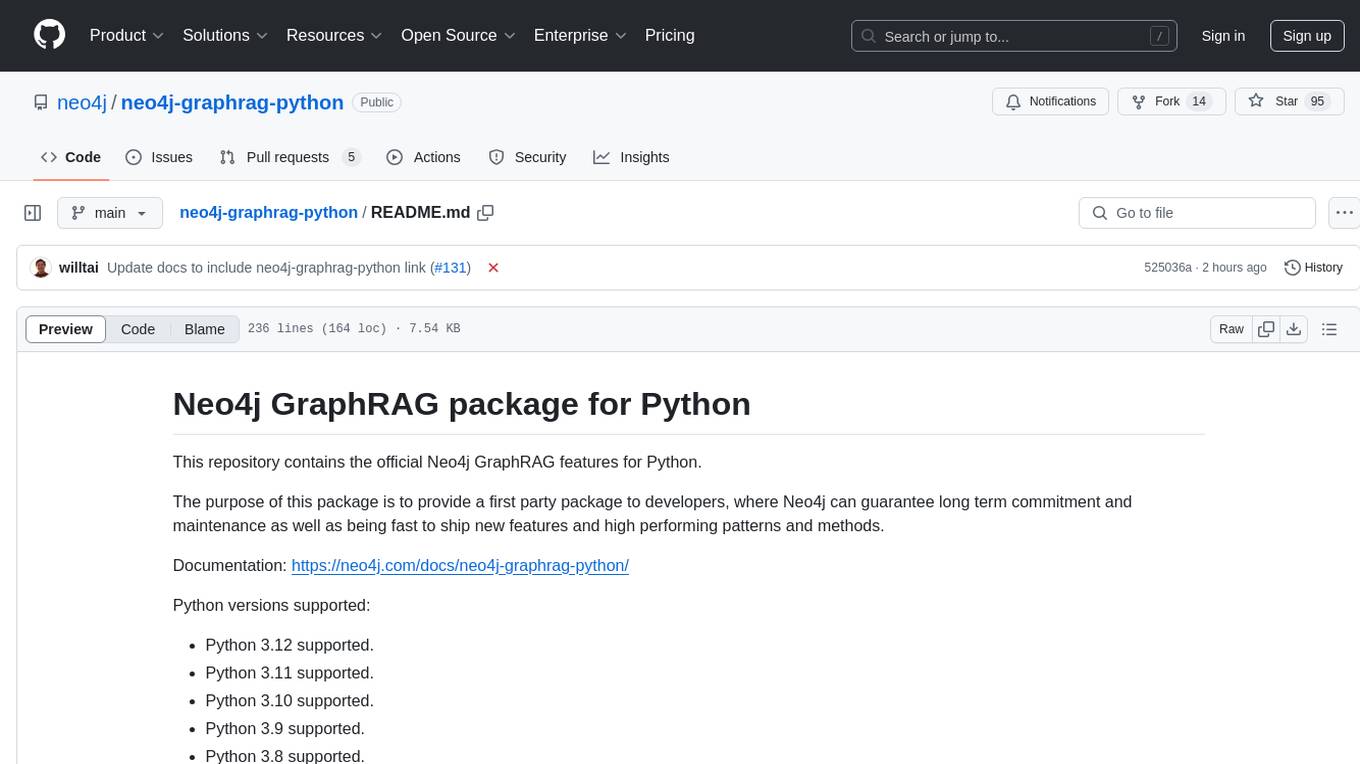
neo4j-graphrag-python
The Neo4j GraphRAG package for Python is an official repository that provides features for creating and managing vector indexes in Neo4j databases. It aims to offer developers a reliable package with long-term commitment, maintenance, and fast feature updates. The package supports various Python versions and includes functionalities for creating vector indexes, populating them, and performing similarity searches. It also provides guidelines for installation, examples, and development processes such as installing dependencies, making changes, and running tests.
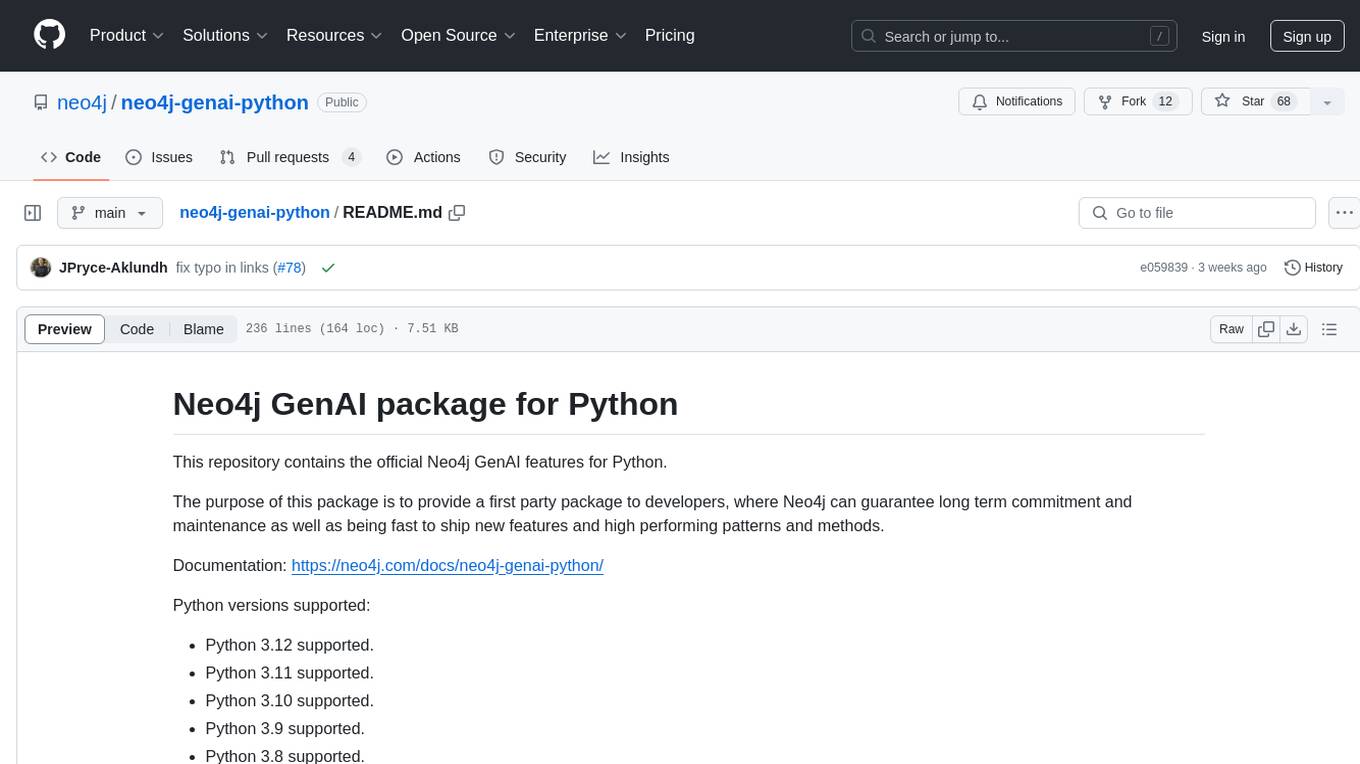
neo4j-genai-python
This repository contains the official Neo4j GenAI features for Python. The purpose of this package is to provide a first-party package to developers, where Neo4j can guarantee long-term commitment and maintenance as well as being fast to ship new features and high-performing patterns and methods.
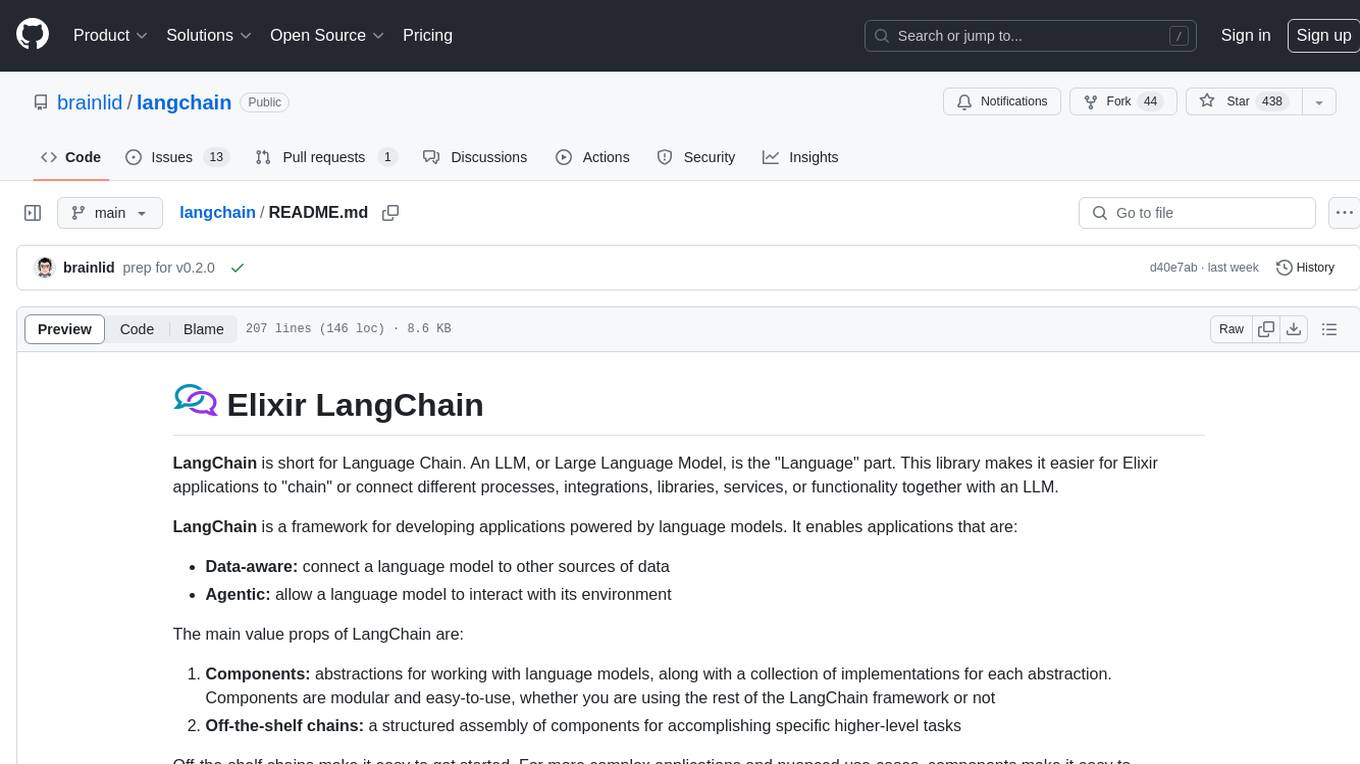
langchain
LangChain is a framework for developing Elixir applications powered by language models. It enables applications to connect language models to other data sources and interact with the environment. The library provides components for working with language models and off-the-shelf chains for specific tasks. It aims to assist in building applications that combine large language models with other sources of computation or knowledge. LangChain is written in Elixir and is not aimed for parity with the JavaScript and Python versions due to differences in programming paradigms and design choices. The library is designed to make it easy to integrate language models into applications and expose features, data, and functionality to the models.
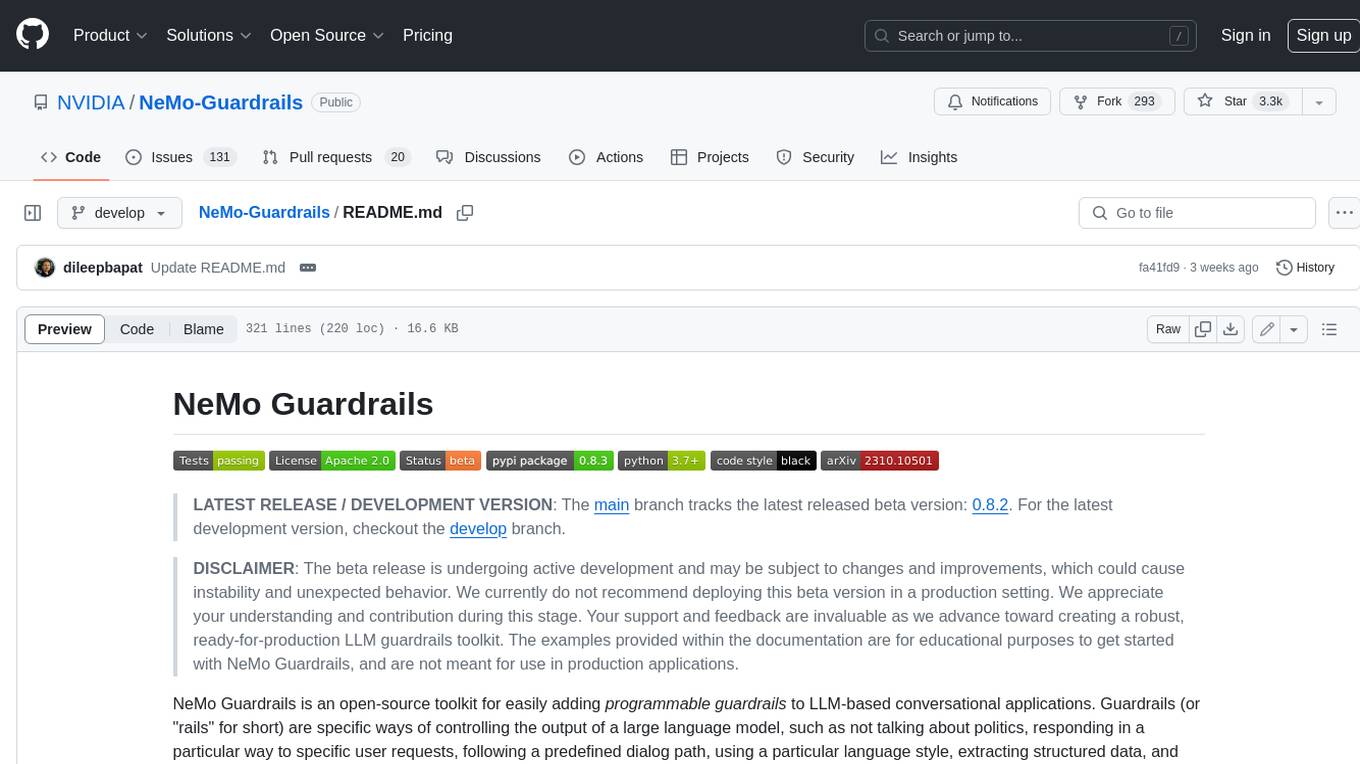
NeMo-Guardrails
NeMo Guardrails is an open-source toolkit for easily adding _programmable guardrails_ to LLM-based conversational applications. Guardrails (or "rails" for short) are specific ways of controlling the output of a large language model, such as not talking about politics, responding in a particular way to specific user requests, following a predefined dialog path, using a particular language style, extracting structured data, and more.
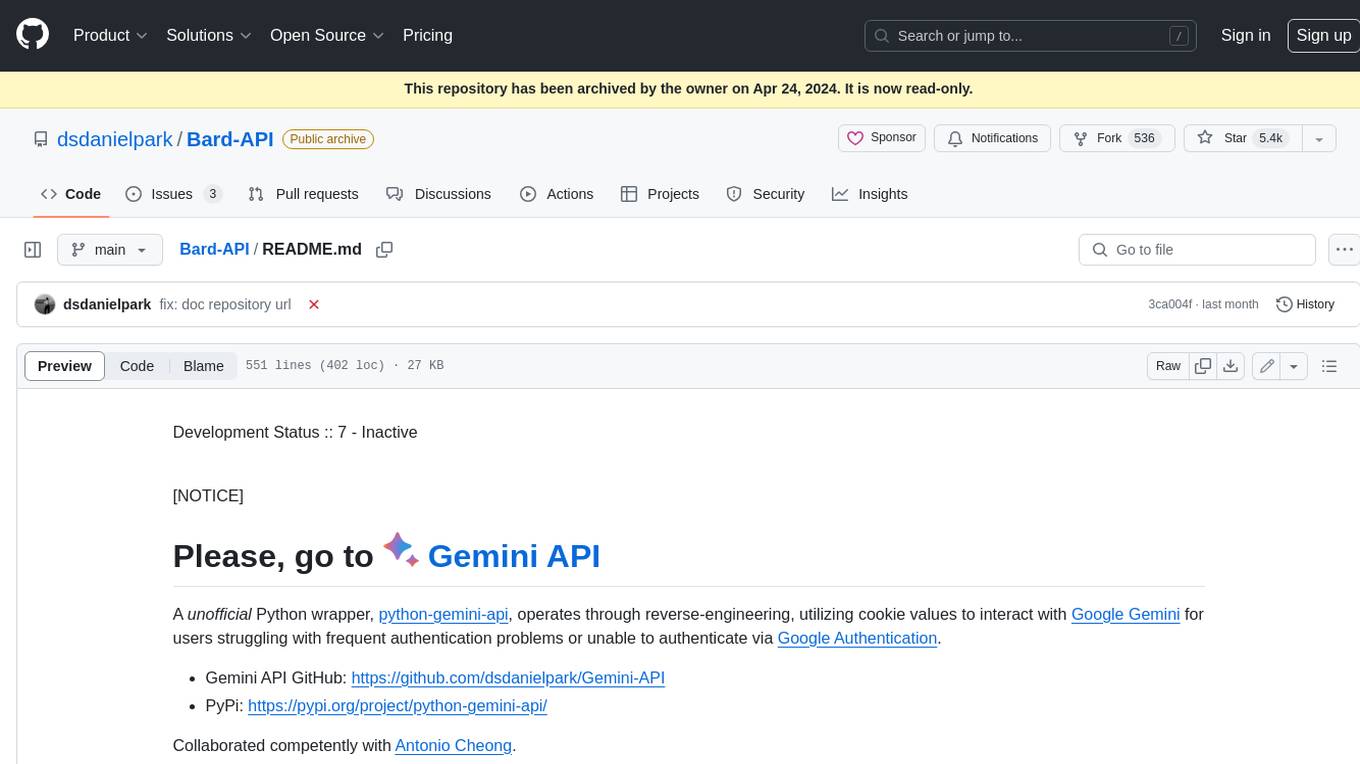
Bard-API
The Bard API is a Python package that returns responses from Google Bard through the value of a cookie. It is an unofficial API that operates through reverse-engineering, utilizing cookie values to interact with Google Bard for users struggling with frequent authentication problems or unable to authenticate via Google Authentication. The Bard API is not a free service, but rather a tool provided to assist developers with testing certain functionalities due to the delayed development and release of Google Bard's API. It has been designed with a lightweight structure that can easily adapt to the emergence of an official API. Therefore, using it for any other purposes is strongly discouraged. If you have access to a reliable official PaLM-2 API or Google Generative AI API, replace the provided response with the corresponding official code. Check out https://github.com/dsdanielpark/Bard-API/issues/262.
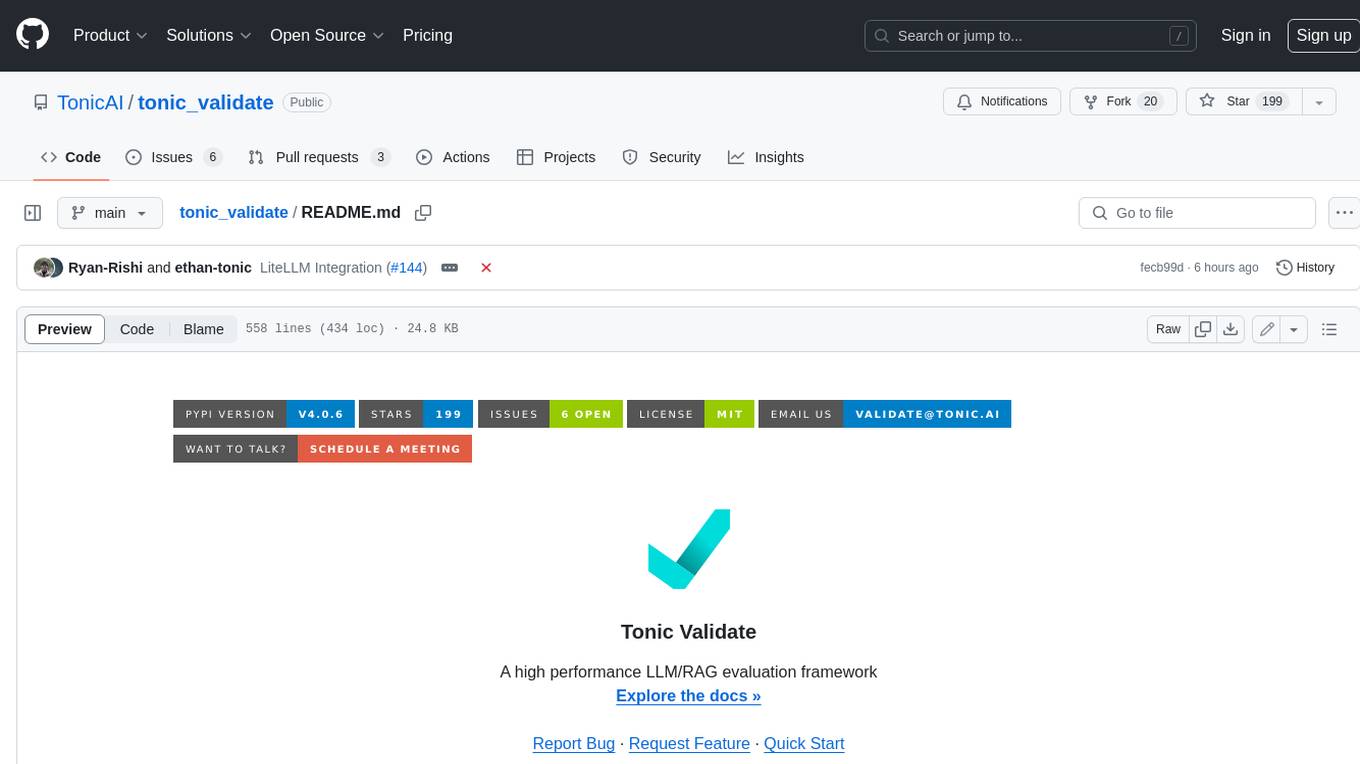
tonic_validate
Tonic Validate is a framework for the evaluation of LLM outputs, such as Retrieval Augmented Generation (RAG) pipelines. Validate makes it easy to evaluate, track, and monitor your LLM and RAG applications. Validate allows you to evaluate your LLM outputs through the use of our provided metrics which measure everything from answer correctness to LLM hallucination. Additionally, Validate has an optional UI to visualize your evaluation results for easy tracking and monitoring.
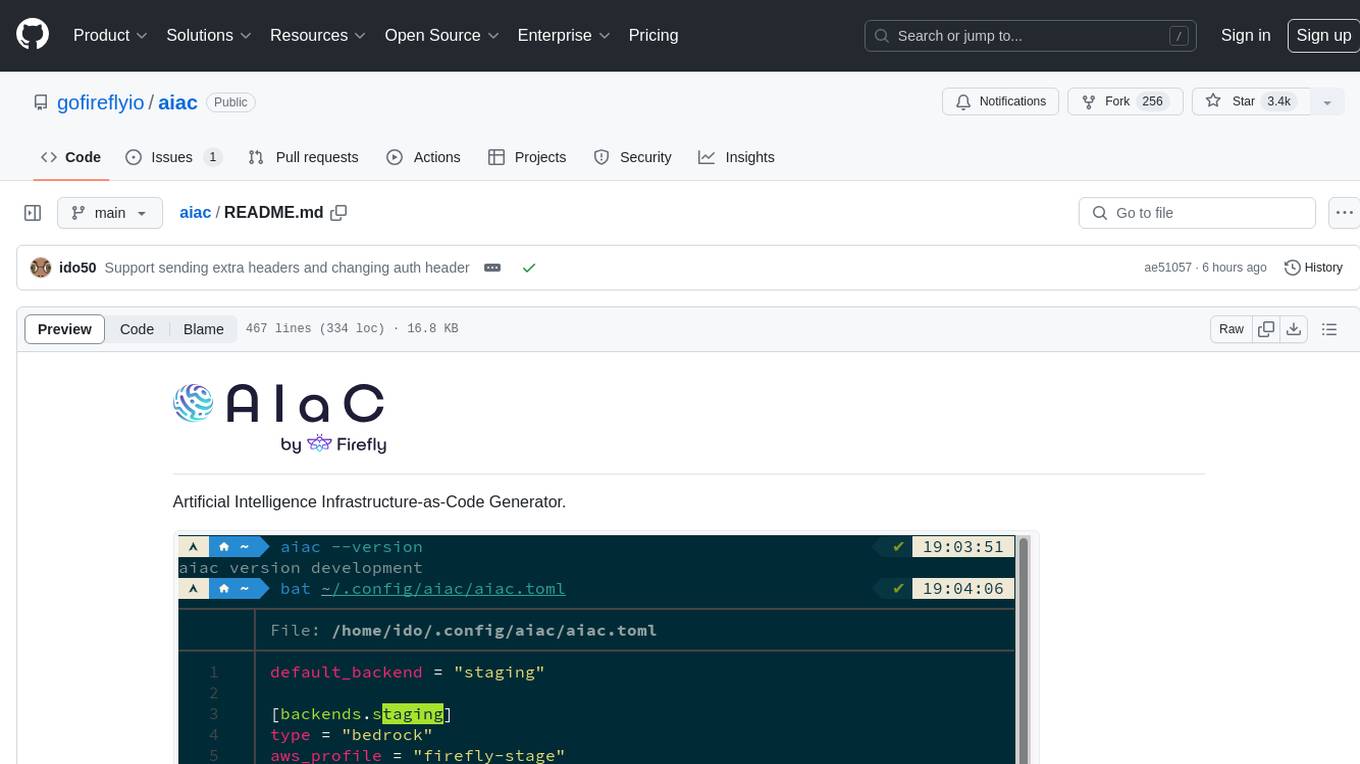
aiac
AIAC is a library and command line tool to generate Infrastructure as Code (IaC) templates, configurations, utilities, queries, and more via LLM providers such as OpenAI, Amazon Bedrock, and Ollama. Users can define multiple 'backends' targeting different LLM providers and environments using a simple configuration file. The tool allows users to ask a model to generate templates for different scenarios and composes an appropriate request to the selected provider, storing the resulting code to a file and/or printing it to standard output.
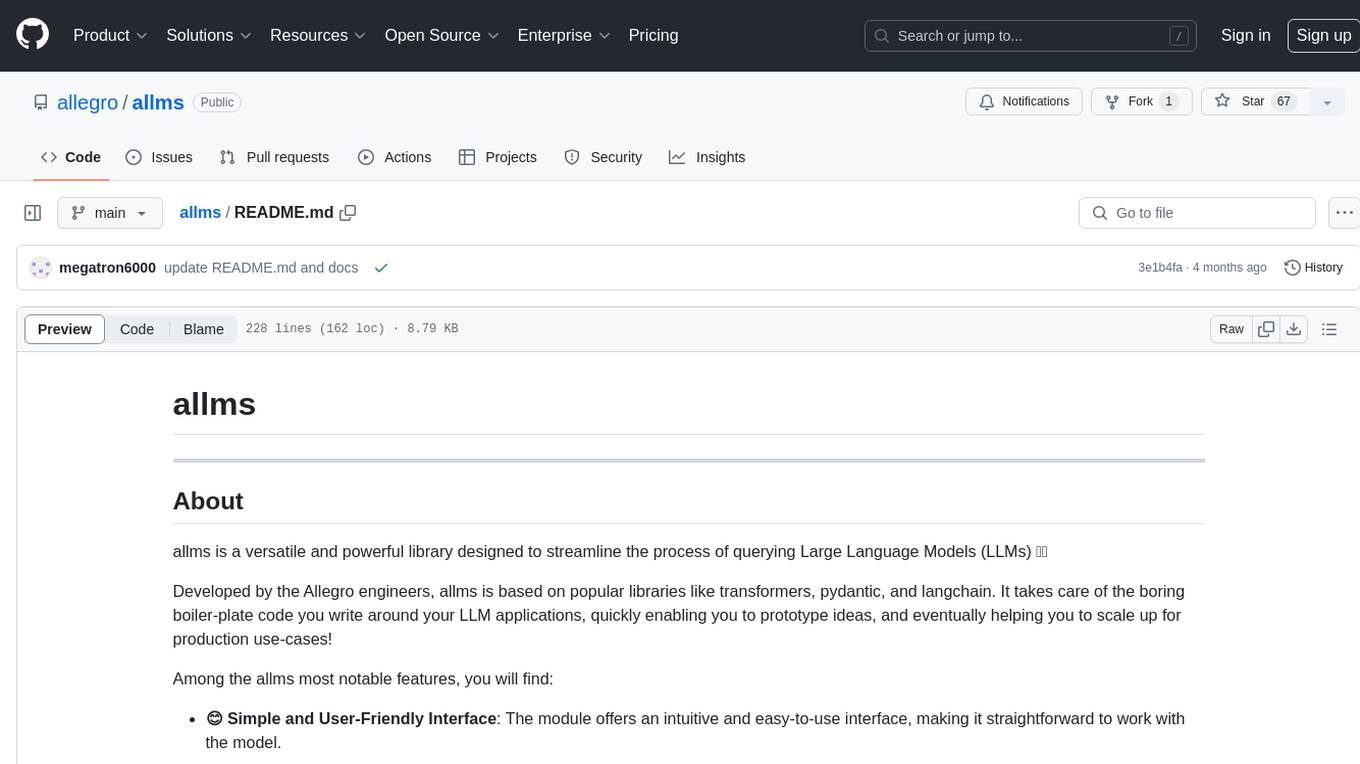
allms
allms is a versatile and powerful library designed to streamline the process of querying Large Language Models (LLMs). Developed by Allegro engineers, it simplifies working with LLM applications by providing a user-friendly interface, asynchronous querying, automatic retrying mechanism, error handling, and output parsing. It supports various LLM families hosted on different platforms like OpenAI, Google, Azure, and GCP. The library offers features for configuring endpoint credentials, batch querying with symbolic variables, and forcing structured output format. It also provides documentation, quickstart guides, and instructions for local development, testing, updating documentation, and making new releases.
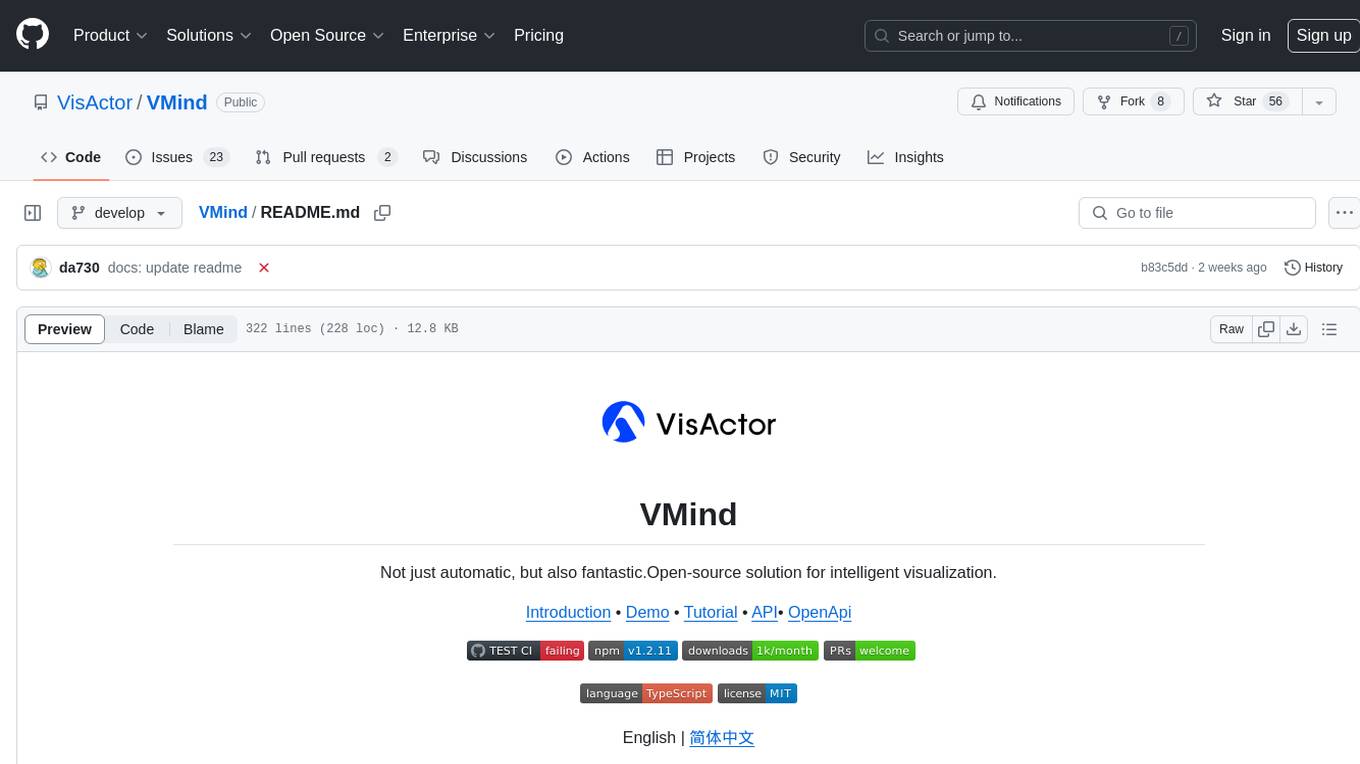
VMind
VMind is an open-source solution for intelligent visualization, providing an intelligent chart component based on LLM by VisActor. It allows users to create chart narrative works with natural language interaction, edit charts through dialogue, and export narratives as videos or GIFs. The tool is easy to use, scalable, supports various chart types, and offers one-click export functionality. Users can customize chart styles, specify themes, and aggregate data using LLM models. VMind aims to enhance efficiency in creating data visualization works through dialogue-based editing and natural language interaction.
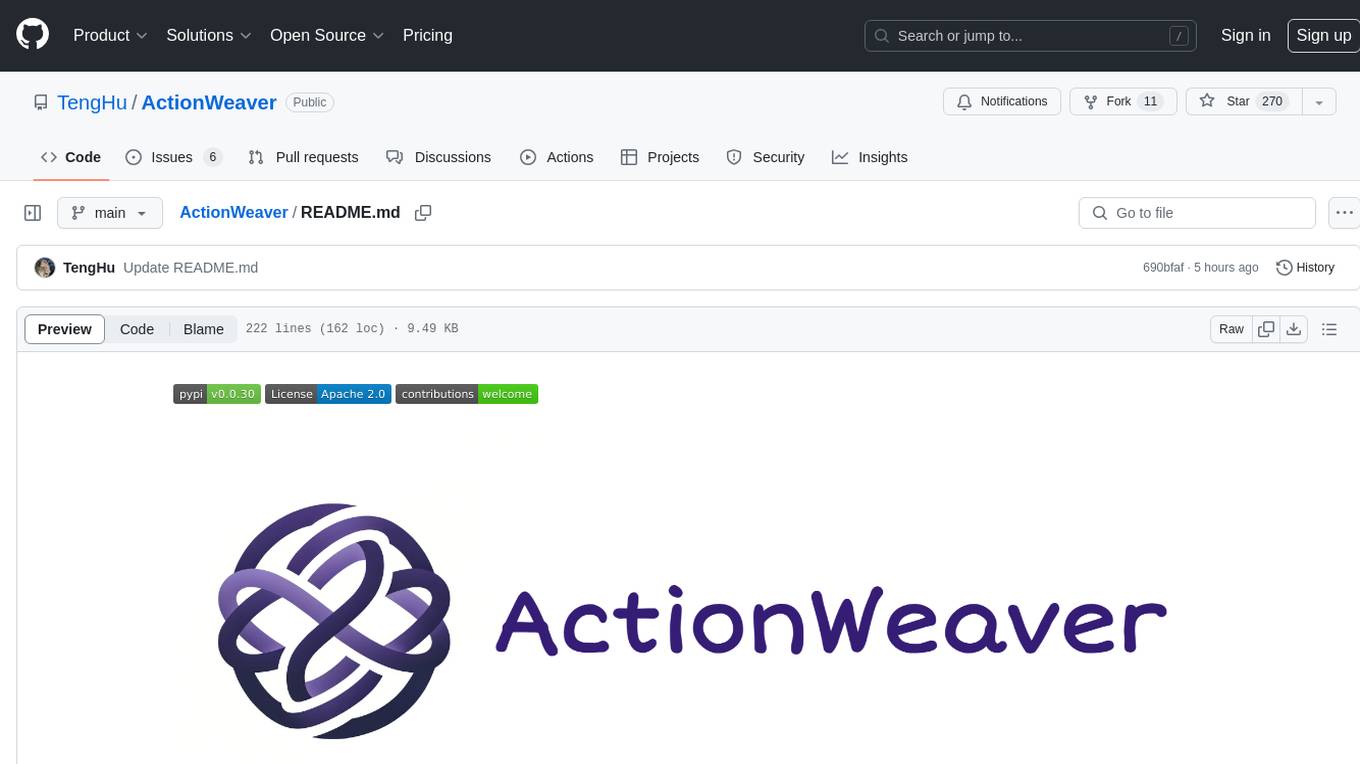
ActionWeaver
ActionWeaver is an AI application framework designed for simplicity, relying on OpenAI and Pydantic. It supports both OpenAI API and Azure OpenAI service. The framework allows for function calling as a core feature, extensibility to integrate any Python code, function orchestration for building complex call hierarchies, and telemetry and observability integration. Users can easily install ActionWeaver using pip and leverage its capabilities to create, invoke, and orchestrate actions with the language model. The framework also provides structured extraction using Pydantic models and allows for exception handling customization. Contributions to the project are welcome, and users are encouraged to cite ActionWeaver if found useful.
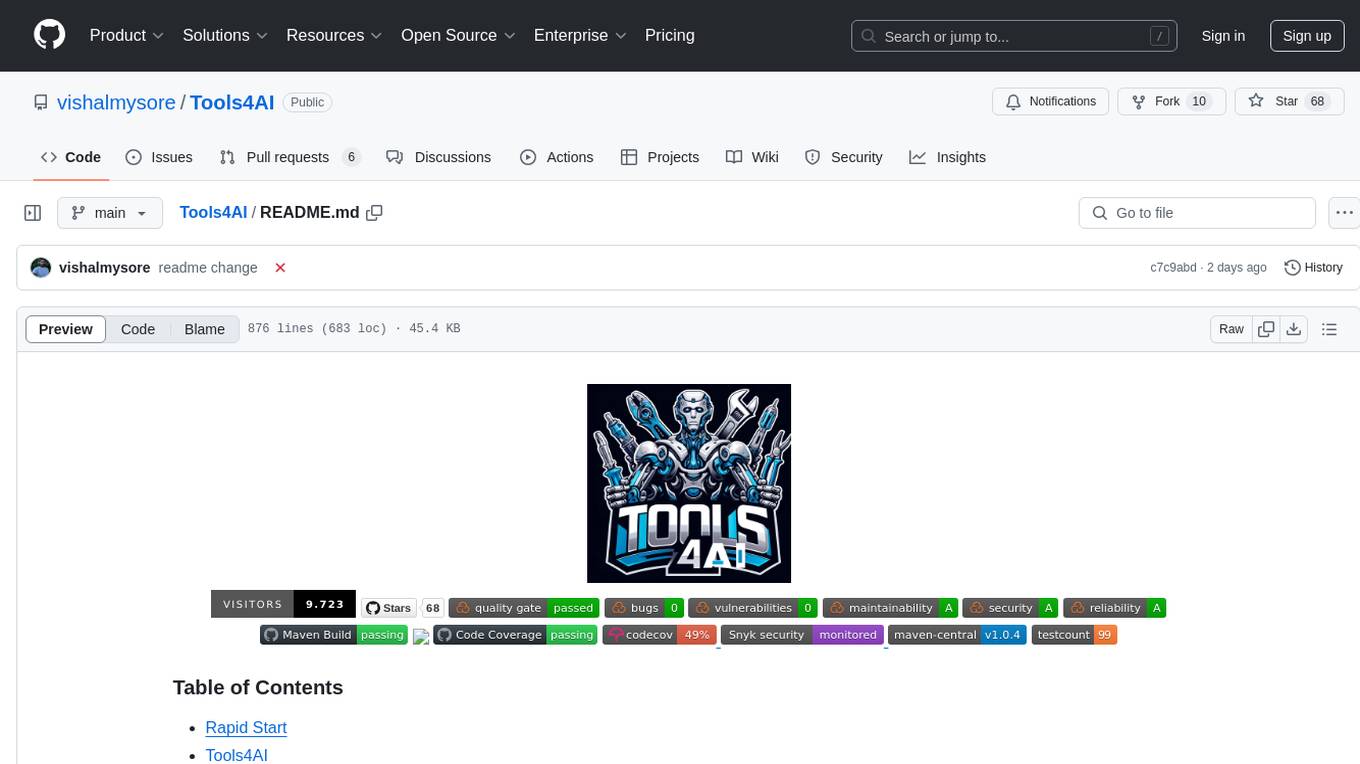
Tools4AI
Tools4AI is a Java-based Agentic Framework for building AI agents to integrate with enterprise Java applications. It enables the conversion of natural language prompts into actionable behaviors, streamlining user interactions with complex systems. By leveraging AI capabilities, it enhances productivity and innovation across diverse applications. The framework allows for seamless integration of AI with various systems, such as customer service applications, to interpret user requests, trigger actions, and streamline workflows. Prompt prediction anticipates user actions based on input prompts, enhancing user experience by proactively suggesting relevant actions or services based on context.
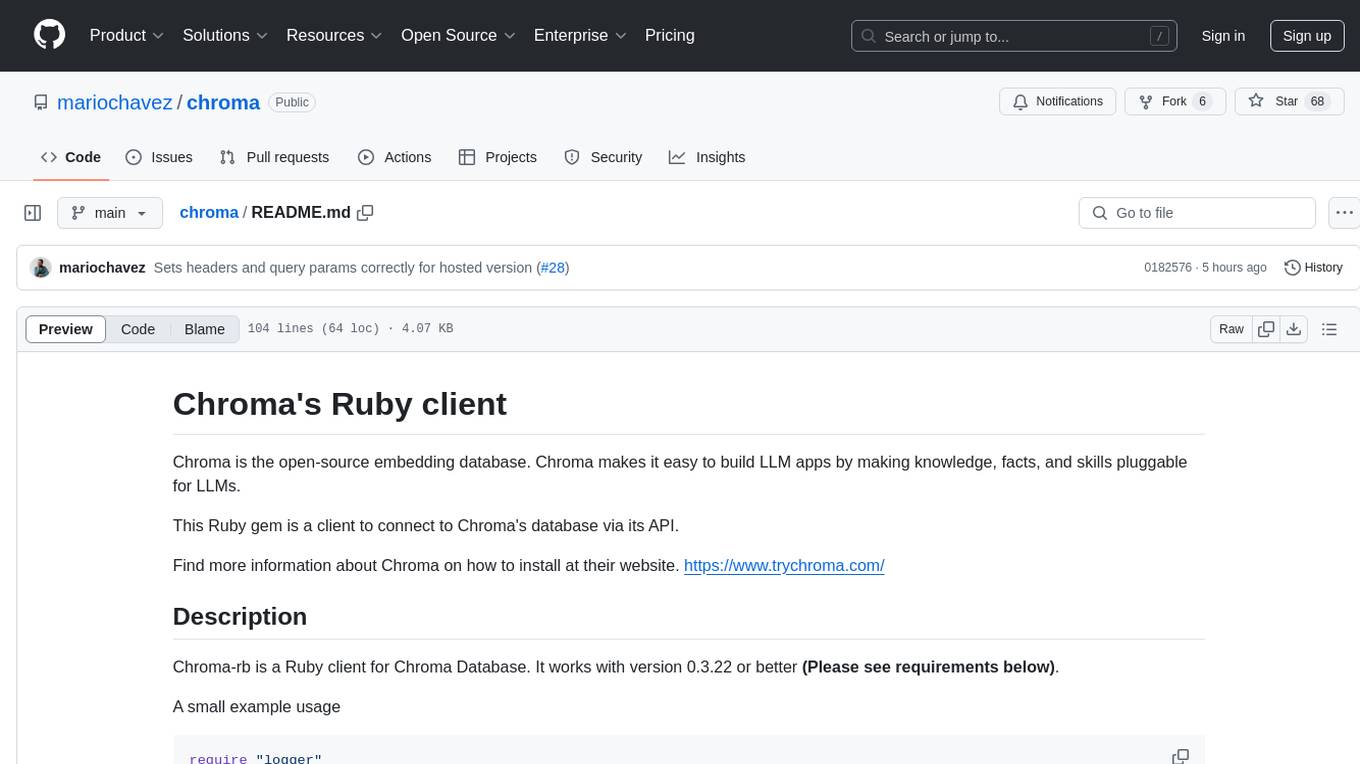
chroma
Chroma is an open-source embedding database that simplifies building LLM apps by enabling the integration of knowledge, facts, and skills for LLMs. The Ruby client for Chroma Database, chroma-rb, facilitates connecting to Chroma's database via its API. Users can configure the host, check server version, create collections, and add embeddings. The gem supports Chroma Database version 0.3.22 or newer, requiring Ruby 3.1.4 or later. It can be used with the hosted Chroma service at trychroma.com by setting configuration options like api_key, tenant, and database. Additionally, the gem provides integration with Jupyter Notebook for creating embeddings using Ollama and Nomic embed text with a Ruby HTTP client.
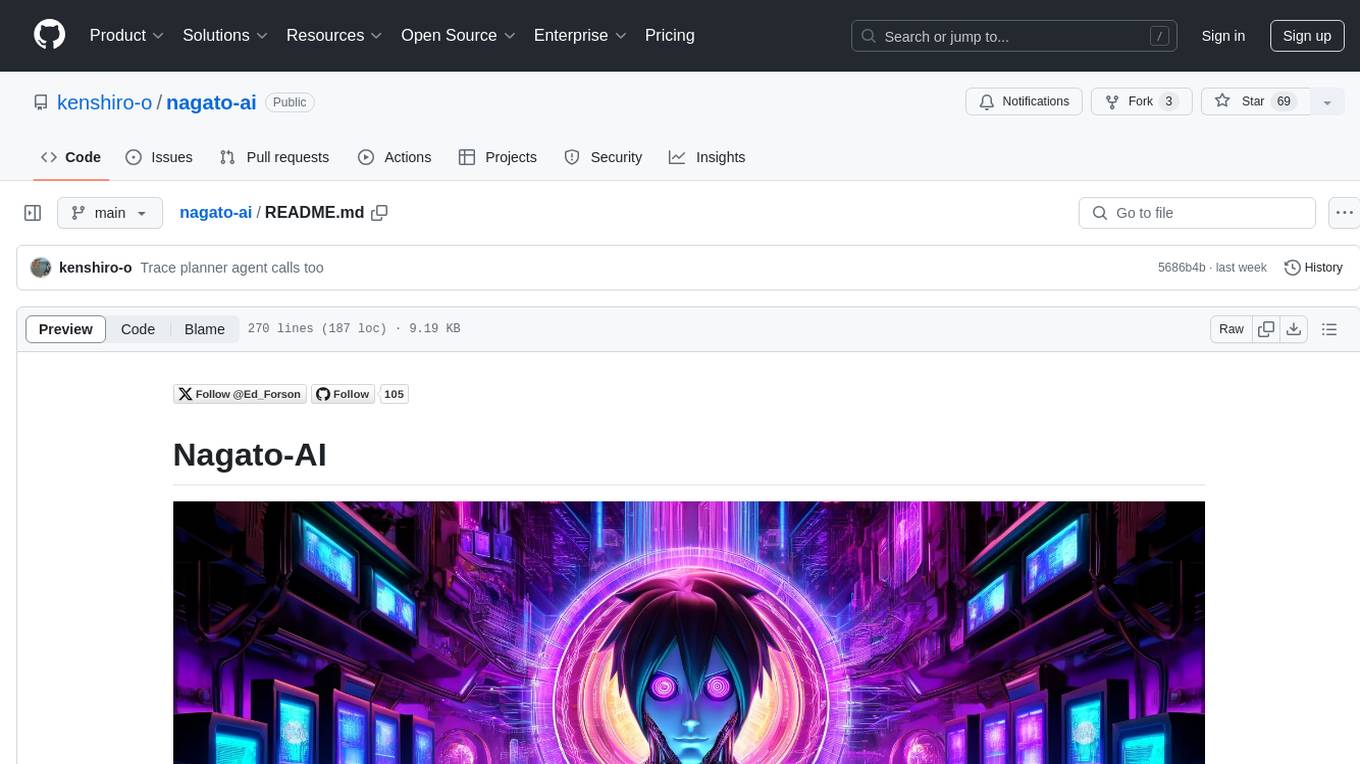
nagato-ai
Nagato-AI is an intuitive AI Agent library that supports multiple LLMs including OpenAI's GPT, Anthropic's Claude, Google's Gemini, and Groq LLMs. Users can create agents from these models and combine them to build an effective AI Agent system. The library is named after the powerful ninja Nagato from the anime Naruto, who can control multiple bodies with different abilities. Nagato-AI acts as a linchpin to summon and coordinate AI Agents for specific missions. It provides flexibility in programming and supports tools like Coordinator, Researcher, Critic agents, and HumanConfirmInputTool.
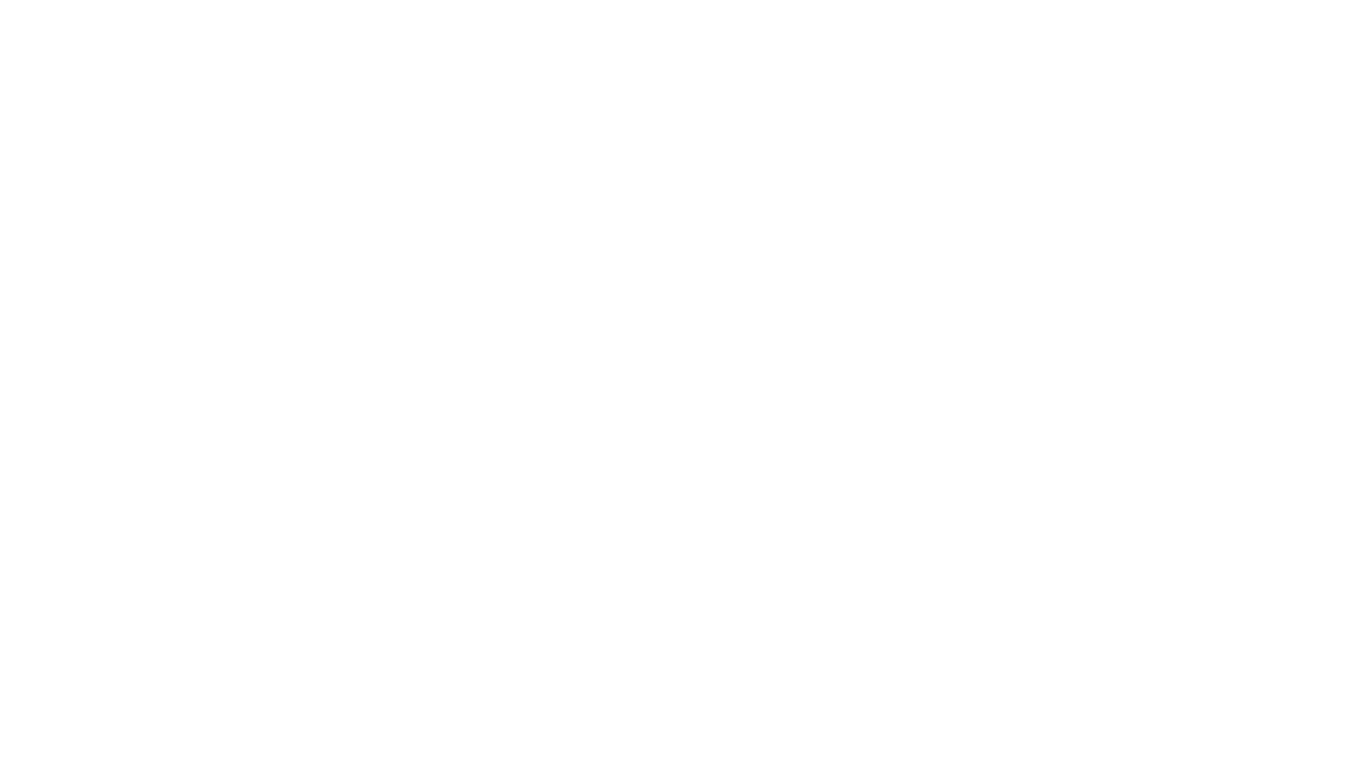
rag-experiment-accelerator
The RAG Experiment Accelerator is a versatile tool that helps you conduct experiments and evaluations using Azure AI Search and RAG pattern. It offers a rich set of features, including experiment setup, integration with Azure AI Search, Azure Machine Learning, MLFlow, and Azure OpenAI, multiple document chunking strategies, query generation, multiple search types, sub-querying, re-ranking, metrics and evaluation, report generation, and multi-lingual support. The tool is designed to make it easier and faster to run experiments and evaluations of search queries and quality of response from OpenAI, and is useful for researchers, data scientists, and developers who want to test the performance of different search and OpenAI related hyperparameters, compare the effectiveness of various search strategies, fine-tune and optimize parameters, find the best combination of hyperparameters, and generate detailed reports and visualizations from experiment results.
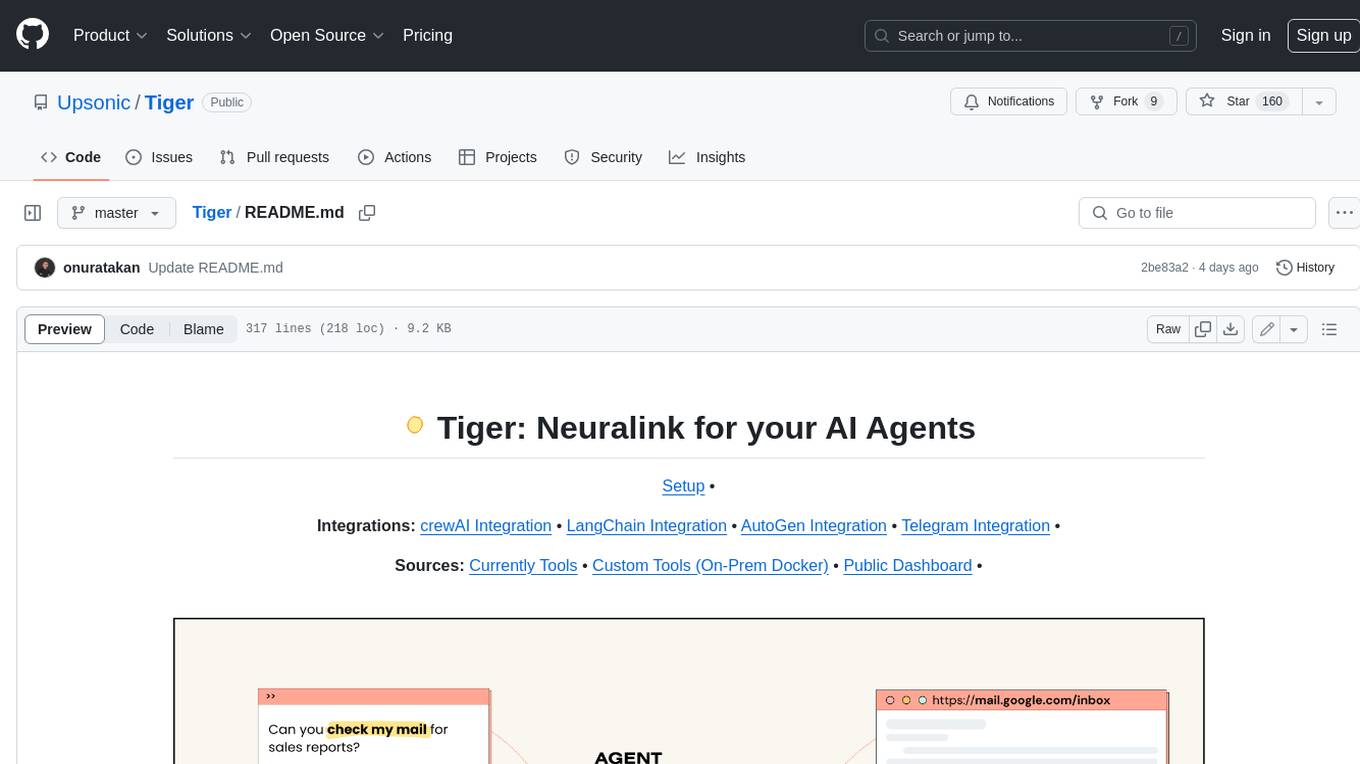
Tiger
Tiger is a community-driven project developing a reusable and integrated tool ecosystem for LLM Agent Revolution. It utilizes Upsonic for isolated tool storage, profiling, and automatic document generation. With Tiger, you can create a customized environment for your agents or leverage the robust and publicly maintained Tiger curated by the community itself.
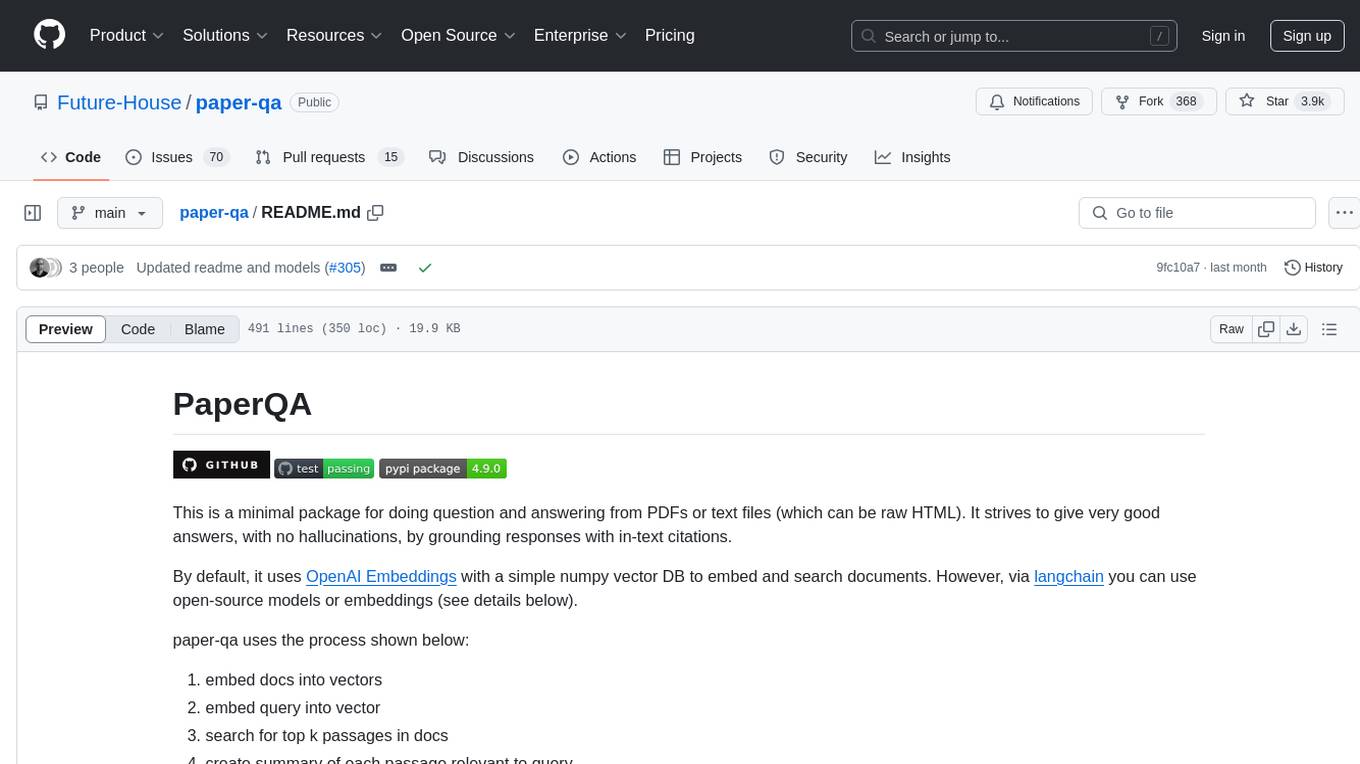
paper-qa
PaperQA is a minimal package for question and answering from PDFs or text files, providing very good answers with in-text citations. It uses OpenAI Embeddings to embed and search documents, and includes a process of embedding docs, queries, searching for top passages, creating summaries, using an LLM to re-score and select relevant summaries, putting summaries into prompt, and generating answers. The tool can be used to answer specific questions related to scientific research by leveraging citations and relevant passages from documents.
For similar tasks
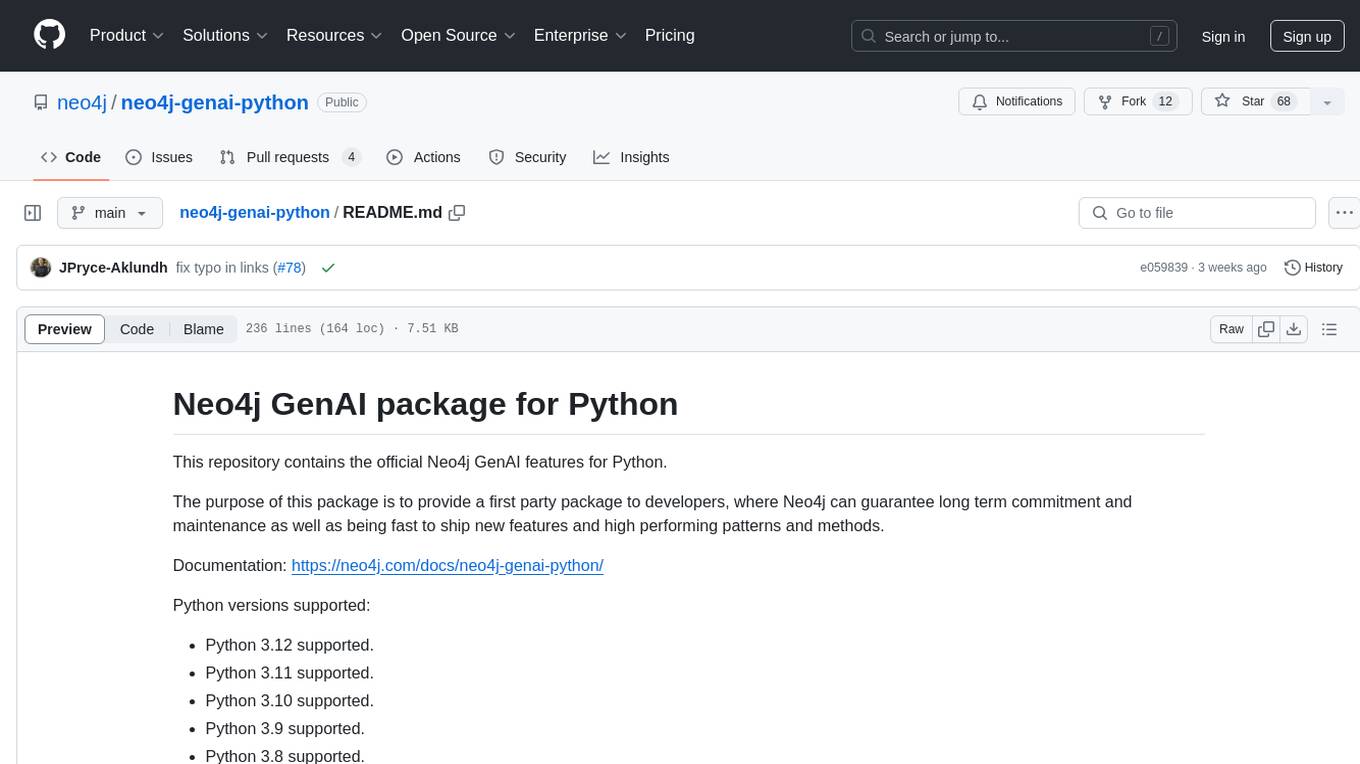
neo4j-genai-python
This repository contains the official Neo4j GenAI features for Python. The purpose of this package is to provide a first-party package to developers, where Neo4j can guarantee long-term commitment and maintenance as well as being fast to ship new features and high-performing patterns and methods.
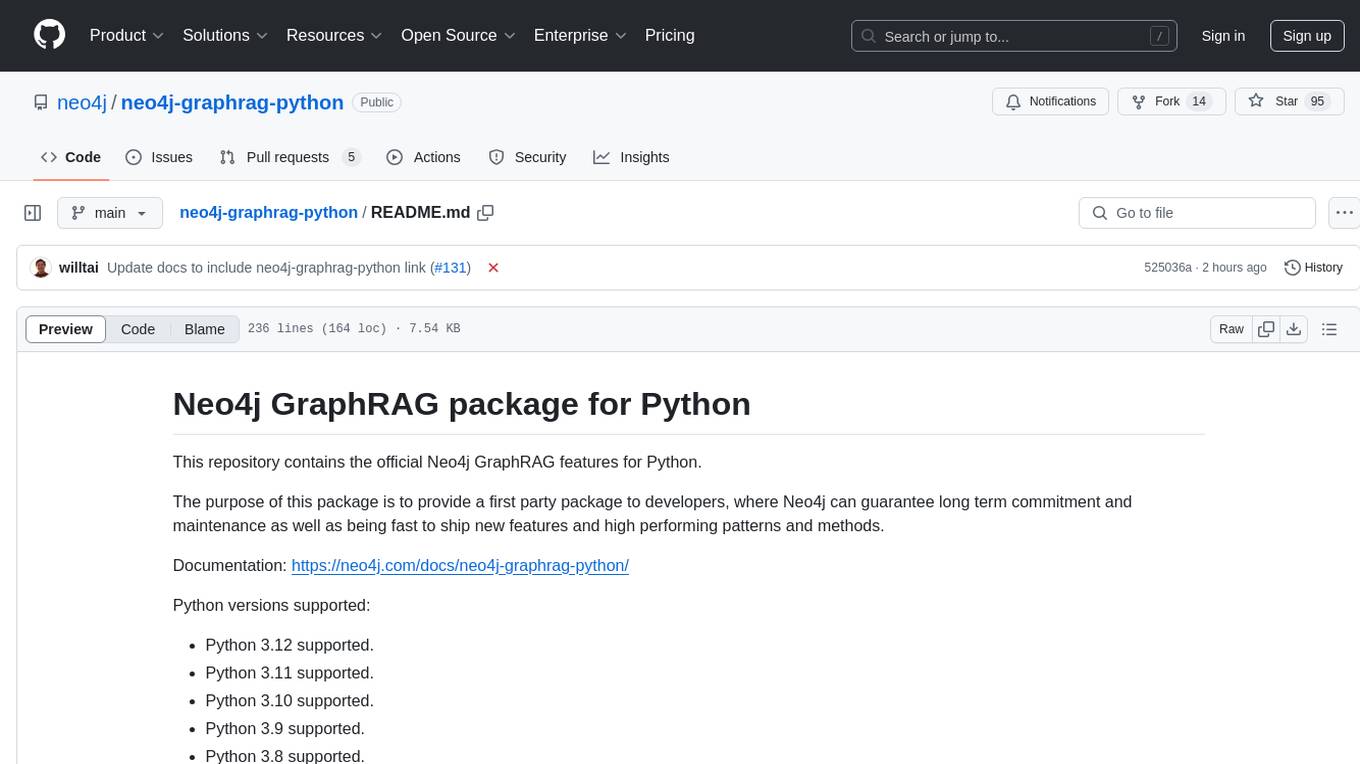
neo4j-graphrag-python
The Neo4j GraphRAG package for Python is an official repository that provides features for creating and managing vector indexes in Neo4j databases. It aims to offer developers a reliable package with long-term commitment, maintenance, and fast feature updates. The package supports various Python versions and includes functionalities for creating vector indexes, populating them, and performing similarity searches. It also provides guidelines for installation, examples, and development processes such as installing dependencies, making changes, and running tests.
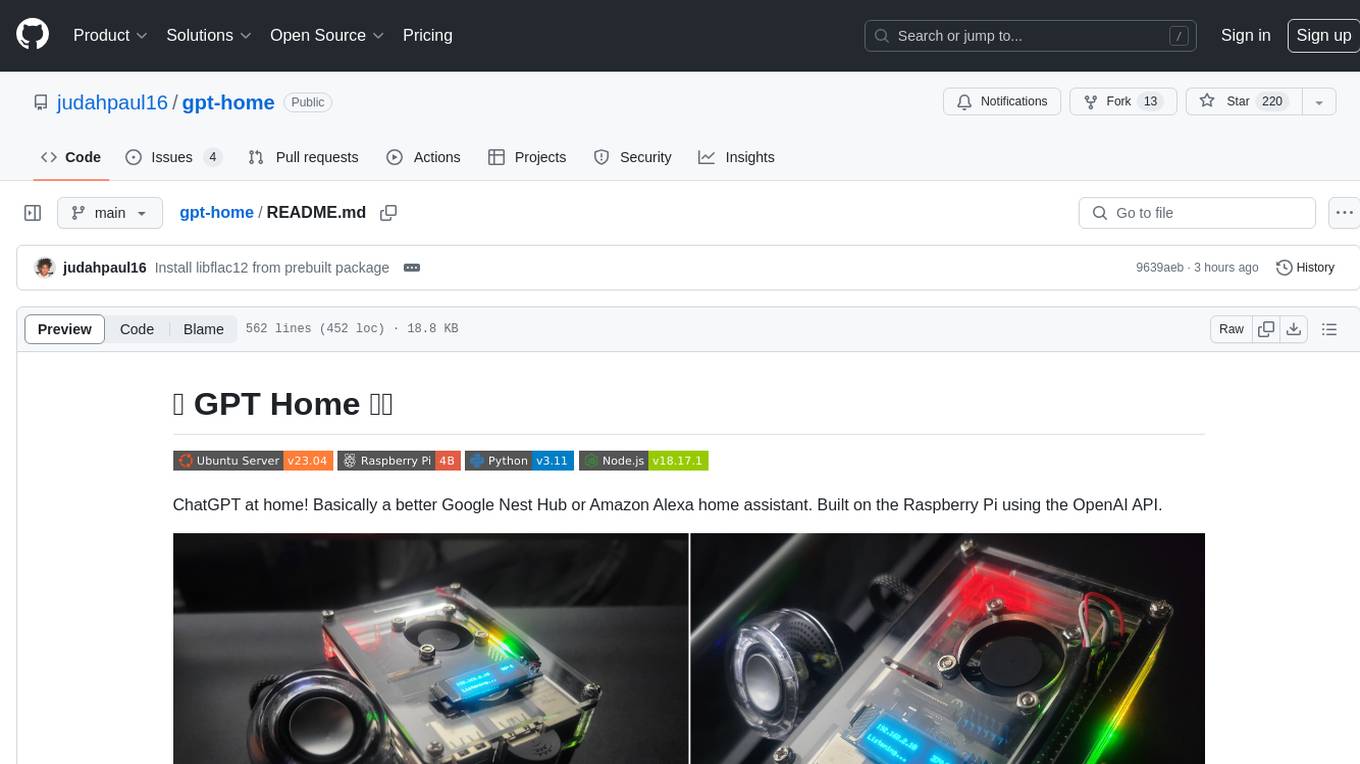
gpt-home
GPT Home is a project that allows users to build their own home assistant using Raspberry Pi and OpenAI API. It serves as a guide for setting up a smart home assistant similar to Google Nest Hub or Amazon Alexa. The project integrates various components like OpenAI, Spotify, Philips Hue, and OpenWeatherMap to provide a personalized home assistant experience. Users can follow the detailed instructions provided to build their own version of the home assistant on Raspberry Pi, with optional components for customization. The project also includes system configurations, dependencies installation, and setup scripts for easy deployment. Overall, GPT Home offers a DIY solution for creating a smart home assistant using Raspberry Pi and OpenAI technology.
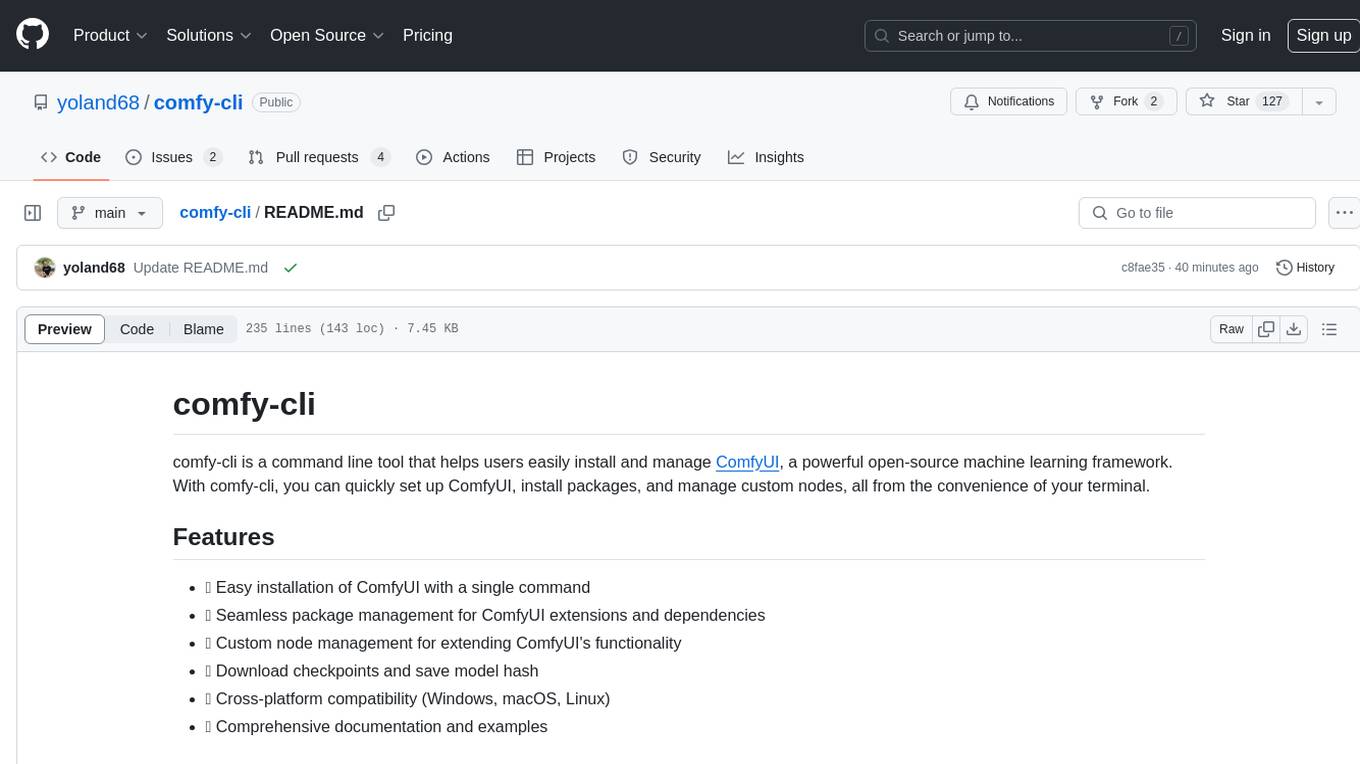
comfy-cli
comfy-cli is a command line tool designed to simplify the installation and management of ComfyUI, an open-source machine learning framework. It allows users to easily set up ComfyUI, install packages, manage custom nodes, download checkpoints, and ensure cross-platform compatibility. The tool provides comprehensive documentation and examples to aid users in utilizing ComfyUI efficiently.
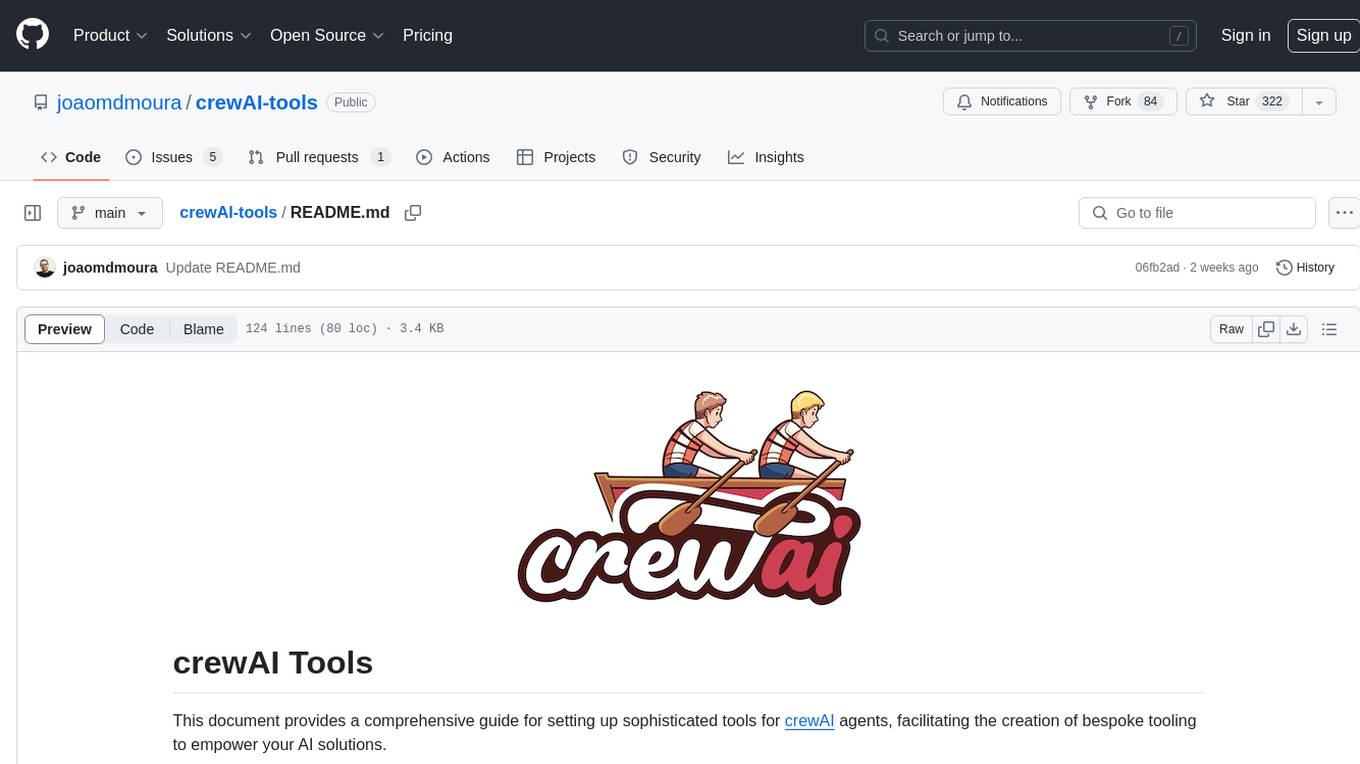
crewAI-tools
The crewAI Tools repository provides a guide for setting up tools for crewAI agents, enabling the creation of custom tools to enhance AI solutions. Tools play a crucial role in improving agent functionality. The guide explains how to equip agents with a range of tools and how to create new tools. Tools are designed to return strings for generating responses. There are two main methods for creating tools: subclassing BaseTool and using the tool decorator. Contributions to the toolset are encouraged, and the development setup includes steps for installing dependencies, activating the virtual environment, setting up pre-commit hooks, running tests, static type checking, packaging, and local installation. Enhance AI agent capabilities with advanced tooling.
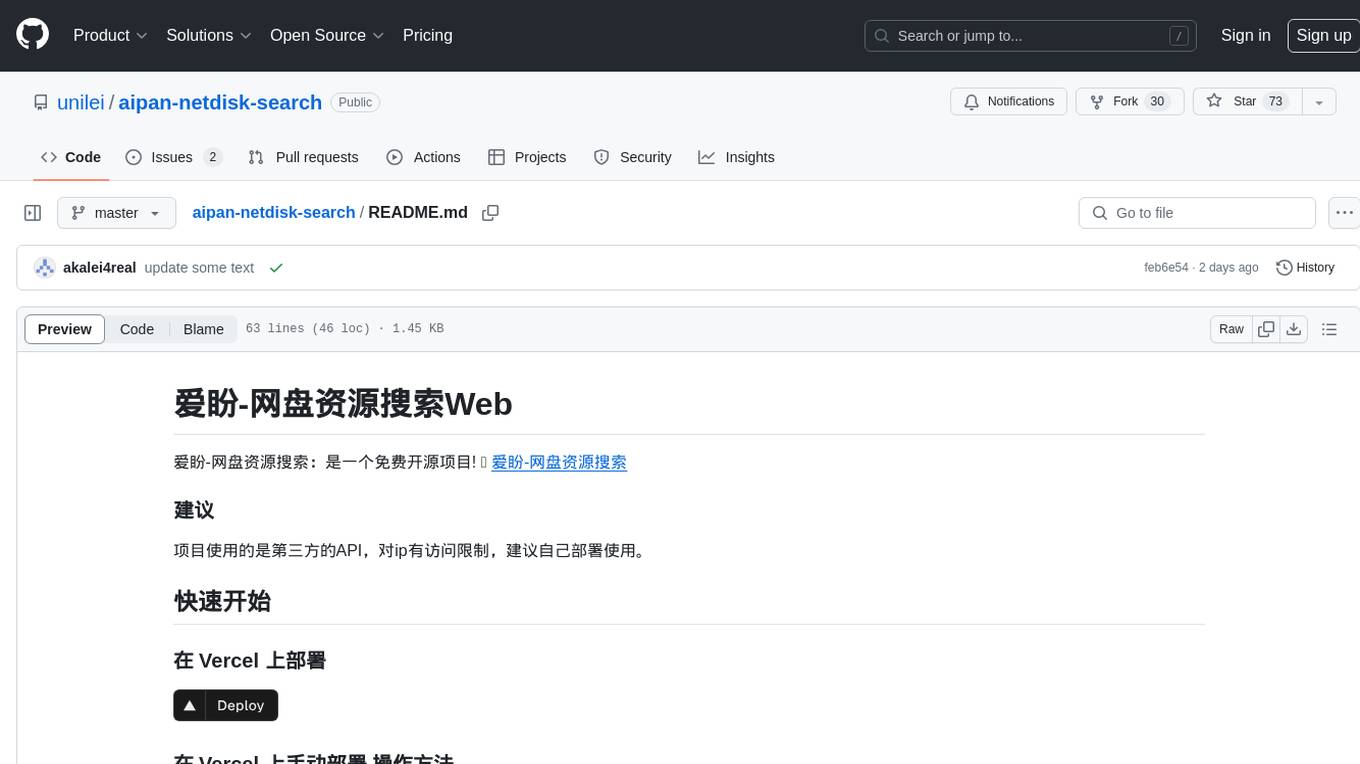
aipan-netdisk-search
Aipan-Netdisk-Search is a free and open-source web project for searching netdisk resources. It utilizes third-party APIs with IP access restrictions, suggesting self-deployment. The project can be easily deployed on Vercel and provides instructions for manual deployment. Users can clone the project, install dependencies, run it in the browser, and access it at localhost:3001. The project also includes documentation for deploying on personal servers using NUXT.JS. Additionally, there are options for donations and communication via WeChat.
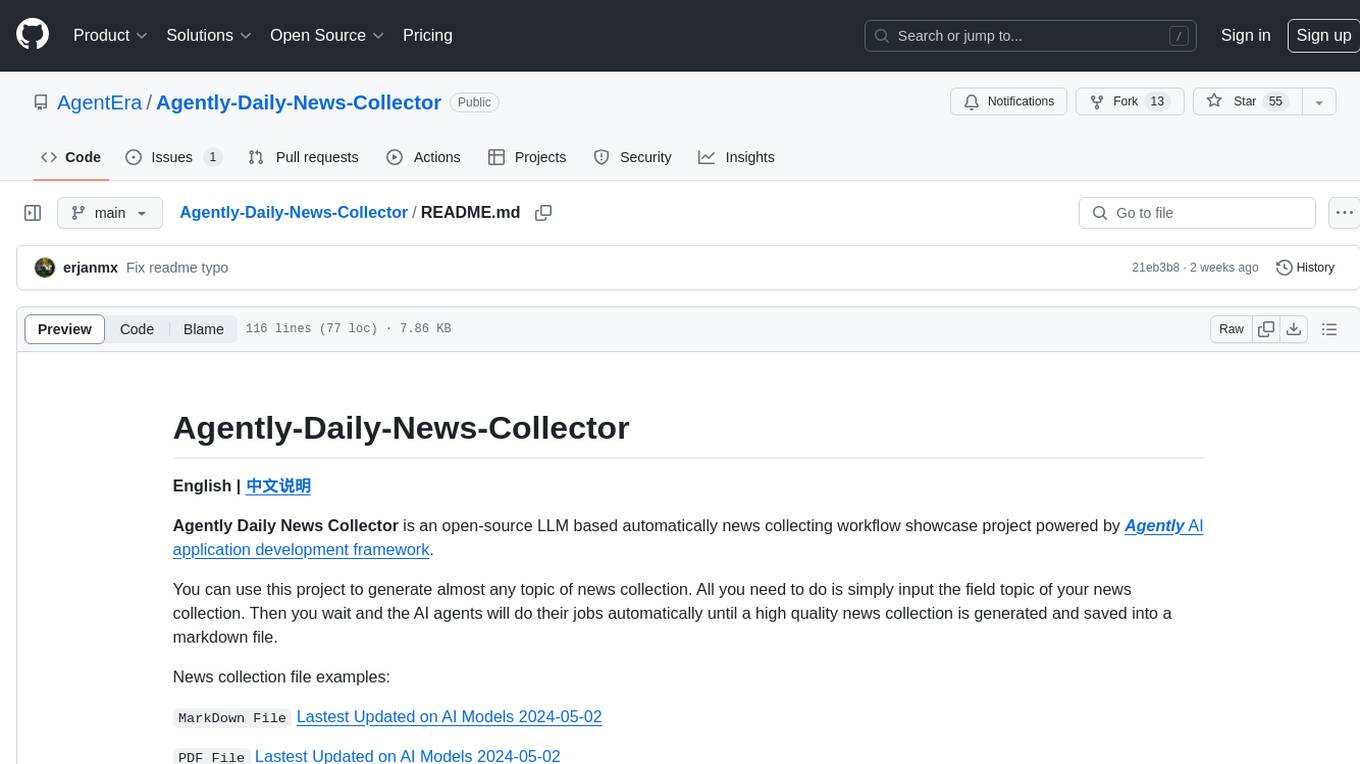
Agently-Daily-News-Collector
Agently Daily News Collector is an open-source project showcasing a workflow powered by the Agent ly AI application development framework. It allows users to generate news collections on various topics by inputting the field topic. The AI agents automatically perform the necessary tasks to generate a high-quality news collection saved in a markdown file. Users can edit settings in the YAML file, install Python and required packages, input their topic idea, and wait for the news collection to be generated. The process involves tasks like outlining, searching, summarizing, and preparing column data. The project dependencies include Agently AI Development Framework, duckduckgo-search, BeautifulSoup4, and PyYAM.
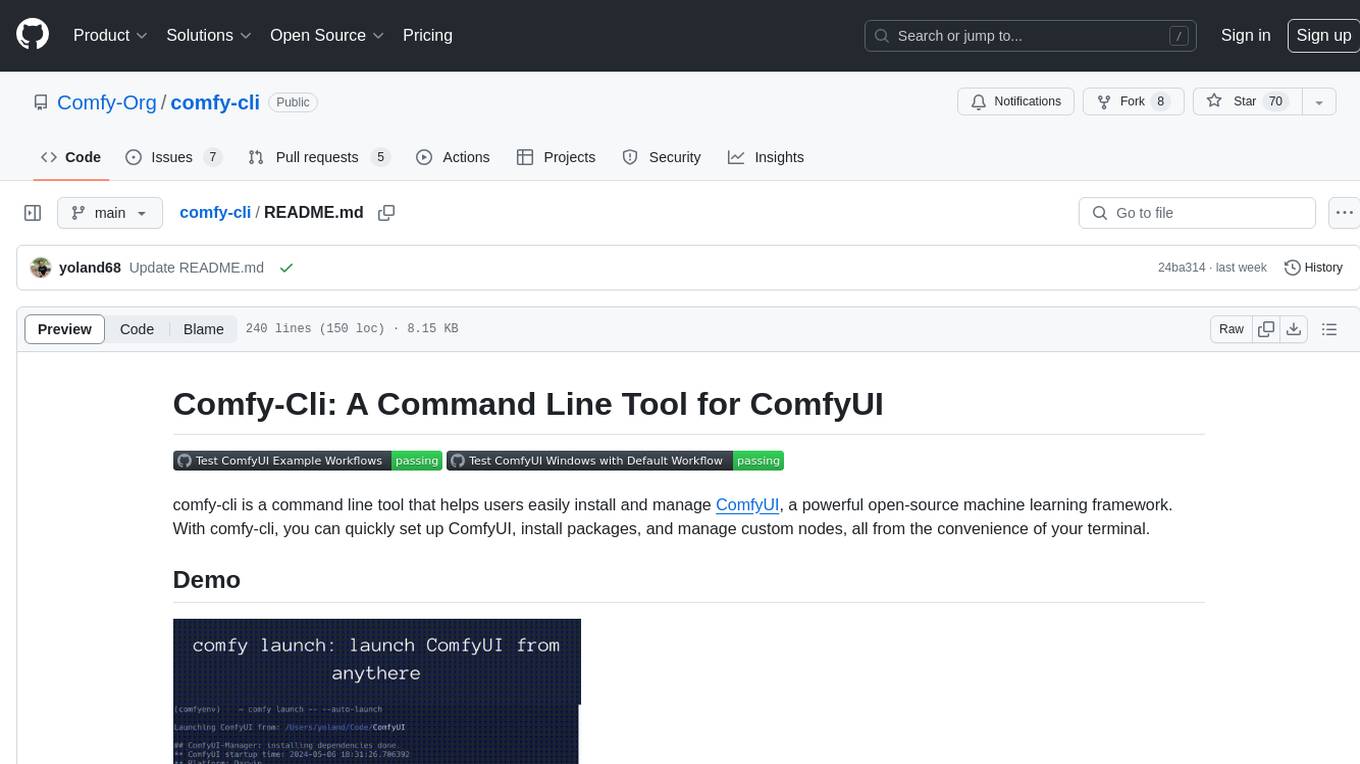
comfy-cli
Comfy-cli is a command line tool designed to facilitate the installation and management of ComfyUI, an open-source machine learning framework. Users can easily set up ComfyUI, install packages, and manage custom nodes directly from the terminal. The tool offers features such as easy installation, seamless package management, custom node management, checkpoint downloads, cross-platform compatibility, and comprehensive documentation. Comfy-cli simplifies the process of working with ComfyUI, making it convenient for users to handle various tasks related to the framework.
For similar jobs
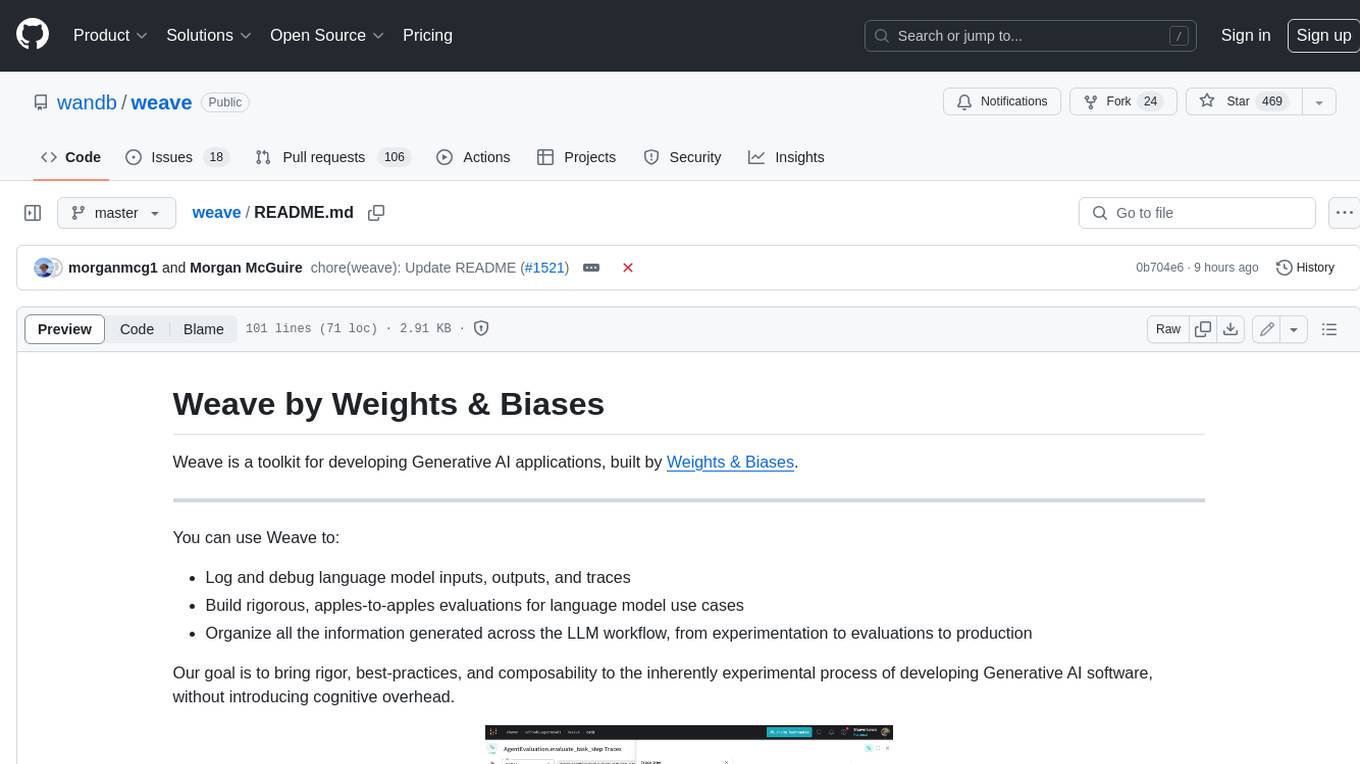
weave
Weave is a toolkit for developing Generative AI applications, built by Weights & Biases. With Weave, you can log and debug language model inputs, outputs, and traces; build rigorous, apples-to-apples evaluations for language model use cases; and organize all the information generated across the LLM workflow, from experimentation to evaluations to production. Weave aims to bring rigor, best-practices, and composability to the inherently experimental process of developing Generative AI software, without introducing cognitive overhead.
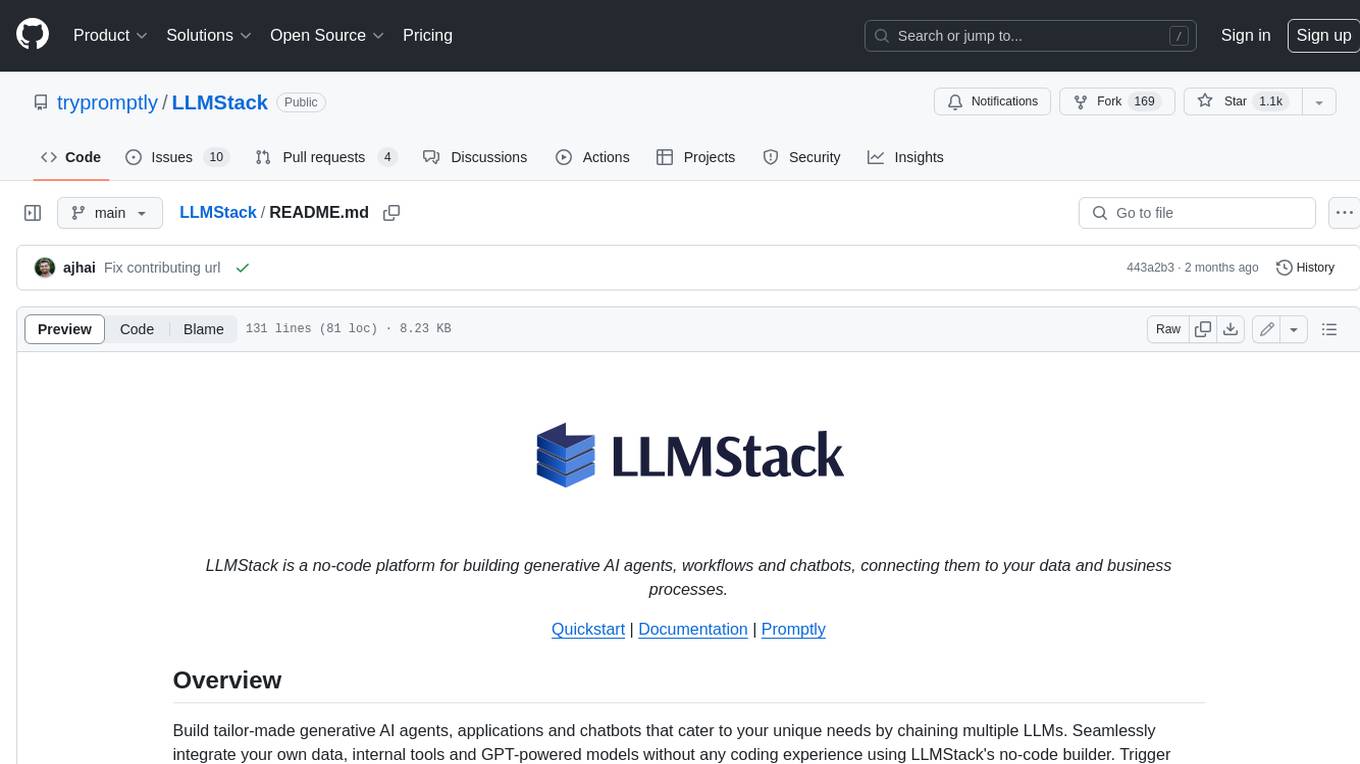
LLMStack
LLMStack is a no-code platform for building generative AI agents, workflows, and chatbots. It allows users to connect their own data, internal tools, and GPT-powered models without any coding experience. LLMStack can be deployed to the cloud or on-premise and can be accessed via HTTP API or triggered from Slack or Discord.
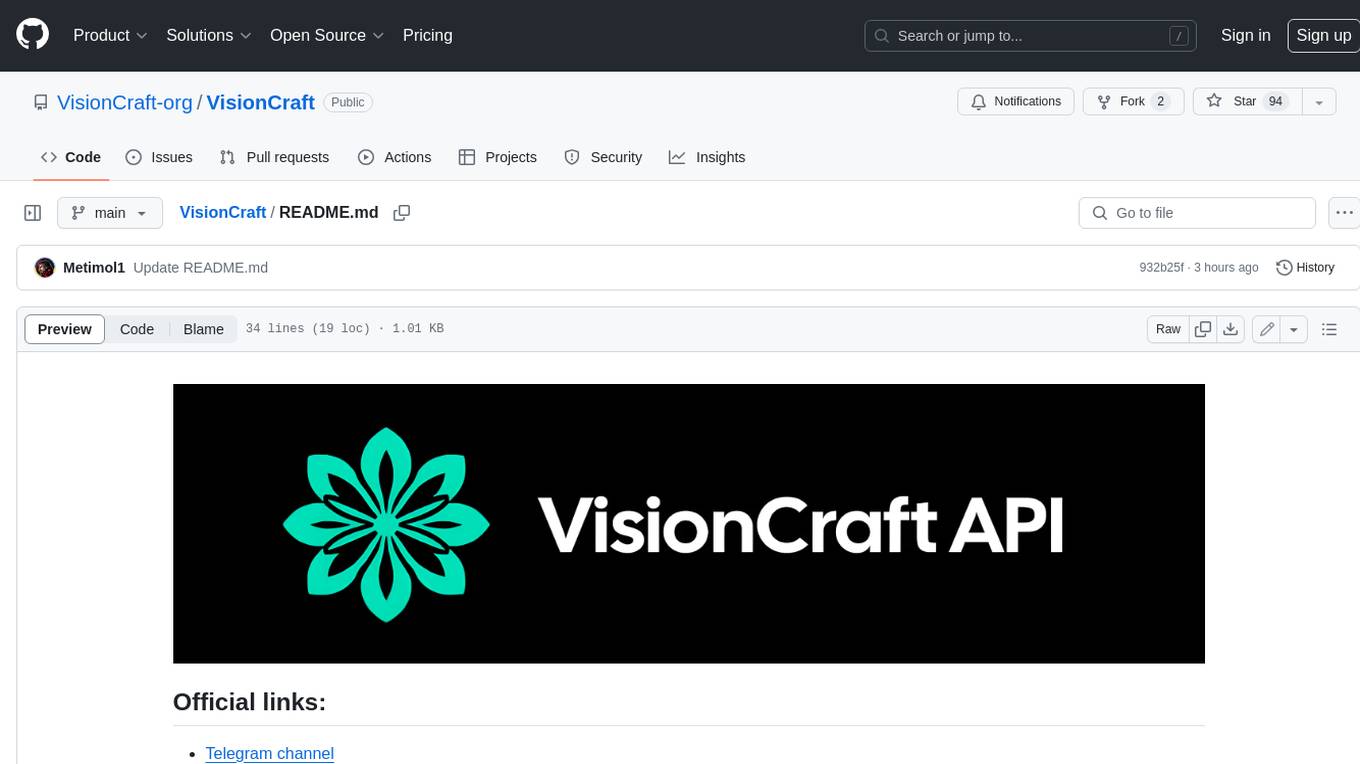
VisionCraft
The VisionCraft API is a free API for using over 100 different AI models. From images to sound.
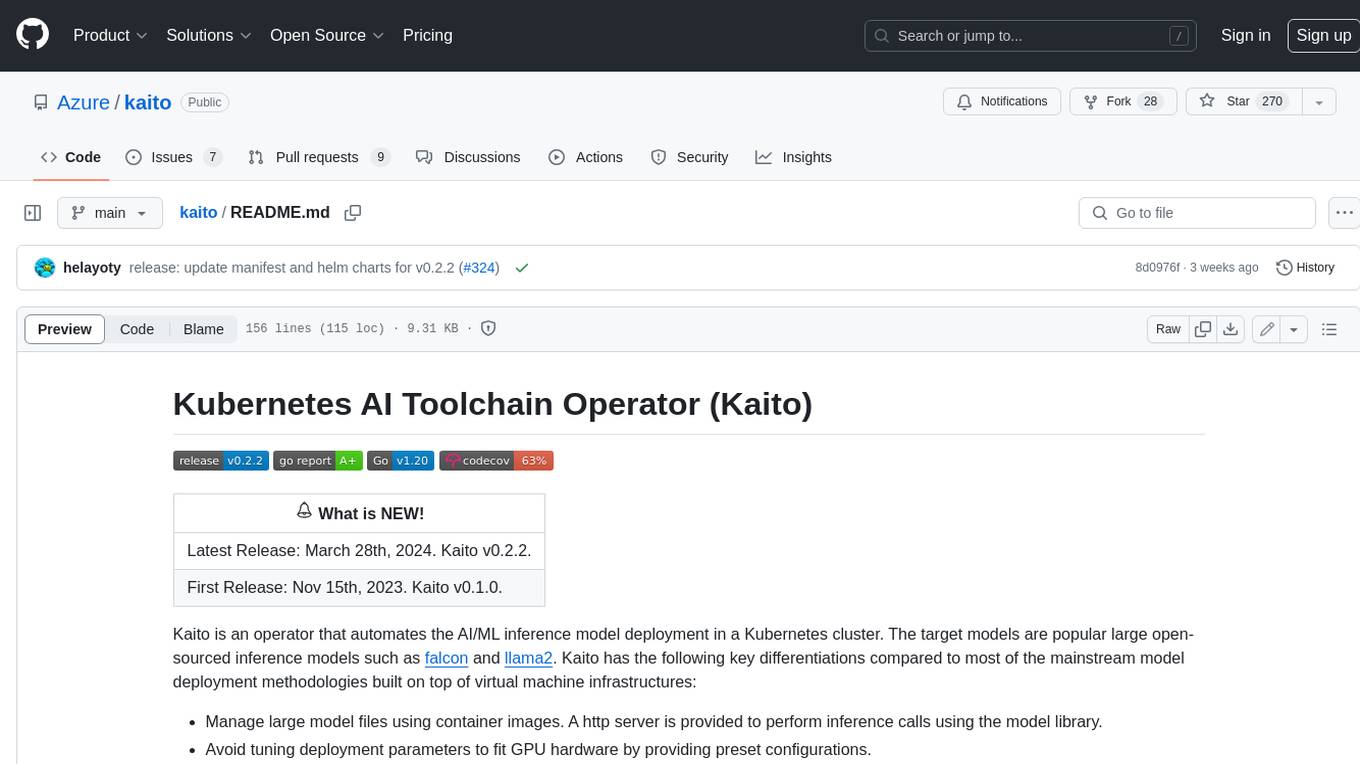
kaito
Kaito is an operator that automates the AI/ML inference model deployment in a Kubernetes cluster. It manages large model files using container images, avoids tuning deployment parameters to fit GPU hardware by providing preset configurations, auto-provisions GPU nodes based on model requirements, and hosts large model images in the public Microsoft Container Registry (MCR) if the license allows. Using Kaito, the workflow of onboarding large AI inference models in Kubernetes is largely simplified.
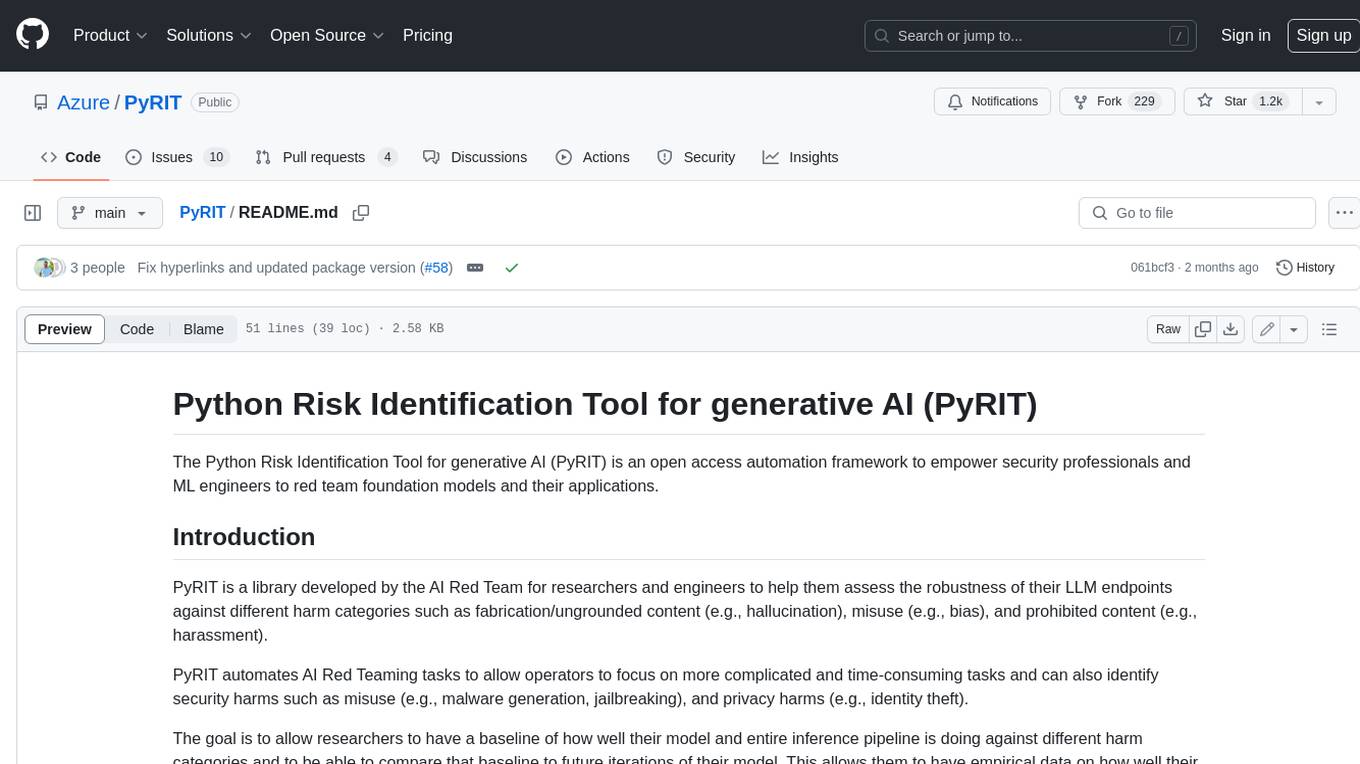
PyRIT
PyRIT is an open access automation framework designed to empower security professionals and ML engineers to red team foundation models and their applications. It automates AI Red Teaming tasks to allow operators to focus on more complicated and time-consuming tasks and can also identify security harms such as misuse (e.g., malware generation, jailbreaking), and privacy harms (e.g., identity theft). The goal is to allow researchers to have a baseline of how well their model and entire inference pipeline is doing against different harm categories and to be able to compare that baseline to future iterations of their model. This allows them to have empirical data on how well their model is doing today, and detect any degradation of performance based on future improvements.
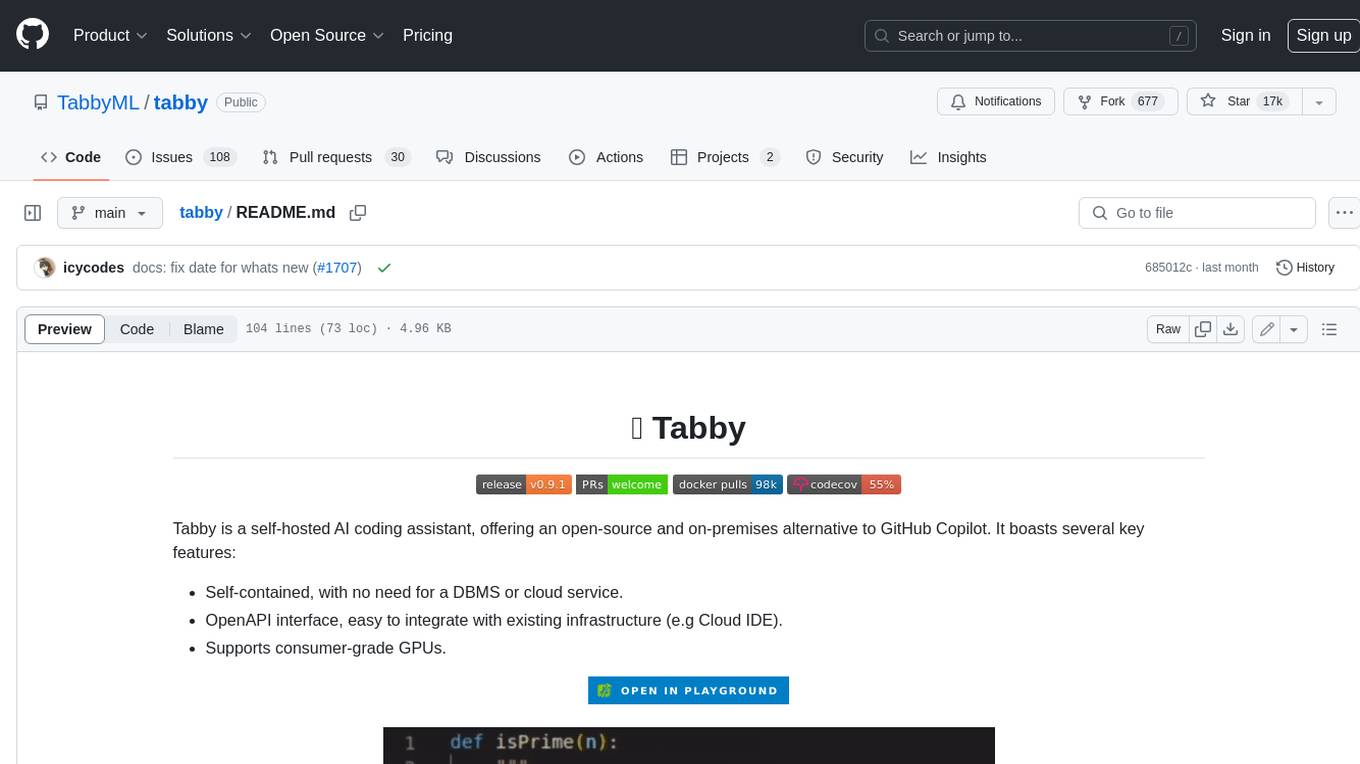
tabby
Tabby is a self-hosted AI coding assistant, offering an open-source and on-premises alternative to GitHub Copilot. It boasts several key features: * Self-contained, with no need for a DBMS or cloud service. * OpenAPI interface, easy to integrate with existing infrastructure (e.g Cloud IDE). * Supports consumer-grade GPUs.
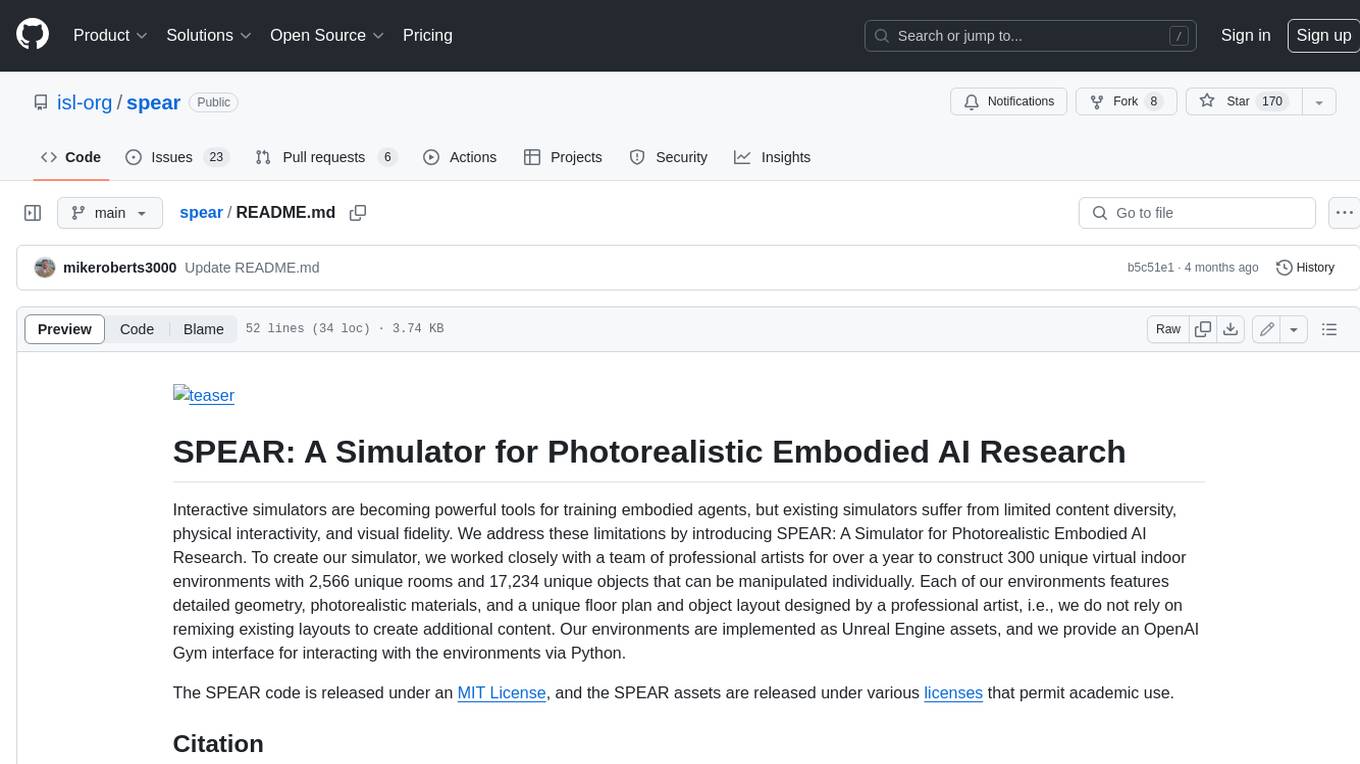
spear
SPEAR (Simulator for Photorealistic Embodied AI Research) is a powerful tool for training embodied agents. It features 300 unique virtual indoor environments with 2,566 unique rooms and 17,234 unique objects that can be manipulated individually. Each environment is designed by a professional artist and features detailed geometry, photorealistic materials, and a unique floor plan and object layout. SPEAR is implemented as Unreal Engine assets and provides an OpenAI Gym interface for interacting with the environments via Python.
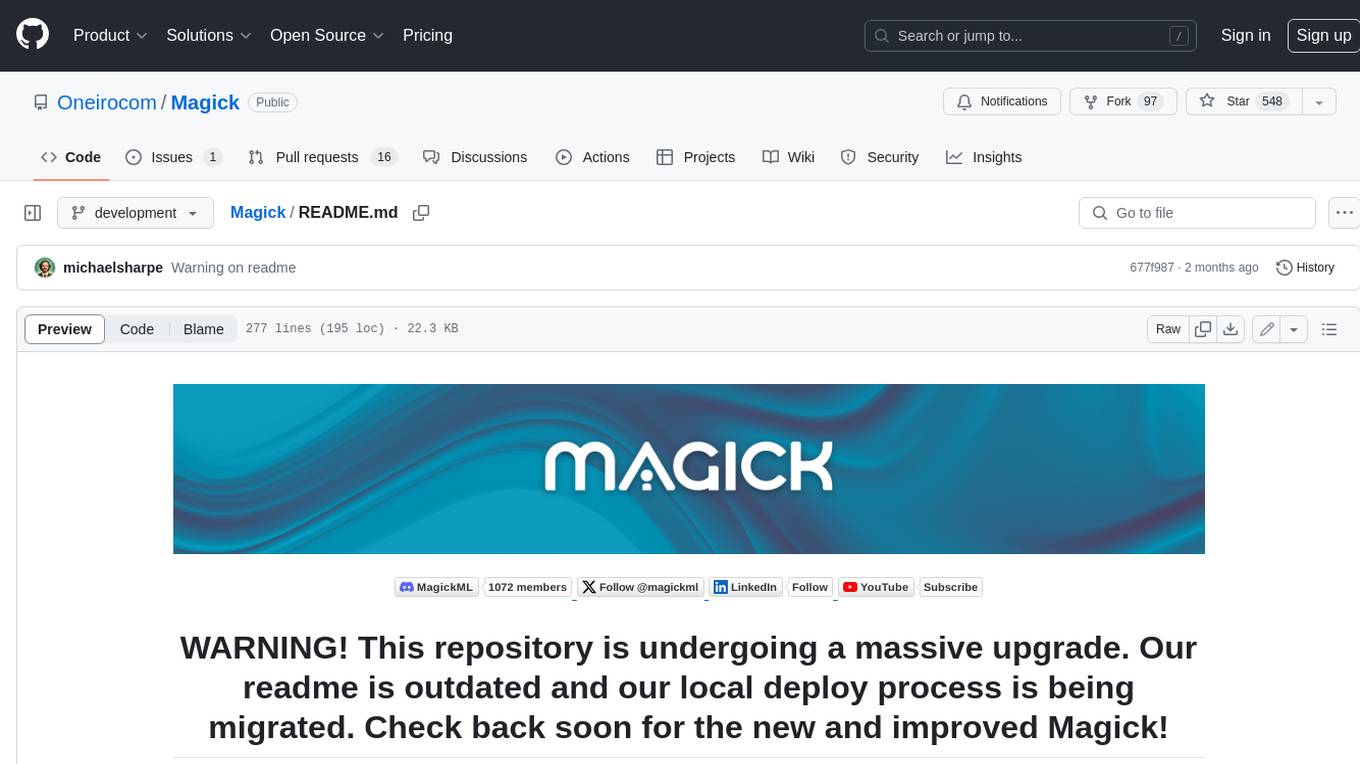
Magick
Magick is a groundbreaking visual AIDE (Artificial Intelligence Development Environment) for no-code data pipelines and multimodal agents. Magick can connect to other services and comes with nodes and templates well-suited for intelligent agents, chatbots, complex reasoning systems and realistic characters.