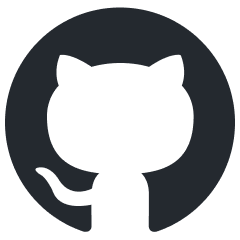
OSWorld
[NeurIPS 2024] OSWorld: Benchmarking Multimodal Agents for Open-Ended Tasks in Real Computer Environments
Stars: 1736
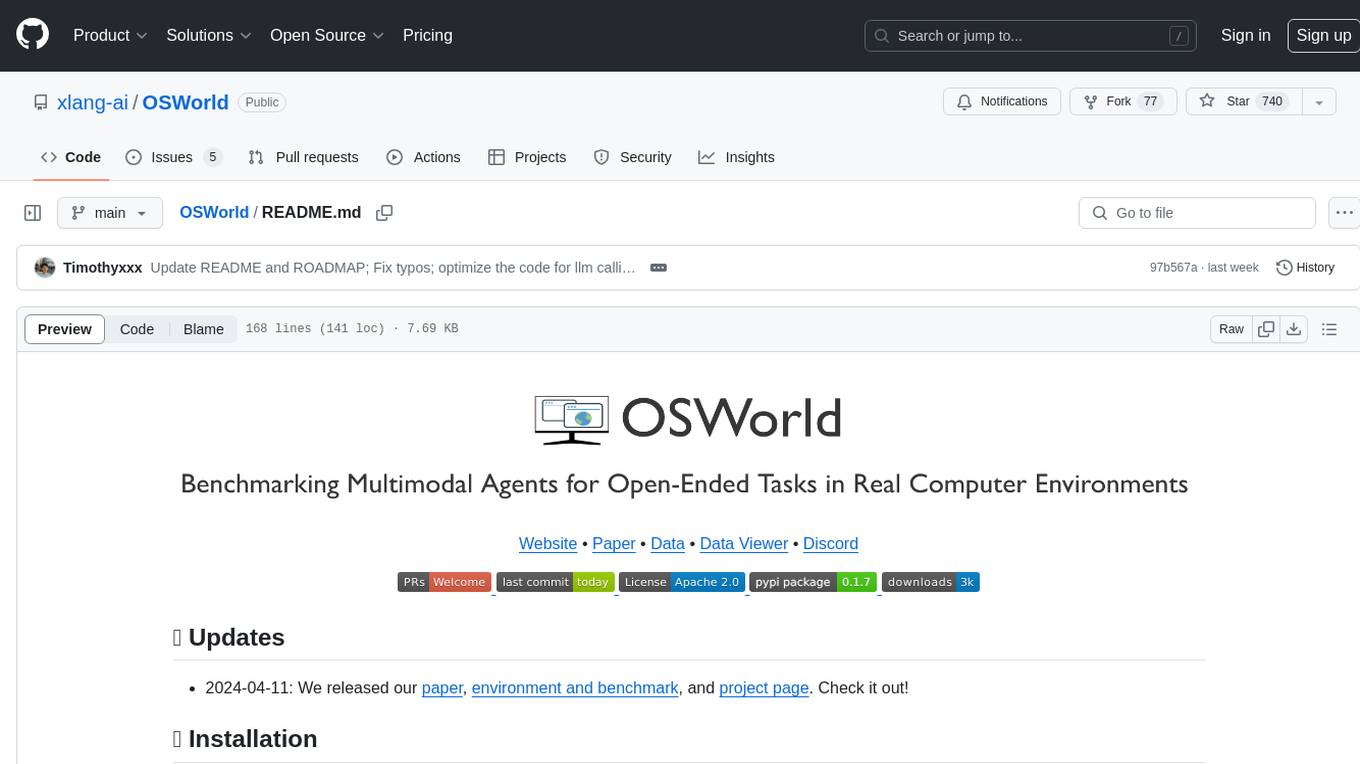
OSWorld is a benchmarking tool designed to evaluate multimodal agents for open-ended tasks in real computer environments. It provides a platform for running experiments, setting up virtual machines, and interacting with the environment using Python scripts. Users can install the tool on their desktop or server, manage dependencies with Conda, and run benchmark tasks. The tool supports actions like executing commands, checking for specific results, and evaluating agent performance. OSWorld aims to facilitate research in AI by providing a standardized environment for testing and comparing different agent baselines.
README:
Website • Paper • Doc • Data • Data Viewer • Discord
- 2024-10-22: We supported Docker🐳 for hosting virtual machines on virtualized platforms. Check below for detailed instructions!
- 2024-06-15: We refactor the code of environment part to decompose VMware Integration, and start to support other platforms such as VitualBox, AWS, Azure, etc. Hold tight!
- 2024-04-11: We released our paper, environment and benchmark, and project page. Check it out!
Suppose you are operating on a system that has not been virtualized (e.g. your desktop, laptop, bare metal machine), meaning you are not utilizing a virtualized environment like AWS, Azure, or k8s. If this is the case, proceed with the instructions below. However, if you are on a virtualized platform, please refer to the Docker section.
- First, clone this repository and
cd
into it. Then, install the dependencies listed inrequirements.txt
. It is recommended that you use the latest version of Conda to manage the environment, but you can also choose to manually install the dependencies. Please ensure that the version of Python is >= 3.9.
# Clone the OSWorld repository
git clone https://github.com/xlang-ai/OSWorld
# Change directory into the cloned repository
cd OSWorld
# Optional: Create a Conda environment for OSWorld
# conda create -n osworld python=3.9
# conda activate osworld
# Install required dependencies
pip install -r requirements.txt
Alternatively, you can install the environment without any benchmark tasks:
pip install desktop-env
- Install VMware Workstation Pro (for systems with Apple Chips, you should install VMware Fusion) and configure the
vmrun
command. The installation process can refer to How to install VMware Worksation Pro. Verify the successful installation by running the following:
vmrun -T ws list
If the installation along with the environment variable set is successful, you will see the message showing the current running virtual machines.
Note: We also support using VirtualBox if you have issues with VMware Pro. However, features such as parallelism and macOS on Apple chips might not be well-supported.
All set! Our setup script will automatically download the necessary virtual machines and configure the environment for you.
If you are running on a non-bare metal server, or prefer not to use VMware and VirtualBox platforms, we recommend using our Docker support.
We recommend running the VM with KVM support. To check if your hosting platform supports KVM, run
egrep -c '(vmx|svm)' /proc/cpuinfo
on Linux. If the return value is greater than zero, the processor should be able to support KVM.
Note: macOS hosts generally do not support KVM. You are advised to use VMware if you would like to run OSWorld on macOS.
If your hosting platform supports a graphical user interface (GUI), you may refer to Install Docker Desktop on Linux or Install Docker Desktop on Windows based on your OS. Otherwise, you may Install Docker Engine.
Add the following arguments when initializing DesktopEnv
:
-
provider_name
:docker
-
os_type
:Ubuntu
orWindows
, depending on the OS of the VM
Note: If the experiment is interrupted abnormally (e.g., by interrupting signals), there may be residual docker containers which could affect system performance over time. Please run
docker stop $(docker ps -q) && docker rm $(docker ps -a -q)
to clean up.
We are working on supporting more 👷. Please hold tight!
Run the following minimal example to interact with the environment:
from desktop_env.desktop_env import DesktopEnv
example = {
"id": "94d95f96-9699-4208-98ba-3c3119edf9c2",
"instruction": "I want to install Spotify on my current system. Could you please help me?",
"config": [
{
"type": "execute",
"parameters": {
"command": [
"python",
"-c",
"import pyautogui; import time; pyautogui.click(960, 540); time.sleep(0.5);"
]
}
}
],
"evaluator": {
"func": "check_include_exclude",
"result": {
"type": "vm_command_line",
"command": "which spotify"
},
"expected": {
"type": "rule",
"rules": {
"include": ["spotify"],
"exclude": ["not found"]
}
}
}
}
env = DesktopEnv(action_space="pyautogui")
obs = env.reset(task_config=example)
obs, reward, done, info = env.step("pyautogui.rightClick()")
You will see all the logs of the system running normally, including the successful creation of the environment, completion of setup, and successful execution of actions. In the end, you will observe a successful right-click on the screen, which means you are ready to go.
If you wish to run the baseline agent used in our paper, you can execute the following command as an example under the GPT-4V pure-screenshot setting:
Set OPENAI_API_KEY environment variable with your API key
export OPENAI_API_KEY='changeme'
python run.py --path_to_vm Ubuntu/Ubuntu.vmx --headless --observation_type screenshot --model gpt-4-vision-preview --result_dir ./results
The results, which include screenshots, actions, and video recordings of the agent's task completion, will be saved in the ./results
directory in this case. You can then run the following command to obtain the result:
python show_result.py
Please start by reading through the agent interface and the environment interface.
Correctly implement the agent interface and import your customized version in the run.py
file.
Afterward, you can execute a command similar to the one in the previous section to run the benchmark on your agent.
The username and password for the virtual machines are as follows:
-
Ubuntu:
user
/password
See Account Guideline.
See Proxy Guideline.
Setting | Expected Time* | Budget Cost (Full Test Set/Small Test Set) |
---|---|---|
GPT-4V (screenshot) | 10h | $100 ($10) |
Gemini-ProV (screenshot) | 15h | $0 ($0) |
Claude-3 Opus (screenshot) | 15h | $150 ($15) |
GPT-4V (a11y tree, SoM, etc.) | 30h | $500 ($50) |
*No environment parallelism. Calculated in April 2024.
Thanks to all the contributors!
If you find this environment useful, please consider citing our work:
@misc{OSWorld,
title={OSWorld: Benchmarking Multimodal Agents for Open-Ended Tasks in Real Computer Environments},
author={Tianbao Xie and Danyang Zhang and Jixuan Chen and Xiaochuan Li and Siheng Zhao and Ruisheng Cao and Toh Jing Hua and Zhoujun Cheng and Dongchan Shin and Fangyu Lei and Yitao Liu and Yiheng Xu and Shuyan Zhou and Silvio Savarese and Caiming Xiong and Victor Zhong and Tao Yu},
year={2024},
eprint={2404.07972},
archivePrefix={arXiv},
primaryClass={cs.AI}
}
For Tasks:
Click tags to check more tools for each tasksFor Jobs:
Alternative AI tools for OSWorld
Similar Open Source Tools
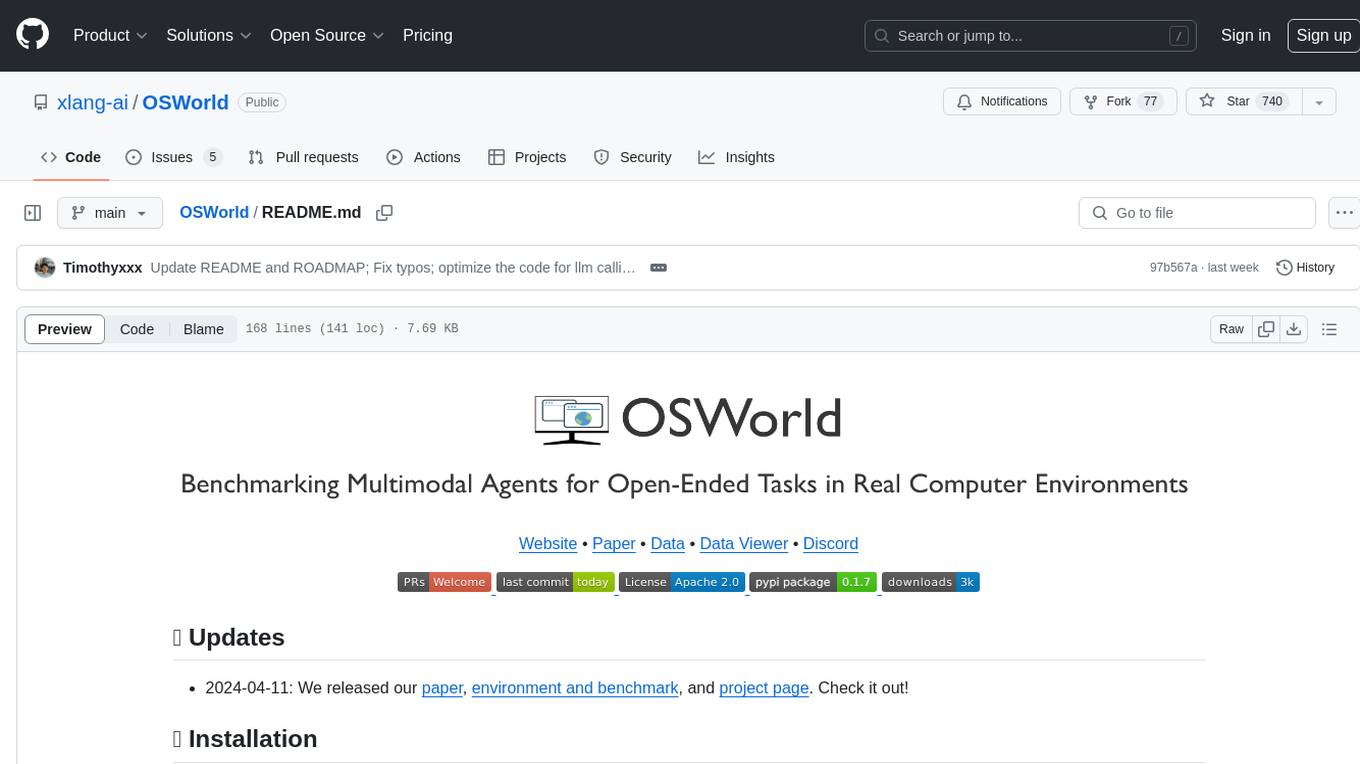
OSWorld
OSWorld is a benchmarking tool designed to evaluate multimodal agents for open-ended tasks in real computer environments. It provides a platform for running experiments, setting up virtual machines, and interacting with the environment using Python scripts. Users can install the tool on their desktop or server, manage dependencies with Conda, and run benchmark tasks. The tool supports actions like executing commands, checking for specific results, and evaluating agent performance. OSWorld aims to facilitate research in AI by providing a standardized environment for testing and comparing different agent baselines.
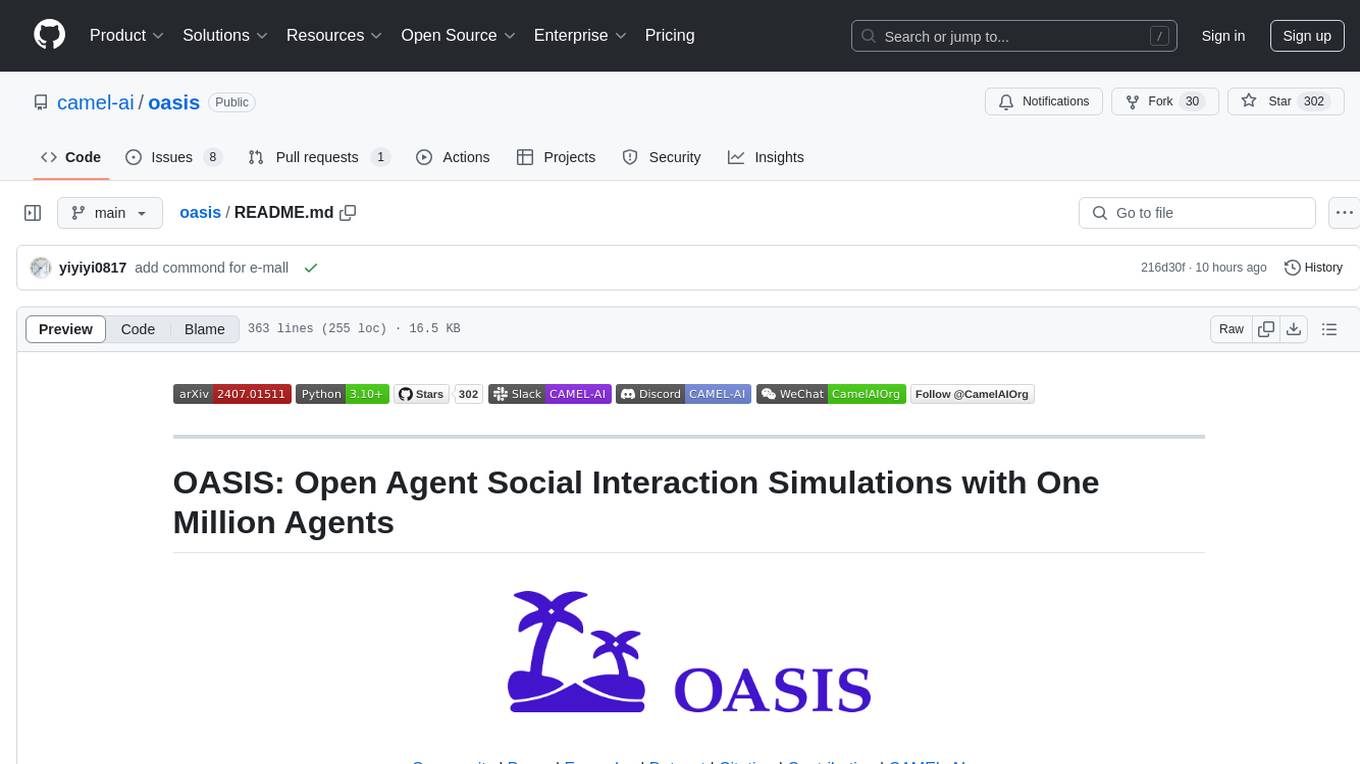
oasis
OASIS is a scalable, open-source social media simulator that integrates large language models with rule-based agents to realistically mimic the behavior of up to one million users on platforms like Twitter and Reddit. It facilitates the study of complex social phenomena such as information spread, group polarization, and herd behavior, offering a versatile tool for exploring diverse social dynamics and user interactions in digital environments. With features like scalability, dynamic environments, diverse action spaces, and integrated recommendation systems, OASIS provides a comprehensive platform for simulating social media interactions at a large scale.
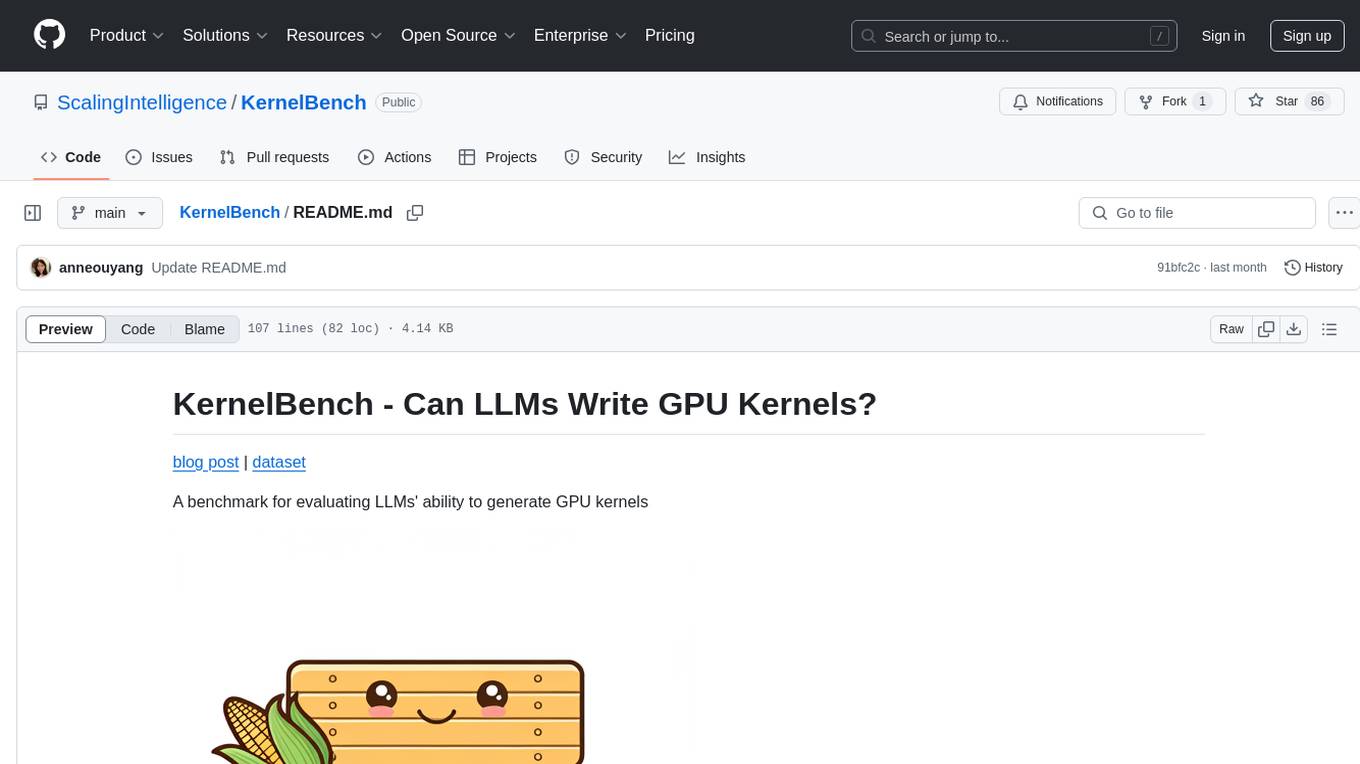
KernelBench
KernelBench is a benchmark tool designed to evaluate Large Language Models' (LLMs) ability to generate GPU kernels. It focuses on transpiling operators from PyTorch to CUDA kernels at different levels of granularity. The tool categorizes problems into four levels, ranging from single-kernel operators to full model architectures, and assesses solutions based on compilation, correctness, and speed. The repository provides a structured directory layout, setup instructions, usage examples for running single or multiple problems, and upcoming roadmap features like additional GPU platform support and integration with other frameworks.
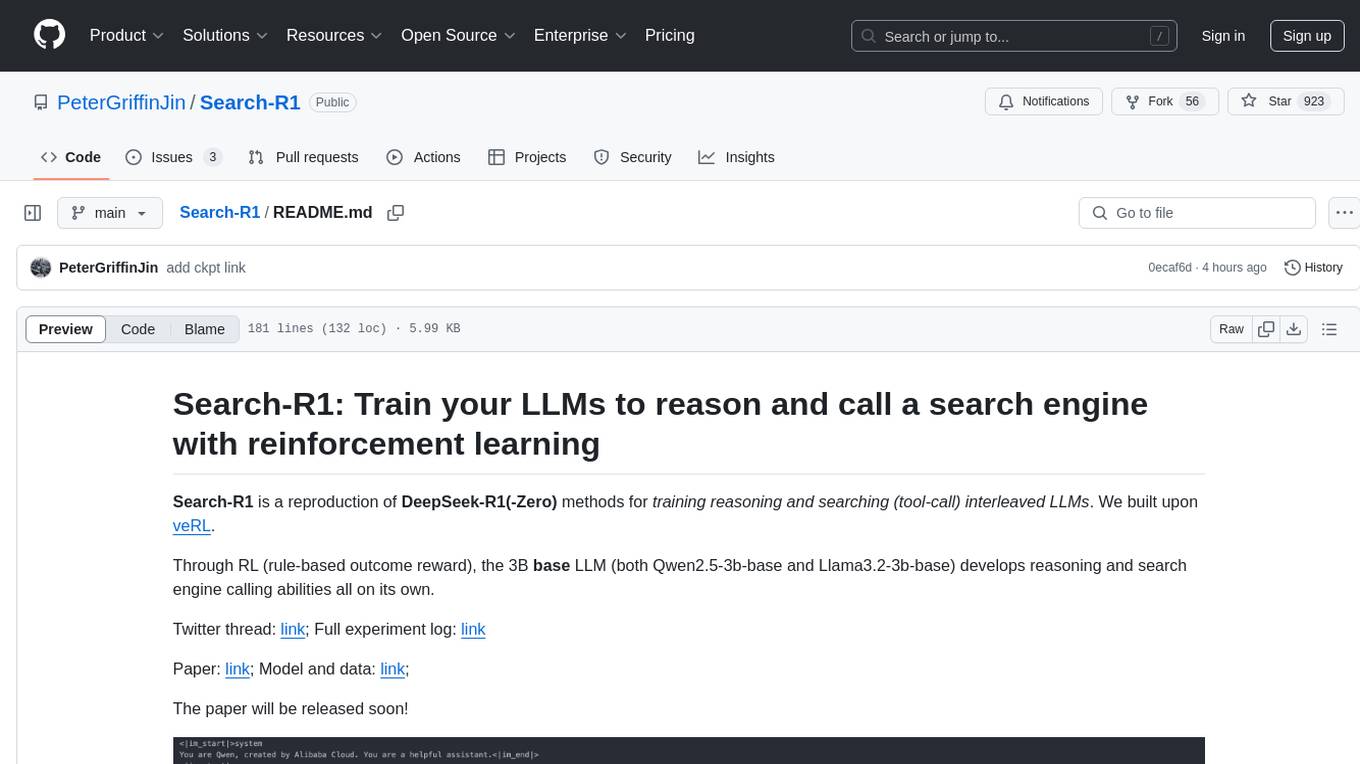
Search-R1
Search-R1 is a tool that trains large language models (LLMs) to reason and call a search engine using reinforcement learning. It is a reproduction of DeepSeek-R1 methods for training reasoning and searching interleaved LLMs, built upon veRL. Through rule-based outcome reward, the base LLM develops reasoning and search engine calling abilities independently. Users can train LLMs on their own datasets and search engines, with preliminary results showing improved performance in search engine calling and reasoning tasks.
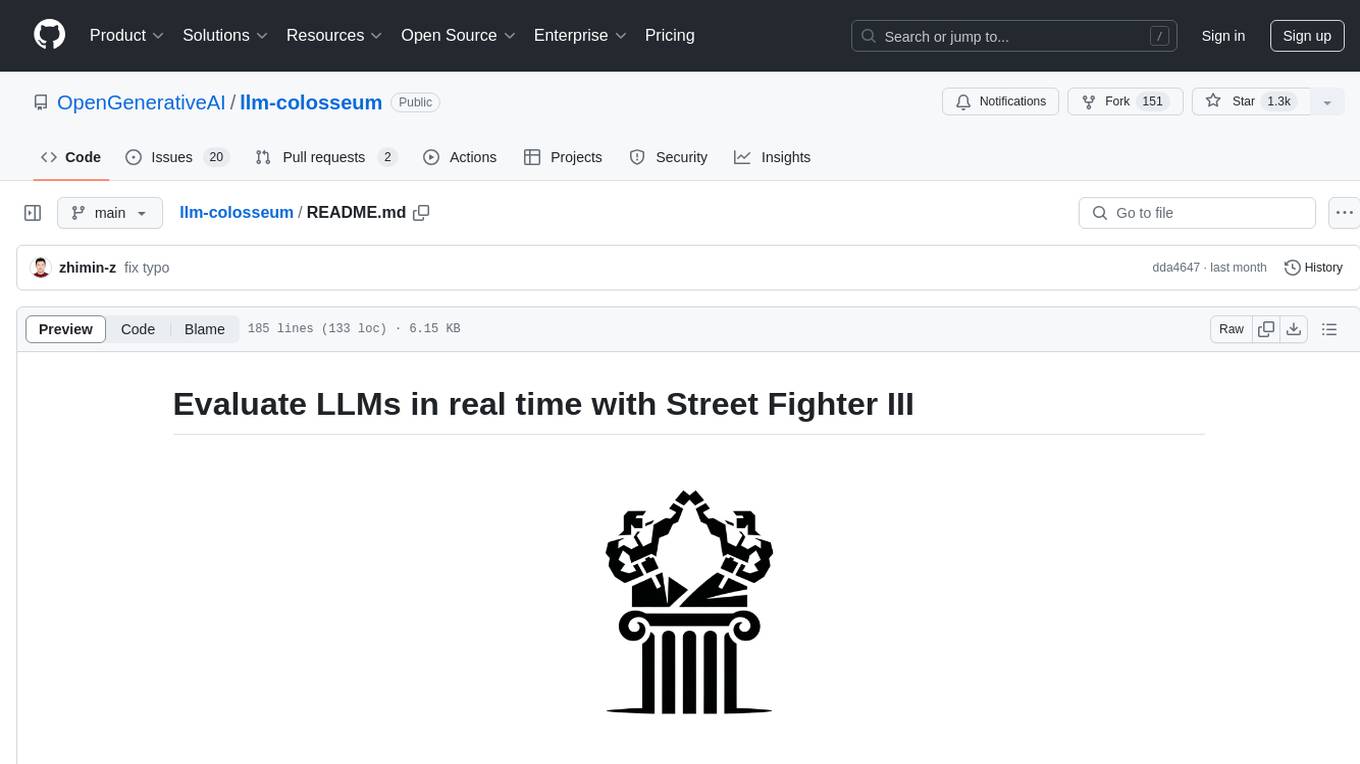
llm-colosseum
llm-colosseum is a tool designed to evaluate Language Model Models (LLMs) in real-time by making them fight each other in Street Fighter III. The tool assesses LLMs based on speed, strategic thinking, adaptability, out-of-the-box thinking, and resilience. It provides a benchmark for LLMs to understand their environment and take context-based actions. Users can analyze the performance of different LLMs through ELO rankings and win rate matrices. The tool allows users to run experiments, test different LLM models, and customize prompts for LLM interactions. It offers installation instructions, test mode options, logging configurations, and the ability to run the tool with local models. Users can also contribute their own LLM models for evaluation and ranking.
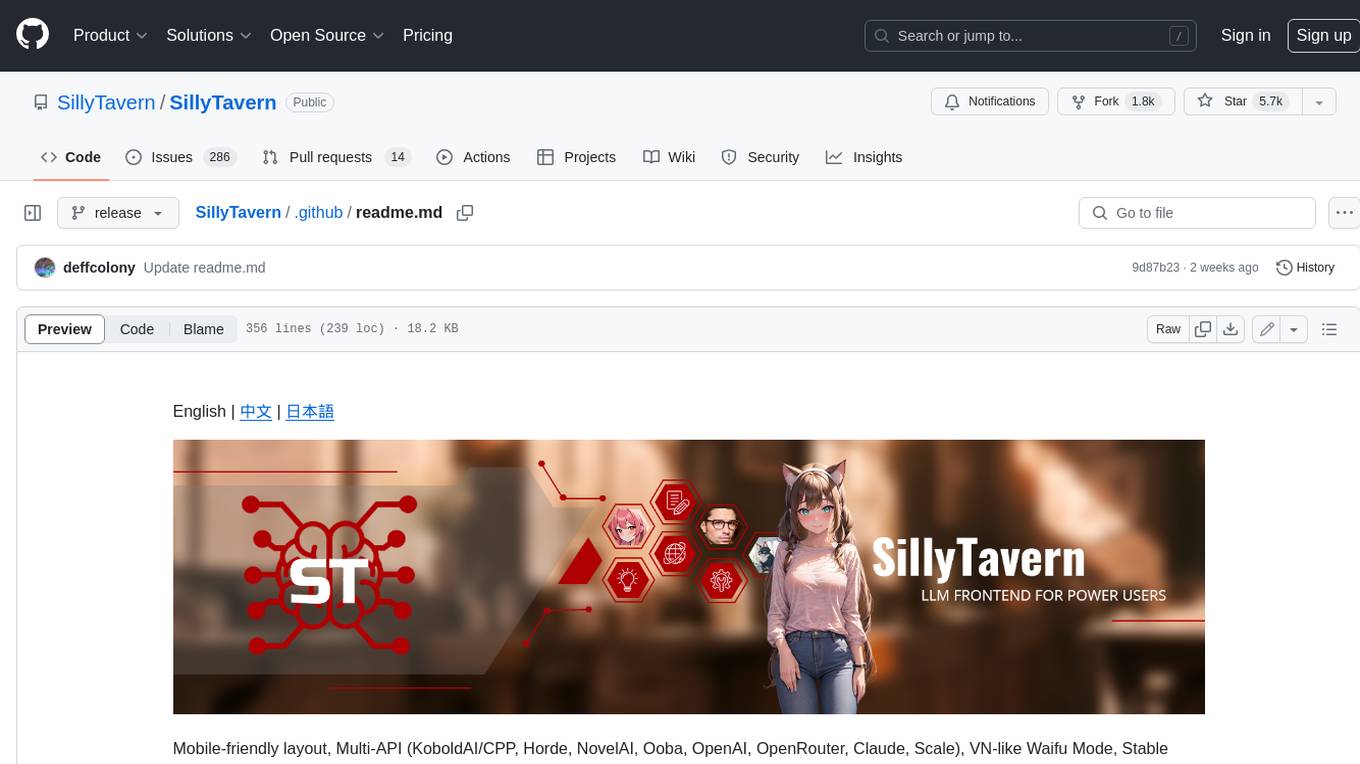
SillyTavern
SillyTavern is a user interface you can install on your computer (and Android phones) that allows you to interact with text generation AIs and chat/roleplay with characters you or the community create. SillyTavern is a fork of TavernAI 1.2.8 which is under more active development and has added many major features. At this point, they can be thought of as completely independent programs.
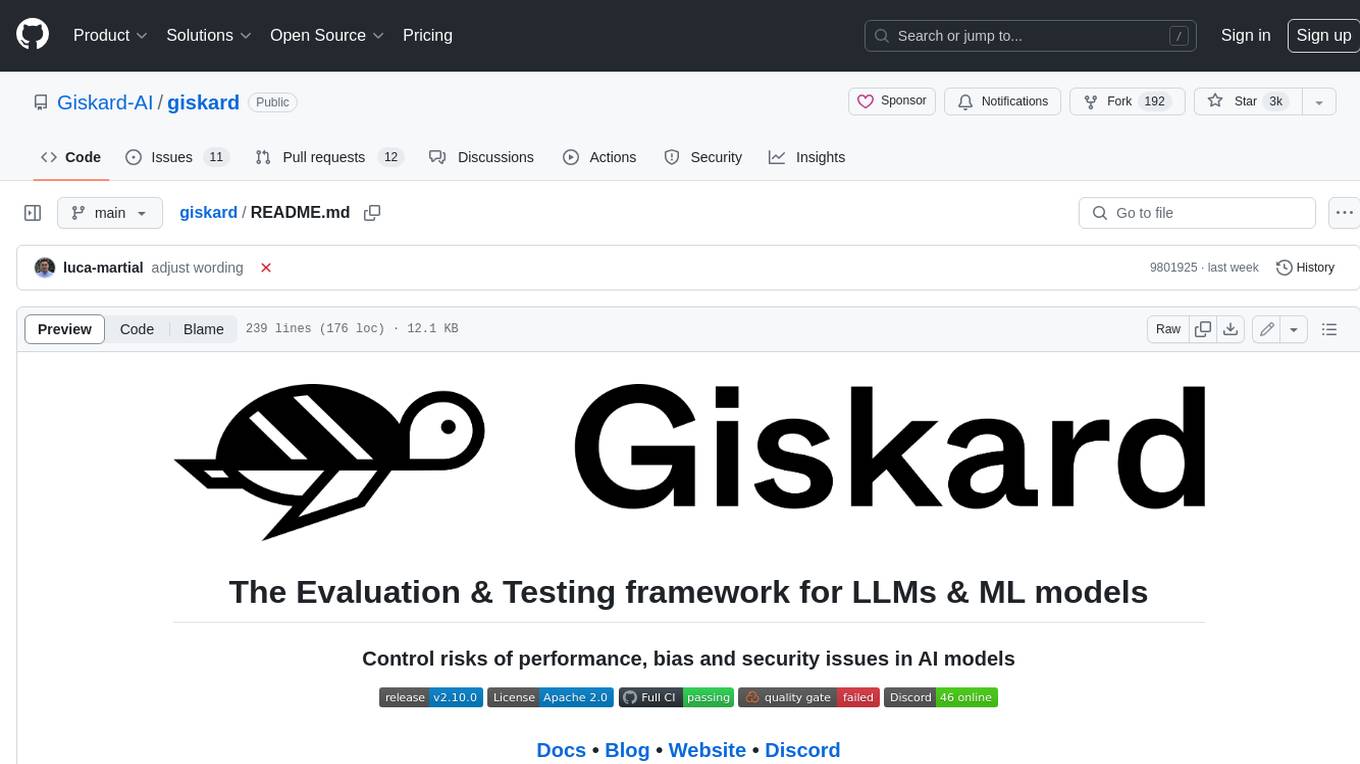
giskard
Giskard is an open-source Python library that automatically detects performance, bias & security issues in AI applications. The library covers LLM-based applications such as RAG agents, all the way to traditional ML models for tabular data.
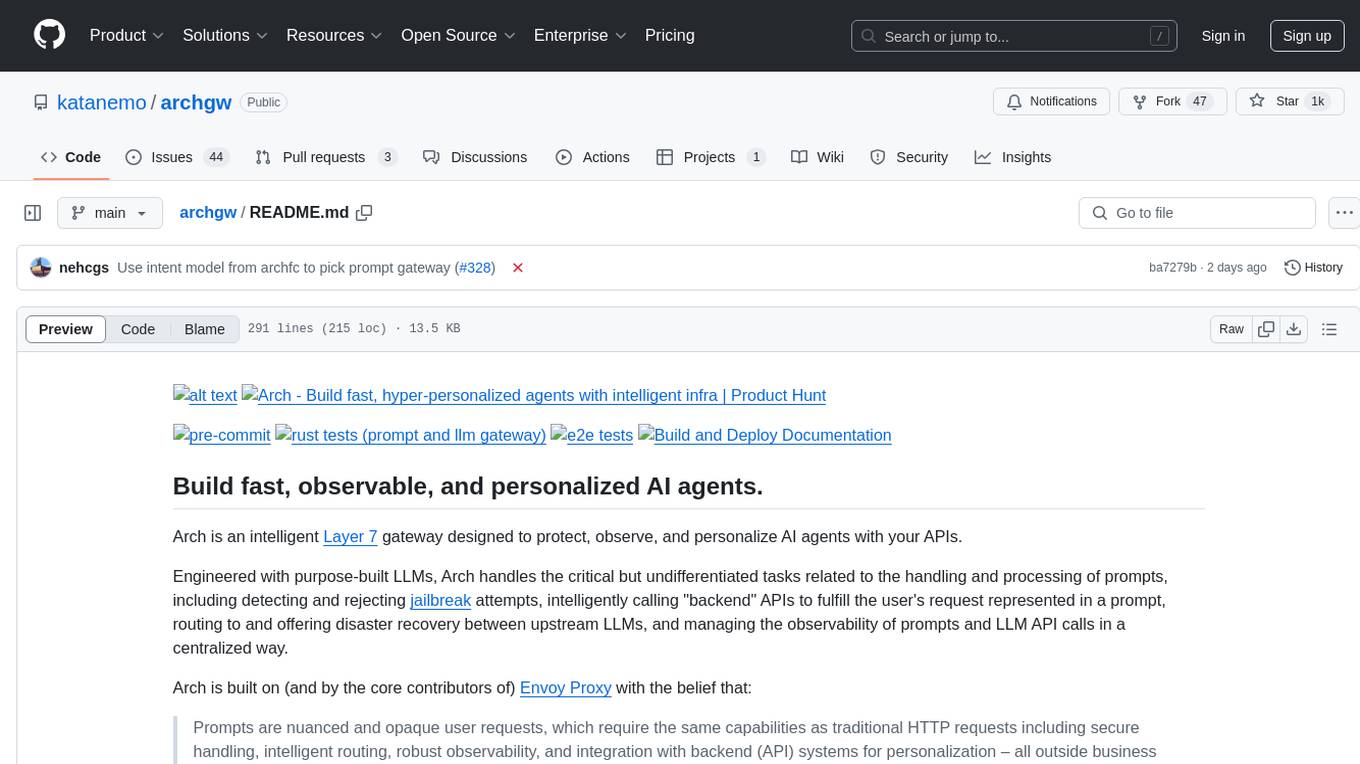
archgw
Arch is an intelligent Layer 7 gateway designed to protect, observe, and personalize AI agents with APIs. It handles tasks related to prompts, including detecting jailbreak attempts, calling backend APIs, routing between LLMs, and managing observability. Built on Envoy Proxy, it offers features like function calling, prompt guardrails, traffic management, and observability. Users can build fast, observable, and personalized AI agents using Arch to improve speed, security, and personalization of GenAI apps.
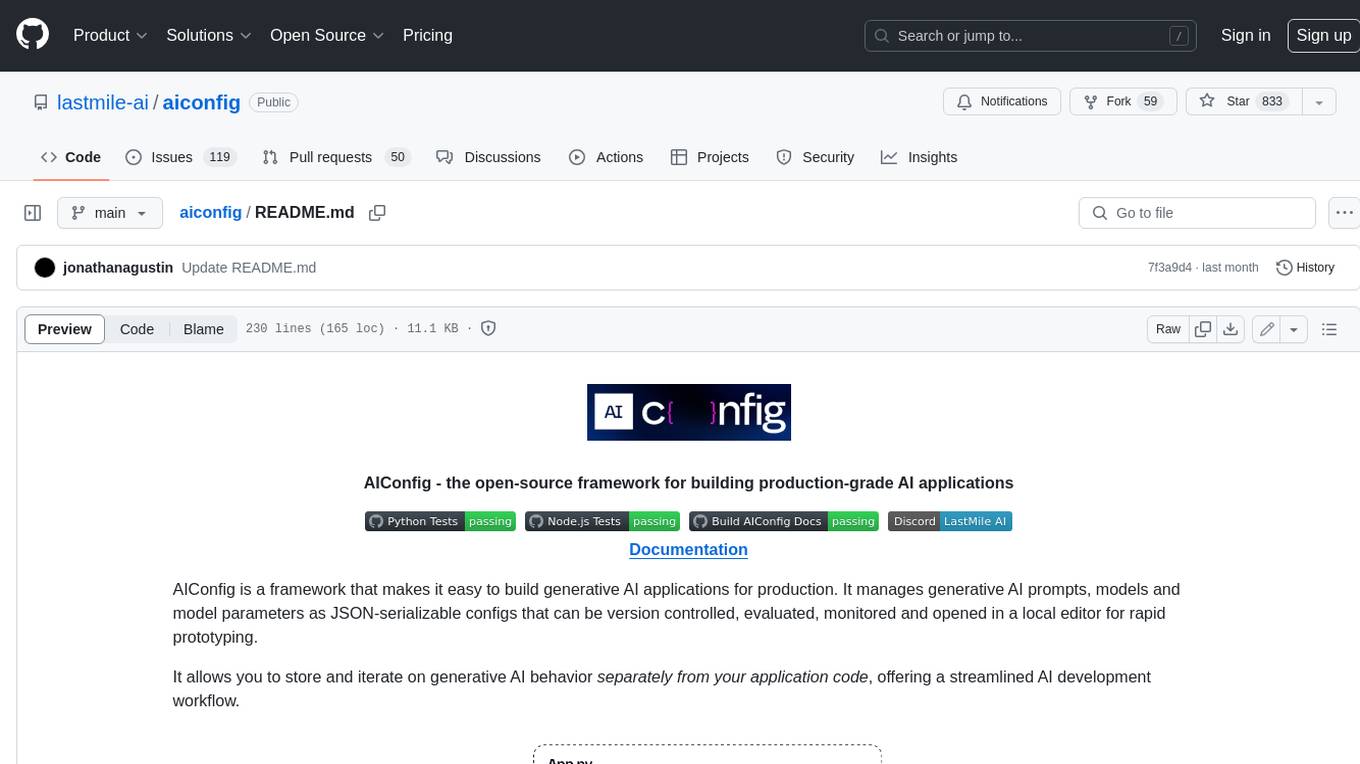
aiconfig
AIConfig is a framework that makes it easy to build generative AI applications for production. It manages generative AI prompts, models and model parameters as JSON-serializable configs that can be version controlled, evaluated, monitored and opened in a local editor for rapid prototyping. It allows you to store and iterate on generative AI behavior separately from your application code, offering a streamlined AI development workflow.
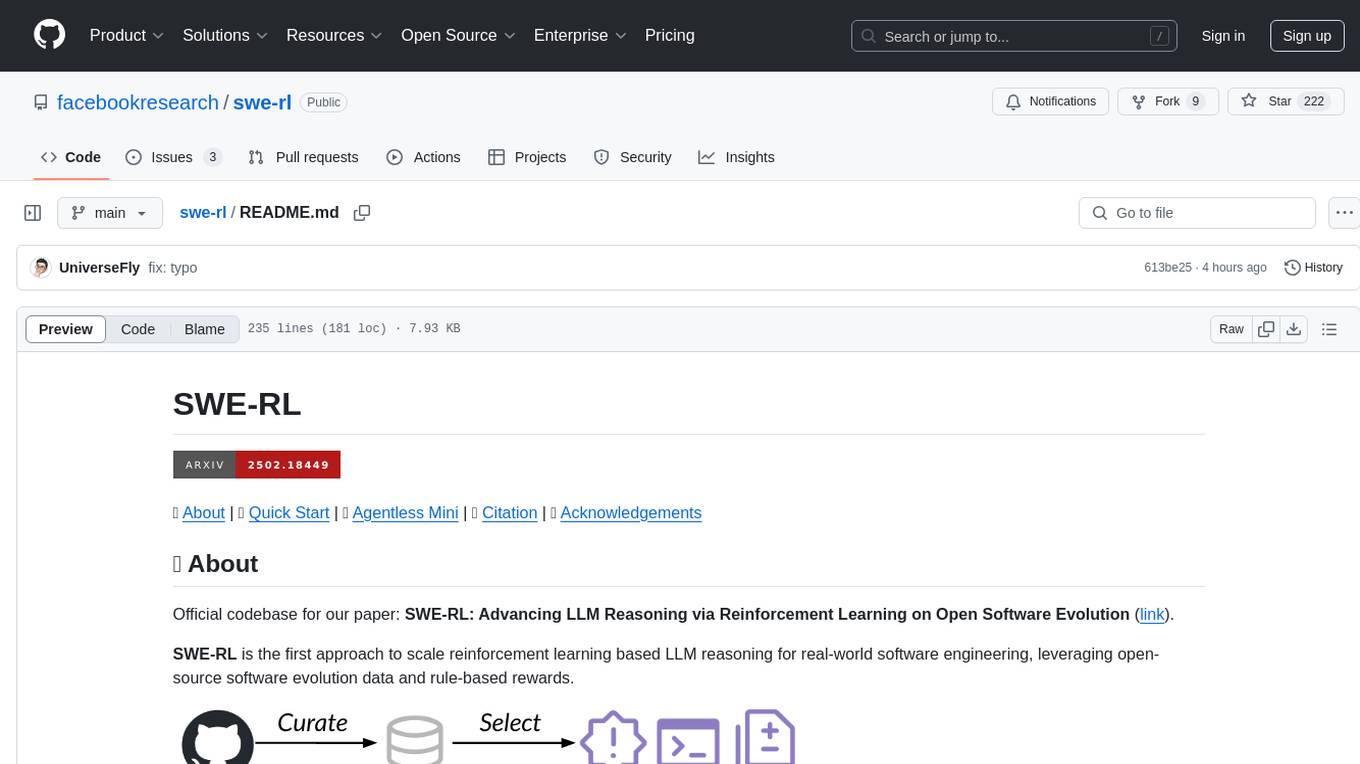
swe-rl
SWE-RL is the official codebase for the paper 'SWE-RL: Advancing LLM Reasoning via Reinforcement Learning on Open Software Evolution'. It is the first approach to scale reinforcement learning based LLM reasoning for real-world software engineering, leveraging open-source software evolution data and rule-based rewards. The code provides prompt templates and the implementation of the reward function based on sequence similarity. Agentless Mini, a part of SWE-RL, builds on top of Agentless with improvements like fast async inference, code refactoring for scalability, and support for using multiple reproduction tests for reranking. The tool can be used for localization, repair, and reproduction test generation in software engineering tasks.
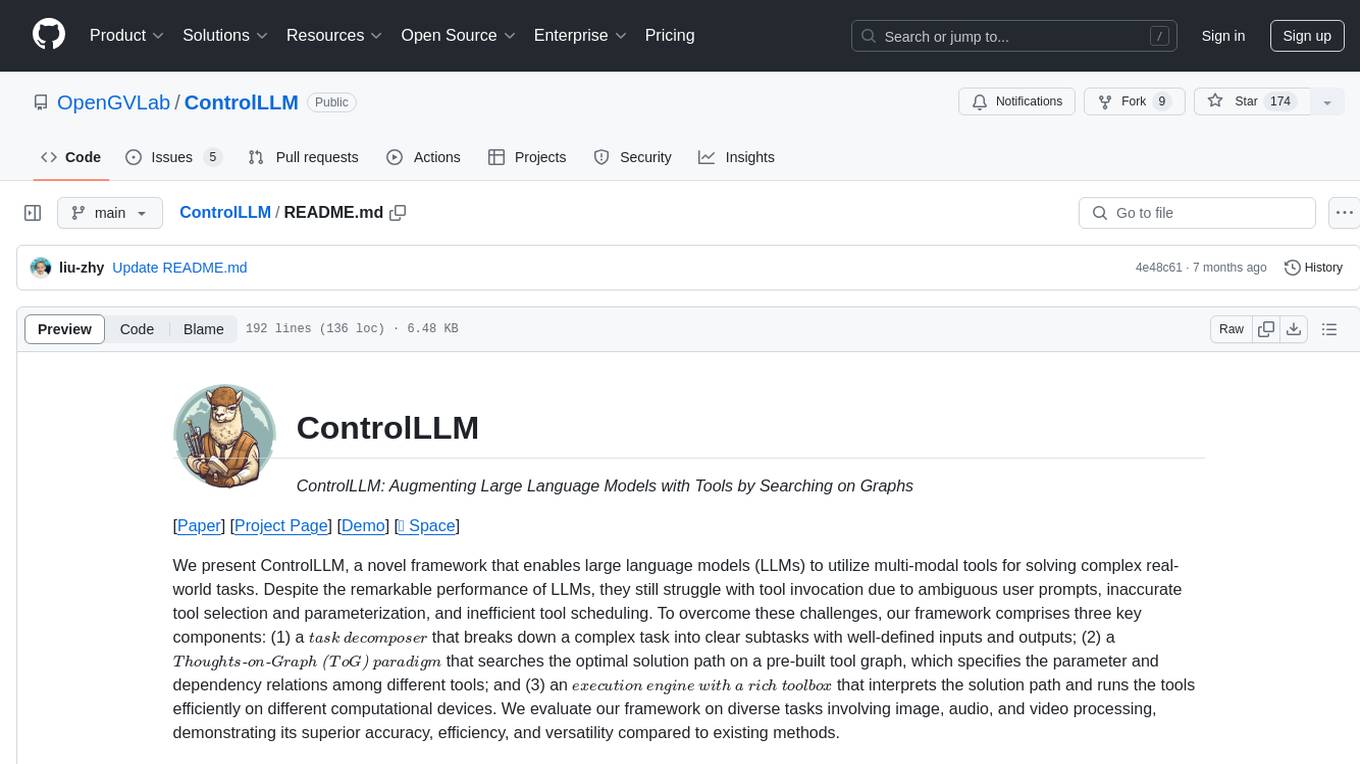
ControlLLM
ControlLLM is a framework that empowers large language models to leverage multi-modal tools for solving complex real-world tasks. It addresses challenges like ambiguous user prompts, inaccurate tool selection, and inefficient tool scheduling by utilizing a task decomposer, a Thoughts-on-Graph paradigm, and an execution engine with a rich toolbox. The framework excels in tasks involving image, audio, and video processing, showcasing superior accuracy, efficiency, and versatility compared to existing methods.
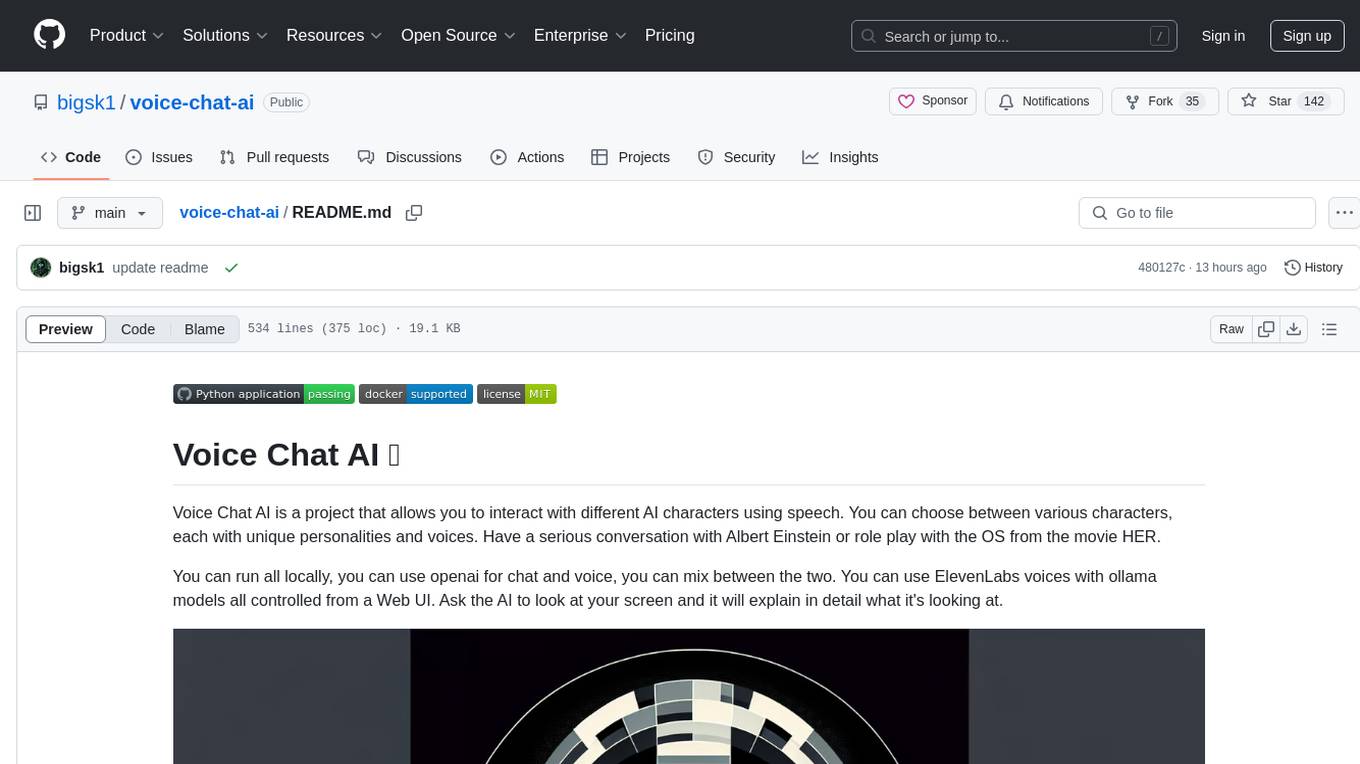
voice-chat-ai
Voice Chat AI is a project that allows users to interact with different AI characters using speech. Users can choose from various characters with unique personalities and voices, and have conversations or role play with them. The project supports OpenAI, xAI, or Ollama language models for chat, and provides text-to-speech synthesis using XTTS, OpenAI TTS, or ElevenLabs. Users can seamlessly integrate visual context into conversations by having the AI analyze their screen. The project offers easy configuration through environment variables and can be run via WebUI or Terminal. It also includes a huge selection of built-in characters for engaging conversations.
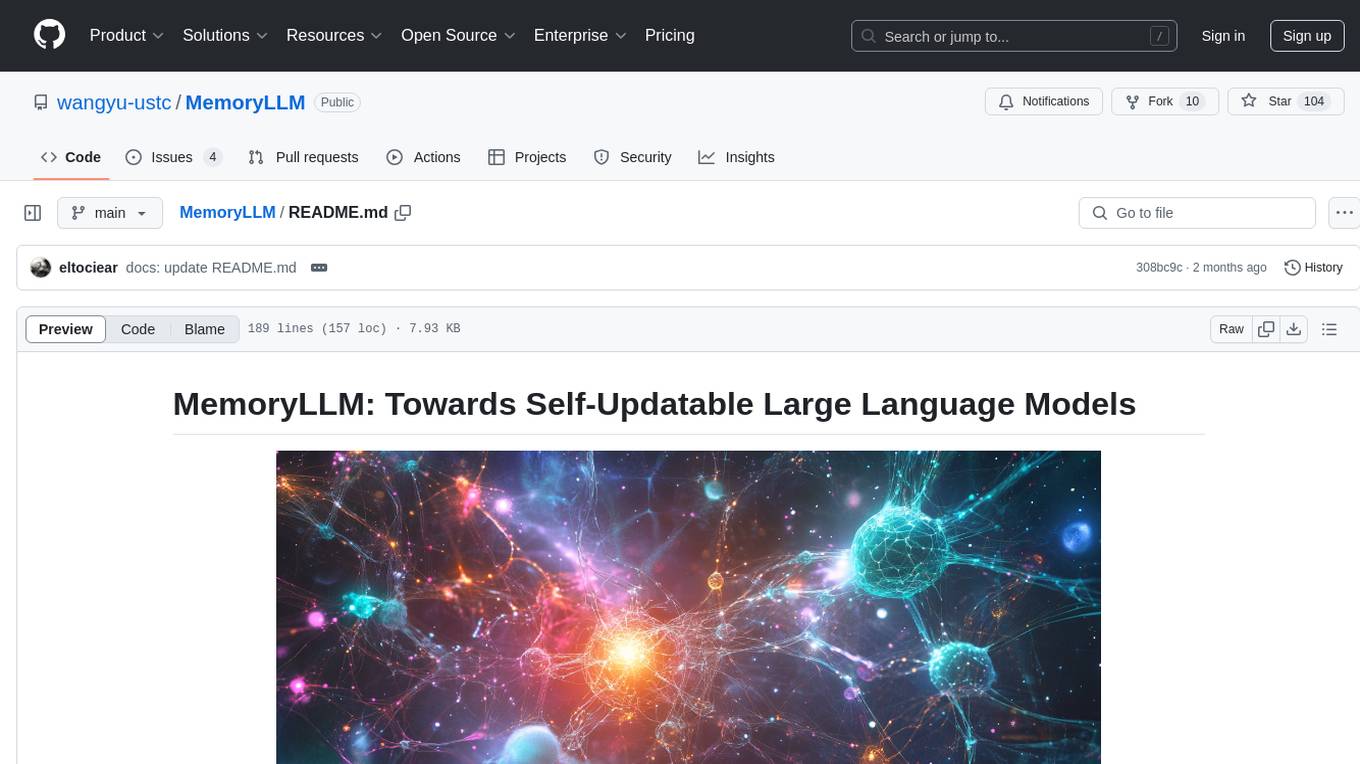
MemoryLLM
MemoryLLM is a large language model designed for self-updating capabilities. It offers pretrained models with different memory capacities and features, such as chat models. The repository provides training code, evaluation scripts, and datasets for custom experiments. MemoryLLM aims to enhance knowledge retention and performance on various natural language processing tasks.
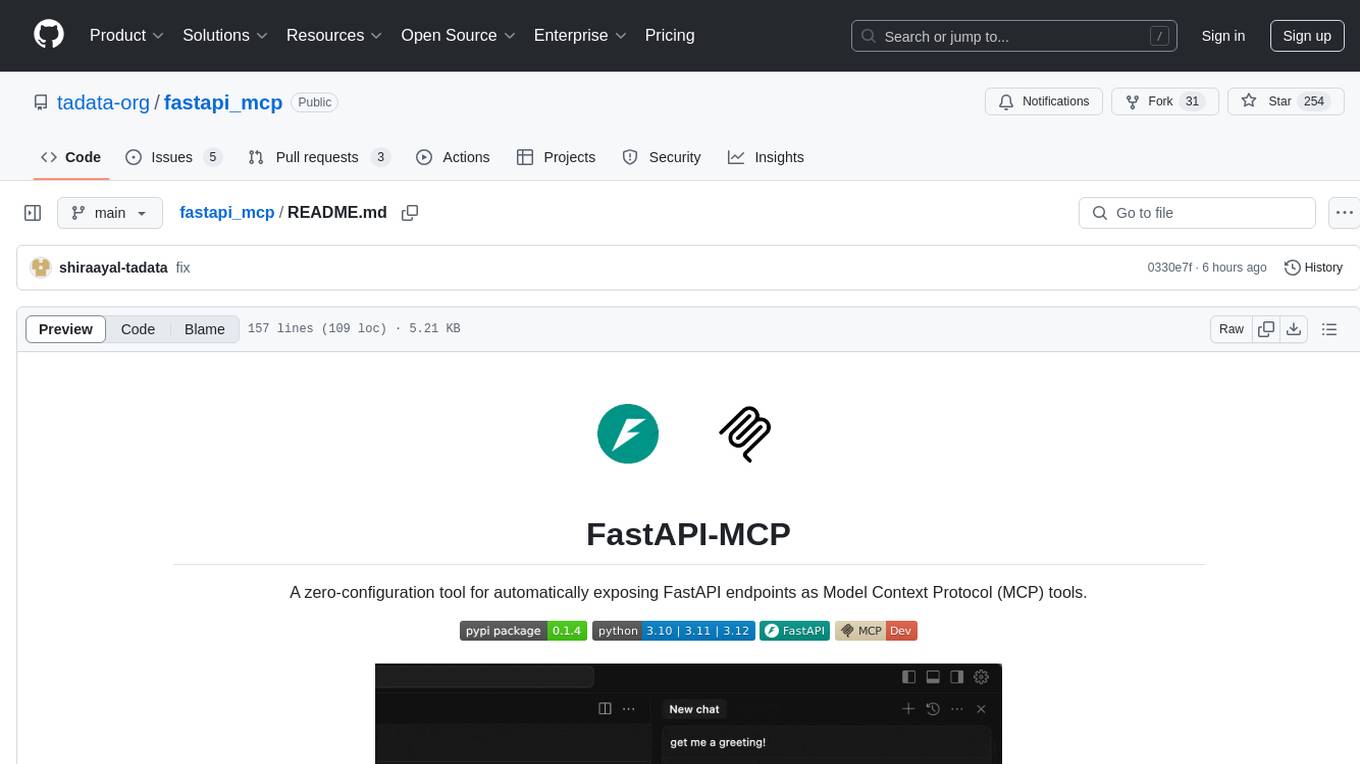
fastapi_mcp
FastAPI-MCP is a zero-configuration tool that automatically exposes FastAPI endpoints as Model Context Protocol (MCP) tools. It allows for direct integration with FastAPI apps, automatic discovery and conversion of endpoints to MCP tools, preservation of request and response schemas, documentation preservation similar to Swagger, and the ability to extend with custom MCP tools. Users can easily add an MCP server to their FastAPI application and customize the server creation and configuration. The tool supports connecting to the MCP server using SSE or mcp-proxy stdio for different MCP clients. FastAPI-MCP is developed and maintained by Tadata Inc.
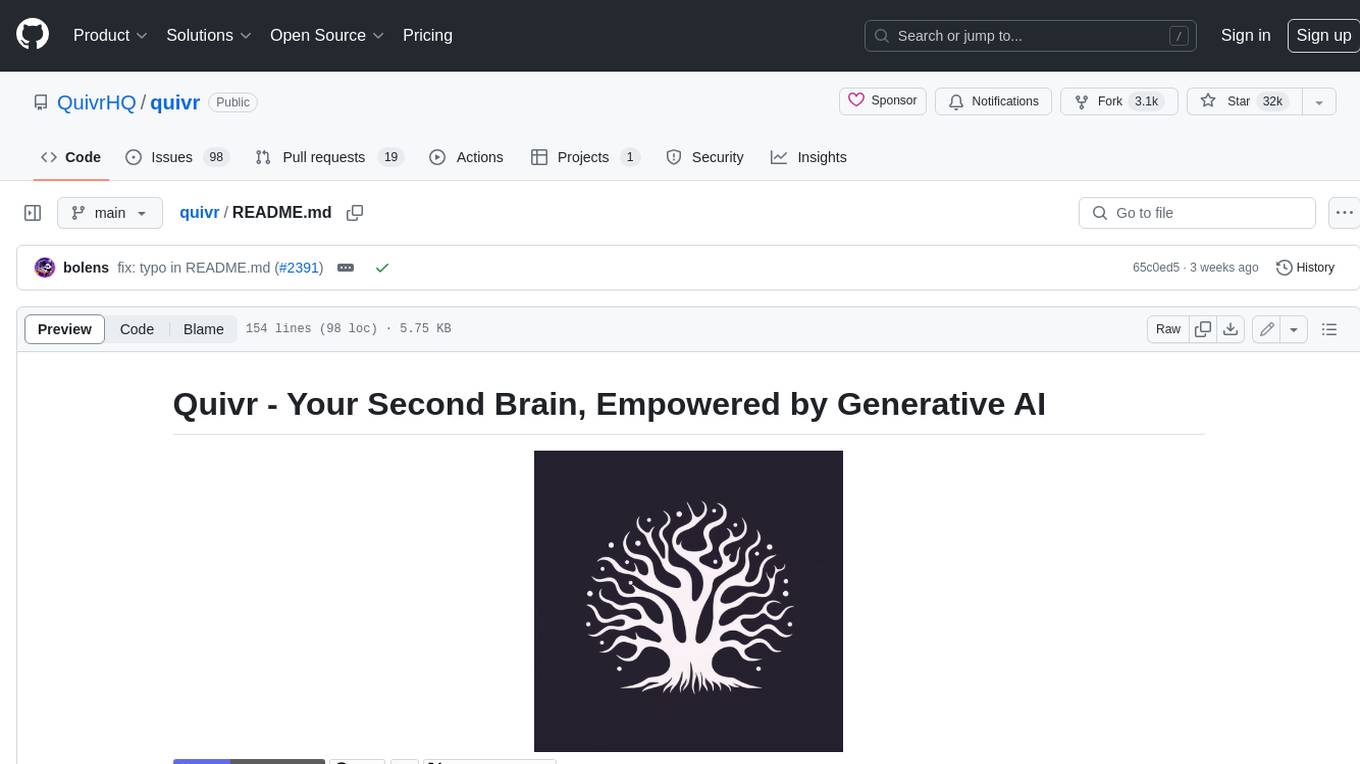
quivr
Quivr is a personal assistant powered by Generative AI, designed to be a second brain for users. It offers fast and efficient access to data, ensuring security and compatibility with various file formats. Quivr is open source and free to use, allowing users to share their brains publicly or keep them private. The marketplace feature enables users to share and utilize brains created by others, boosting productivity. Quivr's offline mode provides anytime, anywhere access to data. Key features include speed, security, OS compatibility, file compatibility, open source nature, public/private sharing options, a marketplace, and offline mode.
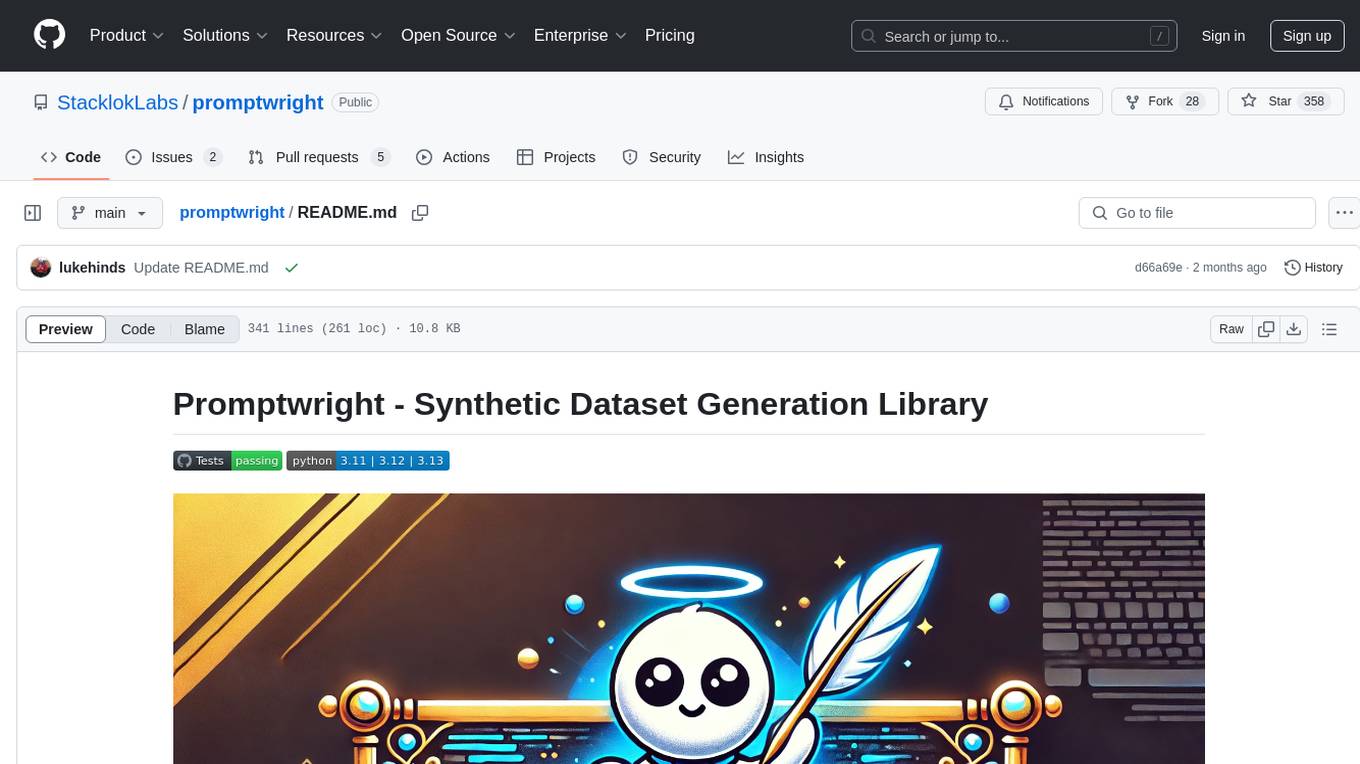
promptwright
Promptwright is a Python library designed for generating large synthetic datasets using a local LLM and various LLM service providers. It offers flexible interfaces for generating prompt-led synthetic datasets. The library supports multiple providers, configurable instructions and prompts, YAML configuration for tasks, command line interface for running tasks, push to Hugging Face Hub for dataset upload, and system message control. Users can define generation tasks using YAML configuration or Python code. Promptwright integrates with LiteLLM to interface with LLM providers and supports automatic dataset upload to Hugging Face Hub.
For similar tasks
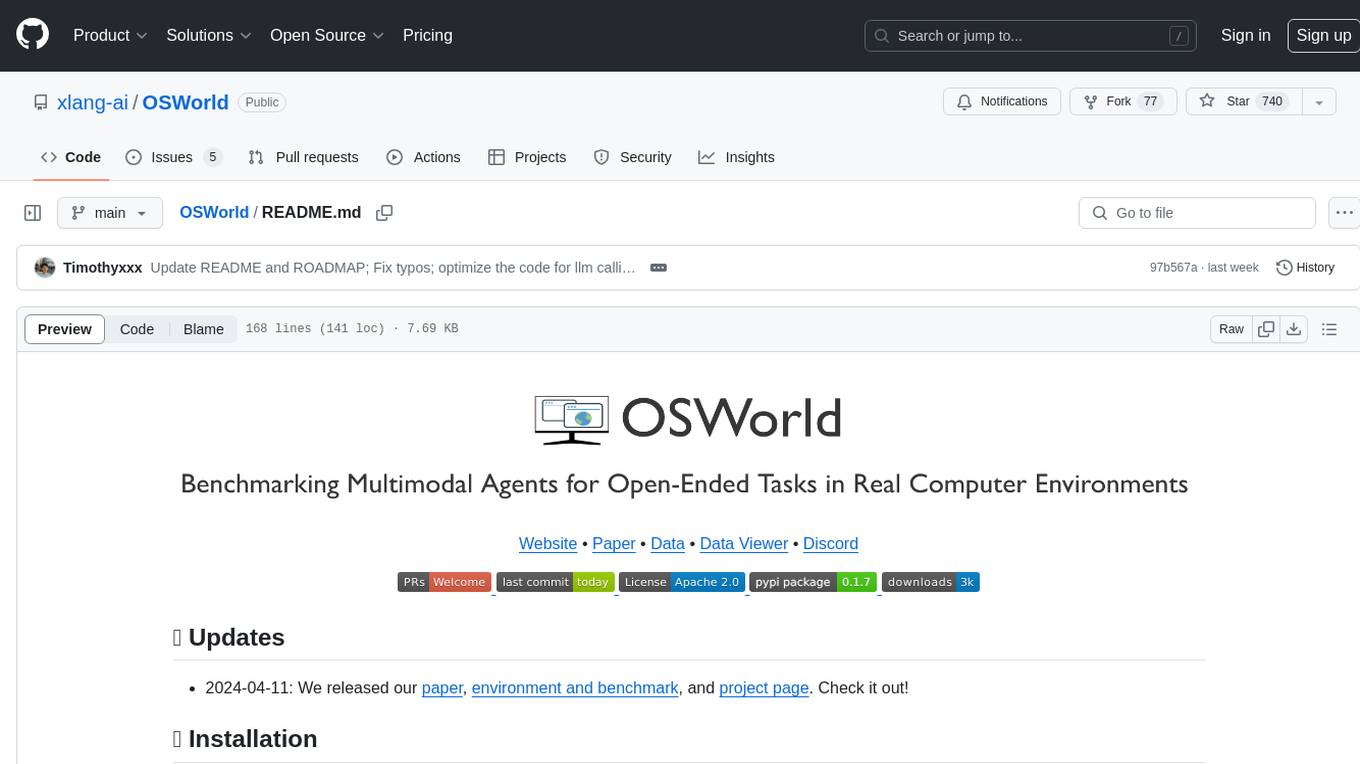
OSWorld
OSWorld is a benchmarking tool designed to evaluate multimodal agents for open-ended tasks in real computer environments. It provides a platform for running experiments, setting up virtual machines, and interacting with the environment using Python scripts. Users can install the tool on their desktop or server, manage dependencies with Conda, and run benchmark tasks. The tool supports actions like executing commands, checking for specific results, and evaluating agent performance. OSWorld aims to facilitate research in AI by providing a standardized environment for testing and comparing different agent baselines.
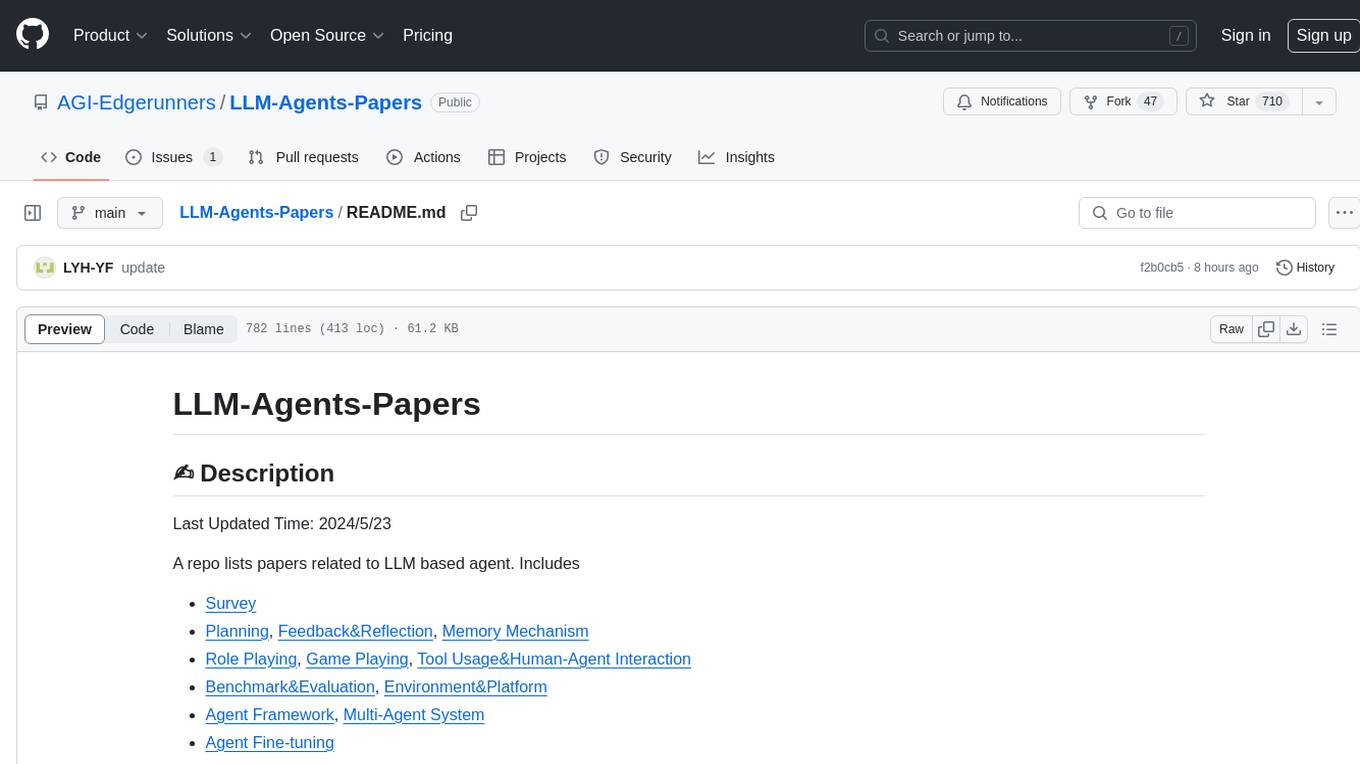
LLM-Agents-Papers
A repository that lists papers related to Large Language Model (LLM) based agents. The repository covers various topics including survey, planning, feedback & reflection, memory mechanism, role playing, game playing, tool usage & human-agent interaction, benchmark & evaluation, environment & platform, agent framework, multi-agent system, and agent fine-tuning. It provides a comprehensive collection of research papers on LLM-based agents, exploring different aspects of AI agent architectures and applications.
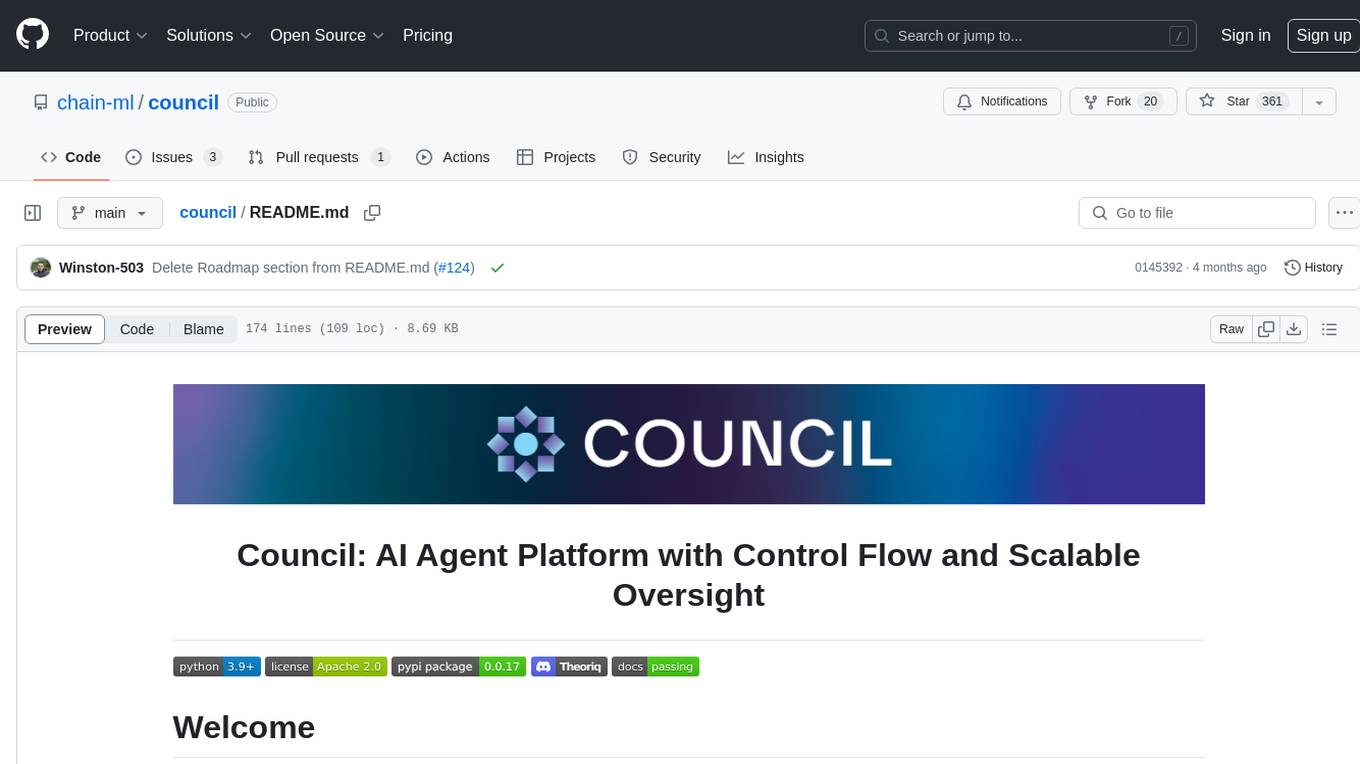
council
Council is an open-source platform designed for the rapid development and deployment of customized generative AI applications using teams of agents. It extends the LLM tool ecosystem by providing advanced control flow and scalable oversight for AI agents. Users can create sophisticated agents with predictable behavior by leveraging Council's powerful approach to control flow using Controllers, Filters, Evaluators, and Budgets. The framework allows for automated routing between agents, comparing, evaluating, and selecting the best results for a task. Council aims to facilitate packaging and deploying agents at scale on multiple platforms while enabling enterprise-grade monitoring and quality control.
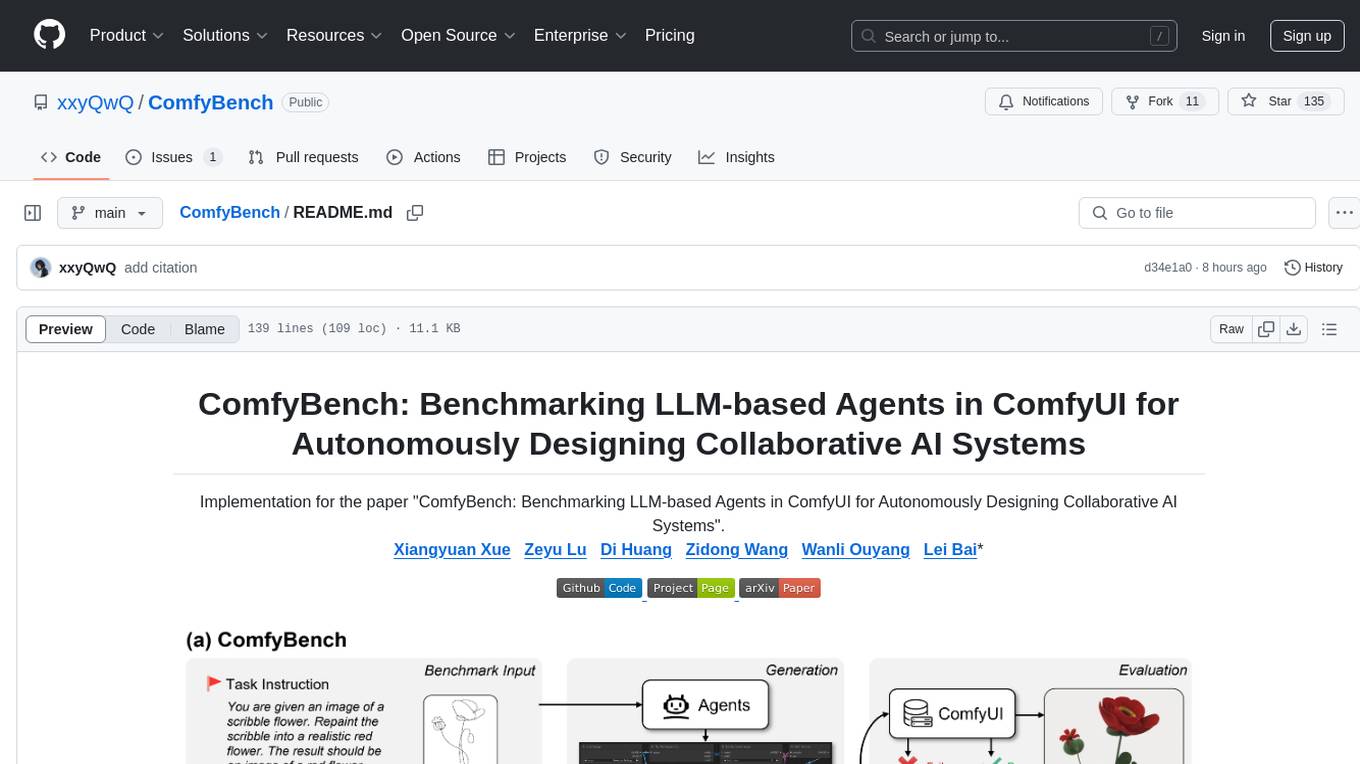
ComfyBench
ComfyBench is a comprehensive benchmark tool designed to evaluate agents' ability to design collaborative AI systems in ComfyUI. It provides tasks for agents to learn from documents and create workflows, which are then converted into code for better understanding by LLMs. The tool measures performance based on pass rate and resolve rate, reflecting the correctness of workflow execution and task realization. ComfyAgent, a component of ComfyBench, autonomously designs new workflows by learning from existing ones, interpreting them as collaborative AI systems to complete given tasks.
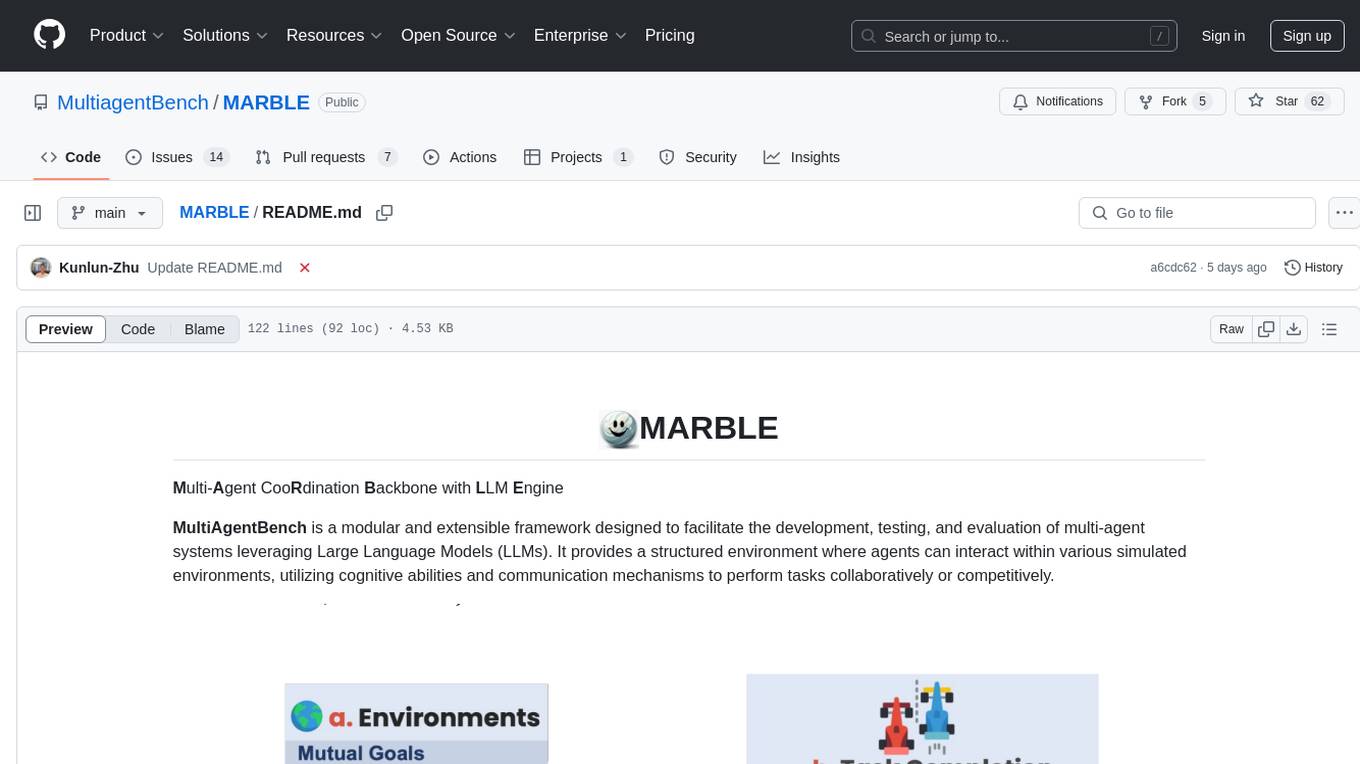
MARBLE
MARBLE (Multi-Agent Coordination Backbone with LLM Engine) is a modular framework for developing, testing, and evaluating multi-agent systems leveraging Large Language Models. It provides a structured environment for agents to interact in simulated environments, utilizing cognitive abilities and communication mechanisms for collaborative or competitive tasks. The framework features modular design, multi-agent support, LLM integration, shared memory, flexible environments, metrics and evaluation, industrial coding standards, and Docker support.
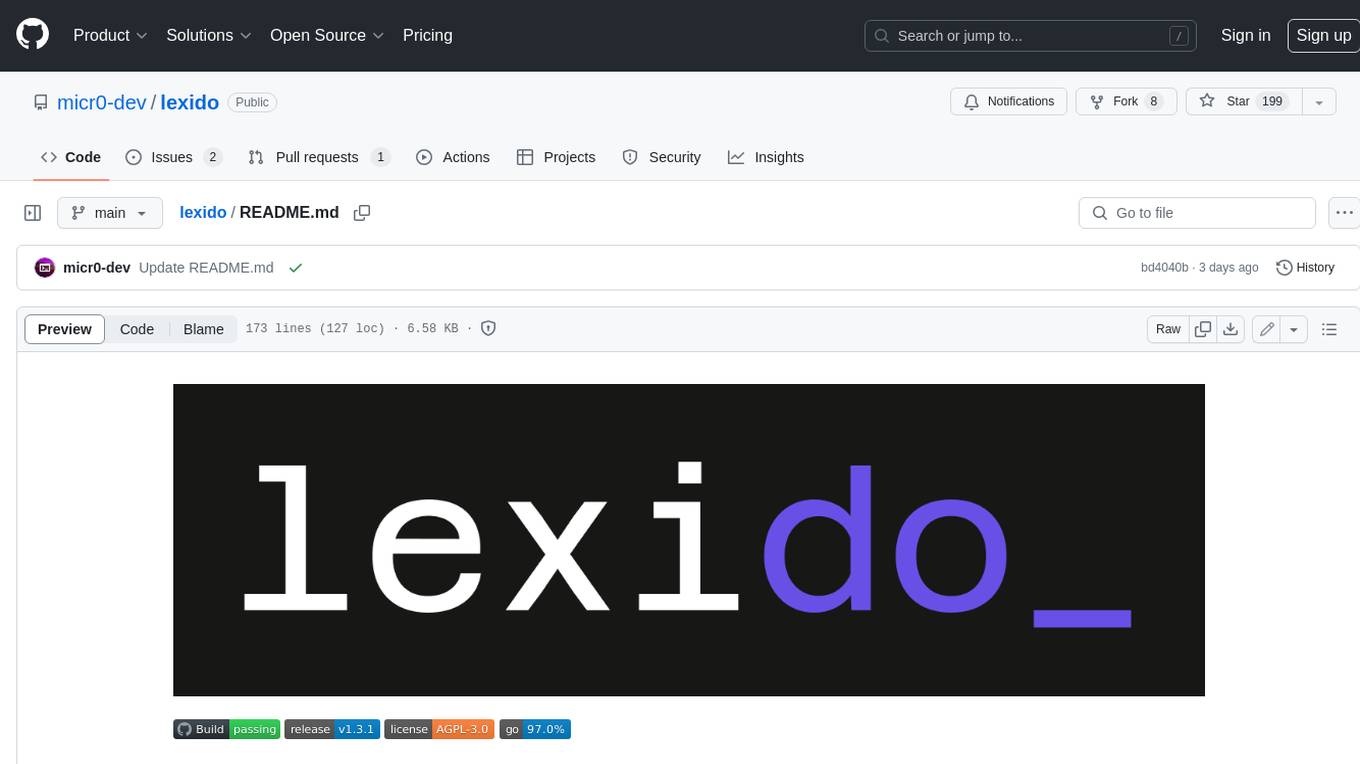
lexido
Lexido is an innovative assistant for the Linux command line, designed to boost your productivity and efficiency. Powered by Gemini Pro 1.0 and utilizing the free API, Lexido offers smart suggestions for commands based on your prompts and importantly your current environment. Whether you're installing software, managing files, or configuring system settings, Lexido streamlines the process, making it faster and more intuitive.
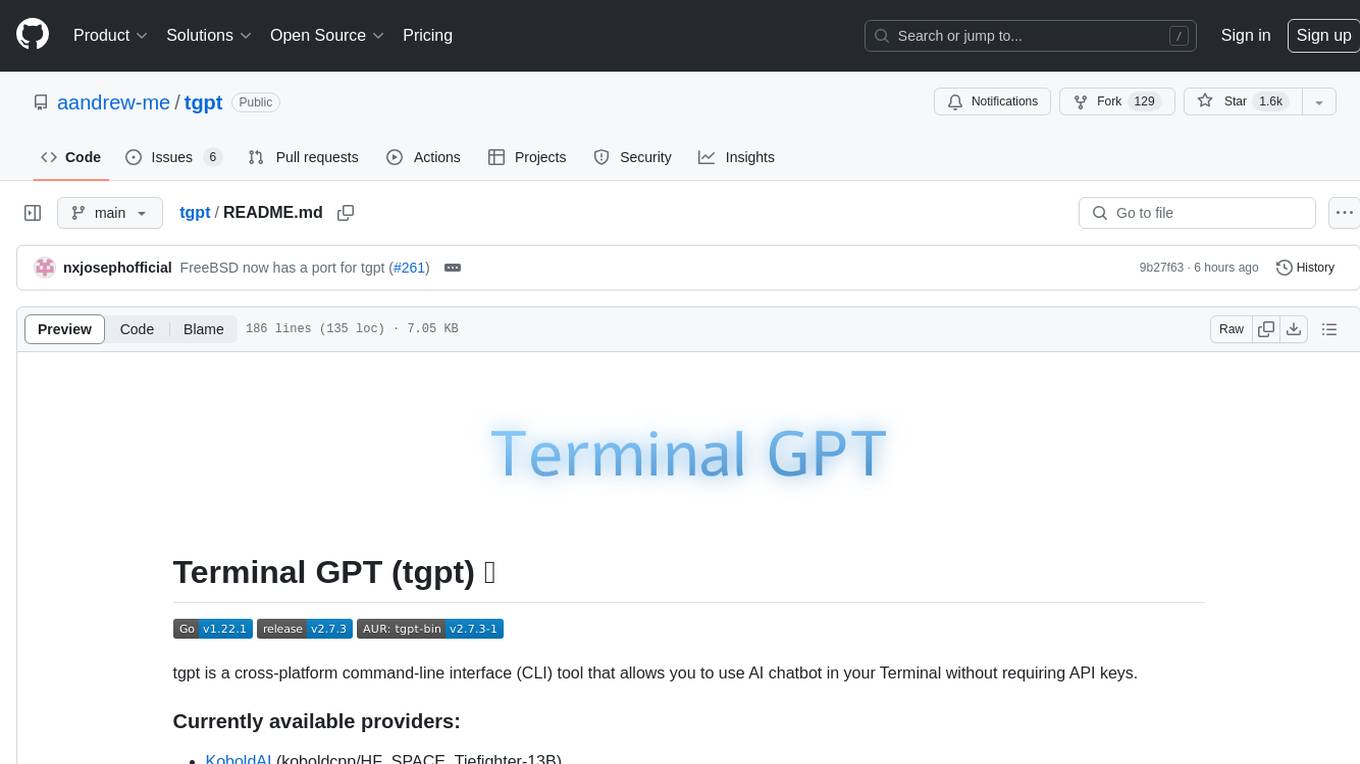
tgpt
tgpt is a cross-platform command-line interface (CLI) tool that allows users to interact with AI chatbots in the Terminal without needing API keys. It supports various AI providers such as KoboldAI, Phind, Llama2, Blackbox AI, and OpenAI. Users can generate text, code, and images using different flags and options. The tool can be installed on GNU/Linux, MacOS, FreeBSD, and Windows systems. It also supports proxy configurations and provides options for updating and uninstalling the tool.
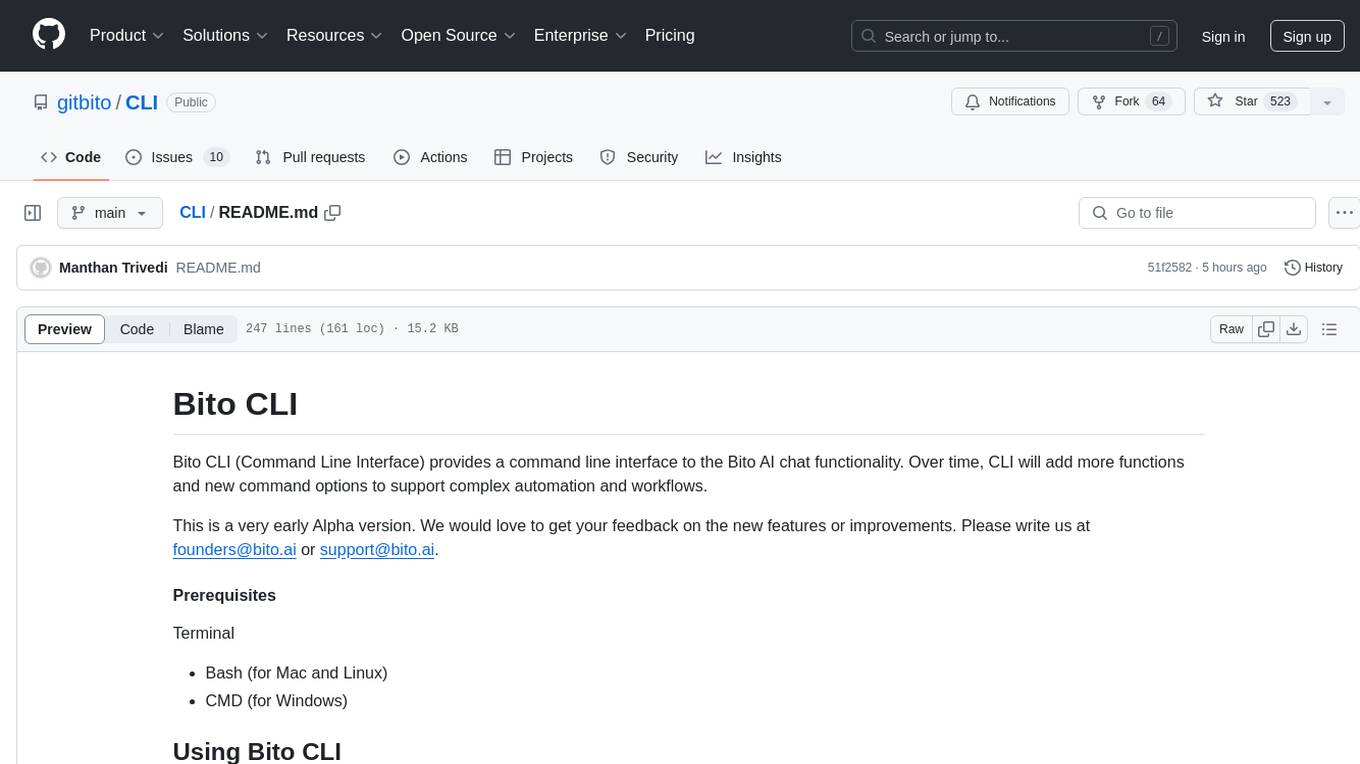
CLI
Bito CLI provides a command line interface to the Bito AI chat functionality, allowing users to interact with the AI through commands. It supports complex automation and workflows, with features like long prompts and slash commands. Users can install Bito CLI on Mac, Linux, and Windows systems using various methods. The tool also offers configuration options for AI model type, access key management, and output language customization. Bito CLI is designed to enhance user experience in querying AI models and automating tasks through the command line interface.
For similar jobs
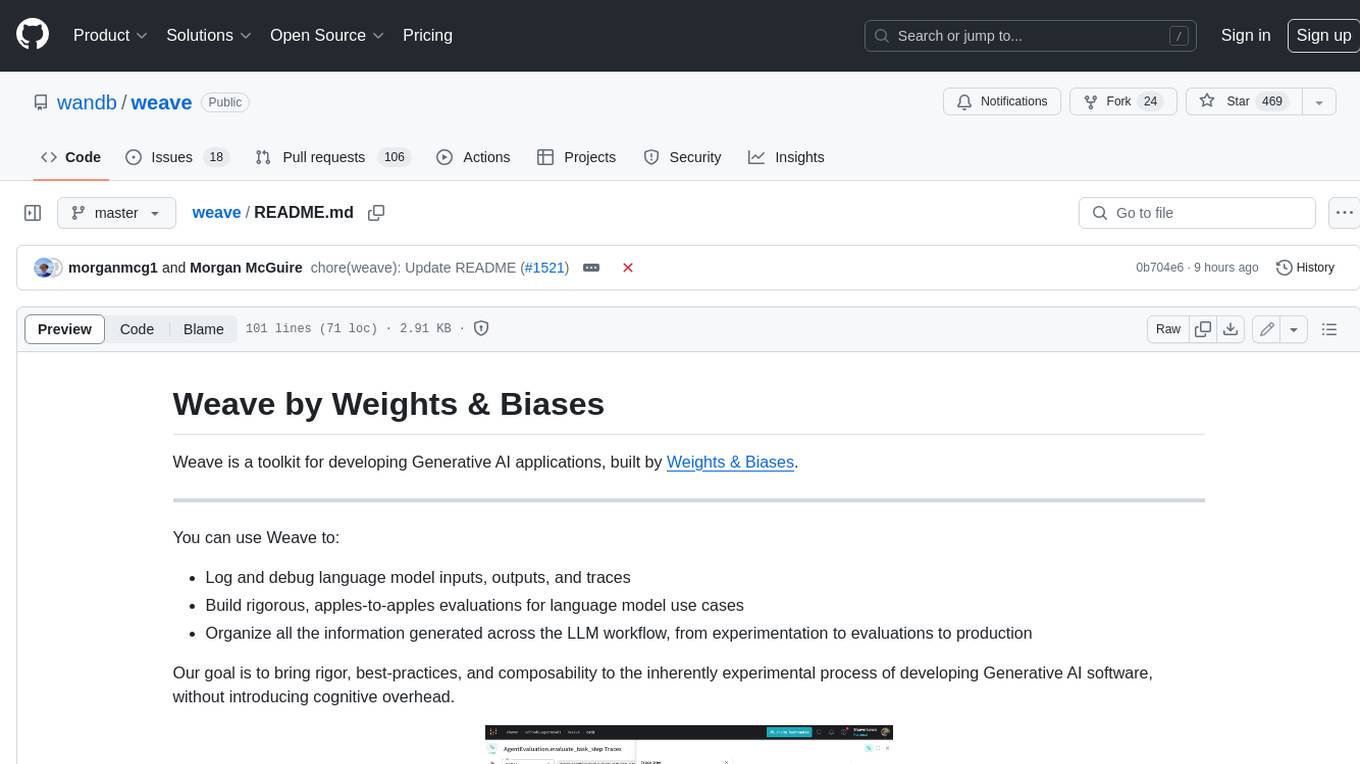
weave
Weave is a toolkit for developing Generative AI applications, built by Weights & Biases. With Weave, you can log and debug language model inputs, outputs, and traces; build rigorous, apples-to-apples evaluations for language model use cases; and organize all the information generated across the LLM workflow, from experimentation to evaluations to production. Weave aims to bring rigor, best-practices, and composability to the inherently experimental process of developing Generative AI software, without introducing cognitive overhead.
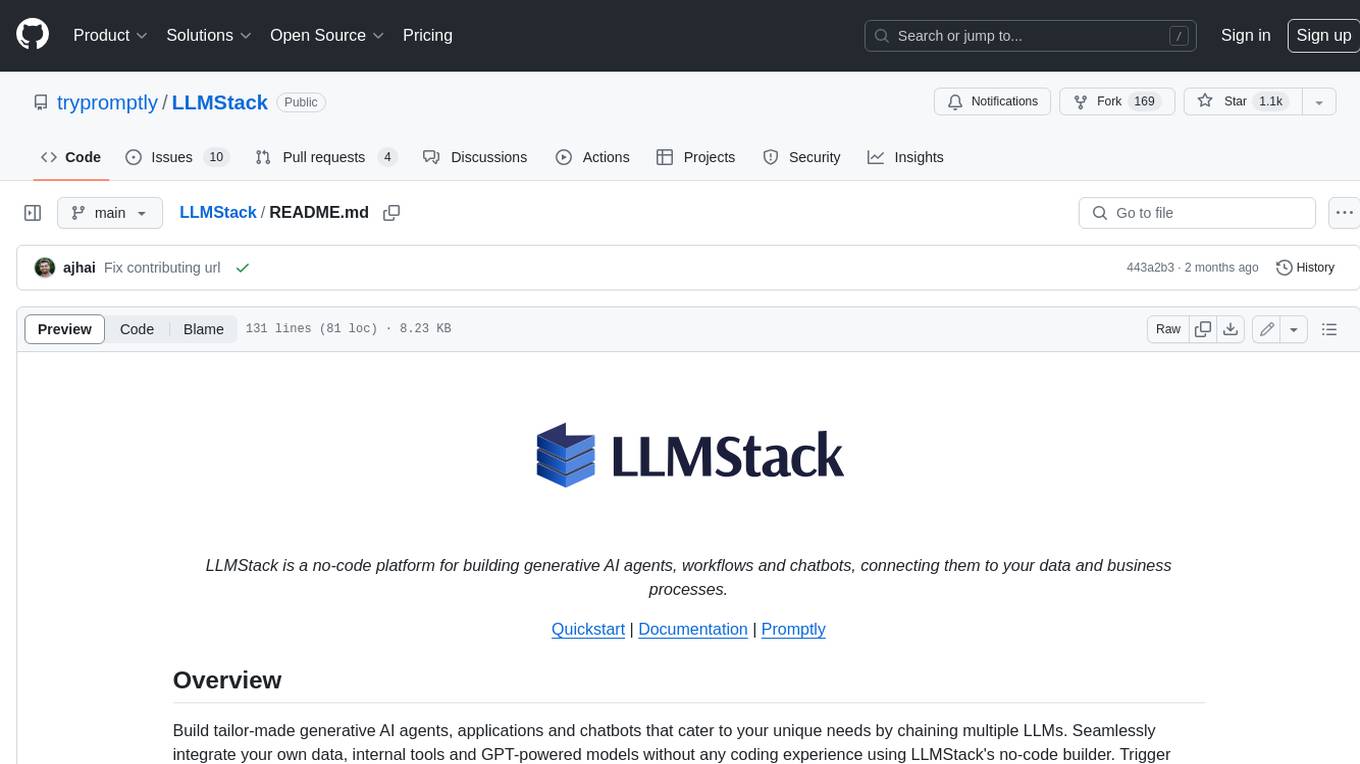
LLMStack
LLMStack is a no-code platform for building generative AI agents, workflows, and chatbots. It allows users to connect their own data, internal tools, and GPT-powered models without any coding experience. LLMStack can be deployed to the cloud or on-premise and can be accessed via HTTP API or triggered from Slack or Discord.
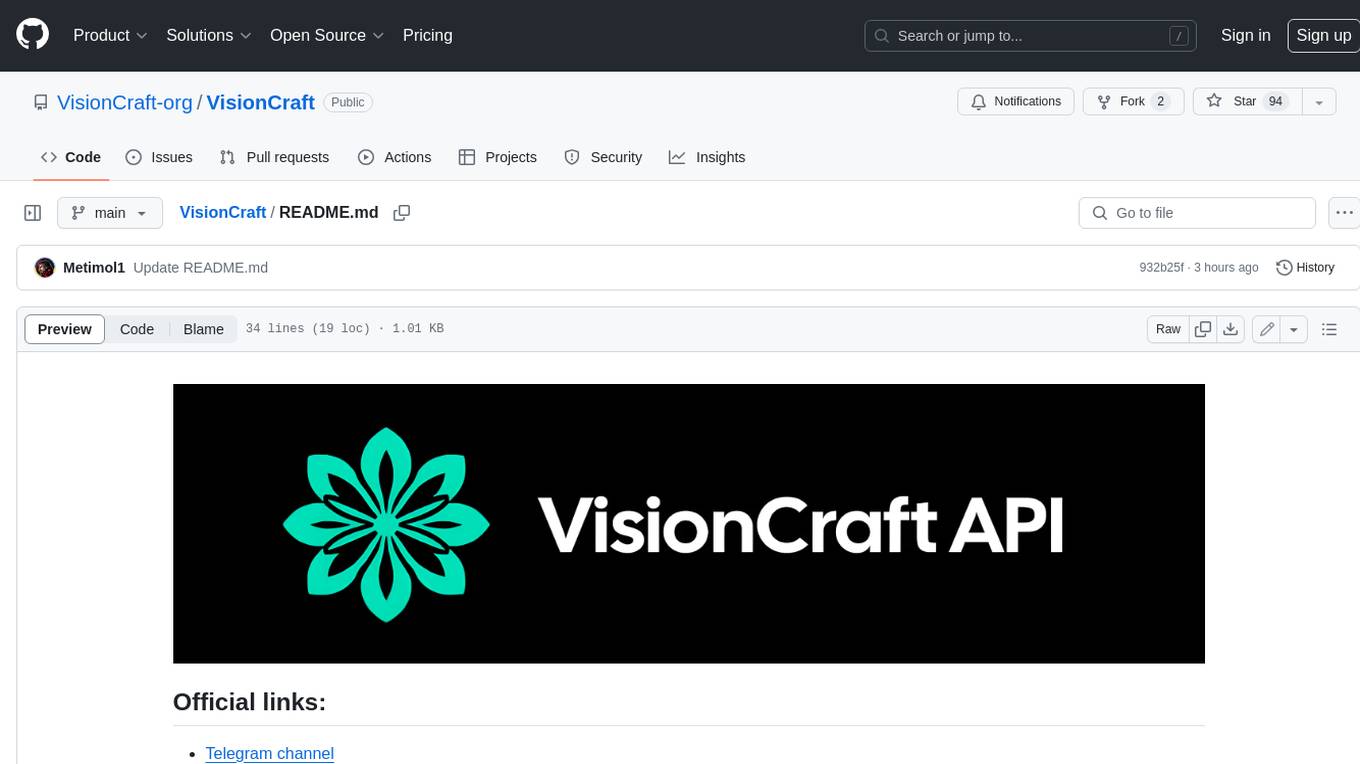
VisionCraft
The VisionCraft API is a free API for using over 100 different AI models. From images to sound.
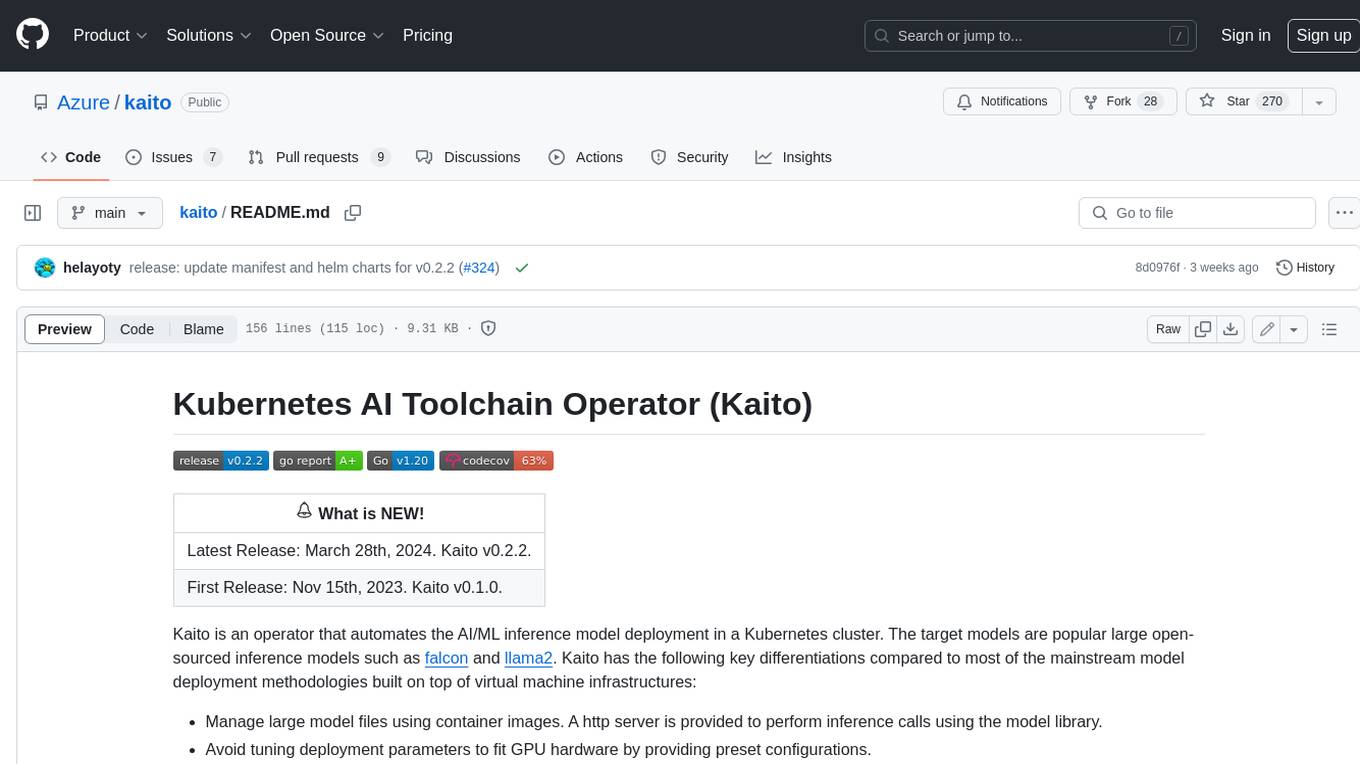
kaito
Kaito is an operator that automates the AI/ML inference model deployment in a Kubernetes cluster. It manages large model files using container images, avoids tuning deployment parameters to fit GPU hardware by providing preset configurations, auto-provisions GPU nodes based on model requirements, and hosts large model images in the public Microsoft Container Registry (MCR) if the license allows. Using Kaito, the workflow of onboarding large AI inference models in Kubernetes is largely simplified.
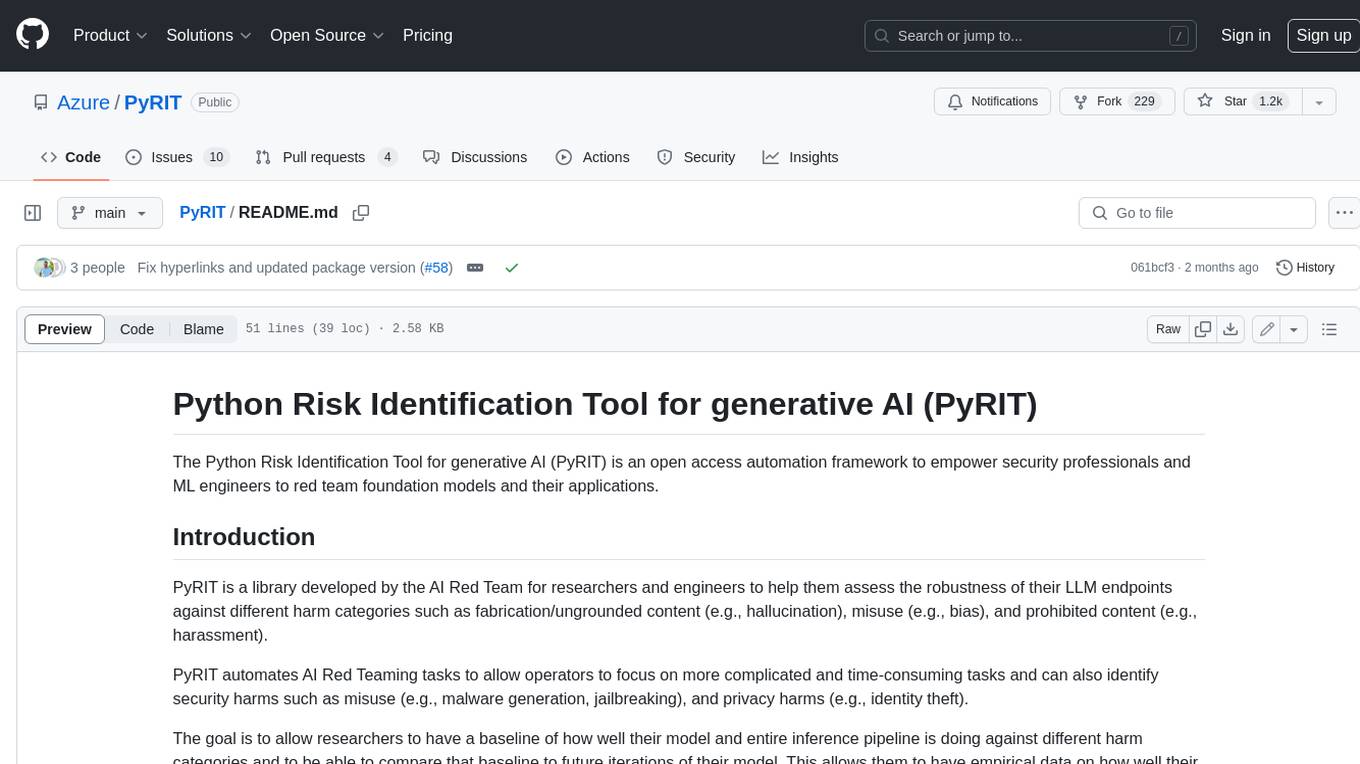
PyRIT
PyRIT is an open access automation framework designed to empower security professionals and ML engineers to red team foundation models and their applications. It automates AI Red Teaming tasks to allow operators to focus on more complicated and time-consuming tasks and can also identify security harms such as misuse (e.g., malware generation, jailbreaking), and privacy harms (e.g., identity theft). The goal is to allow researchers to have a baseline of how well their model and entire inference pipeline is doing against different harm categories and to be able to compare that baseline to future iterations of their model. This allows them to have empirical data on how well their model is doing today, and detect any degradation of performance based on future improvements.
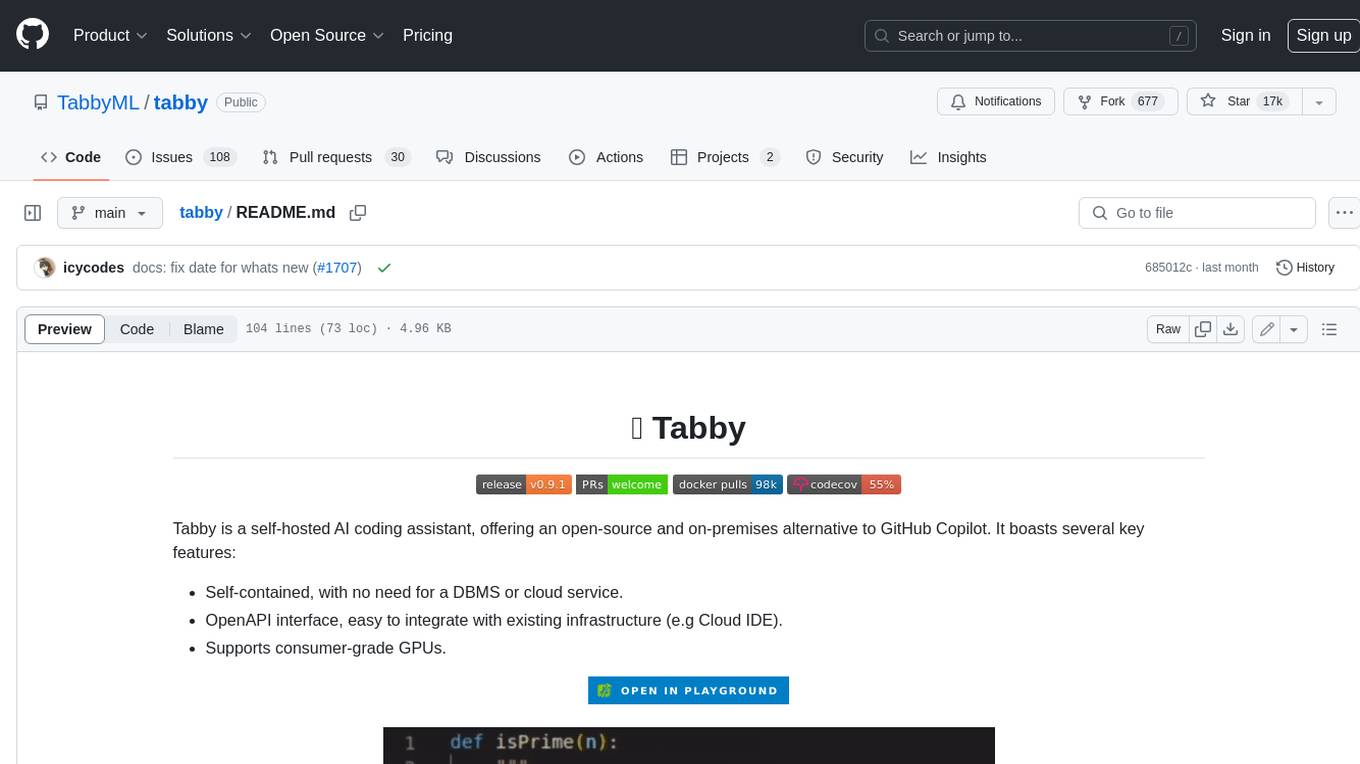
tabby
Tabby is a self-hosted AI coding assistant, offering an open-source and on-premises alternative to GitHub Copilot. It boasts several key features: * Self-contained, with no need for a DBMS or cloud service. * OpenAPI interface, easy to integrate with existing infrastructure (e.g Cloud IDE). * Supports consumer-grade GPUs.
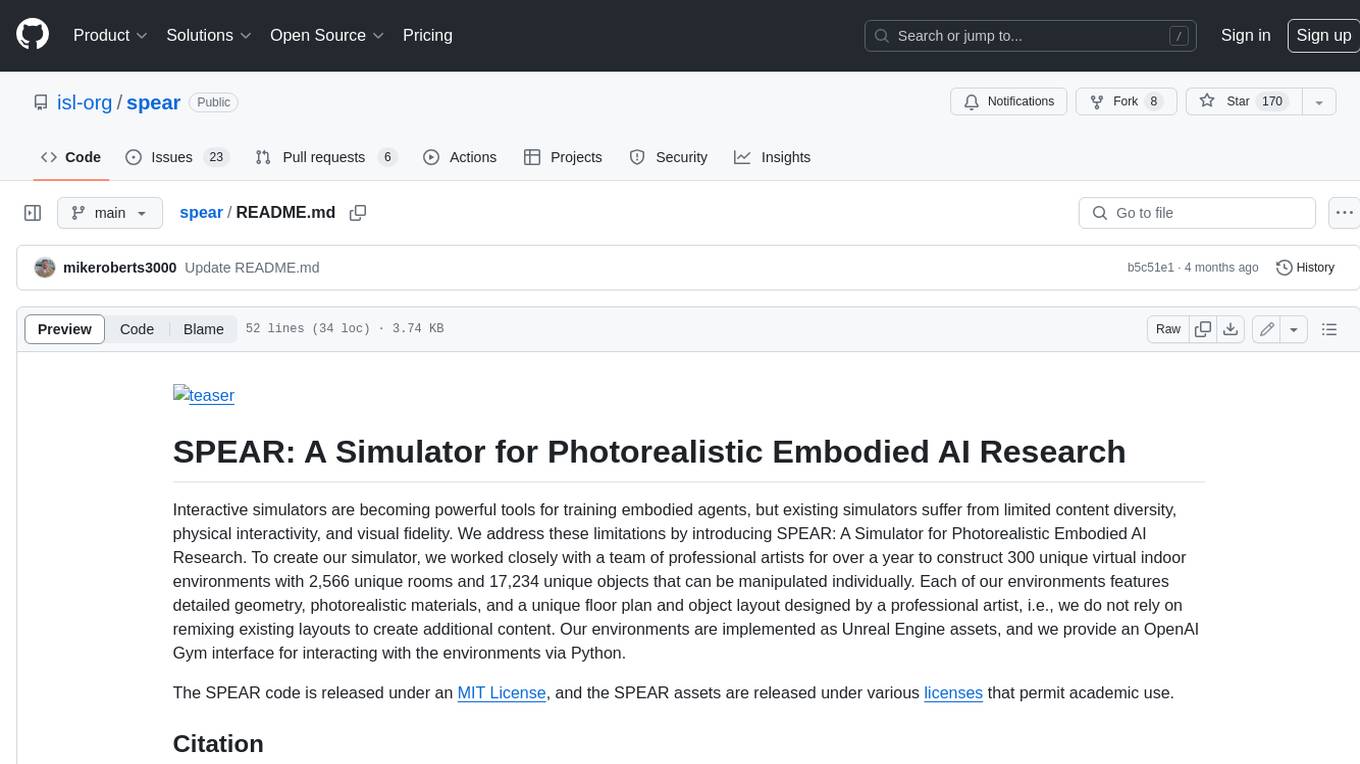
spear
SPEAR (Simulator for Photorealistic Embodied AI Research) is a powerful tool for training embodied agents. It features 300 unique virtual indoor environments with 2,566 unique rooms and 17,234 unique objects that can be manipulated individually. Each environment is designed by a professional artist and features detailed geometry, photorealistic materials, and a unique floor plan and object layout. SPEAR is implemented as Unreal Engine assets and provides an OpenAI Gym interface for interacting with the environments via Python.
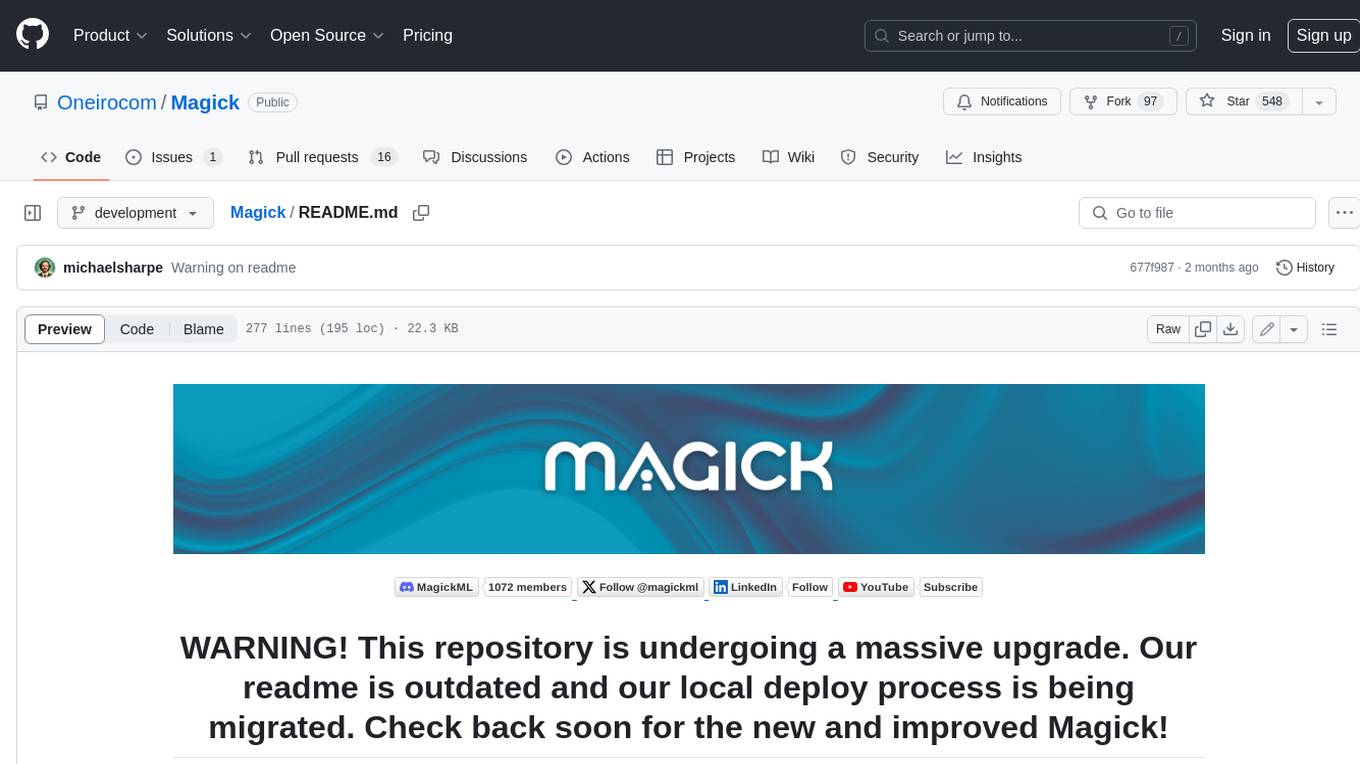
Magick
Magick is a groundbreaking visual AIDE (Artificial Intelligence Development Environment) for no-code data pipelines and multimodal agents. Magick can connect to other services and comes with nodes and templates well-suited for intelligent agents, chatbots, complex reasoning systems and realistic characters.