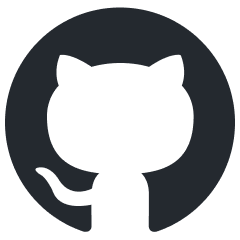
promptwright
Generate large synthetic data using an LLM
Stars: 367
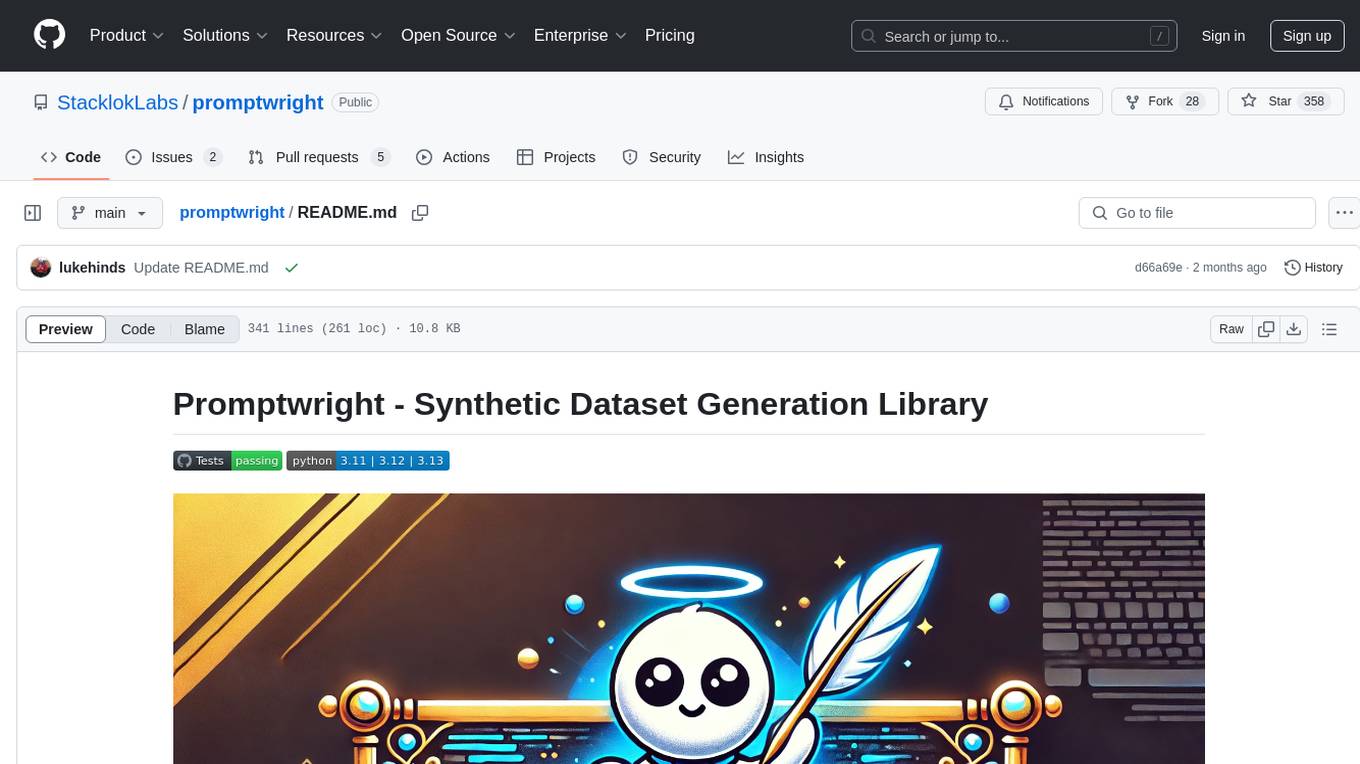
Promptwright is a Python library designed for generating large synthetic datasets using a local LLM and various LLM service providers. It offers flexible interfaces for generating prompt-led synthetic datasets. The library supports multiple providers, configurable instructions and prompts, YAML configuration for tasks, command line interface for running tasks, push to Hugging Face Hub for dataset upload, and system message control. Users can define generation tasks using YAML configuration or Python code. Promptwright integrates with LiteLLM to interface with LLM providers and supports automatic dataset upload to Hugging Face Hub.
README:
Promptwright is a Python library from Stacklok designed for generating large synthetic datasets using a local LLM and most LLM service providers (openAI, Anthropic, OpenRouter etc). The library offers a flexible and easy-to-use set of interfaces, enabling users the ability to generate prompt led synthetic datasets.
Promptwright was inspired by the redotvideo/pluto, in fact it started as fork, but ended up largley being a re-write.
The library interfaces with LiteLLM, making it easy to just pull a model and run locally with say something like Ollama, or call directly to an online LLM provider.
- Multiple Providers Support: Works with most LLM service providers and LocalLLM's via Ollam, VLLM etc
- Configurable Instructions and Prompts: Define custom instructions and system prompts
- YAML Configuration: Define your generation tasks using YAML configuration files
- Command Line Interface: Run generation tasks directly from the command line
- Push to Hugging Face: Push the generated dataset to Hugging Face Hub with automatic dataset cards and tags
- System Message Control: Choose whether to include system messages in the generated dataset
- Python 3.11+
- Poetry (for dependency management)
- Ollama CLI installed and running (see Ollama Installation
- A Model pulled via Ollama (see Model Compatibility)
- (Optional) Hugging Face account and API token for dataset upload
You can install Promptwright using pip:
pip install promptwright
To install the prerequisites, you can use the following commands:
# Install Poetry if you haven't already
curl -sSL https://install.python-poetry.org | python3 -
# Install promptwright and its dependencies
git clone https://github.com/StacklokLabs/promptwright.git
cd promptwright
poetry install
Promptwright offers two ways to define and run your generation tasks:
Create a YAML file defining your generation task:
system_prompt: "You are a helpful assistant. You provide clear and concise answers to user questions."
topic_tree:
args:
root_prompt: "Capital Cities of the World."
model_system_prompt: "<system_prompt_placeholder>"
tree_degree: 3
tree_depth: 2
temperature: 0.7
model_name: "ollama/mistral:latest"
save_as: "basic_prompt_topictree.jsonl"
data_engine:
args:
instructions: "Please provide training examples with questions about capital cities."
system_prompt: "<system_prompt_placeholder>"
model_name: "ollama/mistral:latest"
temperature: 0.9
max_retries: 2
dataset:
creation:
num_steps: 5
batch_size: 1
model_name: "ollama/mistral:latest"
sys_msg: true # Include system message in dataset (default: true)
save_as: "basic_prompt_dataset.jsonl"
# Optional Hugging Face Hub configuration
huggingface:
# Repository in format "username/dataset-name"
repository: "your-username/your-dataset-name"
# Token can also be provided via HF_TOKEN environment variable or --hf-token CLI option
token: "your-hf-token"
# Additional tags for the dataset (optional)
# "promptwright" and "synthetic" tags are added automatically
tags:
- "promptwright-generated-dataset"
- "geography"
Run using the CLI:
promptwright start config.yaml
The CLI supports various options to override configuration values:
promptwright start config.yaml \
--topic-tree-save-as output_tree.jsonl \
--dataset-save-as output_dataset.jsonl \
--model-name ollama/llama3 \
--temperature 0.8 \
--tree-degree 4 \
--tree-depth 3 \
--num-steps 10 \
--batch-size 2 \
--sys-msg true \ # Control system message inclusion (default: true)
--hf-repo username/dataset-name \
--hf-token your-token \
--hf-tags tag1 --hf-tags tag2
Promptwright uses LiteLLM to interface with LLM providers. You can specify the provider in the provider, model section in your config or code:
provider: "openai" # LLM provider
model: "gpt-4-1106-preview" # Model name
Choose any of the listed providers here and following the same naming convention.
e.g.
The LiteLLM convention for Google Gemini would is:
from litellm import completion
import os
os.environ['GEMINI_API_KEY'] = ""
response = completion(
model="gemini/gemini-pro",
messages=[{"role": "user", "content": "write code for saying hi from LiteLLM"}]
)
In Promptwright, you would specify the provider as gemini
and the model as gemini-pro
.
provider: "gemini" # LLM provider
model: "gemini-pro" # Model name
For Ollama, you would specify the provider as ollama
and the model as mistral
and so on.
provider: "ollama" # LLM provider
model: "mistral:latest" # Model name
You can set the API key for the provider in the environment variable. The key
should be set as PROVIDER_API_KEY
. For example, for OpenAI, you would set the
API key as OPENAI_API_KEY
.
export OPENAI_API_KEY
Again, refer to the LiteLLM documentation for more information on setting up the API keys.
Promptwright supports automatic dataset upload to the Hugging Face Hub with the following features:
- Dataset Upload: Upload your generated dataset directly to Hugging Face Hub
- Dataset Cards: Automatically creates and updates dataset cards
- Automatic Tags: Adds "promptwright" and "synthetic" tags automatically
- Custom Tags: Support for additional custom tags
-
Flexible Authentication: HF token can be provided via:
- CLI option:
--hf-token your-token
- Environment variable:
export HF_TOKEN=your-token
- YAML configuration:
huggingface.token
- CLI option:
Example using environment variable:
export HF_TOKEN=your-token
promptwright start config.yaml --hf-repo username/dataset-name
Or pass it in as a CLI option:
promptwright start config.yaml --hf-repo username/dataset-name --hf-token your-token
You can also create generation tasks programmatically using Python code. There
are several examples in the examples
directory that demonstrate this approach.
Example Python usage:
from promptwright import DataEngine, EngineArguments, TopicTree, TopicTreeArguments
tree = TopicTree(
args=TopicTreeArguments(
root_prompt="Creative Writing Prompts",
model_system_prompt=system_prompt,
tree_degree=5,
tree_depth=4,
temperature=0.9,
model_name="ollama/llama3"
)
)
engine = DataEngine(
args=EngineArguments(
instructions="Generate creative writing prompts and example responses.",
system_prompt="You are a creative writing instructor providing writing prompts and example responses.",
model_name="ollama/llama3",
temperature=0.9,
max_retries=2,
sys_msg=True, # Include system message in dataset (default: true)
)
)
The project uses Poetry for dependency management. Here are some common development commands:
# Install dependencies including development dependencies
make install
# Format code
make format
# Run linting
make lint
# Run tests
make test
# Run security checks
make security
# Build the package
make build
# Run all checks and build
make all
With sys_msg=true (default):
{
"messages": [
{
"role": "system",
"content": "You are tasked with designing an immersive virtual reality experience that transports users to a fantastical world of wonder."
},
{
"role": "user",
"content": "Create a descriptive passage about a character discovering their hidden talents."
},
{
"role": "assistant",
"content": "As she stared at the canvas, Emma's fingers hovered above the paintbrushes, as if hesitant to unleash the colors that had been locked within her. The strokes began with bold abandon, swirling blues and greens merging into a mesmerizing dance of light and shadow. With each passing moment, she felt herself becoming the art – her very essence seeping onto the canvas like watercolors in a spring storm. The world around her melted away, leaving only the vibrant symphony of color and creation."
}
]
}
With sys_msg=false:
{
"messages": [
{
"role": "user",
"content": "Create a descriptive passage about a character discovering their hidden talents."
},
{
"role": "assistant",
"content": "As she stared at the canvas, Emma's fingers hovered above the paintbrushes, as if hesitant to unleash the colors that had been locked within her. The strokes began with bold abandon, swirling blues and greens merging into a mesmerizing dance of light and shadow. With each passing moment, she felt herself becoming the art – her very essence seeping onto the canvas like watercolors in a spring storm. The world around her melted away, leaving only the vibrant symphony of color and creation."
}
]
}
The library is designed to generate synthetic data based on the prompts and instructions provided. The quality of the generated data is dependent on the quality of the prompts and the model used. The library does not guarantee the quality of the generated data.
Large Language Models can sometimes generate unpredictable or inappropriate content and the authors of this library are not responsible for the content generated by the models. We recommend reviewing the generated data before using it in any production environment.
Large Language Models also have the potential to fail to stick with the behavior defined by the prompt around JSON formatting, and may generate invalid JSON. This is a known issue with the underlying model and not the library. We handle these errors by retrying the generation process and filtering out invalid JSON. The failure rate is low, but it can happen. We report on each failure within a final summary.
If something here could be improved, please open an issue or submit a pull request.
This project is licensed under the Apache 2 License. See the LICENSE
file for more details.
For Tasks:
Click tags to check more tools for each tasksFor Jobs:
Alternative AI tools for promptwright
Similar Open Source Tools
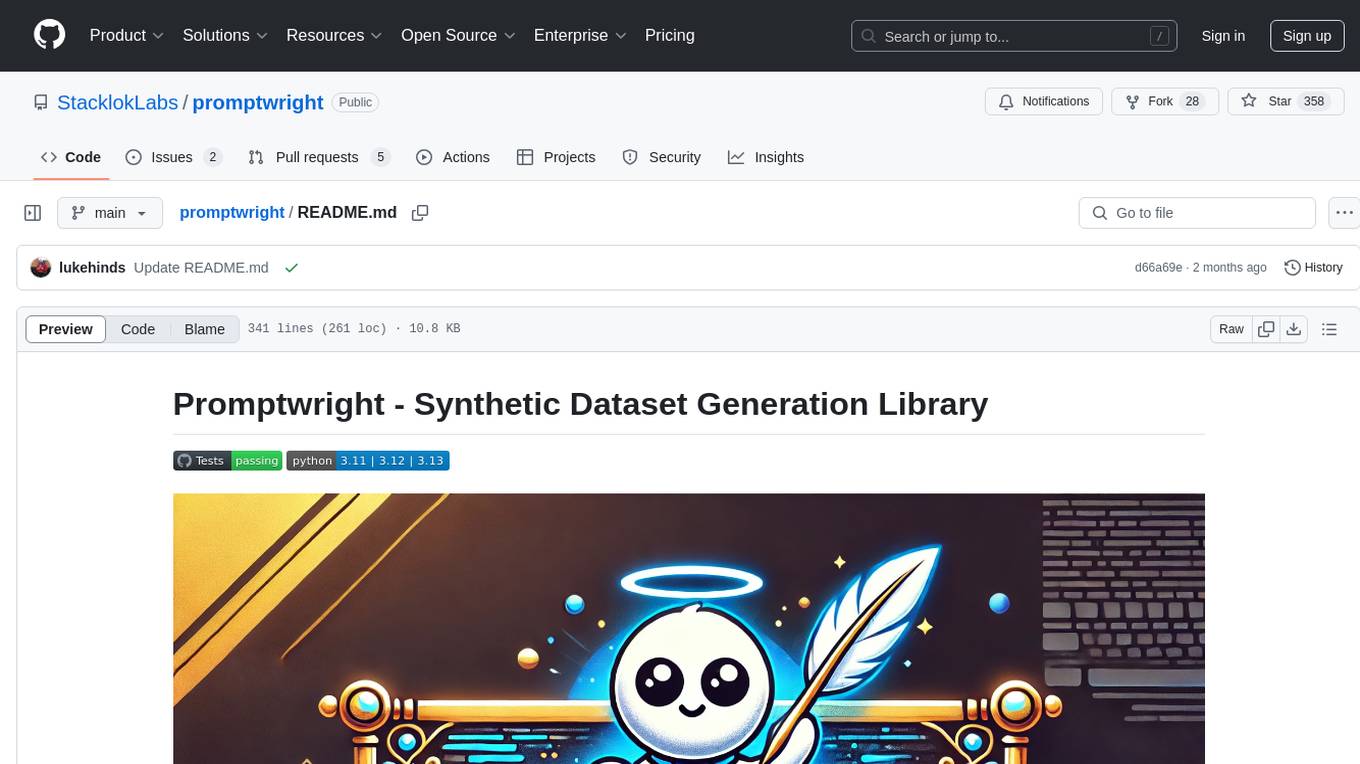
promptwright
Promptwright is a Python library designed for generating large synthetic datasets using a local LLM and various LLM service providers. It offers flexible interfaces for generating prompt-led synthetic datasets. The library supports multiple providers, configurable instructions and prompts, YAML configuration for tasks, command line interface for running tasks, push to Hugging Face Hub for dataset upload, and system message control. Users can define generation tasks using YAML configuration or Python code. Promptwright integrates with LiteLLM to interface with LLM providers and supports automatic dataset upload to Hugging Face Hub.
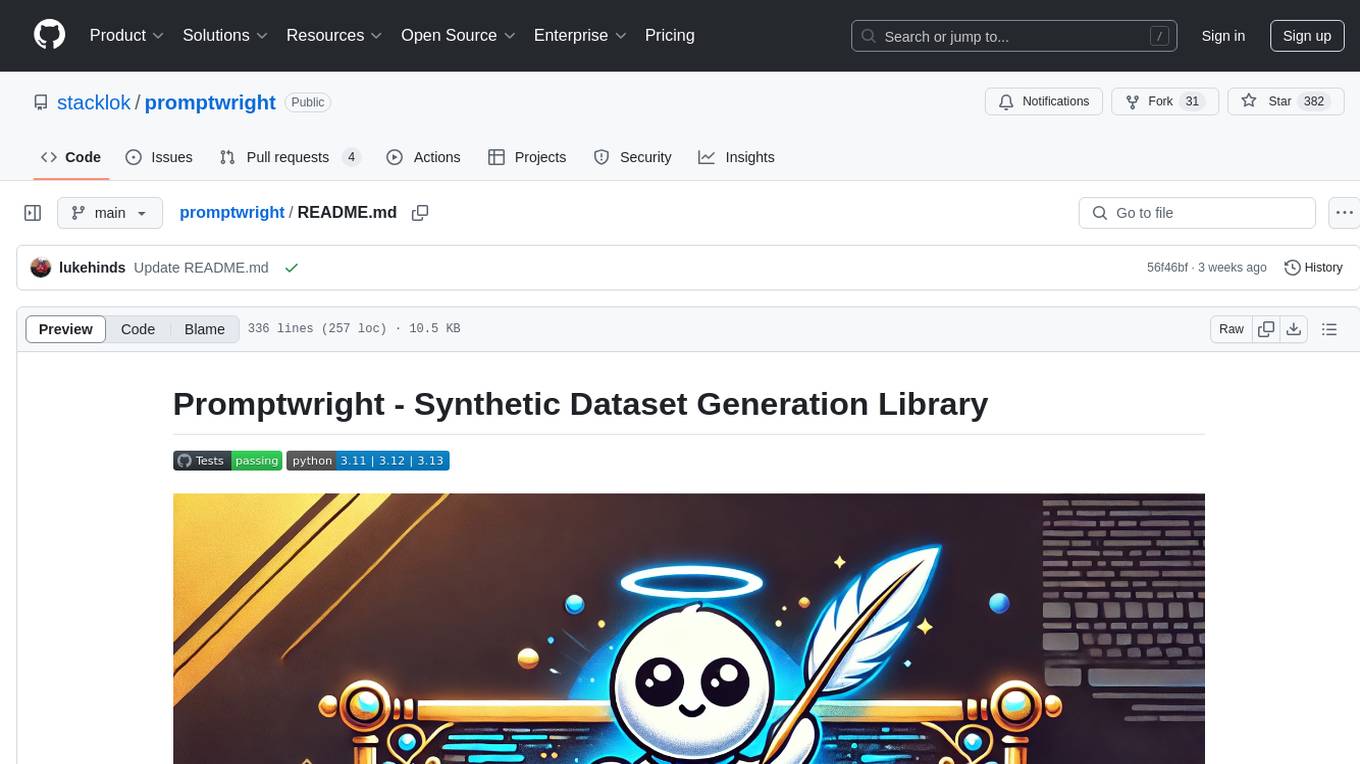
promptwright
Promptwright is a Python library designed for generating large synthetic datasets using local LLM and various LLM service providers. It offers flexible interfaces for generating prompt-led synthetic datasets. The library supports multiple providers, configurable instructions and prompts, YAML configuration, command line interface, push to Hugging Face Hub, and system message control. Users can define generation tasks using YAML configuration files or programmatically using Python code. Promptwright integrates with LiteLLM for LLM providers and supports automatic dataset upload to Hugging Face Hub. The library is not responsible for the content generated by models and advises users to review the data before using it in production environments.
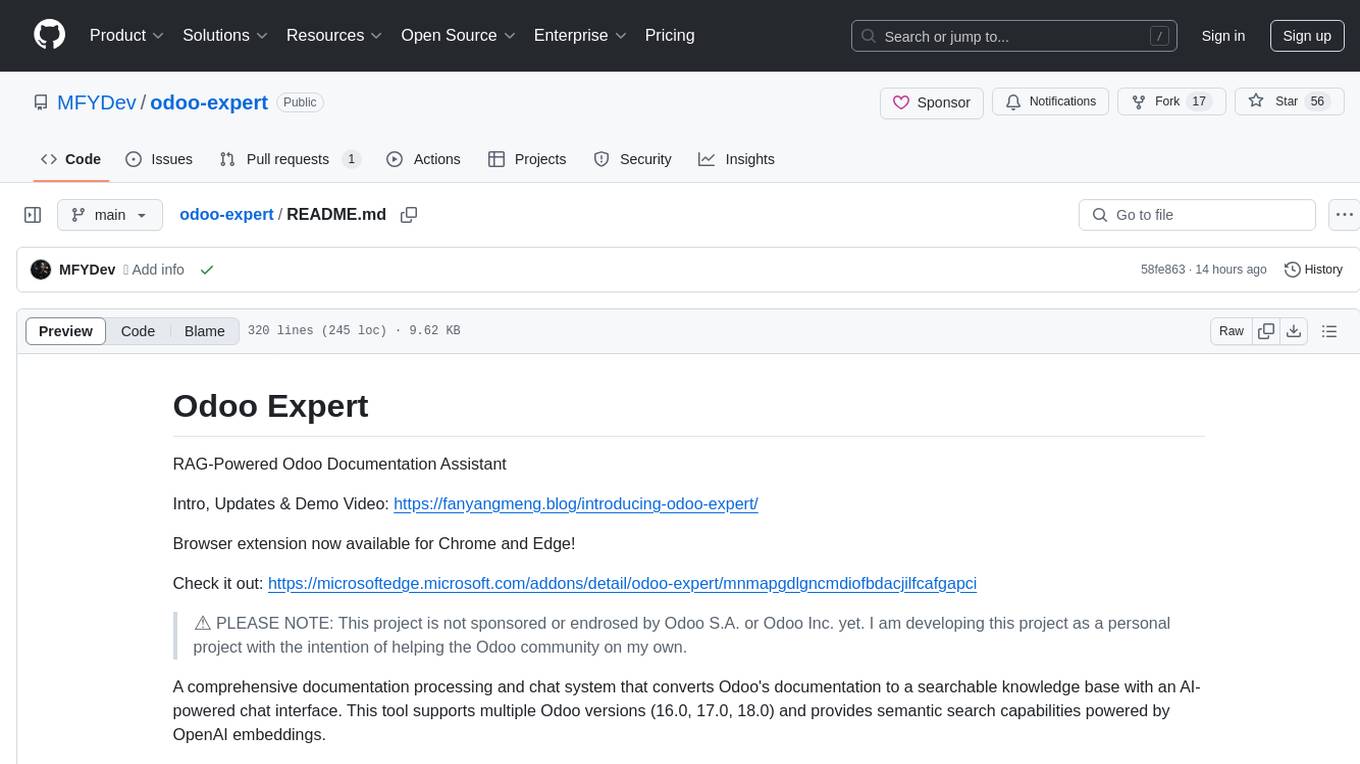
odoo-expert
RAG-Powered Odoo Documentation Assistant is a comprehensive documentation processing and chat system that converts Odoo's documentation to a searchable knowledge base with an AI-powered chat interface. It supports multiple Odoo versions (16.0, 17.0, 18.0) and provides semantic search capabilities powered by OpenAI embeddings. The tool automates the conversion of RST to Markdown, offers real-time semantic search, context-aware AI-powered chat responses, and multi-version support. It includes a Streamlit-based web UI, REST API for programmatic access, and a CLI for document processing and chat. The system operates through a pipeline of data processing steps and an interface layer for UI and API access to the knowledge base.
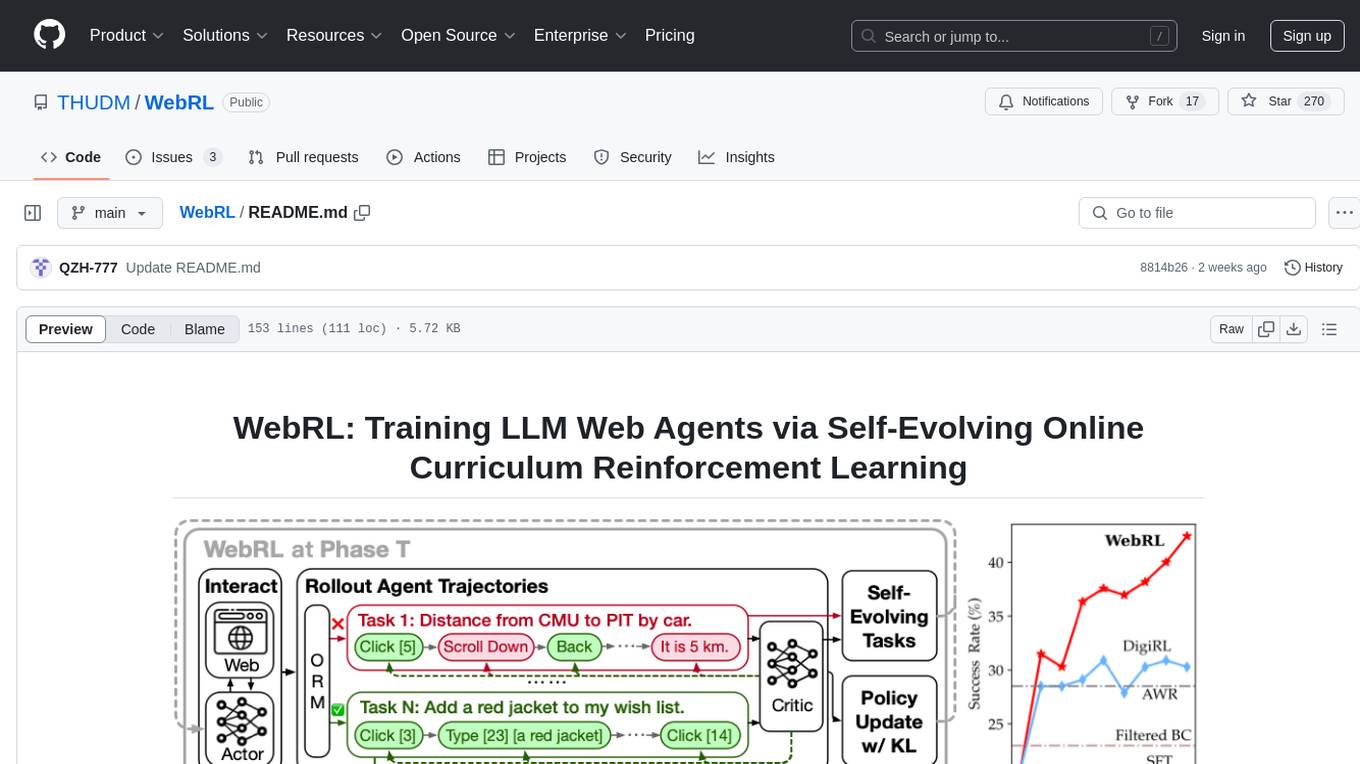
WebRL
WebRL is a self-evolving online curriculum learning framework designed for training web agents in the WebArena environment. It provides model checkpoints, training instructions, and evaluation processes for training the actor and critic models. The tool enables users to generate new instructions and interact with WebArena to configure tasks for training and evaluation.
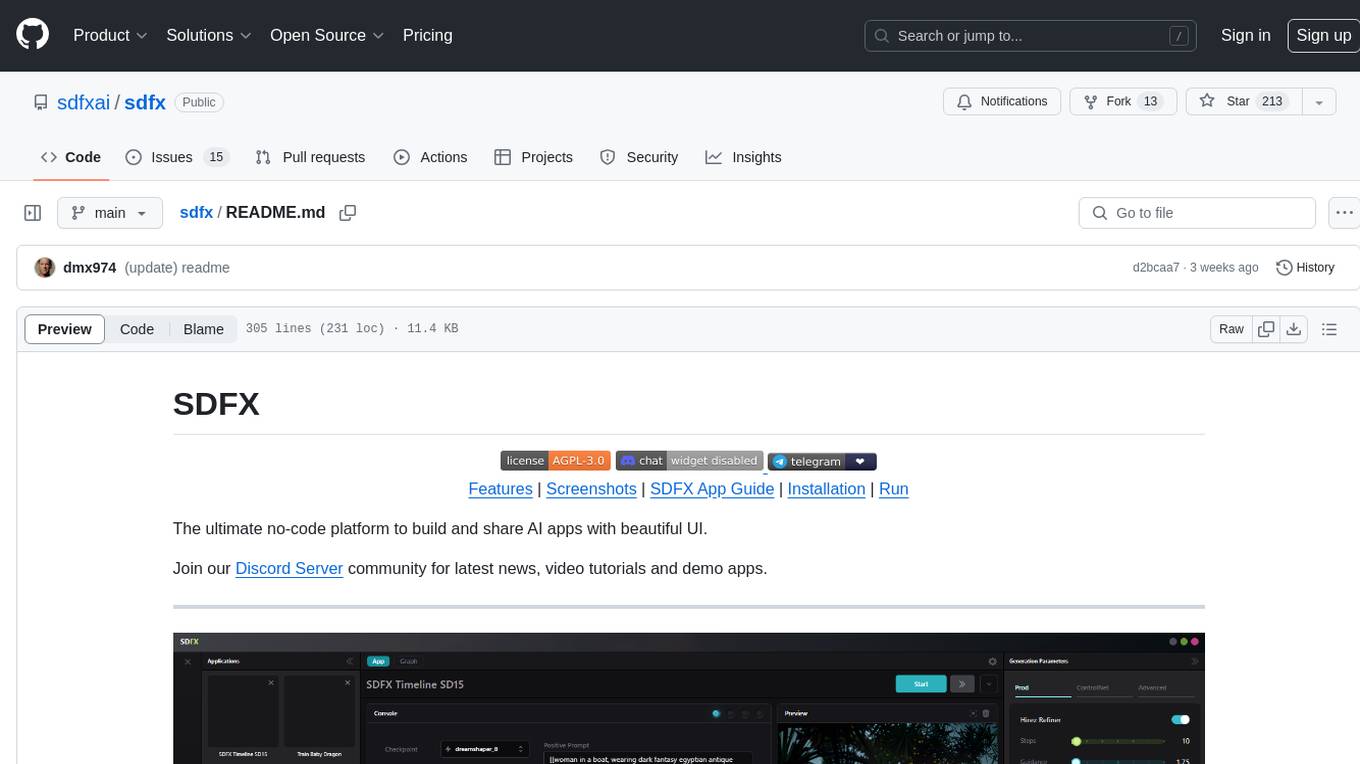
sdfx
SDFX is the ultimate no-code platform for building and sharing AI apps with beautiful UI. It enables the creation of user-friendly interfaces for complex workflows by combining Comfy workflow with a UI. The tool is designed to merge the benefits of form-based UI and graph-node based UI, allowing users to create intricate graphs with a high-level UI overlay. SDFX is fully compatible with ComfyUI, abstracting the need for installing ComfyUI. It offers features like animated graph navigation, node bookmarks, UI debugger, custom nodes manager, app and template export, image and mask editor, and more. The tool compiles as a native app or web app, making it easy to maintain and add new features.
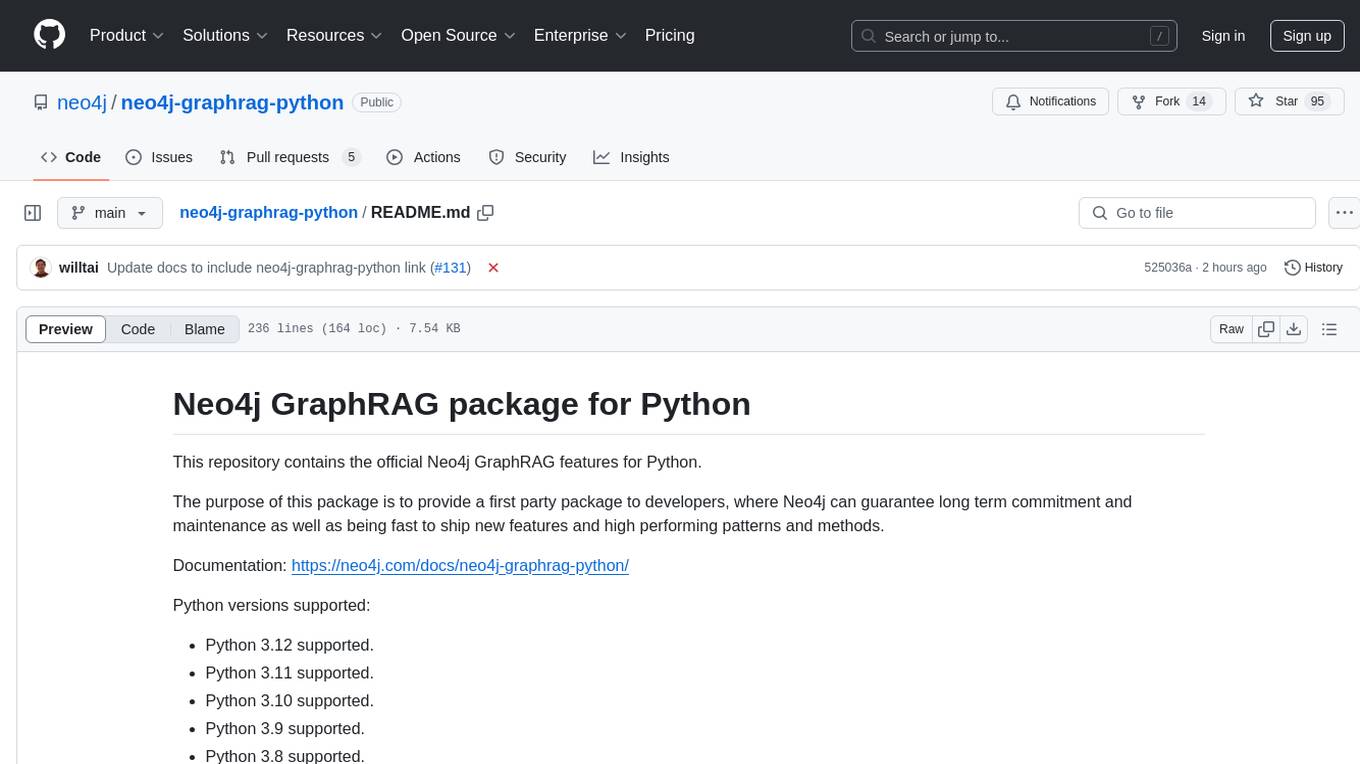
neo4j-graphrag-python
The Neo4j GraphRAG package for Python is an official repository that provides features for creating and managing vector indexes in Neo4j databases. It aims to offer developers a reliable package with long-term commitment, maintenance, and fast feature updates. The package supports various Python versions and includes functionalities for creating vector indexes, populating them, and performing similarity searches. It also provides guidelines for installation, examples, and development processes such as installing dependencies, making changes, and running tests.
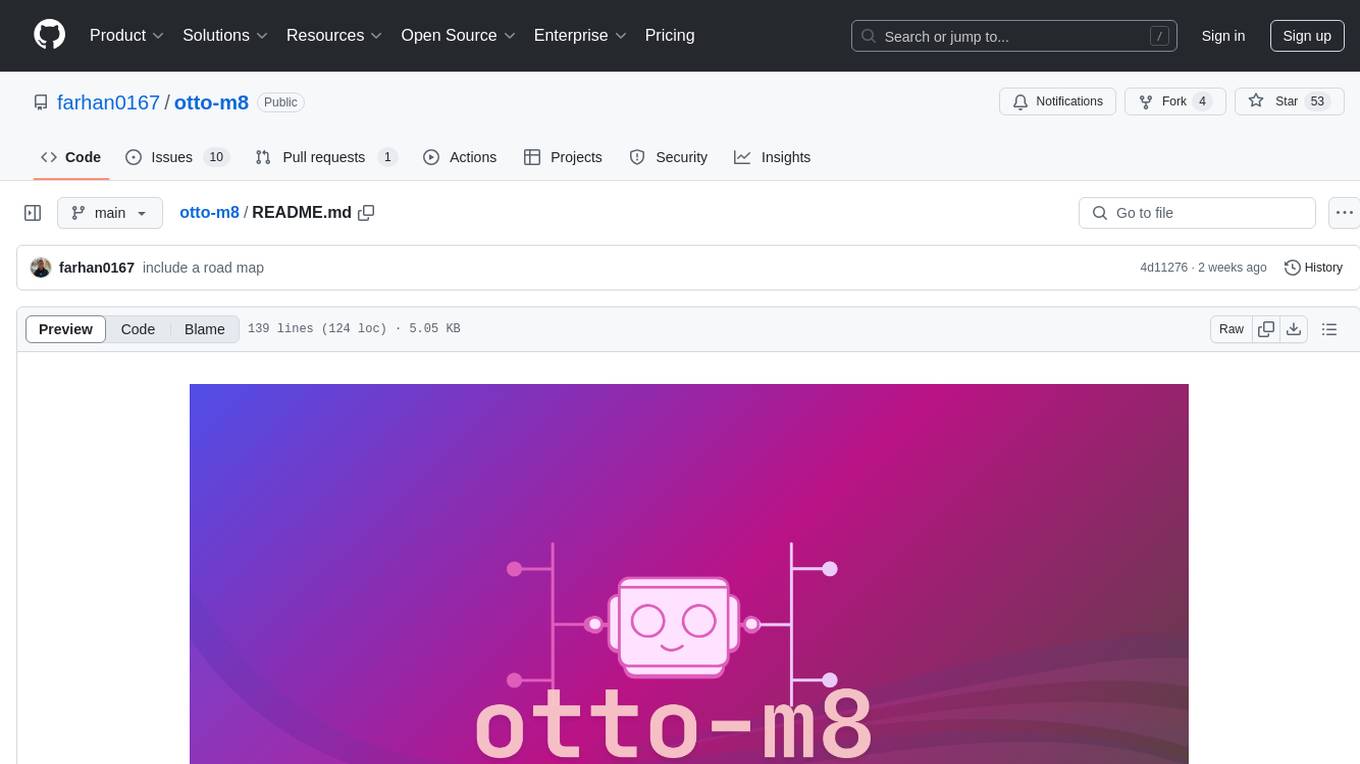
otto-m8
otto-m8 is a flowchart based automation platform designed to run deep learning workloads with minimal to no code. It provides a user-friendly interface to spin up a wide range of AI models, including traditional deep learning models and large language models. The tool deploys Docker containers of workflows as APIs for integration with existing workflows, building AI chatbots, or standalone applications. Otto-m8 operates on an Input, Process, Output paradigm, simplifying the process of running AI models into a flowchart-like UI.
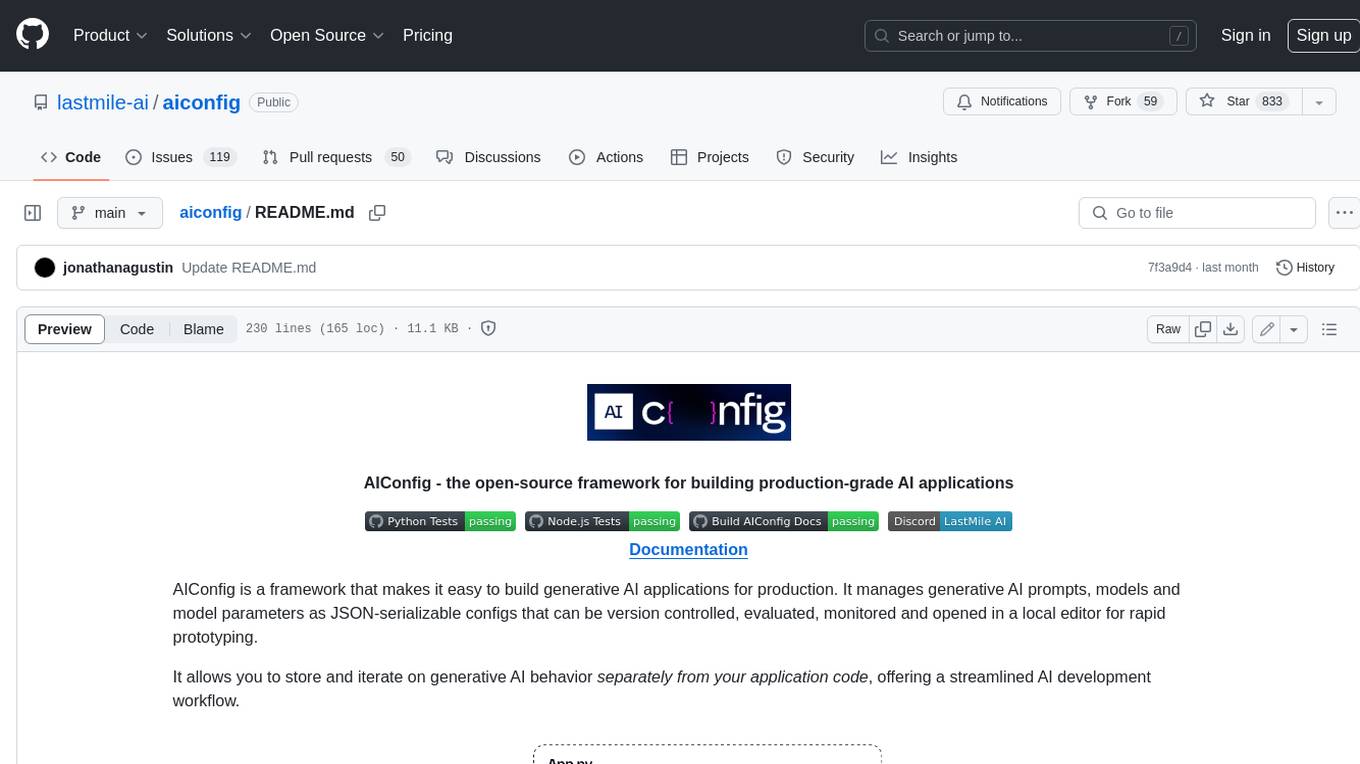
aiconfig
AIConfig is a framework that makes it easy to build generative AI applications for production. It manages generative AI prompts, models and model parameters as JSON-serializable configs that can be version controlled, evaluated, monitored and opened in a local editor for rapid prototyping. It allows you to store and iterate on generative AI behavior separately from your application code, offering a streamlined AI development workflow.
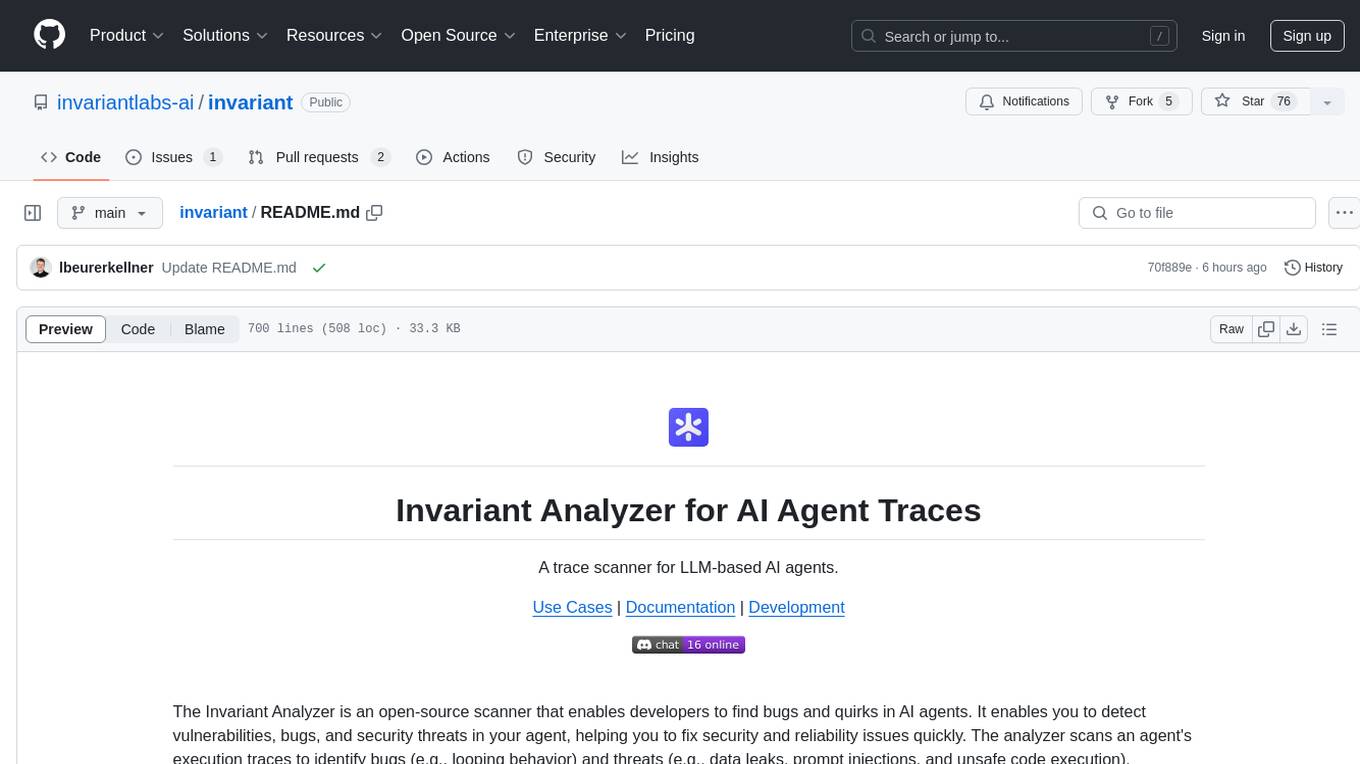
invariant
Invariant Analyzer is an open-source scanner designed for LLM-based AI agents to find bugs, vulnerabilities, and security threats. It scans agent execution traces to identify issues like looping behavior, data leaks, prompt injections, and unsafe code execution. The tool offers a library of built-in checkers, an expressive policy language, data flow analysis, real-time monitoring, and extensible architecture for custom checkers. It helps developers debug AI agents, scan for security violations, and prevent security issues and data breaches during runtime. The analyzer leverages deep contextual understanding and a purpose-built rule matching engine for security policy enforcement.
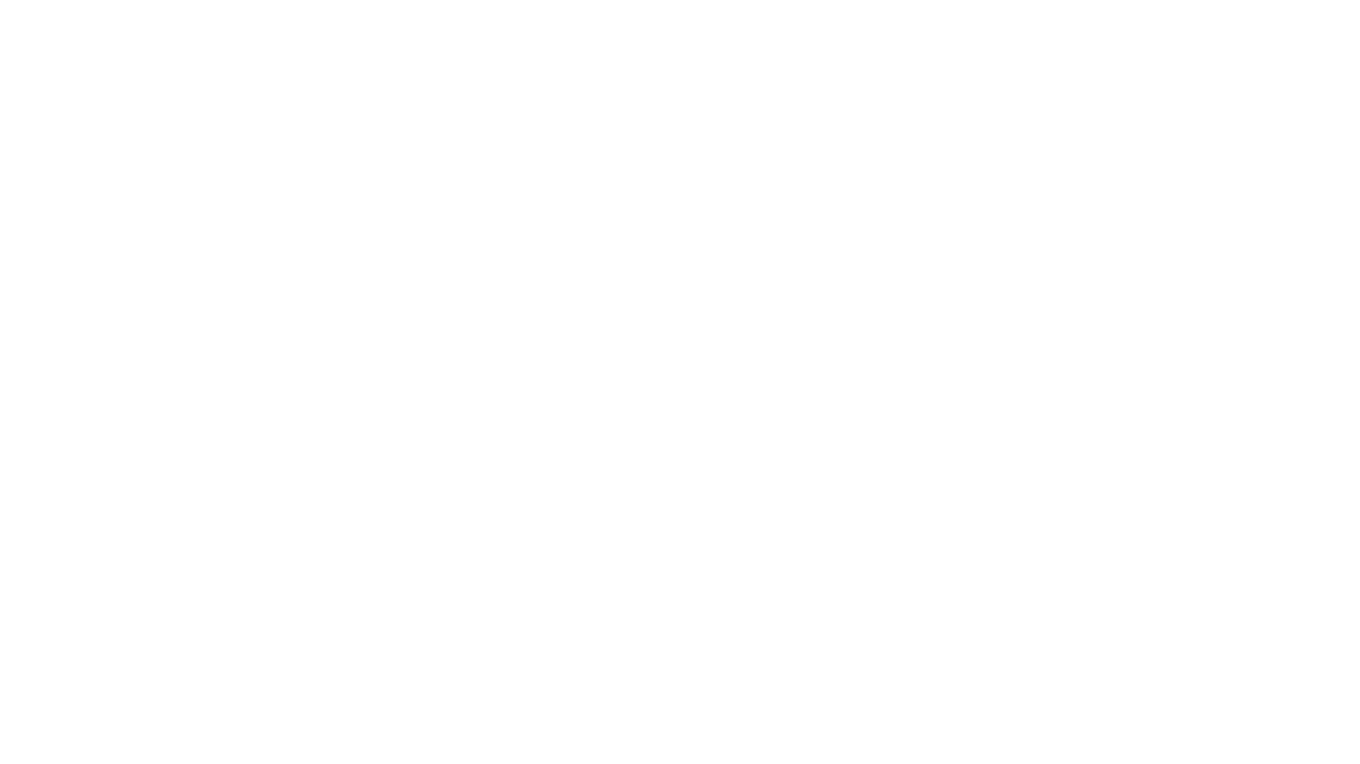
chat-mcp
A Cross-Platform Interface for Large Language Models (LLMs) utilizing the Model Context Protocol (MCP) to connect and interact with various LLMs. The desktop app, built on Electron, ensures compatibility across Linux, macOS, and Windows. It simplifies understanding MCP principles, facilitates testing of multiple servers and LLMs, and supports dynamic LLM configuration and multi-client management. The UI can be extracted for web use, ensuring consistency across web and desktop versions.
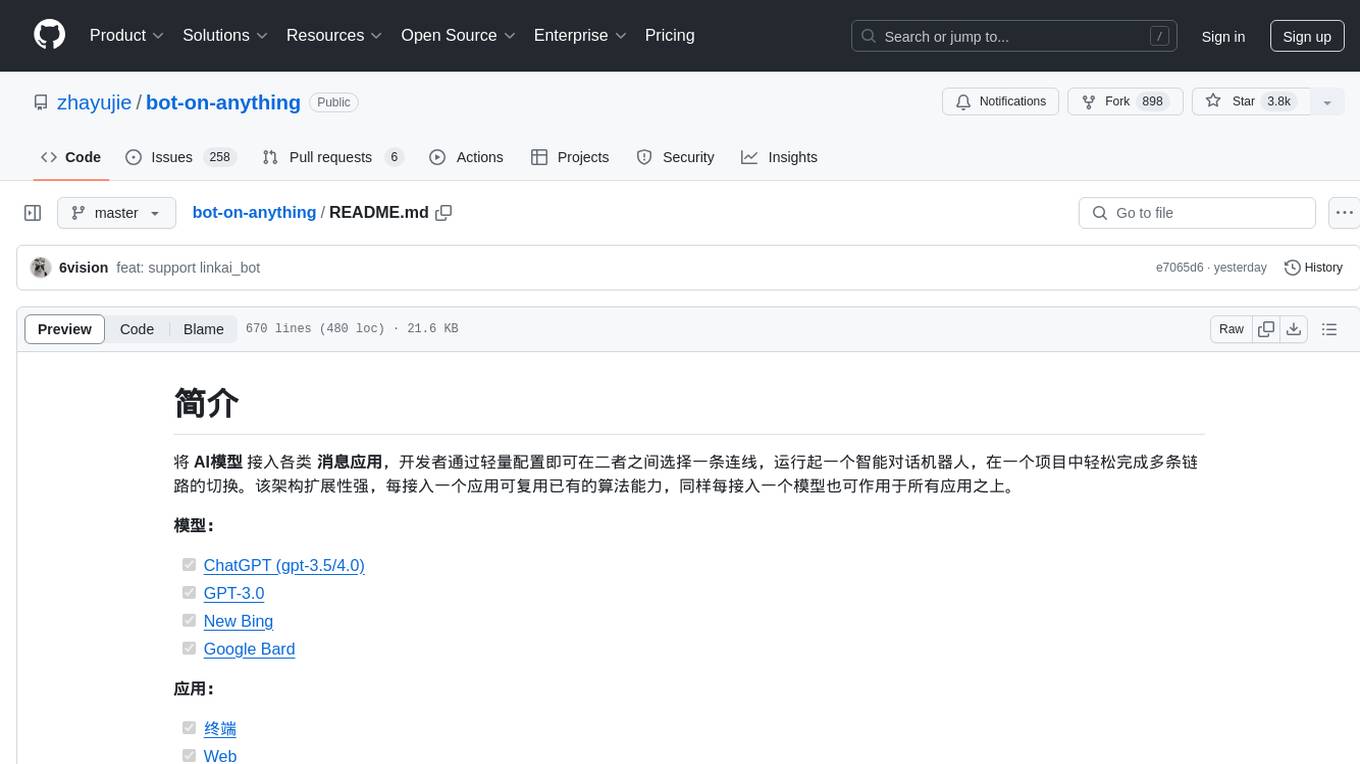
bot-on-anything
The 'bot-on-anything' repository allows developers to integrate various AI models into messaging applications, enabling the creation of intelligent chatbots. By configuring the connections between models and applications, developers can easily switch between multiple channels within a project. The architecture is highly scalable, allowing the reuse of algorithmic capabilities for each new application and model integration. Supported models include ChatGPT, GPT-3.0, New Bing, and Google Bard, while supported applications range from terminals and web platforms to messaging apps like WeChat, Telegram, QQ, and more. The repository provides detailed instructions for setting up the environment, configuring the models and channels, and running the chatbot for various tasks across different messaging platforms.
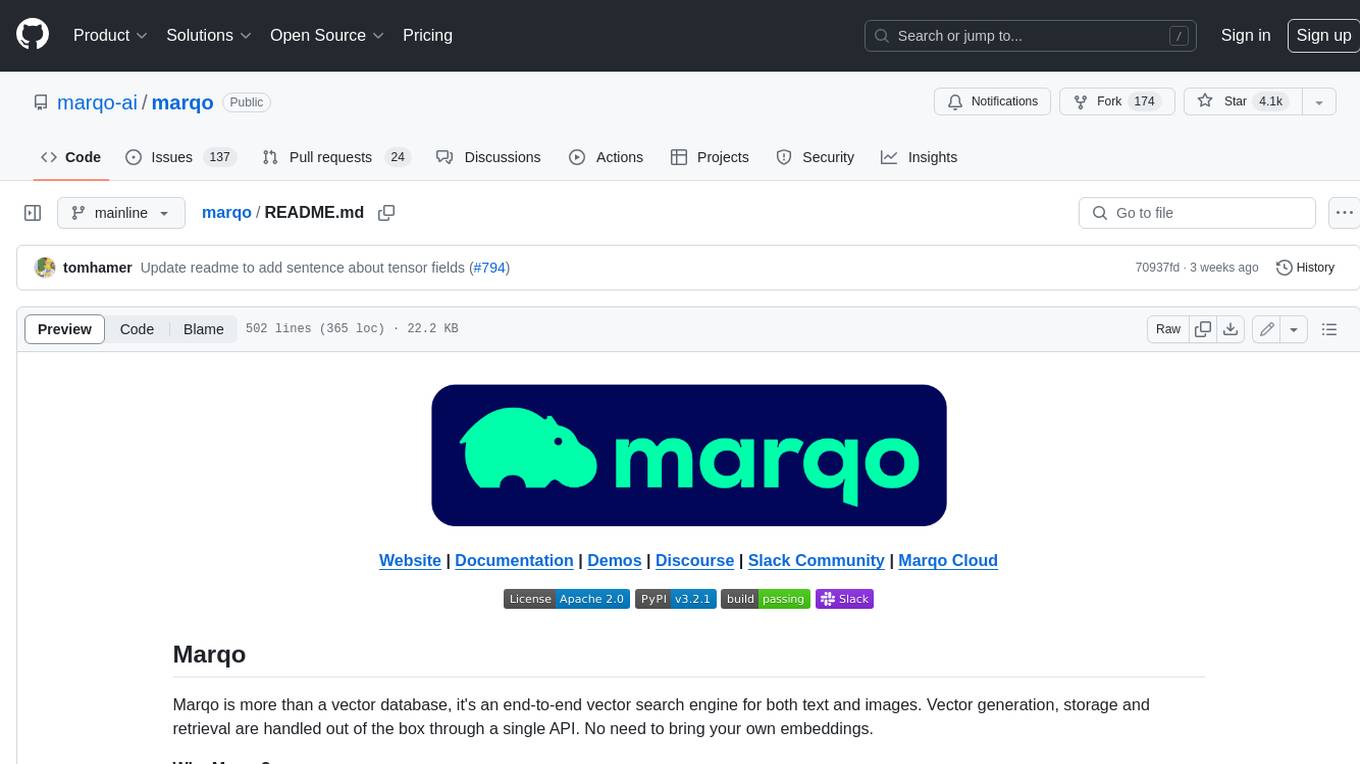
marqo
Marqo is more than a vector database, it's an end-to-end vector search engine for both text and images. Vector generation, storage and retrieval are handled out of the box through a single API. No need to bring your own embeddings.
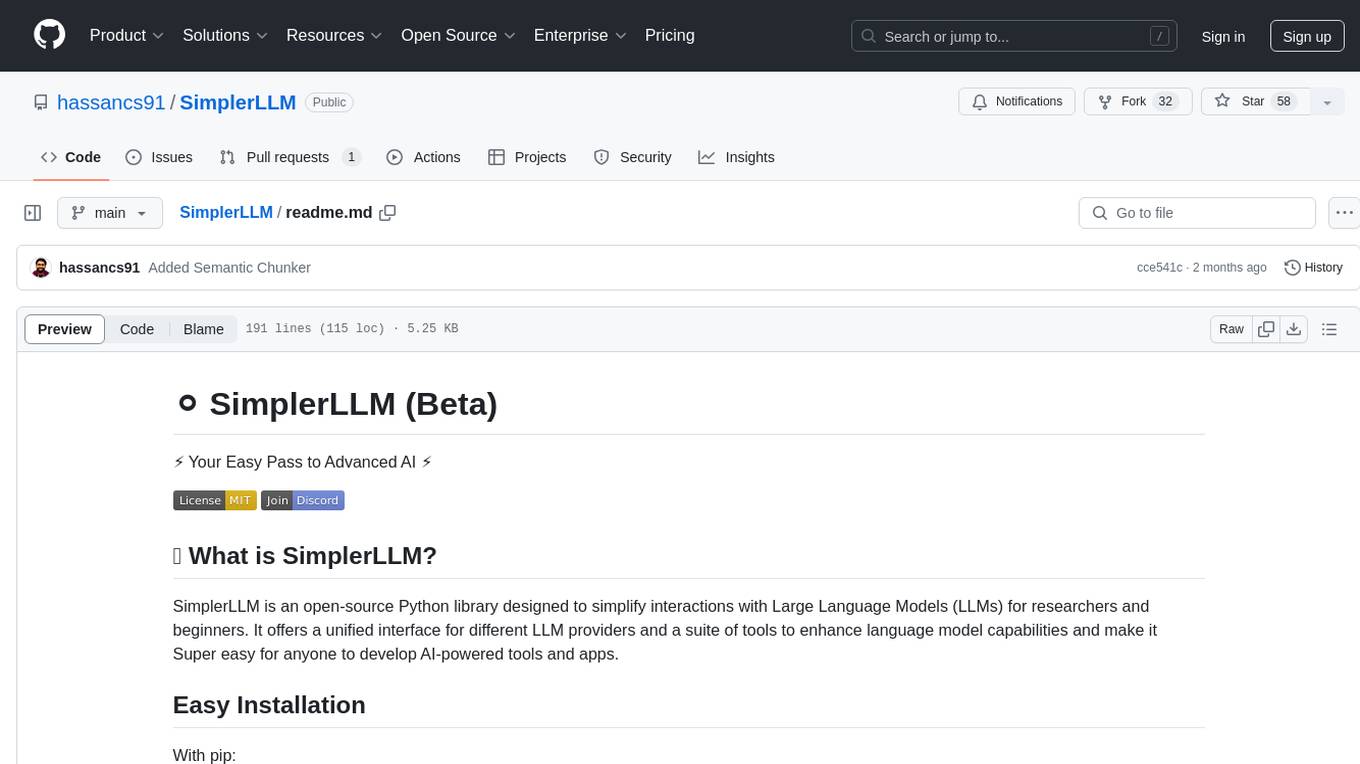
SimplerLLM
SimplerLLM is an open-source Python library that simplifies interactions with Large Language Models (LLMs) for researchers and beginners. It provides a unified interface for different LLM providers, tools for enhancing language model capabilities, and easy development of AI-powered tools and apps. The library offers features like unified LLM interface, generic text loader, RapidAPI connector, SERP integration, prompt template builder, and more. Users can easily set up environment variables, create LLM instances, use tools like SERP, generic text loader, calling RapidAPI APIs, and prompt template builder. Additionally, the library includes chunking functions to split texts into manageable chunks based on different criteria. Future updates will bring more tools, interactions with local LLMs, prompt optimization, response evaluation, GPT Trainer, document chunker, advanced document loader, integration with more providers, Simple RAG with SimplerVectors, integration with vector databases, agent builder, and LLM server.
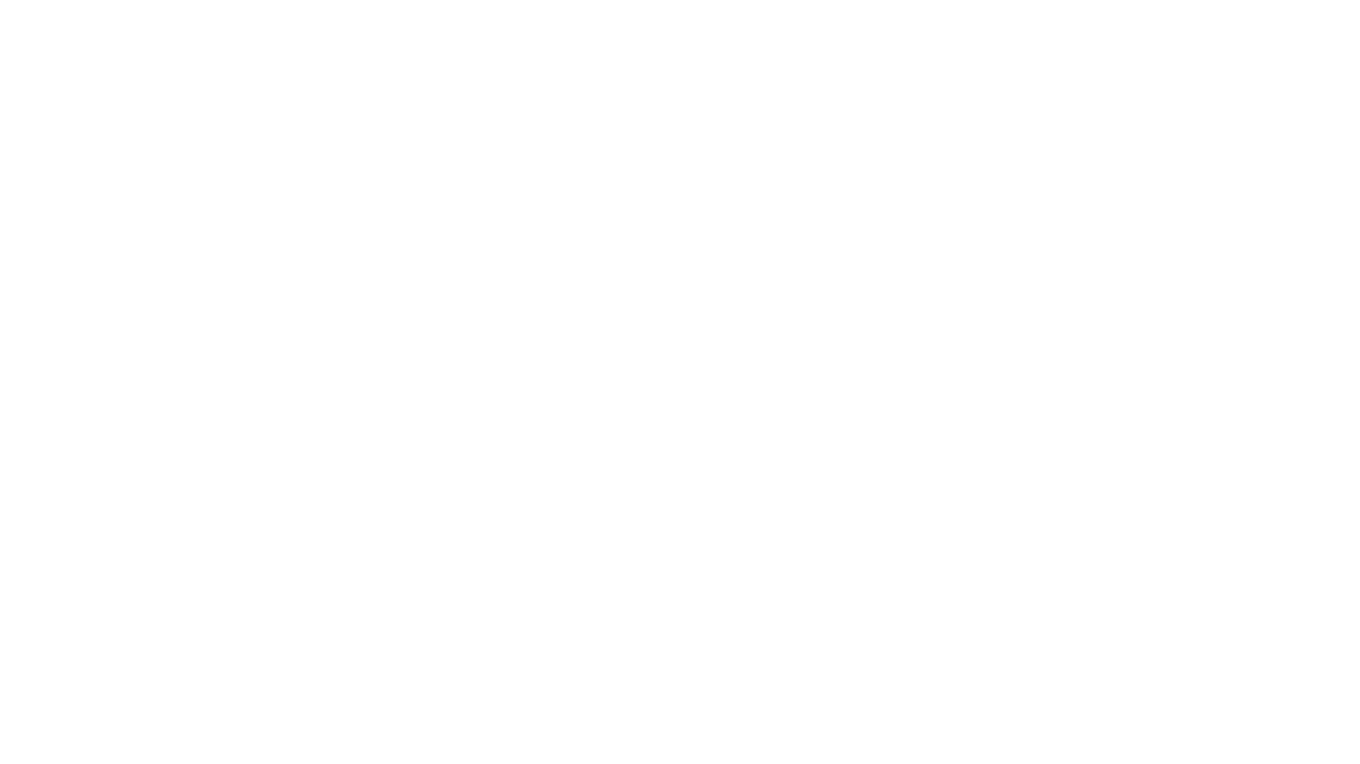
LLamaWorker
LLamaWorker is a HTTP API server developed to provide an OpenAI-compatible API for integrating Large Language Models (LLM) into applications. It supports multi-model configuration, streaming responses, text embedding, chat templates, automatic model release, function calls, API key authentication, and test UI. Users can switch models, complete chats and prompts, manage chat history, and generate tokens through the test UI. Additionally, LLamaWorker offers a Vulkan compiled version for download and provides function call templates for testing. The tool supports various backends and provides API endpoints for chat completion, prompt completion, embeddings, model information, model configuration, and model switching. A Gradio UI demo is also available for testing.
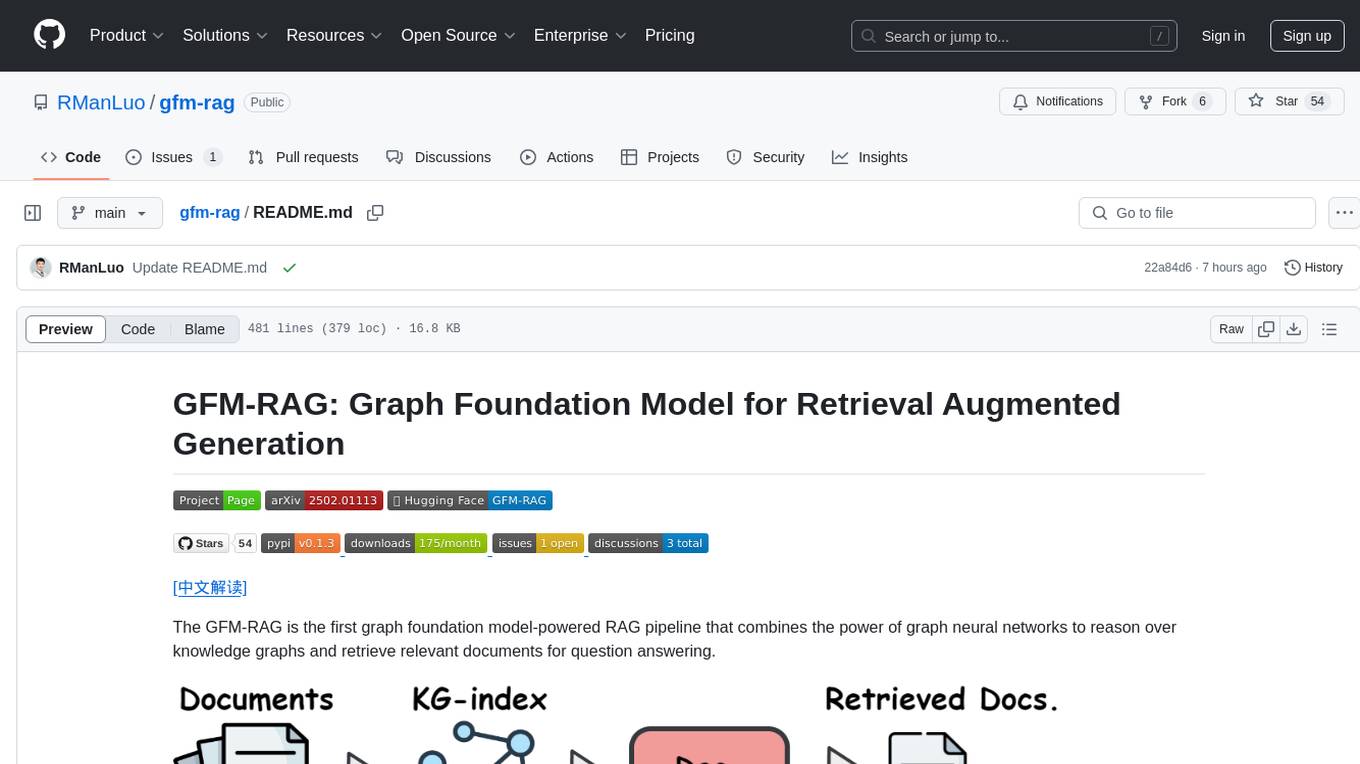
gfm-rag
The GFM-RAG is a graph foundation model-powered pipeline that combines graph neural networks to reason over knowledge graphs and retrieve relevant documents for question answering. It features a knowledge graph index, efficiency in multi-hop reasoning, generalizability to unseen datasets, transferability for fine-tuning, compatibility with agent-based frameworks, and interpretability of reasoning paths. The tool can be used for conducting retrieval and question answering tasks using pre-trained models or fine-tuning on custom datasets.
For similar tasks
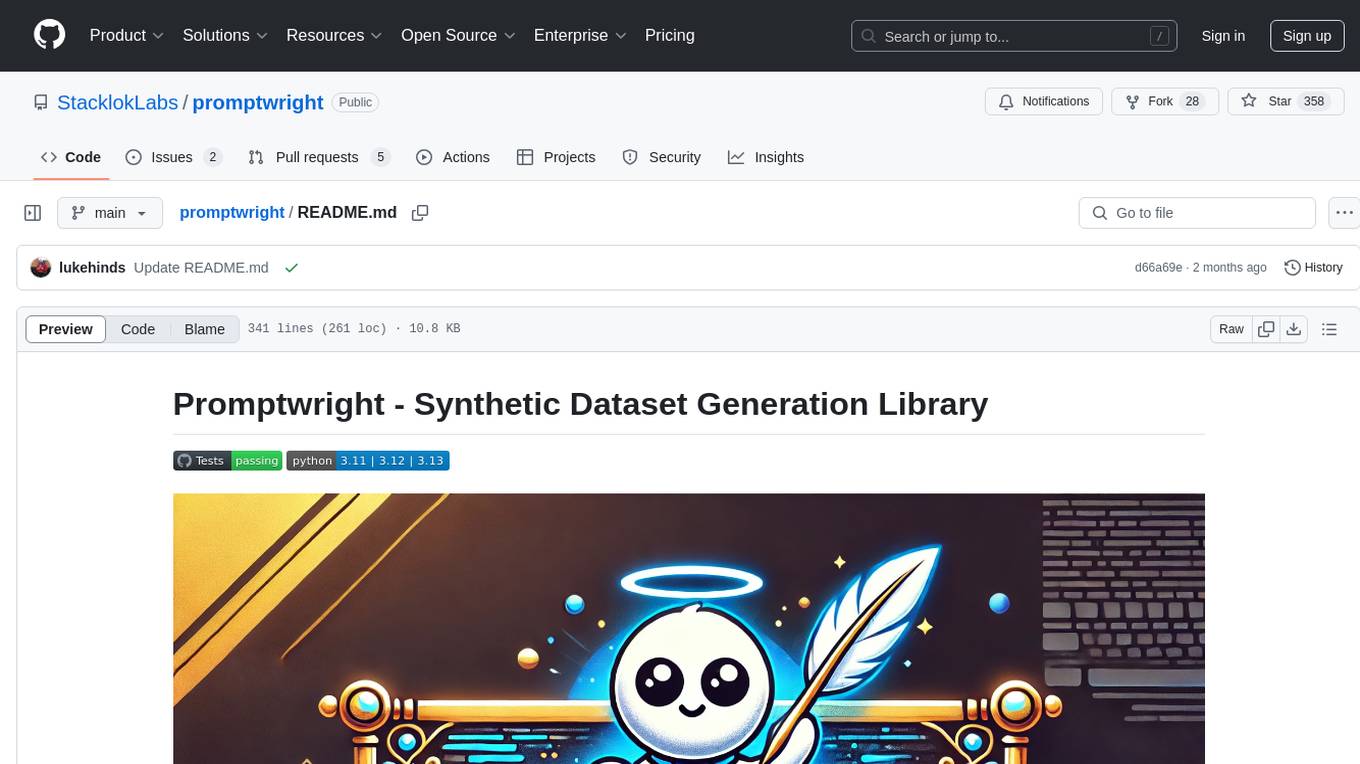
promptwright
Promptwright is a Python library designed for generating large synthetic datasets using a local LLM and various LLM service providers. It offers flexible interfaces for generating prompt-led synthetic datasets. The library supports multiple providers, configurable instructions and prompts, YAML configuration for tasks, command line interface for running tasks, push to Hugging Face Hub for dataset upload, and system message control. Users can define generation tasks using YAML configuration or Python code. Promptwright integrates with LiteLLM to interface with LLM providers and supports automatic dataset upload to Hugging Face Hub.
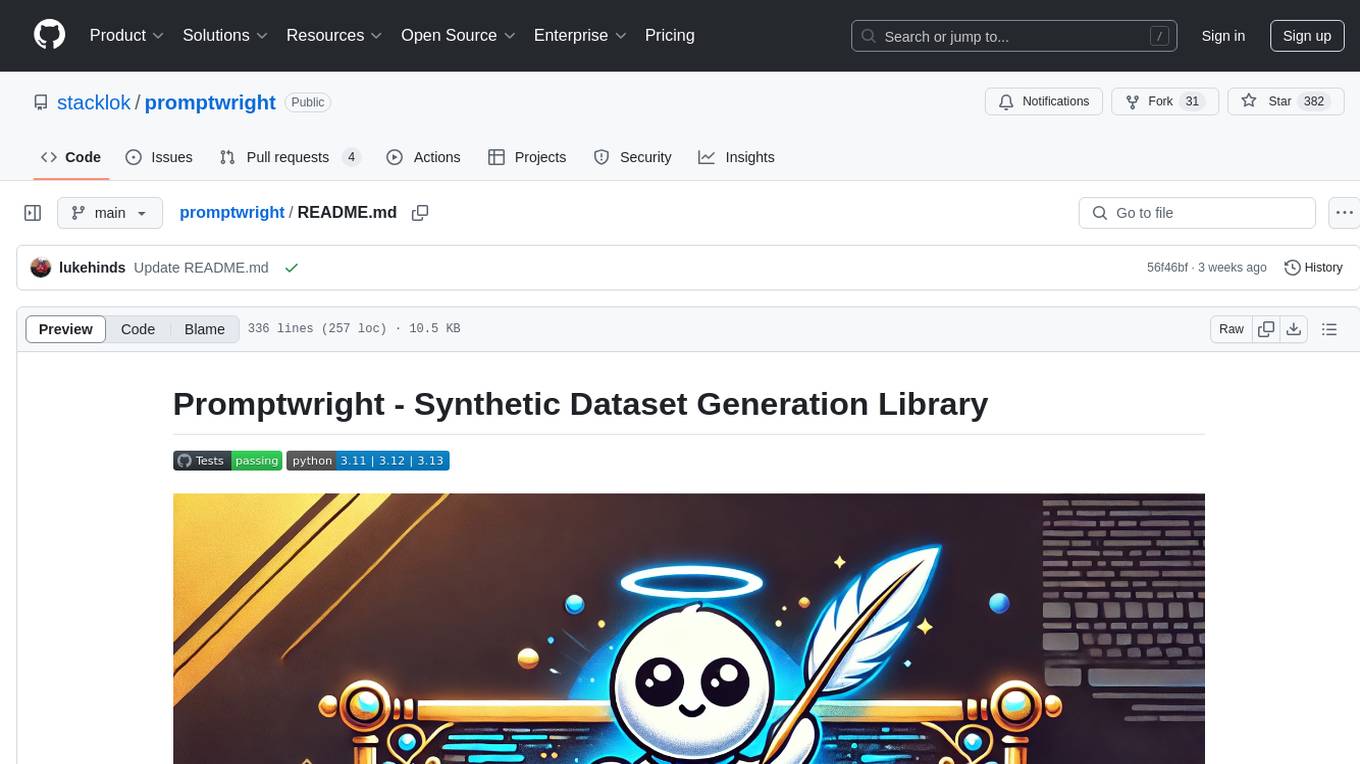
promptwright
Promptwright is a Python library designed for generating large synthetic datasets using local LLM and various LLM service providers. It offers flexible interfaces for generating prompt-led synthetic datasets. The library supports multiple providers, configurable instructions and prompts, YAML configuration, command line interface, push to Hugging Face Hub, and system message control. Users can define generation tasks using YAML configuration files or programmatically using Python code. Promptwright integrates with LiteLLM for LLM providers and supports automatic dataset upload to Hugging Face Hub. The library is not responsible for the content generated by models and advises users to review the data before using it in production environments.
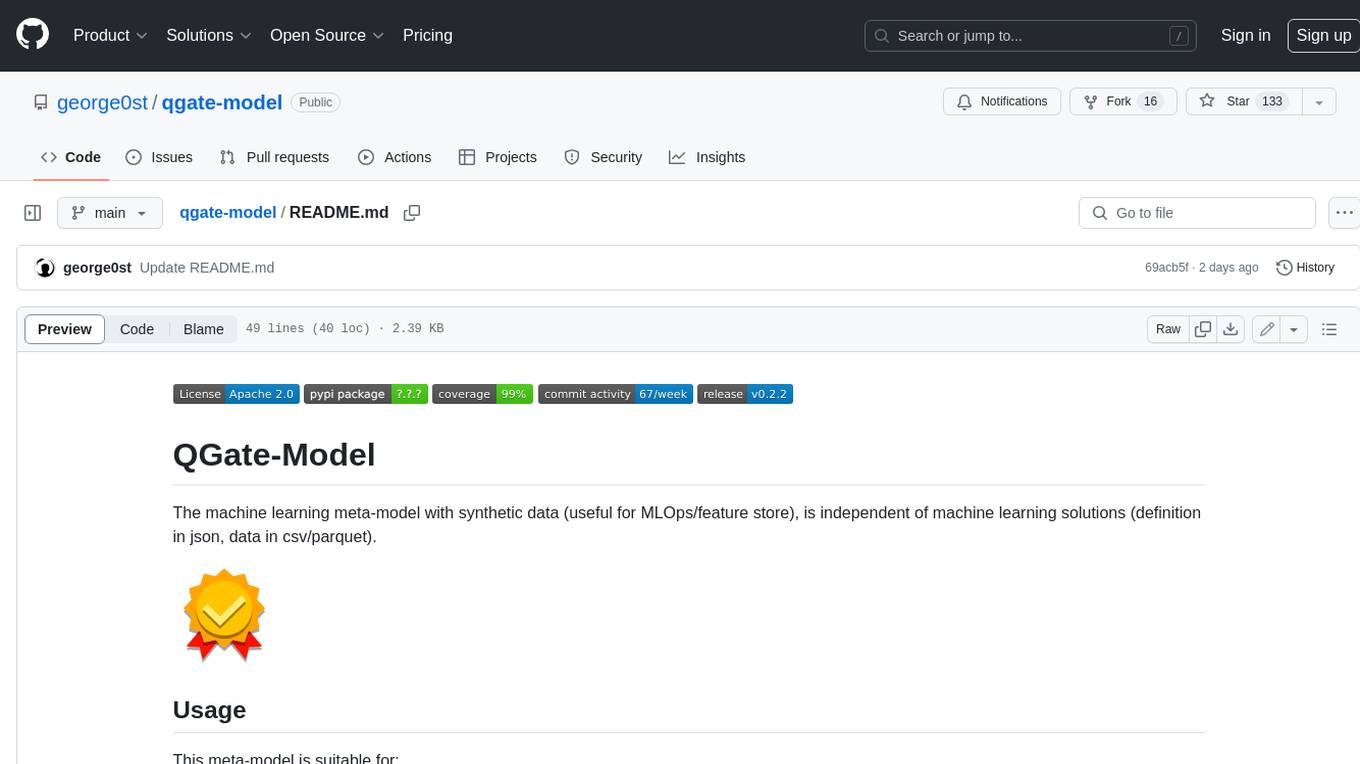
qgate-model
QGate-Model is a machine learning meta-model with synthetic data, designed for MLOps and feature store. It is independent of machine learning solutions, with definitions in JSON and data in CSV/parquet formats. This meta-model is useful for comparing capabilities and functions of machine learning solutions, independently testing new versions of machine learning solutions, and conducting various types of tests (unit, sanity, smoke, system, regression, function, acceptance, performance, shadow, etc.). It can also be used for external test coverage when internal test coverage is not available or weak.
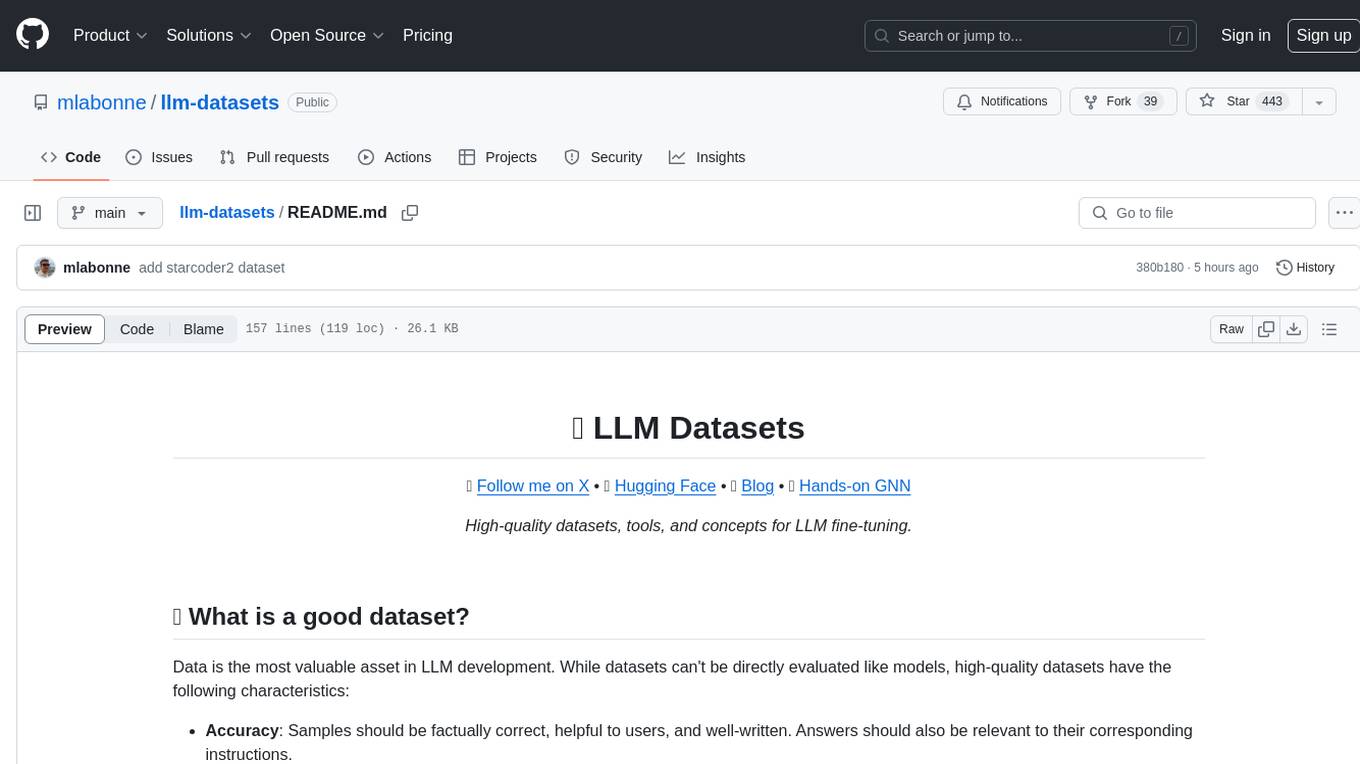
llm-datasets
LLM Datasets is a repository containing high-quality datasets, tools, and concepts for LLM fine-tuning. It provides datasets with characteristics like accuracy, diversity, and complexity to train large language models for various tasks. The repository includes datasets for general-purpose, math & logic, code, conversation & role-play, and agent & function calling domains. It also offers guidance on creating high-quality datasets through data deduplication, data quality assessment, data exploration, and data generation techniques.
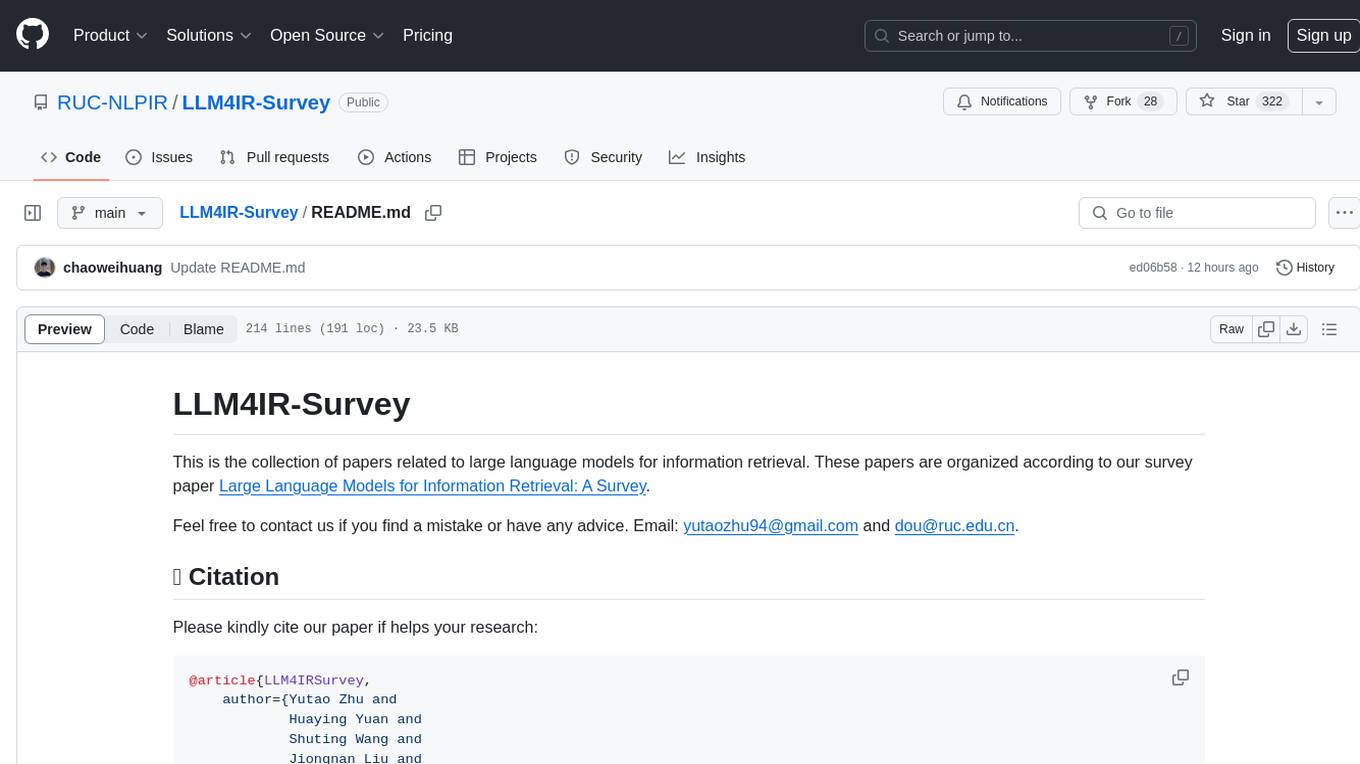
LLM4IR-Survey
LLM4IR-Survey is a collection of papers related to large language models for information retrieval, organized according to the survey paper 'Large Language Models for Information Retrieval: A Survey'. It covers various aspects such as query rewriting, retrievers, rerankers, readers, search agents, and more, providing insights into the integration of large language models with information retrieval systems.
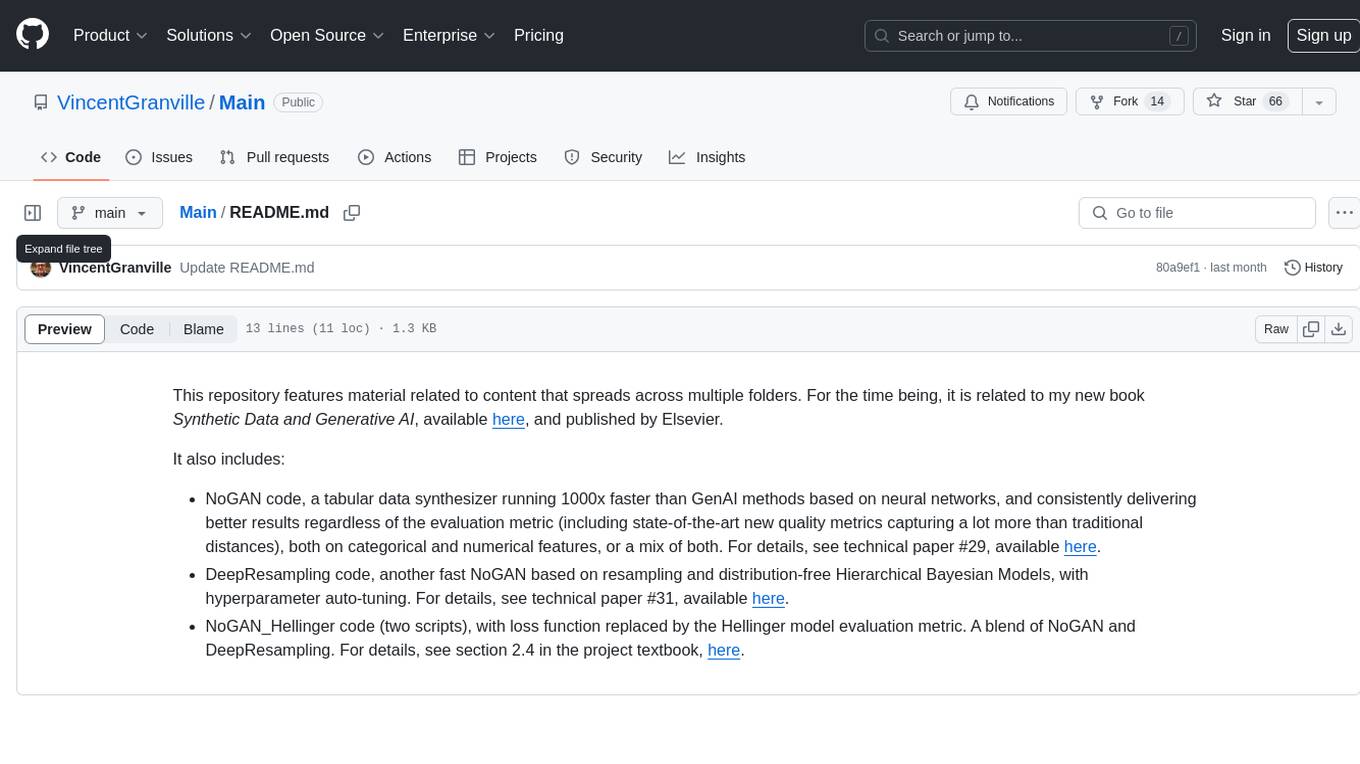
Main
This repository contains material related to the new book _Synthetic Data and Generative AI_ by the author, including code for NoGAN, DeepResampling, and NoGAN_Hellinger. NoGAN is a tabular data synthesizer that outperforms GenAI methods in terms of speed and results, utilizing state-of-the-art quality metrics. DeepResampling is a fast NoGAN based on resampling and Bayesian Models with hyperparameter auto-tuning. NoGAN_Hellinger combines NoGAN and DeepResampling with the Hellinger model evaluation metric.
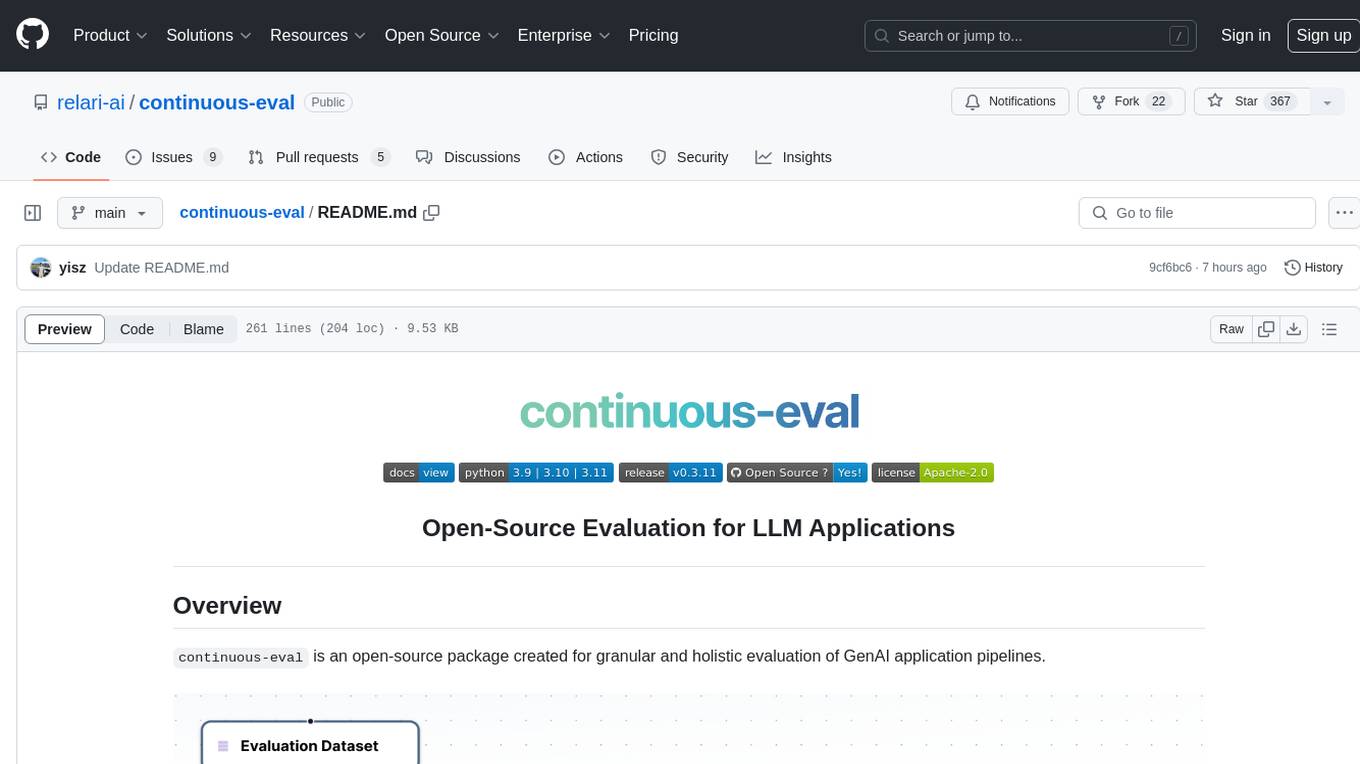
continuous-eval
Open-Source Evaluation for LLM Applications. `continuous-eval` is an open-source package created for granular and holistic evaluation of GenAI application pipelines. It offers modularized evaluation, a comprehensive metric library covering various LLM use cases, the ability to leverage user feedback in evaluation, and synthetic dataset generation for testing pipelines. Users can define their own metrics by extending the Metric class. The tool allows running evaluation on a pipeline defined with modules and corresponding metrics. Additionally, it provides synthetic data generation capabilities to create user interaction data for evaluation or training purposes.
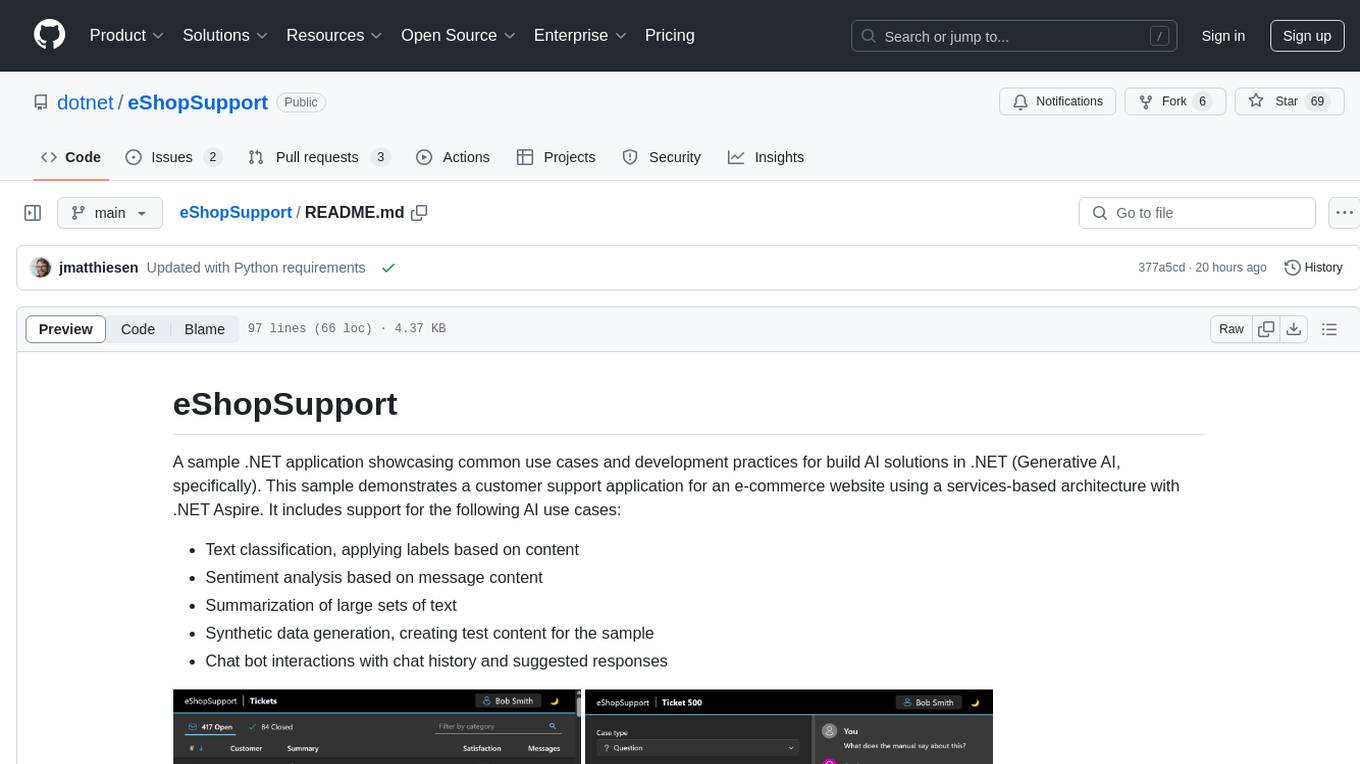
eShopSupport
eShopSupport is a sample .NET application showcasing common use cases and development practices for building AI solutions in .NET, specifically Generative AI. It demonstrates a customer support application for an e-commerce website using a services-based architecture with .NET Aspire. The application includes support for text classification, sentiment analysis, text summarization, synthetic data generation, and chat bot interactions. It also showcases development practices such as developing solutions locally, evaluating AI responses, leveraging Python projects, and deploying applications to the Cloud.
For similar jobs
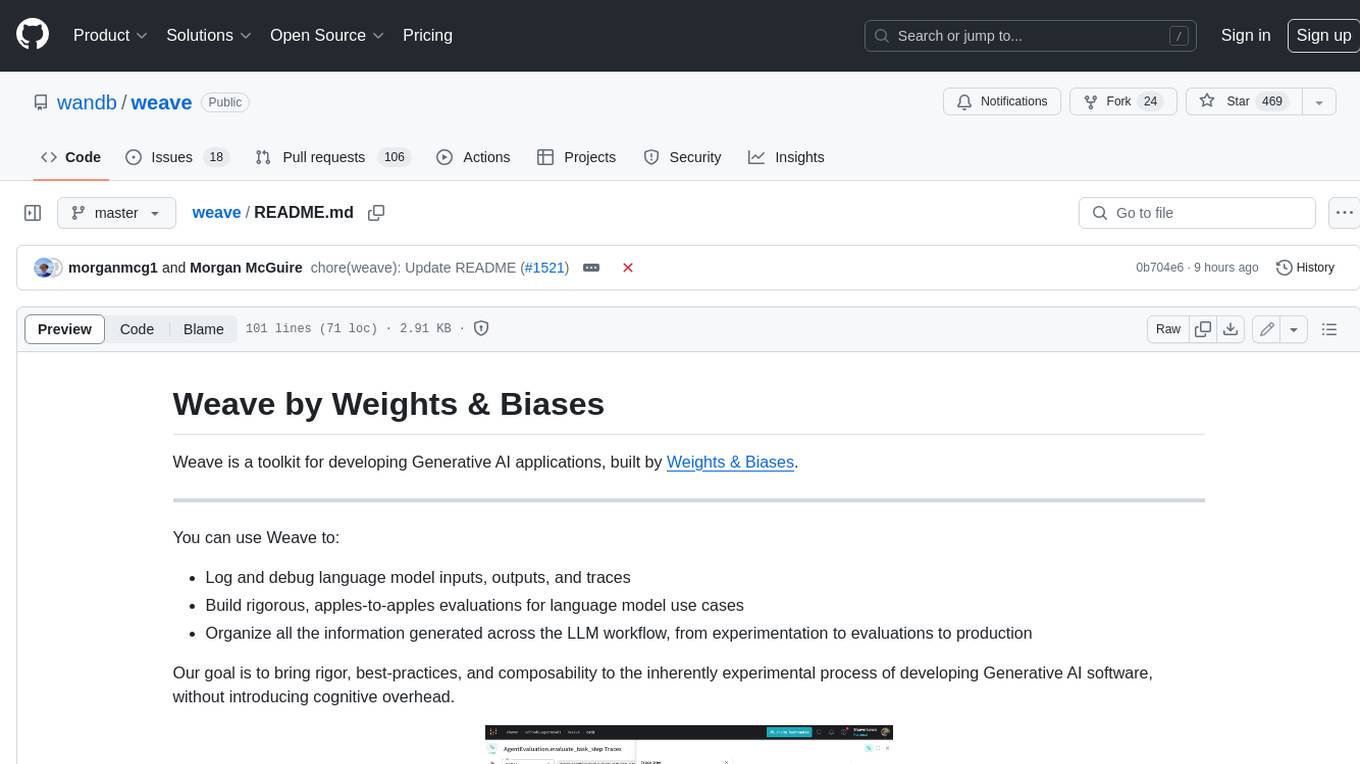
weave
Weave is a toolkit for developing Generative AI applications, built by Weights & Biases. With Weave, you can log and debug language model inputs, outputs, and traces; build rigorous, apples-to-apples evaluations for language model use cases; and organize all the information generated across the LLM workflow, from experimentation to evaluations to production. Weave aims to bring rigor, best-practices, and composability to the inherently experimental process of developing Generative AI software, without introducing cognitive overhead.
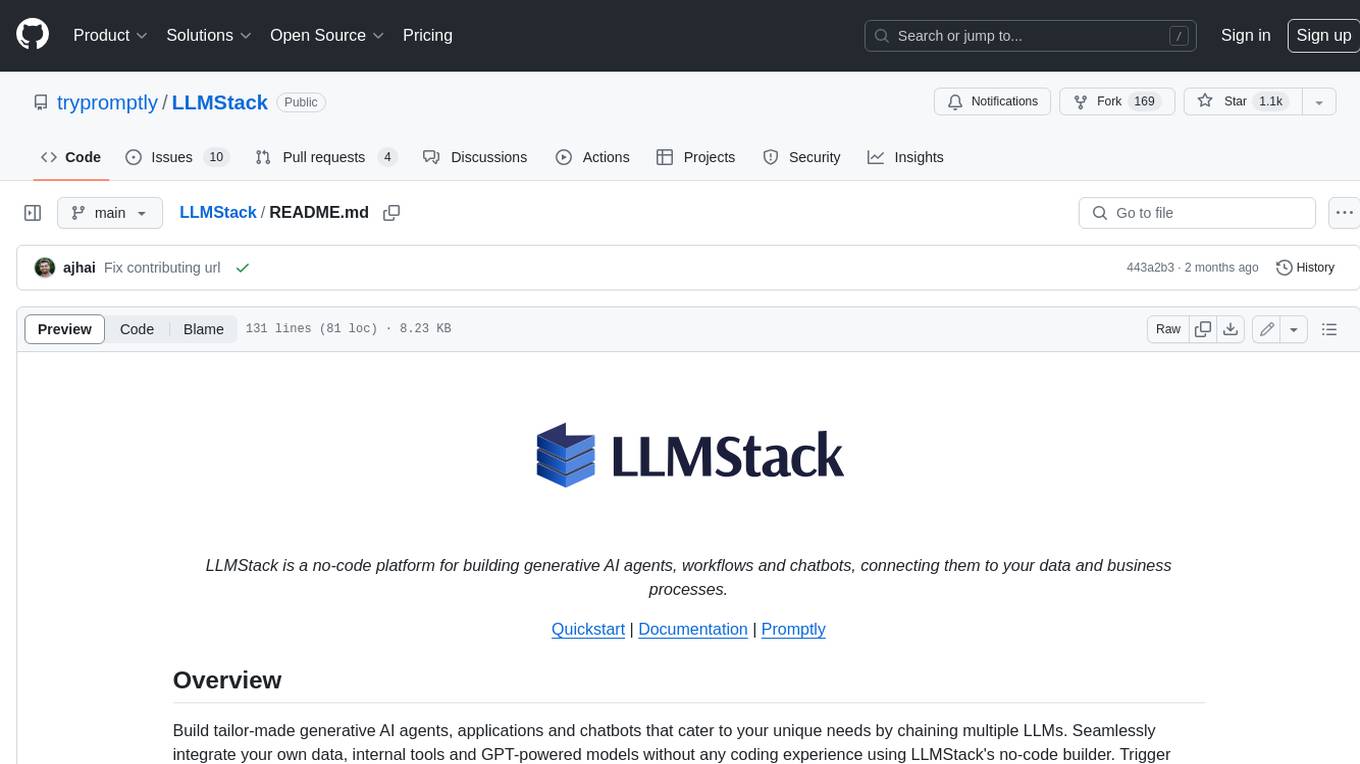
LLMStack
LLMStack is a no-code platform for building generative AI agents, workflows, and chatbots. It allows users to connect their own data, internal tools, and GPT-powered models without any coding experience. LLMStack can be deployed to the cloud or on-premise and can be accessed via HTTP API or triggered from Slack or Discord.
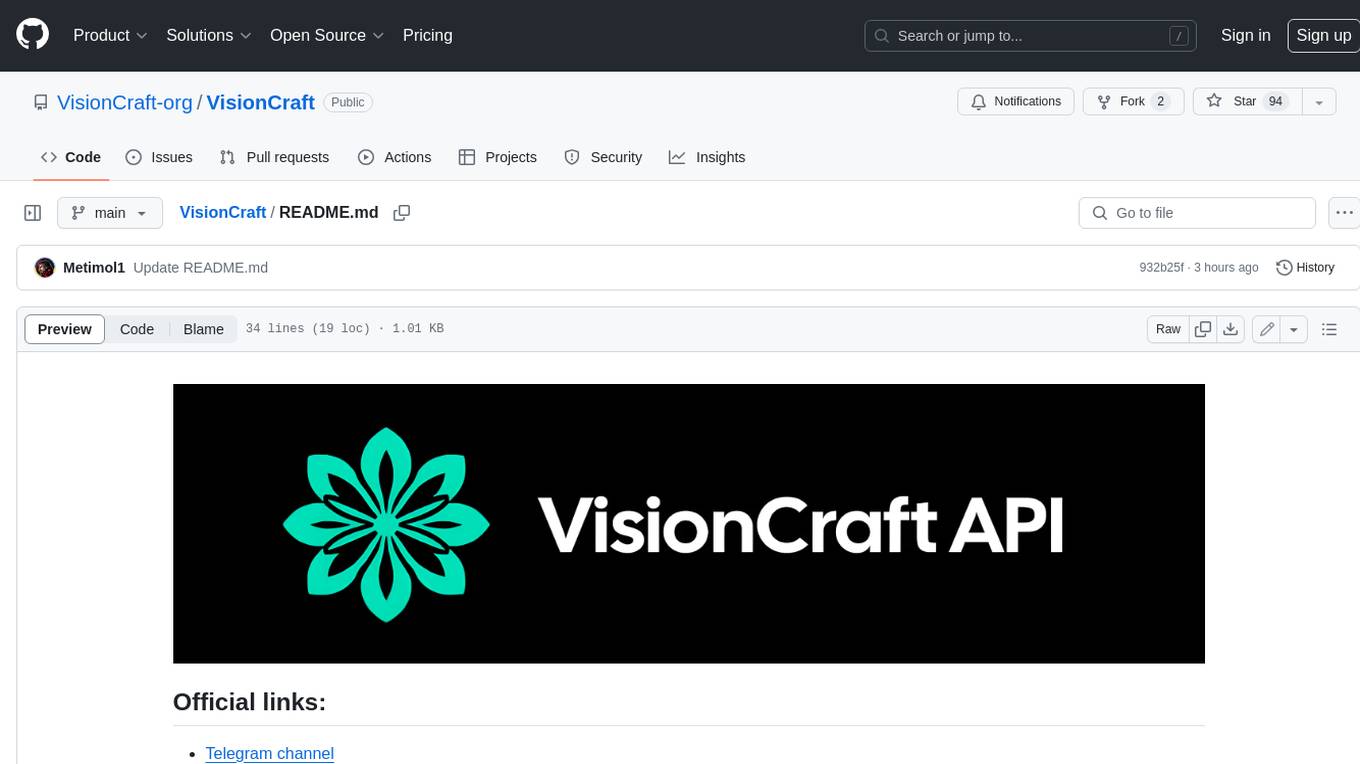
VisionCraft
The VisionCraft API is a free API for using over 100 different AI models. From images to sound.
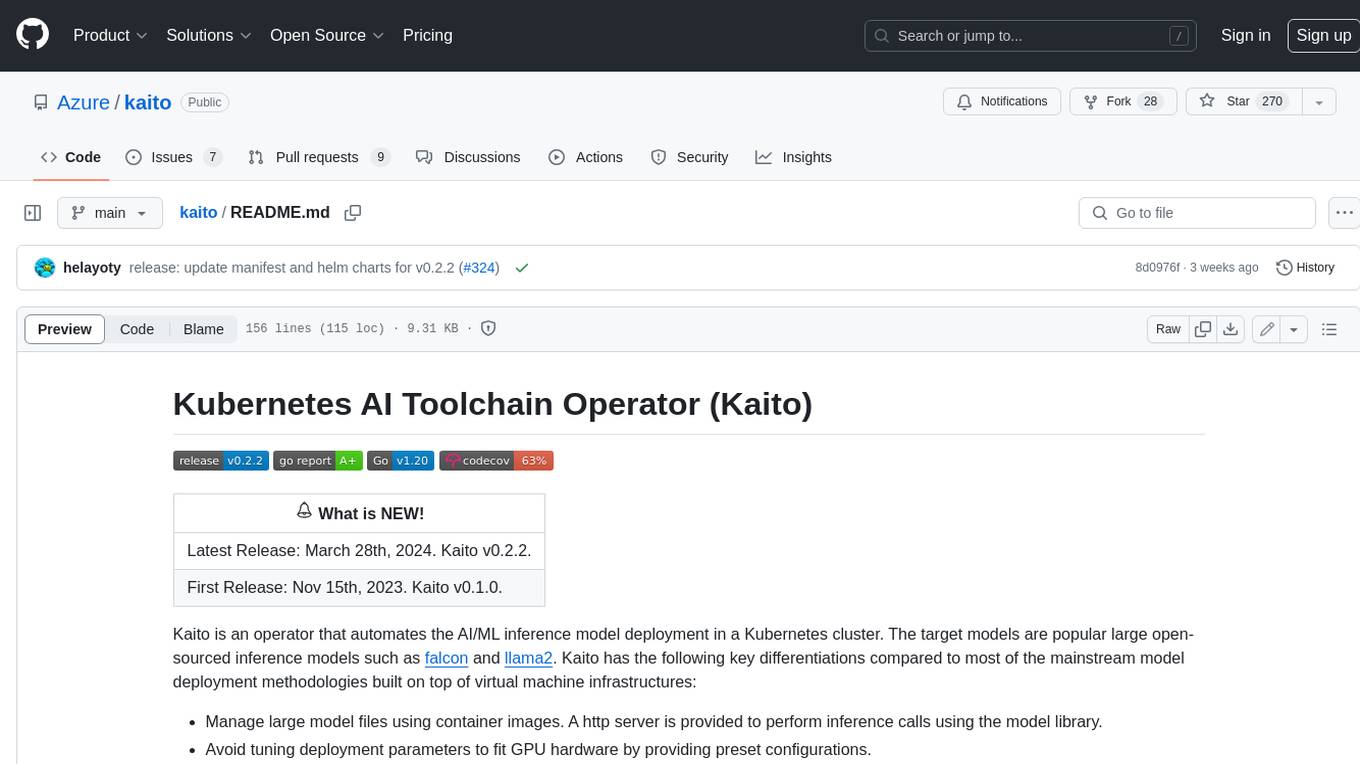
kaito
Kaito is an operator that automates the AI/ML inference model deployment in a Kubernetes cluster. It manages large model files using container images, avoids tuning deployment parameters to fit GPU hardware by providing preset configurations, auto-provisions GPU nodes based on model requirements, and hosts large model images in the public Microsoft Container Registry (MCR) if the license allows. Using Kaito, the workflow of onboarding large AI inference models in Kubernetes is largely simplified.
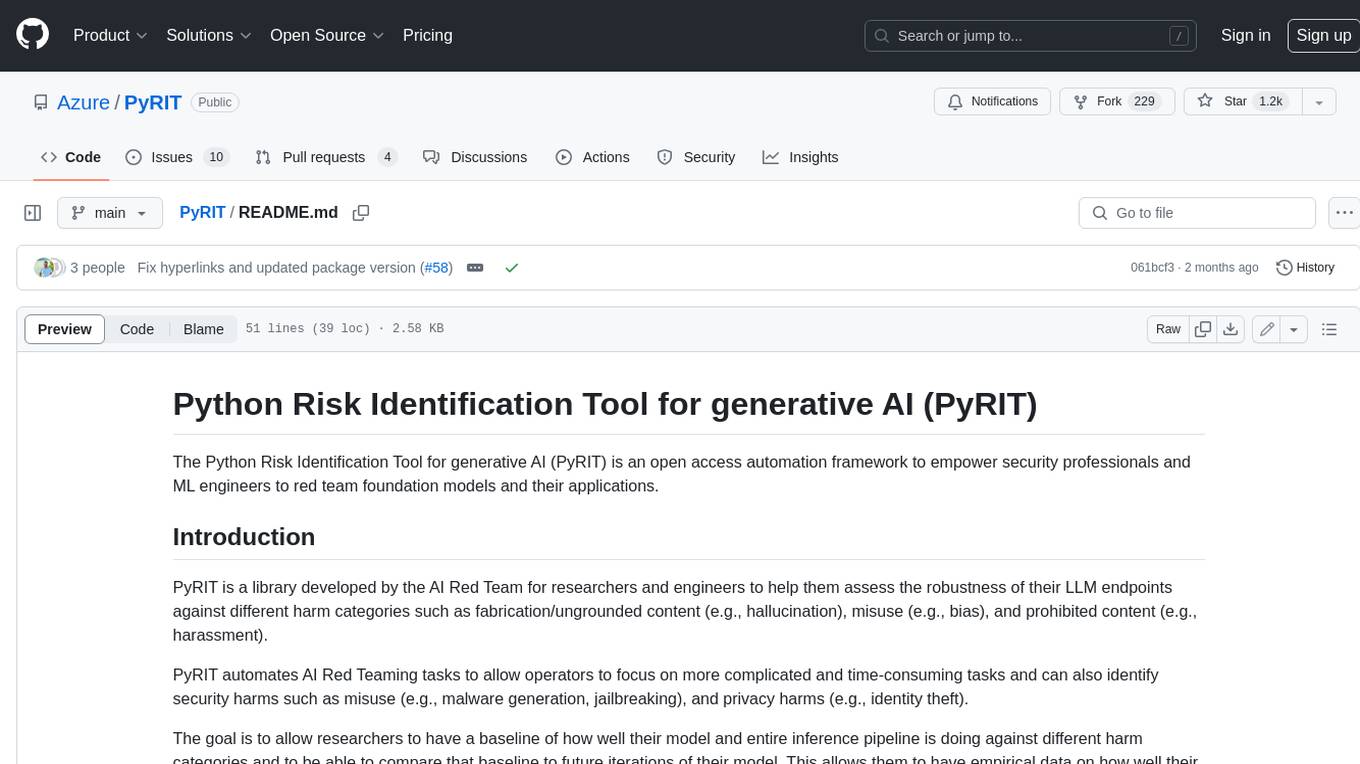
PyRIT
PyRIT is an open access automation framework designed to empower security professionals and ML engineers to red team foundation models and their applications. It automates AI Red Teaming tasks to allow operators to focus on more complicated and time-consuming tasks and can also identify security harms such as misuse (e.g., malware generation, jailbreaking), and privacy harms (e.g., identity theft). The goal is to allow researchers to have a baseline of how well their model and entire inference pipeline is doing against different harm categories and to be able to compare that baseline to future iterations of their model. This allows them to have empirical data on how well their model is doing today, and detect any degradation of performance based on future improvements.
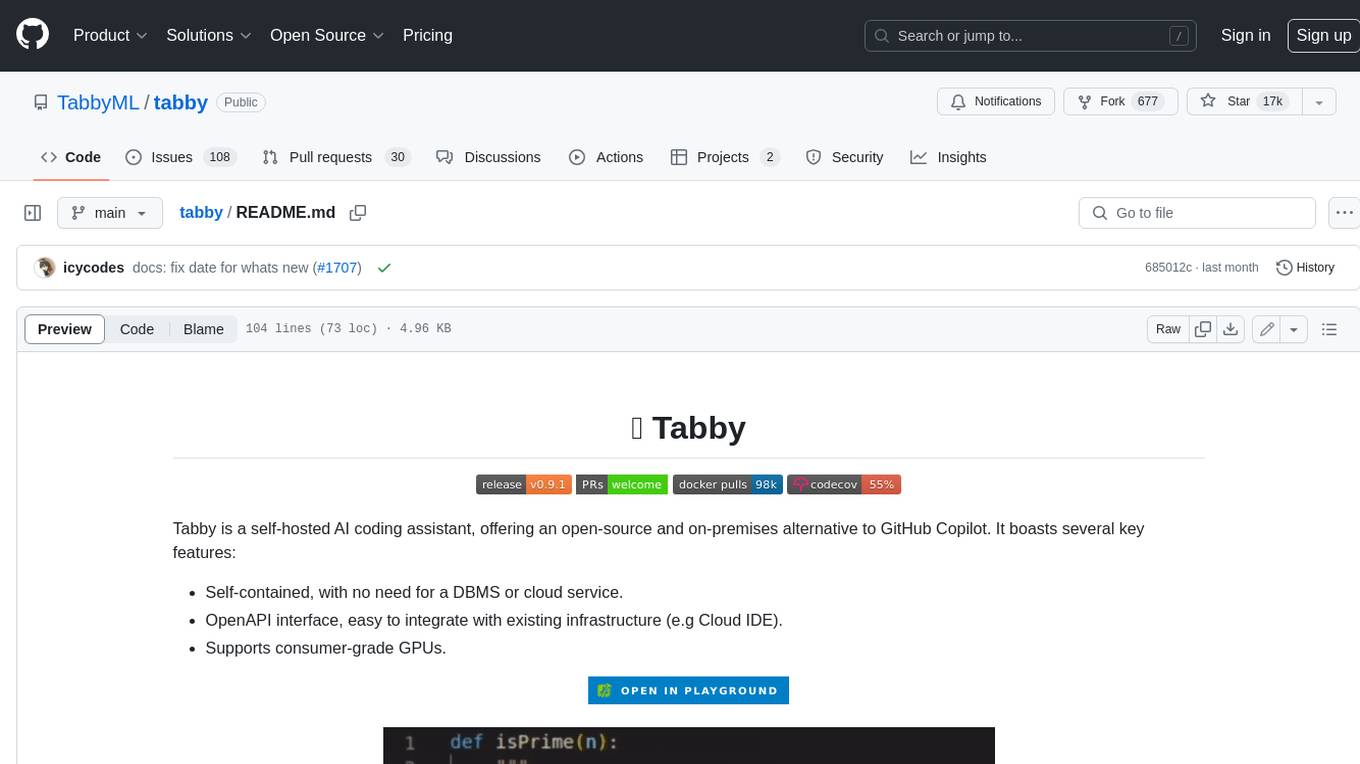
tabby
Tabby is a self-hosted AI coding assistant, offering an open-source and on-premises alternative to GitHub Copilot. It boasts several key features: * Self-contained, with no need for a DBMS or cloud service. * OpenAPI interface, easy to integrate with existing infrastructure (e.g Cloud IDE). * Supports consumer-grade GPUs.
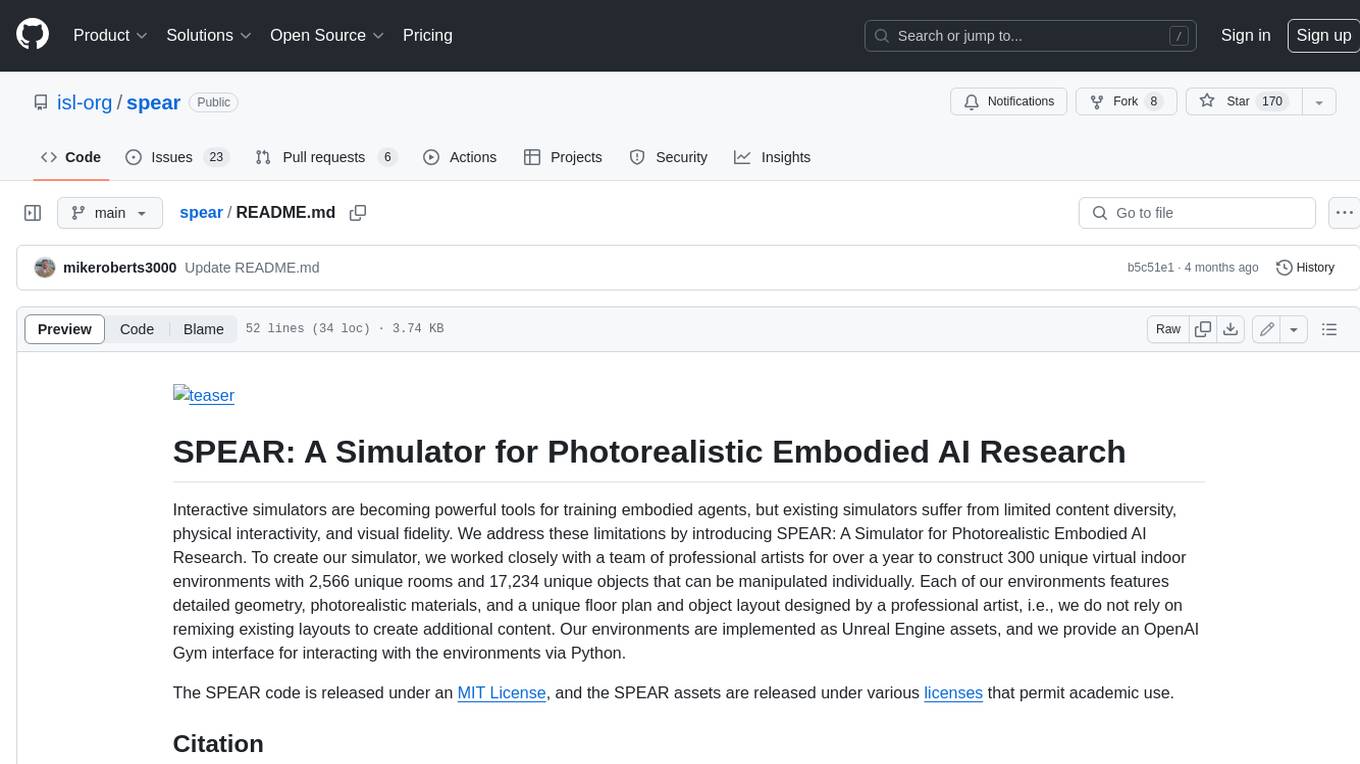
spear
SPEAR (Simulator for Photorealistic Embodied AI Research) is a powerful tool for training embodied agents. It features 300 unique virtual indoor environments with 2,566 unique rooms and 17,234 unique objects that can be manipulated individually. Each environment is designed by a professional artist and features detailed geometry, photorealistic materials, and a unique floor plan and object layout. SPEAR is implemented as Unreal Engine assets and provides an OpenAI Gym interface for interacting with the environments via Python.
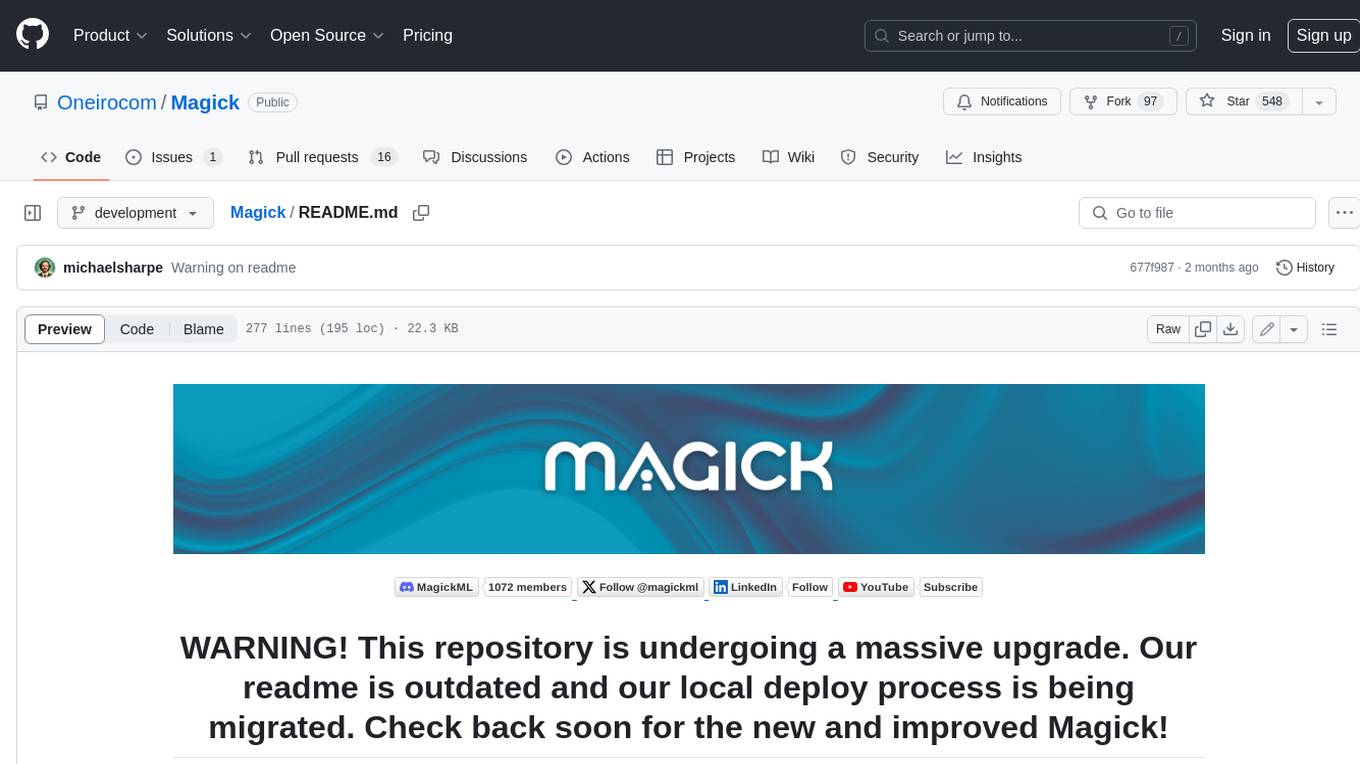
Magick
Magick is a groundbreaking visual AIDE (Artificial Intelligence Development Environment) for no-code data pipelines and multimodal agents. Magick can connect to other services and comes with nodes and templates well-suited for intelligent agents, chatbots, complex reasoning systems and realistic characters.