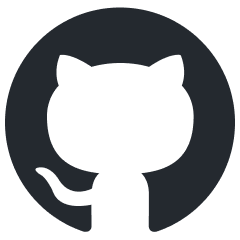
LLM4IR-Survey
This is the repo for the survey of LLM4IR.
Stars: 390
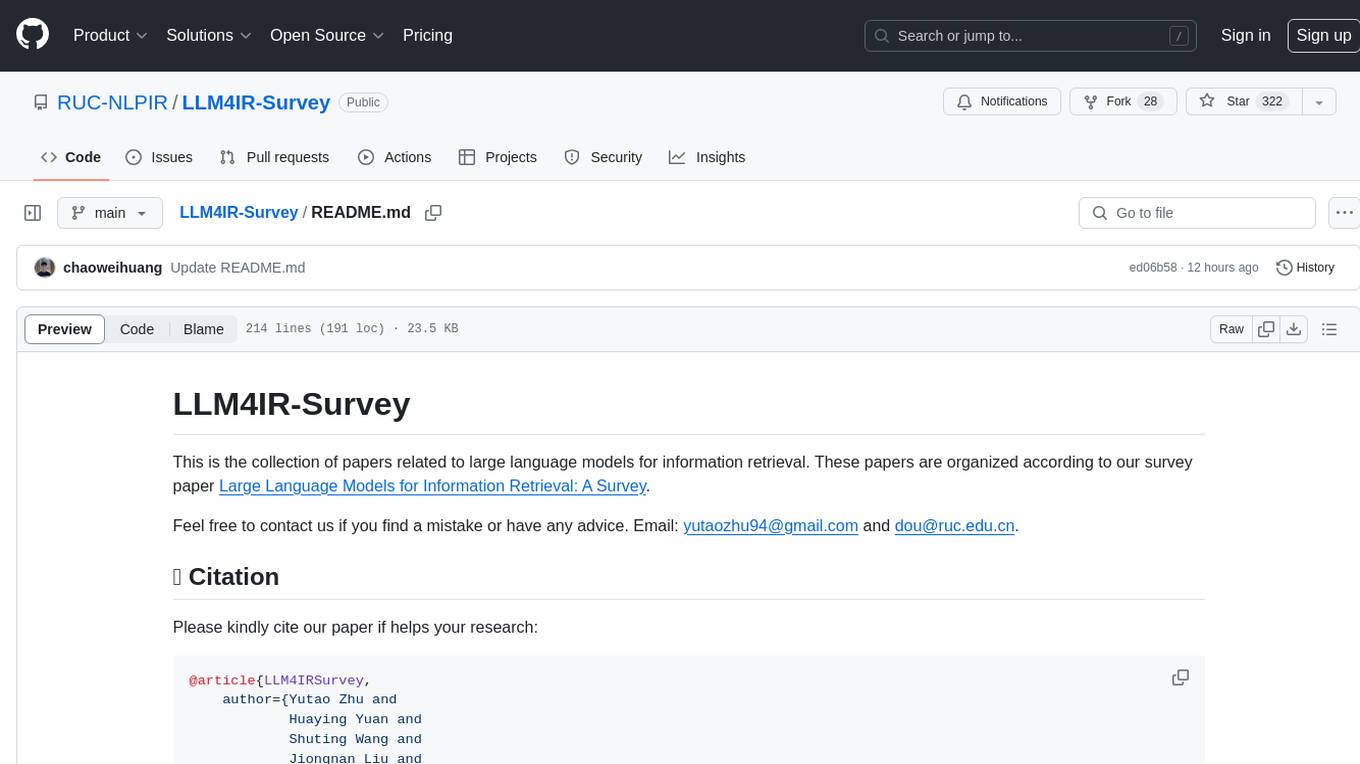
LLM4IR-Survey is a collection of papers related to large language models for information retrieval, organized according to the survey paper 'Large Language Models for Information Retrieval: A Survey'. It covers various aspects such as query rewriting, retrievers, rerankers, readers, search agents, and more, providing insights into the integration of large language models with information retrieval systems.
README:
This is the collection of papers related to large language models for information retrieval. These papers are organized according to our survey paper Large Language Models for Information Retrieval: A Survey.
Feel free to contact us if you find a mistake or have any advice. Email: [email protected] and [email protected].
Please kindly cite our paper if helps your research:
@article{LLM4IRSurvey,
author={Yutao Zhu and
Huaying Yuan and
Shuting Wang and
Jiongnan Liu and
Wenhan Liu and
Chenlong Deng and
Haonan Chen and
Zhicheng Dou and
Ji-Rong Wen},
title={Large Language Models for Information Retrieval: A Survey},
journal={CoRR},
volume={abs/2308.07107},
year={2023},
url={https://arxiv.org/abs/2308.07107},
eprinttype={arXiv},
eprint={2308.07107}
}
-
Version 3 [2024-09-03]
- Retriever: We incorporated up-to-date methods that utilize LLM to enlarge the dataset used for training retrievers or to improve the overall structure and design of retriever systems.
- Re-ranker: We have added some unsupervised rerankers, several studies focusing on training data augmentation, and discussions on the limitations of LLM rerankers.
- Reader: We added the latest studies on readers, particularly enriching the works in the active reader section.
- Search Agent: We added the latest studies on static and dynamic search agents, particularly enriching the works in benchmarking and self-planning.
-
Version 2 [2024-01-19]
- We added a new section to introduce search agents, which represent an innovative approach to integrating LLMs with IR systems.
- Rewriter: We added recent works on LLM-based query rewriting, most of which focus on conversational search.
- Retriever: We added the latest techniques that leverage LLMs to expand the training corpus for retrievers or to enhance retrievers' architectures.
- Reranker: We added recent LLM-based ranking works to each of the three part: Utilizing LLMs as Supervised Rerankers, Utilizing LLMs as Unsupervised Rerankers, and Utilizing LLMs for Training Data Augmentation.
- Reader: We added the latest studies in LLM-enhanced reader area, including a section introducing the reference compression technique, a section discussing the applications of LLM-enhanced readers, and a section analyzing the characteristics of LLM-enhanced readers.
- Future Direction: We added a section about search agents and a section discussing the bias caused by leveraging LLMs into IR systems.
- Query2doc: Query Expansion with Large Language Models, Wang et al., arXiv 2023. [Paper]
- Generative and Pseudo-Relevant Feedback for Sparse, Dense and Learned Sparse Retrieval, Mackie et al., arXiv 2023. [Paper]
- Generative Relevance Feedback with Large Language Models, Mackie et al., SIGIR 2023 (short paper). [Paper]
- GRM: Generative Relevance Modeling Using Relevance-Aware Sample Estimation for Document Retrieval, Mackie et al., arXiv 2023. [Paper]
- Large Language Models Know Your Contextual Search Intent: A Prompting Framework for Conversational Search, Mao et al., arXiv 2023. [Paper]
- Precise Zero-Shot Dense Retrieval without Relevance Labels, Gao et al., ACL 2023. [Paper]
- Query Expansion by Prompting Large Language Models, Jagerman et al., arXiv 2023. [Paper]
- Large Language Models are Strong Zero-Shot Retriever, Shen et al., arXiv 2023. [Paper]
- Enhancing Conversational Search: Large Language Model-Aided Informative Query Rewriting, Ye et al., EMNLP 2023 (Findings). [Paper]
- Can generative llms create query variants for test collections? an exploratory study, M. Alaofi et al., SIGIR 2023 (short paper). [Paper]
- Corpus-Steered Query Expansion with Large Language Models, Lei et al., EACL 2024 (Short Paper). [Paper]
- Large language model based long-tail query rewriting in taobao search, Peng et al., WWW 2024. [Paper]
- Can Query Expansion Improve Generalization of Strong Cross-Encoder Rankers?, Li et al., SIGIR 2024. [Paper]
- Query Performance Prediction using Relevance Judgments Generated by Large Language Models, Meng et al., arXiv 2024. [Paper]
- RaFe: Ranking Feedback Improves Query Rewriting for RAG, Mao et al., arXiv 2024. [Paper]
- Crafting the Path: Robust Query Rewriting for Information Retrieval, Baek et al., arXiv 2024. [Paper]
- Query Rewriting for Retrieval-Augmented Large Language Models, Ma et al., arXiv 2023. [Paper]
- QUILL: Query Intent with Large Language Models using Retrieval Augmentation and Multi-stage Distillation, Srinivasan et al., EMNLP 2022 (Industry). [Paper] (This paper explore fine-tuning methods in baseline experiments.)
- QUILL: Query Intent with Large Language Models using Retrieval Augmentation and Multi-stage Distillation, Srinivasan et al., EMNLP 2022 (Industry). [Paper]
- Knowledge Refinement via Interaction Between Search Engines and Large Language Models, Feng et al., arXiv 2023. [Paper]
- Query Rewriting for Retrieval-Augmented Large Language Models, Ma et al., arXiv 2023. [Paper]
- InPars: Data Augmentation for Information Retrieval using Large Language Models, Bonifacio et al., arXiv 2022. [Paper]
- Pre-training with Large Language Model-based Document Expansion for Dense Passage Retrieval, Ma et al., arXiv 2023. [Paper]
- InPars-v2: Large Language Models as Efficient Dataset Generators for Information Retrieval, Jeronymo et al., arXiv 2023. [Paper]
- Promptagator: Few-shot Dense Retrieval From 8 Examples, Dai et al., ICLR 2023. [Paper]
- AugTriever: Unsupervised Dense Retrieval by Scalable Data Augmentation, Meng et al., arXiv 2023. [Paper]
- UDAPDR: Unsupervised Domain Adaptation via LLM Prompting and Distillation of Rerankers, Saad-Falco et al., arXiv 2023. [Paper]
- Soft Prompt Tuning for Augmenting Dense Retrieval with Large Language Models, Peng et al., arXiv 2023. [Paper]
- CONVERSER: Few-shot Conversational Dense Retrieval with Synthetic Data Generation, Huang et al., ACL 2023. [Paper]
- Leveraging LLMs for Synthesizing Training Data Across Many Languages in Multilingual Dense Retrieval, Thakur et al., arXiv 2023. [Paper]
- Questions Are All You Need to Train a Dense Passage Retriever, Sachan et al., ACL 2023. [Paper]
- Beyond Factuality: A Comprehensive Evaluation of Large Language Models as Knowledge Generators, Chen et al., EMNLP 2023. [Paper]
- Gecko: Versatile Text Embeddings Distilled from Large Language Models, Lee et al., arXiv 2024. [Paper]
- Improving Text Embeddings with Large Language Models, Wang et al., ACL 2024. [Paper]
- Text and Code Embeddings by Contrastive Pre-Training, Neelakantan et al., arXiv 2022. [Paper]
- Fine-Tuning LLaMA for Multi-Stage Text Retrieval, Ma et al., arXiv 2023. [Paper]
- Large Dual Encoders Are Generalizable Retrievers, Ni et al., EMNLP 2022. [Paper]
- Task-aware Retrieval with Instructions, Asai et al., ACL 2023 (Findings). [Paper]
- Transformer memory as a differentiable search index, Tay et al., NeurIPS 2022. [Paper]
- Large Language Models are Built-in Autoregressive Search Engines, Ziems et al., ACL 2023 (Findings). [Paper]
- Chatretriever: Adapting large language models for generalized and robust conversational dense retrieval, Mao et al., arXiv. [Paper]
- How does generative retrieval scale to millions of passages?, Pradeep et al., ACL 2023. [Paper]
- CorpusLM: Towards a Unified Language Model on Corpus for Knowledge-Intensive Tasks, Li et al., SIGIR. [Paper]
- Multi-Stage Document Ranking with BERT, Nogueira et al., arXiv 2019. [Paper]
- Document Ranking with a Pretrained Sequence-to-Sequence Model, Nogueira et al., EMNLP 2020 (Findings). [Paper]
- Text-to-Text Multi-view Learning for Passage Re-ranking, Ju et al., SIGIR 2021 (Short Paper). [Paper]
- The Expando-Mono-Duo Design Pattern for Text Ranking with Pretrained Sequence-to-Sequence Models, Pradeep et al., arXiv 2021. [Paper]
- RankT5: Fine-Tuning T5 for Text Ranking with Ranking Losses, Zhuang et al., SIGIR 2023 (Short Paper). [Paper]
- Fine-Tuning LLaMA for Multi-Stage Text Retrieval, Ma et al., arXiv 2023. [Paper]
- A Two-Stage Adaptation of Large Language Models for Text Ranking, Zhang et al., ACL 2024 (Findings). [Paper]
- Rank-without-GPT: Building GPT-Independent Listwise Rerankers on Open-Source Large Language Models, Zhang et al., arXiv 2023. [Paper]
- ListT5: Listwise Reranking with Fusion-in-Decoder Improves Zero-shot Retrieval, Yoon et al., ACL 2024. [Paper]
- Q-PEFT: Query-dependent Parameter Efficient Fine-tuning for Text Reranking with Large Language Models, Peng et al., arXiv 2024. [Paper]
- Leveraging Passage Embeddings for Efficient Listwise Reranking with Large Language Models, Liu et al., arXiv 2024. [Paper]
- Holistic Evaluation of Language Models, Liang et al., arXiv 2022. [Paper]
- Improving Passage Retrieval with Zero-Shot Question Generation, Sachan et al., EMNLP 2022. [Paper]
- Discrete Prompt Optimization via Constrained Generation for Zero-shot Re-ranker, Cho et al., ACL 2023 (Findings). [Paper]
- Open-source Large Language Models are Strong Zero-shot Query Likelihood Models for Document Ranking, Zhuang et al., EMNLP 2023 (Findings). [Paper]
- PaRaDe: Passage Ranking using Demonstrations with Large Language Models, Drozdov et al., EMNLP 2023 (Findings). [Paper]
- Beyond Yes and No: Improving Zero-Shot LLM Rankers via Scoring Fine-Grained Relevance Labels, Zhuang et al., arXiv 2023. [Paper]
- Is ChatGPT Good at Search? Investigating Large Language Models as Re-Ranking Agent, Sun et al., EMNLP 2023. [Paper]
- Zero-Shot Listwise Document Reranking with a Large Language Model, Ma et al., arXiv 2023. [Paper]
- Found in the Middle: Permutation Self-Consistency Improves Listwise Ranking in Large Language Models, Tang et al., arXiv 2023. [Paper]
- Large Language Models are Effective Text Rankers with Pairwise Ranking Prompting, Qin et al., NAACL 2024 (Findings). [Paper]
- A Setwise Approach for Effective and Highly Efficient Zero-shot Ranking with Large Language Models, Zhuang et al., SIGIR 2024. [Paper]
- InstUPR: Instruction-based Unsupervised Passage Reranking with Large Language Models, Huang and Chen, arXiv 2024. [Paper]
- Generating Diverse Criteria On-the-Fly to Improve Point-wise LLM Rankers, Guo et al., arXiv 2024. [Paper]
- DemoRank: Selecting Effective Demonstrations for Large Language Models in Ranking Task, Liu et al., arXiv 2024. [Paper]
- An Investigation of Prompt Variations for Zero-shot LLM-based Rankers, Sun et al., arXiv 2024. [Paper]
- TourRank: Utilizing Large Language Models for Documents Ranking with a Tournament-Inspired Strategy, Chen et al., arXiv 2024. [Paper]
- Top-Down Partitioning for Efficient List-Wise Ranking, Parry et al., arXiv 2024. [Paper]
- PRP-Graph: Pairwise Ranking Prompting to LLMs with Graph Aggregation for Effective Text Re-ranking, Luo et al., ACL 2024. [Paper]
- Consolidating Ranking and Relevance Predictions of Large Language Models through Post-Processing, Yan et al., arXiv 2024. [Paper]
- ExaRanker: Explanation-Augmented Neural Ranker, Ferraretto et al., SIGIR 2023 (Short Paper). [Paper]
- InPars-Light: Cost-Effective Unsupervised Training of Efficient Rankers, Boytsov et al., arXiv 2023. [Paper]
- Generating Synthetic Documents for Cross-Encoder Re-Rankers, Askari et al., arXiv 2023. [Paper]
- Instruction Distillation Makes Large Language Models Efficient Zero-shot Rankers, Sun et al., arXiv 2023. [Paper]
- RankVicuna: Zero-Shot Listwise Document Reranking with Open-Source Large Language Models, Pradeep et al., arXiv 2023. [Paper]
- RankZephyr: Effective and Robust Zero-Shot Listwise Reranking is a Breeze!, Pradeep et al., arXiv 2023. [Paper]
- ExaRanker-Open: Synthetic Explanation for IR using Open-Source LLMs, Ferraretto et al., arXiv 2024. [Paper]
- Expand, Highlight, Generate: RL-driven Document Generation for Passage Reranking, Askari et al., EMNLP 2023. [Paper]
- FIRST: Faster Improved Listwise Reranking with Single Token Decoding, Reddy et al., arXiv 2024. [Paper]
- REALM: Retrieval-Augmented Language Model Pre-Training, Guu et al., ICML 2020. [Paper]
- Retrieval-Augmented Generation for Knowledge-Intensive NLP Tasks, Lewis et al., NeurIPS 2020. [Paper]
- REPLUG: Retrieval-Augmented Black-Box Language Models, Shi et al., arXiv 2023. [Paper]
- Atlas: Few-shot Learning with Retrieval Augmented Language Models, Izacard et al., JMLR 2023. [Paper]
- Internet-augmented Language Models through Few-shot Prompting for Open-domain Question Answering, Lazaridou et al., arXiv 2022. [Paper]
- Rethinking with Retrieval: Faithful Large Language Model Inference, He et al., arXiv 2023. [Paper]
- FreshLLMs: Refreshing Large Language Models with Search Engine Augmentation, Vu et al., arxiv 2023. [Paper]
- Enabling Large Language Models to Generate Text with Citations, Gao et al., EMNLP 2023. [Paper]
- Chain-of-Note: Enhancing Robustness in Retrieval-Augmented Language Models, Yu et al., arxiv 2023. [Paper]
- Improving Retrieval-Augmented Large Language Models via Data Importance Learning, Lyu et al., arXiv 2023. [Paper]
- Search Augmented Instruction Learning, Luo et al., EMNLP 2023 (Findings). [Paper]
- RADIT: Retrieval-Augmented Dual Instruction Tuning, Lin et al., arXiv 2023. [Paper]
- Improving Language Models by Retrieving from Trillions of Tokens, Borgeaud et al., ICML 2022. [Paper]
- In-Context Retrieval-Augmented Language Models, Ram et al., arXiv 2023. [Paper]
- Interleaving Retrieval with Chain-of-thought Reasoning for Knowledge-intensive Multi-step Questions, Trivedi et al., ACL 2023. [Paper]
- Improving Language Models via Plug-and-Play Retrieval Feedback, Yu et al., arXiv 2023. [Paper]
- Enhancing Retrieval-Augmented Large Language Models with Iterative Retrieval-Generation Synergy, Shao et al., EMNLP 2023 (Findings). [Paper]
- Retrieval-Generation Synergy Augmented Large Language Models, Feng et al., arXiv 2023. [Paper]
- Self-RAG: Learning to Retrieve, Generate, and Critique through Self-Reflection, Asai et al., arXiv 2023. [Paper]
- Active Retrieval Augmented Generation, Jiang et al., EMNLP 2023. [Paper]
- Measuring and Narrowing the Compositionality Gap in Language Models, Press et al., arXiv 2022. [Paper]
- DEMONSTRATE–SEARCH–PREDICT: Composing Retrieval and Language Models for Knowledge-intensive NLP, Khattab et al., arXiv 2022. [Paper]
- Answering Questions by Meta-Reasoning over Multiple Chains of Thought, Yoran et al., arXiv 2023. [Paper]
- PlanRAG: A Plan-then-Retrieval Augmented Generation for Generative Large Language Models as Decision Makers, Lee ei al., arXiv 2024. [Paper]
- Learning to Plan for Retrieval-Augmented Large Language Models from Knowledge Graphs, Wang et al., arXiv 2024. [Paper]
- LeanContext: Cost-Efficient Domain-Specific Question Answering Using LLMs, Arefeen et al., arXiv 2023. [Paper]
- RECOMP: Improving Retrieval-Augmented LMs with Compression and Selective Augmentation, Xu et al., arXiv 2023. [Paper]
- TCRA-LLM: Token Compression Retrieval Augmented Large Language Model for Inference Cost Reduction, Liu et al., EMNLP 2023 (Findings). [Paper]
- Learning to Filter Context for Retrieval-Augmented Generation, Wang et al., arXiv 2023. [Paper]
- Lost in the Middle: How Language Models Use Long Contexts, Liu et al., arXiv 2023. [Paper]
- Investigating the Factual Knowledge Boundary of Large Language Models with Retrieval Augmentation, Ren et al., arXiv 2023. [Paper]
- Exploring the Integration Strategies of Retriever and Large Language Models, Liu et al., arXiv 2023. [Paper]
- Characterizing Attribution and Fluency Tradeoffs for Retrieval-Augmented Large Language Models, Aksitov et al., arXiv 2023. [Paper]
- When Not to Trust Language Models: Investigating Effectiveness of Parametric and Non-Parametric Memories, Mallen et al., ACL 2023. [Paper]
- Augmenting Black-box LLMs with Medical Textbooks for Clinical Question Answering, Wang et al., arXiv 2023. [Paper]
- ATLANTIC: Structure-Aware Retrieval-Augmented Language Model for Interdisciplinary Science, Munikoti et al., arXiv 2023. [Paper]
- Crosslingual Retrieval Augmented In-context Learning for Bangla, Li et al., arXiv 2023. [Paper]
- Clinfo.ai: An Open-Source Retrieval-Augmented Large Language Model System for Answering Medical Questions using Scientific Literature, Lozano et al., arXiv 2023. [Paper]
- Enhancing Financial Sentiment Analysis via Retrieval Augmented Large Language Models, Zhang et al., ICAIF 2023. [Paper]
- Interpretable Long-Form Legal Question Answering with Retrieval-Augmented Large Language Models, Louis et al., arXiv 2023. [Paper]
- RETA-LLM: A Retrieval-Augmented Large Language Model Toolkit, Liu et al., arXiv 2023. [Paper]
- Chameleon: a Heterogeneous and Disaggregated Accelerator System for Retrieval-Augmented Language Models, Jiang et al., arXiv 2023. [Paper]
- RaLLe: A Framework for Developing and Evaluating Retrieval-Augmented Large Language Models, Hoshi et al., EMNLP 2023. [Paper]
- Don't forget private retrieval: distributed private similarity search for large language models, Zyskind et al., arXiv 2023. [Paper]
- LaMDA: Language Models for Dialog Applications, Thoppilan et al., arXiv 2022. [Paper]
- Language Models that Seek for Knowledge: Modular Search & Generation for Dialogue and Prompt Completion, Shuster et al., EMNLP 2022 (Findings). [Paper]
- Teaching language models to support answers with verified quotes, Menick et al., arXiv 2022. [Paper]
- WebGLM: Towards An Efficient Web-Enhanced Question Answering System with Human Preferences, Liu et al., KDD 2023. [Paper]
- A Real-World WebAgent with Planning, Long Context Understanding, and Program Synthesis, Gur et al., arXiv 2023. [Paper]
- Know Where to Go: Make LLM a Relevant, Responsible, and Trustworthy Searcher, Shi et al., arXiv 2023. [Paper]
- CoSearchAgent: A Lightweight Collaborative Search Agent with Large Language Models, Gong et al., SIGIR 2024. [Paper]
- TRAD: Enhancing LLM Agents with Step-Wise Thought Retrieval and Aligned Decision, Zhou et al., SIGIR 2024. [Paper]
- WebGPT: Browser-assisted question-answering with human feedback, Nakano et al., arXiv 2021. [Paper]
- WebShop: Towards Scalable Real-World Web Interaction with Grounded Language Agents, Yao et al., arXiv 2022. [Paper]
- WebCPM: Interactive Web Search for Chinese Long-form Question Answering, Qin et al., ACL 2023. [Paper]
- Mind2Web: Towards a Generalist Agent for the Web, Deng et al., arXiv 2023. [Paper]
- WebArena: A Realistic Web Environment for Building Autonomous Agents, Zhou et al., arXiv 2023. [Paper]
- Hierarchical Prompting Assists Large Language Model on Web Navigation, Sridhar et al., EMNLP 2023 (Findings). [Paper]
- KwaiAgents: Generalized Information-seeking Agent System with Large Language Models, Pan et al., arXiv 2023. [Paper]
- WebVoyager : Building an End-to-End Web Agent with Large Multimodal Models, He et al., arXiv 2024. [Paper]
- AutoWebGLM: Bootstrap And Reinforce A Large Language Model-based Web Navigating Agent, Lai et al., KDD 2024. [Paper]
- WebCanvas: Benchmarking Web Agents in Online Environments, Pan et al., arXiv 2024. [Paper]
- Internet of Agents: Weaving a Web of Heterogeneous Agents for Collaborative Intelligence, Chen et al., arXiv 2024. [Paper]
- Agent-E: From Autonomous Web Navigation to Foundational Design Principles in Agentic Systems, Abuelsaad et al., arXiv 2024. [Paper]
- MindSearch: Mimicking Human Minds Elicits Deep AI Searcher, Chen et al., arXiv 2024. [Paper]
For Tasks:
Click tags to check more tools for each tasksFor Jobs:
Alternative AI tools for LLM4IR-Survey
Similar Open Source Tools
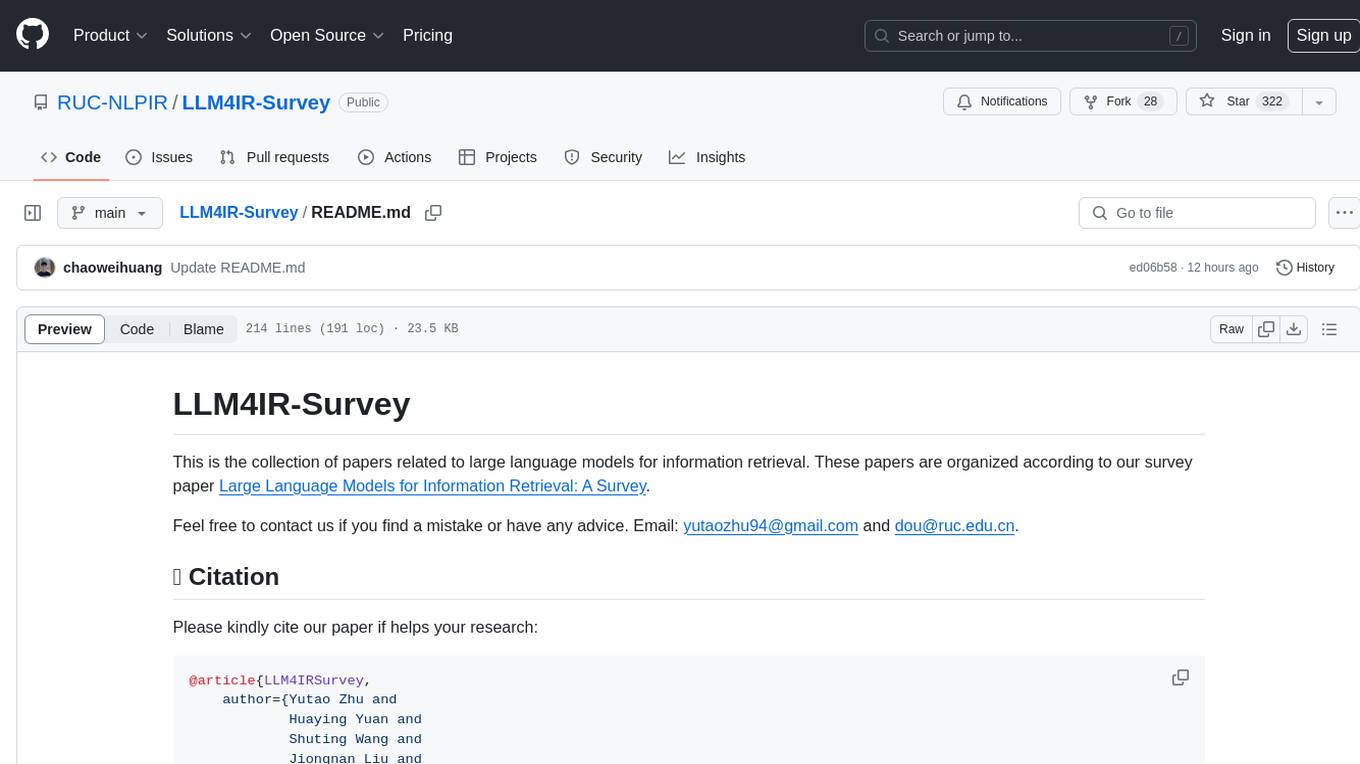
LLM4IR-Survey
LLM4IR-Survey is a collection of papers related to large language models for information retrieval, organized according to the survey paper 'Large Language Models for Information Retrieval: A Survey'. It covers various aspects such as query rewriting, retrievers, rerankers, readers, search agents, and more, providing insights into the integration of large language models with information retrieval systems.
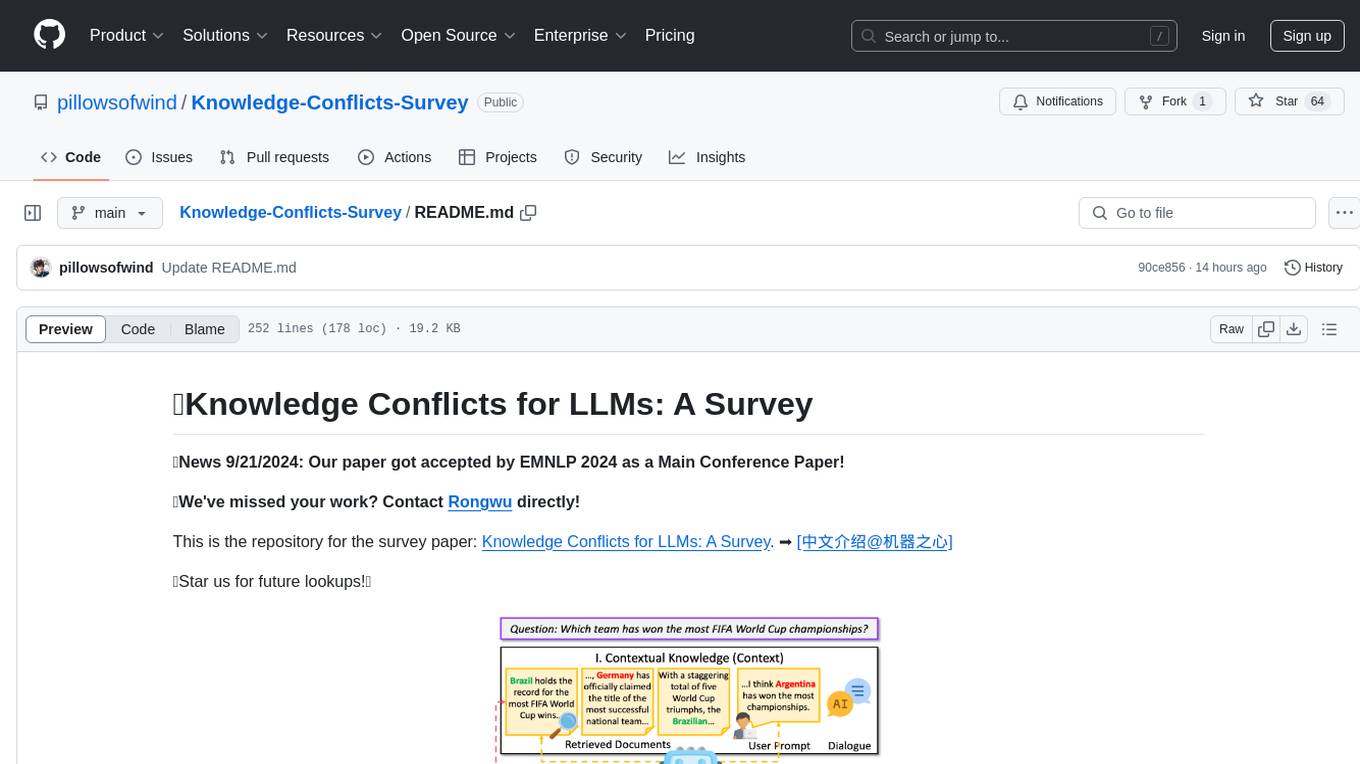
Knowledge-Conflicts-Survey
Knowledge Conflicts for LLMs: A Survey is a repository containing a survey paper that investigates three types of knowledge conflicts: context-memory conflict, inter-context conflict, and intra-memory conflict within Large Language Models (LLMs). The survey reviews the causes, behaviors, and possible solutions to these conflicts, providing a comprehensive analysis of the literature in this area. The repository includes detailed information on the types of conflicts, their causes, behavior analysis, and mitigating solutions, offering insights into how conflicting knowledge affects LLMs and how to address these conflicts.
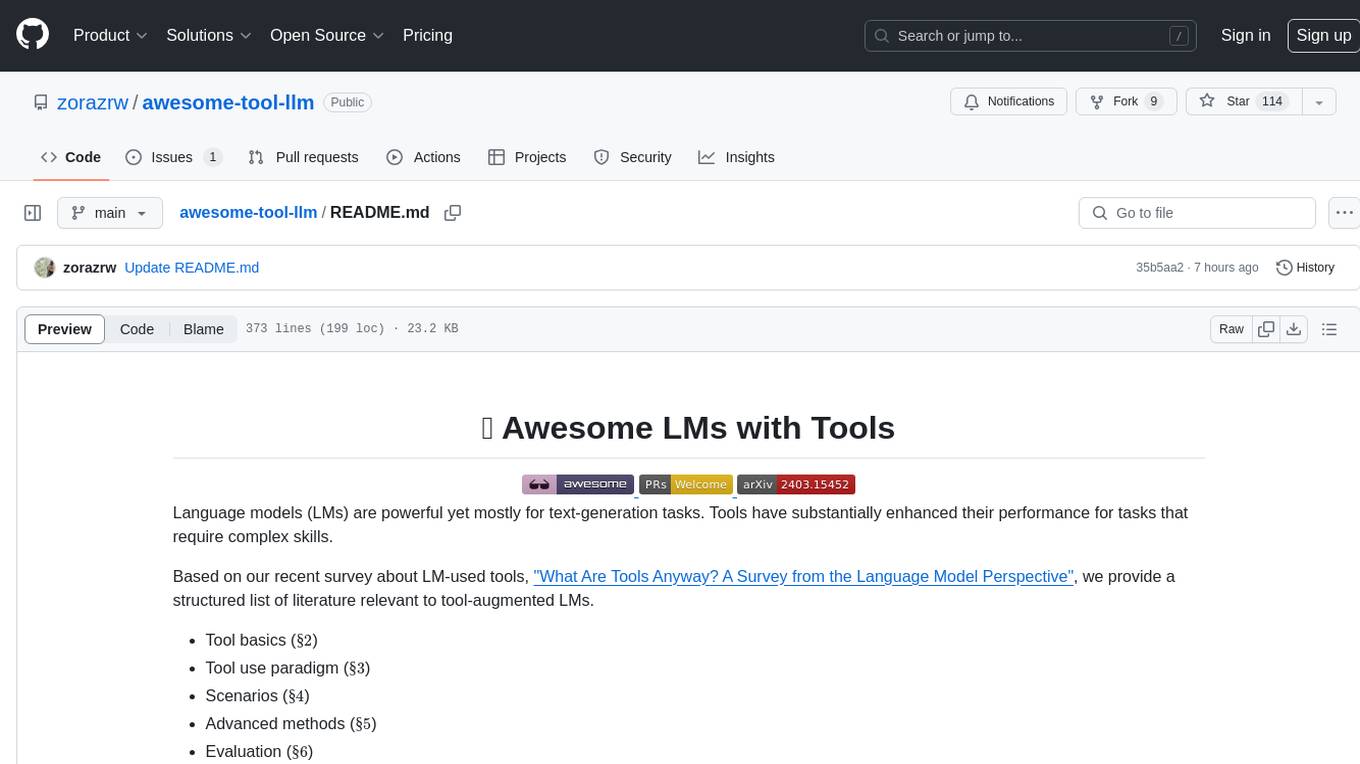
awesome-tool-llm
This repository focuses on exploring tools that enhance the performance of language models for various tasks. It provides a structured list of literature relevant to tool-augmented language models, covering topics such as tool basics, tool use paradigm, scenarios, advanced methods, and evaluation. The repository includes papers, preprints, and books that discuss the use of tools in conjunction with language models for tasks like reasoning, question answering, mathematical calculations, accessing knowledge, interacting with the world, and handling non-textual modalities.
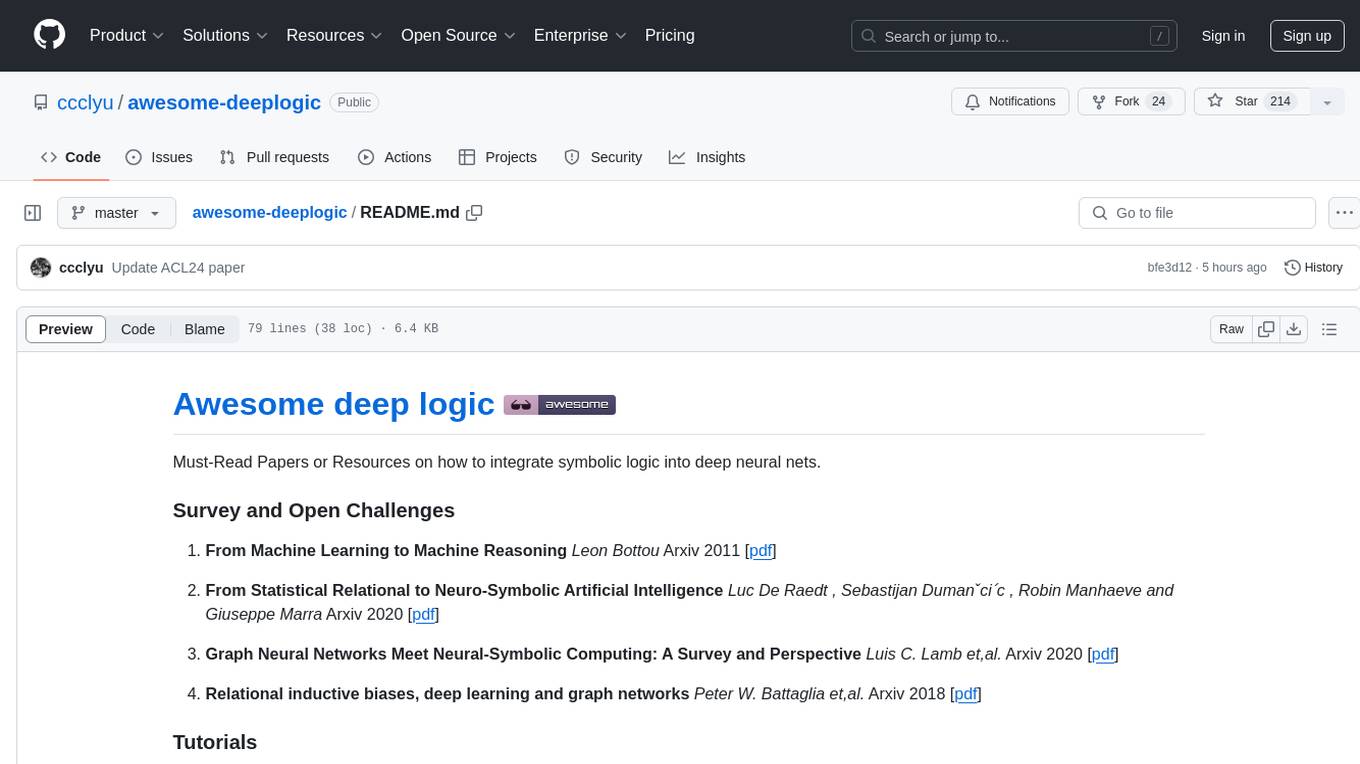
awesome-deeplogic
Awesome deep logic is a curated list of papers and resources focusing on integrating symbolic logic into deep neural networks. It includes surveys, tutorials, and research papers that explore the intersection of logic and deep learning. The repository aims to provide valuable insights and knowledge on how logic can be used to enhance reasoning, knowledge regularization, weak supervision, and explainability in neural networks.
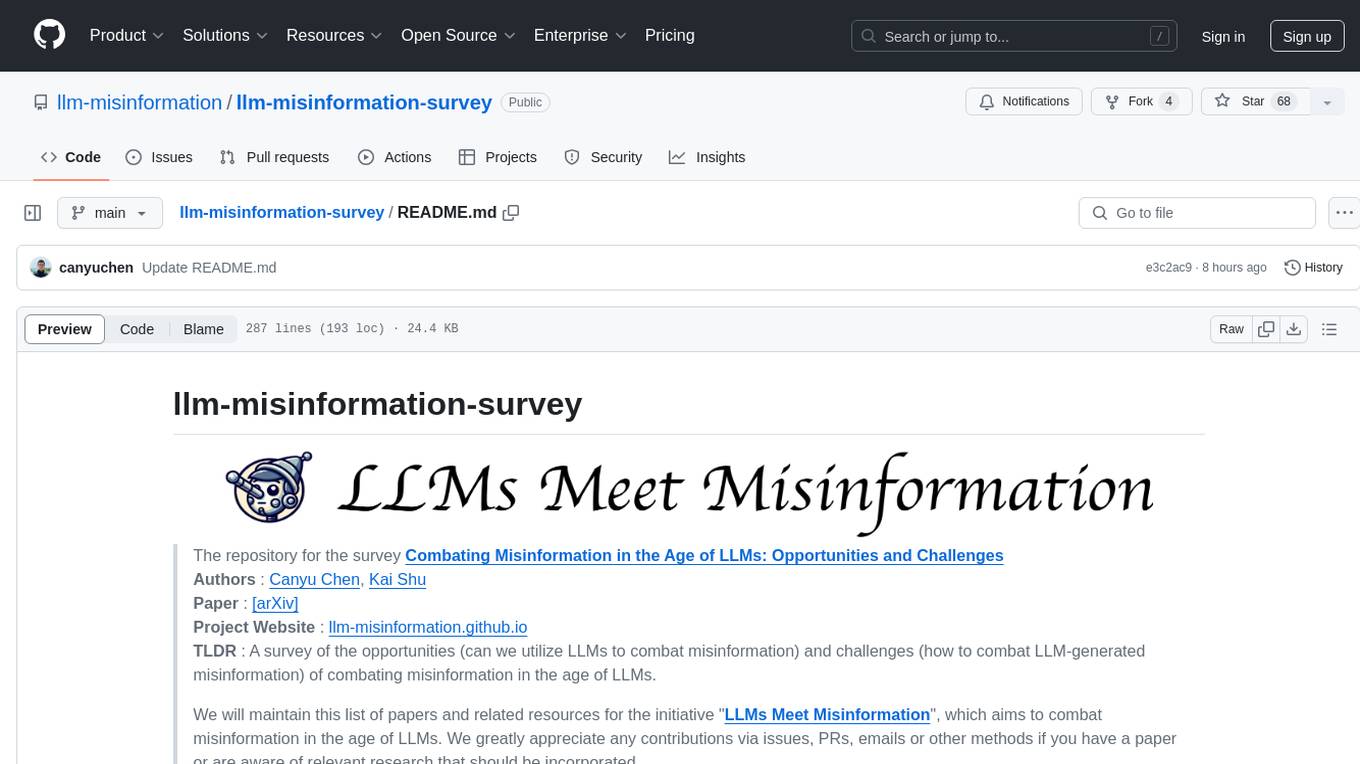
llm-misinformation-survey
The 'llm-misinformation-survey' repository is dedicated to the survey on combating misinformation in the age of Large Language Models (LLMs). It explores the opportunities and challenges of utilizing LLMs to combat misinformation, providing insights into the history of combating misinformation, current efforts, and future outlook. The repository serves as a resource hub for the initiative 'LLMs Meet Misinformation' and welcomes contributions of relevant research papers and resources. The goal is to facilitate interdisciplinary efforts in combating LLM-generated misinformation and promoting the responsible use of LLMs in fighting misinformation.
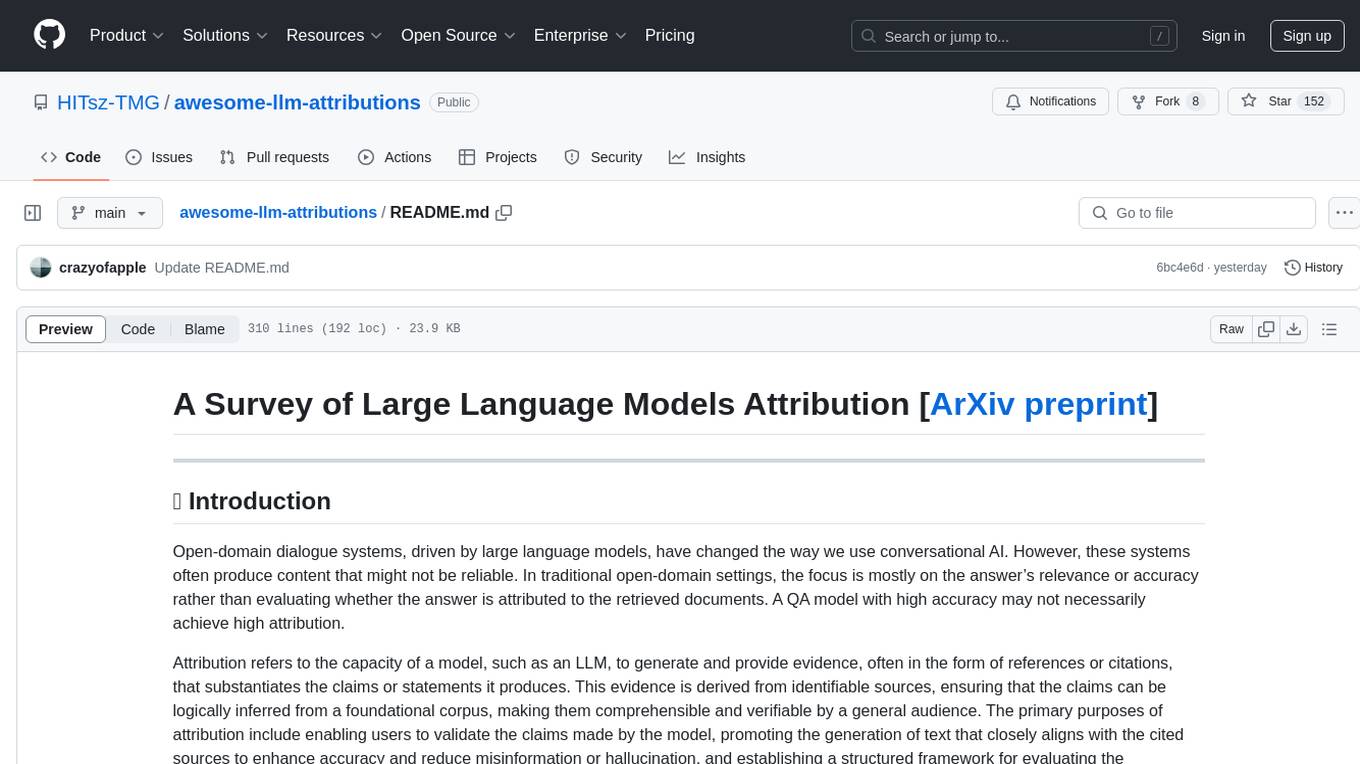
awesome-llm-attributions
This repository focuses on unraveling the sources that large language models tap into for attribution or citation. It delves into the origins of facts, their utilization by the models, the efficacy of attribution methodologies, and challenges tied to ambiguous knowledge reservoirs, biases, and pitfalls of excessive attribution.
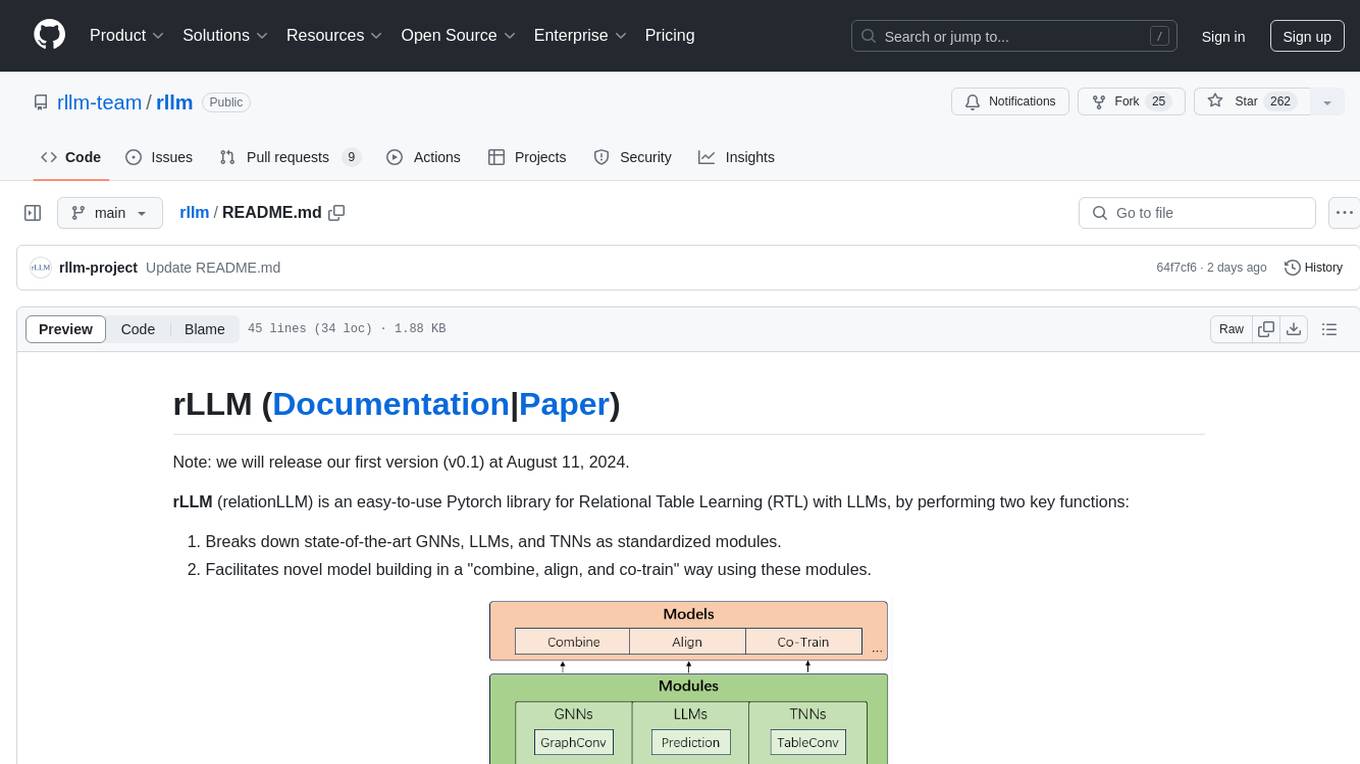
rllm
rLLM (relationLLM) is a Pytorch library for Relational Table Learning (RTL) with LLMs. It breaks down state-of-the-art GNNs, LLMs, and TNNs as standardized modules and facilitates novel model building in a 'combine, align, and co-train' way using these modules. The library is LLM-friendly, processes various graphs as multiple tables linked by foreign keys, introduces new relational table datasets, and is supported by students and teachers from Shanghai Jiao Tong University and Tsinghua University.
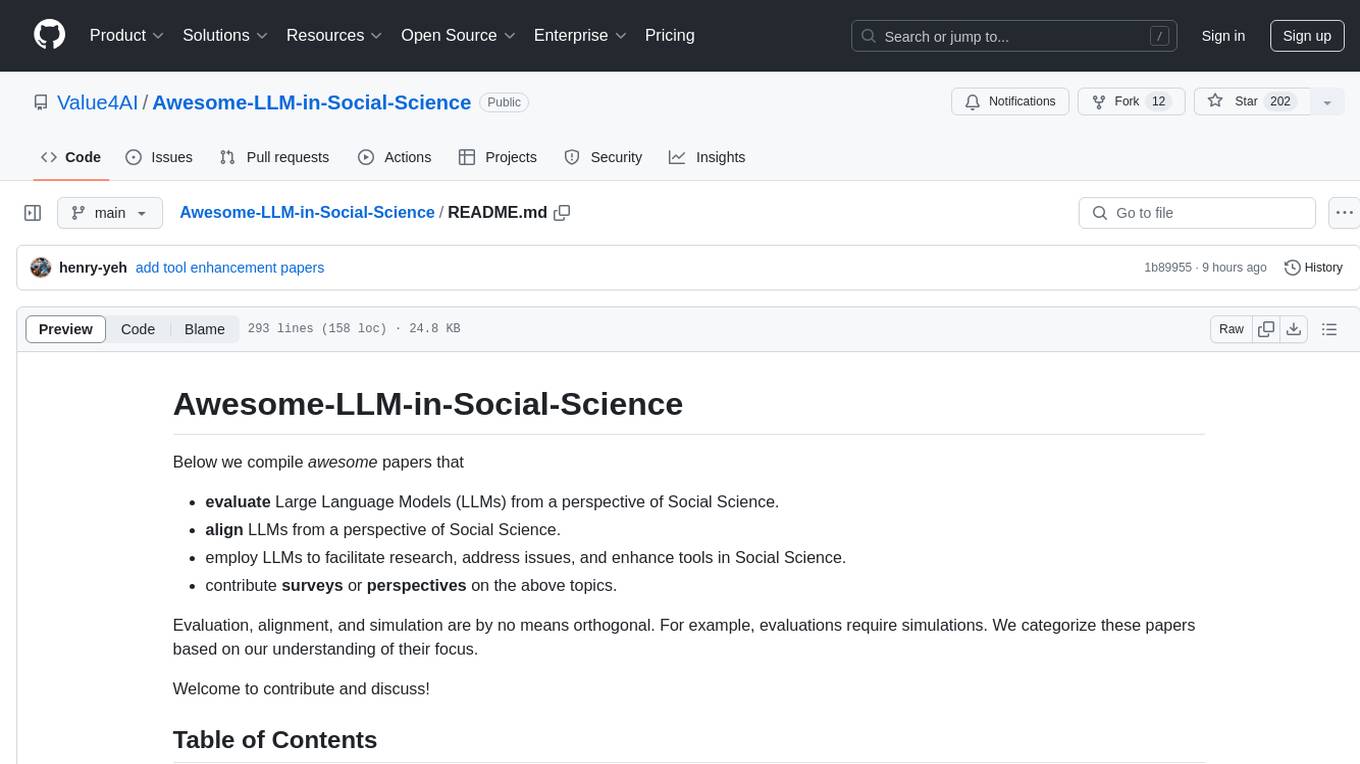
Awesome-LLM-in-Social-Science
Awesome-LLM-in-Social-Science is a repository that compiles papers evaluating Large Language Models (LLMs) from a social science perspective. It includes papers on evaluating, aligning, and simulating LLMs, as well as enhancing tools in social science research. The repository categorizes papers based on their focus on attitudes, opinions, values, personality, morality, and more. It aims to contribute to discussions on the potential and challenges of using LLMs in social science research.
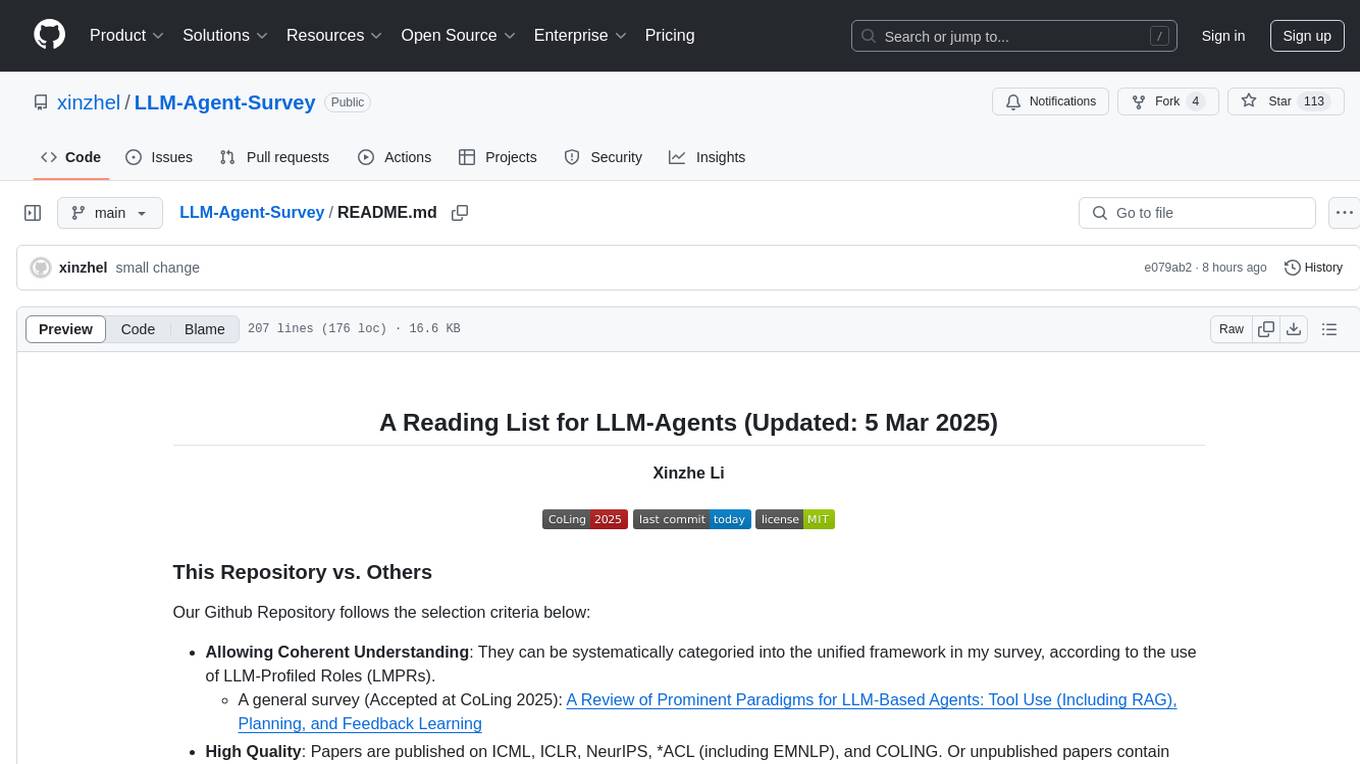
LLM-Agent-Survey
LLM-Agent-Survey is a comprehensive repository that provides a curated list of papers related to Large Language Model (LLM) agents. The repository categorizes papers based on LLM-Profiled Roles and includes high-quality publications from prestigious conferences and journals. It aims to offer a systematic understanding of LLM-based agents, covering topics such as tool use, planning, and feedback learning. The repository also includes unpublished papers with insightful analysis and novelty, marked for future updates. Users can explore a wide range of surveys, tool use cases, planning workflows, and benchmarks related to LLM agents.
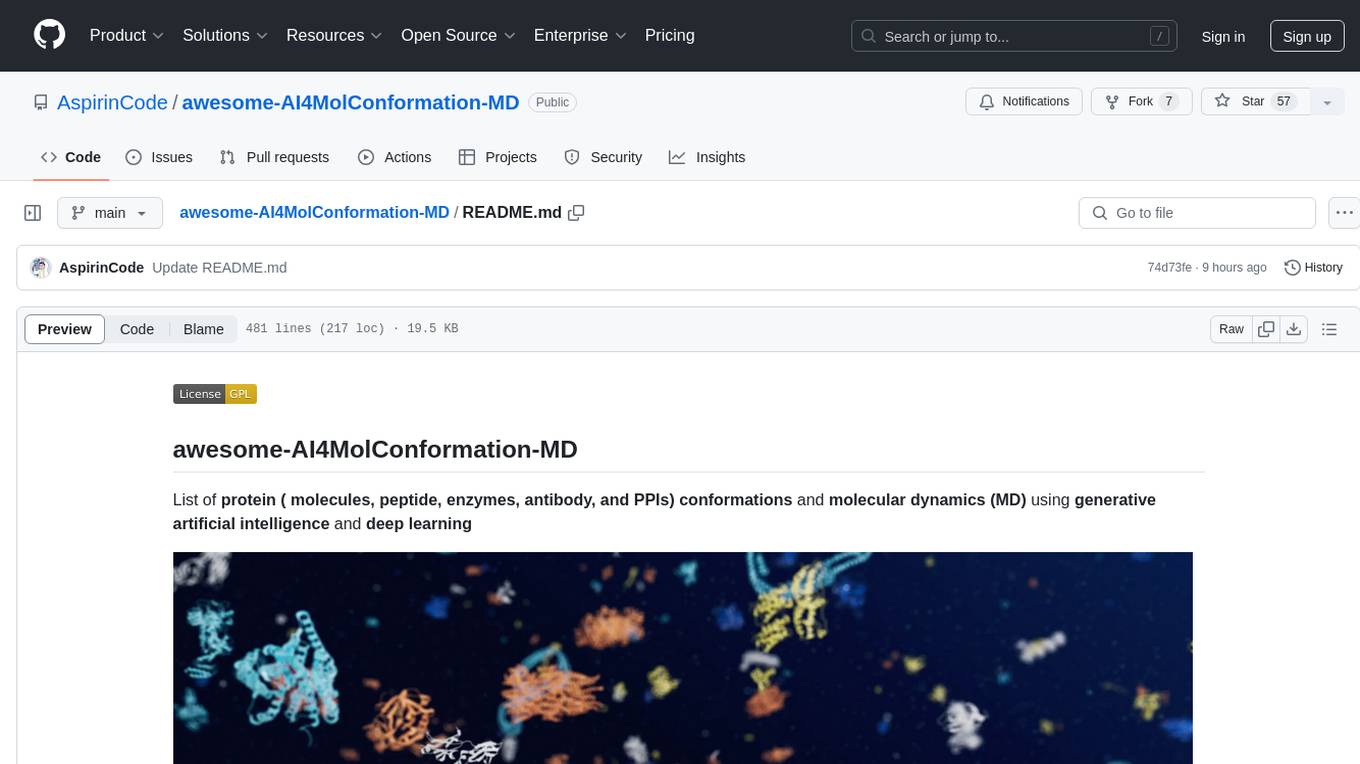
awesome-AI4MolConformation-MD
The 'awesome-AI4MolConformation-MD' repository focuses on protein conformations and molecular dynamics using generative artificial intelligence and deep learning. It provides resources, reviews, datasets, packages, and tools related to AI-driven molecular dynamics simulations. The repository covers a wide range of topics such as neural networks potentials, force fields, AI engines/frameworks, trajectory analysis, visualization tools, and various AI-based models for protein conformational sampling. It serves as a comprehensive guide for researchers and practitioners interested in leveraging AI for studying molecular structures and dynamics.
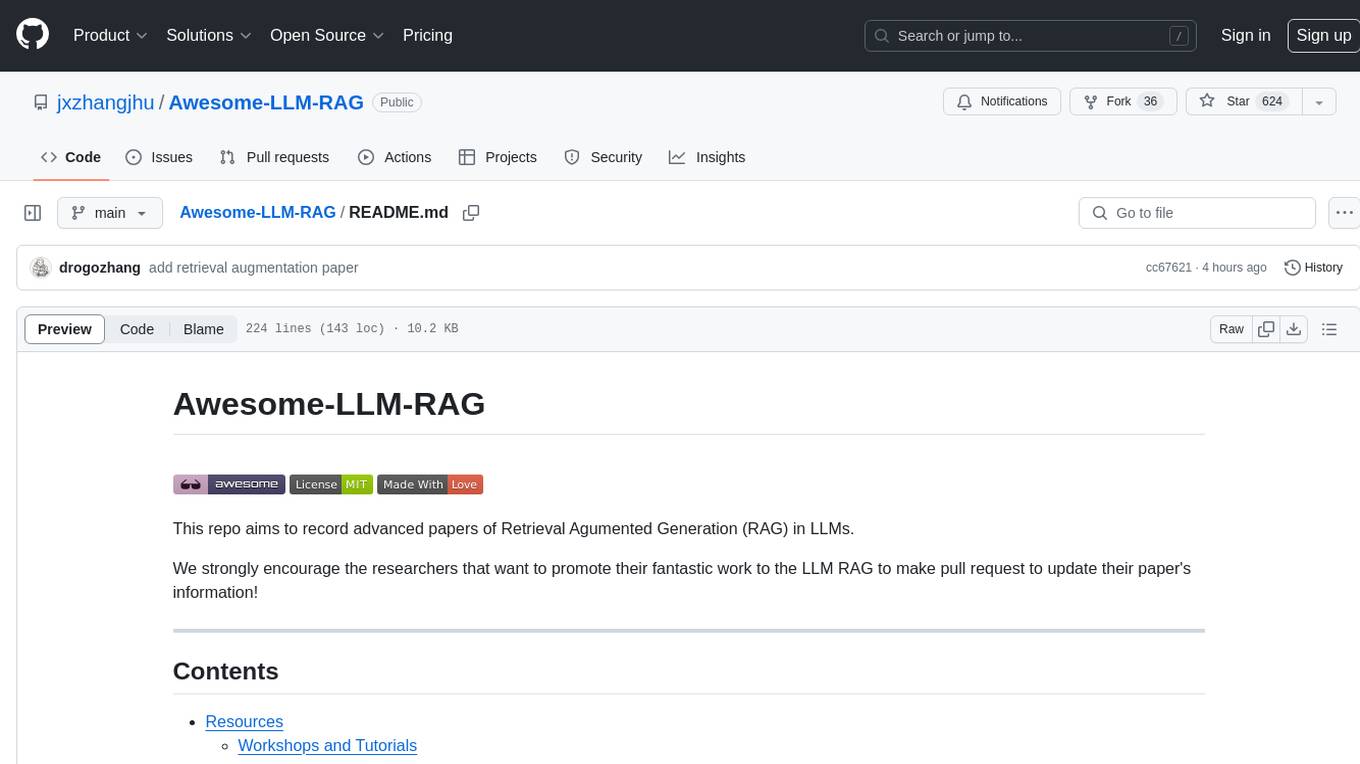
Awesome-LLM-RAG
This repository, Awesome-LLM-RAG, aims to record advanced papers on Retrieval Augmented Generation (RAG) in Large Language Models (LLMs). It serves as a resource hub for researchers interested in promoting their work related to LLM RAG by updating paper information through pull requests. The repository covers various topics such as workshops, tutorials, papers, surveys, benchmarks, retrieval-enhanced LLMs, RAG instruction tuning, RAG in-context learning, RAG embeddings, RAG simulators, RAG search, RAG long-text and memory, RAG evaluation, RAG optimization, and RAG applications.
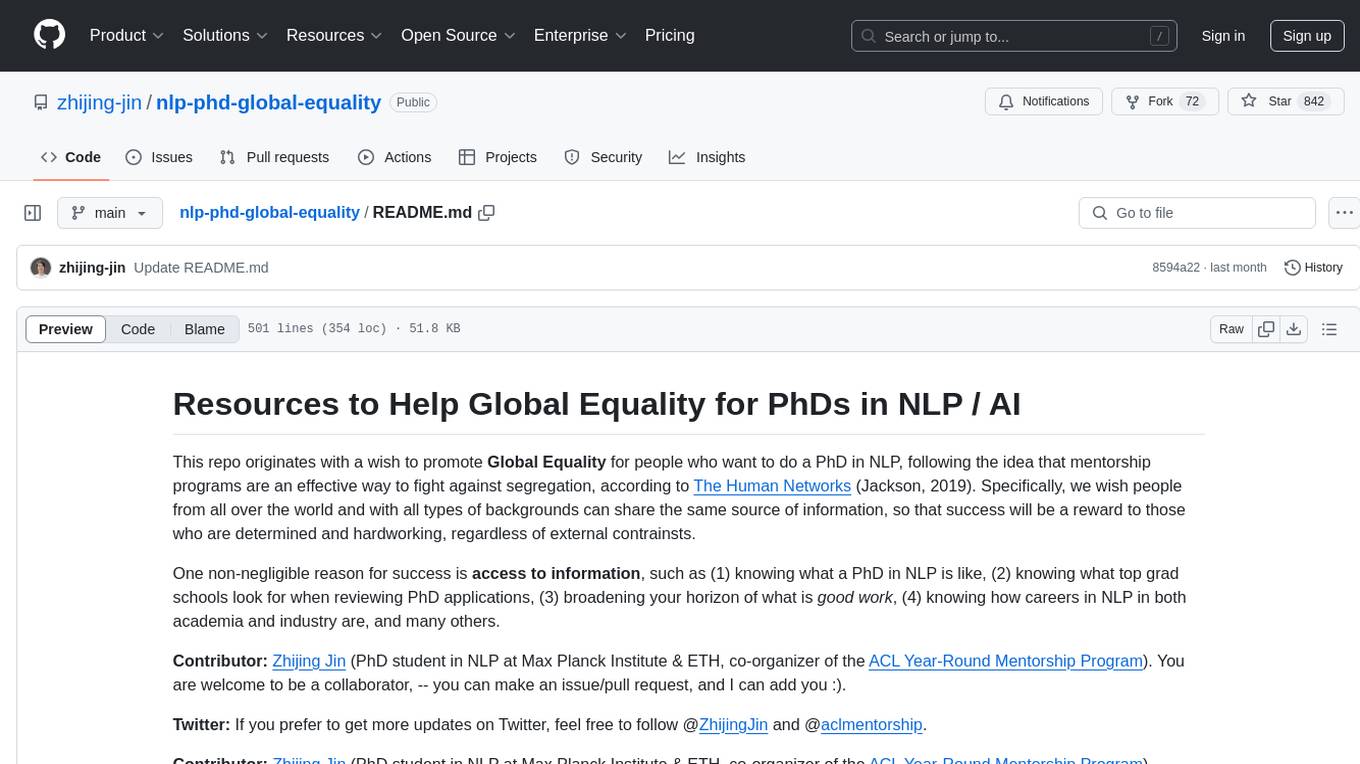
nlp-phd-global-equality
This repository aims to promote global equality for individuals pursuing a PhD in NLP by providing resources and information on various aspects of the academic journey. It covers topics such as applying for a PhD, getting research opportunities, preparing for the job market, and succeeding in academia. The repository is actively updated and includes contributions from experts in the field.
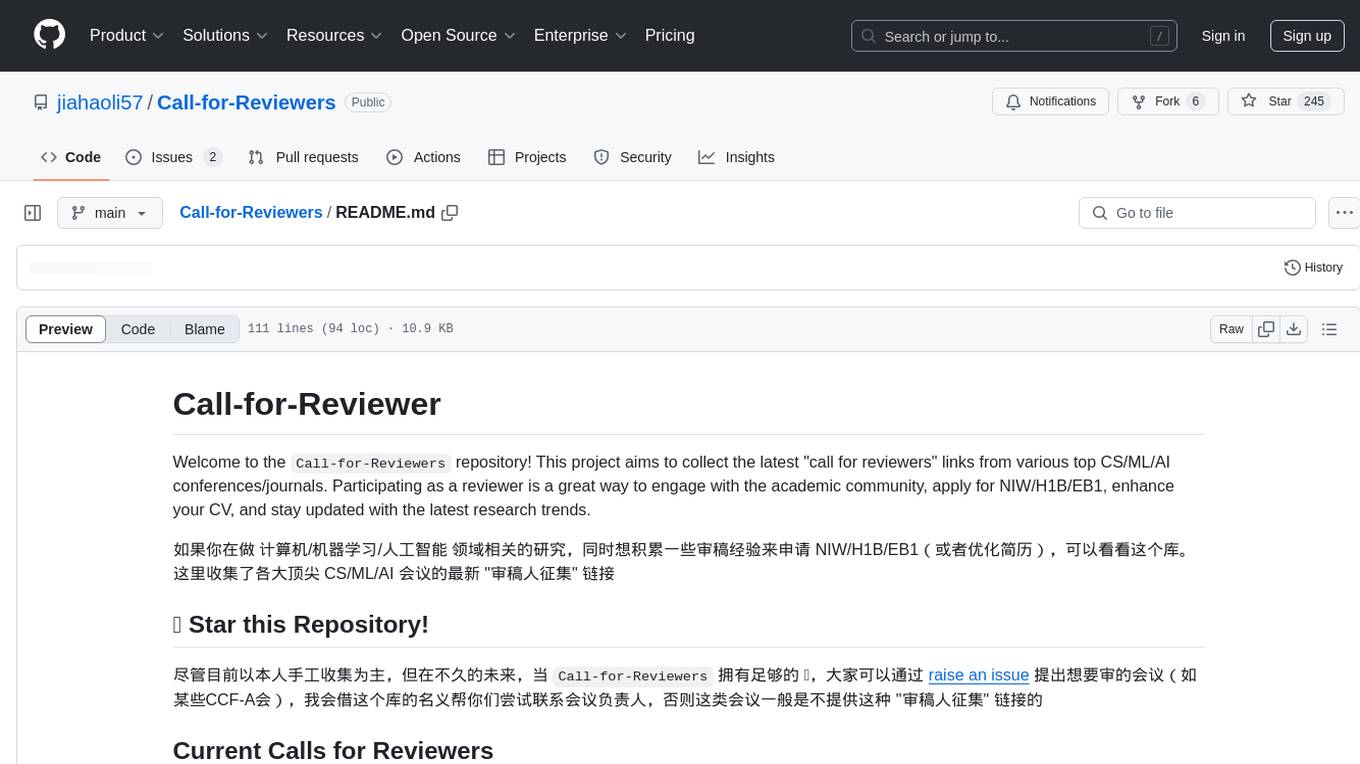
Call-for-Reviewers
The `Call-for-Reviewers` repository aims to collect the latest 'call for reviewers' links from various top CS/ML/AI conferences/journals. It provides an opportunity for individuals in the computer/ machine learning/ artificial intelligence fields to gain review experience for applying for NIW/H1B/EB1 or enhancing their CV. The repository helps users stay updated with the latest research trends and engage with the academic community.
For similar tasks
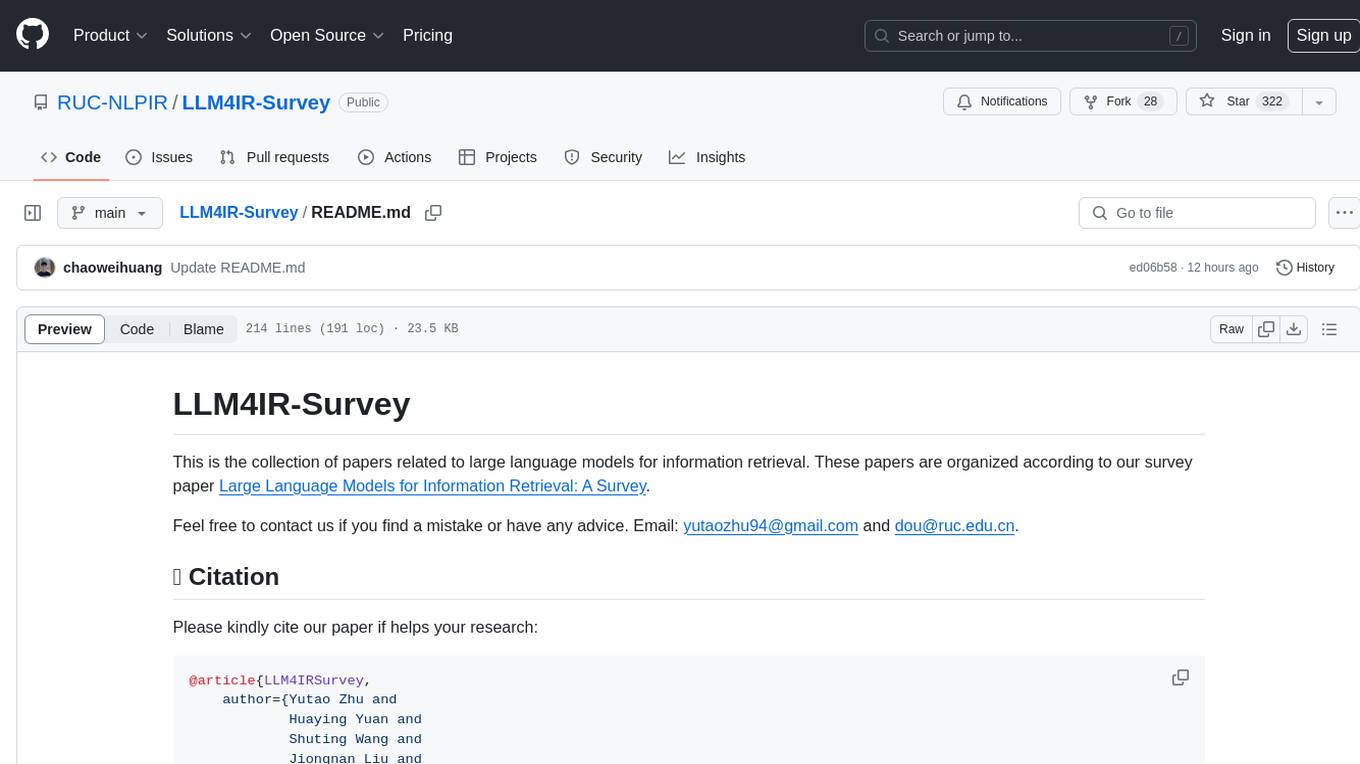
LLM4IR-Survey
LLM4IR-Survey is a collection of papers related to large language models for information retrieval, organized according to the survey paper 'Large Language Models for Information Retrieval: A Survey'. It covers various aspects such as query rewriting, retrievers, rerankers, readers, search agents, and more, providing insights into the integration of large language models with information retrieval systems.
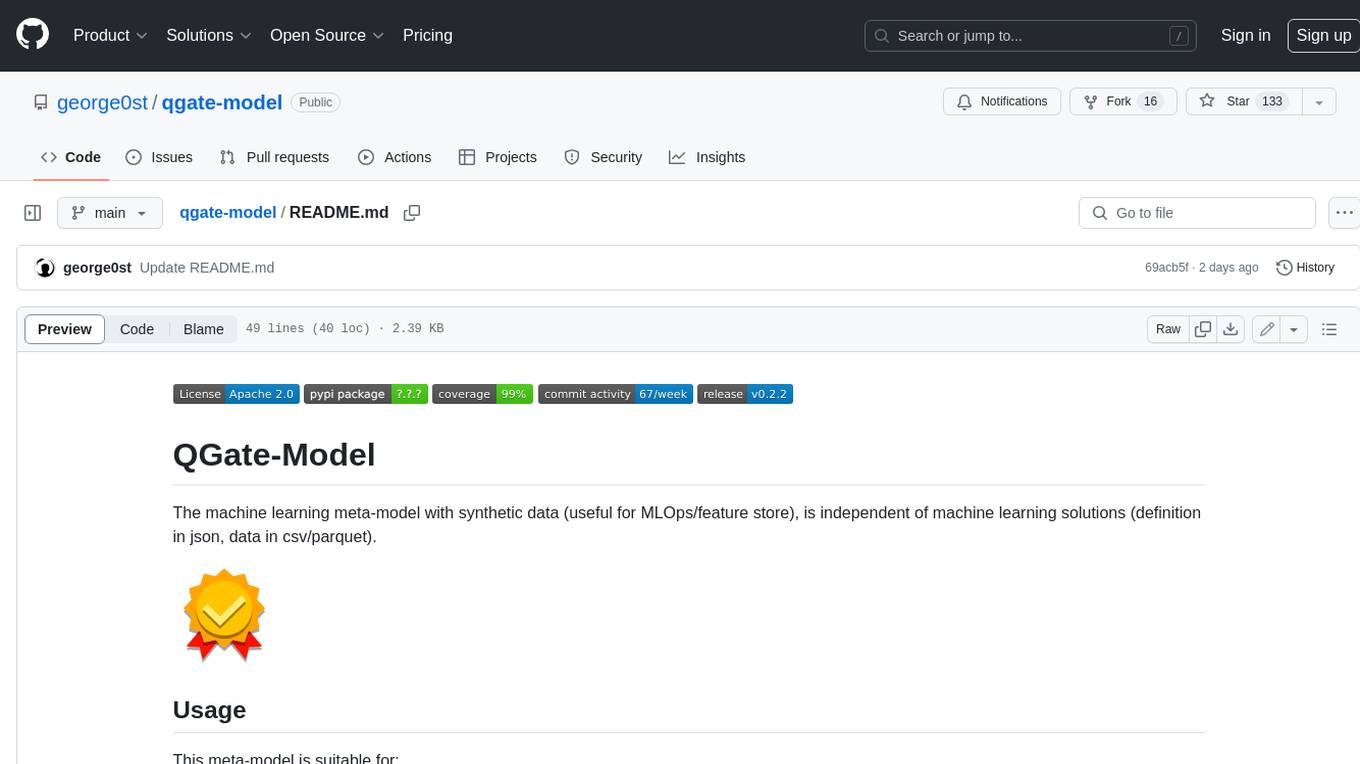
qgate-model
QGate-Model is a machine learning meta-model with synthetic data, designed for MLOps and feature store. It is independent of machine learning solutions, with definitions in JSON and data in CSV/parquet formats. This meta-model is useful for comparing capabilities and functions of machine learning solutions, independently testing new versions of machine learning solutions, and conducting various types of tests (unit, sanity, smoke, system, regression, function, acceptance, performance, shadow, etc.). It can also be used for external test coverage when internal test coverage is not available or weak.
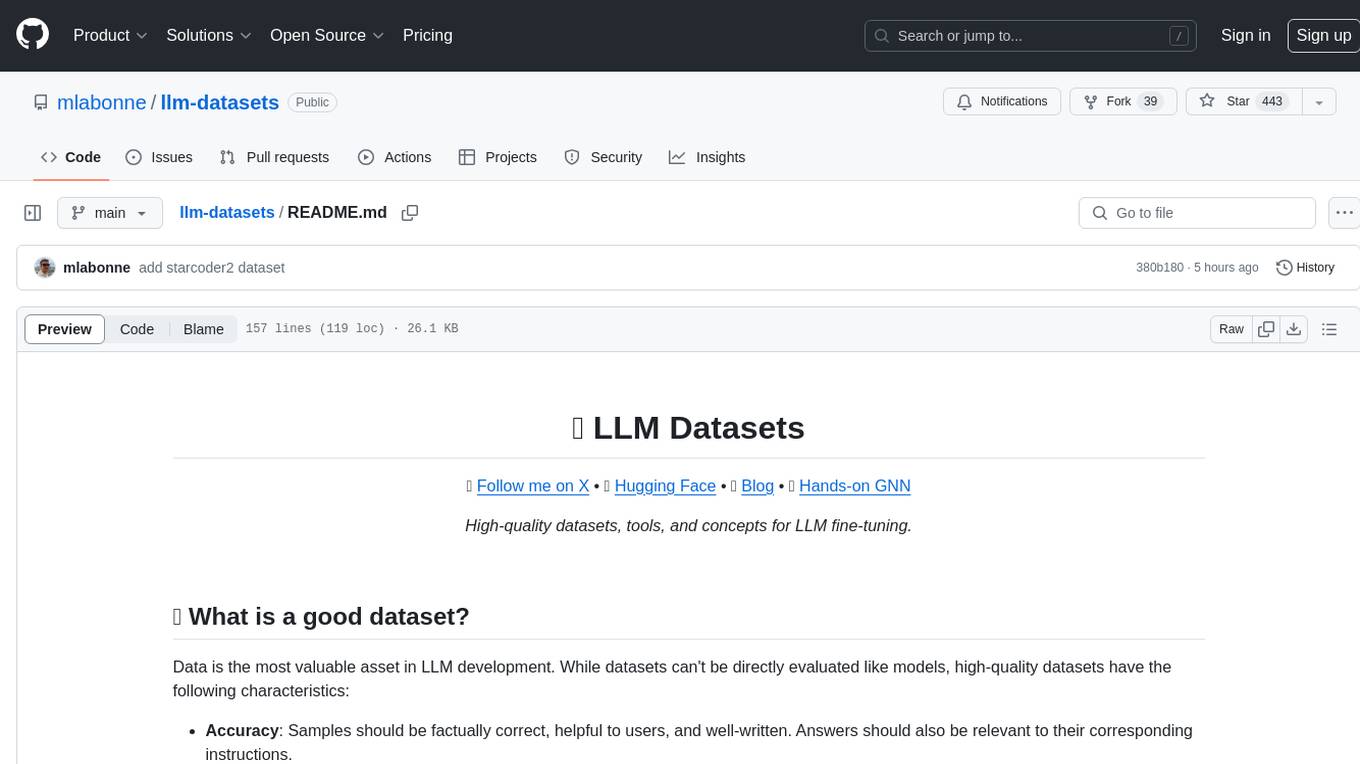
llm-datasets
LLM Datasets is a repository containing high-quality datasets, tools, and concepts for LLM fine-tuning. It provides datasets with characteristics like accuracy, diversity, and complexity to train large language models for various tasks. The repository includes datasets for general-purpose, math & logic, code, conversation & role-play, and agent & function calling domains. It also offers guidance on creating high-quality datasets through data deduplication, data quality assessment, data exploration, and data generation techniques.
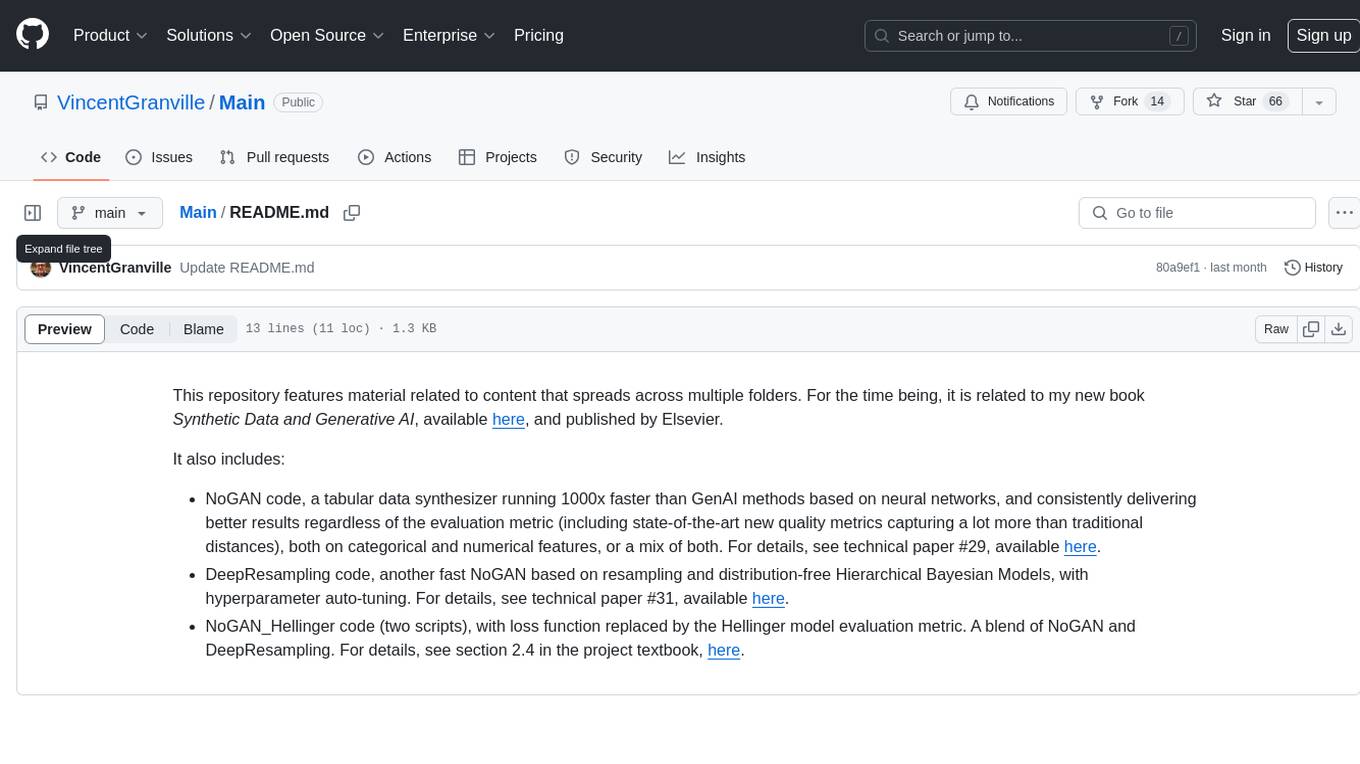
Main
This repository contains material related to the new book _Synthetic Data and Generative AI_ by the author, including code for NoGAN, DeepResampling, and NoGAN_Hellinger. NoGAN is a tabular data synthesizer that outperforms GenAI methods in terms of speed and results, utilizing state-of-the-art quality metrics. DeepResampling is a fast NoGAN based on resampling and Bayesian Models with hyperparameter auto-tuning. NoGAN_Hellinger combines NoGAN and DeepResampling with the Hellinger model evaluation metric.
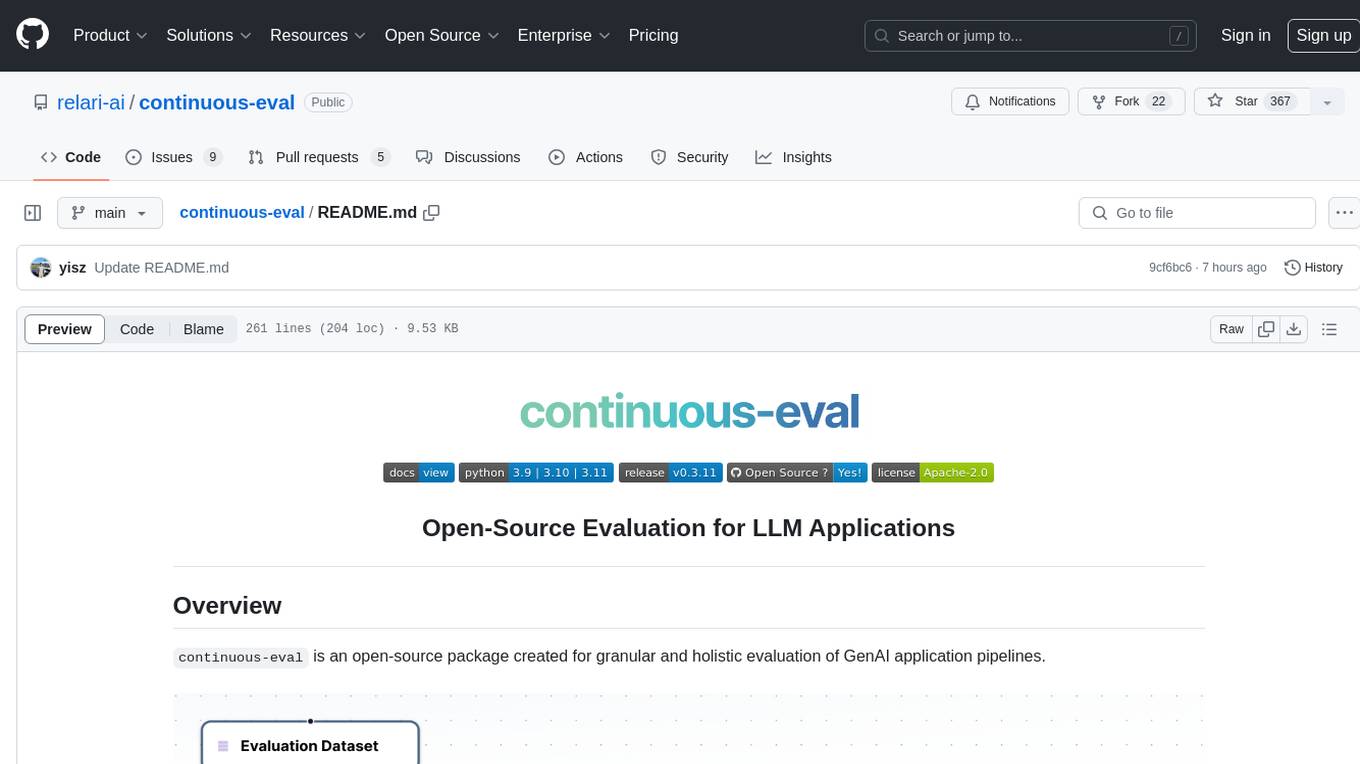
continuous-eval
Open-Source Evaluation for LLM Applications. `continuous-eval` is an open-source package created for granular and holistic evaluation of GenAI application pipelines. It offers modularized evaluation, a comprehensive metric library covering various LLM use cases, the ability to leverage user feedback in evaluation, and synthetic dataset generation for testing pipelines. Users can define their own metrics by extending the Metric class. The tool allows running evaluation on a pipeline defined with modules and corresponding metrics. Additionally, it provides synthetic data generation capabilities to create user interaction data for evaluation or training purposes.
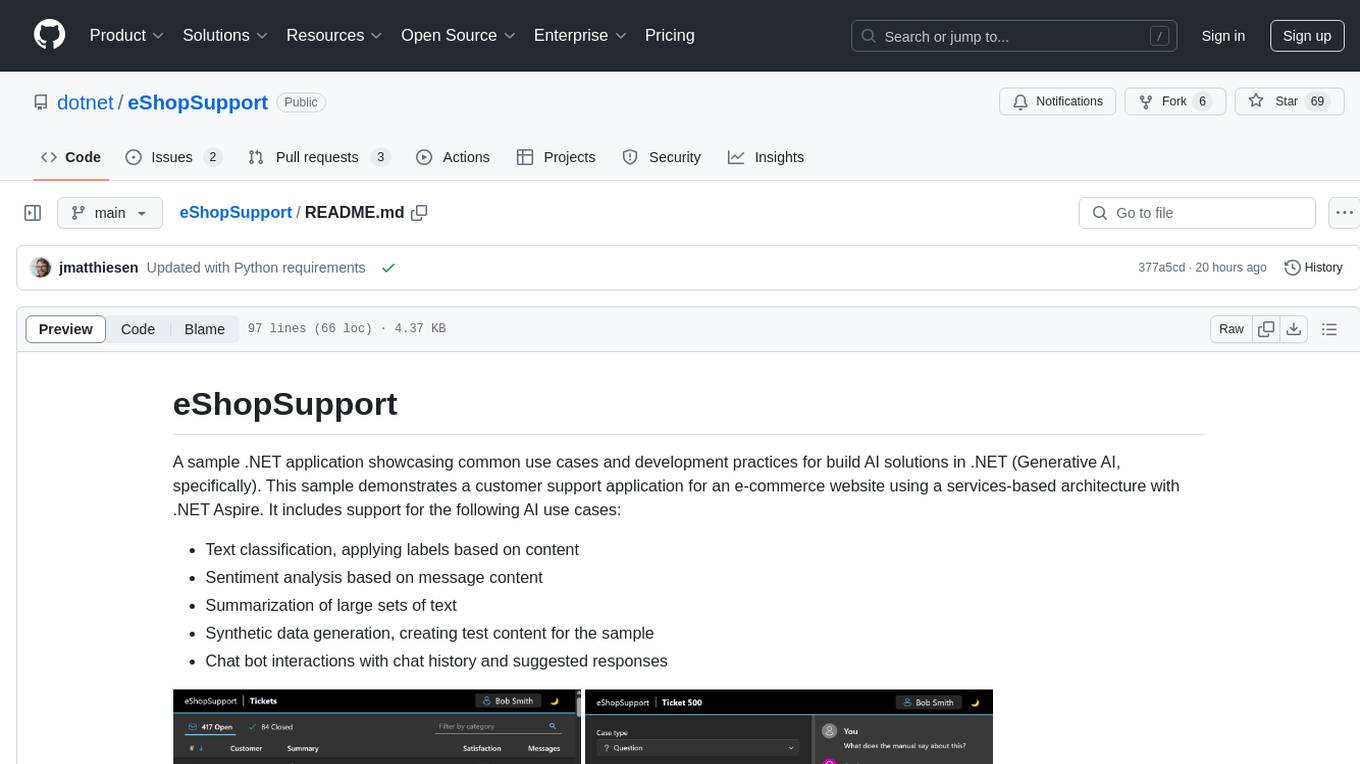
eShopSupport
eShopSupport is a sample .NET application showcasing common use cases and development practices for building AI solutions in .NET, specifically Generative AI. It demonstrates a customer support application for an e-commerce website using a services-based architecture with .NET Aspire. The application includes support for text classification, sentiment analysis, text summarization, synthetic data generation, and chat bot interactions. It also showcases development practices such as developing solutions locally, evaluating AI responses, leveraging Python projects, and deploying applications to the Cloud.
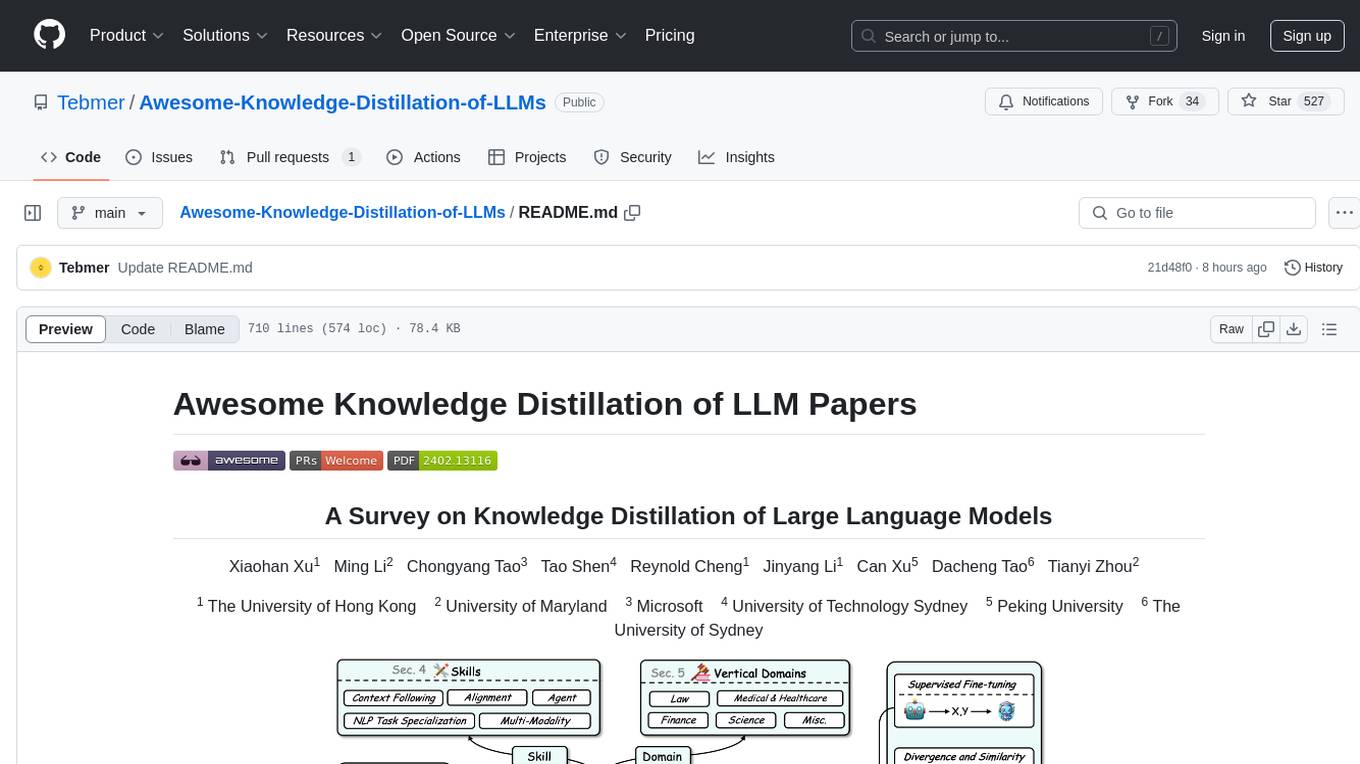
Awesome-Knowledge-Distillation-of-LLMs
A collection of papers related to knowledge distillation of large language models (LLMs). The repository focuses on techniques to transfer advanced capabilities from proprietary LLMs to smaller models, compress open-source LLMs, and refine their performance. It covers various aspects of knowledge distillation, including algorithms, skill distillation, verticalization distillation in fields like law, medical & healthcare, finance, science, and miscellaneous domains. The repository provides a comprehensive overview of the research in the area of knowledge distillation of LLMs.
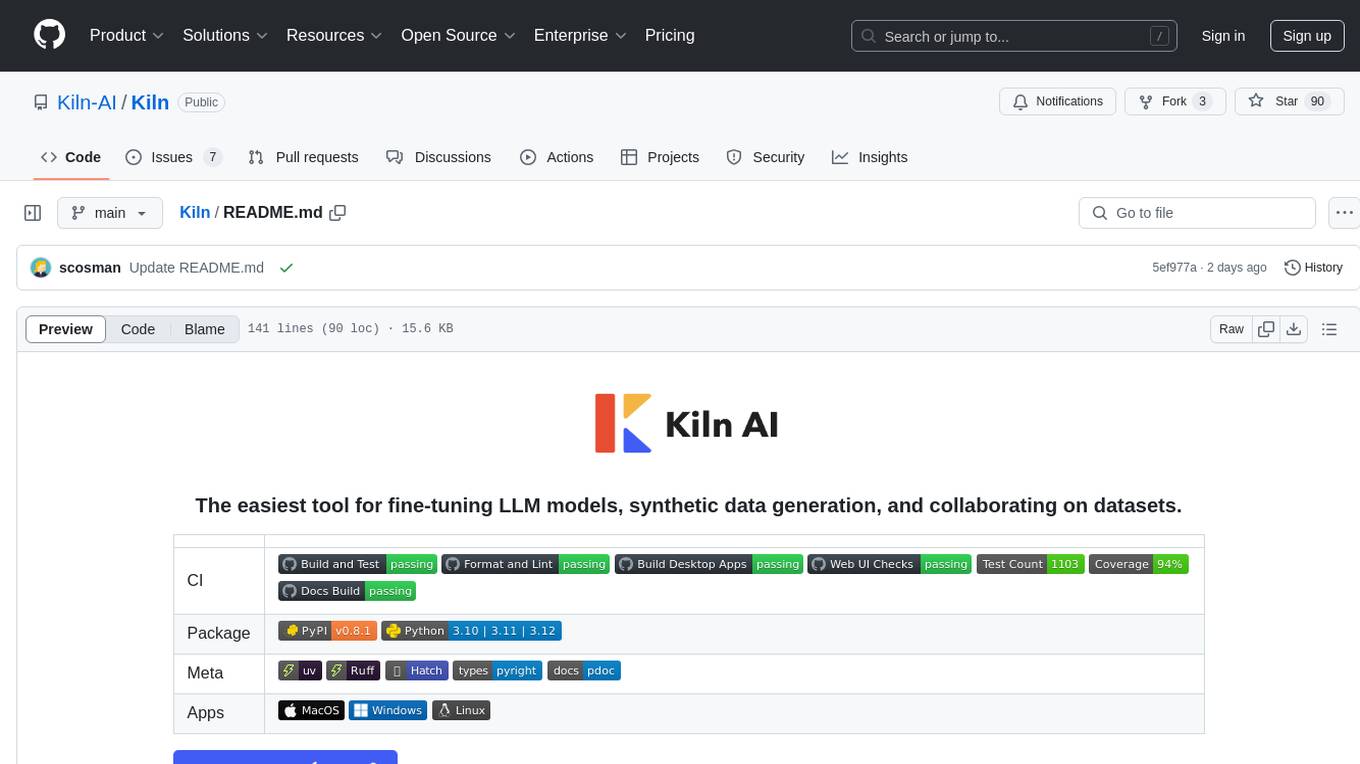
Kiln
Kiln is an intuitive tool for fine-tuning LLM models, generating synthetic data, and collaborating on datasets. It offers desktop apps for Windows, MacOS, and Linux, zero-code fine-tuning for various models, interactive data generation, and Git-based version control. Users can easily collaborate with QA, PM, and subject matter experts, generate auto-prompts, and work with a wide range of models and providers. The tool is open-source, privacy-first, and supports structured data tasks in JSON format. Kiln is free to use and helps build high-quality AI products with datasets, facilitates collaboration between technical and non-technical teams, allows comparison of models and techniques without code, ensures structured data integrity, and prioritizes user privacy.
For similar jobs
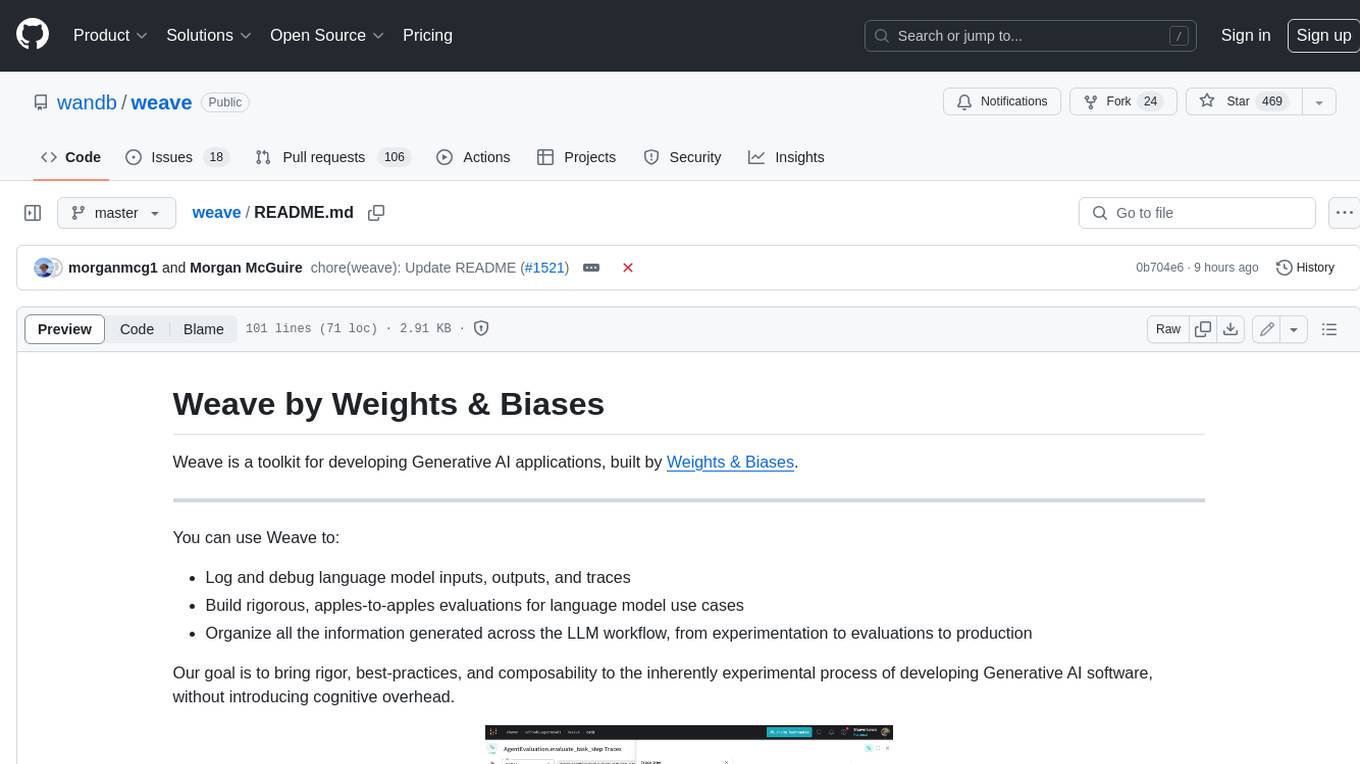
weave
Weave is a toolkit for developing Generative AI applications, built by Weights & Biases. With Weave, you can log and debug language model inputs, outputs, and traces; build rigorous, apples-to-apples evaluations for language model use cases; and organize all the information generated across the LLM workflow, from experimentation to evaluations to production. Weave aims to bring rigor, best-practices, and composability to the inherently experimental process of developing Generative AI software, without introducing cognitive overhead.
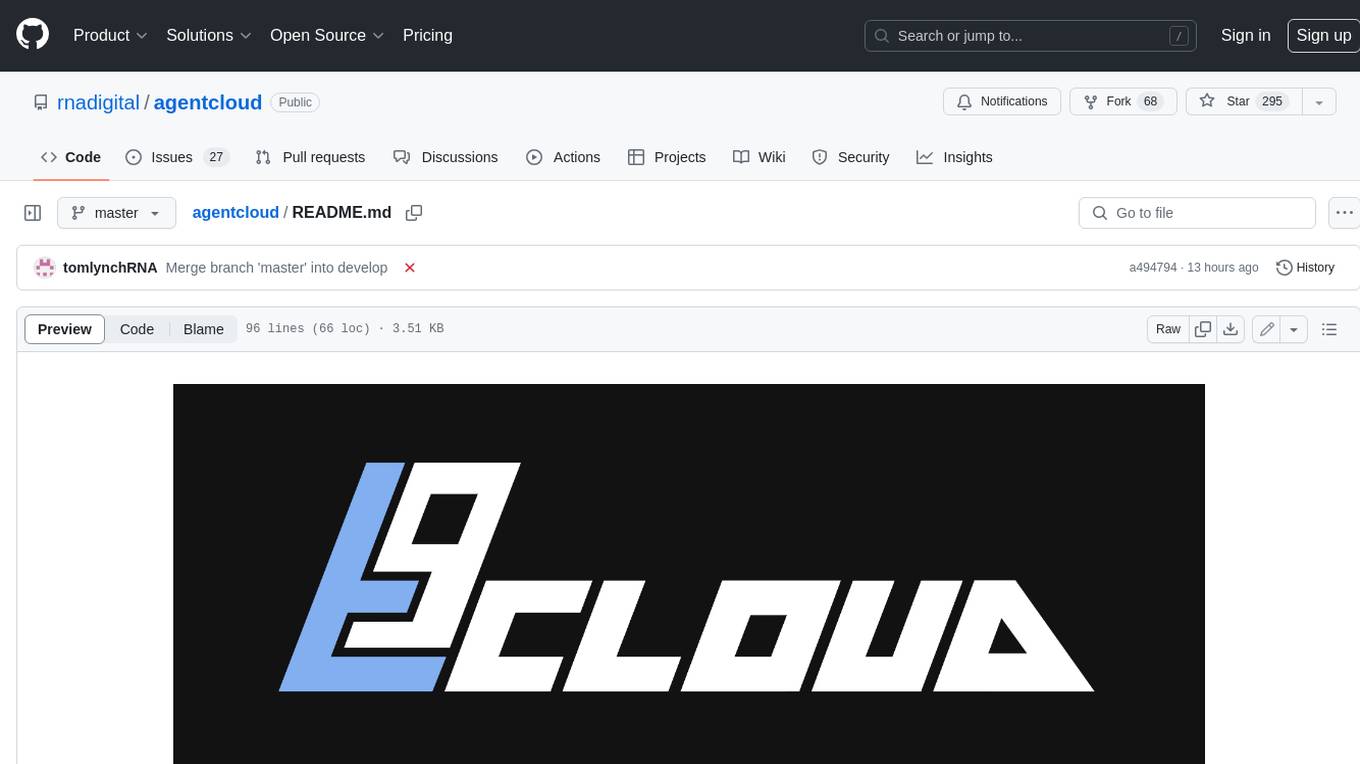
agentcloud
AgentCloud is an open-source platform that enables companies to build and deploy private LLM chat apps, empowering teams to securely interact with their data. It comprises three main components: Agent Backend, Webapp, and Vector Proxy. To run this project locally, clone the repository, install Docker, and start the services. The project is licensed under the GNU Affero General Public License, version 3 only. Contributions and feedback are welcome from the community.
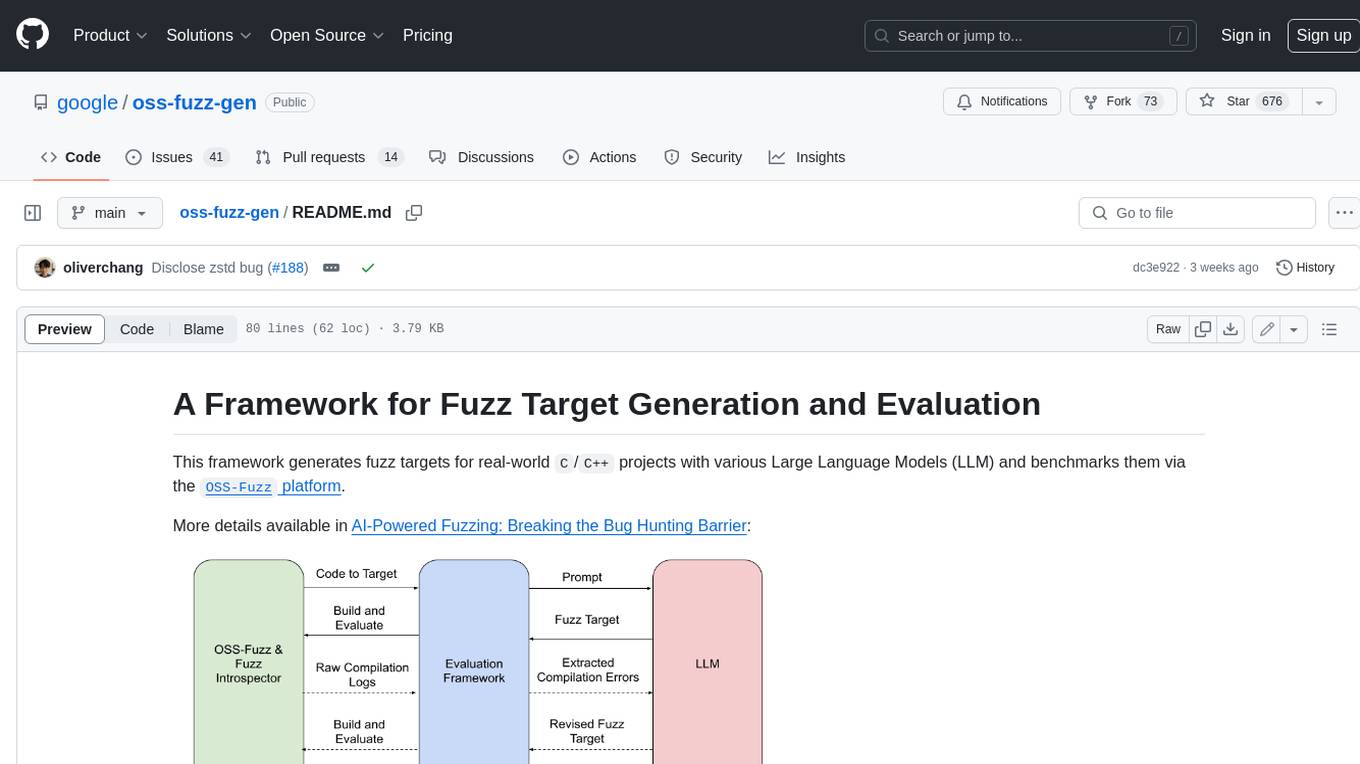
oss-fuzz-gen
This framework generates fuzz targets for real-world `C`/`C++` projects with various Large Language Models (LLM) and benchmarks them via the `OSS-Fuzz` platform. It manages to successfully leverage LLMs to generate valid fuzz targets (which generate non-zero coverage increase) for 160 C/C++ projects. The maximum line coverage increase is 29% from the existing human-written targets.
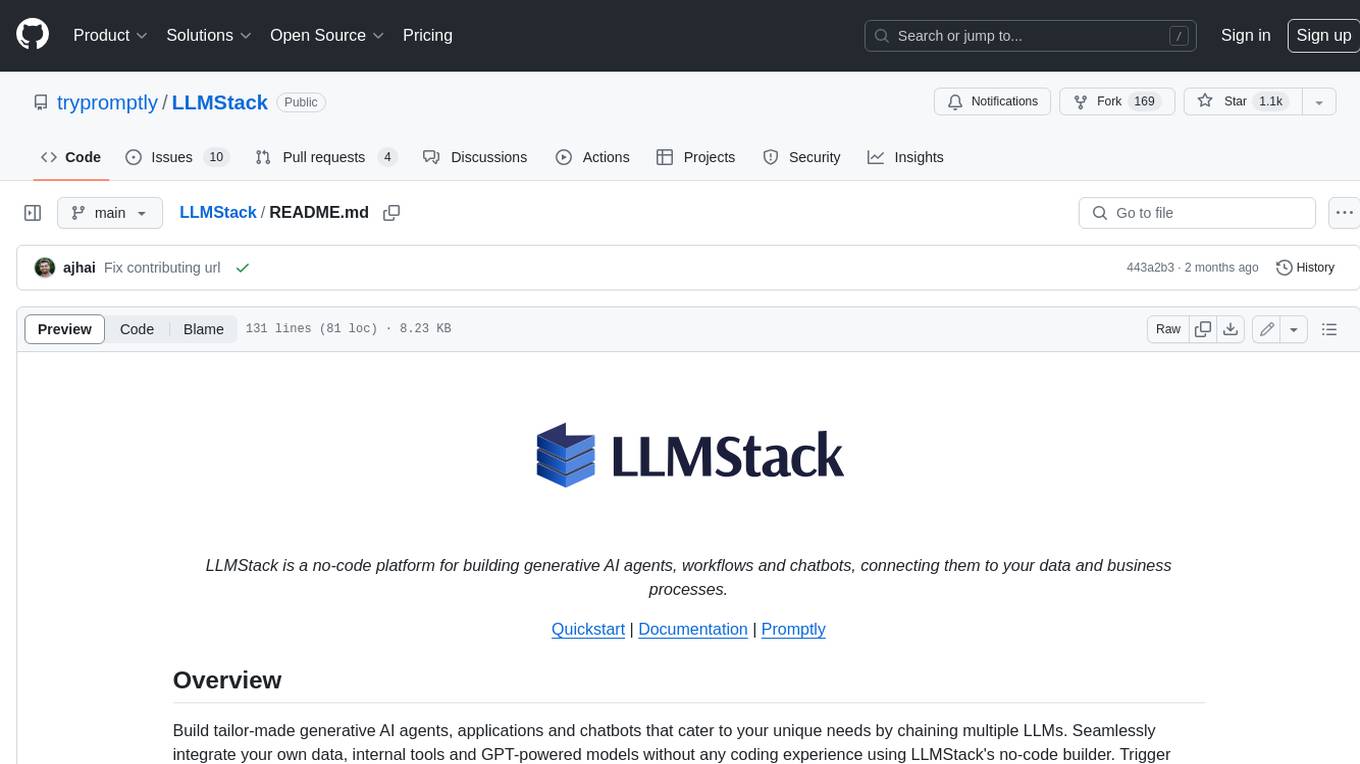
LLMStack
LLMStack is a no-code platform for building generative AI agents, workflows, and chatbots. It allows users to connect their own data, internal tools, and GPT-powered models without any coding experience. LLMStack can be deployed to the cloud or on-premise and can be accessed via HTTP API or triggered from Slack or Discord.
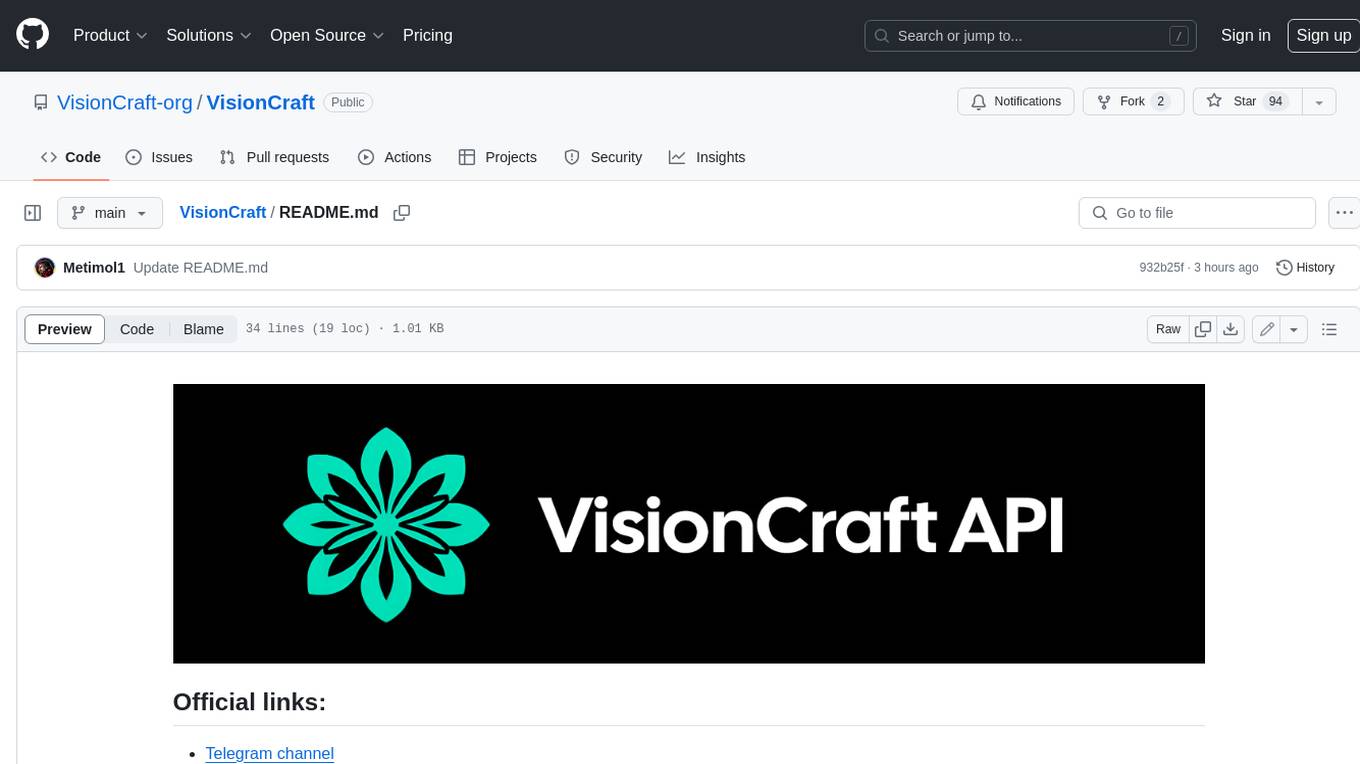
VisionCraft
The VisionCraft API is a free API for using over 100 different AI models. From images to sound.
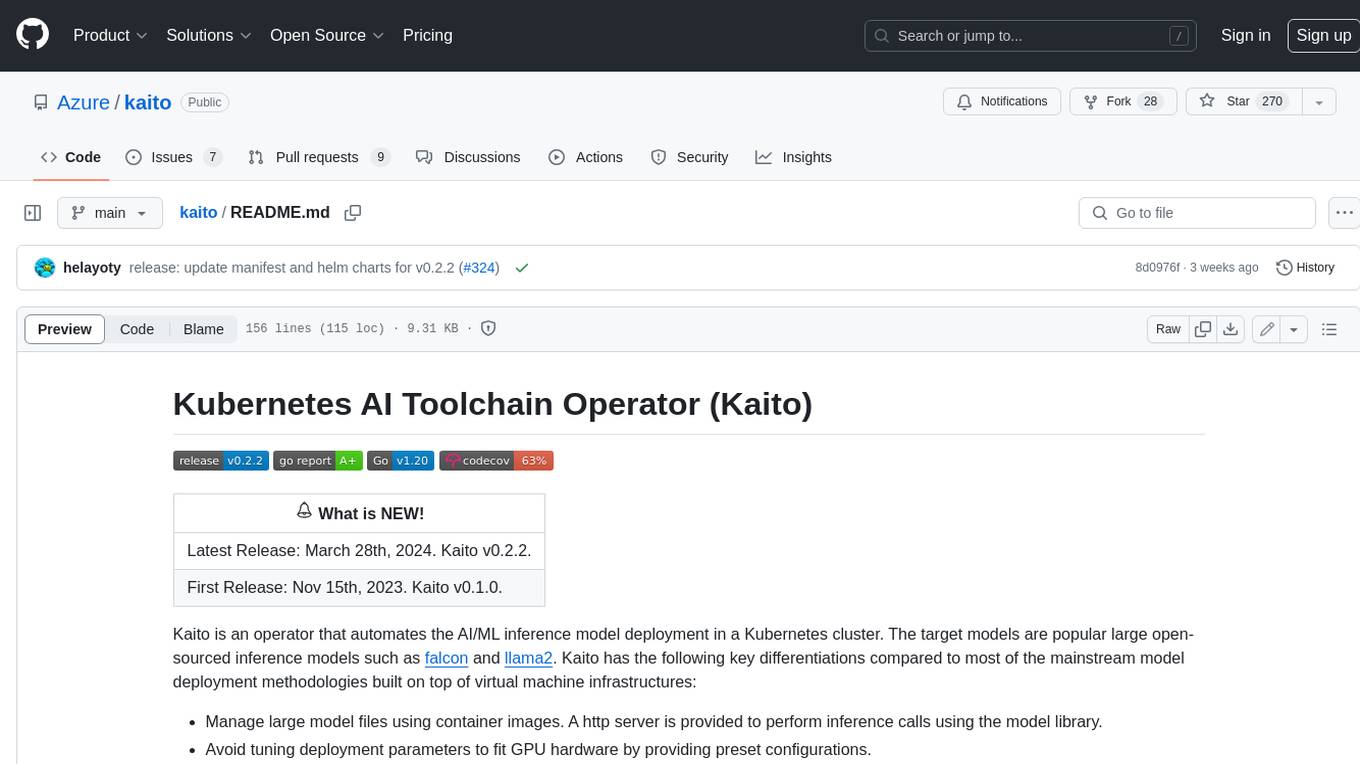
kaito
Kaito is an operator that automates the AI/ML inference model deployment in a Kubernetes cluster. It manages large model files using container images, avoids tuning deployment parameters to fit GPU hardware by providing preset configurations, auto-provisions GPU nodes based on model requirements, and hosts large model images in the public Microsoft Container Registry (MCR) if the license allows. Using Kaito, the workflow of onboarding large AI inference models in Kubernetes is largely simplified.
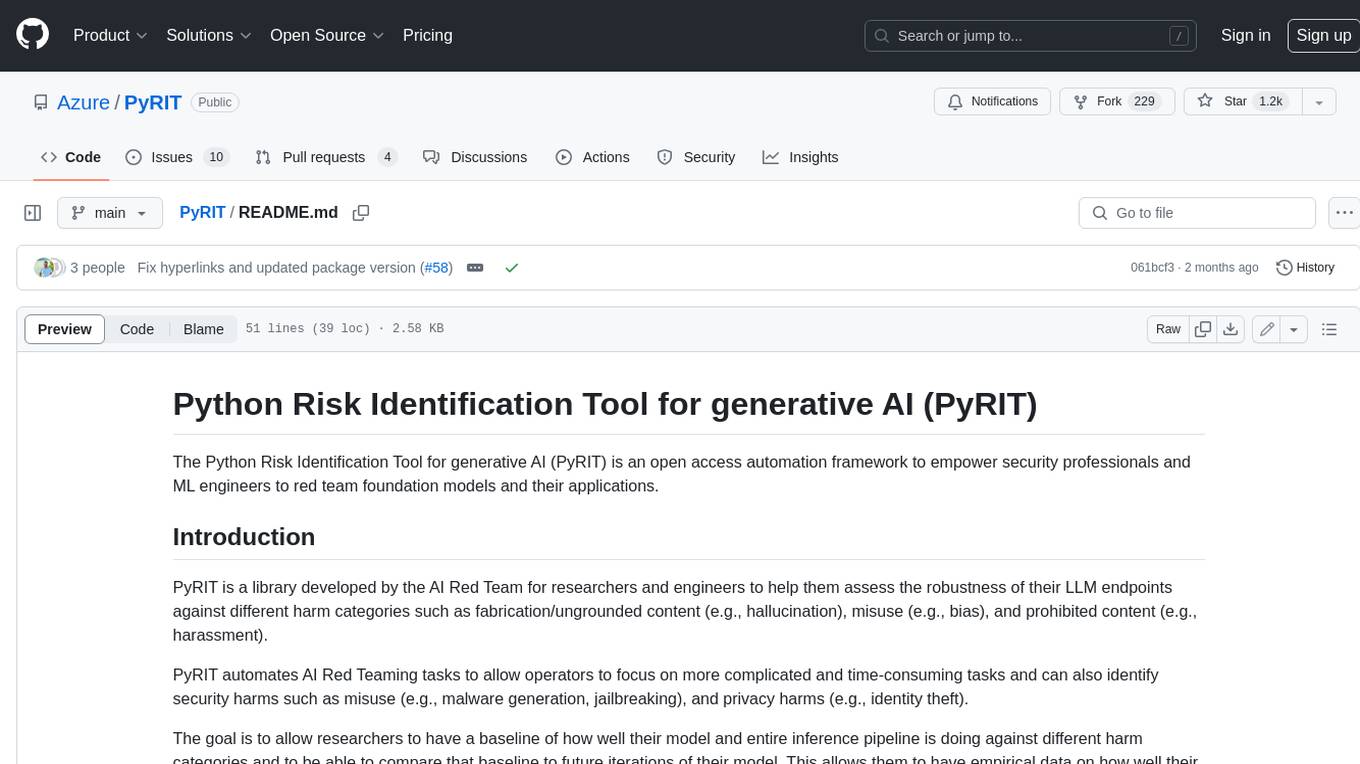
PyRIT
PyRIT is an open access automation framework designed to empower security professionals and ML engineers to red team foundation models and their applications. It automates AI Red Teaming tasks to allow operators to focus on more complicated and time-consuming tasks and can also identify security harms such as misuse (e.g., malware generation, jailbreaking), and privacy harms (e.g., identity theft). The goal is to allow researchers to have a baseline of how well their model and entire inference pipeline is doing against different harm categories and to be able to compare that baseline to future iterations of their model. This allows them to have empirical data on how well their model is doing today, and detect any degradation of performance based on future improvements.
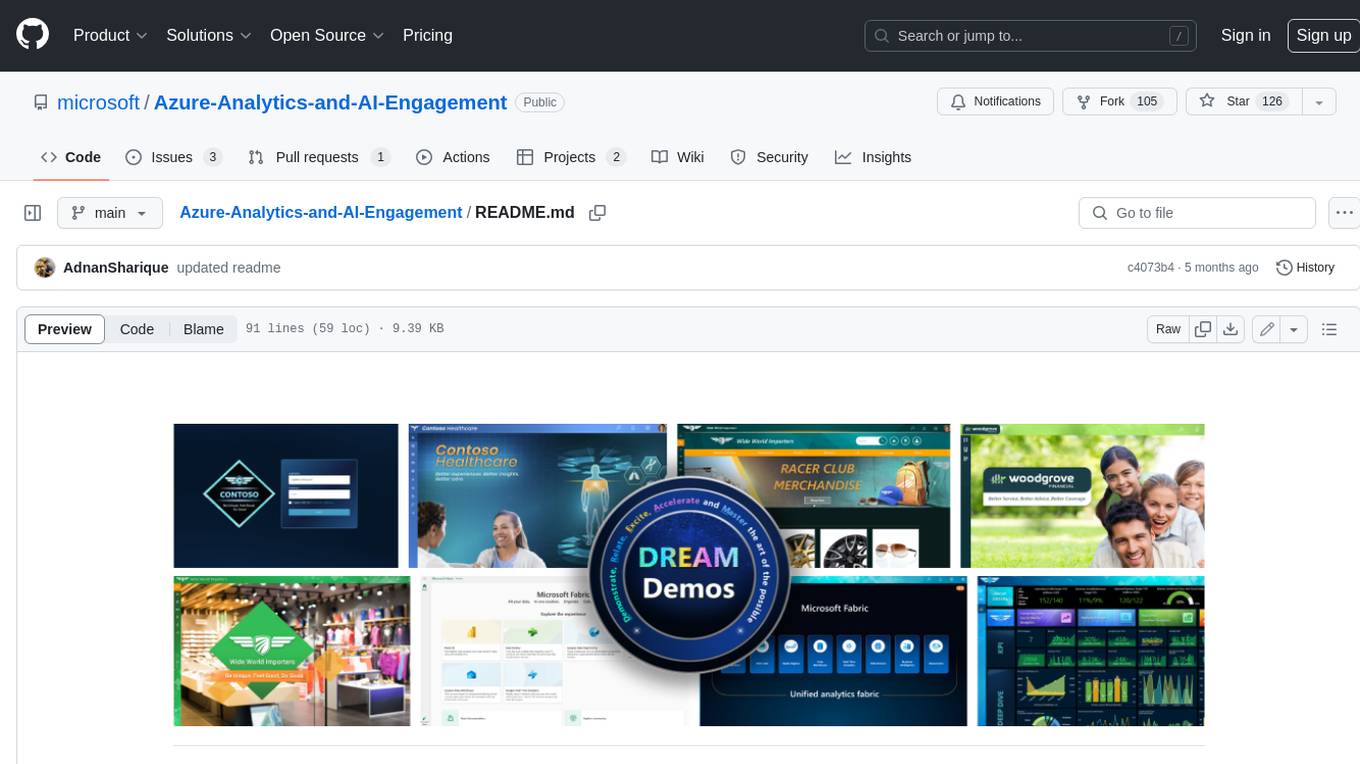
Azure-Analytics-and-AI-Engagement
The Azure-Analytics-and-AI-Engagement repository provides packaged Industry Scenario DREAM Demos with ARM templates (Containing a demo web application, Power BI reports, Synapse resources, AML Notebooks etc.) that can be deployed in a customer’s subscription using the CAPE tool within a matter of few hours. Partners can also deploy DREAM Demos in their own subscriptions using DPoC.