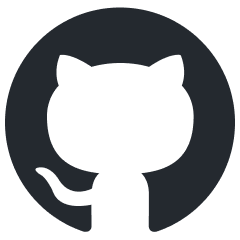
Kiln
The easiest tool for fine-tuning LLM models, synthetic data generation, and collaborating on datasets.
Stars: 3225
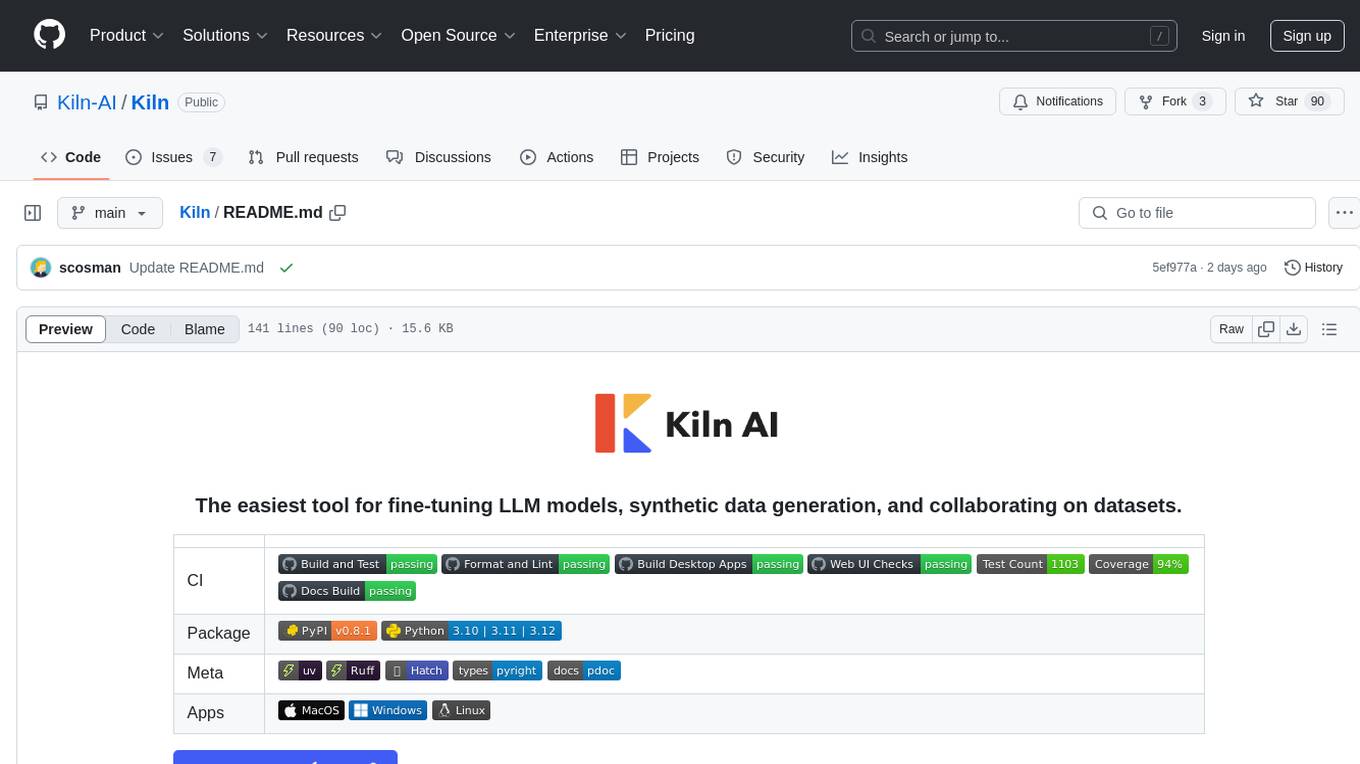
Kiln is an intuitive tool for fine-tuning LLM models, generating synthetic data, and collaborating on datasets. It offers desktop apps for Windows, MacOS, and Linux, zero-code fine-tuning for various models, interactive data generation, and Git-based version control. Users can easily collaborate with QA, PM, and subject matter experts, generate auto-prompts, and work with a wide range of models and providers. The tool is open-source, privacy-first, and supports structured data tasks in JSON format. Kiln is free to use and helps build high-quality AI products with datasets, facilitates collaboration between technical and non-technical teams, allows comparison of models and techniques without code, ensures structured data integrity, and prioritizes user privacy.
README:
Fine Tuning • Synthetic Data Generation • Evals • Collaboration • Docs
CI |
|
Package |
|
Meta |
|
Apps |
|
Connect |
|
- 🚀 Intuitive Desktop Apps: One-click apps for Windows, MacOS, and Linux. Truly intuitive design.
- 🎛️ Fine Tuning: Zero-code fine-tuning for Llama, GPT-4o, and more. Automatic serverless deployment of models.
- 📊 Evals: Evaluate the quality of your models/tasks using state of the art evaluators.
- 🤖 Synthetic Data Generation: Generate training data with our interactive visual tooling.
- 🧠 Reasoning Models: Train or distill your own custom reasoning models.
- 🤝 Team Collaboration: Git-based version control for your AI datasets. Intuitive UI makes it easy to collaborate with QA, PM, and subject matter experts on structured data (examples, prompts, ratings, feedback, issues, etc.).
- 📝 Prompt Generation: Automatically generate prompts from your data, including chain-of-thought, few-shot, and multi-shot, and more.
- 🌐 Wide Model and Provider Support: Use any model via Ollama, OpenAI, OpenRouter, Fireworks, Groq, AWS, or any OpenAI compatible API.
- 🧑💻 Open-Source Library and API: Our Python library and OpenAPI REST API are MIT open source.
- 🔒 Privacy-First: We can't see your data. Bring your own API keys or run locally with Ollama.
- 🗃️ Structured Data: Build AI tasks that speak JSON.
- 💰 Free: Our apps are free, and our library is open-source.
The Kiln desktop app is completely free. Available on MacOS, Windows and Linux.
In this demo, I create 9 fine-tuned models (including Llama 3.x, Mixtral, and GPT-4o-mini) in just 18 minutes, achieving great results for less than $6 total cost. See details.
Kiln is quite intuitive, so we suggest launching the desktop app and diving in. However if you have any questions or want to learn more, our docs are here to help.
- Quick Start
- How to use any AI model or provider in Kiln
- Fine Tuning LLM Models
- Guide: Train a Reasoning Model
- Reasoning & Chain of Thought
- Evaluators
- Synthetic Data Generation
- Collaborating with Kiln
- Rating and Labeling Data
- Prompt Styles
- Structure Data / JSON
- Organizing Kiln Datasets (Tags and Filters)
- Our Data Model
- Repairing Responses
- Keyboard Shortcuts
- Privacy Overview: Private by Design
For developers, see our Kiln Python Library Docs. These include how to load datasets into Kiln, or using Kiln datasets in your own code-base/notebooks.
Our open-source python library allows you to integrate Kiln datasets into your own workflows, build fine tunes, use Kiln in Notebooks, build custom tools, and much more! Read the docs for examples.
pip install kiln-ai
There are new models and techniques emerging all the time. Kiln makes it easy to try a variety of approaches and compare them in a few clicks without writing code. These can result in higher quality and improved performance.
We currently support:
- Various prompting techniques: basic, few-shot, multi-shot, repair & feedback
- Chain of thought / thinking, with optional custom “thinking” instructions
- Many models: GPT, Llama, Claude, Gemini, Mistral, Gemma, Phi
- Fine Tuning: create custom models using your Kiln dataset
In the future, we plan to add more powerful no-code options like evals, and RAG. For experienced data-scientists, you can create these techniques today using Kiln datasets and our python library.
When building AI products, there’s usually a subject matter expert who knows the problem you are trying to solve, and a different technical team assigned to build the model. Kiln bridges that gap as a collaboration tool.
Subject matter experts can use our intuitive desktop apps to generate structured datasets and ratings, without coding or using technical tools. No command line or GPU required.
Data-scientists can consume the dataset created by subject matter experts, using the UI, or deep dive with our python library.
QA and PM can easily identify issues sooner and help generate the dataset content needed to fix the issue at the model layer.
The dataset file format is designed to be be used with Git for powerful collaboration and attribution. Many people can contribute in parallel; collisions are avoided using UUIDs, and attribution is captured inside the dataset files. You can even share a dataset on a shared drive, letting completely non-technical team members contribute data and evals without knowing Git.
Products don’t naturally have “datasets”, but Kiln helps you create one. Every time you use Kiln, we capture the inputs, outputs, human ratings, feedback, and repairs needed to build high quality models for use in your product. The more you use it, the more data you have.
Your model quality improves automatically as the dataset grows, by giving the models more examples of quality content (and mistakes).
If your product goals shift or new bugs are found (as is almost always the case), you can easily iterate the dataset to address issues.
See CONTRIBUTING.md for information on how to setup a development environment and contribute to Kiln.
@software{kiln_ai,
title = {Kiln: Rapid AI Prototyping and Dataset Collaboration Tool},
author = {{Chesterfield Laboratories Inc.}},
year = {2025},
url = {https://github.com/Kiln-AI/Kiln},
version = {latest}
}
- Python Library: MIT License
- Python REST Server/API: MIT License
- Desktop App: free to download and use under our EULA, and source-available. License
- The Kiln names and logos are trademarks of Chesterfield Laboratories Inc.
Copyright 2024 - Chesterfield Laboratories Inc.
For Tasks:
Click tags to check more tools for each tasksFor Jobs:
Alternative AI tools for Kiln
Similar Open Source Tools
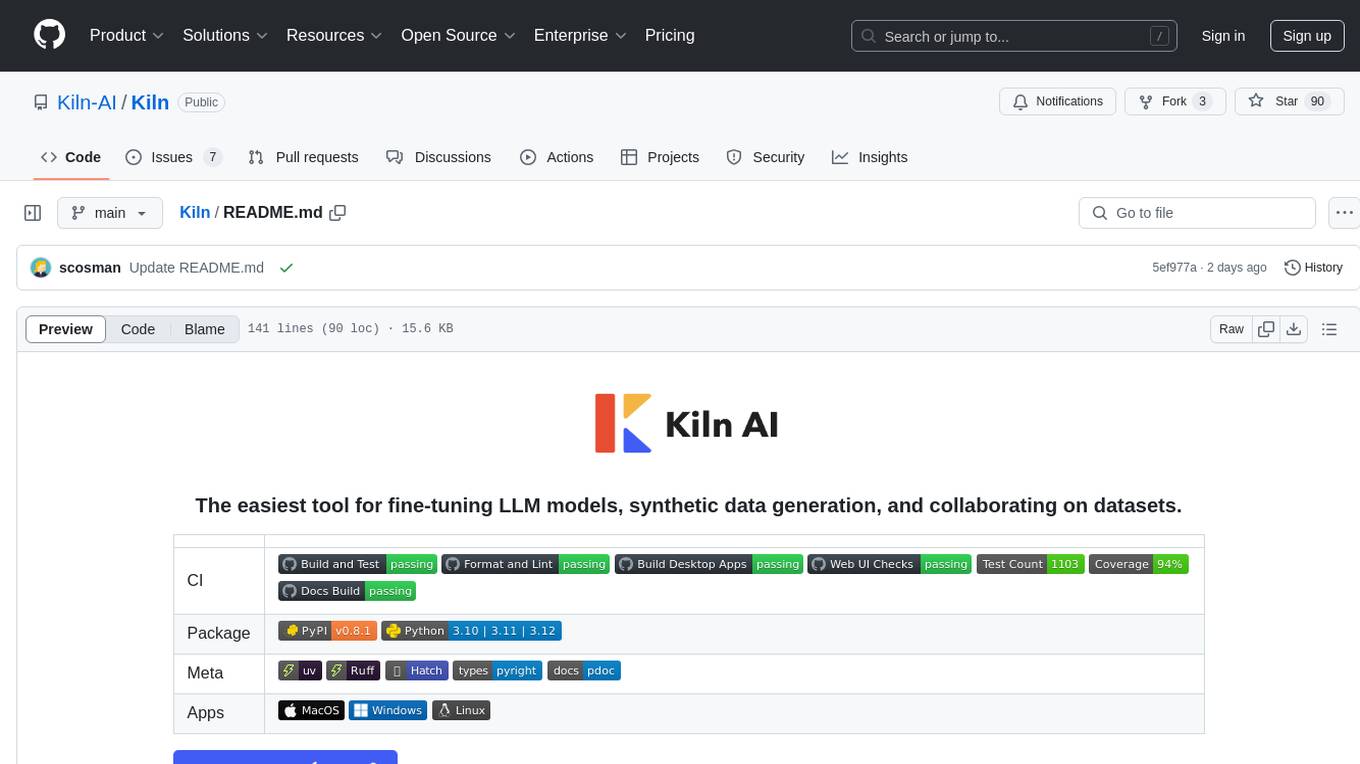
Kiln
Kiln is an intuitive tool for fine-tuning LLM models, generating synthetic data, and collaborating on datasets. It offers desktop apps for Windows, MacOS, and Linux, zero-code fine-tuning for various models, interactive data generation, and Git-based version control. Users can easily collaborate with QA, PM, and subject matter experts, generate auto-prompts, and work with a wide range of models and providers. The tool is open-source, privacy-first, and supports structured data tasks in JSON format. Kiln is free to use and helps build high-quality AI products with datasets, facilitates collaboration between technical and non-technical teams, allows comparison of models and techniques without code, ensures structured data integrity, and prioritizes user privacy.
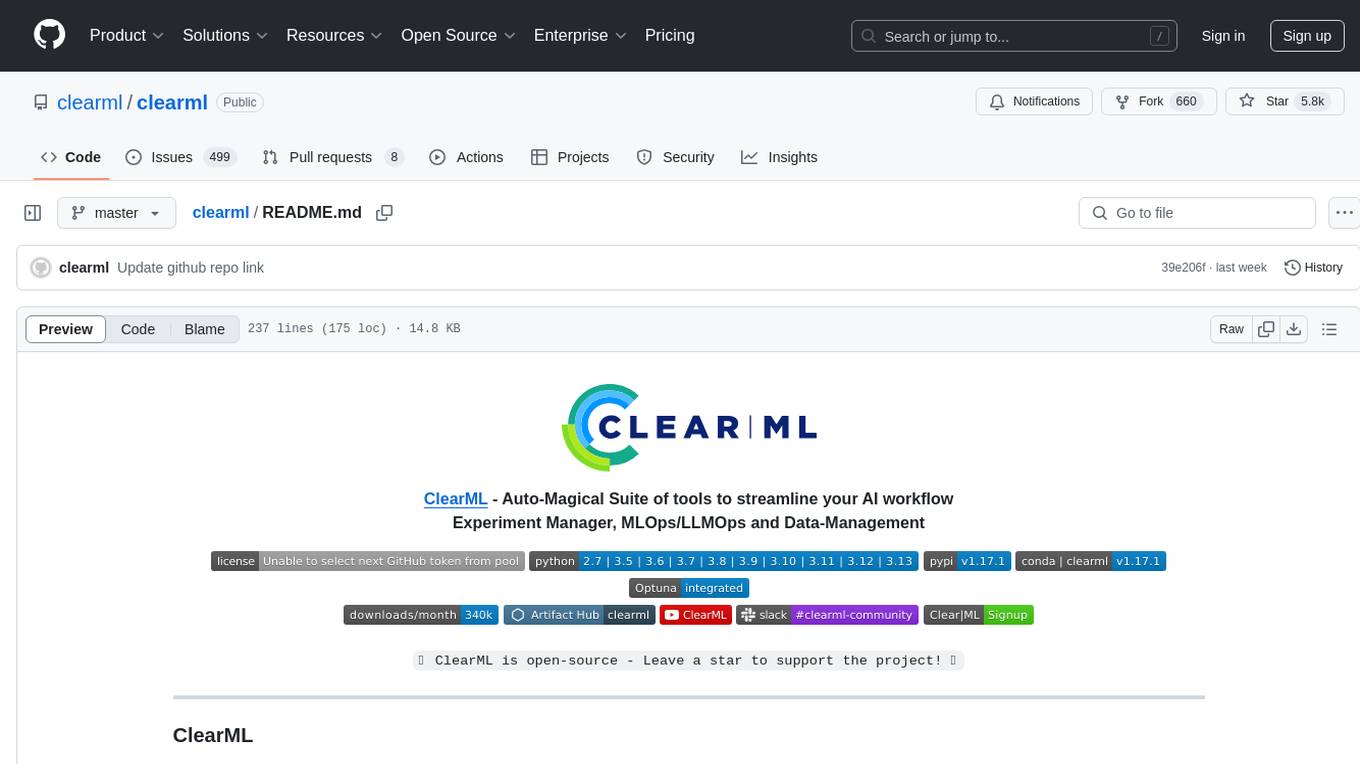
clearml
ClearML is an auto-magical suite of tools designed to streamline AI workflows. It includes modules for experiment management, MLOps/LLMOps, data management, model serving, and more. ClearML offers features like experiment tracking, model serving, orchestration, and automation. It supports various ML/DL frameworks and integrates with Jupyter Notebook and PyCharm for remote debugging. ClearML aims to simplify collaboration, automate processes, and enhance visibility in AI projects.
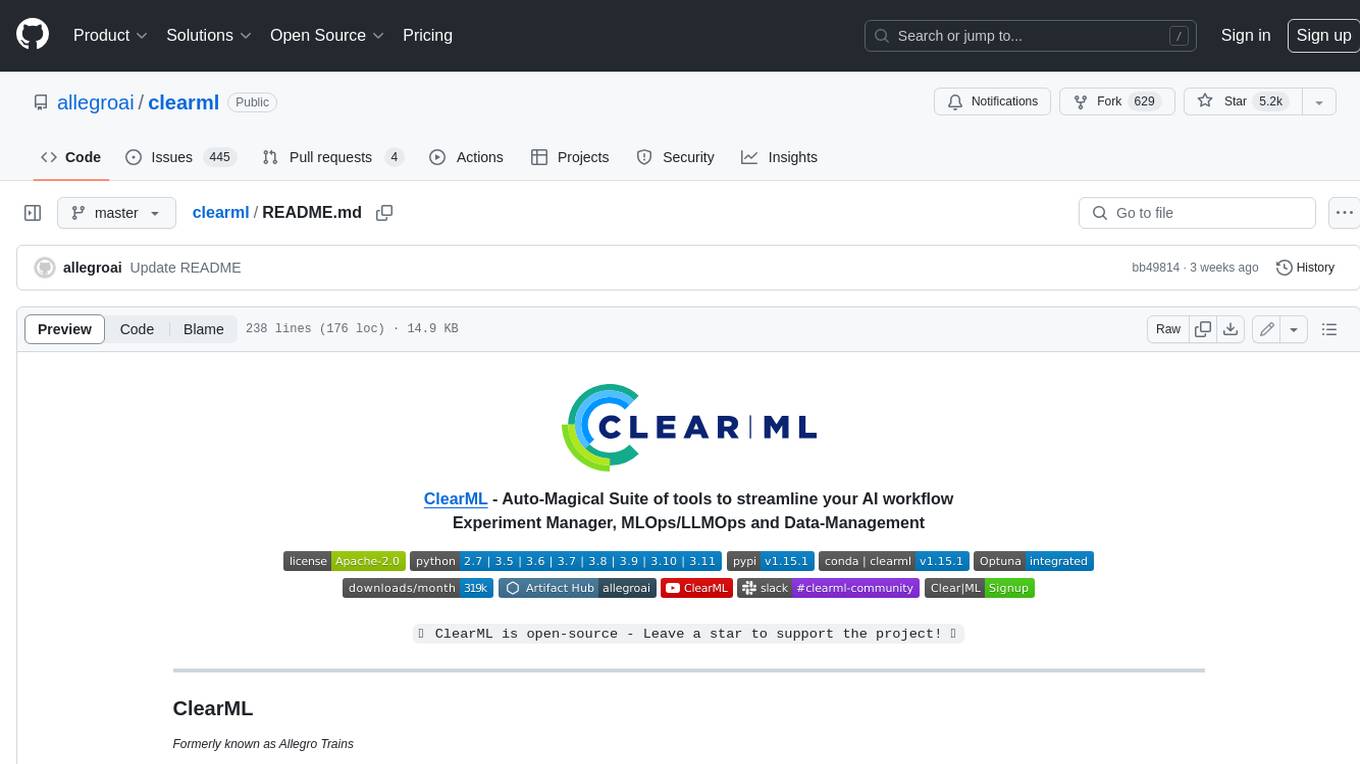
clearml
ClearML is a suite of tools designed to streamline the machine learning workflow. It includes an experiment manager, MLOps/LLMOps, data management, and model serving capabilities. ClearML is open-source and offers a free tier hosting option. It supports various ML/DL frameworks and integrates with Jupyter Notebook and PyCharm. ClearML provides extensive logging capabilities, including source control info, execution environment, hyper-parameters, and experiment outputs. It also offers automation features, such as remote job execution and pipeline creation. ClearML is designed to be easy to integrate, requiring only two lines of code to add to existing scripts. It aims to improve collaboration, visibility, and data transparency within ML teams.
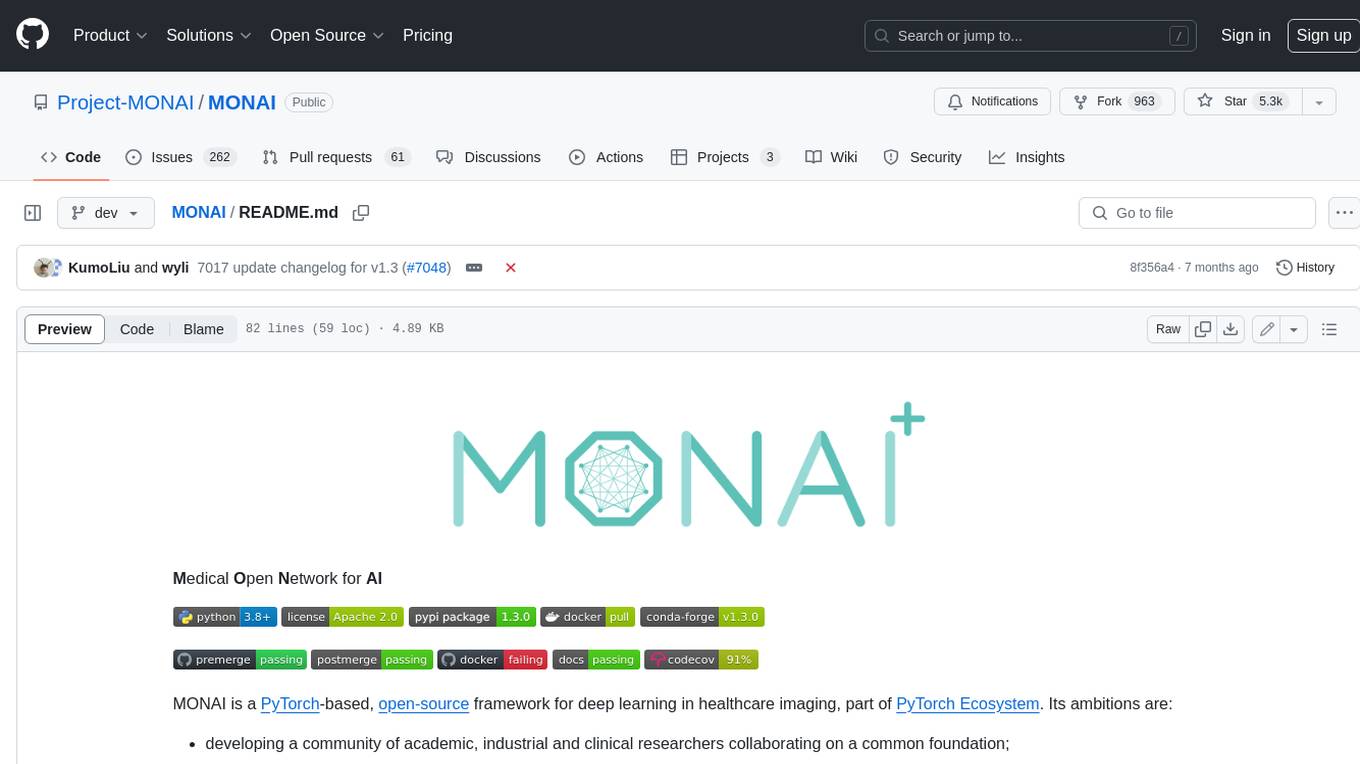
MONAI
MONAI is a PyTorch-based, open-source framework for deep learning in healthcare imaging. It provides a comprehensive set of tools for medical image analysis, including data preprocessing, model training, and evaluation. MONAI is designed to be flexible and easy to use, making it a valuable resource for researchers and developers in the field of medical imaging.
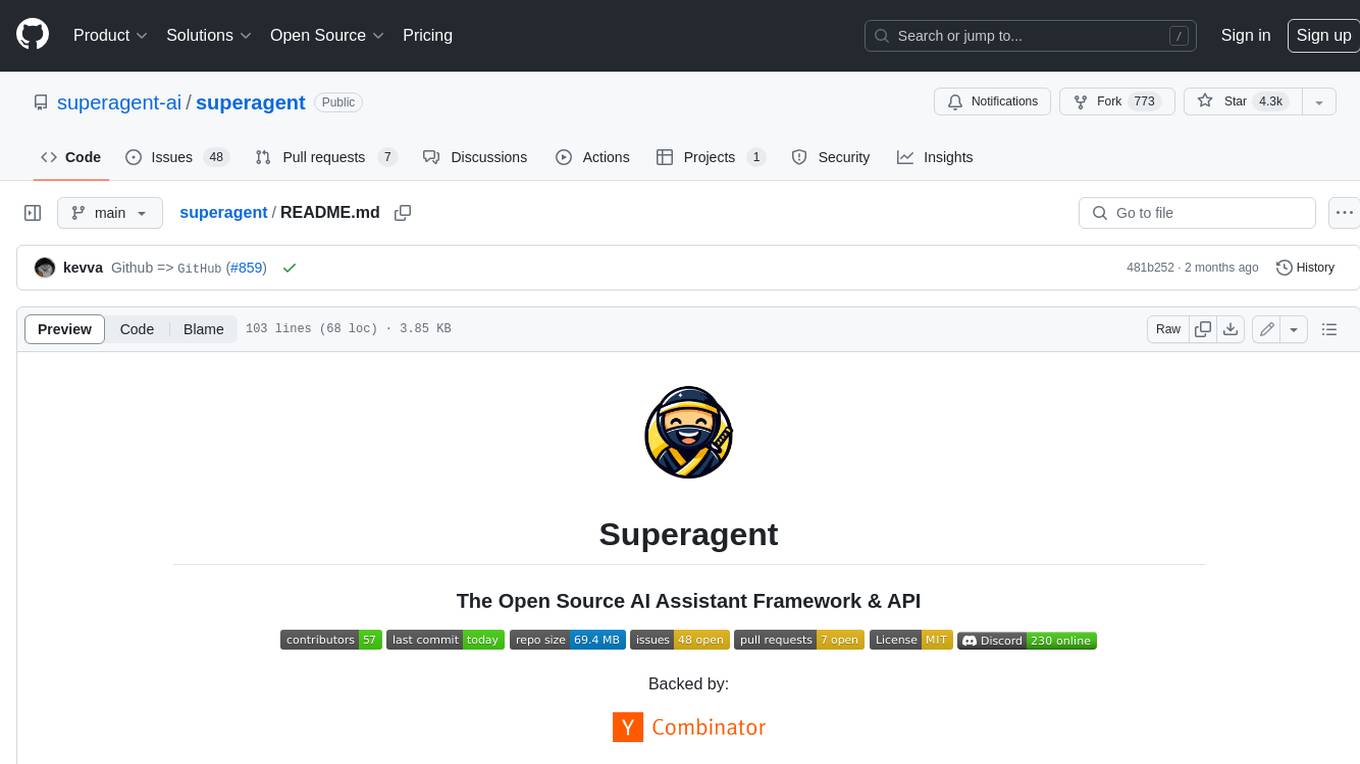
superagent
Superagent is an open-source AI assistant framework and API that allows developers to add powerful AI assistants to their applications. These assistants use large language models (LLMs), retrieval augmented generation (RAG), and generative AI to help users with a variety of tasks, including question answering, chatbot development, content generation, data aggregation, and workflow automation. Superagent is backed by Y Combinator and is part of YC W24.
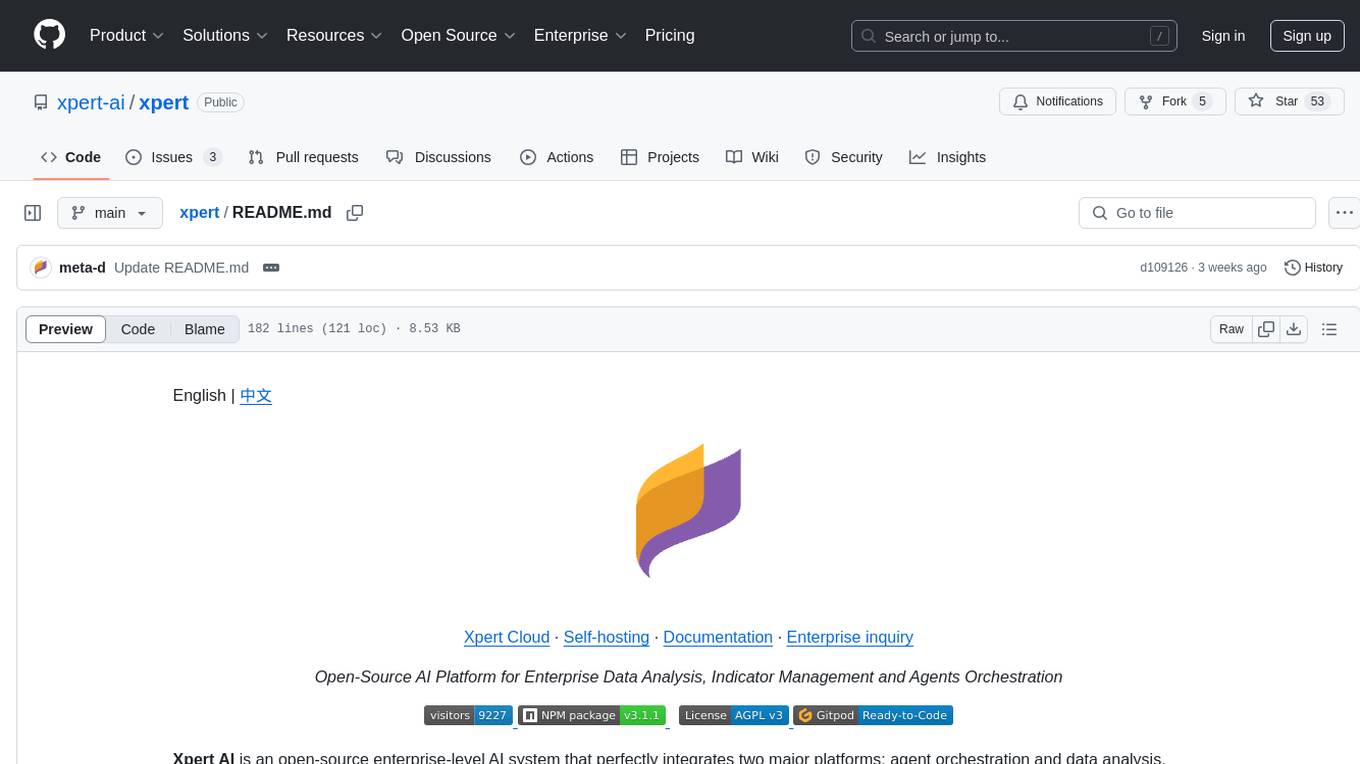
xpert
Xpert is a powerful tool for data analysis and visualization. It provides a user-friendly interface to explore and manipulate datasets, perform statistical analysis, and create insightful visualizations. With Xpert, users can easily import data from various sources, clean and preprocess data, analyze trends and patterns, and generate interactive charts and graphs. Whether you are a data scientist, analyst, researcher, or student, Xpert simplifies the process of data analysis and visualization, making it accessible to users with varying levels of expertise.
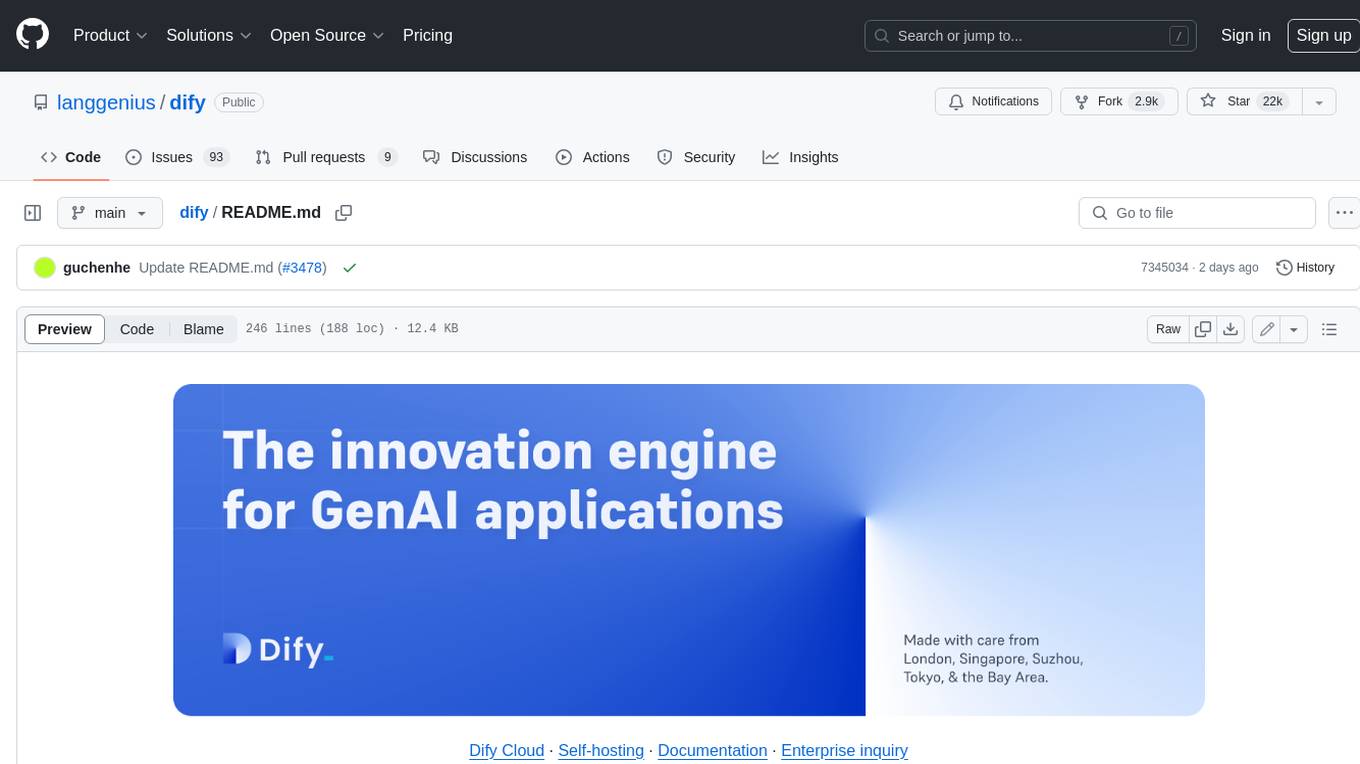
dify
Dify is an open-source LLM app development platform that combines AI workflow, RAG pipeline, agent capabilities, model management, observability features, and more. It allows users to quickly go from prototype to production. Key features include: 1. Workflow: Build and test powerful AI workflows on a visual canvas. 2. Comprehensive model support: Seamless integration with hundreds of proprietary / open-source LLMs from dozens of inference providers and self-hosted solutions. 3. Prompt IDE: Intuitive interface for crafting prompts, comparing model performance, and adding additional features. 4. RAG Pipeline: Extensive RAG capabilities that cover everything from document ingestion to retrieval. 5. Agent capabilities: Define agents based on LLM Function Calling or ReAct, and add pre-built or custom tools. 6. LLMOps: Monitor and analyze application logs and performance over time. 7. Backend-as-a-Service: All of Dify's offerings come with corresponding APIs for easy integration into your own business logic.
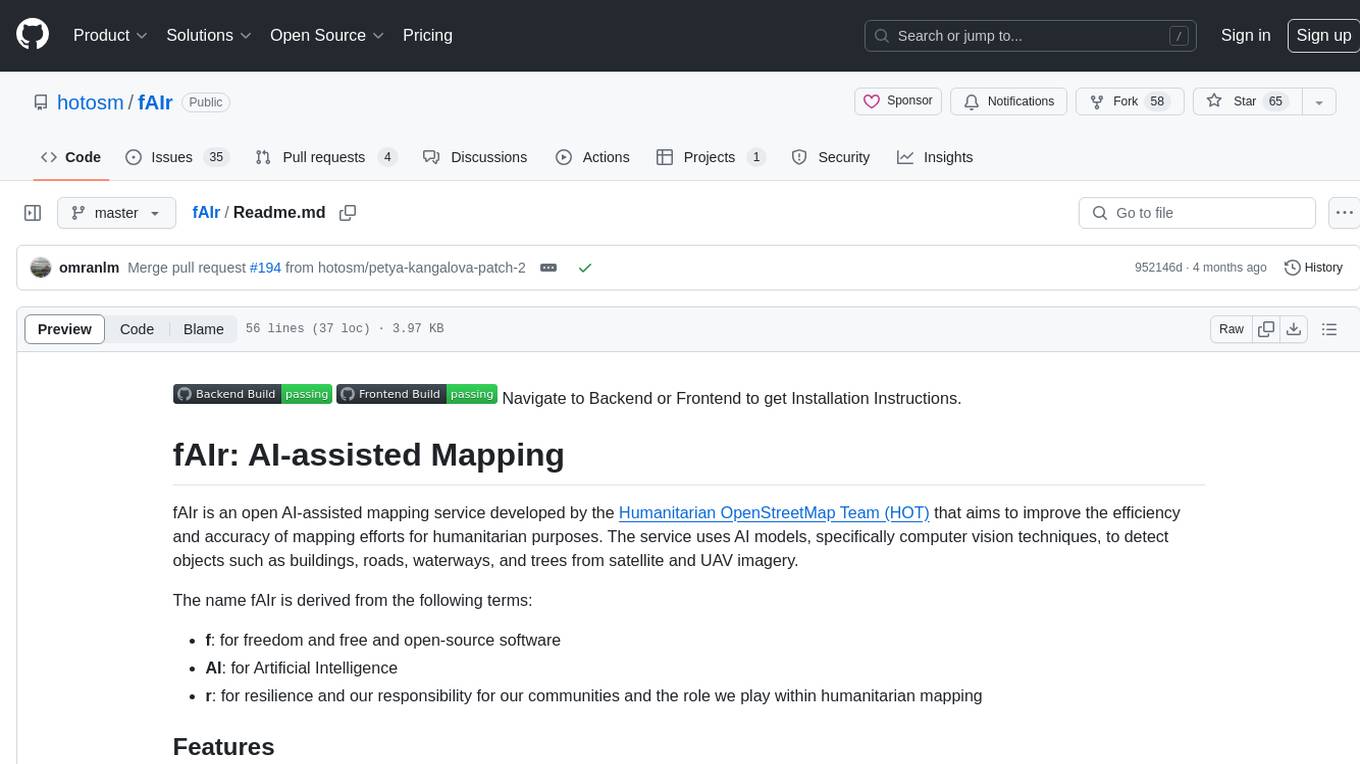
fAIr
fAIr is an open AI-assisted mapping service developed by the Humanitarian OpenStreetMap Team (HOT) to improve mapping efficiency and accuracy for humanitarian purposes. It uses AI models, specifically computer vision techniques, to detect objects like buildings, roads, waterways, and trees from satellite and UAV imagery. The service allows OSM community members to create and train their own AI models for mapping in their region of interest and ensures models are relevant to local communities. Constant feedback loop with local communities helps eliminate model biases and improve model accuracy.
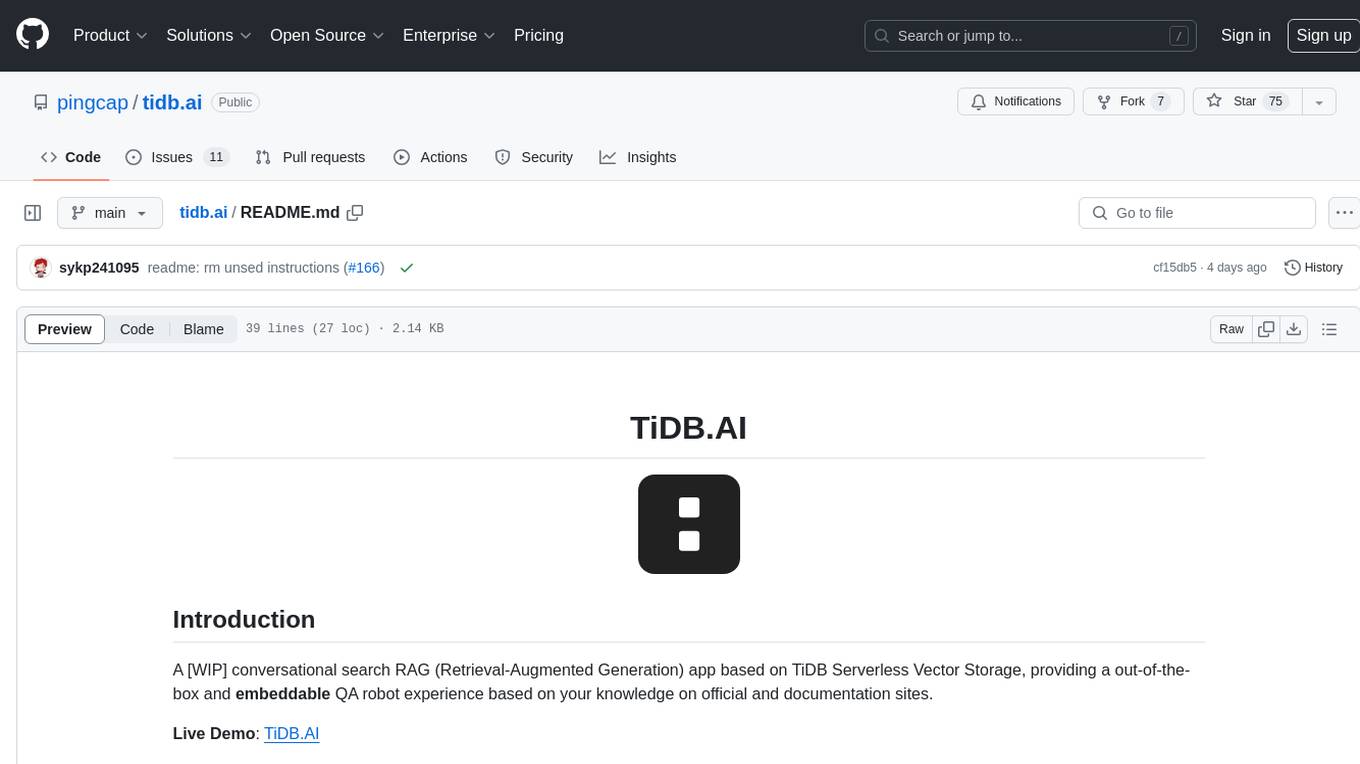
tidb.ai
TiDB.AI is a conversational search RAG (Retrieval-Augmented Generation) app based on TiDB Serverless Vector Storage. It provides an out-of-the-box and embeddable QA robot experience based on knowledge from official and documentation sites. The platform features a Perplexity-style Conversational Search page with an advanced built-in website crawler for comprehensive coverage. Users can integrate an embeddable JavaScript snippet into their website for instant responses to product-related queries. The tech stack includes Next.js, TypeScript, Tailwind CSS, shadcn/ui for design, TiDB for database storage, Kysely for SQL query building, NextAuth.js for authentication, Vercel for deployments, and LlamaIndex for the RAG framework. TiDB.AI is open-source under the Apache License, Version 2.0.
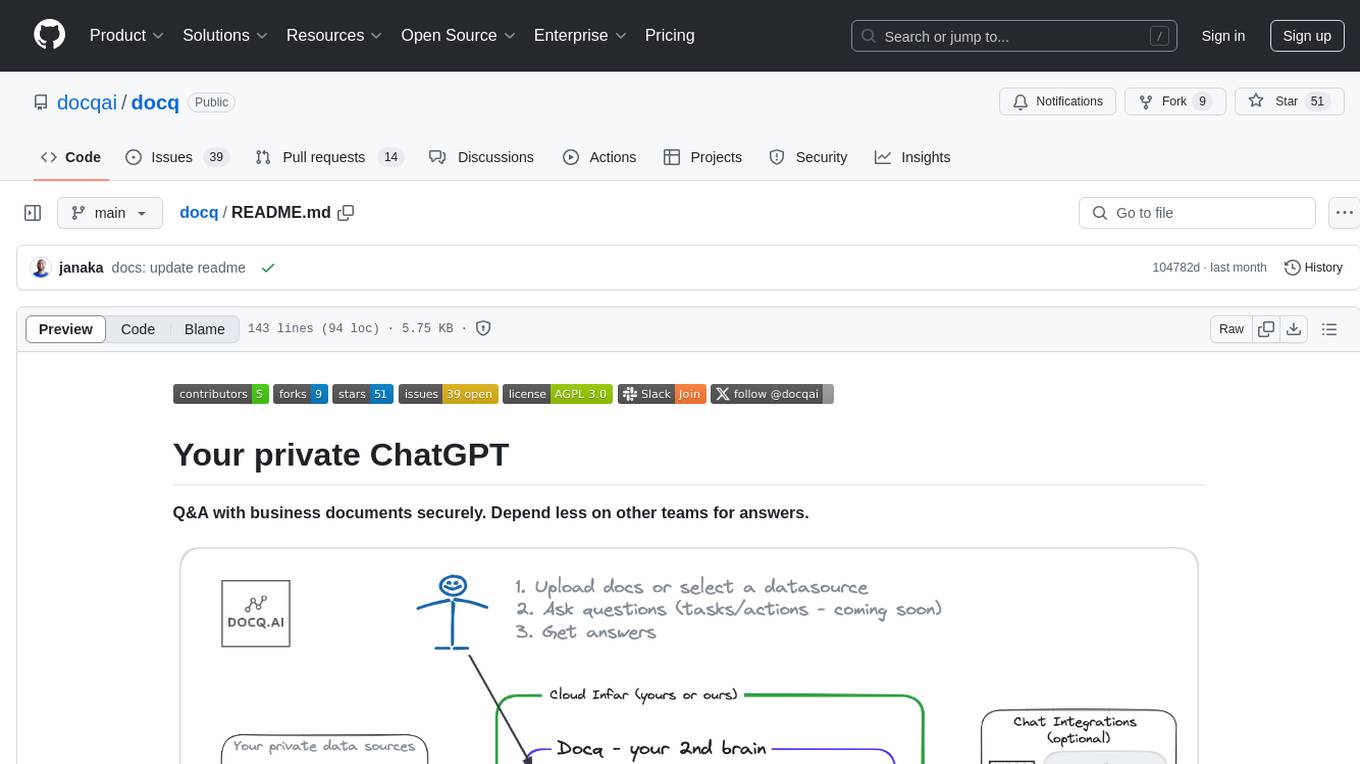
docq
Docq is a private and secure GenAI tool designed to extract knowledge from business documents, enabling users to find answers independently. It allows data to stay within organizational boundaries, supports self-hosting with various cloud vendors, and offers multi-model and multi-modal capabilities. Docq is extensible, open-source (AGPLv3), and provides commercial licensing options. The tool aims to be a turnkey solution for organizations to adopt AI innovation safely, with plans for future features like more data ingestion options and model fine-tuning.
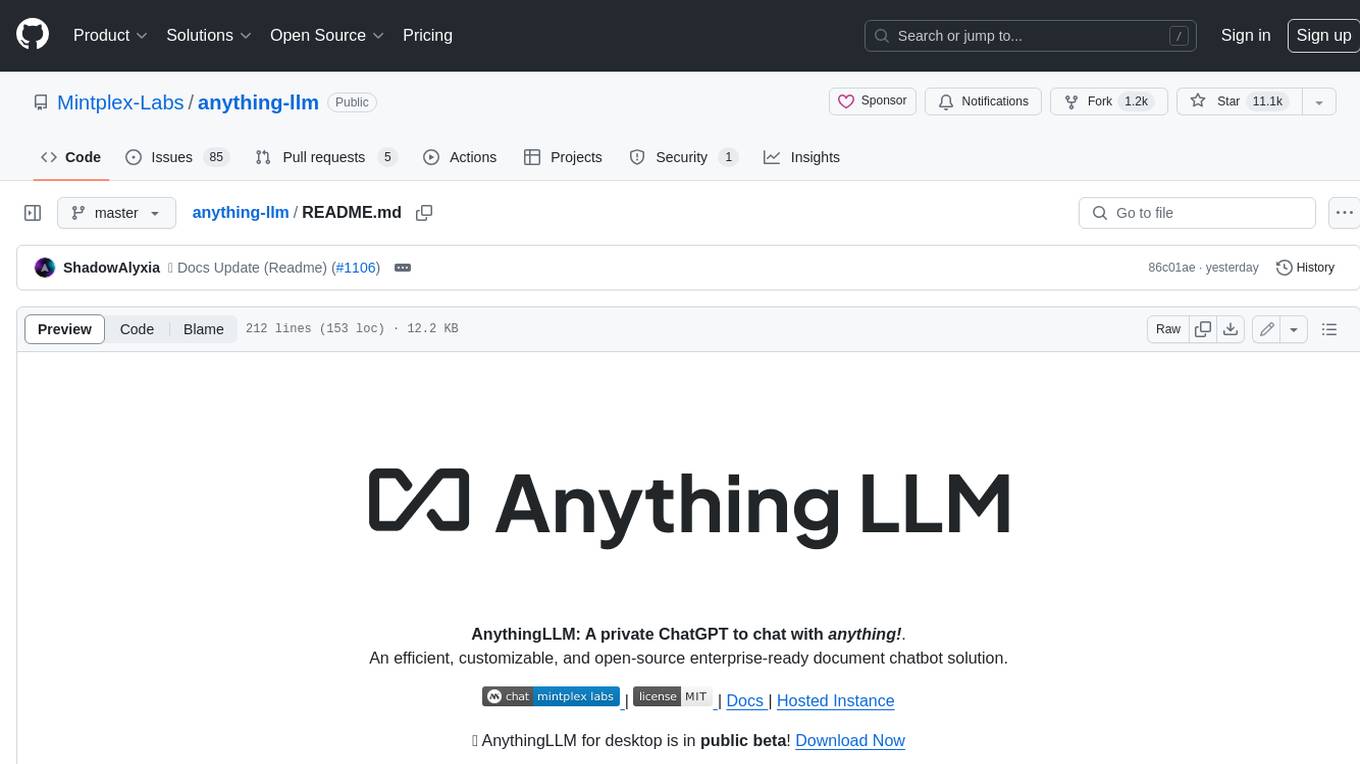
anything-llm
AnythingLLM is a full-stack application that enables you to turn any document, resource, or piece of content into context that any LLM can use as references during chatting. This application allows you to pick and choose which LLM or Vector Database you want to use as well as supporting multi-user management and permissions.
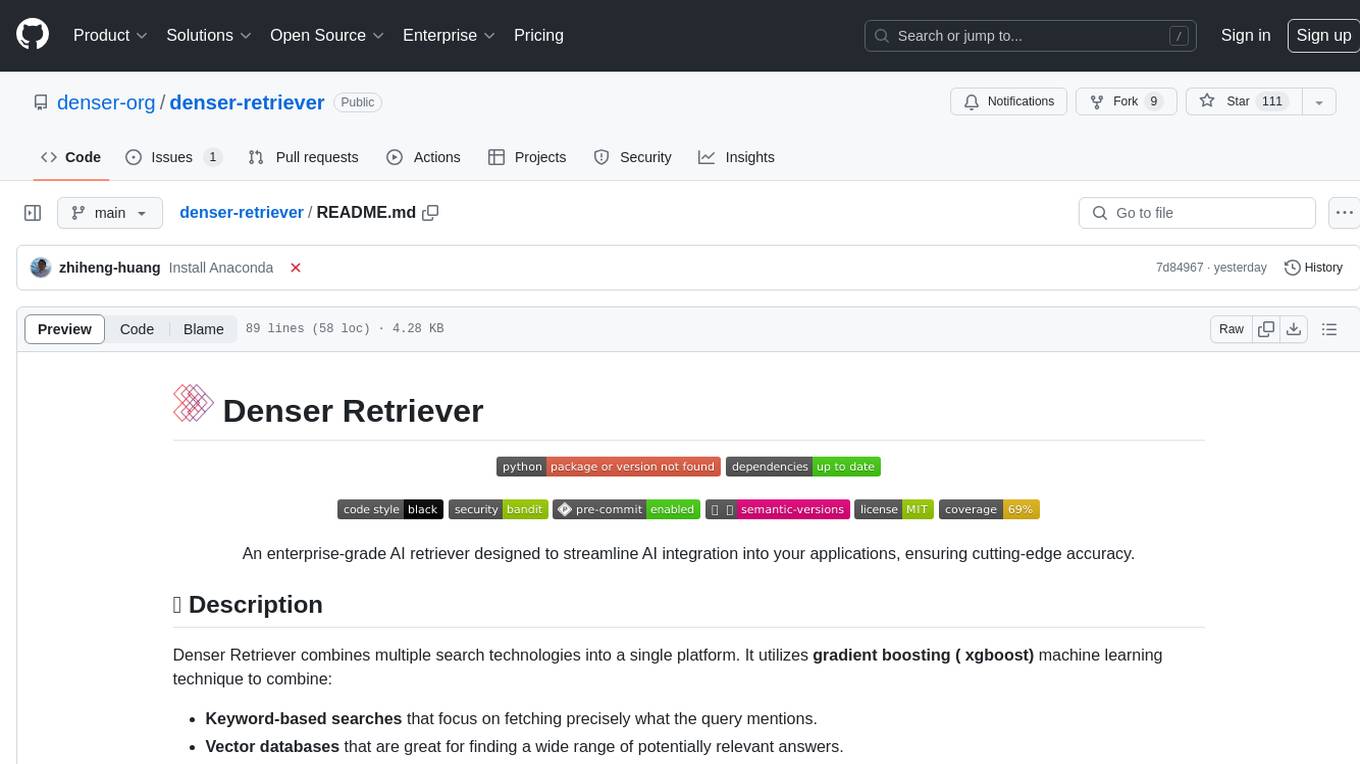
denser-retriever
Denser Retriever is an enterprise-grade AI retriever designed to streamline AI integration into applications, combining keyword-based searches, vector databases, and machine learning rerankers using xgboost. It provides state-of-the-art accuracy on MTEB Retrieval benchmarking and supports various heterogeneous retrievers for end-to-end applications like chatbots and semantic search.
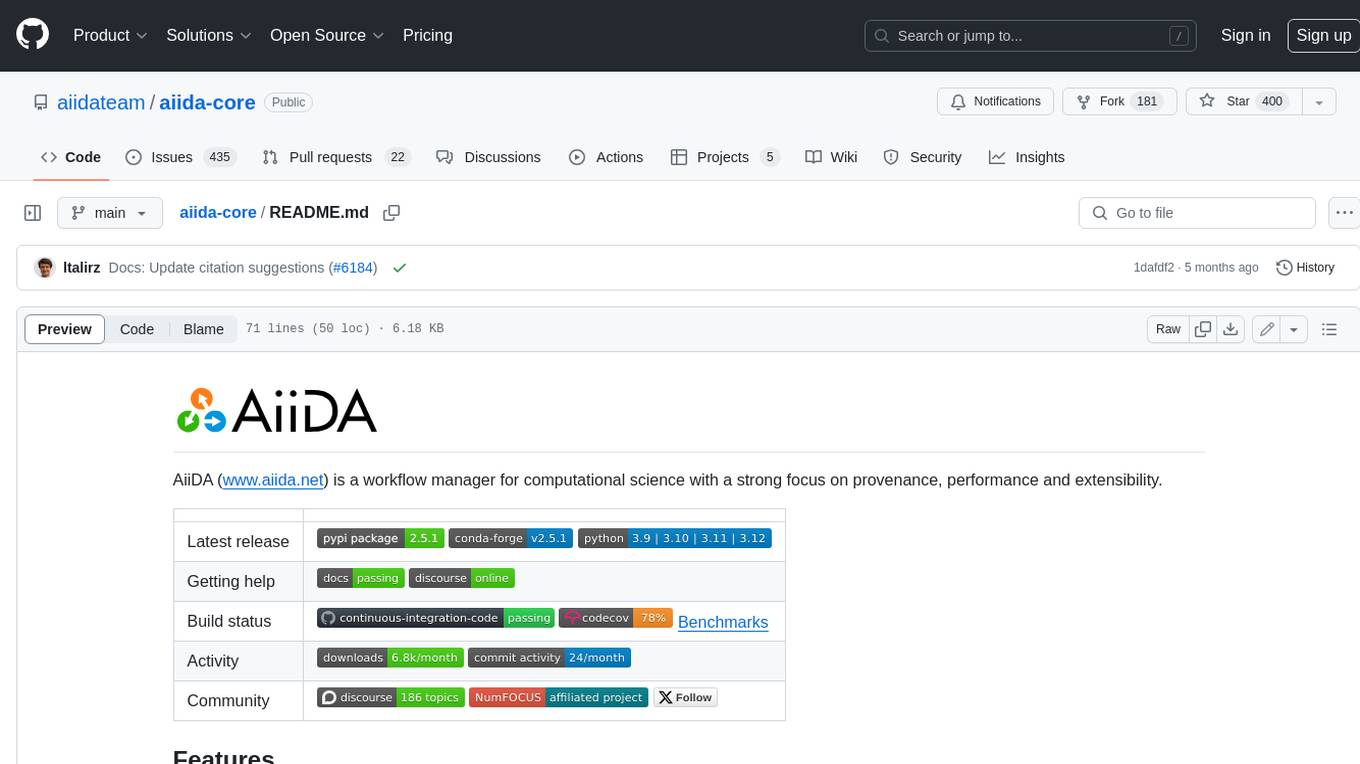
aiida-core
AiiDA (www.aiida.net) is a workflow manager for computational science with a strong focus on provenance, performance and extensibility. **Features** * **Workflows:** Write complex, auto-documenting workflows in python, linked to arbitrary executables on local and remote computers. The event-based workflow engine supports tens of thousands of processes per hour with full checkpointing. * **Data provenance:** Automatically track inputs, outputs & metadata of all calculations in a provenance graph for full reproducibility. Perform fast queries on graphs containing millions of nodes. * **HPC interface:** Move your calculations to a different computer by changing one line of code. AiiDA is compatible with schedulers like SLURM, PBS Pro, torque, SGE or LSF out of the box. * **Plugin interface:** Extend AiiDA with plugins for new simulation codes (input generation & parsing), data types, schedulers, transport modes and more. * **Open Science:** Export subsets of your provenance graph and share them with peers or make them available online for everyone on the Materials Cloud. * **Open source:** AiiDA is released under the MIT open source license
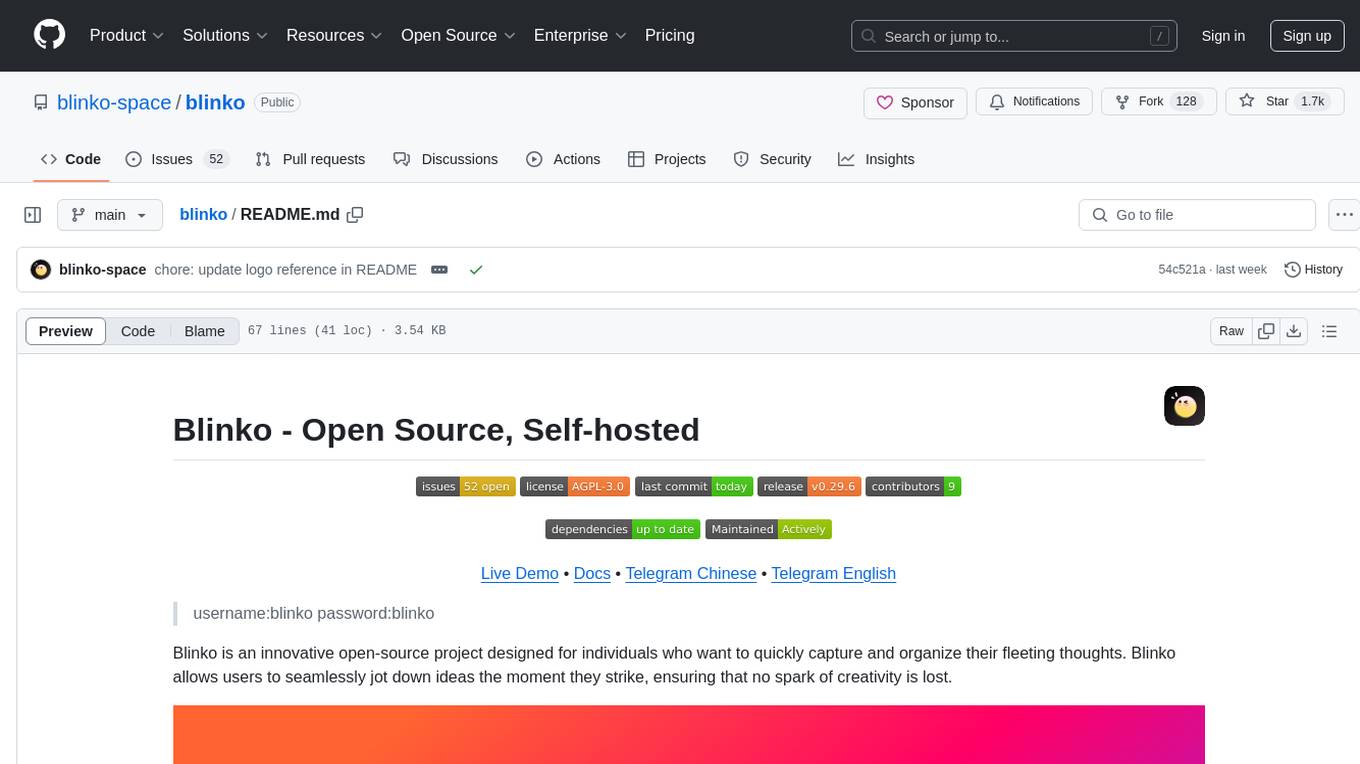
blinko
Blinko is an innovative open-source project designed for individuals who want to quickly capture and organize their fleeting thoughts. It allows users to seamlessly jot down ideas the moment they strike, ensuring that no spark of creativity is lost. With advanced AI-powered note retrieval, data ownership, efficient and fast capturing, lightweight architecture, and open collaboration, Blinko offers a comprehensive solution for managing and accessing notes.
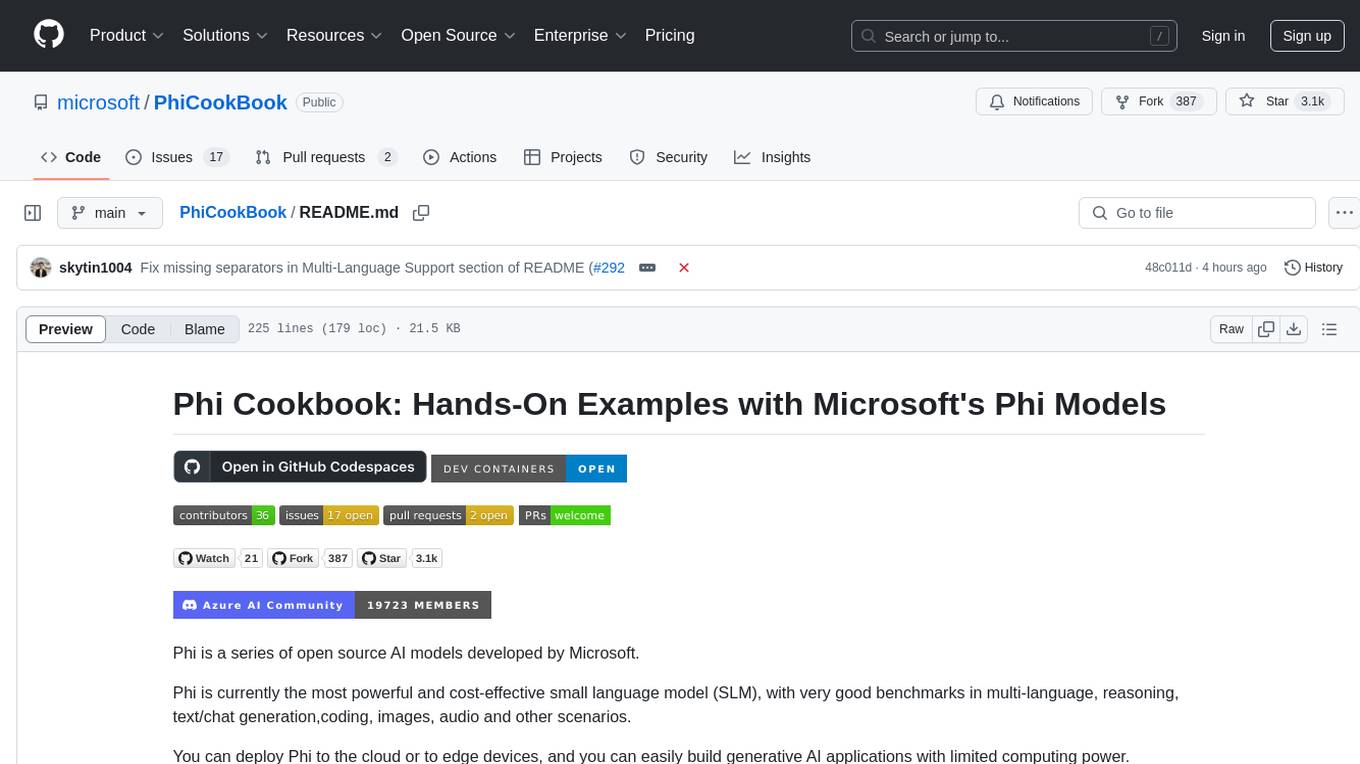
PhiCookBook
Phi Cookbook is a repository containing hands-on examples with Microsoft's Phi models, which are a series of open source AI models developed by Microsoft. Phi is currently the most powerful and cost-effective small language model with benchmarks in various scenarios like multi-language, reasoning, text/chat generation, coding, images, audio, and more. Users can deploy Phi to the cloud or edge devices to build generative AI applications with limited computing power.
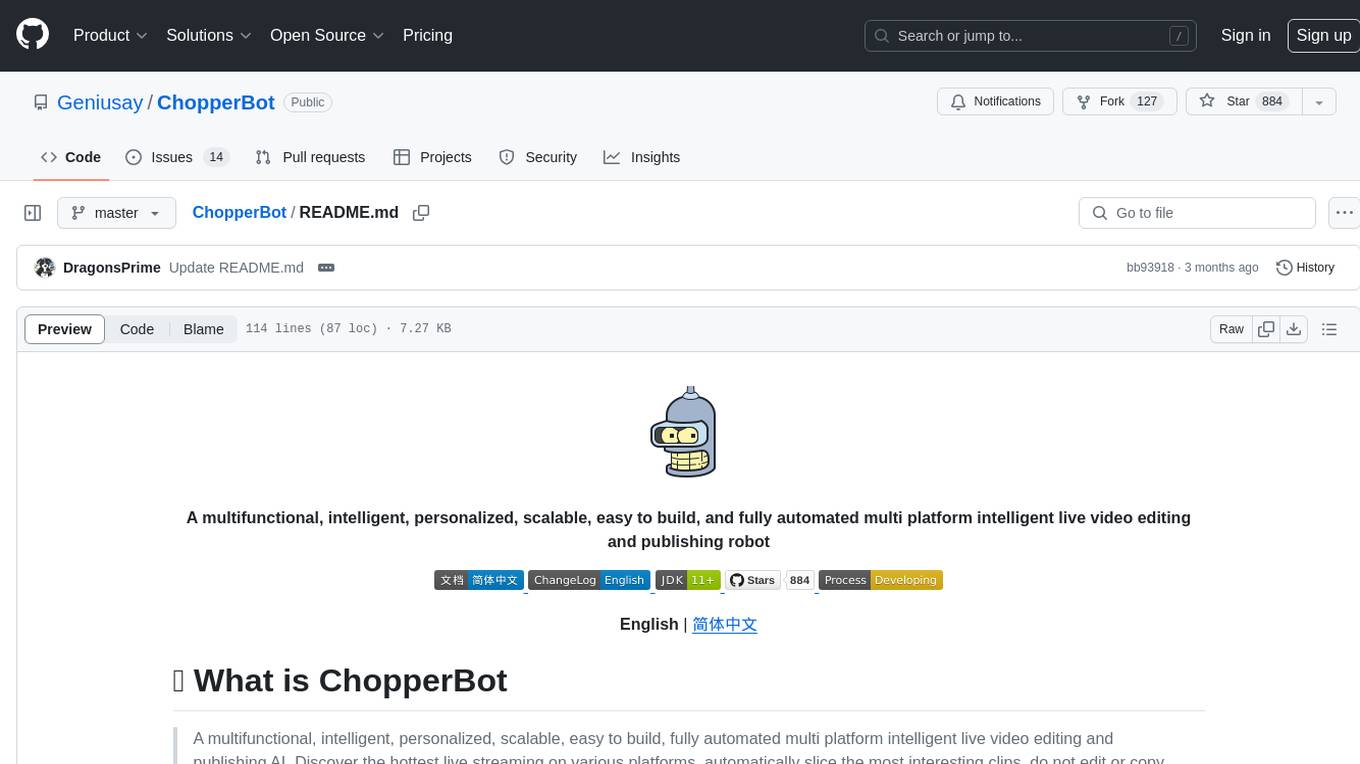
ChopperBot
A multifunctional, intelligent, personalized, scalable, easy to build, and fully automated multi platform intelligent live video editing and publishing robot. ChopperBot is a comprehensive AI tool that automatically analyzes and slices the most interesting clips from popular live streaming platforms, generates and publishes content, and manages accounts. It supports plugin DIY development and hot swapping functionality, making it easy to customize and expand. With ChopperBot, users can quickly build their own live video editing platform without the need to install any software, thanks to its visual management interface.
For similar tasks
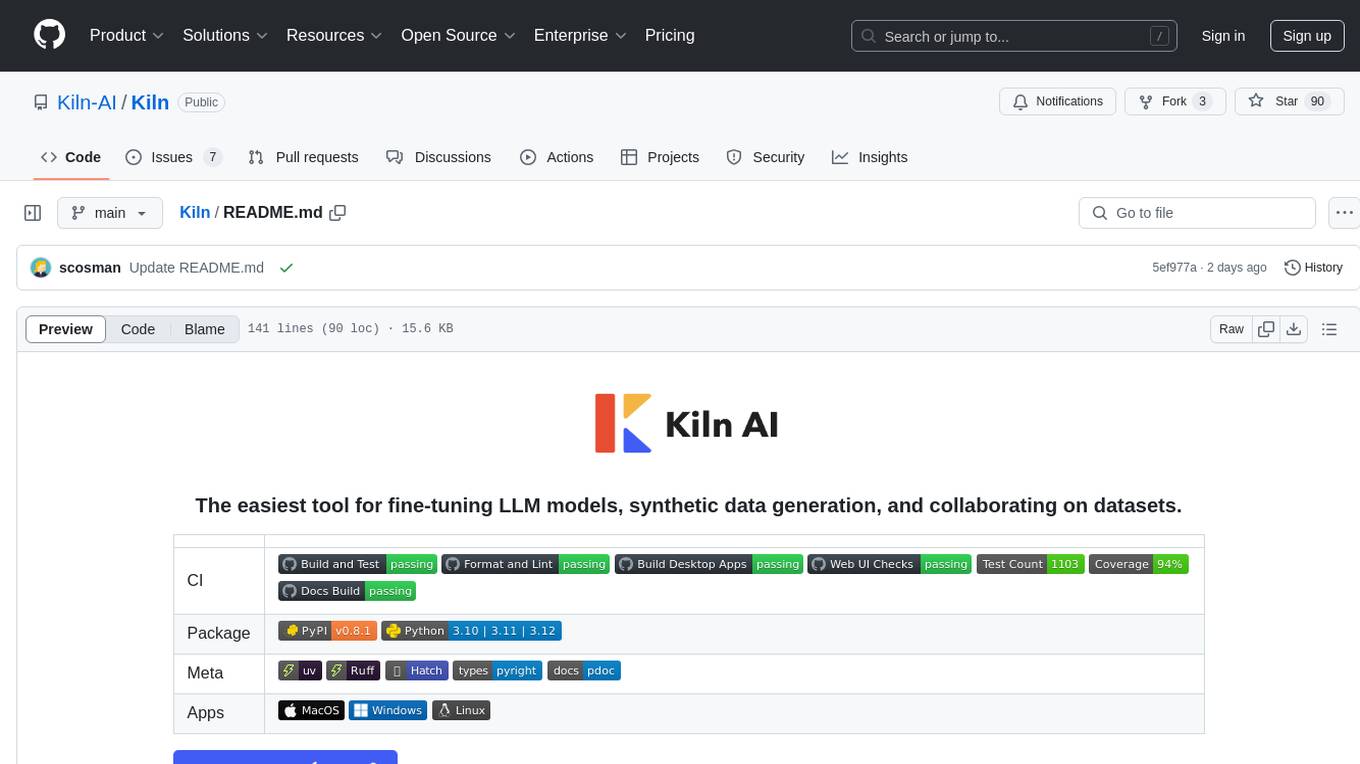
Kiln
Kiln is an intuitive tool for fine-tuning LLM models, generating synthetic data, and collaborating on datasets. It offers desktop apps for Windows, MacOS, and Linux, zero-code fine-tuning for various models, interactive data generation, and Git-based version control. Users can easily collaborate with QA, PM, and subject matter experts, generate auto-prompts, and work with a wide range of models and providers. The tool is open-source, privacy-first, and supports structured data tasks in JSON format. Kiln is free to use and helps build high-quality AI products with datasets, facilitates collaboration between technical and non-technical teams, allows comparison of models and techniques without code, ensures structured data integrity, and prioritizes user privacy.
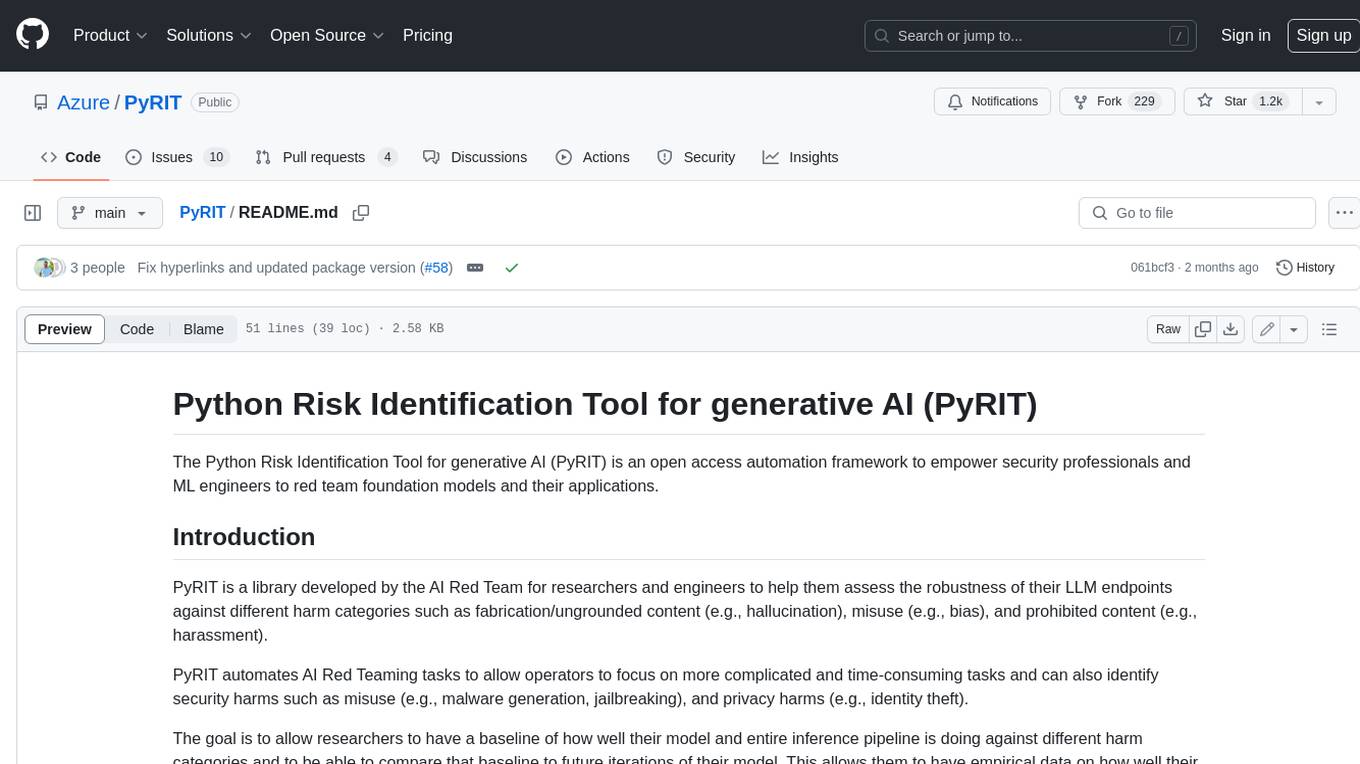
PyRIT
PyRIT is an open access automation framework designed to empower security professionals and ML engineers to red team foundation models and their applications. It automates AI Red Teaming tasks to allow operators to focus on more complicated and time-consuming tasks and can also identify security harms such as misuse (e.g., malware generation, jailbreaking), and privacy harms (e.g., identity theft). The goal is to allow researchers to have a baseline of how well their model and entire inference pipeline is doing against different harm categories and to be able to compare that baseline to future iterations of their model. This allows them to have empirical data on how well their model is doing today, and detect any degradation of performance based on future improvements.
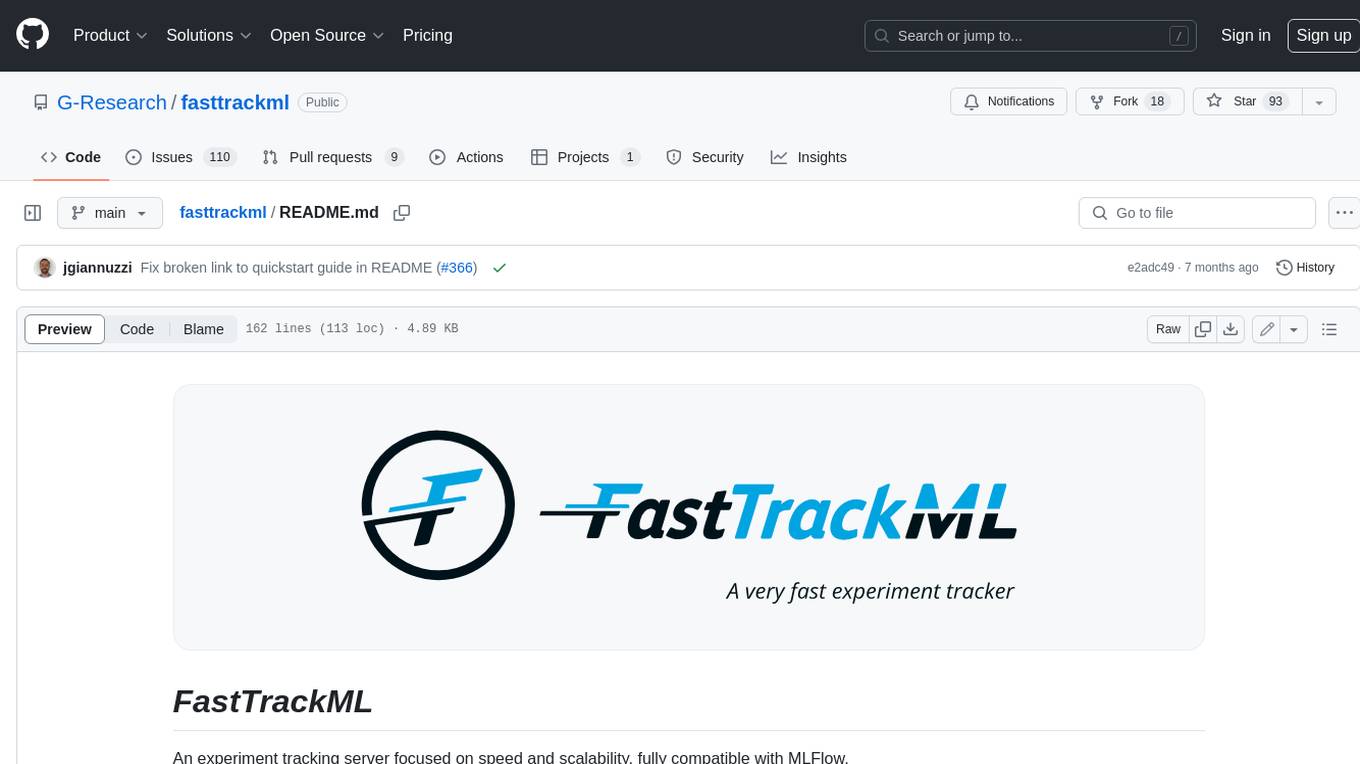
fasttrackml
FastTrackML is an experiment tracking server focused on speed and scalability, fully compatible with MLFlow. It provides a user-friendly interface to track and visualize your machine learning experiments, making it easy to compare different models and identify the best performing ones. FastTrackML is open source and can be easily installed and run with pip or Docker. It is also compatible with the MLFlow Python package, making it easy to integrate with your existing MLFlow workflows.
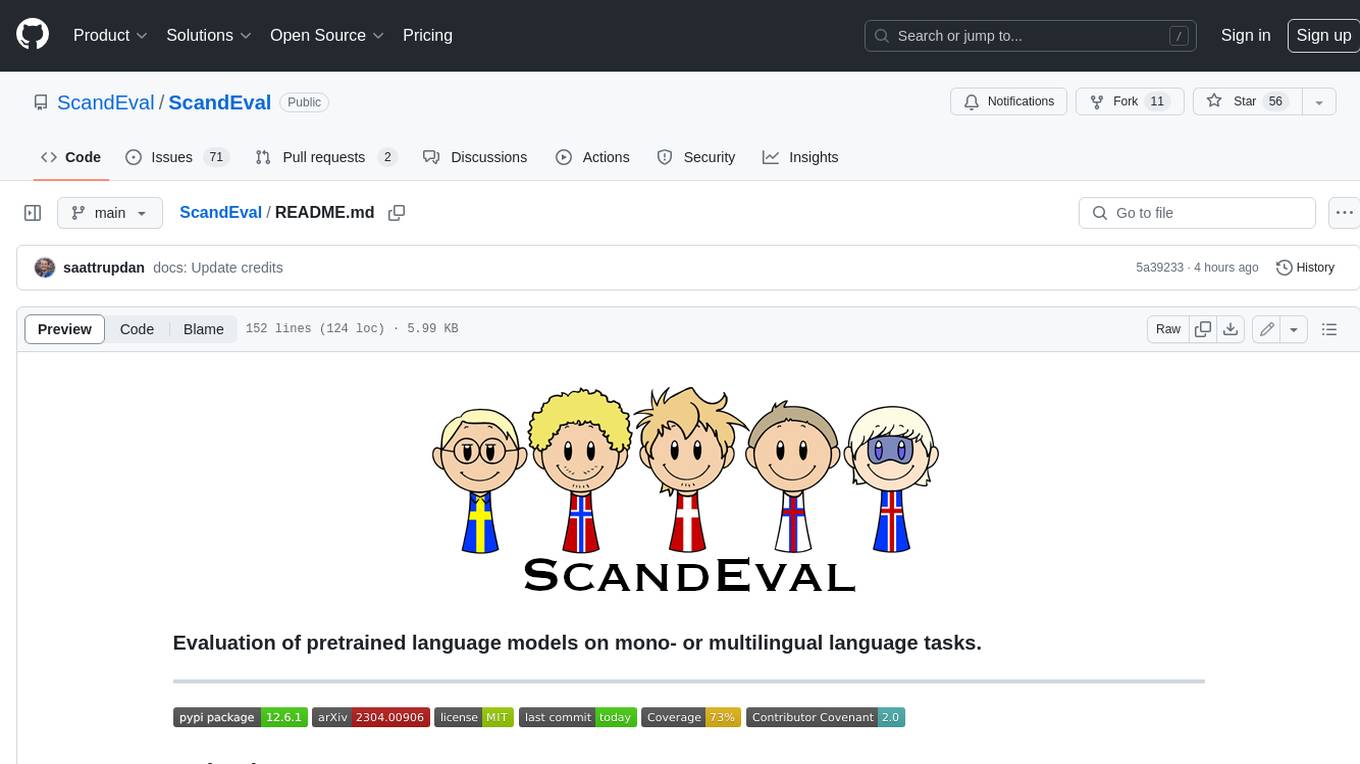
ScandEval
ScandEval is a framework for evaluating pretrained language models on mono- or multilingual language tasks. It provides a unified interface for benchmarking models on a variety of tasks, including sentiment analysis, question answering, and machine translation. ScandEval is designed to be easy to use and extensible, making it a valuable tool for researchers and practitioners alike.
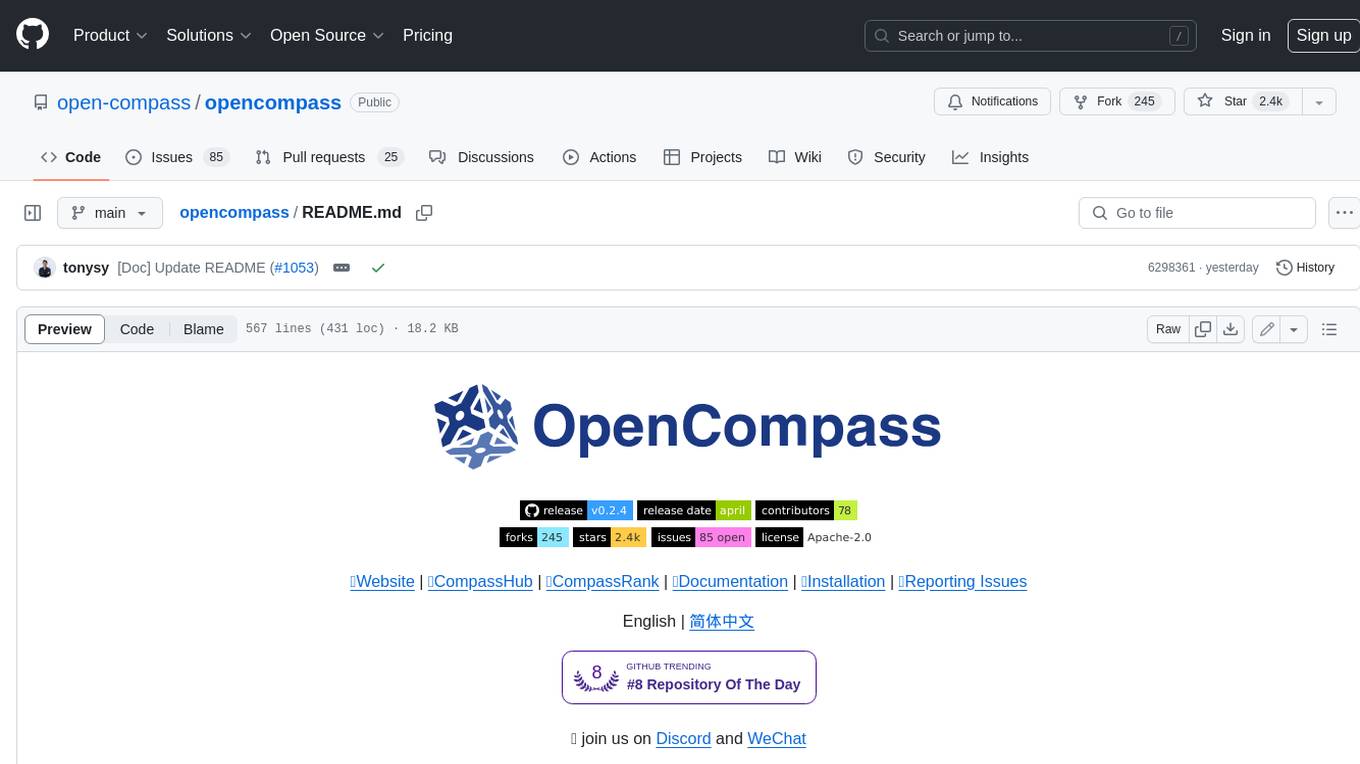
opencompass
OpenCompass is a one-stop platform for large model evaluation, aiming to provide a fair, open, and reproducible benchmark for large model evaluation. Its main features include: * Comprehensive support for models and datasets: Pre-support for 20+ HuggingFace and API models, a model evaluation scheme of 70+ datasets with about 400,000 questions, comprehensively evaluating the capabilities of the models in five dimensions. * Efficient distributed evaluation: One line command to implement task division and distributed evaluation, completing the full evaluation of billion-scale models in just a few hours. * Diversified evaluation paradigms: Support for zero-shot, few-shot, and chain-of-thought evaluations, combined with standard or dialogue-type prompt templates, to easily stimulate the maximum performance of various models. * Modular design with high extensibility: Want to add new models or datasets, customize an advanced task division strategy, or even support a new cluster management system? Everything about OpenCompass can be easily expanded! * Experiment management and reporting mechanism: Use config files to fully record each experiment, and support real-time reporting of results.
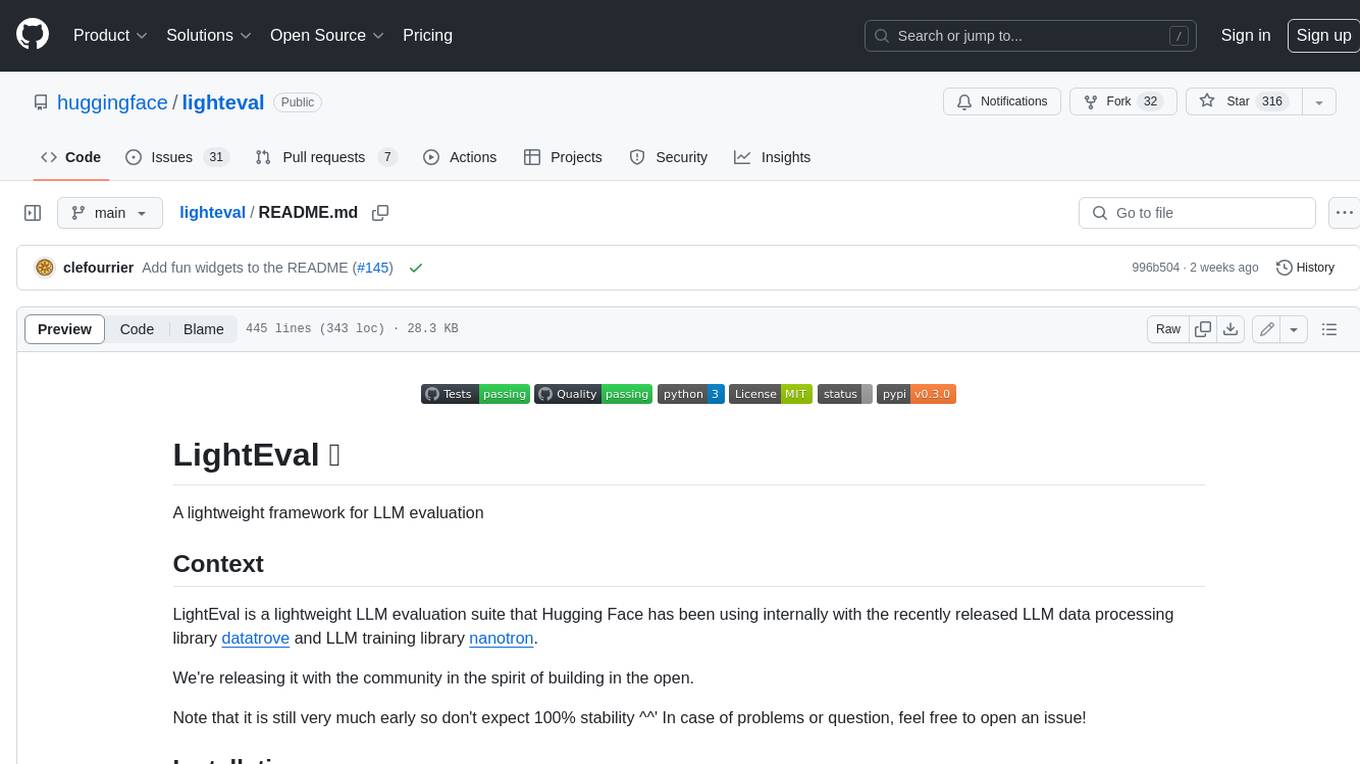
lighteval
LightEval is a lightweight LLM evaluation suite that Hugging Face has been using internally with the recently released LLM data processing library datatrove and LLM training library nanotron. We're releasing it with the community in the spirit of building in the open. Note that it is still very much early so don't expect 100% stability ^^' In case of problems or question, feel free to open an issue!
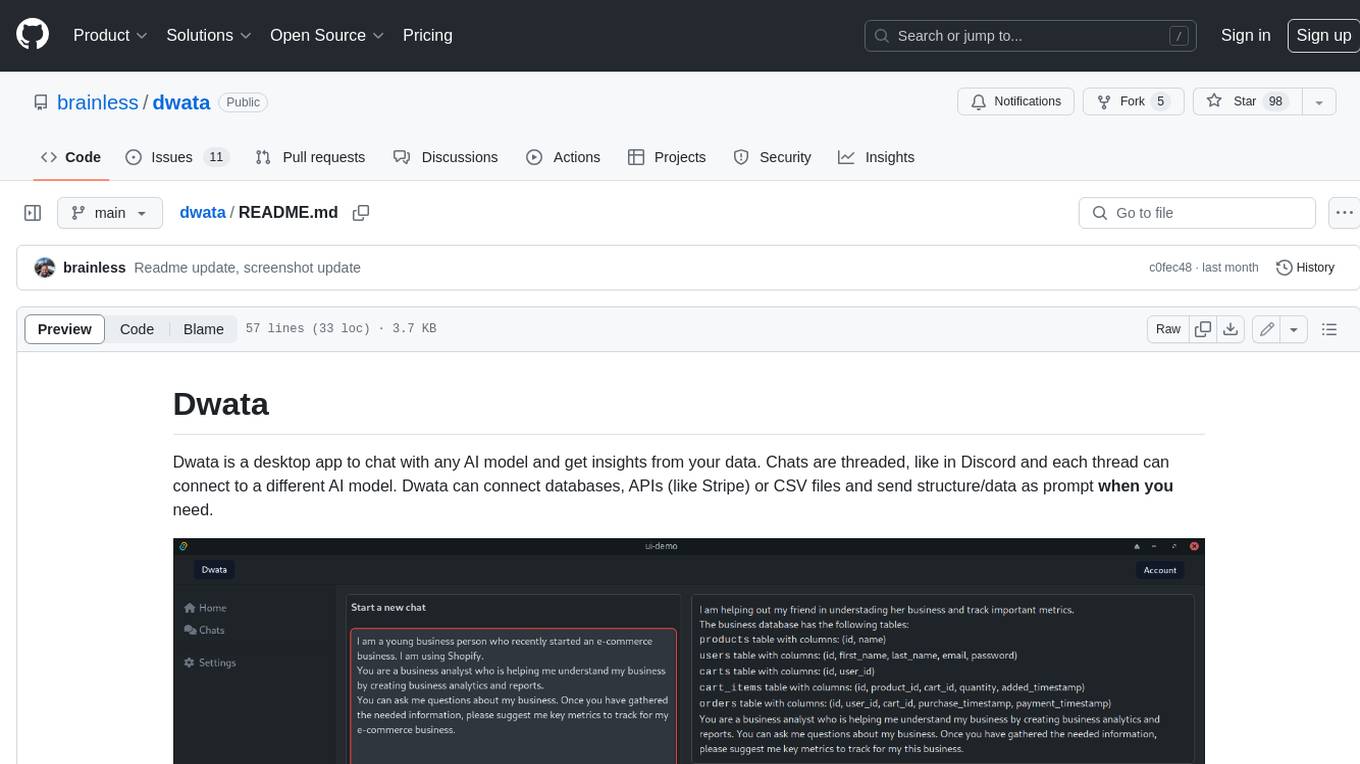
dwata
Dwata is a desktop application that allows users to chat with any AI model and gain insights from their data. Chats are organized into threads, similar to Discord, with each thread connecting to a different AI model. Dwata can connect to databases, APIs (such as Stripe), or CSV files and send structured data as prompts when needed. The AI's response will often include SQL or Python code, which can be used to extract the desired insights. Dwata can validate AI-generated SQL to ensure that the tables and columns referenced are correct and can execute queries against the database from within the application. Python code (typically using Pandas) can also be executed from within Dwata, although this feature is still in development. Dwata supports a range of AI models, including OpenAI's GPT-4, GPT-4 Turbo, and GPT-3.5 Turbo; Groq's LLaMA2-70b and Mixtral-8x7b; Phind's Phind-34B and Phind-70B; Anthropic's Claude; and Ollama's Llama 2, Mistral, and Phi-2 Gemma. Dwata can compare chats from different models, allowing users to see the responses of multiple models to the same prompts. Dwata can connect to various data sources, including databases (PostgreSQL, MySQL, MongoDB), SaaS products (Stripe, Shopify), CSV files/folders, and email (IMAP). The desktop application does not collect any private or business data without the user's explicit consent.
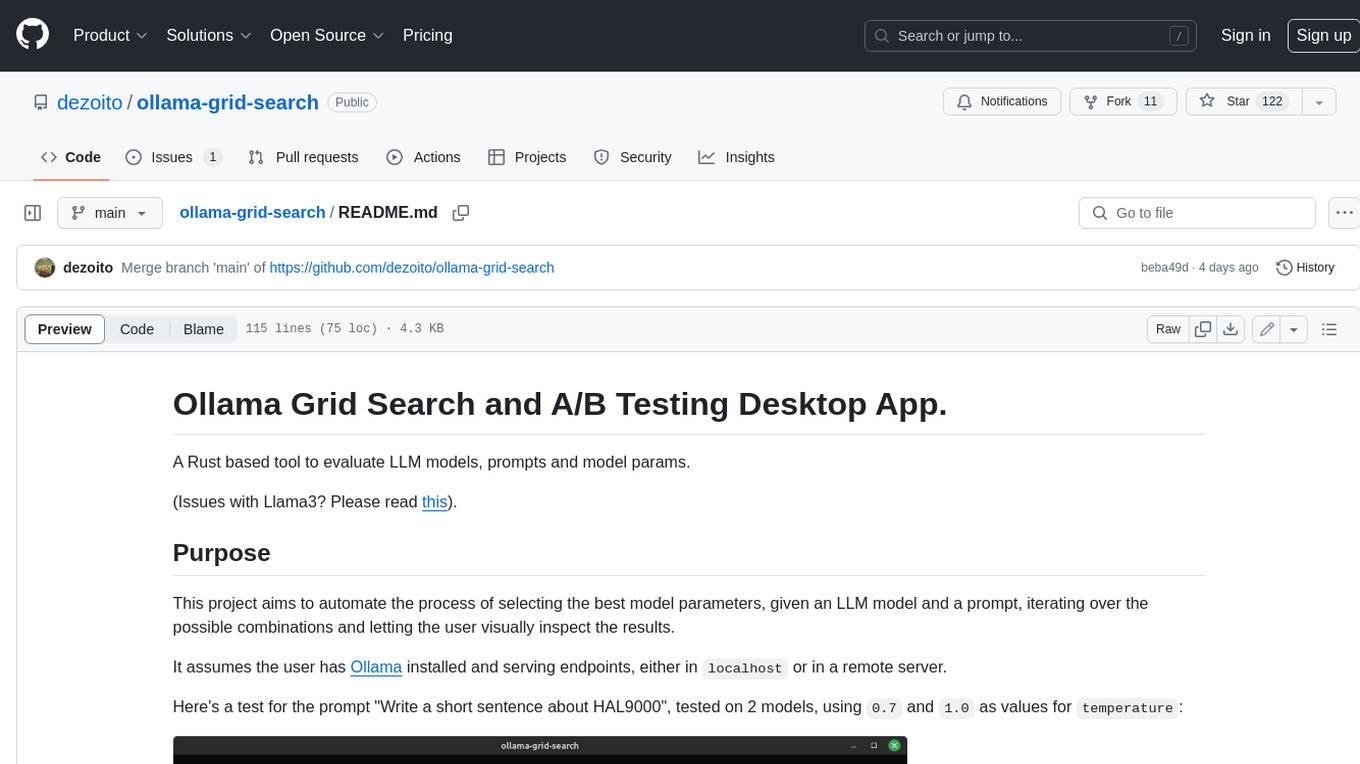
ollama-grid-search
A Rust based tool to evaluate LLM models, prompts and model params. It automates the process of selecting the best model parameters, given an LLM model and a prompt, iterating over the possible combinations and letting the user visually inspect the results. The tool assumes the user has Ollama installed and serving endpoints, either in `localhost` or in a remote server. Key features include: * Automatically fetches models from local or remote Ollama servers * Iterates over different models and params to generate inferences * A/B test prompts on different models simultaneously * Allows multiple iterations for each combination of parameters * Makes synchronous inference calls to avoid spamming servers * Optionally outputs inference parameters and response metadata (inference time, tokens and tokens/s) * Refetching of individual inference calls * Model selection can be filtered by name * List experiments which can be downloaded in JSON format * Configurable inference timeout * Custom default parameters and system prompts can be defined in settings
For similar jobs
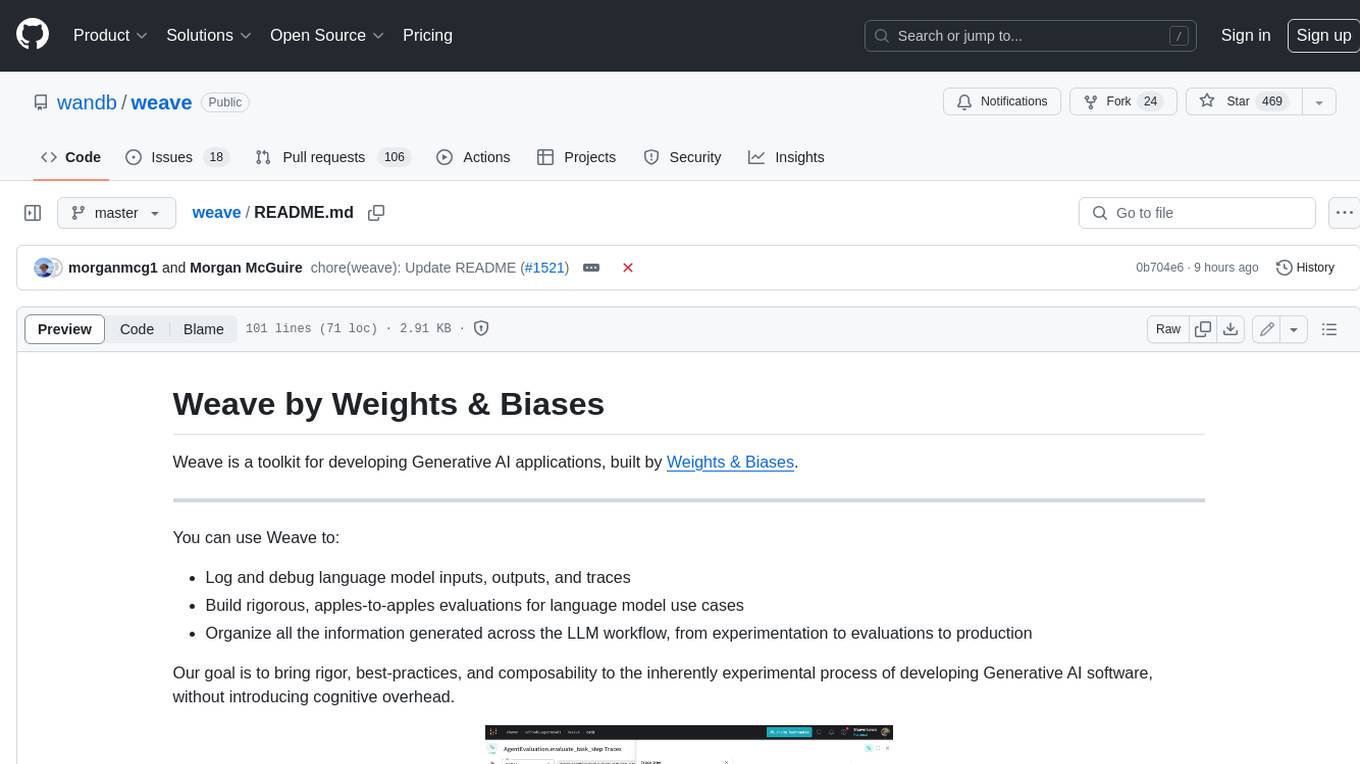
weave
Weave is a toolkit for developing Generative AI applications, built by Weights & Biases. With Weave, you can log and debug language model inputs, outputs, and traces; build rigorous, apples-to-apples evaluations for language model use cases; and organize all the information generated across the LLM workflow, from experimentation to evaluations to production. Weave aims to bring rigor, best-practices, and composability to the inherently experimental process of developing Generative AI software, without introducing cognitive overhead.
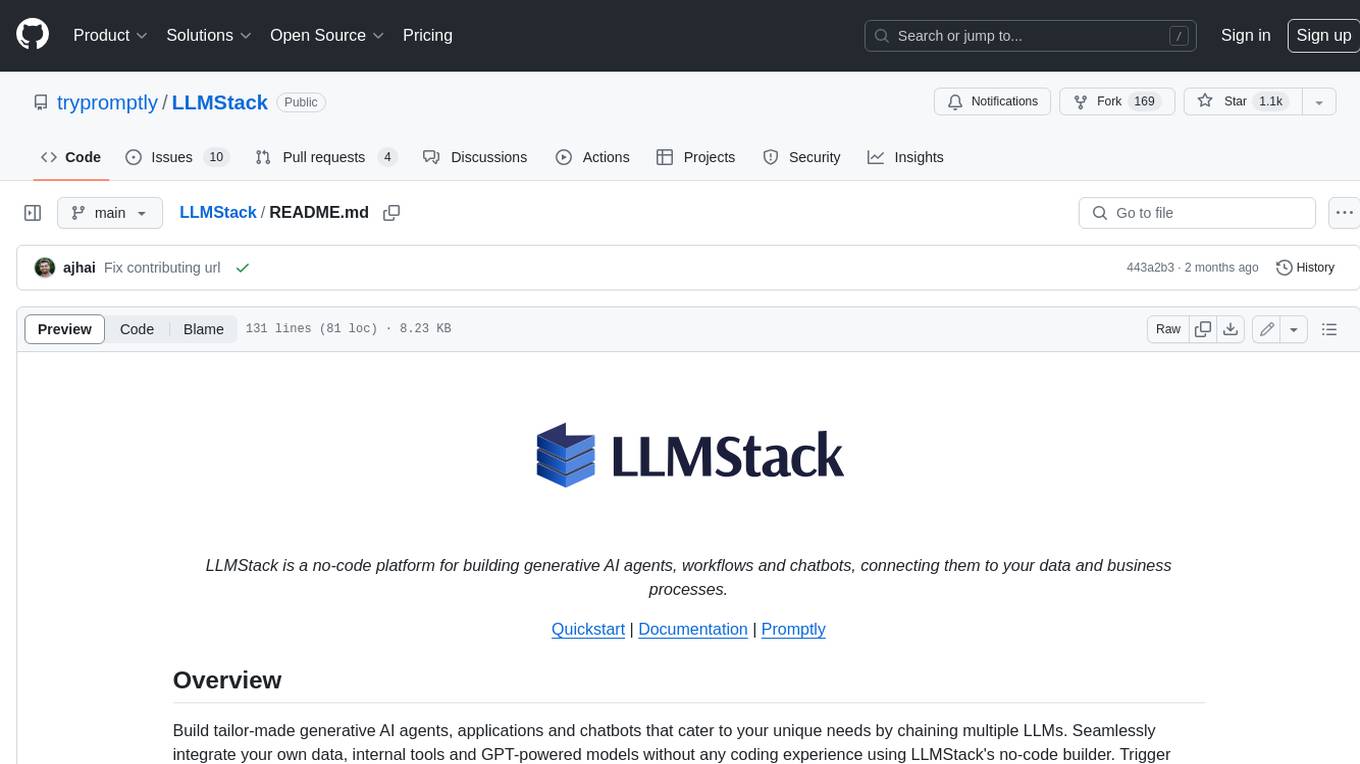
LLMStack
LLMStack is a no-code platform for building generative AI agents, workflows, and chatbots. It allows users to connect their own data, internal tools, and GPT-powered models without any coding experience. LLMStack can be deployed to the cloud or on-premise and can be accessed via HTTP API or triggered from Slack or Discord.
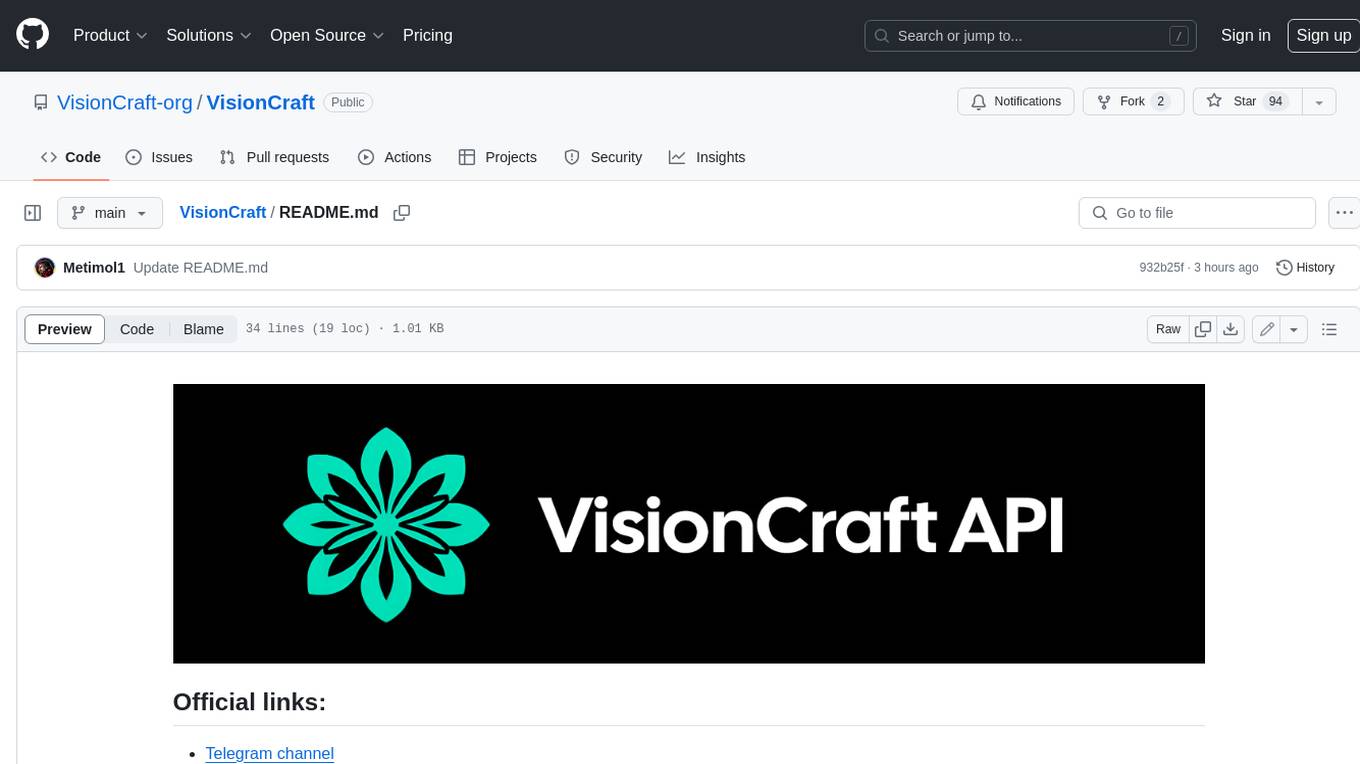
VisionCraft
The VisionCraft API is a free API for using over 100 different AI models. From images to sound.
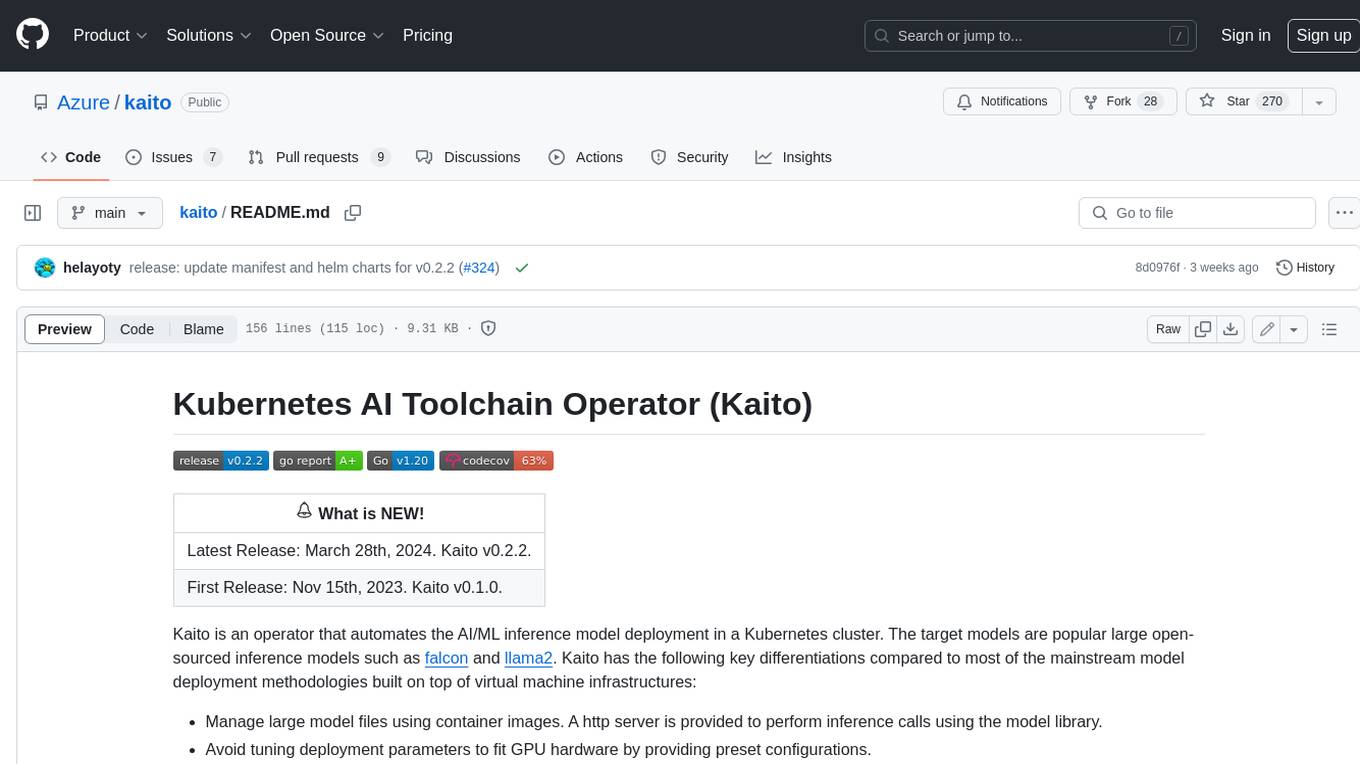
kaito
Kaito is an operator that automates the AI/ML inference model deployment in a Kubernetes cluster. It manages large model files using container images, avoids tuning deployment parameters to fit GPU hardware by providing preset configurations, auto-provisions GPU nodes based on model requirements, and hosts large model images in the public Microsoft Container Registry (MCR) if the license allows. Using Kaito, the workflow of onboarding large AI inference models in Kubernetes is largely simplified.
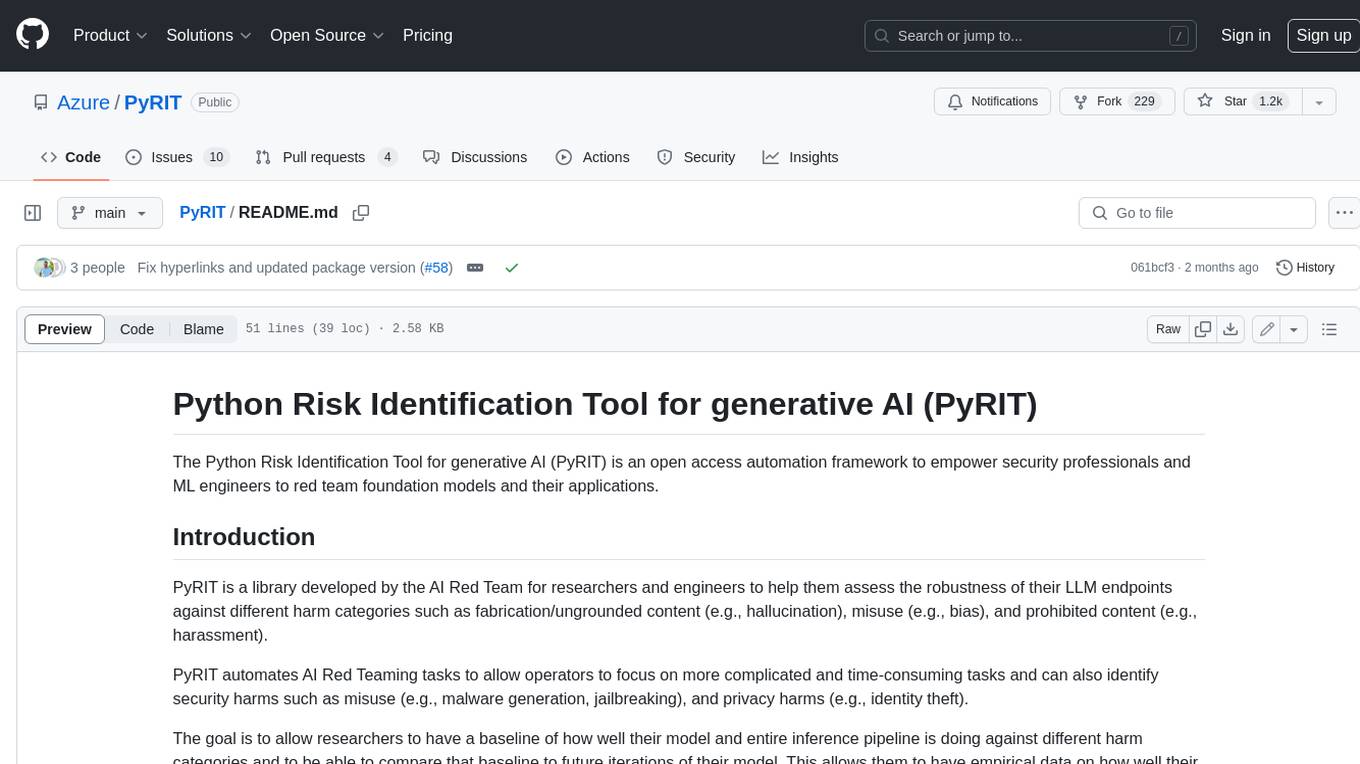
PyRIT
PyRIT is an open access automation framework designed to empower security professionals and ML engineers to red team foundation models and their applications. It automates AI Red Teaming tasks to allow operators to focus on more complicated and time-consuming tasks and can also identify security harms such as misuse (e.g., malware generation, jailbreaking), and privacy harms (e.g., identity theft). The goal is to allow researchers to have a baseline of how well their model and entire inference pipeline is doing against different harm categories and to be able to compare that baseline to future iterations of their model. This allows them to have empirical data on how well their model is doing today, and detect any degradation of performance based on future improvements.
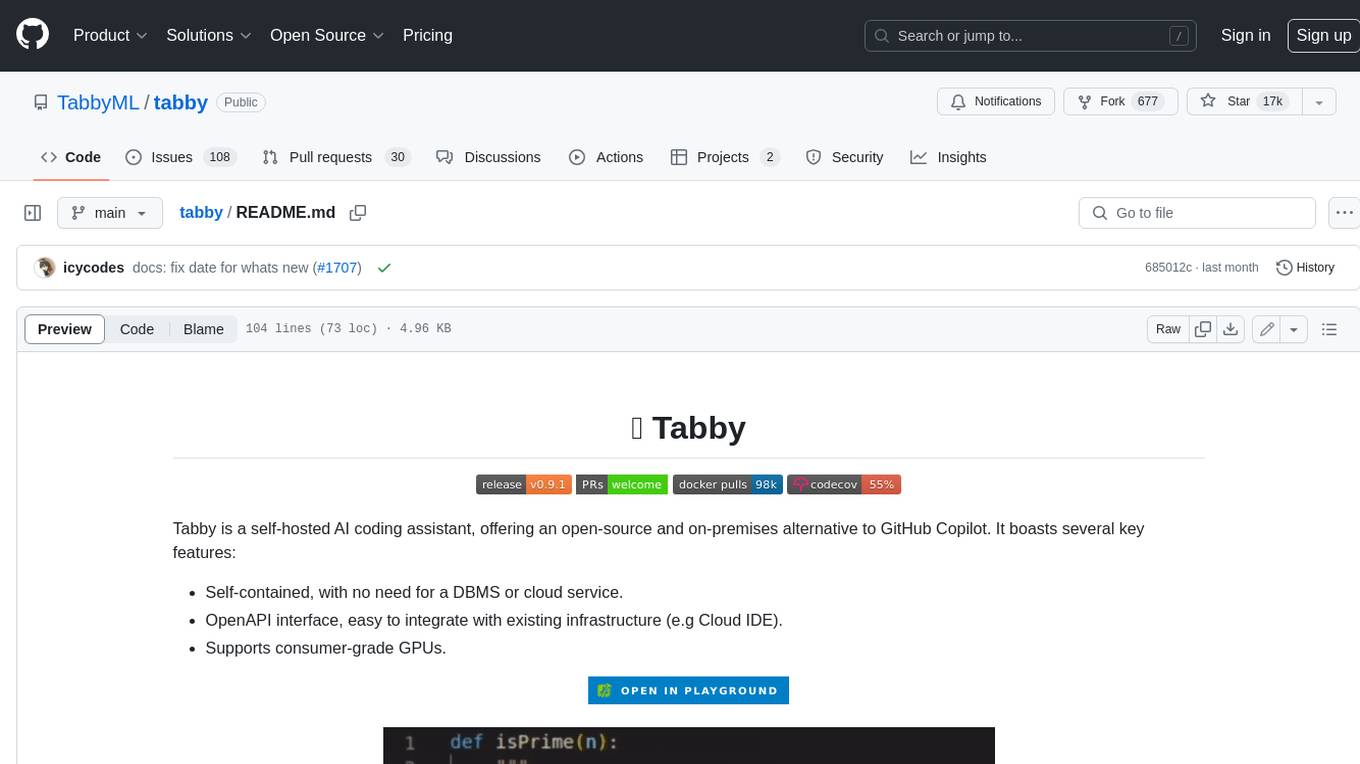
tabby
Tabby is a self-hosted AI coding assistant, offering an open-source and on-premises alternative to GitHub Copilot. It boasts several key features: * Self-contained, with no need for a DBMS or cloud service. * OpenAPI interface, easy to integrate with existing infrastructure (e.g Cloud IDE). * Supports consumer-grade GPUs.
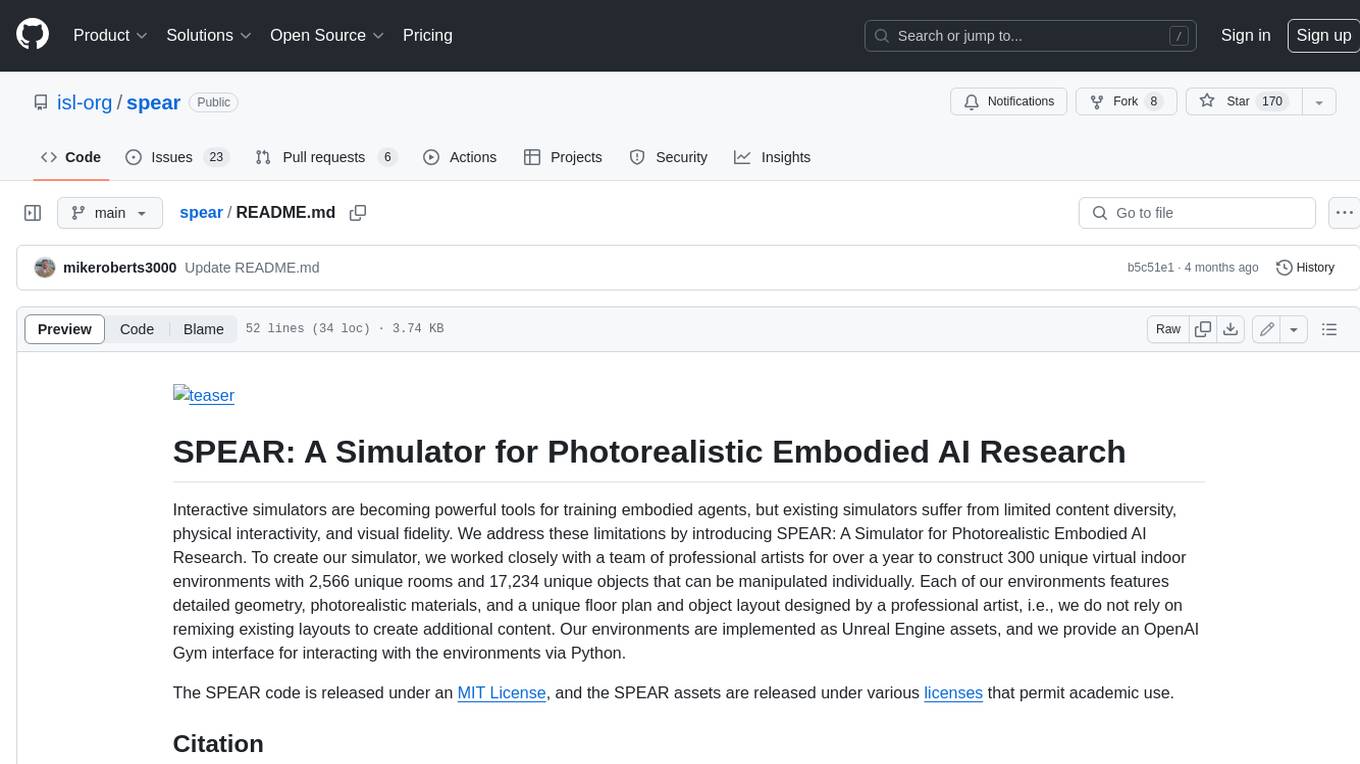
spear
SPEAR (Simulator for Photorealistic Embodied AI Research) is a powerful tool for training embodied agents. It features 300 unique virtual indoor environments with 2,566 unique rooms and 17,234 unique objects that can be manipulated individually. Each environment is designed by a professional artist and features detailed geometry, photorealistic materials, and a unique floor plan and object layout. SPEAR is implemented as Unreal Engine assets and provides an OpenAI Gym interface for interacting with the environments via Python.
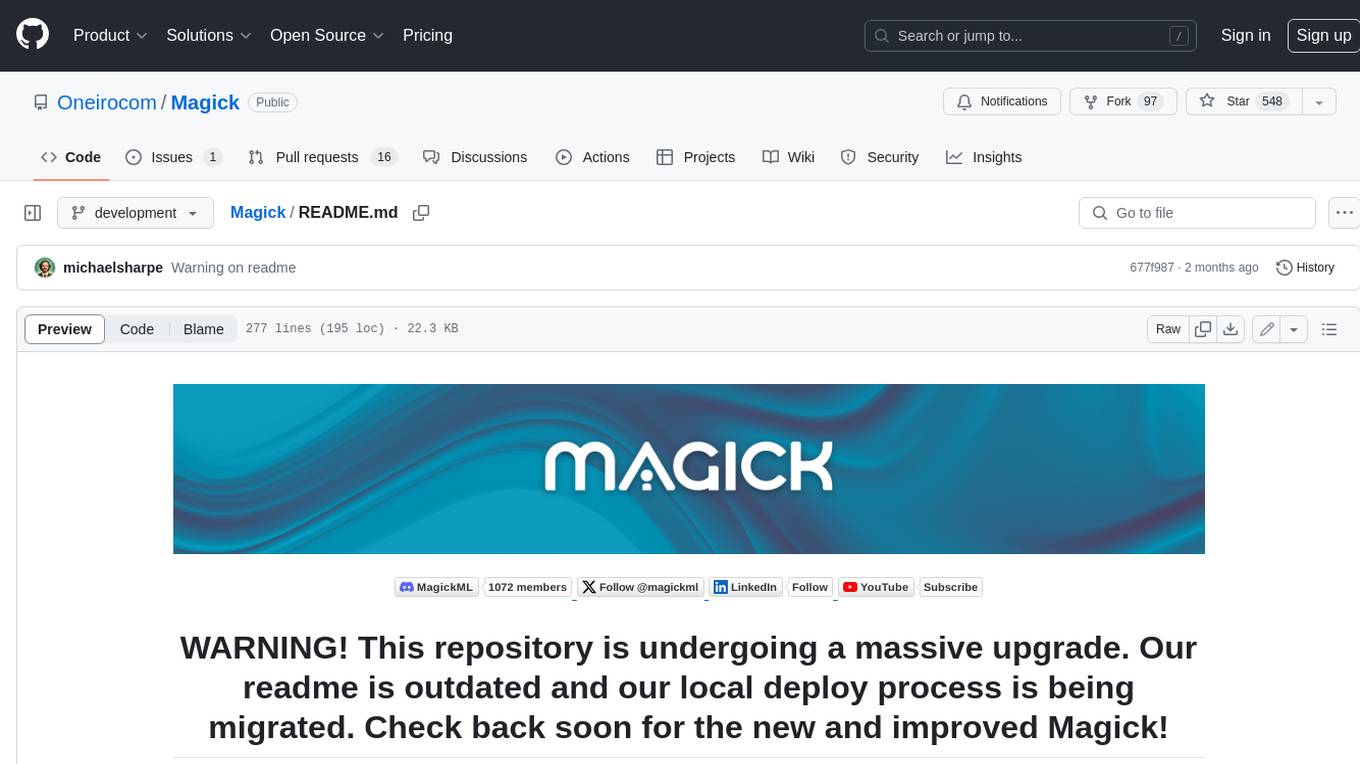
Magick
Magick is a groundbreaking visual AIDE (Artificial Intelligence Development Environment) for no-code data pipelines and multimodal agents. Magick can connect to other services and comes with nodes and templates well-suited for intelligent agents, chatbots, complex reasoning systems and realistic characters.