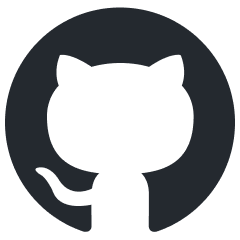
Knowledge-Conflicts-Survey
The official GitHub repo for the survey paper "Knowledge Conflicts for LLMs: A Survey"
Stars: 64
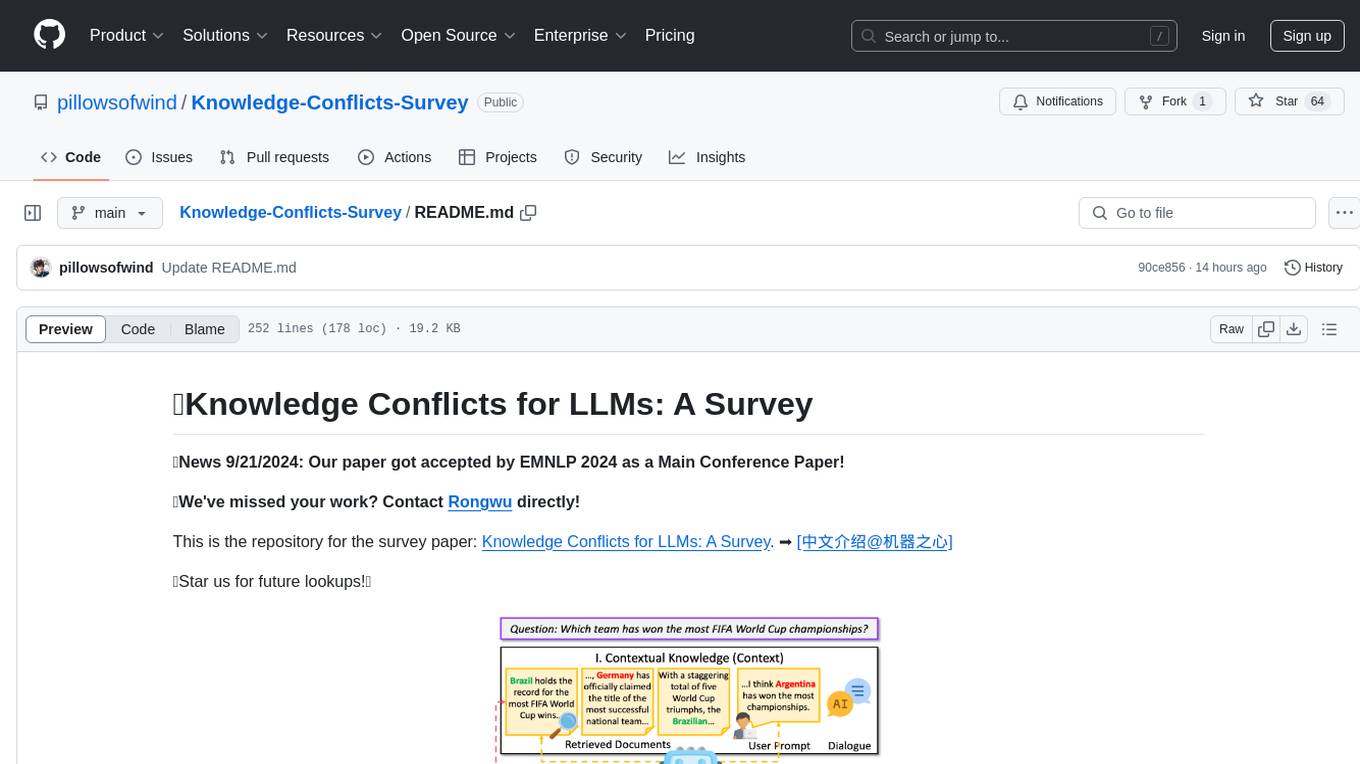
Knowledge Conflicts for LLMs: A Survey is a repository containing a survey paper that investigates three types of knowledge conflicts: context-memory conflict, inter-context conflict, and intra-memory conflict within Large Language Models (LLMs). The survey reviews the causes, behaviors, and possible solutions to these conflicts, providing a comprehensive analysis of the literature in this area. The repository includes detailed information on the types of conflicts, their causes, behavior analysis, and mitigating solutions, offering insights into how conflicting knowledge affects LLMs and how to address these conflicts.
README:
📢News 9/21/2024: Our paper got accepted by EMNLP 2024 as a Main Conference Paper!
📢We've missed your work? Contact Rongwu directly!
This is the repository for the survey paper: Knowledge Conflicts for LLMs: A Survey. ➡️ [中文介绍@机器之心]
🌟Star us for future lookups!🌟
Rongwu Xu1*, Zehan Qi1*, Zhijiang Guo2, Cunxiang Wang3, Hongru Wang4, Yue Zhang3 and Wei Xu1
1. Tsinghua University; 2. University of Cambridge; 3. Westlake University; 4. The Chinese University of Hong Kong
(* Equal Contribution)
If you find our survey useful, please consider citing:
@article{xu2024knowledge,
title={Knowledge Conflicts for LLMs: A Survey},
author={Xu, Rongwu and Qi, Zehan and Wang, Cunxiang and Wang, Hongru and Zhang, Yue and Xu, Wei},
journal={arXiv preprint arXiv:2403.08319},
year={2024}
}
We investigate three types of knowledge conflicts: context-memory conflict, inter-context conflict, and intra-memory conflict.
- Context-memory conflict: Contextual knowledge (context) can conflict with the parametric knowledge (memory) encapsulated within the LLM's parameters.
- Inter-context conflict: Conflict among various pieces of contextual knowledge (e.g., noise, outdated information, misinformation, etc.).
- Intra-memory conflict: LLM's parametric knowledge may yield divergent responses to differently phrased queries, which can be attributed to the conflicting knowledge embedded within the LLM's parameters.
This survey reviews the literature on the causes, behaviors, and possible solutions to knowledge conflicts.
Taxonomy of knowledge conflicts: we consider three distinct types of conflicts and analysis causes, behaviors, and solutions.
- Mind the gap: Assessing temporal generalization in neural language models, Lazaridou et al., Neurips 2021.[Paper]
- Time Waits for No One! Analysis and Challenges of Temporal Misalignment, Luu et al., NAACL 2022. [Paper]
- Time-aware language models as temporal knowledge bases, Dhingra et al., TACL 2022.[Paper]
- Towards continual knowledge learning of language models, Jang et al., ICLR 2022, [Paper]
- Temporalwiki: A lifelong benchmark for training and evaluating ever-evolving language models, Jang et al., EMNLP 2023, [Paper]
- Streamingqa: A benchmark for adaptation to new knowledge over time in question answering models, Liska et al., ICML 2022. [Paper]
- Can LMs Generalize to Future Data? An Empirical Analysis on Text Summarization, Cheang et al., EMNLP 2023, [Paper]
- RealTime QA: What's the Answer Right Now?, Kasai et al., Neurips 2024, [Paper]
- Attacking open-domain question answering by injecting misinformation, Pan et al., AACL 2023, [Paper]
- On the risk of misinformation pollution with large language models, Pan et al., EMNLP 2023, [Paper]
- Defending against misinformation attacks in open-domain question answering, Weller et al., EACL 2024, [Paper]
- The earth is flat because...: Investigating llms’ belief towards misinformation via persuasive conversation, Xu et al., ACL 2024, [Paper]
- Prompt injection attack against llm-integrated applications, Liu et al., arXiv 2024, [Paper]
- Benchmarking and defending against indirect prompt injection attacks on large language models, Yi et al., arXiv 2024, [Paper]
- Adaptive chameleon or stubborn sloth: Unraveling the behavior of large language models in knowledge conflicts, Xie et al., ICLR 2024, [Paper]
- Poisoning web-scale training datasets is practical, Carlini et al., S&P 2024, [Paper]
- Can llm-generated misinformation be detected, Chen and Shu, ICLR 2024, [Paper]
- Entity-Based Knowledge Conflicts in Question Answering, Longpre et al., EMNLP 2021, [Paper]
- Rich Knowledge Sources Bring Complex Knowledge Conflicts: Recalibrating Models to Reflect Conflicting Evidence, Chen et al., EMNLP 2022, [Paper]
- Blinded by Generated Contexts: How Language Models Merge Generated and Retrieved Contexts When Knowledge Conflicts, Tan et al., arXiv 2024, [Paper]
- Adaptive chameleon or stubborn sloth: Unraveling the behavior of large language models in knowledge conflicts, Xie et al., ICLR 2024, [Paper]
- RESOLVING KNOWLEDGE CONFLICTS IN LARGE LANGUAGE MODELS, Wang et al., arXiv 2023, [Paper]
- Intuitive or Dependent? Investigating LLMs’ Behavior Style to Conflicting Prompts, Ying et al., arXiv 2024, [Paper]
- “Merge Conflicts!” Exploring the Impacts of External Distractors to Parametric Knowledge Graphs, Qian et al., arXiv 2023, [Paper]
- Studying Large Language Model Behaviors Under Realistic Knowledge Conflicts, arXiv 2024, [Paper]
- Characterizing mechanisms for factual recall in language models, EMNLP 2023, [Paper]
- Context versus Prior Knowledge in Language Models, ACL 2024, [Paper]
- Cutting Off the Head Ends the Conflict: A Mechanism for Interpreting and Mitigating Knowledge Conflicts in Language Models, arXiv 2024, [Paper]
- ConflictBank: A Benchmark for Evaluating the Influence of Knowledge Conflicts in LLM, Su et al., arXiv 2024, [Paper]
- Large Language Models with Controllable Working Memory, Li et al., ACL 2023, [Paper]
- TrueTeacher: Learning Factual Consistency Evaluation with Large Language Models, Gekhman et al., EMNLP 2023, [Paper]
- Improving Factual Consistency for Knowledge-Grounded Dialogue Systems via Knowledge Enhancement and Alignment, Xue et al., EMNLP 2023, [Paper]
- Improving Temporal Generalization of Pre-trained Language Models with Lexical Semantic Change, Su et al., EMNLP 2022, [Paper]
- Context-faithful Prompting for Large Language Models, Zhou et al., EMNLP 2023, [Paper]
- Trusting Your Evidence: Hallucinate Less with Context-aware Decoding, Shi et al., NAACL 2024, [Paper]
- Contrastive Decoding: Open-ended Text Generation as Optimization, Li et al., ACL 2023, [Paper]
- Tug-of-war between knowledge: Exploring and resolving knowledge conflicts in retrieval-augmented language models, COLING 2024, [Paper]
- Characterizing mechanisms for factual recall in language models, EMNLP 2023, [Paper]
- Cutting Off the Head Ends the Conflict: A Mechanism for Interpreting and Mitigating Knowledge Conflicts in Language Models, arXiv 2024, [Paper]
- Synthetic lies: Understanding ai-generated misinformation and evaluating algorithmic and human solutions, Zhou et al., CHI 2023, [Paper]
- Comparing GPT-4 and Open-Source Language Models in Misinformation Mitigation, Vergho et al., arXiv 2024, [Paperhttps://arxiv.org/abs/2401.06920]
- A dataset for answering time-sensitive questions, Chen et al., Neurips 2021, [Paper]
- SituatedQA: Incorporating extra-linguistic contexts into QA, Zhang et al., EMNLP 2021, [Paper]
- Streamingqa: A benchmark for adaptation to new knowledge over time in question answering models, Liska et al., ICML 2022. [Paper]
- RealTime QA: What's the Answer Right Now?, Kasai et al., Neurips 2024, [Paper]
- SituatedQA: Incorporating extra-linguistic contexts into QA, Zhang et al., EMNLP 2021, [Paper]
- Synthetic Disinformation Attacks on Automated Fact Verification Systems Authors, AAAI 2022, [Paper]
- Attacking open-domain question answering by injecting misinformation, Pan et al., AACL 2023, [Paper]
- Rich Knowledge Sources Bring Complex Knowledge Conflicts: Recalibrating Models to Reflect Conflicting Evidence, Chen et al., EMNLP 2022, [Paper]
- Tug-of-war between knowledge: Exploring and resolving knowledge conflicts in retrieval-augmented language models, Jin et al., LREC-COLING 2024, [Paper]
- ConflictBank: A Benchmark for Evaluating the Influence of Knowledge Conflicts in LLM, Su et al., arXiv 2024, [Paper]
- CDConv: A Benchmark for Contradiction Detection in Chinese Conversations, Zheng et al., EMNLP 2022, [Paper]
- ContraDoc: understanding self-contradictions in documents with large language models, Li et al., arXiv 2023, [Paperhttps://arxiv.org/abs/2311.09182]
- What Evidence Do Language Models Find Convincing?, Wan et al., ACL 2024, [Paper]
- Tug-of-war between knowledge: Exploring and resolving knowledge conflicts in retrieval-augmented language models, Jin et al., LREC-COLING 2024, [Paper]
- WikiContradiction: Detecting Self-Contradiction Articles on Wikipedia, Hsu et al., IEEE Big Data 2021, [Paper]
- Topological analysis of contradictions in text, Wu et al., SIGIR 2022, [Paper]
- FACTOOL: Factuality Detection in Generative AI-A Tool Augmented Framework for Multi-Task and Multi-Domain Scenarios, Chern et al., arXiv 2023, [Paper]
- Detecting Misinformation with LLM-Predicted Credibility Signals and Weak Supervision, Leite et al., arXiv 2023, [Paper]
- Why So Gullible? Enhancing the Robustness of Retrieval-Augmented Models against Counterfactual Noise, Hong et al., arXiv 2024, [Paper]
- Defending Against Disinformation Attacks in Open-Domain Question Answering, Weller et al., EACL 2024, [Paper]
- On the dangers of stochastic parrots: Can language models be too big, Bender et al., FACCT 2021, [Paper]
- Ethical and social risks of harm from language models, Weidinger et al., arXiv 2021, [Paper]
- Measuring Causal Effects of Data Statistics on Language Model's 'Factual' Predictions, Elazar et al., arXiv 2023, [Paper]
- Studying large language model generalization with influence functions, Grosse et al., arXiv 2023, [Paper]
- How pre-trained language models capture factual knowledge? a causal-inspired analysis, Li et al., ACL 2022, [Paper]
- Impact of co-occurrence on factual knowledge of large language models, Kang and Choi, EMNLP 2023, [Paper]
- Factuality enhanced language models for open-ended text generation, Lee et al., NeurIPS 2022, [Paper]
- A survey on hallucination in large language models: Principles, taxonomy, challenges, and open questions, Huang et al., arXiv 2023, [Paper]
- Unveiling the pitfalls of knowledge editing for large language models, Li et al., ICLR 2024, [Paper]
- Editing large language models: Problems, methods, and opportunities, Yao et al., EMNLP 2023, [Paper]
- Measuring and improving consistency in pretrained language models, Elazar et al., TACL 2021, [Paper]
- Methods for measuring, updating, and visualizing factual beliefs in language models, Hase et al., EACL 2023, [Paper]
- Knowing what llms do not know: A simple yet effective self-detection method, Zhao et al., NAACL 2024, [Paper]
- Statistical knowledge assessment for large language models, Dong et al., NeurIPS 2023, [Paper]
- Benchmarking and improving generator-validator consistency of language models, Li et al., ICLR 2024, [Paper]
- How pre-trained language models capture factual knowledge? a causal-inspired analysis, Li et al., ACL 2022, [Paper]
- Impact of co-occurrence on factual knowledge of large language models, Kang and Choi, EMNLP 2023, [Paper]
- ConflictBank: A Benchmark for Evaluating the Influence of Knowledge Conflicts in LLM, Su et al., arXiv 2024, [Paper]
- Dola: Decoding by contrasting layers improves factuality in large language models, Chuang et al., ICLR 2024, [Paper]
- Inferencetime intervention: Eliciting truthful answers from a language model, Li et al., NeurIPS 2023, [Paper]
- Cross-lingual knowledge editing in large language models, Wan et al., arXiv 2023, [Paper]
- Cross-lingual consistency of factual knowledge in multilingual language models, Qi et al., EMNLP 2023, [Paper]
- Measuring and improving consistency in pretrained language models, Elazar et al., TACL 2021, [Paper]
- Benchmarking and improving generator-validator consistency of language models, Li et al., ICLR 2024, [Paper]
- Improving language models meaning understanding and consistency by learning conceptual roles from dictionary, Jang et al., EMNLP 2023, [Paper]
- Enhancing selfconsistency and performance of pre-trained language models through natural language inference, Mitchell et al., EMNLP 2022, [Paper]
- Knowing what llms do not know: A simple yet effective self-detection method, Zhao et al., NAACL 2024, [Paper]
For Tasks:
Click tags to check more tools for each tasksFor Jobs:
Alternative AI tools for Knowledge-Conflicts-Survey
Similar Open Source Tools
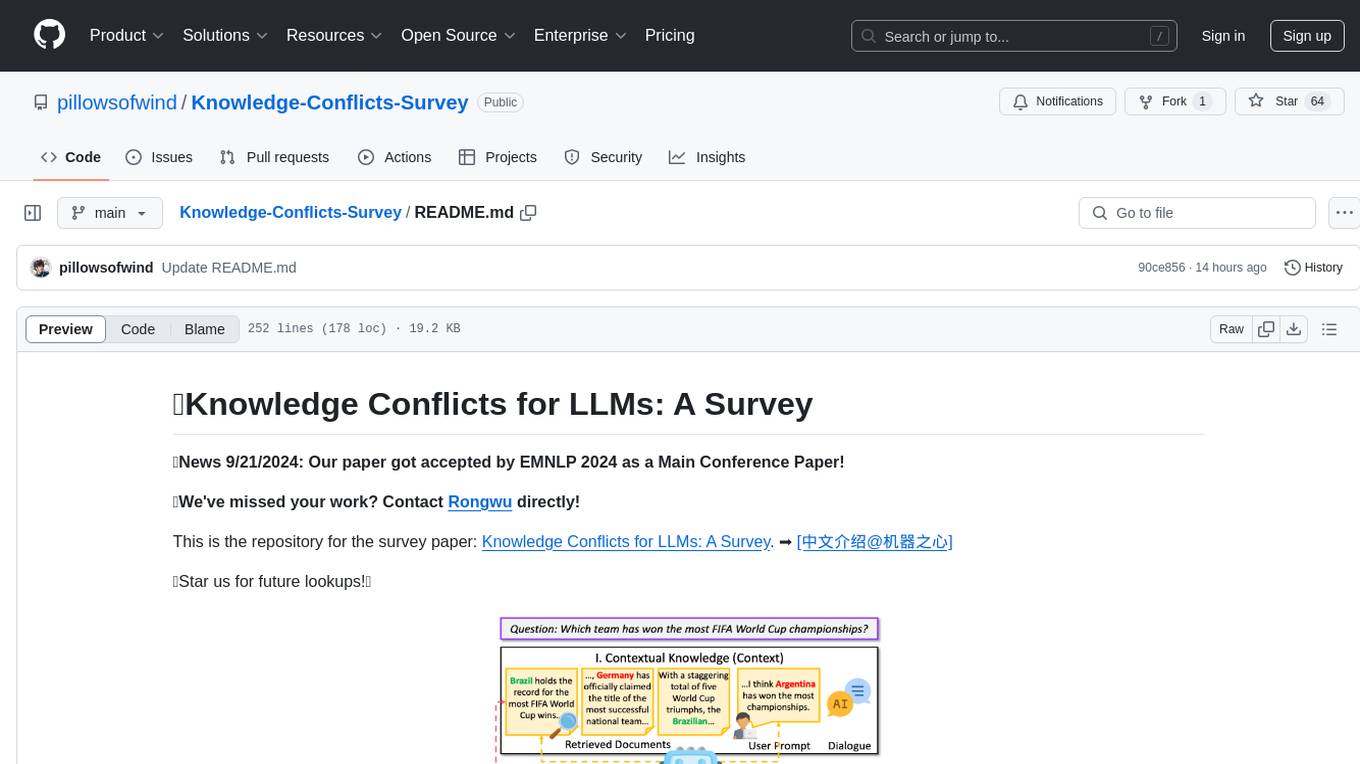
Knowledge-Conflicts-Survey
Knowledge Conflicts for LLMs: A Survey is a repository containing a survey paper that investigates three types of knowledge conflicts: context-memory conflict, inter-context conflict, and intra-memory conflict within Large Language Models (LLMs). The survey reviews the causes, behaviors, and possible solutions to these conflicts, providing a comprehensive analysis of the literature in this area. The repository includes detailed information on the types of conflicts, their causes, behavior analysis, and mitigating solutions, offering insights into how conflicting knowledge affects LLMs and how to address these conflicts.
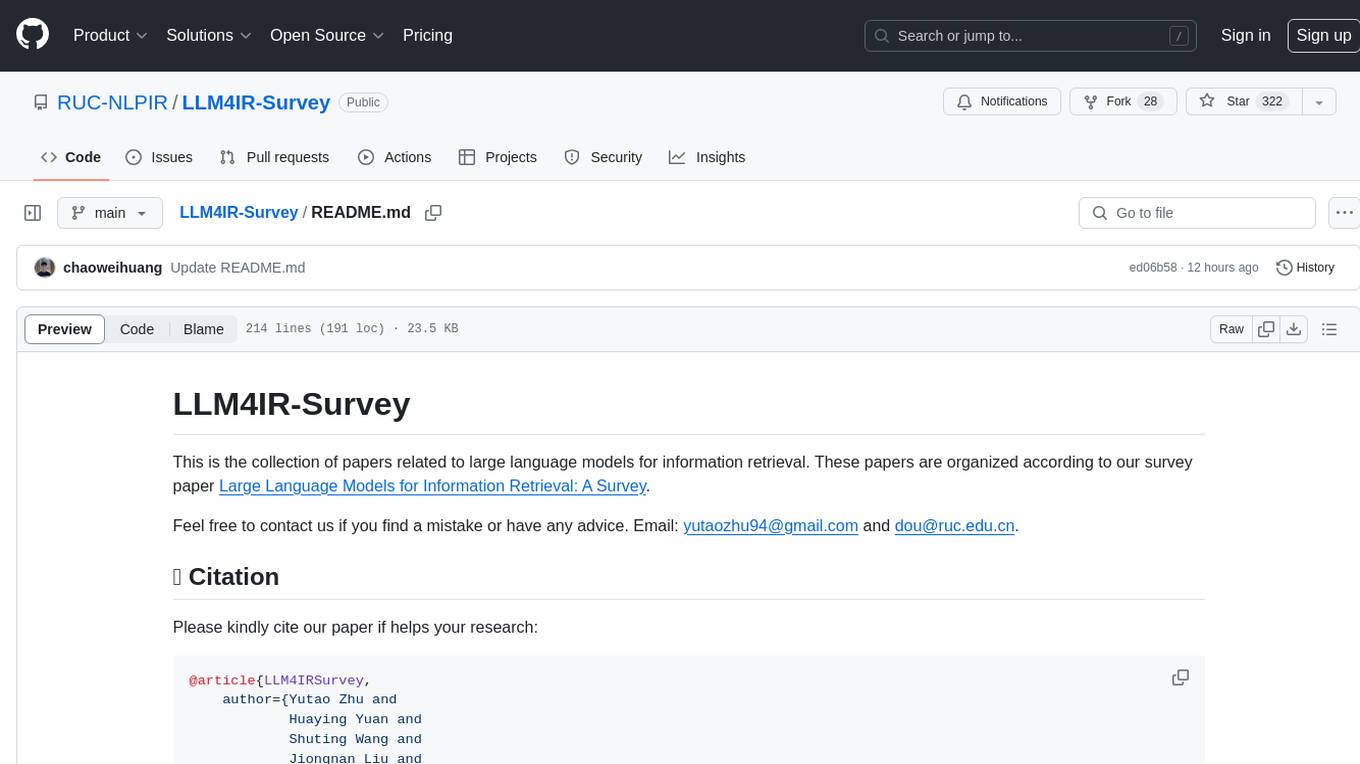
LLM4IR-Survey
LLM4IR-Survey is a collection of papers related to large language models for information retrieval, organized according to the survey paper 'Large Language Models for Information Retrieval: A Survey'. It covers various aspects such as query rewriting, retrievers, rerankers, readers, search agents, and more, providing insights into the integration of large language models with information retrieval systems.
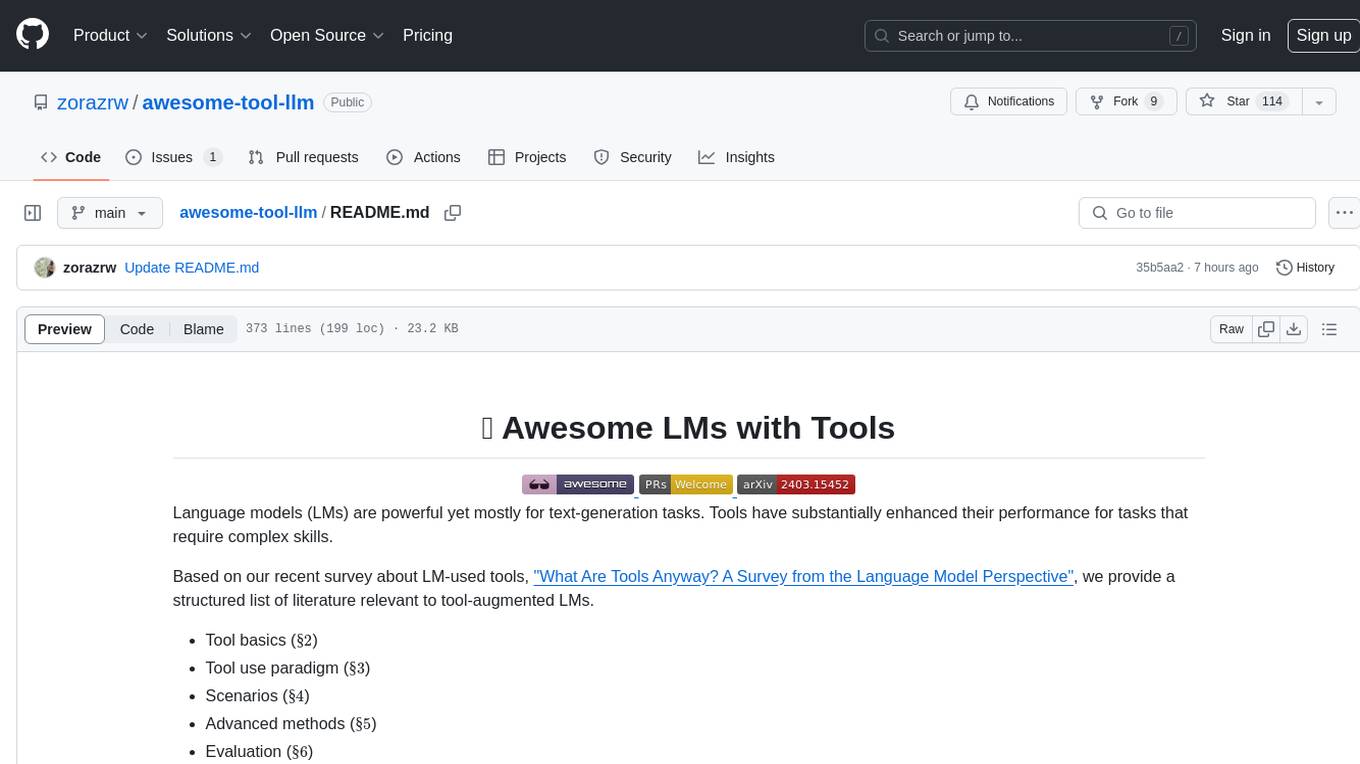
awesome-tool-llm
This repository focuses on exploring tools that enhance the performance of language models for various tasks. It provides a structured list of literature relevant to tool-augmented language models, covering topics such as tool basics, tool use paradigm, scenarios, advanced methods, and evaluation. The repository includes papers, preprints, and books that discuss the use of tools in conjunction with language models for tasks like reasoning, question answering, mathematical calculations, accessing knowledge, interacting with the world, and handling non-textual modalities.
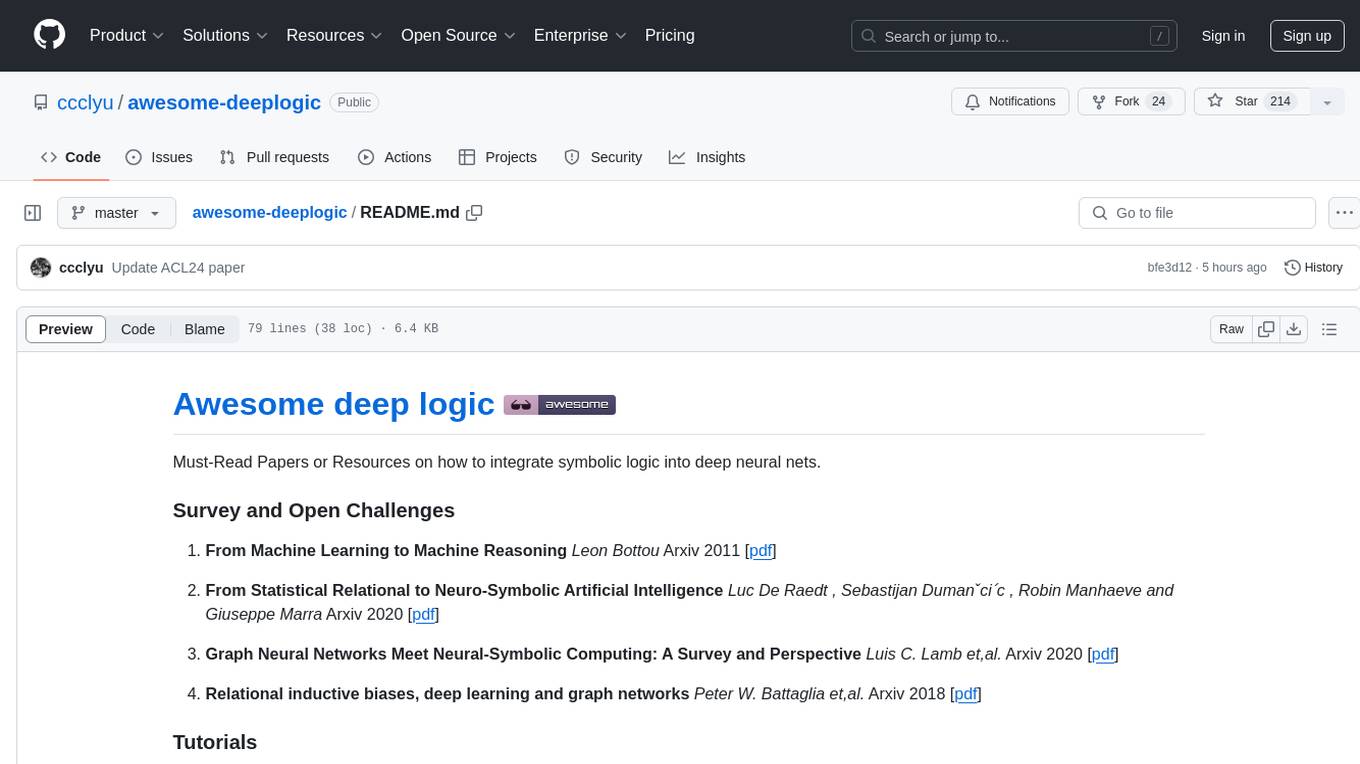
awesome-deeplogic
Awesome deep logic is a curated list of papers and resources focusing on integrating symbolic logic into deep neural networks. It includes surveys, tutorials, and research papers that explore the intersection of logic and deep learning. The repository aims to provide valuable insights and knowledge on how logic can be used to enhance reasoning, knowledge regularization, weak supervision, and explainability in neural networks.
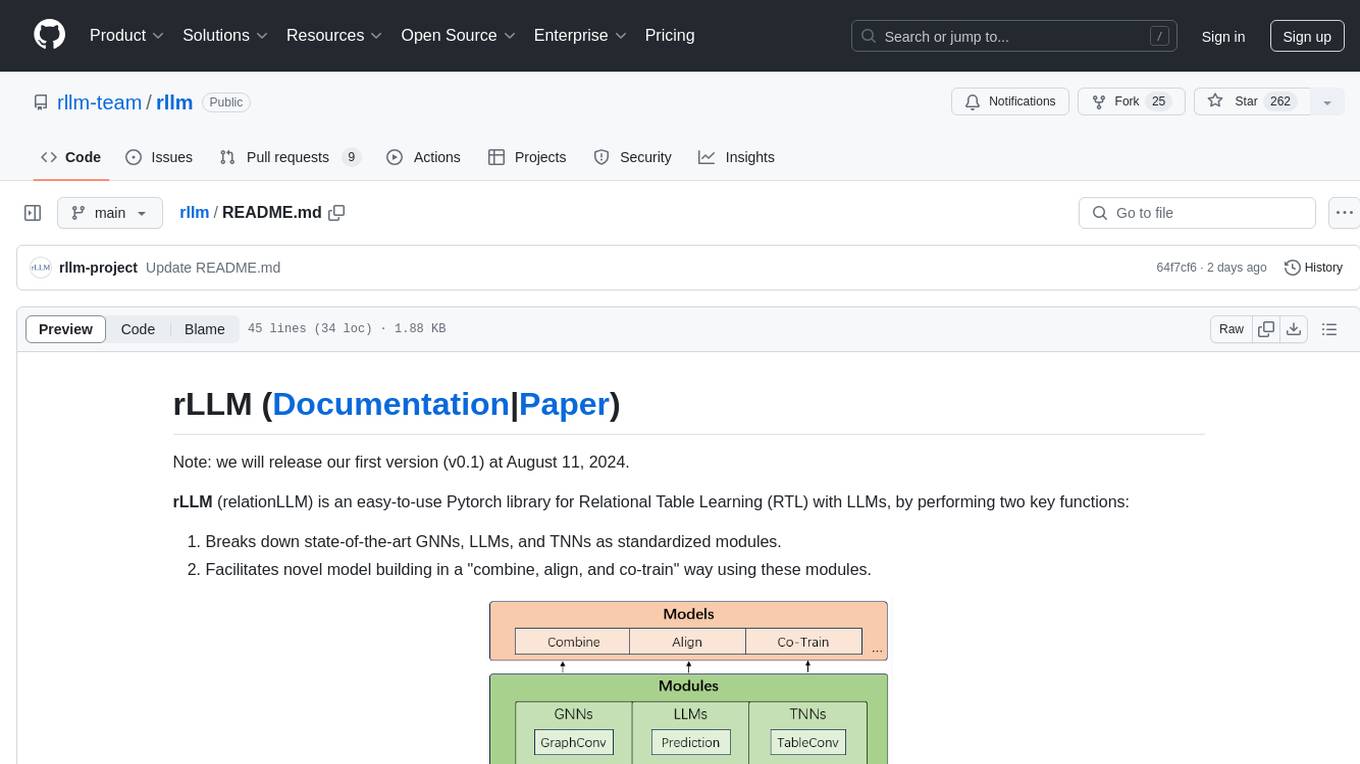
rllm
rLLM (relationLLM) is a Pytorch library for Relational Table Learning (RTL) with LLMs. It breaks down state-of-the-art GNNs, LLMs, and TNNs as standardized modules and facilitates novel model building in a 'combine, align, and co-train' way using these modules. The library is LLM-friendly, processes various graphs as multiple tables linked by foreign keys, introduces new relational table datasets, and is supported by students and teachers from Shanghai Jiao Tong University and Tsinghua University.
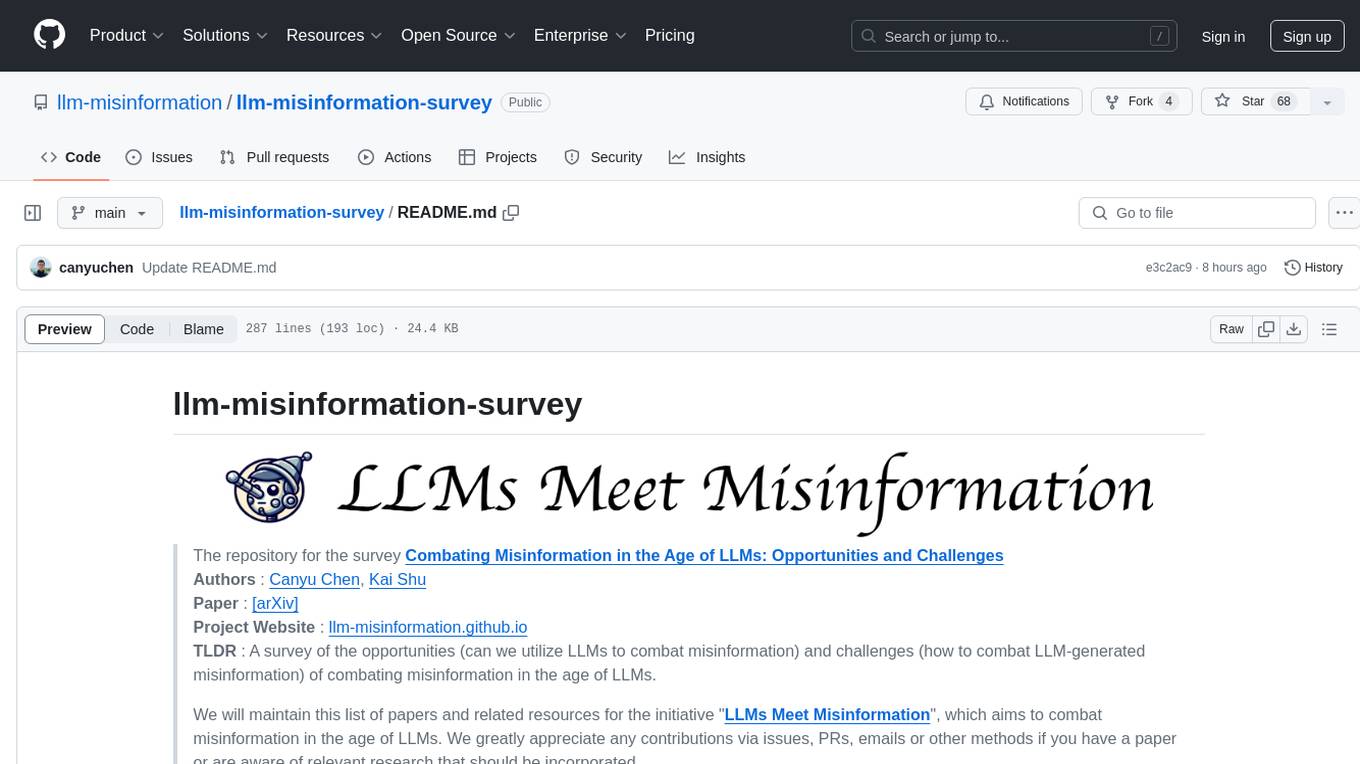
llm-misinformation-survey
The 'llm-misinformation-survey' repository is dedicated to the survey on combating misinformation in the age of Large Language Models (LLMs). It explores the opportunities and challenges of utilizing LLMs to combat misinformation, providing insights into the history of combating misinformation, current efforts, and future outlook. The repository serves as a resource hub for the initiative 'LLMs Meet Misinformation' and welcomes contributions of relevant research papers and resources. The goal is to facilitate interdisciplinary efforts in combating LLM-generated misinformation and promoting the responsible use of LLMs in fighting misinformation.
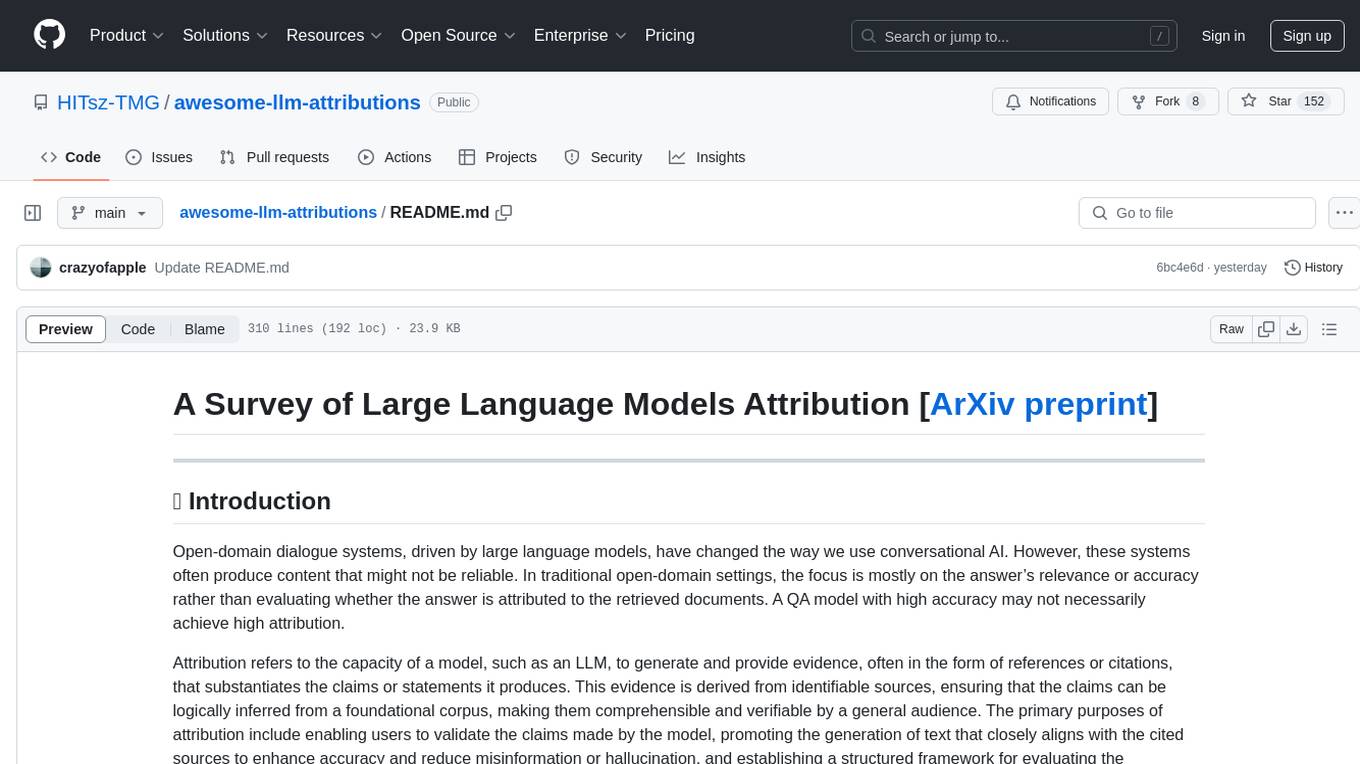
awesome-llm-attributions
This repository focuses on unraveling the sources that large language models tap into for attribution or citation. It delves into the origins of facts, their utilization by the models, the efficacy of attribution methodologies, and challenges tied to ambiguous knowledge reservoirs, biases, and pitfalls of excessive attribution.
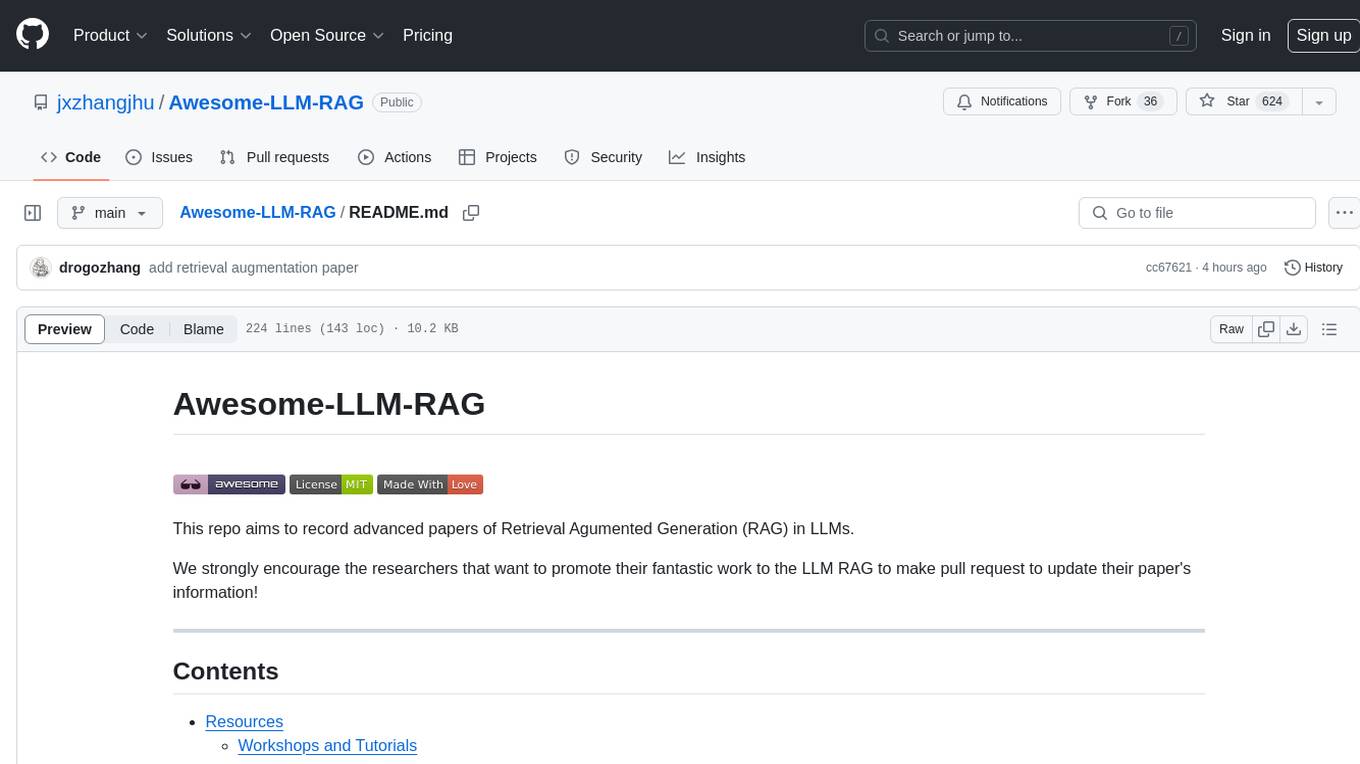
Awesome-LLM-RAG
This repository, Awesome-LLM-RAG, aims to record advanced papers on Retrieval Augmented Generation (RAG) in Large Language Models (LLMs). It serves as a resource hub for researchers interested in promoting their work related to LLM RAG by updating paper information through pull requests. The repository covers various topics such as workshops, tutorials, papers, surveys, benchmarks, retrieval-enhanced LLMs, RAG instruction tuning, RAG in-context learning, RAG embeddings, RAG simulators, RAG search, RAG long-text and memory, RAG evaluation, RAG optimization, and RAG applications.
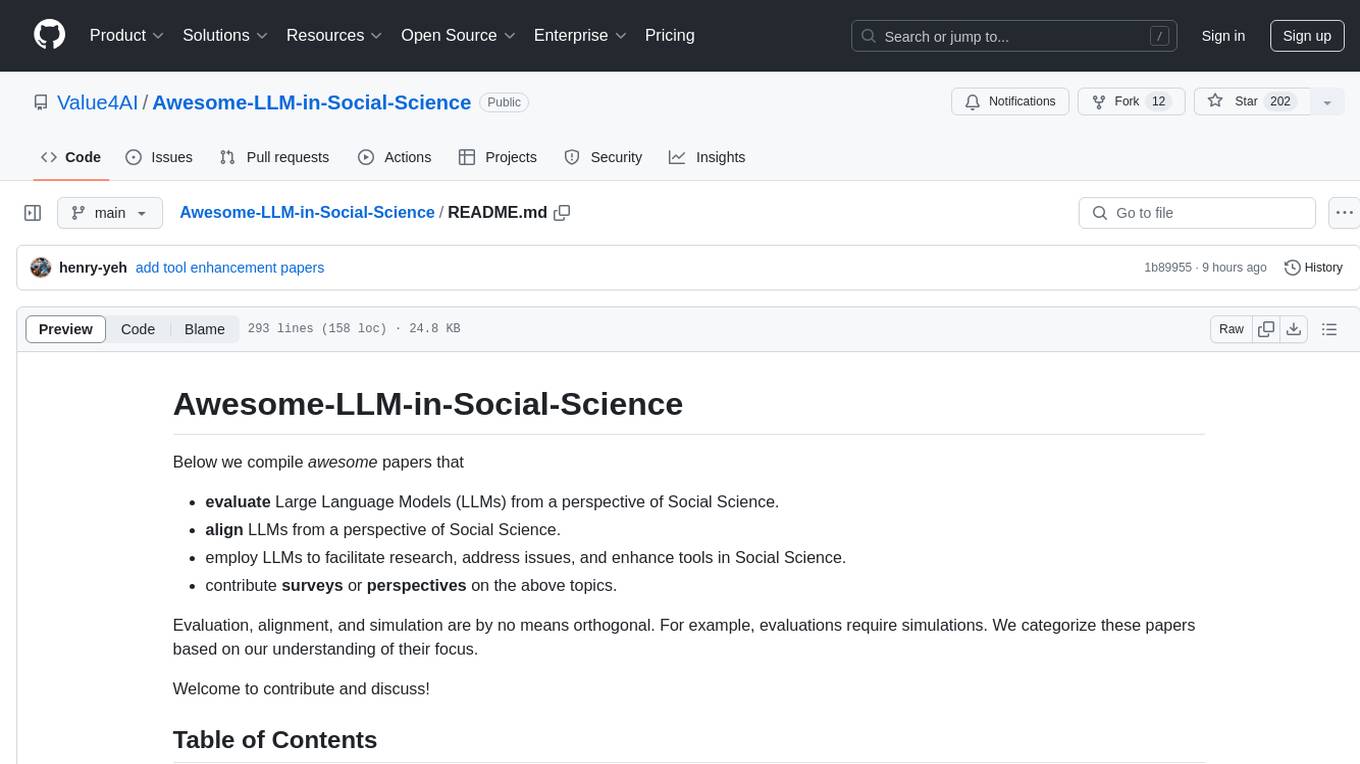
Awesome-LLM-in-Social-Science
Awesome-LLM-in-Social-Science is a repository that compiles papers evaluating Large Language Models (LLMs) from a social science perspective. It includes papers on evaluating, aligning, and simulating LLMs, as well as enhancing tools in social science research. The repository categorizes papers based on their focus on attitudes, opinions, values, personality, morality, and more. It aims to contribute to discussions on the potential and challenges of using LLMs in social science research.
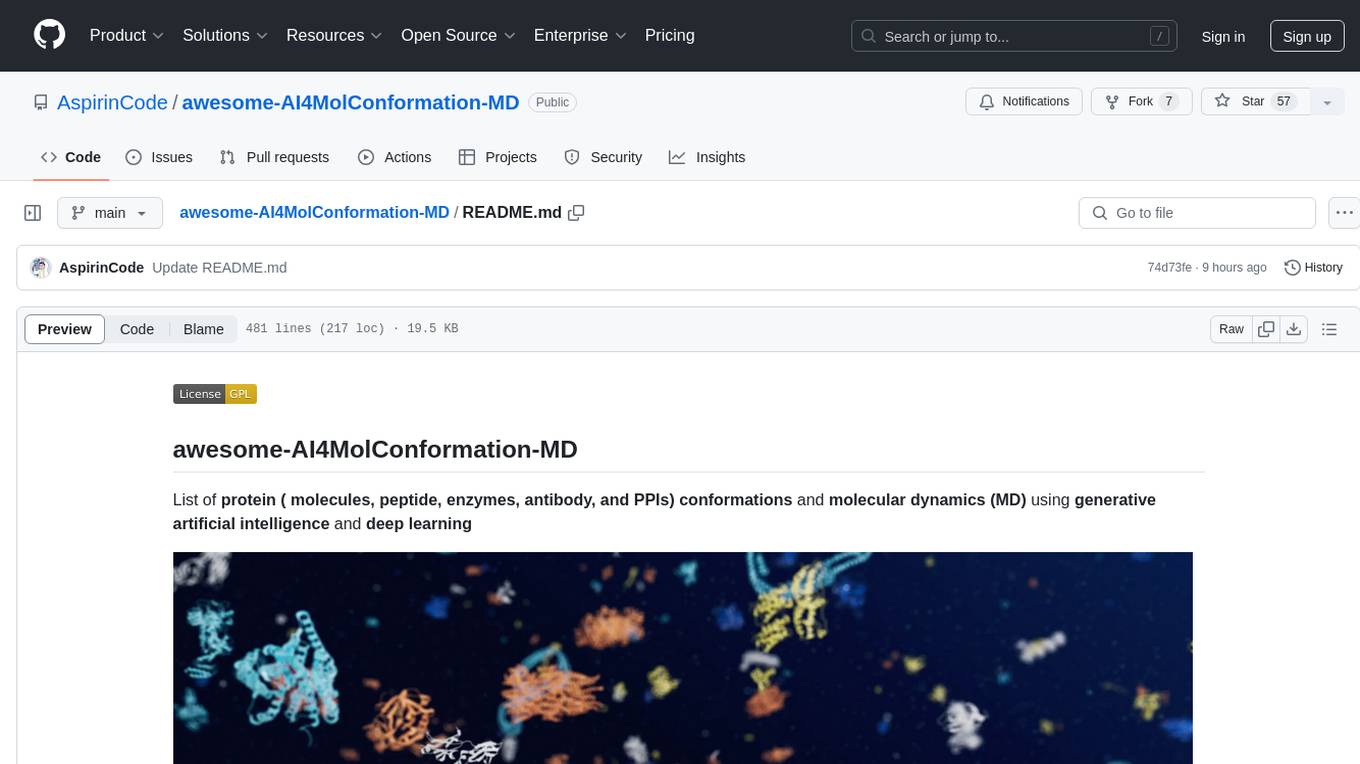
awesome-AI4MolConformation-MD
The 'awesome-AI4MolConformation-MD' repository focuses on protein conformations and molecular dynamics using generative artificial intelligence and deep learning. It provides resources, reviews, datasets, packages, and tools related to AI-driven molecular dynamics simulations. The repository covers a wide range of topics such as neural networks potentials, force fields, AI engines/frameworks, trajectory analysis, visualization tools, and various AI-based models for protein conformational sampling. It serves as a comprehensive guide for researchers and practitioners interested in leveraging AI for studying molecular structures and dynamics.
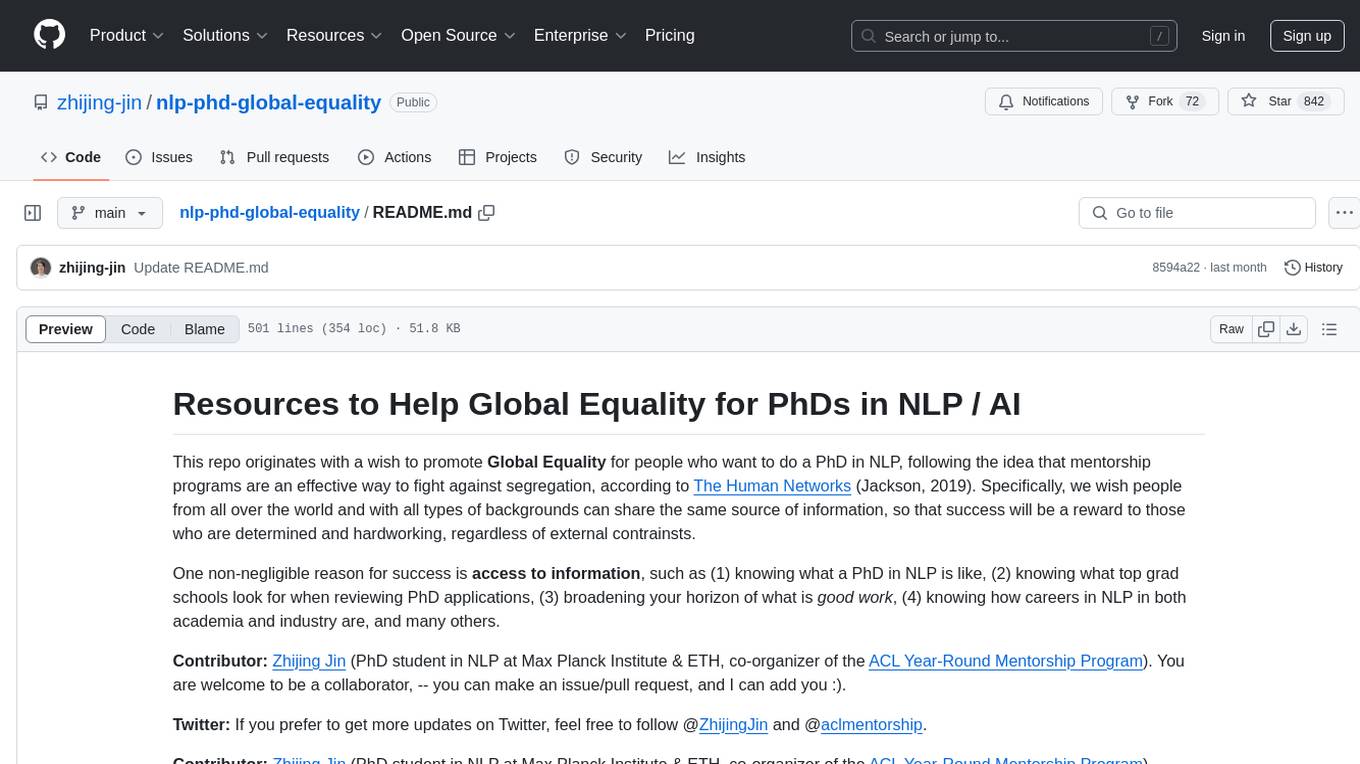
nlp-phd-global-equality
This repository aims to promote global equality for individuals pursuing a PhD in NLP by providing resources and information on various aspects of the academic journey. It covers topics such as applying for a PhD, getting research opportunities, preparing for the job market, and succeeding in academia. The repository is actively updated and includes contributions from experts in the field.
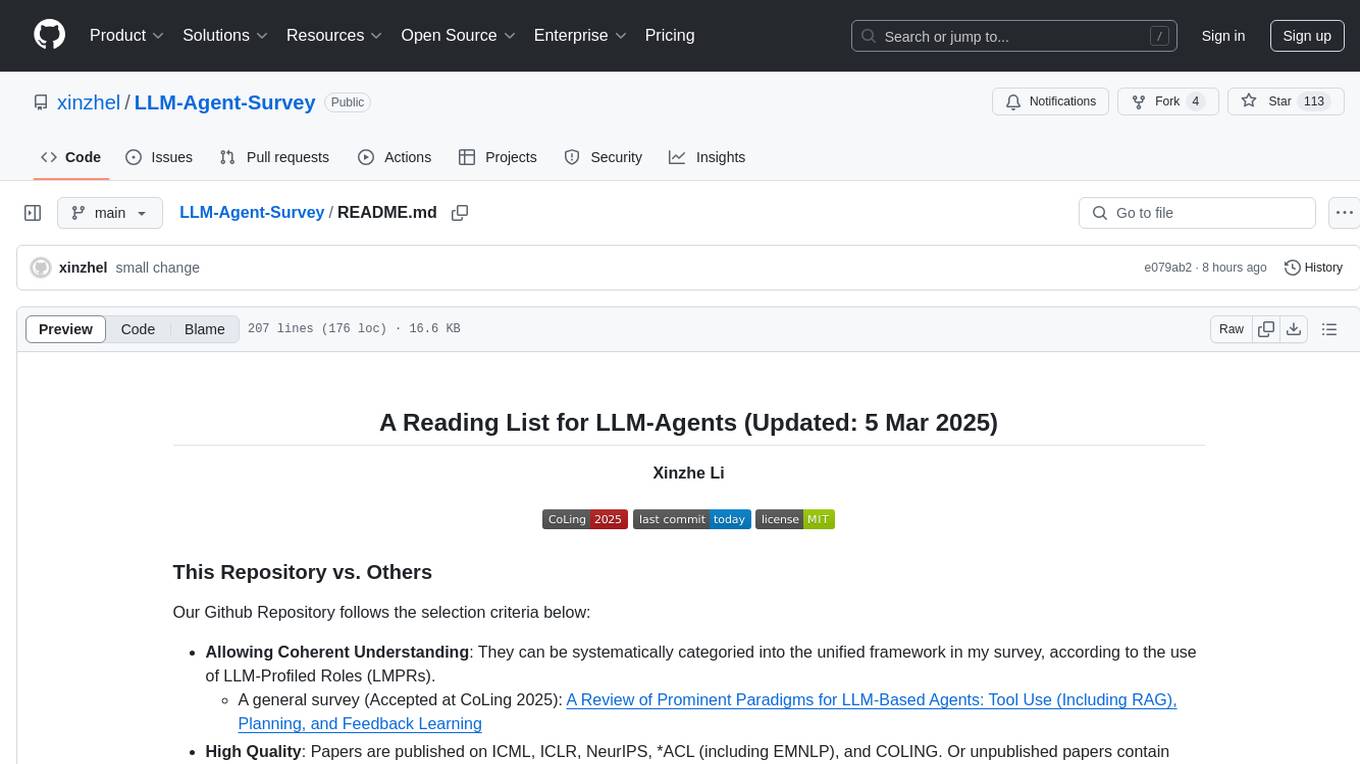
LLM-Agent-Survey
LLM-Agent-Survey is a comprehensive repository that provides a curated list of papers related to Large Language Model (LLM) agents. The repository categorizes papers based on LLM-Profiled Roles and includes high-quality publications from prestigious conferences and journals. It aims to offer a systematic understanding of LLM-based agents, covering topics such as tool use, planning, and feedback learning. The repository also includes unpublished papers with insightful analysis and novelty, marked for future updates. Users can explore a wide range of surveys, tool use cases, planning workflows, and benchmarks related to LLM agents.
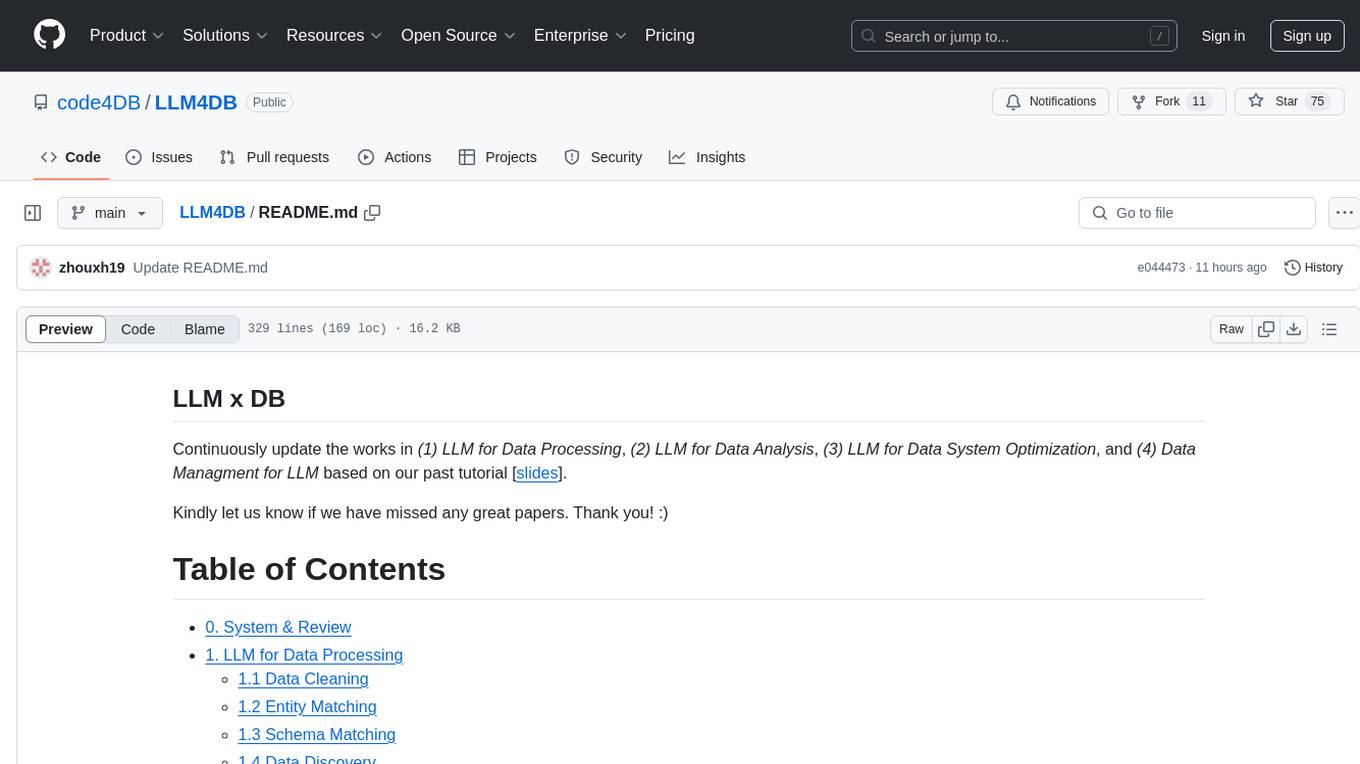
LLM4DB
LLM4DB is a repository focused on the intersection of Large Language Models (LLMs) and Database technologies. It covers various aspects such as data processing, data analysis, database optimization, and data management for LLMs. The repository includes research papers, tools, and techniques related to leveraging LLMs for tasks like data cleaning, entity matching, schema matching, data discovery, NL2SQL, data exploration, data visualization, knob tuning, query optimization, and database diagnosis.
For similar tasks
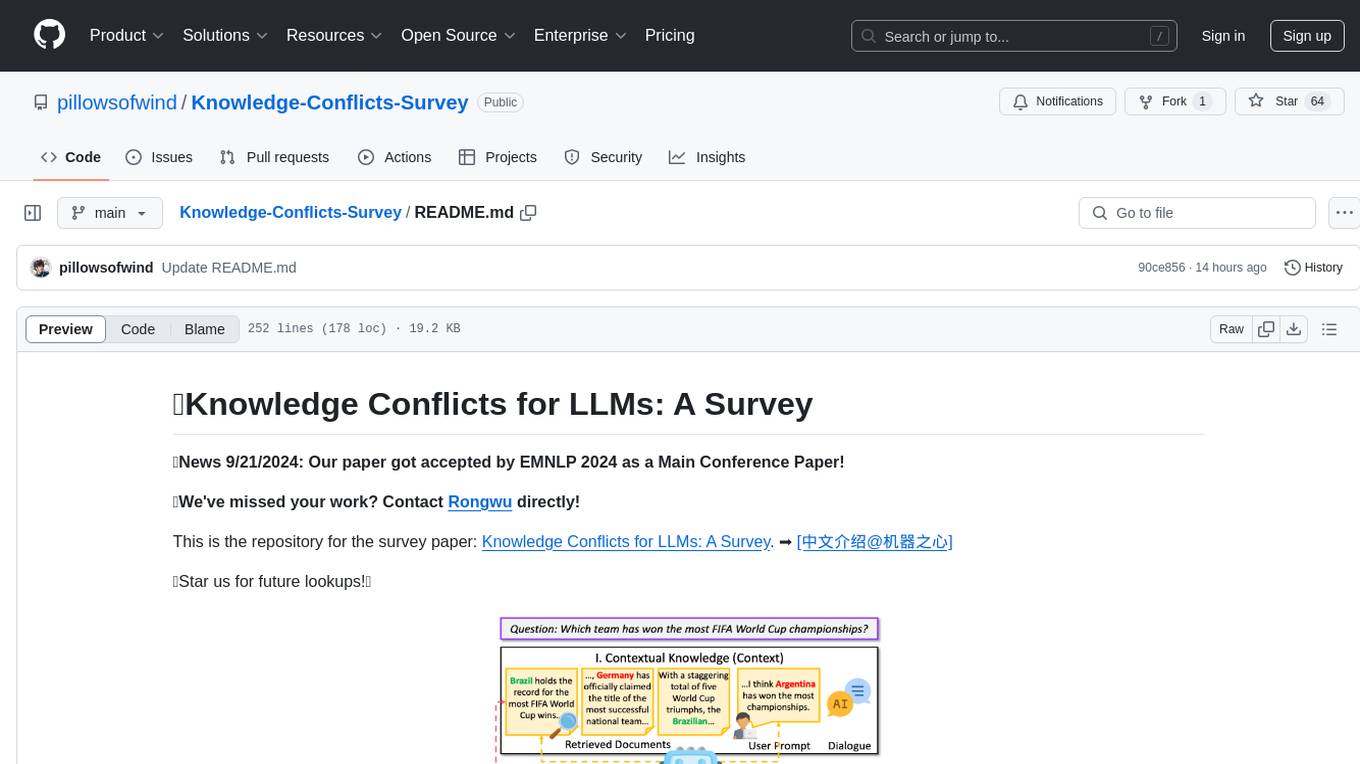
Knowledge-Conflicts-Survey
Knowledge Conflicts for LLMs: A Survey is a repository containing a survey paper that investigates three types of knowledge conflicts: context-memory conflict, inter-context conflict, and intra-memory conflict within Large Language Models (LLMs). The survey reviews the causes, behaviors, and possible solutions to these conflicts, providing a comprehensive analysis of the literature in this area. The repository includes detailed information on the types of conflicts, their causes, behavior analysis, and mitigating solutions, offering insights into how conflicting knowledge affects LLMs and how to address these conflicts.
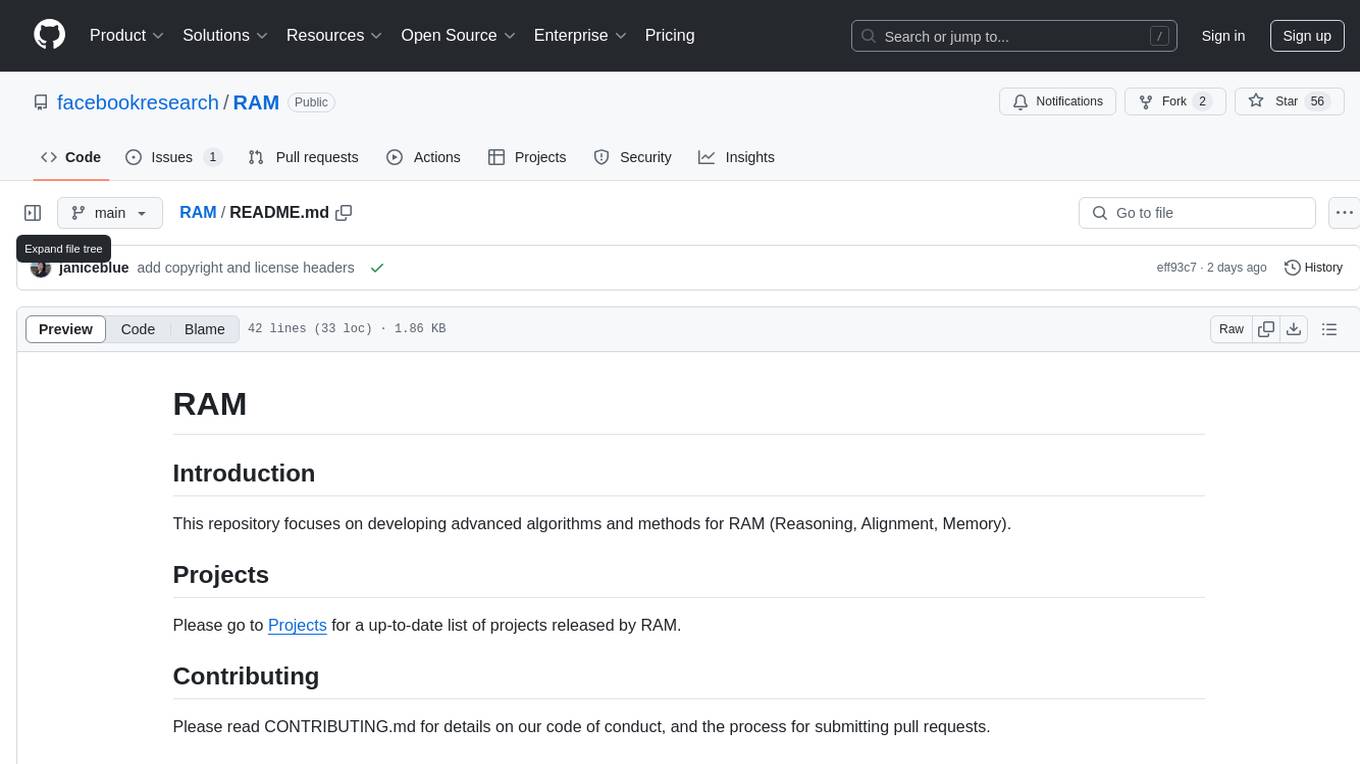
RAM
This repository, RAM, focuses on developing advanced algorithms and methods for Reasoning, Alignment, Memory. It contains projects related to these areas and is maintained by a team of individuals. The repository is licensed under the MIT License.
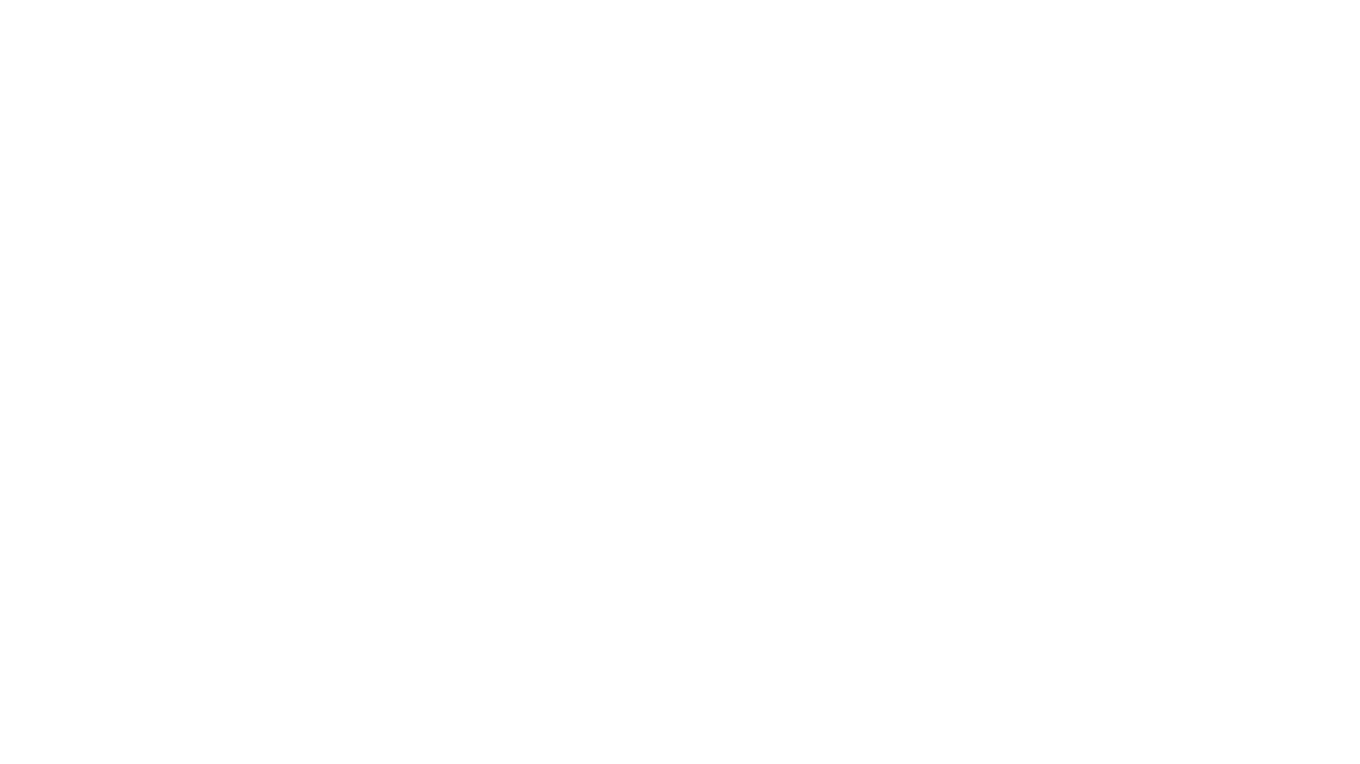
Lecture_AI_in_Automotive_Technology
This Github repository contains practice session materials for the TUM course on Artificial Intelligence in Automotive Technology. It includes coding examples used in the lectures to teach the foundations of AI in automotive technology. The repository aims to provide hands-on experience and practical knowledge in applying AI concepts to the automotive industry.
For similar jobs
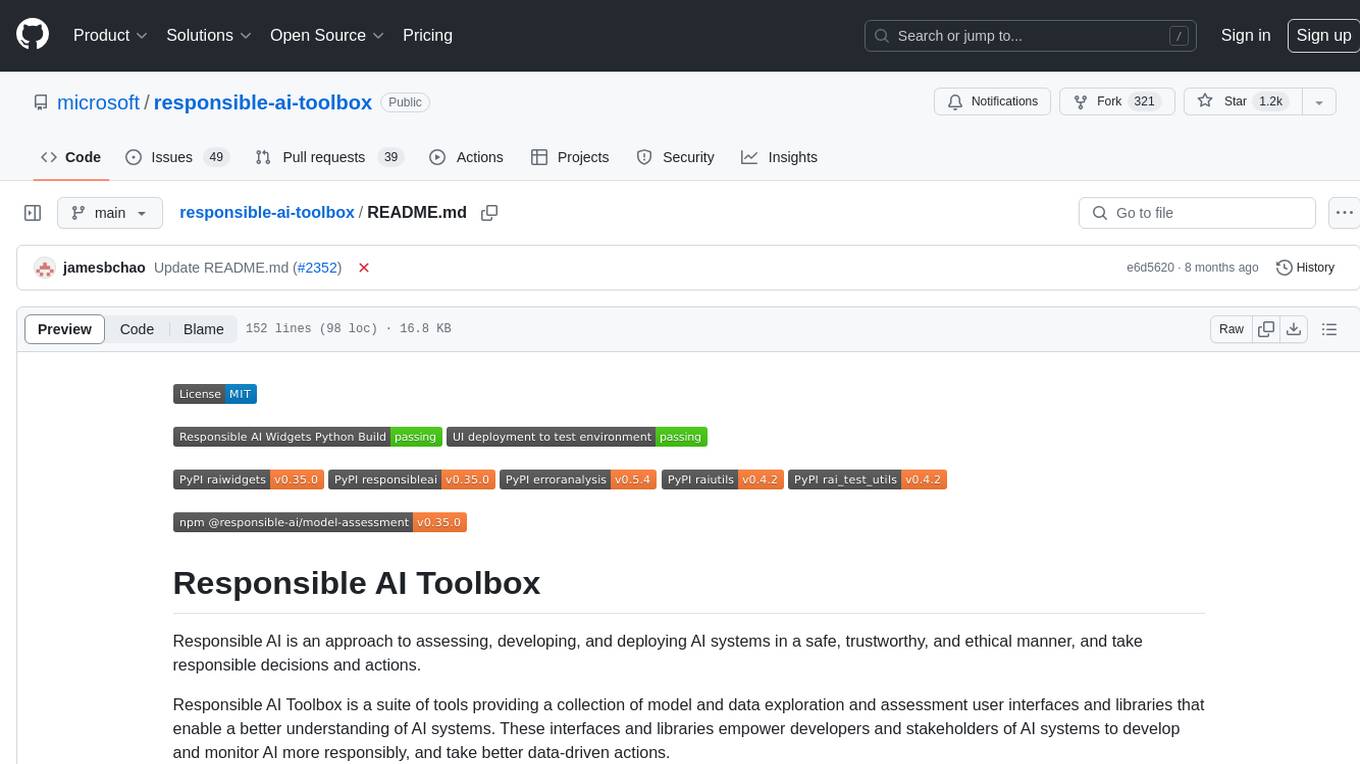
responsible-ai-toolbox
Responsible AI Toolbox is a suite of tools providing model and data exploration and assessment interfaces and libraries for understanding AI systems. It empowers developers and stakeholders to develop and monitor AI responsibly, enabling better data-driven actions. The toolbox includes visualization widgets for model assessment, error analysis, interpretability, fairness assessment, and mitigations library. It also offers a JupyterLab extension for managing machine learning experiments and a library for measuring gender bias in NLP datasets.
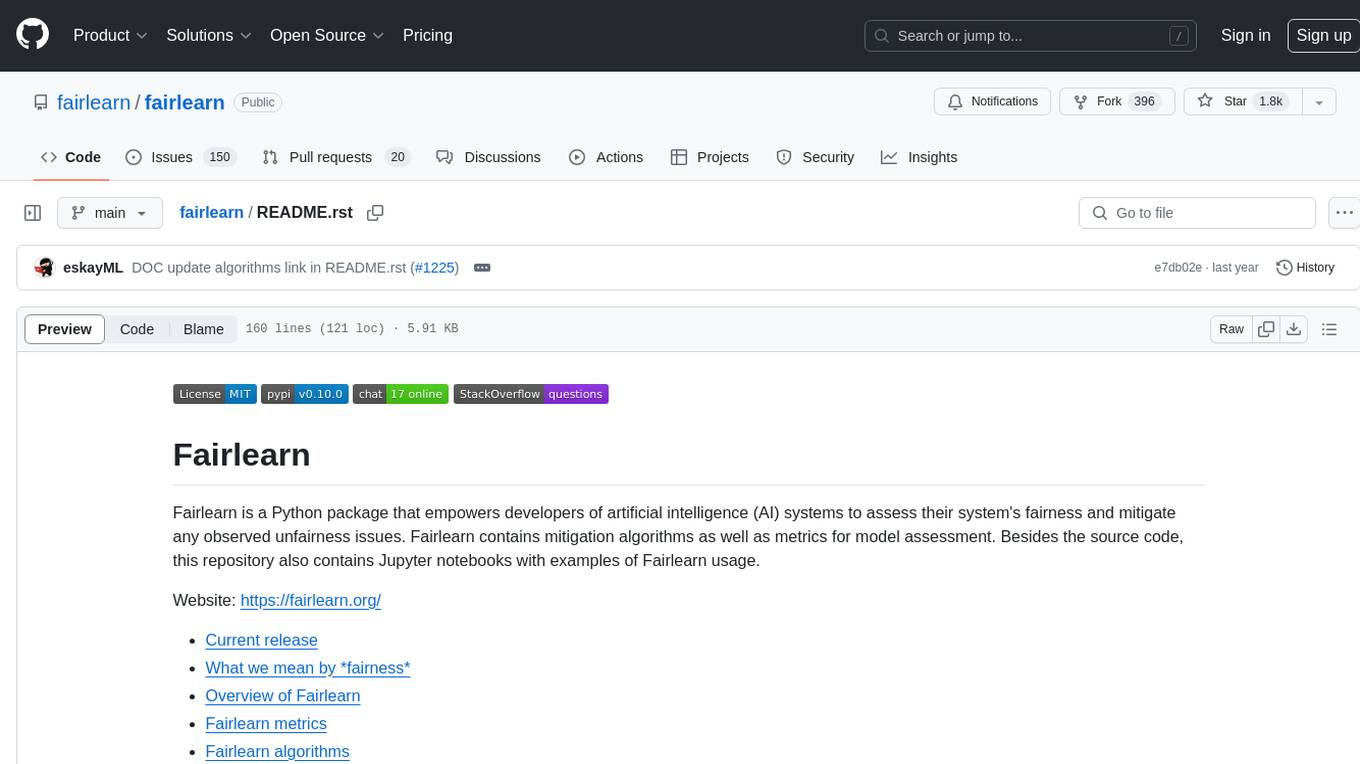
fairlearn
Fairlearn is a Python package designed to help developers assess and mitigate fairness issues in artificial intelligence (AI) systems. It provides mitigation algorithms and metrics for model assessment. Fairlearn focuses on two types of harms: allocation harms and quality-of-service harms. The package follows the group fairness approach, aiming to identify groups at risk of experiencing harms and ensuring comparable behavior across these groups. Fairlearn consists of metrics for assessing model impacts and algorithms for mitigating unfairness in various AI tasks under different fairness definitions.
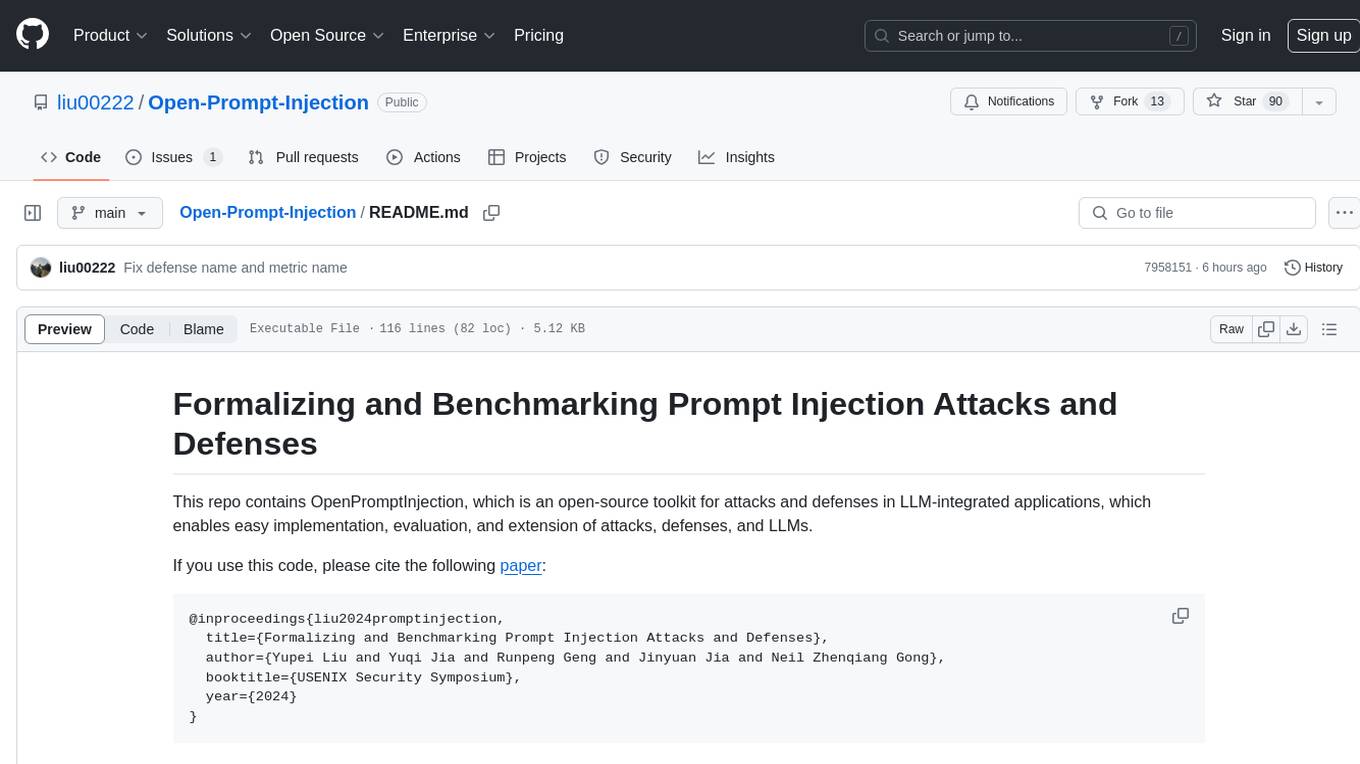
Open-Prompt-Injection
OpenPromptInjection is an open-source toolkit for attacks and defenses in LLM-integrated applications, enabling easy implementation, evaluation, and extension of attacks, defenses, and LLMs. It supports various attack and defense strategies, including prompt injection, paraphrasing, retokenization, data prompt isolation, instructional prevention, sandwich prevention, perplexity-based detection, LLM-based detection, response-based detection, and know-answer detection. Users can create models, tasks, and apps to evaluate different scenarios. The toolkit currently supports PaLM2 and provides a demo for querying models with prompts. Users can also evaluate ASV for different scenarios by injecting tasks and querying models with attacked data prompts.
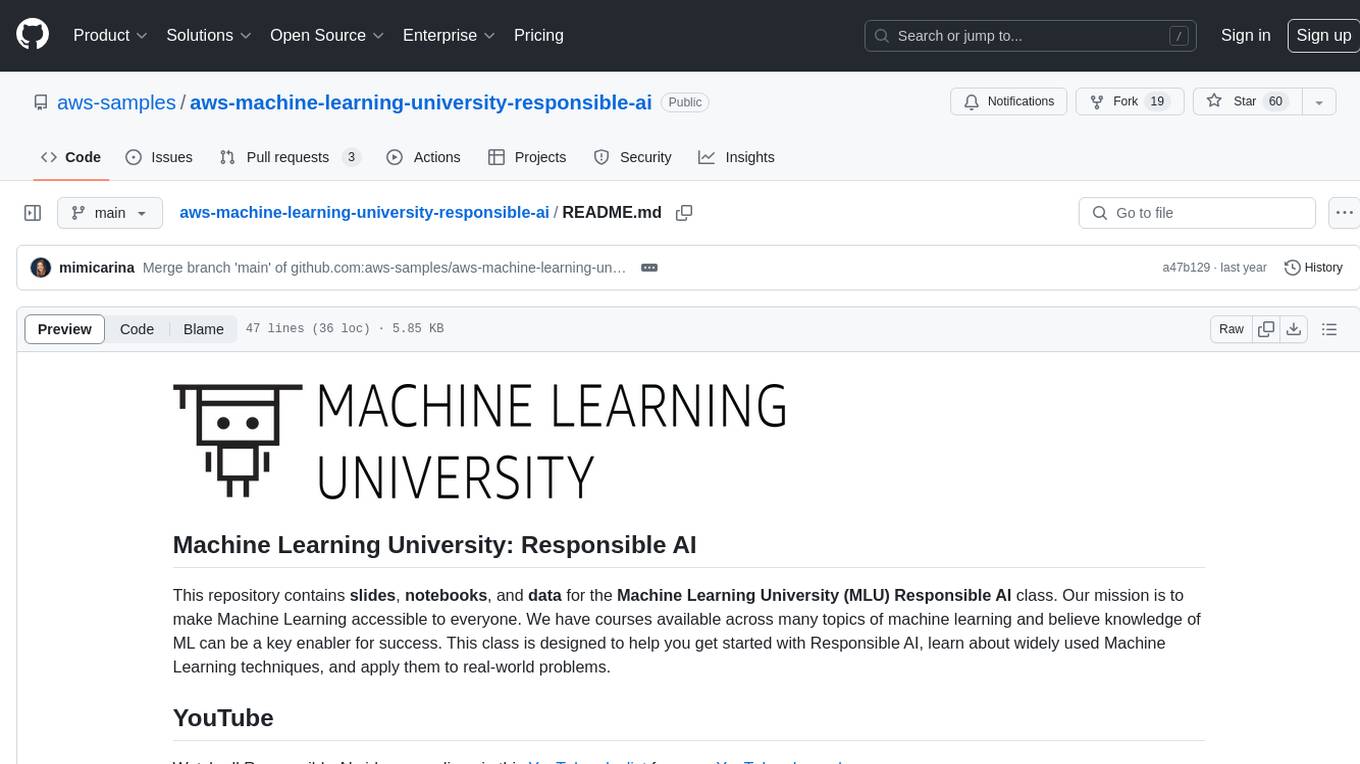
aws-machine-learning-university-responsible-ai
This repository contains slides, notebooks, and data for the Machine Learning University (MLU) Responsible AI class. The mission is to make Machine Learning accessible to everyone, covering widely used ML techniques and applying them to real-world problems. The class includes lectures, final projects, and interactive visuals to help users learn about Responsible AI and core ML concepts.
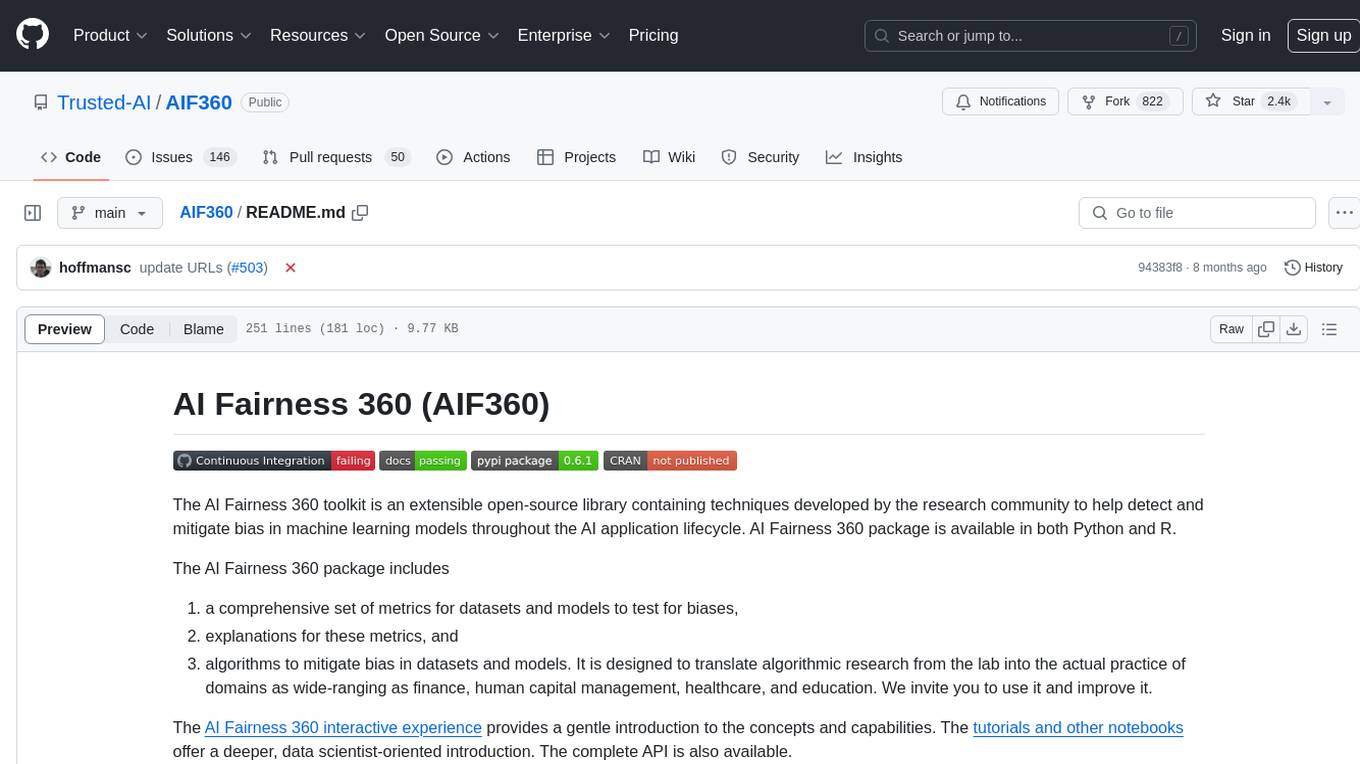
AIF360
The AI Fairness 360 toolkit is an open-source library designed to detect and mitigate bias in machine learning models. It provides a comprehensive set of metrics, explanations, and algorithms for bias mitigation in various domains such as finance, healthcare, and education. The toolkit supports multiple bias mitigation algorithms and fairness metrics, and is available in both Python and R. Users can leverage the toolkit to ensure fairness in AI applications and contribute to its development for extensibility.
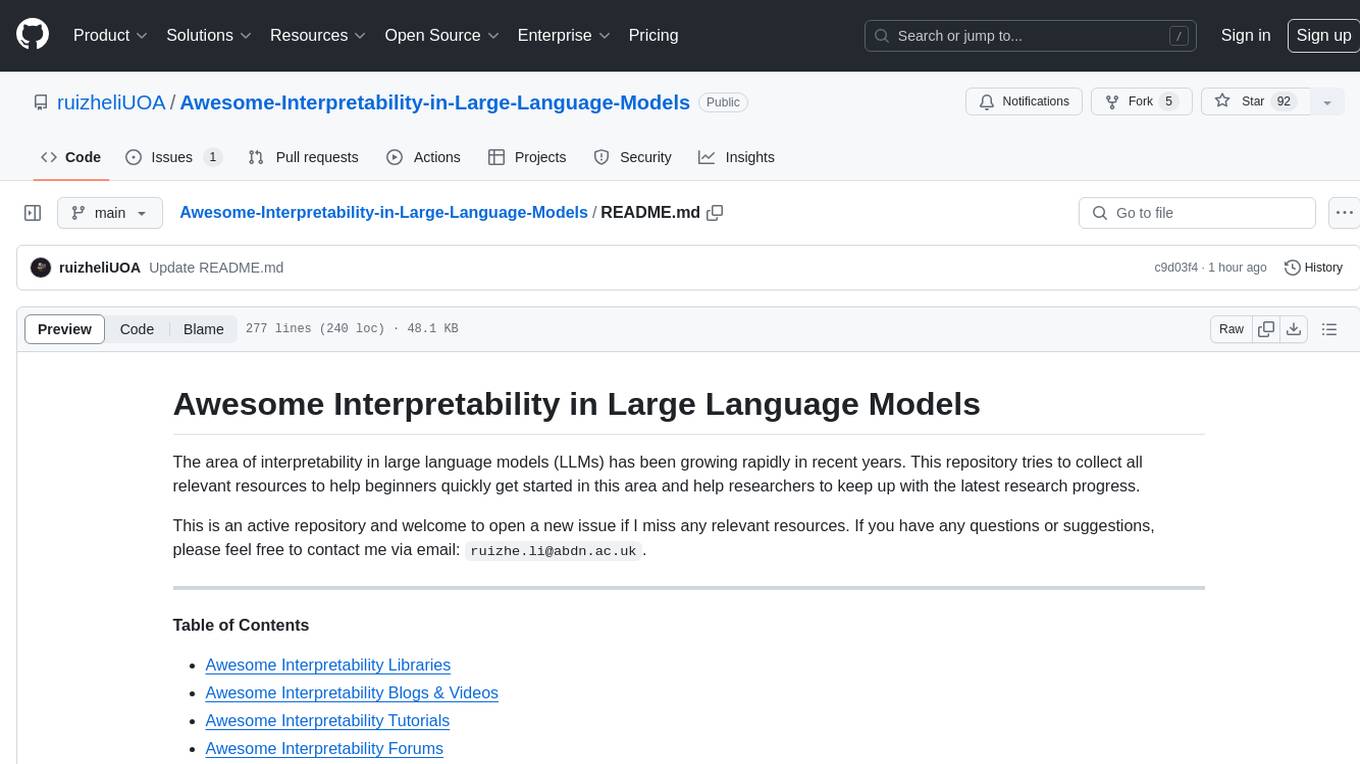
Awesome-Interpretability-in-Large-Language-Models
This repository is a collection of resources focused on interpretability in large language models (LLMs). It aims to help beginners get started in the area and keep researchers updated on the latest progress. It includes libraries, blogs, tutorials, forums, tools, programs, papers, and more related to interpretability in LLMs.
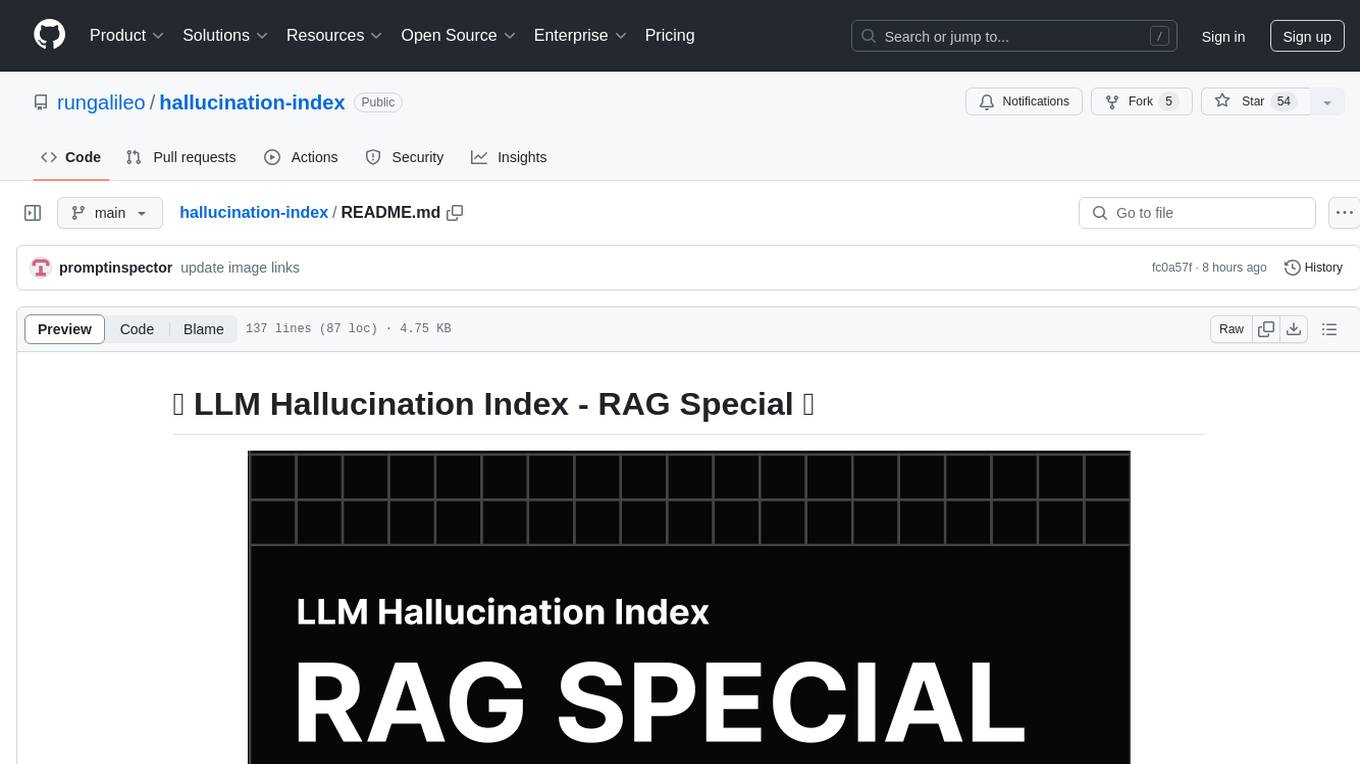
hallucination-index
LLM Hallucination Index - RAG Special is a comprehensive evaluation of large language models (LLMs) focusing on context length and open vs. closed-source attributes. The index explores the impact of context length on model performance and tests the assumption that closed-source LLMs outperform open-source ones. It also investigates the effectiveness of prompting techniques like Chain-of-Note across different context lengths. The evaluation includes 22 models from various brands, analyzing major trends and declaring overall winners based on short, medium, and long context insights. Methodologies involve rigorous testing with different context lengths and prompting techniques to assess models' abilities in handling extensive texts and detecting hallucinations.
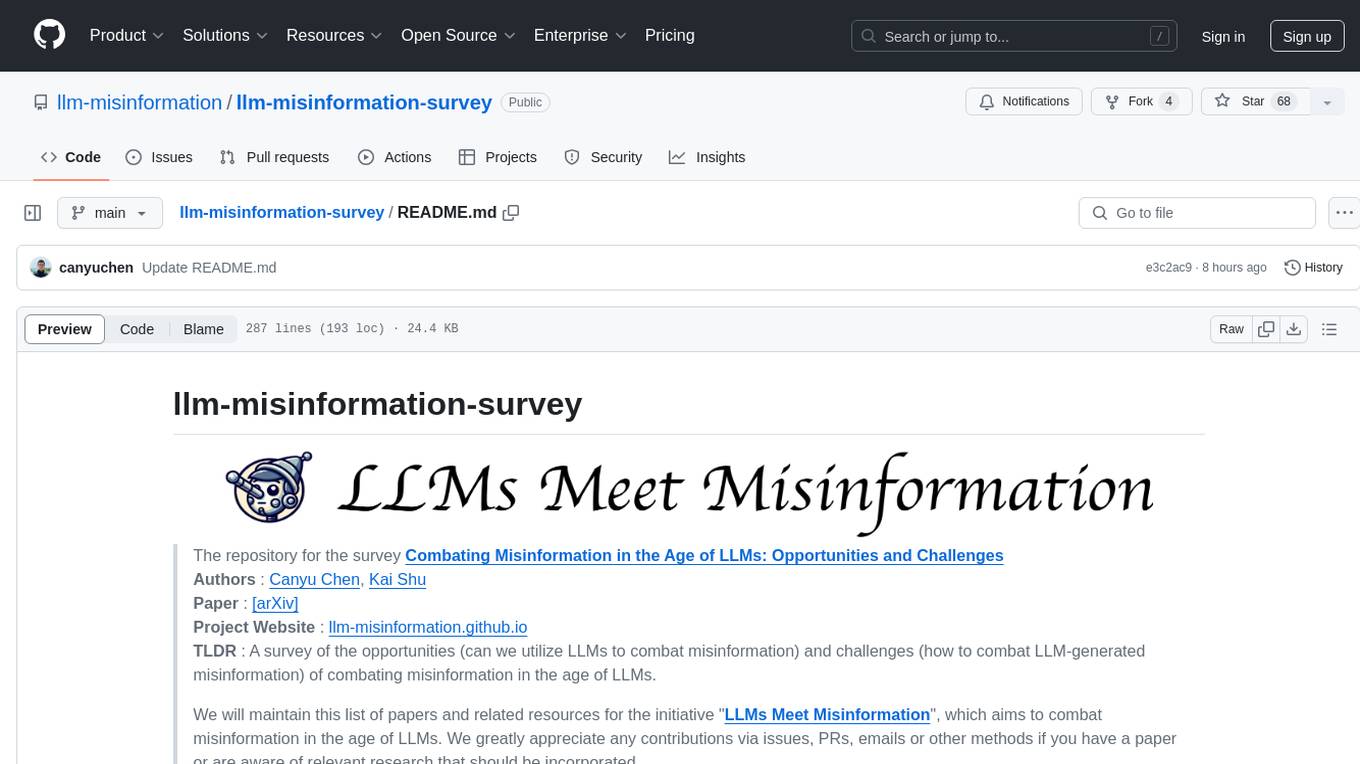
llm-misinformation-survey
The 'llm-misinformation-survey' repository is dedicated to the survey on combating misinformation in the age of Large Language Models (LLMs). It explores the opportunities and challenges of utilizing LLMs to combat misinformation, providing insights into the history of combating misinformation, current efforts, and future outlook. The repository serves as a resource hub for the initiative 'LLMs Meet Misinformation' and welcomes contributions of relevant research papers and resources. The goal is to facilitate interdisciplinary efforts in combating LLM-generated misinformation and promoting the responsible use of LLMs in fighting misinformation.