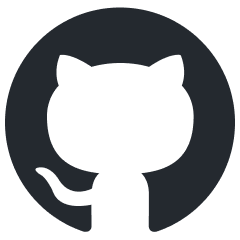
responsible-ai-toolbox
Responsible AI Toolbox is a suite of tools providing model and data exploration and assessment user interfaces and libraries that enable a better understanding of AI systems. These interfaces and libraries empower developers and stakeholders of AI systems to develop and monitor AI more responsibly, and take better data-driven actions.
Stars: 1275
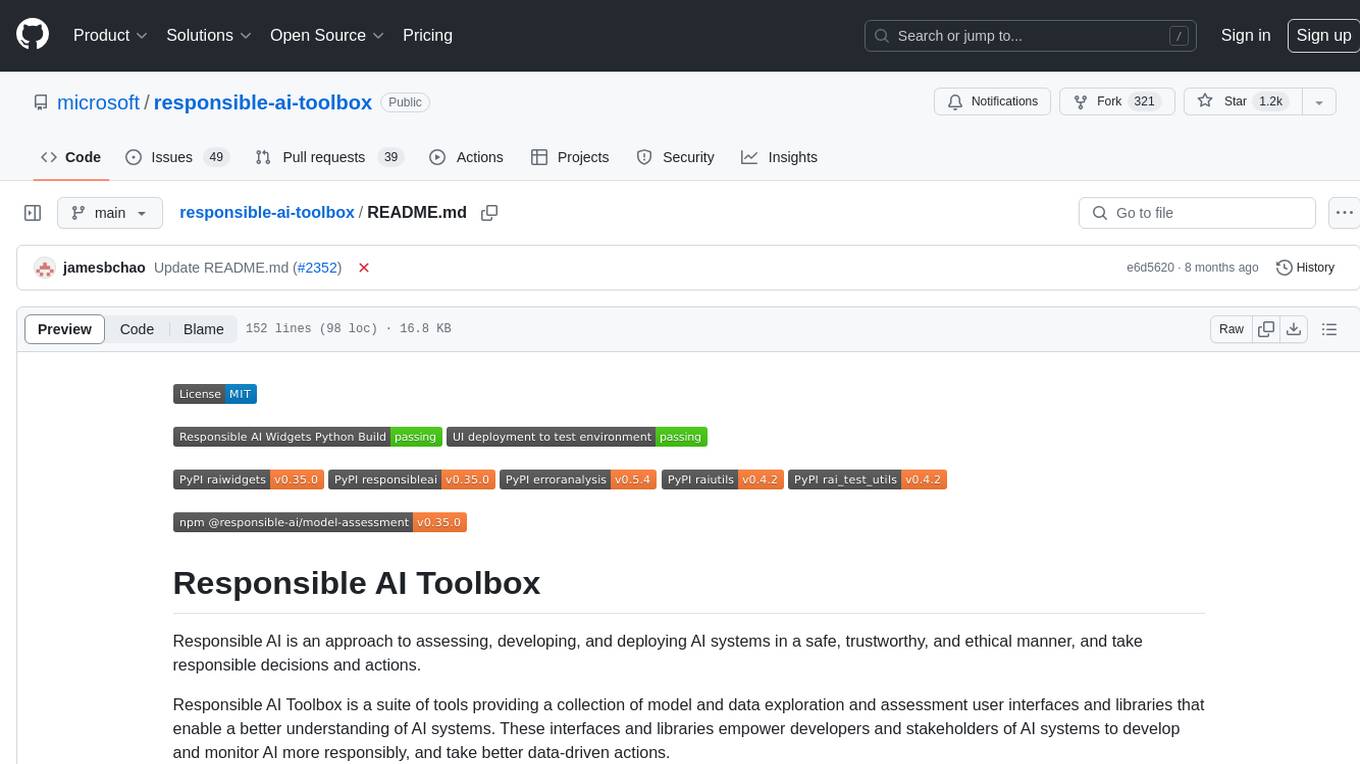
Responsible AI Toolbox is a suite of tools providing model and data exploration and assessment interfaces and libraries for understanding AI systems. It empowers developers and stakeholders to develop and monitor AI responsibly, enabling better data-driven actions. The toolbox includes visualization widgets for model assessment, error analysis, interpretability, fairness assessment, and mitigations library. It also offers a JupyterLab extension for managing machine learning experiments and a library for measuring gender bias in NLP datasets.
README:
Responsible AI is an approach to assessing, developing, and deploying AI systems in a safe, trustworthy, and ethical manner, and take responsible decisions and actions.
Responsible AI Toolbox is a suite of tools providing a collection of model and data exploration and assessment user interfaces and libraries that enable a better understanding of AI systems. These interfaces and libraries empower developers and stakeholders of AI systems to develop and monitor AI more responsibly, and take better data-driven actions.
The Toolbox consists of three repositories:
Repository | Tools Covered |
---|---|
Responsible-AI-Toolbox Repository (Here) | This repository contains four visualization widgets for model assessment and decision making: 1. Responsible AI dashboard, a single pane of glass bringing together several mature Responsible AI tools from the toolbox for a holistic responsible assessment and debugging of models and making informed business decisions. With this dashboard, you can identify model errors, diagnose why those errors are happening, and mitigate them. Moreover, the causal decision-making capabilities provide actionable insights to your stakeholders and customers. 2. Error Analysis dashboard, for identifying model errors and discovering cohorts of data for which the model underperforms. 3. Interpretability dashboard, for understanding model predictions. This dashboard is powered by InterpretML. 4. Fairness dashboard, for understanding model’s fairness issues using various group-fairness metrics across sensitive features and cohorts. This dashboard is powered by Fairlearn. |
Responsible-AI-Toolbox-Mitigations Repository | The Responsible AI Mitigations Library helps AI practitioners explore different measurements and mitigation steps that may be most appropriate when the model underperforms for a given data cohort. The library currently has two modules: 1. DataProcessing, which offers mitigation techniques for improving model performance for specific cohorts. 2. DataBalanceAnalysis, which provides metrics for diagnosing errors that originate from data imbalance either on class labels or feature values. 3. Cohort: provides classes for handling and managing cohorts, which allows the creation of custom pipelines for each cohort in an easy and intuitive interface. The module also provides techniques for learning different decoupled estimators (models) for different cohorts and combining them in a way that optimizes different definitions of group fairness. |
Responsible-AI-Tracker Repository | Responsible AI Toolbox Tracker is a JupyterLab extension for managing, tracking, and comparing results of machine learning experiments for model improvement. Using this extension, users can view models, code, and visualization artifacts within the same framework enabling therefore fast model iteration and evaluation processes. Main functionalities include: 1. Managing and linking model improvement artifacts 2. Disaggregated model evaluation and comparisons 3. Integration with the Responsible AI Mitigations library 4. Integration with mlflow |
Responsible-AI-Toolbox-GenBit Repository | The Responsible AI Gender Bias (GenBit) Library helps AI practitioners measure gender bias in Natural Language Processing (NLP) datasets. The main goal of GenBit is to analyze your text corpora and compute metrics that give insights into the gender bias present in a corpus. |
Responsible AI dashboard is a single pane of glass, enabling you to easily flow through different stages of model debugging and decision-making. This customizable experience can be taken in a multitude of directions, from analyzing the model or data holistically, to conducting a deep dive or comparison on cohorts of interest, to explaining and perturbing model predictions for individual instances, and to informing users on business decisions and actions.
In order to achieve these capabilities, the dashboard integrates together ideas and technologies from several open-source toolkits in the areas of
-
Error Analysis powered by Error Analysis, which identifies cohorts of data with higher error rate than the overall benchmark. These discrepancies might occur when the system or model underperforms for specific demographic groups or infrequently observed input conditions in the training data.
-
Fairness Assessment powered by Fairlearn, which identifies which groups of people may be disproportionately negatively impacted by an AI system and in what ways.
-
Model Interpretability powered by InterpretML, which explains blackbox models, helping users understand their model's global behavior, or the reasons behind individual predictions.
-
Counterfactual Analysis powered by DiCE, which shows feature-perturbed versions of the same datapoint who would have received a different prediction outcome, e.g., Taylor's loan has been rejected by the model. But they would have received the loan if their income was higher by $10,000.
-
Causal Analysis powered by EconML, which focuses on answering What If-style questions to apply data-driven decision-making – how would revenue be affected if a corporation pursues a new pricing strategy? Would a new medication improve a patient’s condition, all else equal?
-
Data Balance powered by Responsible AI, which helps users gain an overall understanding of their data, identify features receiving the positive outcome more than others, and visualize feature distributions.
Responsible AI dashboard is designed to achieve the following goals:
- To help further accelerate engineering processes in machine learning by enabling practitioners to design customizable workflows and tailor Responsible AI dashboards that best fit with their model assessment and data-driven decision making scenarios.
- To help model developers create end to end and fluid debugging experiences and navigate seamlessly through error identification and diagnosis by using interactive visualizations that identify errors, inspect the data, generate global and local explanations models, and potentially inspect problematic examples.
- To help business stakeholders explore causal relationships in the data and take informed decisions in the real world.
This repository contains the Jupyter notebooks with examples to showcase how to use this widget. Get started here.
Use the following pip command to install the Responsible AI Toolbox.
If running in jupyter, please make sure to restart the jupyter kernel after installing.
pip install raiwidgets
The Responsible AI Toolbox’s strength lies in its customizability. It empowers users to design tailored, end-to-end model debugging and decision-making workflows that address their particular needs. Need some inspiration? Here are some examples of how Toolbox components can be put together to analyze scenarios in different ways:
Please note that model overview (including fairness analysis) and data explorer components are activated by default!
Responsible AI Dashboard Flow | Use Case |
---|---|
Model Overview -> Error Analysis -> Data Explorer | To identify model errors and diagnose them by understanding the underlying data distribution |
Model Overview -> Fairness Assessment -> Data Explorer | To identify model fairness issues and diagnose them by understanding the underlying data distribution |
Model Overview -> Error Analysis -> Counterfactuals Analysis and What-If | To diagnose errors in individual instances with counterfactual analysis (minimum change to lead to a different model prediction) |
Model Overview -> Data Explorer -> Data Balance | To understand the root cause of errors and fairness issues introduced via data imbalances or lack of representation of a particular data cohort |
Model Overview -> Interpretability | To diagnose model errors through understanding how the model has made its predictions |
Data Explorer -> Causal Inference | To distinguish between correlations and causations in the data or decide the best treatments to apply to see a positive outcome |
Interpretability -> Causal Inference | To learn whether the factors that model has used for decision making has any causal effect on the real-world outcome. |
Data Explorer -> Counterfactuals Analysis and What-If | To address customer questions about what they can do next time to get a different outcome from an AI. |
Data Explorer -> Data Balance | To gain an overall understanding of the data, identify features receiving the positive outcome more than others, and visualize feature distributions |
Tabular Examples:
- Try the tool: make decisions for house improvements (decision making)
- Try the tool: provide recommendations to patients using diabetes data (decision making)
- Try the tool: model debugging of a census income prediction model (classification)
- Try the tool: model debugging of a housing price prediction model (classification)
- Try the tool: model debugging of a diabetes progression prediction model (regression)
Text Examples:
- Try the tool: model debugging of an OpenAI Question Answering model on SQuAD
- Try the tool: model debugging of a HuggingFace Question Answering model on SQuAD
- Try the tool: model debugging of a DBPedia text classification model
- Try the tool: model debugging of a binary text classification model
- Try the tool: model debugging of a COVID-19 multilabel text classification model
Vision Examples:
- Try the tool: model debugging of a fridge image classification model
- Try the tool: model debugging of a fridge multilabel image classification model
- Try the tool: model debugging of a fridge object detection model
This Responsible AI Toolbox API supports models that are trained on datasets in Python numpy.ndarray
, pandas.DataFrame
, iml.datatypes.DenseData
, or scipy.sparse.csr_matrix
format.
The explanation functions of Interpret-Community accept both models and pipelines as input as long as the model or pipeline implements a predict
or predict_proba
function that conforms to the Scikit convention. If not compatible, you can wrap your model's prediction function into a wrapper function that transforms the output into the format that is supported (predict or predict_proba of Scikit), and pass that wrapper function to your selected interpretability techniques.
If a pipeline script is provided, the explanation function assumes that the running pipeline script returns a prediction. The repository also supports models trained via PyTorch, TensorFlow, and Keras deep learning frameworks.
Tools within the Responsible AI Toolbox can also be used with AI models offered as APIs by providers such as Azure Cognitive Services. To see example use cases, see the folders below:
For Tasks:
Click tags to check more tools for each tasksFor Jobs:
Alternative AI tools for responsible-ai-toolbox
Similar Open Source Tools
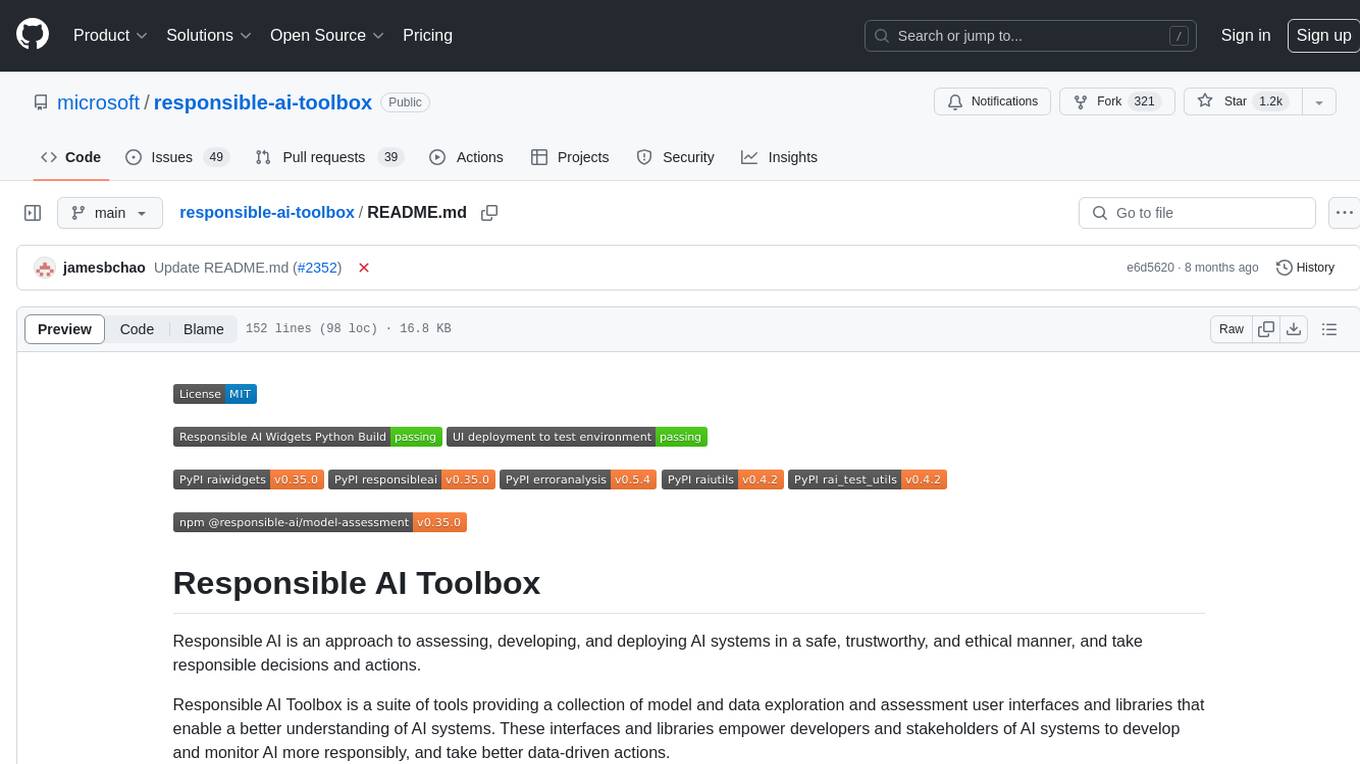
responsible-ai-toolbox
Responsible AI Toolbox is a suite of tools providing model and data exploration and assessment interfaces and libraries for understanding AI systems. It empowers developers and stakeholders to develop and monitor AI responsibly, enabling better data-driven actions. The toolbox includes visualization widgets for model assessment, error analysis, interpretability, fairness assessment, and mitigations library. It also offers a JupyterLab extension for managing machine learning experiments and a library for measuring gender bias in NLP datasets.
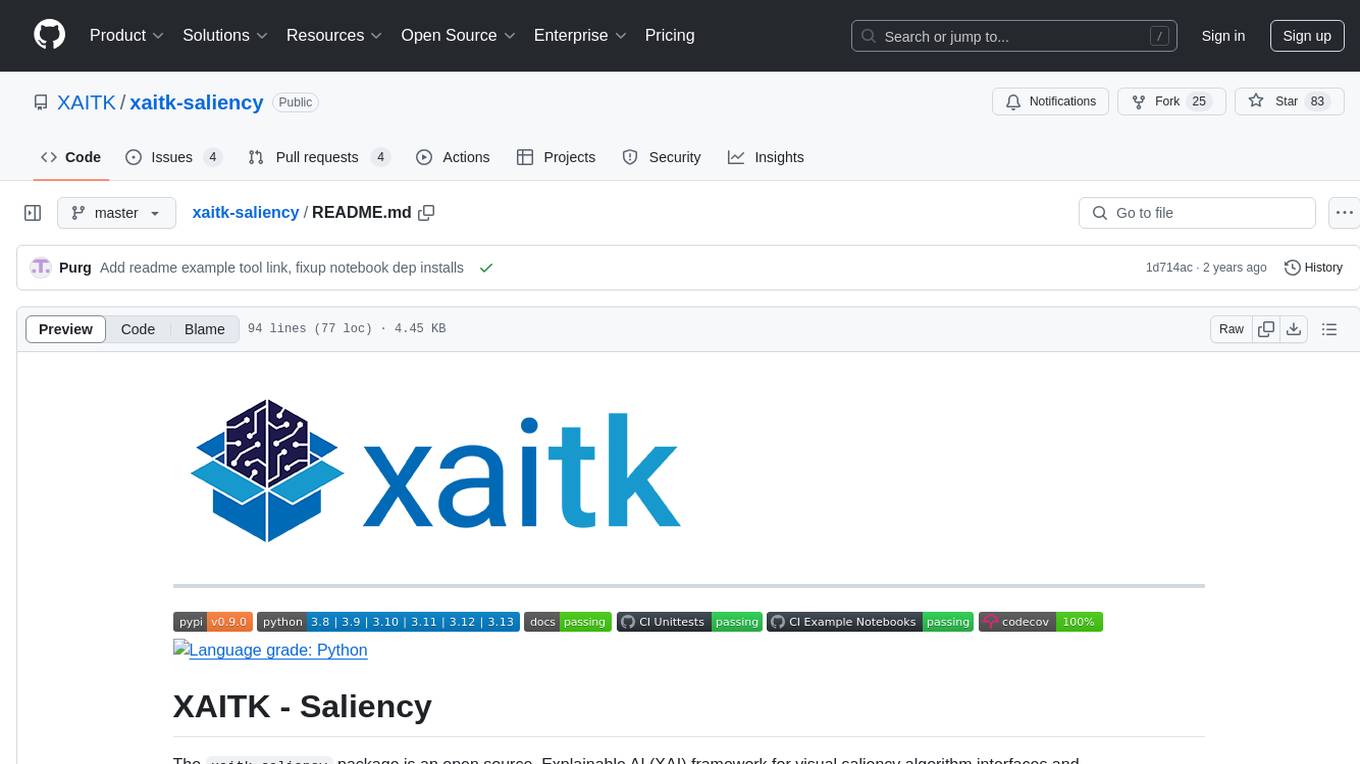
xaitk-saliency
The `xaitk-saliency` package is an open source Explainable AI (XAI) framework for visual saliency algorithm interfaces and implementations, designed for analytics and autonomy applications. It provides saliency algorithms for various image understanding tasks such as image classification, image similarity, object detection, and reinforcement learning. The toolkit targets data scientists and developers who aim to incorporate visual saliency explanations into their workflow or product, offering both direct accessibility for experimentation and modular integration into systems and applications through Strategy and Adapter patterns. The package includes documentation, examples, and a demonstration tool for visual saliency generation in a user-interface.
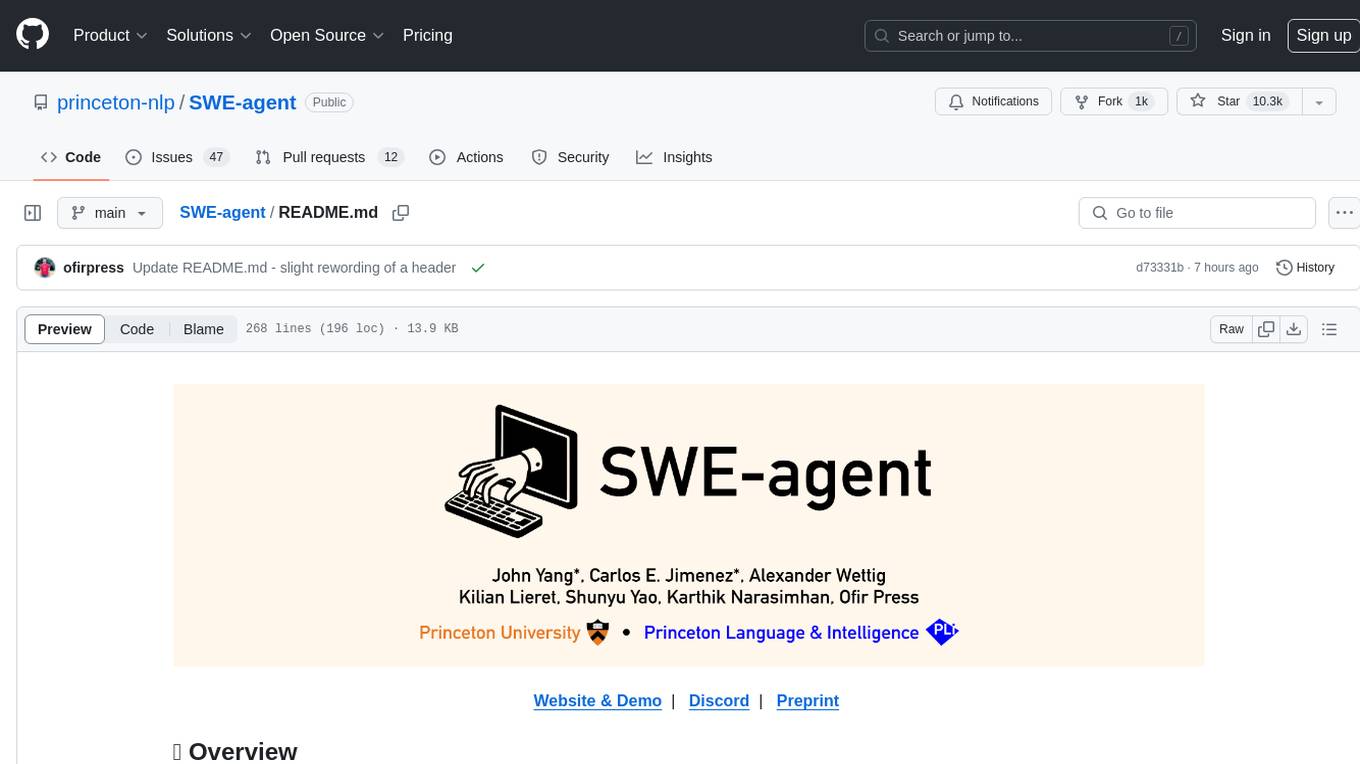
SWE-agent
SWE-agent is a tool that turns language models (e.g. GPT-4) into software engineering agents capable of fixing bugs and issues in real GitHub repositories. It achieves state-of-the-art performance on the full test set by resolving 12.29% of issues. The tool is built and maintained by researchers from Princeton University. SWE-agent provides a command line tool and a graphical web interface for developers to interact with. It introduces an Agent-Computer Interface (ACI) to facilitate browsing, viewing, editing, and executing code files within repositories. The tool includes features such as a linter for syntax checking, a specialized file viewer, and a full-directory string searching command to enhance the agent's capabilities. SWE-agent aims to improve prompt engineering and ACI design to enhance the performance of language models in software engineering tasks.
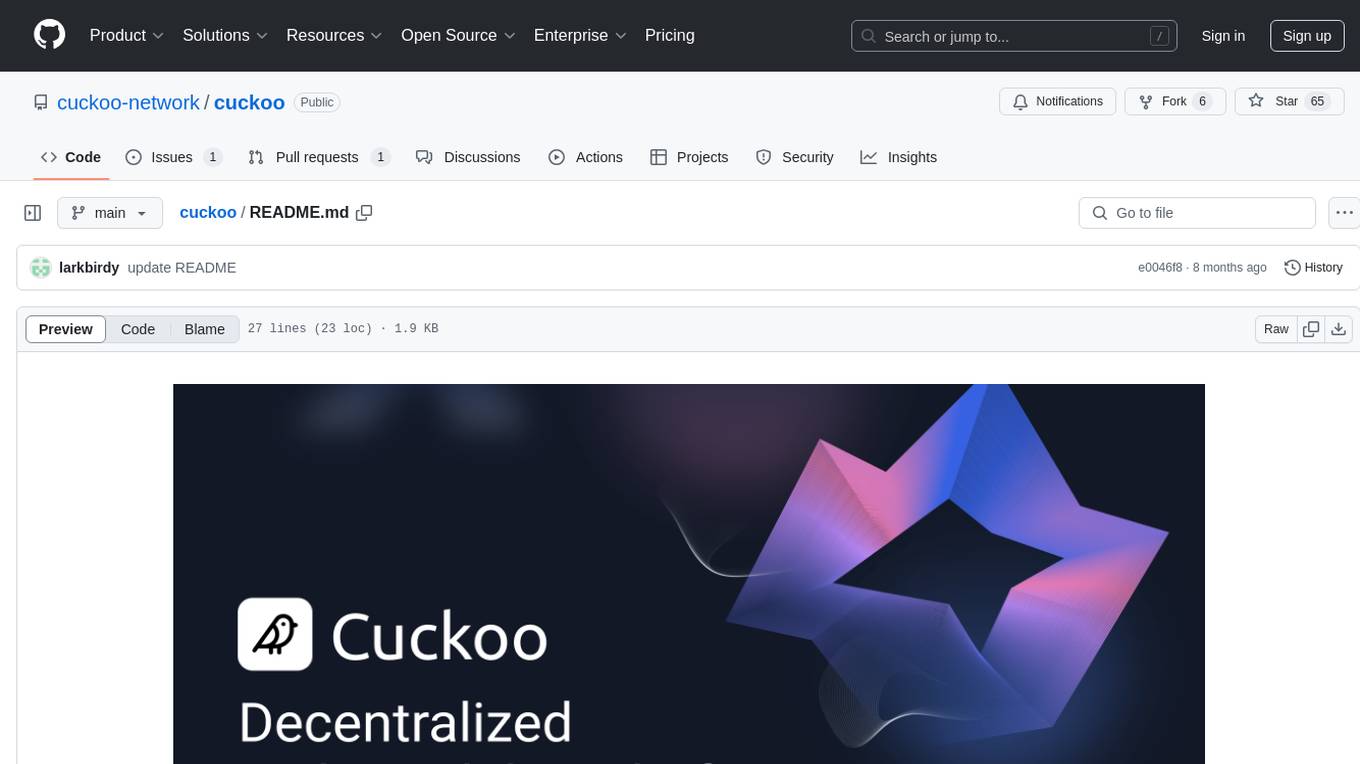
cuckoo
Cuckoo is a Decentralized AI Platform that focuses on GPU-sharing for text-to-image generation and LLM inference. It provides a platform for users to generate images using Telegram or Discord.
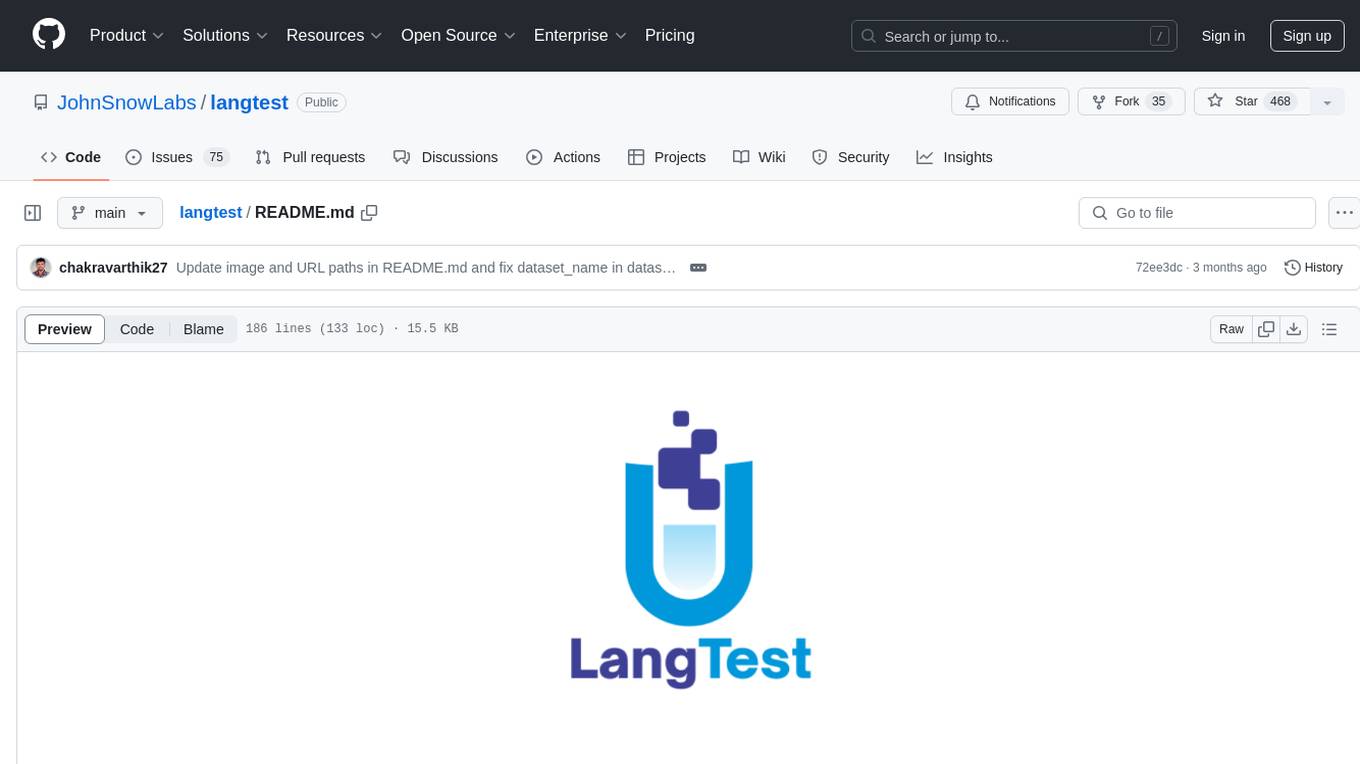
langtest
LangTest is a comprehensive evaluation library for custom LLM and NLP models. It aims to deliver safe and effective language models by providing tools to test model quality, augment training data, and support popular NLP frameworks. LangTest comes with benchmark datasets to challenge and enhance language models, ensuring peak performance in various linguistic tasks. The tool offers more than 60 distinct types of tests with just one line of code, covering aspects like robustness, bias, representation, fairness, and accuracy. It supports testing LLMS for question answering, toxicity, clinical tests, legal support, factuality, sycophancy, and summarization.
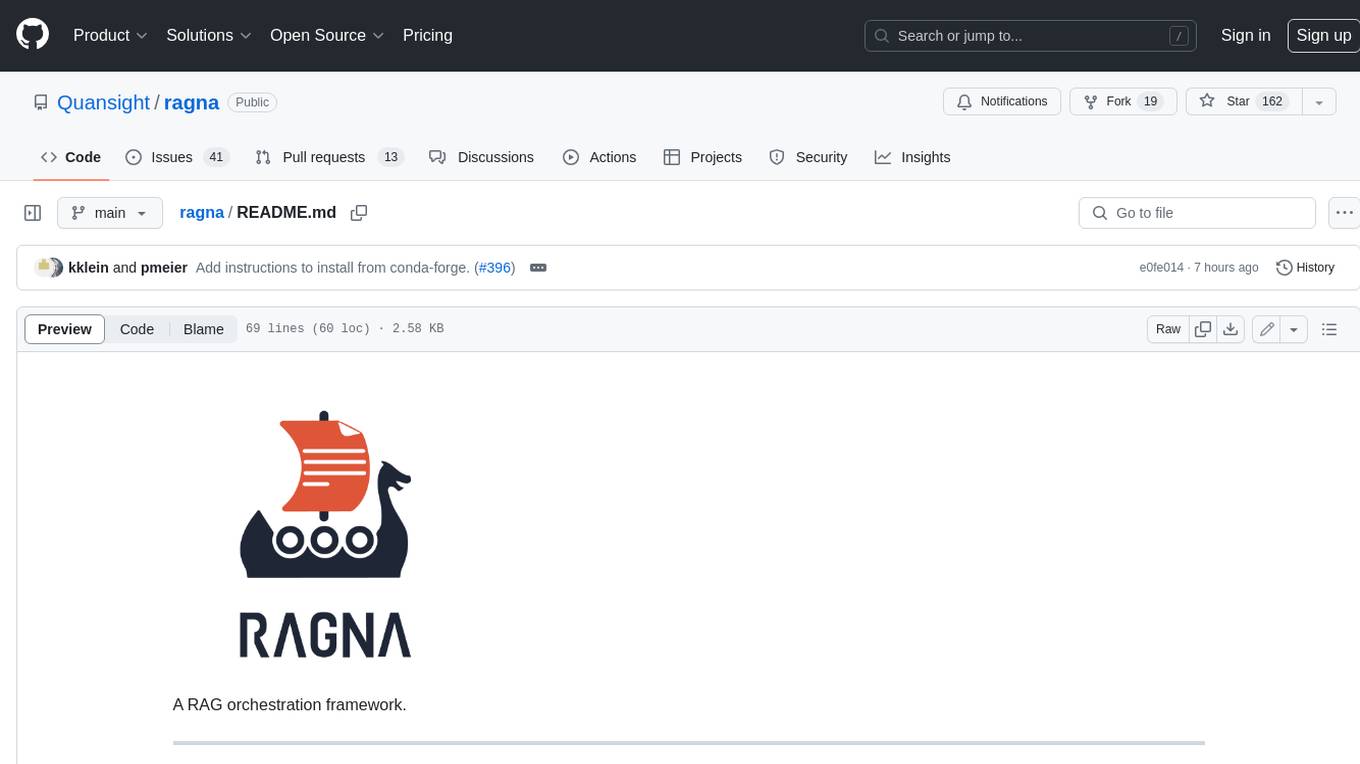
ragna
Ragna is a RAG orchestration framework designed for managing workflows and orchestrating tasks. It provides a comprehensive set of features for users to streamline their processes and automate repetitive tasks. With Ragna, users can easily create, schedule, and monitor workflows, making it an ideal tool for teams and individuals looking to improve their productivity and efficiency. The framework offers extensive documentation, community support, and a user-friendly interface, making it accessible to users of all skill levels. Whether you are a developer, data scientist, or project manager, Ragna can help you simplify your workflow management and boost your overall performance.
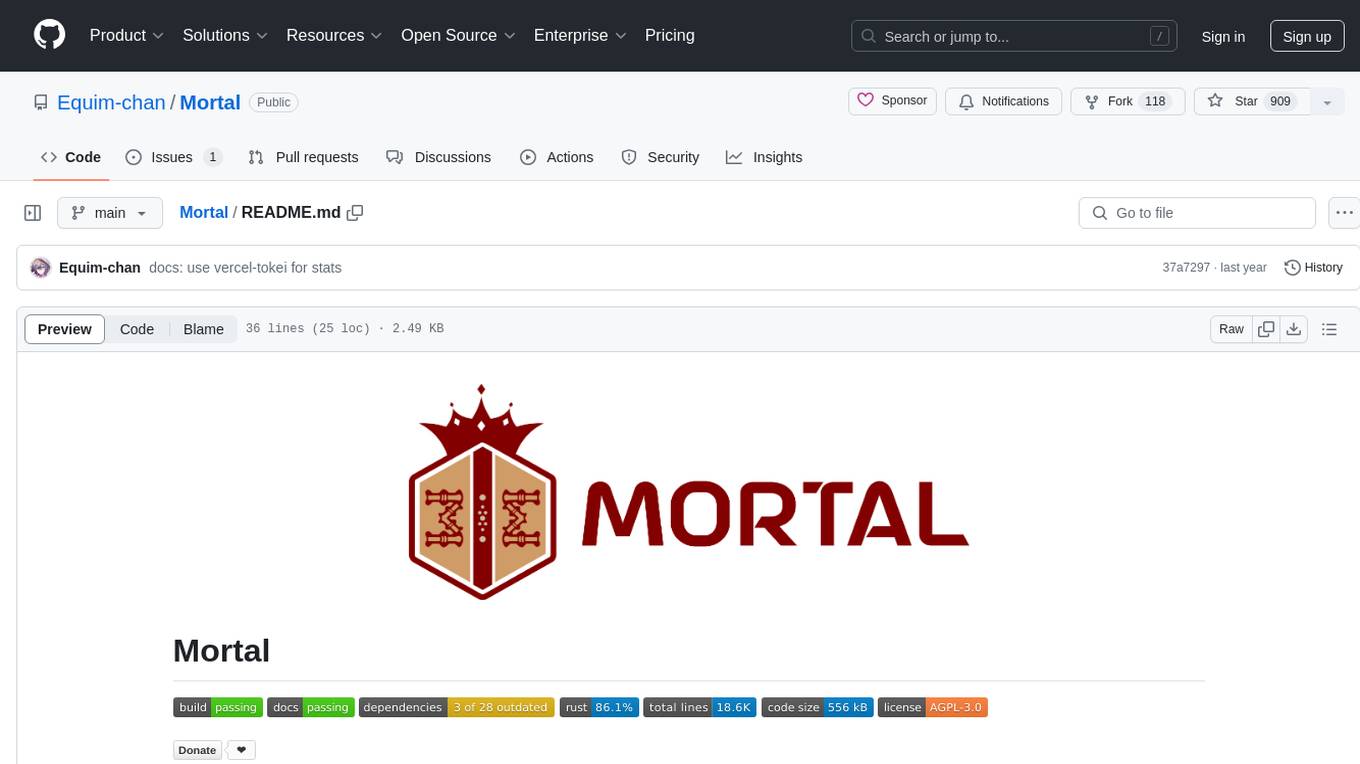
Mortal
Mortal (凡夫) is a free and open source AI for Japanese mahjong, powered by deep reinforcement learning. It provides a comprehensive solution for playing Japanese mahjong with AI assistance. The project focuses on utilizing deep reinforcement learning techniques to enhance gameplay and decision-making in Japanese mahjong. Mortal offers a user-friendly interface and detailed documentation to assist users in understanding and utilizing the AI effectively. The project is actively maintained and welcomes contributions from the community to further improve the AI's capabilities and performance.
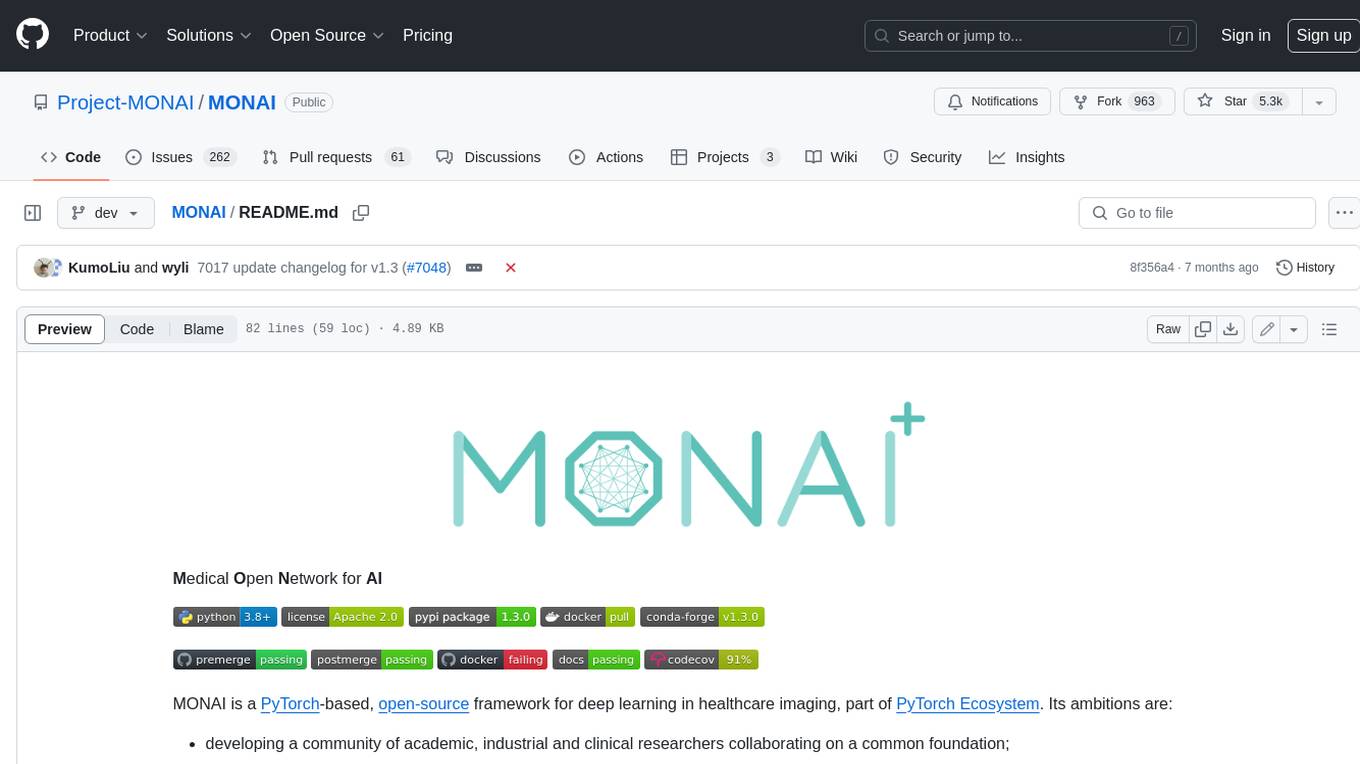
MONAI
MONAI is a PyTorch-based, open-source framework for deep learning in healthcare imaging. It provides a comprehensive set of tools for medical image analysis, including data preprocessing, model training, and evaluation. MONAI is designed to be flexible and easy to use, making it a valuable resource for researchers and developers in the field of medical imaging.
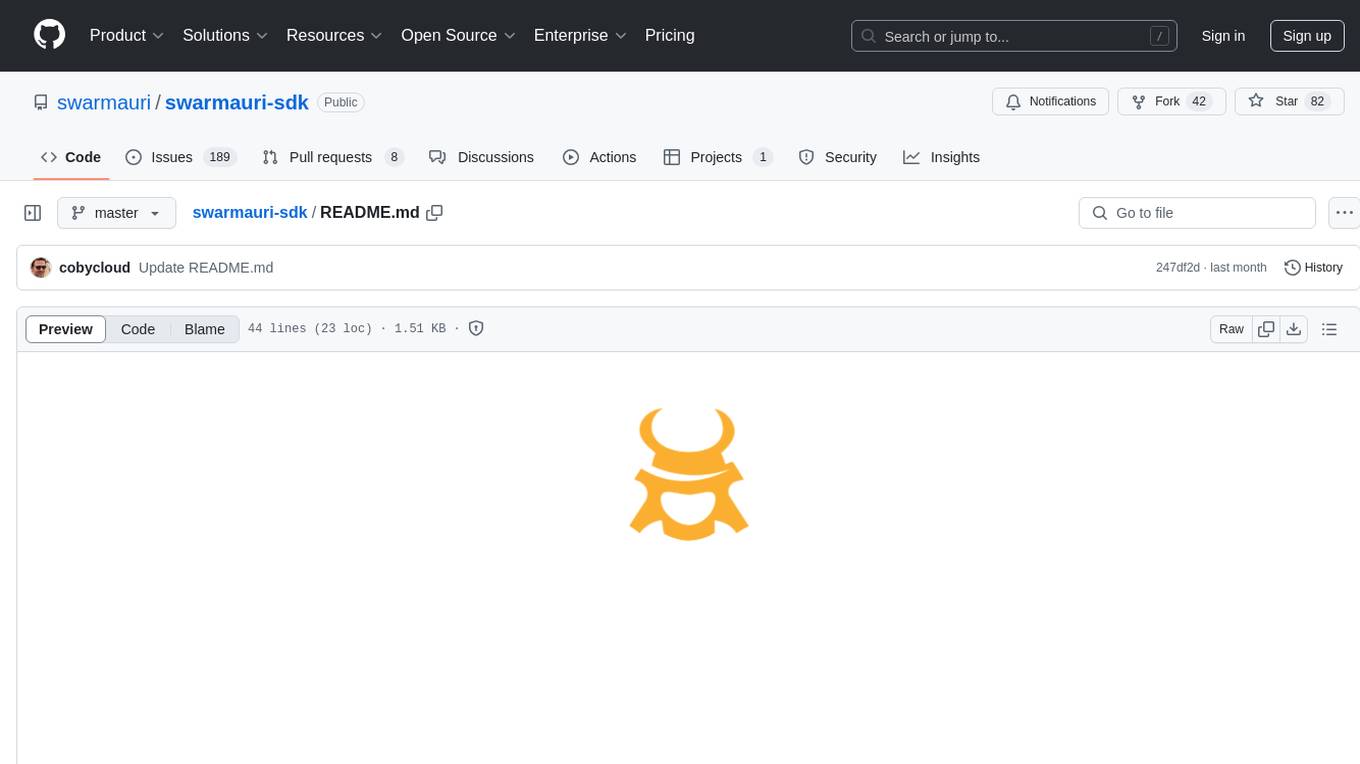
swarmauri-sdk
Swarmauri SDK is a repository containing core interfaces, standard ABCs, and standard concrete references of the SwarmaURI Framework. It provides a set of tools and functionalities for developers to work with the SwarmaURI ecosystem. The SDK aims to streamline the development process and enhance the interoperability of applications within the framework. Developers can easily integrate SwarmaURI features into their projects by leveraging the resources available in this repository.
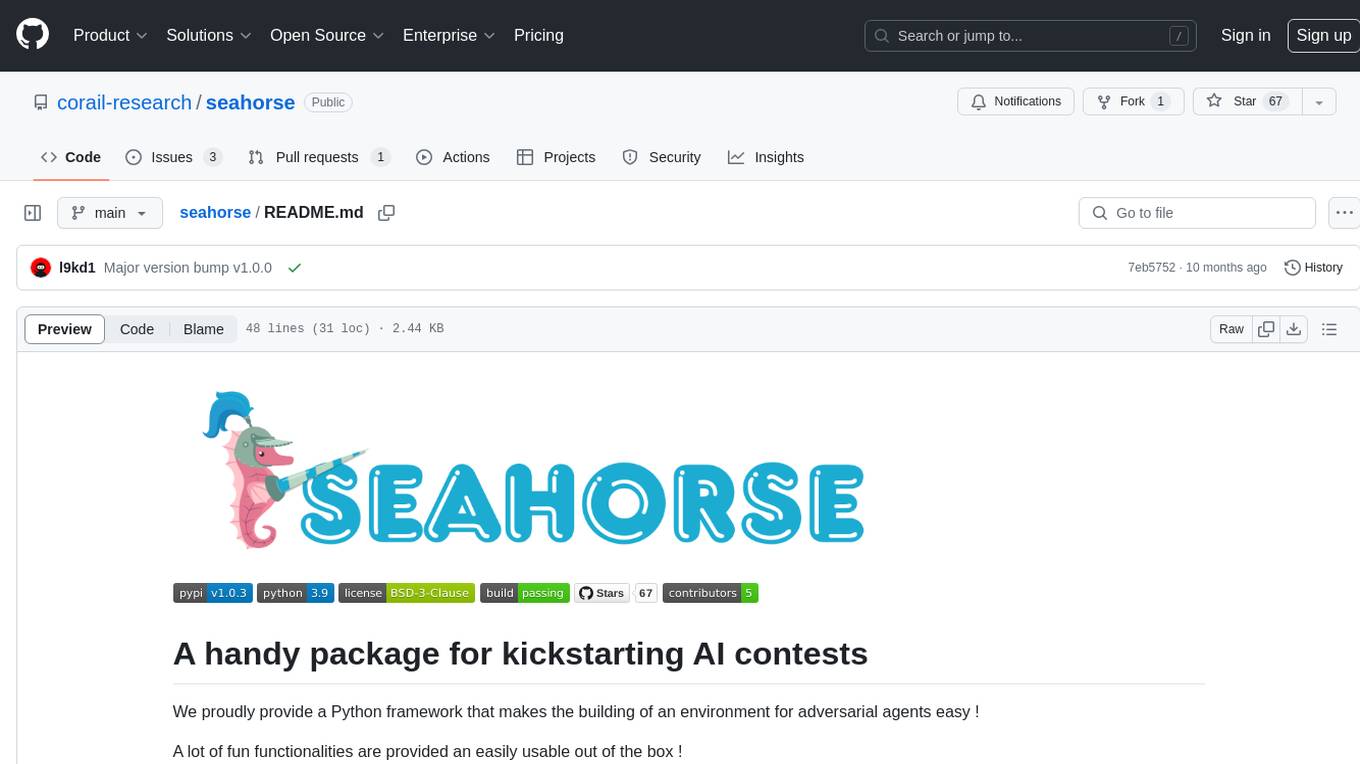
seahorse
A handy package for kickstarting AI contests. This Python framework simplifies the creation of an environment for adversarial agents, offering various functionalities for game setup, playing against remote agents, data generation, and contest organization. The package is user-friendly and provides easy-to-use features out of the box. Developed by an enthusiastic team of M.Sc candidates at Polytechnique Montréal, 'seahorse' is distributed under the 3-Clause BSD License.
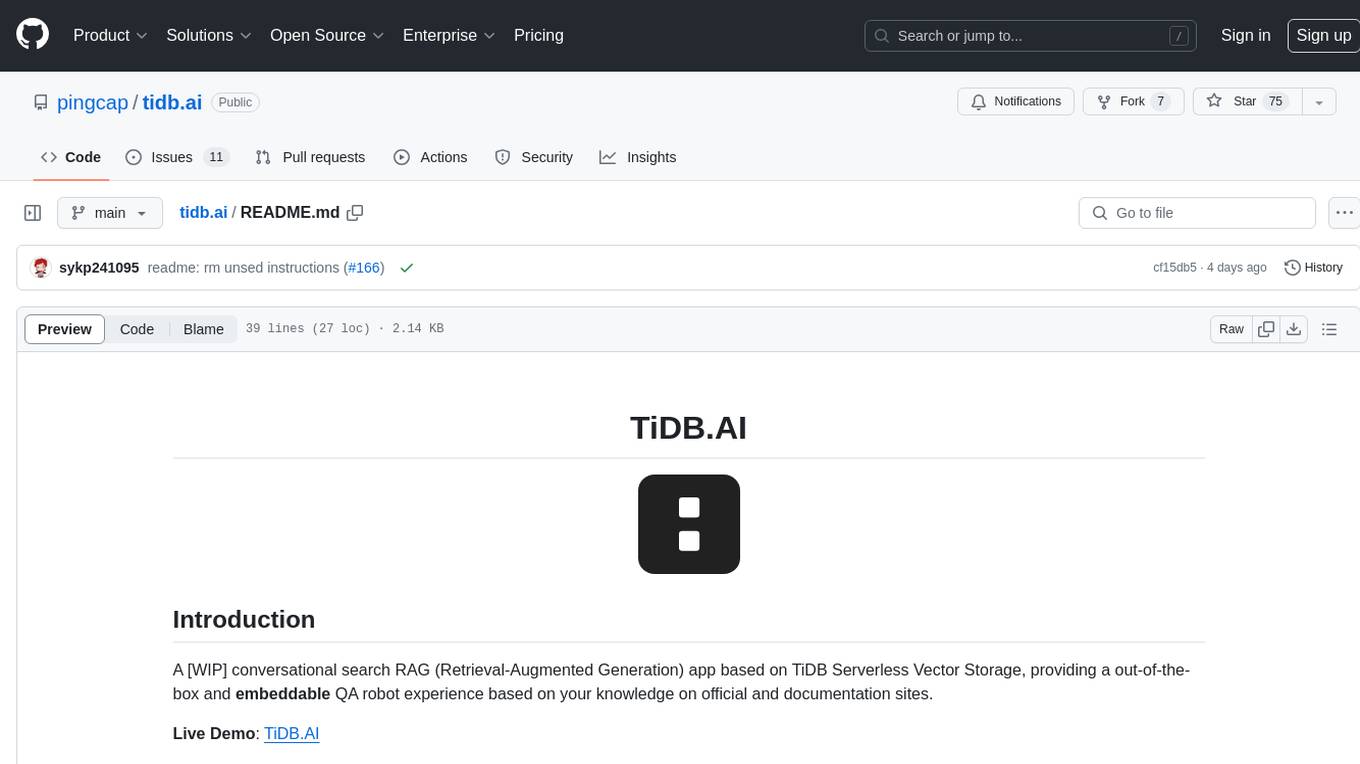
tidb.ai
TiDB.AI is a conversational search RAG (Retrieval-Augmented Generation) app based on TiDB Serverless Vector Storage. It provides an out-of-the-box and embeddable QA robot experience based on knowledge from official and documentation sites. The platform features a Perplexity-style Conversational Search page with an advanced built-in website crawler for comprehensive coverage. Users can integrate an embeddable JavaScript snippet into their website for instant responses to product-related queries. The tech stack includes Next.js, TypeScript, Tailwind CSS, shadcn/ui for design, TiDB for database storage, Kysely for SQL query building, NextAuth.js for authentication, Vercel for deployments, and LlamaIndex for the RAG framework. TiDB.AI is open-source under the Apache License, Version 2.0.
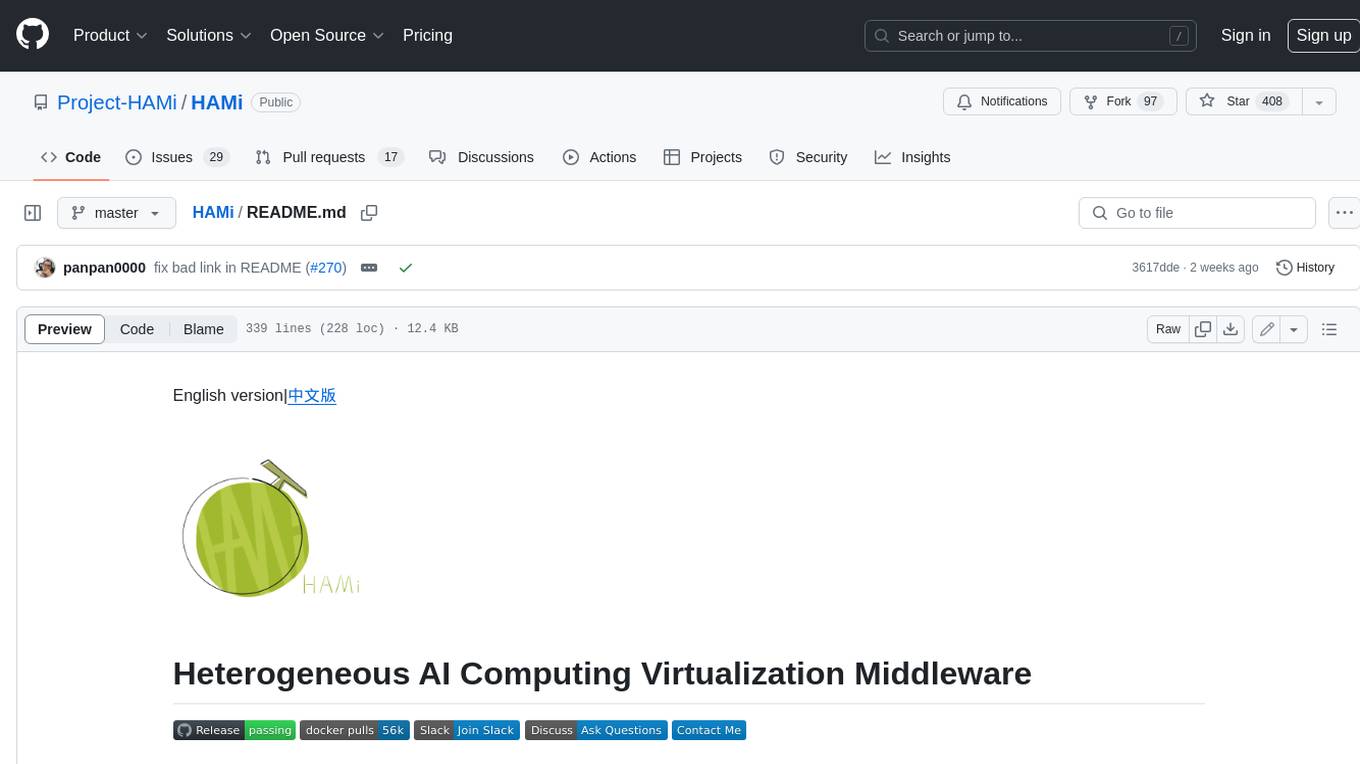
HAMi
HAMi is a Heterogeneous AI Computing Virtualization Middleware designed to manage Heterogeneous AI Computing Devices in a Kubernetes cluster. It allows for device sharing, device memory control, device type specification, and device UUID specification. The tool is easy to use and does not require modifying task YAML files. It includes features like hard limits on device memory, partial device allocation, streaming multiprocessor limits, and core usage specification. HAMi consists of components like a mutating webhook, scheduler extender, device plugins, and in-container virtualization techniques. It is suitable for scenarios requiring device sharing, specific device memory allocation, GPU balancing, low utilization optimization, and scenarios needing multiple small GPUs. The tool requires prerequisites like NVIDIA drivers, CUDA version, nvidia-docker, Kubernetes version, glibc version, and helm. Users can install, upgrade, and uninstall HAMi, submit tasks, and monitor cluster information. The tool's roadmap includes supporting additional AI computing devices, video codec processing, and Multi-Instance GPUs (MIG).
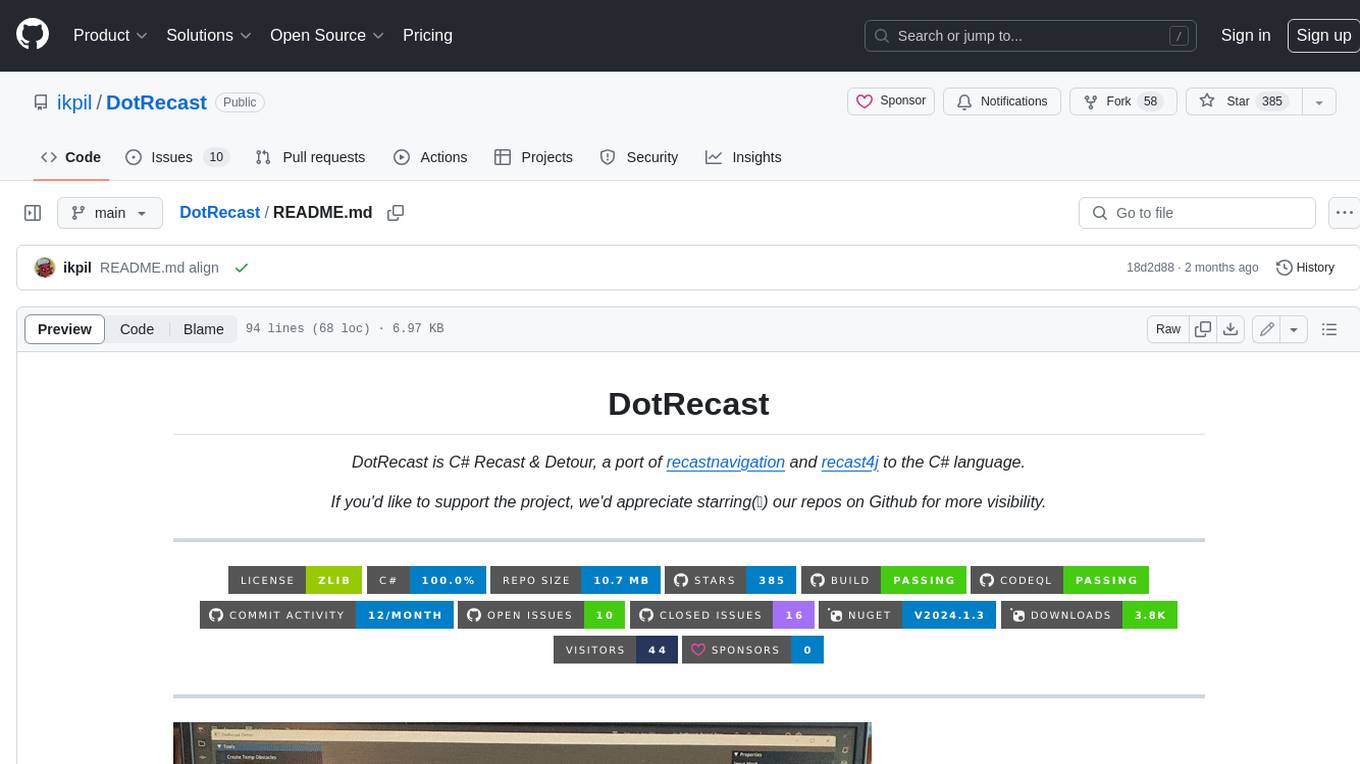
DotRecast
DotRecast is a C# port of Recast & Detour, a navigation library used in many AAA and indie games and engines. It provides automatic navmesh generation, fast turnaround times, detailed customization options, and is dependency-free. Recast Navigation is divided into multiple modules, each contained in its own folder: - DotRecast.Core: Core utils - DotRecast.Recast: Navmesh generation - DotRecast.Detour: Runtime loading of navmesh data, pathfinding, navmesh queries - DotRecast.Detour.TileCache: Navmesh streaming. Useful for large levels and open-world games - DotRecast.Detour.Crowd: Agent movement, collision avoidance, and crowd simulation - DotRecast.Detour.Dynamic: Robust support for dynamic nav meshes combining pre-built voxels with dynamic objects which can be freely added and removed - DotRecast.Detour.Extras: Simple tool to import navmeshes created with A* Pathfinding Project - DotRecast.Recast.Toolset: All modules - DotRecast.Recast.Demo: Standalone, comprehensive demo app showcasing all aspects of Recast & Detour's functionality - Tests: Unit tests Recast constructs a navmesh through a multi-step mesh rasterization process: 1. First Recast rasterizes the input triangle meshes into voxels. 2. Voxels in areas where agents would not be able to move are filtered and removed. 3. The walkable areas described by the voxel grid are then divided into sets of polygonal regions. 4. The navigation polygons are generated by re-triangulating the generated polygonal regions into a navmesh. You can use Recast to build a single navmesh, or a tiled navmesh. Single meshes are suitable for many simple, static cases and are easy to work with. Tiled navmeshes are more complex to work with but better support larger, more dynamic environments. Tiled meshes enable advanced Detour features like re-baking, hierarchical path-planning, and navmesh data-streaming.
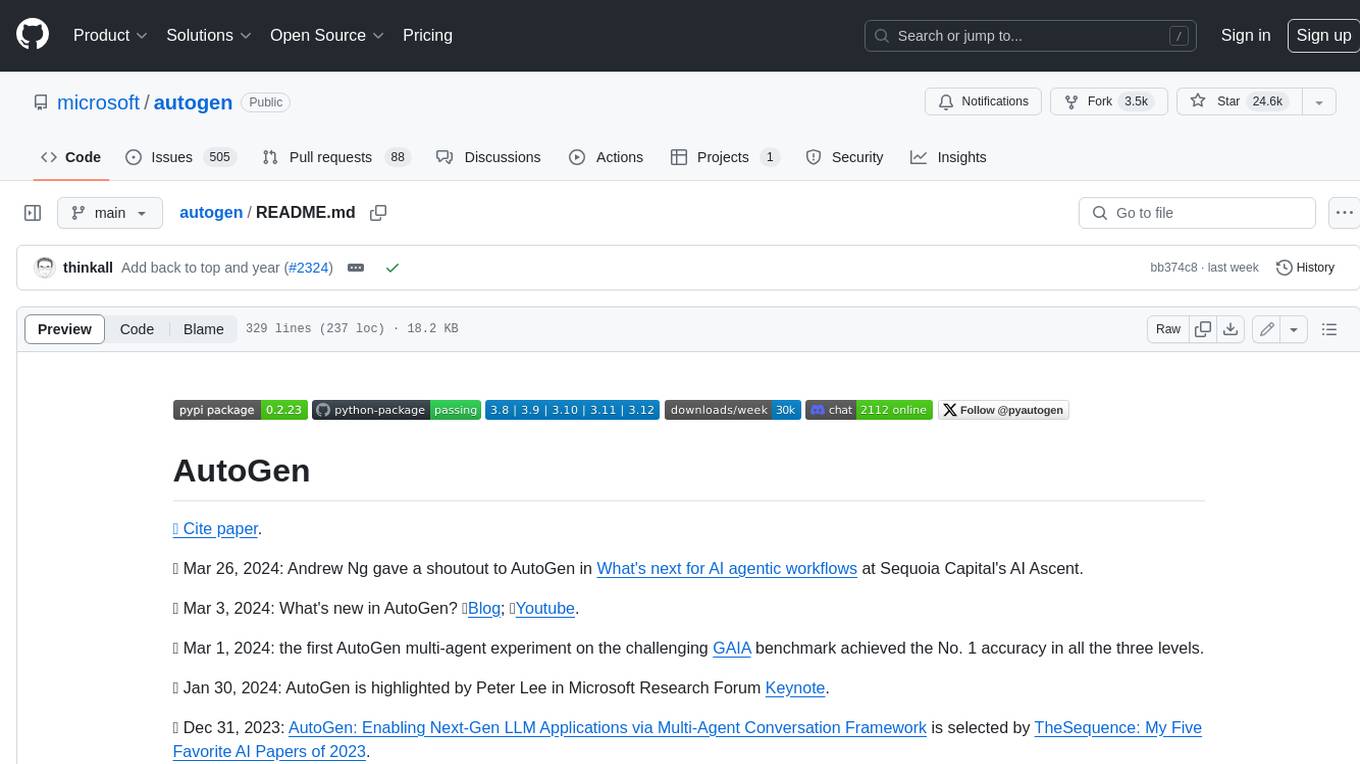
autogen
AutoGen is a framework that enables the development of LLM applications using multiple agents that can converse with each other to solve tasks. AutoGen agents are customizable, conversable, and seamlessly allow human participation. They can operate in various modes that employ combinations of LLMs, human inputs, and tools.
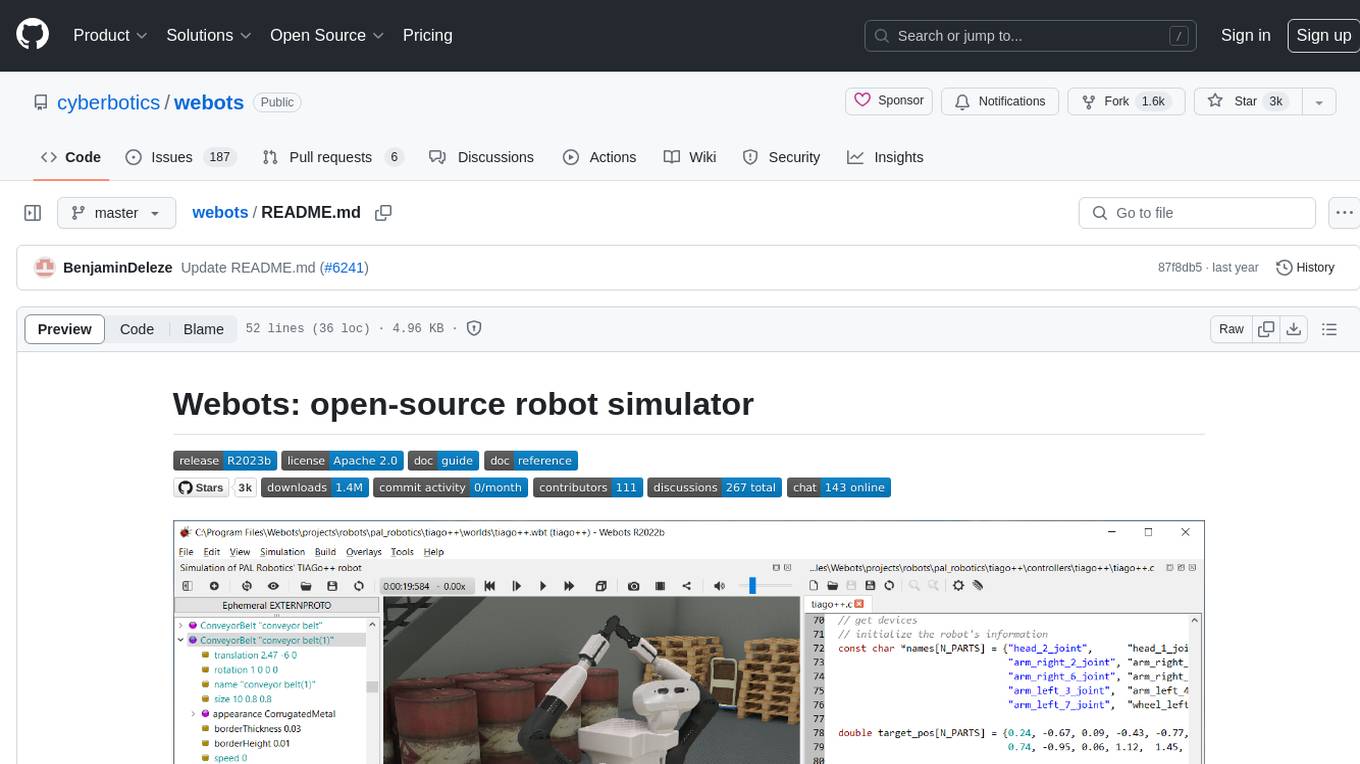
webots
Webots is an open-source robot simulator that provides a complete development environment to model, program, and simulate robots, vehicles, and mechanical systems. It was originally designed at EPFL in 1996 and further developed and commercialized by Cyberbotics since 1998. Webots was open-sourced in December 2018 and continues to be developed by Cyberbotics with paid customer support, training, and consulting services for industry and academic research projects.
For similar tasks
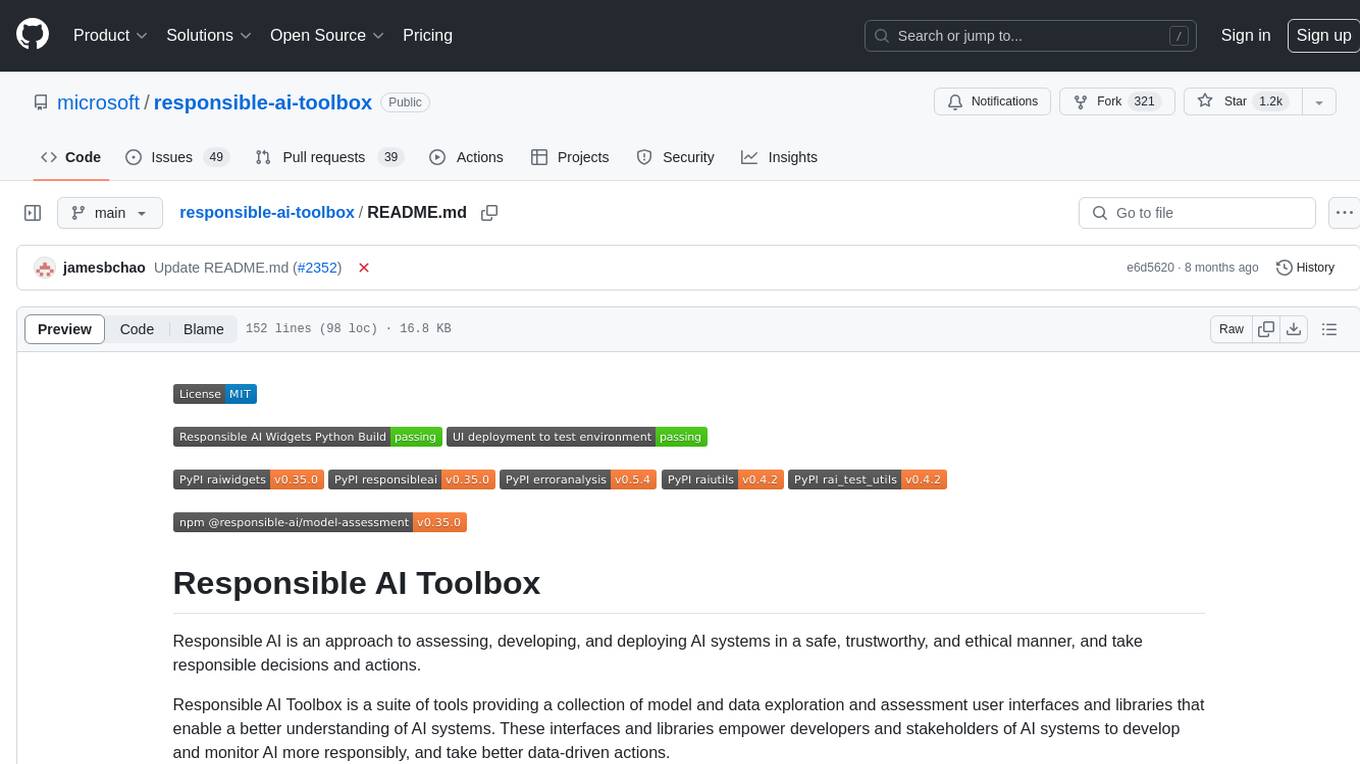
responsible-ai-toolbox
Responsible AI Toolbox is a suite of tools providing model and data exploration and assessment interfaces and libraries for understanding AI systems. It empowers developers and stakeholders to develop and monitor AI responsibly, enabling better data-driven actions. The toolbox includes visualization widgets for model assessment, error analysis, interpretability, fairness assessment, and mitigations library. It also offers a JupyterLab extension for managing machine learning experiments and a library for measuring gender bias in NLP datasets.
For similar jobs
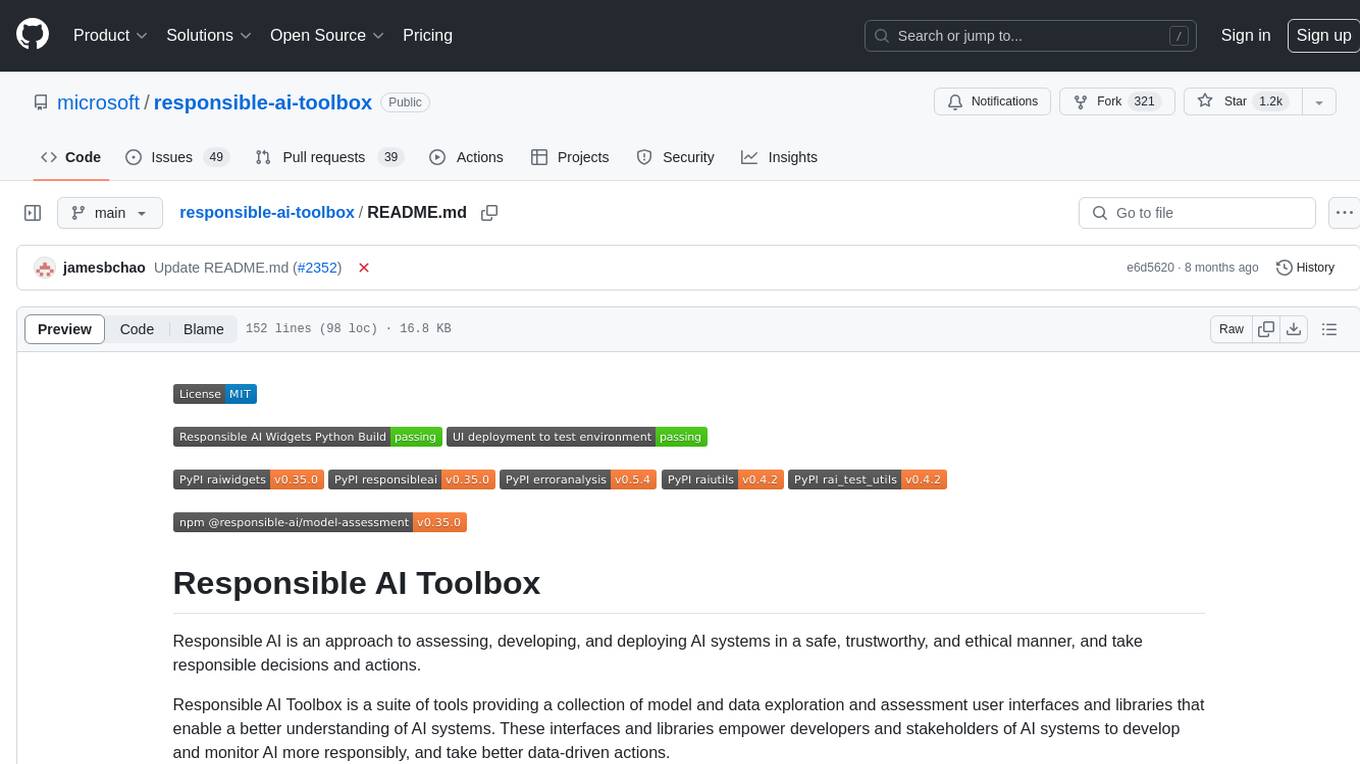
responsible-ai-toolbox
Responsible AI Toolbox is a suite of tools providing model and data exploration and assessment interfaces and libraries for understanding AI systems. It empowers developers and stakeholders to develop and monitor AI responsibly, enabling better data-driven actions. The toolbox includes visualization widgets for model assessment, error analysis, interpretability, fairness assessment, and mitigations library. It also offers a JupyterLab extension for managing machine learning experiments and a library for measuring gender bias in NLP datasets.
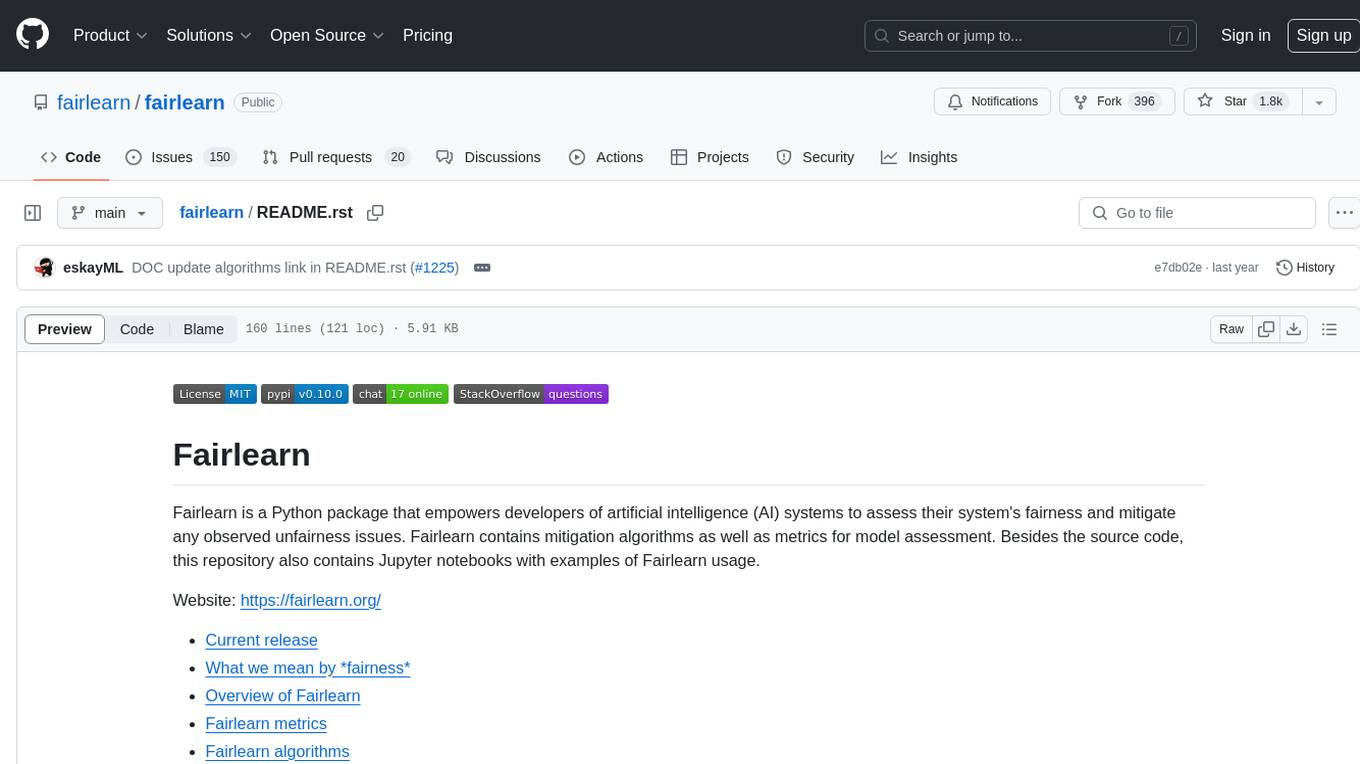
fairlearn
Fairlearn is a Python package designed to help developers assess and mitigate fairness issues in artificial intelligence (AI) systems. It provides mitigation algorithms and metrics for model assessment. Fairlearn focuses on two types of harms: allocation harms and quality-of-service harms. The package follows the group fairness approach, aiming to identify groups at risk of experiencing harms and ensuring comparable behavior across these groups. Fairlearn consists of metrics for assessing model impacts and algorithms for mitigating unfairness in various AI tasks under different fairness definitions.
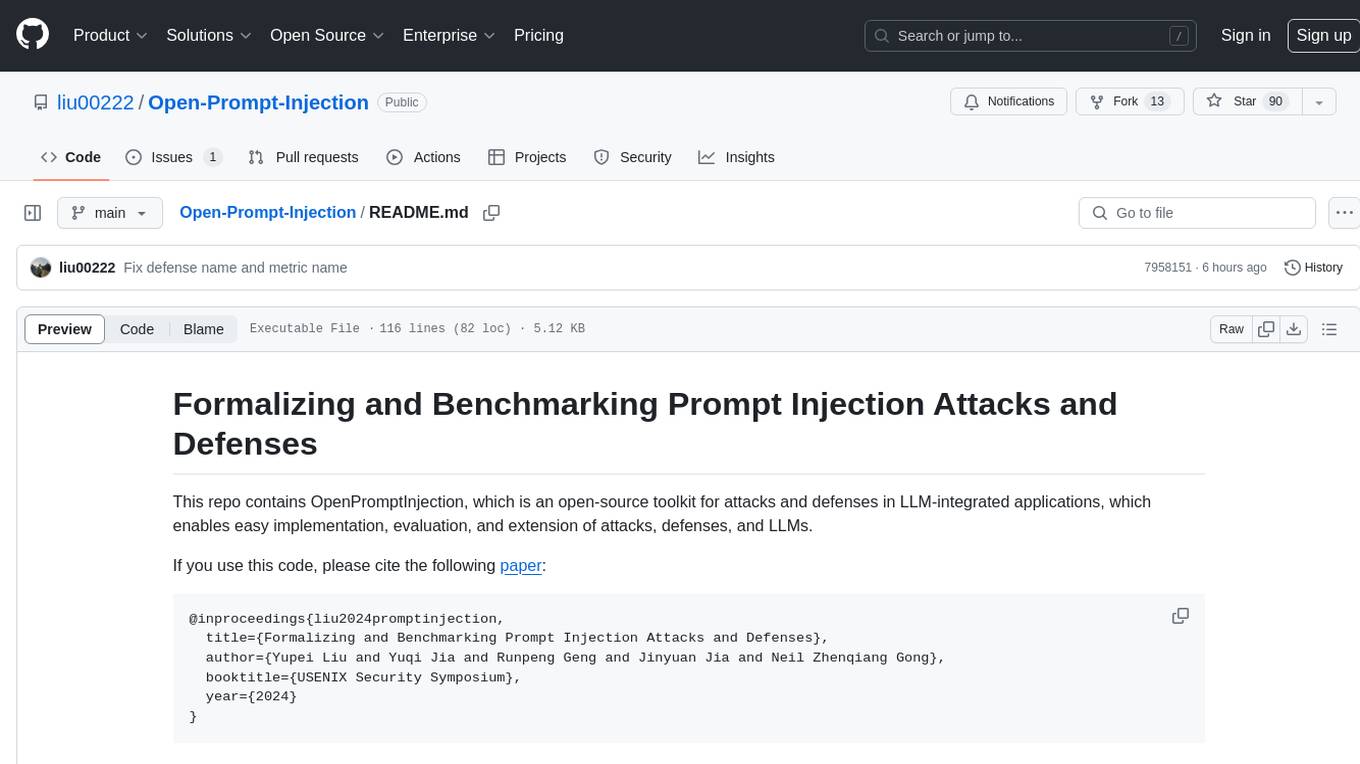
Open-Prompt-Injection
OpenPromptInjection is an open-source toolkit for attacks and defenses in LLM-integrated applications, enabling easy implementation, evaluation, and extension of attacks, defenses, and LLMs. It supports various attack and defense strategies, including prompt injection, paraphrasing, retokenization, data prompt isolation, instructional prevention, sandwich prevention, perplexity-based detection, LLM-based detection, response-based detection, and know-answer detection. Users can create models, tasks, and apps to evaluate different scenarios. The toolkit currently supports PaLM2 and provides a demo for querying models with prompts. Users can also evaluate ASV for different scenarios by injecting tasks and querying models with attacked data prompts.
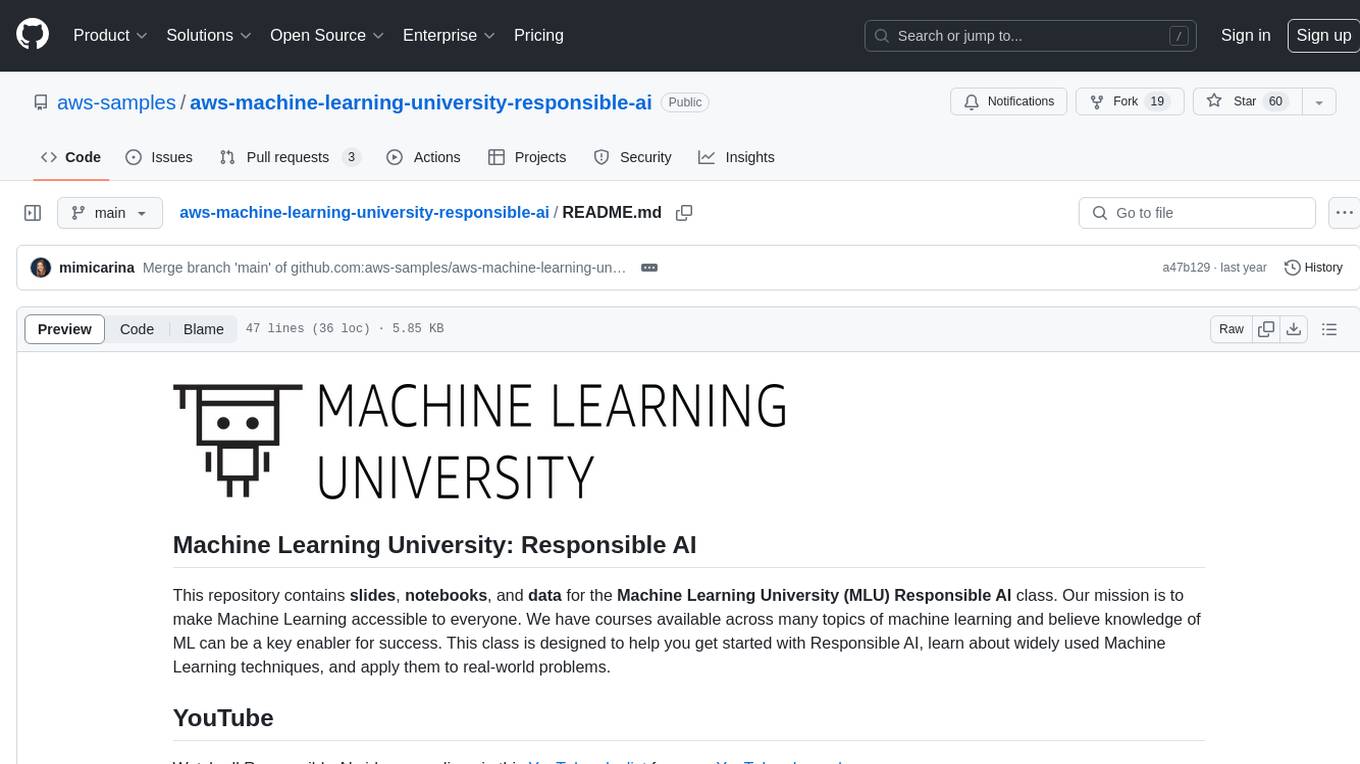
aws-machine-learning-university-responsible-ai
This repository contains slides, notebooks, and data for the Machine Learning University (MLU) Responsible AI class. The mission is to make Machine Learning accessible to everyone, covering widely used ML techniques and applying them to real-world problems. The class includes lectures, final projects, and interactive visuals to help users learn about Responsible AI and core ML concepts.
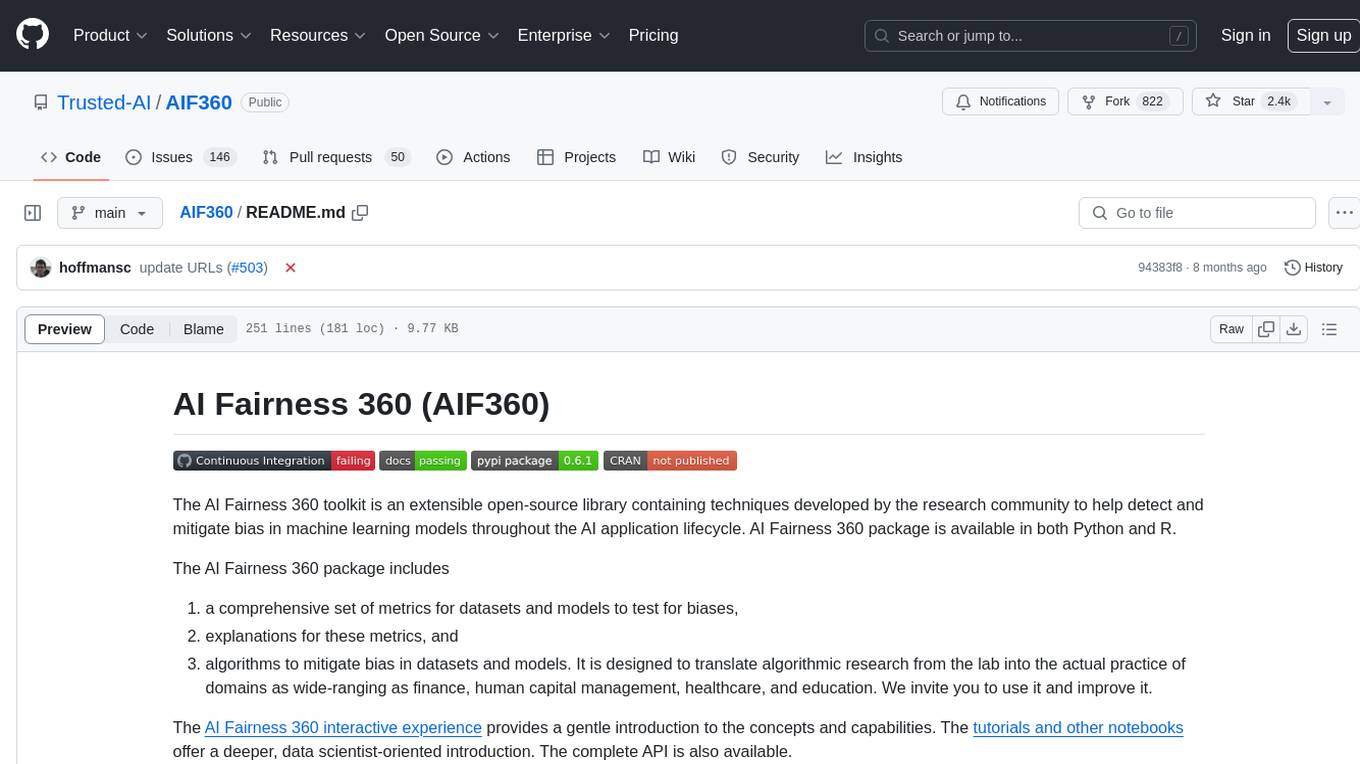
AIF360
The AI Fairness 360 toolkit is an open-source library designed to detect and mitigate bias in machine learning models. It provides a comprehensive set of metrics, explanations, and algorithms for bias mitigation in various domains such as finance, healthcare, and education. The toolkit supports multiple bias mitigation algorithms and fairness metrics, and is available in both Python and R. Users can leverage the toolkit to ensure fairness in AI applications and contribute to its development for extensibility.
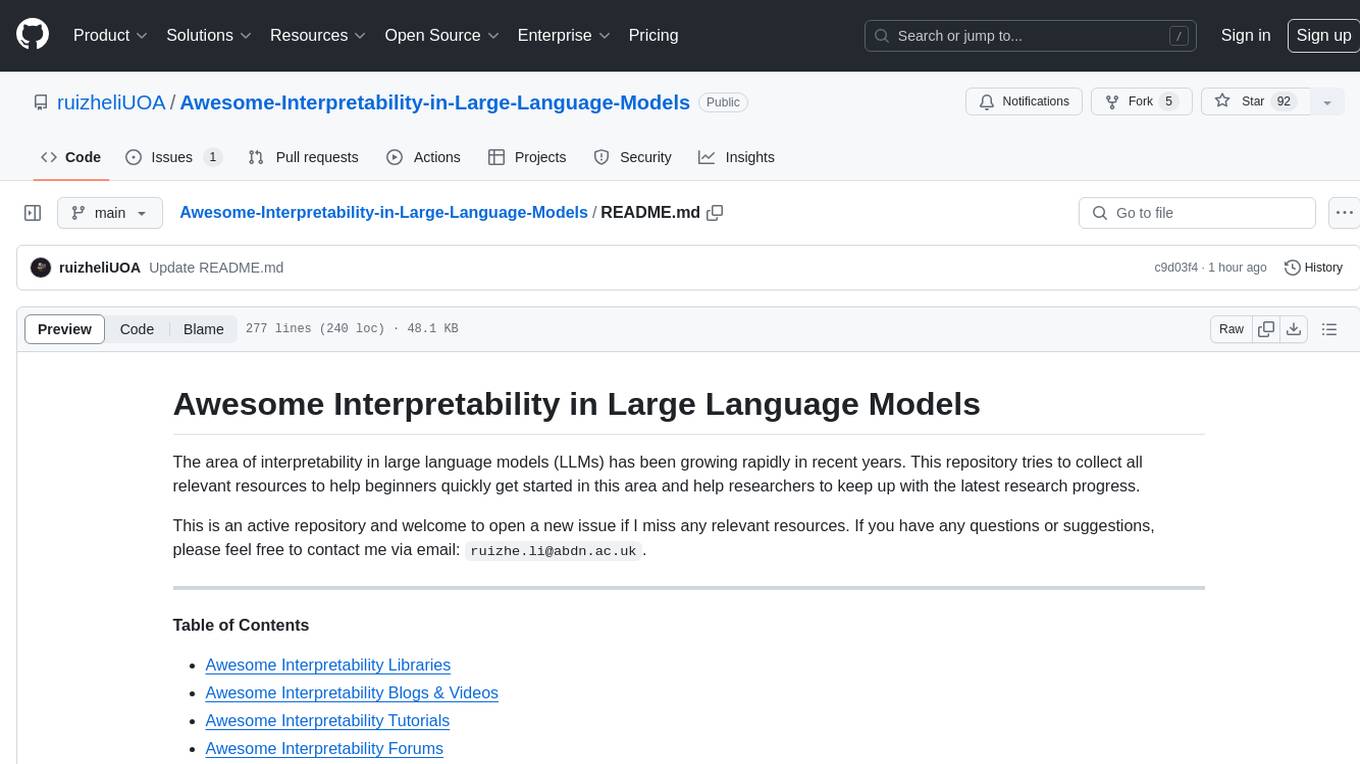
Awesome-Interpretability-in-Large-Language-Models
This repository is a collection of resources focused on interpretability in large language models (LLMs). It aims to help beginners get started in the area and keep researchers updated on the latest progress. It includes libraries, blogs, tutorials, forums, tools, programs, papers, and more related to interpretability in LLMs.
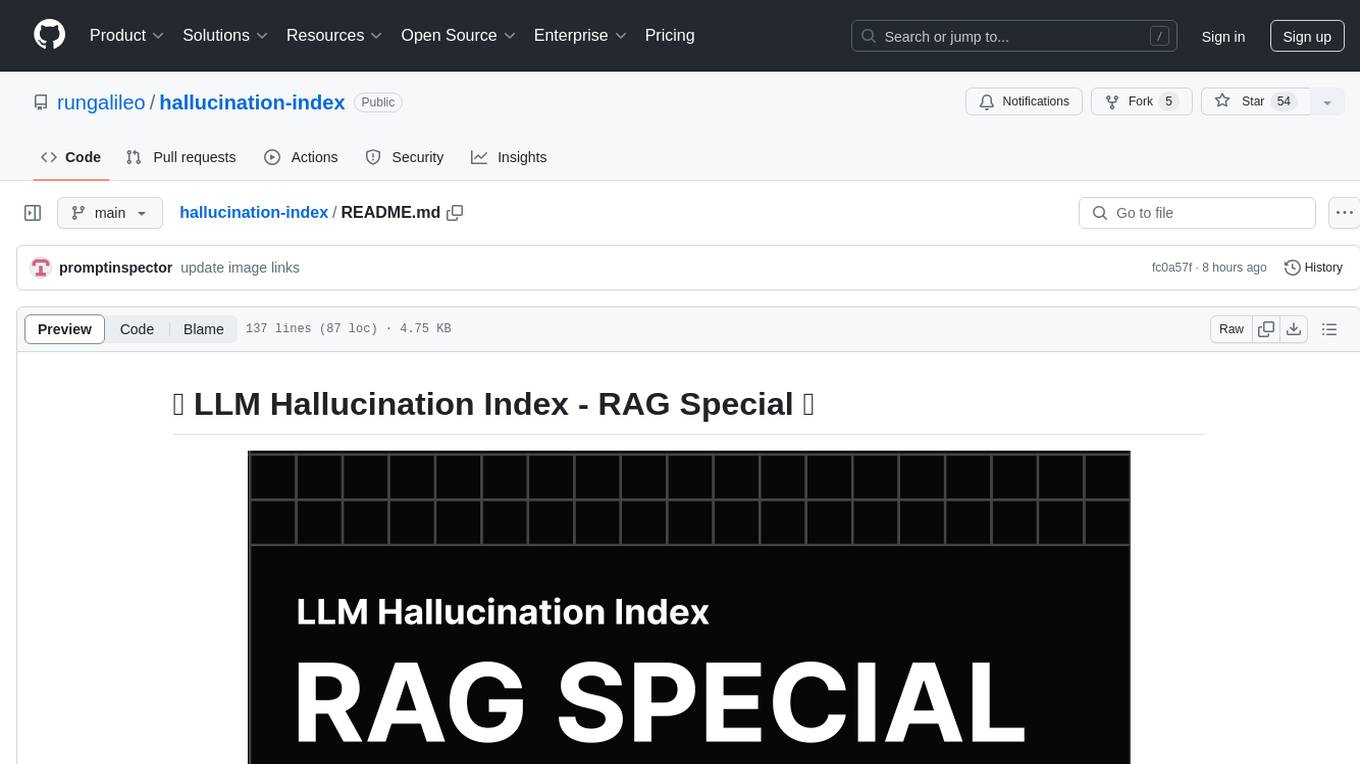
hallucination-index
LLM Hallucination Index - RAG Special is a comprehensive evaluation of large language models (LLMs) focusing on context length and open vs. closed-source attributes. The index explores the impact of context length on model performance and tests the assumption that closed-source LLMs outperform open-source ones. It also investigates the effectiveness of prompting techniques like Chain-of-Note across different context lengths. The evaluation includes 22 models from various brands, analyzing major trends and declaring overall winners based on short, medium, and long context insights. Methodologies involve rigorous testing with different context lengths and prompting techniques to assess models' abilities in handling extensive texts and detecting hallucinations.
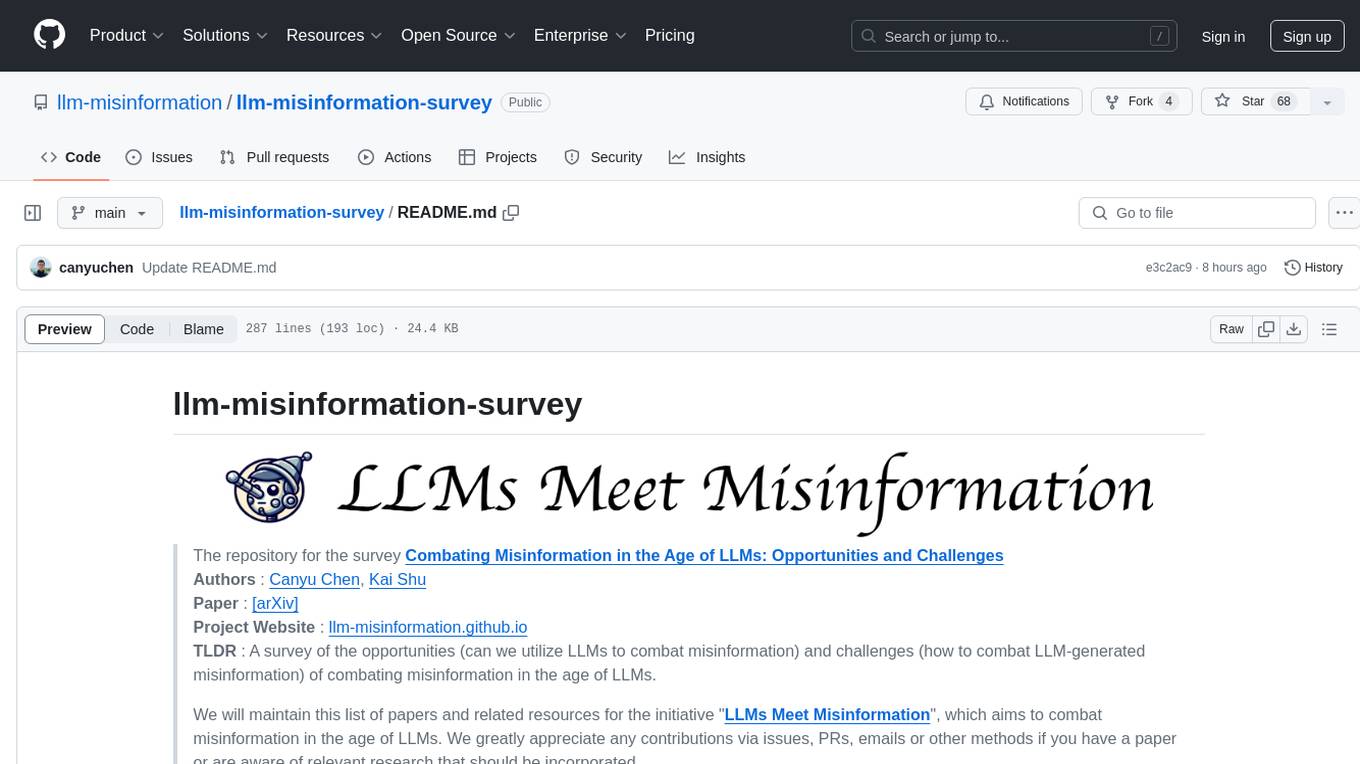
llm-misinformation-survey
The 'llm-misinformation-survey' repository is dedicated to the survey on combating misinformation in the age of Large Language Models (LLMs). It explores the opportunities and challenges of utilizing LLMs to combat misinformation, providing insights into the history of combating misinformation, current efforts, and future outlook. The repository serves as a resource hub for the initiative 'LLMs Meet Misinformation' and welcomes contributions of relevant research papers and resources. The goal is to facilitate interdisciplinary efforts in combating LLM-generated misinformation and promoting the responsible use of LLMs in fighting misinformation.