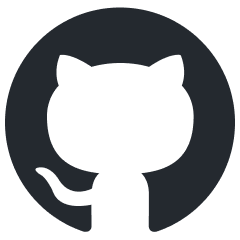
langtest
Deliver safe & effective language models
Stars: 515
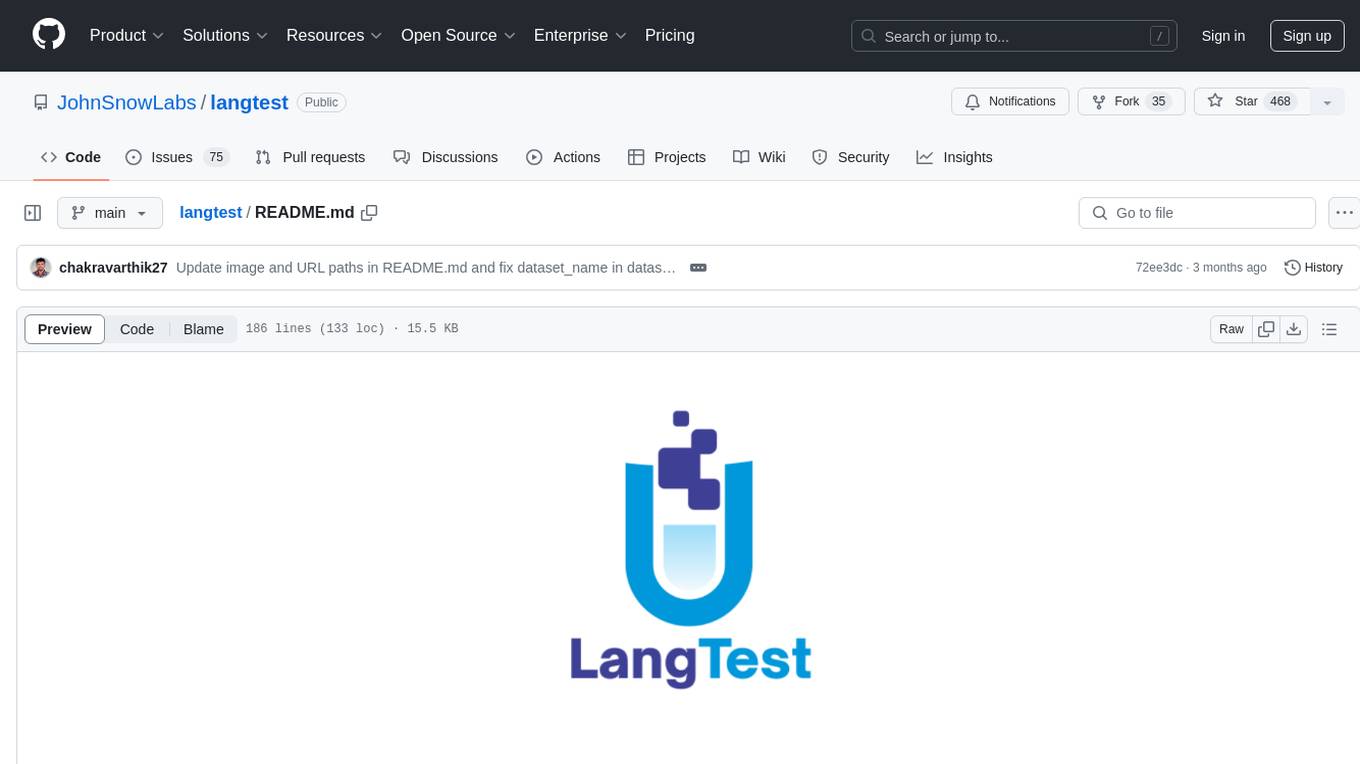
LangTest is a comprehensive evaluation library for custom LLM and NLP models. It aims to deliver safe and effective language models by providing tools to test model quality, augment training data, and support popular NLP frameworks. LangTest comes with benchmark datasets to challenge and enhance language models, ensuring peak performance in various linguistic tasks. The tool offers more than 60 distinct types of tests with just one line of code, covering aspects like robustness, bias, representation, fairness, and accuracy. It supports testing LLMS for question answering, toxicity, clinical tests, legal support, factuality, sycophancy, and summarization.
README:
Project's Website • Key Features • How To Use • Benchmark Datasets • Community Support • Contributing • Mission • License
Take a look at our official page for user documentation and examples: langtest.org
- Generate and execute more than 60 distinct types of tests only with 1 line of code
- Test all aspects of model quality: robustness, bias, representation, fairness and accuracy.
- Automatically augment training data based on test results (for select models)
- Support for popular NLP frameworks for NER, Translation and Text-Classifcation: Spark NLP, Hugging Face & Transformers.
- Support for testing LLMS ( OpenAI, Cohere, AI21, Hugging Face Inference API and Azure-OpenAI LLMs) for question answering, toxicity, clinical-tests, legal-support, factuality, sycophancy, summarization and other popular tests.
LangTest comes with different datasets to test your models, covering a wide range of use cases and evaluation scenarios. You can explore all the benchmark datasets available here, each meticulously curated to challenge and enhance your language models. Whether you're focused on Question-Answering, text summarization etc, LangTest ensures you have the right data to push your models to their limits and achieve peak performance in diverse linguistic tasks.
# Install langtest
!pip install langtest[transformers]
# Import and create a Harness object
from langtest import Harness
h = Harness(task='ner', model={"model":'dslim/bert-base-NER', "hub":'huggingface'})
# Generate test cases, run them and view a report
h.generate().run().report()
Note For more extended examples of usage and documentation, head over to langtest.org
You can check out the following LangTest articles:
Blog | Description |
---|---|
Automatically Testing for Demographic Bias in Clinical Treatment Plans Generated by Large Language Models | Helps in understanding and testing demographic bias in clinical treatment plans generated by LLM. |
LangTest: Unveiling & Fixing Biases with End-to-End NLP Pipelines | The end-to-end language pipeline in LangTest empowers NLP practitioners to tackle biases in language models with a comprehensive, data-driven, and iterative approach. |
Beyond Accuracy: Robustness Testing of Named Entity Recognition Models with LangTest | While accuracy is undoubtedly crucial, robustness testing takes natural language processing (NLP) models evaluation to the next level by ensuring that models can perform reliably and consistently across a wide array of real-world conditions. |
Elevate Your NLP Models with Automated Data Augmentation for Enhanced Performance | In this article, we discuss how automated data augmentation may supercharge your NLP models and improve their performance and how we do that using LangTest. |
Mitigating Gender-Occupational Stereotypes in AI: Evaluating Models with the Wino Bias Test through Langtest Library | In this article, we discuss how we can test the "Wino Bias” using LangTest. It specifically refers to testing biases arising from gender-occupational stereotypes. |
Automating Responsible AI: Integrating Hugging Face and LangTest for More Robust Models | In this article, we have explored the integration between Hugging Face, your go-to source for state-of-the-art NLP models and datasets, and LangTest, your NLP pipeline’s secret weapon for testing and optimization. |
Detecting and Evaluating Sycophancy Bias: An Analysis of LLM and AI Solutions | In this blog post, we discuss the pervasive issue of sycophantic AI behavior and the challenges it presents in the world of artificial intelligence. We explore how language models sometimes prioritize agreement over authenticity, hindering meaningful and unbiased conversations. Furthermore, we unveil a potential game-changing solution to this problem, synthetic data, which promises to revolutionize the way AI companions engage in discussions, making them more reliable and accurate across various real-world conditions. |
Unmasking Language Model Sensitivity in Negation and Toxicity Evaluations | In this blog post, we delve into Language Model Sensitivity, examining how models handle negations and toxicity in language. Through these tests, we gain insights into the models' adaptability and responsiveness, emphasizing the continuous need for improvement in NLP models. |
Unveiling Bias in Language Models: Gender, Race, Disability, and Socioeconomic Perspectives | In this blog post, we explore bias in Language Models, focusing on gender, race, disability, and socioeconomic factors. We assess this bias using the CrowS-Pairs dataset, designed to measure stereotypical biases. To address these biases, we discuss the importance of tools like LangTest in promoting fairness in NLP systems. |
Unmasking the Biases Within AI: How Gender, Ethnicity, Religion, and Economics Shape NLP and Beyond | In this blog post, we tackle AI bias on how Gender, Ethnicity, Religion, and Economics Shape NLP systems. We discussed strategies for reducing bias and promoting fairness in AI systems. |
Evaluating Large Language Models on Gender-Occupational Stereotypes Using the Wino Bias Test | In this blog post, we dive into testing the WinoBias dataset on LLMs, examining language models’ handling of gender and occupational roles, evaluation metrics, and the wider implications. Let’s explore the evaluation of language models with LangTest on the WinoBias dataset and confront the challenges of addressing bias in AI. |
Streamlining ML Workflows: Integrating MLFlow Tracking with LangTest for Enhanced Model Evaluations | In this blog post, we dive into the growing need for transparent, systematic, and comprehensive tracking of models. Enter MLFlow and LangTest: two tools that, when combined, create a revolutionary approach to ML development. |
Testing the Question Answering Capabilities of Large Language Models | In this blog post, we dive into enhancing the QA evaluation capabilities using LangTest library. Explore about different evaluation methods that LangTest offers to address the complexities of evaluating Question Answering (QA) tasks. |
Evaluating Stereotype Bias with LangTest | In this blog post, we are focusing on using the StereoSet dataset to assess bias related to gender, profession, and race. |
Testing the Robustness of LSTM-Based Sentiment Analysis Models | Explore the robustness of custom models with LangTest Insights. |
LangTest Insights: A Deep Dive into LLM Robustness on OpenBookQA | Explore the robustness of Language Models (LLMs) on the OpenBookQA dataset with LangTest Insights. |
LangTest: A Secret Weapon for Improving the Robustness of Your Transformers Language Models | Explore the robustness of Transformers Language Models with LangTest Insights. |
Mastering Model Evaluation: Introducing the Comprehensive Ranking & Leaderboard System in LangTest | The Model Ranking & Leaderboard system by John Snow Labs' LangTest offers a systematic approach to evaluating AI models with comprehensive ranking, historical comparisons, and dataset-specific insights, empowering researchers and data scientists to make data-driven decisions on model performance. |
Evaluating Long-Form Responses with Prometheus-Eval and Langtest | Prometheus-Eval and LangTest unite to offer an open-source, reliable, and cost-effective solution for evaluating long-form responses, combining Prometheus's GPT-4-level performance and LangTest's robust testing framework to provide detailed, interpretable feedback and high accuracy in assessments. |
Ensuring Precision of LLMs in Medical Domain: The Challenge of Drug Name Swapping | Accurate drug name identification is crucial for patient safety. Testing GPT-4o with LangTest's drug_generic_to_brand conversion test revealed potential errors in predicting drug names when brand names are replaced by ingredients, highlighting the need for ongoing refinement and rigorous testing to ensure medical LLM accuracy and reliability. |
Note To check all blogs, head over to Blogs
-
Slack For live discussion with the LangTest community, join the
#langtest
channel - GitHub For bug reports, feature requests, and contributions
- Discussions To engage with other community members, share ideas, and show off how you use LangTest!
While there is a lot of talk about the need to train AI models that are safe, robust, and fair - few tools have been made available to data scientists to meet these goals. As a result, the front line of NLP models in production systems reflects a sorry state of affairs.
We propose here an early stage open-source community project that aims to fill this gap, and would love for you to join us on this mission. We aim to build on the foundation laid by previous research such as Ribeiro et al. (2020), Song et al. (2020), Parrish et al. (2021), van Aken et al. (2021) and many others.
John Snow Labs has a full development team allocated to the project and is committed to improving the library for years, as we do with other open-source libraries. Expect frequent releases with new test types, tasks, languages, and platforms to be added regularly. We look forward to working together to make safe, reliable, and responsible NLP an everyday reality.
Note For usage and documentation, head over to langtest.org
We welcome all sorts of contributions:
A detailed overview of contributing can be found in the contributing guide.
If you are looking to start working with the LangTest codebase, navigate to the GitHub "issues" tab and start looking through interesting issues. There are a number of issues listed under where you could start out. Or maybe through using LangTest you have an idea of your own or are looking for something in the documentation and thinking ‘This can be improved’...you can do something about it!
Feel free to ask questions on the Q&A discussions.
As contributors and maintainers to this project, you are expected to abide by LangTest's code of conduct. More information can be found at: Contributor Code of Conduct
We have published a paper that you can cite for the LangTest library:
@article{nazir2024langtest,
title={LangTest: A comprehensive evaluation library for custom LLM and NLP models},
author={Arshaan Nazir, Thadaka Kalyan Chakravarthy, David Amore Cecchini, Rakshit Khajuria, Prikshit Sharma, Ali Tarik Mirik, Veysel Kocaman and David Talby},
journal={Software Impacts},
pages={100619},
year={2024},
publisher={Elsevier}
}
We would like to acknowledge all contributors of this open-source community project.
LangTest is released under the Apache License 2.0, which guarantees commercial use, modification, distribution, patent use, private use and sets limitations on trademark use, liability and warranty.
For Tasks:
Click tags to check more tools for each tasksFor Jobs:
Alternative AI tools for langtest
Similar Open Source Tools
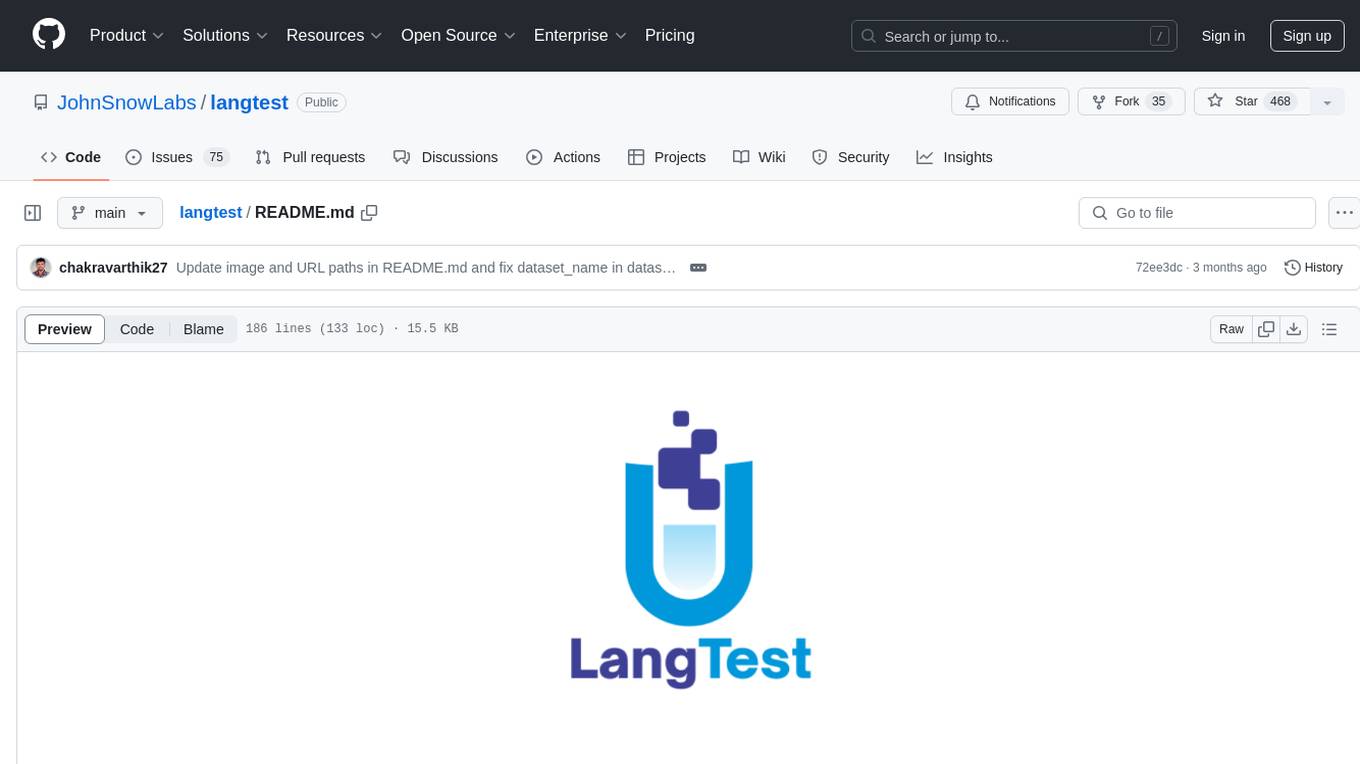
langtest
LangTest is a comprehensive evaluation library for custom LLM and NLP models. It aims to deliver safe and effective language models by providing tools to test model quality, augment training data, and support popular NLP frameworks. LangTest comes with benchmark datasets to challenge and enhance language models, ensuring peak performance in various linguistic tasks. The tool offers more than 60 distinct types of tests with just one line of code, covering aspects like robustness, bias, representation, fairness, and accuracy. It supports testing LLMS for question answering, toxicity, clinical tests, legal support, factuality, sycophancy, and summarization.
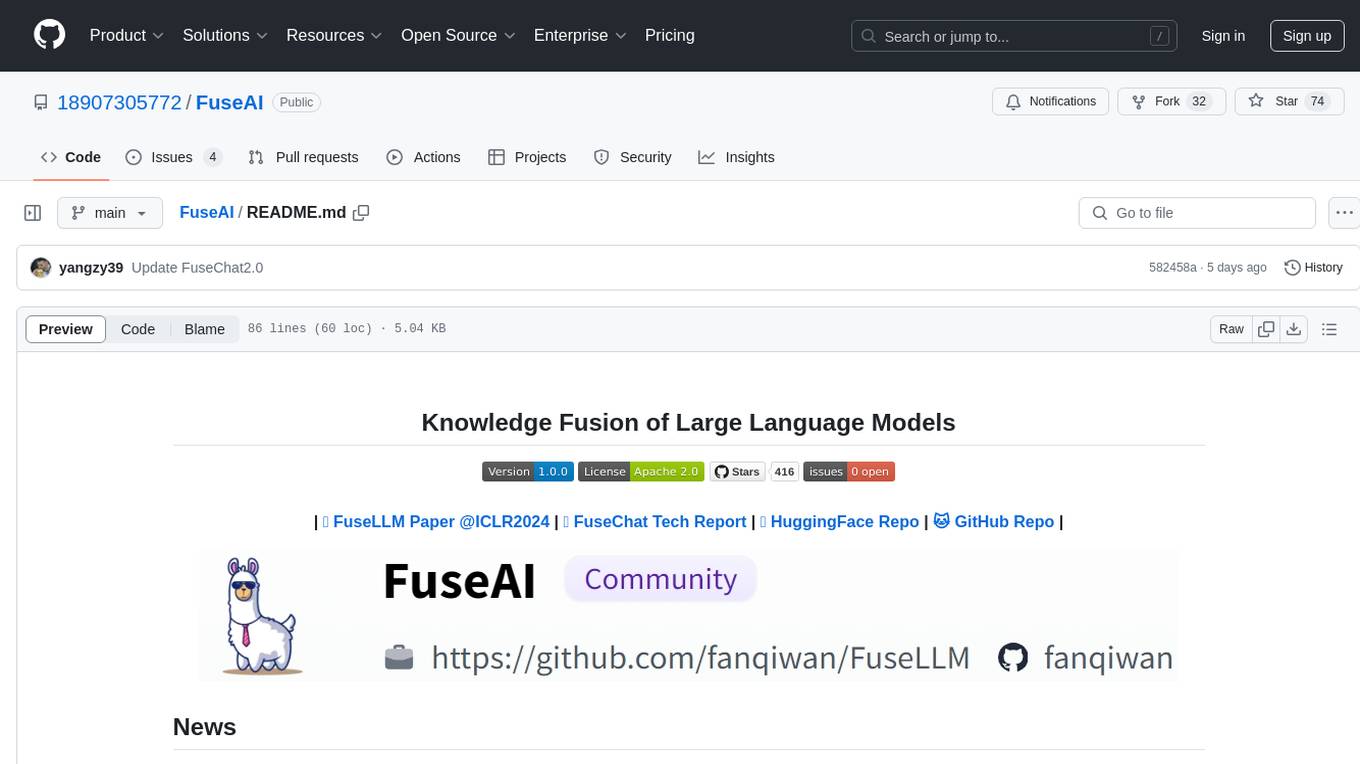
FuseAI
FuseAI is a repository that focuses on knowledge fusion of large language models. It includes FuseChat, a state-of-the-art 7B LLM on MT-Bench, and FuseLLM, which surpasses Llama-2-7B by fusing three open-source foundation LLMs. The repository provides tech reports, releases, and datasets for FuseChat and FuseLLM, showcasing their performance and advancements in the field of chat models and large language models.
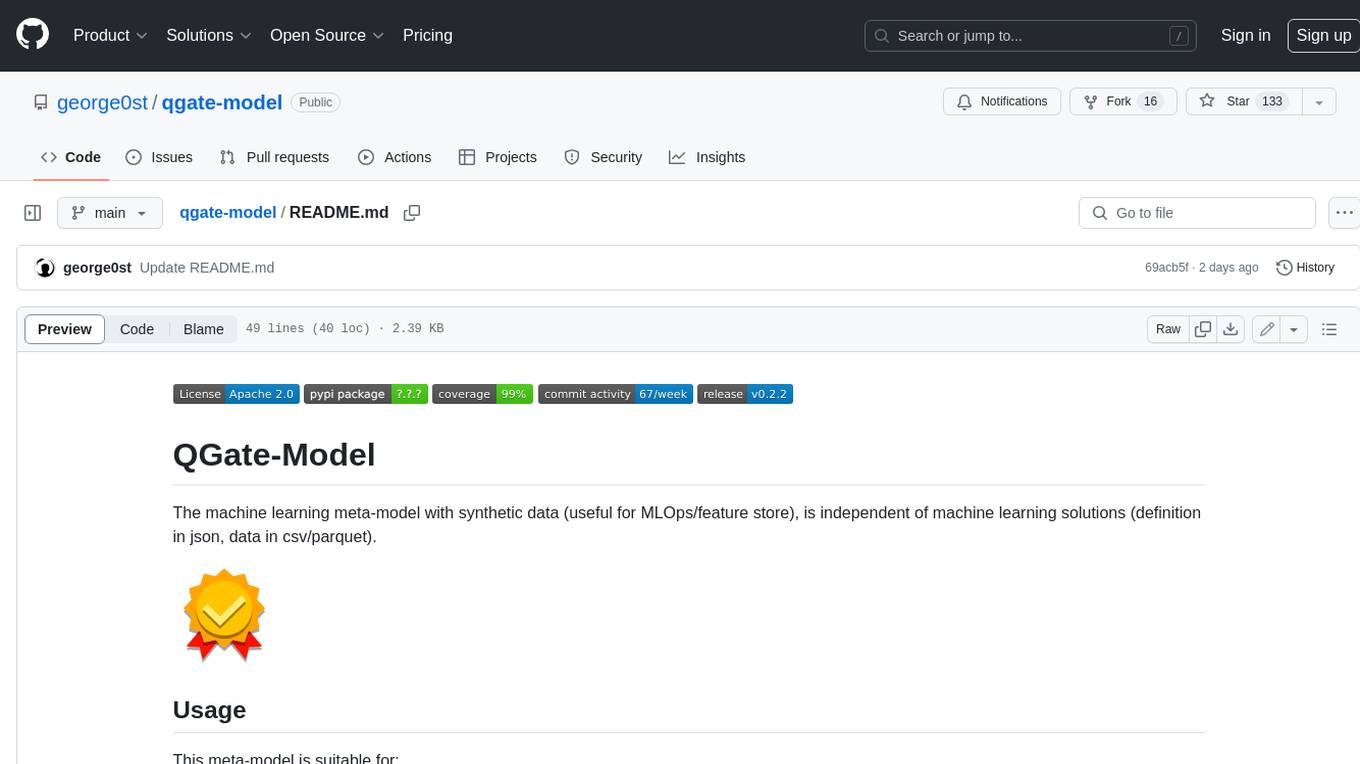
qgate-model
QGate-Model is a machine learning meta-model with synthetic data, designed for MLOps and feature store. It is independent of machine learning solutions, with definitions in JSON and data in CSV/parquet formats. This meta-model is useful for comparing capabilities and functions of machine learning solutions, independently testing new versions of machine learning solutions, and conducting various types of tests (unit, sanity, smoke, system, regression, function, acceptance, performance, shadow, etc.). It can also be used for external test coverage when internal test coverage is not available or weak.
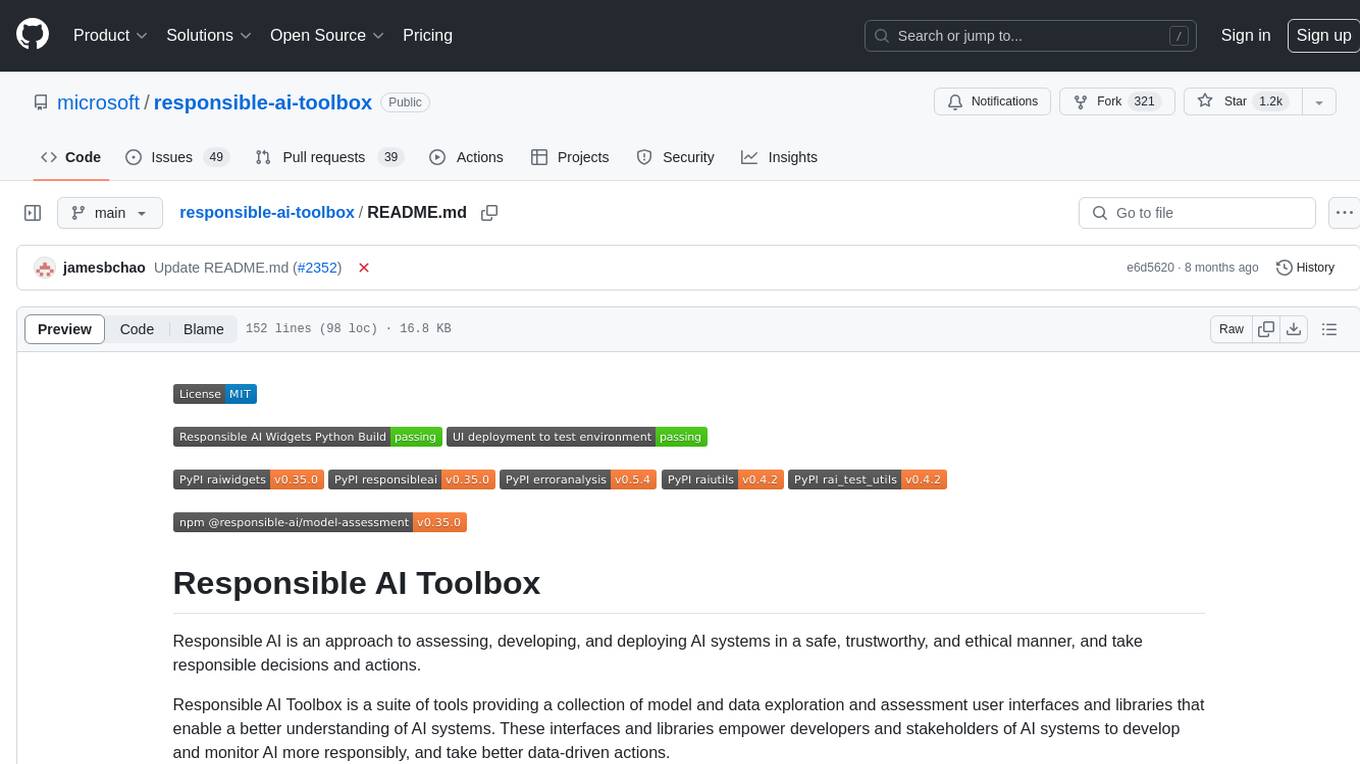
responsible-ai-toolbox
Responsible AI Toolbox is a suite of tools providing model and data exploration and assessment interfaces and libraries for understanding AI systems. It empowers developers and stakeholders to develop and monitor AI responsibly, enabling better data-driven actions. The toolbox includes visualization widgets for model assessment, error analysis, interpretability, fairness assessment, and mitigations library. It also offers a JupyterLab extension for managing machine learning experiments and a library for measuring gender bias in NLP datasets.
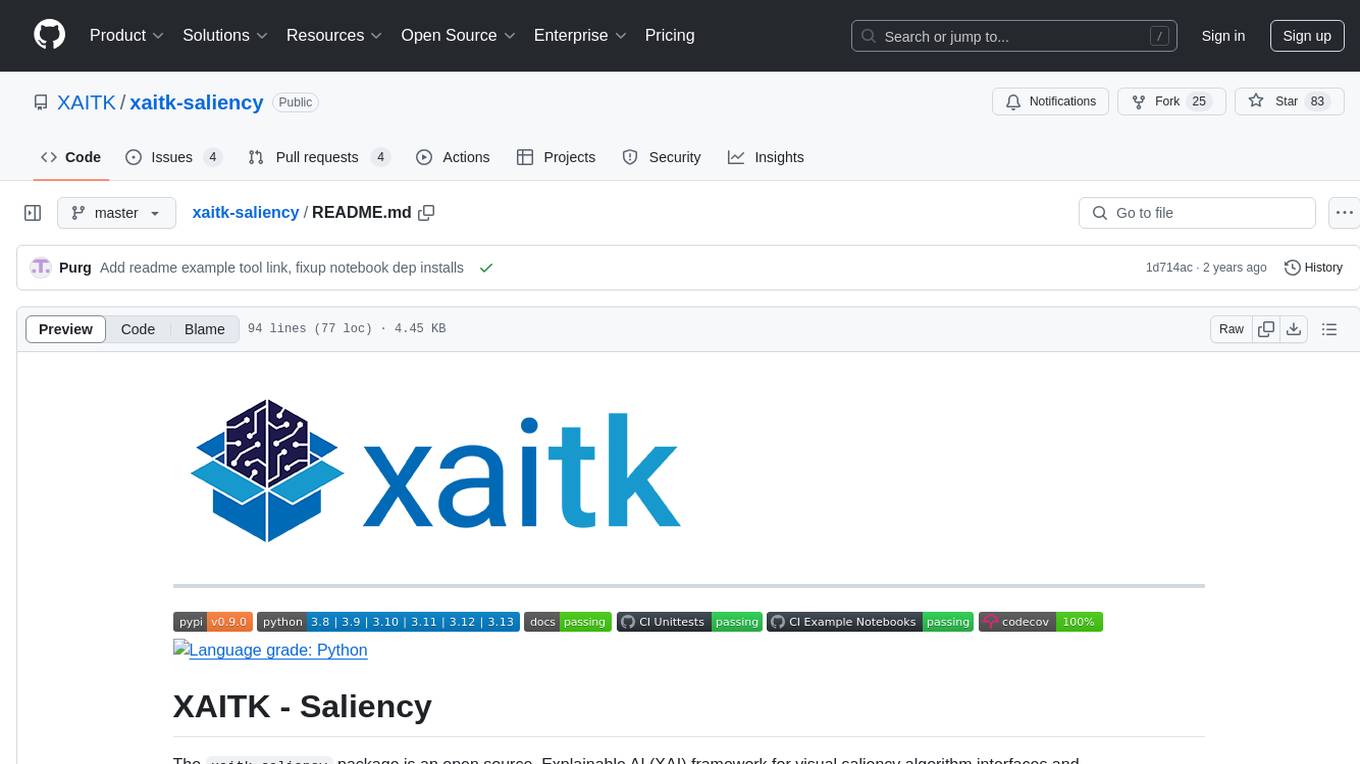
xaitk-saliency
The `xaitk-saliency` package is an open source Explainable AI (XAI) framework for visual saliency algorithm interfaces and implementations, designed for analytics and autonomy applications. It provides saliency algorithms for various image understanding tasks such as image classification, image similarity, object detection, and reinforcement learning. The toolkit targets data scientists and developers who aim to incorporate visual saliency explanations into their workflow or product, offering both direct accessibility for experimentation and modular integration into systems and applications through Strategy and Adapter patterns. The package includes documentation, examples, and a demonstration tool for visual saliency generation in a user-interface.
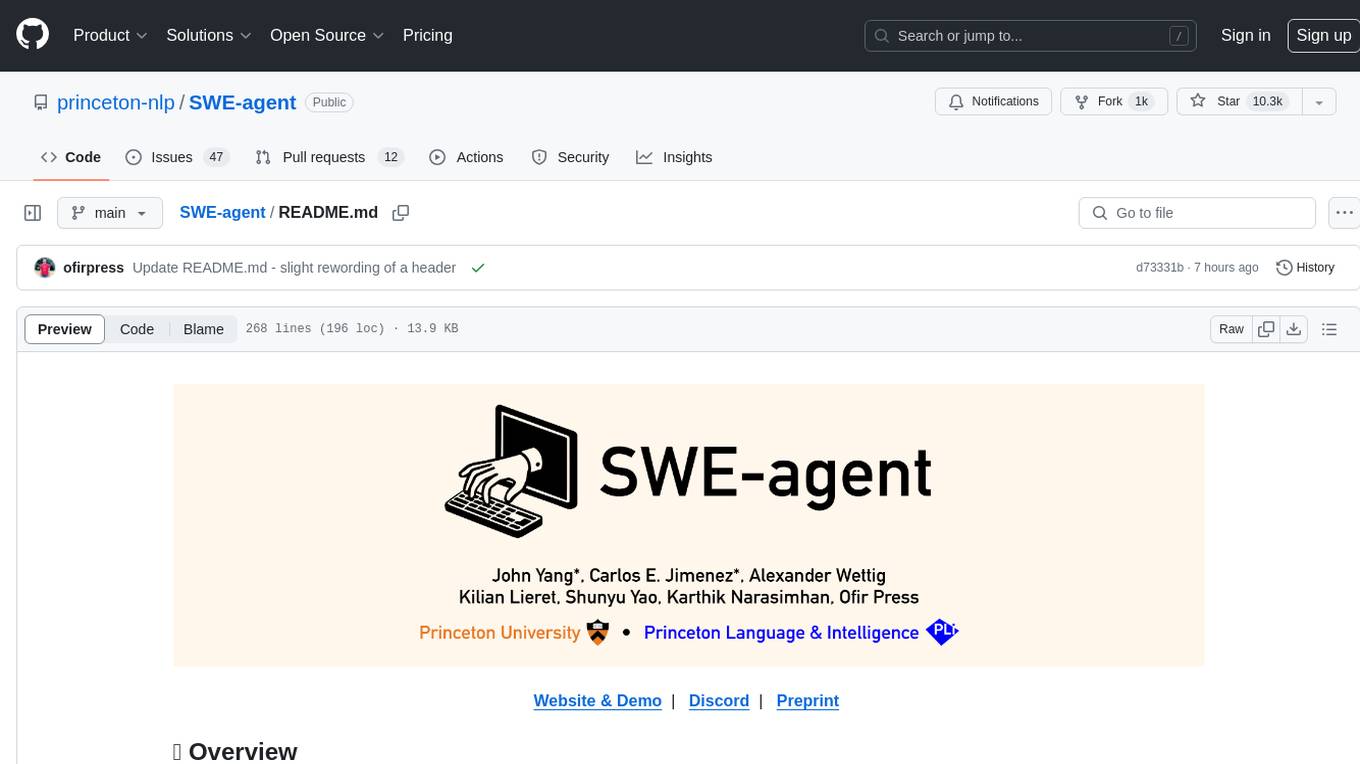
SWE-agent
SWE-agent is a tool that turns language models (e.g. GPT-4) into software engineering agents capable of fixing bugs and issues in real GitHub repositories. It achieves state-of-the-art performance on the full test set by resolving 12.29% of issues. The tool is built and maintained by researchers from Princeton University. SWE-agent provides a command line tool and a graphical web interface for developers to interact with. It introduces an Agent-Computer Interface (ACI) to facilitate browsing, viewing, editing, and executing code files within repositories. The tool includes features such as a linter for syntax checking, a specialized file viewer, and a full-directory string searching command to enhance the agent's capabilities. SWE-agent aims to improve prompt engineering and ACI design to enhance the performance of language models in software engineering tasks.
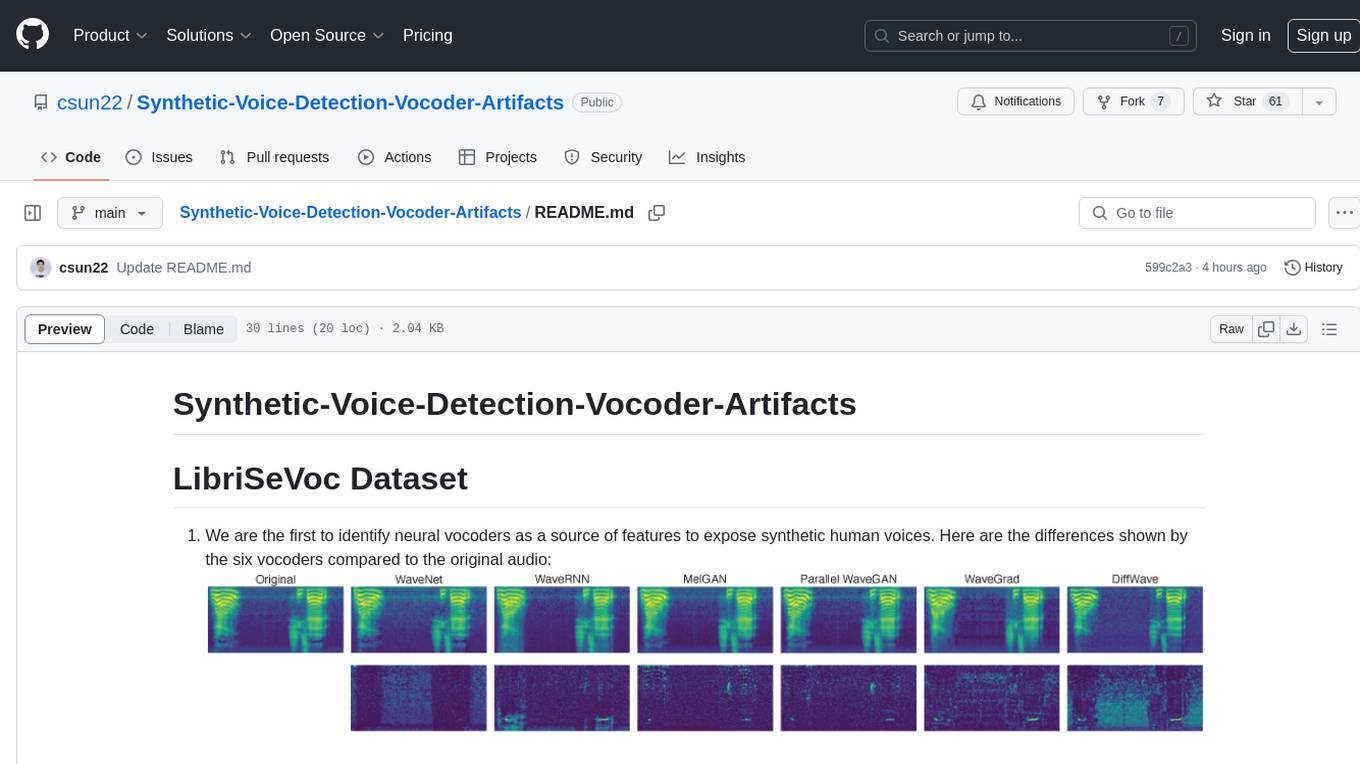
Synthetic-Voice-Detection-Vocoder-Artifacts
The Synthetic-Voice-Detection-Vocoder-Artifacts repository provides the LibriSeVoc dataset containing self-vocoding samples created with six state-of-the-art vocoders to expose and exploit vocoder artifacts. It also introduces a new approach for detecting synthetic human voices by identifying signal artifacts left by neural vocoders and enhancing the RawNet2 baseline. The repository includes a paper and dataset for further reference and offers instructions for training the model and testing it in the wild.
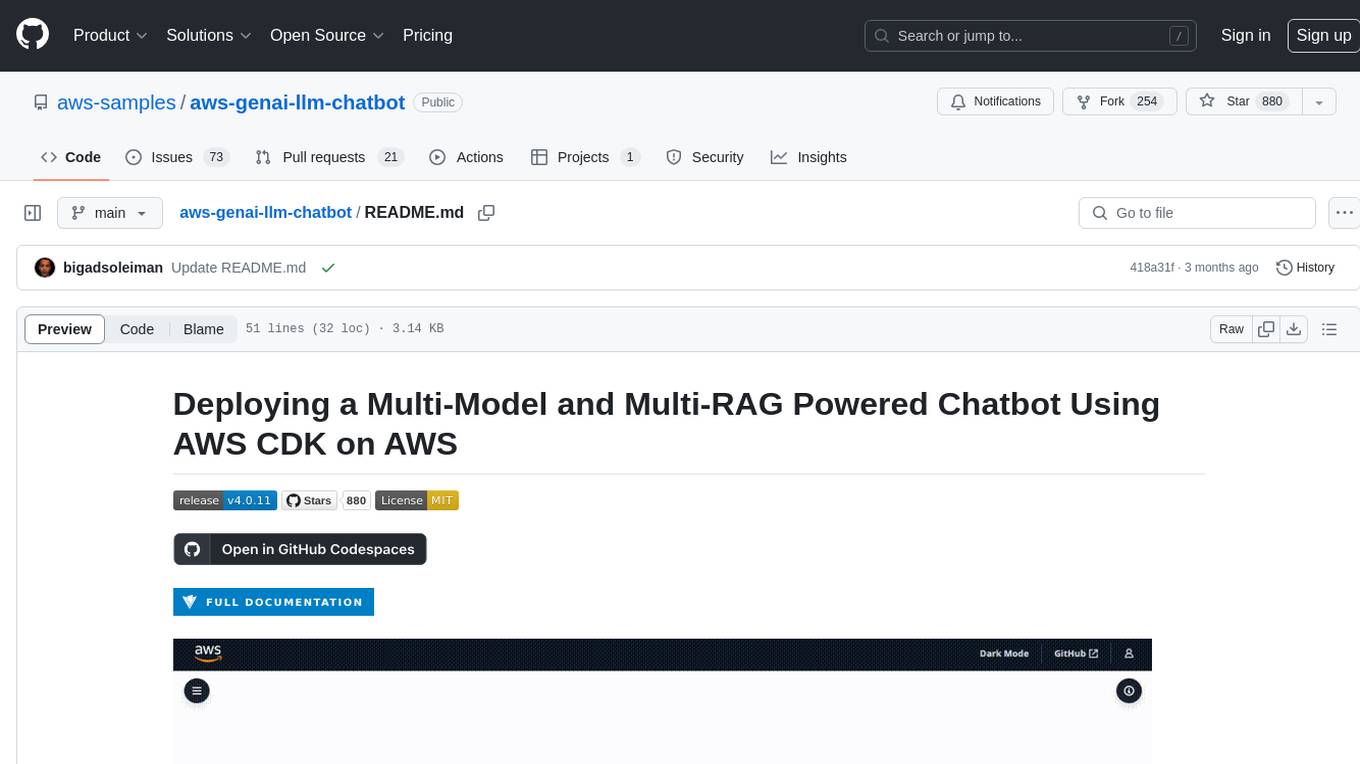
aws-genai-llm-chatbot
This repository provides code to deploy a chatbot powered by Multi-Model and Multi-RAG using AWS CDK on AWS. Users can experiment with various Large Language Models and Multimodal Language Models from different providers. The solution supports Amazon Bedrock, Amazon SageMaker self-hosted models, and third-party providers via API. It also offers additional resources like AWS Generative AI CDK Constructs and Project Lakechain for building generative AI solutions and document processing. The roadmap and authors are listed, along with contributors. The library is licensed under the MIT-0 License with information on changelog, code of conduct, and contributing guidelines. A legal disclaimer advises users to conduct their own assessment before using the content for production purposes.
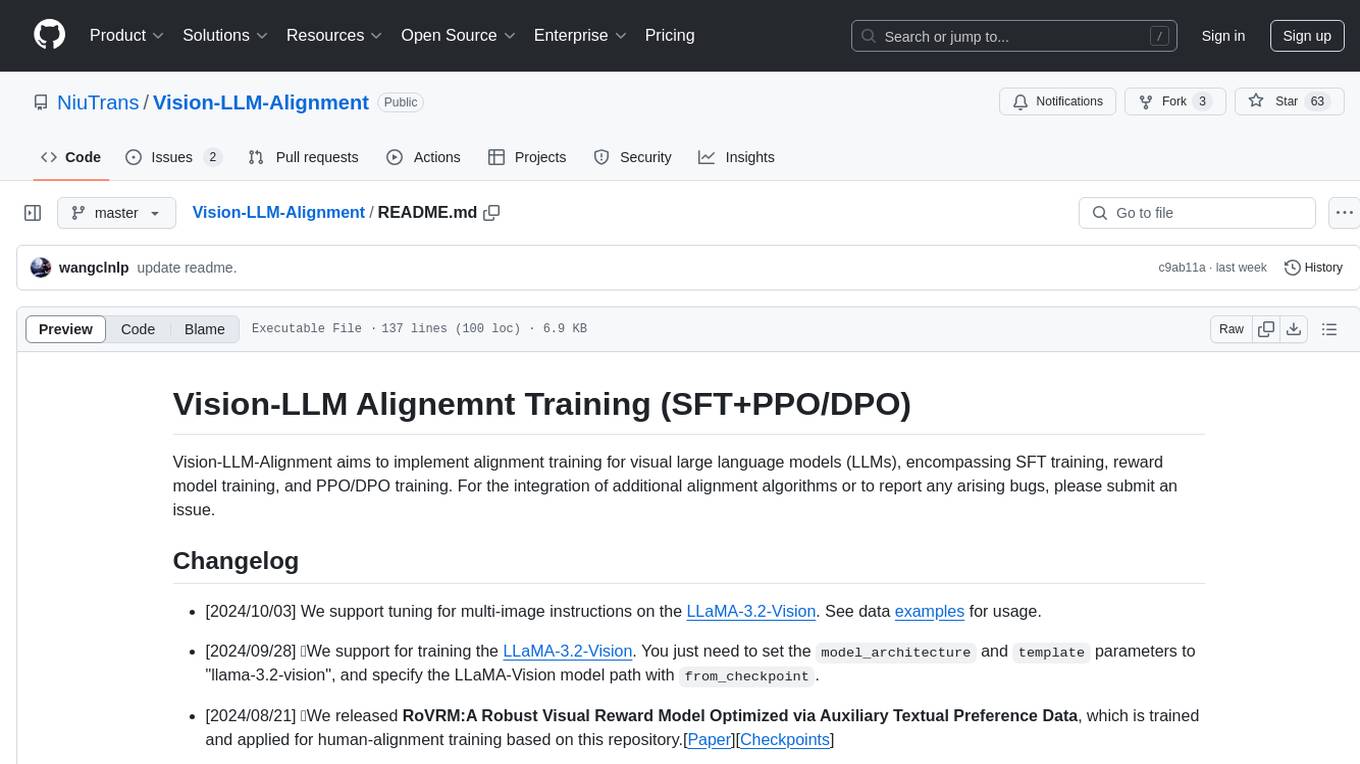
Vision-LLM-Alignment
Vision-LLM-Alignment is a repository focused on implementing alignment training for visual large language models (LLMs), including SFT training, reward model training, and PPO/DPO training. It supports various model architectures and provides datasets for training. The repository also offers benchmark results and installation instructions for users.
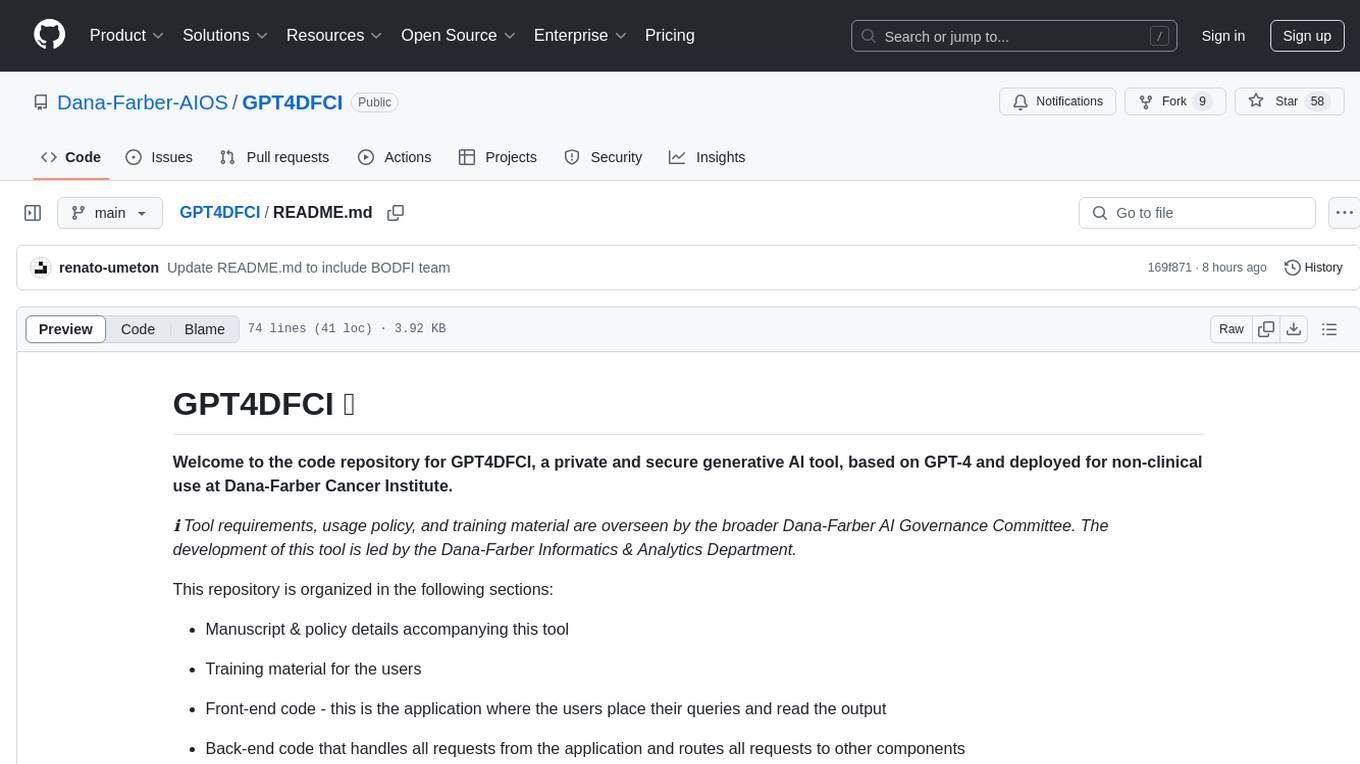
GPT4DFCI
GPT4DFCI is a private and secure generative AI tool based on GPT-4, deployed for non-clinical use at Dana-Farber Cancer Institute. The tool is overseen by the Dana-Farber AI Governance Committee and developed by the Dana-Farber Informatics & Analytics Department. The repository includes manuscript & policy details, training material, front-end and back-end code, infrastructure information, API client for programmatic use, licensing details, and contact information.
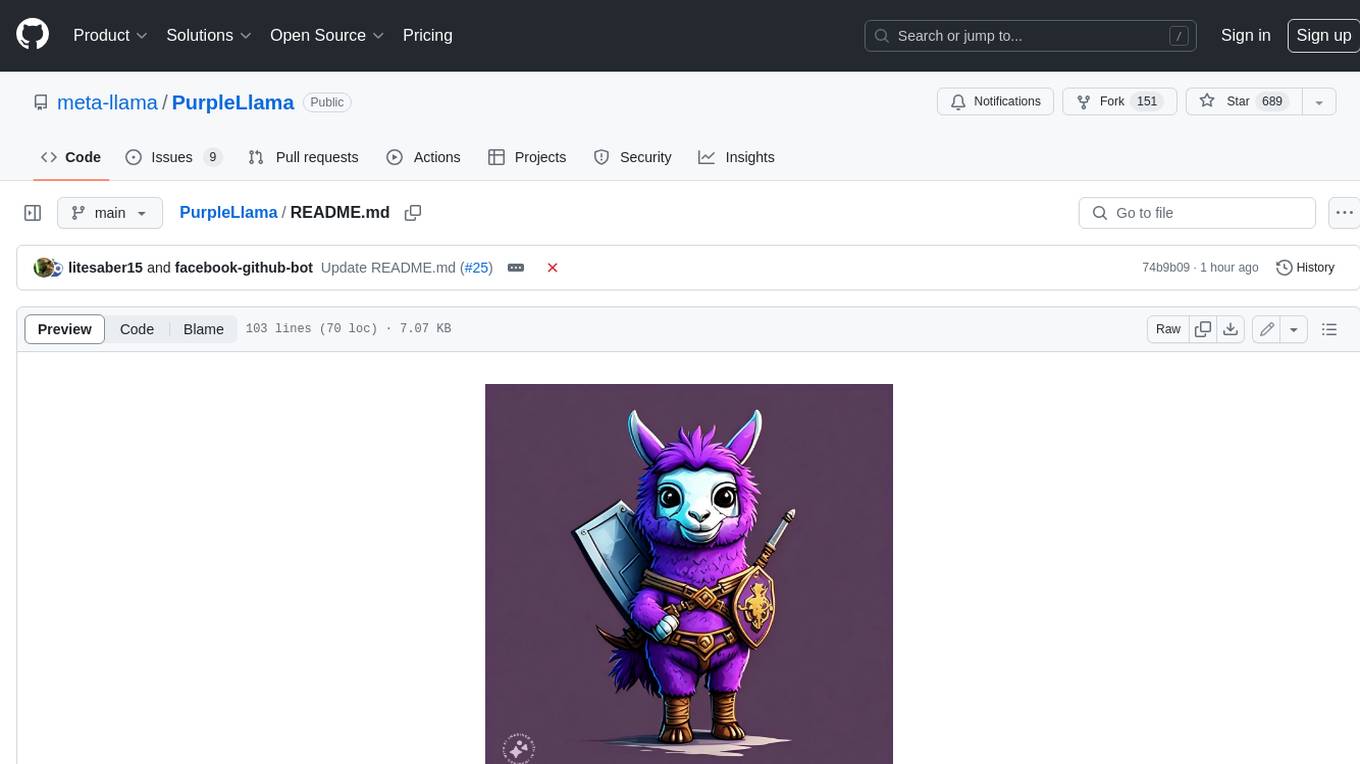
PurpleLlama
Purple Llama is an umbrella project that aims to provide tools and evaluations to support responsible development and usage of generative AI models. It encompasses components for cybersecurity and input/output safeguards, with plans to expand in the future. The project emphasizes a collaborative approach, borrowing the concept of purple teaming from cybersecurity, to address potential risks and challenges posed by generative AI. Components within Purple Llama are licensed permissively to foster community collaboration and standardize the development of trust and safety tools for generative AI.
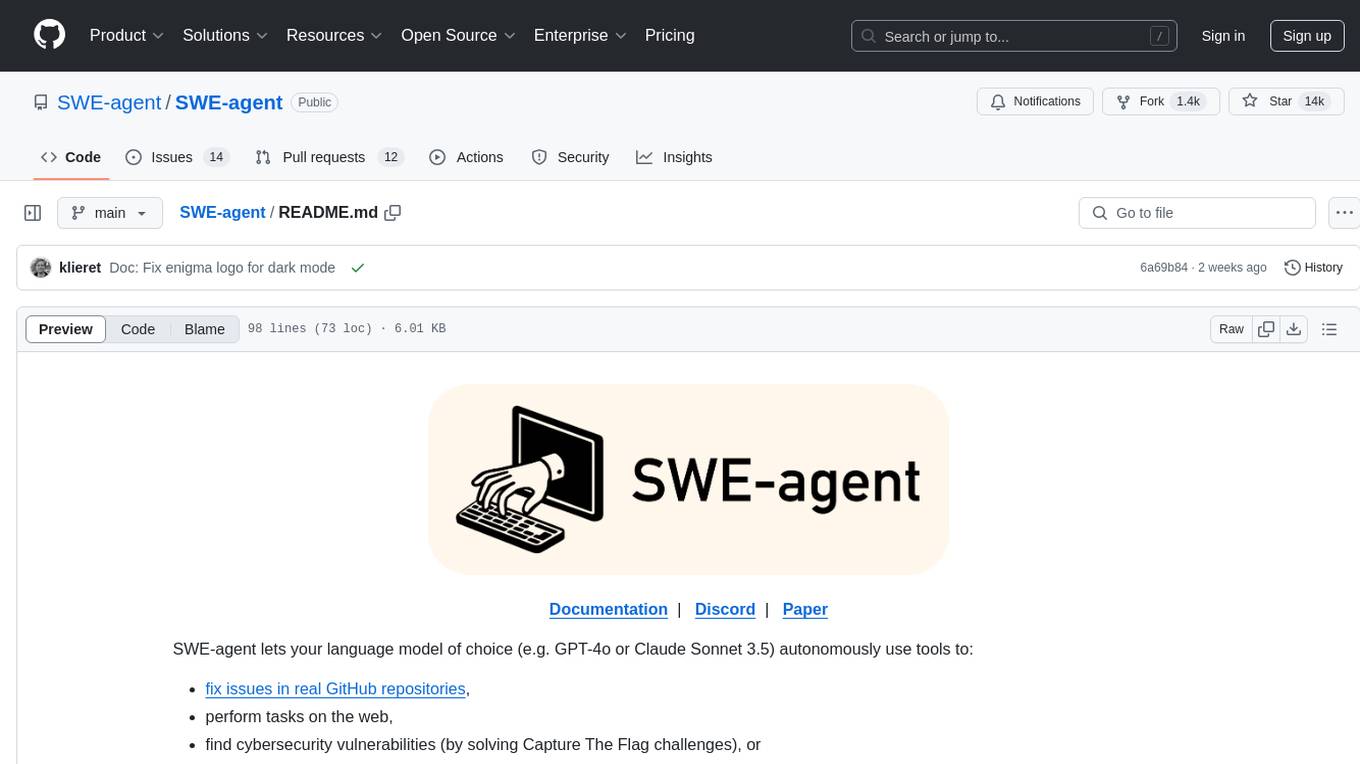
SWE-agent
SWE-agent is a tool that allows language models to autonomously fix issues in GitHub repositories, perform tasks on the web, find cybersecurity vulnerabilities, and handle custom tasks. It uses configurable agent-computer interfaces (ACIs) to interact with isolated computer environments. The tool is built and maintained by researchers from Princeton University and Stanford University.
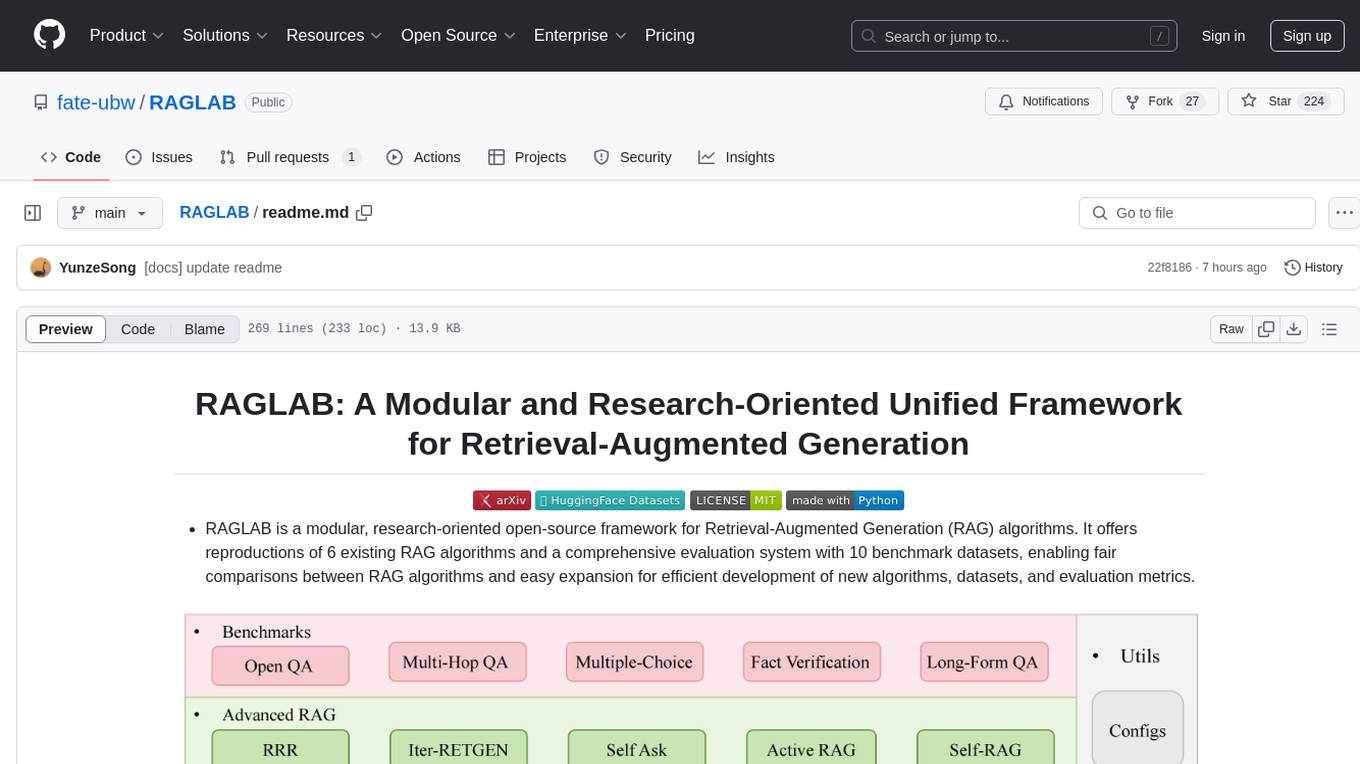
RAGLAB
RAGLAB is a modular, research-oriented open-source framework for Retrieval-Augmented Generation (RAG) algorithms. It offers reproductions of 6 existing RAG algorithms and a comprehensive evaluation system with 10 benchmark datasets, enabling fair comparisons between RAG algorithms and easy expansion for efficient development of new algorithms, datasets, and evaluation metrics. The framework supports the entire RAG pipeline, provides advanced algorithm implementations, fair comparison platform, efficient retriever client, versatile generator support, and flexible instruction lab. It also includes features like Interact Mode for quick understanding of algorithms and Evaluation Mode for reproducing paper results and scientific research.
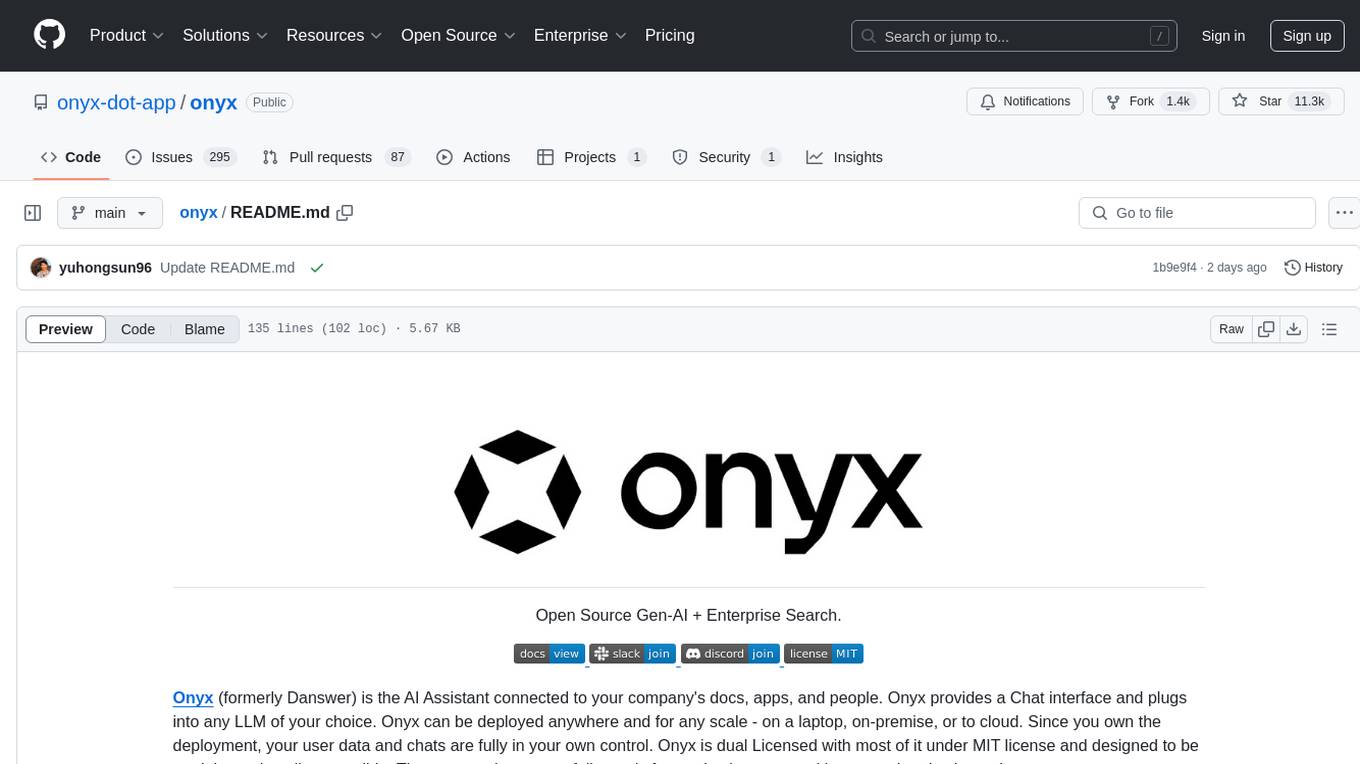
onyx
Onyx is an open-source Gen-AI and Enterprise Search tool that serves as an AI Assistant connected to company documents, apps, and people. It provides a chat interface, can be deployed anywhere, and offers features like user authentication, role management, chat persistence, and UI for configuring AI Assistants. Onyx acts as an Enterprise Search tool across various workplace platforms, enabling users to access team-specific knowledge and perform tasks like document search, AI answers for natural language queries, and integration with common workplace tools like Slack, Google Drive, Confluence, etc.
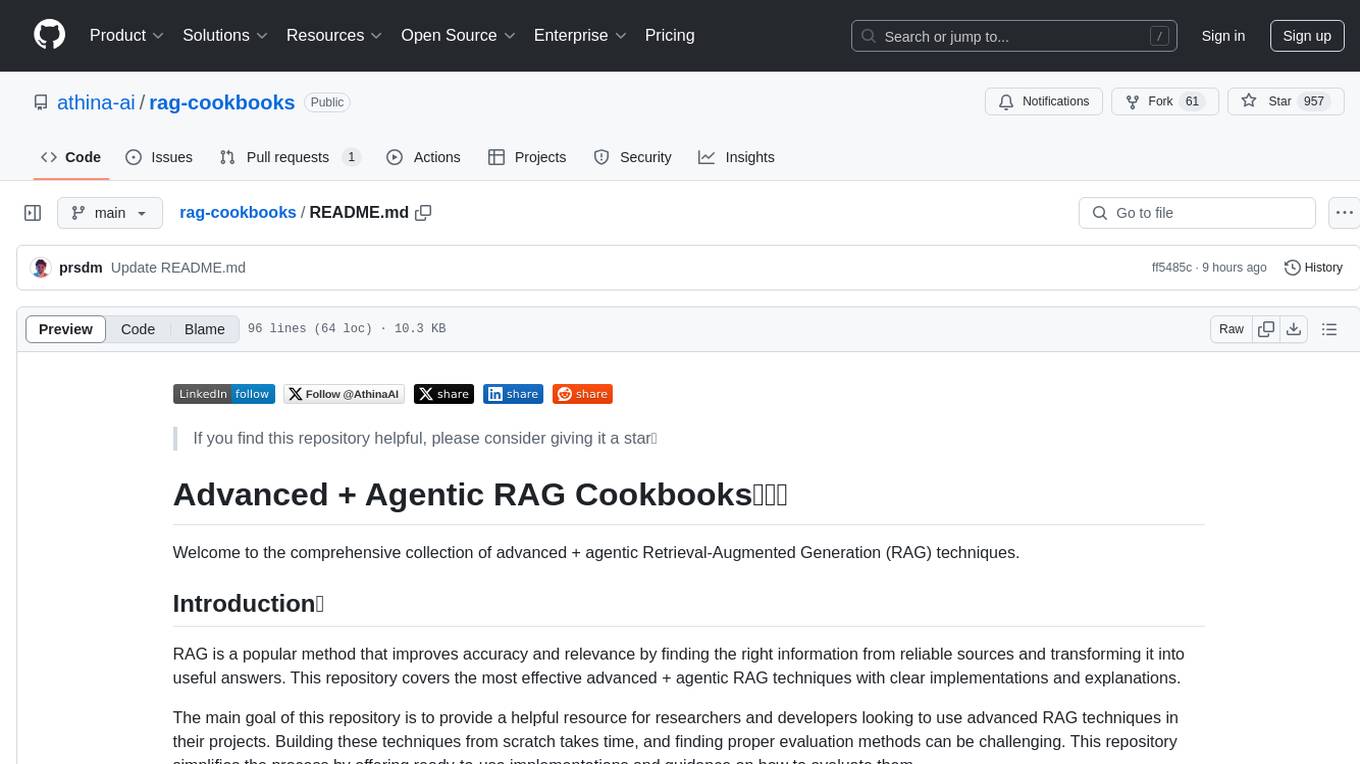
rag-cookbooks
Welcome to the comprehensive collection of advanced + agentic Retrieval-Augmented Generation (RAG) techniques. This repository covers the most effective advanced + agentic RAG techniques with clear implementations and explanations. It aims to provide a helpful resource for researchers and developers looking to use advanced RAG techniques in their projects, offering ready-to-use implementations and guidance on evaluation methods. The RAG framework addresses limitations of Large Language Models by using external documents for in-context learning, ensuring contextually relevant and accurate responses. The repository includes detailed descriptions of various RAG techniques, tools used, and implementation guidance for each technique.
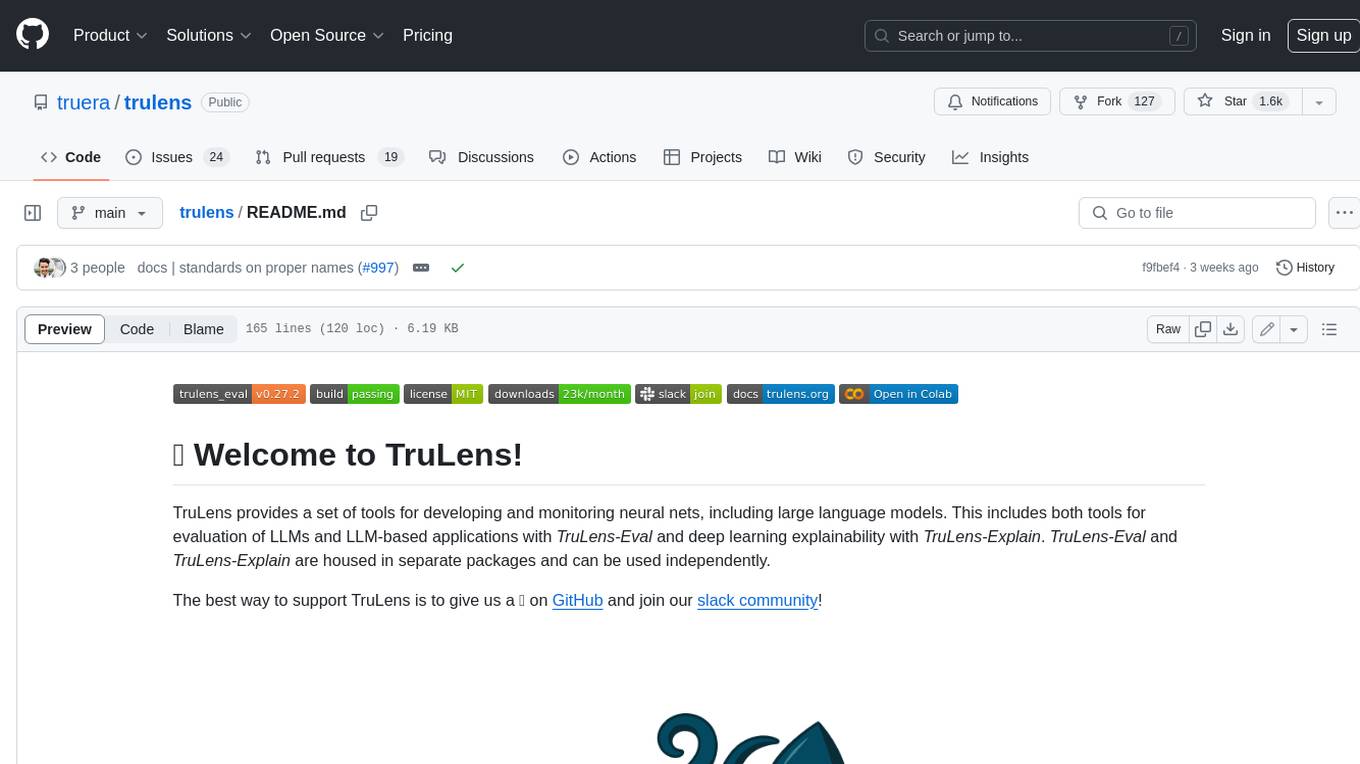
trulens
TruLens provides a set of tools for developing and monitoring neural nets, including large language models. This includes both tools for evaluation of LLMs and LLM-based applications with _TruLens-Eval_ and deep learning explainability with _TruLens-Explain_. _TruLens-Eval_ and _TruLens-Explain_ are housed in separate packages and can be used independently.
For similar tasks
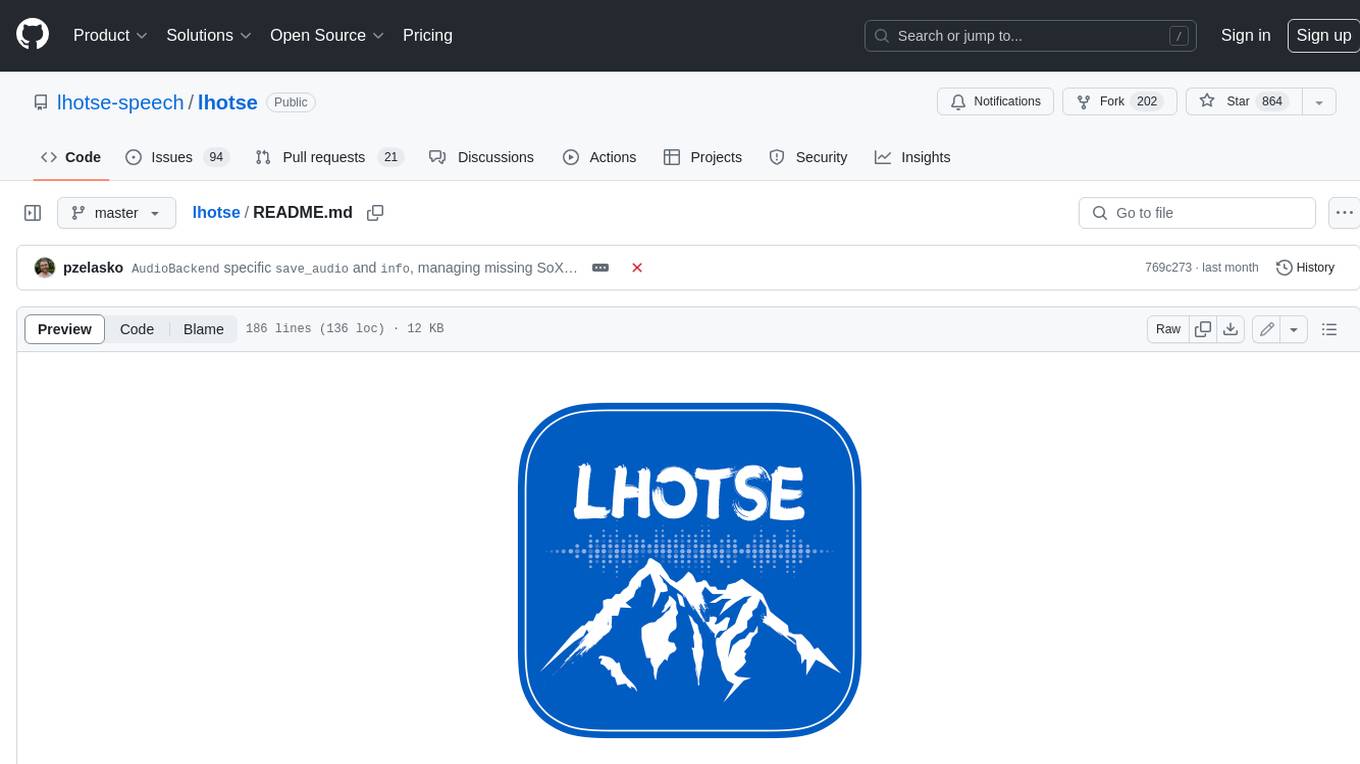
lhotse
Lhotse is a Python library designed to make speech and audio data preparation flexible and accessible. It aims to attract a wider community to speech processing tasks by providing a Python-centric design and an expressive command-line interface. Lhotse offers standard data preparation recipes, PyTorch Dataset classes for speech tasks, and efficient data preparation for model training with audio cuts. It supports data augmentation, feature extraction, and feature-space cut mixing. The tool extends Kaldi's data preparation recipes with seamless PyTorch integration, human-readable text manifests, and convenient Python classes.
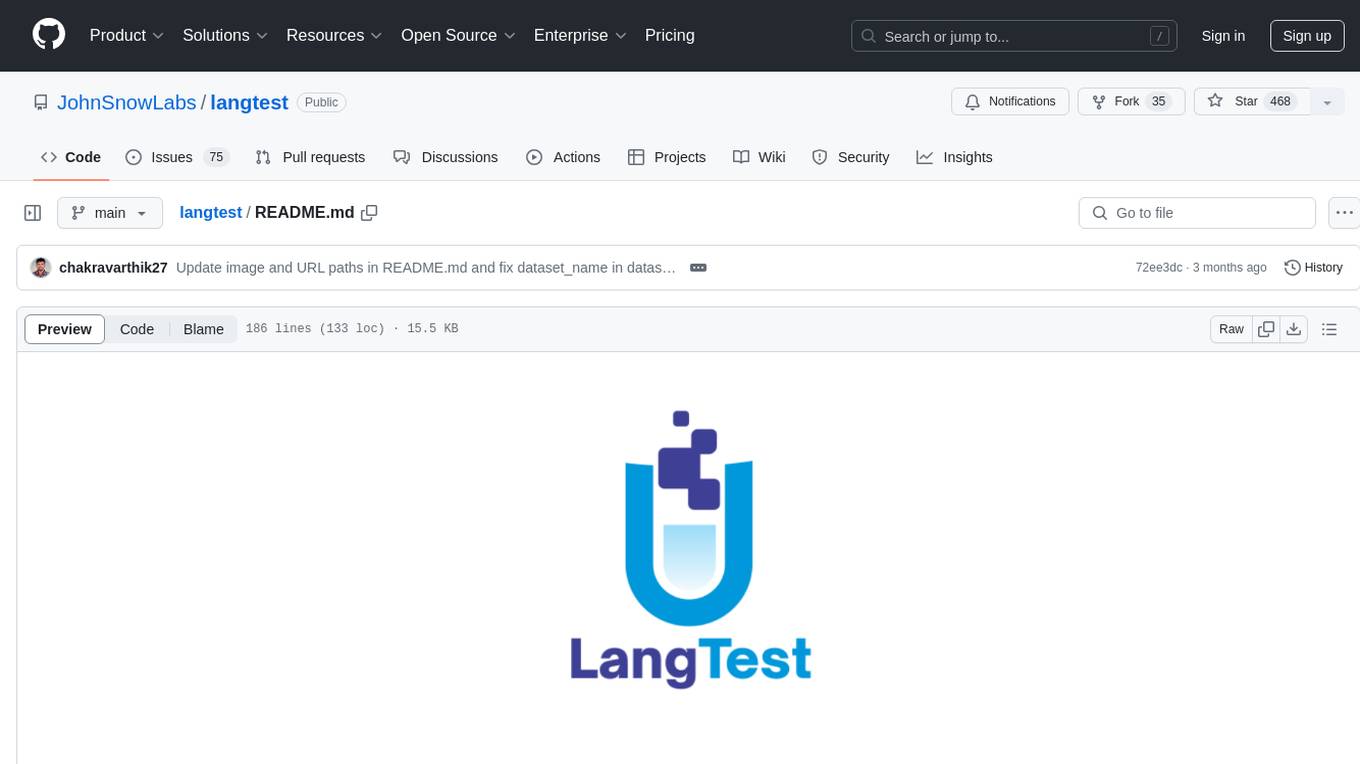
langtest
LangTest is a comprehensive evaluation library for custom LLM and NLP models. It aims to deliver safe and effective language models by providing tools to test model quality, augment training data, and support popular NLP frameworks. LangTest comes with benchmark datasets to challenge and enhance language models, ensuring peak performance in various linguistic tasks. The tool offers more than 60 distinct types of tests with just one line of code, covering aspects like robustness, bias, representation, fairness, and accuracy. It supports testing LLMS for question answering, toxicity, clinical tests, legal support, factuality, sycophancy, and summarization.
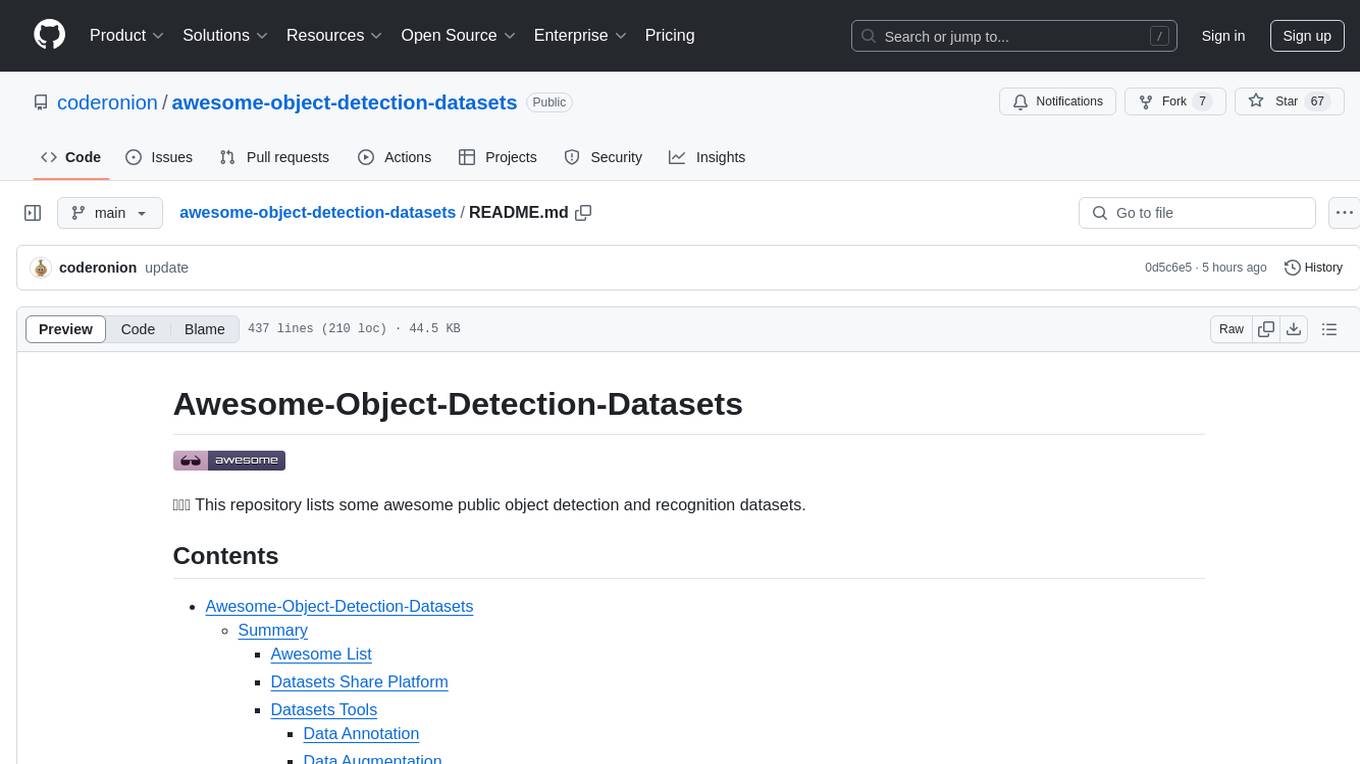
awesome-object-detection-datasets
This repository is a curated list of awesome public object detection and recognition datasets. It includes a wide range of datasets related to object detection and recognition tasks, such as general detection and recognition datasets, autonomous driving datasets, adverse weather datasets, person detection datasets, anti-UAV datasets, optical aerial imagery datasets, low-light image datasets, infrared image datasets, SAR image datasets, multispectral image datasets, 3D object detection datasets, vehicle-to-everything field datasets, super-resolution field datasets, and face detection and recognition datasets. The repository also provides information on tools for data annotation, data augmentation, and data management related to object detection tasks.
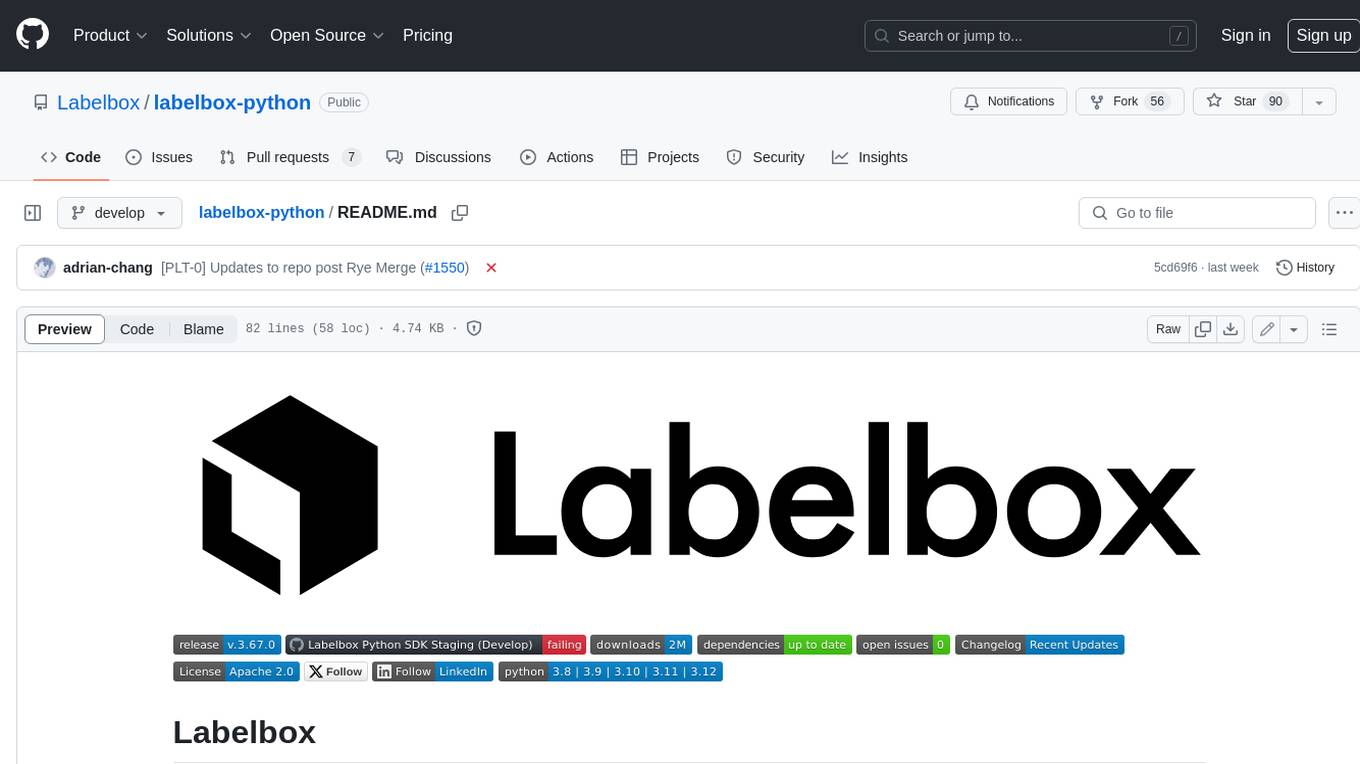
labelbox-python
Labelbox is a data-centric AI platform for enterprises to develop, optimize, and use AI to solve problems and power new products and services. Enterprises use Labelbox to curate data, generate high-quality human feedback data for computer vision and LLMs, evaluate model performance, and automate tasks by combining AI and human-centric workflows. The academic & research community uses Labelbox for cutting-edge AI research.
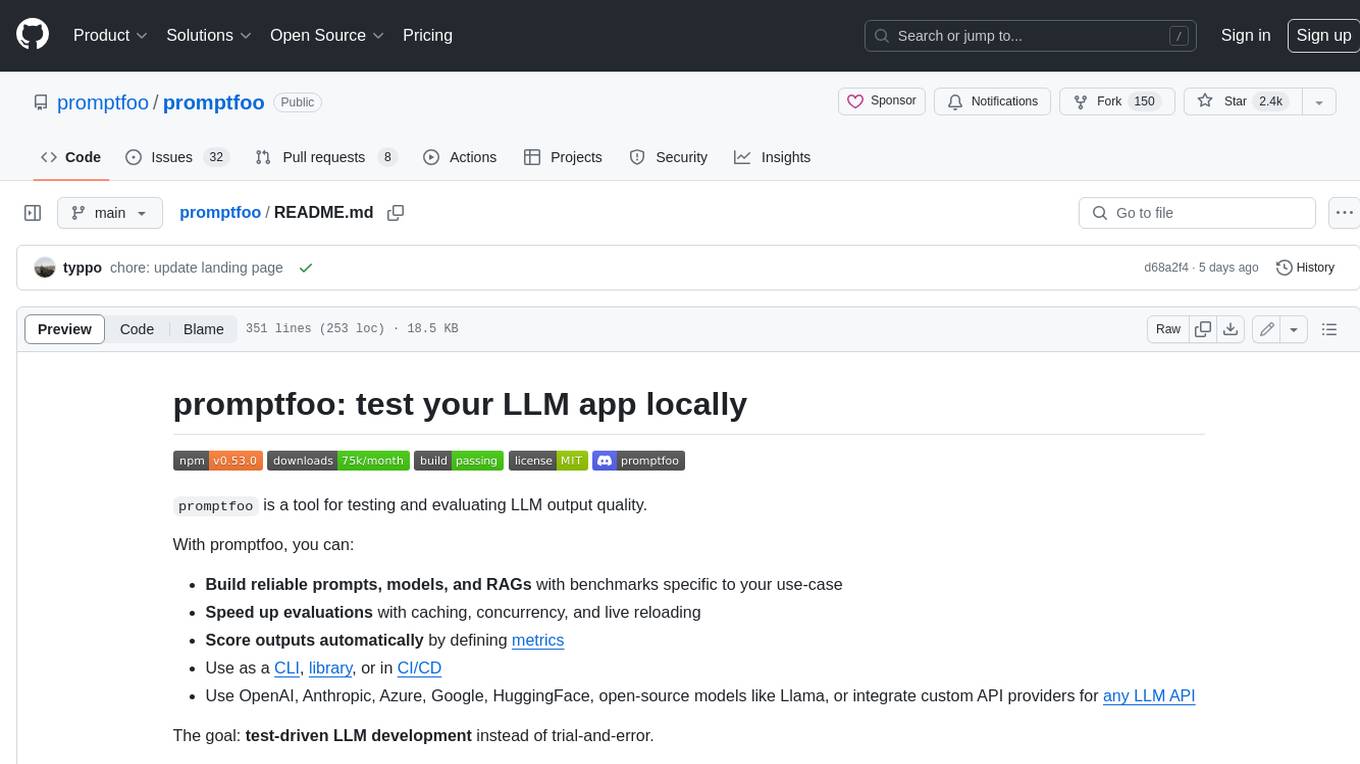
promptfoo
Promptfoo is a tool for testing and evaluating LLM output quality. With promptfoo, you can build reliable prompts, models, and RAGs with benchmarks specific to your use-case, speed up evaluations with caching, concurrency, and live reloading, score outputs automatically by defining metrics, use as a CLI, library, or in CI/CD, and use OpenAI, Anthropic, Azure, Google, HuggingFace, open-source models like Llama, or integrate custom API providers for any LLM API.
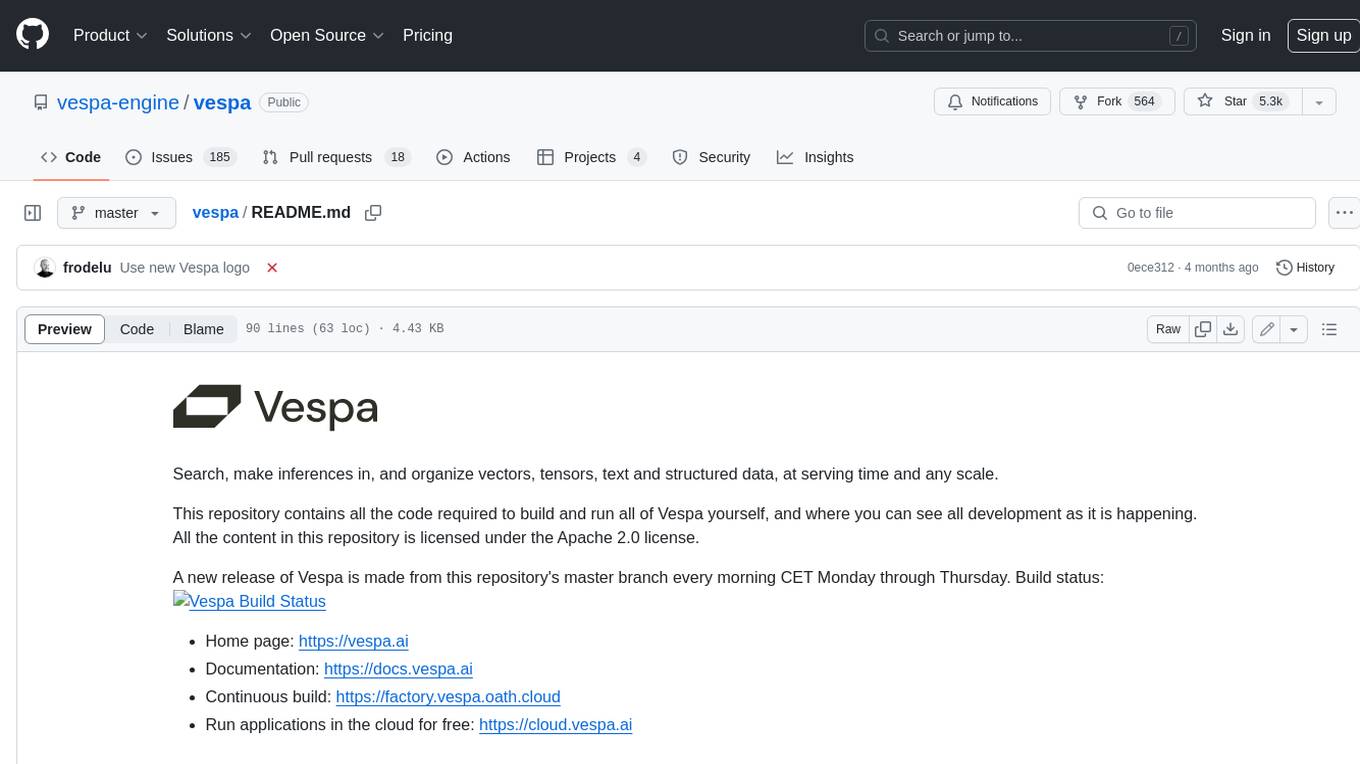
vespa
Vespa is a platform that performs operations such as selecting a subset of data in a large corpus, evaluating machine-learned models over the selected data, organizing and aggregating it, and returning it, typically in less than 100 milliseconds, all while the data corpus is continuously changing. It has been in development for many years and is used on a number of large internet services and apps which serve hundreds of thousands of queries from Vespa per second.
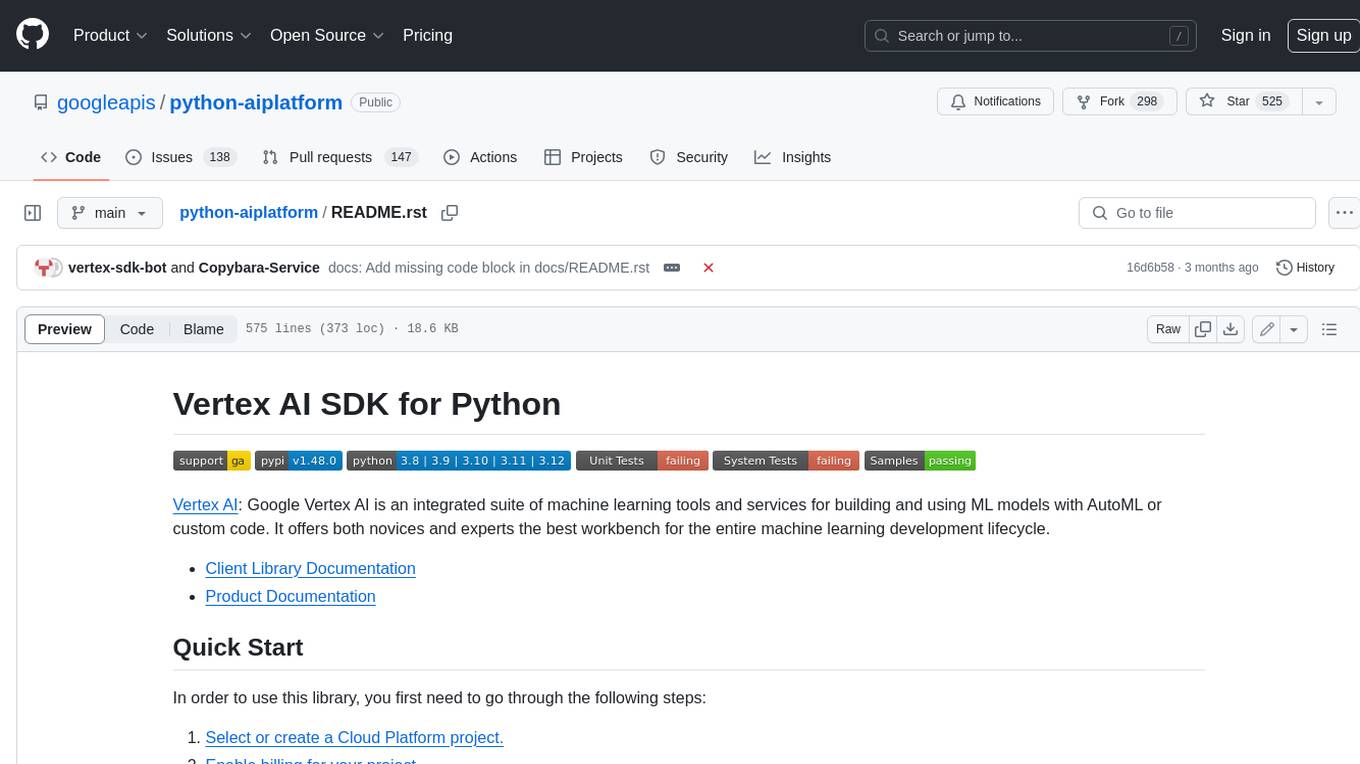
python-aiplatform
The Vertex AI SDK for Python is a library that provides a convenient way to use the Vertex AI API. It offers a high-level interface for creating and managing Vertex AI resources, such as datasets, models, and endpoints. The SDK also provides support for training and deploying custom models, as well as using AutoML models. With the Vertex AI SDK for Python, you can quickly and easily build and deploy machine learning models on Vertex AI.
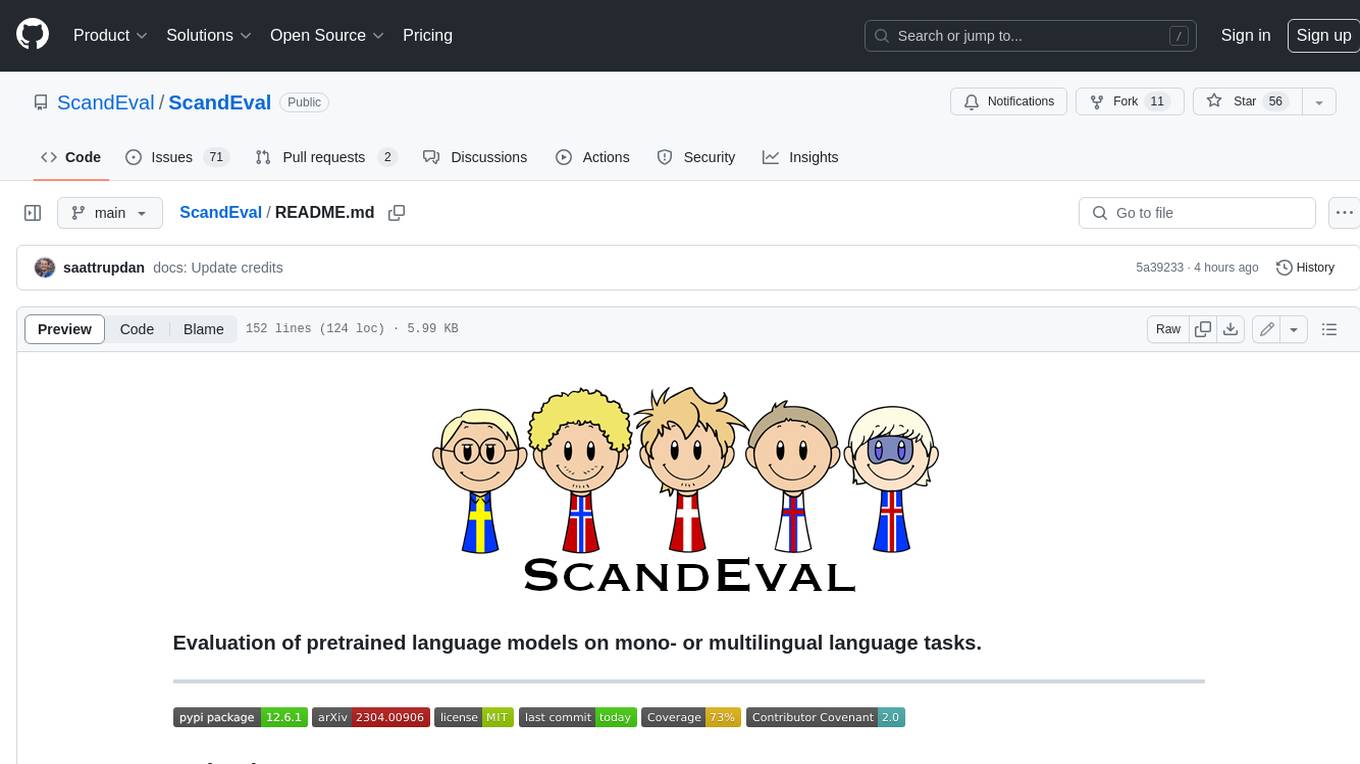
ScandEval
ScandEval is a framework for evaluating pretrained language models on mono- or multilingual language tasks. It provides a unified interface for benchmarking models on a variety of tasks, including sentiment analysis, question answering, and machine translation. ScandEval is designed to be easy to use and extensible, making it a valuable tool for researchers and practitioners alike.
For similar jobs
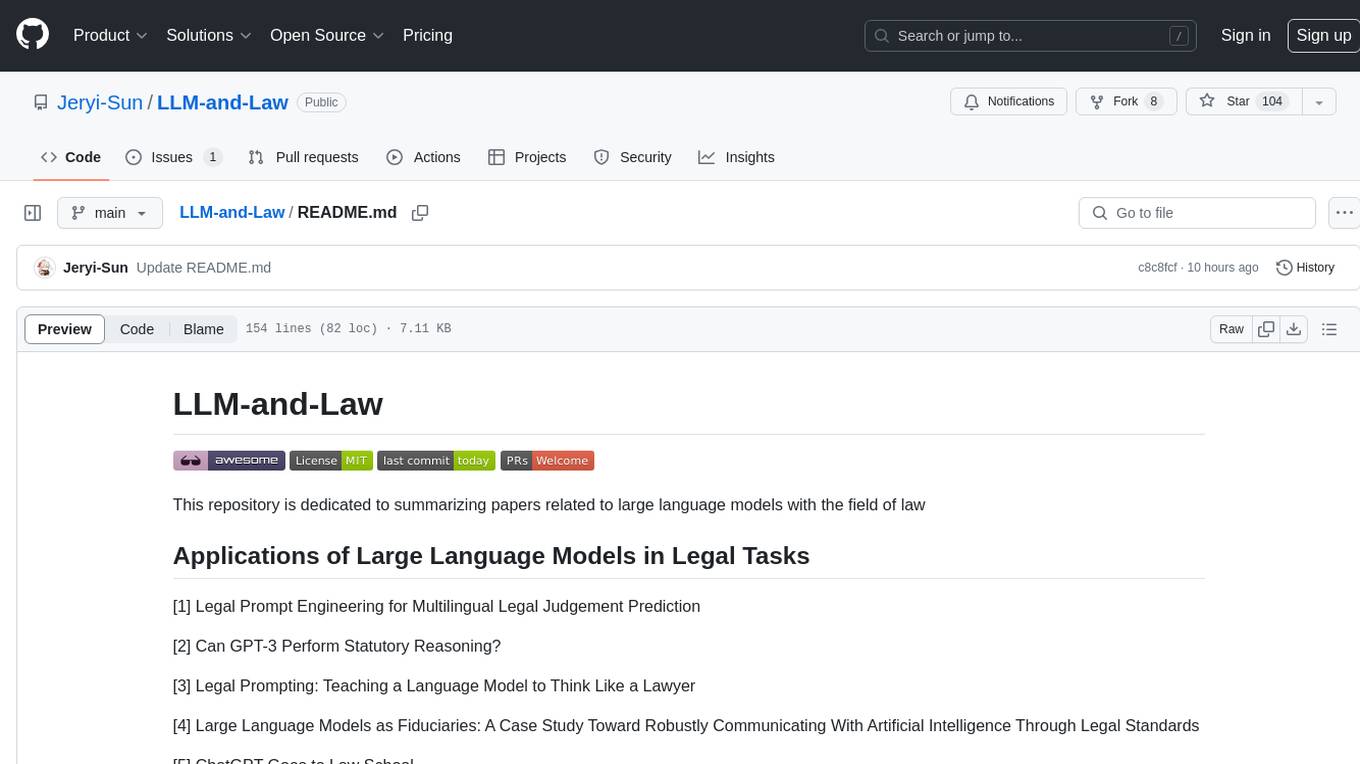
LLM-and-Law
This repository is dedicated to summarizing papers related to large language models with the field of law. It includes applications of large language models in legal tasks, legal agents, legal problems of large language models, data resources for large language models in law, law LLMs, and evaluation of large language models in the legal domain.
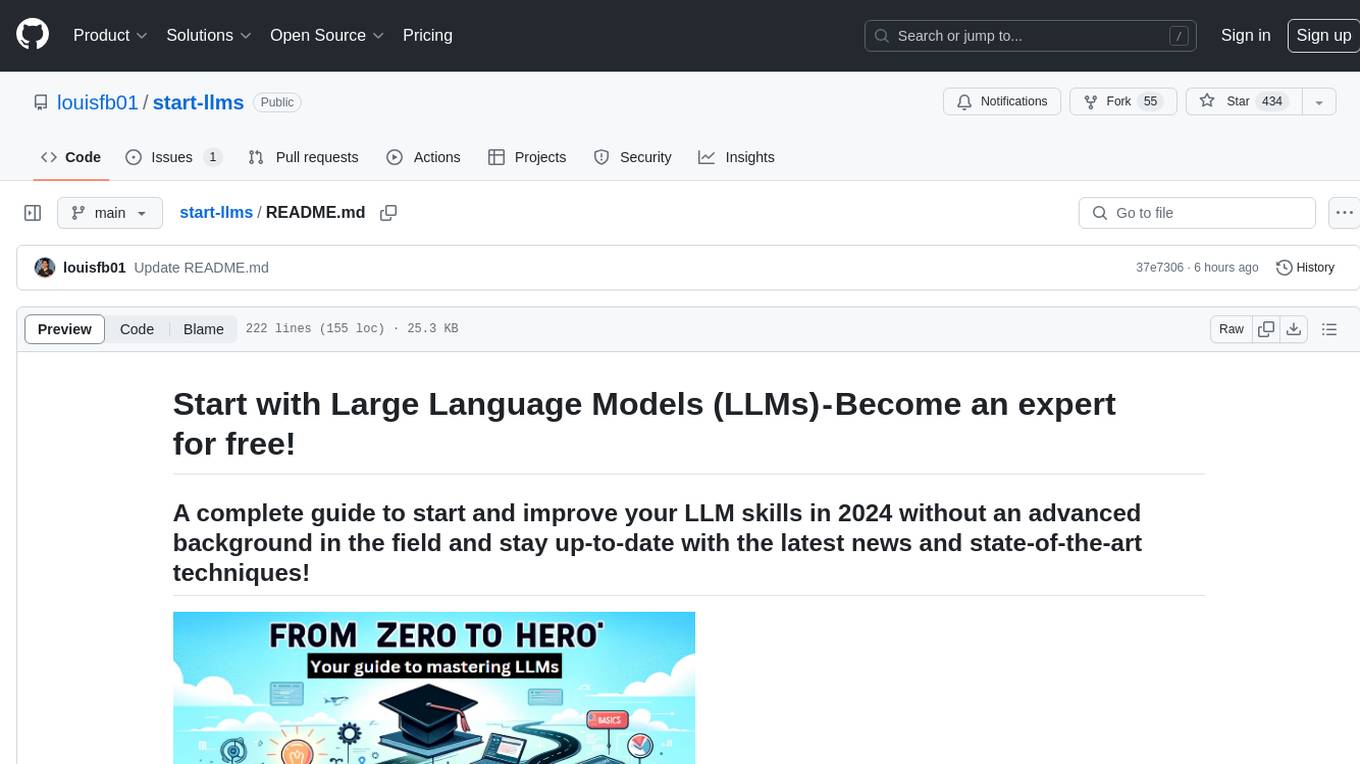
start-llms
This repository is a comprehensive guide for individuals looking to start and improve their skills in Large Language Models (LLMs) without an advanced background in the field. It provides free resources, online courses, books, articles, and practical tips to become an expert in machine learning. The guide covers topics such as terminology, transformers, prompting, retrieval augmented generation (RAG), and more. It also includes recommendations for podcasts, YouTube videos, and communities to stay updated with the latest news in AI and LLMs.
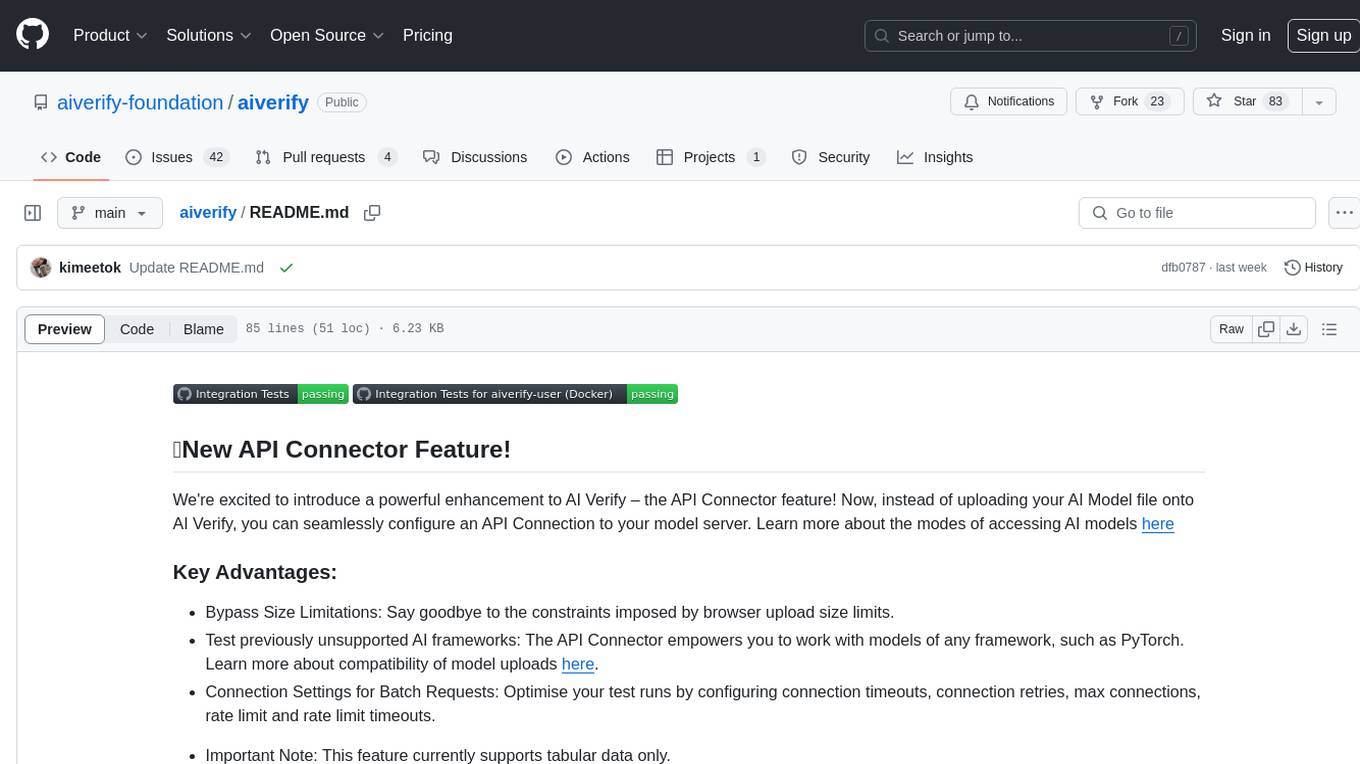
aiverify
AI Verify is an AI governance testing framework and software toolkit that validates the performance of AI systems against internationally recognised principles through standardised tests. It offers a new API Connector feature to bypass size limitations, test various AI frameworks, and configure connection settings for batch requests. The toolkit operates within an enterprise environment, conducting technical tests on common supervised learning models for tabular and image datasets. It does not define AI ethical standards or guarantee complete safety from risks or biases.
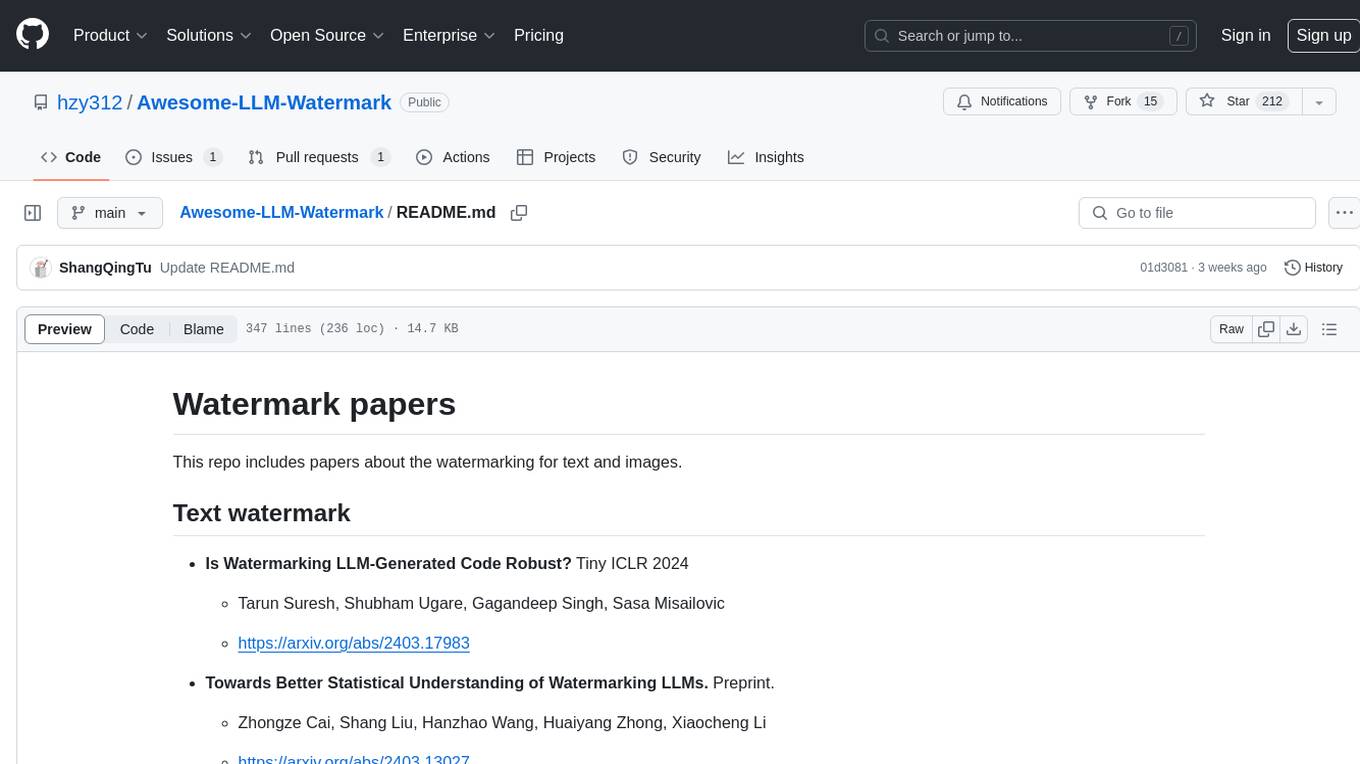
Awesome-LLM-Watermark
This repository contains a collection of research papers related to watermarking techniques for text and images, specifically focusing on large language models (LLMs). The papers cover various aspects of watermarking LLM-generated content, including robustness, statistical understanding, topic-based watermarks, quality-detection trade-offs, dual watermarks, watermark collision, and more. Researchers have explored different methods and frameworks for watermarking LLMs to protect intellectual property, detect machine-generated text, improve generation quality, and evaluate watermarking techniques. The repository serves as a valuable resource for those interested in the field of watermarking for LLMs.
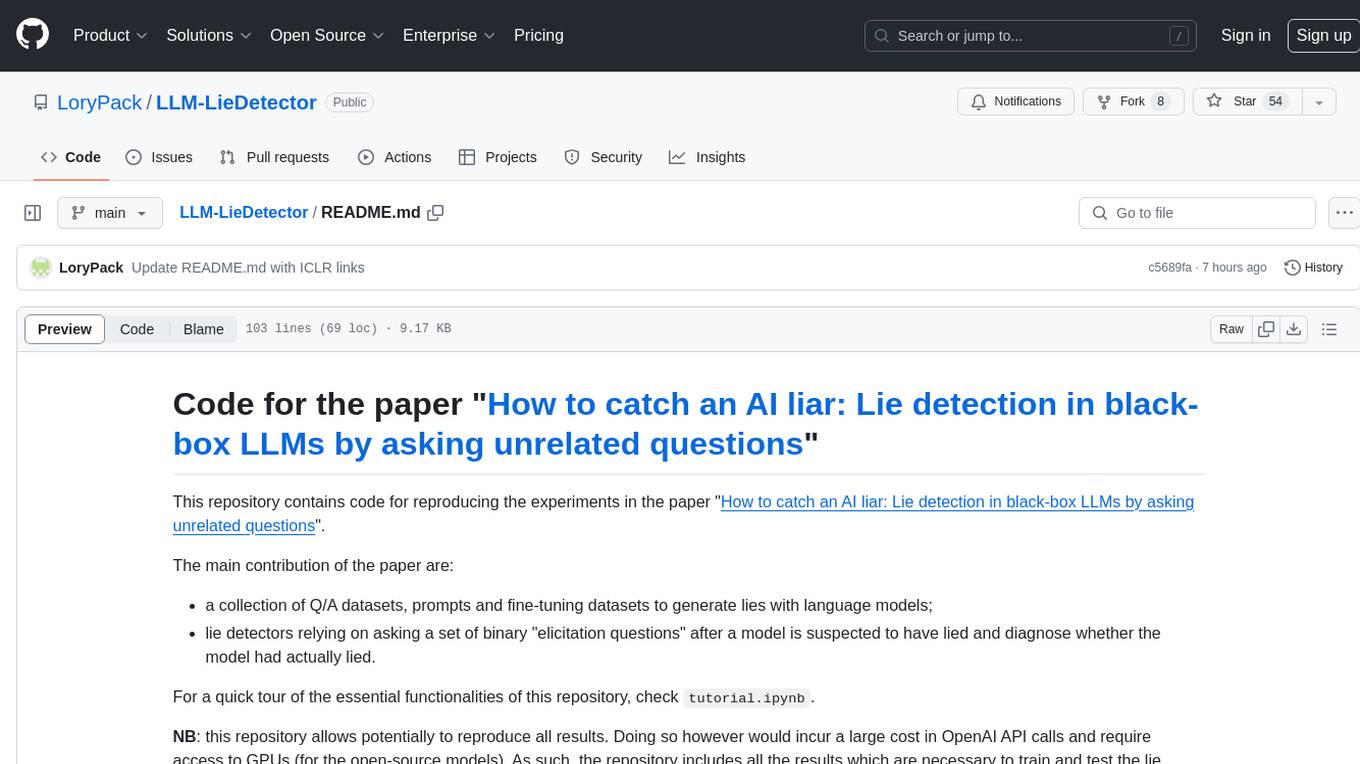
LLM-LieDetector
This repository contains code for reproducing experiments on lie detection in black-box LLMs by asking unrelated questions. It includes Q/A datasets, prompts, and fine-tuning datasets for generating lies with language models. The lie detectors rely on asking binary 'elicitation questions' to diagnose whether the model has lied. The code covers generating lies from language models, training and testing lie detectors, and generalization experiments. It requires access to GPUs and OpenAI API calls for running experiments with open-source models. Results are stored in the repository for reproducibility.
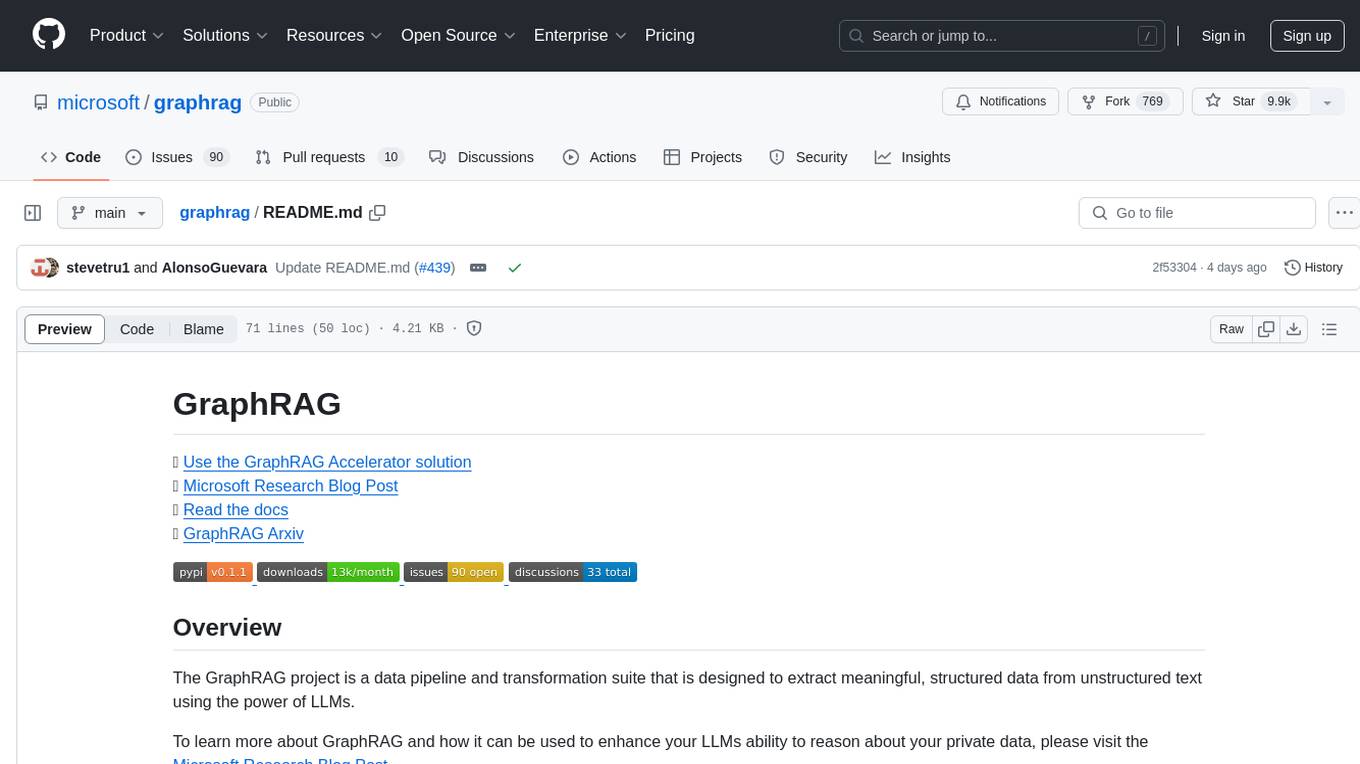
graphrag
The GraphRAG project is a data pipeline and transformation suite designed to extract meaningful, structured data from unstructured text using LLMs. It enhances LLMs' ability to reason about private data. The repository provides guidance on using knowledge graph memory structures to enhance LLM outputs, with a warning about the potential costs of GraphRAG indexing. It offers contribution guidelines, development resources, and encourages prompt tuning for optimal results. The Responsible AI FAQ addresses GraphRAG's capabilities, intended uses, evaluation metrics, limitations, and operational factors for effective and responsible use.
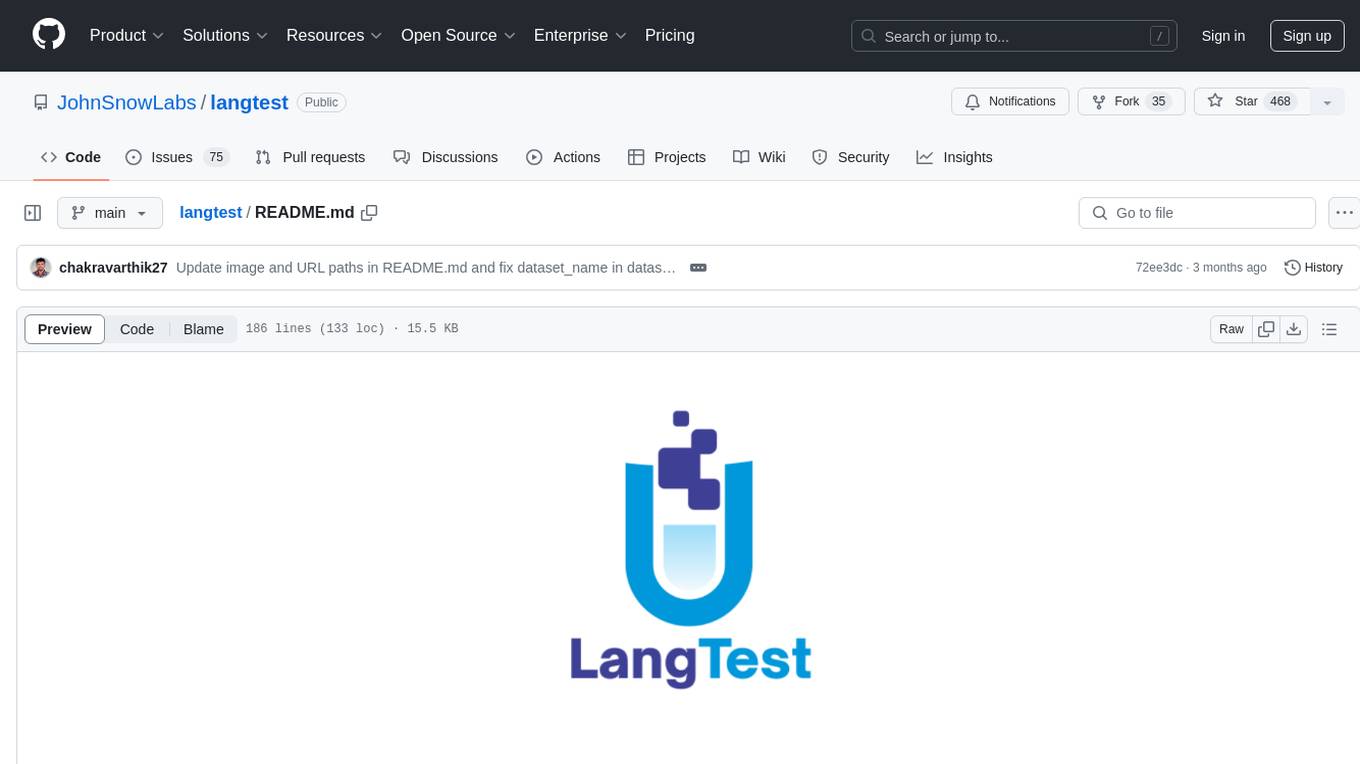
langtest
LangTest is a comprehensive evaluation library for custom LLM and NLP models. It aims to deliver safe and effective language models by providing tools to test model quality, augment training data, and support popular NLP frameworks. LangTest comes with benchmark datasets to challenge and enhance language models, ensuring peak performance in various linguistic tasks. The tool offers more than 60 distinct types of tests with just one line of code, covering aspects like robustness, bias, representation, fairness, and accuracy. It supports testing LLMS for question answering, toxicity, clinical tests, legal support, factuality, sycophancy, and summarization.
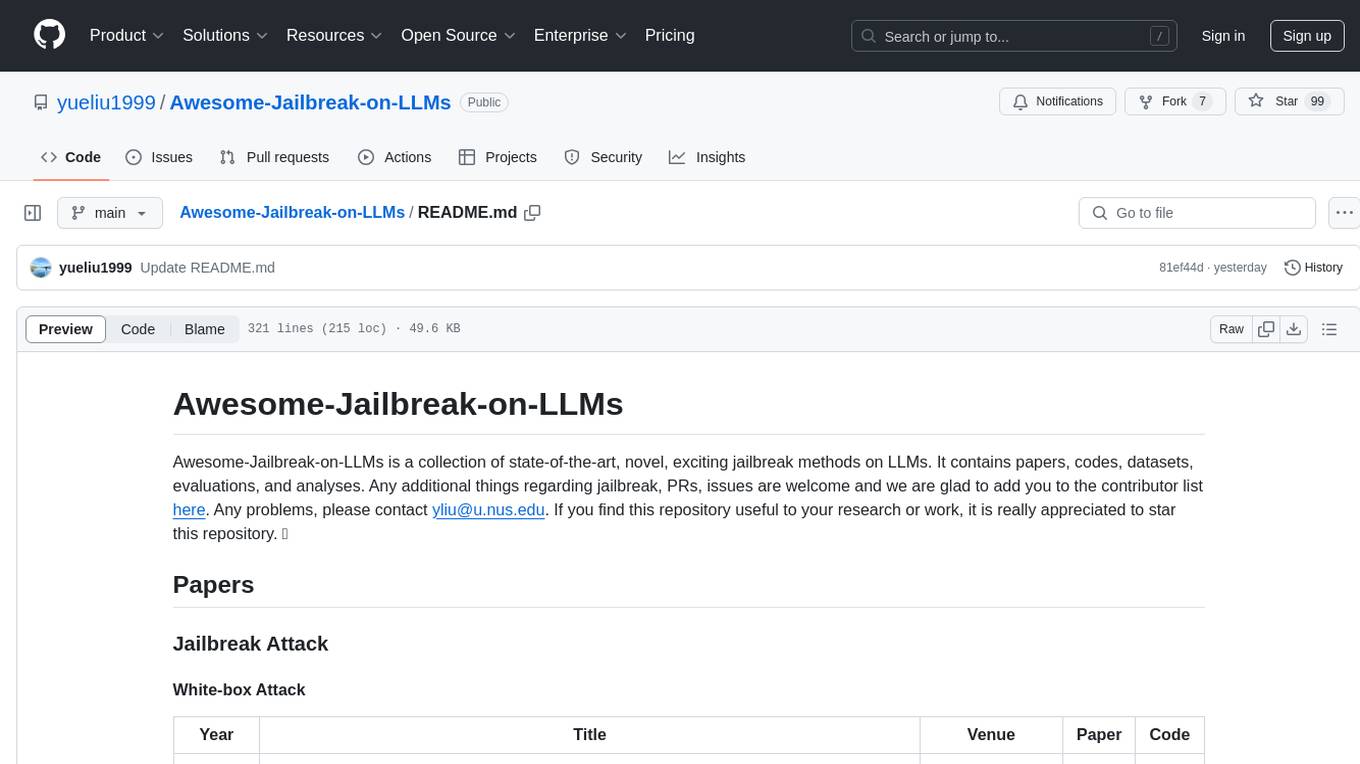
Awesome-Jailbreak-on-LLMs
Awesome-Jailbreak-on-LLMs is a collection of state-of-the-art, novel, and exciting jailbreak methods on Large Language Models (LLMs). The repository contains papers, codes, datasets, evaluations, and analyses related to jailbreak attacks on LLMs. It serves as a comprehensive resource for researchers and practitioners interested in exploring various jailbreak techniques and defenses in the context of LLMs. Contributions such as additional jailbreak-related content, pull requests, and issue reports are welcome, and contributors are acknowledged. For any inquiries or issues, contact [email protected]. If you find this repository useful for your research or work, consider starring it to show appreciation.