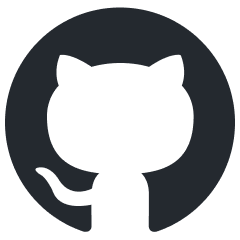
xaitk-saliency
As part of the Explainable AI Toolkit (XAITK), XAITK-Saliency is an open source, explainable AI framework for visual saliency algorithm interfaces and implementations, built for analytics and autonomy applications.
Stars: 83
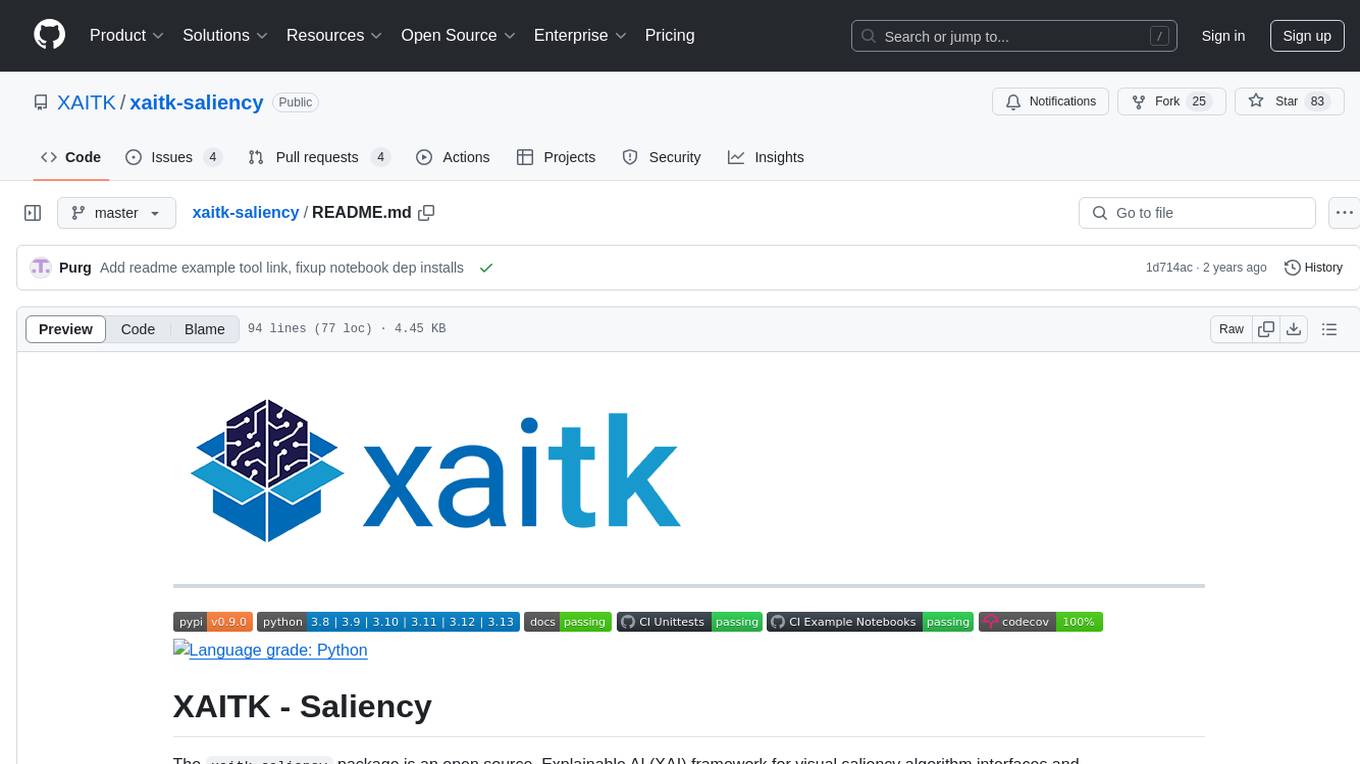
The `xaitk-saliency` package is an open source Explainable AI (XAI) framework for visual saliency algorithm interfaces and implementations, designed for analytics and autonomy applications. It provides saliency algorithms for various image understanding tasks such as image classification, image similarity, object detection, and reinforcement learning. The toolkit targets data scientists and developers who aim to incorporate visual saliency explanations into their workflow or product, offering both direct accessibility for experimentation and modular integration into systems and applications through Strategy and Adapter patterns. The package includes documentation, examples, and a demonstration tool for visual saliency generation in a user-interface.
README:
The xaitk-saliency
package is an open source, Explainable AI (XAI) framework
for visual saliency algorithm interfaces and implementations, built for
analytics and autonomy applications.
See here for a more formal introduction to the topic of XAI and visual saliency explanations.
This framework is a part of the Explainable AI Toolkit (XAITK).
The xaitk-saliency
package provides saliency algorithms for a wide range of image understanding
tasks, including image classification, image similarity, object detection, and reinforcement learning.
The current list of supported saliency algorithms can be found here.
This toolkit is intended to help data scientists and developers who want to add visual saliency explanations to their workflow or product. Functionality provided here is both directly accessible for targeted experimentation, and through Strategy and Adapter patterns to allow for modular integration into systems and applications.
Install the latest release via pip:
pip install xaitk-saliency
Some plugins may require additional dependencies in order to be utilized at runtime. Such details are described here.
See here for more installation documentation.
We provide a number of examples based on Jupyter notebooks in the ./examples/
directory to show usage of the xaitk-saliency
package in a number of
different contexts.
Contributions are welcome! See the CONTRIBUTING.md file for details.
Documentation snapshots for releases as well as the latest master are hosted on ReadTheDocs.
The sphinx-based documentation may also be built locally for the most up-to-date reference:
# Install dependencies
poetry install
# Navigate to the documentation root.
cd docs
# Build the docs.
poetry run make html
# Open in your favorite browser!
firefox _build/html/index.html
This associated project provides a local web-application that provides a demonstration of visual saliency generation in a user-interface. This provides an example of how visual saliency, as generated by this package, can be utilized in a user-interface to facilitate model and results exploration. This tool uses the trame framework.
![]() |
![]() |
![]() |
![]() |
---|
For Tasks:
Click tags to check more tools for each tasksFor Jobs:
Alternative AI tools for xaitk-saliency
Similar Open Source Tools
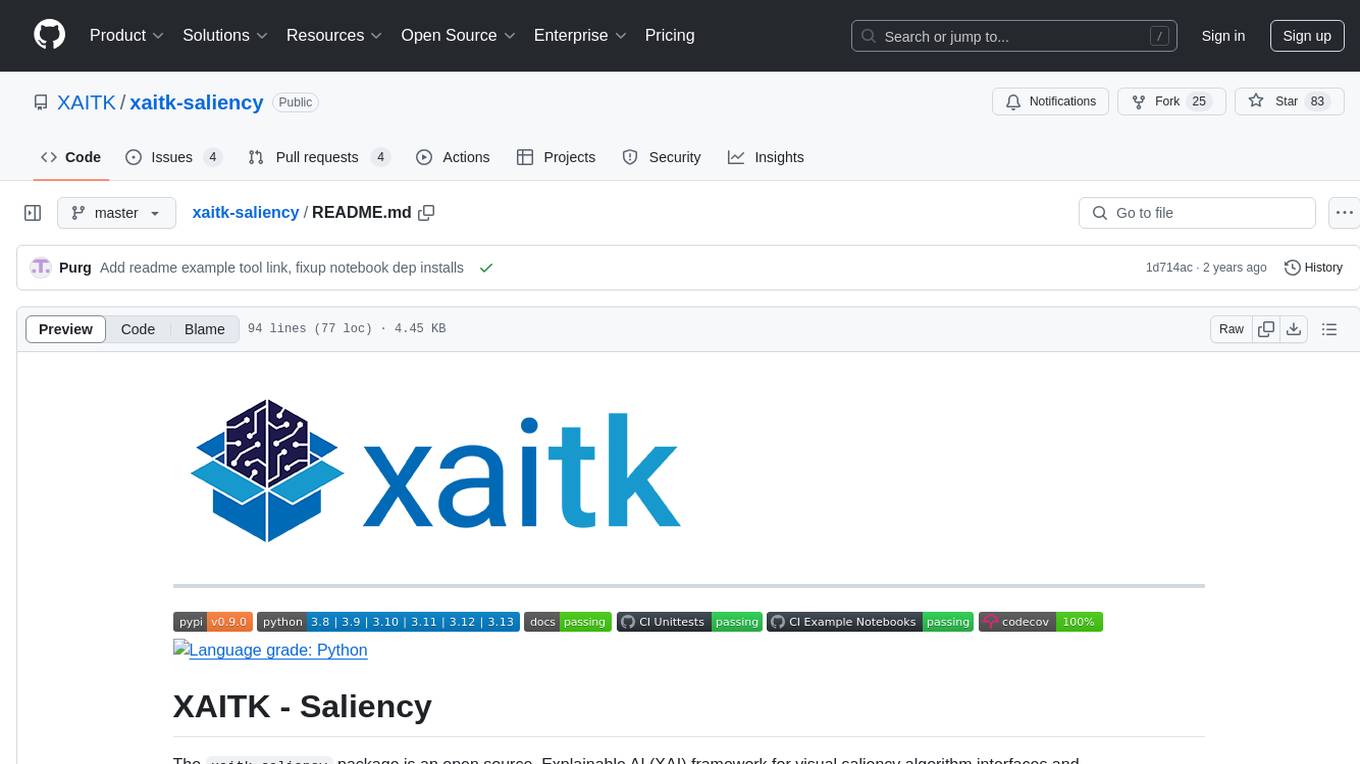
xaitk-saliency
The `xaitk-saliency` package is an open source Explainable AI (XAI) framework for visual saliency algorithm interfaces and implementations, designed for analytics and autonomy applications. It provides saliency algorithms for various image understanding tasks such as image classification, image similarity, object detection, and reinforcement learning. The toolkit targets data scientists and developers who aim to incorporate visual saliency explanations into their workflow or product, offering both direct accessibility for experimentation and modular integration into systems and applications through Strategy and Adapter patterns. The package includes documentation, examples, and a demonstration tool for visual saliency generation in a user-interface.
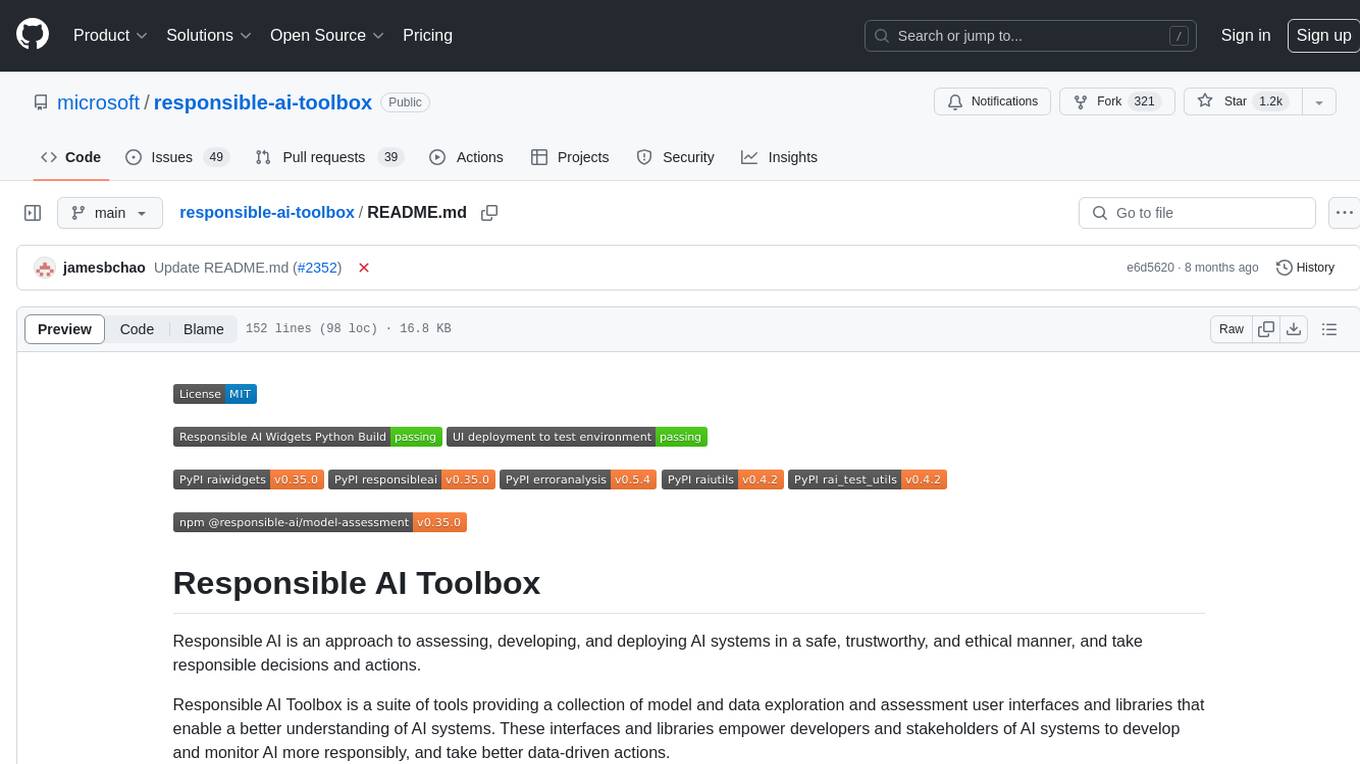
responsible-ai-toolbox
Responsible AI Toolbox is a suite of tools providing model and data exploration and assessment interfaces and libraries for understanding AI systems. It empowers developers and stakeholders to develop and monitor AI responsibly, enabling better data-driven actions. The toolbox includes visualization widgets for model assessment, error analysis, interpretability, fairness assessment, and mitigations library. It also offers a JupyterLab extension for managing machine learning experiments and a library for measuring gender bias in NLP datasets.
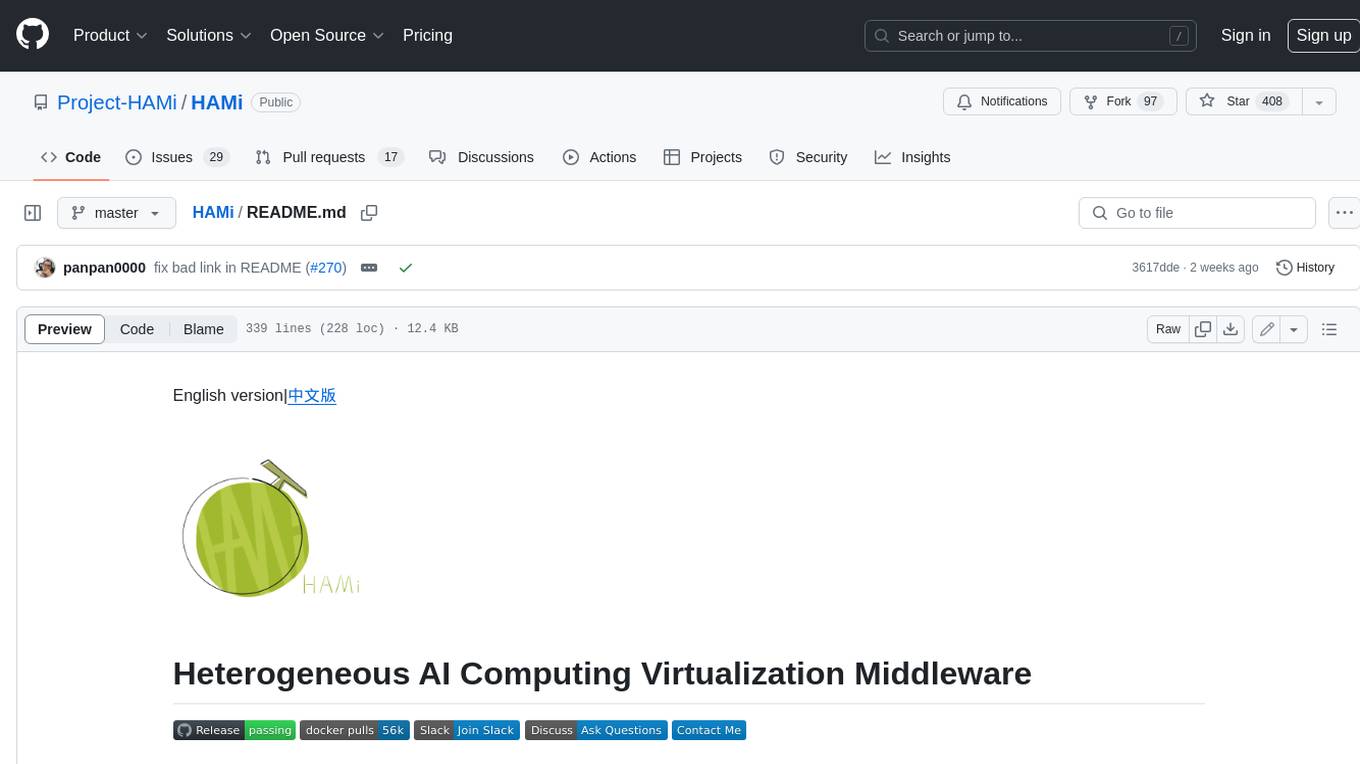
HAMi
HAMi is a Heterogeneous AI Computing Virtualization Middleware designed to manage Heterogeneous AI Computing Devices in a Kubernetes cluster. It allows for device sharing, device memory control, device type specification, and device UUID specification. The tool is easy to use and does not require modifying task YAML files. It includes features like hard limits on device memory, partial device allocation, streaming multiprocessor limits, and core usage specification. HAMi consists of components like a mutating webhook, scheduler extender, device plugins, and in-container virtualization techniques. It is suitable for scenarios requiring device sharing, specific device memory allocation, GPU balancing, low utilization optimization, and scenarios needing multiple small GPUs. The tool requires prerequisites like NVIDIA drivers, CUDA version, nvidia-docker, Kubernetes version, glibc version, and helm. Users can install, upgrade, and uninstall HAMi, submit tasks, and monitor cluster information. The tool's roadmap includes supporting additional AI computing devices, video codec processing, and Multi-Instance GPUs (MIG).
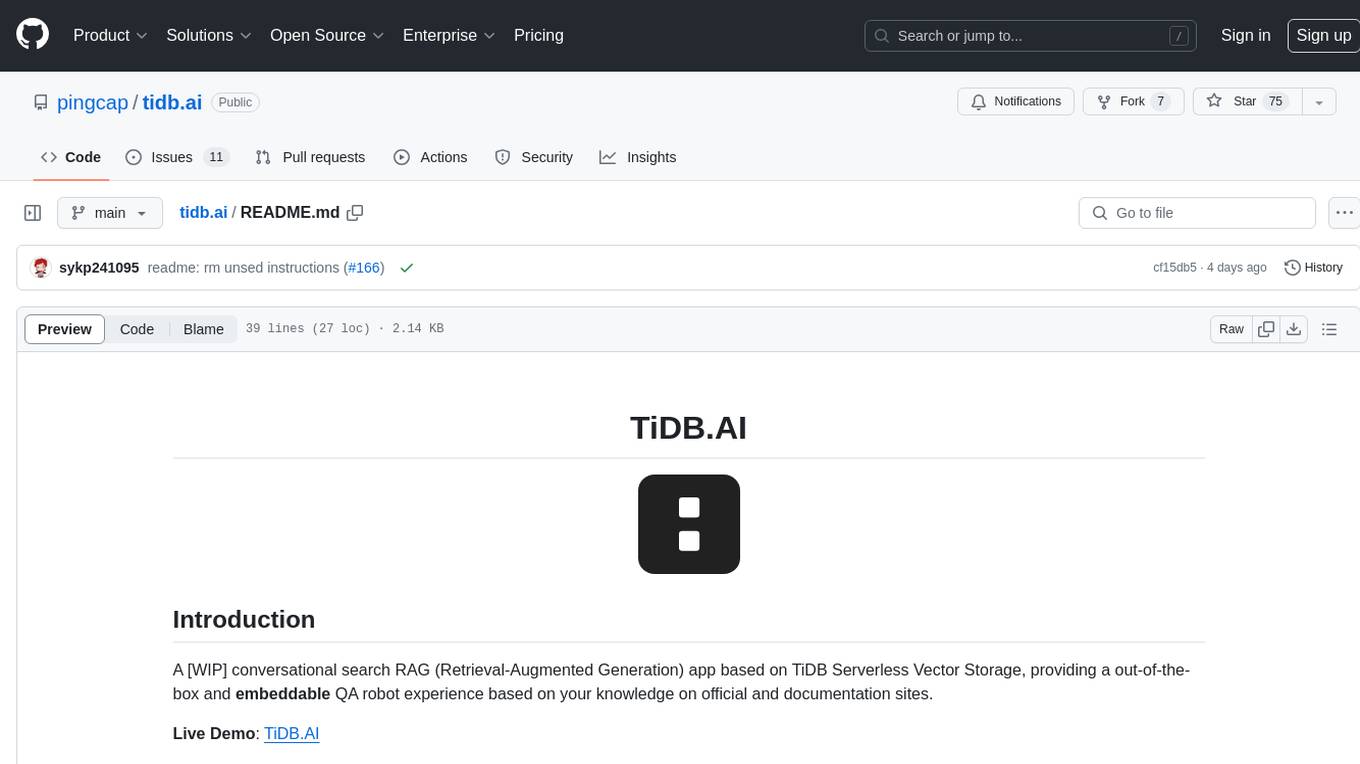
tidb.ai
TiDB.AI is a conversational search RAG (Retrieval-Augmented Generation) app based on TiDB Serverless Vector Storage. It provides an out-of-the-box and embeddable QA robot experience based on knowledge from official and documentation sites. The platform features a Perplexity-style Conversational Search page with an advanced built-in website crawler for comprehensive coverage. Users can integrate an embeddable JavaScript snippet into their website for instant responses to product-related queries. The tech stack includes Next.js, TypeScript, Tailwind CSS, shadcn/ui for design, TiDB for database storage, Kysely for SQL query building, NextAuth.js for authentication, Vercel for deployments, and LlamaIndex for the RAG framework. TiDB.AI is open-source under the Apache License, Version 2.0.
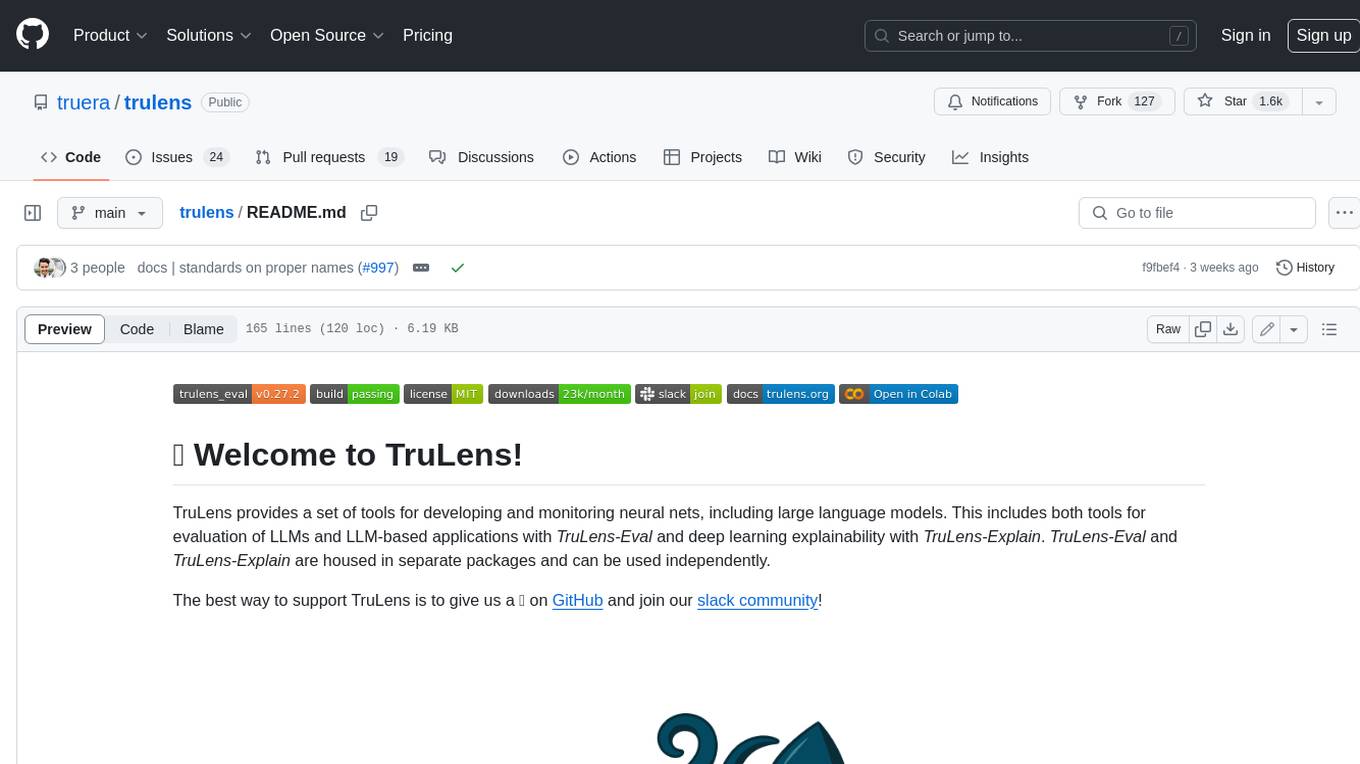
trulens
TruLens provides a set of tools for developing and monitoring neural nets, including large language models. This includes both tools for evaluation of LLMs and LLM-based applications with _TruLens-Eval_ and deep learning explainability with _TruLens-Explain_. _TruLens-Eval_ and _TruLens-Explain_ are housed in separate packages and can be used independently.
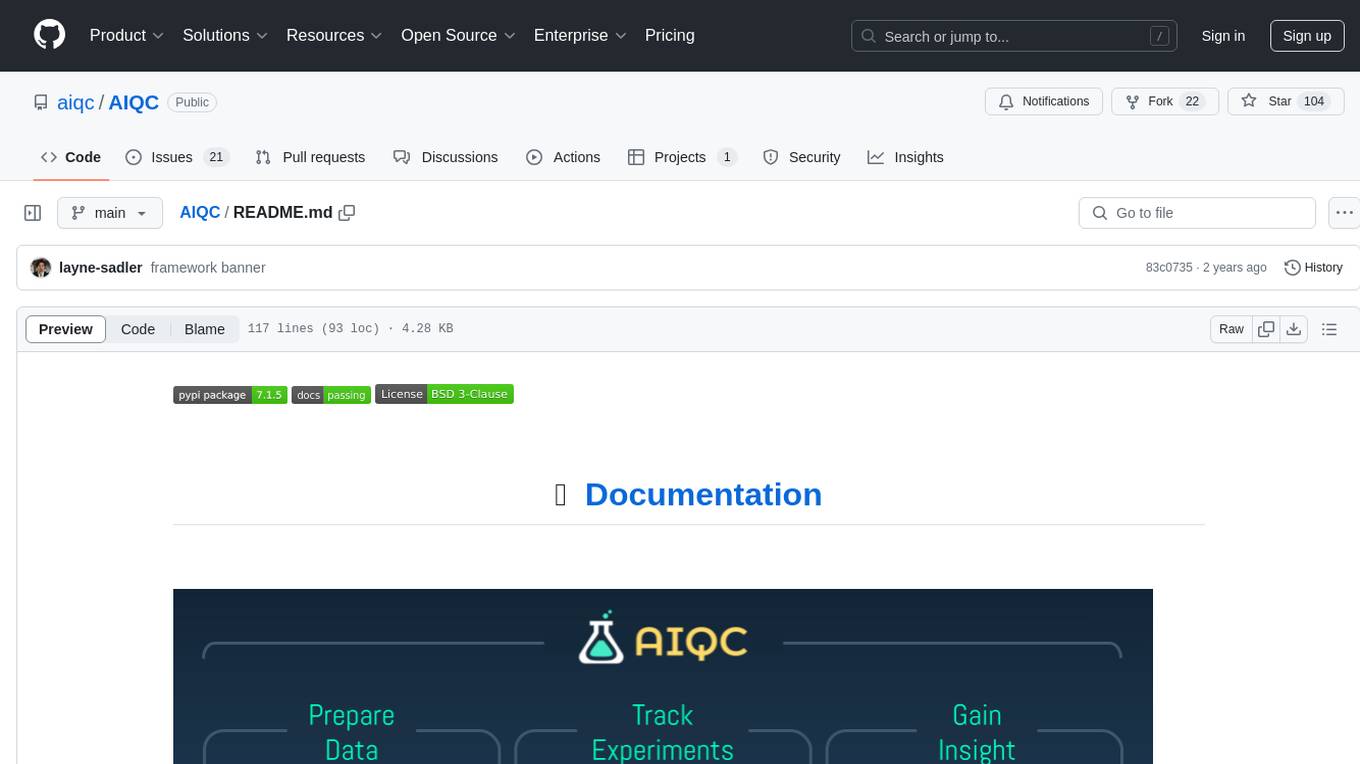
AIQC
AIQC is an open source Python package that provides a declarative API for end-to-end MLOps in order to make deep learning more accessible to researchers. It utilizes a SQLite object-relational model for machine learning objects and stacks standardized workflows for various analyses, data types, and libraries. The benefits include a 90% reduction in data wrangling, reproducibility, and no need to install and maintain application and database servers for experiment tracking. AIQC is pip-installable and provides a Dash-Plotly UI for real-time experiment tracking.
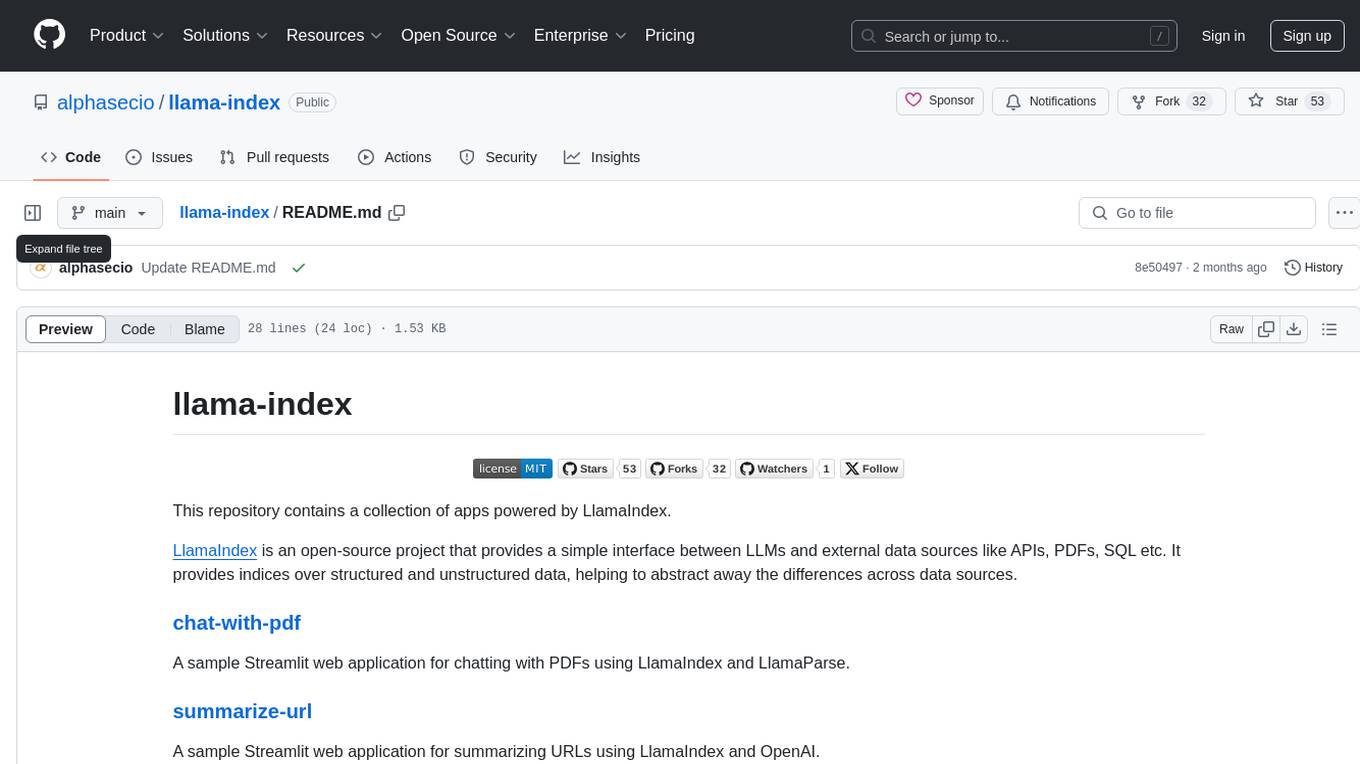
llama-index
This repository, llama-index, contains a collection of apps powered by LlamaIndex. LlamaIndex is an open-source project that provides a simple interface between LLMs and external data sources like APIs, PDFs, SQL etc. It provides indices over structured and unstructured data, helping to abstract away the differences across data sources. The repository includes apps like chat-with-pdf and summarize-url, showcasing the capabilities of LlamaIndex in interacting with PDFs and summarizing URLs.
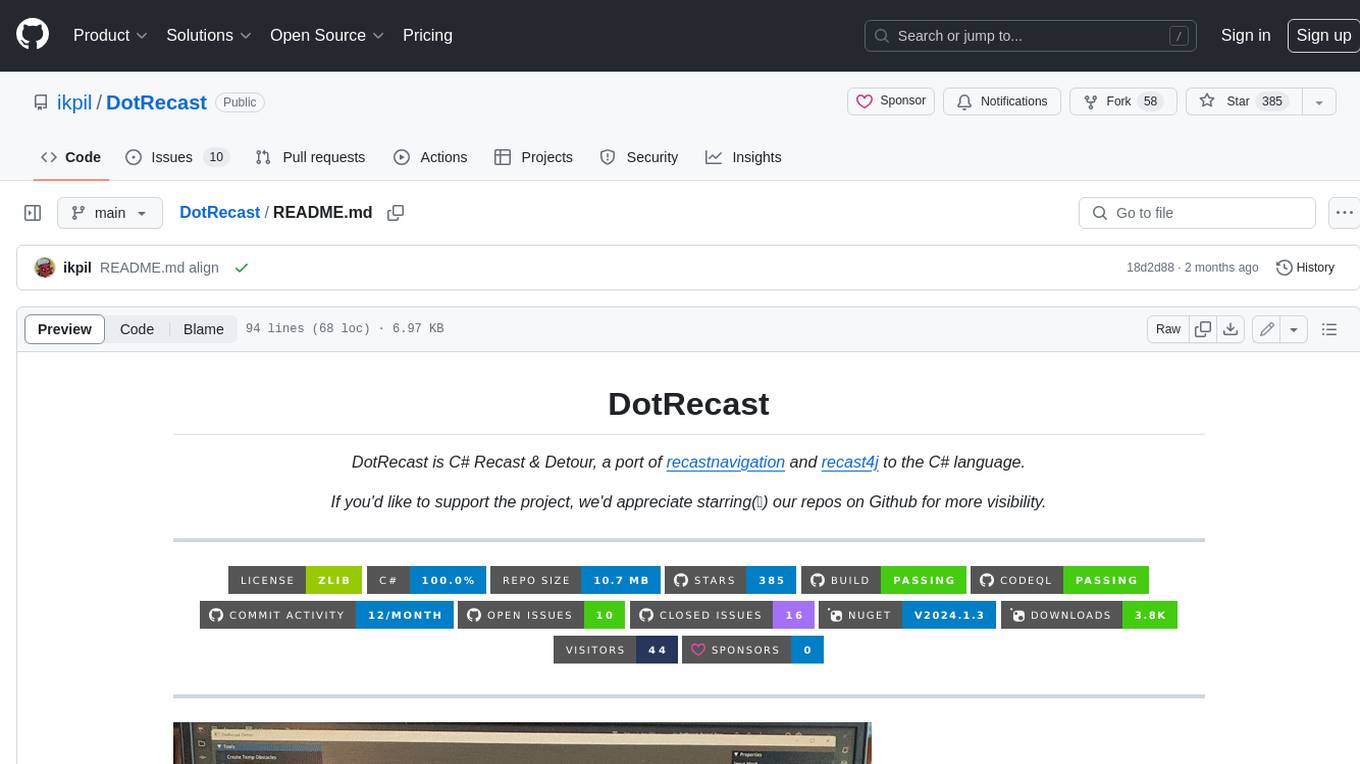
DotRecast
DotRecast is a C# port of Recast & Detour, a navigation library used in many AAA and indie games and engines. It provides automatic navmesh generation, fast turnaround times, detailed customization options, and is dependency-free. Recast Navigation is divided into multiple modules, each contained in its own folder: - DotRecast.Core: Core utils - DotRecast.Recast: Navmesh generation - DotRecast.Detour: Runtime loading of navmesh data, pathfinding, navmesh queries - DotRecast.Detour.TileCache: Navmesh streaming. Useful for large levels and open-world games - DotRecast.Detour.Crowd: Agent movement, collision avoidance, and crowd simulation - DotRecast.Detour.Dynamic: Robust support for dynamic nav meshes combining pre-built voxels with dynamic objects which can be freely added and removed - DotRecast.Detour.Extras: Simple tool to import navmeshes created with A* Pathfinding Project - DotRecast.Recast.Toolset: All modules - DotRecast.Recast.Demo: Standalone, comprehensive demo app showcasing all aspects of Recast & Detour's functionality - Tests: Unit tests Recast constructs a navmesh through a multi-step mesh rasterization process: 1. First Recast rasterizes the input triangle meshes into voxels. 2. Voxels in areas where agents would not be able to move are filtered and removed. 3. The walkable areas described by the voxel grid are then divided into sets of polygonal regions. 4. The navigation polygons are generated by re-triangulating the generated polygonal regions into a navmesh. You can use Recast to build a single navmesh, or a tiled navmesh. Single meshes are suitable for many simple, static cases and are easy to work with. Tiled navmeshes are more complex to work with but better support larger, more dynamic environments. Tiled meshes enable advanced Detour features like re-baking, hierarchical path-planning, and navmesh data-streaming.
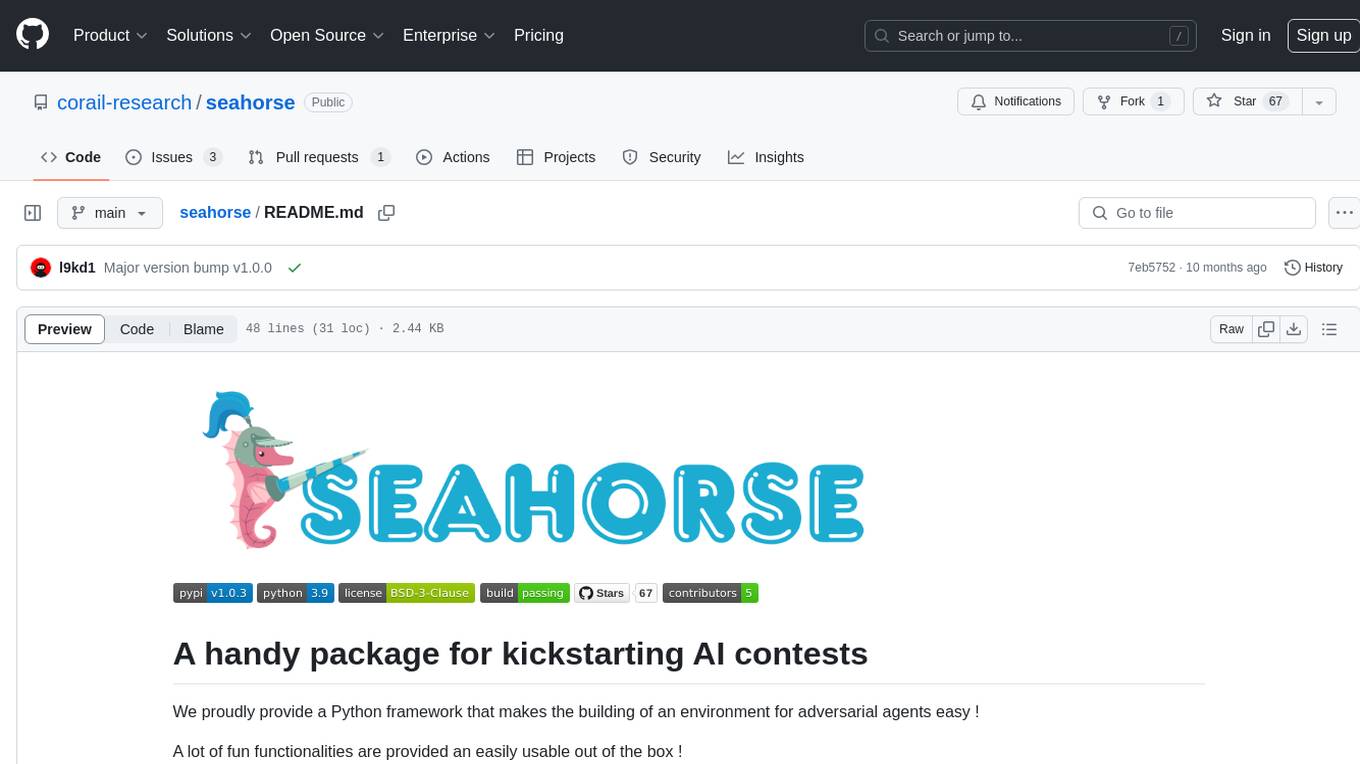
seahorse
A handy package for kickstarting AI contests. This Python framework simplifies the creation of an environment for adversarial agents, offering various functionalities for game setup, playing against remote agents, data generation, and contest organization. The package is user-friendly and provides easy-to-use features out of the box. Developed by an enthusiastic team of M.Sc candidates at Polytechnique Montréal, 'seahorse' is distributed under the 3-Clause BSD License.
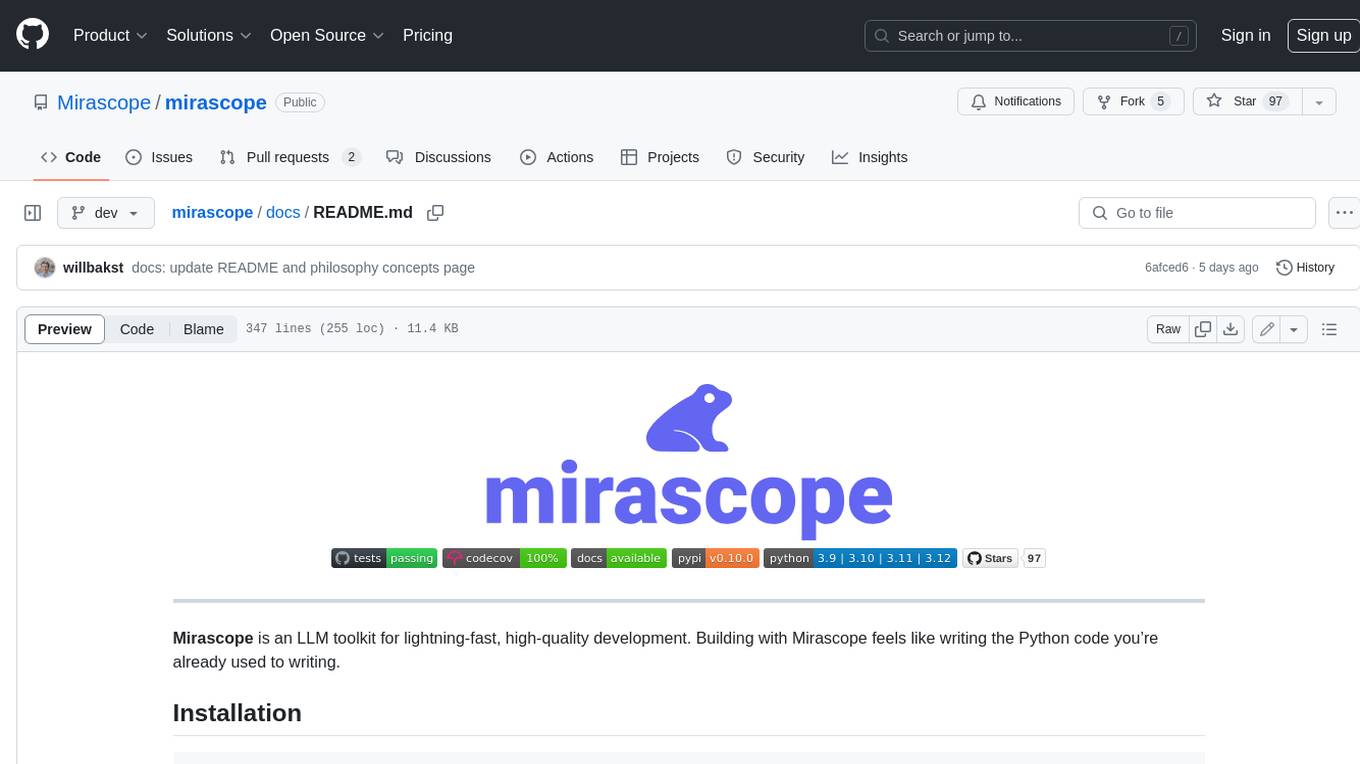
mirascope
Mirascope is an LLM toolkit for lightning-fast, high-quality development. Building with Mirascope feels like writing the Python code you’re already used to writing.
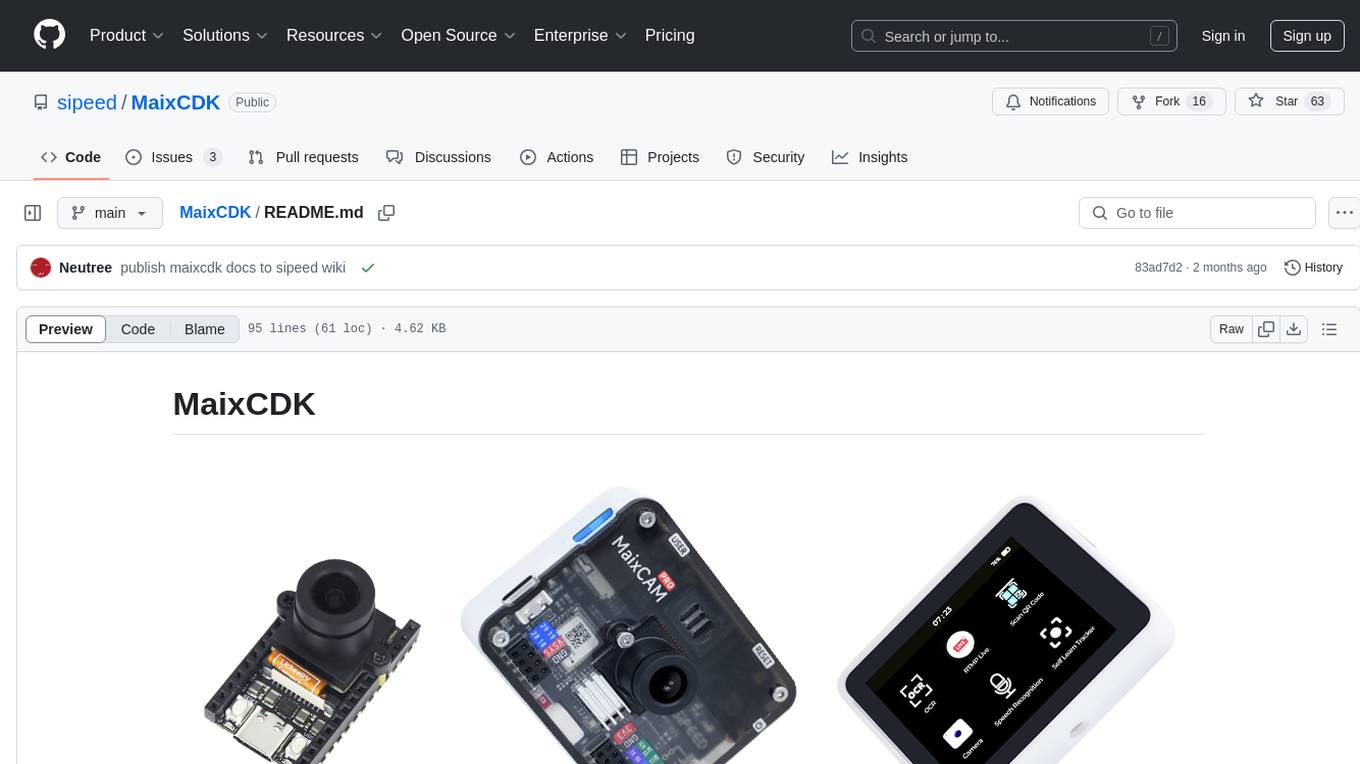
MaixCDK
MaixCDK (Maix C/CPP Development Kit) is a C/C++ development kit that integrates practical functions such as AI, machine vision, and IoT. It provides easy-to-use encapsulation for quickly building projects in vision, artificial intelligence, IoT, robotics, industrial cameras, and more. It supports hardware-accelerated execution of AI models, common vision algorithms, OpenCV, and interfaces for peripheral operations. MaixCDK offers cross-platform support, easy-to-use API, simple environment setup, online debugging, and a complete ecosystem including MaixPy and MaixVision. Supported devices include Sipeed MaixCAM, Sipeed MaixCAM-Pro, and partial support for Common Linux.
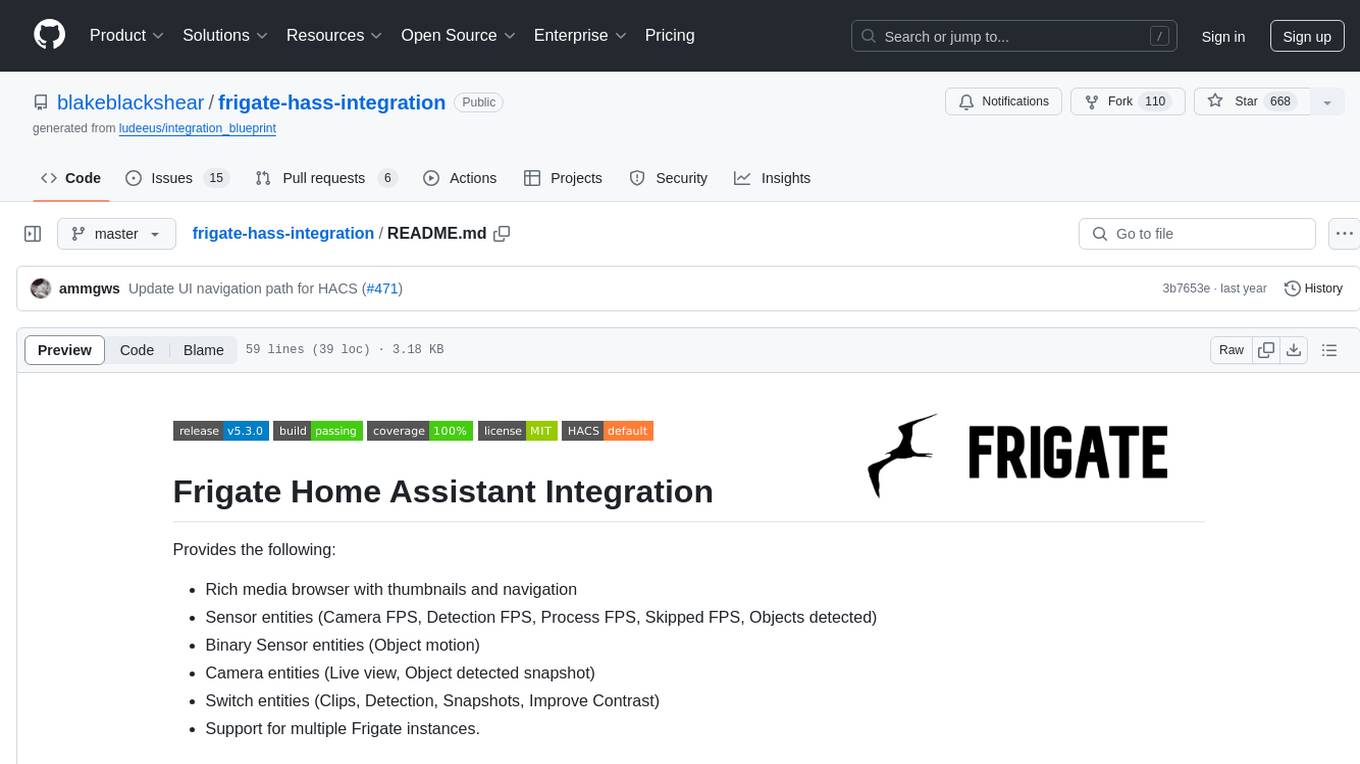
frigate-hass-integration
Frigate Home Assistant Integration provides a rich media browser with thumbnails and navigation, sensor entities for camera FPS, detection FPS, process FPS, skipped FPS, and objects detected, binary sensor entities for object motion, camera entities for live view and object detected snapshot, switch entities for clips, detection, snapshots, and improve contrast, and support for multiple Frigate instances. It offers easy installation via HACS and manual installation options for advanced users. Users need to configure the `mqtt` integration for Frigate to work. Additionally, media browsing and a companion Lovelace card are available for enhanced user experience. Refer to the main Frigate documentation for detailed installation instructions and usage guidance.
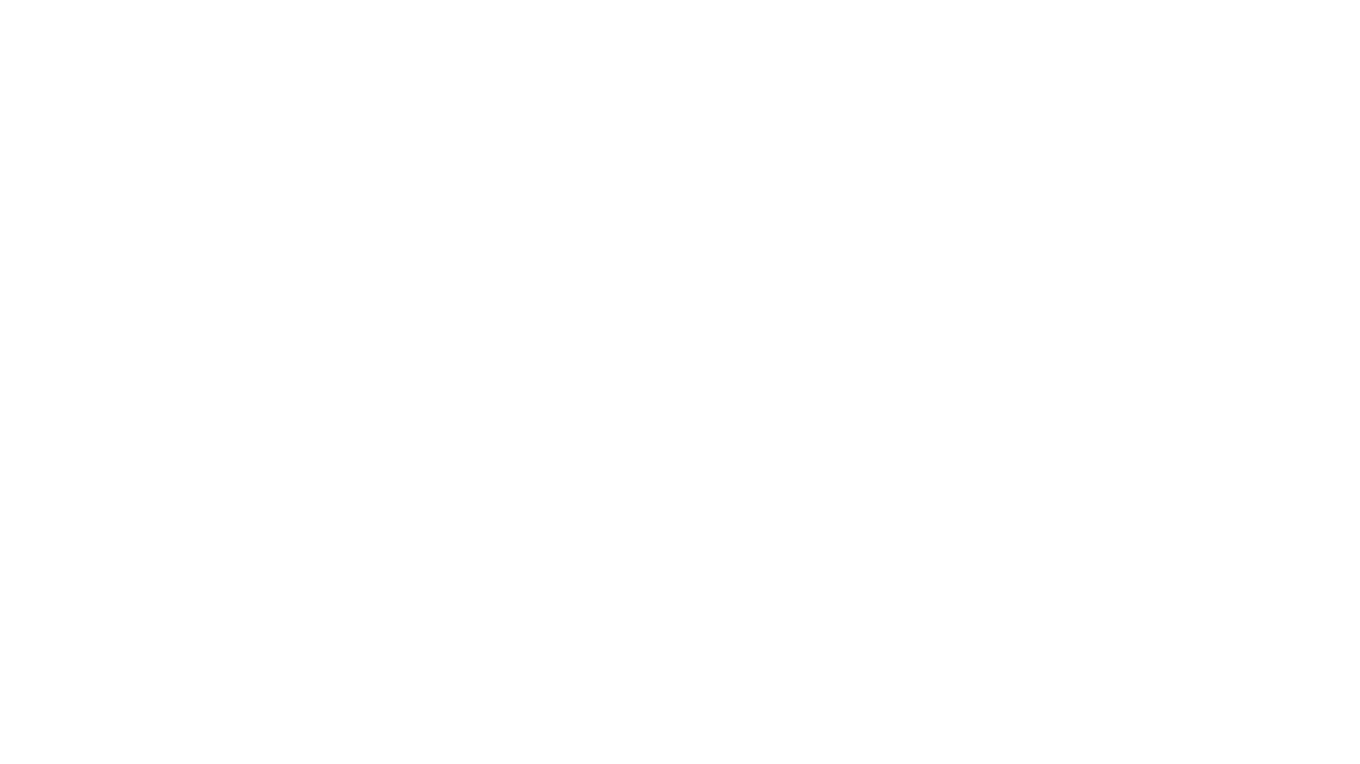
TestSpark
TestSpark is a plugin for generating unit tests that integrates AI-based test generation tools. It supports LLM-based test generation using OpenAI, HuggingFace, and JetBrains internal AI Assistant platform, as well as local search-based test generation using EvoSuite. Users can configure test generation settings, interact with test cases, view coverage statistics, and integrate tests into projects. The plugin is designed for experimental use to augment existing test suites, not replace manual test writing.
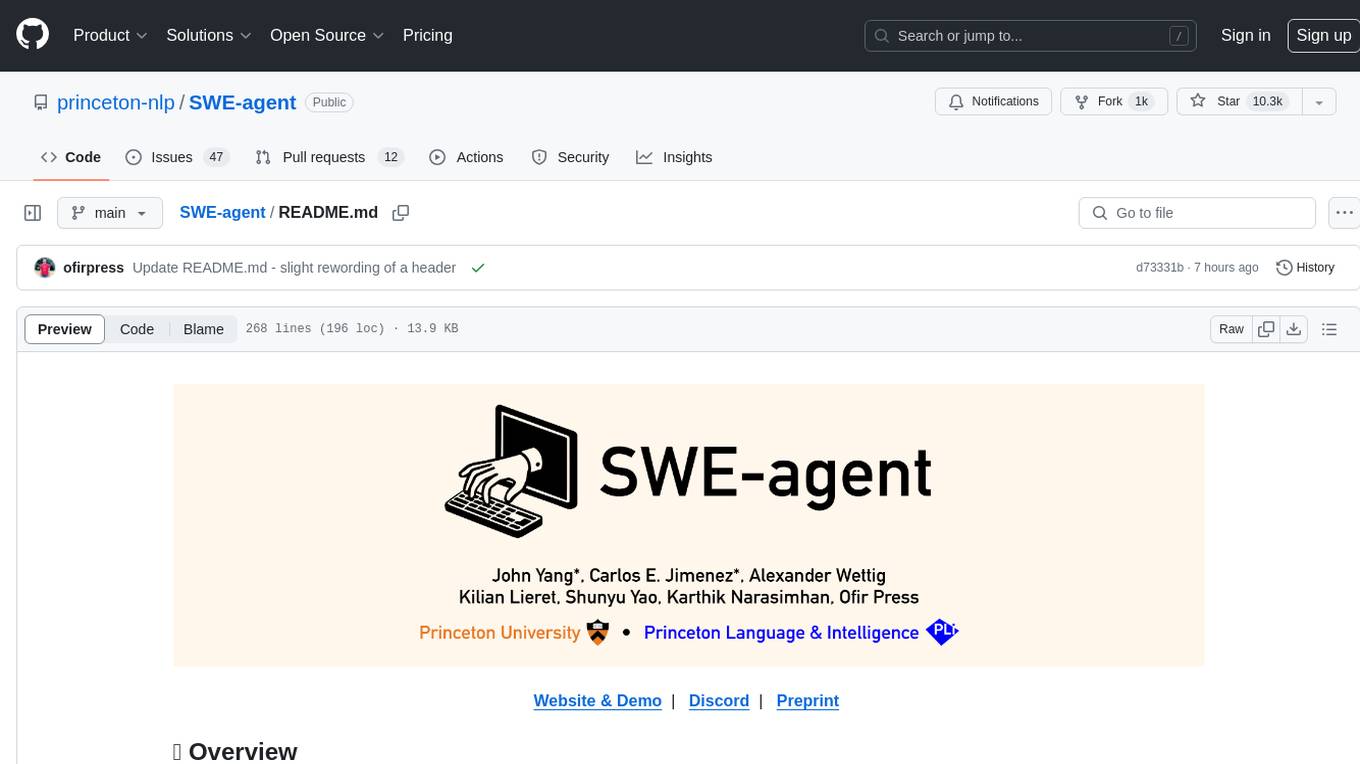
SWE-agent
SWE-agent is a tool that turns language models (e.g. GPT-4) into software engineering agents capable of fixing bugs and issues in real GitHub repositories. It achieves state-of-the-art performance on the full test set by resolving 12.29% of issues. The tool is built and maintained by researchers from Princeton University. SWE-agent provides a command line tool and a graphical web interface for developers to interact with. It introduces an Agent-Computer Interface (ACI) to facilitate browsing, viewing, editing, and executing code files within repositories. The tool includes features such as a linter for syntax checking, a specialized file viewer, and a full-directory string searching command to enhance the agent's capabilities. SWE-agent aims to improve prompt engineering and ACI design to enhance the performance of language models in software engineering tasks.
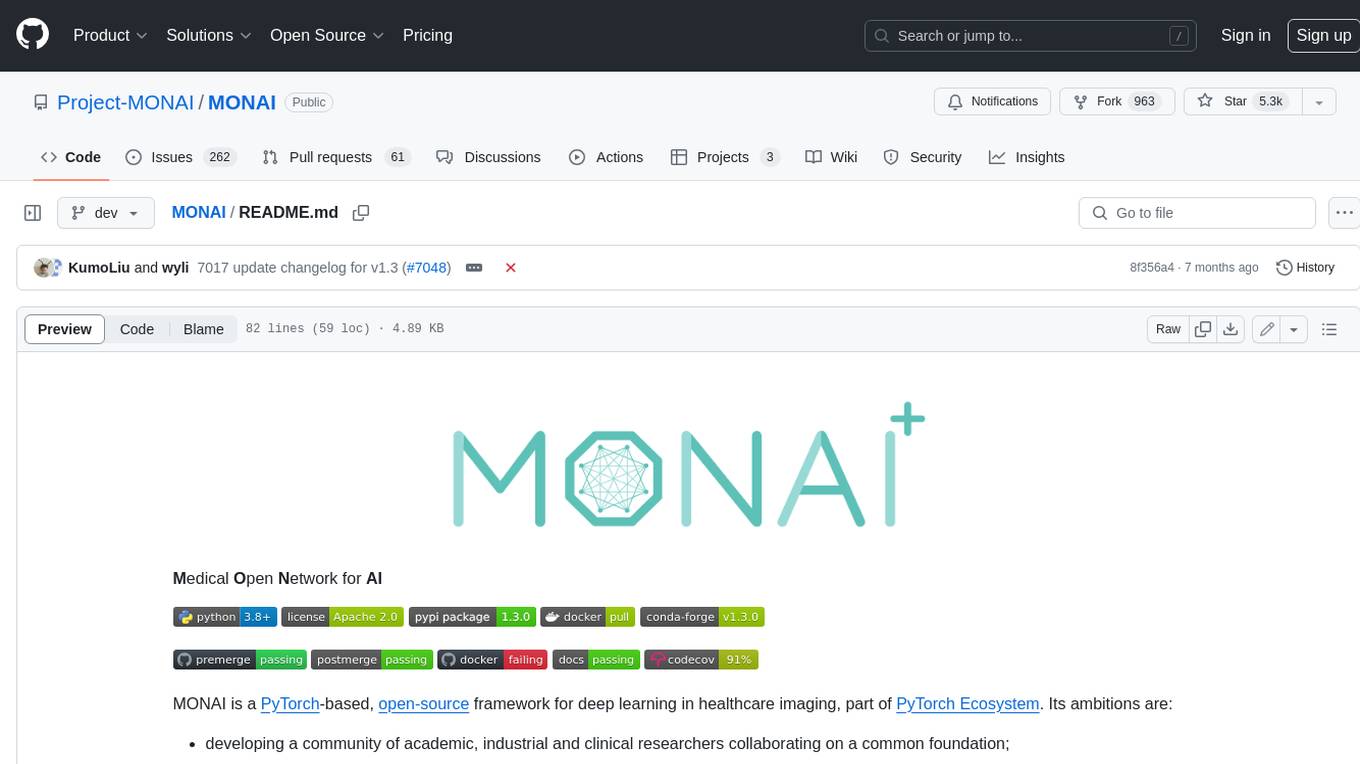
MONAI
MONAI is a PyTorch-based, open-source framework for deep learning in healthcare imaging. It provides a comprehensive set of tools for medical image analysis, including data preprocessing, model training, and evaluation. MONAI is designed to be flexible and easy to use, making it a valuable resource for researchers and developers in the field of medical imaging.
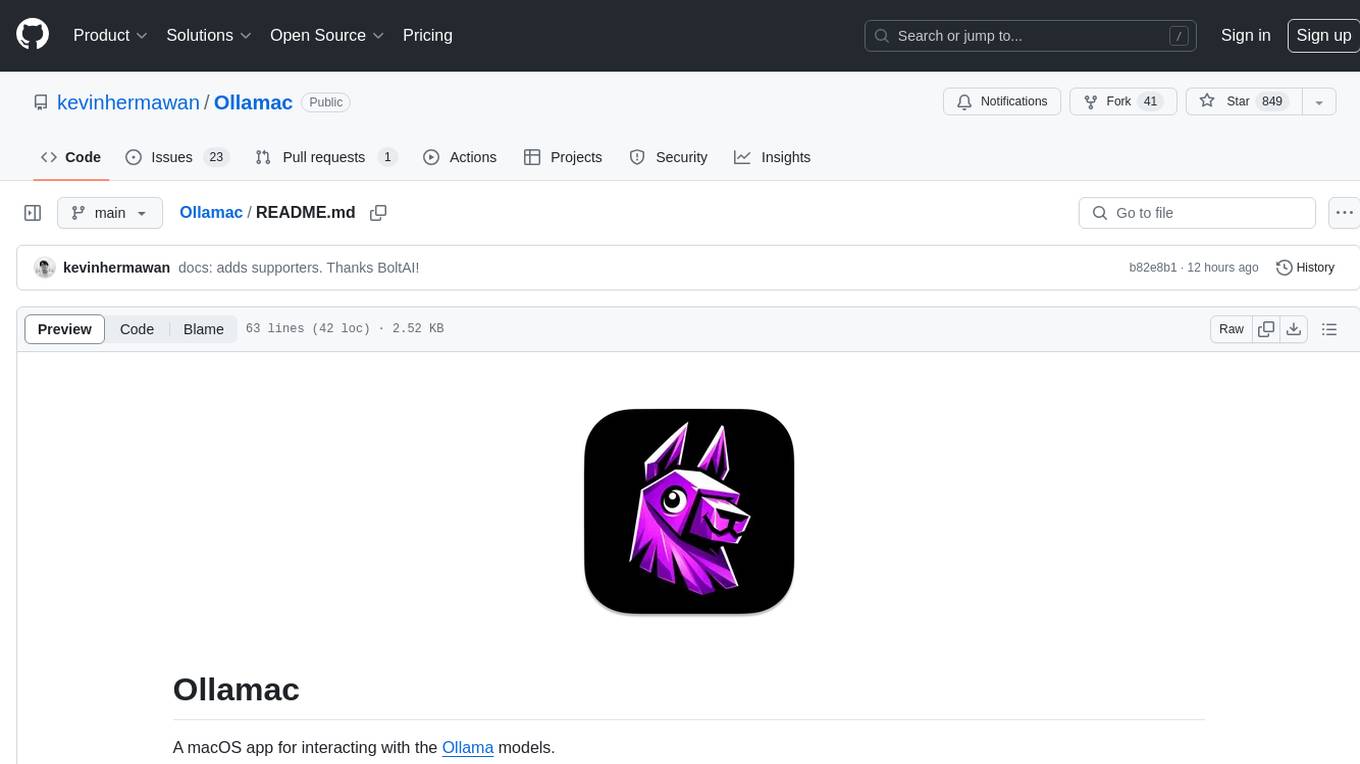
Ollamac
Ollamac is a macOS app designed for interacting with Ollama models. It is optimized for macOS, allowing users to easily use any model from the Ollama library. The app features a user-friendly interface, chat archive for saving interactions, and real-time communication using HTTP streaming technology. Ollamac is open-source, enabling users to contribute to its development and enhance its capabilities. It requires macOS 14 or later and the Ollama system to be installed on the user's Mac with at least one Ollama model downloaded.
For similar tasks
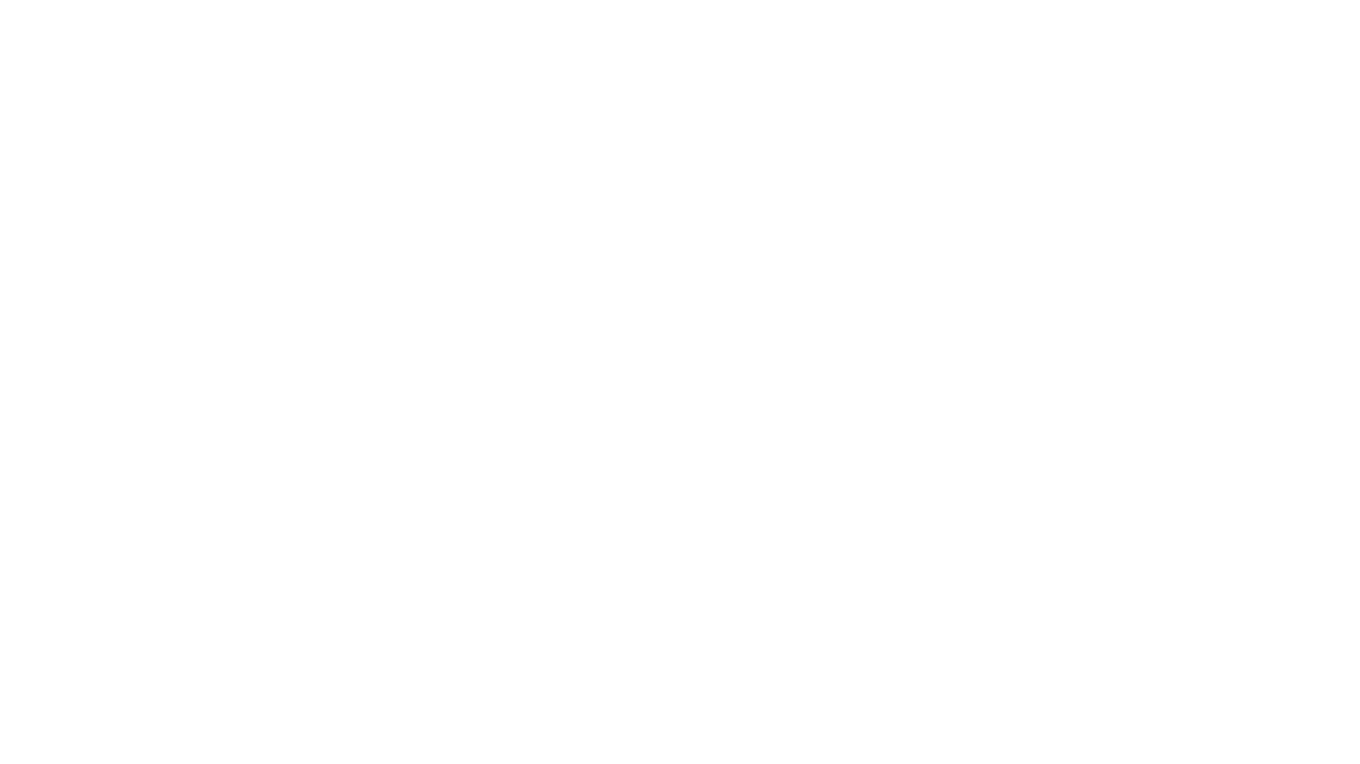
HPT
Hyper-Pretrained Transformers (HPT) is a novel multimodal LLM framework from HyperGAI, trained for vision-language models capable of understanding both textual and visual inputs. The repository contains the open-source implementation of inference code to reproduce the evaluation results of HPT Air on different benchmarks. HPT has achieved competitive results with state-of-the-art models on various multimodal LLM benchmarks. It offers models like HPT 1.5 Air and HPT 1.0 Air, providing efficient solutions for vision-and-language tasks.
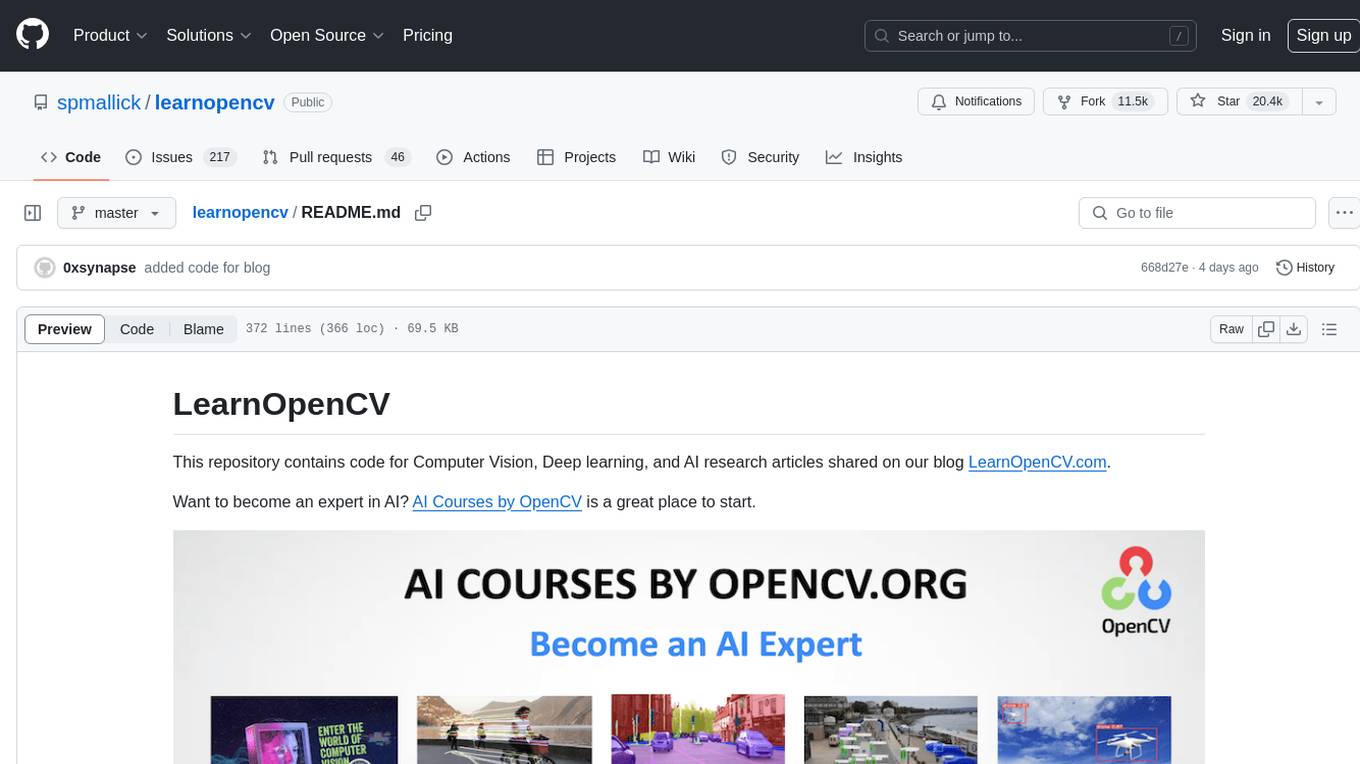
learnopencv
LearnOpenCV is a repository containing code for Computer Vision, Deep learning, and AI research articles shared on the blog LearnOpenCV.com. It serves as a resource for individuals looking to enhance their expertise in AI through various courses offered by OpenCV. The repository includes a wide range of topics such as image inpainting, instance segmentation, robotics, deep learning models, and more, providing practical implementations and code examples for readers to explore and learn from.
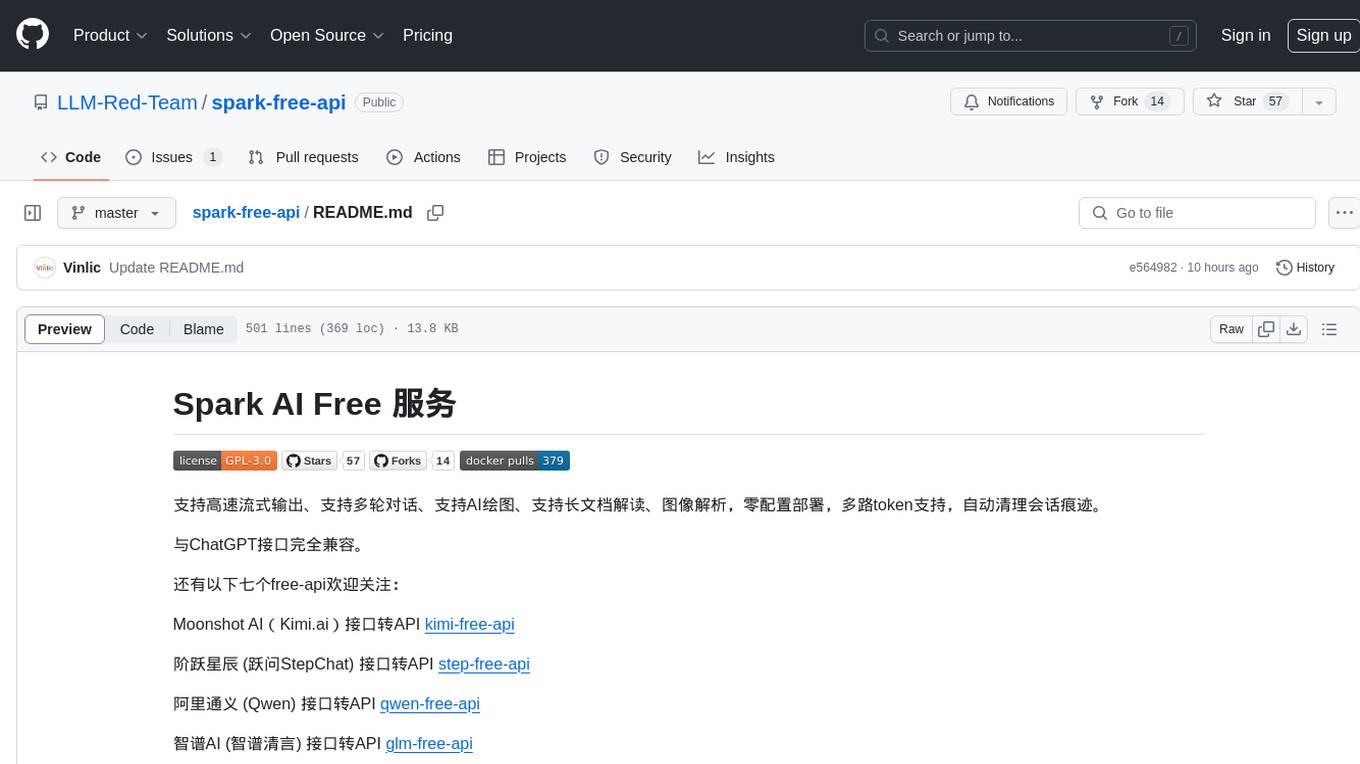
spark-free-api
Spark AI Free 服务 provides high-speed streaming output, multi-turn dialogue support, AI drawing support, long document interpretation, and image parsing. It offers zero-configuration deployment, multi-token support, and automatic session trace cleaning. It is fully compatible with the ChatGPT interface. The repository includes multiple free-api projects for various AI services. Users can access the API for tasks such as chat completions, AI drawing, document interpretation, image analysis, and ssoSessionId live checking. The project also provides guidelines for deployment using Docker, Docker-compose, Render, Vercel, and native deployment methods. It recommends using custom clients for faster and simpler access to the free-api series projects.
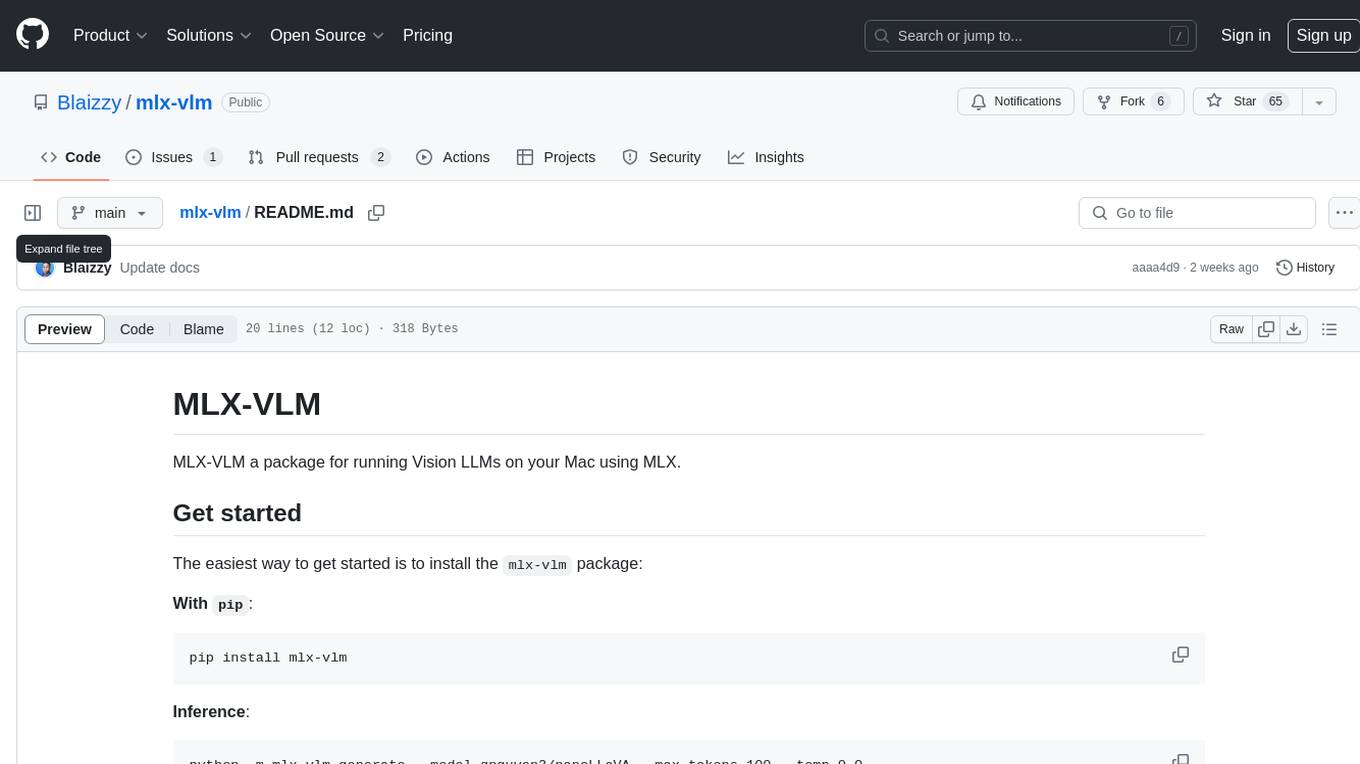
mlx-vlm
MLX-VLM is a package designed for running Vision LLMs on Mac systems using MLX. It provides a convenient way to install and utilize the package for processing large language models related to vision tasks. The tool simplifies the process of running LLMs on Mac computers, offering a seamless experience for users interested in leveraging MLX for vision-related projects.
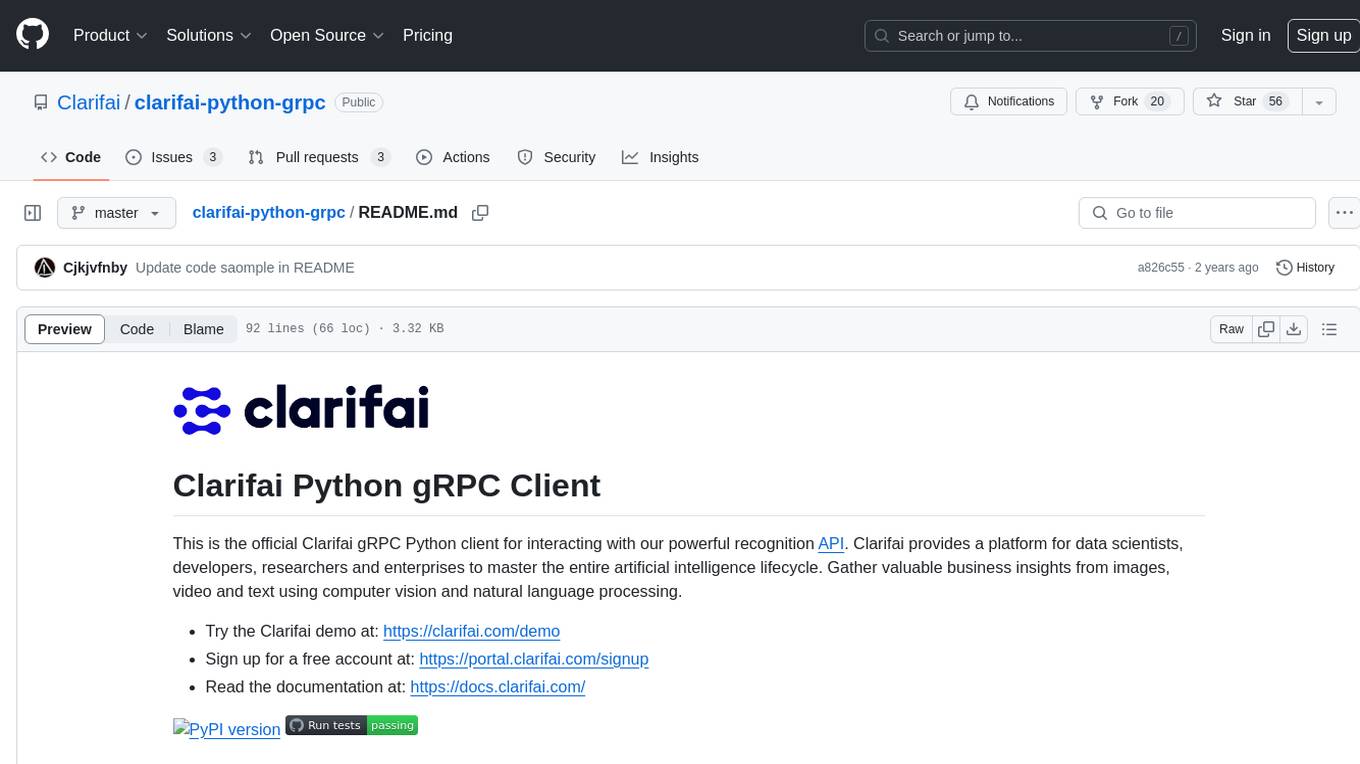
clarifai-python-grpc
This is the official Clarifai gRPC Python client for interacting with their recognition API. Clarifai offers a platform for data scientists, developers, researchers, and enterprises to utilize artificial intelligence for image, video, and text analysis through computer vision and natural language processing. The client allows users to authenticate, predict concepts in images, and access various functionalities provided by the Clarifai API. It follows a versioning scheme that aligns with the backend API updates and includes specific instructions for installation and troubleshooting. Users can explore the Clarifai demo, sign up for an account, and refer to the documentation for detailed information.
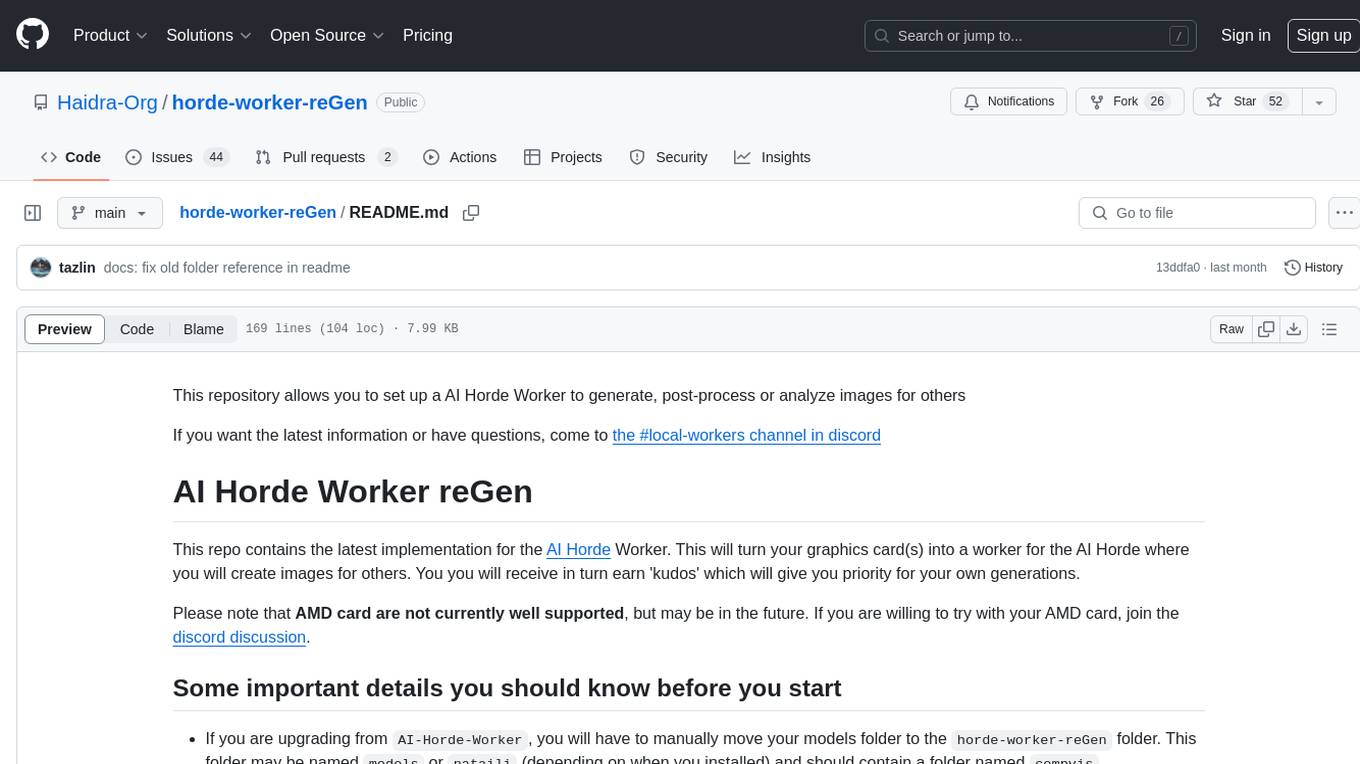
horde-worker-reGen
This repository provides the latest implementation for the AI Horde Worker, allowing users to utilize their graphics card(s) to generate, post-process, or analyze images for others. It offers a platform where users can create images and earn 'kudos' in return, granting priority for their own image generations. The repository includes important details for setup, recommendations for system configurations, instructions for installation on Windows and Linux, basic usage guidelines, and information on updating the AI Horde Worker. Users can also run the worker with multiple GPUs and receive notifications for updates through Discord. Additionally, the repository contains models that are licensed under the CreativeML OpenRAIL License.
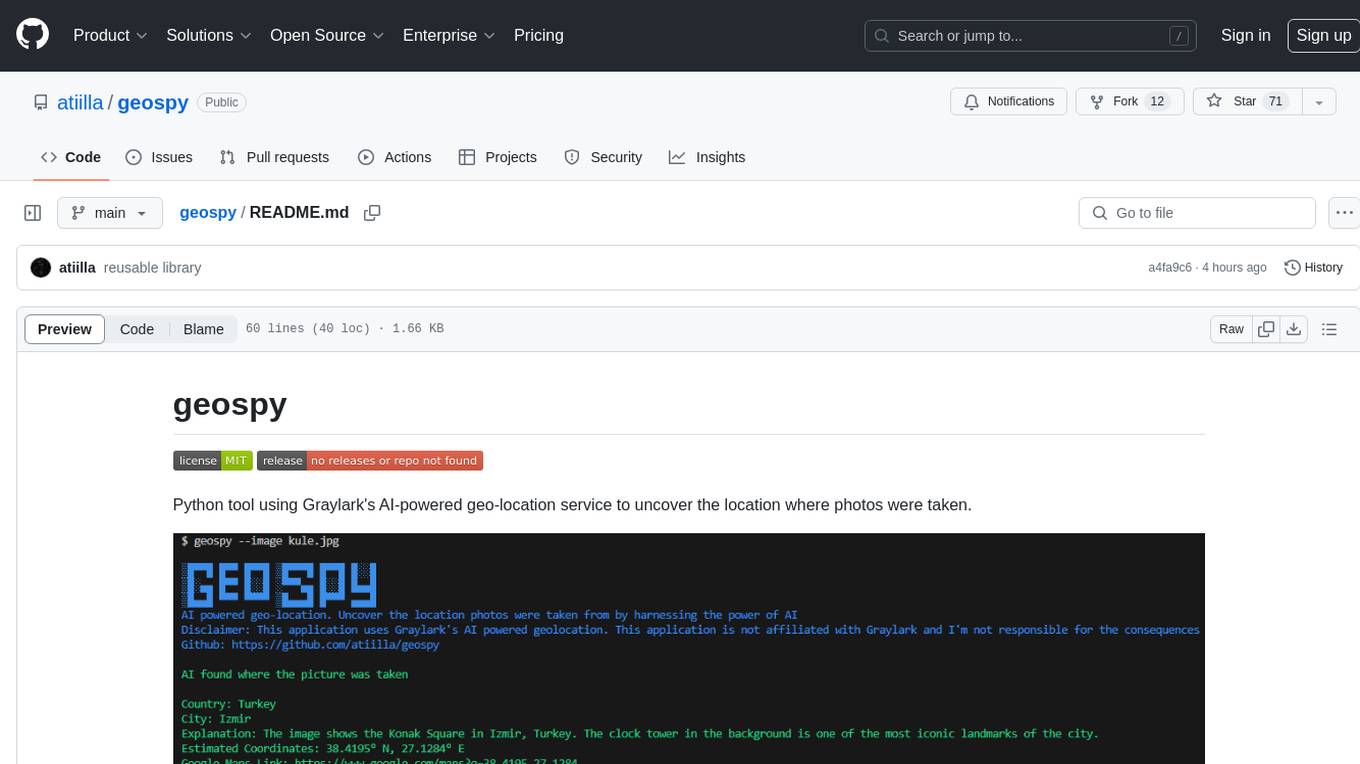
geospy
Geospy is a Python tool that utilizes Graylark's AI-powered geolocation service to determine the location where photos were taken. It allows users to analyze images and retrieve information such as country, city, explanation, coordinates, and Google Maps links. The tool provides a seamless way to integrate geolocation services into various projects and applications.
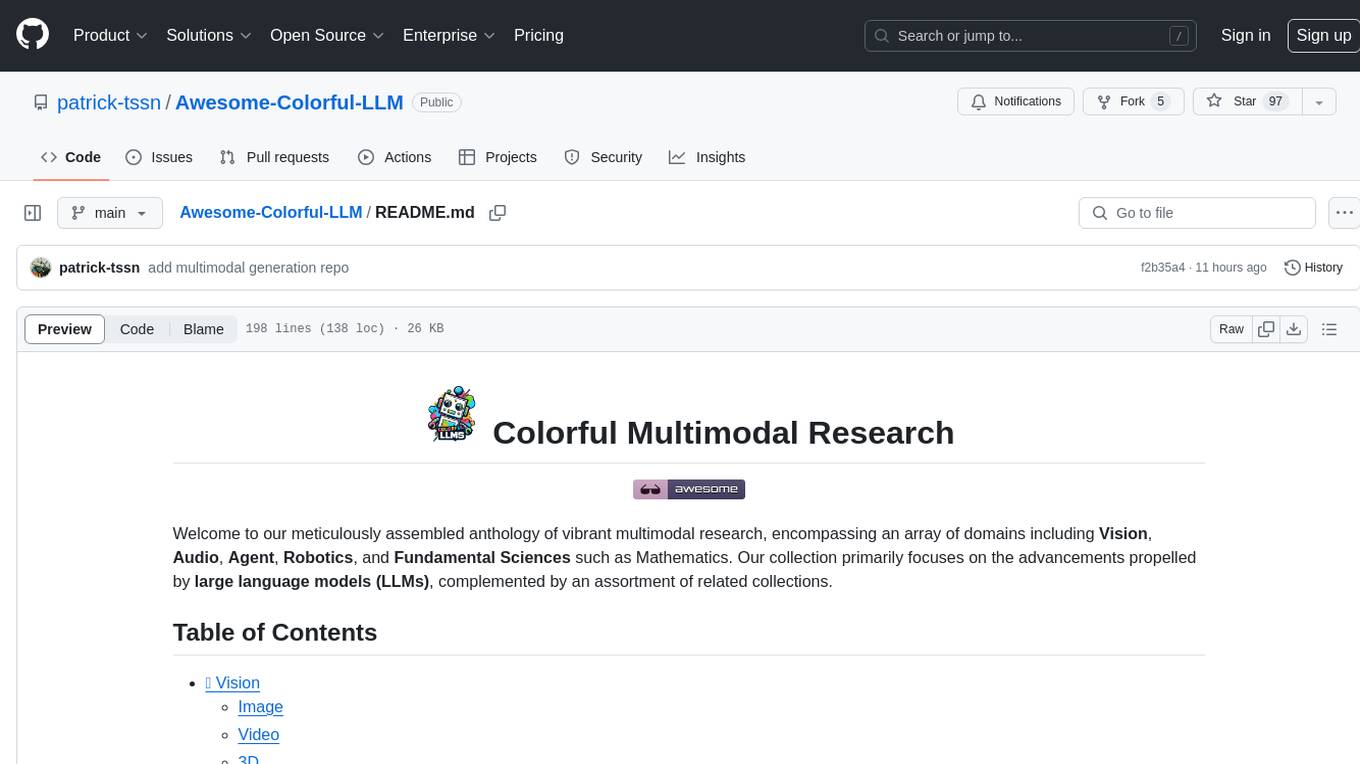
Awesome-Colorful-LLM
Awesome-Colorful-LLM is a meticulously assembled anthology of vibrant multimodal research focusing on advancements propelled by large language models (LLMs) in domains such as Vision, Audio, Agent, Robotics, and Fundamental Sciences like Mathematics. The repository contains curated collections of works, datasets, benchmarks, projects, and tools related to LLMs and multimodal learning. It serves as a comprehensive resource for researchers and practitioners interested in exploring the intersection of language models and various modalities for tasks like image understanding, video pretraining, 3D modeling, document understanding, audio analysis, agent learning, robotic applications, and mathematical research.
For similar jobs
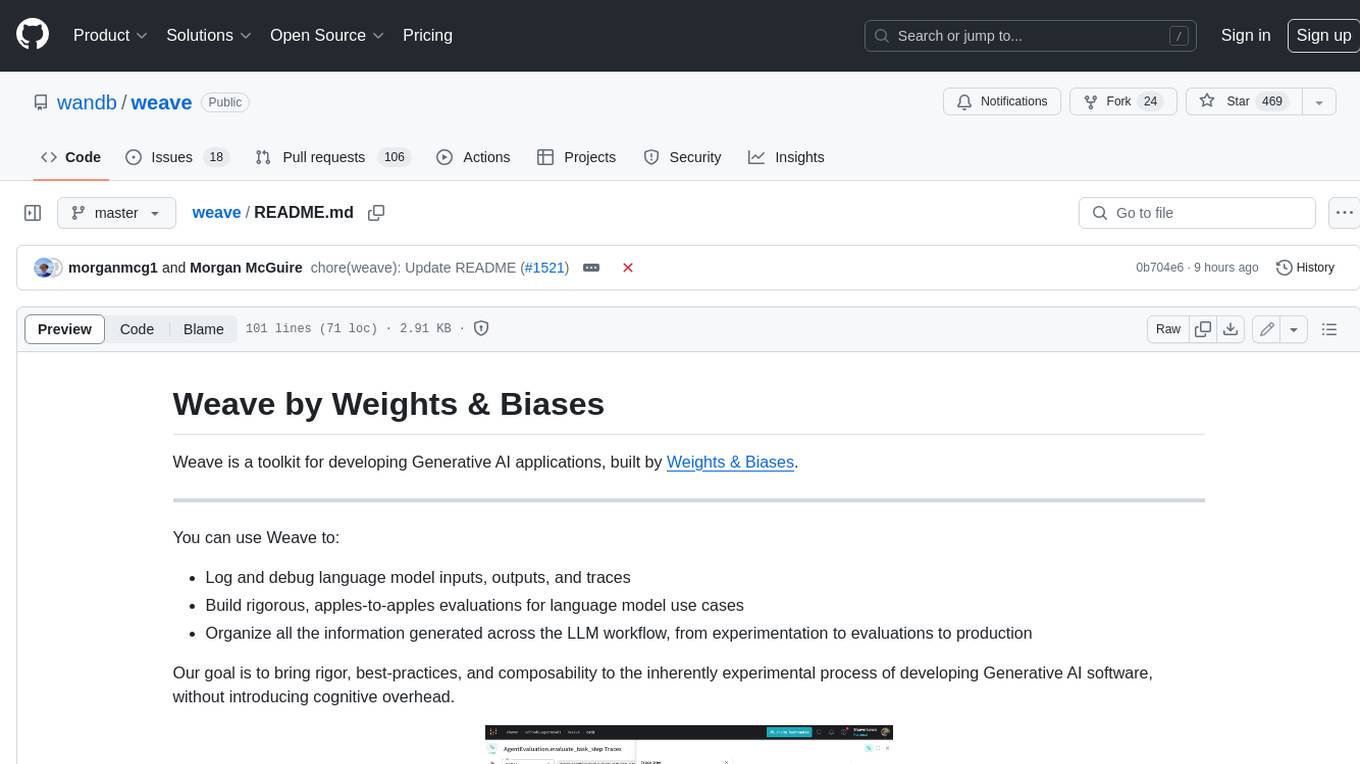
weave
Weave is a toolkit for developing Generative AI applications, built by Weights & Biases. With Weave, you can log and debug language model inputs, outputs, and traces; build rigorous, apples-to-apples evaluations for language model use cases; and organize all the information generated across the LLM workflow, from experimentation to evaluations to production. Weave aims to bring rigor, best-practices, and composability to the inherently experimental process of developing Generative AI software, without introducing cognitive overhead.
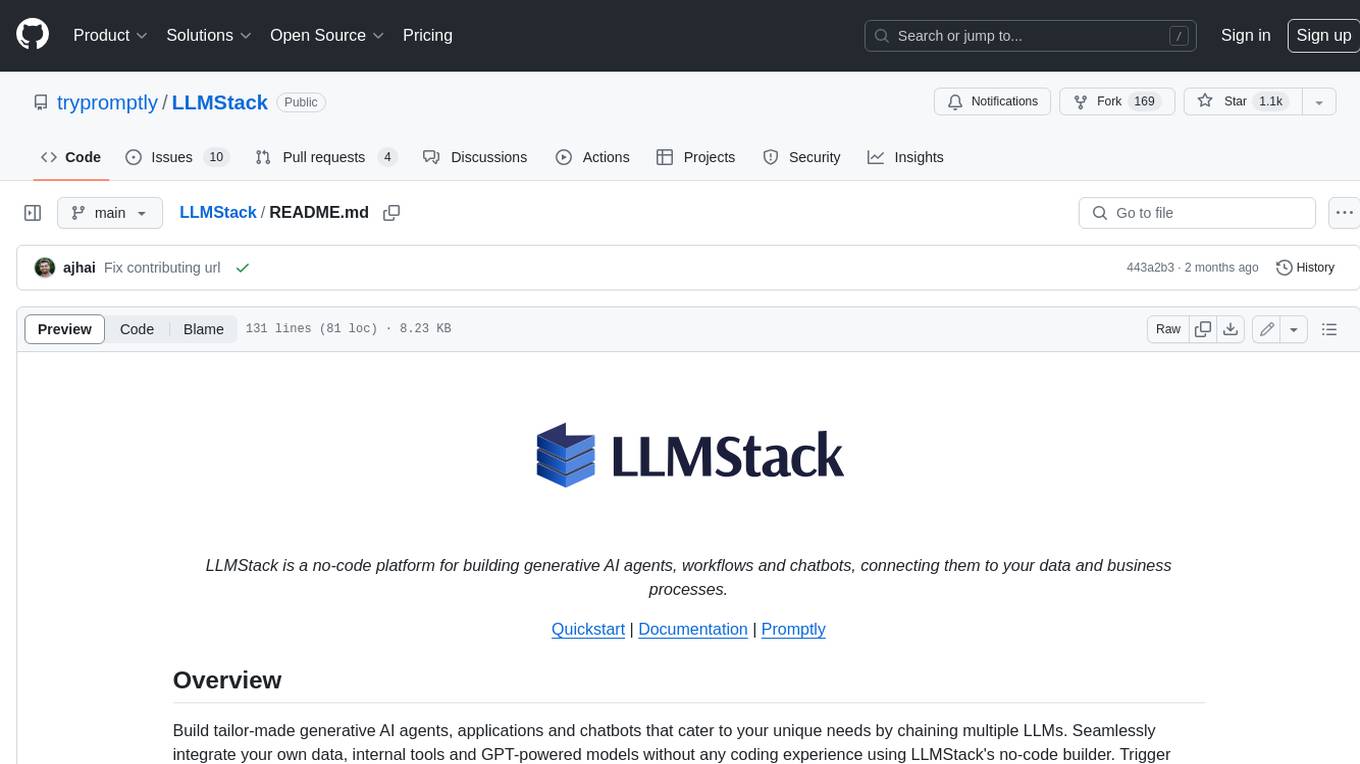
LLMStack
LLMStack is a no-code platform for building generative AI agents, workflows, and chatbots. It allows users to connect their own data, internal tools, and GPT-powered models without any coding experience. LLMStack can be deployed to the cloud or on-premise and can be accessed via HTTP API or triggered from Slack or Discord.
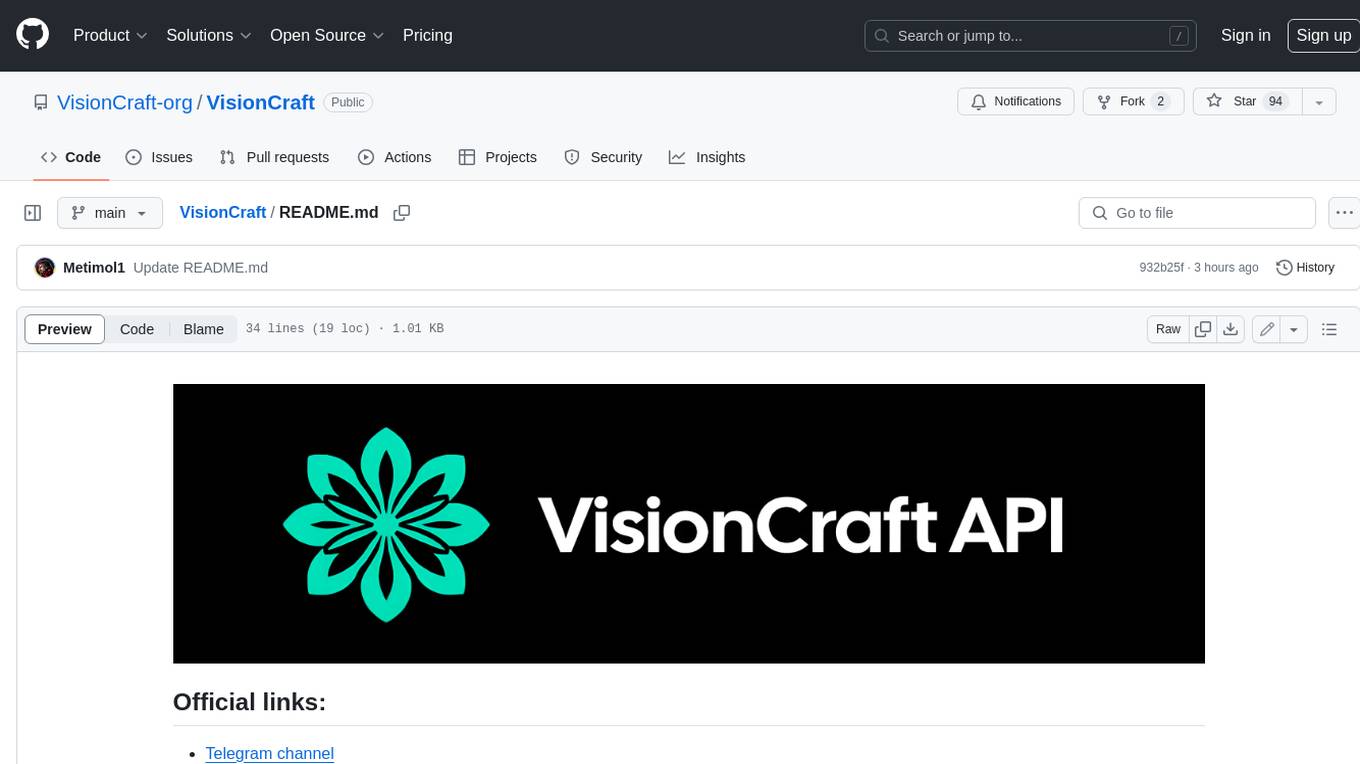
VisionCraft
The VisionCraft API is a free API for using over 100 different AI models. From images to sound.
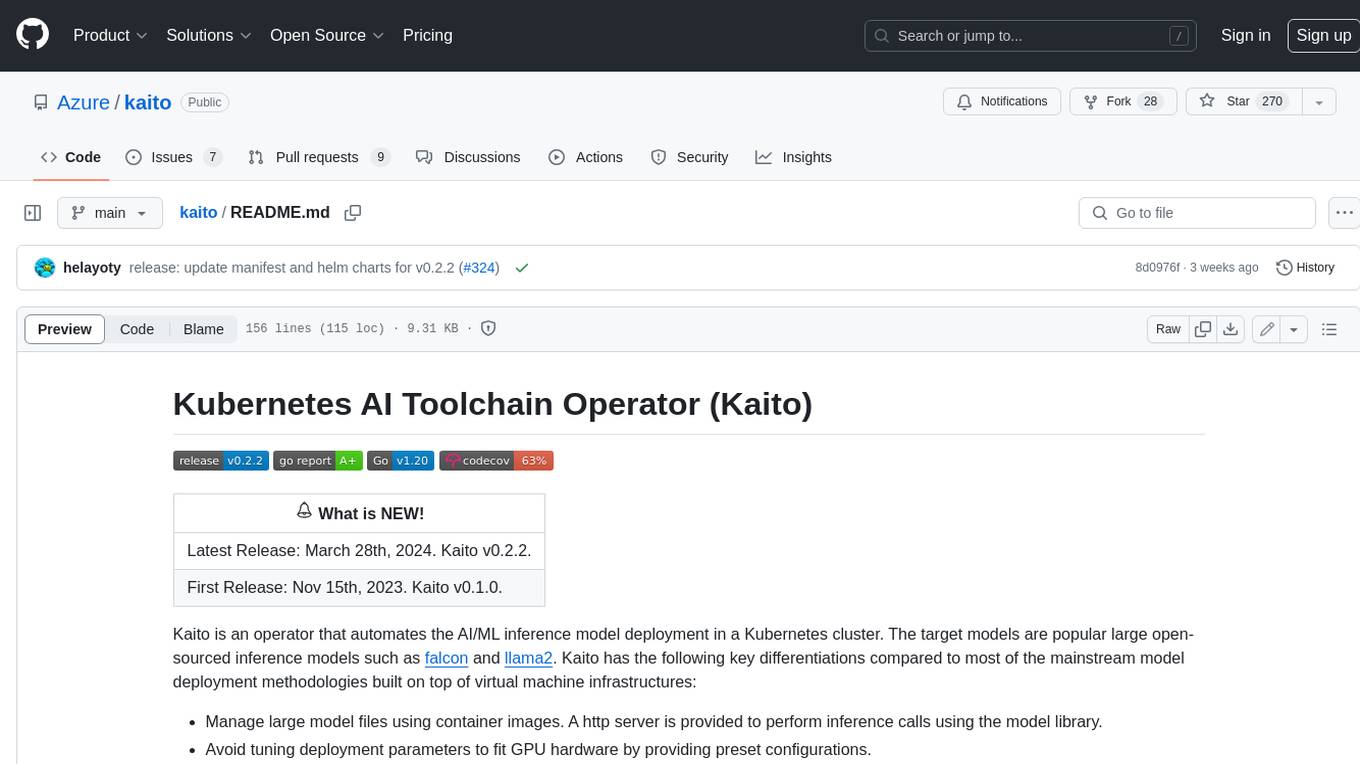
kaito
Kaito is an operator that automates the AI/ML inference model deployment in a Kubernetes cluster. It manages large model files using container images, avoids tuning deployment parameters to fit GPU hardware by providing preset configurations, auto-provisions GPU nodes based on model requirements, and hosts large model images in the public Microsoft Container Registry (MCR) if the license allows. Using Kaito, the workflow of onboarding large AI inference models in Kubernetes is largely simplified.
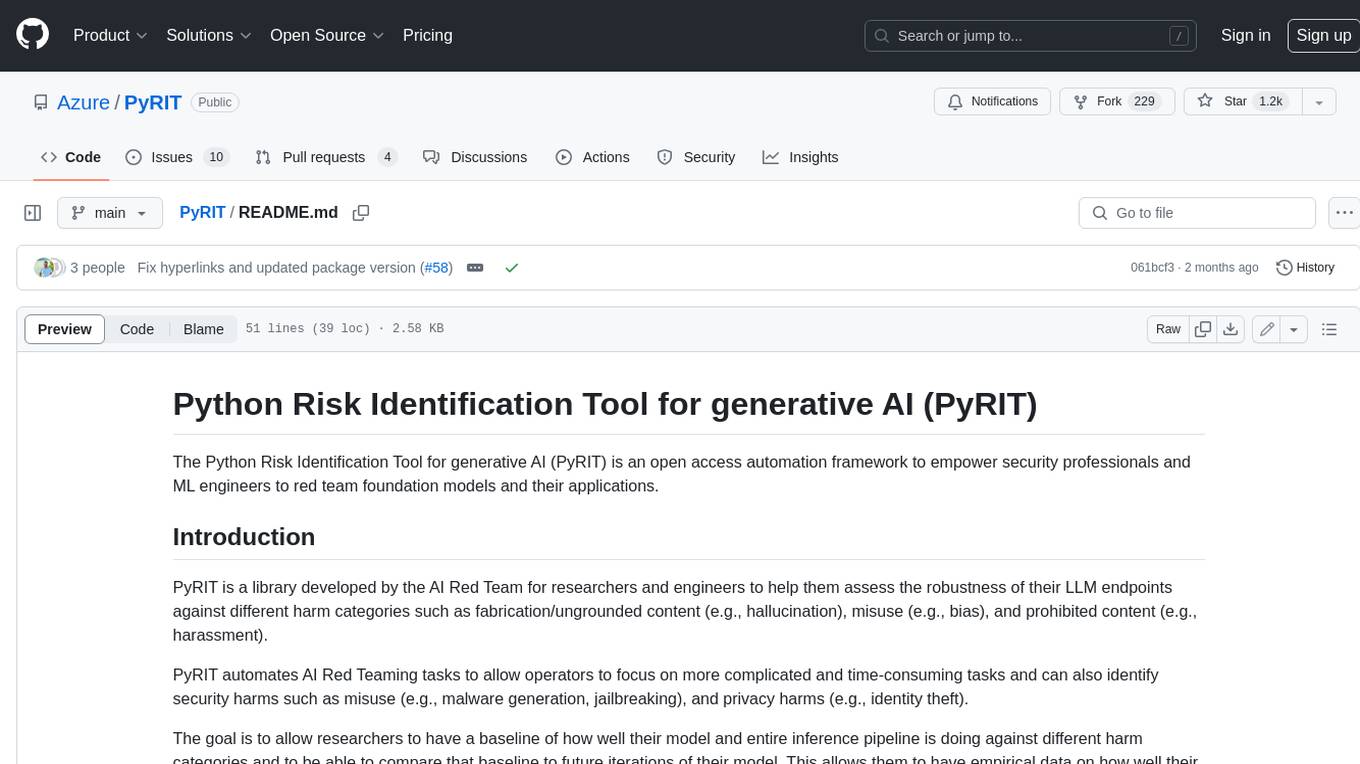
PyRIT
PyRIT is an open access automation framework designed to empower security professionals and ML engineers to red team foundation models and their applications. It automates AI Red Teaming tasks to allow operators to focus on more complicated and time-consuming tasks and can also identify security harms such as misuse (e.g., malware generation, jailbreaking), and privacy harms (e.g., identity theft). The goal is to allow researchers to have a baseline of how well their model and entire inference pipeline is doing against different harm categories and to be able to compare that baseline to future iterations of their model. This allows them to have empirical data on how well their model is doing today, and detect any degradation of performance based on future improvements.
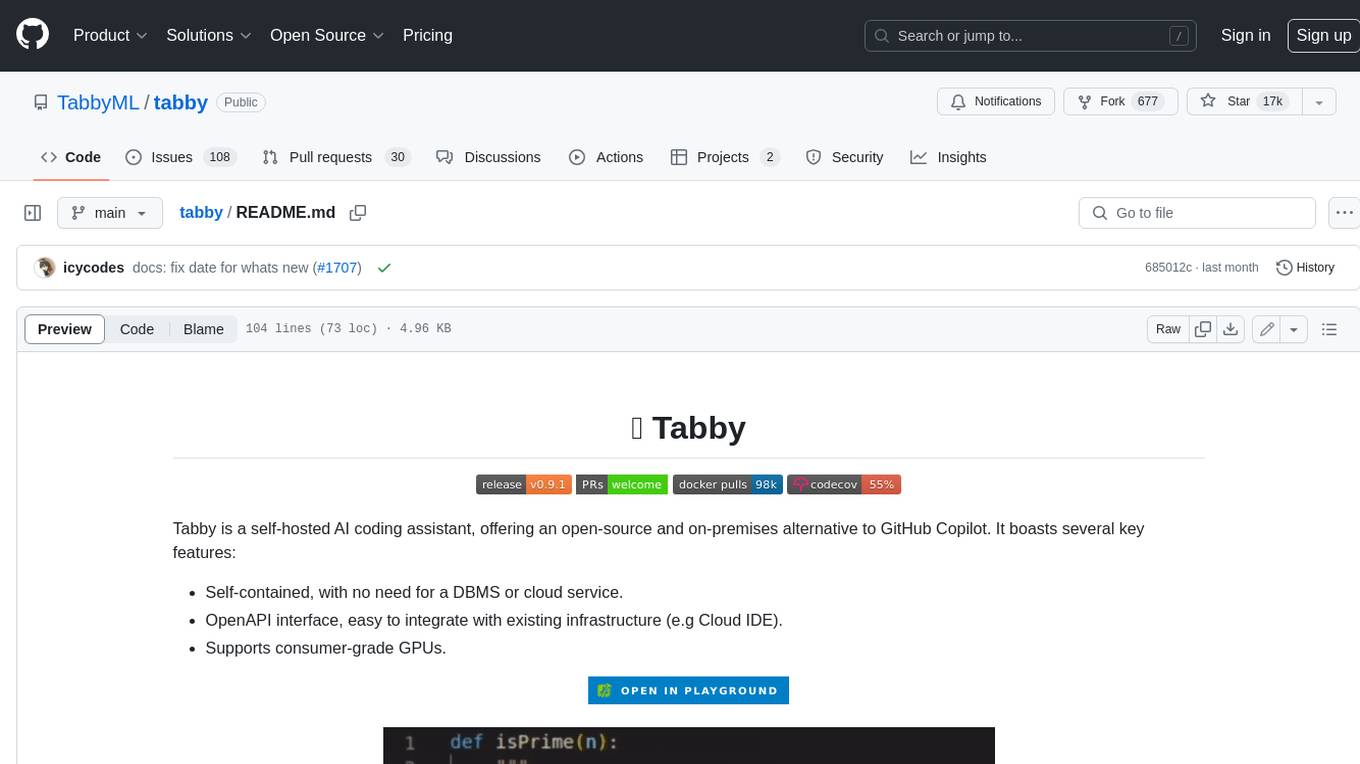
tabby
Tabby is a self-hosted AI coding assistant, offering an open-source and on-premises alternative to GitHub Copilot. It boasts several key features: * Self-contained, with no need for a DBMS or cloud service. * OpenAPI interface, easy to integrate with existing infrastructure (e.g Cloud IDE). * Supports consumer-grade GPUs.
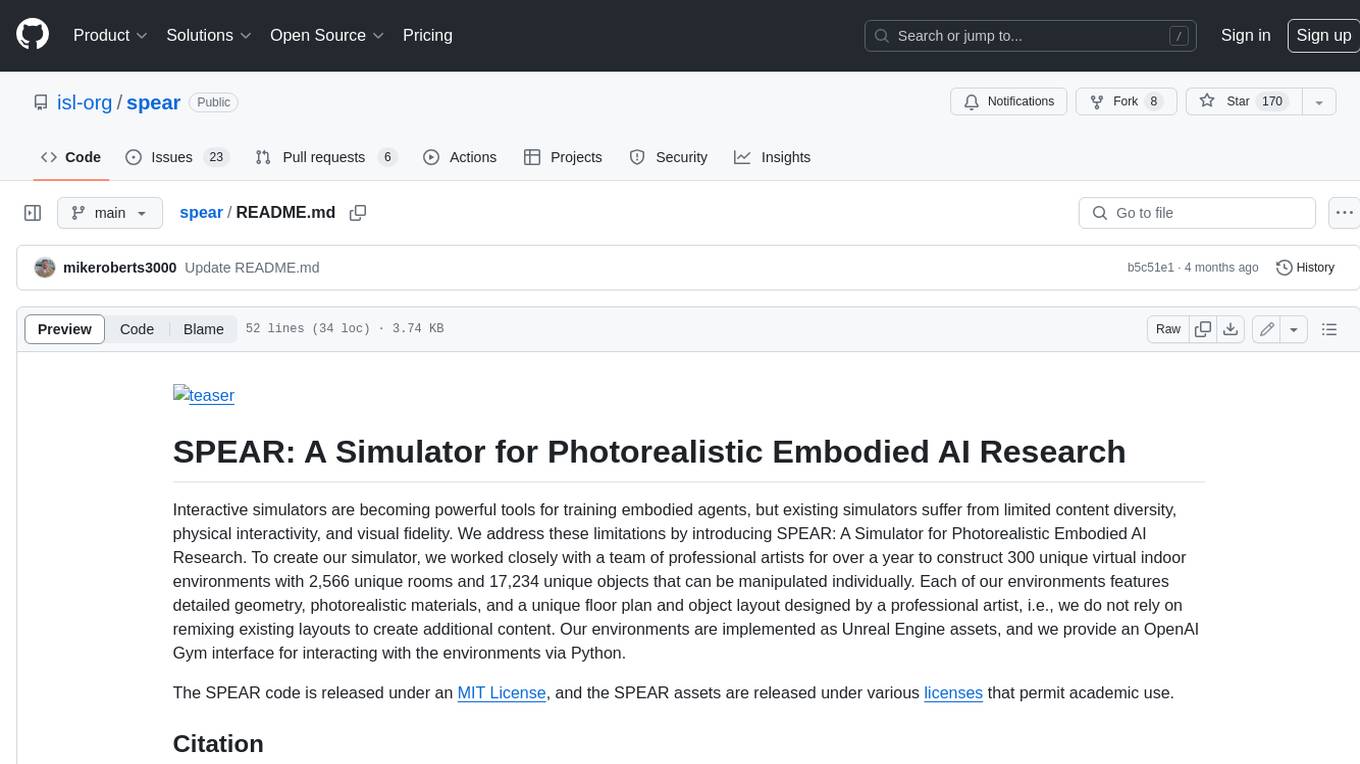
spear
SPEAR (Simulator for Photorealistic Embodied AI Research) is a powerful tool for training embodied agents. It features 300 unique virtual indoor environments with 2,566 unique rooms and 17,234 unique objects that can be manipulated individually. Each environment is designed by a professional artist and features detailed geometry, photorealistic materials, and a unique floor plan and object layout. SPEAR is implemented as Unreal Engine assets and provides an OpenAI Gym interface for interacting with the environments via Python.
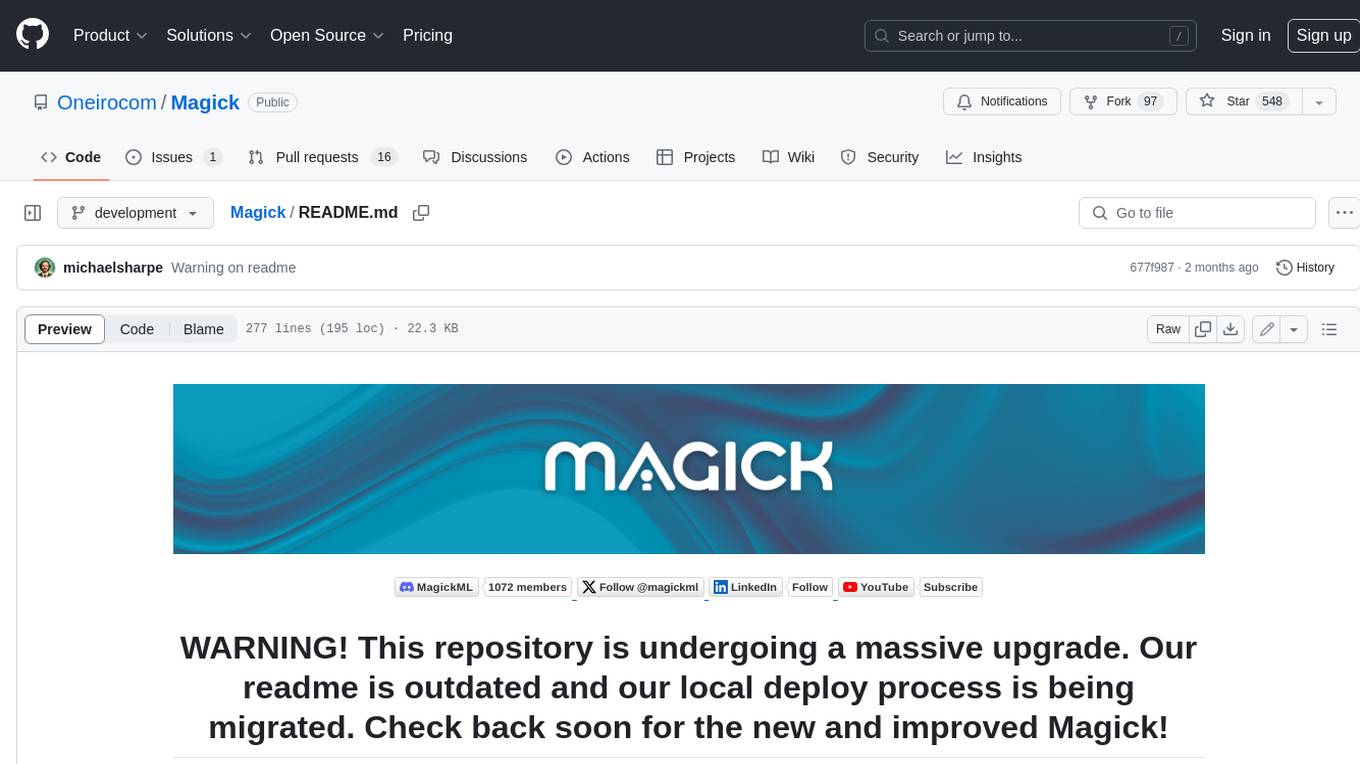
Magick
Magick is a groundbreaking visual AIDE (Artificial Intelligence Development Environment) for no-code data pipelines and multimodal agents. Magick can connect to other services and comes with nodes and templates well-suited for intelligent agents, chatbots, complex reasoning systems and realistic characters.