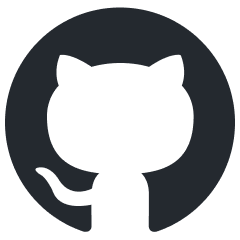
habitat-sim
A flexible, high-performance 3D simulator for Embodied AI research.
Stars: 2575
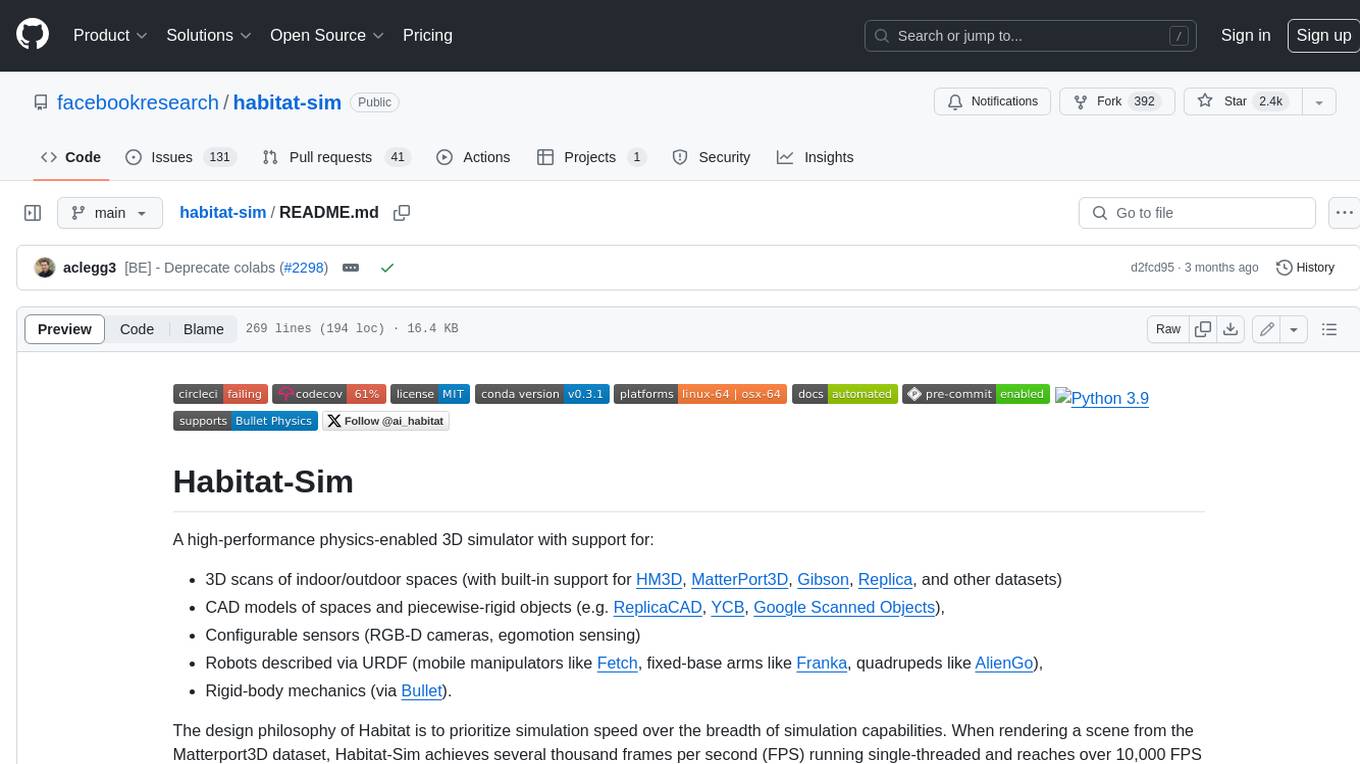
Habitat-Sim is a high-performance physics-enabled 3D simulator with support for 3D scans of indoor/outdoor spaces, CAD models of spaces and piecewise-rigid objects, configurable sensors, robots described via URDF, and rigid-body mechanics. It prioritizes simulation speed over the breadth of simulation capabilities, achieving several thousand frames per second (FPS) running single-threaded and over 10,000 FPS multi-process on a single GPU when rendering a scene from the Matterport3D dataset. Habitat-Sim simulates a Fetch robot interacting in ReplicaCAD scenes at over 8,000 steps per second (SPS), where each ‘step’ involves rendering 1 RGBD observation (128×128 pixels) and rigid-body dynamics for 1/30sec.
README:
A high-performance physics-enabled 3D simulator with support for:
- 3D scans of indoor/outdoor spaces (with built-in support for HM3D, MatterPort3D, Gibson, Replica, and other datasets)
- CAD models of spaces and piecewise-rigid objects (e.g. ReplicaCAD, YCB, Google Scanned Objects),
- Configurable sensors (RGB-D cameras, egomotion sensing)
- Robots described via URDF (mobile manipulators like Fetch, fixed-base arms like Franka, quadrupeds like AlienGo),
- Rigid-body mechanics (via Bullet).
The design philosophy of Habitat is to prioritize simulation speed over the breadth of simulation capabilities. When rendering a scene from the Matterport3D dataset, Habitat-Sim achieves several thousand frames per second (FPS) running single-threaded and reaches over 10,000 FPS multi-process on a single GPU. Habitat-Sim simulates a Fetch robot interacting in ReplicaCAD scenes at over 8,000 steps per second (SPS), where each ‘step’ involves rendering 1 RGBD observation (128×128 pixels) and rigid-body dynamics for 1/30sec.
Habitat-Sim is typically used with Habitat-Lab, a modular high-level library for end-to-end experiments in embodied AI -- defining embodied AI tasks (e.g. navigation, instruction following, question answering), training agents (via imitation or reinforcement learning, or no learning at all as in classical SensePlanAct pipelines), and benchmarking their performance on the defined tasks using standard metrics.
https://user-images.githubusercontent.com/2941091/126080914-36dc8045-01d4-4a68-8c2e-74d0bca1b9b8.mp4
If you use the Habitat platform in your research, please cite the Habitat 1.0, Habitat 2.0, and Habitat 3.0 papers:
@misc{puig2023habitat3,
title = {Habitat 3.0: A Co-Habitat for Humans, Avatars and Robots},
author = {Xavi Puig and Eric Undersander and Andrew Szot and Mikael Dallaire Cote and Ruslan Partsey and Jimmy Yang and Ruta Desai and Alexander William Clegg and Michal Hlavac and Tiffany Min and Theo Gervet and Vladimír Vondruš and Vincent-Pierre Berges and John Turner and Oleksandr Maksymets and Zsolt Kira and Mrinal Kalakrishnan and Jitendra Malik and Devendra Singh Chaplot and Unnat Jain and Dhruv Batra and Akshara Rai and Roozbeh Mottaghi},
year={2023},
archivePrefix={arXiv},
}
@inproceedings{szot2021habitat,
title = {Habitat 2.0: Training Home Assistants to Rearrange their Habitat},
author = {Andrew Szot and Alex Clegg and Eric Undersander and Erik Wijmans and Yili Zhao and John Turner and Noah Maestre and Mustafa Mukadam and Devendra Chaplot and Oleksandr Maksymets and Aaron Gokaslan and Vladimir Vondrus and Sameer Dharur and Franziska Meier and Wojciech Galuba and Angel Chang and Zsolt Kira and Vladlen Koltun and Jitendra Malik and Manolis Savva and Dhruv Batra},
booktitle = {Advances in Neural Information Processing Systems (NeurIPS)},
year = {2021}
}
@inproceedings{habitat19iccv,
title = {Habitat: {A} {P}latform for {E}mbodied {AI} {R}esearch},
author = {Manolis Savva and Abhishek Kadian and Oleksandr Maksymets and Yili Zhao and Erik Wijmans and Bhavana Jain and Julian Straub and Jia Liu and Vladlen Koltun and Jitendra Malik and Devi Parikh and Dhruv Batra},
booktitle = {Proceedings of the IEEE/CVF International Conference on Computer Vision (ICCV)},
year = {2019}
}
Habitat-Sim also builds on work contributed by others. If you use contributed methods/models, please cite their works. See the External Contributions section for a list of what was externally contributed and the corresponding work/citation.
Habitat-Sim can be installed in 3 ways:
- Via Conda - Recommended method for most users. Stable release and nightly builds.
- [Experimental] Via PIP -
pip install .
to compile the latest headless build with Bullet. Read build instructions and common build issues. - Via Docker - Updated approximately once per year for the Habitat Challenge. Read habitat-docker-setup.
- Via Source - For active development. Read build instructions and common build issues.
Habitat is under active development, and we advise users to restrict themselves to stable releases. Starting with v0.1.4, we provide conda packages for each release.
-
Preparing conda env
Assuming you have conda installed, let's prepare a conda env:
# We require python>=3.9 and cmake>=3.10 conda create -n habitat python=3.9 cmake=3.14.0 conda activate habitat
-
conda install habitat-sim
Pick one of the options below depending on your system/needs:
-
To install on machines with an attached display:
conda install habitat-sim -c conda-forge -c aihabitat
-
To install on headless machines (i.e. without an attached display, e.g. in a cluster) and machines with multiple GPUs (this parameter relies on EGL and thus does not work on MacOS):
conda install habitat-sim headless -c conda-forge -c aihabitat
-
[Most common scenario] To install habitat-sim with bullet physics
conda install habitat-sim withbullet -c conda-forge -c aihabitat
-
Note: Build parameters can be chained together. For instance, to install habitat-sim with physics on headless machines:
conda install habitat-sim withbullet headless -c conda-forge -c aihabitat
-
Conda packages for older versions can installed by explicitly specifying the version, e.g. conda install habitat-sim=0.1.6 -c conda-forge -c aihabitat
.
We also provide a nightly conda build for the main branch. However, this should only be used if you need a specific feature not yet in the latest release version. To get the nightly build of the latest main, simply swap -c aihabitat
for -c aihabitat-nightly
.
-
Let's download some 3D assets using our python data download utility:
-
Download (testing) 3D scenes
python -m habitat_sim.utils.datasets_download --uids habitat_test_scenes --data-path /path/to/data/
Note that these testing scenes do not provide semantic annotations. If you would like to test the semantic sensors via
example.py
, please use the data from the Matterport3D dataset (see Datasets). -
Download example objects
python -m habitat_sim.utils.datasets_download --uids habitat_example_objects --data-path /path/to/data/
-
-
Interactive testing: Use the interactive viewer included with Habitat-Sim in either C++ or python:
#C++ # ./build/viewer if compiling locally habitat-viewer /path/to/data/scene_datasets/habitat-test-scenes/skokloster-castle.glb #Python #NOTE: depending on your choice of installation, you may need to add '/path/to/habitat-sim' to your PYTHONPATH. #e.g. from 'habitat-sim/' directory run 'export PYTHONPATH=$(pwd)' python examples/viewer.py --scene /path/to/data/scene_datasets/habitat-test-scenes/skokloster-castle.glb
You should be able to control an agent in this test scene. Use W/A/S/D keys to move forward/left/backward/right and arrow keys or mouse (LEFT click) to control gaze direction (look up/down/left/right). Try to find the picture of a woman surrounded by a wreath. Have fun!
-
Physical interactions: Habitat-sim provides rigid and articulated dynamics simulation via integration with Bullet physics. Try it out now with our interactive viewer functionality in C++ or python.
First, download our fully interactive ReplicaCAD apartment dataset (140 MB):
#NOTE: by default, data will be downloaded into habitat-sim/data/. Optionally modify the data path by adding: `--data-path /path/to/data/` # with conda install python -m habitat_sim.utils.datasets_download --uids replica_cad_dataset # with source (from inside habitat_sim/) python src_python/habitat_sim/utils/datasets_download.py --uids replica_cad_dataset
- Alternatively, 105 scene variations with pre-baked lighting are available via
--uids replica_cad_baked_lighting
(480 MB).
Then load a ReplicaCAD scene in the viewer application with physics enabled. If you modified the data path above, also modify it in viewer calls below.
#C++ # ./build/viewer if compiling locally habitat-viewer --enable-physics --dataset data/replica_cad/replicaCAD.scene_dataset_config.json -- apt_1 #python #NOTE: habitat-sim/ directory must be on your `PYTHONPATH` python examples/viewer.py --dataset data/replica_cad/replicaCAD.scene_dataset_config.json --scene apt_1
- Using scenes with pre-baked lighting instead? Use
--dataset data/replica_cad_baked_lighting/replicaCAD_baked.scene_dataset_config.json --scene Baked_sc1_staging_00
The viewer application outputs the full list of keyboard and mouse interface options to the console at runtime.
Quickstart Example:
-
WASD
to move -
LEFT
click and drag the mouse to look around - press
SPACE
to toggle simulation off/on (default on) - press
'm'
to switch to "GRAB" mouse mode - now
LEFT
orRIGHT
click and drag to move objects or open doors/drawers and release to drop the object - with an object gripped, scroll the mouse wheel to:
- (default): move it closer or farther away
- (+
ALT
): rotate object fixed constraint frame (yaw) - (+
CTRL
): rotate object fixed constraint frame (pitch) - (+
ALT
+CTRL
): rotate object fixed constraint frame (roll)
- Alternatively, 105 scene variations with pre-baked lighting are available via
-
Non-interactive testing (e.g. for headless systems): Run the example script:
python /path/to/habitat-sim/examples/example.py --scene /path/to/data/scene_datasets/habitat-test-scenes/skokloster-castle.glb
The agent will traverse a particular path and you should see the performance stats at the very end, something like this:
640 x 480, total time: 3.208 sec. FPS: 311.7
.To reproduce the benchmark table from Habitat ICCV'19 run
examples/benchmark.py --scene /path/to/mp3d_example/17DRP5sb8fy/17DRP5sb8fy.glb
.Additional arguments to
example.py
are provided to change the sensor configuration, print statistics of the semantic annotations in a scene, compute action-space shortest path trajectories, and set other useful functionality. Refer to theexample.py
anddemo_runner.py
source files for an overview.Load a specific MP3D or Gibson house:
examples/example.py --scene path/to/mp3d/house_id.glb
.We have also provided an example demo for reference.
To run a physics example in python (after building with "Physics simulation via Bullet"):
python examples/example.py --scene /path/to/data/scene_datasets/habitat-test-scenes/skokloster-castle.glb --enable_physics
Note that in this mode the agent will be frozen and oriented toward the spawned physical objects. Additionally,
--save_png
can be used to output agent visual observation frames of the physical scene to the current directory.
-
If you are running on a remote machine and experience display errors when initializing the simulator, e.g.
X11: The DISPLAY environment variable is missing Could not initialize GLFW
ensure you do not have
DISPLAY
defined in your environment (rununset DISPLAY
to undefine the variable) -
If you see libGL errors like:
X11: The DISPLAY environment variable is missing Could not initialize GLFW
chances are your libGL is located at a non-standard location. See e.g. this issue.
Browse the online Habitat-Sim documentation.
Check out our ECCV tutorial series for a hands-on quickstart experience.
Can't find the answer to your question? Try asking the developers and community on our Discussions forum.
HowTo use common supported datasets with Habitat-Sim.
-
If you use the noise model from PyRobot, please cite the their technical report.
Specifically, the noise model used for the noisy control functions named
pyrobot_*
and defined insrc_python/habitat_sim/agent/controls/pyrobot_noisy_controls.py
-
If you use the Redwood Depth Noise Model, please cite their paper
Specifically, the noise model defined in
src_python/habitat_sim/sensors/noise_models/redwood_depth_noise_model.py
andsrc/esp/sensor/RedwoodNoiseModel.*
Habitat-Sim is MIT licensed. See the LICENSE for details.
The WebGL demo and demo scripts use:
- The King´s Hall by Skokloster Castle (Skoklosters slott) licensed under Creative Commons Attribution
- Van Gogh Room by ruslans3d licensed under Creative Commons Attribution
For Tasks:
Click tags to check more tools for each tasksFor Jobs:
Alternative AI tools for habitat-sim
Similar Open Source Tools
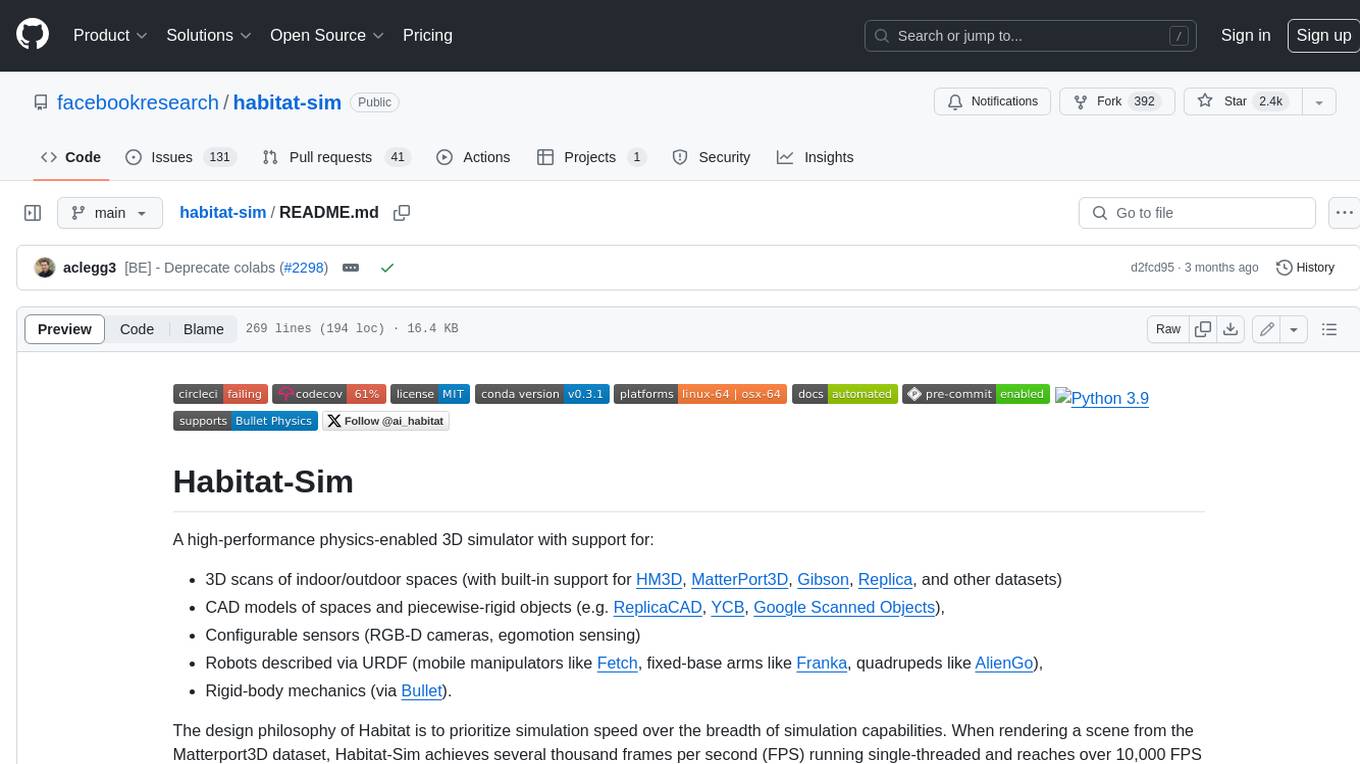
habitat-sim
Habitat-Sim is a high-performance physics-enabled 3D simulator with support for 3D scans of indoor/outdoor spaces, CAD models of spaces and piecewise-rigid objects, configurable sensors, robots described via URDF, and rigid-body mechanics. It prioritizes simulation speed over the breadth of simulation capabilities, achieving several thousand frames per second (FPS) running single-threaded and over 10,000 FPS multi-process on a single GPU when rendering a scene from the Matterport3D dataset. Habitat-Sim simulates a Fetch robot interacting in ReplicaCAD scenes at over 8,000 steps per second (SPS), where each ‘step’ involves rendering 1 RGBD observation (128×128 pixels) and rigid-body dynamics for 1/30sec.
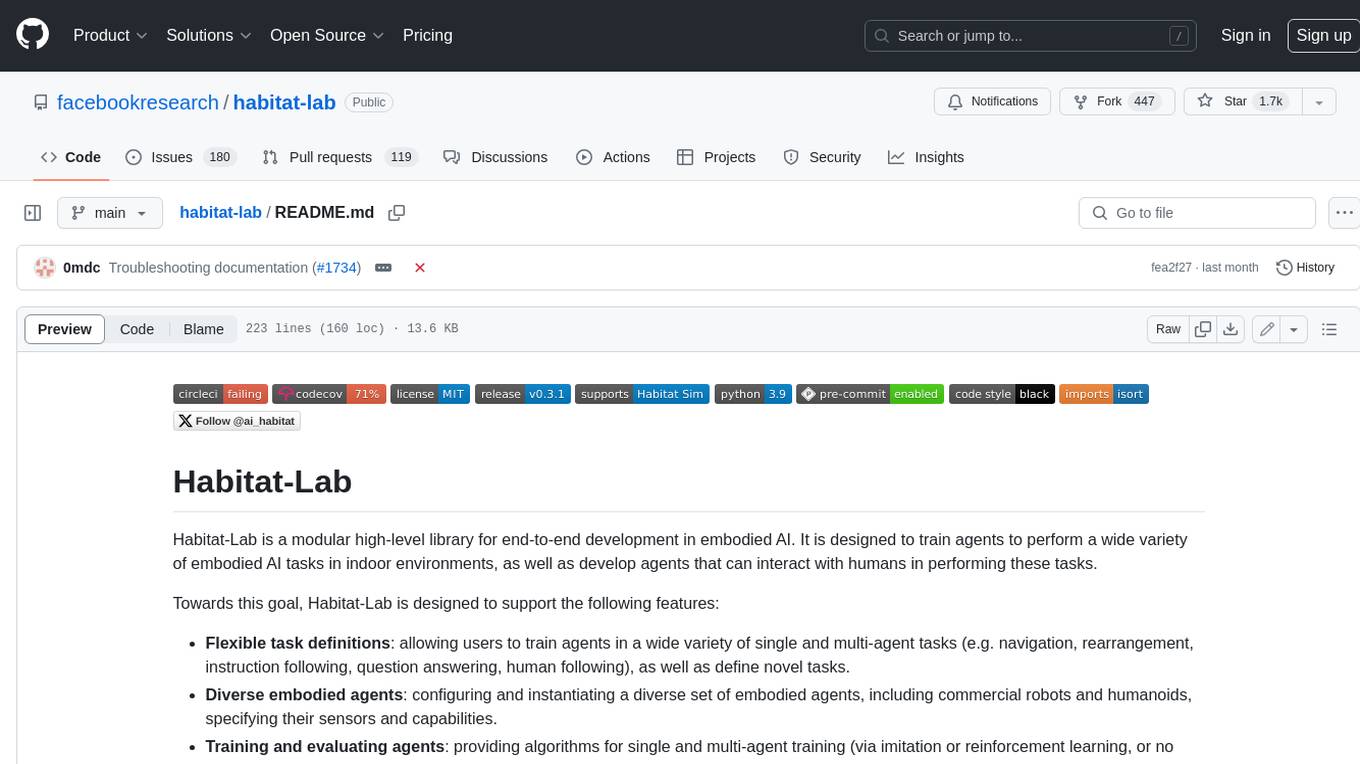
habitat-lab
Habitat-Lab is a modular high-level library for end-to-end development in embodied AI. It is designed to train agents to perform a wide variety of embodied AI tasks in indoor environments, as well as develop agents that can interact with humans in performing these tasks.
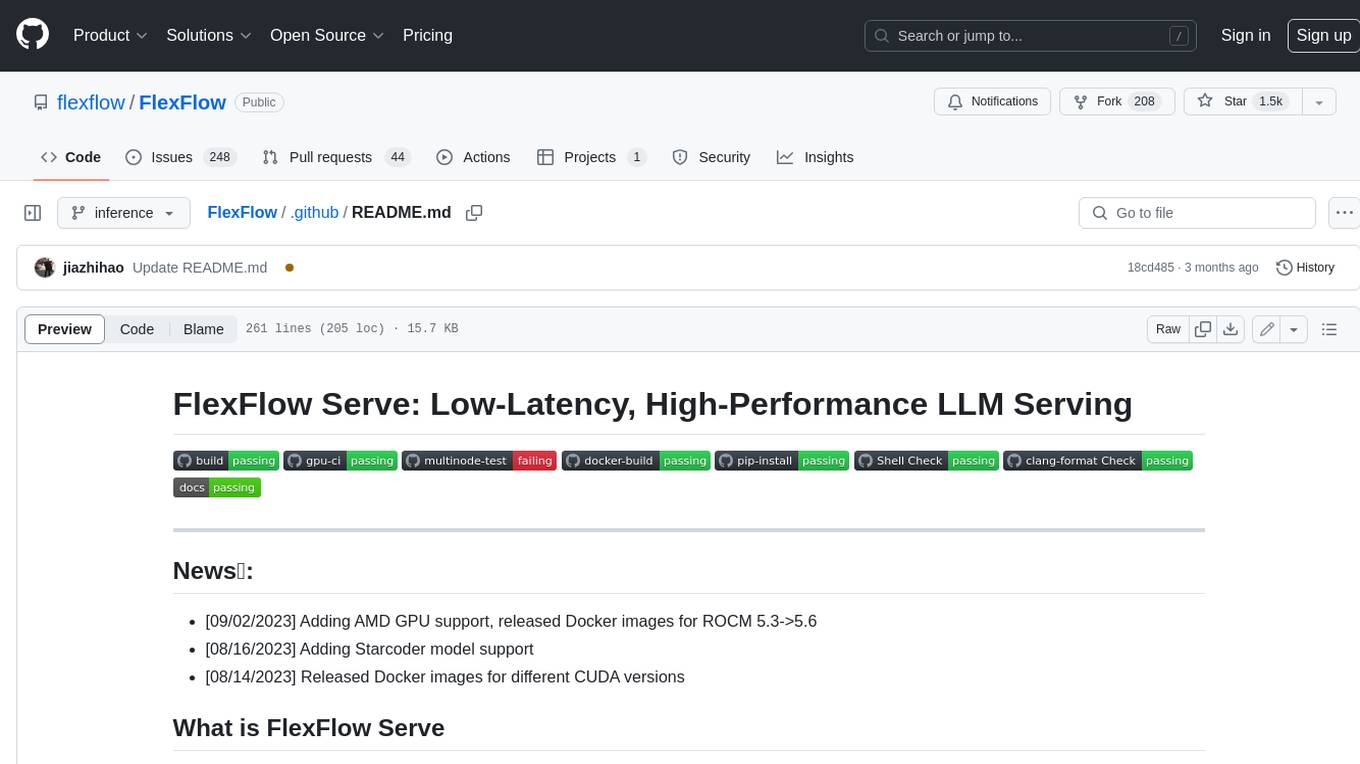
FlexFlow
FlexFlow Serve is an open-source compiler and distributed system for **low latency**, **high performance** LLM serving. FlexFlow Serve outperforms existing systems by 1.3-2.0x for single-node, multi-GPU inference and by 1.4-2.4x for multi-node, multi-GPU inference.
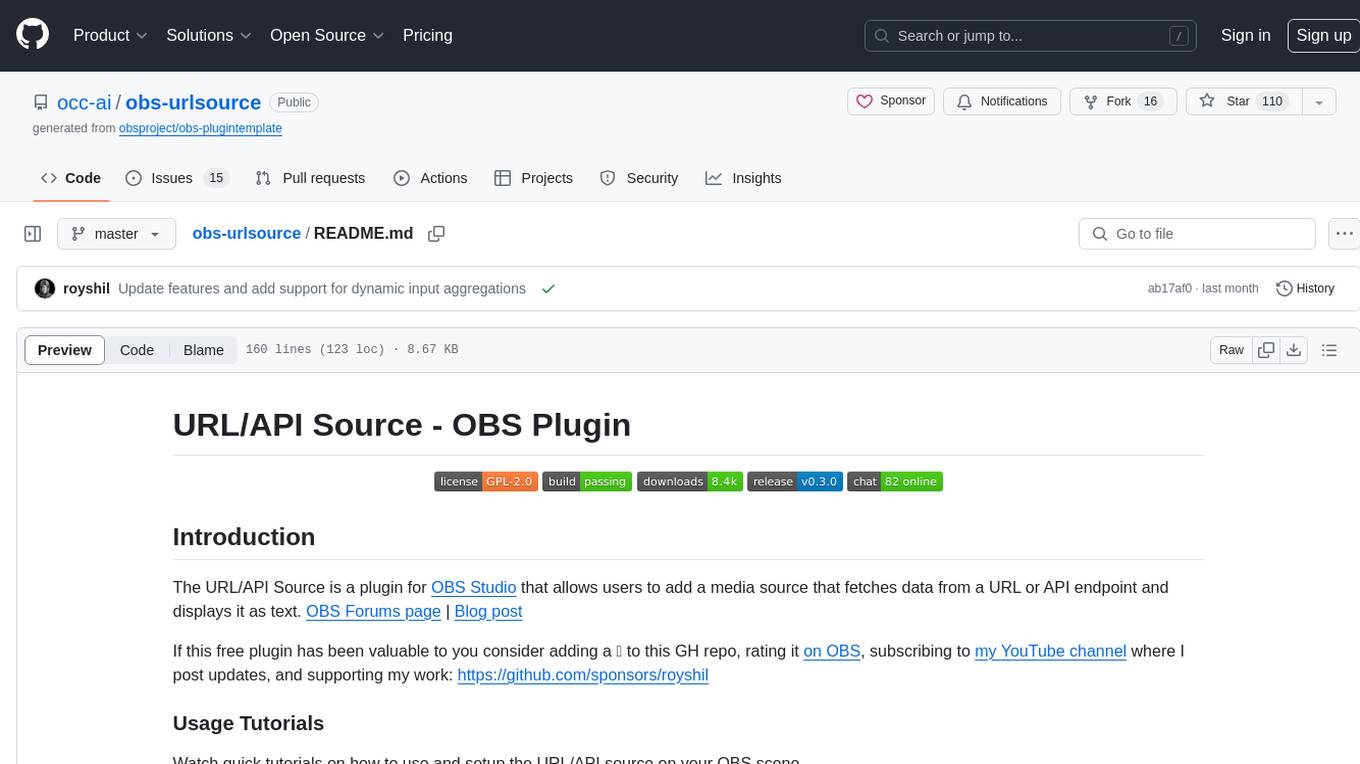
obs-urlsource
The URL/API Source is a plugin for OBS Studio that allows users to add a media source fetching data from a URL or API endpoint and displaying it as text. It supports input and output templating, various request types, output parsing (JSON, XML/HTML, Regex, CSS selectors), live data updating, output styling, and formatting. Future features include authentication, websocket support, more parsing options, request types, and output formats. The plugin is cross-platform compatible and actively maintained by the developer. Users can support the project on GitHub.
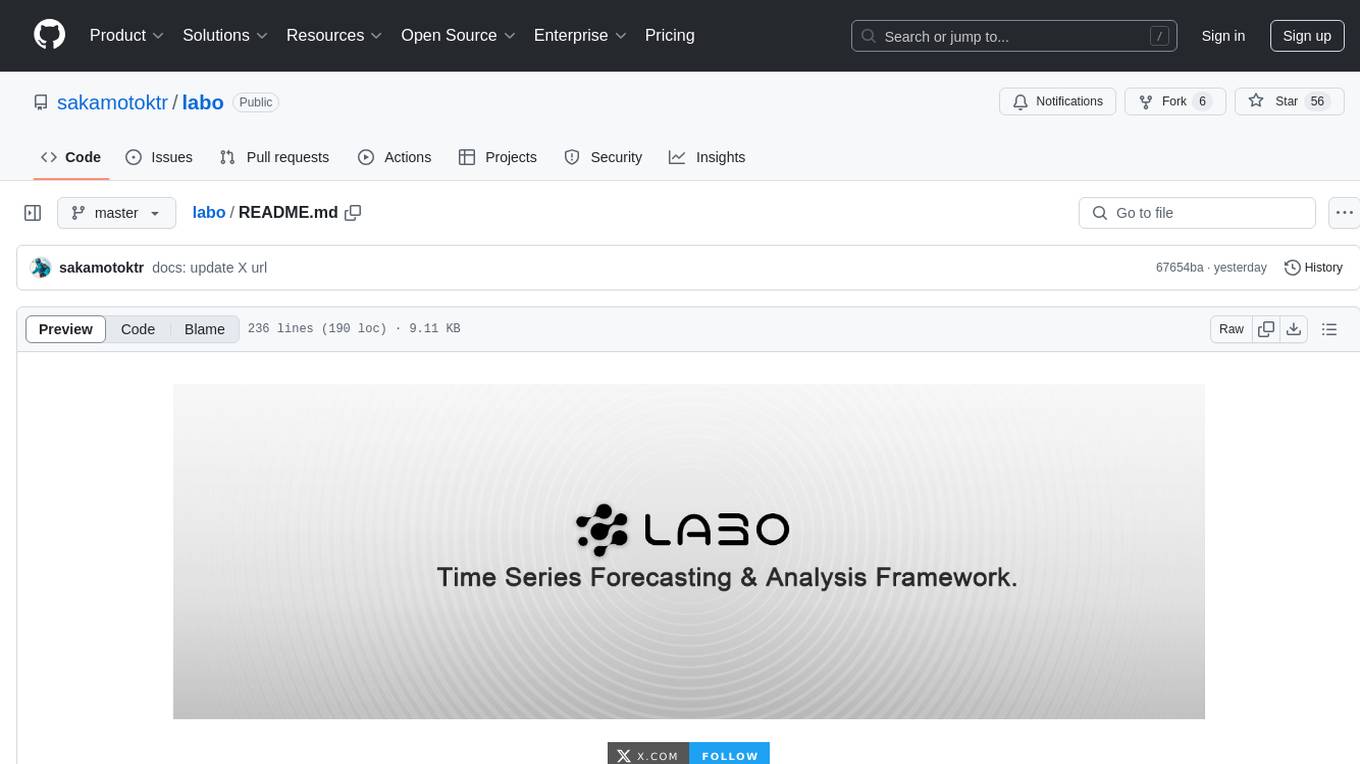
labo
LABO is a time series forecasting and analysis framework that integrates pre-trained and fine-tuned LLMs with multi-domain agent-based systems. It allows users to create and tune agents easily for various scenarios, such as stock market trend prediction and web public opinion analysis. LABO requires a specific runtime environment setup, including system requirements, Python environment, dependency installations, and configurations. Users can fine-tune their own models using LABO's Low-Rank Adaptation (LoRA) for computational efficiency and continuous model updates. Additionally, LABO provides a Python library for building model training pipelines and customizing agents for specific tasks.
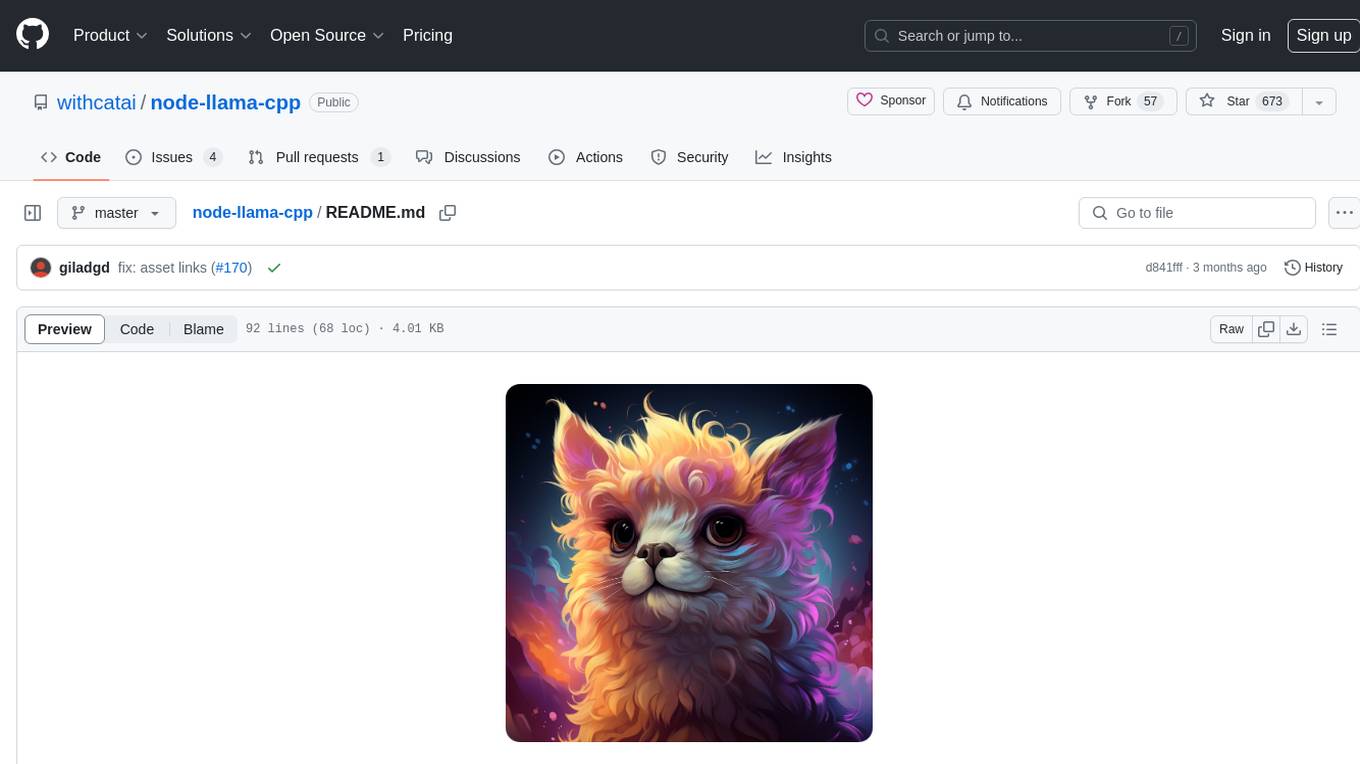
node-llama-cpp
node-llama-cpp is a tool that allows users to run AI models locally on their machines. It provides pre-built bindings with the option to build from source using cmake. Users can interact with text generation models, chat with models using a chat wrapper, and force models to generate output in a parseable format like JSON. The tool supports Metal and CUDA, offers CLI functionality for chatting with models without coding, and ensures up-to-date compatibility with the latest version of llama.cpp. Installation includes pre-built binaries for macOS, Linux, and Windows, with the option to build from source if binaries are not available for the platform.
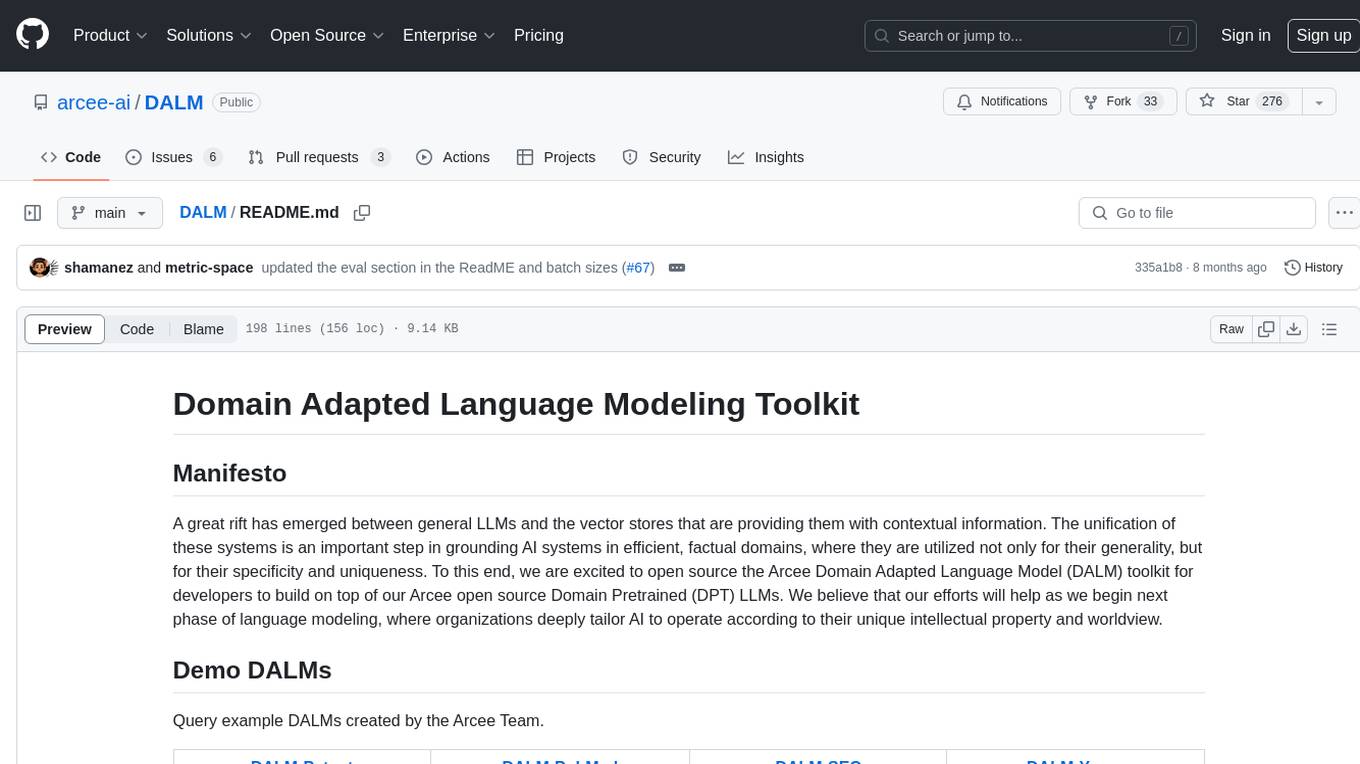
DALM
The DALM (Domain Adapted Language Modeling) toolkit is designed to unify general LLMs with vector stores to ground AI systems in efficient, factual domains. It provides developers with tools to build on top of Arcee's open source Domain Pretrained LLMs, enabling organizations to deeply tailor AI according to their unique intellectual property and worldview. The toolkit contains code for fine-tuning a fully differential Retrieval Augmented Generation (RAG-end2end) architecture, incorporating in-batch negative concept alongside RAG's marginalization for efficiency. It includes training scripts for both retriever and generator models, evaluation scripts, data processing codes, and synthetic data generation code.
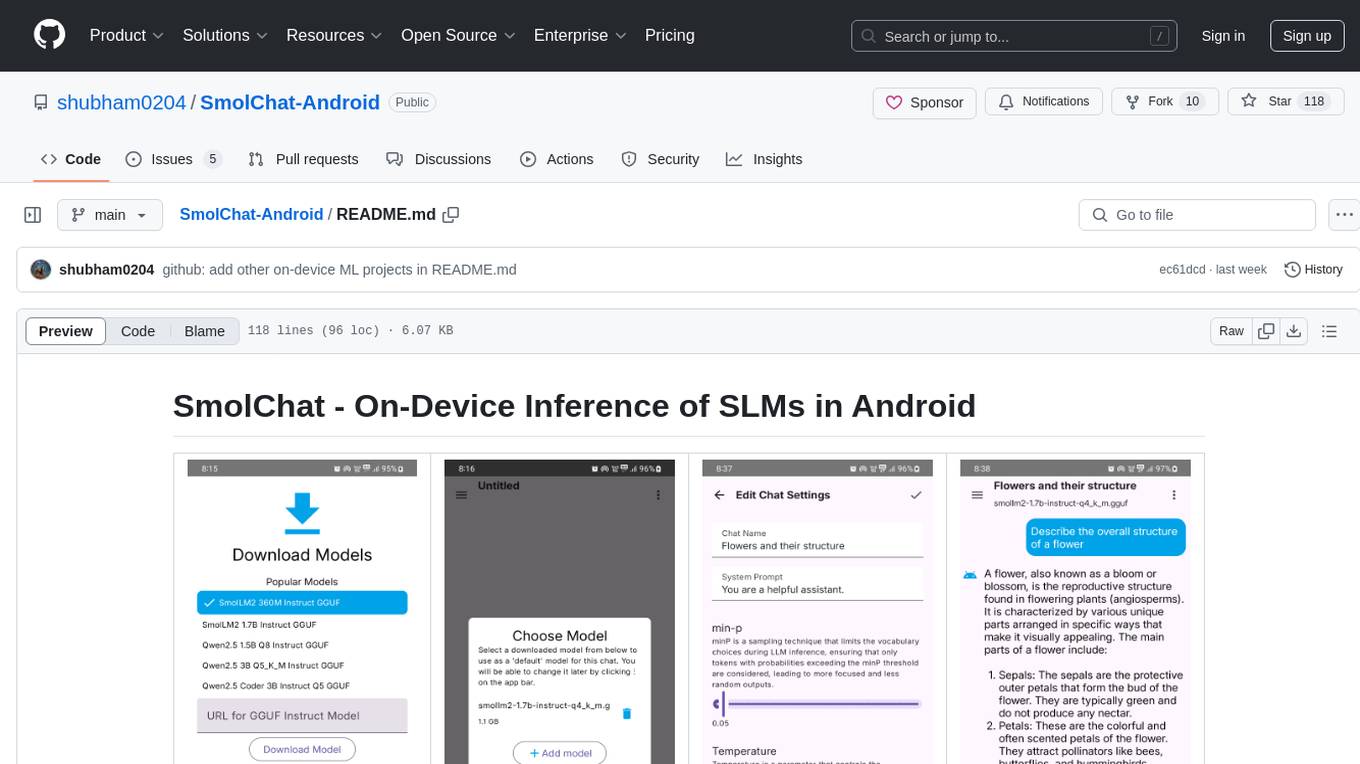
SmolChat-Android
SmolChat-Android is a mobile application that enables users to interact with local small language models (SLMs) on-device. Users can add/remove SLMs, modify system prompts and inference parameters, create downstream tasks, and generate responses. The app uses llama.cpp for model execution, ObjectBox for database storage, and Markwon for markdown rendering. It provides a simple, extensible codebase for on-device machine learning projects.
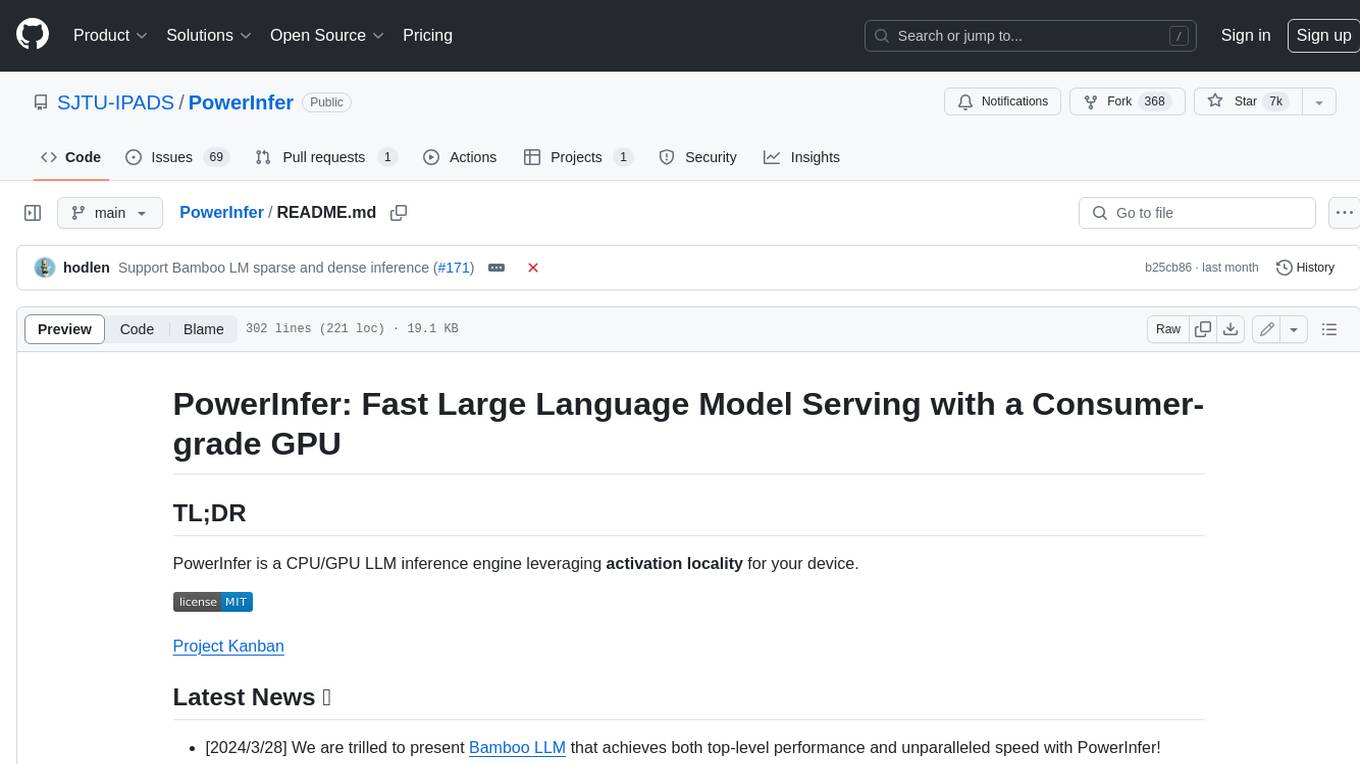
PowerInfer
PowerInfer is a high-speed Large Language Model (LLM) inference engine designed for local deployment on consumer-grade hardware, leveraging activation locality to optimize efficiency. It features a locality-centric design, hybrid CPU/GPU utilization, easy integration with popular ReLU-sparse models, and support for various platforms. PowerInfer achieves high speed with lower resource demands and is flexible for easy deployment and compatibility with existing models like Falcon-40B, Llama2 family, ProSparse Llama2 family, and Bamboo-7B.
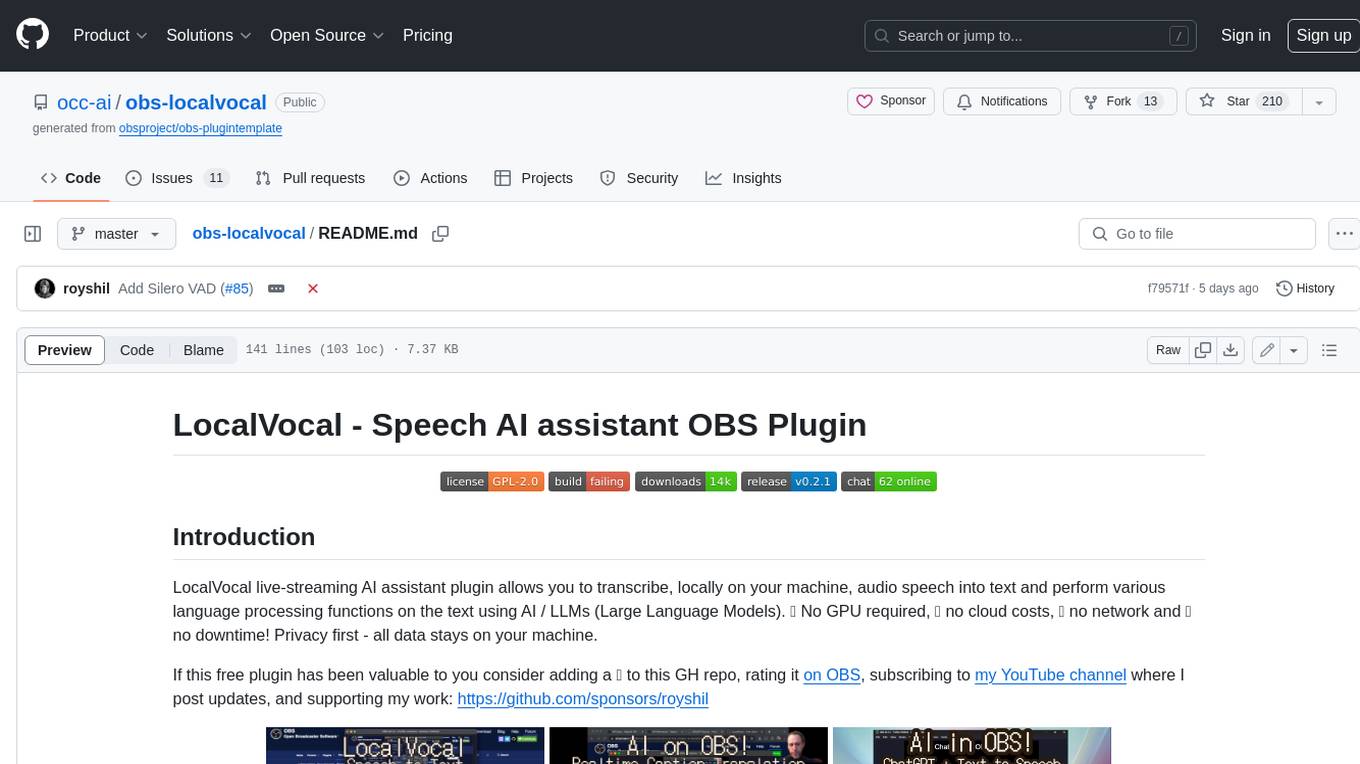
obs-localvocal
LocalVocal is a live-streaming AI assistant plugin for OBS that allows you to transcribe audio speech into text and perform various language processing functions on the text using AI / LLMs (Large Language Models). It's privacy-first, with all data staying on your machine, and requires no GPU, cloud costs, network, or downtime.
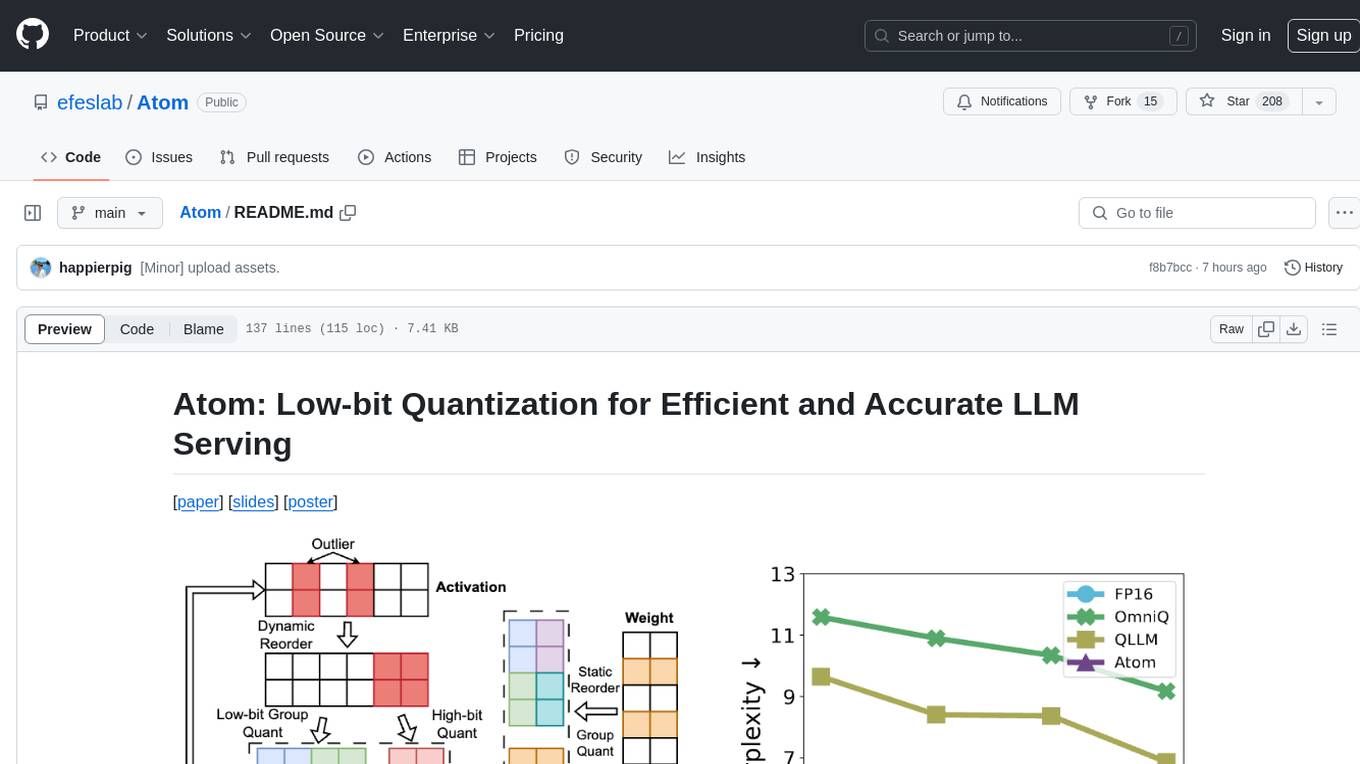
Atom
Atom is an accurate low-bit weight-activation quantization algorithm that combines mixed-precision, fine-grained group quantization, dynamic activation quantization, KV-cache quantization, and efficient CUDA kernels co-design. It introduces a low-bit quantization method, Atom, to maximize Large Language Models (LLMs) serving throughput with negligible accuracy loss. The codebase includes evaluation of perplexity and zero-shot accuracy, kernel benchmarking, and end-to-end evaluation. Atom significantly boosts serving throughput by using low-bit operators and reduces memory consumption via low-bit quantization.
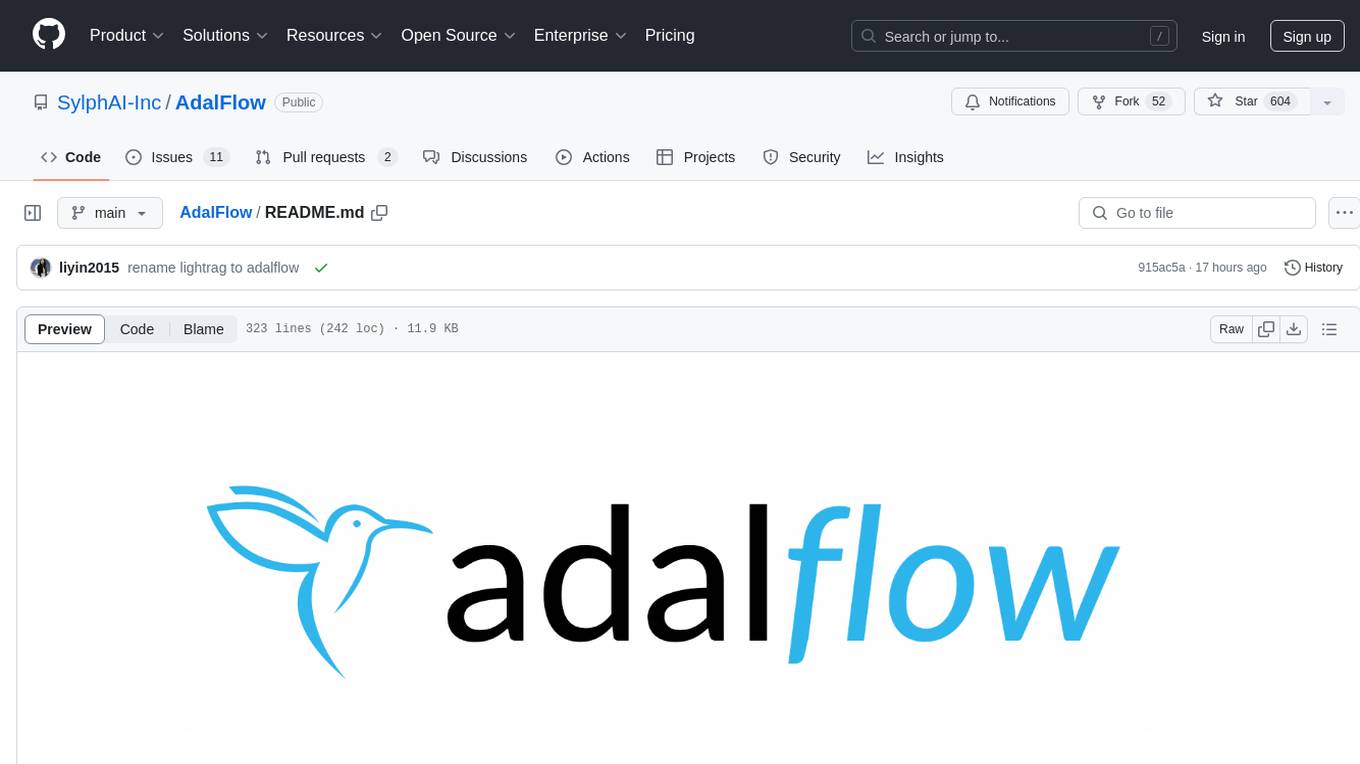
AdalFlow
AdalFlow is a library designed to help developers build and optimize Large Language Model (LLM) task pipelines. It follows a design pattern similar to PyTorch, offering a light, modular, and robust codebase. Named in honor of Ada Lovelace, AdalFlow aims to inspire more women to enter the AI field. The library is tailored for various GenAI applications like chatbots, translation, summarization, code generation, and autonomous agents, as well as classical NLP tasks such as text classification and named entity recognition. AdalFlow emphasizes modularity, robustness, and readability to support users in customizing and iterating code for their specific use cases.
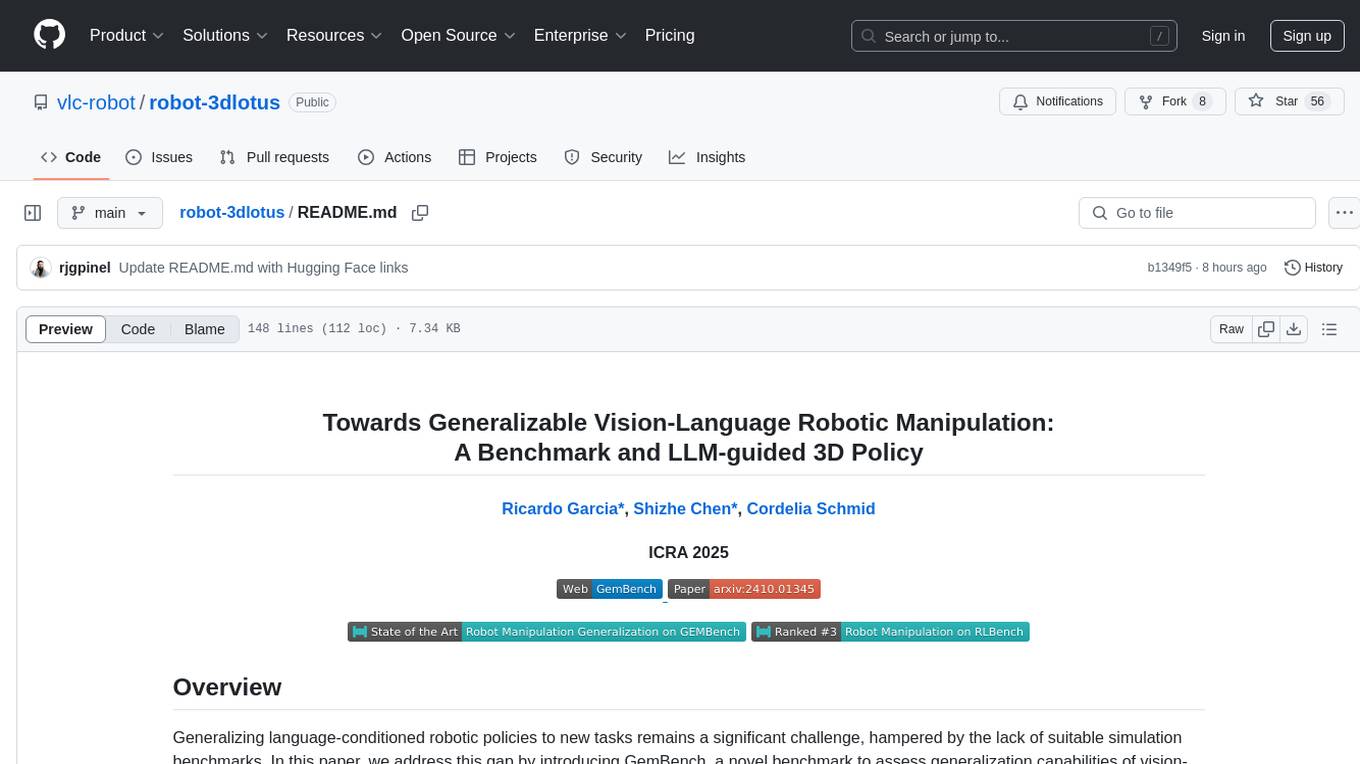
robot-3dlotus
Towards Generalizable Vision-Language Robotic Manipulation: A Benchmark and LLM-guided 3D Policy is a research project focusing on addressing the challenge of generalizing language-conditioned robotic policies to new tasks. The project introduces GemBench, a benchmark to evaluate the generalization capabilities of vision-language robotic manipulation policies. It also presents the 3D-LOTUS approach, which leverages rich 3D information for action prediction conditioned on language. Additionally, the project introduces 3D-LOTUS++, a framework that integrates 3D-LOTUS's motion planning capabilities with the task planning capabilities of LLMs and the object grounding accuracy of VLMs to achieve state-of-the-art performance on novel tasks in robotic manipulation.
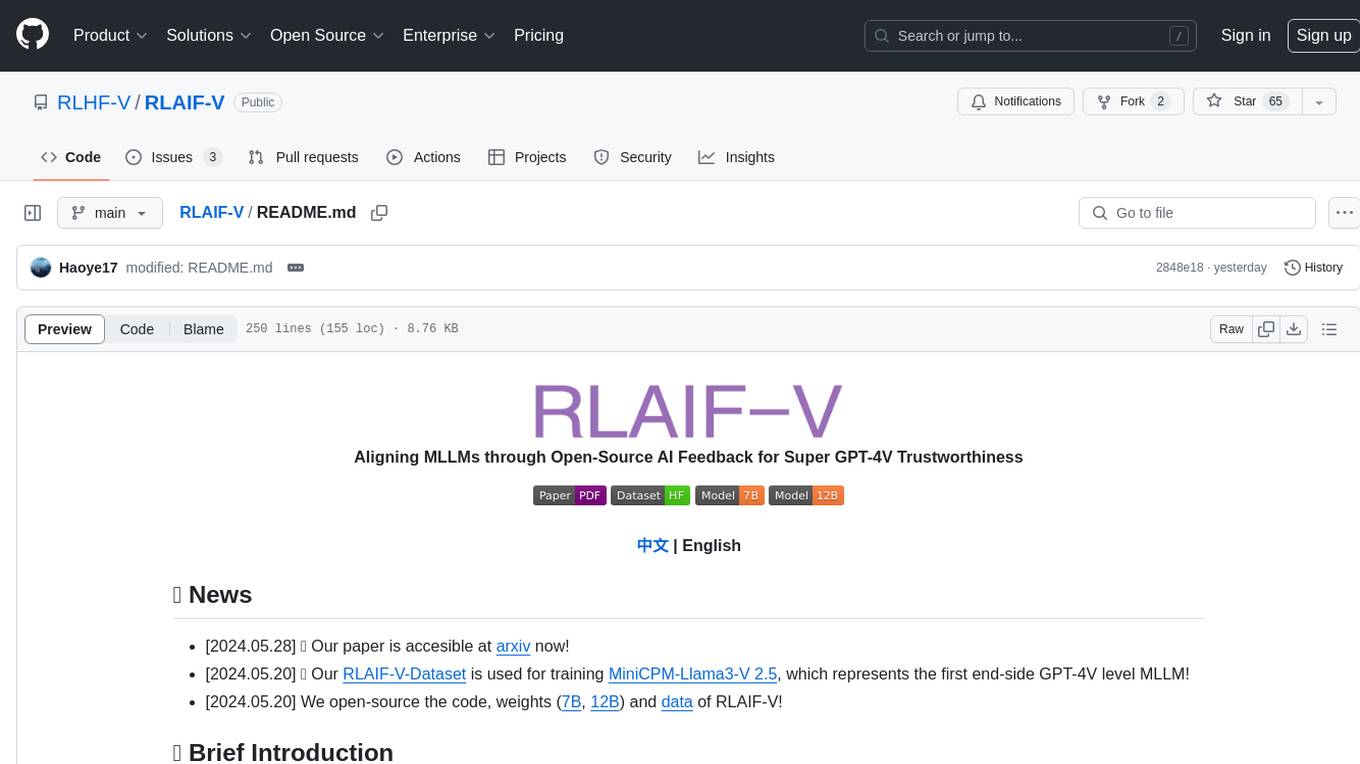
RLAIF-V
RLAIF-V is a novel framework that aligns MLLMs in a fully open-source paradigm for super GPT-4V trustworthiness. It maximally exploits open-source feedback from high-quality feedback data and online feedback learning algorithm. Notable features include achieving super GPT-4V trustworthiness in both generative and discriminative tasks, using high-quality generalizable feedback data to reduce hallucination of different MLLMs, and exhibiting better learning efficiency and higher performance through iterative alignment.
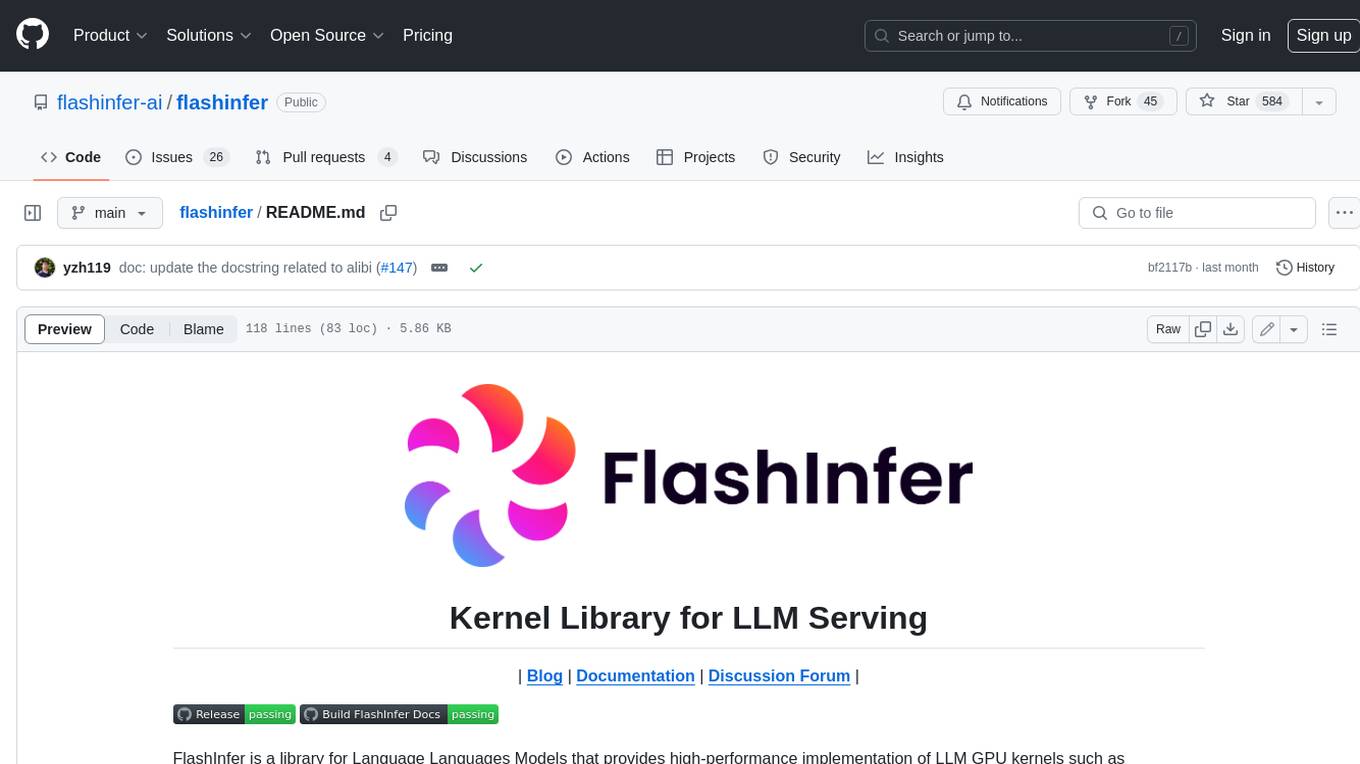
flashinfer
FlashInfer is a library for Language Languages Models that provides high-performance implementation of LLM GPU kernels such as FlashAttention, PageAttention and LoRA. FlashInfer focus on LLM serving and inference, and delivers state-the-art performance across diverse scenarios.
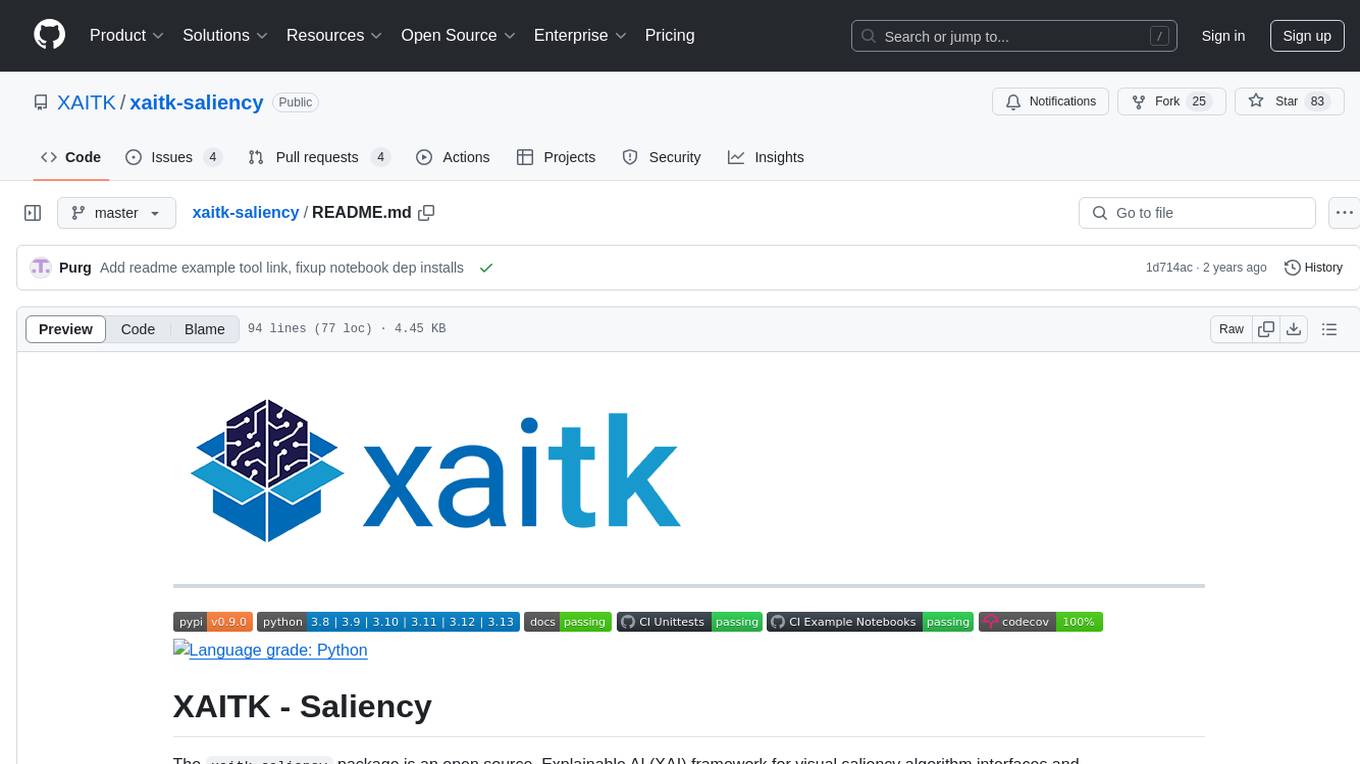
xaitk-saliency
The `xaitk-saliency` package is an open source Explainable AI (XAI) framework for visual saliency algorithm interfaces and implementations, designed for analytics and autonomy applications. It provides saliency algorithms for various image understanding tasks such as image classification, image similarity, object detection, and reinforcement learning. The toolkit targets data scientists and developers who aim to incorporate visual saliency explanations into their workflow or product, offering both direct accessibility for experimentation and modular integration into systems and applications through Strategy and Adapter patterns. The package includes documentation, examples, and a demonstration tool for visual saliency generation in a user-interface.
For similar tasks
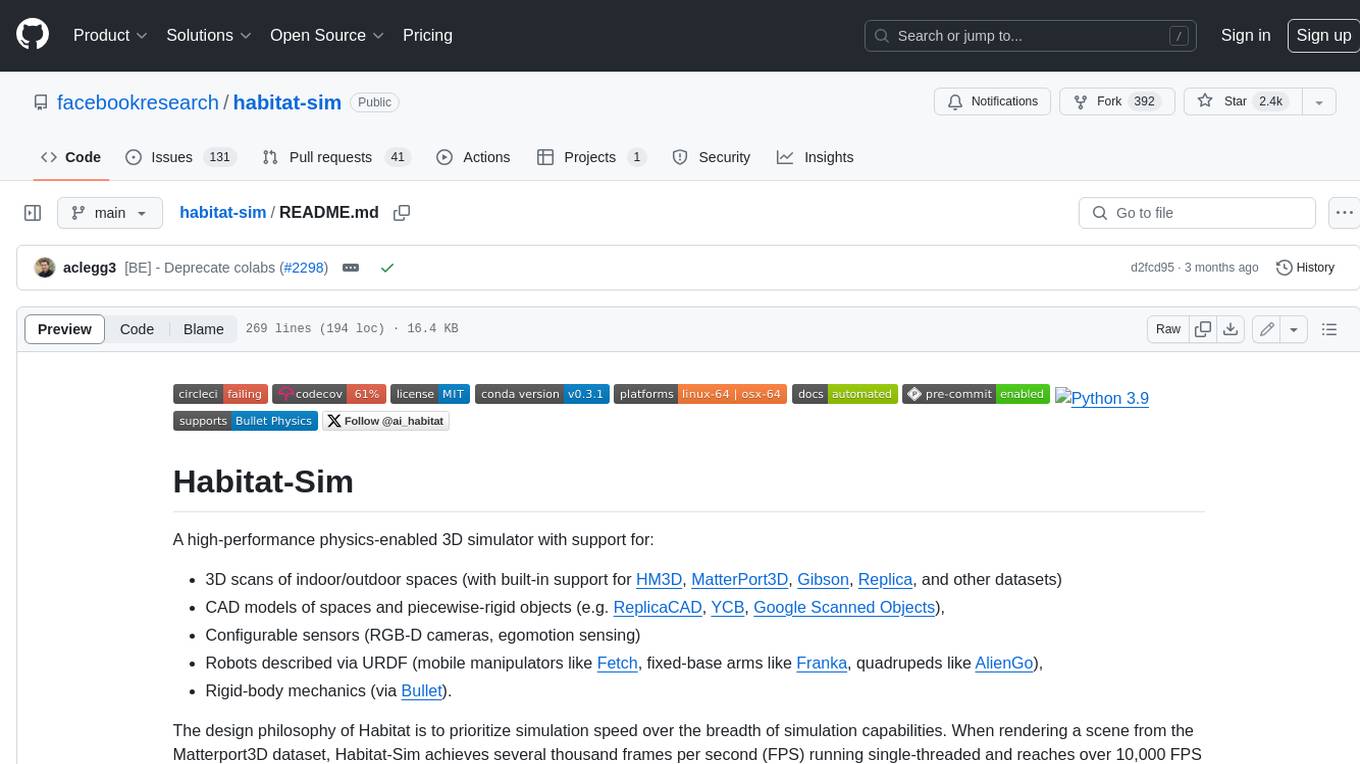
habitat-sim
Habitat-Sim is a high-performance physics-enabled 3D simulator with support for 3D scans of indoor/outdoor spaces, CAD models of spaces and piecewise-rigid objects, configurable sensors, robots described via URDF, and rigid-body mechanics. It prioritizes simulation speed over the breadth of simulation capabilities, achieving several thousand frames per second (FPS) running single-threaded and over 10,000 FPS multi-process on a single GPU when rendering a scene from the Matterport3D dataset. Habitat-Sim simulates a Fetch robot interacting in ReplicaCAD scenes at over 8,000 steps per second (SPS), where each ‘step’ involves rendering 1 RGBD observation (128×128 pixels) and rigid-body dynamics for 1/30sec.
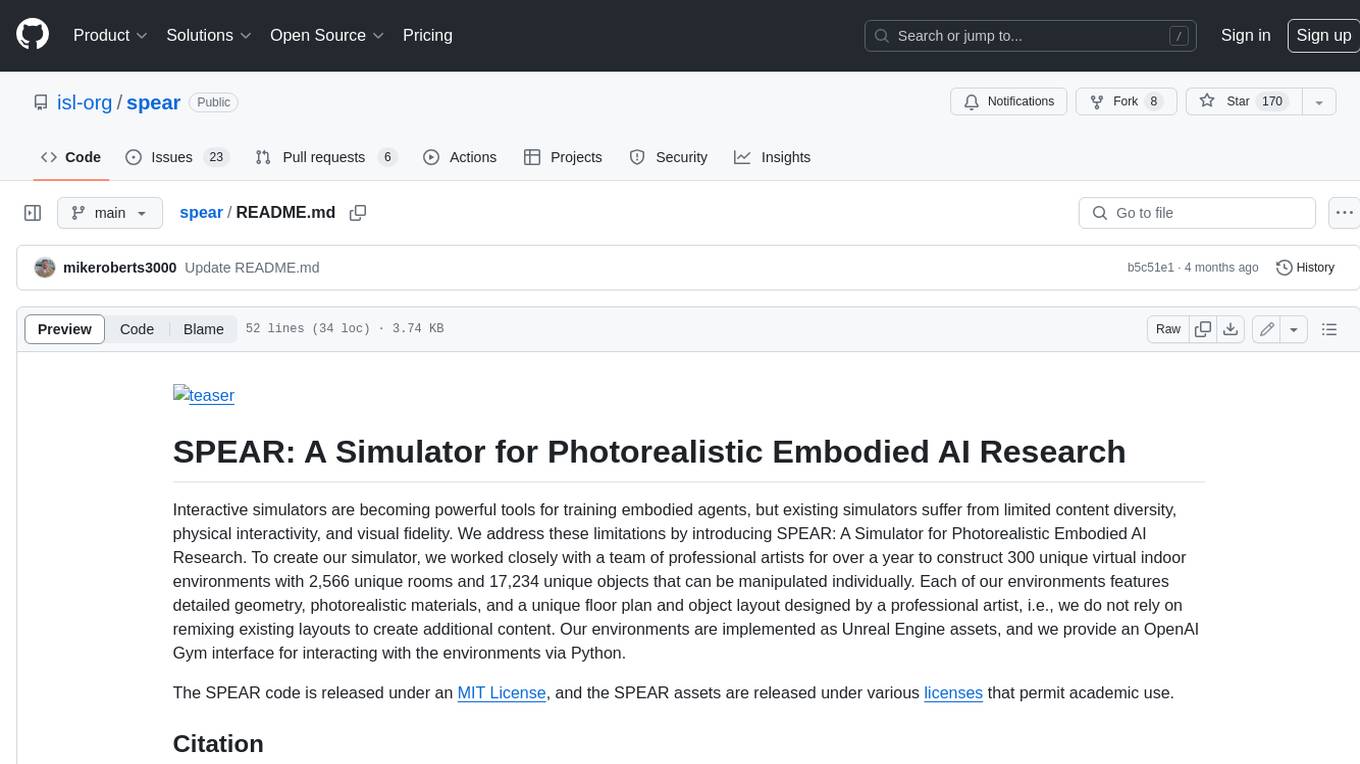
spear
SPEAR (Simulator for Photorealistic Embodied AI Research) is a powerful tool for training embodied agents. It features 300 unique virtual indoor environments with 2,566 unique rooms and 17,234 unique objects that can be manipulated individually. Each environment is designed by a professional artist and features detailed geometry, photorealistic materials, and a unique floor plan and object layout. SPEAR is implemented as Unreal Engine assets and provides an OpenAI Gym interface for interacting with the environments via Python.
For similar jobs
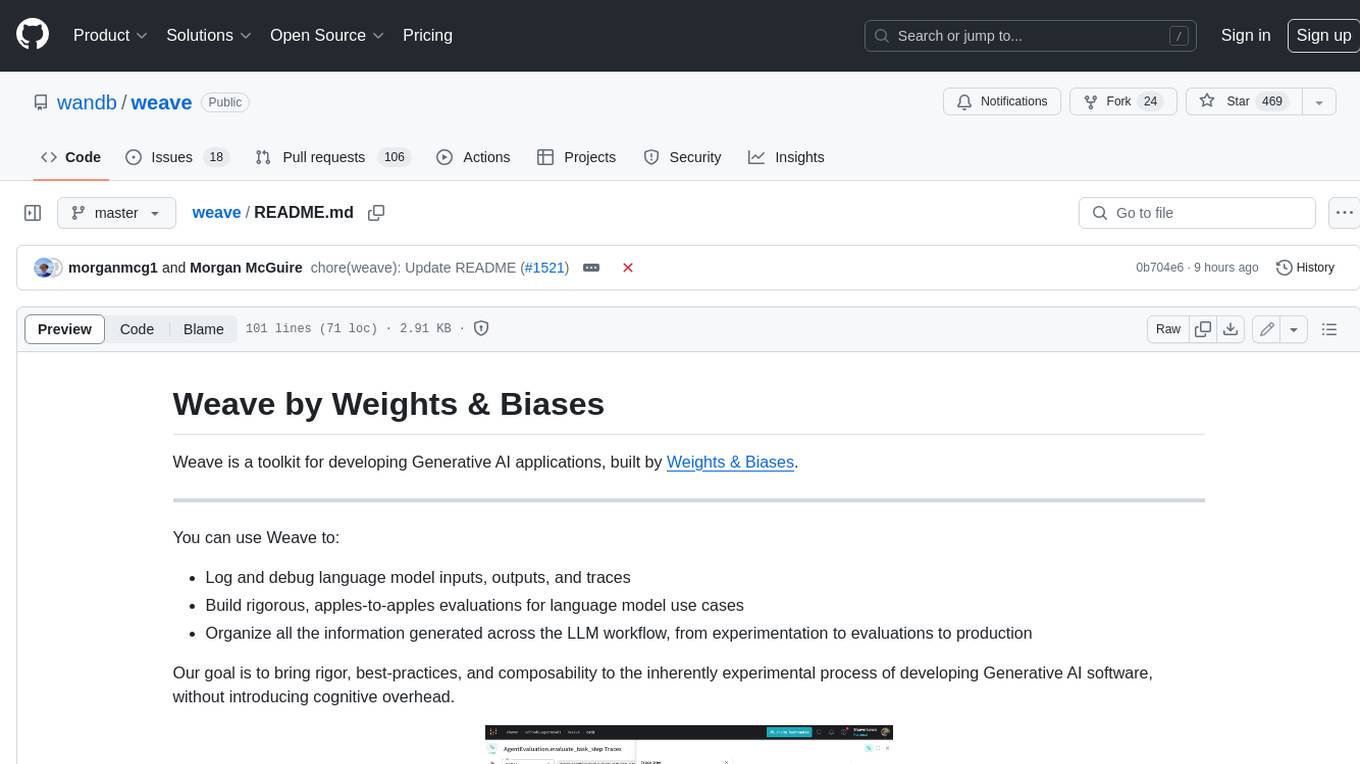
weave
Weave is a toolkit for developing Generative AI applications, built by Weights & Biases. With Weave, you can log and debug language model inputs, outputs, and traces; build rigorous, apples-to-apples evaluations for language model use cases; and organize all the information generated across the LLM workflow, from experimentation to evaluations to production. Weave aims to bring rigor, best-practices, and composability to the inherently experimental process of developing Generative AI software, without introducing cognitive overhead.
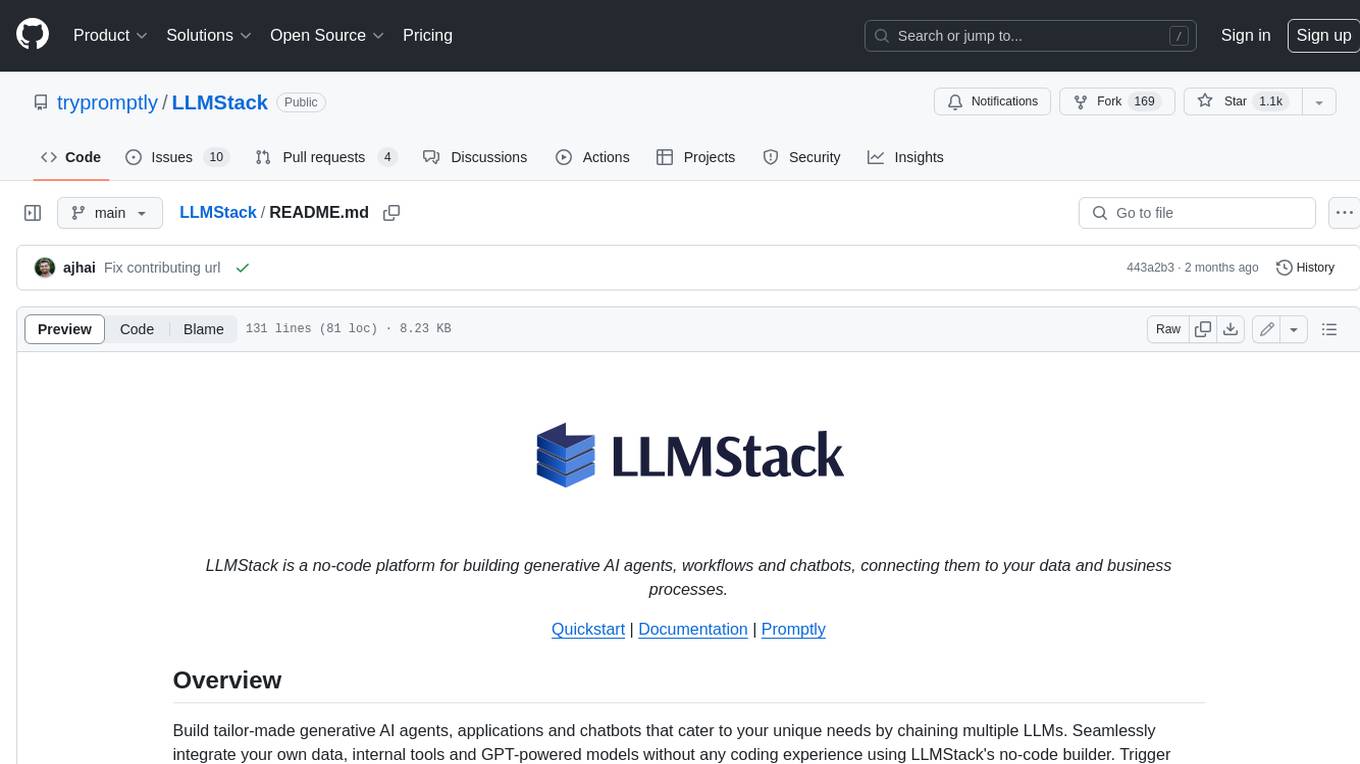
LLMStack
LLMStack is a no-code platform for building generative AI agents, workflows, and chatbots. It allows users to connect their own data, internal tools, and GPT-powered models without any coding experience. LLMStack can be deployed to the cloud or on-premise and can be accessed via HTTP API or triggered from Slack or Discord.
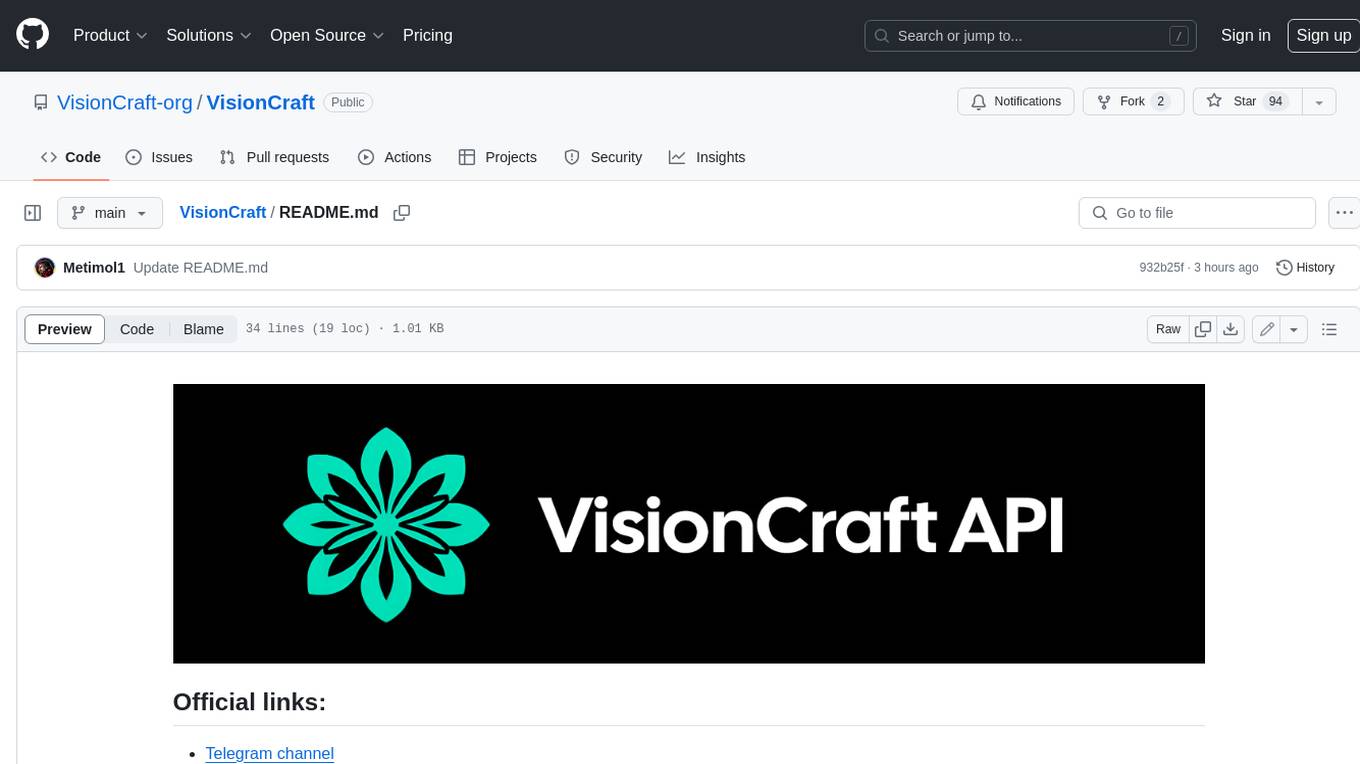
VisionCraft
The VisionCraft API is a free API for using over 100 different AI models. From images to sound.
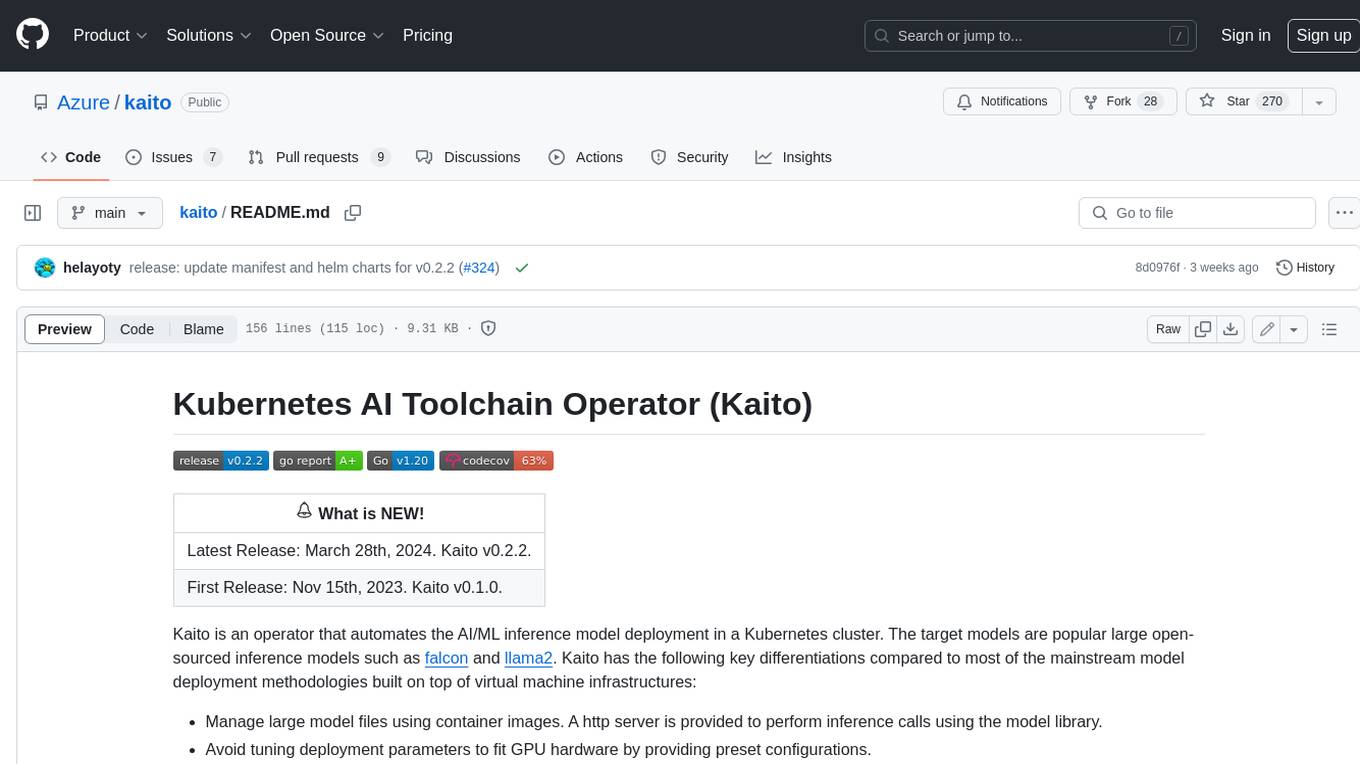
kaito
Kaito is an operator that automates the AI/ML inference model deployment in a Kubernetes cluster. It manages large model files using container images, avoids tuning deployment parameters to fit GPU hardware by providing preset configurations, auto-provisions GPU nodes based on model requirements, and hosts large model images in the public Microsoft Container Registry (MCR) if the license allows. Using Kaito, the workflow of onboarding large AI inference models in Kubernetes is largely simplified.
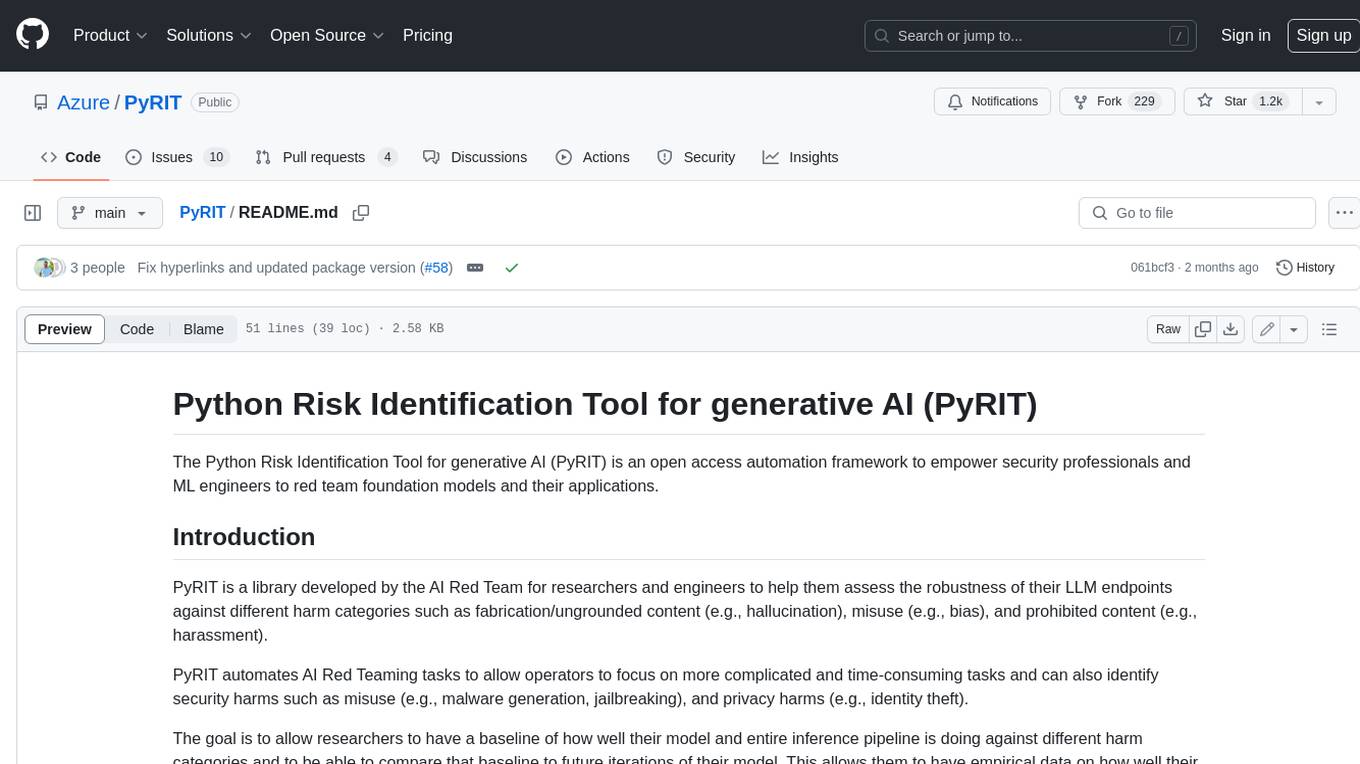
PyRIT
PyRIT is an open access automation framework designed to empower security professionals and ML engineers to red team foundation models and their applications. It automates AI Red Teaming tasks to allow operators to focus on more complicated and time-consuming tasks and can also identify security harms such as misuse (e.g., malware generation, jailbreaking), and privacy harms (e.g., identity theft). The goal is to allow researchers to have a baseline of how well their model and entire inference pipeline is doing against different harm categories and to be able to compare that baseline to future iterations of their model. This allows them to have empirical data on how well their model is doing today, and detect any degradation of performance based on future improvements.
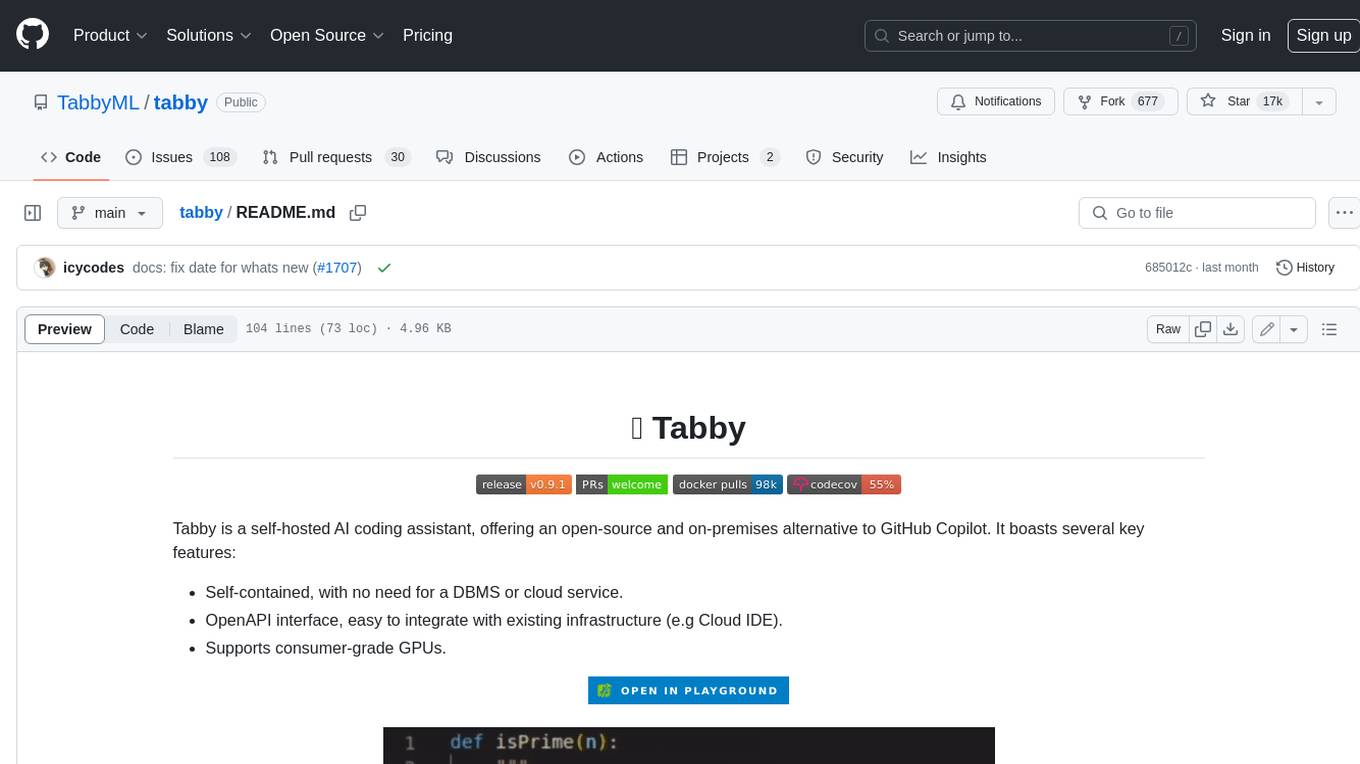
tabby
Tabby is a self-hosted AI coding assistant, offering an open-source and on-premises alternative to GitHub Copilot. It boasts several key features: * Self-contained, with no need for a DBMS or cloud service. * OpenAPI interface, easy to integrate with existing infrastructure (e.g Cloud IDE). * Supports consumer-grade GPUs.
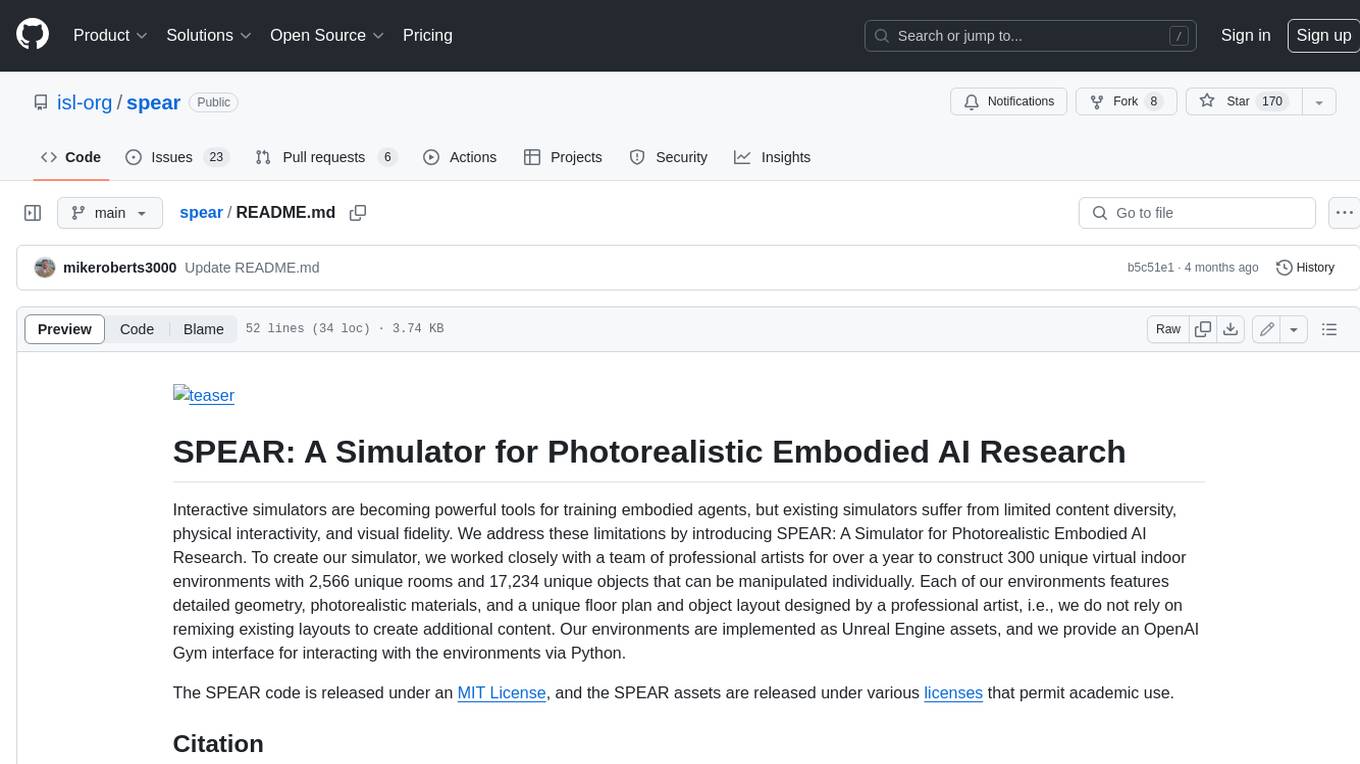
spear
SPEAR (Simulator for Photorealistic Embodied AI Research) is a powerful tool for training embodied agents. It features 300 unique virtual indoor environments with 2,566 unique rooms and 17,234 unique objects that can be manipulated individually. Each environment is designed by a professional artist and features detailed geometry, photorealistic materials, and a unique floor plan and object layout. SPEAR is implemented as Unreal Engine assets and provides an OpenAI Gym interface for interacting with the environments via Python.
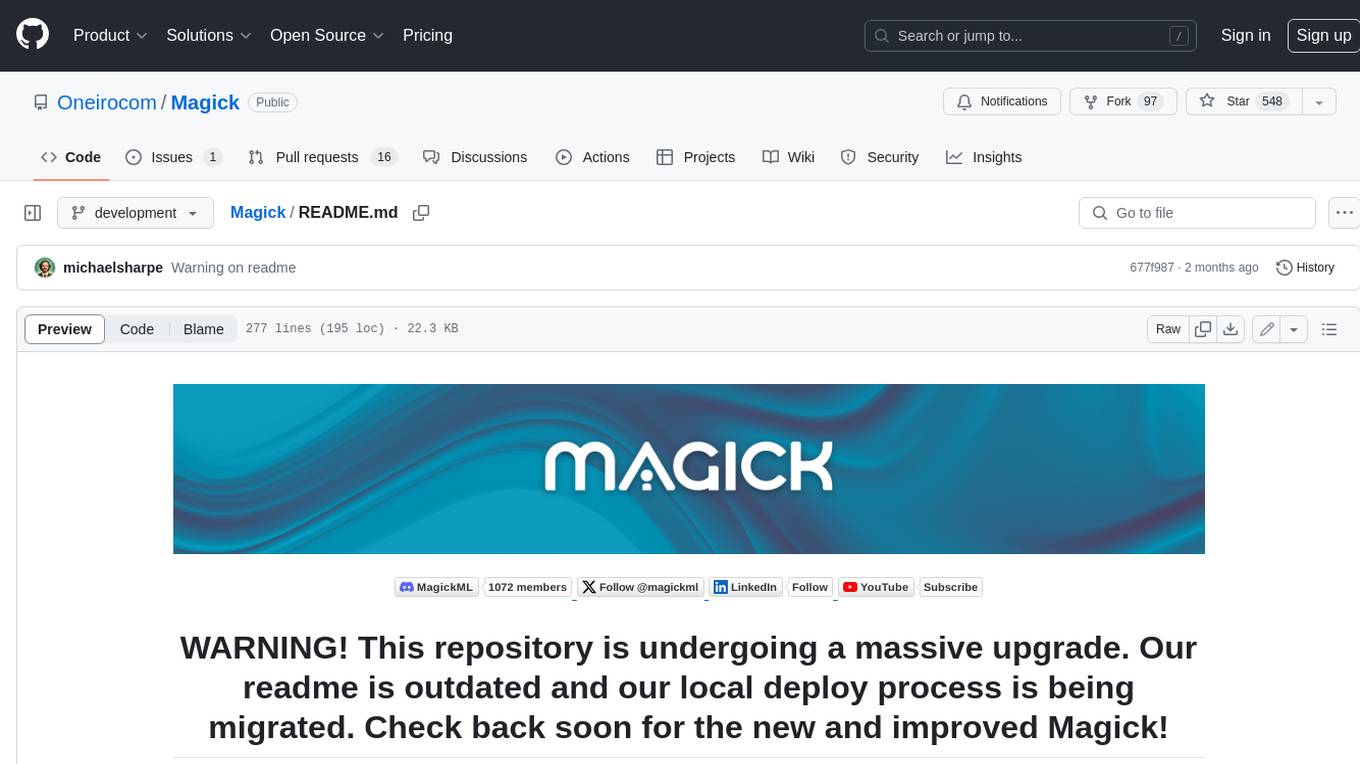
Magick
Magick is a groundbreaking visual AIDE (Artificial Intelligence Development Environment) for no-code data pipelines and multimodal agents. Magick can connect to other services and comes with nodes and templates well-suited for intelligent agents, chatbots, complex reasoning systems and realistic characters.