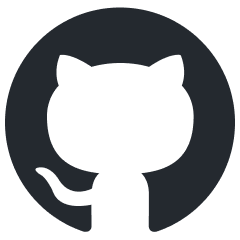
HAMi
Heterogeneous AI Computing Virtualization Middleware
Stars: 1205
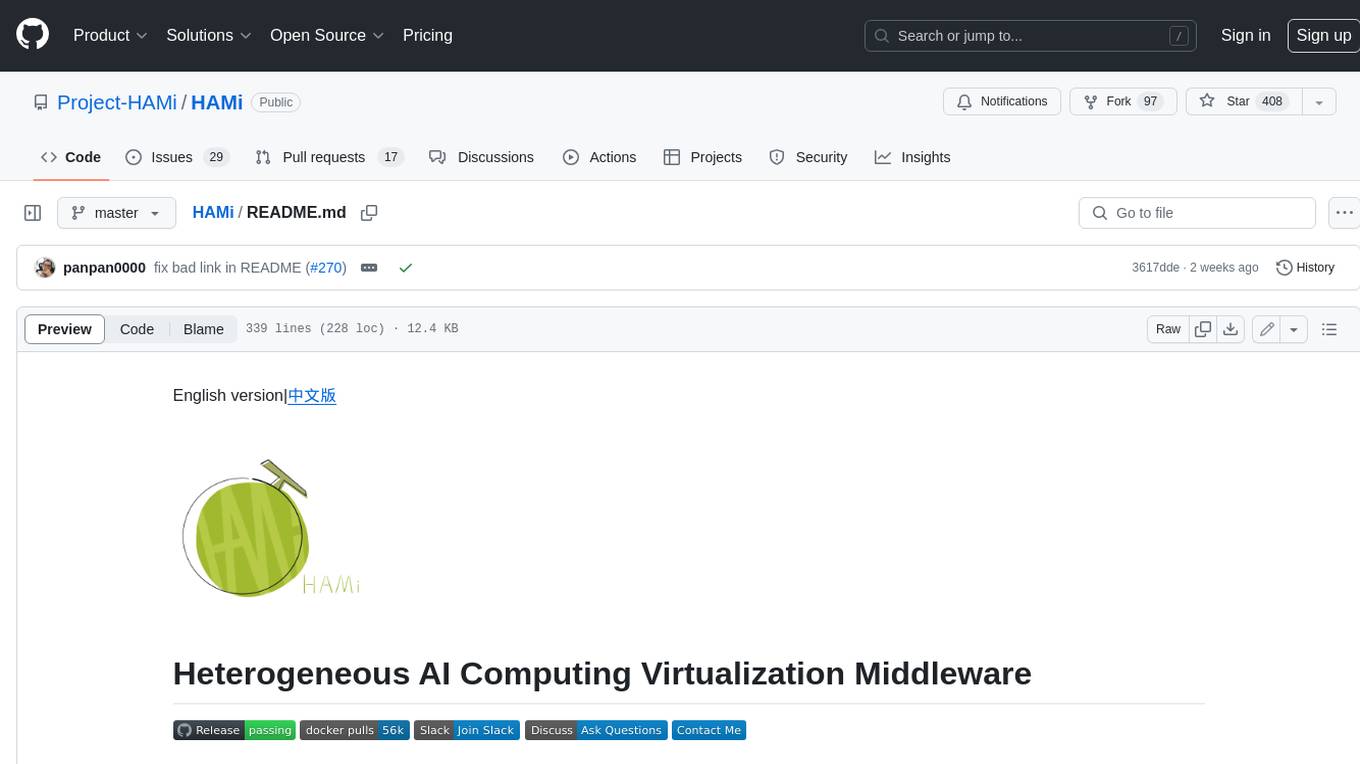
HAMi is a Heterogeneous AI Computing Virtualization Middleware designed to manage Heterogeneous AI Computing Devices in a Kubernetes cluster. It allows for device sharing, device memory control, device type specification, and device UUID specification. The tool is easy to use and does not require modifying task YAML files. It includes features like hard limits on device memory, partial device allocation, streaming multiprocessor limits, and core usage specification. HAMi consists of components like a mutating webhook, scheduler extender, device plugins, and in-container virtualization techniques. It is suitable for scenarios requiring device sharing, specific device memory allocation, GPU balancing, low utilization optimization, and scenarios needing multiple small GPUs. The tool requires prerequisites like NVIDIA drivers, CUDA version, nvidia-docker, Kubernetes version, glibc version, and helm. Users can install, upgrade, and uninstall HAMi, submit tasks, and monitor cluster information. The tool's roadmap includes supporting additional AI computing devices, video codec processing, and Multi-Instance GPUs (MIG).
README:
English version | 中文版
HAMi, formerly known as 'k8s-vGPU-scheduler', is a Heterogeneous device management middleware for Kubernetes. It can manage different types of heterogeneous devices (like GPU, NPU, etc.), share heterogeneous devices among pods, make better scheduling decisions based on topology of devices and scheduling policies.
It aims to remove the gap between different Heterogeneous devices, and provide a unified interface for users to manage with no changes to their applications. As of December 2024, HAMi has been widely used not only in Internet, public cloud and private cloud, but also broadly adopted in various vertical industries including finance, securities, energy, telecommunications, education, and manufacturing. More than 50 companies or institutions are not only end users but also active contributors.
HAMi is a sandbox and landscape project of
Cloud Native Computing Foundation(CNCF),
CNAI Landscape project.
HAMi provides device virtualization for several heterogeneous devices including GPU, by supporting device sharing and device resource isolation. For the list of devices supporting device virtualization, see supported devices
- Allows partial device allocation by specifying device core usage.
- Allows partial device allocation by specifying device memory.
- Imposes a hard limit on streaming multiprocessors.
- Requires zero changes to existing programs.
- Support dynamic-mig feature, example
A simple demonstration of device isolation: A task with the following resources:
resources:
limits:
nvidia.com/gpu: 1 # declare how many physical GPUs the pod needs
nvidia.com/gpumem: 3000 # identifies 3G GPU memory each physical GPU allocates to the pod
will see 3G device memory inside container
Note:
- After installing HAMi, the value of
nvidia.com/gpu
registered on the node defaults to the number of vGPUs. - When requesting resources in a pod,
nvidia.com/gpu
refers to the number of physical GPUs required by the current pod.
HAMi consists of several components, including a unified mutatingwebhook, a unified scheduler extender, different device-plugins and different in-container virtualization technics for each heterogeneous AI devices.
The list of prerequisites for running the NVIDIA device plugin is described below:
- NVIDIA drivers >= 440
- nvidia-docker version > 2.0
- default runtime configured as nvidia for containerd/docker/cri-o container runtime
- Kubernetes version >= 1.16
- glibc >= 2.17 & glibc < 2.3.0
- kernel version >= 3.10
- helm > 3.0
First, Label your GPU nodes for scheduling with HAMi by adding the label "gpu=on". Without this label, the nodes cannot be managed by our scheduler.
kubectl label nodes {nodeid} gpu=on
Add our repo in helm
helm repo add hami-charts https://project-hami.github.io/HAMi/
Use the following command for deployment:
helm install hami hami-charts/hami -n kube-system
Customize your installation by adjusting the configs.
Verify your installation using the following command:
kubectl get pods -n kube-system
If both vgpu-device-plugin
and vgpu-scheduler
pods are in the Running state, your installation is successful. You can try examples here
HAMi-WebUI is available after HAMi v2.4
For installation guide, click here
Monitoring is automatically enabled after installation. Obtain an overview of cluster information by visiting the following URL:
http://{scheduler ip}:{monitorPort}/metrics
The default monitorPort is 31993; other values can be set using --set devicePlugin.service.httpPort
during installation.
Grafana dashboard example
Note The status of a node won't be collected before you submit a task
- If you don't request vGPUs when using the device plugin with NVIDIA images all the GPUs on the machine may be exposed inside your container
- Currently, A100 MIG can be supported in only "none" and "mixed" modes.
- Tasks with the "nodeName" field cannot be scheduled at the moment; please use "nodeSelector" instead.
The project is governed by a group of Maintainers and Contributors. How they are selected and govern is outlined in our Governance Document.
If you're interested in being a contributor and want to get involved in developing the HAMi code, please see CONTRIBUTING for details on submitting patches and the contribution workflow.
See RoadMap to see anything you interested.
The HAMi community is committed to fostering an open and welcoming environment, with several ways to engage with other users and developers.
If you have any questions, please feel free to reach out to us through the following channels:
- Regular Community Meeting: Friday at 16:00 UTC+8 (Chinese)(weekly). Convert to your timezone.
- Email: refer to the MAINTAINERS.md to find the email addresses of all maintainers. Feel free to contact them via email to report any issues or ask questions.
- mailing list
- slack | Join
Link | |
---|---|
CHINA CLOUD COMPUTING INFRASTRUCTURE DEVELOPER CONFERENCE (Beijing 2024) | Unlocking heterogeneous AI infrastructure on k8s clusters Starting from 03:06:15 |
KubeDay(Japan 2024) | Unlocking Heterogeneous AI Infrastructure K8s Cluster:Leveraging the Power of HAMi |
KubeCon & AI_dev Open Source GenAI & ML Summit(China 2024) | Is Your GPU Really Working Efficiently in the Data Center?N Ways to Improve GPU Usage |
KubeCon & AI_dev Open Source GenAI & ML Summit(China 2024) | Unlocking Heterogeneous AI Infrastructure K8s Cluster |
KubeCon(EU 2024) | Cloud Native Batch Computing with Volcano: Updates and Future |
HAMi is under the Apache 2.0 license. See the LICENSE file for details.
For Tasks:
Click tags to check more tools for each tasksFor Jobs:
Alternative AI tools for HAMi
Similar Open Source Tools
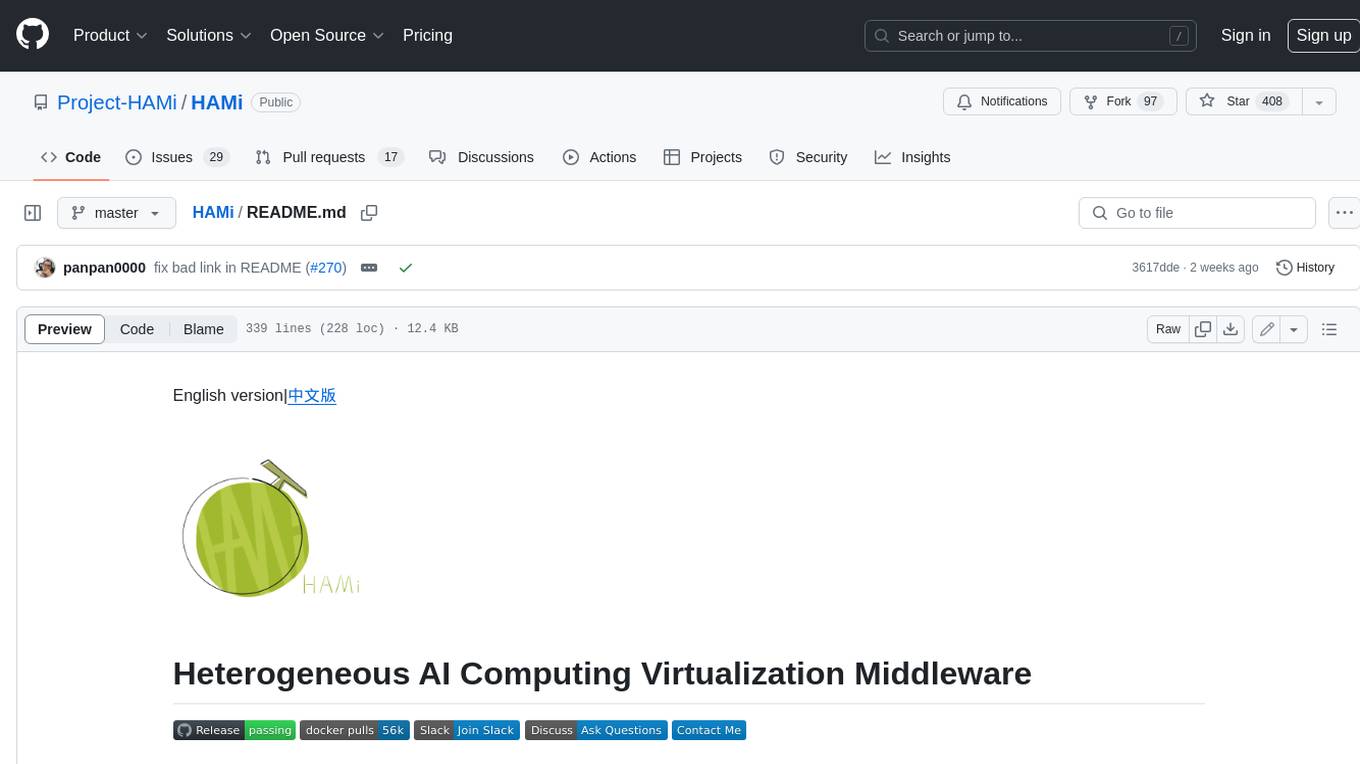
HAMi
HAMi is a Heterogeneous AI Computing Virtualization Middleware designed to manage Heterogeneous AI Computing Devices in a Kubernetes cluster. It allows for device sharing, device memory control, device type specification, and device UUID specification. The tool is easy to use and does not require modifying task YAML files. It includes features like hard limits on device memory, partial device allocation, streaming multiprocessor limits, and core usage specification. HAMi consists of components like a mutating webhook, scheduler extender, device plugins, and in-container virtualization techniques. It is suitable for scenarios requiring device sharing, specific device memory allocation, GPU balancing, low utilization optimization, and scenarios needing multiple small GPUs. The tool requires prerequisites like NVIDIA drivers, CUDA version, nvidia-docker, Kubernetes version, glibc version, and helm. Users can install, upgrade, and uninstall HAMi, submit tasks, and monitor cluster information. The tool's roadmap includes supporting additional AI computing devices, video codec processing, and Multi-Instance GPUs (MIG).
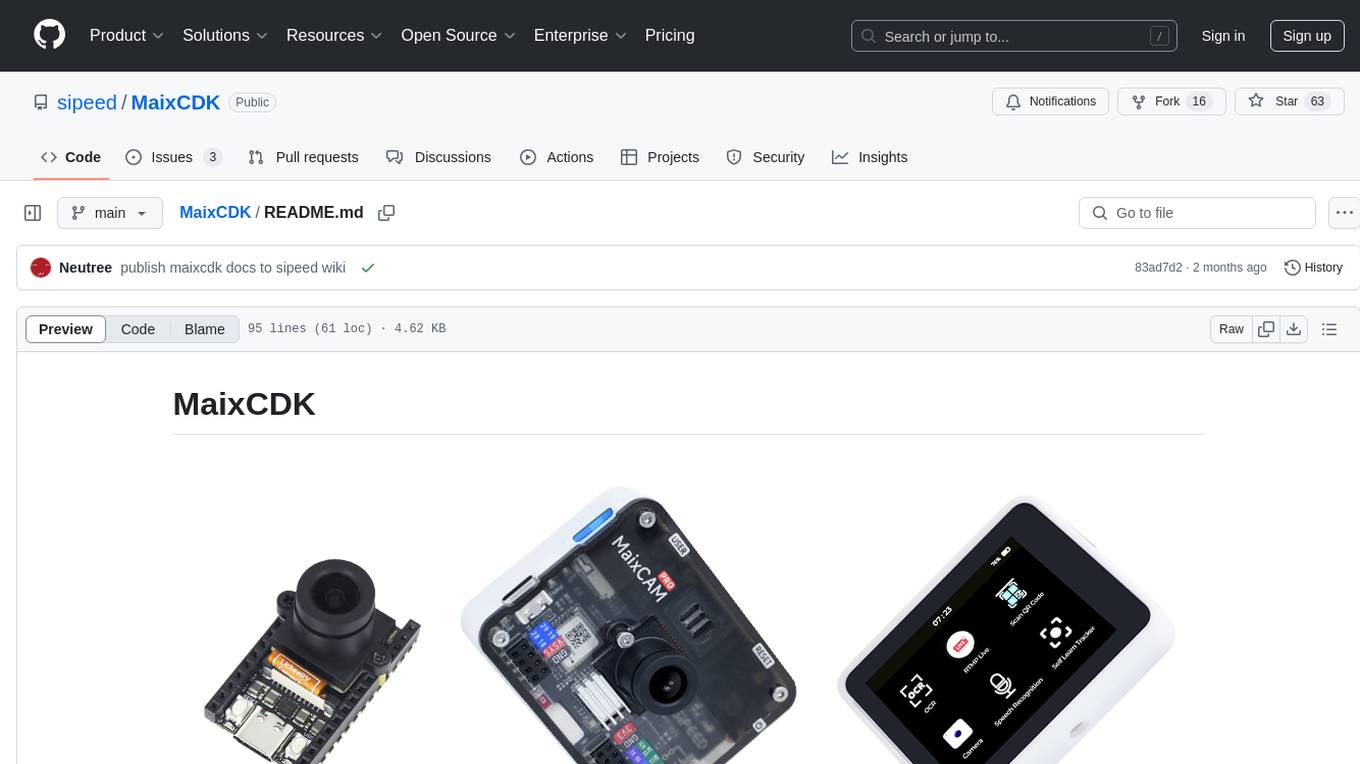
MaixCDK
MaixCDK (Maix C/CPP Development Kit) is a C/C++ development kit that integrates practical functions such as AI, machine vision, and IoT. It provides easy-to-use encapsulation for quickly building projects in vision, artificial intelligence, IoT, robotics, industrial cameras, and more. It supports hardware-accelerated execution of AI models, common vision algorithms, OpenCV, and interfaces for peripheral operations. MaixCDK offers cross-platform support, easy-to-use API, simple environment setup, online debugging, and a complete ecosystem including MaixPy and MaixVision. Supported devices include Sipeed MaixCAM, Sipeed MaixCAM-Pro, and partial support for Common Linux.
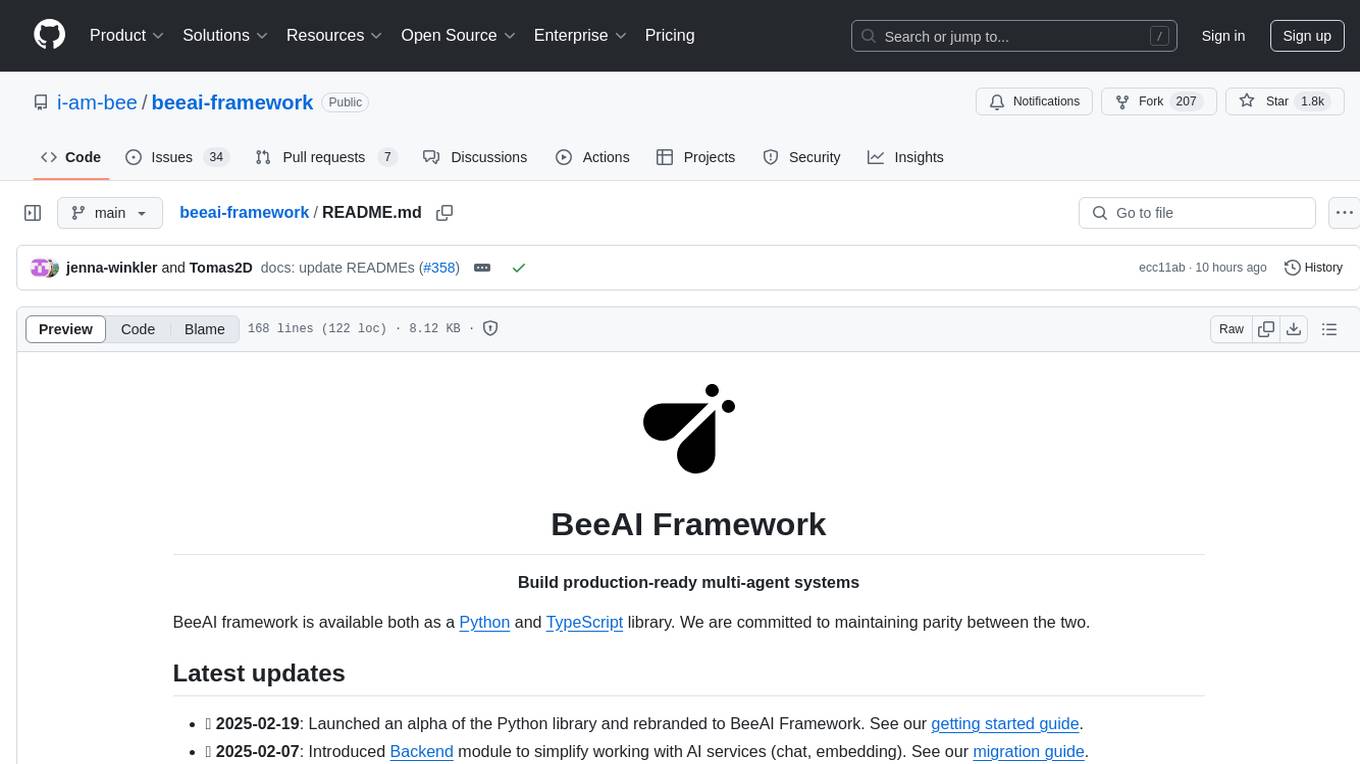
beeai-framework
BeeAI Framework is a versatile tool for building production-ready multi-agent systems. It offers flexibility in orchestrating agents, seamless integration with various models and tools, and production-grade controls for scaling. The framework supports Python and TypeScript libraries, enabling users to implement simple to complex multi-agent patterns, connect with AI services, and optimize token usage and resource management.
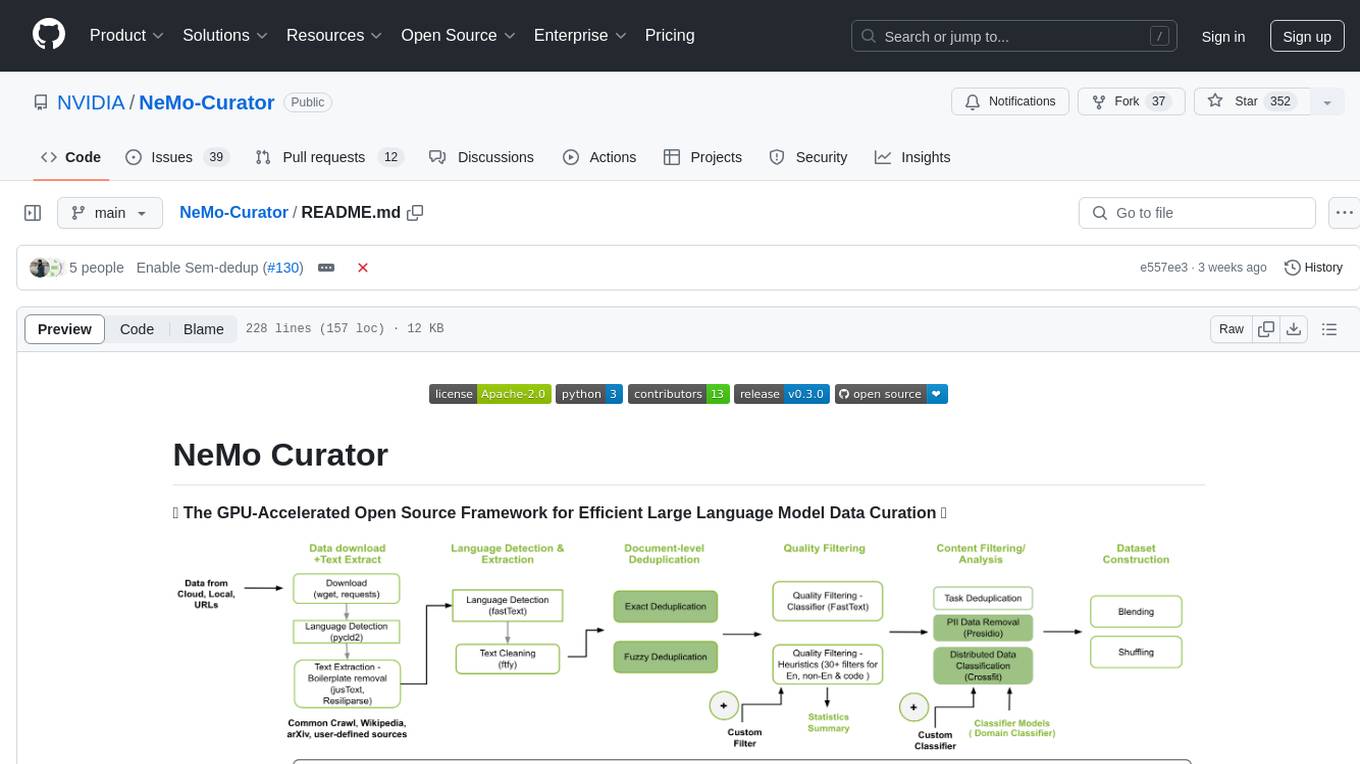
NeMo-Curator
NeMo Curator is a GPU-accelerated open-source framework designed for efficient large language model data curation. It provides scalable dataset preparation for tasks like foundation model pretraining, domain-adaptive pretraining, supervised fine-tuning, and parameter-efficient fine-tuning. The library leverages GPUs with Dask and RAPIDS to accelerate data curation, offering customizable and modular interfaces for pipeline expansion and model convergence. Key features include data download, text extraction, quality filtering, deduplication, downstream-task decontamination, distributed data classification, and PII redaction. NeMo Curator is suitable for curating high-quality datasets for large language model training.
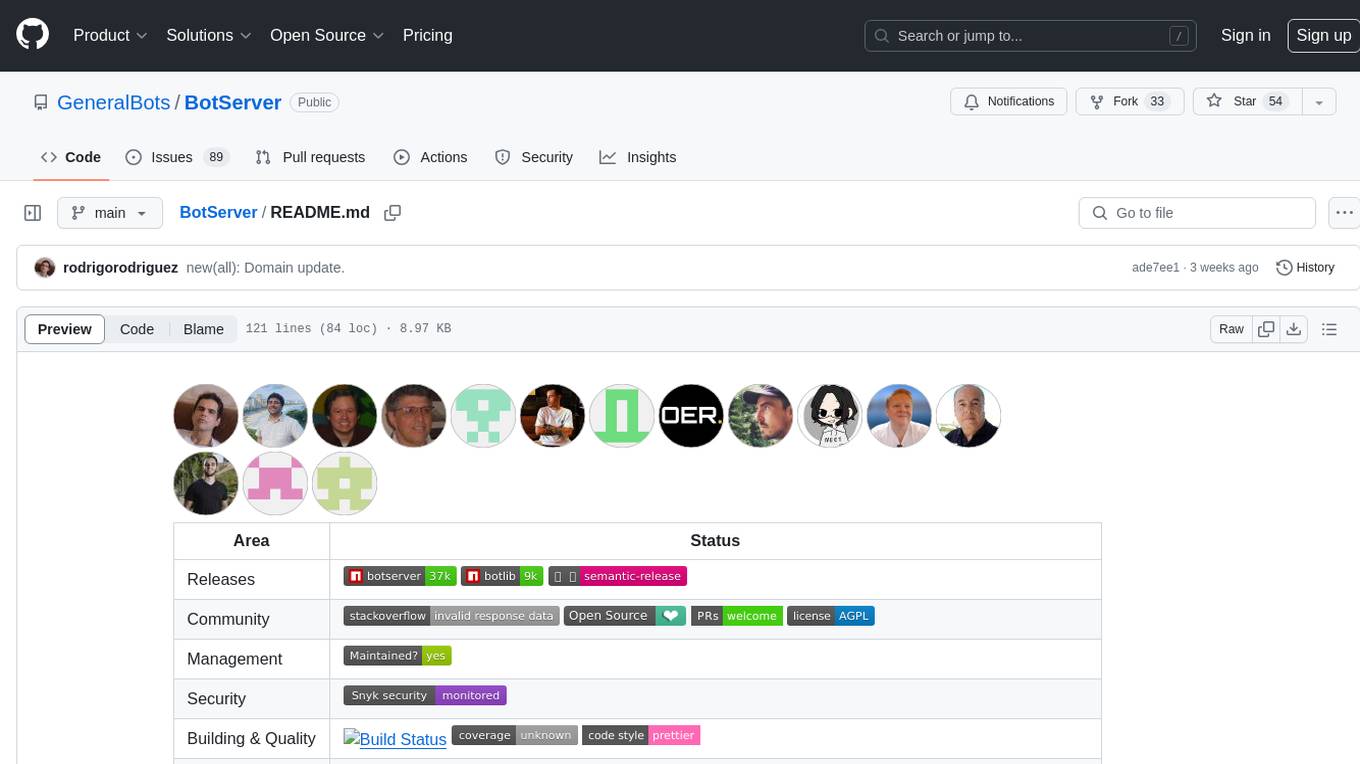
BotServer
General Bot is a chat bot server that accelerates bot development by providing code base, resources, deployment to the cloud, and templates for creating new bots. It allows modification of bot packages without code through a database and service backend. Users can develop bot packages using custom code in editors like Visual Studio Code, Atom, or Brackets. The tool supports creating bots by copying and pasting files and using favorite tools from Office or Photoshop. It also enables building custom dialogs with BASIC for extending bots.
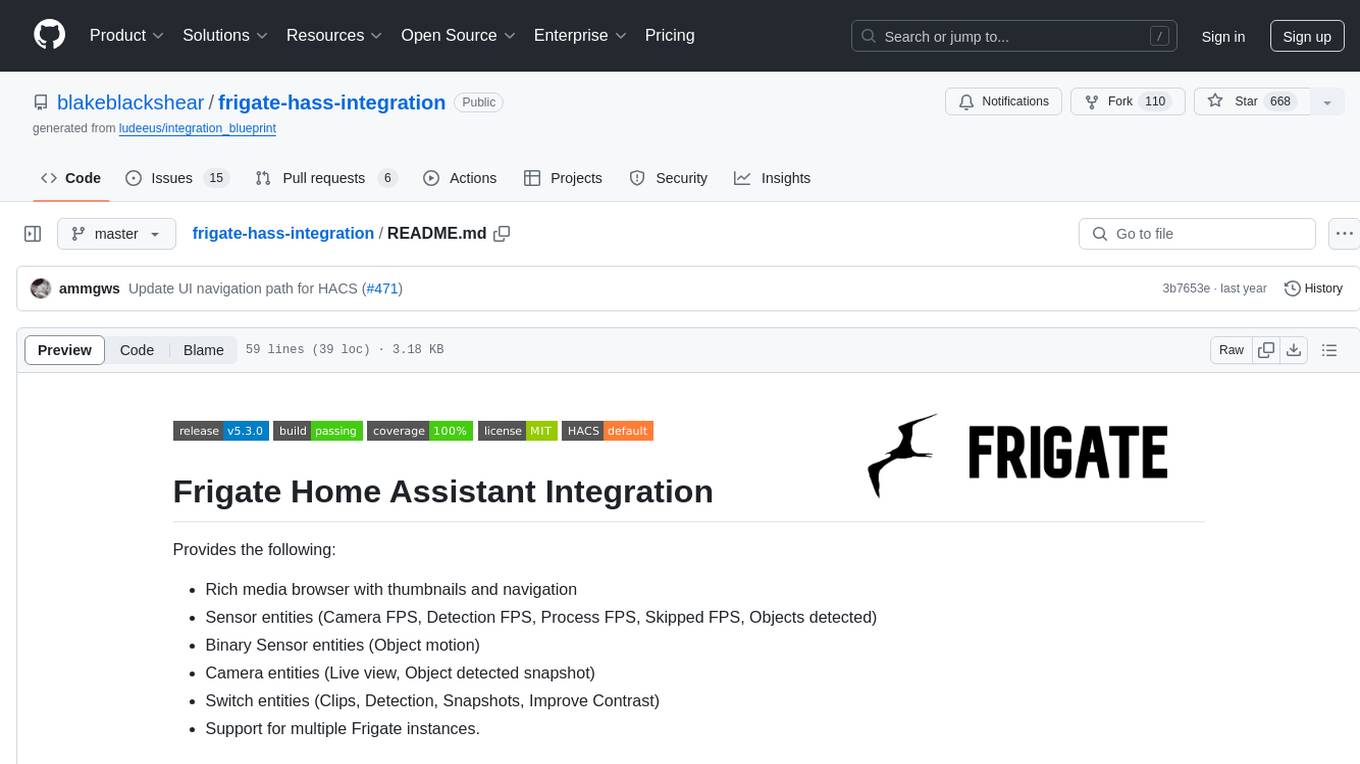
frigate-hass-integration
Frigate Home Assistant Integration provides a rich media browser with thumbnails and navigation, sensor entities for camera FPS, detection FPS, process FPS, skipped FPS, and objects detected, binary sensor entities for object motion, camera entities for live view and object detected snapshot, switch entities for clips, detection, snapshots, and improve contrast, and support for multiple Frigate instances. It offers easy installation via HACS and manual installation options for advanced users. Users need to configure the `mqtt` integration for Frigate to work. Additionally, media browsing and a companion Lovelace card are available for enhanced user experience. Refer to the main Frigate documentation for detailed installation instructions and usage guidance.
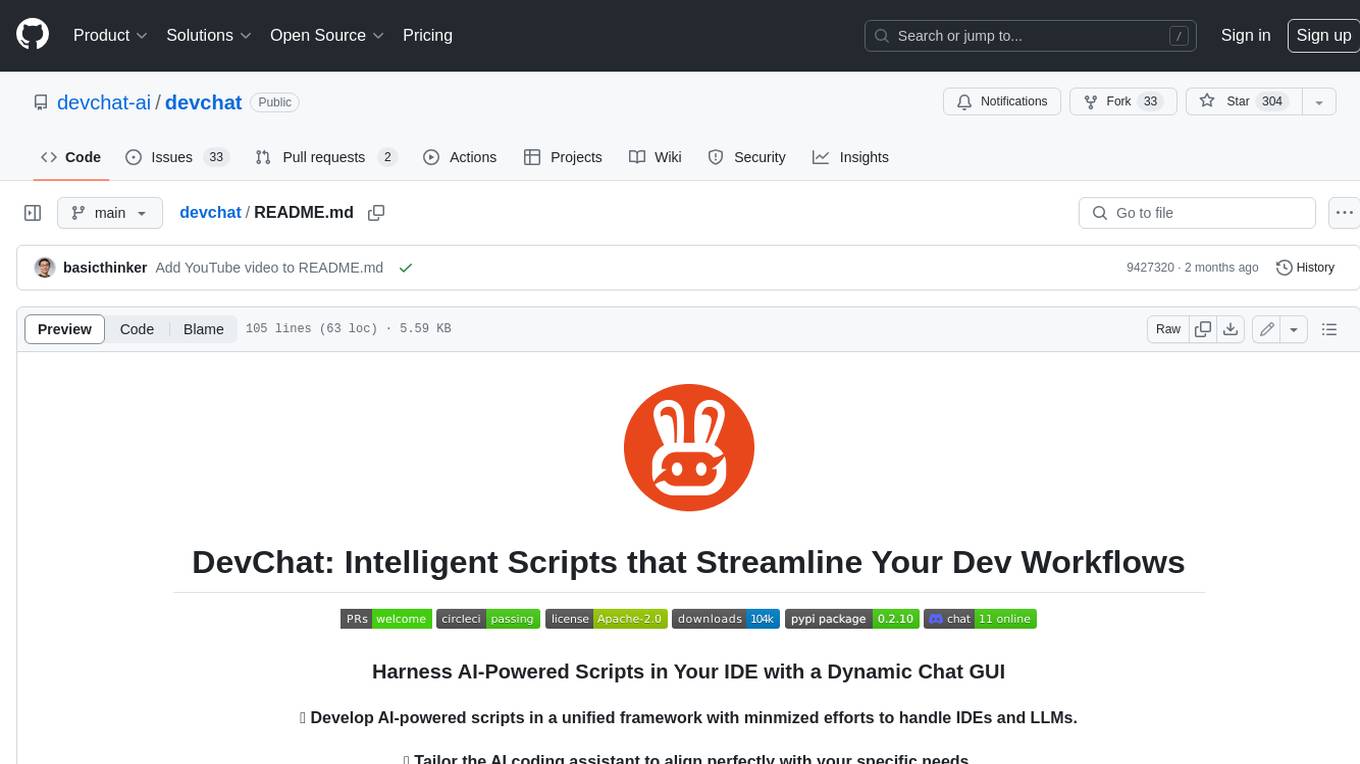
devchat
DevChat is an open-source workflow engine that enables developers to create intelligent, automated workflows for engaging with users through a chat panel within their IDEs. It combines script writing flexibility, latest AI models, and an intuitive chat GUI to enhance user experience and productivity. DevChat simplifies the integration of AI in software development, unlocking new possibilities for developers.
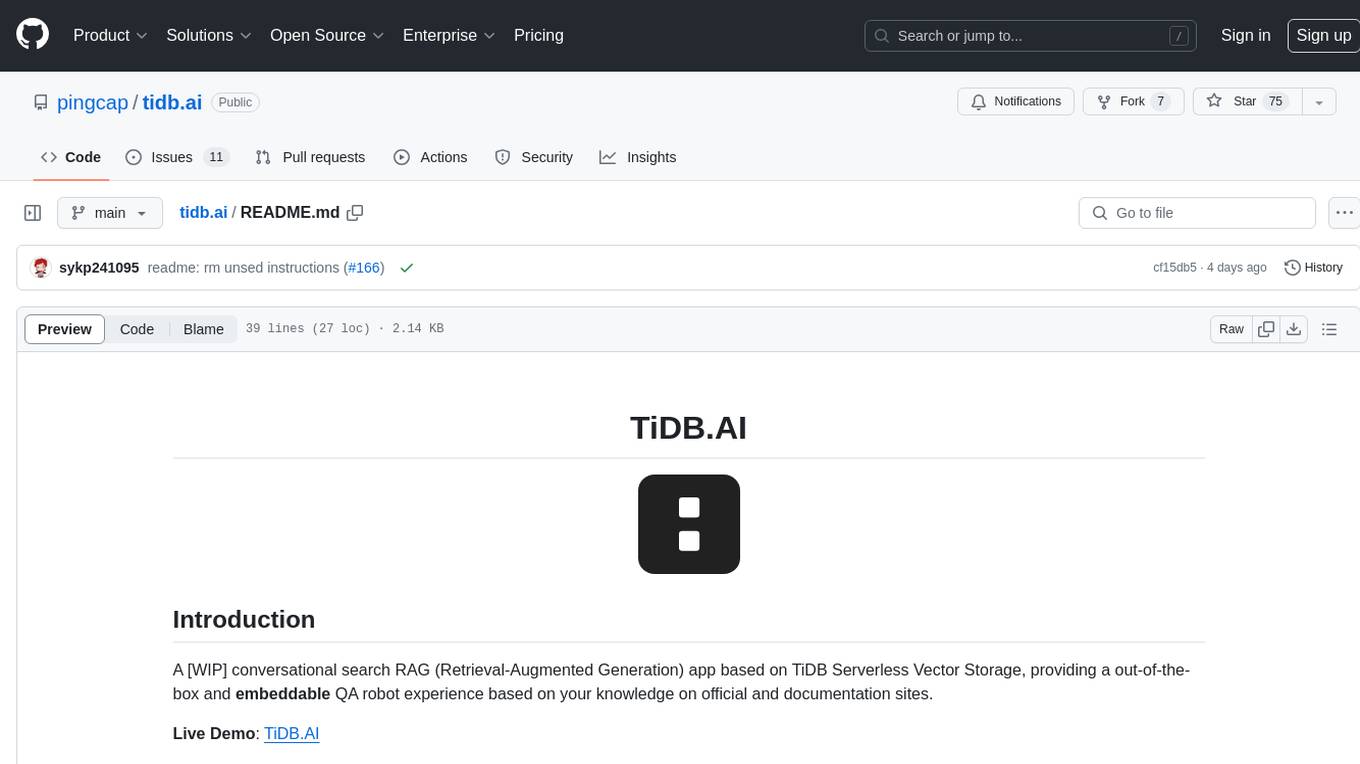
tidb.ai
TiDB.AI is a conversational search RAG (Retrieval-Augmented Generation) app based on TiDB Serverless Vector Storage. It provides an out-of-the-box and embeddable QA robot experience based on knowledge from official and documentation sites. The platform features a Perplexity-style Conversational Search page with an advanced built-in website crawler for comprehensive coverage. Users can integrate an embeddable JavaScript snippet into their website for instant responses to product-related queries. The tech stack includes Next.js, TypeScript, Tailwind CSS, shadcn/ui for design, TiDB for database storage, Kysely for SQL query building, NextAuth.js for authentication, Vercel for deployments, and LlamaIndex for the RAG framework. TiDB.AI is open-source under the Apache License, Version 2.0.
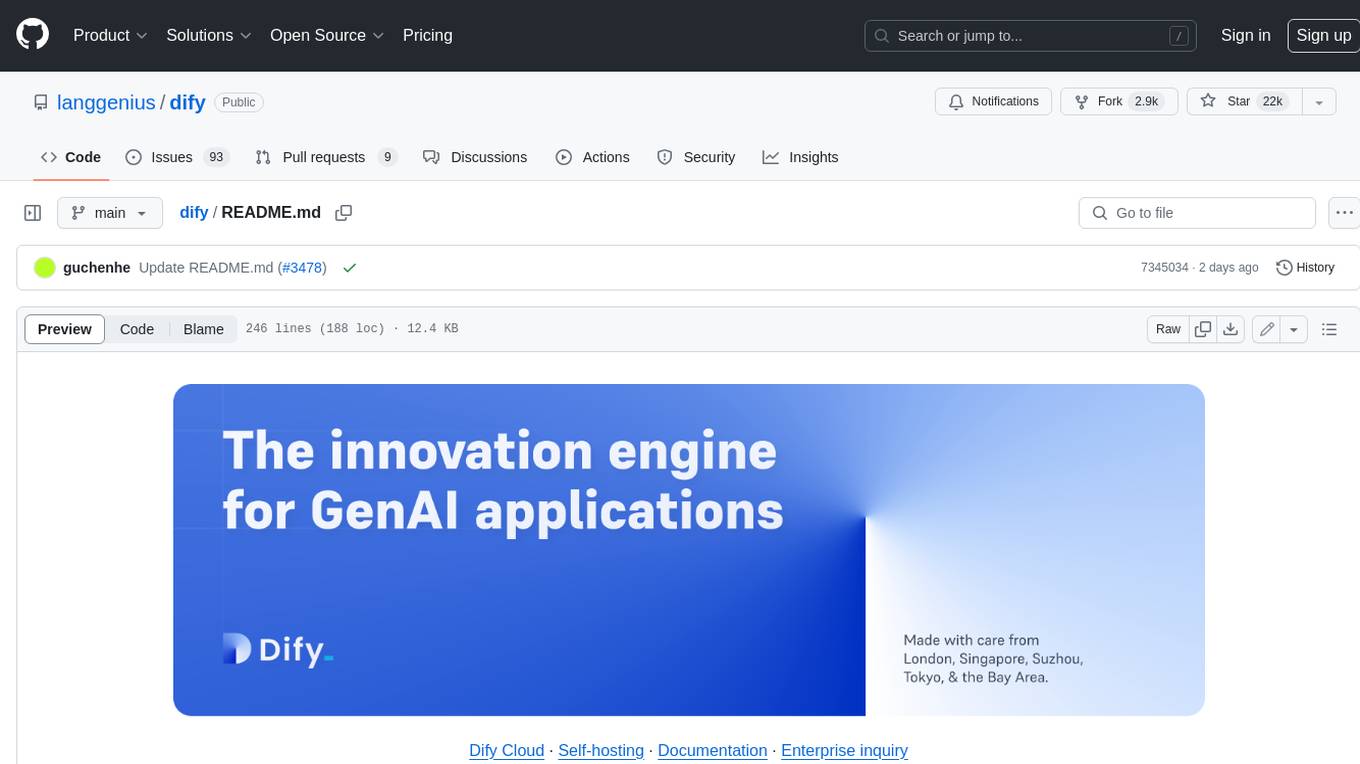
dify
Dify is an open-source LLM app development platform that combines AI workflow, RAG pipeline, agent capabilities, model management, observability features, and more. It allows users to quickly go from prototype to production. Key features include: 1. Workflow: Build and test powerful AI workflows on a visual canvas. 2. Comprehensive model support: Seamless integration with hundreds of proprietary / open-source LLMs from dozens of inference providers and self-hosted solutions. 3. Prompt IDE: Intuitive interface for crafting prompts, comparing model performance, and adding additional features. 4. RAG Pipeline: Extensive RAG capabilities that cover everything from document ingestion to retrieval. 5. Agent capabilities: Define agents based on LLM Function Calling or ReAct, and add pre-built or custom tools. 6. LLMOps: Monitor and analyze application logs and performance over time. 7. Backend-as-a-Service: All of Dify's offerings come with corresponding APIs for easy integration into your own business logic.
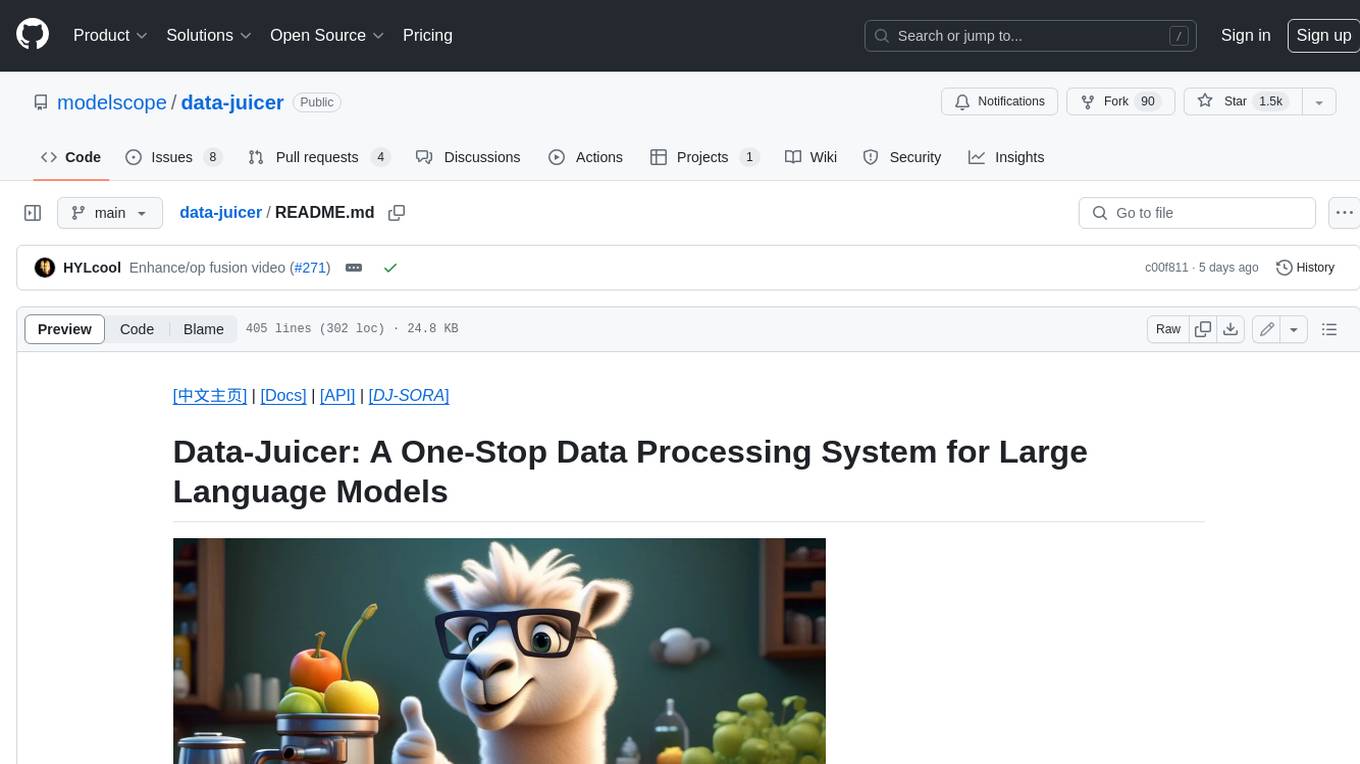
data-juicer
Data-Juicer is a one-stop data processing system to make data higher-quality, juicier, and more digestible for LLMs. It is a systematic & reusable library of 80+ core OPs, 20+ reusable config recipes, and 20+ feature-rich dedicated toolkits, designed to function independently of specific LLM datasets and processing pipelines. Data-Juicer allows detailed data analyses with an automated report generation feature for a deeper understanding of your dataset. Coupled with multi-dimension automatic evaluation capabilities, it supports a timely feedback loop at multiple stages in the LLM development process. Data-Juicer offers tens of pre-built data processing recipes for pre-training, fine-tuning, en, zh, and more scenarios. It provides a speedy data processing pipeline requiring less memory and CPU usage, optimized for maximum productivity. Data-Juicer is flexible & extensible, accommodating most types of data formats and allowing flexible combinations of OPs. It is designed for simplicity, with comprehensive documentation, easy start guides and demo configs, and intuitive configuration with simple adding/removing OPs from existing configs.
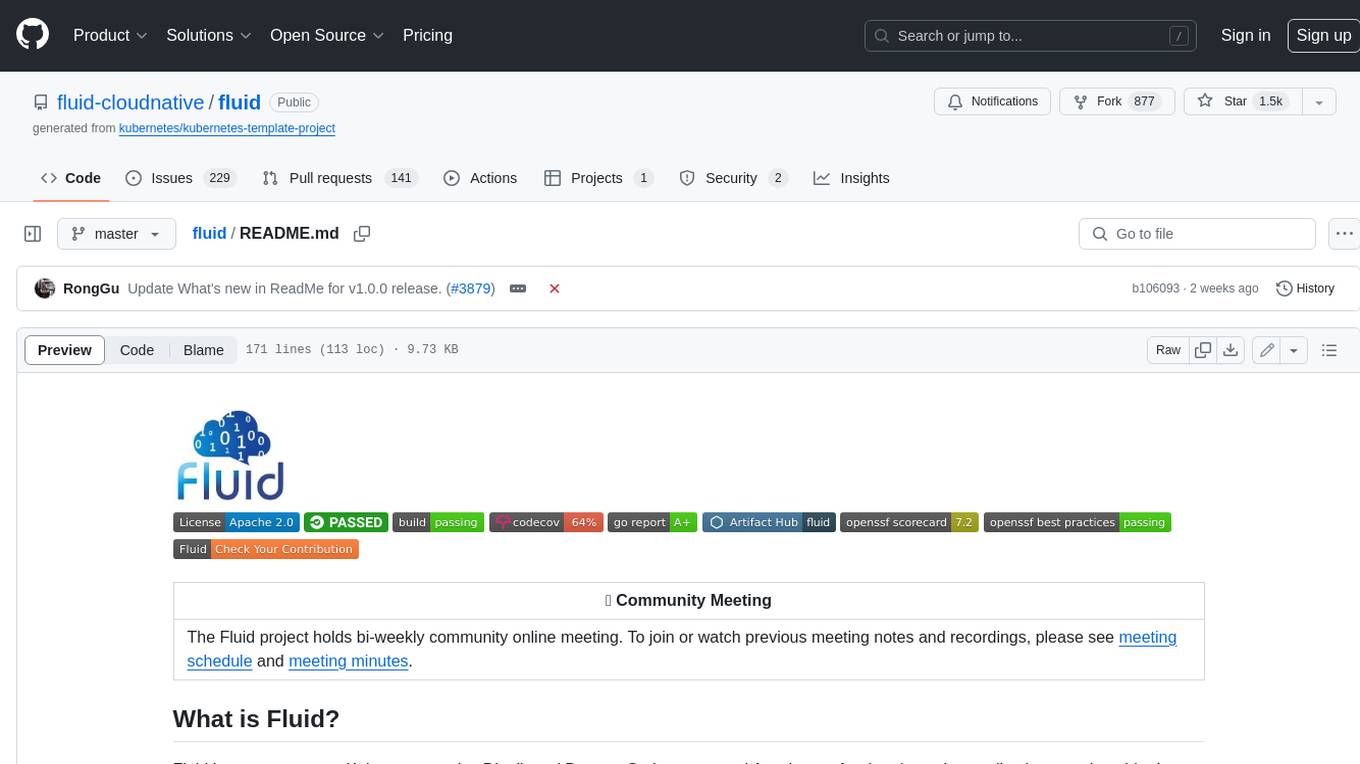
fluid
Fluid is an open source Kubernetes-native Distributed Dataset Orchestrator and Accelerator for data-intensive applications, such as big data and AI applications. It implements dataset abstraction, scalable cache runtime, automated data operations, elasticity and scheduling, and is runtime platform agnostic. Key concepts include Dataset and Runtime. Prerequisites include Kubernetes version > 1.16, Golang 1.18+, and Helm 3. The tool offers features like accelerating remote file accessing, machine learning, accelerating PVC, preloading dataset, and on-the-fly dataset cache scaling. Contributions are welcomed, and the project is under the Apache 2.0 license with a vendor-neutral approach.
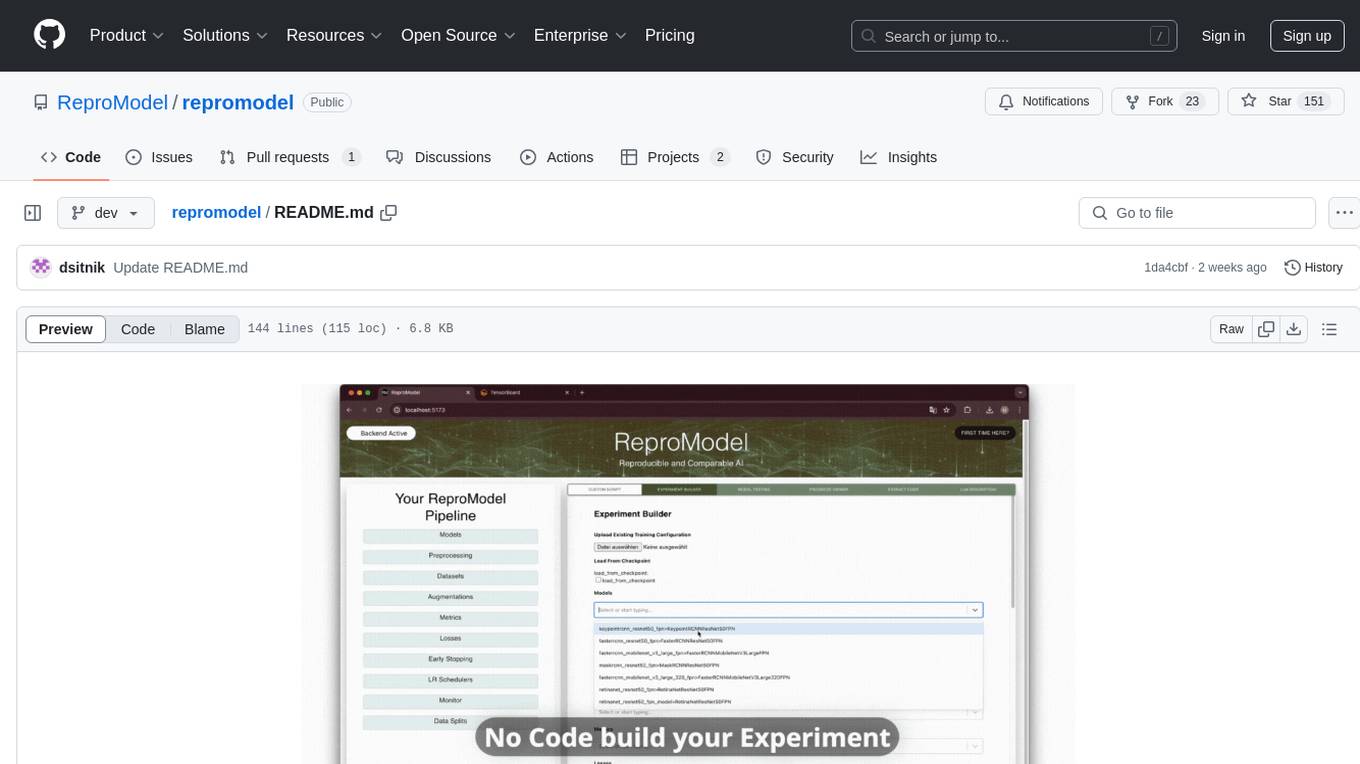
repromodel
ReproModel is an open-source toolbox designed to boost AI research efficiency by enabling researchers to reproduce, compare, train, and test AI models faster. It provides standardized models, dataloaders, and processing procedures, allowing researchers to focus on new datasets and model development. With a no-code solution, users can access benchmark and SOTA models and datasets, utilize training visualizations, extract code for publication, and leverage an LLM-powered automated methodology description writer. The toolbox helps researchers modularize development, compare pipeline performance reproducibly, and reduce time for model development, computation, and writing. Future versions aim to facilitate building upon state-of-the-art research by loading previously published study IDs with verified code, experiments, and results stored in the system.
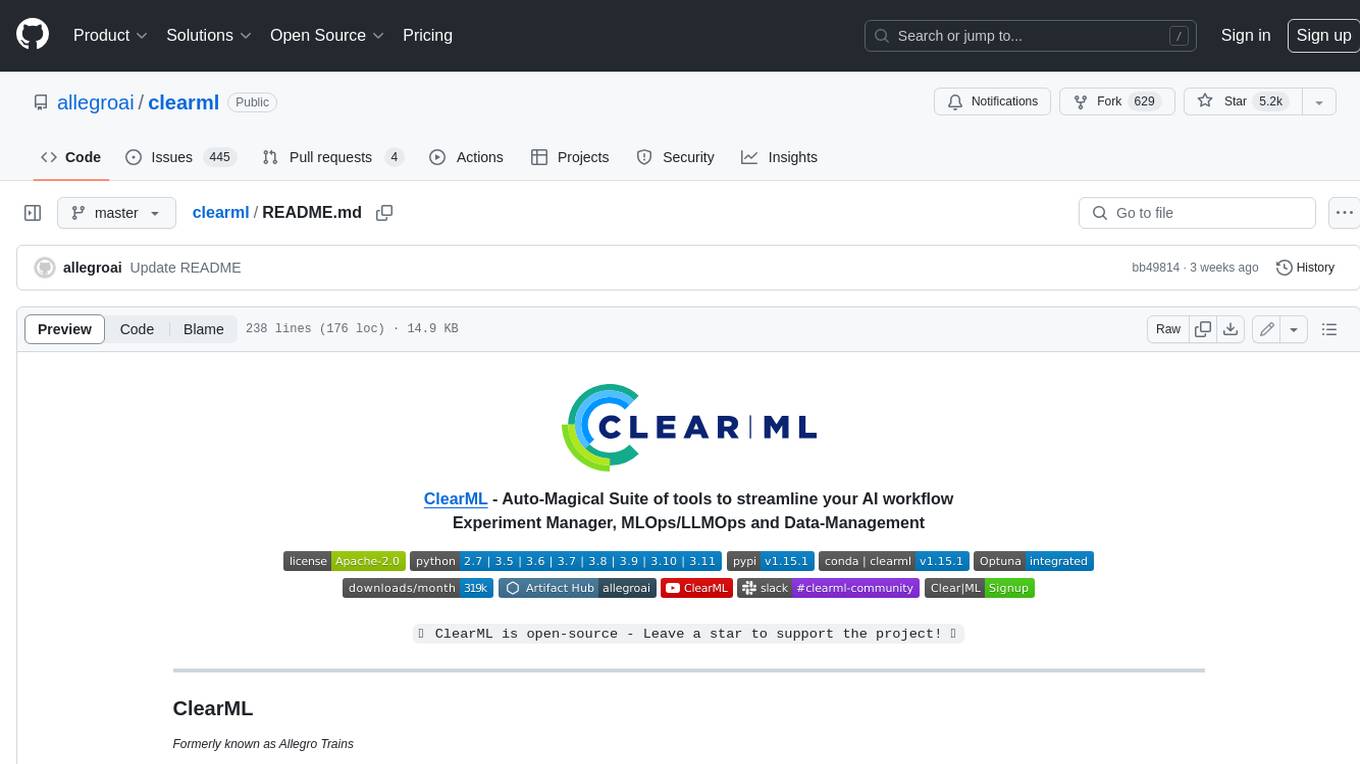
clearml
ClearML is a suite of tools designed to streamline the machine learning workflow. It includes an experiment manager, MLOps/LLMOps, data management, and model serving capabilities. ClearML is open-source and offers a free tier hosting option. It supports various ML/DL frameworks and integrates with Jupyter Notebook and PyCharm. ClearML provides extensive logging capabilities, including source control info, execution environment, hyper-parameters, and experiment outputs. It also offers automation features, such as remote job execution and pipeline creation. ClearML is designed to be easy to integrate, requiring only two lines of code to add to existing scripts. It aims to improve collaboration, visibility, and data transparency within ML teams.
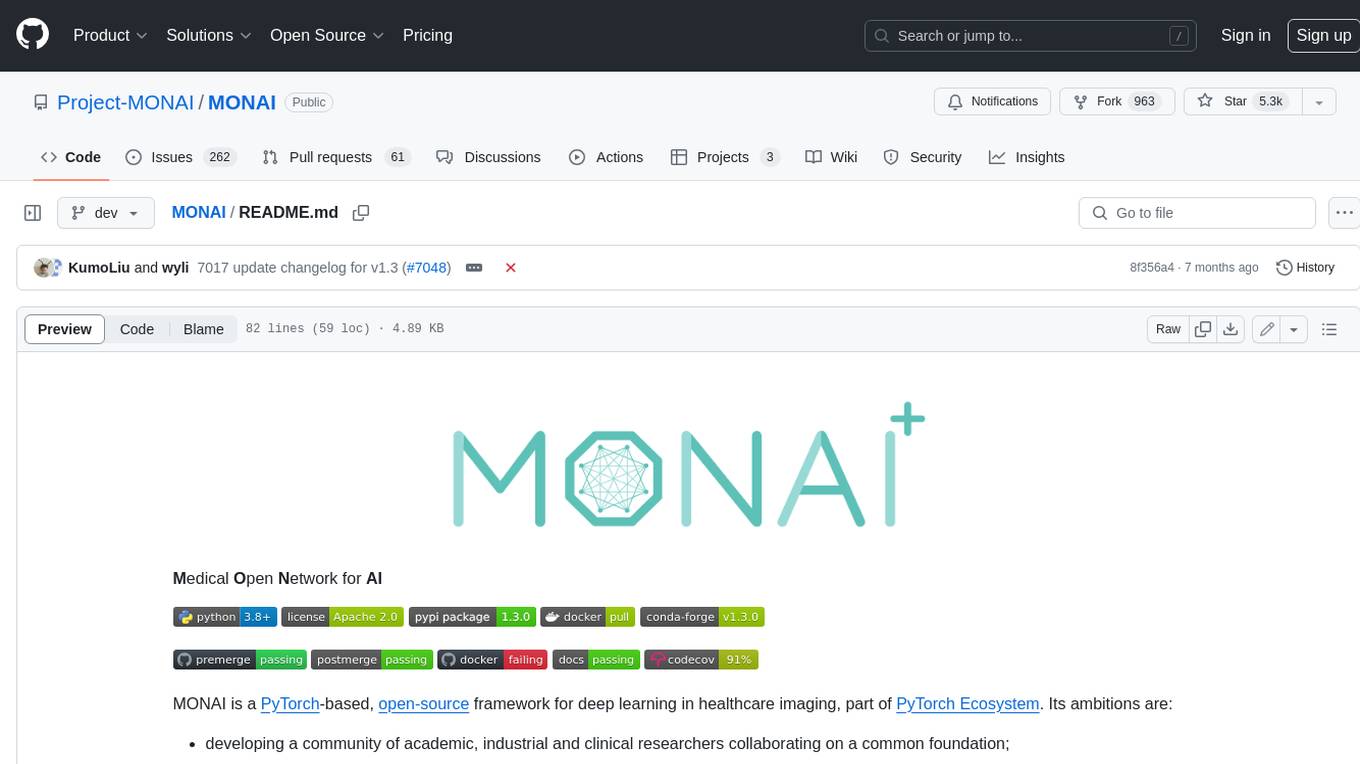
MONAI
MONAI is a PyTorch-based, open-source framework for deep learning in healthcare imaging. It provides a comprehensive set of tools for medical image analysis, including data preprocessing, model training, and evaluation. MONAI is designed to be flexible and easy to use, making it a valuable resource for researchers and developers in the field of medical imaging.
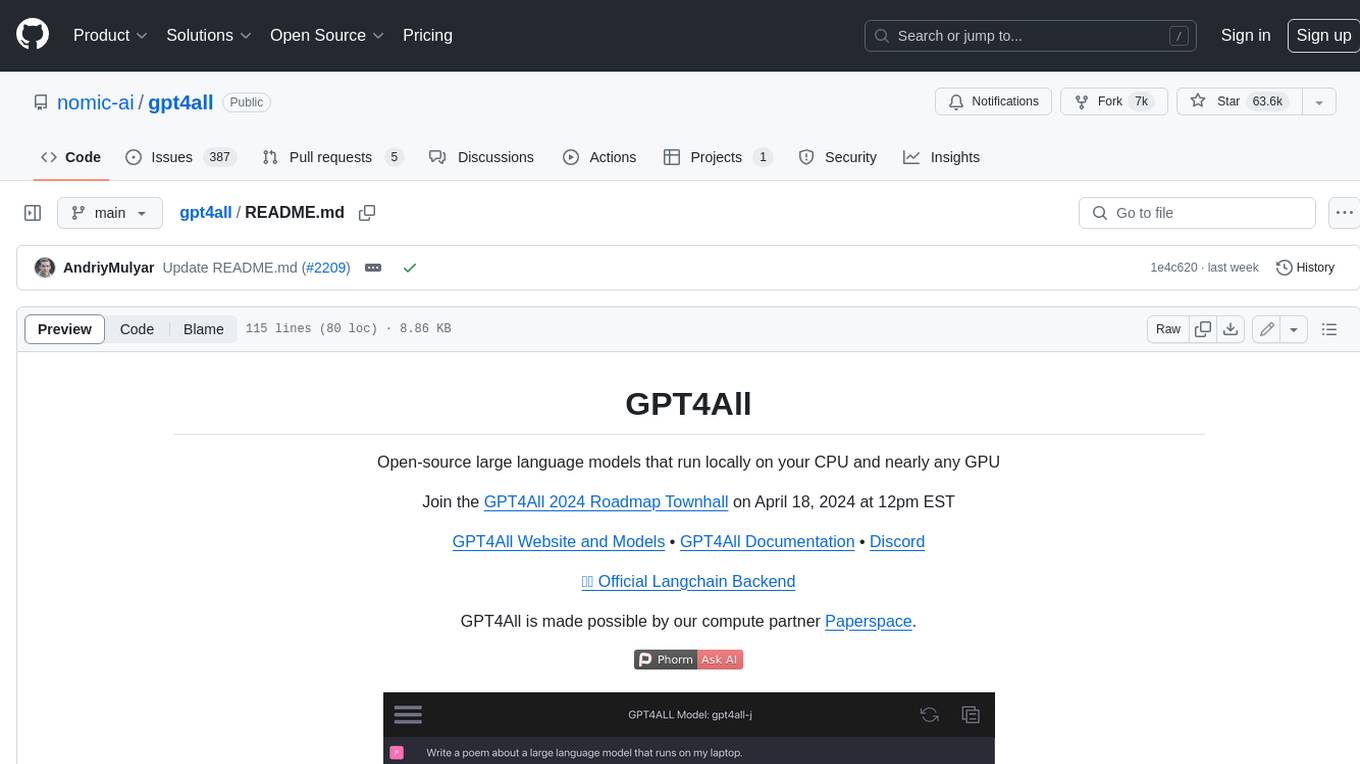
gpt4all
GPT4All is an ecosystem to run powerful and customized large language models that work locally on consumer grade CPUs and any GPU. Note that your CPU needs to support AVX or AVX2 instructions. Learn more in the documentation. A GPT4All model is a 3GB - 8GB file that you can download and plug into the GPT4All open-source ecosystem software. Nomic AI supports and maintains this software ecosystem to enforce quality and security alongside spearheading the effort to allow any person or enterprise to easily train and deploy their own on-edge large language models.
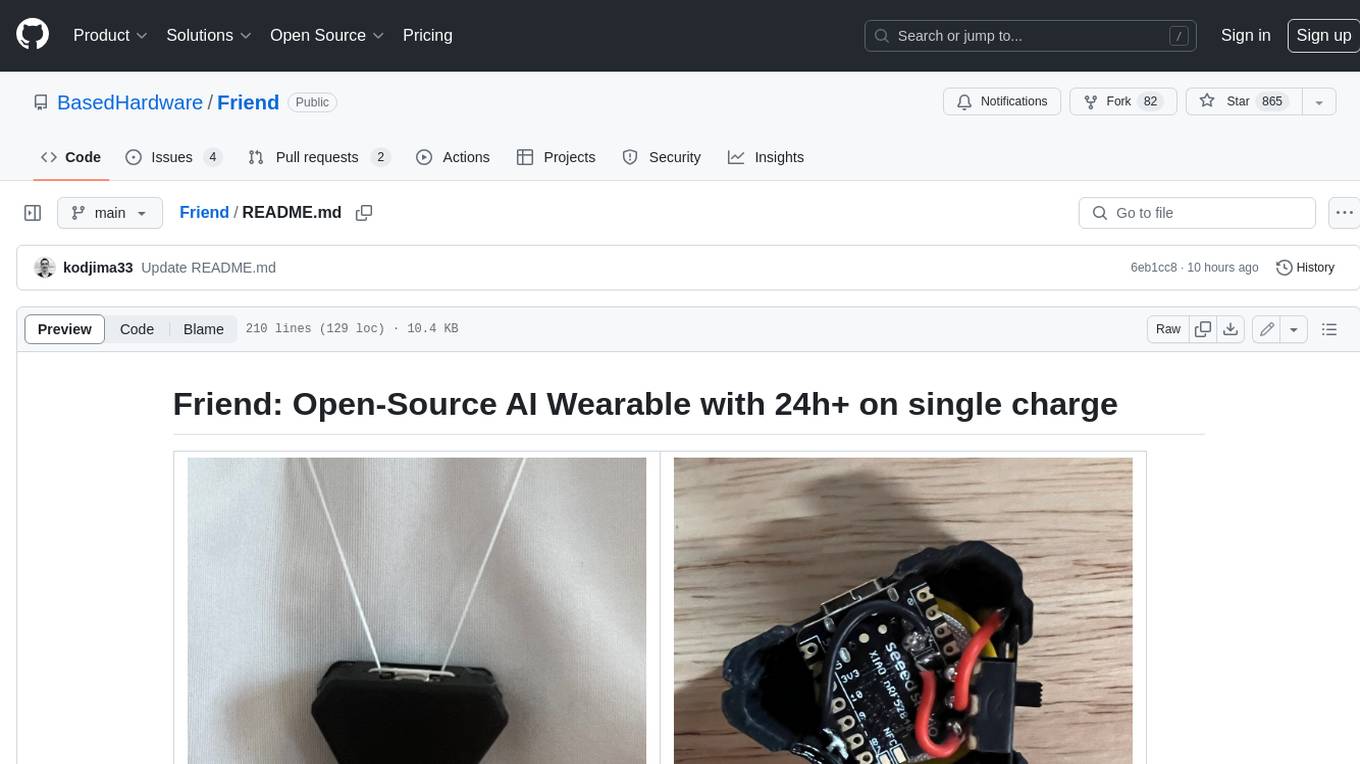
Friend
Friend is an open-source AI wearable device that records everything you say, gives you proactive feedback and advice. It has real-time AI audio processing capabilities, low-powered Bluetooth, open-source software, and a wearable design. The device is designed to be affordable and easy to use, with a total cost of less than $20. To get started, you can clone the repo, choose the version of the app you want to install, and follow the instructions for installing the firmware and assembling the device. Friend is still a prototype project and is provided "as is", without warranty of any kind. Use of the device should comply with all local laws and regulations concerning privacy and data protection.
For similar tasks
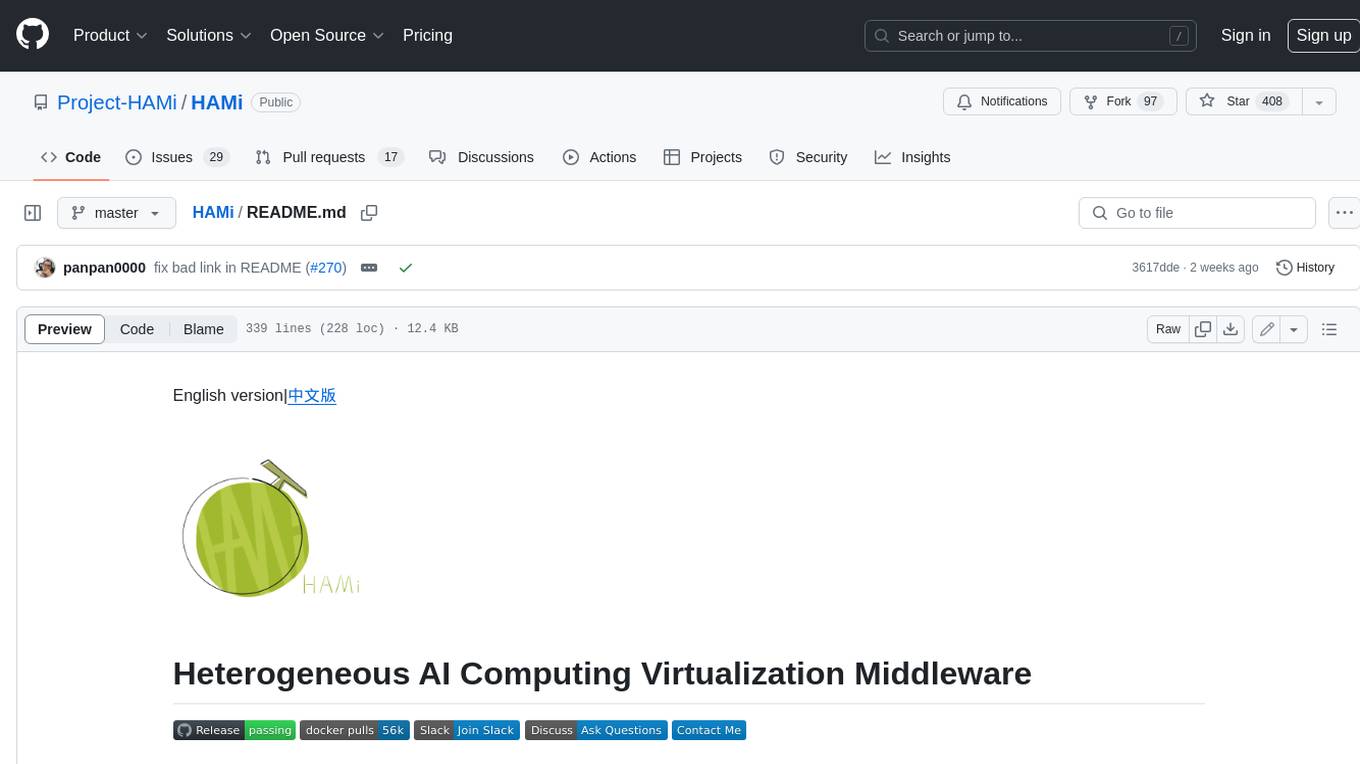
HAMi
HAMi is a Heterogeneous AI Computing Virtualization Middleware designed to manage Heterogeneous AI Computing Devices in a Kubernetes cluster. It allows for device sharing, device memory control, device type specification, and device UUID specification. The tool is easy to use and does not require modifying task YAML files. It includes features like hard limits on device memory, partial device allocation, streaming multiprocessor limits, and core usage specification. HAMi consists of components like a mutating webhook, scheduler extender, device plugins, and in-container virtualization techniques. It is suitable for scenarios requiring device sharing, specific device memory allocation, GPU balancing, low utilization optimization, and scenarios needing multiple small GPUs. The tool requires prerequisites like NVIDIA drivers, CUDA version, nvidia-docker, Kubernetes version, glibc version, and helm. Users can install, upgrade, and uninstall HAMi, submit tasks, and monitor cluster information. The tool's roadmap includes supporting additional AI computing devices, video codec processing, and Multi-Instance GPUs (MIG).
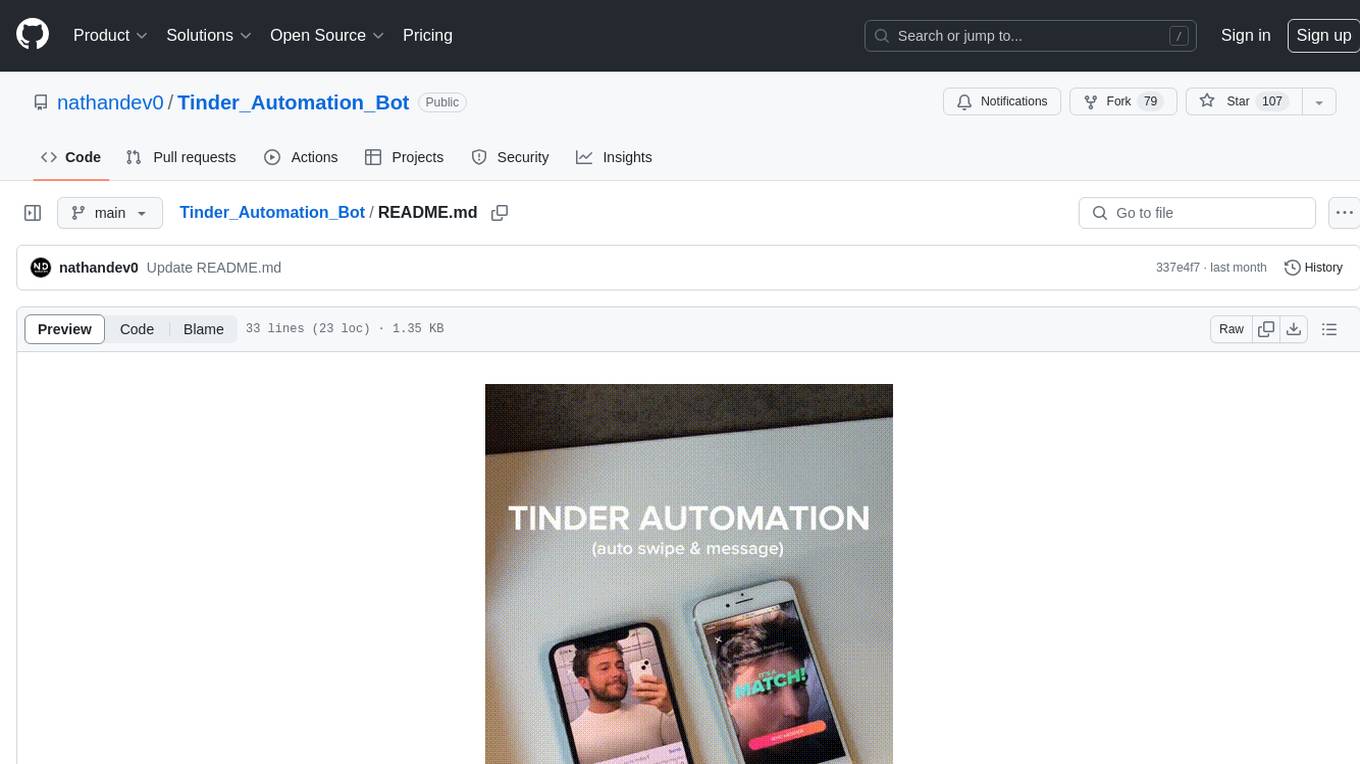
Tinder_Automation_Bot
Tinder Automation Bot is an Appium-based tool designed for automated Tinder account creation and swiping on real devices. It offers functionalities such as automated account creation and swiping, along with integrations like Crane tweak and SMSPool service. The tool also provides features like device and automation management system, anti-bot system for human behavior modeling, IP rotation system for different IP addresses, and GPS location spoofing for different GPS coordinates. It is part of a series of automation bots including TikTok, Bumble, and Badoo automation bots.
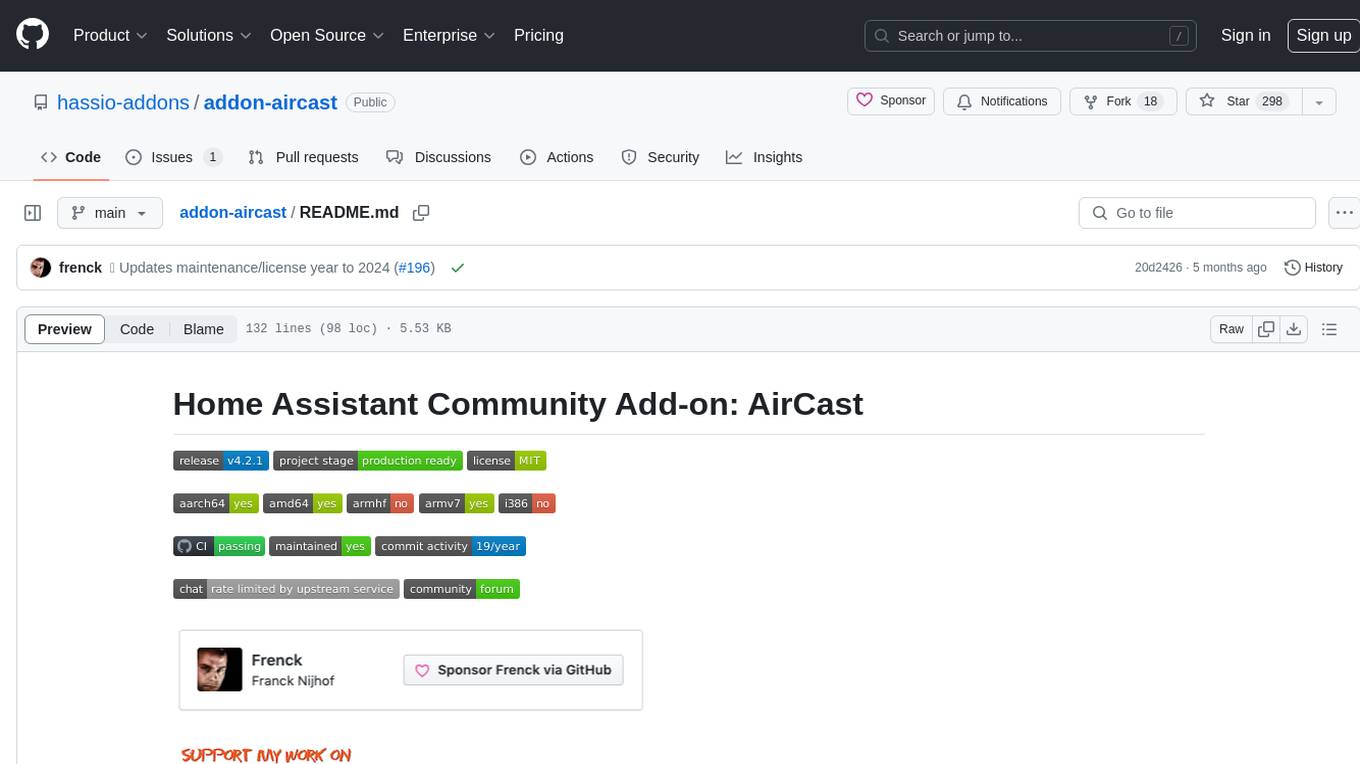
addon-aircast
AirCast is a Home Assistant Community Add-on that provides AirPlay capabilities for Chromecast players. It bridges the compatibility gap between Apple's AirPlay and Google's Chromecast by creating virtual AirPlay devices for Chromecast players on the network. The add-on is based on the AirConnect project and allows users to stream audio from Apple devices to Chromecast players.
For similar jobs
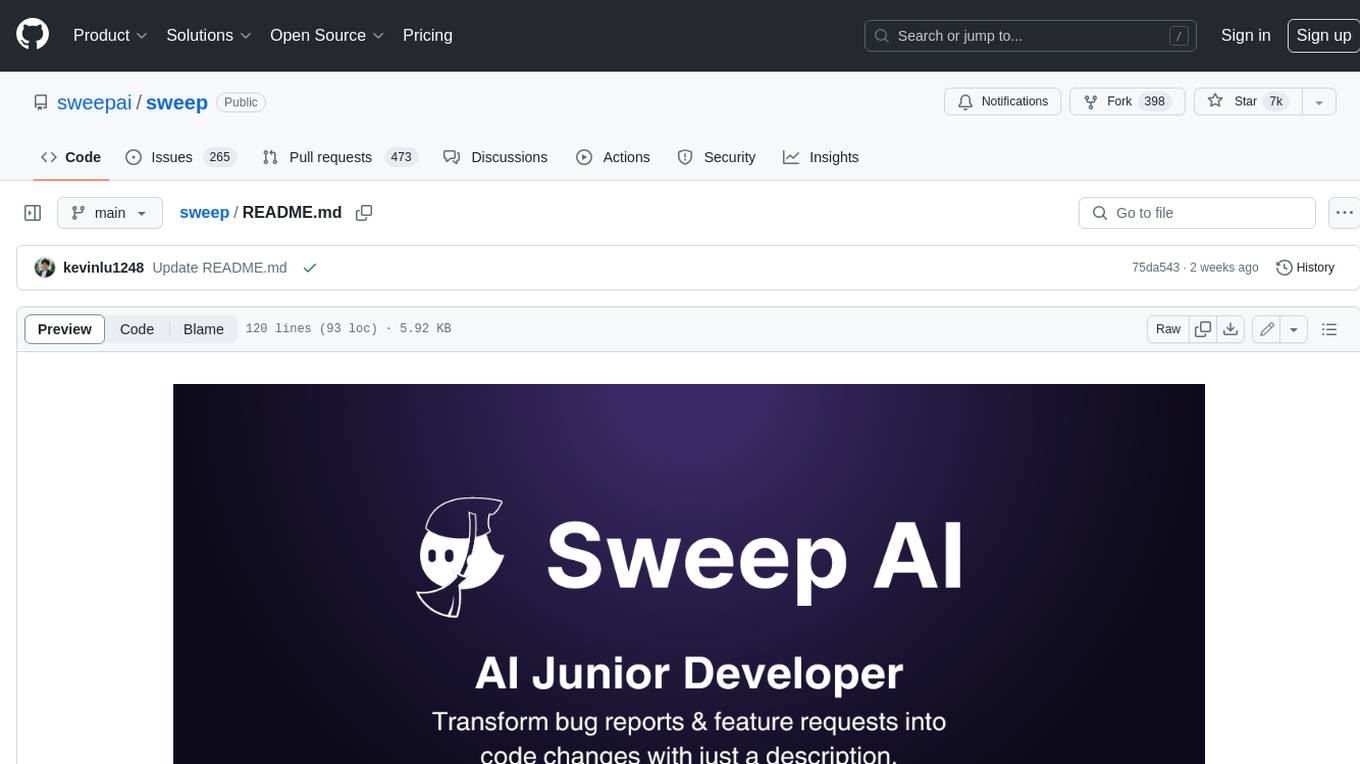
sweep
Sweep is an AI junior developer that turns bugs and feature requests into code changes. It automatically handles developer experience improvements like adding type hints and improving test coverage.
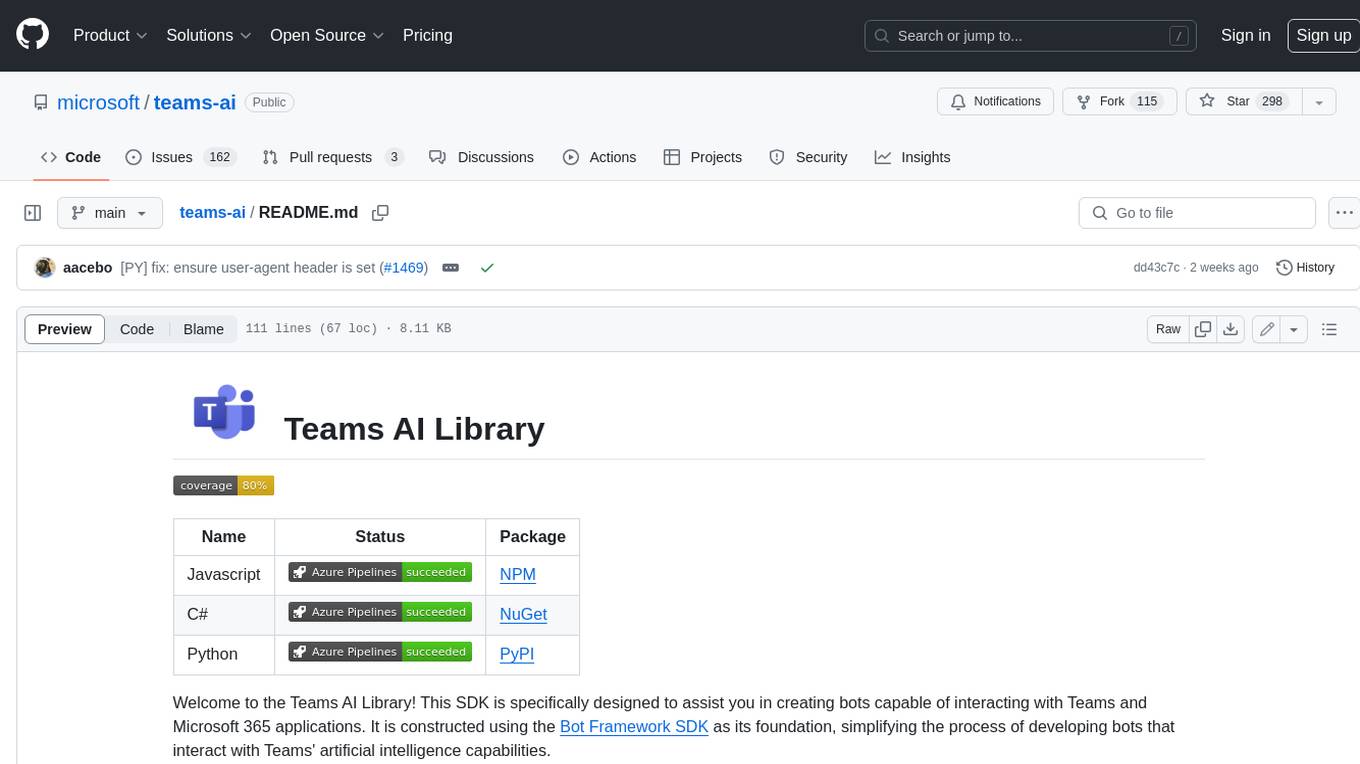
teams-ai
The Teams AI Library is a software development kit (SDK) that helps developers create bots that can interact with Teams and Microsoft 365 applications. It is built on top of the Bot Framework SDK and simplifies the process of developing bots that interact with Teams' artificial intelligence capabilities. The SDK is available for JavaScript/TypeScript, .NET, and Python.
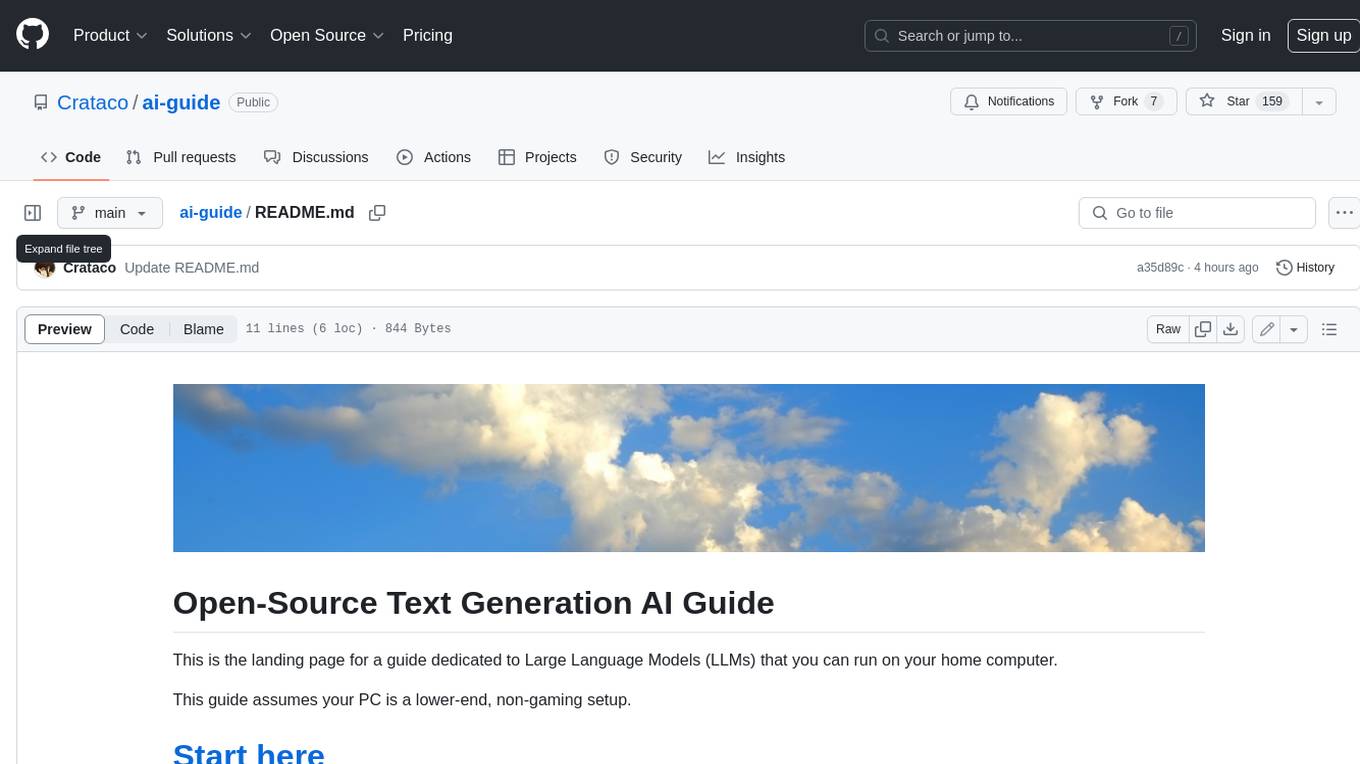
ai-guide
This guide is dedicated to Large Language Models (LLMs) that you can run on your home computer. It assumes your PC is a lower-end, non-gaming setup.
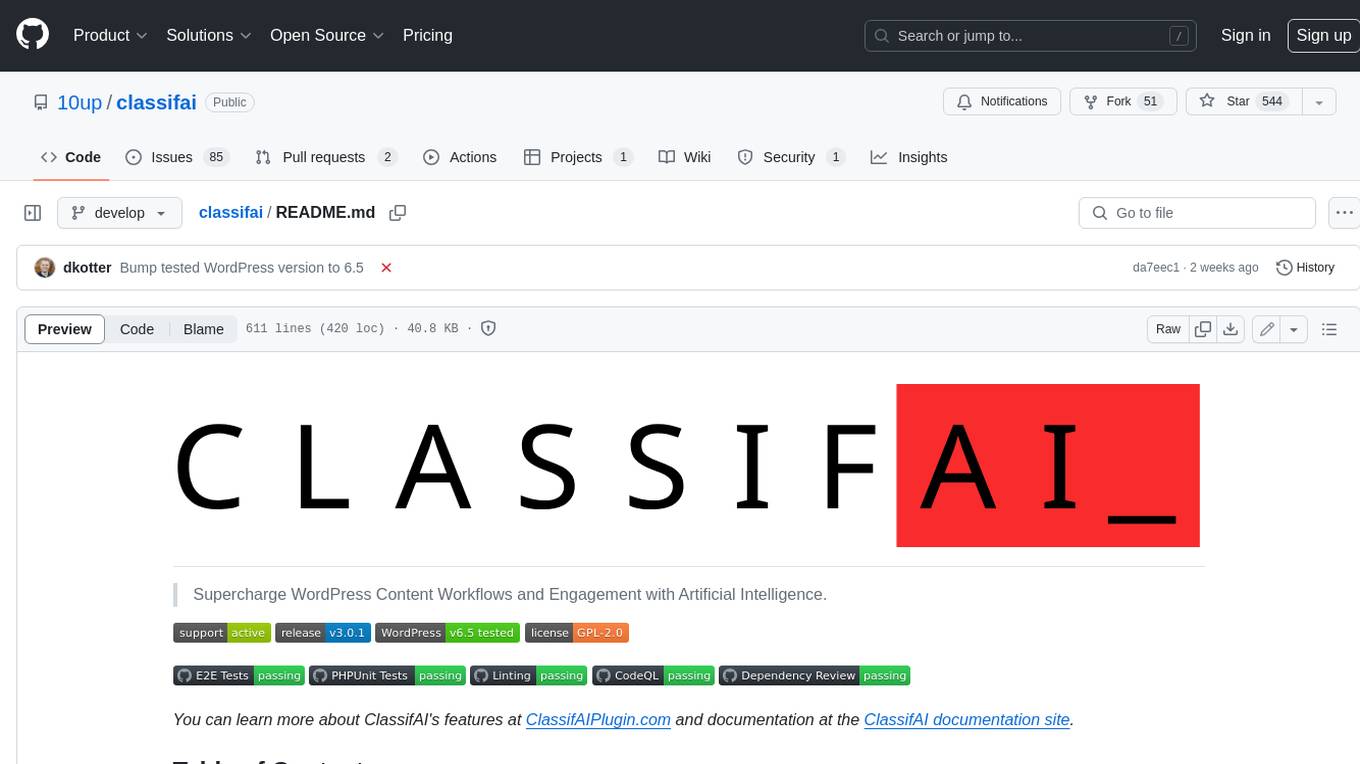
classifai
Supercharge WordPress Content Workflows and Engagement with Artificial Intelligence. Tap into leading cloud-based services like OpenAI, Microsoft Azure AI, Google Gemini and IBM Watson to augment your WordPress-powered websites. Publish content faster while improving SEO performance and increasing audience engagement. ClassifAI integrates Artificial Intelligence and Machine Learning technologies to lighten your workload and eliminate tedious tasks, giving you more time to create original content that matters.
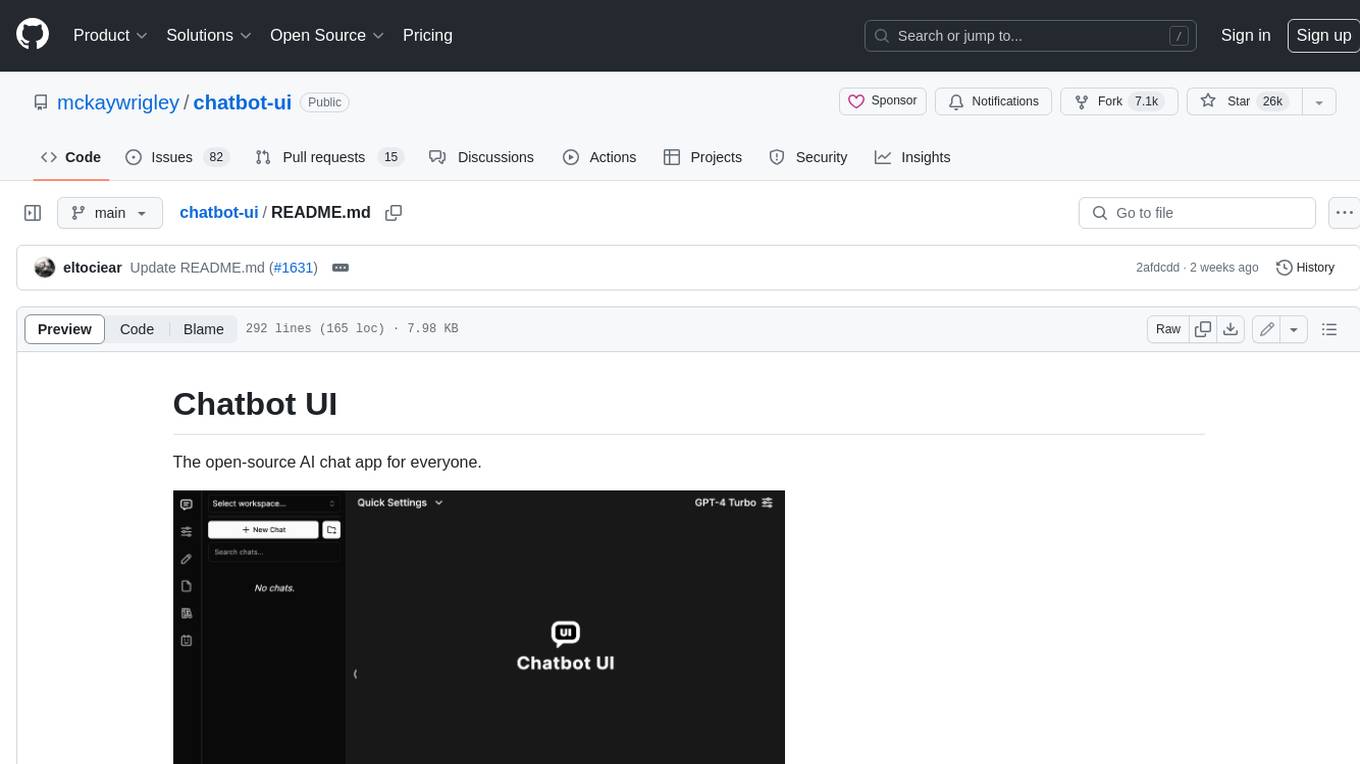
chatbot-ui
Chatbot UI is an open-source AI chat app that allows users to create and deploy their own AI chatbots. It is easy to use and can be customized to fit any need. Chatbot UI is perfect for businesses, developers, and anyone who wants to create a chatbot.
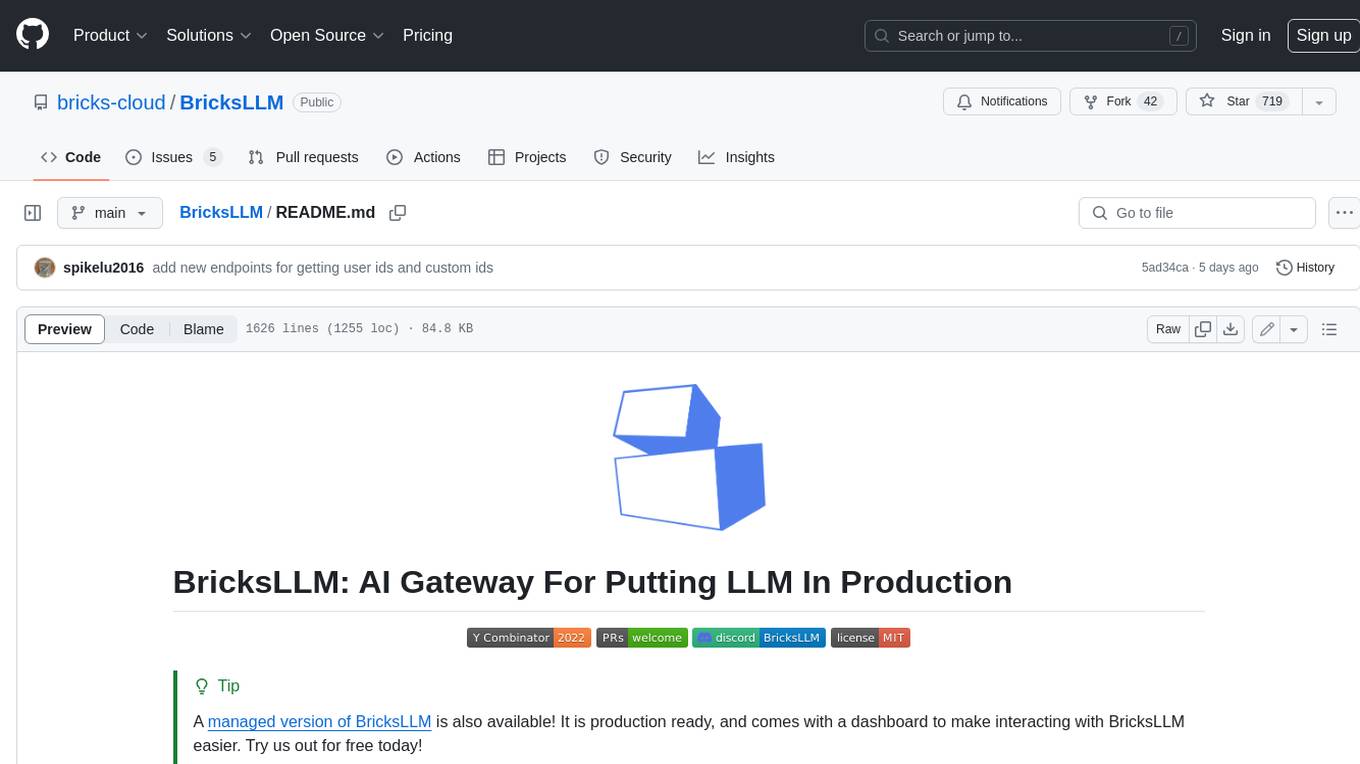
BricksLLM
BricksLLM is a cloud native AI gateway written in Go. Currently, it provides native support for OpenAI, Anthropic, Azure OpenAI and vLLM. BricksLLM aims to provide enterprise level infrastructure that can power any LLM production use cases. Here are some use cases for BricksLLM: * Set LLM usage limits for users on different pricing tiers * Track LLM usage on a per user and per organization basis * Block or redact requests containing PIIs * Improve LLM reliability with failovers, retries and caching * Distribute API keys with rate limits and cost limits for internal development/production use cases * Distribute API keys with rate limits and cost limits for students
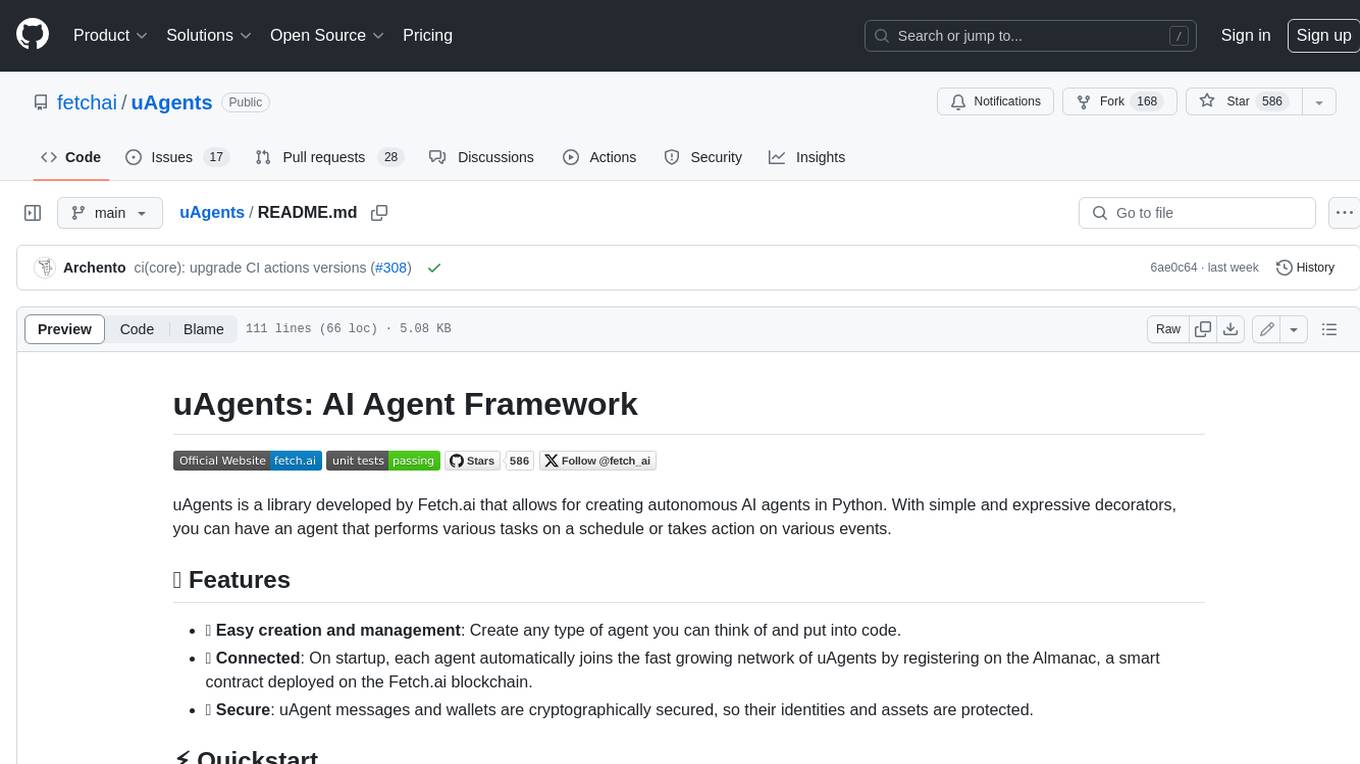
uAgents
uAgents is a Python library developed by Fetch.ai that allows for the creation of autonomous AI agents. These agents can perform various tasks on a schedule or take action on various events. uAgents are easy to create and manage, and they are connected to a fast-growing network of other uAgents. They are also secure, with cryptographically secured messages and wallets.
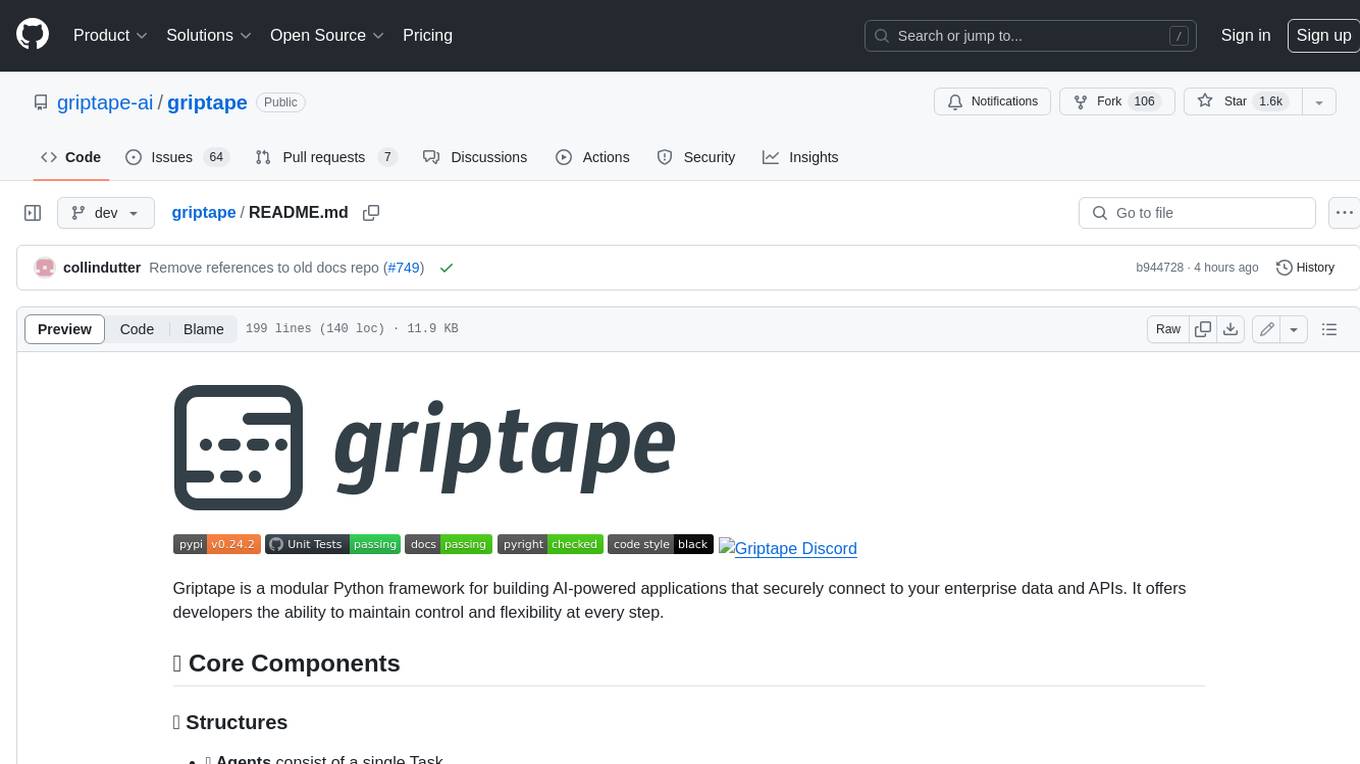
griptape
Griptape is a modular Python framework for building AI-powered applications that securely connect to your enterprise data and APIs. It offers developers the ability to maintain control and flexibility at every step. Griptape's core components include Structures (Agents, Pipelines, and Workflows), Tasks, Tools, Memory (Conversation Memory, Task Memory, and Meta Memory), Drivers (Prompt and Embedding Drivers, Vector Store Drivers, Image Generation Drivers, Image Query Drivers, SQL Drivers, Web Scraper Drivers, and Conversation Memory Drivers), Engines (Query Engines, Extraction Engines, Summary Engines, Image Generation Engines, and Image Query Engines), and additional components (Rulesets, Loaders, Artifacts, Chunkers, and Tokenizers). Griptape enables developers to create AI-powered applications with ease and efficiency.