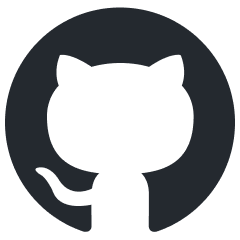
data-juicer
Data processing for and with foundation models! 🍎 🍋 🌽 ➡️ ➡️🍸 🍹 🍷
Stars: 4085
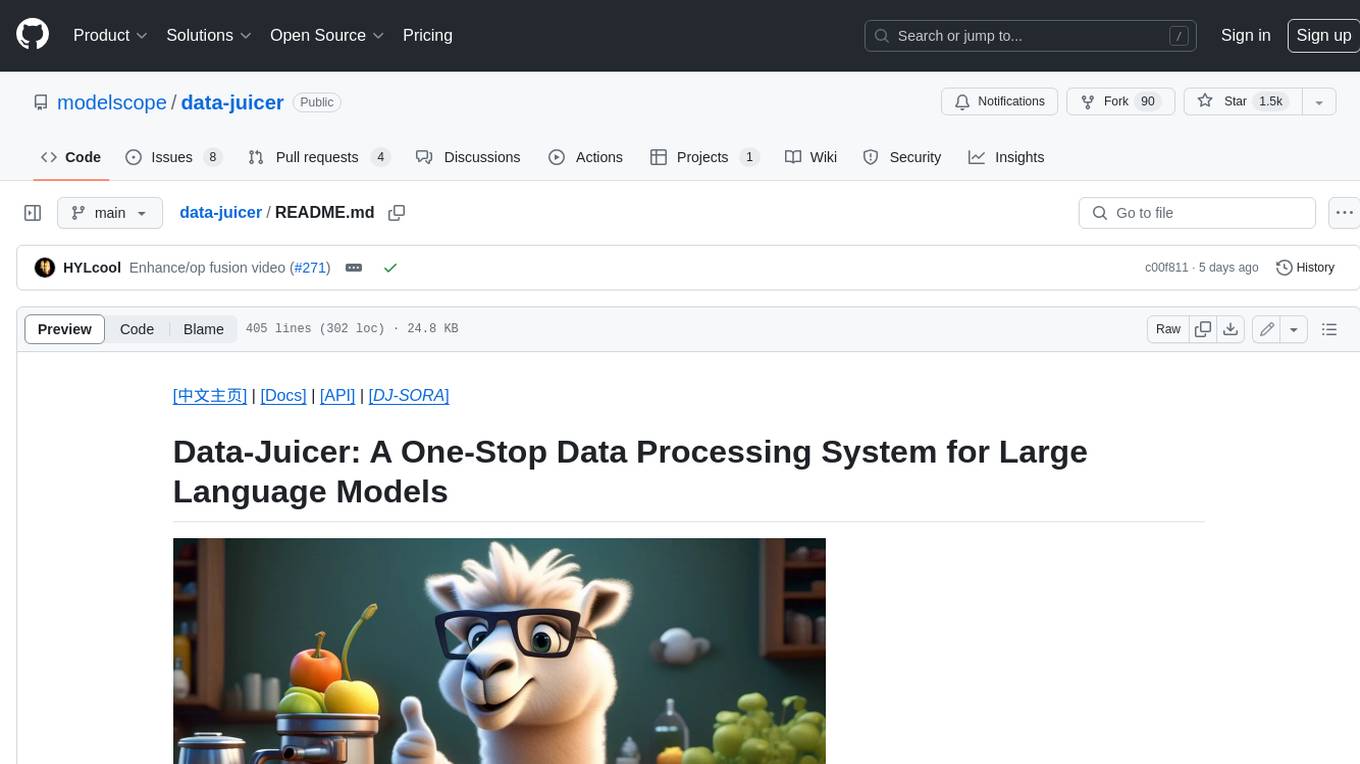
Data-Juicer is a one-stop data processing system to make data higher-quality, juicier, and more digestible for LLMs. It is a systematic & reusable library of 80+ core OPs, 20+ reusable config recipes, and 20+ feature-rich dedicated toolkits, designed to function independently of specific LLM datasets and processing pipelines. Data-Juicer allows detailed data analyses with an automated report generation feature for a deeper understanding of your dataset. Coupled with multi-dimension automatic evaluation capabilities, it supports a timely feedback loop at multiple stages in the LLM development process. Data-Juicer offers tens of pre-built data processing recipes for pre-training, fine-tuning, en, zh, and more scenarios. It provides a speedy data processing pipeline requiring less memory and CPU usage, optimized for maximum productivity. Data-Juicer is flexible & extensible, accommodating most types of data formats and allowing flexible combinations of OPs. It is designed for simplicity, with comprehensive documentation, easy start guides and demo configs, and intuitive configuration with simple adding/removing OPs from existing configs.
README:
[中文主页] | [DJ-Cookbook] | [OperatorZoo] | [API] | [Awesome LLM Data]
Data-Juicer is a one-stop system to process text and multimodal data for and with foundation models (typically LLMs). We provide a playground with a managed JupyterLab. Try Data-Juicer straight away in your browser! If you find Data-Juicer useful for your research or development, please kindly support us by starting it (then be instantly notified of our new releases) and citing our works.
Platform for AI of Alibaba Cloud (PAI) has cited our work and integrated Data-Juicer into its data processing products. PAI is an AI Native large model and AIGC engineering platform that provides dataset management, computing power management, model tool chain, model development, model training, model deployment, and AI asset management. For documentation on data processing, please refer to: PAI-Data Processing for Large Models.
Data-Juicer is being actively updated and maintained. We will periodically enhance and add more features, data recipes and datasets. We welcome you to join us (via issues, PRs, Slack channel, DingDing group, ...), in promoting data-model co-development along with research and applications of foundation models!
-
[2025-03-13] We propose a new data synthesis method, MindGym, which encourages LLMs to self-generate challenging cognitive questions, achieving superior data efficiency, cross-modality generalization, and SFT effects over SOTA baselines (e.g., 16% gain on MathVision using only 400 samples). See more details in MindGym: Enhancing Vision-Language Models via Synthetic Self-Challenging Questions.
-
[2025-02-28] DJ has been integrated in Ray's official Ecosystem and Example Gallery. Besides, our patch in DJ2.0 for the streaming JSON reader has been officially integrated by Apache Arrow.
-
[2025-02-27] Our work on contrastive data synthesis, ImgDiff, has been accepted by CVPR 2025!
-
[2025-02-05] We propose a new data selection method, DaaR, which is theoretically informed, via treating diversity as a reward, achieves better overall performance across 7 benchmarks when post-training SOTA LLMs. See more details in Diversity as a Reward: Fine-Tuning LLMs on a Mixture of Domain-Undetermined Data.
-
[2025-01-11] We release our 2.0 paper, Data-Juicer 2.0: Cloud-Scale Adaptive Data Processing for Foundation Models. It now can process 70B data samples within 2.1h, using 6400 CPU cores on 50 Ray nodes from Alibaba Cloud cluster, and deduplicate 5TB data within 2.8h using 1280 CPU cores on 8 Ray nodes.
-
[2025-01-03] We support post-tuning scenarios better, via 20+ related new OPs, and via unified dataset format compatible to LLaMA-Factory and ModelScope-Swift.
-
[2025-12-17] We propose HumanVBench, which comprises 16 human-centric tasks with synthetic data, benchmarking 22 video-MLLMs' capabilities from views of inner emotion and outer manifestations. See more details in our paper, and try to evaluate your models with it.
History News:
>-
[2024-11-22] We release DJ v1.0.0, in which we refactored Data-Juicer's Operator, Dataset, Sandbox and many other modules for better usability, such as supporting fault-tolerant, FastAPI and adaptive resource management.
-
[2024-08-25] We give a tutorial about data processing for multimodal LLMs in KDD'2024.
-
[2024-08-09] We propose Img-Diff, which enhances the performance of multimodal large language models through contrastive data synthesis, achieving a score that is 12 points higher than GPT-4V on the MMVP benchmark. See more details in our paper, and download the dataset from huggingface and modelscope.
-
[2024-07-24] "Tianchi Better Synth Data Synthesis Competition for Multimodal Large Models" — Our 4th data-centric LLM competition has kicked off! Please visit the competition's official website for more information.
-
[2024-07-17] We utilized the Data-Juicer Sandbox Laboratory Suite to systematically optimize data and models through a co-development workflow between data and models, achieving a new top spot on the VBench text-to-video leaderboard. The related achievements have been compiled and published in a paper, and the model has been released on the ModelScope and HuggingFace platforms.
-
[2024-07-12] Our awesome list of MLLM-Data has evolved into a systemic survey from model-data co-development perspective. Welcome to explore and contribute!
-
[2024-06-01] ModelScope-Sora "Data Directors" creative sprint—Our third data-centric LLM competition has kicked off! Please visit the competition's official website for more information.
-
[2024-03-07] We release Data-Juicer v0.2.0 now! In this new version, we support more features for multimodal data (including video now), and introduce DJ-SORA to provide open large-scale, high-quality datasets for SORA-like models.
-
[2024-02-20] We have actively maintained an awesome list of LLM-Data, welcome to visit and contribute!
-
[2024-02-05] Our paper has been accepted by SIGMOD'24 industrial track!
-
[2024-01-10] Discover new horizons in "Data Mixture"—Our second data-centric LLM competition has kicked off! Please visit the competition's official website for more information.
-
[2024-01-05] We release Data-Juicer v0.1.3 now! In this new version, we support more Python versions (3.8-3.10), and support multimodal dataset converting/processing (Including texts, images, and audios. More modalities will be supported in the future). Besides, our paper is also updated to v3.
-
[2023-10-13] Our first data-centric LLM competition begins! Please visit the competition's official websites, FT-Data Ranker (1B Track, 7B Track), for more information.
- News
- Why Data-Juicer?
- DJ-Cookbook
- Installation
- Quick Start
- License
- Contributing
- Acknowledgement
- References
-
Systematic & Reusable: Empowering users with a systematic library of 100+ core OPs, and 50+ reusable config recipes and dedicated toolkits, designed to function independently of specific multimodal LLM datasets and processing pipelines. Supporting data analysis, cleaning, and synthesis in pre-training, post-tuning, en, zh, and more scenarios.
-
User-Friendly & Extensible: Designed for simplicity and flexibility, with easy-start guides, and DJ-Cookbook containing fruitful demo usages. Feel free to implement your own OPs for customizable data processing.
-
Efficient & Robust: Providing performance-optimized parallel data processing (Aliyun-PAI\Ray\CUDA\OP Fusion), faster with less resource usage, verified in large-scale production environments.
-
Effect-Proven & Sandbox: Supporting data-model co-development, enabling rapid iteration through the sandbox laboratory, and providing features such as feedback loops and visualization, so that you can better understand and improve your data and models. Many effect-proven datasets and models have been derived from DJ, in scenarios such as pre-training, text-to-video and image-to-text generation.
- Basics
- Lookup Materials
- Advanced
-
Data Recipe Gallery
- Data-Juicer Minimal Example Recipe
- Reproducing Open Source Text Datasets
- Improving Open Source Pre-training Text Datasets
- Improving Open Source Post-tuning Text Datasets
- Synthetic Contrastive Learning Image-text Datasets
- Improving Open Source Image-text Datasets
- Basic Example Recipes for Video Data
- Synthesizing Human-centric Video Benchmarks
- Improving Existing Open Source Video Datasets
- Data-Juicer related Competitions
- Better Synth, explore the impact of large model synthetic data on image understanding ability with DJ-Sandbox Lab and multimodal large models
- Modelscope-Sora Challenge, based on Data-Juicer and EasyAnimate framework, optimize data and train SORA-like small models to generate better videos
- Better Mixture, only adjust data mixing and sampling strategies for given multiple candidate datasets
- FT-Data Ranker (1B Track, 7B Track), For a specified candidate dataset, only adjust the data filtering and enhancement strategies
- Kolors-LoRA Stylized Story Challenge, based on Data-Juicer and DiffSynth-Studio framework, explore Diffusion model fine-tuning
- DJ-SORA
- Based on Data-Juicer and AgentScope framework, leverage agents to call DJ Filters and call DJ Mappers
- Introduction to Data-Juicer [ModelScope] [HuggingFace]
- Data Visualization:
- Basic Statistics [ModelScope] [HuggingFace]
- Lexical Diversity [ModelScope] [HuggingFace]
- Operator Insight (Single OP) [ModelScope] [HuggingFace]
- Operator Effect (Multiple OPs) [ModelScope] [HuggingFace]
- Data Processing:
- Scientific Literature (e.g. arXiv) [ModelScope] [HuggingFace]
- Programming Code (e.g. TheStack) [ModelScope] [HuggingFace]
- Chinese Instruction Data (e.g. Alpaca-CoT) [ModelScope] [HuggingFace]
- Tool Pool:
- Dataset Splitting by Language [ModelScope] [HuggingFace]
- Quality Classifier for CommonCrawl [ModelScope] [HuggingFace]
- Auto Evaluation on HELM [ModelScope] [HuggingFace]
- Data Sampling and Mixture [ModelScope] [HuggingFace]
- Data Processing Loop [ModelScope] [HuggingFace]
- Recommend Python>=3.9,<=3.10
- gcc >= 5 (at least C++14 support)
- Run the following commands to install the latest basic
data_juicer
version in editable mode:
cd <path_to_data_juicer>
pip install -v -e .
- Some OPs rely on some other too large or low-platform-compatibility third-party libraries. You can install optional dependencies as needed:
cd <path_to_data_juicer>
pip install -v -e . # Install minimal dependencies, which support the basic functions
pip install -v -e .[tools] # Install a subset of tools dependencies
The dependency options are listed below:
Tag | Description |
---|---|
. or .[mini]
|
Install minimal dependencies for basic Data-Juicer. |
.[all] |
Install all dependencies except sandbox. |
.[sci] |
Install all dependencies for all OPs. |
.[dist] |
Install dependencies for distributed data processing. (Experimental) |
.[dev] |
Install dependencies for developing the package as contributors. |
.[tools] |
Install dependencies for dedicated tools, such as quality classifiers. |
.[sandbox] |
Install all dependencies for sandbox. |
- Install dependencies for specific OPs
With the growth of the number of OPs, the dependencies of all OPs become very heavy. Instead of using the command pip install -v -e .[sci]
to install all dependencies,
we provide two alternative, lighter options:
-
Automatic Minimal Dependency Installation: During the execution of Data-Juicer, minimal dependencies will be automatically installed. This allows for immediate execution, but may potentially lead to dependency conflicts.
-
Manual Minimal Dependency Installation: To manually install minimal dependencies tailored to a specific execution configuration, run the following command:
# only for installation from source python tools/dj_install.py --config path_to_your_data-juicer_config_file # use command line tool dj-install --config path_to_your_data-juicer_config_file
- Run the following command to install the latest released
data_juicer
usingpip
:
pip install py-data-juicer
-
Note:
- only the basic APIs in
data_juicer
and two basic tools (data processing and analysis) are available in this way. If you want customizable and complete functions, we recommend you installdata_juicer
from source. - The release versions from pypi have a certain lag compared to the latest version from source.
So if you want to follow the latest functions of
data_juicer
, we recommend you install from source.
- only the basic APIs in
- You can
-
either pull our pre-built image from DockerHub:
docker pull datajuicer/data-juicer:<version_tag>
-
or run the following command to build the docker image including the latest
data-juicer
with provided Dockerfile:docker build -t datajuicer/data-juicer:<version_tag> .
-
The format of
<version_tag>
is likev0.2.0
, which is the same as the release version tag.
-
import data_juicer as dj
print(dj.__version__)
Before using video-related operators, FFmpeg should be installed and accessible via the $PATH environment variable.
You can install FFmpeg using package managers(e.g. sudo apt install ffmpeg on Debian/Ubuntu, brew install ffmpeg on OS X) or visit the official ffmpeg link.
Check if your environment path is set correctly by running the ffmpeg command from the terminal.
DJ supports various dataset input types, including local files, remote datasets like huggingface; it also supports data validation and data mixture.
Two ways to configure a input file
- Simple scenarios, single path for local/HF file
dataset_path: '/path/to/your/dataset' # path to your dataset directory or file
- advanced method, supports sub-configuration items and more features
dataset:
configs:
- type: 'local'
path: 'path/to/your/dataset' # path to your dataset directory or file
Refer to Dataset Configuration Guide for more details.
- Run
process_data.py
tool ordj-process
command line tool with your config as the argument to process your dataset.
# only for installation from source
python tools/process_data.py --config configs/demo/process.yaml
# use command line tool
dj-process --config configs/demo/process.yaml
-
Note: For some operators that involve third-party models or resources that are not stored locally on your computer, it might be slow for the first running because these ops need to download corresponding resources into a directory first. The default download cache directory is
~/.cache/data_juicer
. Change the cache location by setting the shell environment variable,DATA_JUICER_CACHE_HOME
to another directory, and you can also changeDATA_JUICER_MODELS_CACHE
orDATA_JUICER_ASSETS_CACHE
in the same way: -
Note: When using operators with third-party models, it's necessary to declare the corresponding
mem_required
in the configuration file (you can refer to the settings in theconfig_all.yaml
file). During runtime, Data-Juicer will control the number of processes based on memory availability and the memory requirements of the operator models to achieve better data processing efficiency. When running with CUDA environments, if the mem_required for an operator is not declared correctly, it could potentially lead to a CUDA Out of Memory issue.
# cache home
export DATA_JUICER_CACHE_HOME="/path/to/another/directory"
# cache models
export DATA_JUICER_MODELS_CACHE="/path/to/another/directory/models"
# cache assets
export DATA_JUICER_ASSETS_CACHE="/path/to/another/directory/assets"
- Flexible Programming Interface: We provide various simple interfaces for users to choose from as follows.
#... init op & dataset ...
# Chain call style, support single operator or operator list
dataset = dataset.process(op)
dataset = dataset.process([op1, op2])
# Functional programming style for quick integration or script prototype iteration
dataset = op(dataset)
dataset = op.run(dataset)
We have now implemented multi-machine distributed data processing based on RAY. The corresponding demos can be run using the following commands:
# Run text data processing
python tools/process_data.py --config ./demos/process_on_ray/configs/demo.yaml
# Run video data processing
python tools/process_data.py --config ./demos/process_video_on_ray/configs/demo.yaml
- To run data processing across multiple machines, it is necessary to ensure that all distributed nodes can access the corresponding data paths (for example, by mounting the respective data paths on a file-sharing system such as NAS).
- The deduplication operators for RAY mode are different from the single-machine version, and all those operators are prefixed with
ray
, e.g.ray_video_deduplicator
andray_document_deduplicator
. - More details can be found in the doc for distributed processing.
Users can also opt not to use RAY and instead split the dataset to run on a cluster with Slurm. In this case, please use the default Data-Juicer without RAY. Aliyun PAI-DLC supports the RAY framework, Slurm framework, etc. Users can directly create RAY jobs and Slurm jobs on the DLC cluster.
- Run
analyze_data.py
tool ordj-analyze
command line tool with your config as the argument to analyze your dataset.
# only for installation from source
python tools/analyze_data.py --config configs/demo/analyzer.yaml
# use command line tool
dj-analyze --config configs/demo/analyzer.yaml
# you can also use auto mode to avoid writing a recipe. It will analyze a small
# part (e.g. 1000 samples, specified by argument `auto_num`) of your dataset
# with all Filters that produce stats.
dj-analyze --auto --dataset_path xx.jsonl [--auto_num 1000]
-
Note: Analyzer only computes stats for Filters that produce stats or other OPs that produce tags/categories in meta. So other OPs will be ignored in the analysis process. We use the following registries to decorate OPs:
-
NON_STATS_FILTERS
: decorate Filters that DO NOT produce any stats. -
TAGGING_OPS
: decorate OPs that DO produce tags/categories in meta field.
-
- Run
app.py
tool to visualize your dataset in your browser. - Note: only available for installation from source.
streamlit run app.py
- Config files specify some global arguments, and an operator list for the
data process. You need to set:
- Global arguments: input/output dataset path, number of workers, etc.
- Operator list: list operators with their arguments used to process the dataset.
- You can build up your own config files by:
- ➖:Modify from our example config file
config_all.yaml
which includes all ops and default arguments. You just need to remove ops that you won't use and refine some arguments of ops. - ➕:Build up your own config files from scratch. You can refer our
example config file
config_all.yaml
, op documents, and advanced Build-Up Guide for developers. - Besides the yaml files, you also have the flexibility to specify just one (of several) parameters on the command line, which will override the values in yaml files.
- ➖:Modify from our example config file
python xxx.py --config configs/demo/process.yaml --language_id_score_filter.lang=en
The data sandbox laboratory (DJ-Sandbox) provides users with the best practices for continuously producing data recipes. It features low overhead, portability, and guidance.
- In the sandbox, users can quickly experiment, iterate, and refine data recipes based on small-scale datasets and models, before scaling up to produce high-quality data to serve large-scale models.
- In addition to the basic data optimization and recipe refinement features offered by Data-Juicer, users can seamlessly use configurable components such as data probe and analysis, model training and evaluation, and data and model feedback-based recipe refinement to form a complete one-stop data-model research and development pipeline.
The sandbox is run using the following commands by default, and for more information and details, please refer to the sandbox documentation.
python tools/sandbox_starter.py --config configs/demo/sandbox/sandbox.yaml
- Our Formatters support some common input dataset formats for now:
- Multi-sample in one file: jsonl/json, parquet, csv/tsv, etc.
- Single-sample in one file: txt, code, docx, pdf, etc.
- However, data from different sources are complicated and diverse. Such as:
- Raw arXiv data downloaded from S3 include thousands of tar files and even more gzip files in them, and expected tex files are embedded in the gzip files so they are hard to obtain directly.
- Some crawled data include different kinds of files (pdf, html, docx, etc.). And extra information like tables, charts, and so on is hard to extract.
- It's impossible to handle all kinds of data in Data-Juicer, issues/PRs are welcome to contribute to processing new data types!
- Thus, we provide some common preprocessing tools in
tools/preprocess
for you to preprocess these data.- You are welcome to make your contributions to new preprocessing tools for the community.
- We highly recommend that complicated data can be preprocessed to jsonl or parquet files.
- If you build or pull the docker image of
data-juicer
, you can run the commands or tools mentioned above using this docker image. - Run directly:
# run the data processing directly
docker run --rm \ # remove container after the processing
--privileged \
--shm-size 256g \
--network host \
--gpus all \
--name dj \ # name of the container
-v <host_data_path>:<image_data_path> \ # mount data or config directory into the container
-v ~/.cache/:/root/.cache/ \ # mount the cache directory into the container to reuse caches and models (recommended)
datajuicer/data-juicer:<version_tag> \ # image to run
dj-process --config /path/to/config.yaml # similar data processing commands
- Or enter into the running container and run commands in editable mode:
# start the container
docker run -dit \ # run the container in the background
--privileged \
--shm-size 256g \
--network host \
--gpus all \
--rm \
--name dj \
-v <host_data_path>:<image_data_path> \
-v ~/.cache/:/root/.cache/ \
datajuicer/data-juicer:latest /bin/bash
# enter into this container and then you can use data-juicer in editable mode
docker exec -it <container_id> bash
Data-Juicer is released under Apache License 2.0.
We are in a rapidly developing field and greatly welcome contributions of new features, bug fixes, and better documentation. Please refer to How-to Guide for Developers.
Data-Juicer is used across various foundation model applications and research initiatives, such as industrial scenarios in Alibaba Tongyi and Alibaba Cloud's platform for AI (PAI). We look forward to more of your experience, suggestions, and discussions for collaboration!
Data-Juicer thanks many community contributors and open-source projects, such as Huggingface-Datasets, Bloom, RedPajama, Arrow, Ray, ....
If you find Data-Juicer useful for your research or development, please kindly cite the following works, 1.0paper, 2.0paper.
@inproceedings{djv1,
title={Data-Juicer: A One-Stop Data Processing System for Large Language Models},
author={Daoyuan Chen and Yilun Huang and Zhijian Ma and Hesen Chen and Xuchen Pan and Ce Ge and Dawei Gao and Yuexiang Xie and Zhaoyang Liu and Jinyang Gao and Yaliang Li and Bolin Ding and Jingren Zhou},
booktitle={International Conference on Management of Data},
year={2024}
}
@article{djv2,
title={Data-Juicer 2.0: Cloud-Scale Adaptive Data Processing for Foundation Models},
author={Chen, Daoyuan and Huang, Yilun and Pan, Xuchen and Jiang, Nana and Wang, Haibin and Ge, Ce and Chen, Yushuo and Zhang, Wenhao and Ma, Zhijian and Zhang, Yilei and Huang, Jun and Lin, Wei and Li, Yaliang and Ding, Bolin and Zhou, Jingren},
journal={arXiv preprint arXiv:2501.14755},
year={2024}
}
More data-related papers from the Data-Juicer Team:
>-
Data-Juicer Sandbox: A Feedback-Driven Suite for Multimodal Data-Model Co-development
-
ImgDiff: Contrastive Data Synthesis for Vision Large Language Models
-
Diversity as a Reward: Fine-Tuning LLMs on a Mixture of Domain-Undetermined Data
-
MindGym: Enhancing Vision-Language Models via Synthetic Self-Challenging Questions
-
BiMix: A Bivariate Data Mixing Law for Language Model Pretraining
For Tasks:
Click tags to check more tools for each tasksFor Jobs:
Alternative AI tools for data-juicer
Similar Open Source Tools
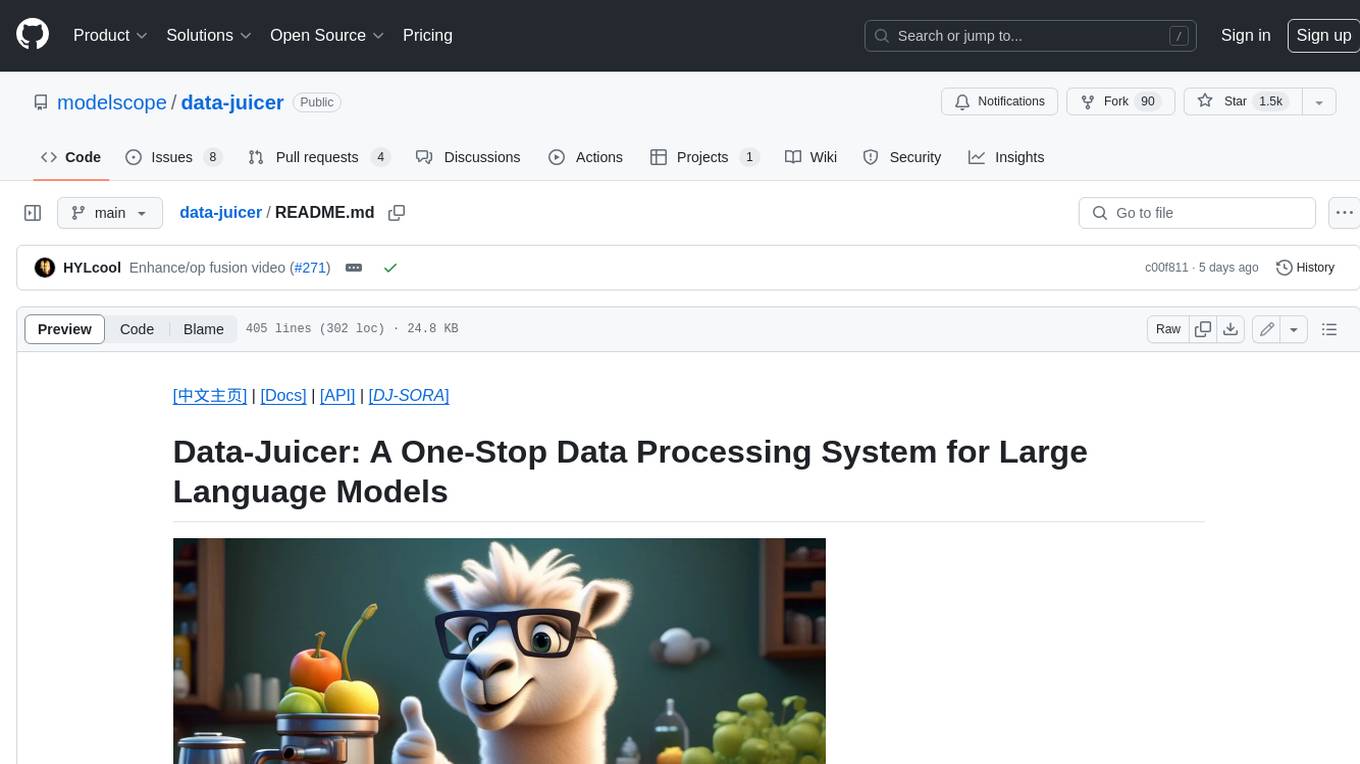
data-juicer
Data-Juicer is a one-stop data processing system to make data higher-quality, juicier, and more digestible for LLMs. It is a systematic & reusable library of 80+ core OPs, 20+ reusable config recipes, and 20+ feature-rich dedicated toolkits, designed to function independently of specific LLM datasets and processing pipelines. Data-Juicer allows detailed data analyses with an automated report generation feature for a deeper understanding of your dataset. Coupled with multi-dimension automatic evaluation capabilities, it supports a timely feedback loop at multiple stages in the LLM development process. Data-Juicer offers tens of pre-built data processing recipes for pre-training, fine-tuning, en, zh, and more scenarios. It provides a speedy data processing pipeline requiring less memory and CPU usage, optimized for maximum productivity. Data-Juicer is flexible & extensible, accommodating most types of data formats and allowing flexible combinations of OPs. It is designed for simplicity, with comprehensive documentation, easy start guides and demo configs, and intuitive configuration with simple adding/removing OPs from existing configs.
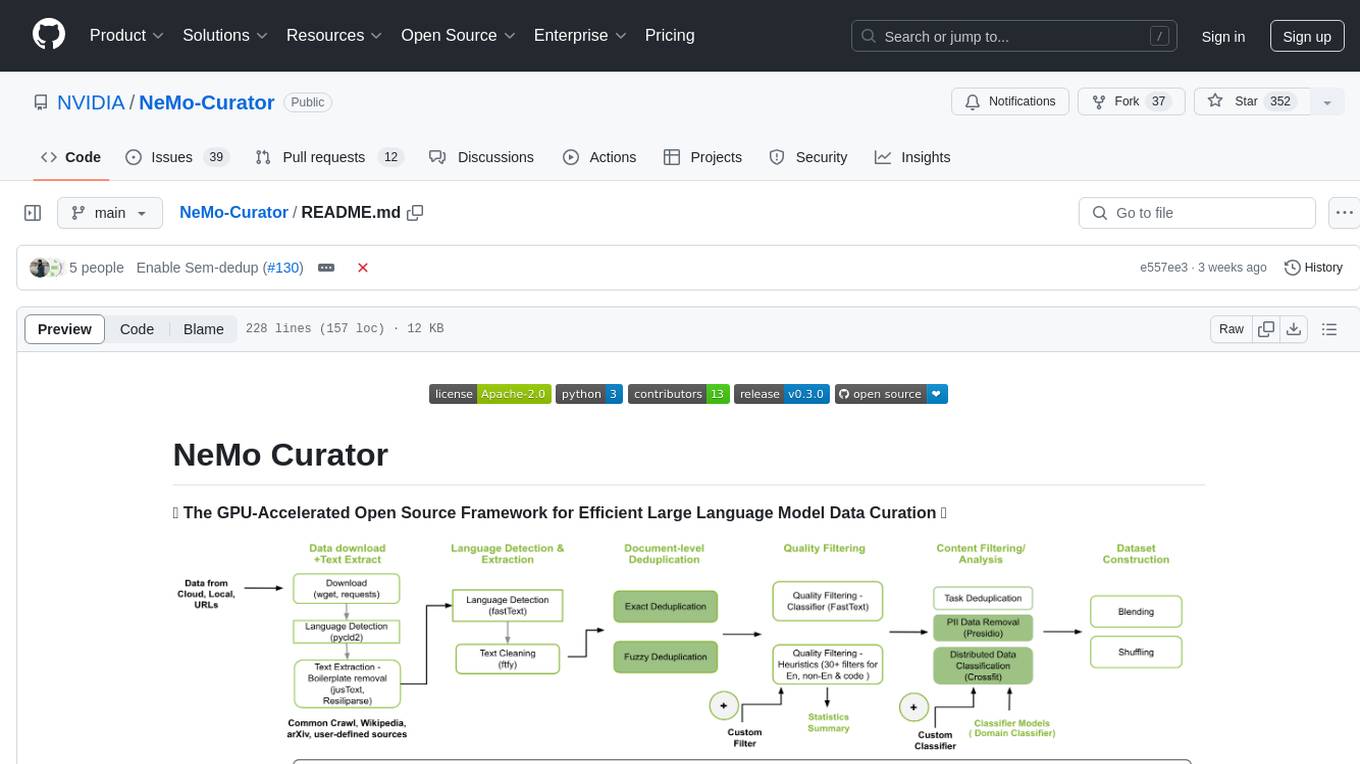
NeMo-Curator
NeMo Curator is a GPU-accelerated open-source framework designed for efficient large language model data curation. It provides scalable dataset preparation for tasks like foundation model pretraining, domain-adaptive pretraining, supervised fine-tuning, and parameter-efficient fine-tuning. The library leverages GPUs with Dask and RAPIDS to accelerate data curation, offering customizable and modular interfaces for pipeline expansion and model convergence. Key features include data download, text extraction, quality filtering, deduplication, downstream-task decontamination, distributed data classification, and PII redaction. NeMo Curator is suitable for curating high-quality datasets for large language model training.
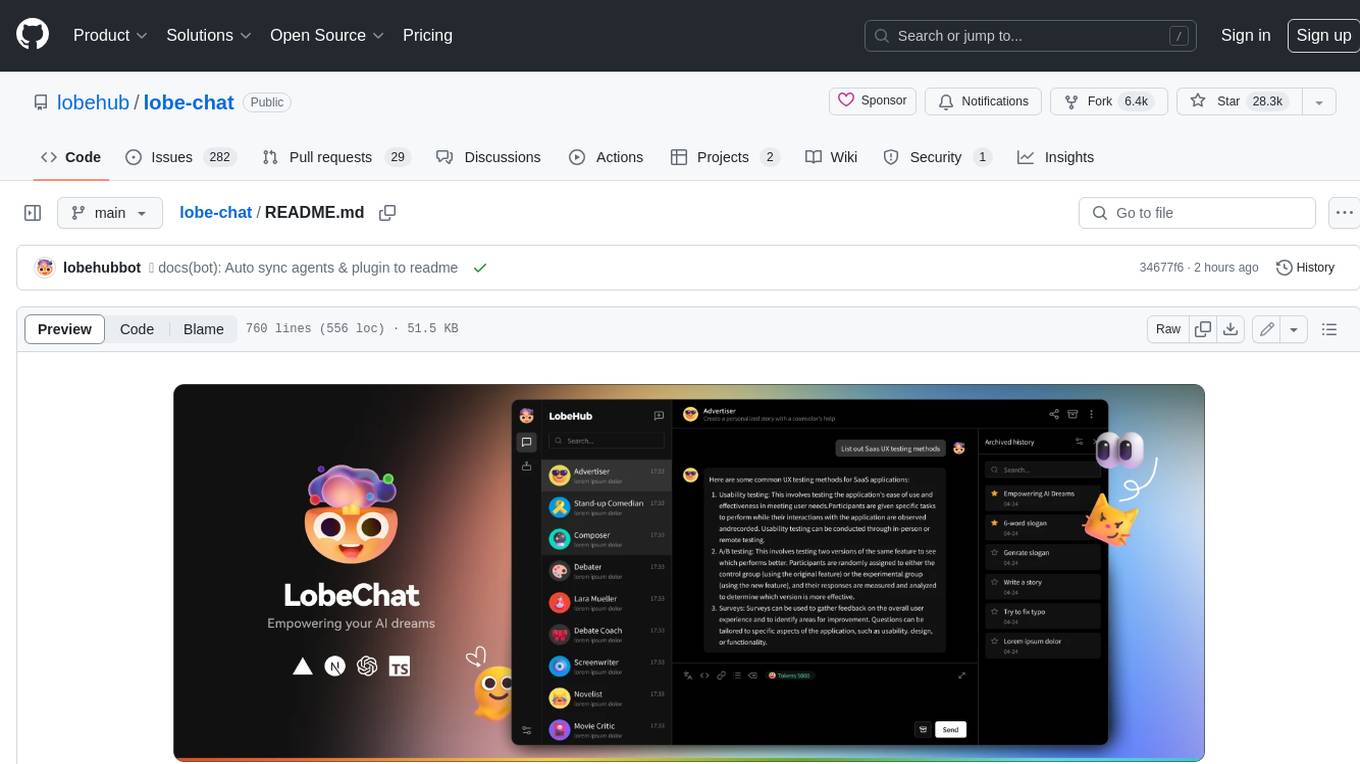
lobe-chat
Lobe Chat is an open-source, modern-design ChatGPT/LLMs UI/Framework. Supports speech-synthesis, multi-modal, and extensible ([function call][docs-functionc-call]) plugin system. One-click **FREE** deployment of your private OpenAI ChatGPT/Claude/Gemini/Groq/Ollama chat application.
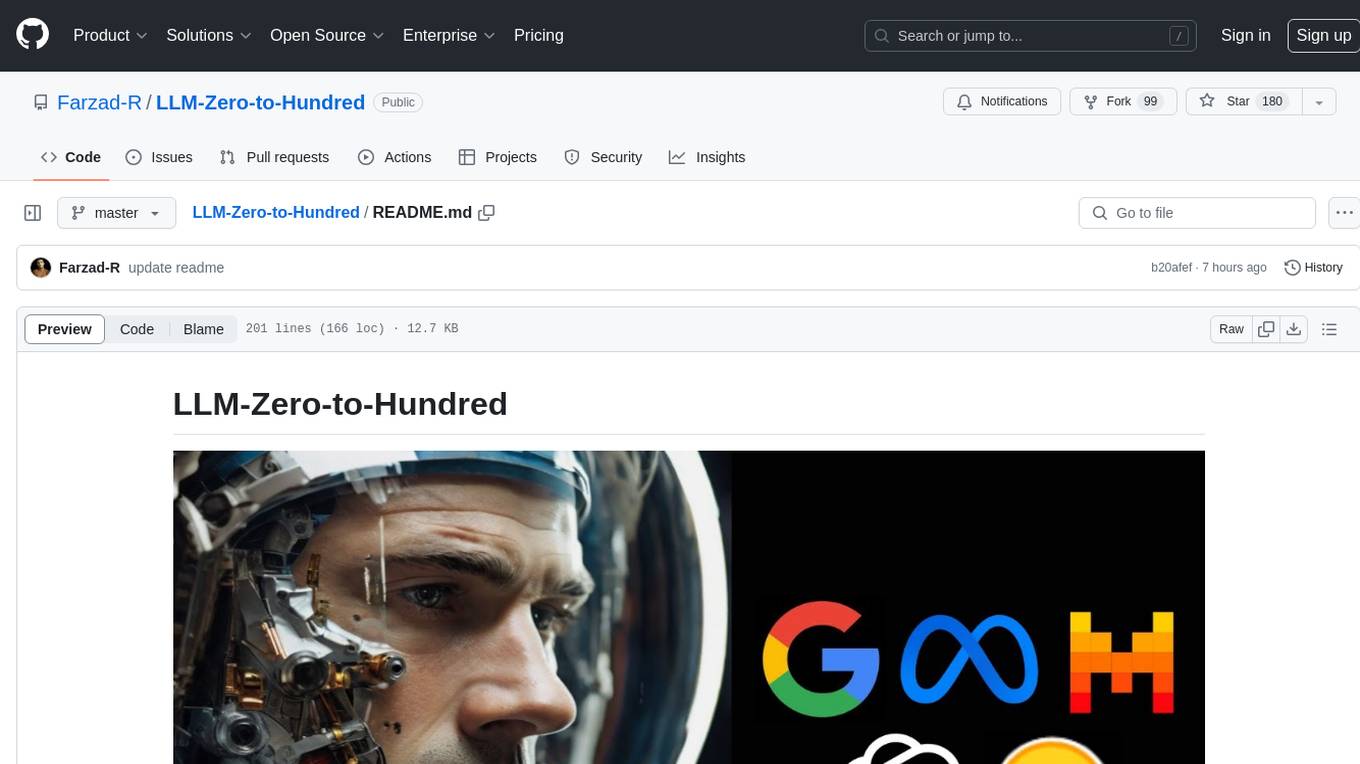
LLM-Zero-to-Hundred
LLM-Zero-to-Hundred is a repository showcasing various applications of LLM chatbots and providing insights into training and fine-tuning Language Models. It includes projects like WebGPT, RAG-GPT, WebRAGQuery, LLM Full Finetuning, RAG-Master LLamaindex vs Langchain, open-source-RAG-GEMMA, and HUMAIN: Advanced Multimodal, Multitask Chatbot. The projects cover features like ChatGPT-like interaction, RAG capabilities, image generation and understanding, DuckDuckGo integration, summarization, text and voice interaction, and memory access. Tutorials include LLM Function Calling and Visualizing Text Vectorization. The projects have a general structure with folders for README, HELPER, .env, configs, data, src, images, and utils.
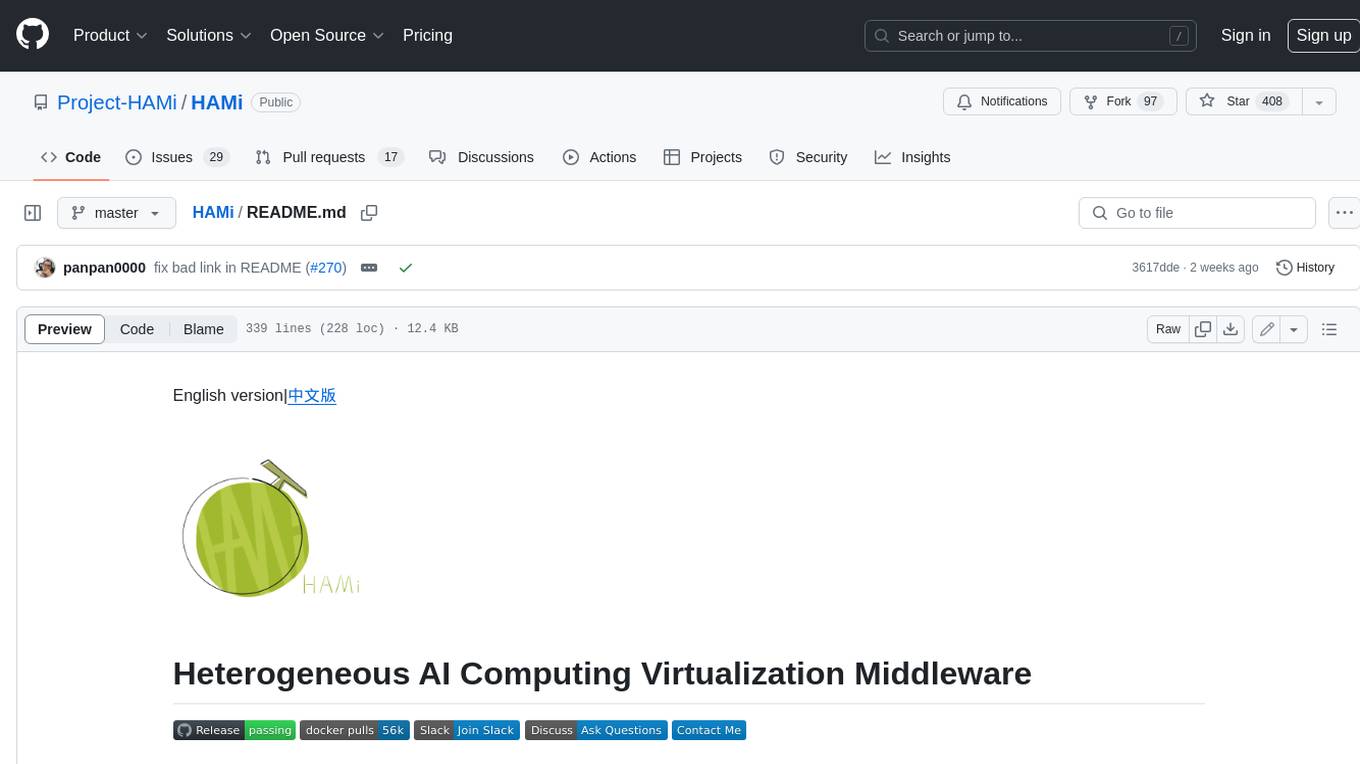
HAMi
HAMi is a Heterogeneous AI Computing Virtualization Middleware designed to manage Heterogeneous AI Computing Devices in a Kubernetes cluster. It allows for device sharing, device memory control, device type specification, and device UUID specification. The tool is easy to use and does not require modifying task YAML files. It includes features like hard limits on device memory, partial device allocation, streaming multiprocessor limits, and core usage specification. HAMi consists of components like a mutating webhook, scheduler extender, device plugins, and in-container virtualization techniques. It is suitable for scenarios requiring device sharing, specific device memory allocation, GPU balancing, low utilization optimization, and scenarios needing multiple small GPUs. The tool requires prerequisites like NVIDIA drivers, CUDA version, nvidia-docker, Kubernetes version, glibc version, and helm. Users can install, upgrade, and uninstall HAMi, submit tasks, and monitor cluster information. The tool's roadmap includes supporting additional AI computing devices, video codec processing, and Multi-Instance GPUs (MIG).
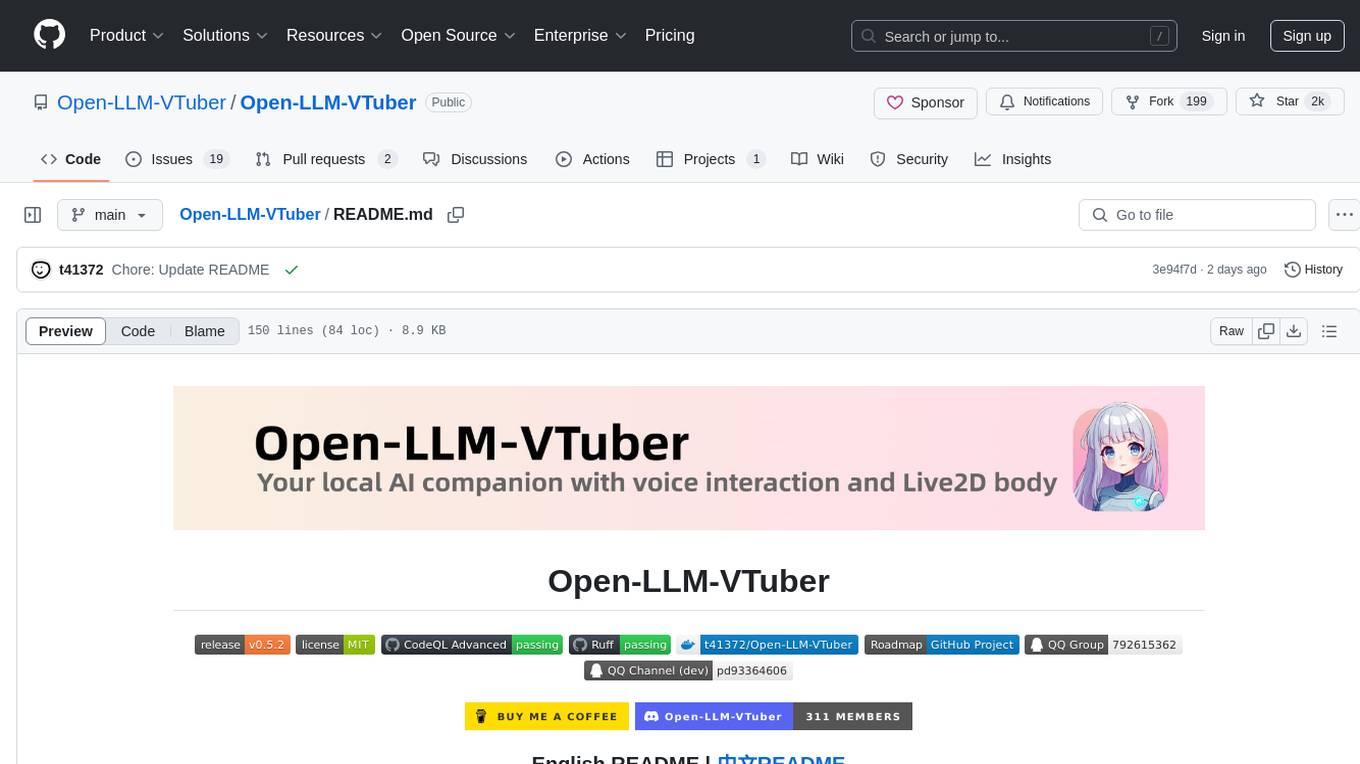
Open-LLM-VTuber
Open-LLM-VTuber is a voice-interactive AI companion supporting real-time voice conversations and featuring a Live2D avatar. It can run offline on Windows, macOS, and Linux, offering web and desktop client modes. Users can customize appearance and persona, with rich LLM inference, text-to-speech, and speech recognition support. The project is highly customizable, extensible, and actively developed with exciting features planned. It provides privacy with offline mode, persistent chat logs, and various interaction features like voice interruption, touch feedback, Live2D expressions, pet mode, and more.
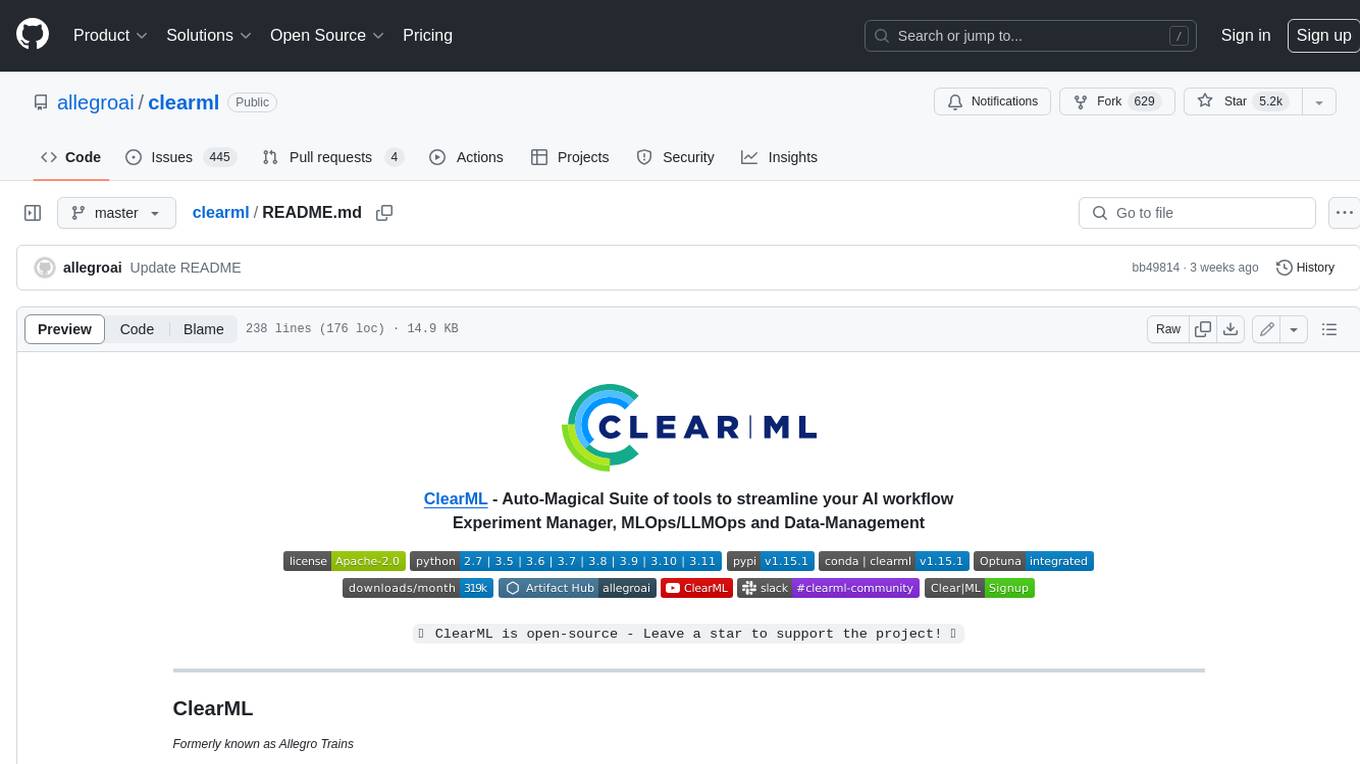
clearml
ClearML is a suite of tools designed to streamline the machine learning workflow. It includes an experiment manager, MLOps/LLMOps, data management, and model serving capabilities. ClearML is open-source and offers a free tier hosting option. It supports various ML/DL frameworks and integrates with Jupyter Notebook and PyCharm. ClearML provides extensive logging capabilities, including source control info, execution environment, hyper-parameters, and experiment outputs. It also offers automation features, such as remote job execution and pipeline creation. ClearML is designed to be easy to integrate, requiring only two lines of code to add to existing scripts. It aims to improve collaboration, visibility, and data transparency within ML teams.
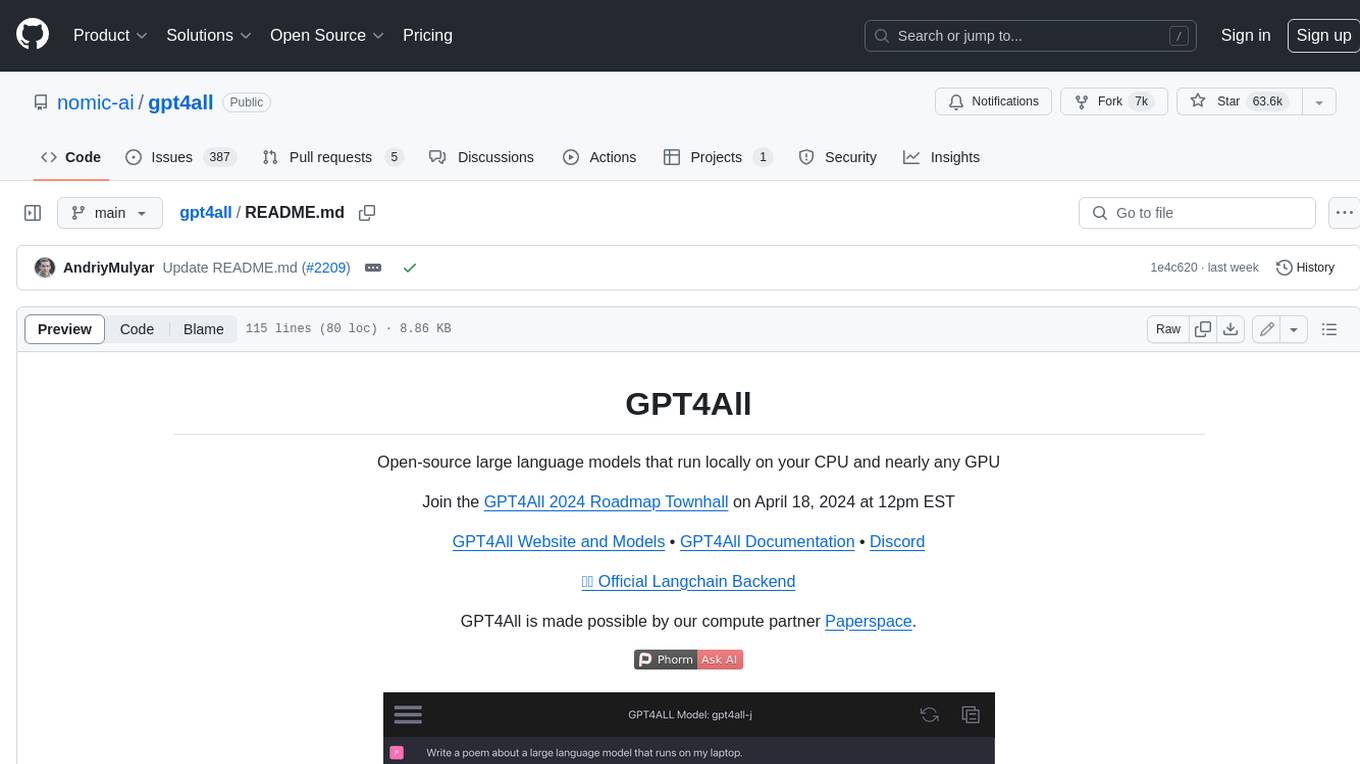
gpt4all
GPT4All is an ecosystem to run powerful and customized large language models that work locally on consumer grade CPUs and any GPU. Note that your CPU needs to support AVX or AVX2 instructions. Learn more in the documentation. A GPT4All model is a 3GB - 8GB file that you can download and plug into the GPT4All open-source ecosystem software. Nomic AI supports and maintains this software ecosystem to enforce quality and security alongside spearheading the effort to allow any person or enterprise to easily train and deploy their own on-edge large language models.
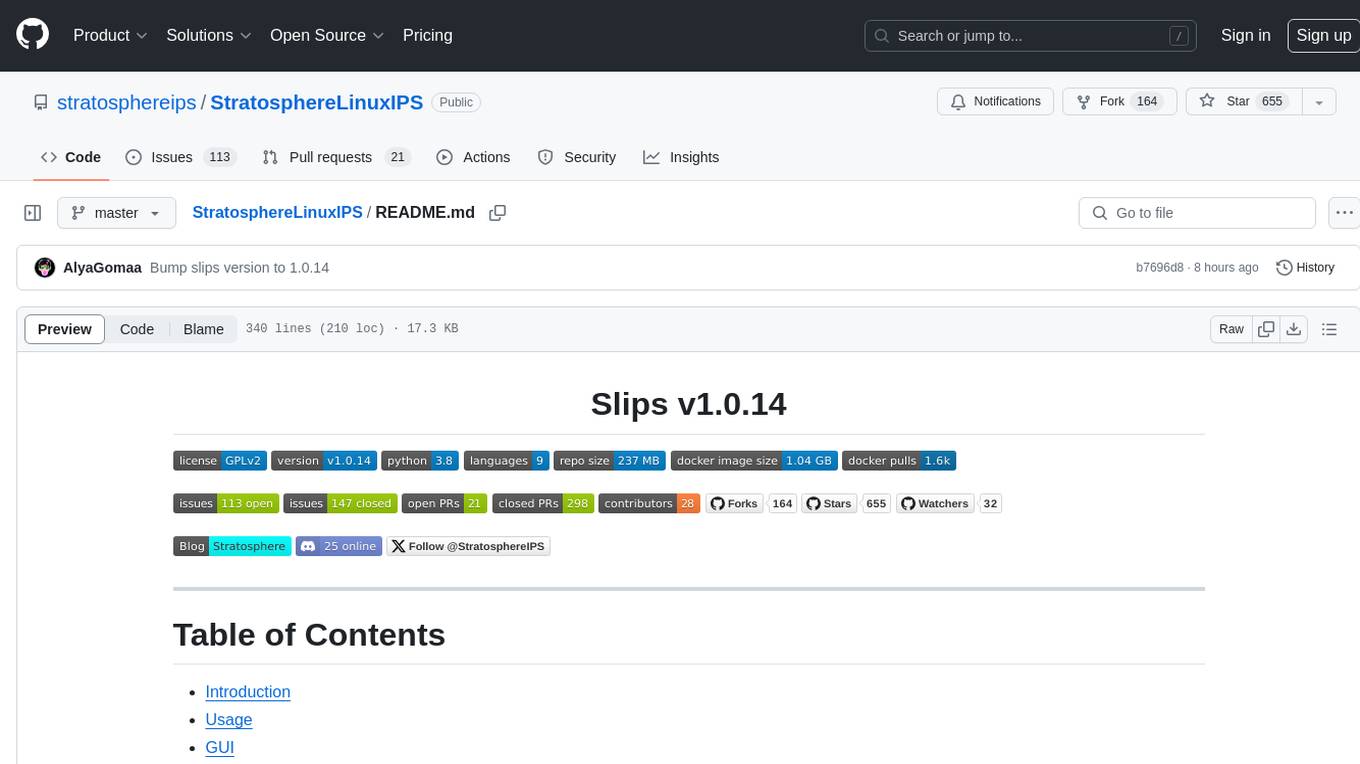
StratosphereLinuxIPS
Slips is a powerful endpoint behavioral intrusion prevention and detection system that uses machine learning to detect malicious behaviors in network traffic. It can work with network traffic in real-time, PCAP files, and network flows from tools like Suricata, Zeek/Bro, and Argus. Slips threat detection is based on machine learning models, threat intelligence feeds, and expert heuristics. It gathers evidence of malicious behavior and triggers alerts when enough evidence is accumulated. The tool is Python-based and supported on Linux and MacOS, with blocking features only on Linux. Slips relies on Zeek network analysis framework and Redis for interprocess communication. It offers a graphical user interface for easy monitoring and analysis.
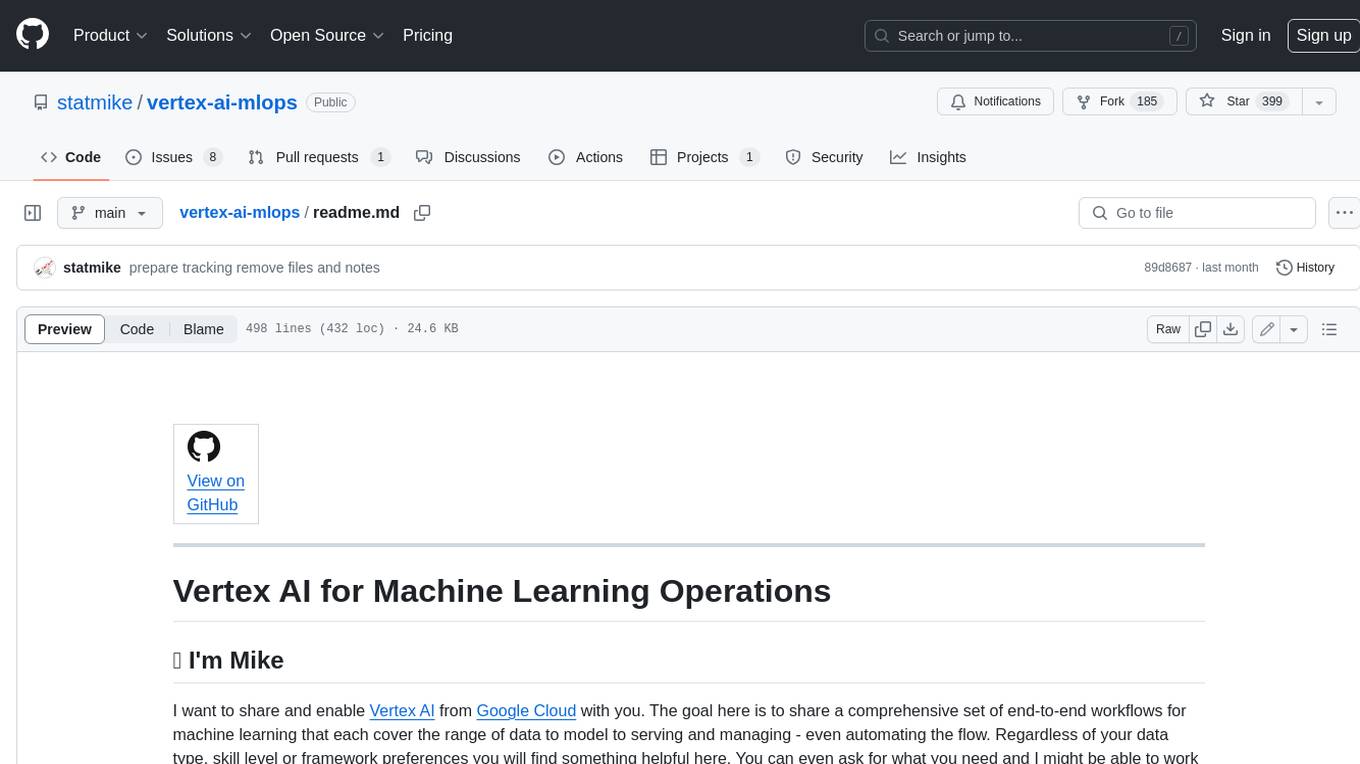
vertex-ai-mlops
Vertex AI is a platform for end-to-end model development. It consist of core components that make the processes of MLOps possible for design patterns of all types.
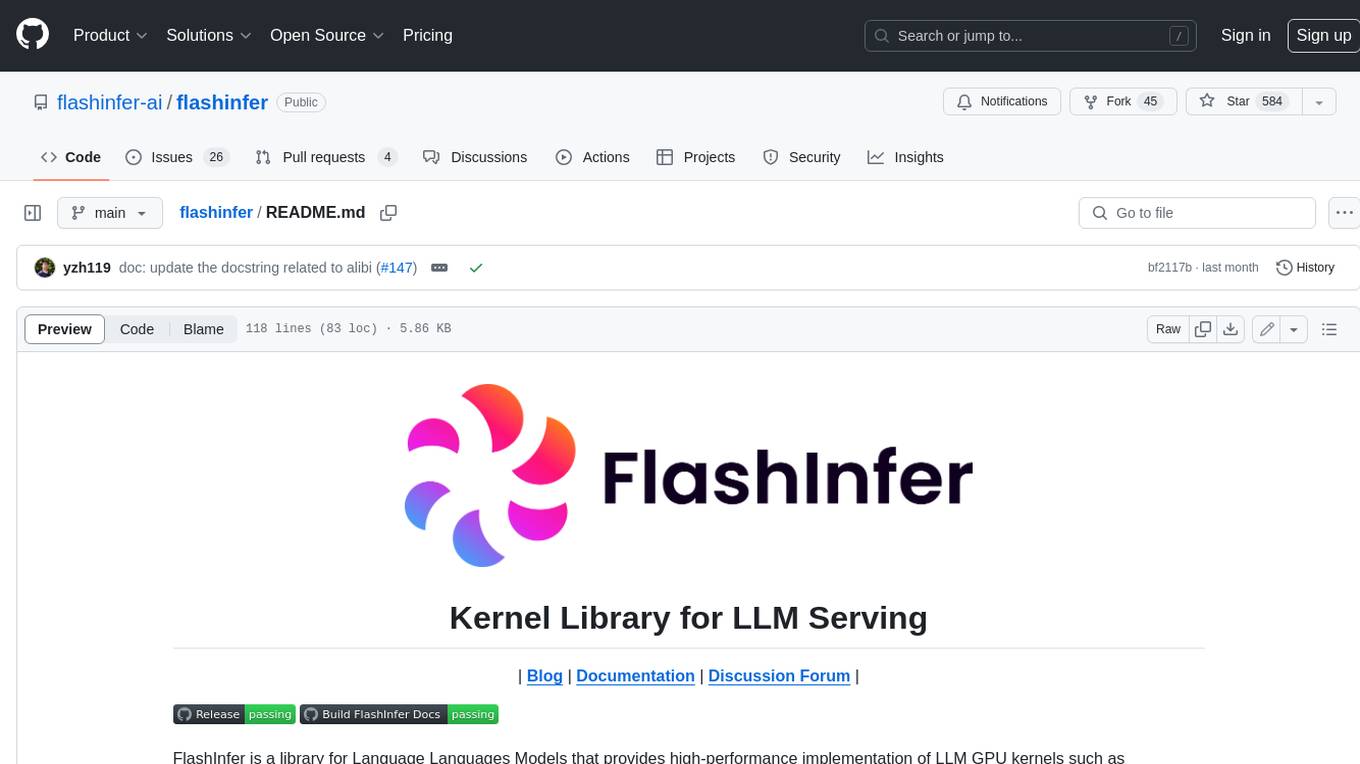
flashinfer
FlashInfer is a library for Language Languages Models that provides high-performance implementation of LLM GPU kernels such as FlashAttention, PageAttention and LoRA. FlashInfer focus on LLM serving and inference, and delivers state-the-art performance across diverse scenarios.
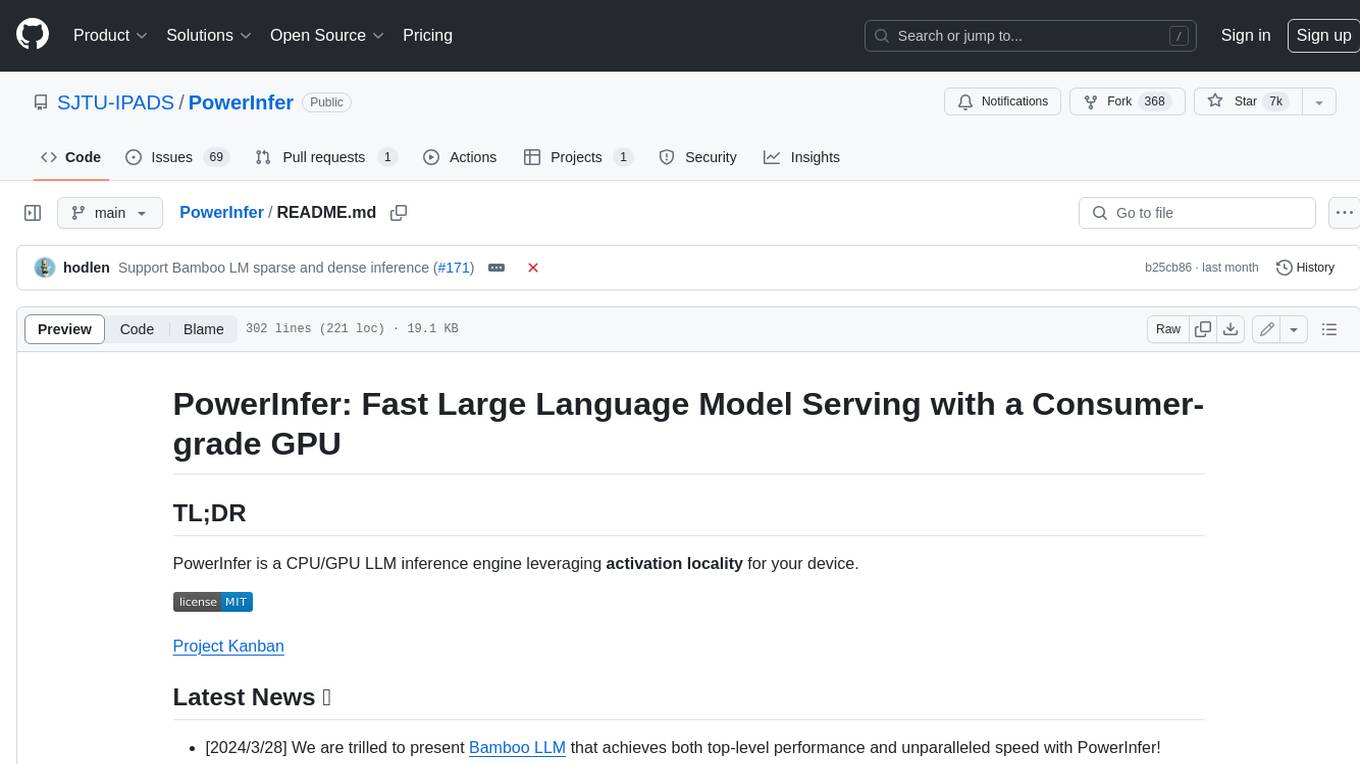
PowerInfer
PowerInfer is a high-speed Large Language Model (LLM) inference engine designed for local deployment on consumer-grade hardware, leveraging activation locality to optimize efficiency. It features a locality-centric design, hybrid CPU/GPU utilization, easy integration with popular ReLU-sparse models, and support for various platforms. PowerInfer achieves high speed with lower resource demands and is flexible for easy deployment and compatibility with existing models like Falcon-40B, Llama2 family, ProSparse Llama2 family, and Bamboo-7B.
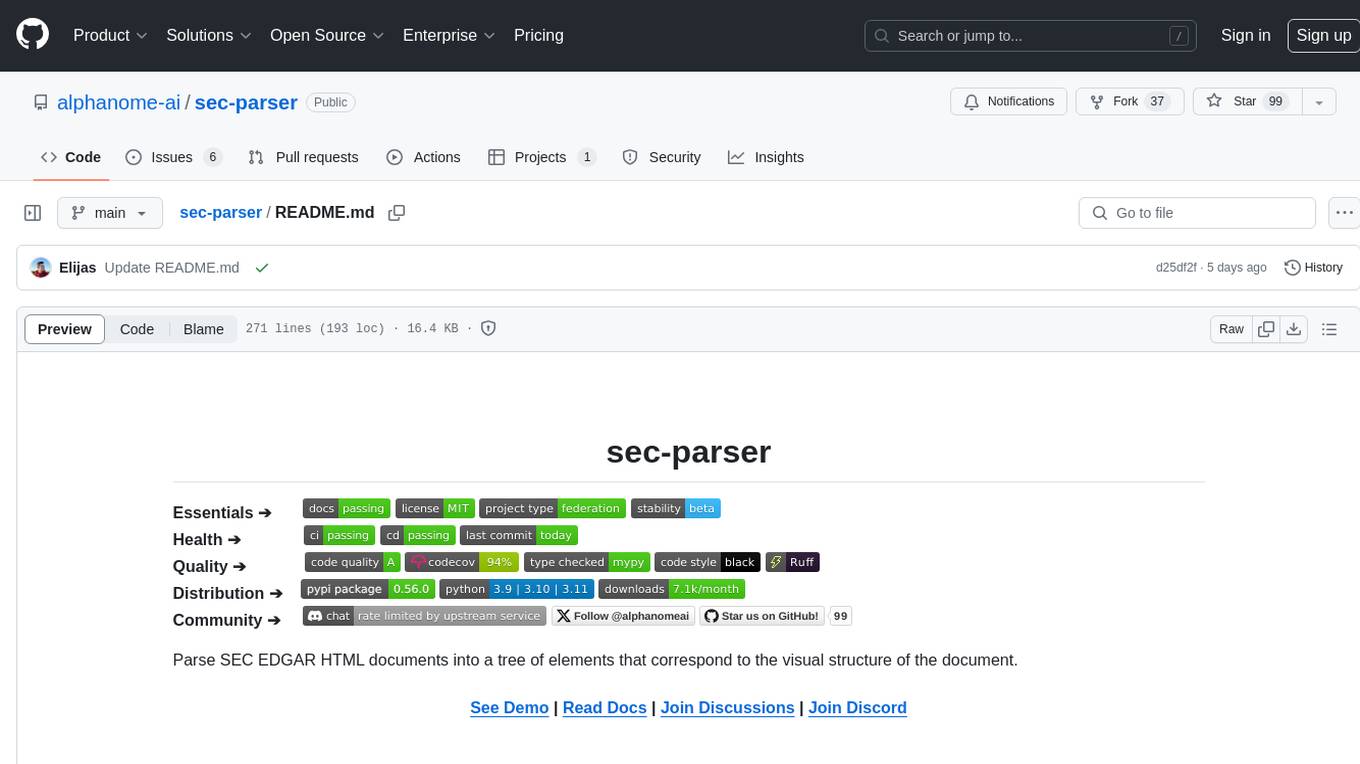
sec-parser
The `sec-parser` project simplifies extracting meaningful information from SEC EDGAR HTML documents by organizing them into semantic elements and a tree structure. It helps in parsing SEC filings for financial and regulatory analysis, analytics and data science, AI and machine learning, causal AI, and large language models. The tool is especially beneficial for AI, ML, and LLM applications by streamlining data pre-processing and feature extraction.
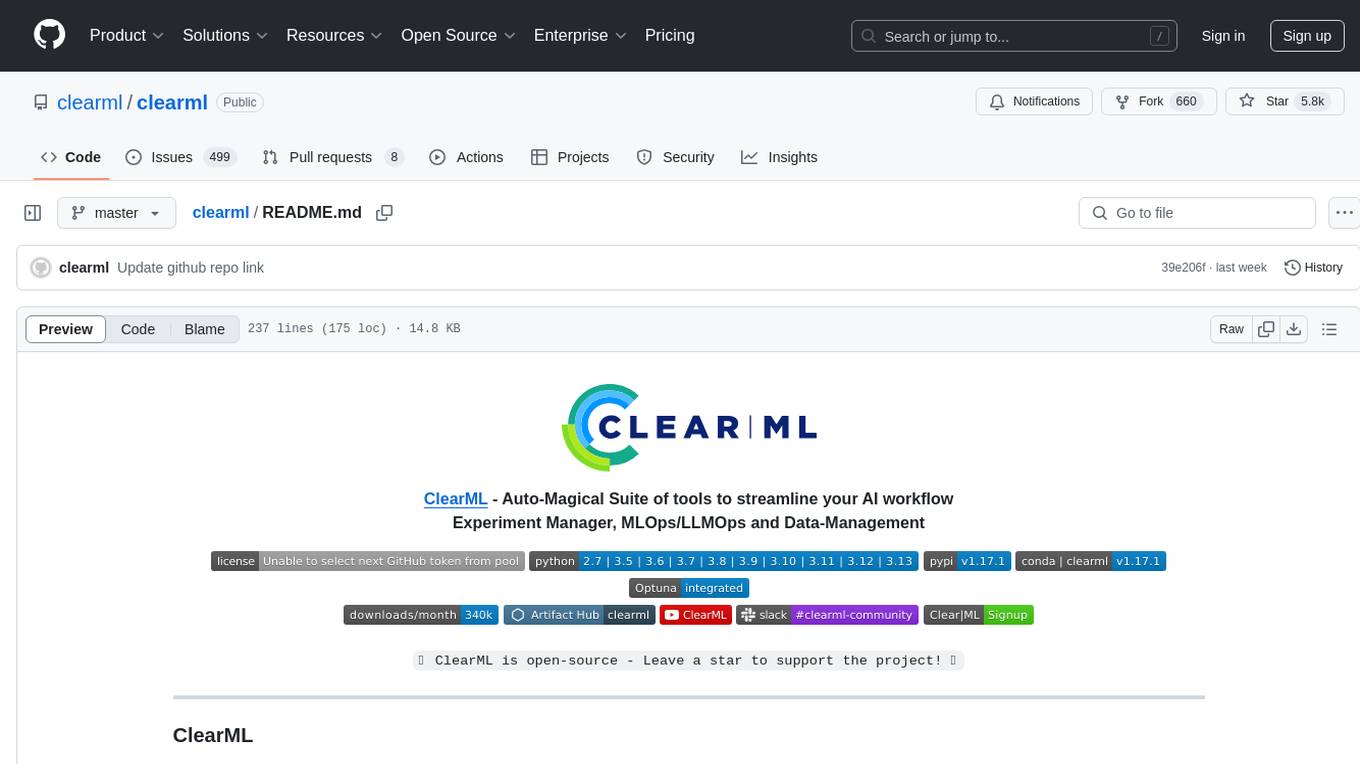
clearml
ClearML is an auto-magical suite of tools designed to streamline AI workflows. It includes modules for experiment management, MLOps/LLMOps, data management, model serving, and more. ClearML offers features like experiment tracking, model serving, orchestration, and automation. It supports various ML/DL frameworks and integrates with Jupyter Notebook and PyCharm for remote debugging. ClearML aims to simplify collaboration, automate processes, and enhance visibility in AI projects.
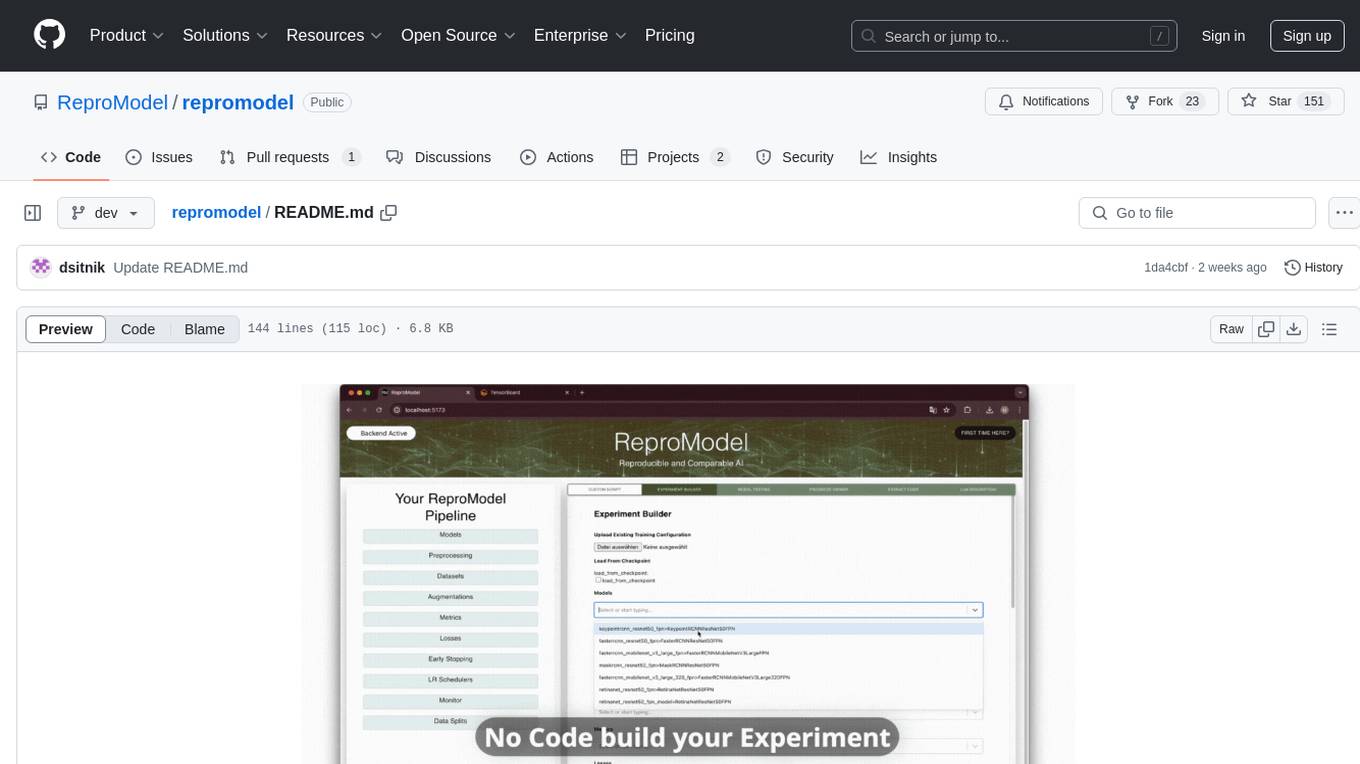
repromodel
ReproModel is an open-source toolbox designed to boost AI research efficiency by enabling researchers to reproduce, compare, train, and test AI models faster. It provides standardized models, dataloaders, and processing procedures, allowing researchers to focus on new datasets and model development. With a no-code solution, users can access benchmark and SOTA models and datasets, utilize training visualizations, extract code for publication, and leverage an LLM-powered automated methodology description writer. The toolbox helps researchers modularize development, compare pipeline performance reproducibly, and reduce time for model development, computation, and writing. Future versions aim to facilitate building upon state-of-the-art research by loading previously published study IDs with verified code, experiments, and results stored in the system.
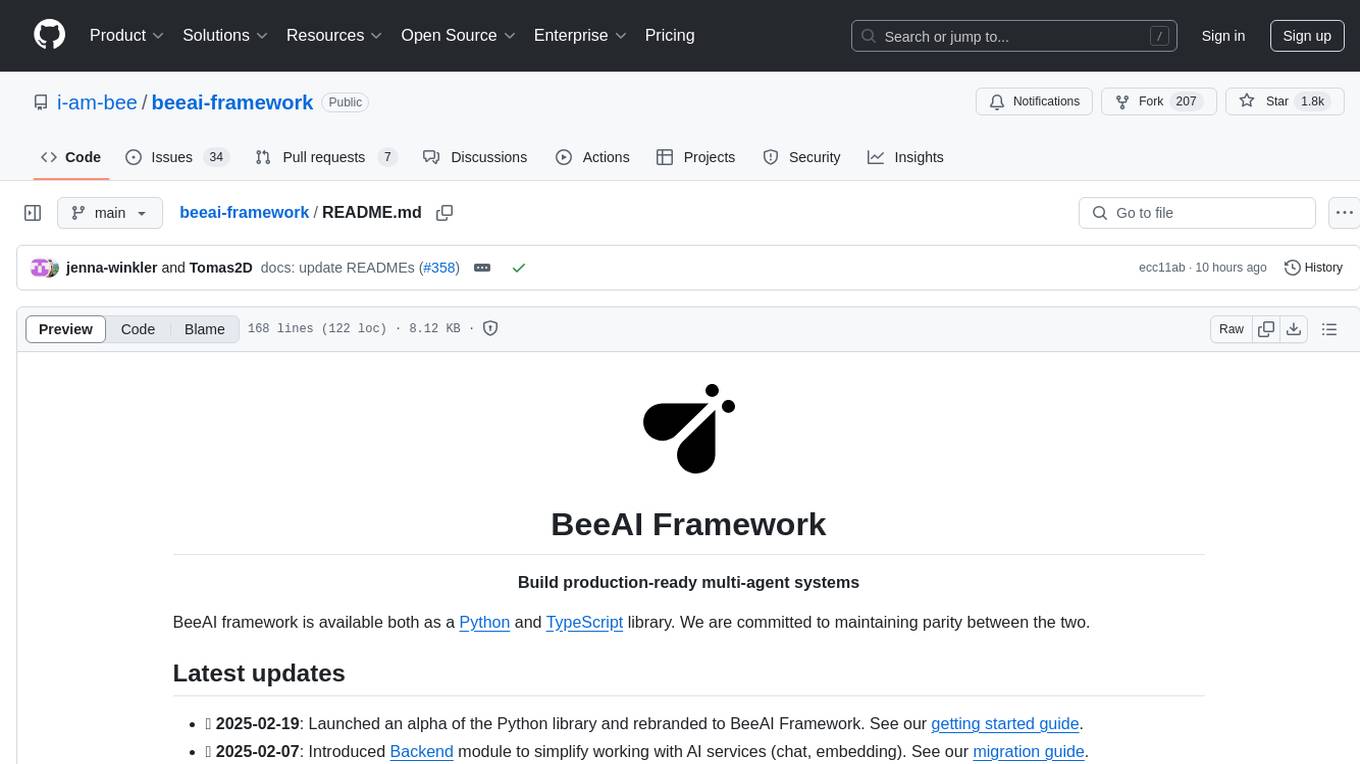
beeai-framework
BeeAI Framework is a versatile tool for building production-ready multi-agent systems. It offers flexibility in orchestrating agents, seamless integration with various models and tools, and production-grade controls for scaling. The framework supports Python and TypeScript libraries, enabling users to implement simple to complex multi-agent patterns, connect with AI services, and optimize token usage and resource management.
For similar tasks
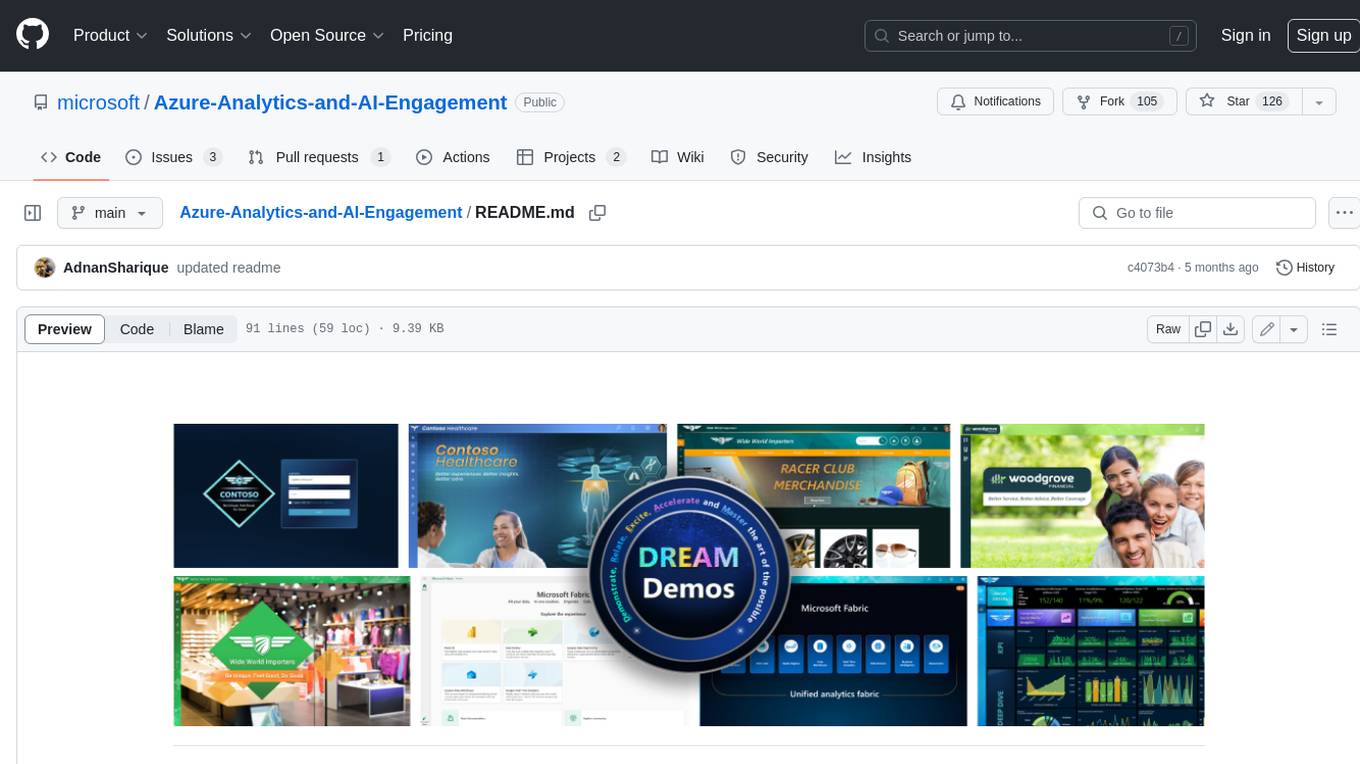
Azure-Analytics-and-AI-Engagement
The Azure-Analytics-and-AI-Engagement repository provides packaged Industry Scenario DREAM Demos with ARM templates (Containing a demo web application, Power BI reports, Synapse resources, AML Notebooks etc.) that can be deployed in a customer’s subscription using the CAPE tool within a matter of few hours. Partners can also deploy DREAM Demos in their own subscriptions using DPoC.
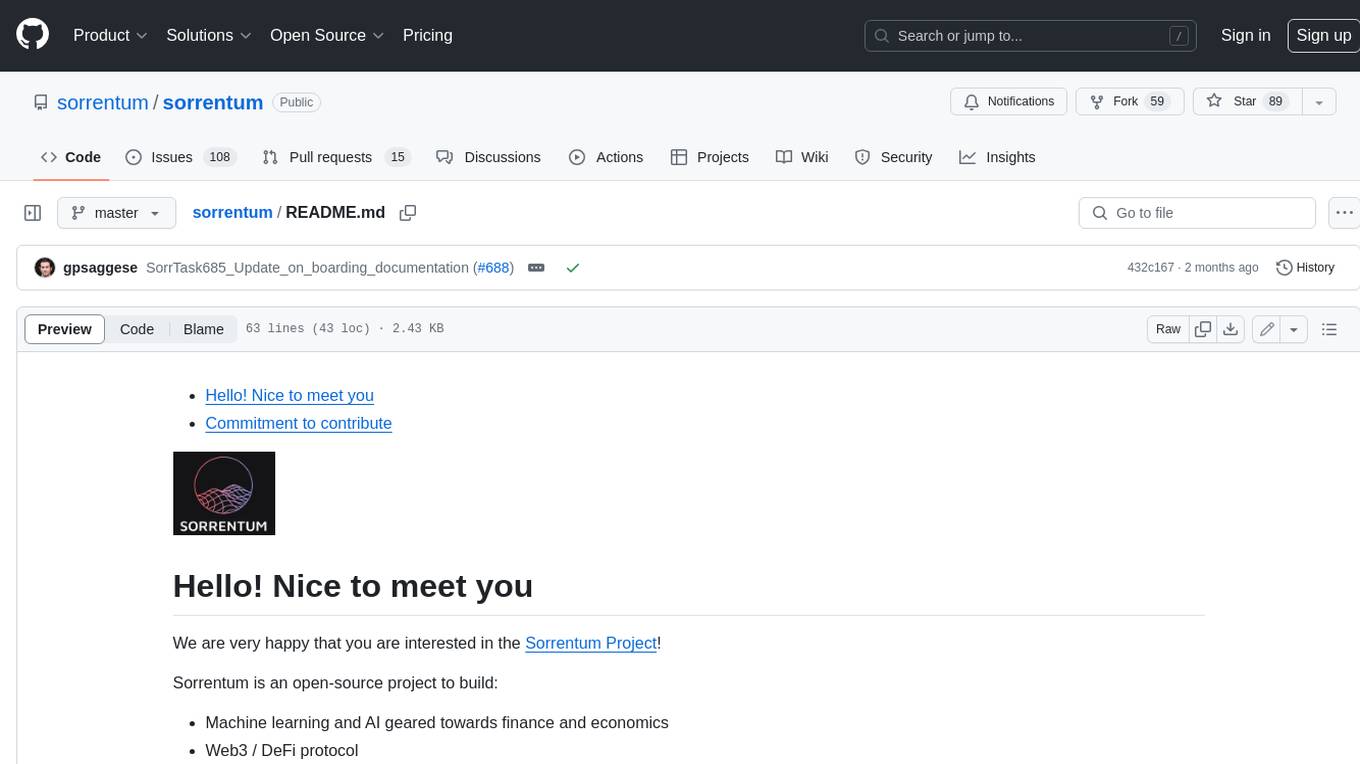
sorrentum
Sorrentum is an open-source project that aims to combine open-source development, startups, and brilliant students to build machine learning, AI, and Web3 / DeFi protocols geared towards finance and economics. The project provides opportunities for internships, research assistantships, and development grants, as well as the chance to work on cutting-edge problems, learn about startups, write academic papers, and get internships and full-time positions at companies working on Sorrentum applications.
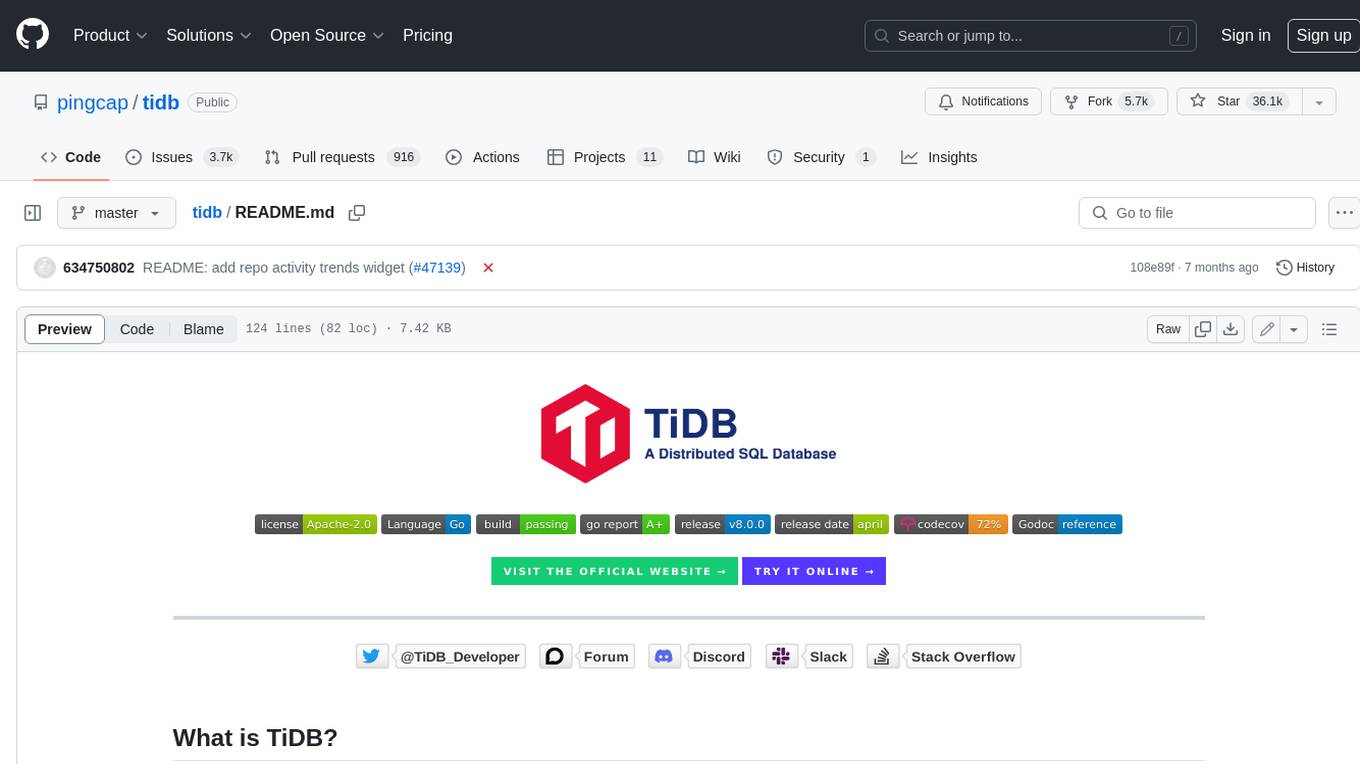
tidb
TiDB is an open-source distributed SQL database that supports Hybrid Transactional and Analytical Processing (HTAP) workloads. It is MySQL compatible and features horizontal scalability, strong consistency, and high availability.
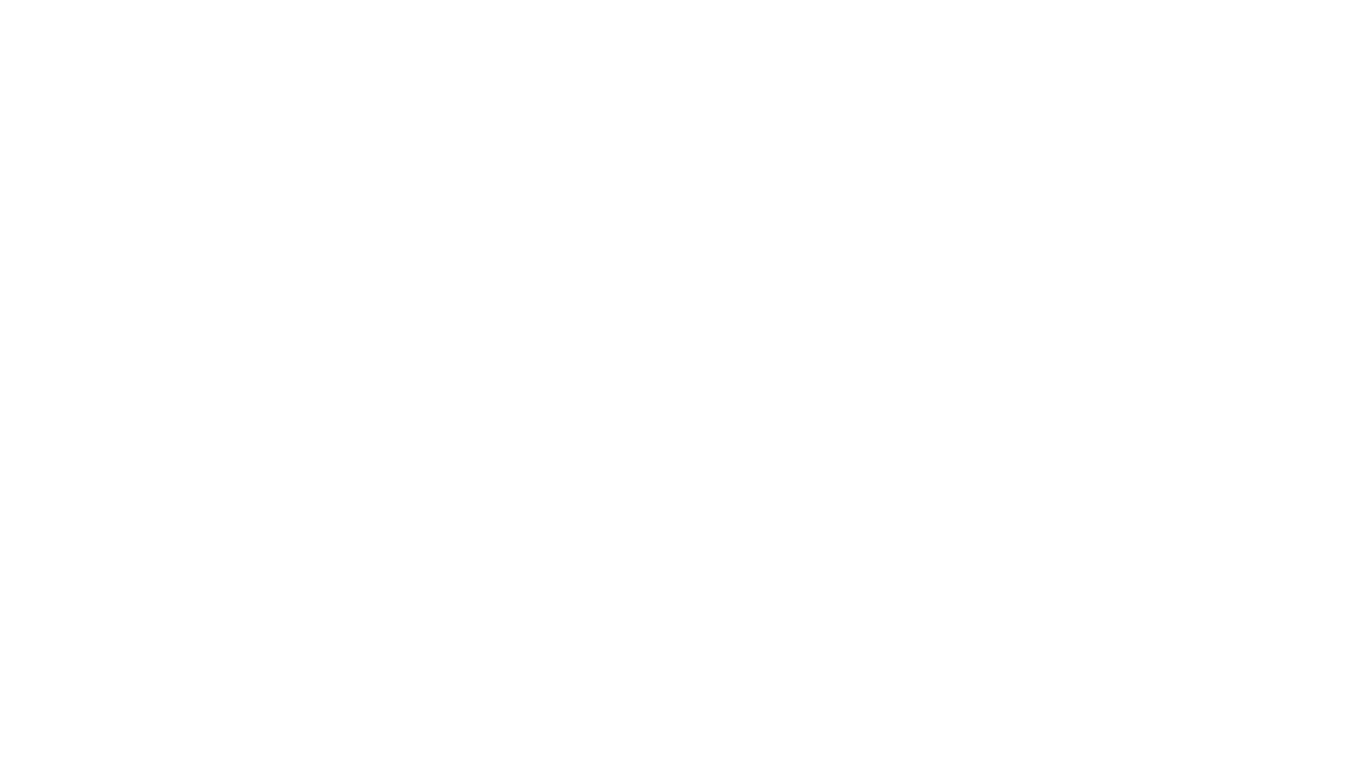
zep-python
Zep is an open-source platform for building and deploying large language model (LLM) applications. It provides a suite of tools and services that make it easy to integrate LLMs into your applications, including chat history memory, embedding, vector search, and data enrichment. Zep is designed to be scalable, reliable, and easy to use, making it a great choice for developers who want to build LLM-powered applications quickly and easily.
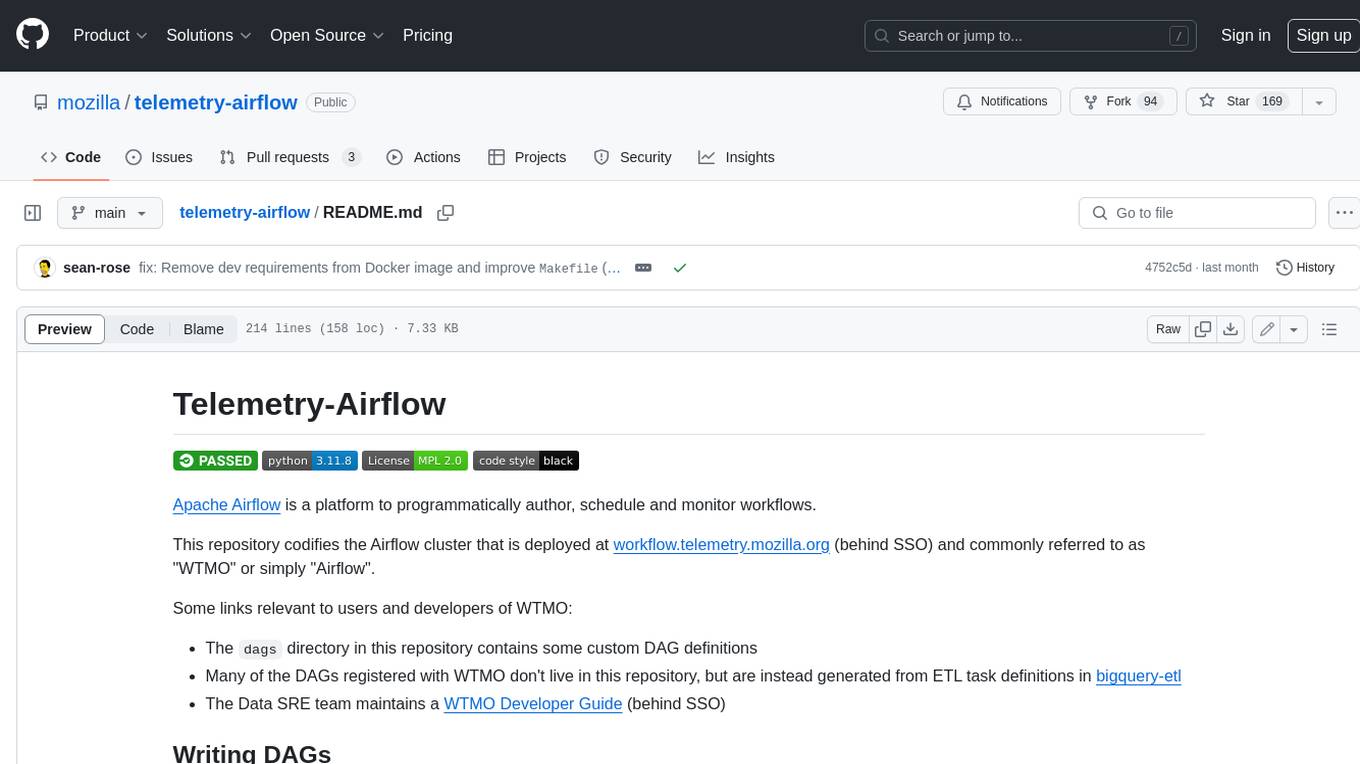
telemetry-airflow
This repository codifies the Airflow cluster that is deployed at workflow.telemetry.mozilla.org (behind SSO) and commonly referred to as "WTMO" or simply "Airflow". Some links relevant to users and developers of WTMO: * The `dags` directory in this repository contains some custom DAG definitions * Many of the DAGs registered with WTMO don't live in this repository, but are instead generated from ETL task definitions in bigquery-etl * The Data SRE team maintains a WTMO Developer Guide (behind SSO)
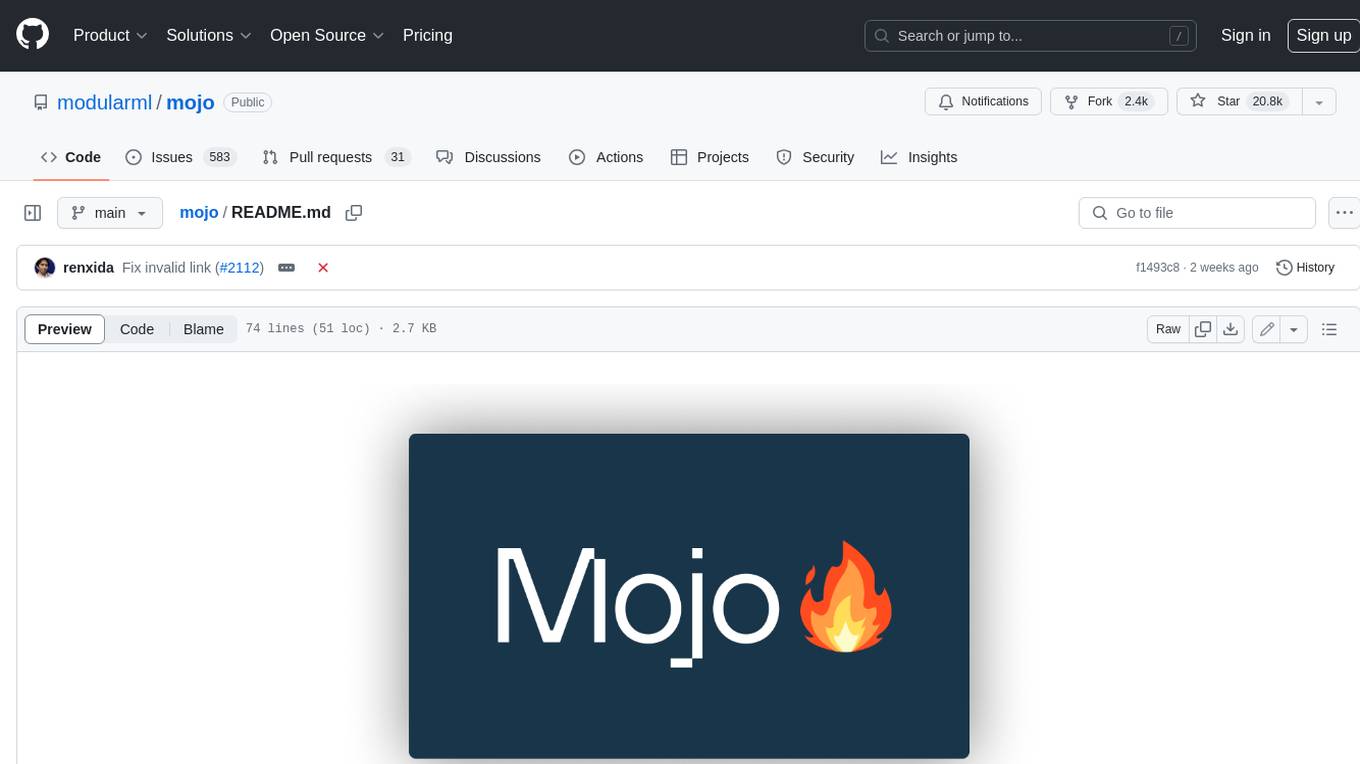
mojo
Mojo is a new programming language that bridges the gap between research and production by combining Python syntax and ecosystem with systems programming and metaprogramming features. Mojo is still young, but it is designed to become a superset of Python over time.
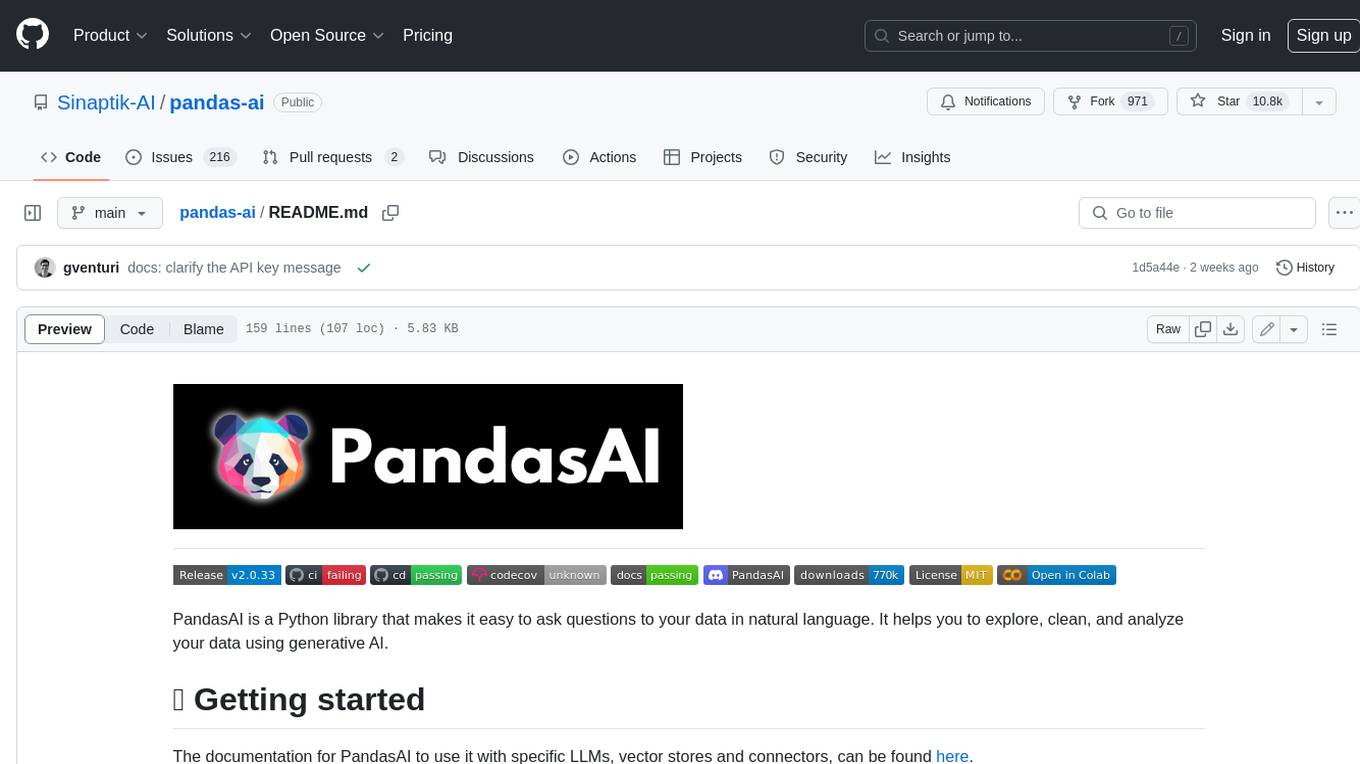
pandas-ai
PandasAI is a Python library that makes it easy to ask questions to your data in natural language. It helps you to explore, clean, and analyze your data using generative AI.
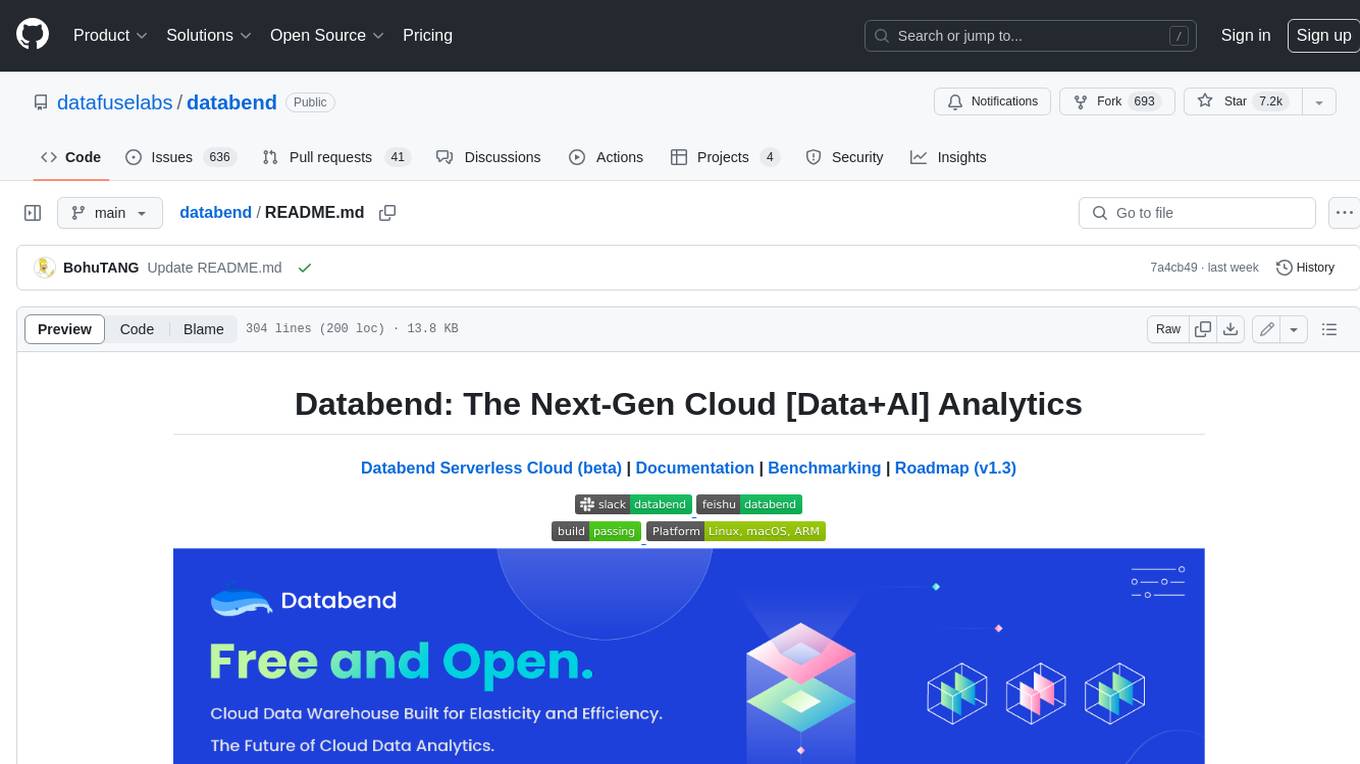
databend
Databend is an open-source cloud data warehouse that serves as a cost-effective alternative to Snowflake. With its focus on fast query execution and data ingestion, it's designed for complex analysis of the world's largest datasets.
For similar jobs
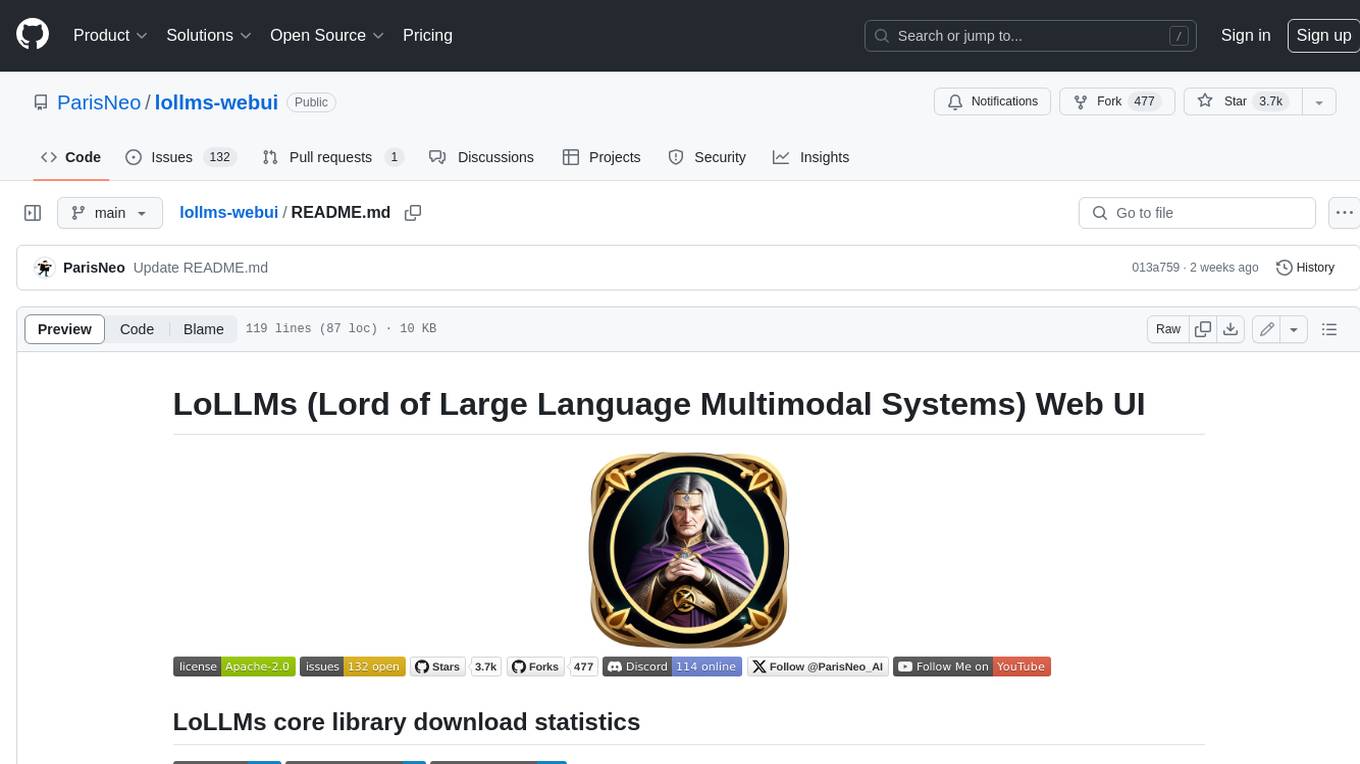
lollms-webui
LoLLMs WebUI (Lord of Large Language Multimodal Systems: One tool to rule them all) is a user-friendly interface to access and utilize various LLM (Large Language Models) and other AI models for a wide range of tasks. With over 500 AI expert conditionings across diverse domains and more than 2500 fine tuned models over multiple domains, LoLLMs WebUI provides an immediate resource for any problem, from car repair to coding assistance, legal matters, medical diagnosis, entertainment, and more. The easy-to-use UI with light and dark mode options, integration with GitHub repository, support for different personalities, and features like thumb up/down rating, copy, edit, and remove messages, local database storage, search, export, and delete multiple discussions, make LoLLMs WebUI a powerful and versatile tool.
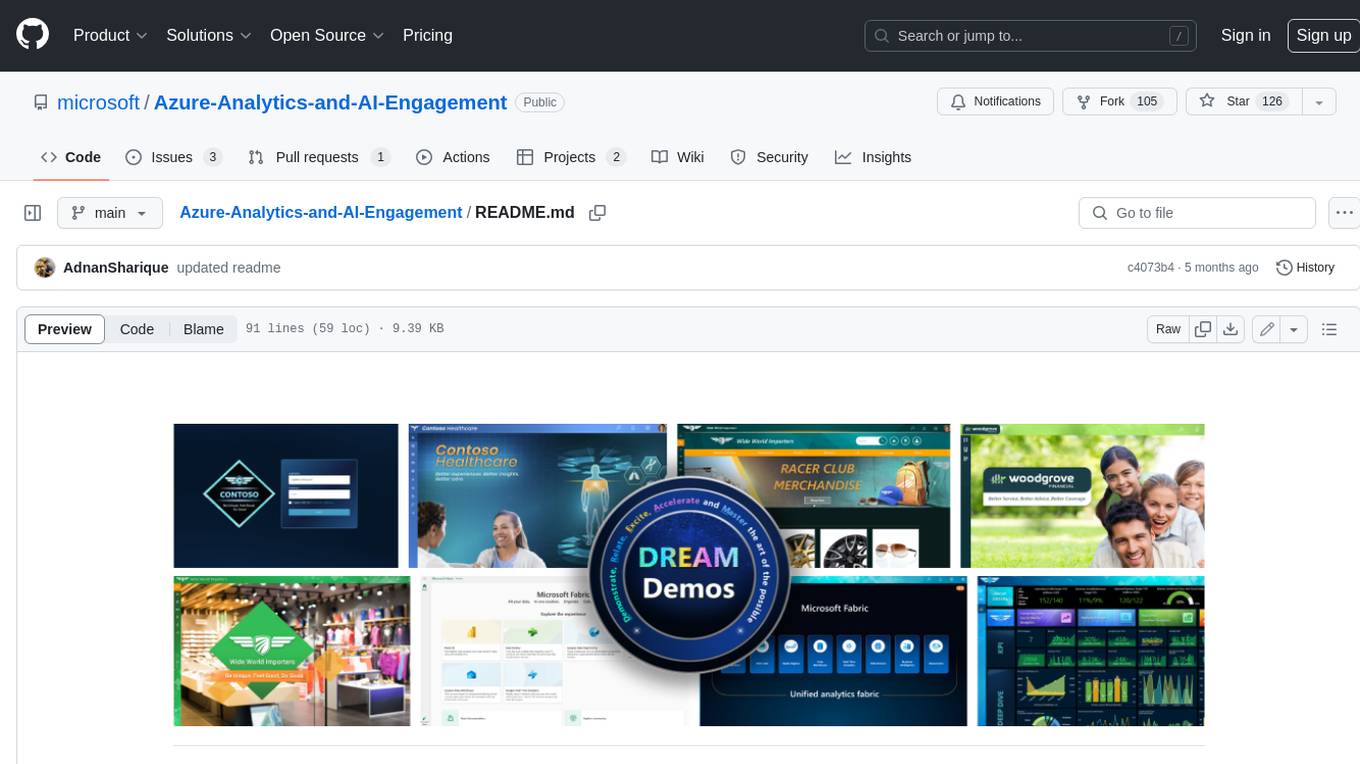
Azure-Analytics-and-AI-Engagement
The Azure-Analytics-and-AI-Engagement repository provides packaged Industry Scenario DREAM Demos with ARM templates (Containing a demo web application, Power BI reports, Synapse resources, AML Notebooks etc.) that can be deployed in a customer’s subscription using the CAPE tool within a matter of few hours. Partners can also deploy DREAM Demos in their own subscriptions using DPoC.
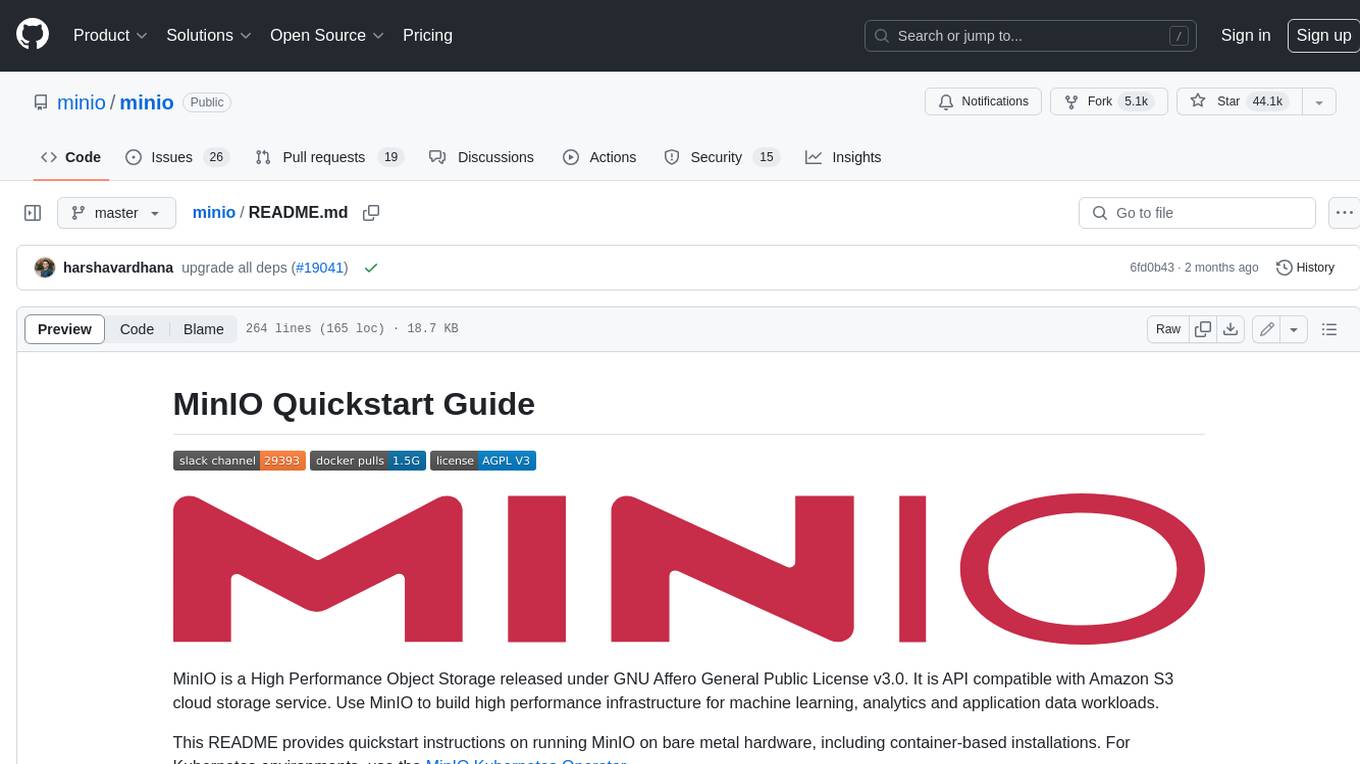
minio
MinIO is a High Performance Object Storage released under GNU Affero General Public License v3.0. It is API compatible with Amazon S3 cloud storage service. Use MinIO to build high performance infrastructure for machine learning, analytics and application data workloads.
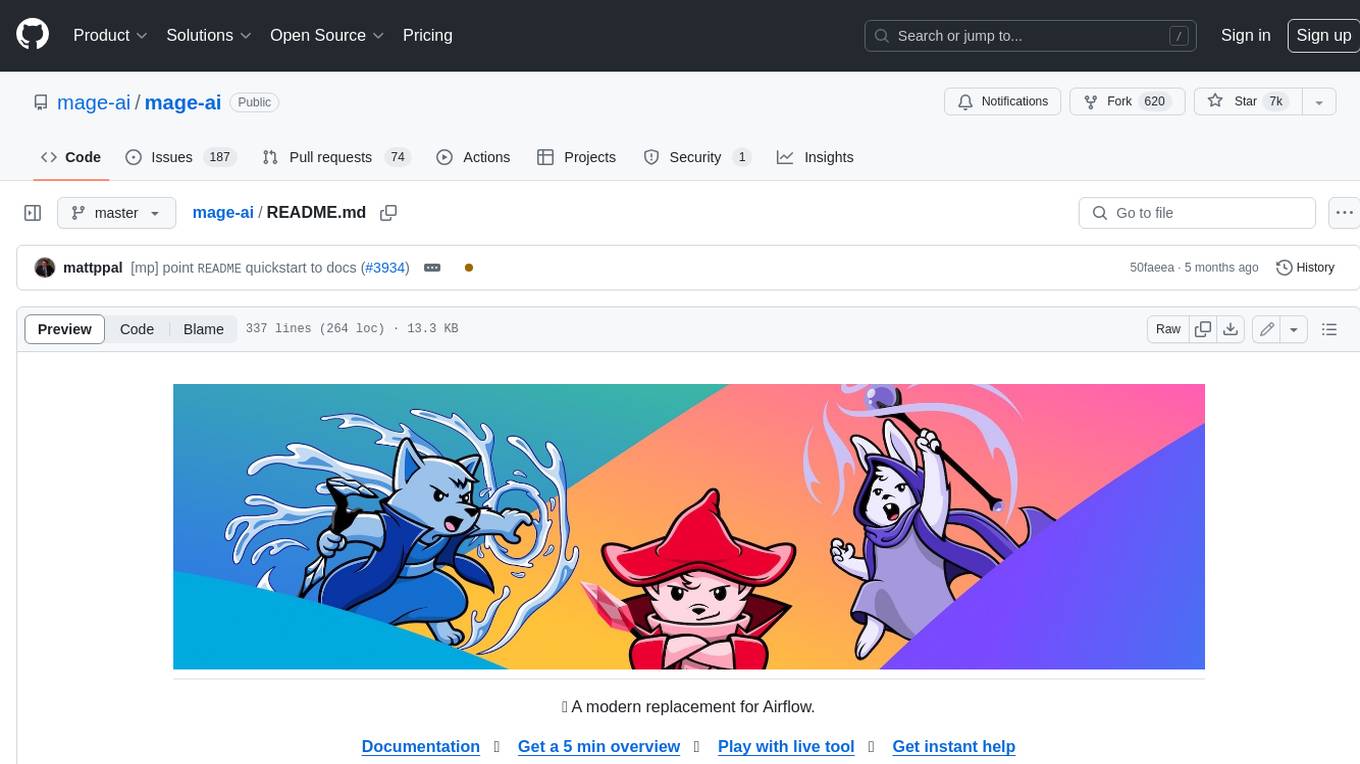
mage-ai
Mage is an open-source data pipeline tool for transforming and integrating data. It offers an easy developer experience, engineering best practices built-in, and data as a first-class citizen. Mage makes it easy to build, preview, and launch data pipelines, and provides observability and scaling capabilities. It supports data integrations, streaming pipelines, and dbt integration.
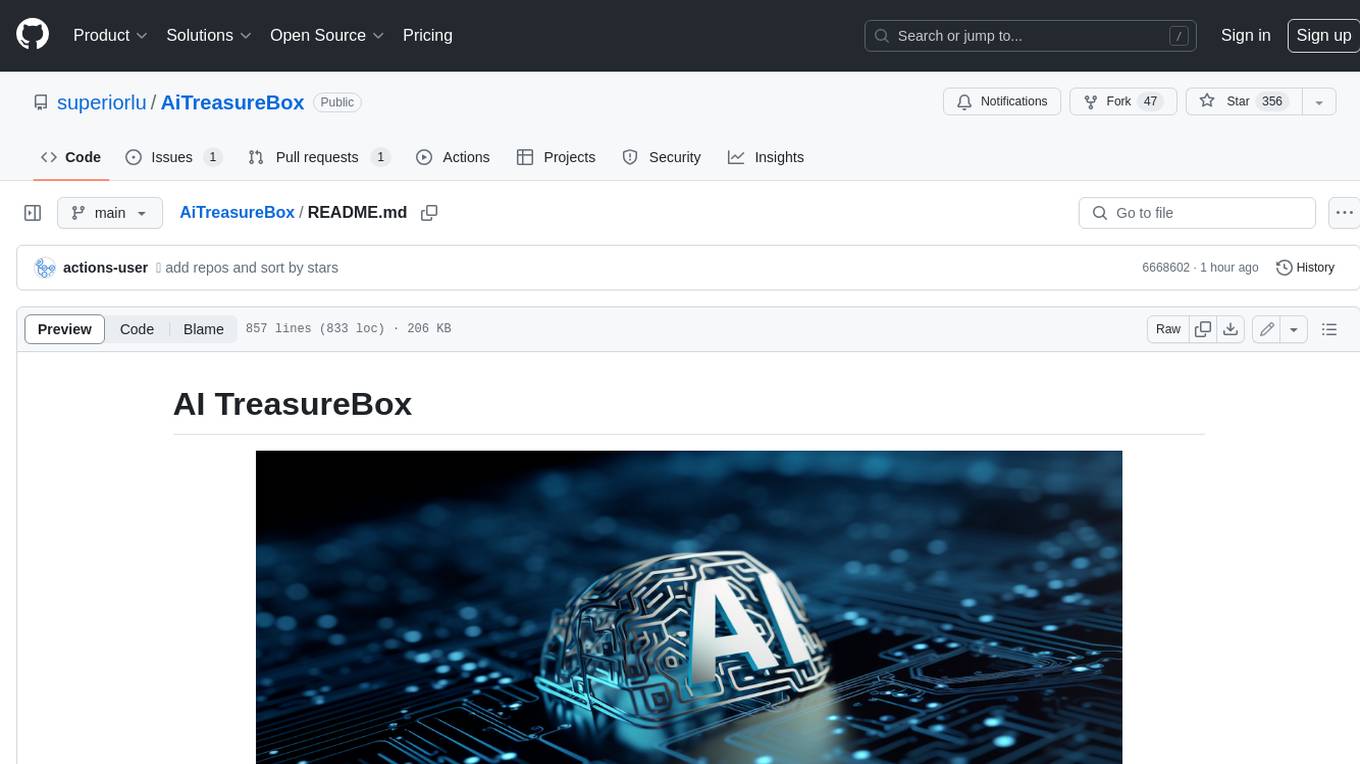
AiTreasureBox
AiTreasureBox is a versatile AI tool that provides a collection of pre-trained models and algorithms for various machine learning tasks. It simplifies the process of implementing AI solutions by offering ready-to-use components that can be easily integrated into projects. With AiTreasureBox, users can quickly prototype and deploy AI applications without the need for extensive knowledge in machine learning or deep learning. The tool covers a wide range of tasks such as image classification, text generation, sentiment analysis, object detection, and more. It is designed to be user-friendly and accessible to both beginners and experienced developers, making AI development more efficient and accessible to a wider audience.
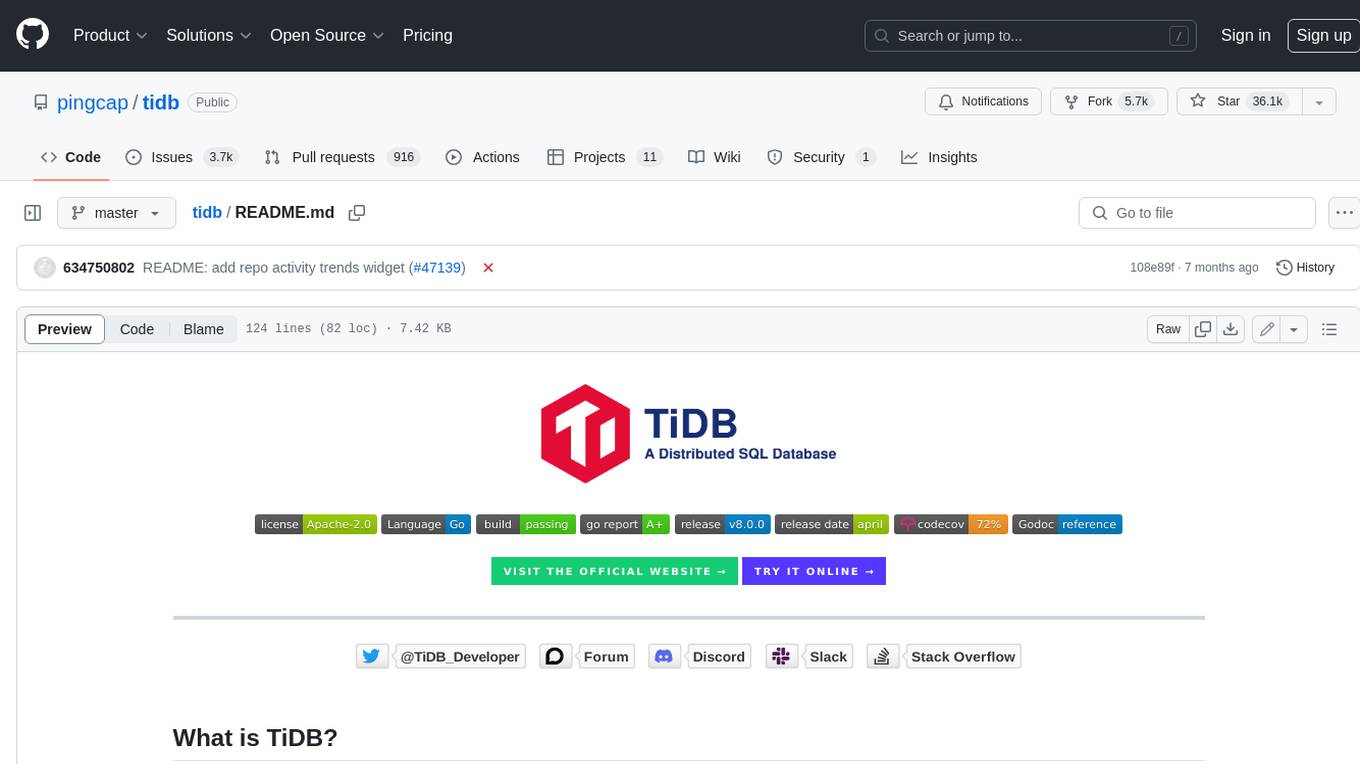
tidb
TiDB is an open-source distributed SQL database that supports Hybrid Transactional and Analytical Processing (HTAP) workloads. It is MySQL compatible and features horizontal scalability, strong consistency, and high availability.
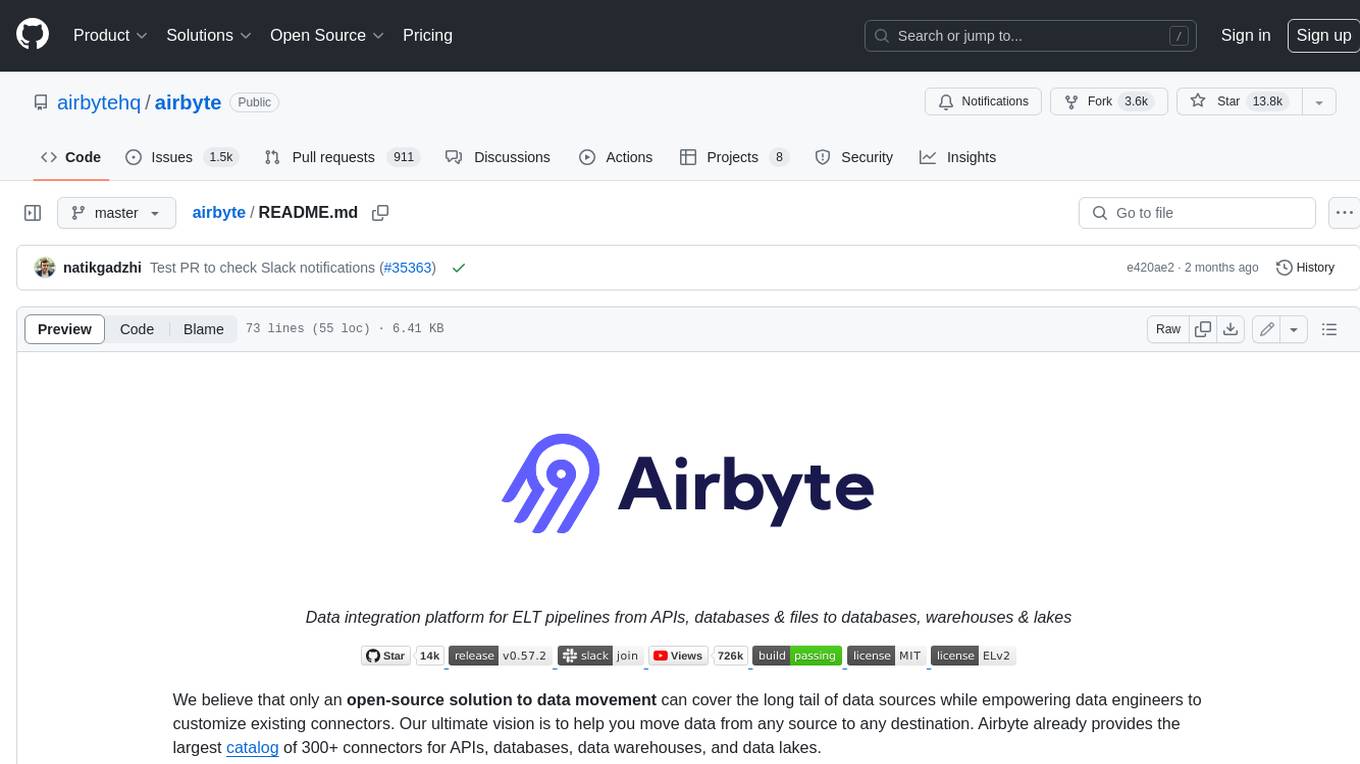
airbyte
Airbyte is an open-source data integration platform that makes it easy to move data from any source to any destination. With Airbyte, you can build and manage data pipelines without writing any code. Airbyte provides a library of pre-built connectors that make it easy to connect to popular data sources and destinations. You can also create your own connectors using Airbyte's no-code Connector Builder or low-code CDK. Airbyte is used by data engineers and analysts at companies of all sizes to build and manage their data pipelines.
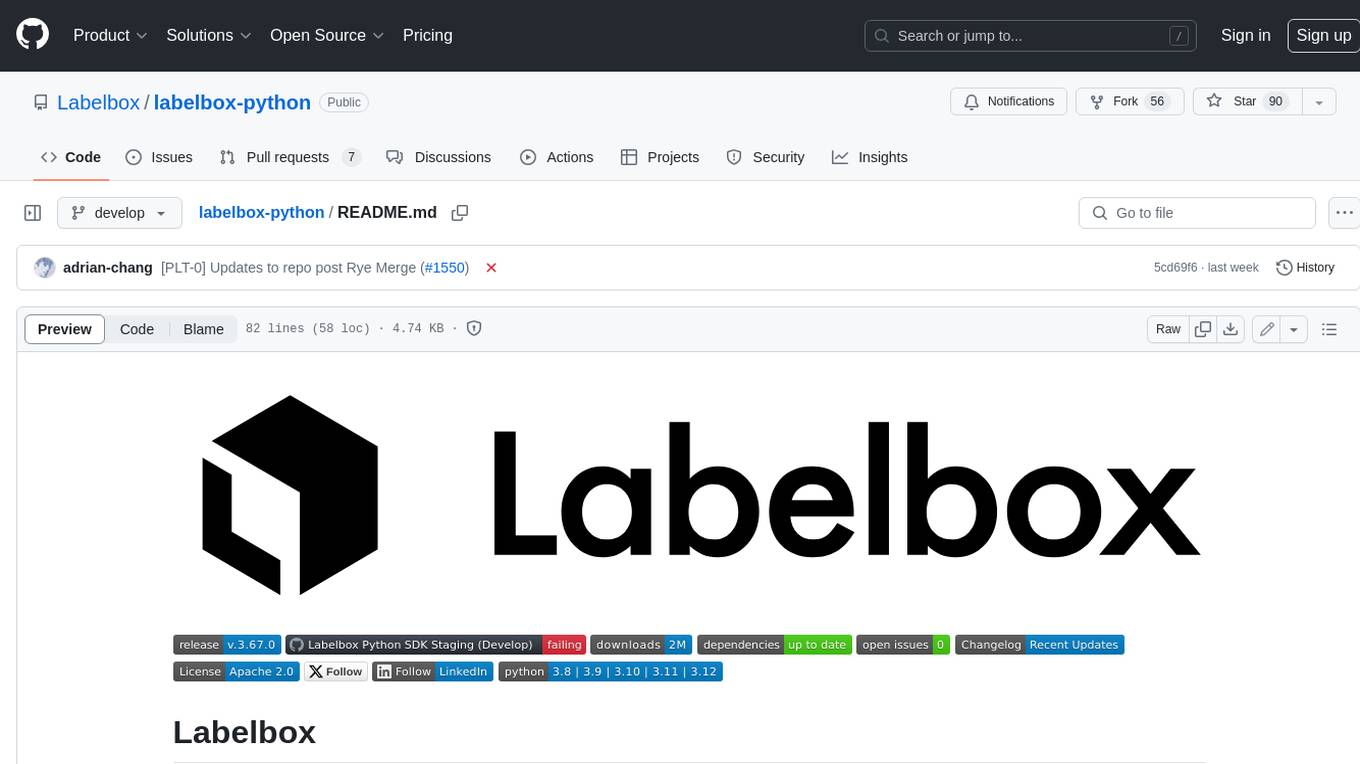
labelbox-python
Labelbox is a data-centric AI platform for enterprises to develop, optimize, and use AI to solve problems and power new products and services. Enterprises use Labelbox to curate data, generate high-quality human feedback data for computer vision and LLMs, evaluate model performance, and automate tasks by combining AI and human-centric workflows. The academic & research community uses Labelbox for cutting-edge AI research.