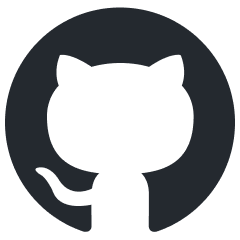
RAGLAB
[EMNLP 2024: Demo Oral] RAGLAB: A Modular and Research-Oriented Unified Framework for Retrieval-Augmented Generation
Stars: 232
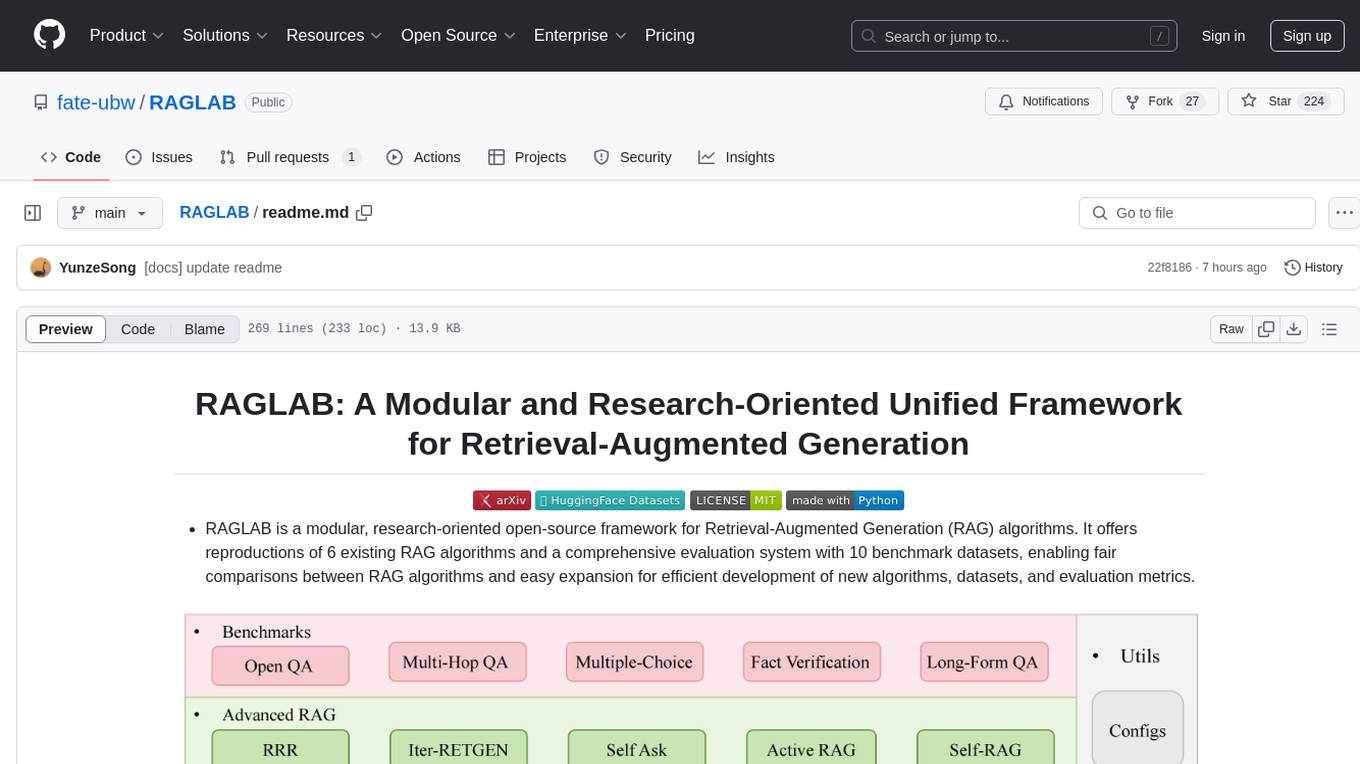
RAGLAB is a modular, research-oriented open-source framework for Retrieval-Augmented Generation (RAG) algorithms. It offers reproductions of 6 existing RAG algorithms and a comprehensive evaluation system with 10 benchmark datasets, enabling fair comparisons between RAG algorithms and easy expansion for efficient development of new algorithms, datasets, and evaluation metrics. The framework supports the entire RAG pipeline, provides advanced algorithm implementations, fair comparison platform, efficient retriever client, versatile generator support, and flexible instruction lab. It also includes features like Interact Mode for quick understanding of algorithms and Evaluation Mode for reproducing paper results and scientific research.
README:
- RAGLAB is a modular, research-oriented open-source framework for Retrieval-Augmented Generation (RAG) algorithms. It offers reproductions of 6 existing RAG algorithms and a comprehensive evaluation system with 10 benchmark datasets, enabling fair comparisons between RAG algorithms and easy expansion for efficient development of new algorithms, datasets, and evaluation metrics.
- 2024.10.6: Our paper has been accepted by EMNLP 2024 System Demonstration.🎉 You can find our paper in RAGLAB.
- 2024.9.9: RAGLAB has open-sourced all log files and evaluation files in evaluation results📌
- 2024.8.20: RAGLAB has open-sourced 4 models🤗: llama3-8B-baseline selfrag-llama3-8b llama3-70B-adaptor selfrag-llama3-70B-adaptor
- 2024.8.6: RAGLAB is released🌈.
- Comprehensive RAG Ecosystem: Supports the entire RAG pipeline from data collection and training to auto-evaluation.
- Advanced Algorithm Implementations: Reproduces 6 state-of-the-art RAG algorithms, with an easy-to-extend framework for developing new algorithms.
- Interact Mode & Evaluation Mode: Interact Mode is specifically designed for quickly understanding algorithms. Evaluation Mode is specifically designed for reproducing paper results and scientific research.
- Fair Comparison Platform: Provides benchmark results for 6 algorithms across 5 task types and 10 datasets.
- Efficient Retriever Client: Offers local API for parallel access and caching, with average latency under 1 second.
- Versatile Generator Support: Compatible with 70B+ models, VLLM, and quantization techniques.
- Flexible Instruction Lab: Customizable instruction templates for various RAG scenarios.
- Interesting RAG applications
-
dev environment:pytorch:2.0.1-py3.10-cuda11.8.0-devel-ubuntu22.04
-
git clone RAGLAB
https://github.com/fate-ubw/RAGLAB.git
-
create environment from yml file
cd RAGLAB conda env create -f environment.yml
-
install flash-attn, en_core_web_sm, punkt manually
pip install flash-attn==2.2 python -m spacy download en_core_web_sm python -m nltk.downloader punkt
raglab need several models please download them
cd RAGLAB
mkdir model
cd model
mkdir output_models
# retriever model
mkdir colbertv2.0
huggingface-cli download colbert-ir/colbertv2.0 --local-dir colbertv2.0/ --local-dir-use-symlinks False
mkdir contriever-msmarco
huggingface-cli download facebook/contriever-msmarco --local-dir contriever-msmarco/ --local-dir-use-symlinks False
# finetuned generator
# 8B model
mkdir Llama3-8B-baseline
huggingface-cli download RAGLAB/Llama3-8B-baseline --local-dir Llama3-8B-baseline/ --local-dir-use-symlinks False
mkdir selfrag_llama3_8b-epoch_0_1
huggingface-cli download RAGLAB/selfrag_llama3-8B --local-dir selfrag_llama3_8b-epoch_0_1/ --local-dir-use-symlinks False
# 70B model
mkdir Llama3-70B-baseline-adapter
huggingface-cli download RAGLAB/Llama3-70B-baseline-adapter --local-dir Llama3-70B-baseline-adapter/ --local-dir-use-symlinks False
mkdir selfrag_llama3_70B-adapter
huggingface-cli download RAGLAB/selfrag_llama3-70B-adapter --local-dir selfrag_llama3_70B-adapter/ --local-dir-use-symlinks False
mkdir Meta-Llama-3-70B
huggingface-cli download meta-llama/Meta-Llama-3-70B --local-dir Meta-Llama-3-70B/ --local-dir-use-symlinks False
# base model for finetune and LoRA
mkdir Meta-Llama-3-8B
huggingface-cli download meta-llama/Meta-Llama-3-8B --local-dir Meta-Llama-3-8B/ --local-dir-use-symlinks False
# ALCE Metric Models
mkdir gpt2-large
huggingface-cli download openai-community/gpt2-large --local-dir gpt2-large/ --local-dir-use-symlinks False
mkdir roberta-large-squad
huggingface-cli download gaotianyu1350/roberta-large-squad --local-dir roberta-large-squad/ --local-dir-use-symlinks False
mkdir t5_xxl_true_nli_mixture
huggingface-cli download google/t5_xxl_true_nli_mixture --local-dir t5_xxl_true_nli_mixture/ --local-dir-use-symlinks False
# factscore model we use gpt3.5 for evaluation, so no need to download local models
# models from official selfrag repo
mkdir selfrag_llama2_7b
huggingface-cli download selfrag/selfrag_llama2_7b --local-dir selfrag_llama2_7b/ --local-dir-use-symlinks False
# you can download other model as generator from huggingface
- If you only need to understand how different algorithms work, the interact mode developed by RAGLAB can meet your needs.
- If you want to reproduce the results from the papers, you need to download all the required data from Hugging Face, including training data, knowledge data, and evaluation data. We have packaged all the data for you, so you just need to download it and it's ready to use.
cd RAGLAB huggingface-cli download RAGLAB/data --local-dir data --repo-type dataset
- Interact Mode is specifically designed for quickly understanding algorithms. In interact mode, you can run various algorithms very quickly, understand the reasoning process of different algorithms, without needing to download any additional data.
- All algorithms integrated in raglab include two modes:
interact
andevaluation
. The test stage demonstrates ininteract
mode, just for demostration and eduction 🤗.
[!NOTE]
- Due to colbert's requirement for absolute paths, you need to modify the index_dbPath and text_dbPath in the config file to use absolute paths.
- Modify the
index_dbPath
andtext_dbPath
in config file:colbert_server-10samples.yamlindex_dbPath: /your_root_path/RAGLAB/data/retrieval/colbertv2.0_embedding/wiki2023-10samples text_dbPath: /your_root_path/RAGLAB/data/retrieval/colbertv2.0_passages/wiki2023-10samples/enwiki-20230401-10samples.tsv
- run colbert server
cd RAGLAB sh run/colbert_server/colbert_server-10samples.sh
[!NOTE]
- At this point, colbert embedding will prompt that due to path errors, colbert embedding needs to be reprocessed. Please enter
yes
and then raglab will automatically help you process the embedding and start the colbert server.
- Now please open another terminal and try to request the colbert server
cd RAGLAB sh run/colbert_server/ask_api.sh
- If a result is returned, it means the colbert server has started successfully! 🌈
- run selfrag (short form & adaptive retrieval) interact mode test 10-samples embedding
cd RAGLAB sh run/rag_inference/3-selfrag_reproduction-interact-short_form-adaptive_retrieval.sh
- Congratulations!!!Now you have already know how to run raglab 🌈
- In raglab, each algorithm has 10 queries built-in in interact mode which are sampled from different benchmarks
[!NOTE]
- remember download wiki2018 konwledge database and model before runing paper results
- Due to colbert's requirement for absolute paths, you need to modify the
index_dbPath
andtext_dbPath
in config file and process the wiki2018 embedding database- Modify the paths in the config file
cd RAGLAB/config/colbert_server vim colbert_server.yaml index_dbPath: {your_root_path}/RAGLAB/data/retrieval/colbertv2.0_embedding/wiki2018 text_dbPath: {your_root_path}/RAGLAB/data/retrieval/colbertv2.0_passages/wiki2018/wiki2018.tsv
- Modify the absolute paths bound in the wiki2018 embedding source file
vim /data/retrieval/colbertv2.0_embedding/wiki2018/indexes/wiki2018/metadata.json # change root path, other parameters do not need to be modified "collection": "/{your_root_path}/RAGLAB/data/retrieval/colbertv2.0_passages/wiki2018/wiki2018.tsv", "experiment": "/{your_root_path}/RAGLAB/data/retrieval/colbertv2.0_embedding/wiki2018",
- Attention: colbert_server need atleast 60GB ram
cd RAGLAB sh run/colbert_server/colbert_server.sh
- open another terminal test your ColBERT server
cd RAGLAB sh run/colbert_server/ask_api.sh
- ColBERT server started successfully!!! 🌈
- inference experiments require running hundreds of scripts in parallel, the automatic gpu scheduler needs to be used to automatically allocate GPUs for different bash scripts in Parallel.
- install
simple_gpu_scheduler
pip install simple_gpu_scheduler
- run hundreds of experiments in one line 😎
cd RAGLAB simple_gpu_scheduler --gpus 0,1,2,3,4,5,6,7 < auto_gpu_scheduling_scripts/auto_run-llama3_8b-baseline-scripts.txt # Other scripts can be run using the same method
- how to write your_script.txt?
- here is an example
# auto_inference_selfreg-7b.txt sh run/rag_inference/selfrag_reproduction/selfrag_reproduction-evaluation-short_form-PubHealth-adaptive_retrieval-pregiven_passages.sh sh run/rag_inference/selfrag_reproduction/selfrag_reproduction-evaluation-short_form-PubHealth-always_retrieval-pregiven_passages.sh
-
RAGLAB includes 3 classic evaluation methods: accuracy, F1, and EM (Exact Match). These 3 methods are simple to calculate, so they can be computed dynamically during the inference process. However, ALCE and Factscore, two advanced metrics, require the completion of the inference process before evaluation.
-
ALCE: RAGLAB has integrated the ALCE repository into RAGLAB. You only need to set the path for the inference results in the config file.
cd RAGLAB cd run/ALCE/ # Change the path in each sh file for the inference generated files # For example: # python ./ALCE/eval.py --f './data/eval_results/ASQA/{your_input_file_path}.jsonl' \ # --mauve \ # --qa simple_gpu_scheduler --gpus 0,1,2,3,4,5,6,7 < auto_gpu_scheduling_scripts/auto_eval_ALCE.txt
-
The evaluation results will be in the same directory as the input file, with the file name suffix
.score
-
Factscore: The Factscore environment requires installation of
torch 1.13.1
, which conflicts with the flash-attn version needed in RAGLAB's training and inference modules. Therefore, RAGLAB currently cannot integrate the Factscore environment, so users need to install the Factscore environment separately for evaluation. -
After installing the Factscore environment, please modify the path of the inference results in the bash file
cd RAGLAB/run/Factscore/ # change the path in each sh file for the inference generated files # For example: # python ./FActScore/factscore/factscorer.py \ # --input_path './data/eval_results/Factscore/{your_input_file_path}.jsonl' \ # --model_name "retrieval+ChatGPT"\ # --openai_key ./api_keys.txt \ # --data_dir ./data/retrieval/colbertv2.0_passages/wiki2023 \ # --verbose simple_gpu_scheduler --gpus 0,1,2,3,4,5,6,7 < auto_gpu_scheduling_scripts/auto_eval_Factscore.txt
-
The evaluation results will be in the same directory as the input file, with the file name suffix
_factscore_output.json
[!NOTE]
- During the Factscore evaluation process, we used GPT-3.5 as the evaluation model, so there's no need to download a local model. If you need to use a local model to evaluate Factscore, please refer to Factscore
- If you wish to process the knowledge database yourself, please refer to the following steps. RAGLAB has already uploaded the processed knowledge database to Hugging Face
- document: process_wiki.md
- This section covers the process of training models in RAGLAB. You can either download all pre-trained models from HuggingFace🤗, or use the tutorial below to train from scratch📝.
- All data provides all data necessary for finetuning.
- document: train_docs.md
If you find this repository useful, please cite our work.
@inproceedings{zhang-etal-2024-raglab,
title = "{RAGLAB}: A Modular and Research-Oriented Unified Framework for Retrieval-Augmented Generation",
author = "Zhang, Xuanwang and
Song, Yunze and
Wang, Yidong and
Tang, Shuyun and
others",
booktitle = "Proceedings of the 2024 Conference on Empirical Methods in Natural Language Processing: System Demonstrations",
month = dec,
year = "2024",
publisher = "Association for Computational Linguistics",
}
RAGLAB is licensed under the MIT License.
For Tasks:
Click tags to check more tools for each tasksFor Jobs:
Alternative AI tools for RAGLAB
Similar Open Source Tools
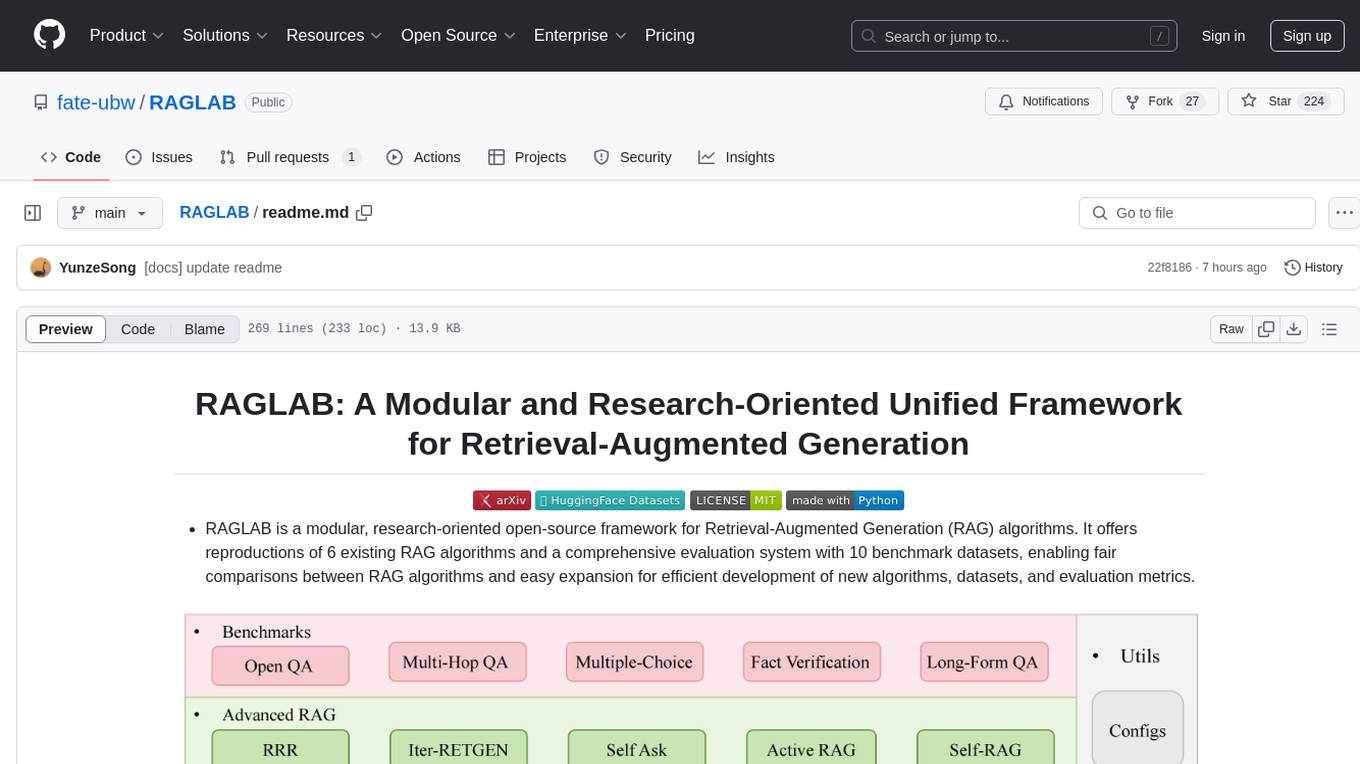
RAGLAB
RAGLAB is a modular, research-oriented open-source framework for Retrieval-Augmented Generation (RAG) algorithms. It offers reproductions of 6 existing RAG algorithms and a comprehensive evaluation system with 10 benchmark datasets, enabling fair comparisons between RAG algorithms and easy expansion for efficient development of new algorithms, datasets, and evaluation metrics. The framework supports the entire RAG pipeline, provides advanced algorithm implementations, fair comparison platform, efficient retriever client, versatile generator support, and flexible instruction lab. It also includes features like Interact Mode for quick understanding of algorithms and Evaluation Mode for reproducing paper results and scientific research.
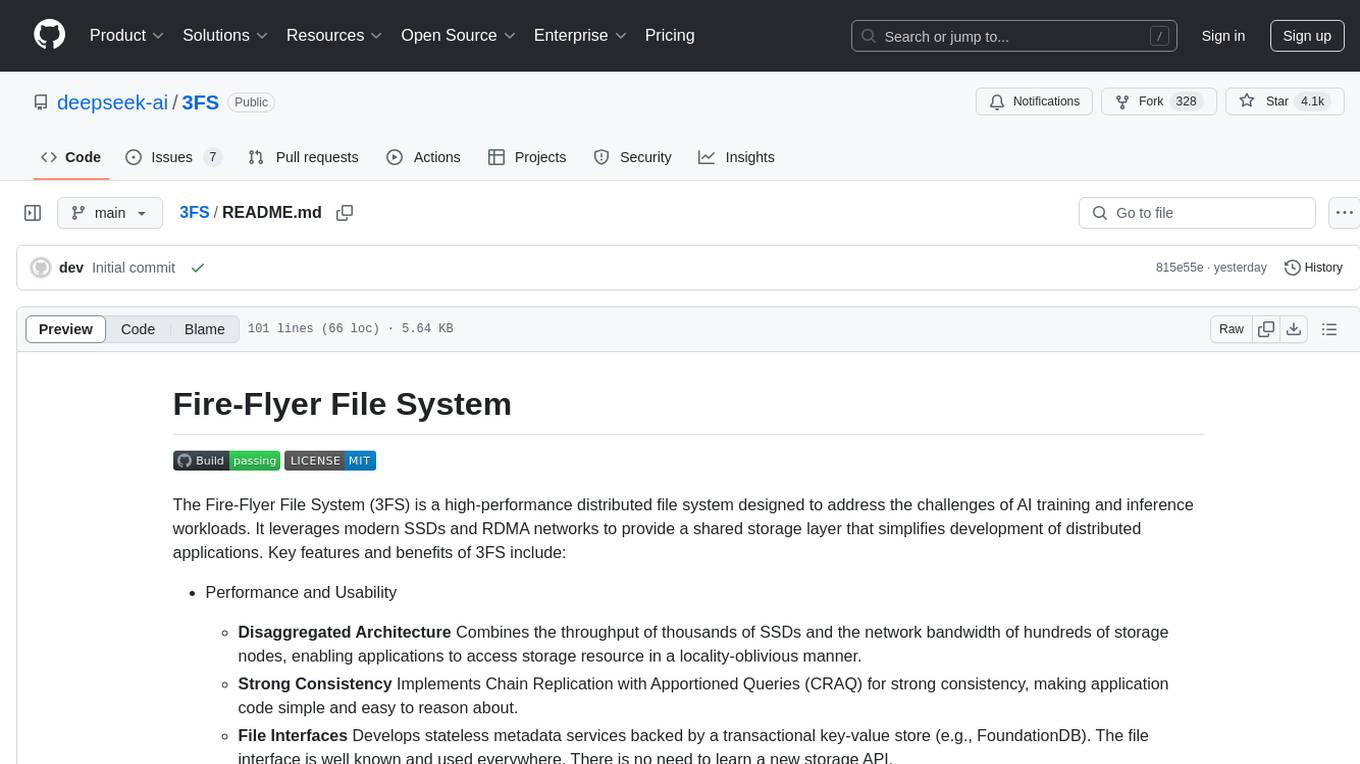
3FS
The Fire-Flyer File System (3FS) is a high-performance distributed file system designed for AI training and inference workloads. It leverages modern SSDs and RDMA networks to provide a shared storage layer that simplifies development of distributed applications. Key features include performance, disaggregated architecture, strong consistency, file interfaces, data preparation, dataloaders, checkpointing, and KVCache for inference. The system is well-documented with design notes, setup guide, USRBIO API reference, and P specifications. Performance metrics include peak throughput, GraySort benchmark results, and KVCache optimization. The source code is available on GitHub for cloning and installation of dependencies. Users can build 3FS and run test clusters following the provided instructions. Issues can be reported on the GitHub repository.
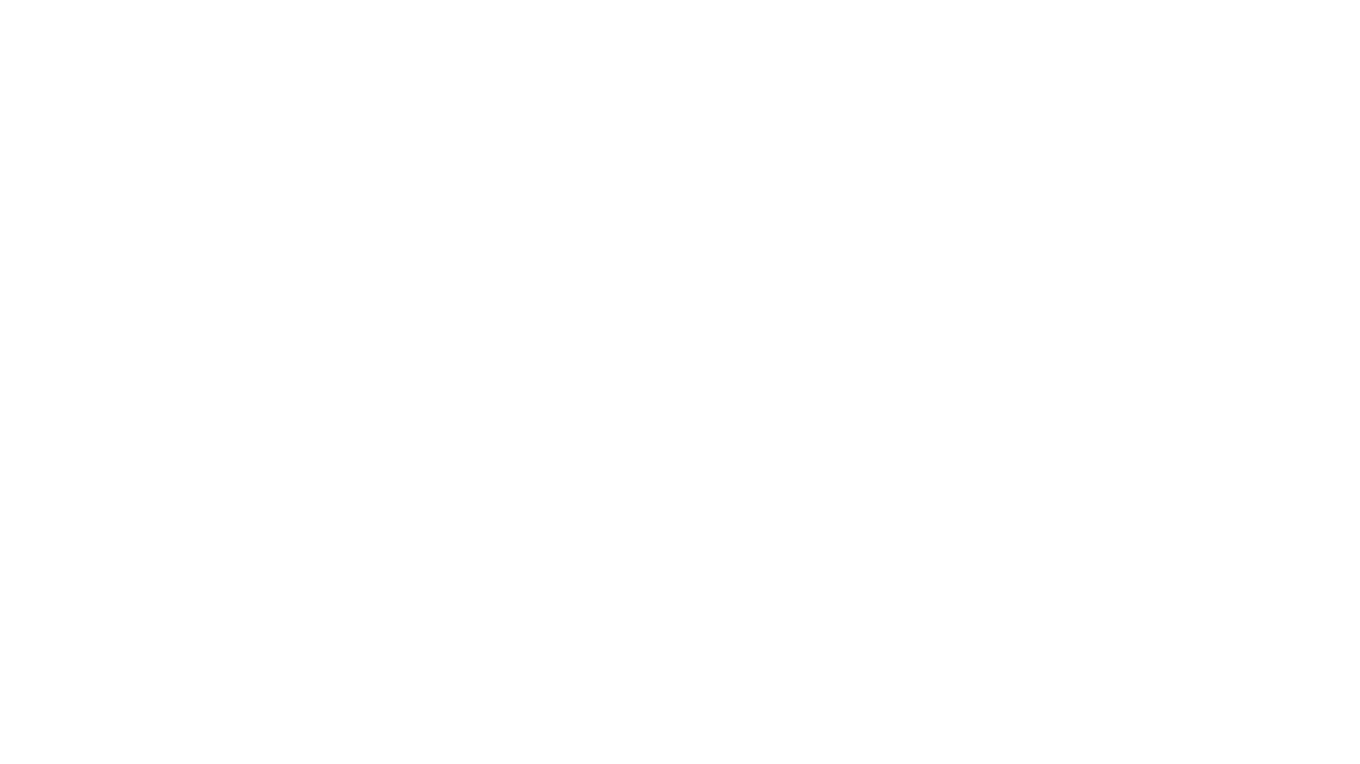
TestSpark
TestSpark is a plugin for generating unit tests that integrates AI-based test generation tools. It supports LLM-based test generation using OpenAI, HuggingFace, and JetBrains internal AI Assistant platform, as well as local search-based test generation using EvoSuite. Users can configure test generation settings, interact with test cases, view coverage statistics, and integrate tests into projects. The plugin is designed for experimental use to augment existing test suites, not replace manual test writing.
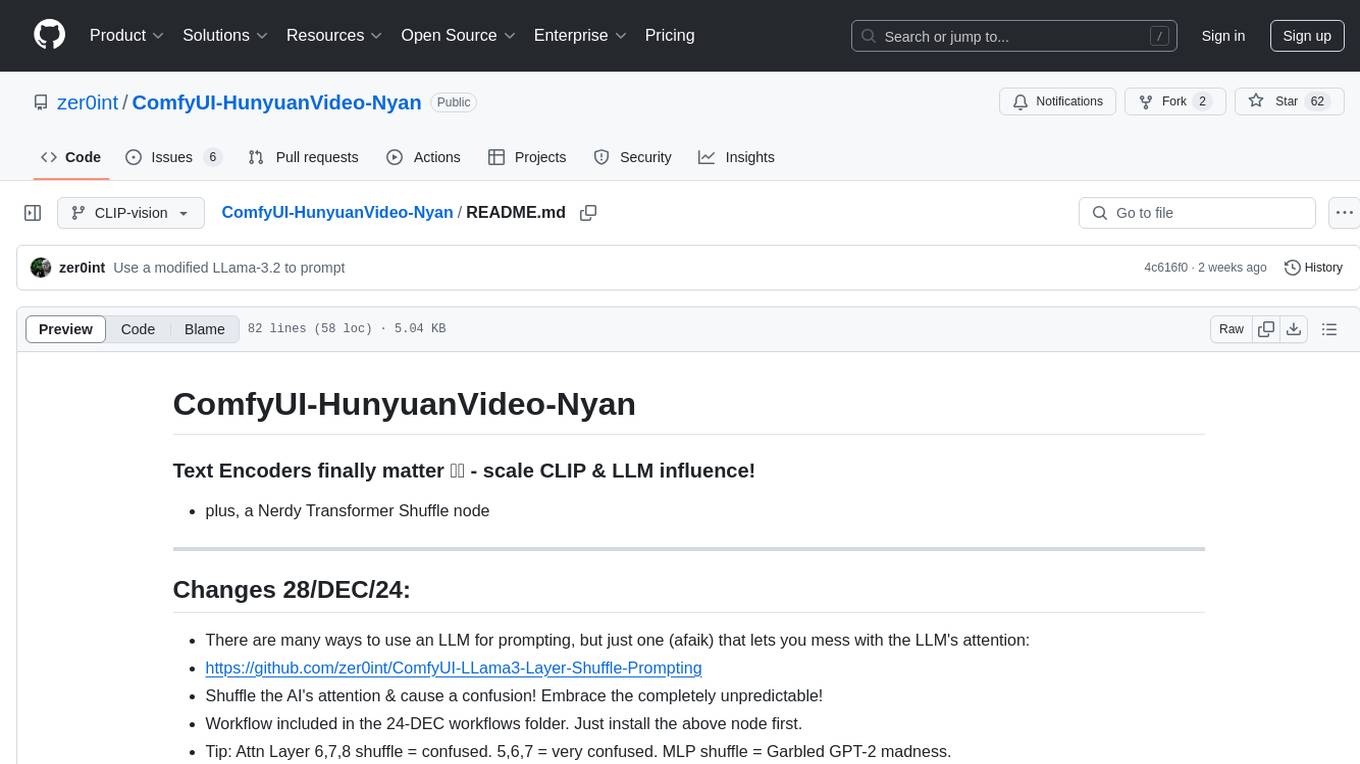
ComfyUI-HunyuanVideo-Nyan
ComfyUI-HunyuanVideo-Nyan is a repository that provides tools for manipulating the attention of LLM models, allowing users to shuffle the AI's attention and cause confusion. The repository includes a Nerdy Transformer Shuffle node that enables users to mess with the LLM's attention layers, providing a workflow for installation and usage. It also offers a new SAE-informed Long-CLIP model with high accuracy, along with recommendations for CLIP models. Users can find detailed instructions on how to use the provided nodes to scale CLIP & LLM factors and create high-quality nature videos. The repository emphasizes compatibility with other related tools and provides insights into the functionality of the included nodes.
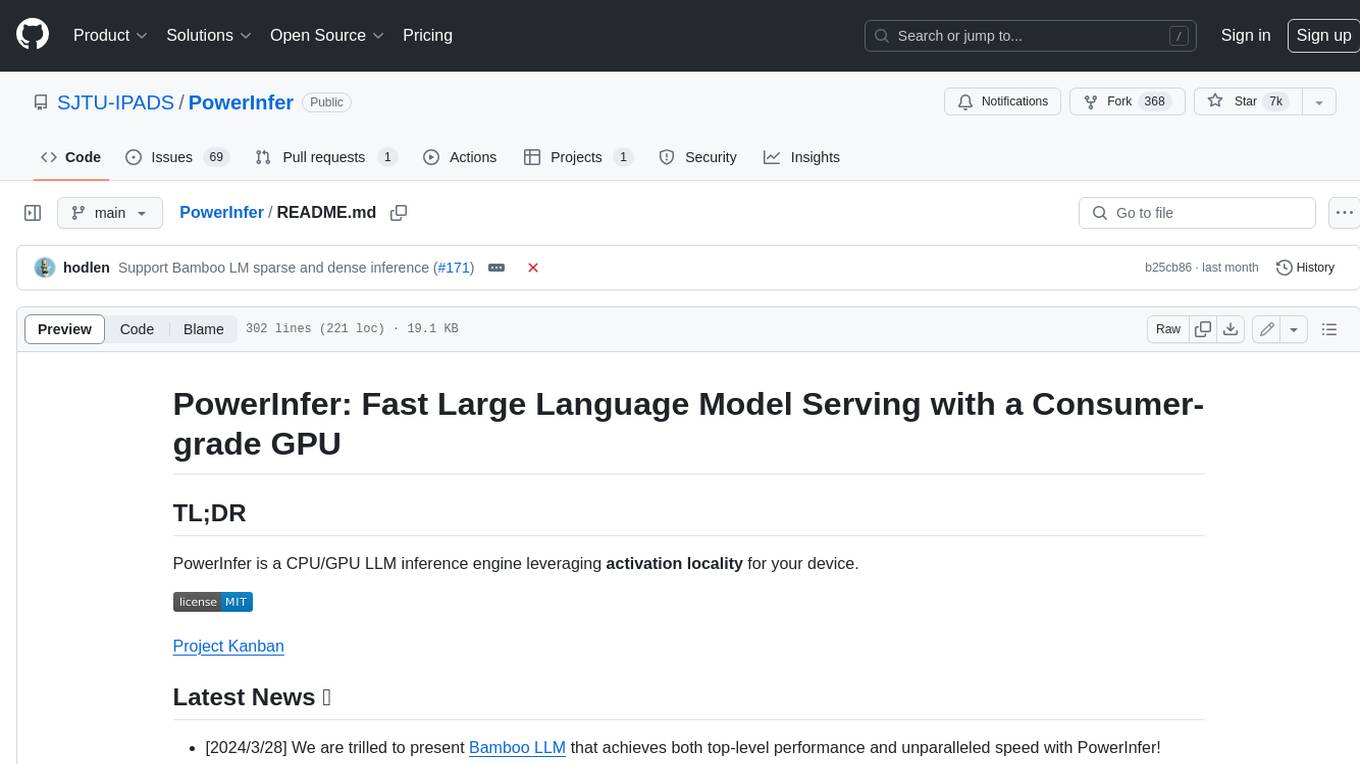
PowerInfer
PowerInfer is a high-speed Large Language Model (LLM) inference engine designed for local deployment on consumer-grade hardware, leveraging activation locality to optimize efficiency. It features a locality-centric design, hybrid CPU/GPU utilization, easy integration with popular ReLU-sparse models, and support for various platforms. PowerInfer achieves high speed with lower resource demands and is flexible for easy deployment and compatibility with existing models like Falcon-40B, Llama2 family, ProSparse Llama2 family, and Bamboo-7B.
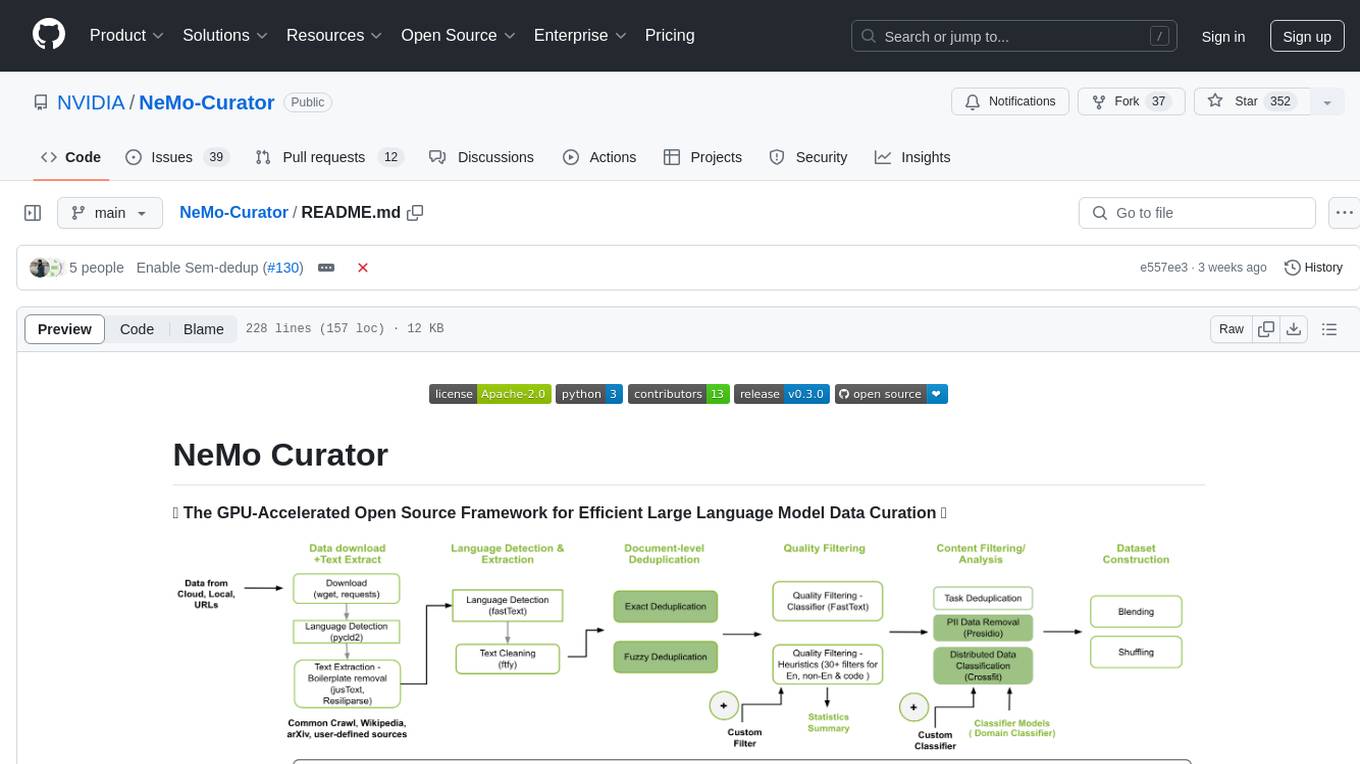
NeMo-Curator
NeMo Curator is a GPU-accelerated open-source framework designed for efficient large language model data curation. It provides scalable dataset preparation for tasks like foundation model pretraining, domain-adaptive pretraining, supervised fine-tuning, and parameter-efficient fine-tuning. The library leverages GPUs with Dask and RAPIDS to accelerate data curation, offering customizable and modular interfaces for pipeline expansion and model convergence. Key features include data download, text extraction, quality filtering, deduplication, downstream-task decontamination, distributed data classification, and PII redaction. NeMo Curator is suitable for curating high-quality datasets for large language model training.
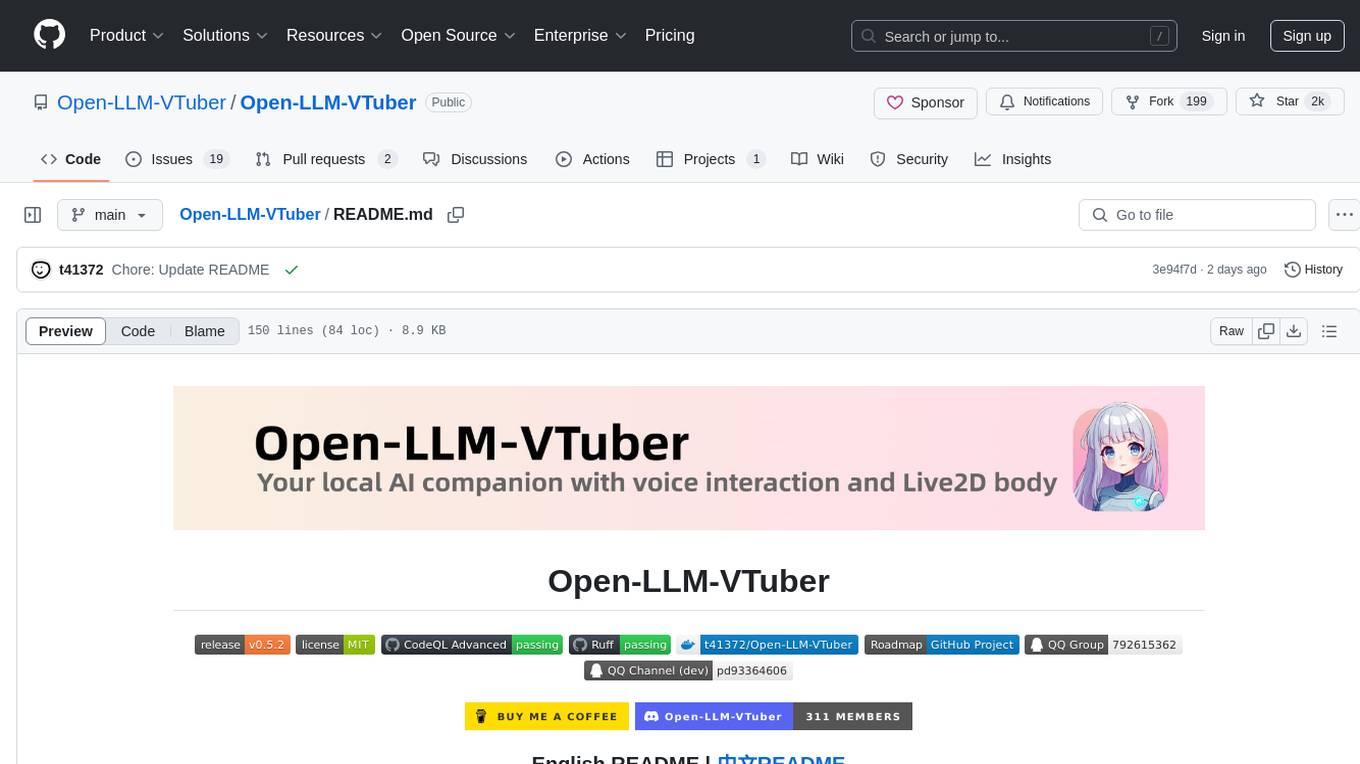
Open-LLM-VTuber
Open-LLM-VTuber is a voice-interactive AI companion supporting real-time voice conversations and featuring a Live2D avatar. It can run offline on Windows, macOS, and Linux, offering web and desktop client modes. Users can customize appearance and persona, with rich LLM inference, text-to-speech, and speech recognition support. The project is highly customizable, extensible, and actively developed with exciting features planned. It provides privacy with offline mode, persistent chat logs, and various interaction features like voice interruption, touch feedback, Live2D expressions, pet mode, and more.
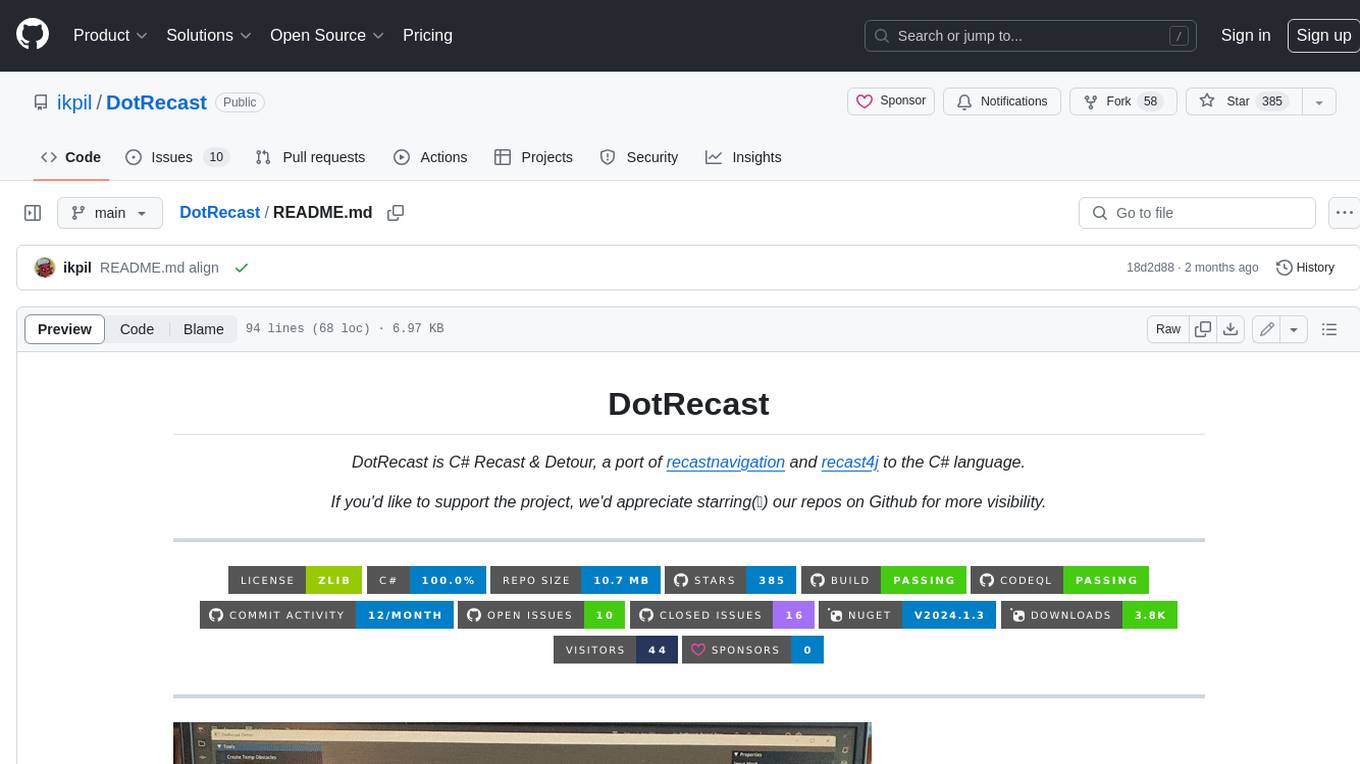
DotRecast
DotRecast is a C# port of Recast & Detour, a navigation library used in many AAA and indie games and engines. It provides automatic navmesh generation, fast turnaround times, detailed customization options, and is dependency-free. Recast Navigation is divided into multiple modules, each contained in its own folder: - DotRecast.Core: Core utils - DotRecast.Recast: Navmesh generation - DotRecast.Detour: Runtime loading of navmesh data, pathfinding, navmesh queries - DotRecast.Detour.TileCache: Navmesh streaming. Useful for large levels and open-world games - DotRecast.Detour.Crowd: Agent movement, collision avoidance, and crowd simulation - DotRecast.Detour.Dynamic: Robust support for dynamic nav meshes combining pre-built voxels with dynamic objects which can be freely added and removed - DotRecast.Detour.Extras: Simple tool to import navmeshes created with A* Pathfinding Project - DotRecast.Recast.Toolset: All modules - DotRecast.Recast.Demo: Standalone, comprehensive demo app showcasing all aspects of Recast & Detour's functionality - Tests: Unit tests Recast constructs a navmesh through a multi-step mesh rasterization process: 1. First Recast rasterizes the input triangle meshes into voxels. 2. Voxels in areas where agents would not be able to move are filtered and removed. 3. The walkable areas described by the voxel grid are then divided into sets of polygonal regions. 4. The navigation polygons are generated by re-triangulating the generated polygonal regions into a navmesh. You can use Recast to build a single navmesh, or a tiled navmesh. Single meshes are suitable for many simple, static cases and are easy to work with. Tiled navmeshes are more complex to work with but better support larger, more dynamic environments. Tiled meshes enable advanced Detour features like re-baking, hierarchical path-planning, and navmesh data-streaming.
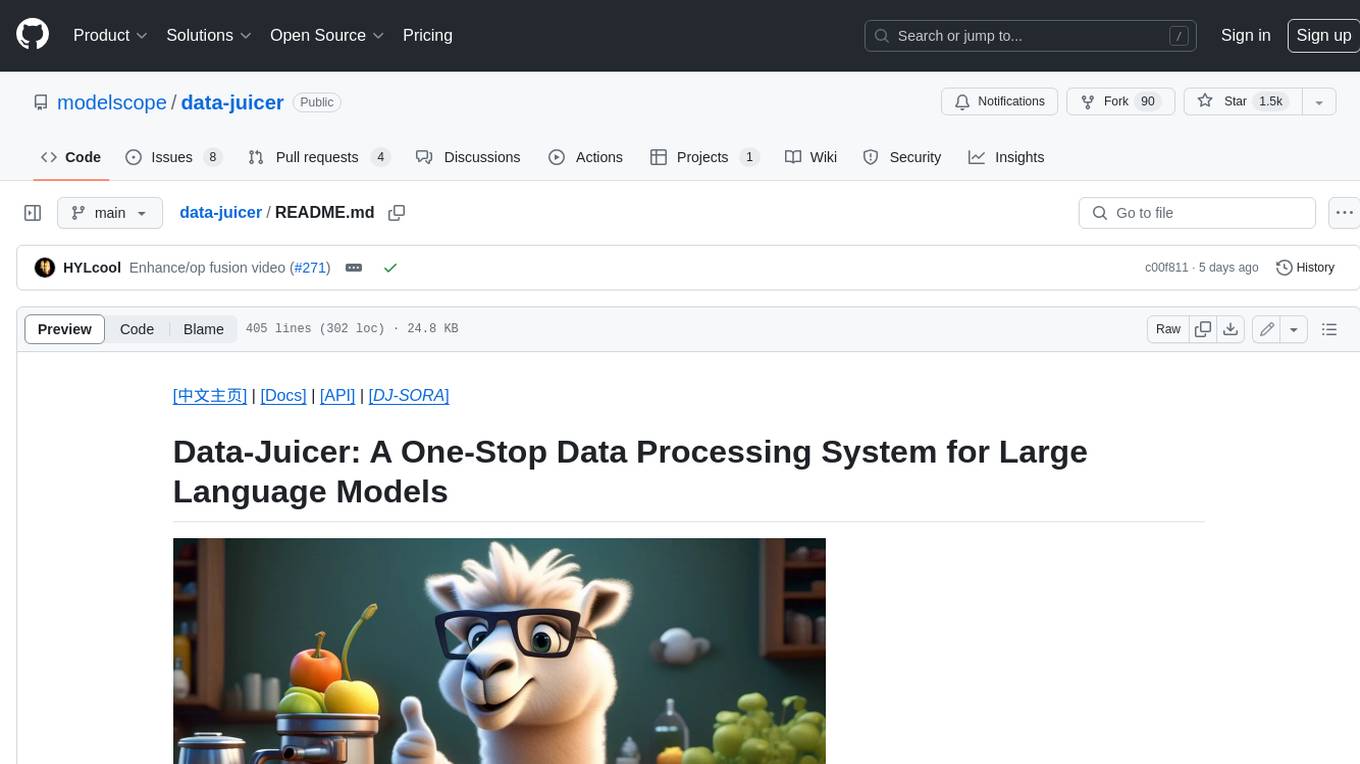
data-juicer
Data-Juicer is a one-stop data processing system to make data higher-quality, juicier, and more digestible for LLMs. It is a systematic & reusable library of 80+ core OPs, 20+ reusable config recipes, and 20+ feature-rich dedicated toolkits, designed to function independently of specific LLM datasets and processing pipelines. Data-Juicer allows detailed data analyses with an automated report generation feature for a deeper understanding of your dataset. Coupled with multi-dimension automatic evaluation capabilities, it supports a timely feedback loop at multiple stages in the LLM development process. Data-Juicer offers tens of pre-built data processing recipes for pre-training, fine-tuning, en, zh, and more scenarios. It provides a speedy data processing pipeline requiring less memory and CPU usage, optimized for maximum productivity. Data-Juicer is flexible & extensible, accommodating most types of data formats and allowing flexible combinations of OPs. It is designed for simplicity, with comprehensive documentation, easy start guides and demo configs, and intuitive configuration with simple adding/removing OPs from existing configs.
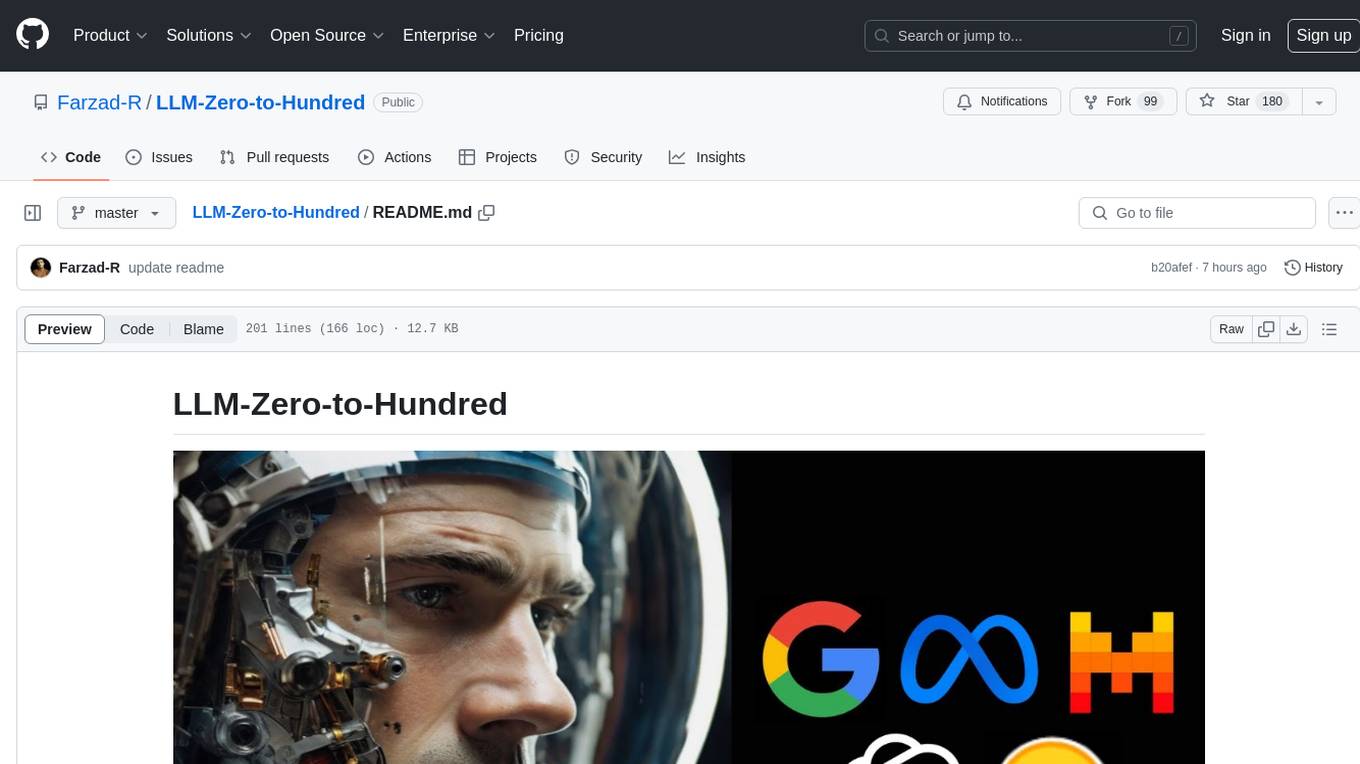
LLM-Zero-to-Hundred
LLM-Zero-to-Hundred is a repository showcasing various applications of LLM chatbots and providing insights into training and fine-tuning Language Models. It includes projects like WebGPT, RAG-GPT, WebRAGQuery, LLM Full Finetuning, RAG-Master LLamaindex vs Langchain, open-source-RAG-GEMMA, and HUMAIN: Advanced Multimodal, Multitask Chatbot. The projects cover features like ChatGPT-like interaction, RAG capabilities, image generation and understanding, DuckDuckGo integration, summarization, text and voice interaction, and memory access. Tutorials include LLM Function Calling and Visualizing Text Vectorization. The projects have a general structure with folders for README, HELPER, .env, configs, data, src, images, and utils.
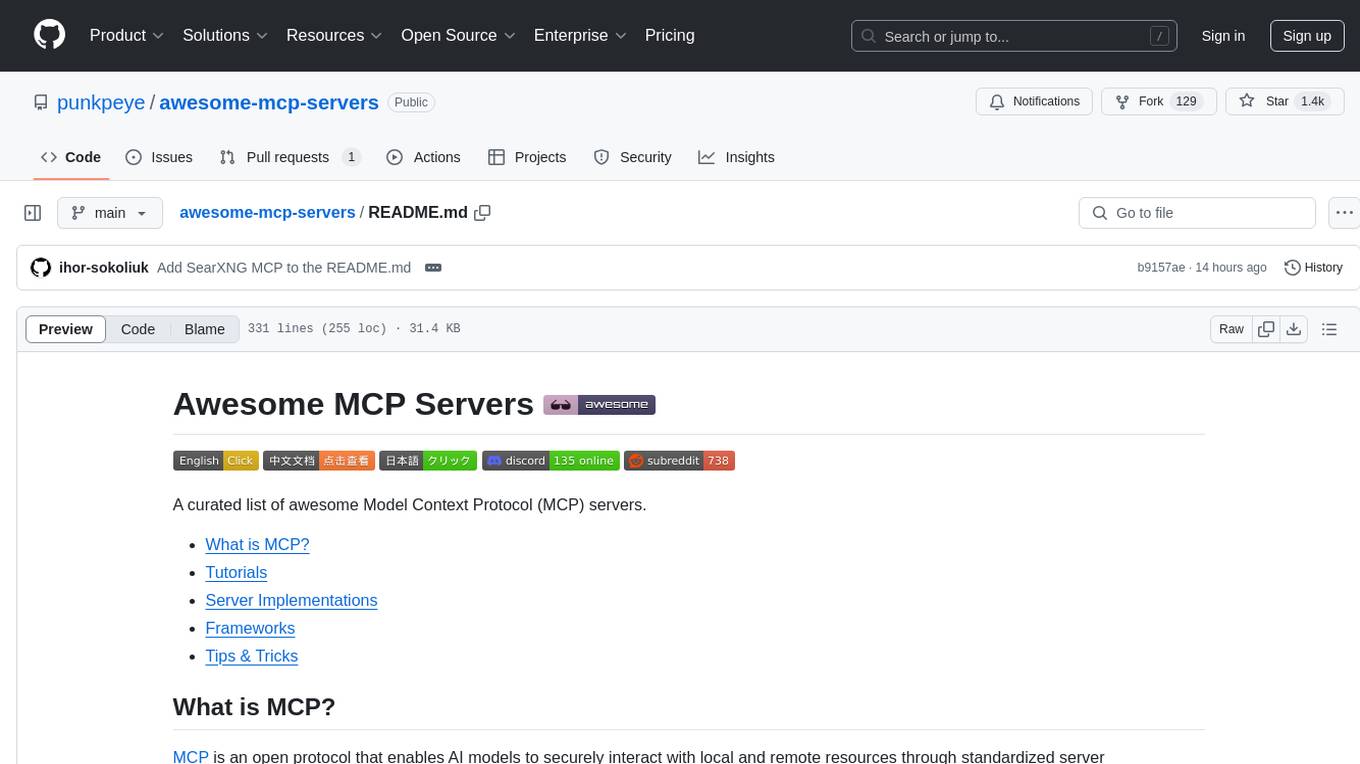
awesome-mcp-servers
Awesome MCP Servers is a curated list of Model Context Protocol (MCP) servers that enable AI models to securely interact with local and remote resources through standardized server implementations. The list includes production-ready and experimental servers that extend AI capabilities through file access, database connections, API integrations, and other contextual services.
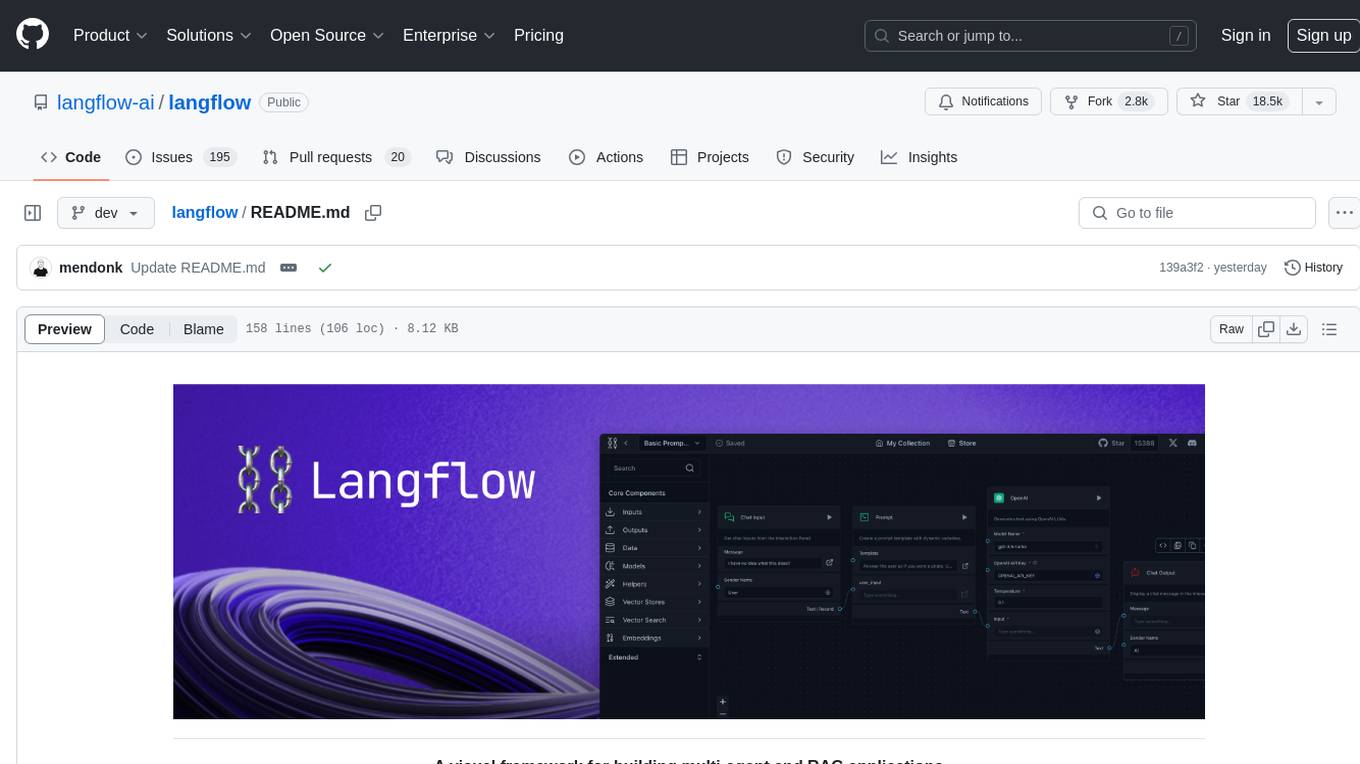
langflow
Langflow is an open-source Python-powered visual framework designed for building multi-agent and RAG applications. It is fully customizable, language model agnostic, and vector store agnostic. Users can easily create flows by dragging components onto the canvas, connect them, and export the flow as a JSON file. Langflow also provides a command-line interface (CLI) for easy management and configuration, allowing users to customize the behavior of Langflow for development or specialized deployment scenarios. The tool can be deployed on various platforms such as Google Cloud Platform, Railway, and Render. Contributors are welcome to enhance the project on GitHub by following the contributing guidelines.
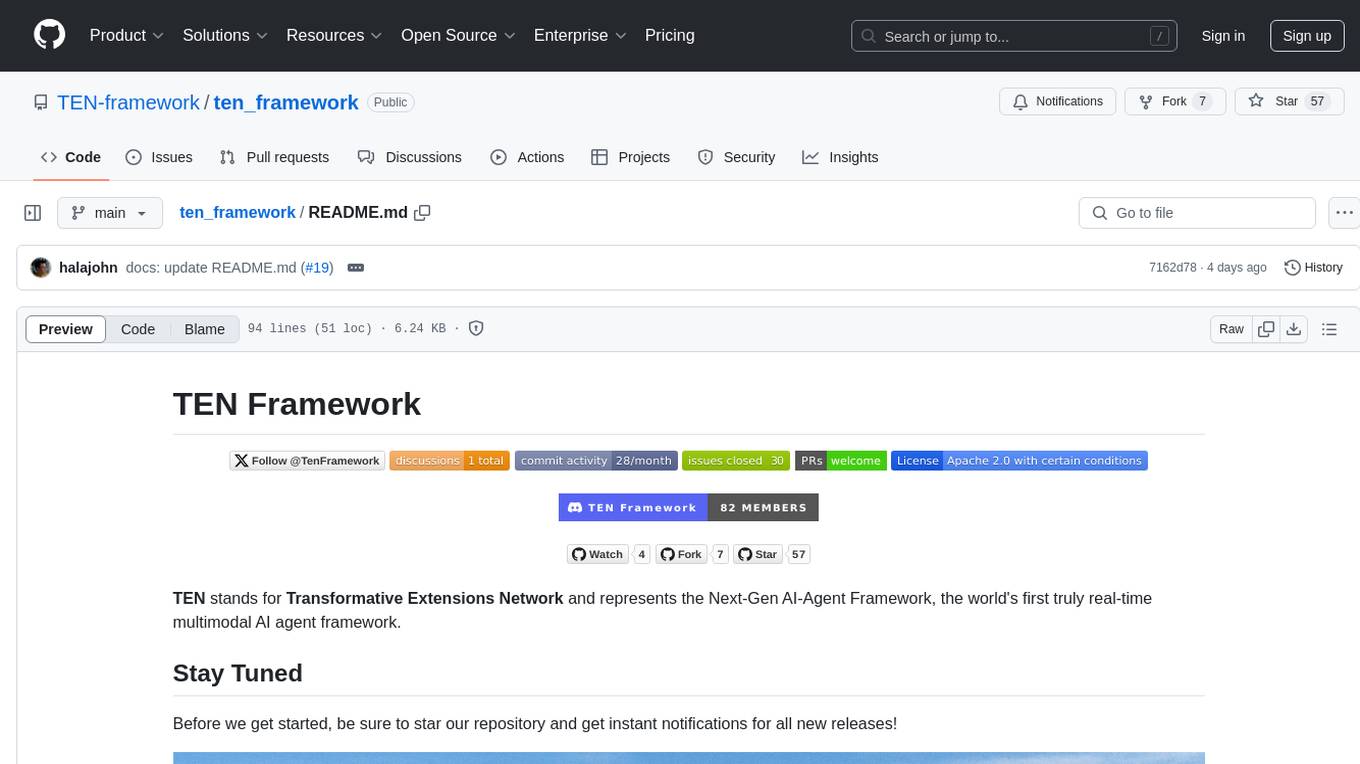
ten_framework
TEN Framework, short for Transformative Extensions Network, is the world's first real-time multimodal AI agent framework. It offers native support for high-performance, real-time multimodal interactions, supports multiple languages and platforms, enables edge-cloud integration, provides flexibility beyond model limitations, and allows for real-time agent state management. The framework facilitates the development of complex AI applications that transcend the limitations of large models by offering a drag-and-drop programming approach. It is suitable for scenarios like simultaneous interpretation, speech-to-text conversion, multilingual chat rooms, audio interaction, and audio-visual interaction.
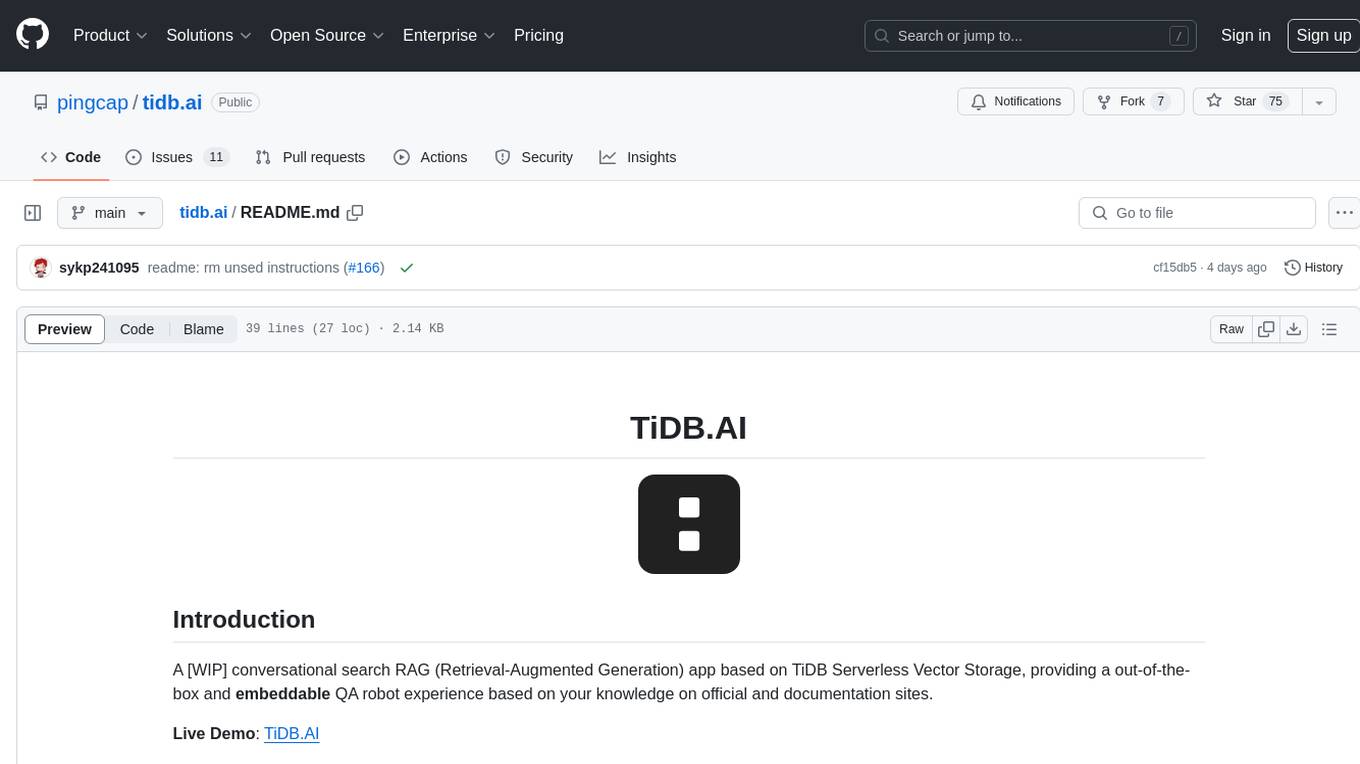
tidb.ai
TiDB.AI is a conversational search RAG (Retrieval-Augmented Generation) app based on TiDB Serverless Vector Storage. It provides an out-of-the-box and embeddable QA robot experience based on knowledge from official and documentation sites. The platform features a Perplexity-style Conversational Search page with an advanced built-in website crawler for comprehensive coverage. Users can integrate an embeddable JavaScript snippet into their website for instant responses to product-related queries. The tech stack includes Next.js, TypeScript, Tailwind CSS, shadcn/ui for design, TiDB for database storage, Kysely for SQL query building, NextAuth.js for authentication, Vercel for deployments, and LlamaIndex for the RAG framework. TiDB.AI is open-source under the Apache License, Version 2.0.
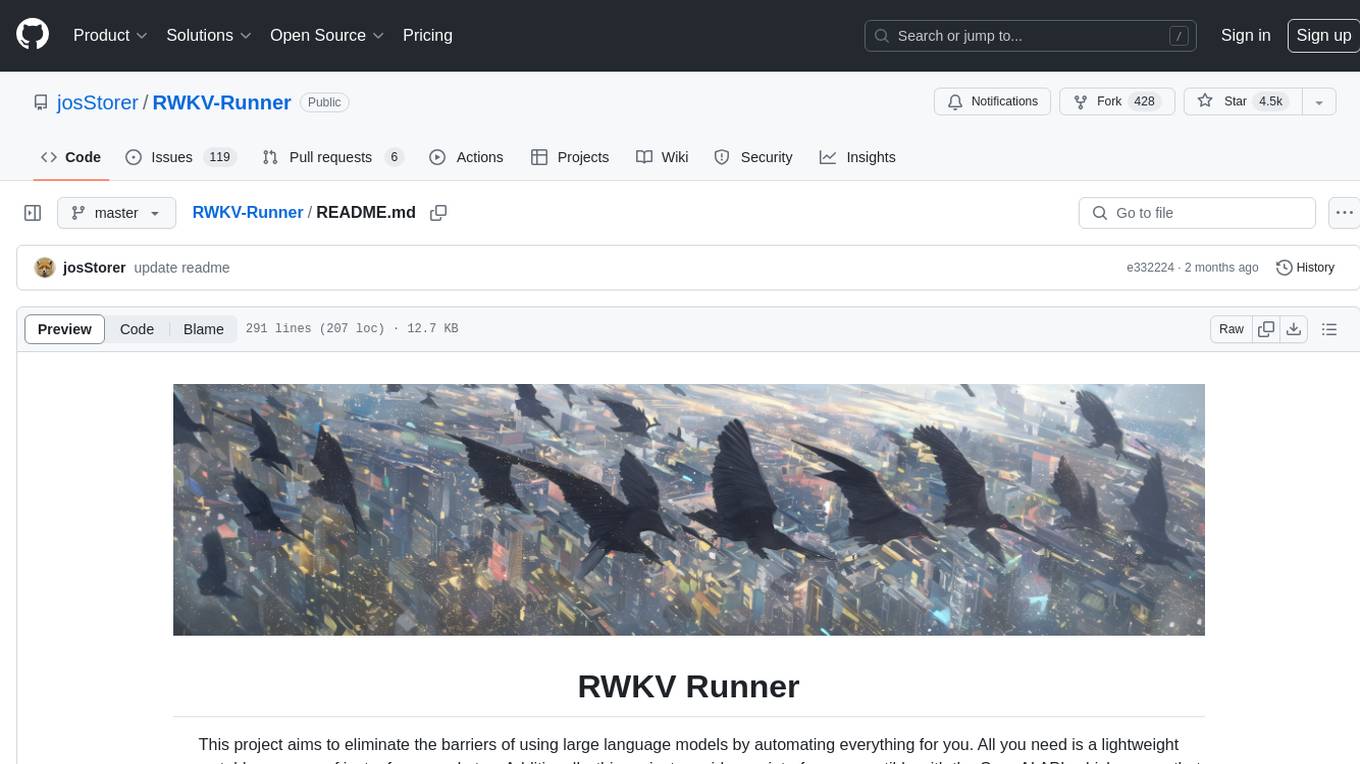
RWKV-Runner
RWKV Runner is a project designed to simplify the usage of large language models by automating various processes. It provides a lightweight executable program and is compatible with the OpenAI API. Users can deploy the backend on a server and use the program as a client. The project offers features like model management, VRAM configurations, user-friendly chat interface, WebUI option, parameter configuration, model conversion tool, download management, LoRA Finetune, and multilingual localization. It can be used for various tasks such as chat, completion, composition, and model inspection.
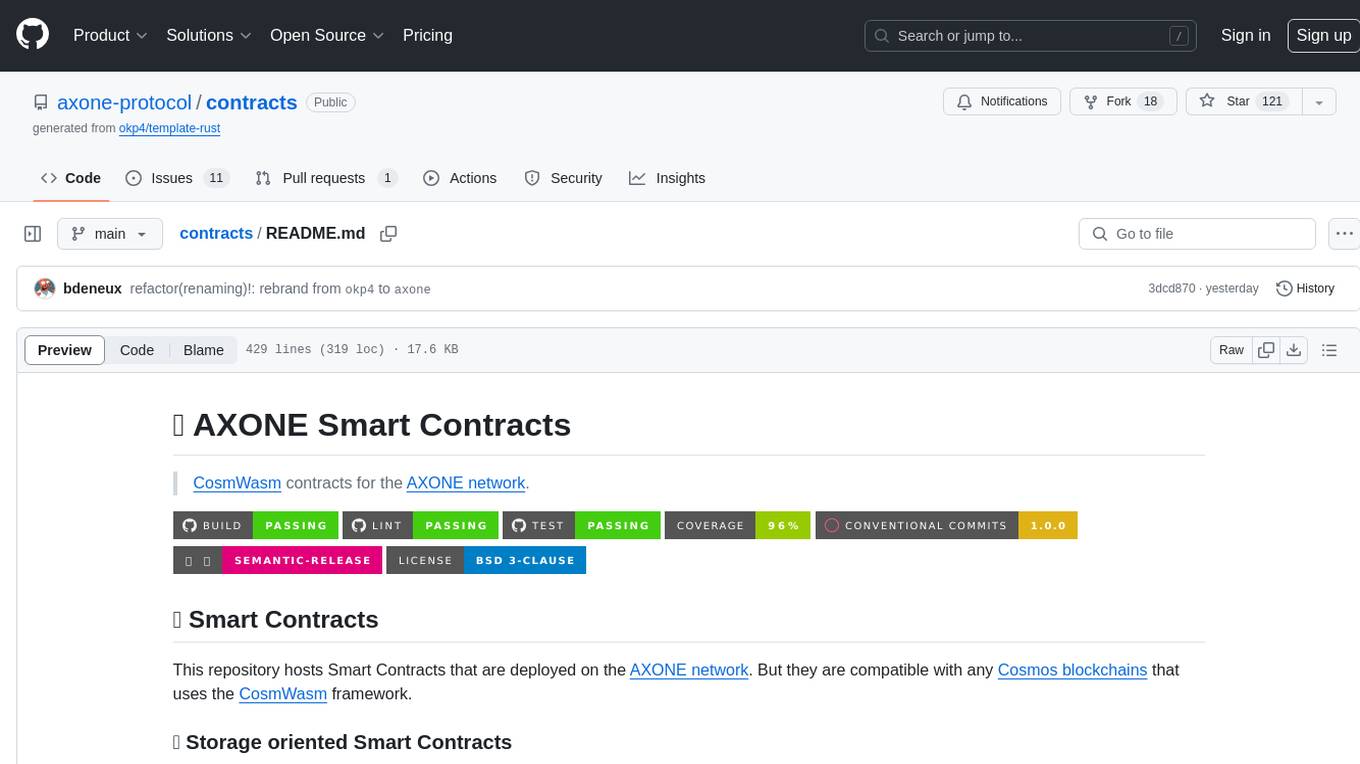
contracts
AXONE Smart Contracts repository hosts Smart Contracts for the AXONE network, compatible with any Cosmos blockchains using the CosmWasm framework. It includes storage, sovereignty, and resource management oriented Smart Contracts. Each contract has different functionalities and maturity stages, with detailed tech documentation and emojis indicating maturity levels. The repository provides tools for building, testing, deploying, and interacting with Smart Contracts, along with guidelines for contributing and community engagement.
For similar tasks
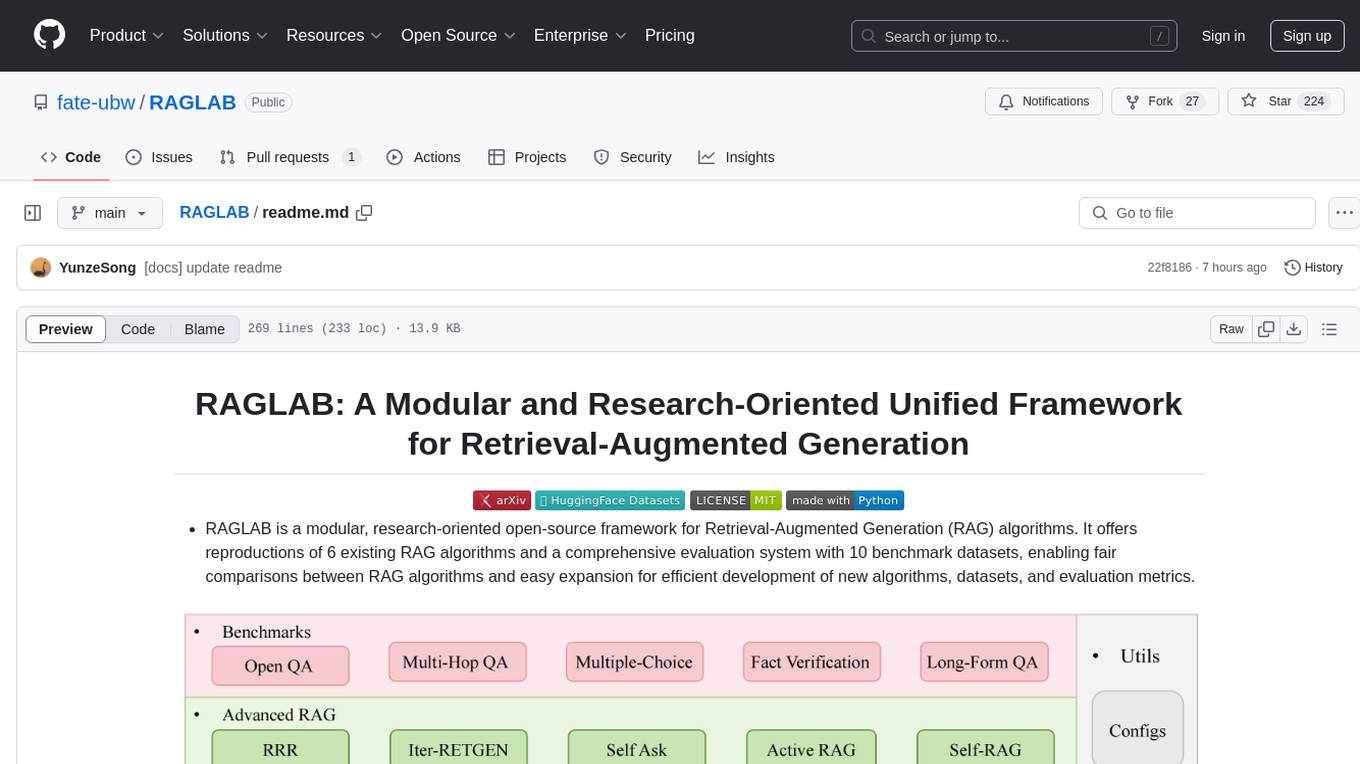
RAGLAB
RAGLAB is a modular, research-oriented open-source framework for Retrieval-Augmented Generation (RAG) algorithms. It offers reproductions of 6 existing RAG algorithms and a comprehensive evaluation system with 10 benchmark datasets, enabling fair comparisons between RAG algorithms and easy expansion for efficient development of new algorithms, datasets, and evaluation metrics. The framework supports the entire RAG pipeline, provides advanced algorithm implementations, fair comparison platform, efficient retriever client, versatile generator support, and flexible instruction lab. It also includes features like Interact Mode for quick understanding of algorithms and Evaluation Mode for reproducing paper results and scientific research.
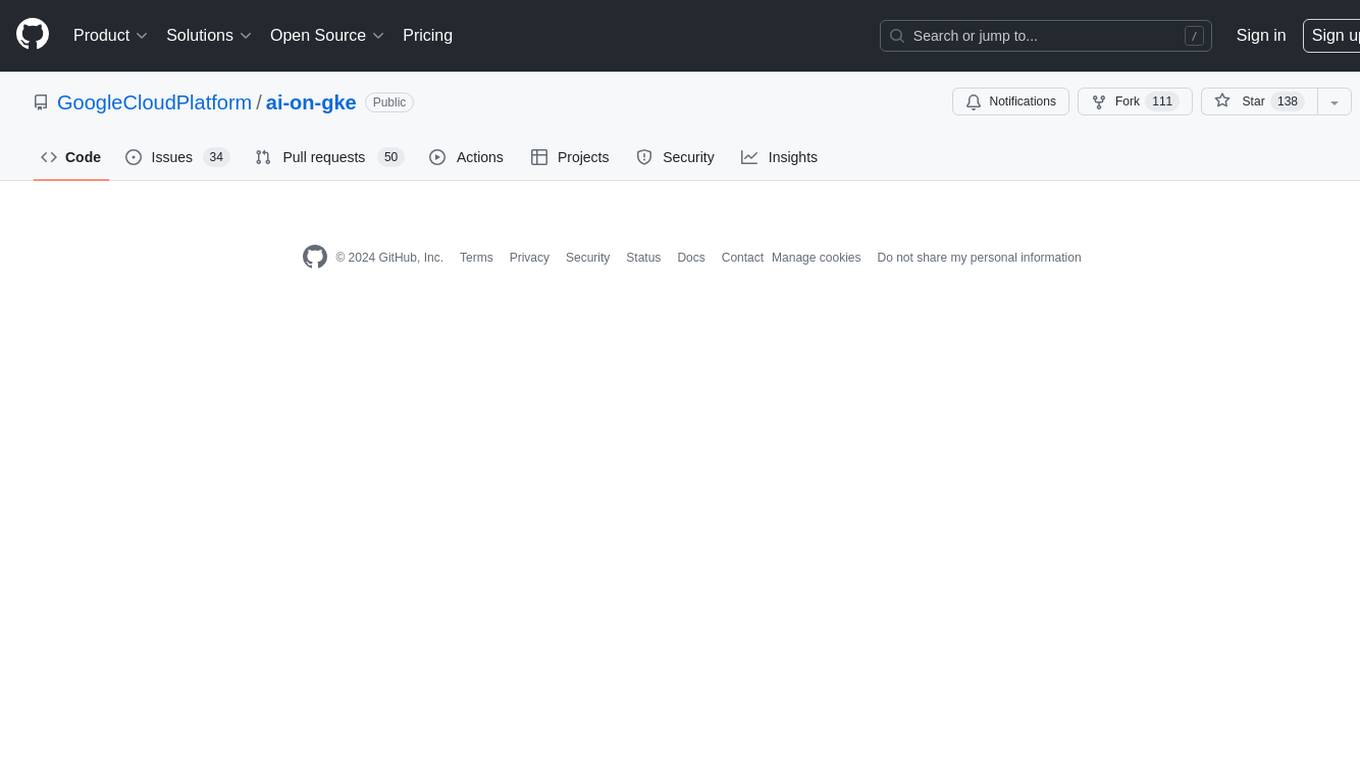
ai-on-gke
This repository contains assets related to AI/ML workloads on Google Kubernetes Engine (GKE). Run optimized AI/ML workloads with Google Kubernetes Engine (GKE) platform orchestration capabilities. A robust AI/ML platform considers the following layers: Infrastructure orchestration that support GPUs and TPUs for training and serving workloads at scale Flexible integration with distributed computing and data processing frameworks Support for multiple teams on the same infrastructure to maximize utilization of resources
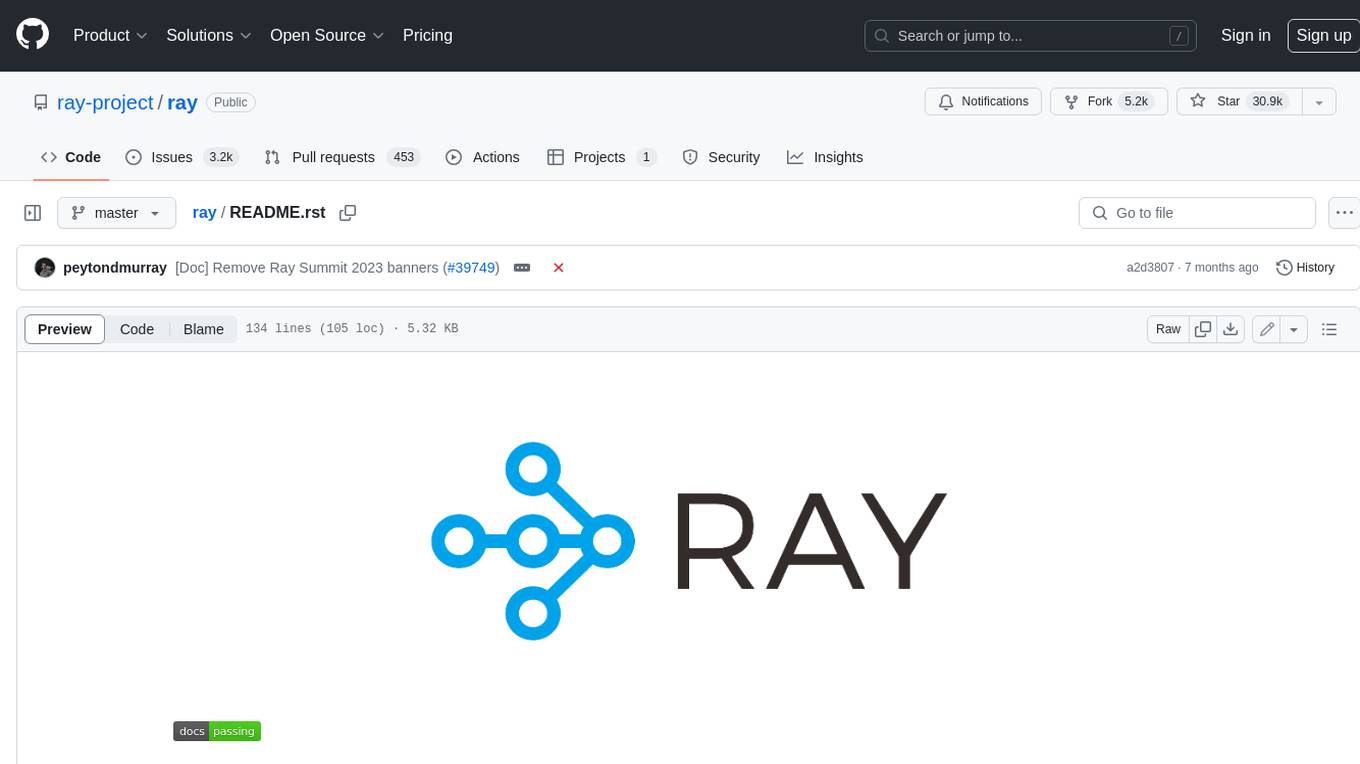
ray
Ray is a unified framework for scaling AI and Python applications. It consists of a core distributed runtime and a set of AI libraries for simplifying ML compute, including Data, Train, Tune, RLlib, and Serve. Ray runs on any machine, cluster, cloud provider, and Kubernetes, and features a growing ecosystem of community integrations. With Ray, you can seamlessly scale the same code from a laptop to a cluster, making it easy to meet the compute-intensive demands of modern ML workloads.
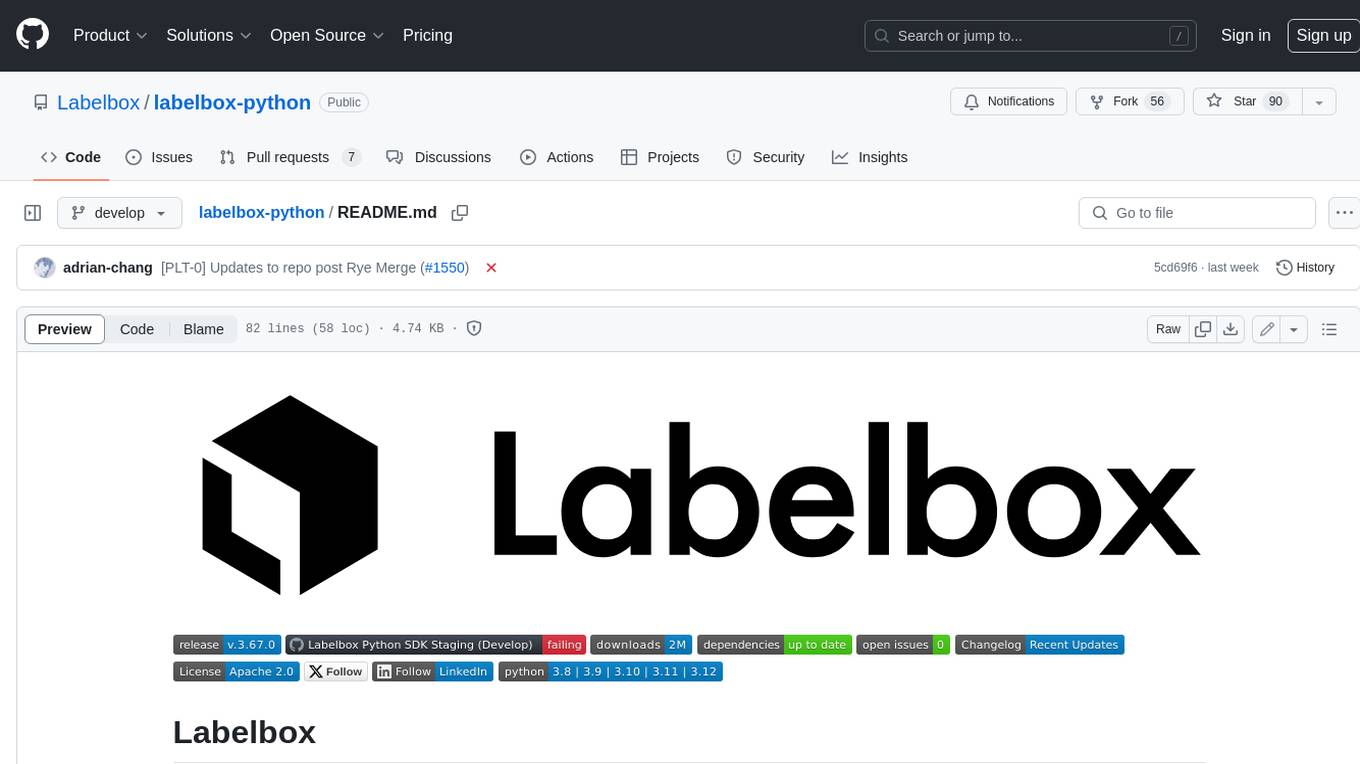
labelbox-python
Labelbox is a data-centric AI platform for enterprises to develop, optimize, and use AI to solve problems and power new products and services. Enterprises use Labelbox to curate data, generate high-quality human feedback data for computer vision and LLMs, evaluate model performance, and automate tasks by combining AI and human-centric workflows. The academic & research community uses Labelbox for cutting-edge AI research.
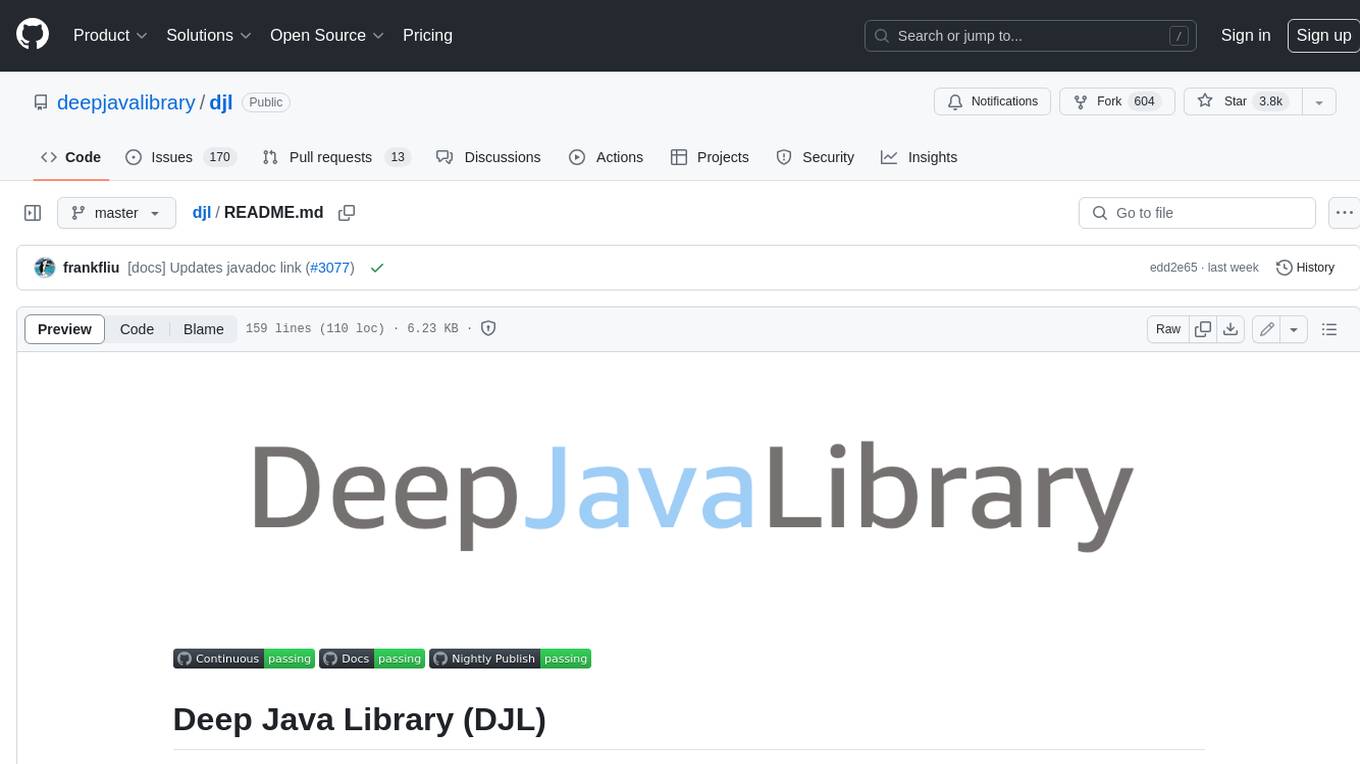
djl
Deep Java Library (DJL) is an open-source, high-level, engine-agnostic Java framework for deep learning. It is designed to be easy to get started with and simple to use for Java developers. DJL provides a native Java development experience and allows users to integrate machine learning and deep learning models with their Java applications. The framework is deep learning engine agnostic, enabling users to switch engines at any point for optimal performance. DJL's ergonomic API interface guides users with best practices to accomplish deep learning tasks, such as running inference and training neural networks.
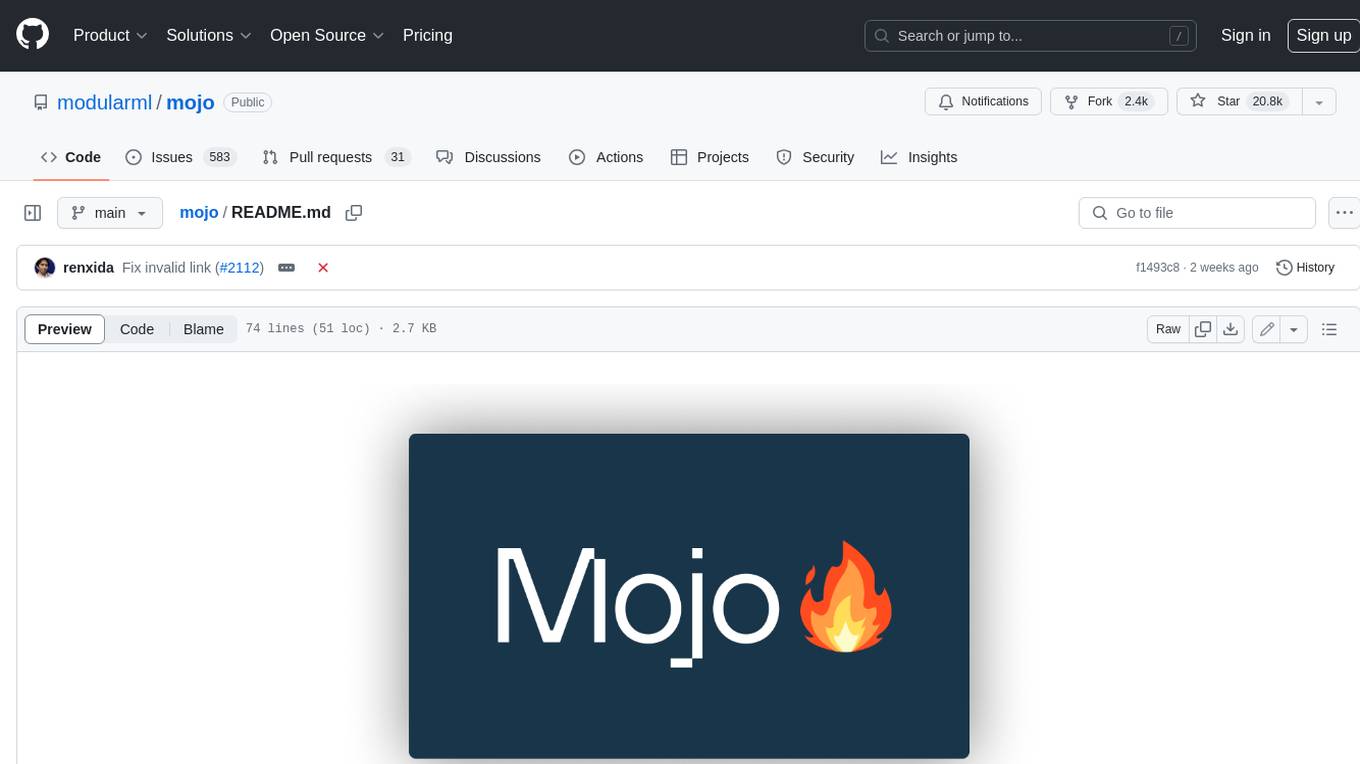
mojo
Mojo is a new programming language that bridges the gap between research and production by combining Python syntax and ecosystem with systems programming and metaprogramming features. Mojo is still young, but it is designed to become a superset of Python over time.
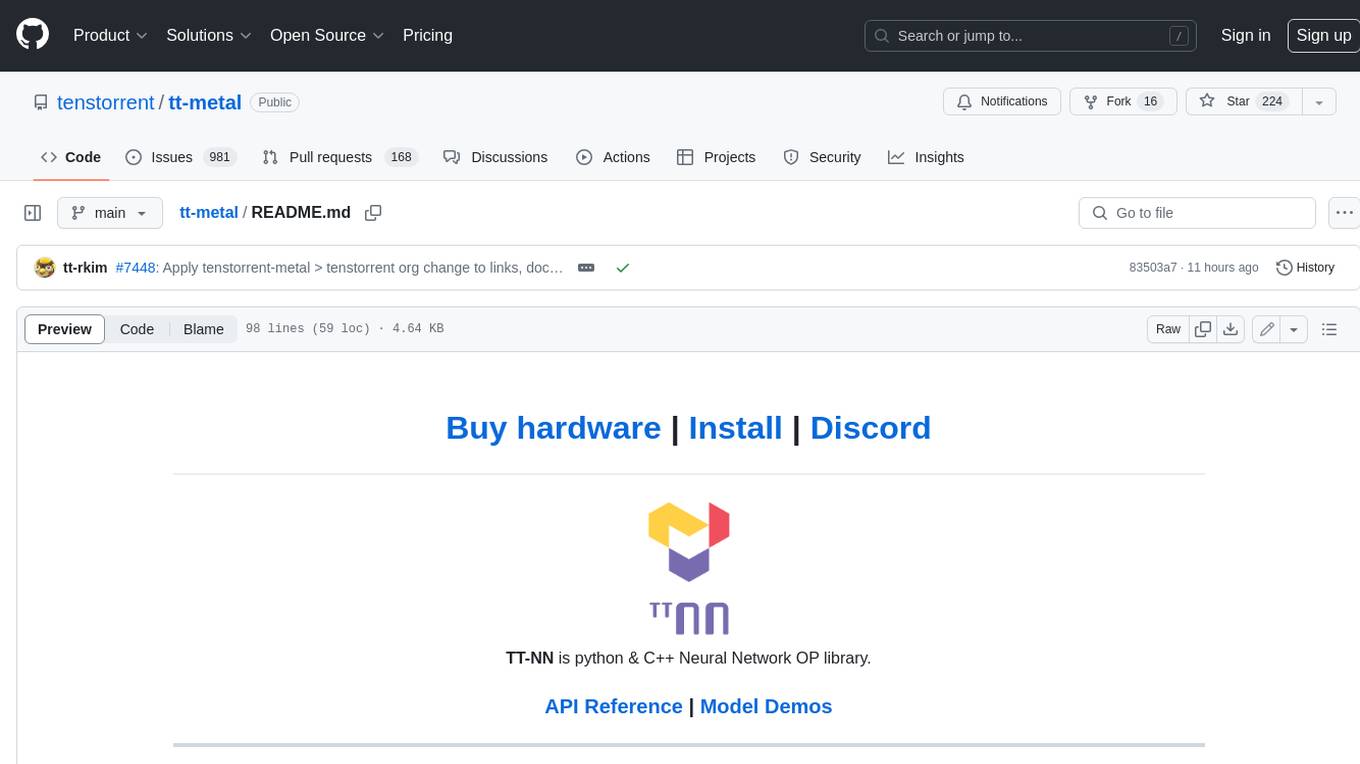
tt-metal
TT-NN is a python & C++ Neural Network OP library. It provides a low-level programming model, TT-Metalium, enabling kernel development for Tenstorrent hardware.
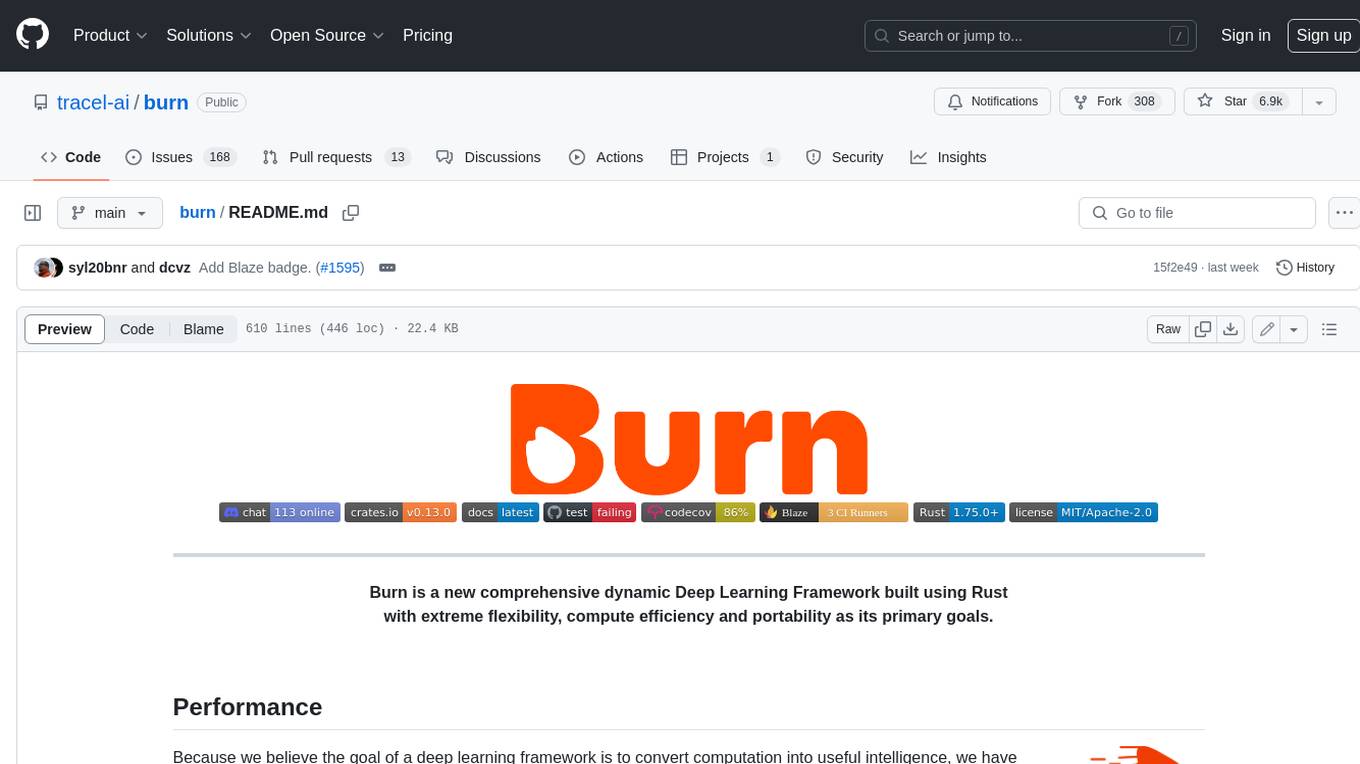
burn
Burn is a new comprehensive dynamic Deep Learning Framework built using Rust with extreme flexibility, compute efficiency and portability as its primary goals.
For similar jobs
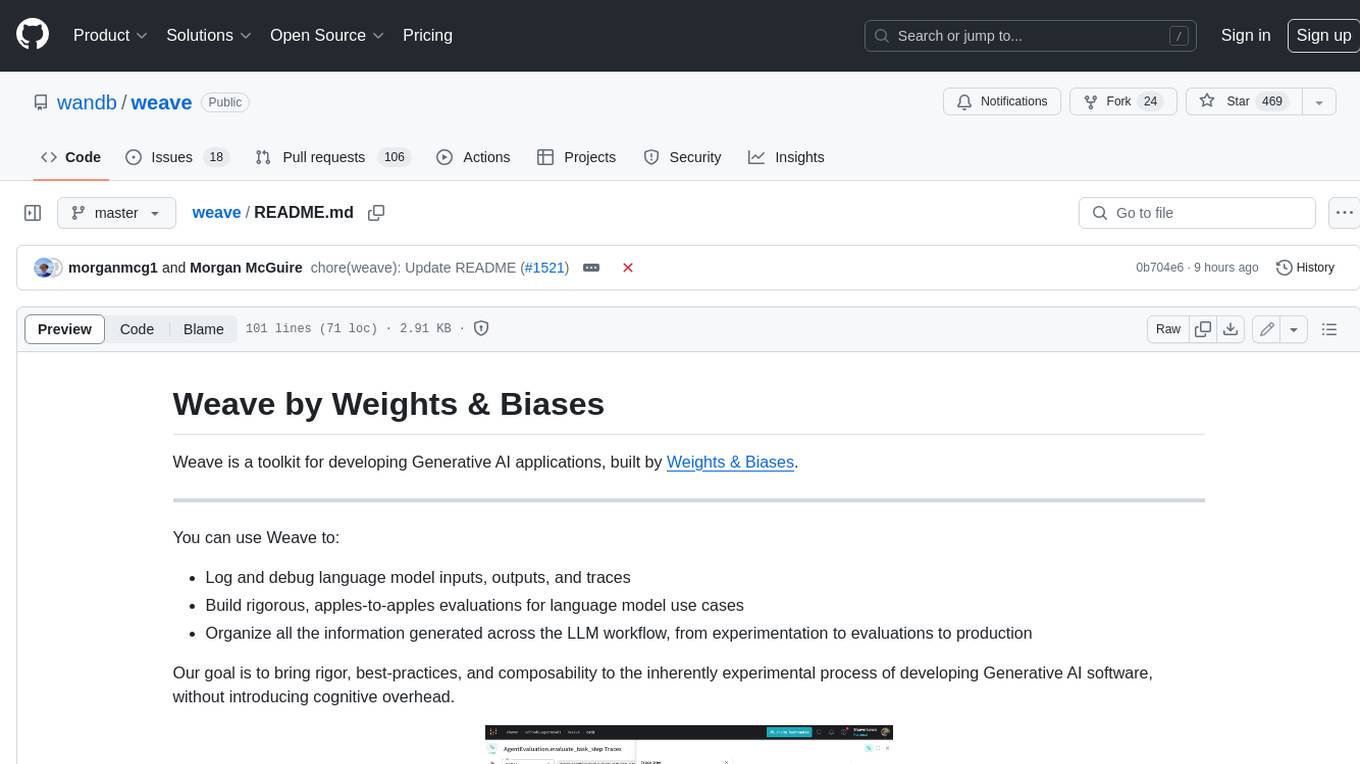
weave
Weave is a toolkit for developing Generative AI applications, built by Weights & Biases. With Weave, you can log and debug language model inputs, outputs, and traces; build rigorous, apples-to-apples evaluations for language model use cases; and organize all the information generated across the LLM workflow, from experimentation to evaluations to production. Weave aims to bring rigor, best-practices, and composability to the inherently experimental process of developing Generative AI software, without introducing cognitive overhead.
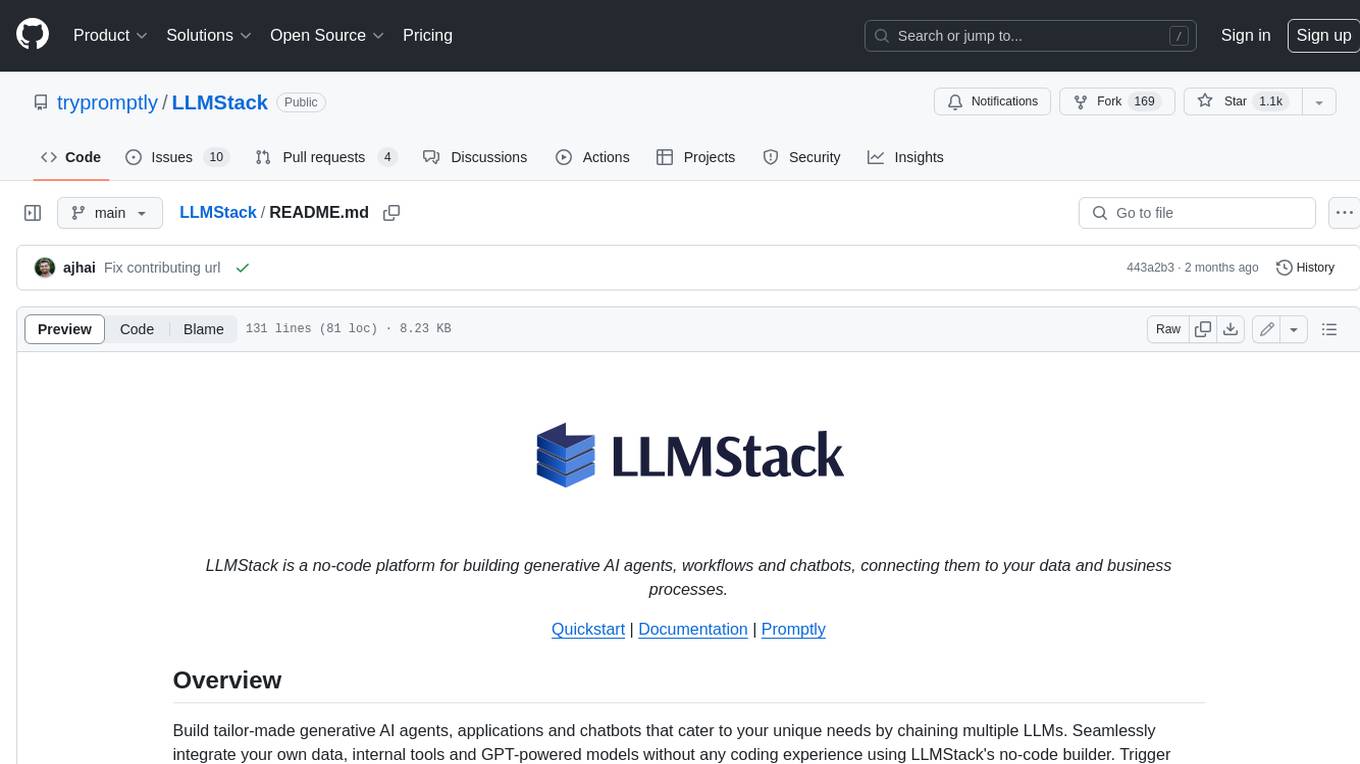
LLMStack
LLMStack is a no-code platform for building generative AI agents, workflows, and chatbots. It allows users to connect their own data, internal tools, and GPT-powered models without any coding experience. LLMStack can be deployed to the cloud or on-premise and can be accessed via HTTP API or triggered from Slack or Discord.
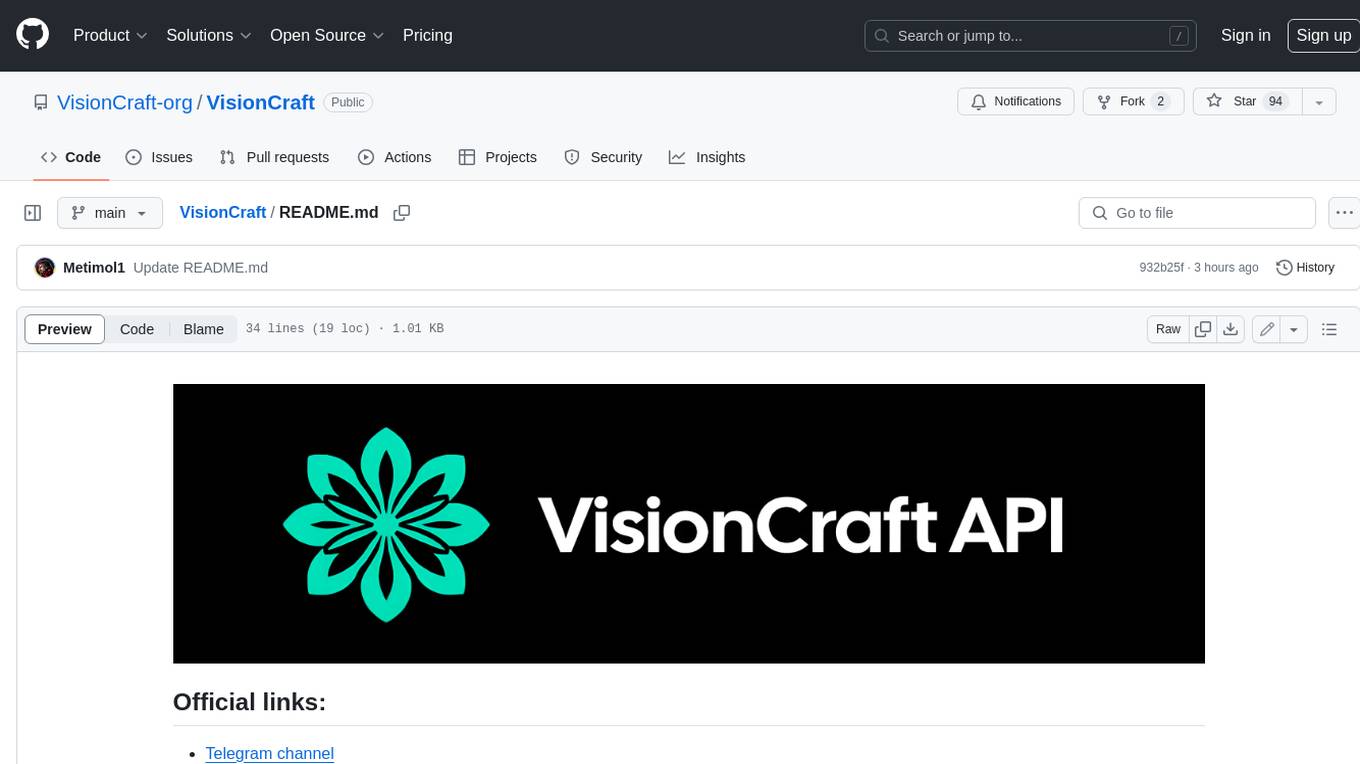
VisionCraft
The VisionCraft API is a free API for using over 100 different AI models. From images to sound.
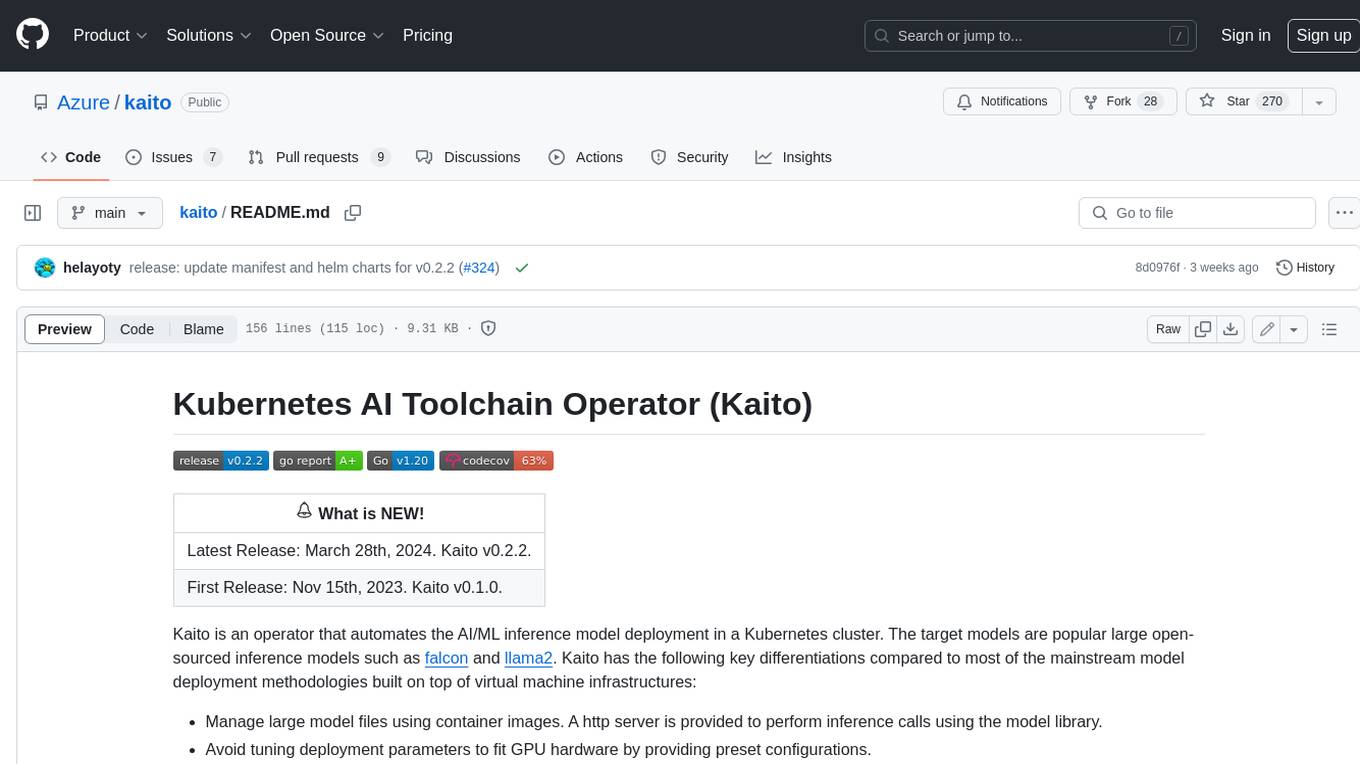
kaito
Kaito is an operator that automates the AI/ML inference model deployment in a Kubernetes cluster. It manages large model files using container images, avoids tuning deployment parameters to fit GPU hardware by providing preset configurations, auto-provisions GPU nodes based on model requirements, and hosts large model images in the public Microsoft Container Registry (MCR) if the license allows. Using Kaito, the workflow of onboarding large AI inference models in Kubernetes is largely simplified.
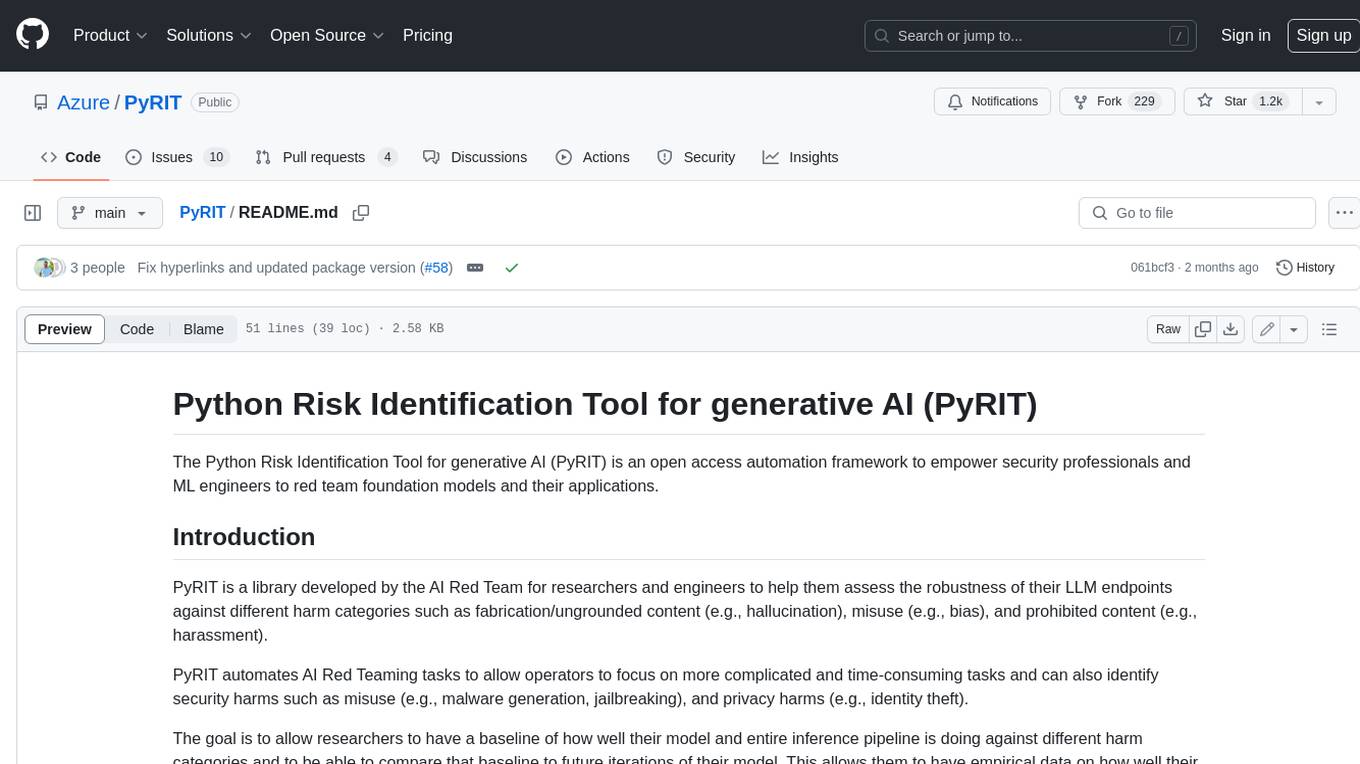
PyRIT
PyRIT is an open access automation framework designed to empower security professionals and ML engineers to red team foundation models and their applications. It automates AI Red Teaming tasks to allow operators to focus on more complicated and time-consuming tasks and can also identify security harms such as misuse (e.g., malware generation, jailbreaking), and privacy harms (e.g., identity theft). The goal is to allow researchers to have a baseline of how well their model and entire inference pipeline is doing against different harm categories and to be able to compare that baseline to future iterations of their model. This allows them to have empirical data on how well their model is doing today, and detect any degradation of performance based on future improvements.
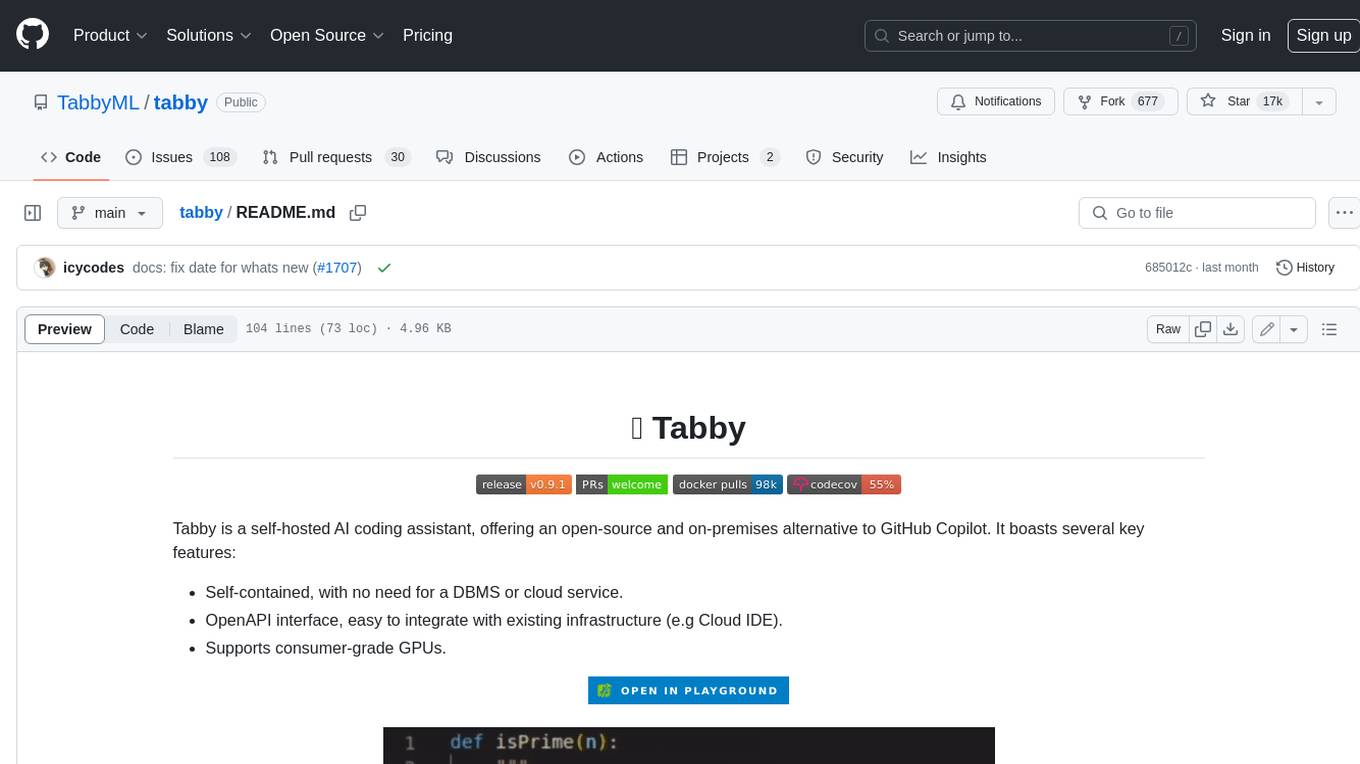
tabby
Tabby is a self-hosted AI coding assistant, offering an open-source and on-premises alternative to GitHub Copilot. It boasts several key features: * Self-contained, with no need for a DBMS or cloud service. * OpenAPI interface, easy to integrate with existing infrastructure (e.g Cloud IDE). * Supports consumer-grade GPUs.
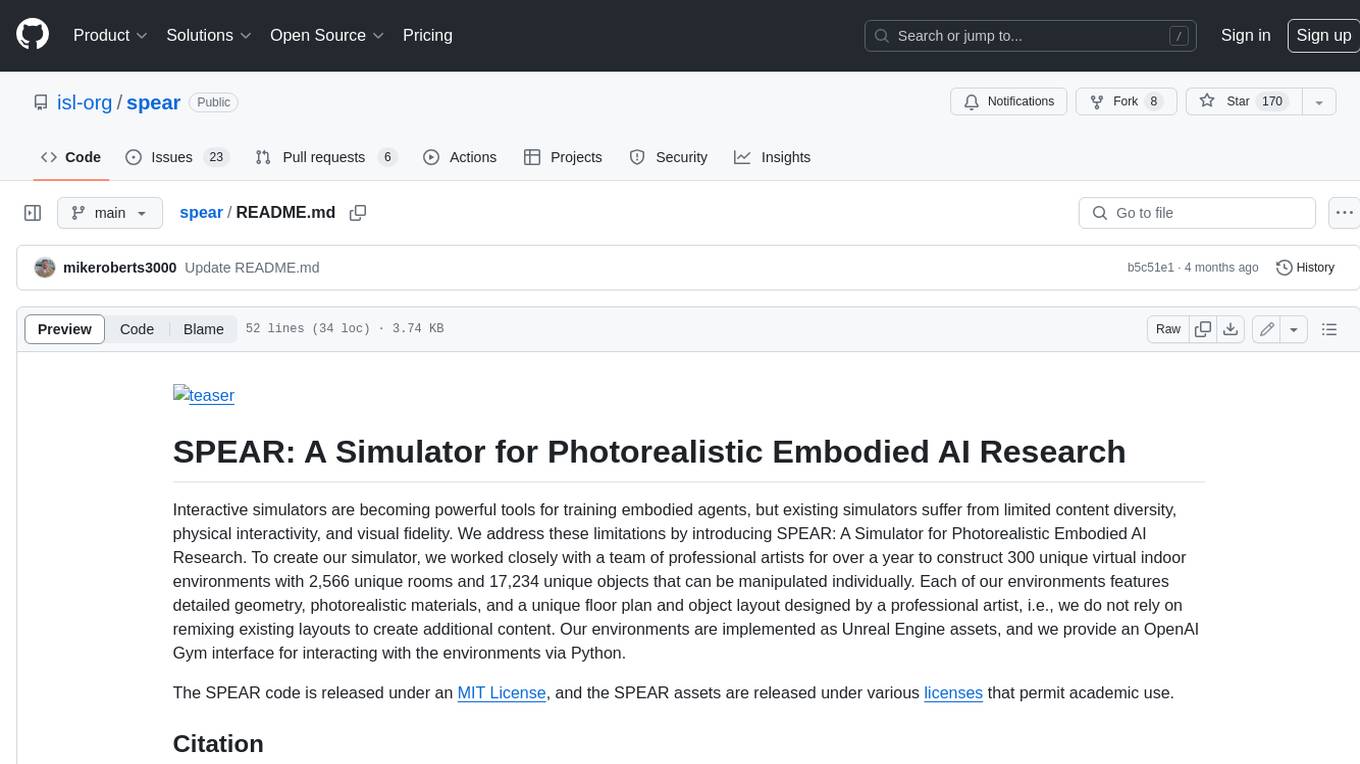
spear
SPEAR (Simulator for Photorealistic Embodied AI Research) is a powerful tool for training embodied agents. It features 300 unique virtual indoor environments with 2,566 unique rooms and 17,234 unique objects that can be manipulated individually. Each environment is designed by a professional artist and features detailed geometry, photorealistic materials, and a unique floor plan and object layout. SPEAR is implemented as Unreal Engine assets and provides an OpenAI Gym interface for interacting with the environments via Python.
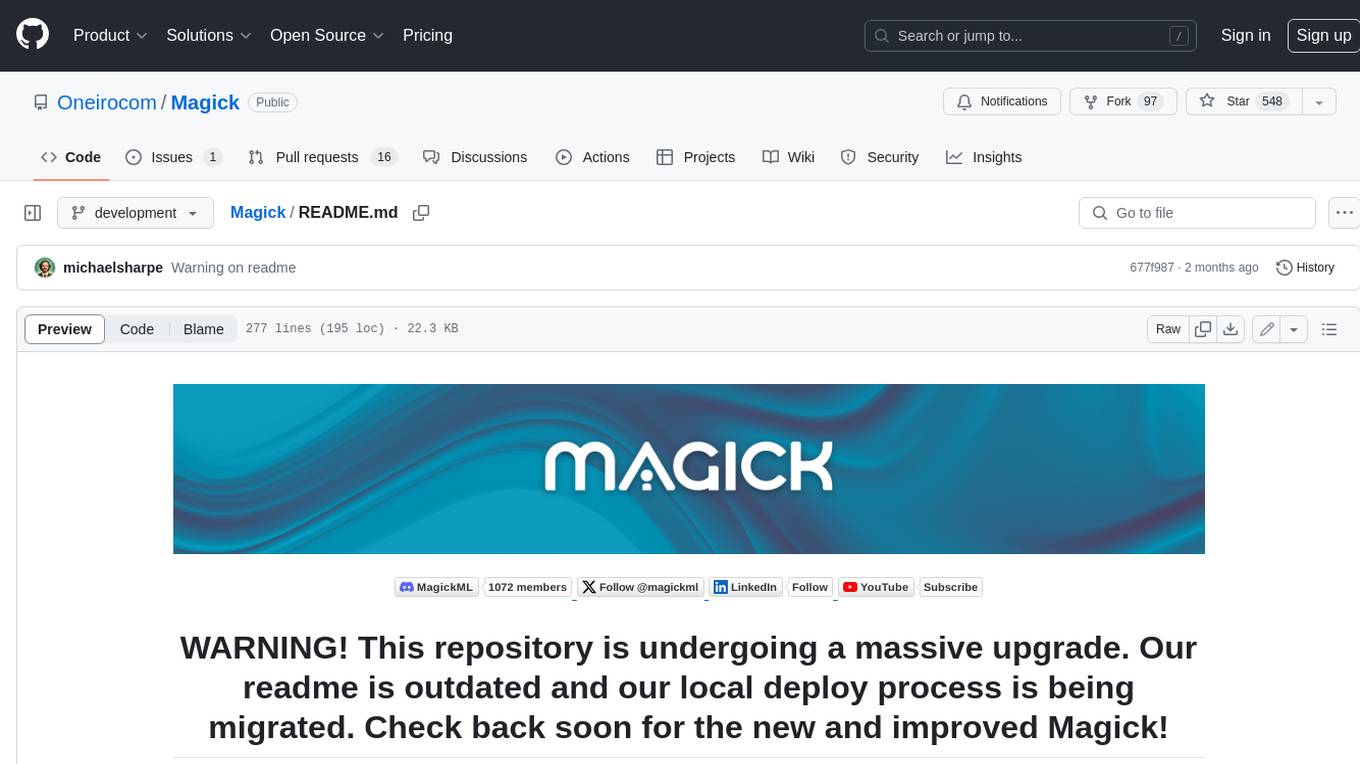
Magick
Magick is a groundbreaking visual AIDE (Artificial Intelligence Development Environment) for no-code data pipelines and multimodal agents. Magick can connect to other services and comes with nodes and templates well-suited for intelligent agents, chatbots, complex reasoning systems and realistic characters.