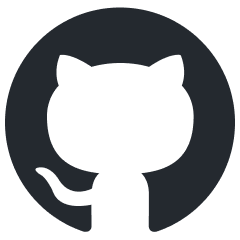
marqo
Unified embedding generation and search engine. Also available on cloud - cloud.marqo.ai
Stars: 4785
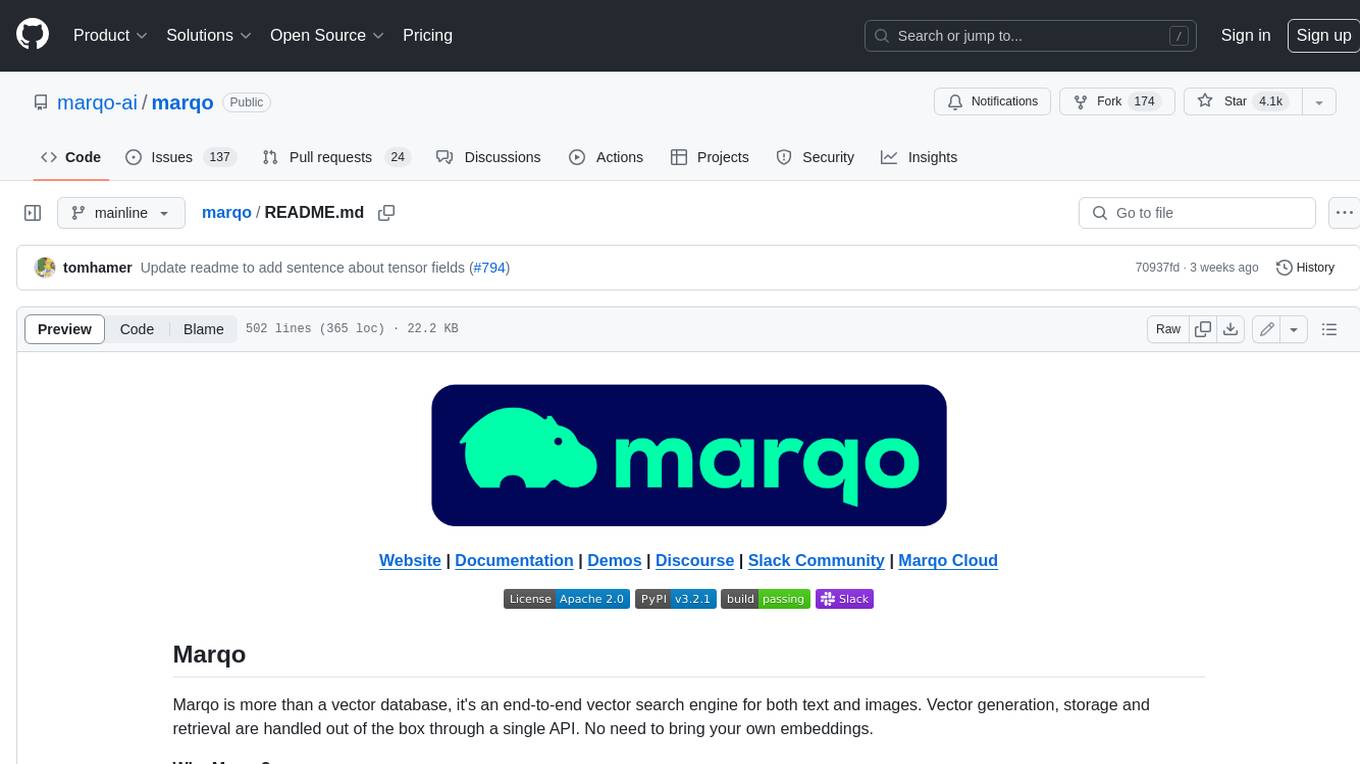
Marqo is more than a vector database, it's an end-to-end vector search engine for both text and images. Vector generation, storage and retrieval are handled out of the box through a single API. No need to bring your own embeddings.
README:
Website | Documentation | Demos | Discourse | Slack Community | Marqo Cloud
Marqo is more than a vector database, it's an end-to-end vector search engine for both text and images. Vector generation, storage and retrieval are handled out of the box through a single API. No need to bring your own embeddings.
Why Marqo?
Vector similarity alone is not enough for vector search. Vector search requires more than a vector database - it also requires machine learning (ML) deployment and management, preprocessing and transformations of inputs as well as the ability to modify search behavior without retraining a model. Marqo contains all these pieces, enabling developers to build vector search into their application with minimal effort. A full list of features can be found below.
Why bundle embedding generation with vector search?
Vector databases are specialized components for vector similarity and only service one component of a vector search system. They are “vectors in - vectors out”. They still require the production of vectors, management of the ML models, associated orchestration and processing of the inputs. Marqo makes this easy by being “documents in, documents out”. Preprocessing of text and images, embedding the content, storing meta-data and deployment of inference and storage is all taken care of by Marqo.
Quick start
Here is a code snippet for a minimal example of vector search with Marqo (see Getting Started):
-
Marqo requires Docker. To install Docker go to the Docker Official website. Ensure that docker has at least 8GB memory and 50GB storage. In Docker desktop, you can do this by clicking the settings icon, then resources, and selecting 8GB memory.
-
Use docker to run Marqo:
docker rm -f marqo
docker pull marqoai/marqo:latest
docker run --name marqo -it -p 8882:8882 marqoai/marqo:latest
- Install the Marqo client:
pip install marqo
- Start indexing and searching! Let's look at a simple example below:
import marqo
mq = marqo.Client(url='http://localhost:8882')
mq.create_index("my-first-index", model="hf/e5-base-v2")
mq.index("my-first-index").add_documents([
{
"Title": "The Travels of Marco Polo",
"Description": "A 13th-century travelogue describing Polo's travels"
},
{
"Title": "Extravehicular Mobility Unit (EMU)",
"Description": "The EMU is a spacesuit that provides environmental protection, "
"mobility, life support, and communications for astronauts",
"_id": "article_591"
}],
tensor_fields=["Description"]
)
results = mq.index("my-first-index").search(
q="What is the best outfit to wear on the moon?"
)
🤖 State of the art embeddings
- Use the latest machine learning models from PyTorch, Huggingface, OpenAI and more.
- Start with a pre-configured model or bring your own.
- CPU and GPU support.
⚡ Performance
- Embeddings stored in in-memory HNSW indexes, achieving cutting edge search speeds.
- Scale to hundred-million document indexes with horizontal index sharding.
- Async and non-blocking data upload and search.
🌌 Documents-in-documents-out
- Vector generation, storage, and retrieval are provided out of the box.
- Build search, entity resolution, and data exploration application with using your text and images.
- Build complex semantic queries by combining weighted search terms.
- Filter search results using Marqo’s query DSL.
- Store unstructured data and semi-structured metadata together in documents, using a range of supported datatypes like bools, ints and keywords.
🍱 Managed cloud
- Low latency optimised deployment of Marqo.
- Scale inference at the click of a button.
- High availability.
- 24/7 support.
- Access control.
- Learn more here.
Marqo is integrated into popular AI and data processing frameworks, with more on the way.
💙 Haystack
Haystack is an open-source framework for building applications that make use of NLP technology such as LLMs, embedding models and more. This integration allows you to use Marqo as your Document Store for Haystack pipelines such as retrieval-augmentation, question answering, document search and more.
🛹 Griptape
Griptape enables safe and reliable deployment of LLM-based agents for enterprise applications, the MarqoVectorStoreDriver gives these agents access to scalable search with your own data. This integration lets you leverage open source or custom fine-tuned models through Marqo to deliver relevant results to your LLMs.
🦜🔗 Langchain
This integration lets you leverage open source or custom fine tuned models through Marqo for LangChain applications with a vector search component. The Marqo vector store implementation can plug into existing chains such as the Retrieval QA and Conversational Retrieval QA.
⋙ Hamilton
This integration lets you leverage open source or custom fine tuned models through Marqo for Hamilton LLM applications.
📗 Quick start | Build your first application with Marqo in under 5 minutes. |
🖼 Marqo for image data | Building advanced image search with Marqo. |
📚 Marqo for text | Building a multilingual database in Marqo. |
🔮 Integrating Marqo with GPT | Making GPT a subject matter expert by using Marqo as a knowledge base. |
🎨 Marqo for Creative AI | Combining stable diffusion with semantic search to generate and categorise 100k images of hotdogs. |
🔊 Marqo and Speech Data | Add diarisation and transcription to preprocess audio for Q&A with Marqo and ChatGPT. |
🚫 Marqo for content moderation | Building advanced image search with Marqo to find and remove content. |
☁️ Getting started with Marqo Cloud | Go through how to get set up and running with Marqo Cloud starting from your first time login through to building your first application with Marqo |
👗 Marqo for e-commerce | This project is a web application with frontend and backend using Python, Flask, ReactJS, and Typescript. The frontend is a ReactJS application that makes requests to the backend which is a Flask application. The backend makes requests to your Marqo cloud API. |
🤖 Marqo chatbot | In this guide we will build a chat bot application using Marqo and OpenAI's ChatGPT API. We will start with an existing code base and then walk through how to customise the behaviour. |
🦾 Features | Marqo's core features. |
-
Marqo requires Docker. To install Docker go to the Docker Official website. Ensure that docker has at least 8GB memory and 50GB storage.
-
Use docker to run Marqo:
docker rm -f marqo
docker pull marqoai/marqo:latest
docker run --name marqo -p 8882:8882 marqoai/marqo:latest
Note: If your marqo
container keeps getting killed, this is most likely due to a lack of memory being allocated to Docker. Increasing the memory limit for Docker to at least 6GB (8GB recommended) in your Docker settings may fix the problem.
- Install the Marqo client:
pip install marqo
- Start indexing and searching! Let's look at a simple example below:
import marqo
mq = marqo.Client(url='http://localhost:8882')
mq.create_index("my-first-index")
mq.index("my-first-index").add_documents([
{
"Title": "The Travels of Marco Polo",
"Description": "A 13th-century travelogue describing Polo's travels"
},
{
"Title": "Extravehicular Mobility Unit (EMU)",
"Description": "The EMU is a spacesuit that provides environmental protection, "
"mobility, life support, and communications for astronauts",
"_id": "article_591"
}],
tensor_fields=["Description"]
)
results = mq.index("my-first-index").search(
q="What is the best outfit to wear on the moon?"
)
-
mq
is the client that wraps themarqo
API. -
create_index()
creates a new index with default settings. You have the option to specify what model to use. For example,mq.create_index("my-first-index", model="hf/all_datasets_v4_MiniLM-L6")
will create an index with the default text modelhf/all_datasets_v4_MiniLM-L6
. Experimentation with different models is often required to achieve the best retrieval for your specific use case. Different models also offer a tradeoff between inference speed and relevancy. See here for the full list of models. -
add_documents()
takes a list of documents, represented as python dicts for indexing.tensor_fields
refers to the fields that will be indexed as vector collections and made searchable. - You can optionally set a document's ID with the special
_id
field. Otherwise, Marqo will generate one.
Let's have a look at the results:
# let's print out the results:
import pprint
pprint.pprint(results)
{
'hits': [
{
'Title': 'Extravehicular Mobility Unit (EMU)',
'Description': 'The EMU is a spacesuit that provides environmental protection, mobility, life support, and'
'communications for astronauts',
'_highlights': [{
'Description': 'The EMU is a spacesuit that provides environmental protection, '
'mobility, life support, and communications for astronauts'
}],
'_id': 'article_591',
'_score': 0.61938936
},
{
'Title': 'The Travels of Marco Polo',
'Description': "A 13th-century travelogue describing Polo's travels",
'_highlights': [{'Title': 'The Travels of Marco Polo'}],
'_id': 'e00d1a8d-894c-41a1-8e3b-d8b2a8fce12a',
'_score': 0.60237324
}
],
'limit': 10,
'processingTimeMs': 49,
'query': 'What is the best outfit to wear on the moon?'
}
- Each hit corresponds to a document that matched the search query.
- They are ordered from most to least matching.
-
limit
is the maximum number of hits to be returned. This can be set as a parameter during search. - Each hit has a
_highlights
field. This was the part of the document that matched the query the best.
Retrieve a document by ID.
result = mq.index("my-first-index").get_document(document_id="article_591")
Note that by adding the document using add_documents
again using the same _id
will cause a document to be updated.
Get information about an index.
results = mq.index("my-first-index").get_stats()
Perform a keyword search.
result = mq.index("my-first-index").search('marco polo', search_method=marqo.SearchMethods.LEXICAL)
To power image and text search, Marqo allows users to plug and play with CLIP models from HuggingFace. Note that if you do not configure multi modal search, image urls will be treated as strings. To start indexing and searching with images, first create an index with a CLIP configuration, as below:
settings = {
"treat_urls_and_pointers_as_images":True, # allows us to find an image file and index it
"model":"ViT-L/14"
}
response = mq.create_index("my-multimodal-index", **settings)
Images can then be added within documents as follows. You can use urls from the internet (for example S3) or from the disk of the machine:
response = mq.index("my-multimodal-index").add_documents([{
"My_Image": "https://raw.githubusercontent.com/marqo-ai/marqo-api-tests/mainline/assets/ai_hippo_realistic.png",
"Description": "The hippopotamus, also called the common hippopotamus or river hippopotamus, is a large semiaquatic mammal native to sub-Saharan Africa",
"_id": "hippo-facts"
}], tensor_fields=["My_Image"])
You can then search the image field using text.
results = mq.index("my-multimodal-index").search('animal')
Searching using an image can be achieved by providing the image link.
results = mq.index("my-multimodal-index").search('https://raw.githubusercontent.com/marqo-ai/marqo-api-tests/mainline/assets/ai_hippo_statue.png')
Queries can also be provided as dictionaries where each key is a query and their corresponding values are weights. This allows for more advanced queries consisting of multiple components with weightings towards or against them, queries can have negations via negative weighting.
The example below shows the application of this to a scenario where a user may want to ask a question but also negate results that match a certain semantic criterion.
import marqo
import pprint
mq = marqo.Client(url="http://localhost:8882")
mq.create_index("my-weighted-query-index")
mq.index("my-weighted-query-index").add_documents(
[
{
"Title": "Smartphone",
"Description": "A smartphone is a portable computer device that combines mobile telephone "
"functions and computing functions into one unit.",
},
{
"Title": "Telephone",
"Description": "A telephone is a telecommunications device that permits two or more users to"
"conduct a conversation when they are too far apart to be easily heard directly.",
},
{
"Title": "Thylacine",
"Description": "The thylacine, also commonly known as the Tasmanian tiger or Tasmanian wolf, "
"is an extinct carnivorous marsupial."
"The last known of its species died in 1936.",
}
],
tensor_fields=["Description"]
)
# initially we ask for a type of communications device which is popular in the 21st century
query = {
# a weighting of 1.1 gives this query slightly more importance
"I need to buy a communications device, what should I get?": 1.1,
# a weighting of 1 gives this query a neutral importance
# this will lead to 'Smartphone' being the top result
"The device should work like an intelligent computer.": 1.0,
}
results = mq.index("my-weighted-query-index").search(q=query)
print("Query 1:")
pprint.pprint(results)
# now we ask for a type of communications which predates the 21st century
query = {
# a weighting of 1 gives this query a neutral importance
"I need to buy a communications device, what should I get?": 1.0,
# a weighting of -1 gives this query a negation effect
# this will lead to 'Telephone' being the top result
"The device should work like an intelligent computer.": -0.3,
}
results = mq.index("my-weighted-query-index").search(q=query)
print("\nQuery 2:")
pprint.pprint(results)
Marqo lets you have indexes with multimodal combination fields. Multimodal combination fields can combine text and images into one field. This allows scoring of documents across the combined text and image fields together. It also allows for a single vector representation instead of needing many which saves on storage. The relative weighting of each component can be set per document.
The example below demonstrates this with retrieval of caption and image pairs using multiple types of queries.
import marqo
import pprint
mq = marqo.Client(url="http://localhost:8882")
settings = {"treat_urls_and_pointers_as_images": True, "model": "ViT-L/14"}
mq.create_index("my-first-multimodal-index", **settings)
mq.index("my-first-multimodal-index").add_documents(
[
{
"Title": "Flying Plane",
"caption": "An image of a passenger plane flying in front of the moon.",
"image": "https://raw.githubusercontent.com/marqo-ai/marqo/mainline/examples/ImageSearchGuide/data/image2.jpg",
},
{
"Title": "Red Bus",
"caption": "A red double decker London bus traveling to Aldwych",
"image": "https://raw.githubusercontent.com/marqo-ai/marqo/mainline/examples/ImageSearchGuide/data/image4.jpg",
},
{
"Title": "Horse Jumping",
"caption": "A person riding a horse over a jump in a competition.",
"image": "https://raw.githubusercontent.com/marqo-ai/marqo/mainline/examples/ImageSearchGuide/data/image1.jpg",
},
],
# Create the mappings, here we define our captioned_image mapping
# which weights the image more heavily than the caption - these pairs
# will be represented by a single vector in the index
mappings={
"captioned_image": {
"type": "multimodal_combination",
"weights": {
"caption": 0.3,
"image": 0.7
}
}
},
# We specify which fields to create vectors for.
# Note that captioned_image is treated as a single field.
tensor_fields=["captioned_image"]
)
# Search this index with a simple text query
results = mq.index("my-first-multimodal-index").search(
q="Give me some images of vehicles and modes of transport. I am especially interested in air travel and commercial aeroplanes."
)
print("Query 1:")
pprint.pprint(results)
# search the index with a query that uses weighted components
results = mq.index("my-first-multimodal-index").search(
q={
"What are some vehicles and modes of transport?": 1.0,
"Aeroplanes and other things that fly": -1.0
},
)
print("\nQuery 2:")
pprint.pprint(results)
results = mq.index("my-first-multimodal-index").search(
q={"Animals of the Perissodactyla order": -1.0}
)
print("\nQuery 3:")
pprint.pprint(results)
Delete documents.
results = mq.index("my-first-index").delete_documents(ids=["article_591", "article_602"])
Delete an index.
results = mq.index("my-first-index").delete()
We support Kubernetes templates for Marqo which you can deploy on a cloud provider of your choice. Marqo's Kubernetes implementation allows you to deploy clusters with replicas, multiple storage shards and multiple inference nodes. The repo can be found here: https://github.com/marqo-ai/marqo-on-kubernetes
If you're looking for a fully managed cloud service, you can sign up for Marqo Cloud here: https://cloud.marqo.ai.
The full documentation for Marqo can be found here https://docs.marqo.ai/.
Note that you should not run other applications on Marqo's Vespa cluster as Marqo automatically changes and adapts the settings on the cluster.
Marqo is a community project with the goal of making tensor search accessible to the wider developer community. We are glad that you are interested in helping out! Please read this to get started.
-
Create a virtual env
python -m venv ./venv
. -
Activate the virtual environment
source ./venv/bin/activate
. -
Install requirements from the requirements file:
pip install -r requirements.txt
. -
Run tests by running the tox file. CD into this dir and then run "tox".
-
If you update dependencies, make sure to delete the .tox dir and rerun.
-
Run the full test suite (by using the command
tox
in this dir). -
Create a pull request with an attached github issue.
- Ask questions and share your creations with the community on our Discourse forum.
- Join our Slack community and chat with other community members about ideas.
For Tasks:
Click tags to check more tools for each tasksFor Jobs:
Alternative AI tools for marqo
Similar Open Source Tools
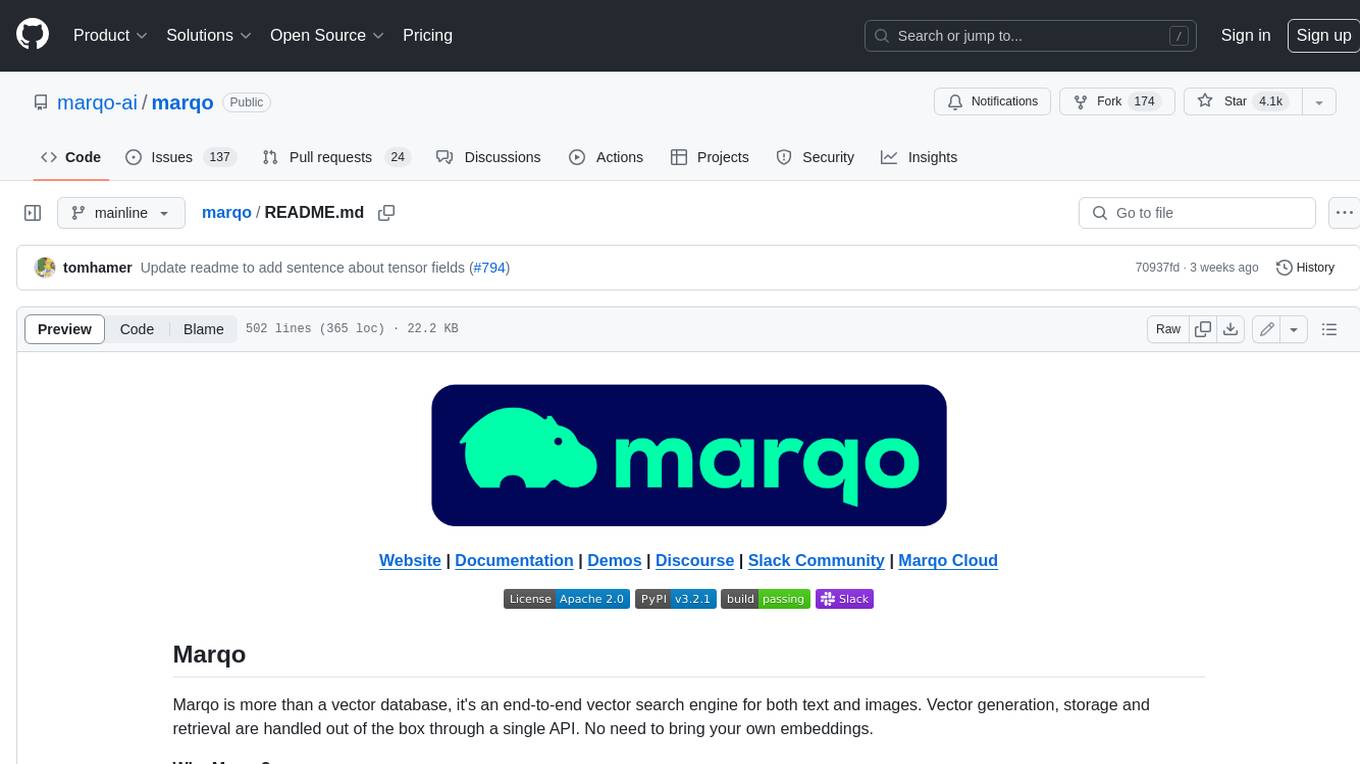
marqo
Marqo is more than a vector database, it's an end-to-end vector search engine for both text and images. Vector generation, storage and retrieval are handled out of the box through a single API. No need to bring your own embeddings.
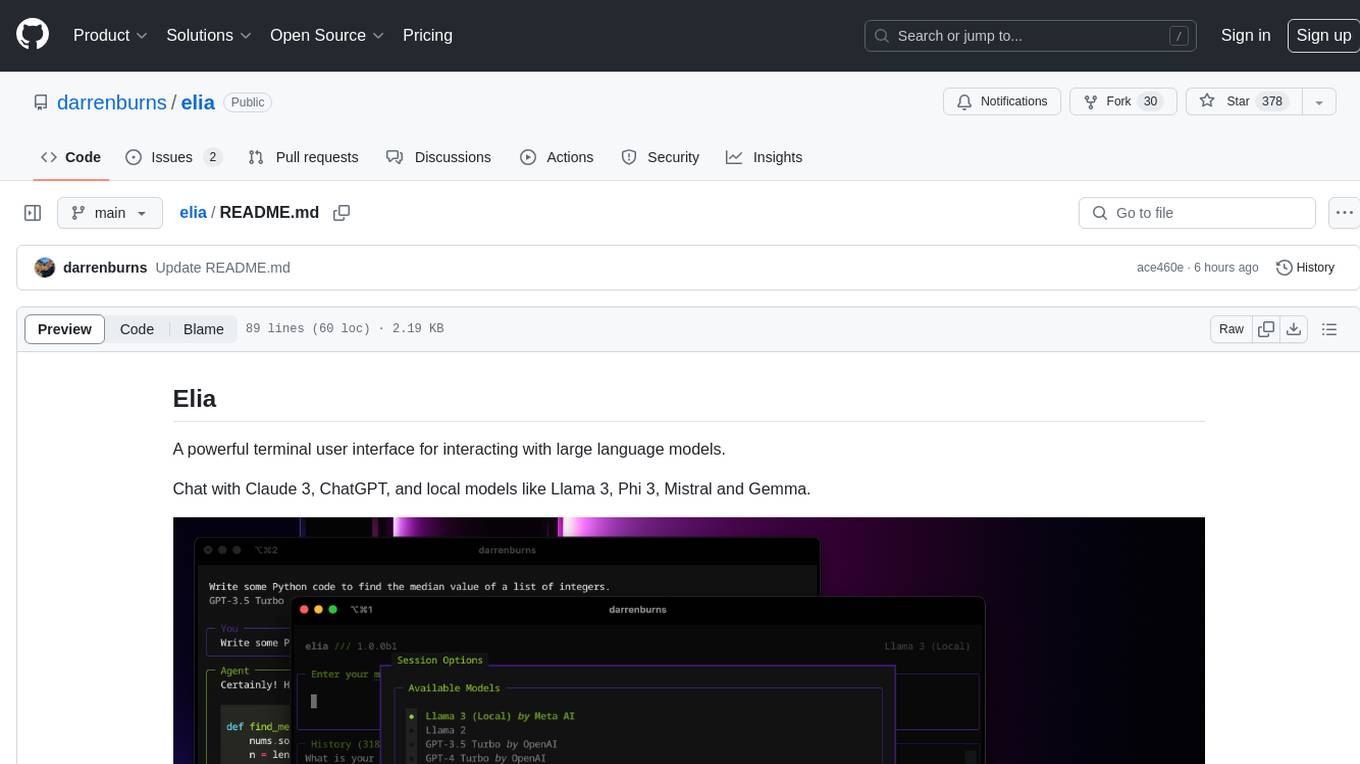
elia
Elia is a powerful terminal user interface designed for interacting with large language models. It allows users to chat with models like Claude 3, ChatGPT, Llama 3, Phi 3, Mistral, and Gemma. Conversations are stored locally in a SQLite database, ensuring privacy. Users can run local models through 'ollama' without data leaving their machine. Elia offers easy installation with pipx and supports various environment variables for different models. It provides a quick start to launch chats and manage local models. Configuration options are available to customize default models, system prompts, and add new models. Users can import conversations from ChatGPT and wipe the database when needed. Elia aims to enhance user experience in interacting with language models through a user-friendly interface.
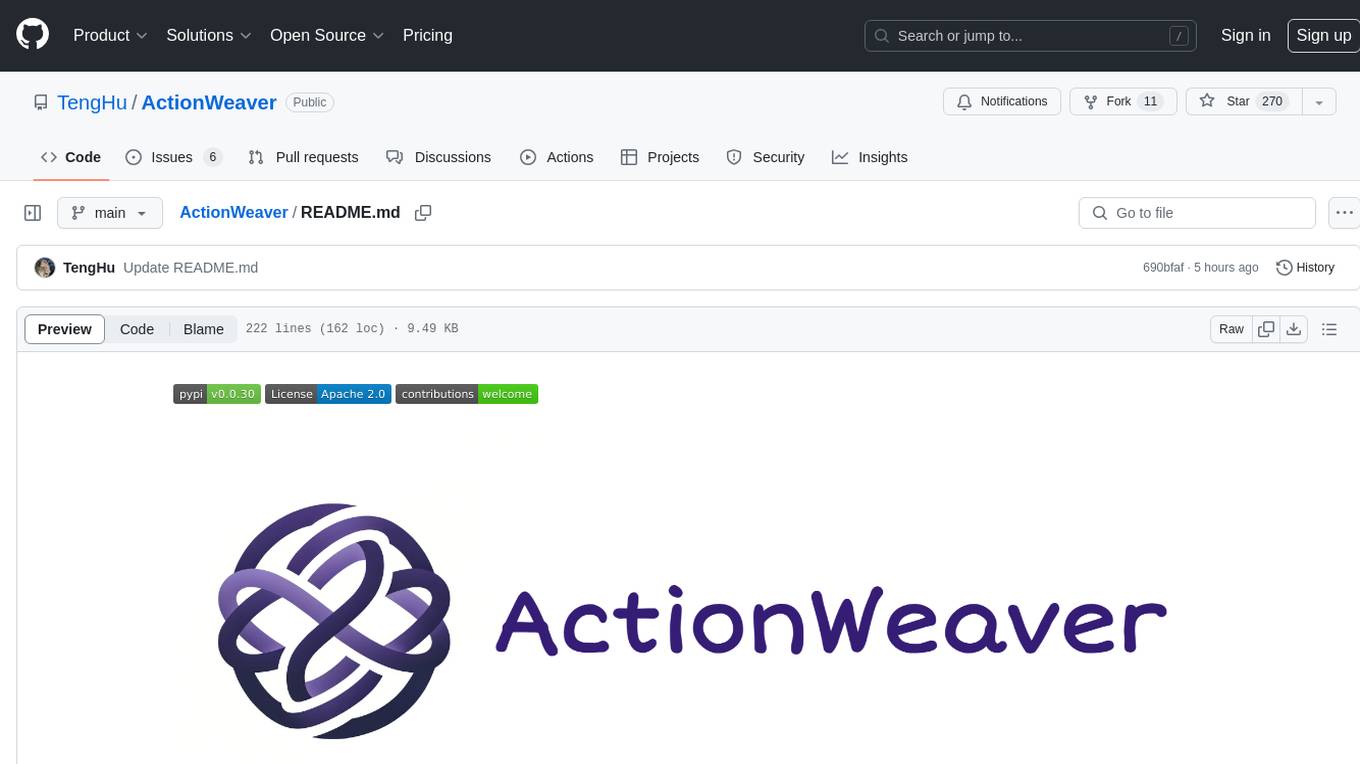
ActionWeaver
ActionWeaver is an AI application framework designed for simplicity, relying on OpenAI and Pydantic. It supports both OpenAI API and Azure OpenAI service. The framework allows for function calling as a core feature, extensibility to integrate any Python code, function orchestration for building complex call hierarchies, and telemetry and observability integration. Users can easily install ActionWeaver using pip and leverage its capabilities to create, invoke, and orchestrate actions with the language model. The framework also provides structured extraction using Pydantic models and allows for exception handling customization. Contributions to the project are welcome, and users are encouraged to cite ActionWeaver if found useful.
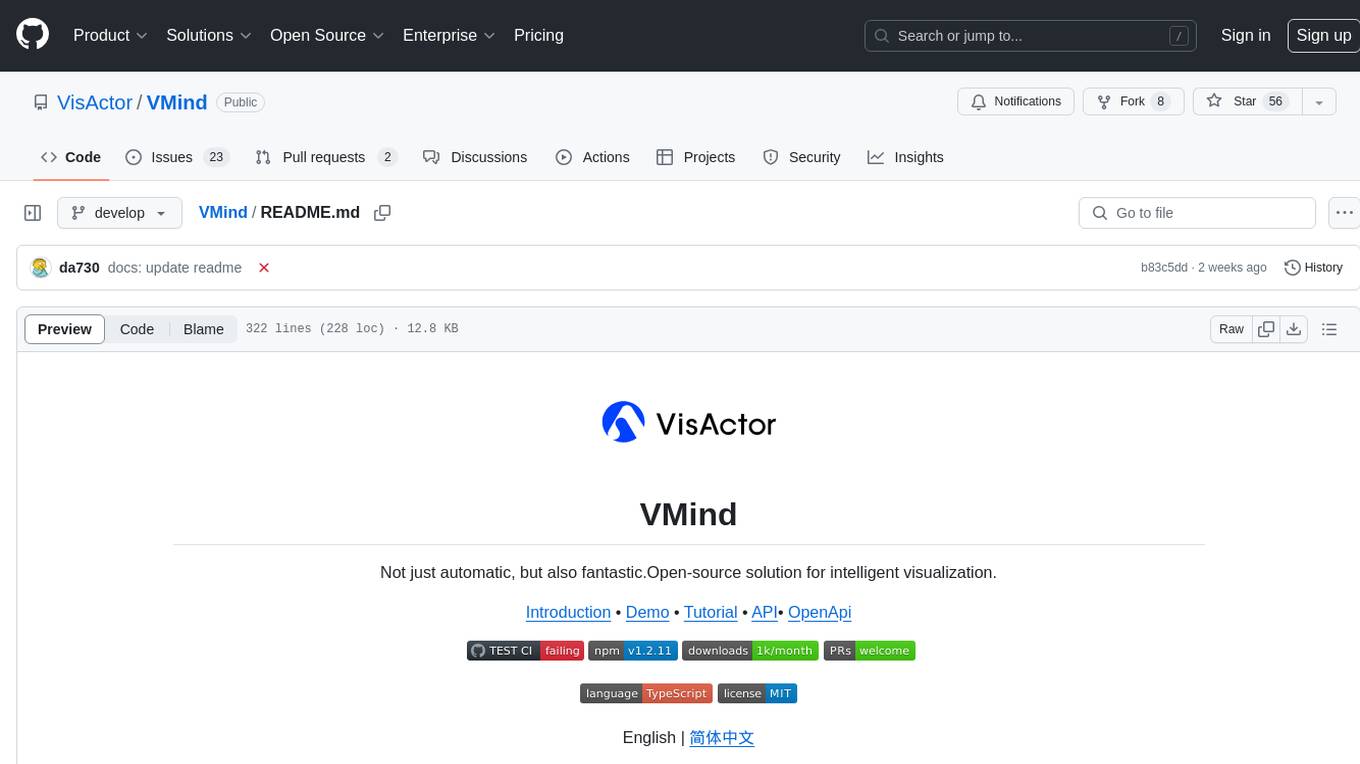
VMind
VMind is an open-source solution for intelligent visualization, providing an intelligent chart component based on LLM by VisActor. It allows users to create chart narrative works with natural language interaction, edit charts through dialogue, and export narratives as videos or GIFs. The tool is easy to use, scalable, supports various chart types, and offers one-click export functionality. Users can customize chart styles, specify themes, and aggregate data using LLM models. VMind aims to enhance efficiency in creating data visualization works through dialogue-based editing and natural language interaction.
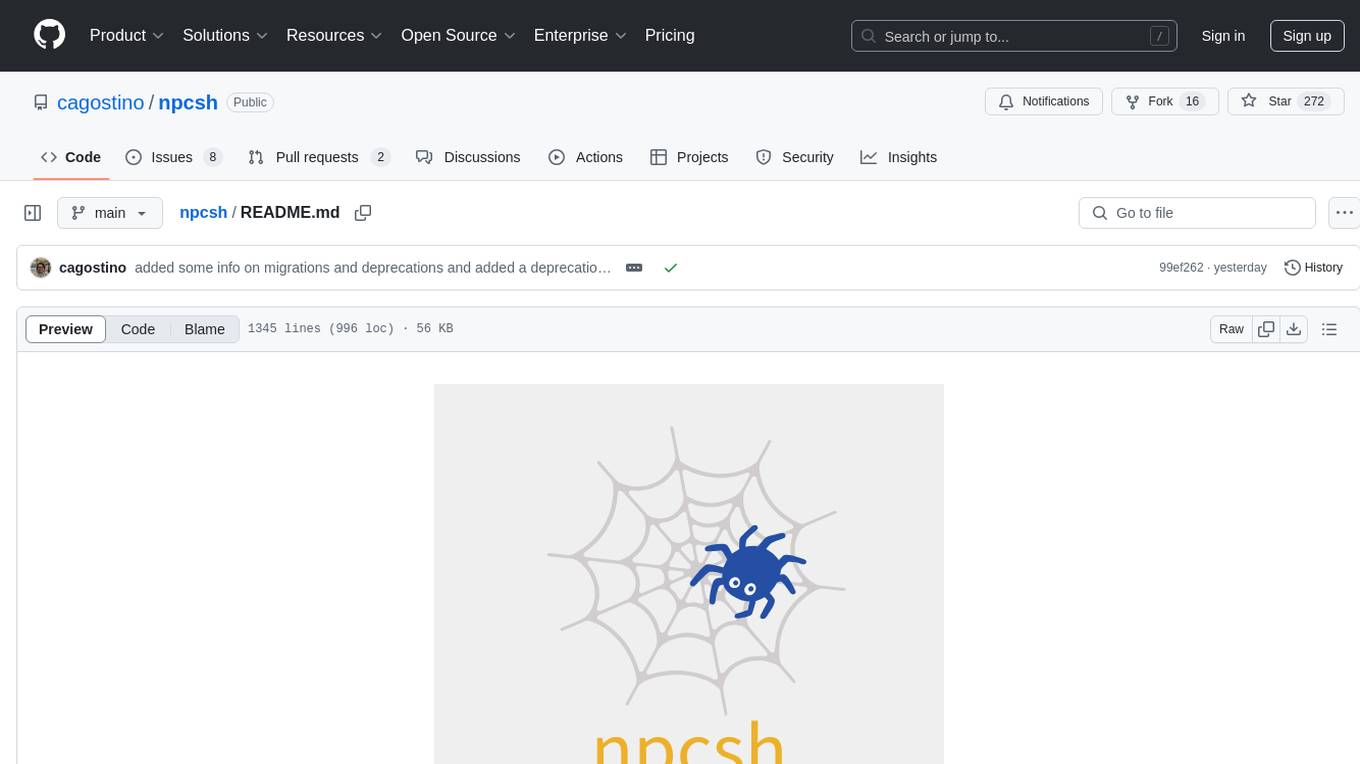
npcsh
`npcsh` is a python-based command-line tool designed to integrate Large Language Models (LLMs) and Agents into one's daily workflow by making them available and easily configurable through the command line shell. It leverages the power of LLMs to understand natural language commands and questions, execute tasks, answer queries, and provide relevant information from local files and the web. Users can also build their own tools and call them like macros from the shell. `npcsh` allows users to take advantage of agents (i.e. NPCs) through a managed system, tailoring NPCs to specific tasks and workflows. The tool is extensible with Python, providing useful functions for interacting with LLMs, including explicit coverage for popular providers like ollama, anthropic, openai, gemini, deepseek, and openai-like providers. Users can set up a flask server to expose their NPC team for use as a backend service, run SQL models defined in their project, execute assembly lines, and verify the integrity of their NPC team's interrelations. Users can execute bash commands directly, use favorite command-line tools like VIM, Emacs, ipython, sqlite3, git, pipe the output of these commands to LLMs, or pass LLM results to bash commands.
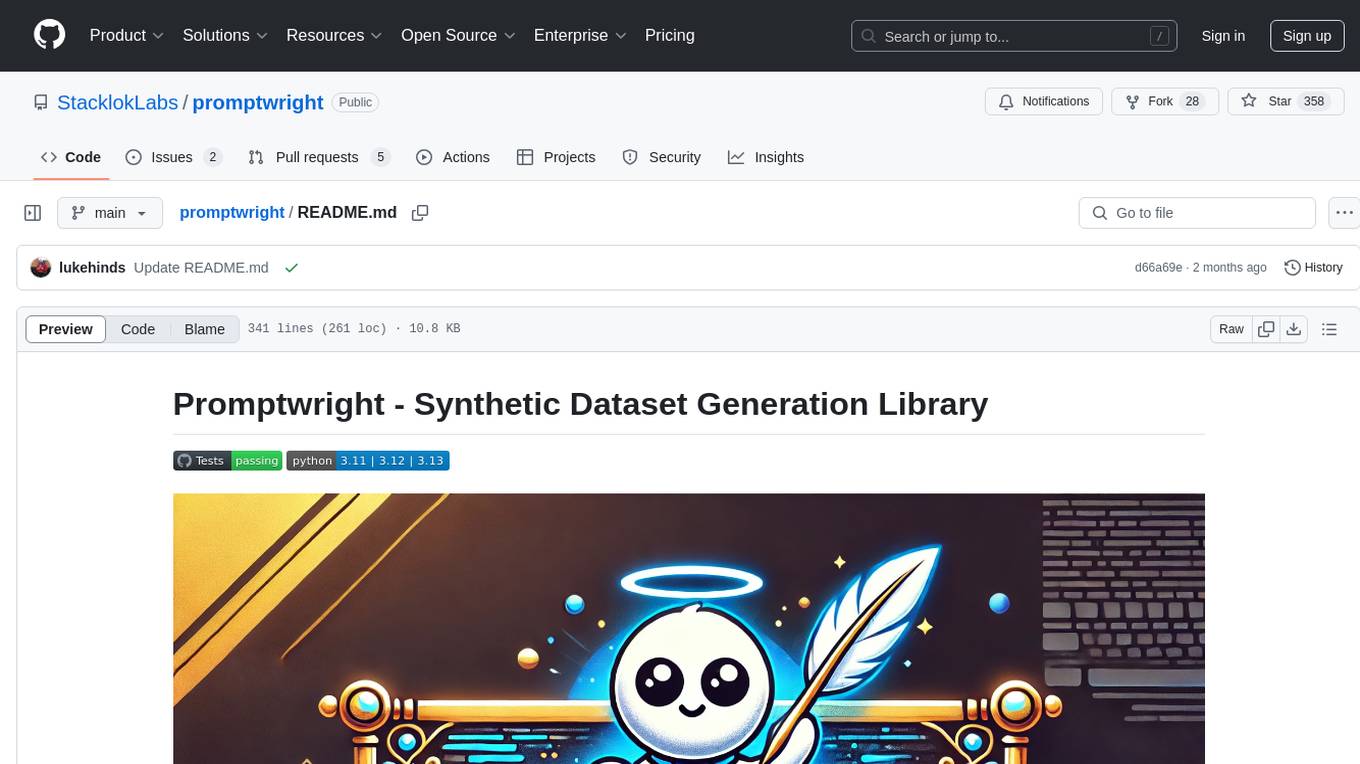
promptwright
Promptwright is a Python library designed for generating large synthetic datasets using a local LLM and various LLM service providers. It offers flexible interfaces for generating prompt-led synthetic datasets. The library supports multiple providers, configurable instructions and prompts, YAML configuration for tasks, command line interface for running tasks, push to Hugging Face Hub for dataset upload, and system message control. Users can define generation tasks using YAML configuration or Python code. Promptwright integrates with LiteLLM to interface with LLM providers and supports automatic dataset upload to Hugging Face Hub.
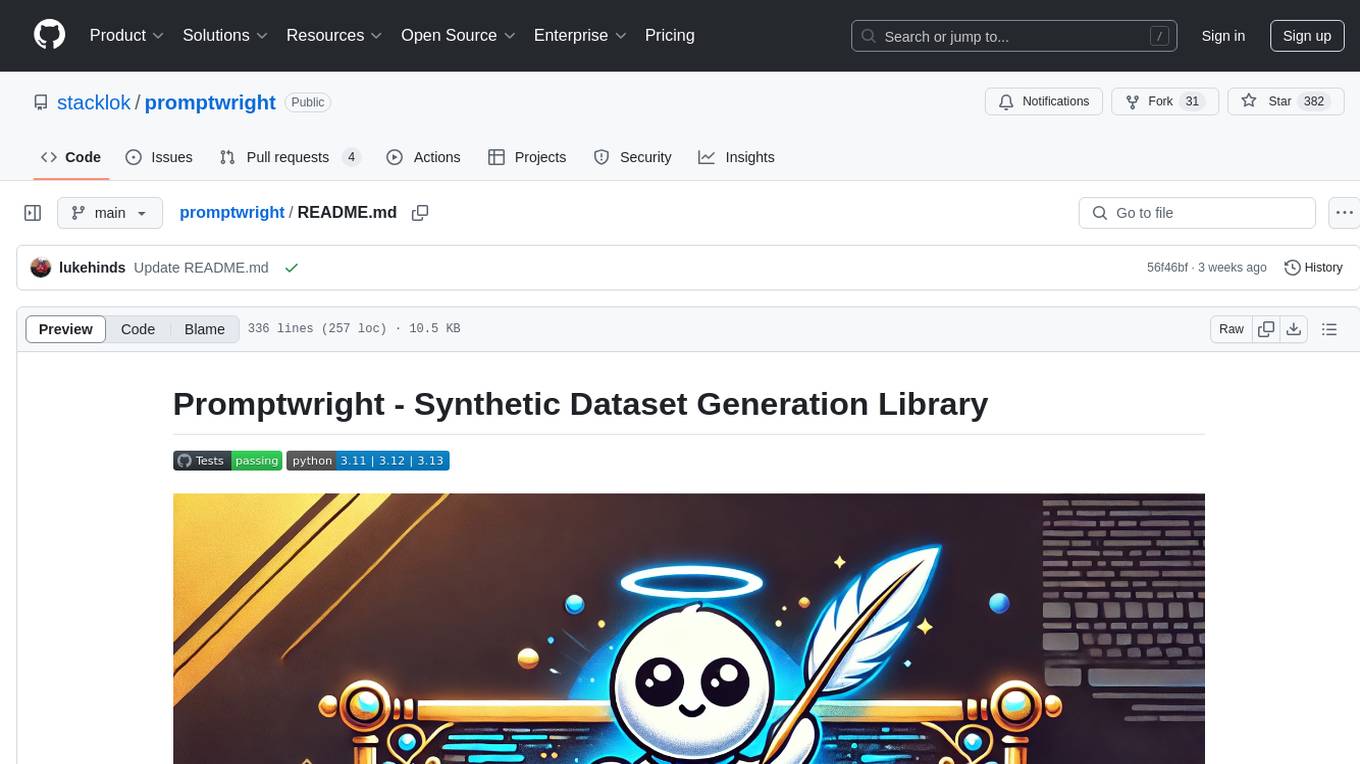
promptwright
Promptwright is a Python library designed for generating large synthetic datasets using local LLM and various LLM service providers. It offers flexible interfaces for generating prompt-led synthetic datasets. The library supports multiple providers, configurable instructions and prompts, YAML configuration, command line interface, push to Hugging Face Hub, and system message control. Users can define generation tasks using YAML configuration files or programmatically using Python code. Promptwright integrates with LiteLLM for LLM providers and supports automatic dataset upload to Hugging Face Hub. The library is not responsible for the content generated by models and advises users to review the data before using it in production environments.
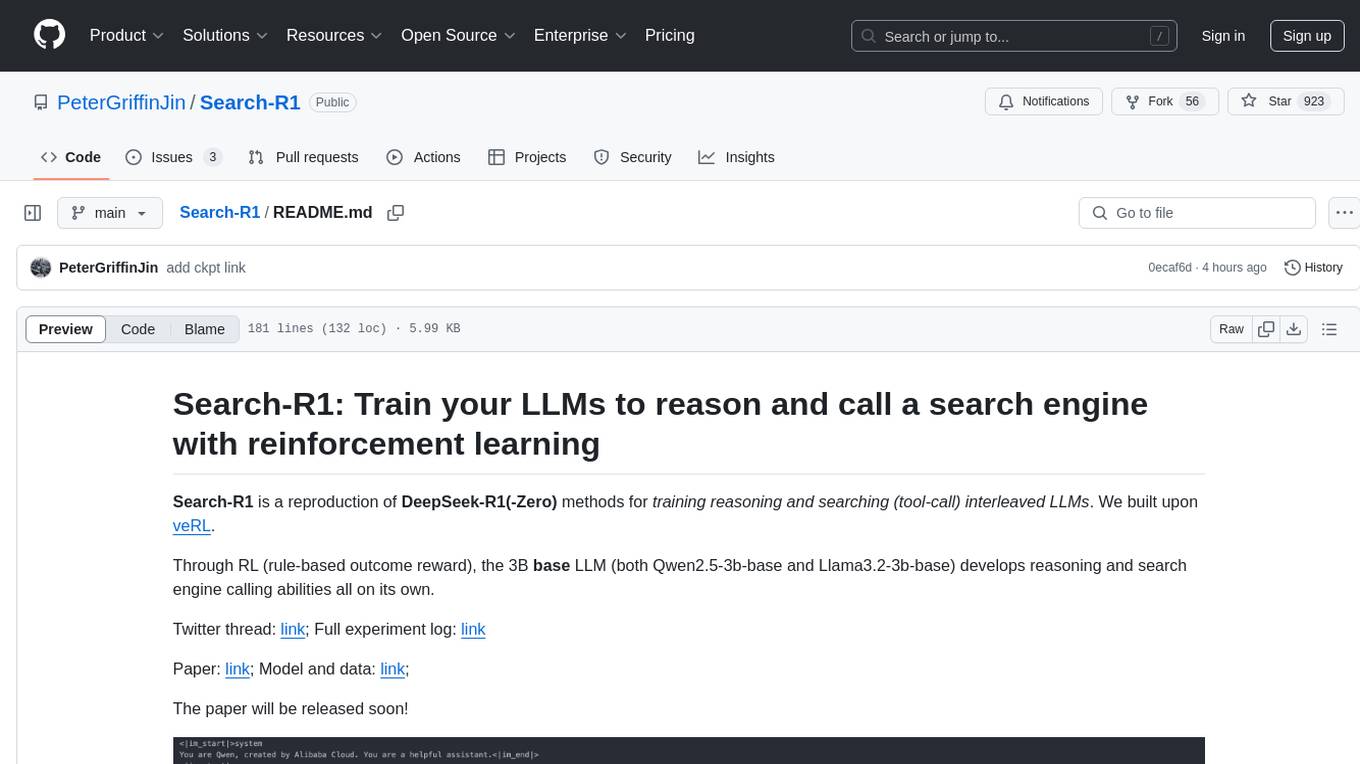
Search-R1
Search-R1 is a tool that trains large language models (LLMs) to reason and call a search engine using reinforcement learning. It is a reproduction of DeepSeek-R1 methods for training reasoning and searching interleaved LLMs, built upon veRL. Through rule-based outcome reward, the base LLM develops reasoning and search engine calling abilities independently. Users can train LLMs on their own datasets and search engines, with preliminary results showing improved performance in search engine calling and reasoning tasks.
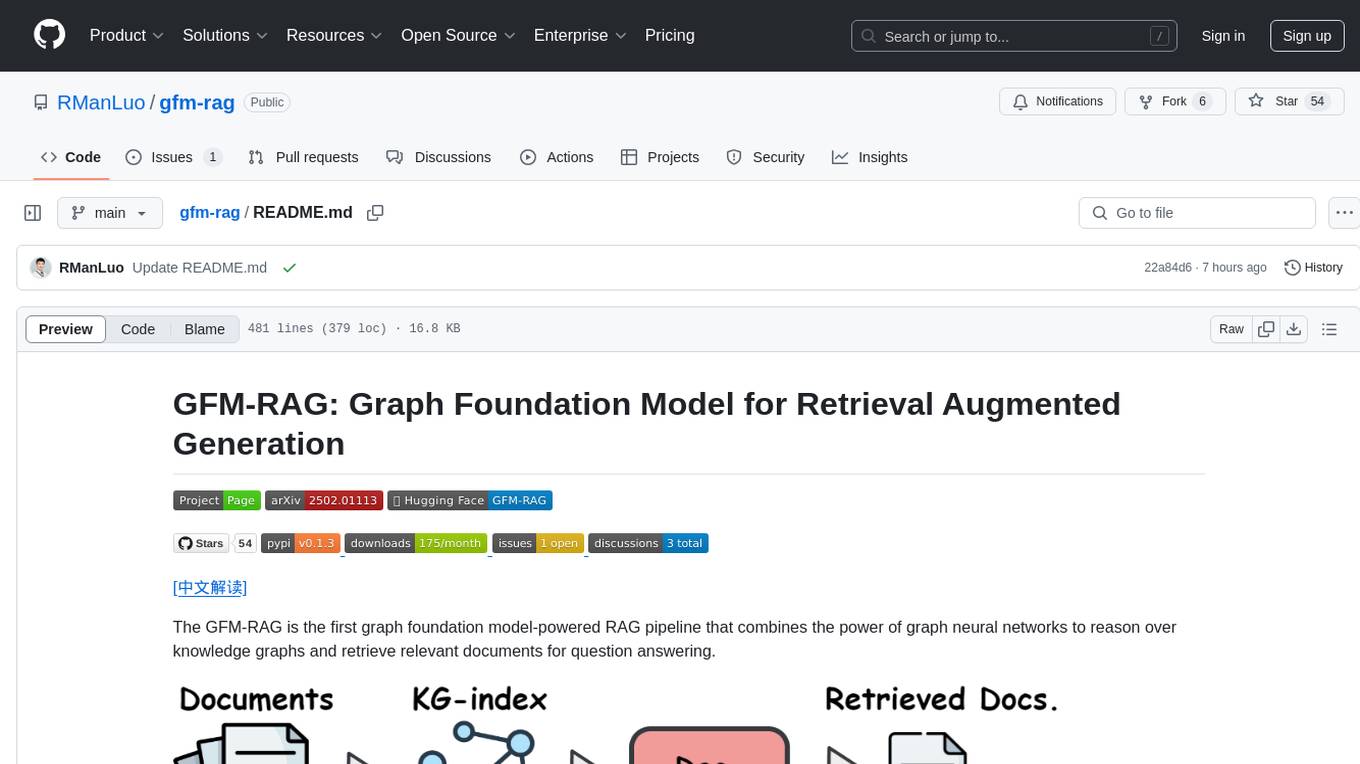
gfm-rag
The GFM-RAG is a graph foundation model-powered pipeline that combines graph neural networks to reason over knowledge graphs and retrieve relevant documents for question answering. It features a knowledge graph index, efficiency in multi-hop reasoning, generalizability to unseen datasets, transferability for fine-tuning, compatibility with agent-based frameworks, and interpretability of reasoning paths. The tool can be used for conducting retrieval and question answering tasks using pre-trained models or fine-tuning on custom datasets.
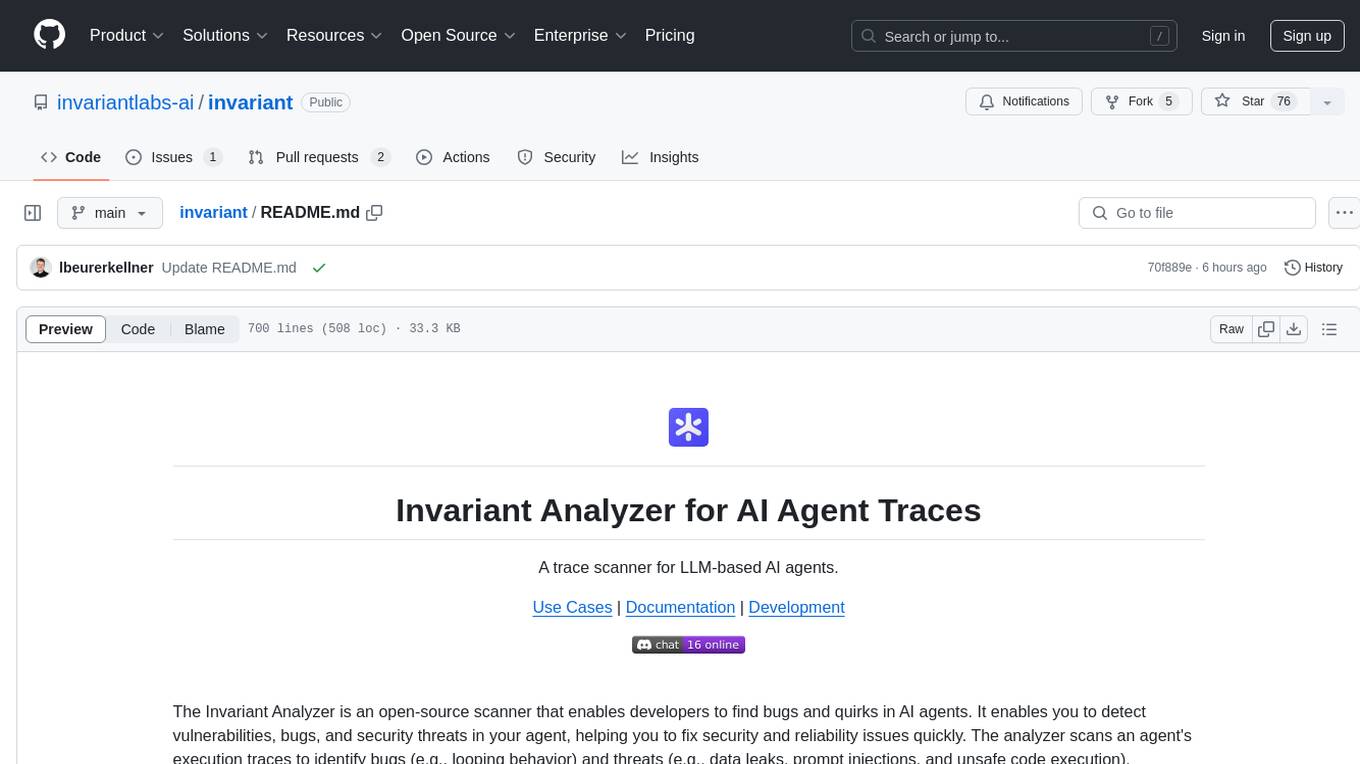
invariant
Invariant Analyzer is an open-source scanner designed for LLM-based AI agents to find bugs, vulnerabilities, and security threats. It scans agent execution traces to identify issues like looping behavior, data leaks, prompt injections, and unsafe code execution. The tool offers a library of built-in checkers, an expressive policy language, data flow analysis, real-time monitoring, and extensible architecture for custom checkers. It helps developers debug AI agents, scan for security violations, and prevent security issues and data breaches during runtime. The analyzer leverages deep contextual understanding and a purpose-built rule matching engine for security policy enforcement.
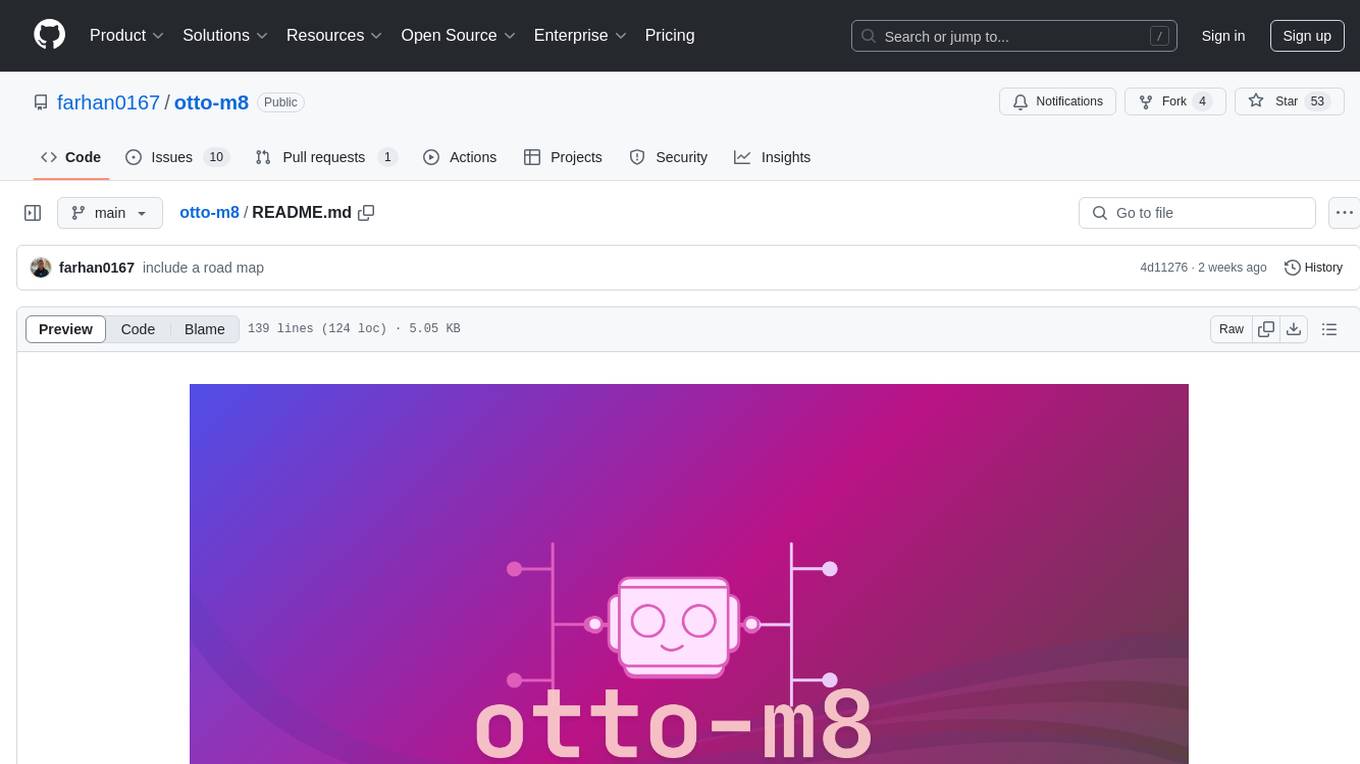
otto-m8
otto-m8 is a flowchart based automation platform designed to run deep learning workloads with minimal to no code. It provides a user-friendly interface to spin up a wide range of AI models, including traditional deep learning models and large language models. The tool deploys Docker containers of workflows as APIs for integration with existing workflows, building AI chatbots, or standalone applications. Otto-m8 operates on an Input, Process, Output paradigm, simplifying the process of running AI models into a flowchart-like UI.
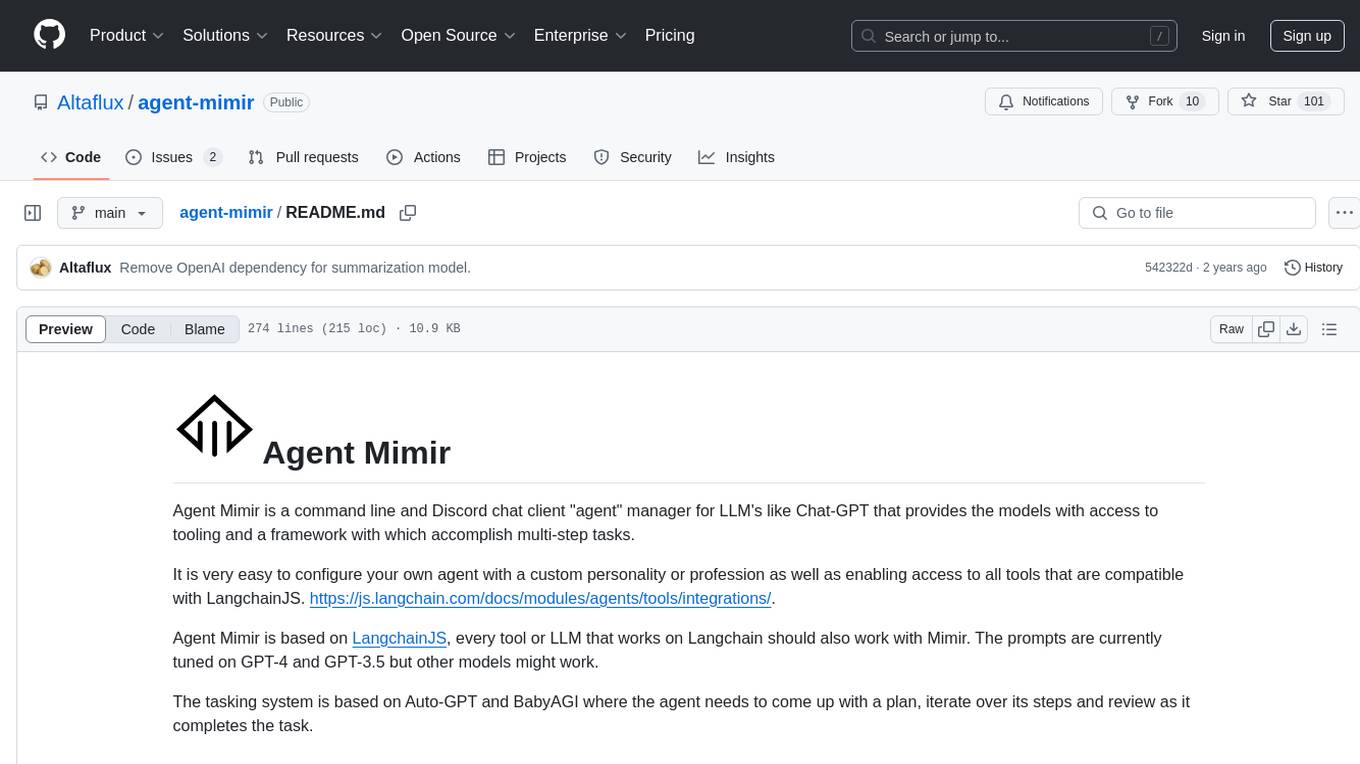
agent-mimir
Agent Mimir is a command line and Discord chat client 'agent' manager for LLM's like Chat-GPT that provides the models with access to tooling and a framework with which accomplish multi-step tasks. It is easy to configure your own agent with a custom personality or profession as well as enabling access to all tools that are compatible with LangchainJS. Agent Mimir is based on LangchainJS, every tool or LLM that works on Langchain should also work with Mimir. The tasking system is based on Auto-GPT and BabyAGI where the agent needs to come up with a plan, iterate over its steps and review as it completes the task.
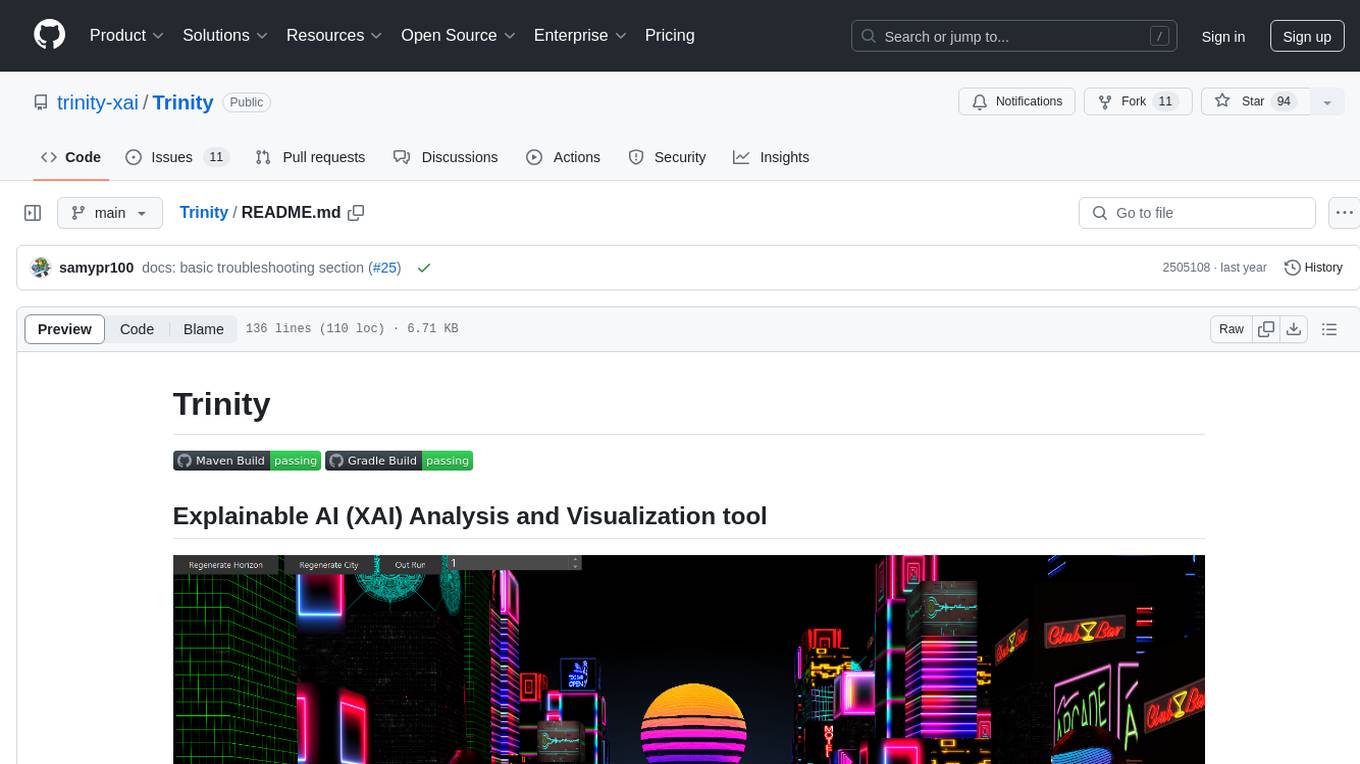
Trinity
Trinity is an Explainable AI (XAI) Analysis and Visualization tool designed for Deep Learning systems or other models performing complex classification or decoding. It provides performance analysis through interactive 3D projections that are hyper-dimensional aware, allowing users to explore hyperspace, hypersurface, projections, and manifolds. Trinity primarily works with JSON data formats and supports the visualization of FeatureVector objects. Users can analyze and visualize data points, correlate inputs with classification results, and create custom color maps for better data interpretation. Trinity has been successfully applied to various use cases including Deep Learning Object detection models, COVID gene/tissue classification, Brain Computer Interface decoders, and Large Language Model (ChatGPT) Embeddings Analysis.
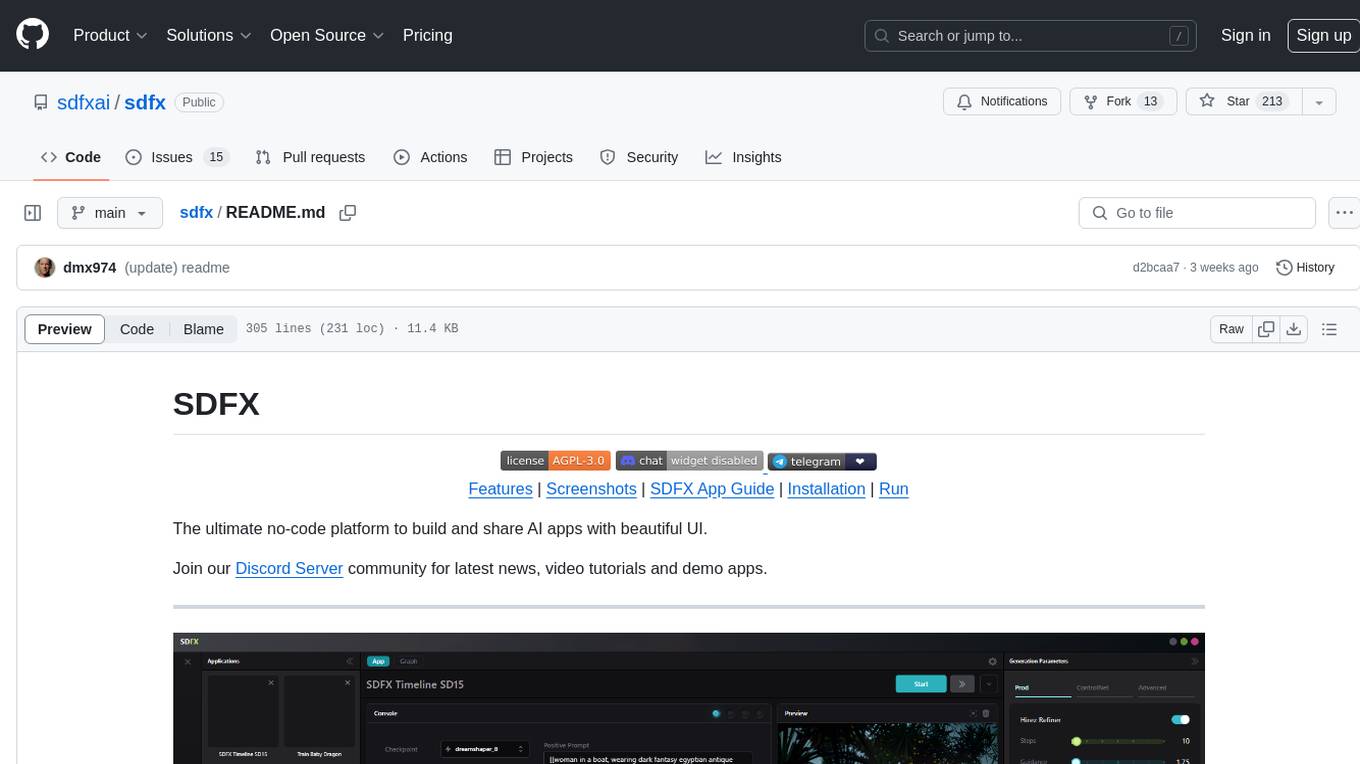
sdfx
SDFX is the ultimate no-code platform for building and sharing AI apps with beautiful UI. It enables the creation of user-friendly interfaces for complex workflows by combining Comfy workflow with a UI. The tool is designed to merge the benefits of form-based UI and graph-node based UI, allowing users to create intricate graphs with a high-level UI overlay. SDFX is fully compatible with ComfyUI, abstracting the need for installing ComfyUI. It offers features like animated graph navigation, node bookmarks, UI debugger, custom nodes manager, app and template export, image and mask editor, and more. The tool compiles as a native app or web app, making it easy to maintain and add new features.
For similar tasks
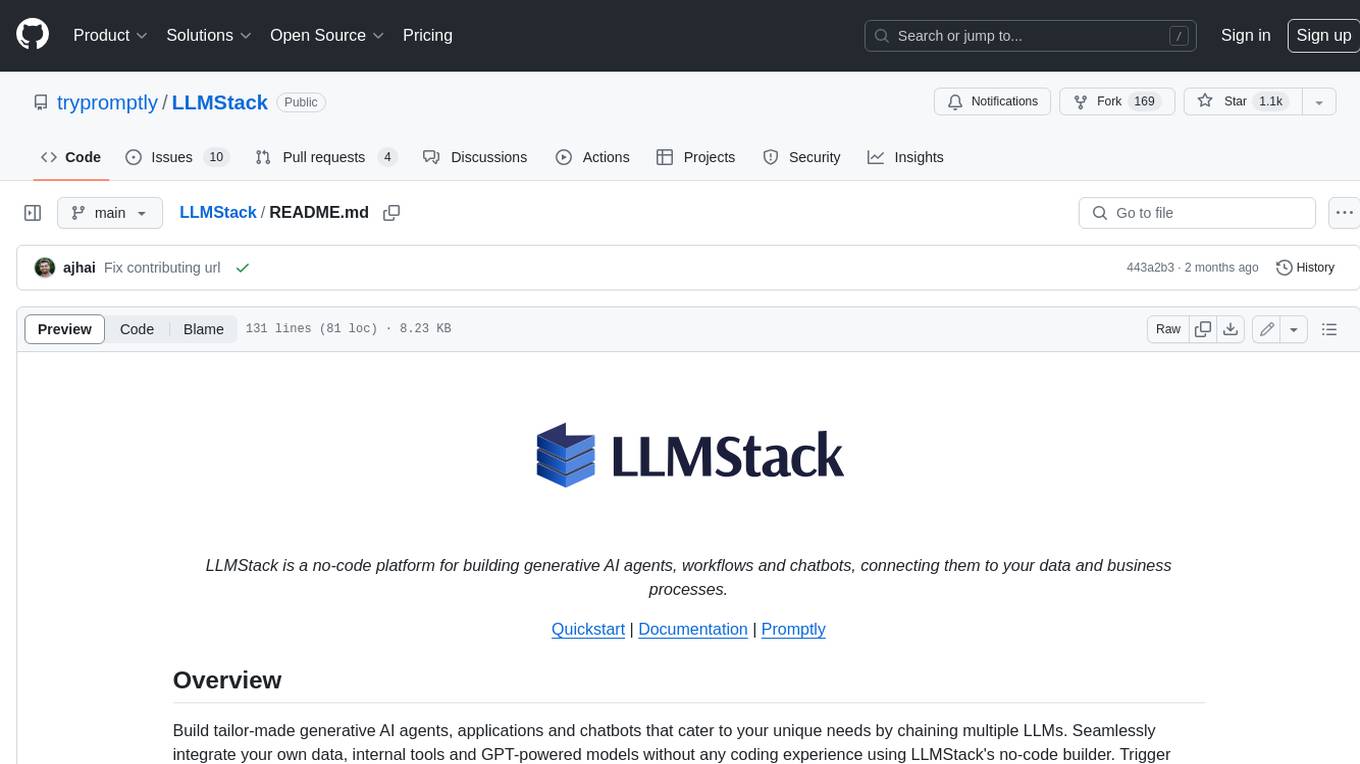
LLMStack
LLMStack is a no-code platform for building generative AI agents, workflows, and chatbots. It allows users to connect their own data, internal tools, and GPT-powered models without any coding experience. LLMStack can be deployed to the cloud or on-premise and can be accessed via HTTP API or triggered from Slack or Discord.
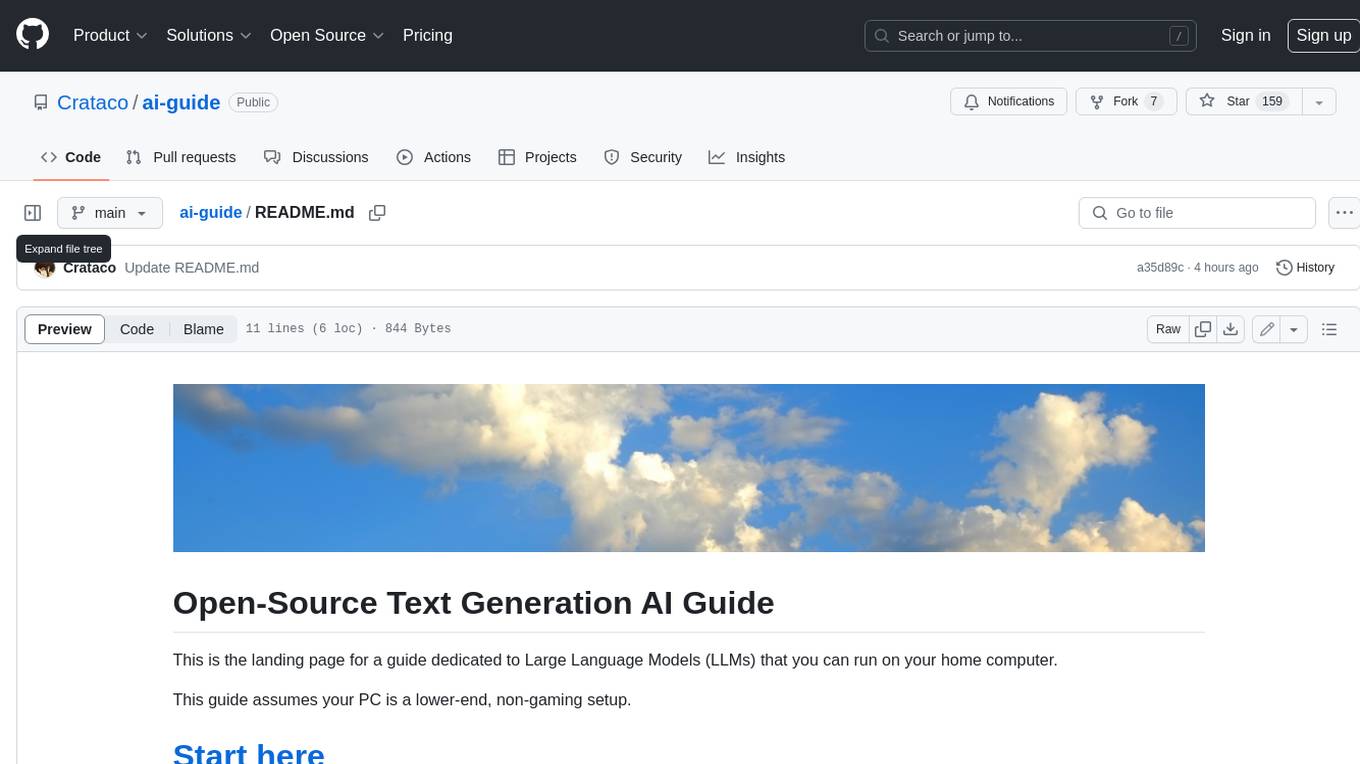
ai-guide
This guide is dedicated to Large Language Models (LLMs) that you can run on your home computer. It assumes your PC is a lower-end, non-gaming setup.
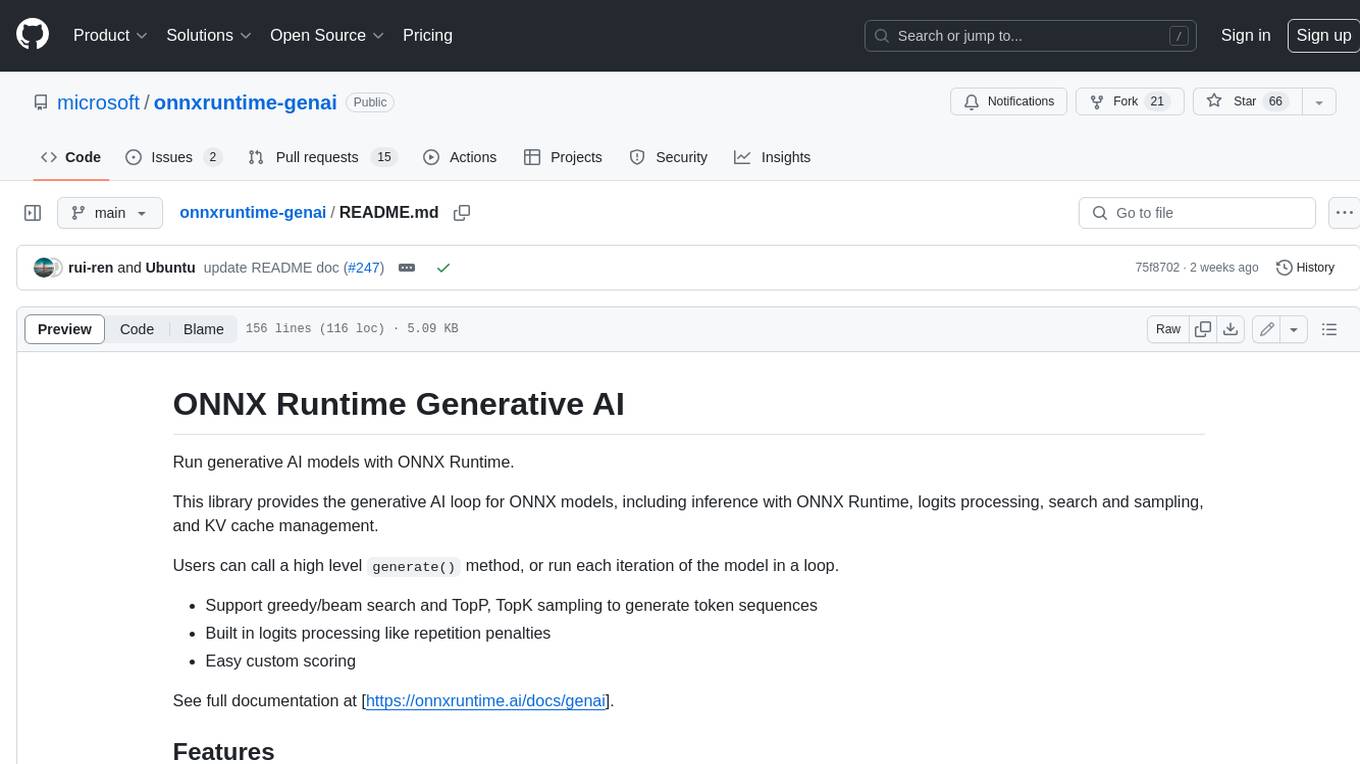
onnxruntime-genai
ONNX Runtime Generative AI is a library that provides the generative AI loop for ONNX models, including inference with ONNX Runtime, logits processing, search and sampling, and KV cache management. Users can call a high level `generate()` method, or run each iteration of the model in a loop. It supports greedy/beam search and TopP, TopK sampling to generate token sequences, has built in logits processing like repetition penalties, and allows for easy custom scoring.
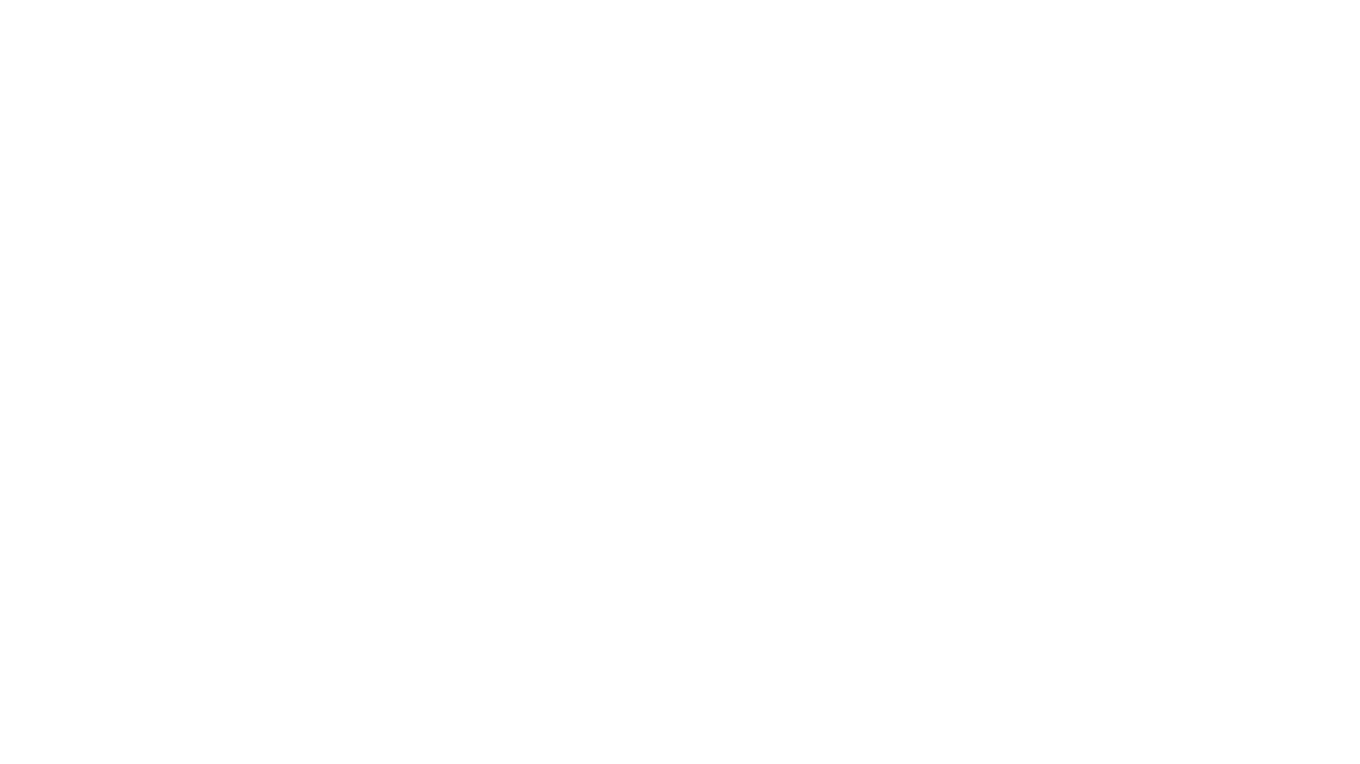
jupyter-ai
Jupyter AI connects generative AI with Jupyter notebooks. It provides a user-friendly and powerful way to explore generative AI models in notebooks and improve your productivity in JupyterLab and the Jupyter Notebook. Specifically, Jupyter AI offers: * An `%%ai` magic that turns the Jupyter notebook into a reproducible generative AI playground. This works anywhere the IPython kernel runs (JupyterLab, Jupyter Notebook, Google Colab, Kaggle, VSCode, etc.). * A native chat UI in JupyterLab that enables you to work with generative AI as a conversational assistant. * Support for a wide range of generative model providers, including AI21, Anthropic, AWS, Cohere, Gemini, Hugging Face, NVIDIA, and OpenAI. * Local model support through GPT4All, enabling use of generative AI models on consumer grade machines with ease and privacy.
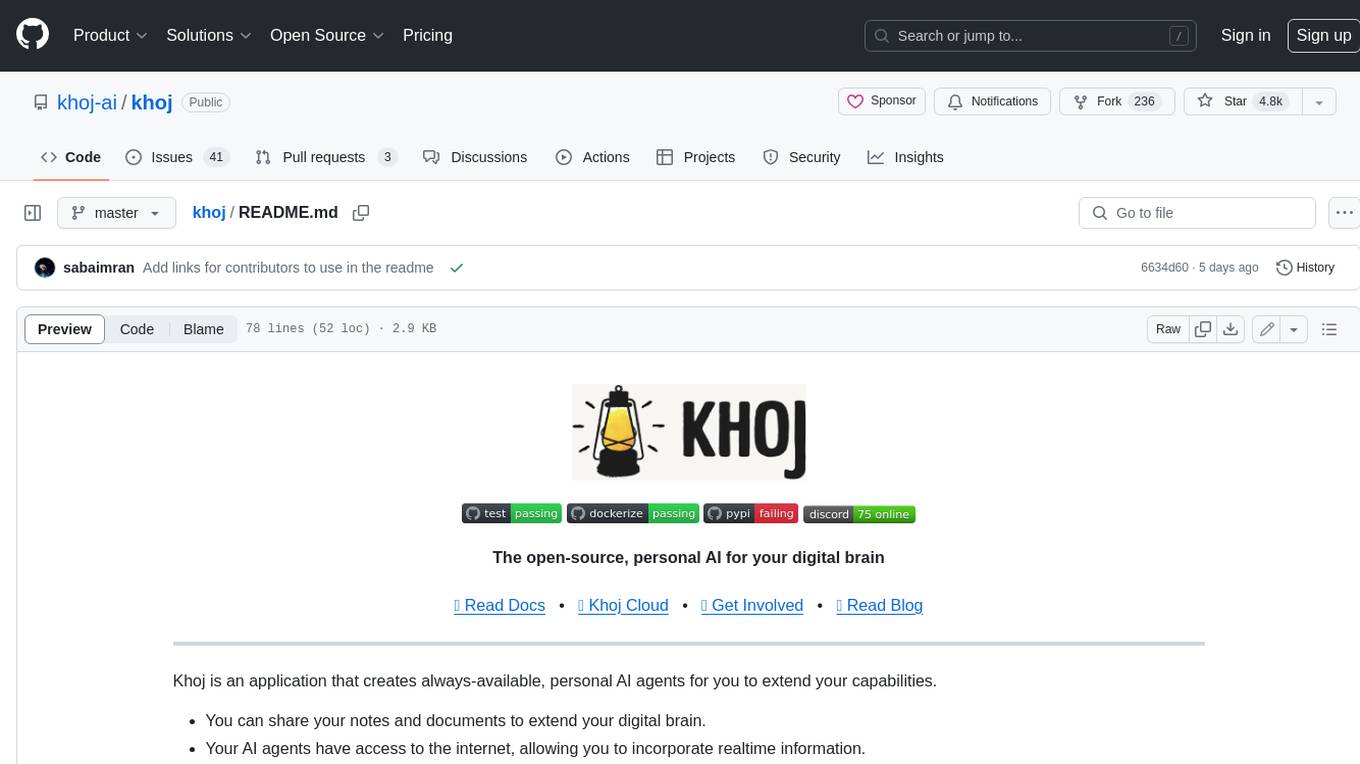
khoj
Khoj is an open-source, personal AI assistant that extends your capabilities by creating always-available AI agents. You can share your notes and documents to extend your digital brain, and your AI agents have access to the internet, allowing you to incorporate real-time information. Khoj is accessible on Desktop, Emacs, Obsidian, Web, and Whatsapp, and you can share PDF, markdown, org-mode, notion files, and GitHub repositories. You'll get fast, accurate semantic search on top of your docs, and your agents can create deeply personal images and understand your speech. Khoj is self-hostable and always will be.
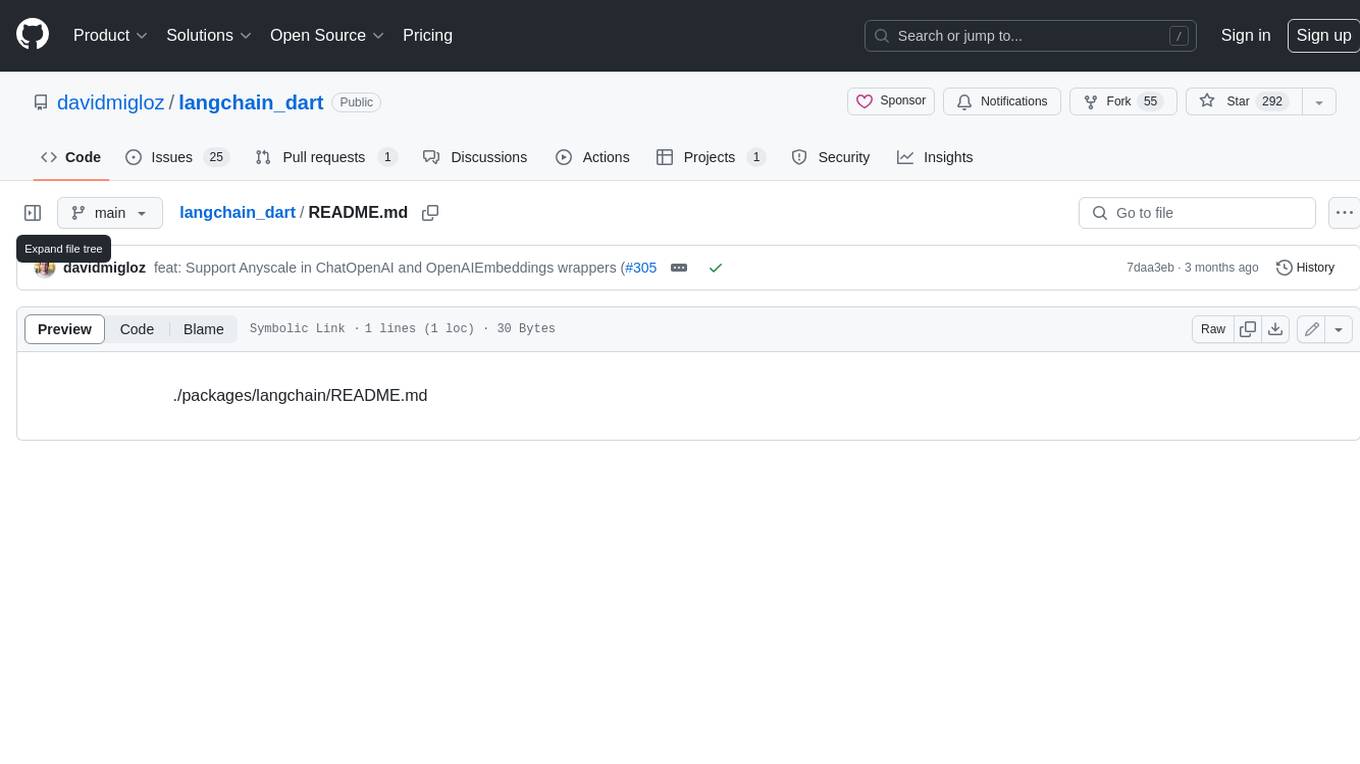
langchain_dart
LangChain.dart is a Dart port of the popular LangChain Python framework created by Harrison Chase. LangChain provides a set of ready-to-use components for working with language models and a standard interface for chaining them together to formulate more advanced use cases (e.g. chatbots, Q&A with RAG, agents, summarization, extraction, etc.). The components can be grouped into a few core modules: * **Model I/O:** LangChain offers a unified API for interacting with various LLM providers (e.g. OpenAI, Google, Mistral, Ollama, etc.), allowing developers to switch between them with ease. Additionally, it provides tools for managing model inputs (prompt templates and example selectors) and parsing the resulting model outputs (output parsers). * **Retrieval:** assists in loading user data (via document loaders), transforming it (with text splitters), extracting its meaning (using embedding models), storing (in vector stores) and retrieving it (through retrievers) so that it can be used to ground the model's responses (i.e. Retrieval-Augmented Generation or RAG). * **Agents:** "bots" that leverage LLMs to make informed decisions about which available tools (such as web search, calculators, database lookup, etc.) to use to accomplish the designated task. The different components can be composed together using the LangChain Expression Language (LCEL).
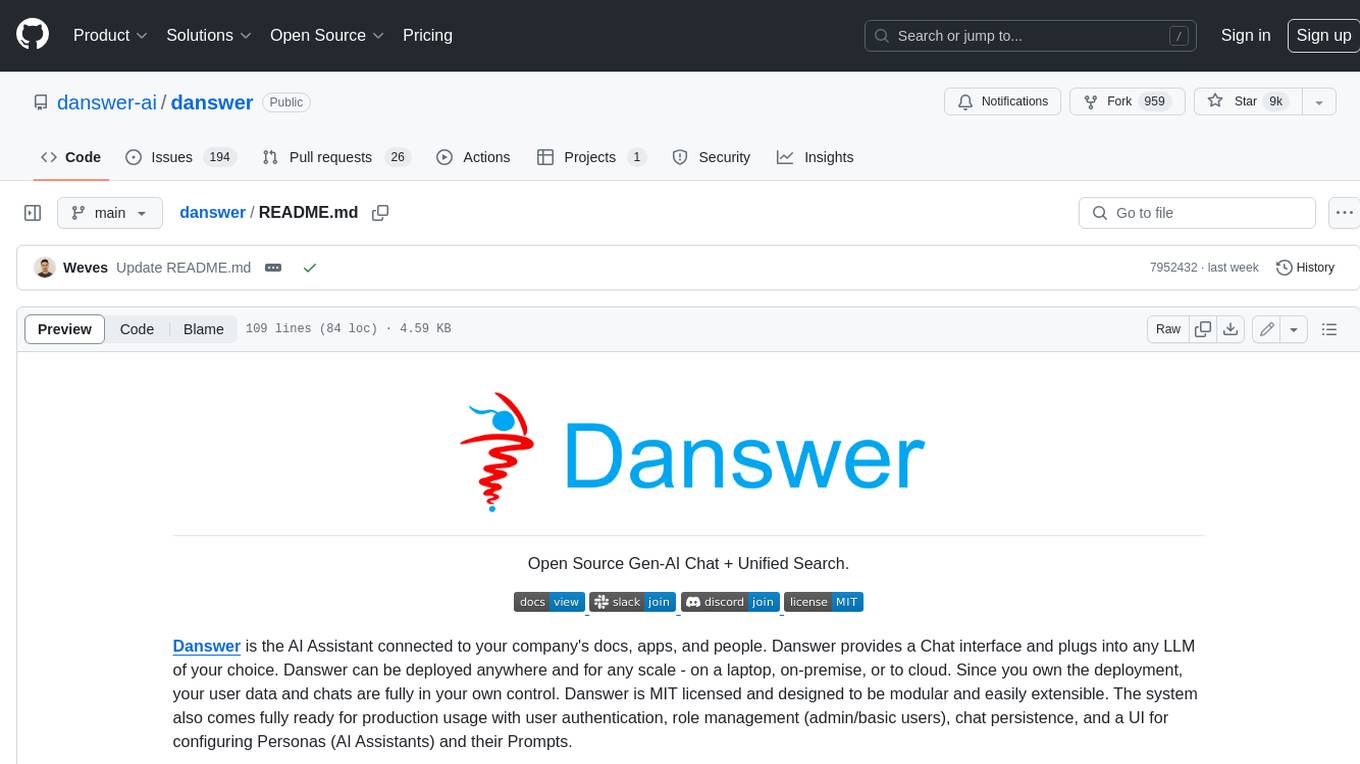
danswer
Danswer is an open-source Gen-AI Chat and Unified Search tool that connects to your company's docs, apps, and people. It provides a Chat interface and plugs into any LLM of your choice. Danswer can be deployed anywhere and for any scale - on a laptop, on-premise, or to cloud. Since you own the deployment, your user data and chats are fully in your own control. Danswer is MIT licensed and designed to be modular and easily extensible. The system also comes fully ready for production usage with user authentication, role management (admin/basic users), chat persistence, and a UI for configuring Personas (AI Assistants) and their Prompts. Danswer also serves as a Unified Search across all common workplace tools such as Slack, Google Drive, Confluence, etc. By combining LLMs and team specific knowledge, Danswer becomes a subject matter expert for the team. Imagine ChatGPT if it had access to your team's unique knowledge! It enables questions such as "A customer wants feature X, is this already supported?" or "Where's the pull request for feature Y?"
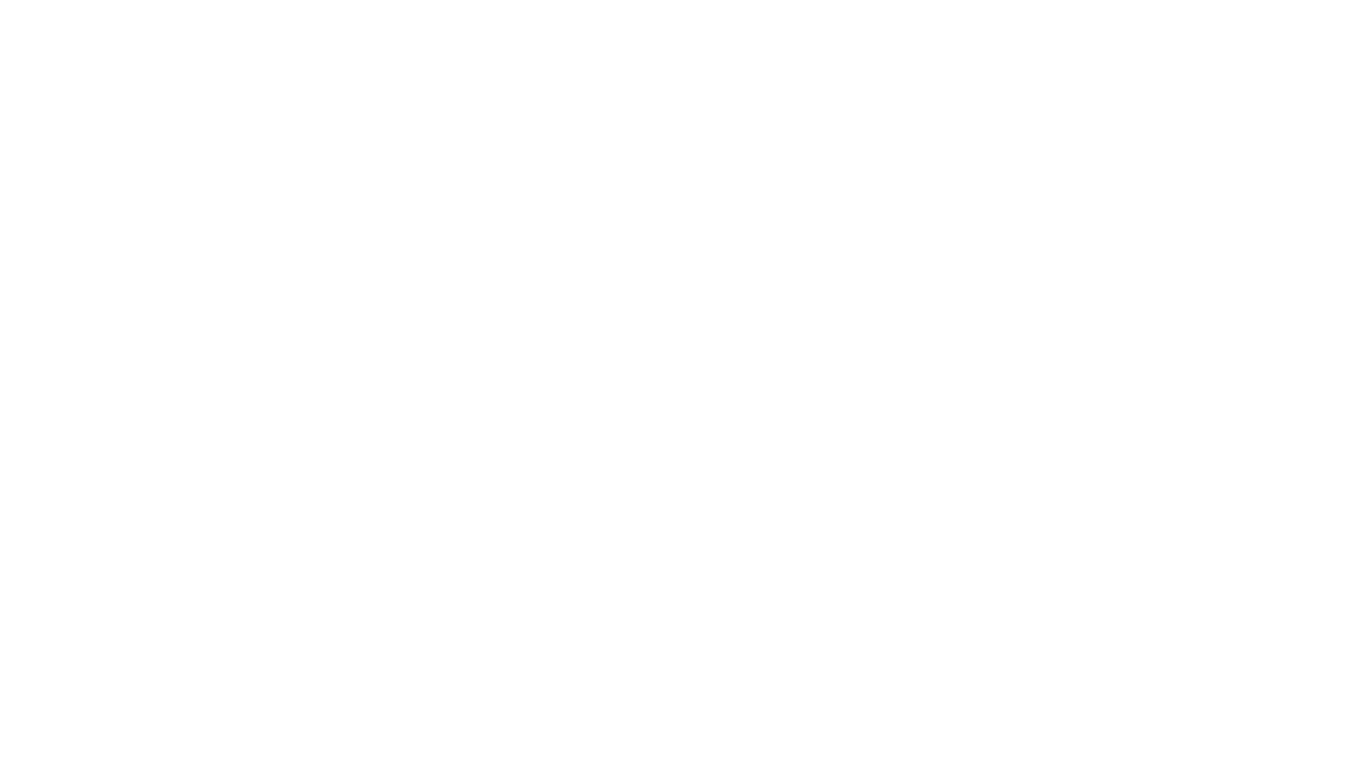
infinity
Infinity is an AI-native database designed for LLM applications, providing incredibly fast full-text and vector search capabilities. It supports a wide range of data types, including vectors, full-text, and structured data, and offers a fused search feature that combines multiple embeddings and full text. Infinity is easy to use, with an intuitive Python API and a single-binary architecture that simplifies deployment. It achieves high performance, with 0.1 milliseconds query latency on million-scale vector datasets and up to 15K QPS.
For similar jobs
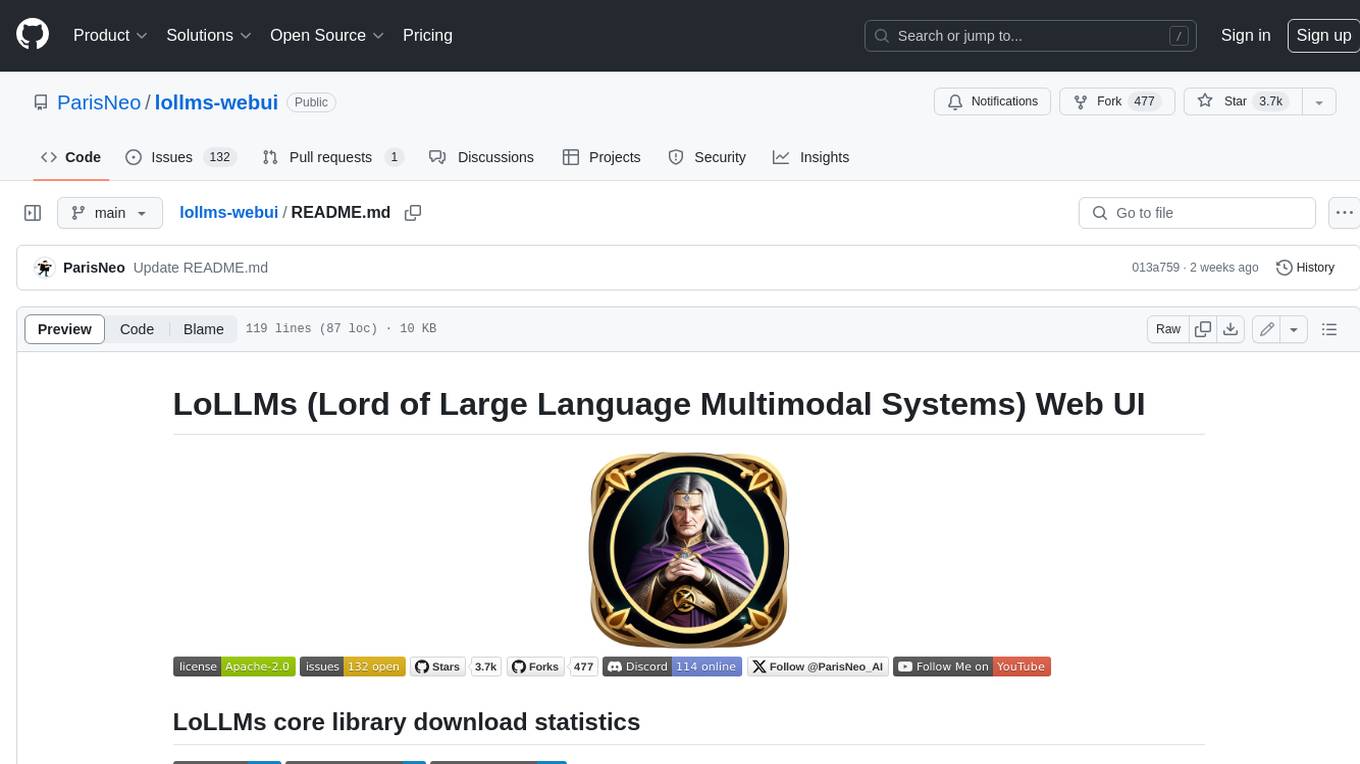
lollms-webui
LoLLMs WebUI (Lord of Large Language Multimodal Systems: One tool to rule them all) is a user-friendly interface to access and utilize various LLM (Large Language Models) and other AI models for a wide range of tasks. With over 500 AI expert conditionings across diverse domains and more than 2500 fine tuned models over multiple domains, LoLLMs WebUI provides an immediate resource for any problem, from car repair to coding assistance, legal matters, medical diagnosis, entertainment, and more. The easy-to-use UI with light and dark mode options, integration with GitHub repository, support for different personalities, and features like thumb up/down rating, copy, edit, and remove messages, local database storage, search, export, and delete multiple discussions, make LoLLMs WebUI a powerful and versatile tool.
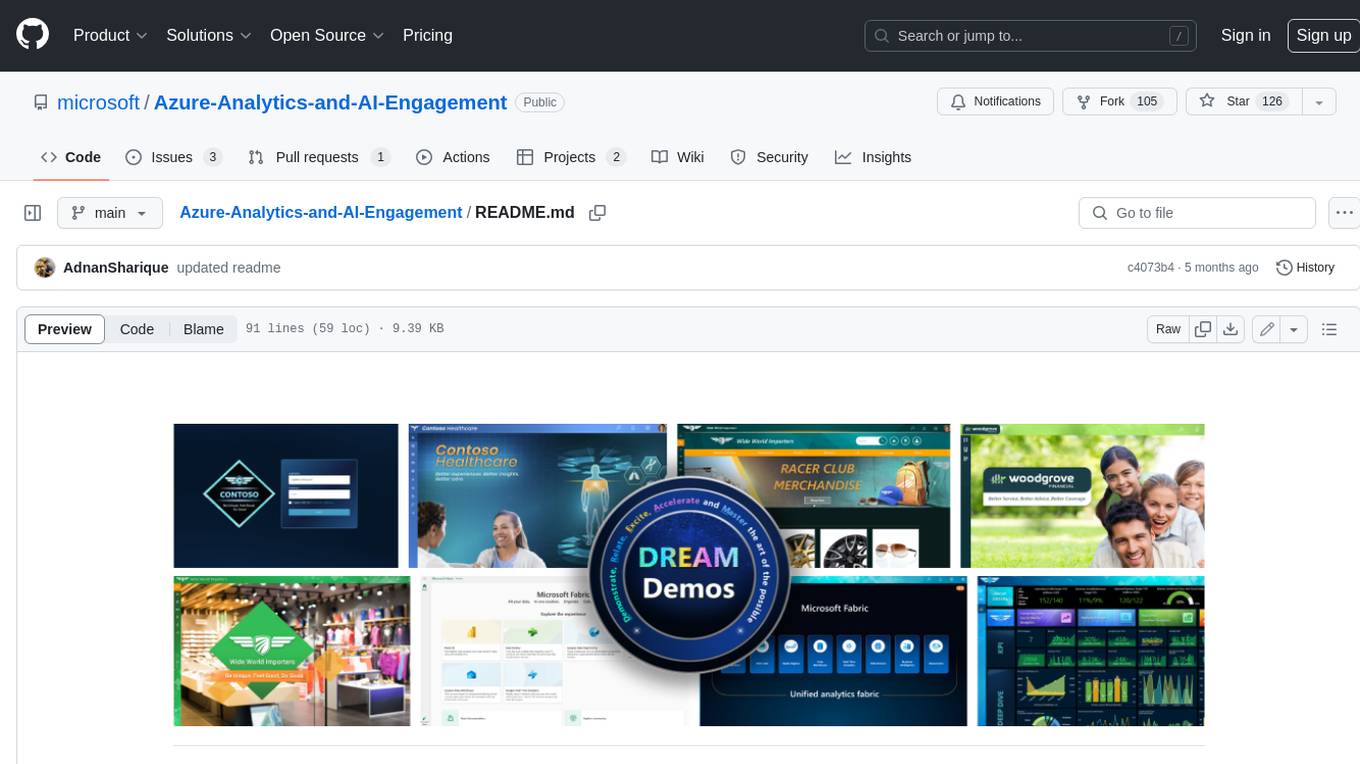
Azure-Analytics-and-AI-Engagement
The Azure-Analytics-and-AI-Engagement repository provides packaged Industry Scenario DREAM Demos with ARM templates (Containing a demo web application, Power BI reports, Synapse resources, AML Notebooks etc.) that can be deployed in a customer’s subscription using the CAPE tool within a matter of few hours. Partners can also deploy DREAM Demos in their own subscriptions using DPoC.
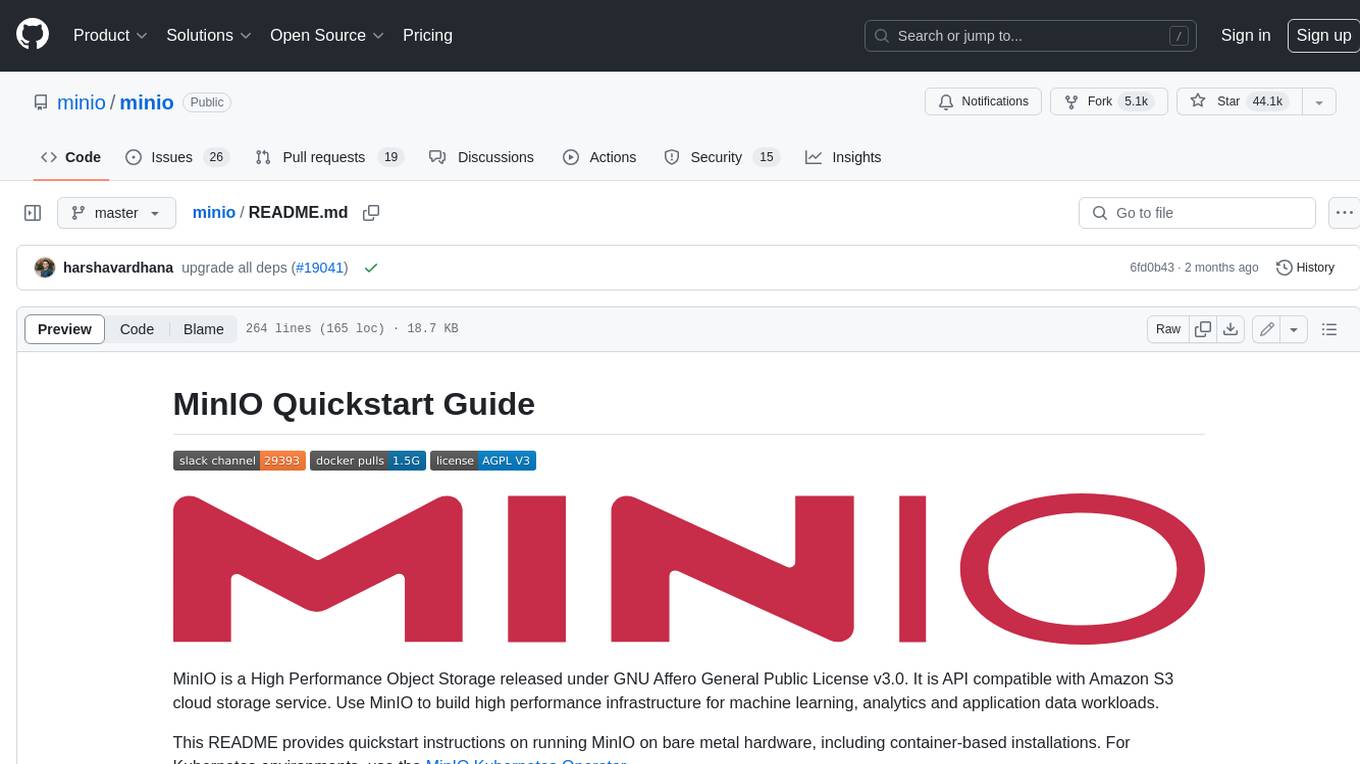
minio
MinIO is a High Performance Object Storage released under GNU Affero General Public License v3.0. It is API compatible with Amazon S3 cloud storage service. Use MinIO to build high performance infrastructure for machine learning, analytics and application data workloads.
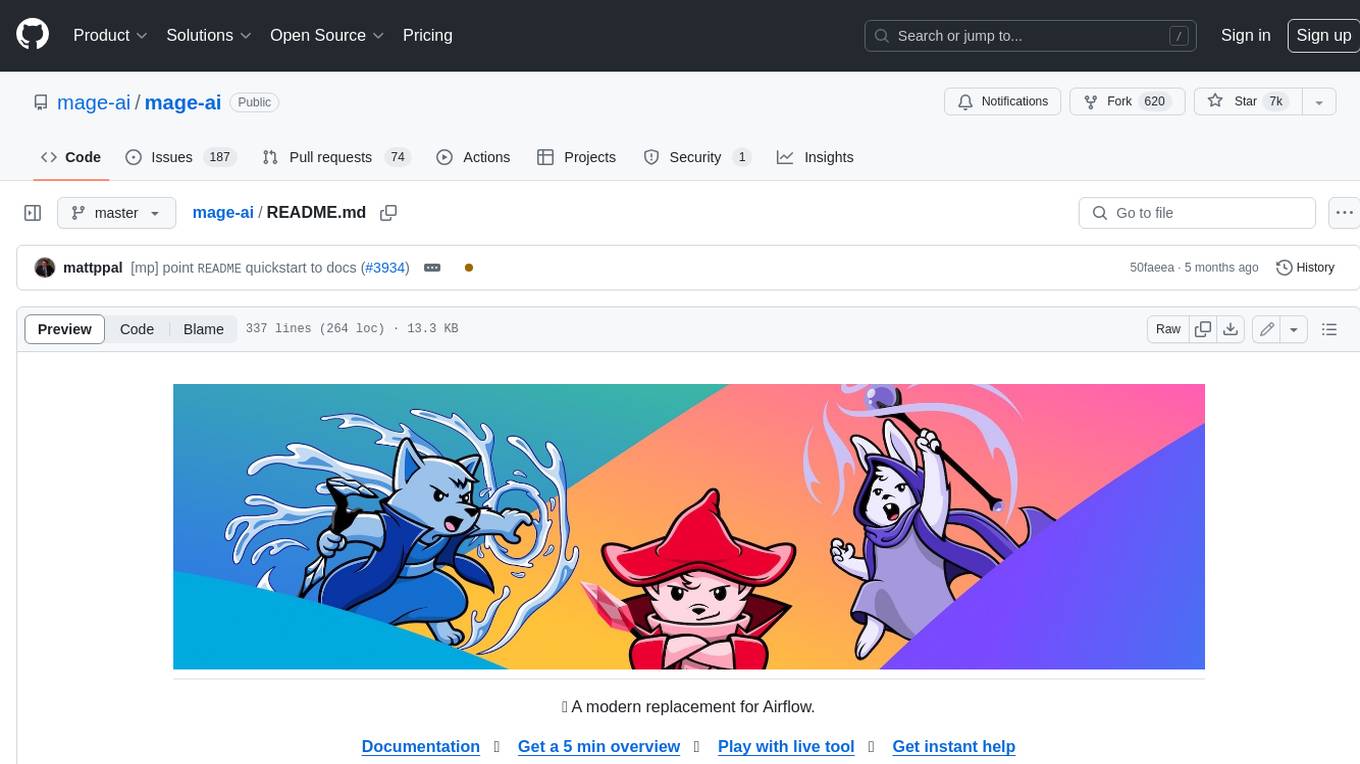
mage-ai
Mage is an open-source data pipeline tool for transforming and integrating data. It offers an easy developer experience, engineering best practices built-in, and data as a first-class citizen. Mage makes it easy to build, preview, and launch data pipelines, and provides observability and scaling capabilities. It supports data integrations, streaming pipelines, and dbt integration.
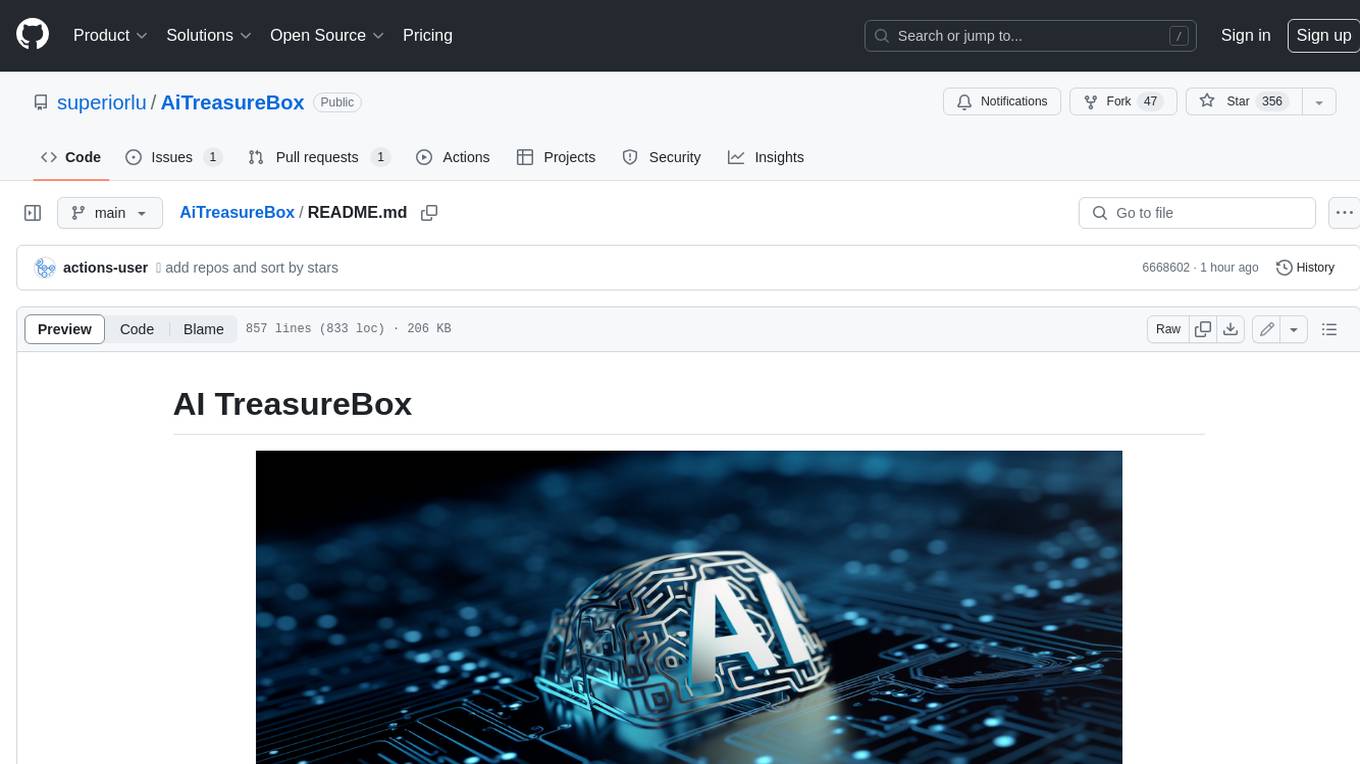
AiTreasureBox
AiTreasureBox is a versatile AI tool that provides a collection of pre-trained models and algorithms for various machine learning tasks. It simplifies the process of implementing AI solutions by offering ready-to-use components that can be easily integrated into projects. With AiTreasureBox, users can quickly prototype and deploy AI applications without the need for extensive knowledge in machine learning or deep learning. The tool covers a wide range of tasks such as image classification, text generation, sentiment analysis, object detection, and more. It is designed to be user-friendly and accessible to both beginners and experienced developers, making AI development more efficient and accessible to a wider audience.
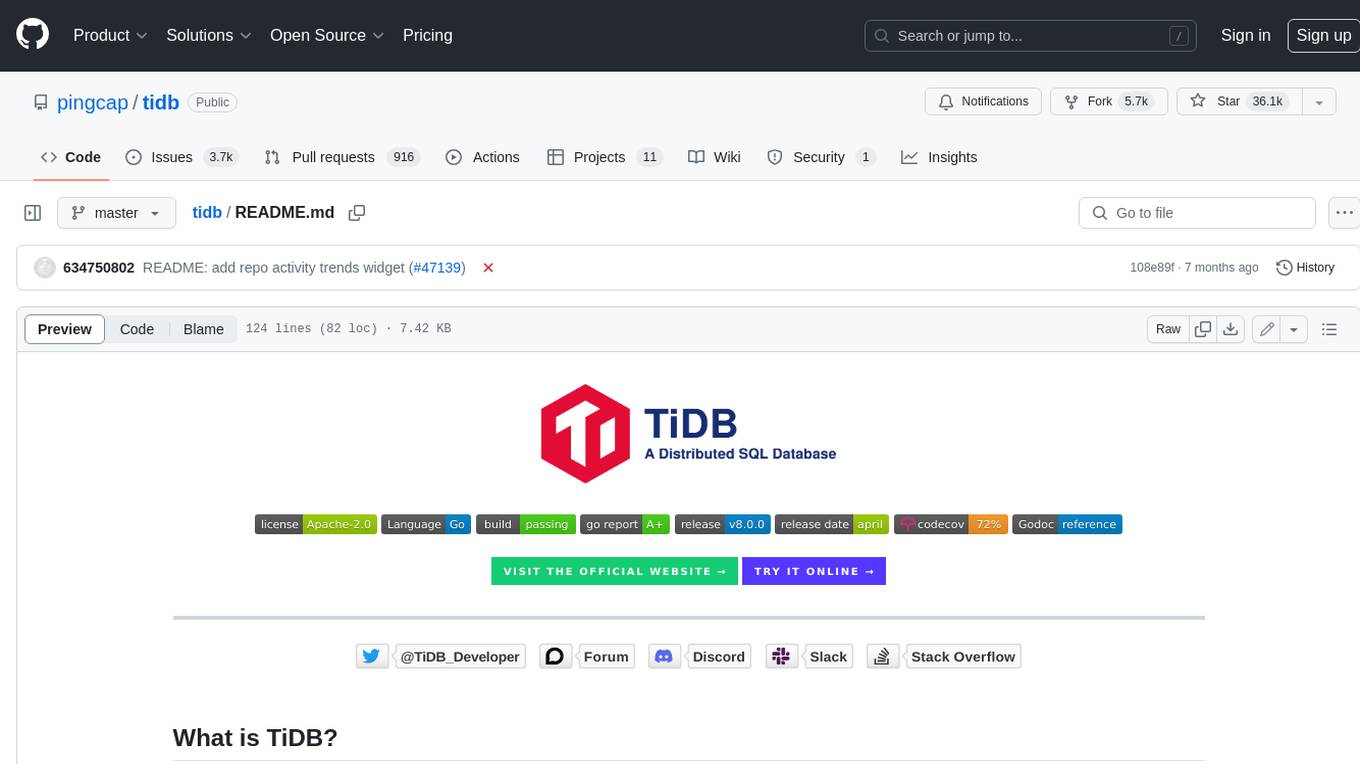
tidb
TiDB is an open-source distributed SQL database that supports Hybrid Transactional and Analytical Processing (HTAP) workloads. It is MySQL compatible and features horizontal scalability, strong consistency, and high availability.
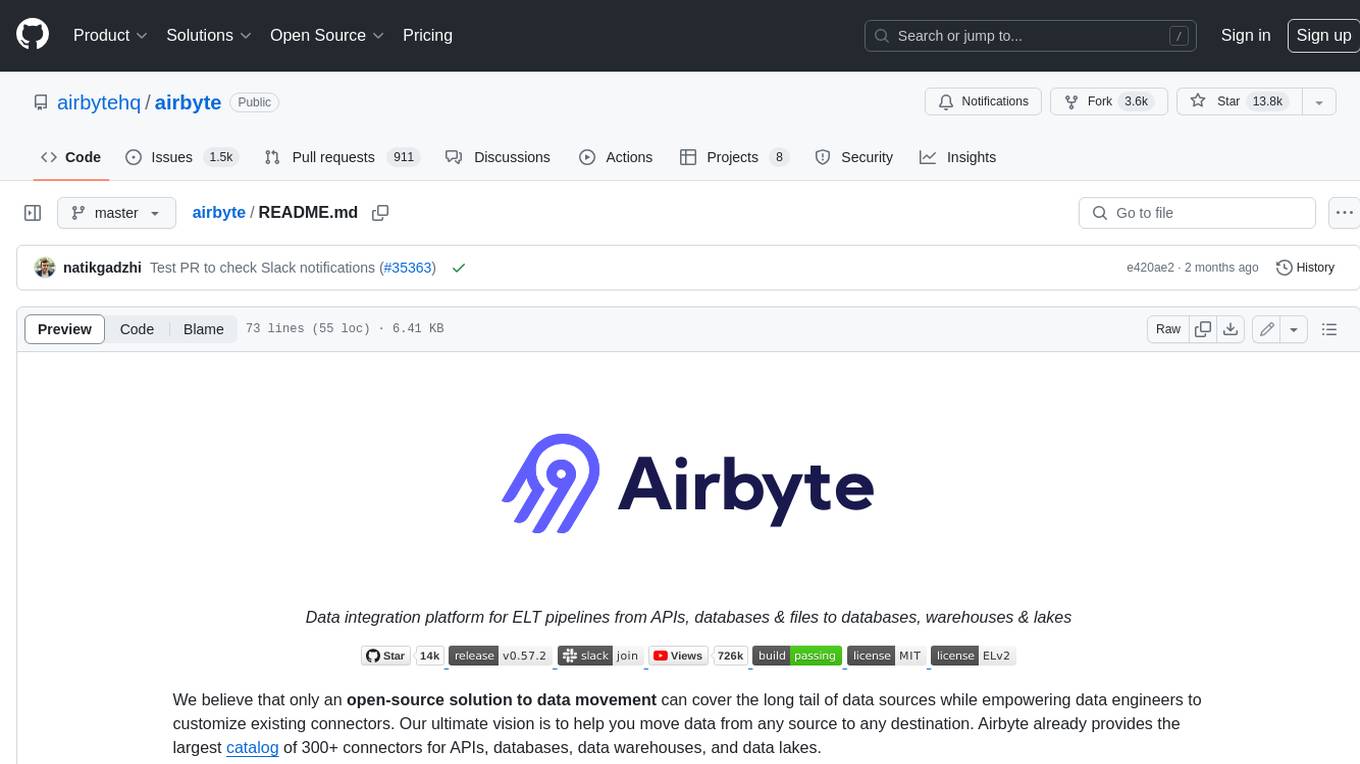
airbyte
Airbyte is an open-source data integration platform that makes it easy to move data from any source to any destination. With Airbyte, you can build and manage data pipelines without writing any code. Airbyte provides a library of pre-built connectors that make it easy to connect to popular data sources and destinations. You can also create your own connectors using Airbyte's no-code Connector Builder or low-code CDK. Airbyte is used by data engineers and analysts at companies of all sizes to build and manage their data pipelines.
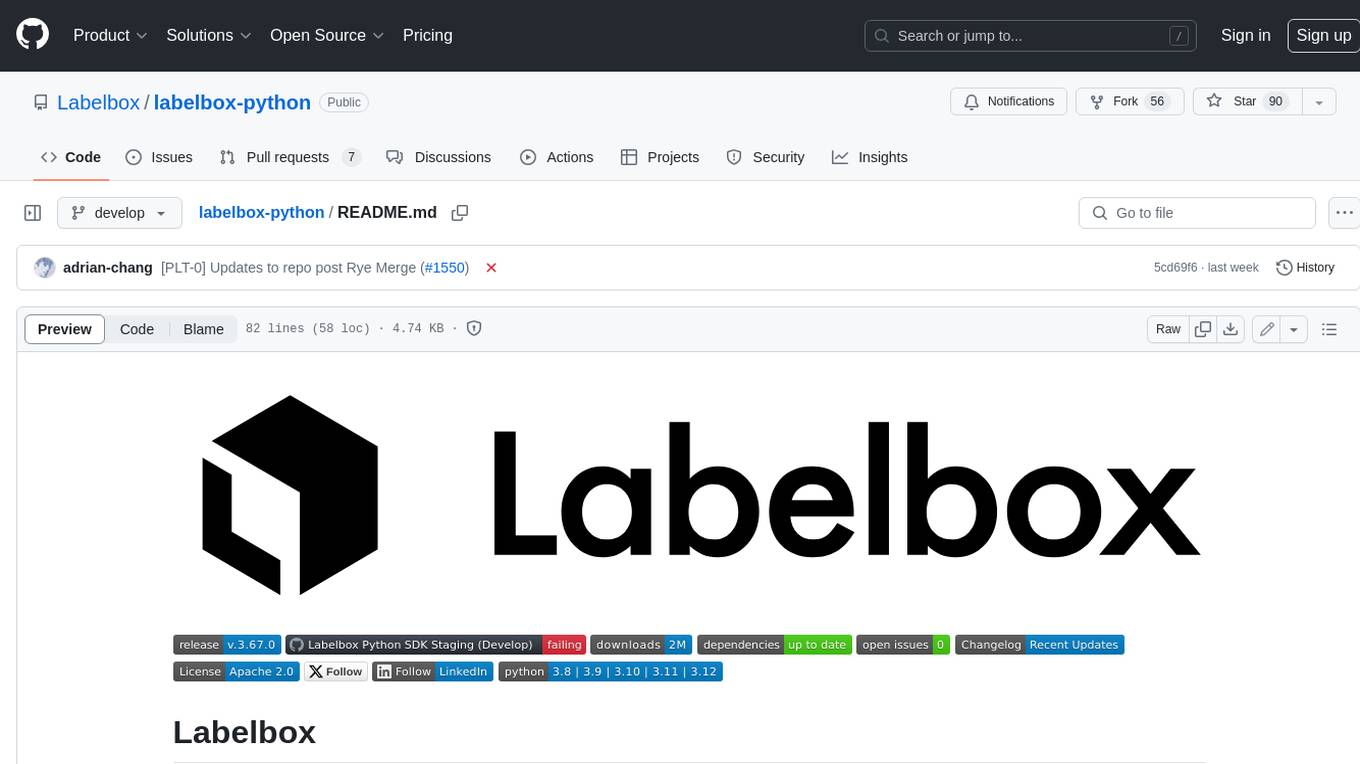
labelbox-python
Labelbox is a data-centric AI platform for enterprises to develop, optimize, and use AI to solve problems and power new products and services. Enterprises use Labelbox to curate data, generate high-quality human feedback data for computer vision and LLMs, evaluate model performance, and automate tasks by combining AI and human-centric workflows. The academic & research community uses Labelbox for cutting-edge AI research.