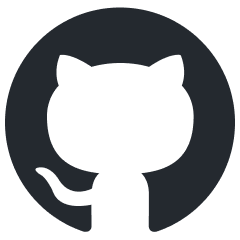
VMind
Not only automatic, but also intelligent. An Intelligent data Visualization System, based on LLM.
Stars: 263
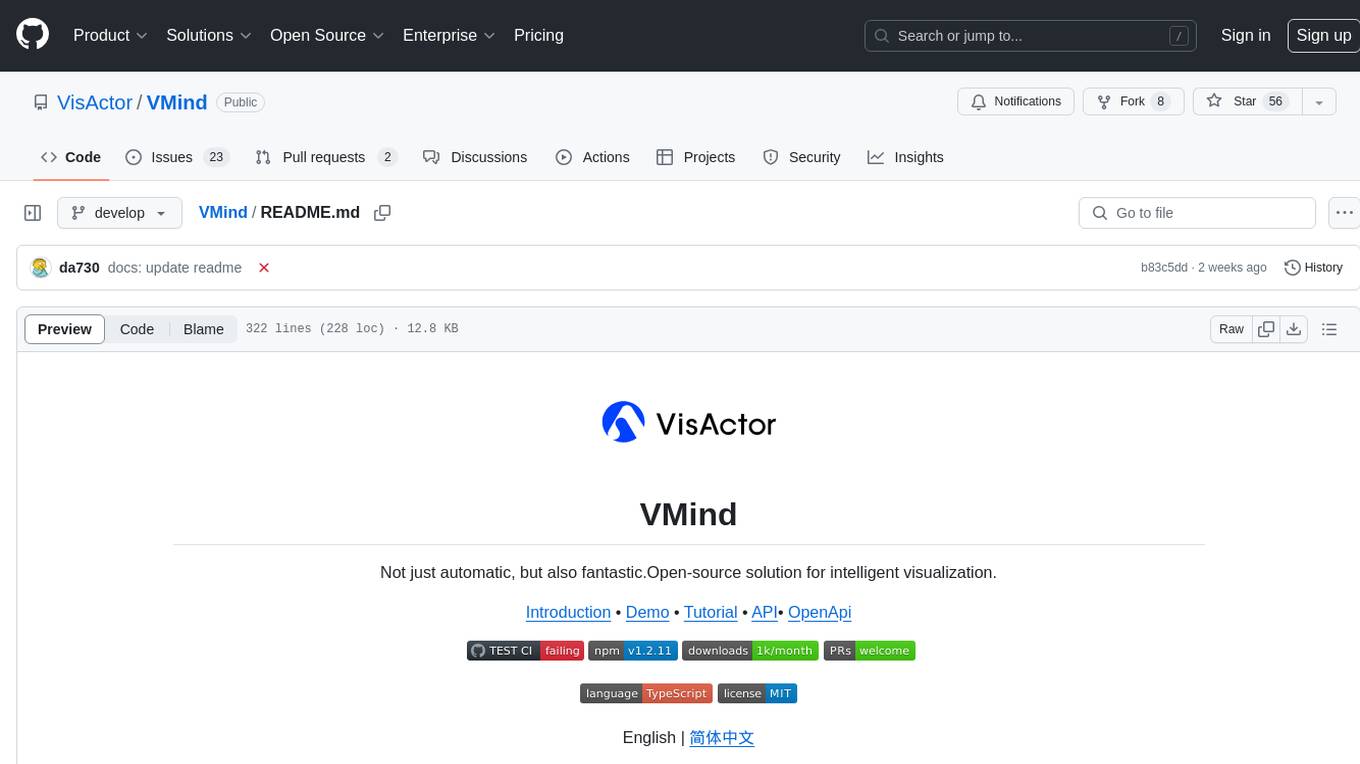
VMind is an open-source solution for intelligent visualization, providing an intelligent chart component based on LLM by VisActor. It allows users to create chart narrative works with natural language interaction, edit charts through dialogue, and export narratives as videos or GIFs. The tool is easy to use, scalable, supports various chart types, and offers one-click export functionality. Users can customize chart styles, specify themes, and aggregate data using LLM models. VMind aims to enhance efficiency in creating data visualization works through dialogue-based editing and natural language interaction.
README:
Not just automatic, but also fantastic.Open-source solution for intelligent visualization.
Introduction • Demo • Tutorial • API• OpenApi
@visactor/vmind
is an intelligent chart component based on LLM provided by VisActor, including dialog-based chart generation and editing capabilities. It provides a natural language interaction interface, allowing you to easily create chart narrative works with @visactor/VMind
with just one sentence, and edit them through continuous dialogue, greatly improving your efficiency in creating data visualization works.
The main features of @visactor/vmind
include:
-
Easy to use: Just provide the data you want to display and a sentence describing the information you want to display, and
@visactor/vmind
will automatically generate the chart for you. Based on the existing chart, describe the modifications you want to make to the chart in one sentence, and@visactor/VMind
will help you achieve the desired effect. -
Strong scalability: The components of
@visactor/VMind
can be easily extended and customized, and new functions and features can be added as needed. By default, the OpenAI GPT model is used, and you can easily replace it with any LLM service. -
Easy narrative: Based on the powerful chart narrative ability of
@visactor/vchart
,@visactor/VMind
supports the generation of various types of charts, including line charts, bar charts, pie charts, etc., and can also generate dynamic bar charts and other dynamic charts, making it easy for you to narrate data. More chart types are being added. You can also use the dialog-based editing function to easily modify chart styles and animation effects, making it easy for you to create narratives. -
One-click export:
@visactor/VMind
comes with a chart export module, and you can export the created chart narrative as a video or GIF for display.
Enter the VMind repository and execute:
# Install dependencies
$ rush update
# Start the demo page
$ rush docs
Enter the VMind repository and execute:
# Install dependencies
$ rush update
# Start the VMind development page
$ rush vmind
You can start the vmind development page. You need to set your LLM service URL and API key to use it normally. You can modify the headers when calling the LLM in packages/vmind/__tests__/browser/src/pages/DataInput.tsx. You can create a new .env.local file in the packages/vmind folder and write in it:
VITE_GPT_URL="Your service url of gpt model"
VITE_GPT_KEY="Your api-key of gpt model"
VITE_DEEPSEEK_URL="https://api.deepseek.com/chat/completions"
VITE_DEEPSEEK_KEY="Your api-key of deepseek model"
VITE_CUSTOM_URL="Your service url of custom model"
VITE_CUSTOM_KEY="Your api-key of custom model"
VITE_CUSTOM_MODEL="Your Custom Model Name"
VITE_PROXY_CONFIG="Your Vite proxy config for forwarding requests. Must be in JSON string format and is optional. Example: {"proxy": {"/v1": {"target": "https://api.openai.com/","changeOrigin": true},"/openapi": {"target": "https://api.openai.com/","changeOrigin": true}}}"
These configurations will be automatically loaded when starting the development environment.
# npm
$ npm install @visactor/vmind
# yarn
$ yarn add @visactor/vmind
First, we need to install VMind in the project:
# Install with npm
npm install @visactor/vmind
# Install with yarn
yarn add @visactor/vmind
Next, import VMind at the top of the JavaScript file
import VMind from '@visactor/vmind';
VMind currently supports GPT, deepseek, doubao and any other models with API Keys. Users can specify the model type to be called when initializing the VMind object, and pass in the URL of the LLM service. Next, we initialize a VMind instance and pass in the model type and model url:
import { Model } from '@visactor/vmind';
const vmind = new VMind({
url: LLM_SERVICE_URL, // URL of the LLM service
model: Model.GPT4o, // Model to use
headers: {
'api-key': LLM_API_KEY
} //headers will be used directly as the request header in the LLM request. You can put the model api key in the header
});
Here is a list of supported models:
//models that VMind support
export enum Model {
GPT3_5 = 'gpt-3.5-turbo',
GPT3_5_1106 = 'gpt-3.5-turbo-1106',
GPT4 = 'gpt-4',
GPT_4_0613 = 'gpt-4-0613',
GPT_4o = 'gpt-4o-2024-08-06',
DOUBAO_LITE = 'doubao-lite-32K',
DOUBAO_PRO = 'doubao-pro-128k',
CHART_ADVISOR = 'chart-advisor',
DEEPSEEK_V3 = 'deepseek-chat',
DEEPSEEK_R1 = 'deepseek-reasoner'
}
And also you can use other model you like:
import { Model } from '@visactor/vmind';
const vmind = new VMind({
url: LLM_SERVICE_URL,
model: LLM_MODEL_NAME, // Model You Choose
headers: {
'api-key': LLM_API_KEY
}
});
VMind supports datasets in both CSV and JSON formats.
To use CSV data in subsequent processes, you need to call the data processing method to extract field information and convert it into a structured dataset. VMind provides a rule-based method parseCSVData
to obtain field information:
// Pass in the CSV string to obtain the fieldInfo and the JSON-structured dataset
const { fieldInfo, dataset } = vmind.parseCSVData(csv);
We can also call the getFieldInfo
method to obtain the fieldInfo by passing in a JSON-formatted dataset:
// Pass in a JSON-formatted dataset to obtain the fieldInfo
const dataset=[
{
"Product name": "Coke",
"region": "south",
"Sales": 2350
},
{
"Product name": "Coke",
"region": "east",
"Sales": 1027
},
{
"Product name": "Coke",
"region": "west",
"Sales": 1027
},
{
"Product name": "Coke",
"region": "north",
"Sales": 1027
}
]
const fieldInfo = vmind.getFieldInfo(dataset);
We want to show "the changes in sales rankings of various car brands". Call the generateChart method and pass the data and display content description directly to VMind:
const userPrompt = 'show me the changes in sales rankings of various car brand';
//Call the chart generation interface to get spec and chart animation duration
const { spec, time } = await vmind.generateChart(userPrompt, fieldInfo, dataset);
In this way, we get the VChart spec of the corresponding dynamic chart. We can render the chart based on this spec:
import VChart from '@visactor/vchart';
<body>
<!-- Prepare a DOM with size (width and height) for vchart, of course you can also specify it in the spec configuration -->
<div id="chart" style="width: 600px;height:400px;"></div>
</body>
// Create a vchart instance
const vchart = new VChart(spec, { dom: 'chart' });
// Draw
vchart.renderAsync();
Thanks to the capabilities of the large language model, users can describe more requirements in natural language and "customize" dishes. Users can specify different theme styles (currently only gpt chart generation supports this feature). For example, users can specify to generate a tech-style chart:
//userPrompt can be in both Chinese and English
//Specify to generate a tech-style chart
const userPrompt = 'show me the changes in sales rankings of various car brand,tech style';
const { spec, time } = await vmind.generateChart(userPrompt, fieldInfo, dataset);
You can also specify the chart type, field mapping, etc. supported by VMind. For example:
//Specify to generate a line chart, with car manufacturers as the x-axis
const userPrompt =
'show me the changes in sales rankings of various car brands,tech style.Using a line chart, Manufacturer makes the x-axis';
const { spec, time } = await vmind.generateChart(userPrompt, fieldInfo, dataset);
When using the chart library to draw bar charts, line charts, etc., if the data is not aggregated, it will affect the visualization effect. At the same time, because no filtering and sorting of fields has been done, some visualization intentions cannot be met, for example: show me the top 10 departments with the most cost, show me the sales of various products in the north, etc.
VMind supports intelligent data aggregation since version 1.2.2. This function uses the data input by the user as a data table, uses a LLM to generate SQL queries according to the user's command, queries data from the data table, and uses GROUP BY and SQL aggregation methods to group, aggregate, sort, and filter data. Supported SQL statements include: SELECT, GROUP BY, WHERE, HAVING, ORDER BY, LIMIT. Supported aggregation methods are: MAX(), MIN(), SUM(), COUNT(), AVG(). Complex SQL operations such as subqueries, JOIN, and conditional statements are not supported.
Use the dataQuery
function of the VMind object to aggregate data. This method has three parameters:
- userInput: user input. You can use the same input as generateChart
- fieldInfo: Dataset field information. The same as generateChart, it can be obtained by parseCSVData, or built by the user.
- dataset: Dataset. The same as generateChart, it can be obtained by parseCSVData, or built by the user.
const { fieldInfo, dataset } = await vmind?.dataQuery(userInput, fieldInfo, dataset);
The fieldInfo and dataset returned by this method are the field information and dataset after data aggregation, which can be used for chart generation.
By default, the generateChart
function will perform a data aggregation using the same user input before generating the chart. You can disable data aggregation by passing in the fourth parameter:
const userInput = 'show me the changes in sales rankings of various car brand';
const { spec, time } = await vmind.generateChart(userInput, fieldInfo, dataset, false); //pass false as the forth parameter to disable data aggregation before generating a chart.
Under development, stay tuned
If you would like to contribute, please read the Code of Conduct and Guide first。
Small streams converge to make great rivers and seas!
For Tasks:
Click tags to check more tools for each tasksFor Jobs:
Alternative AI tools for VMind
Similar Open Source Tools
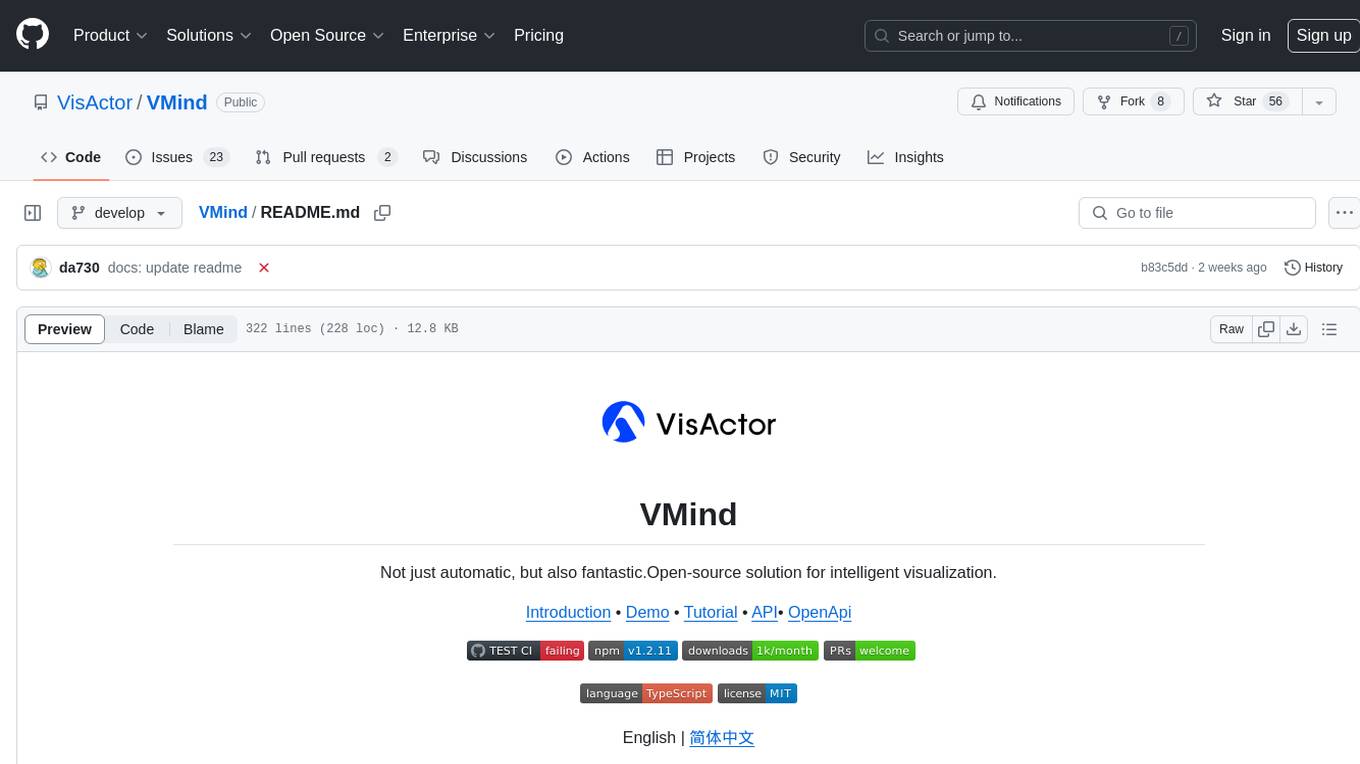
VMind
VMind is an open-source solution for intelligent visualization, providing an intelligent chart component based on LLM by VisActor. It allows users to create chart narrative works with natural language interaction, edit charts through dialogue, and export narratives as videos or GIFs. The tool is easy to use, scalable, supports various chart types, and offers one-click export functionality. Users can customize chart styles, specify themes, and aggregate data using LLM models. VMind aims to enhance efficiency in creating data visualization works through dialogue-based editing and natural language interaction.
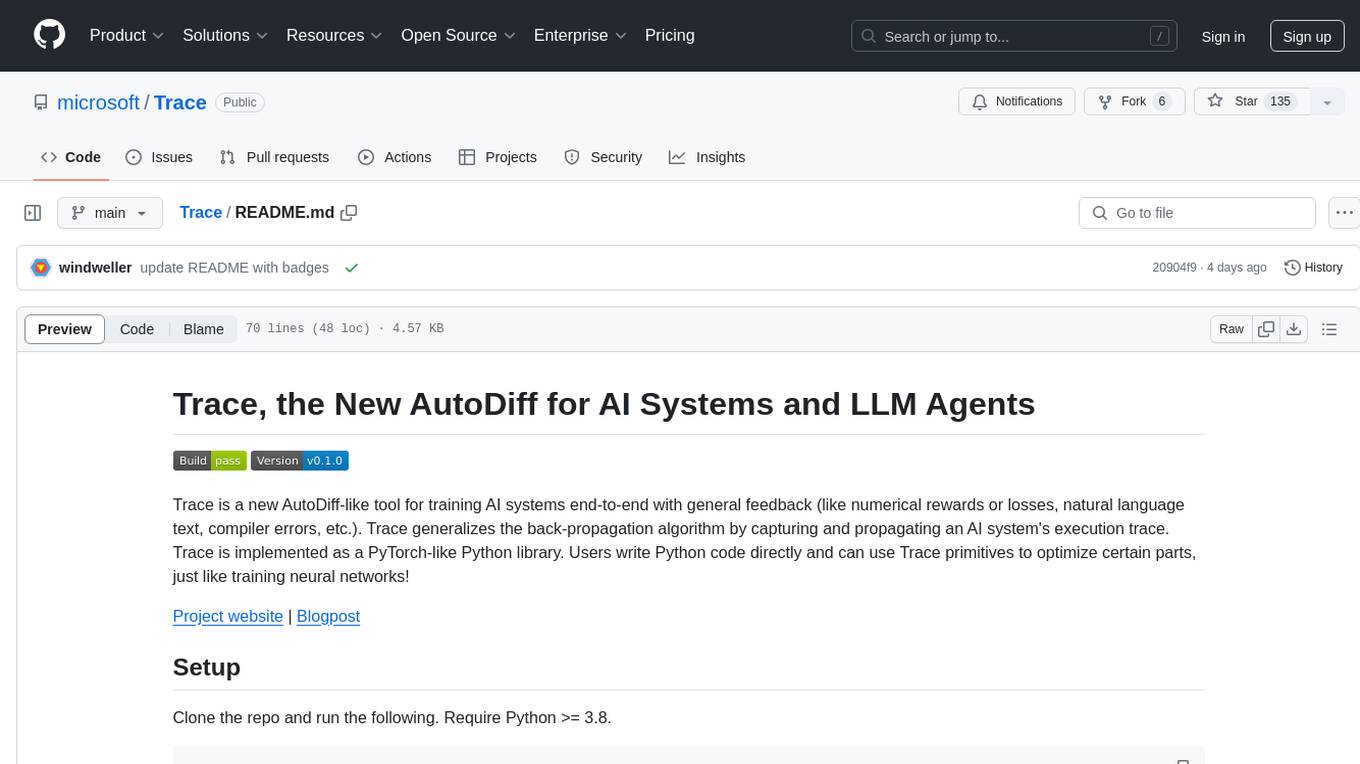
Trace
Trace is a new AutoDiff-like tool for training AI systems end-to-end with general feedback. It generalizes the back-propagation algorithm by capturing and propagating an AI system's execution trace. Implemented as a PyTorch-like Python library, users can write Python code directly and use Trace primitives to optimize certain parts, similar to training neural networks.
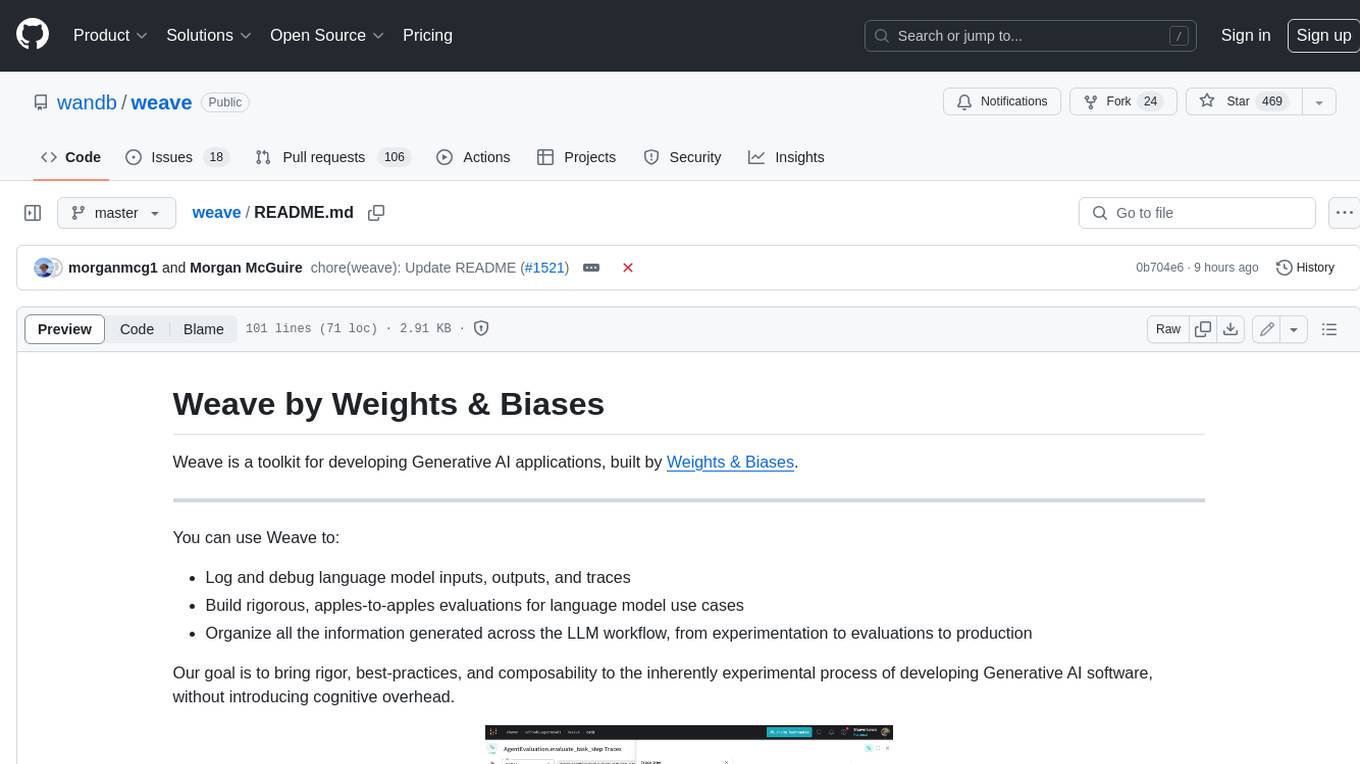
weave
Weave is a toolkit for developing Generative AI applications, built by Weights & Biases. With Weave, you can log and debug language model inputs, outputs, and traces; build rigorous, apples-to-apples evaluations for language model use cases; and organize all the information generated across the LLM workflow, from experimentation to evaluations to production. Weave aims to bring rigor, best-practices, and composability to the inherently experimental process of developing Generative AI software, without introducing cognitive overhead.
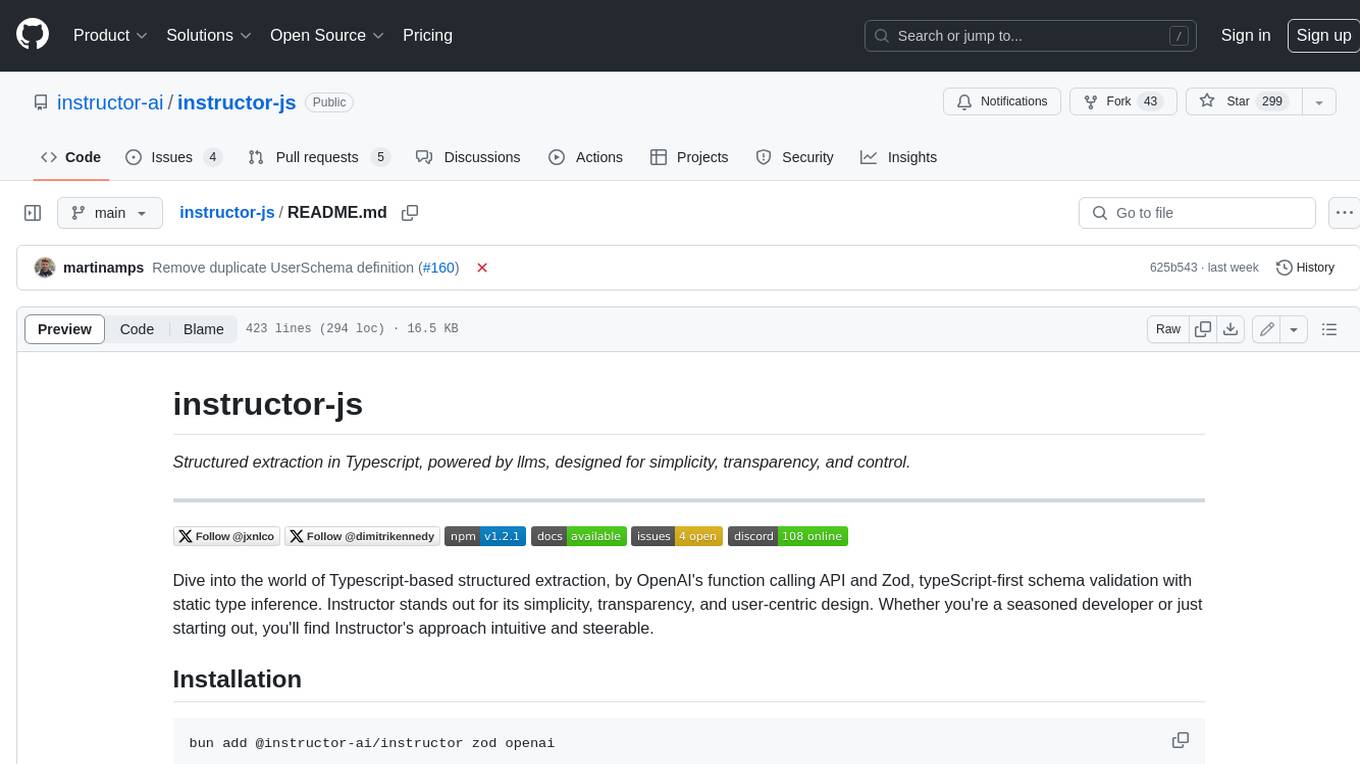
instructor-js
Instructor is a Typescript library for structured extraction in Typescript, powered by llms, designed for simplicity, transparency, and control. It stands out for its simplicity, transparency, and user-centric design. Whether you're a seasoned developer or just starting out, you'll find Instructor's approach intuitive and steerable.
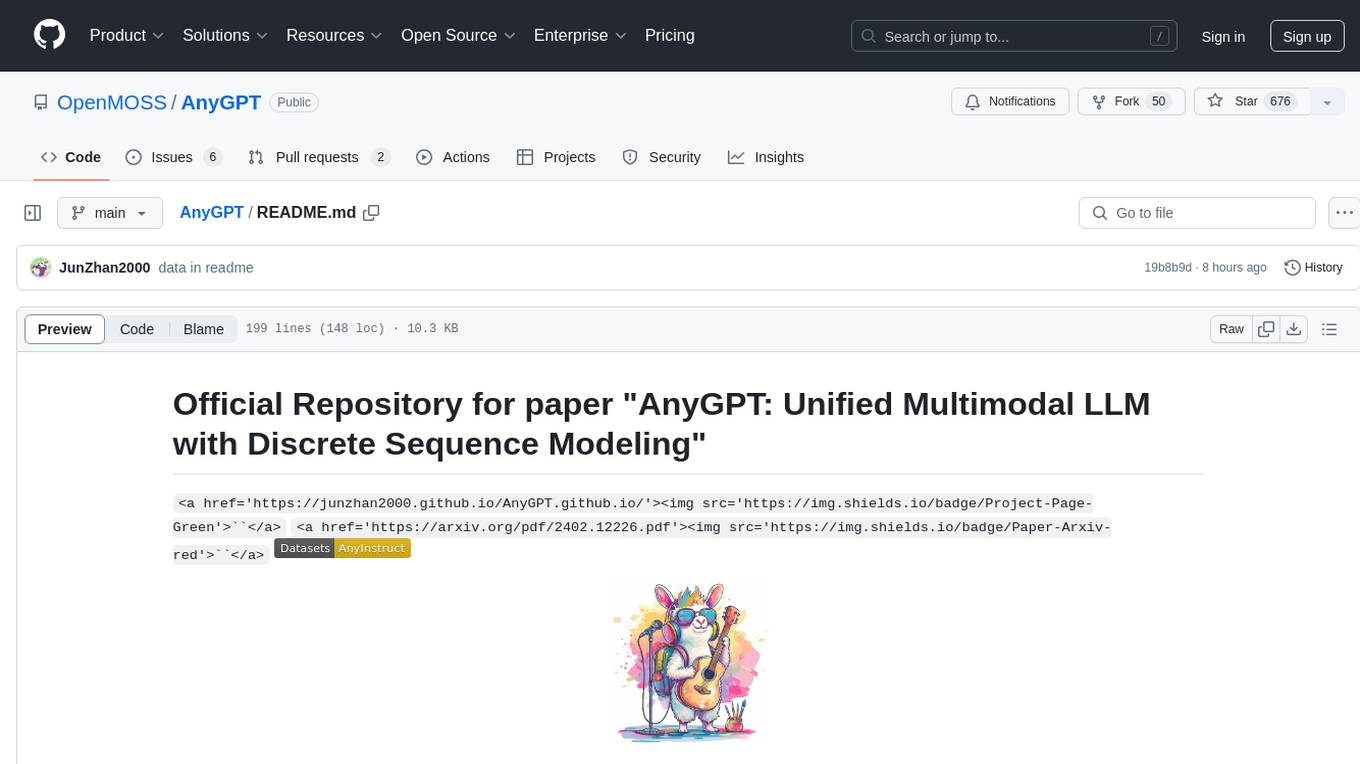
AnyGPT
AnyGPT is a unified multimodal language model that utilizes discrete representations for processing various modalities like speech, text, images, and music. It aligns the modalities for intermodal conversions and text processing. AnyInstruct dataset is constructed for generative models. The model proposes a generative training scheme using Next Token Prediction task for training on a Large Language Model (LLM). It aims to compress vast multimodal data on the internet into a single model for emerging capabilities. The tool supports tasks like text-to-image, image captioning, ASR, TTS, text-to-music, and music captioning.
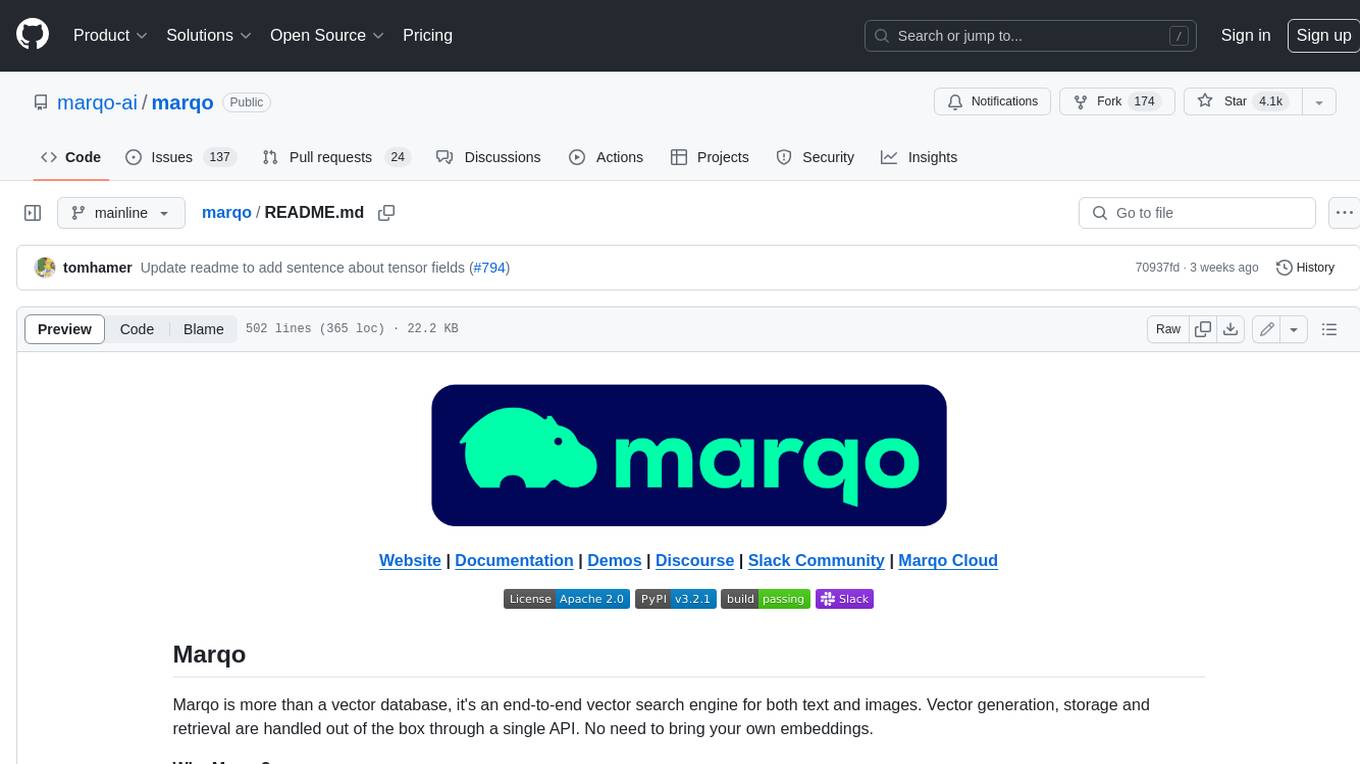
marqo
Marqo is more than a vector database, it's an end-to-end vector search engine for both text and images. Vector generation, storage and retrieval are handled out of the box through a single API. No need to bring your own embeddings.
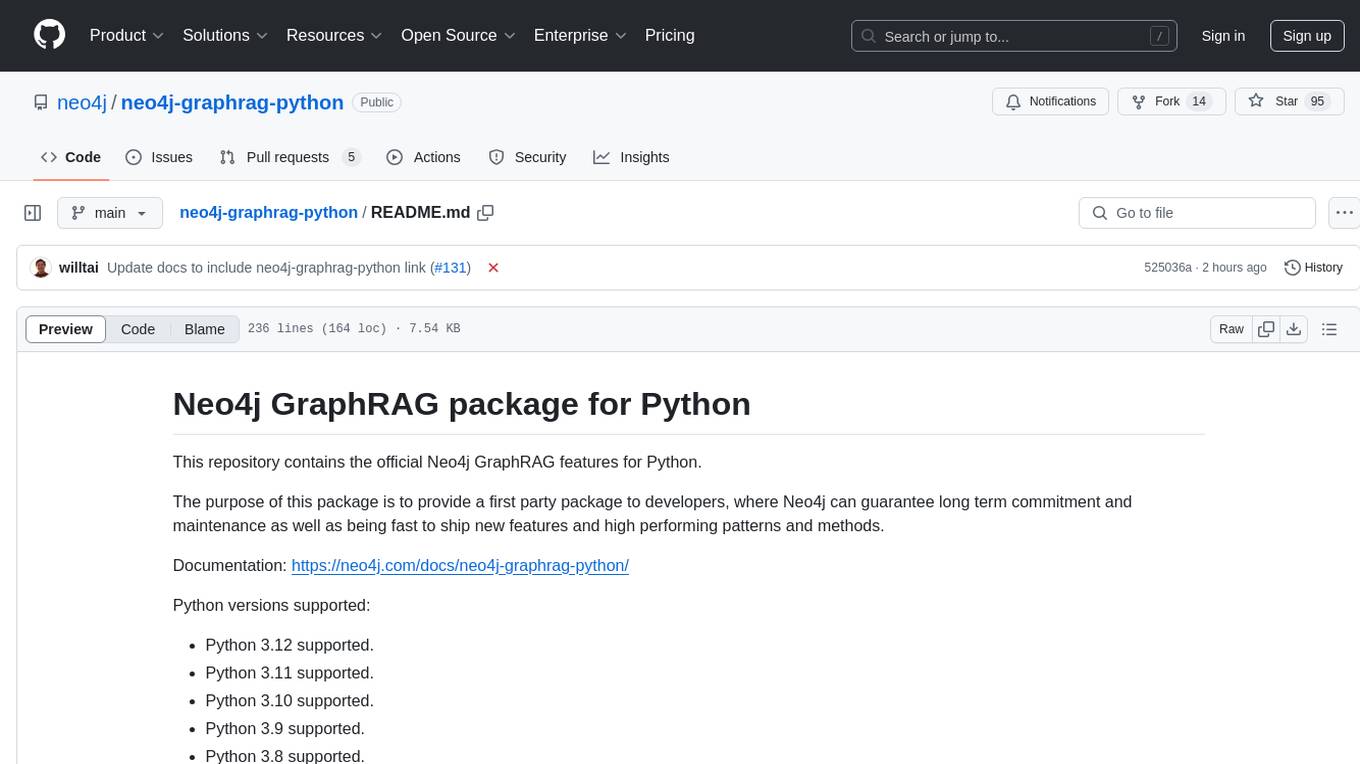
neo4j-graphrag-python
The Neo4j GraphRAG package for Python is an official repository that provides features for creating and managing vector indexes in Neo4j databases. It aims to offer developers a reliable package with long-term commitment, maintenance, and fast feature updates. The package supports various Python versions and includes functionalities for creating vector indexes, populating them, and performing similarity searches. It also provides guidelines for installation, examples, and development processes such as installing dependencies, making changes, and running tests.
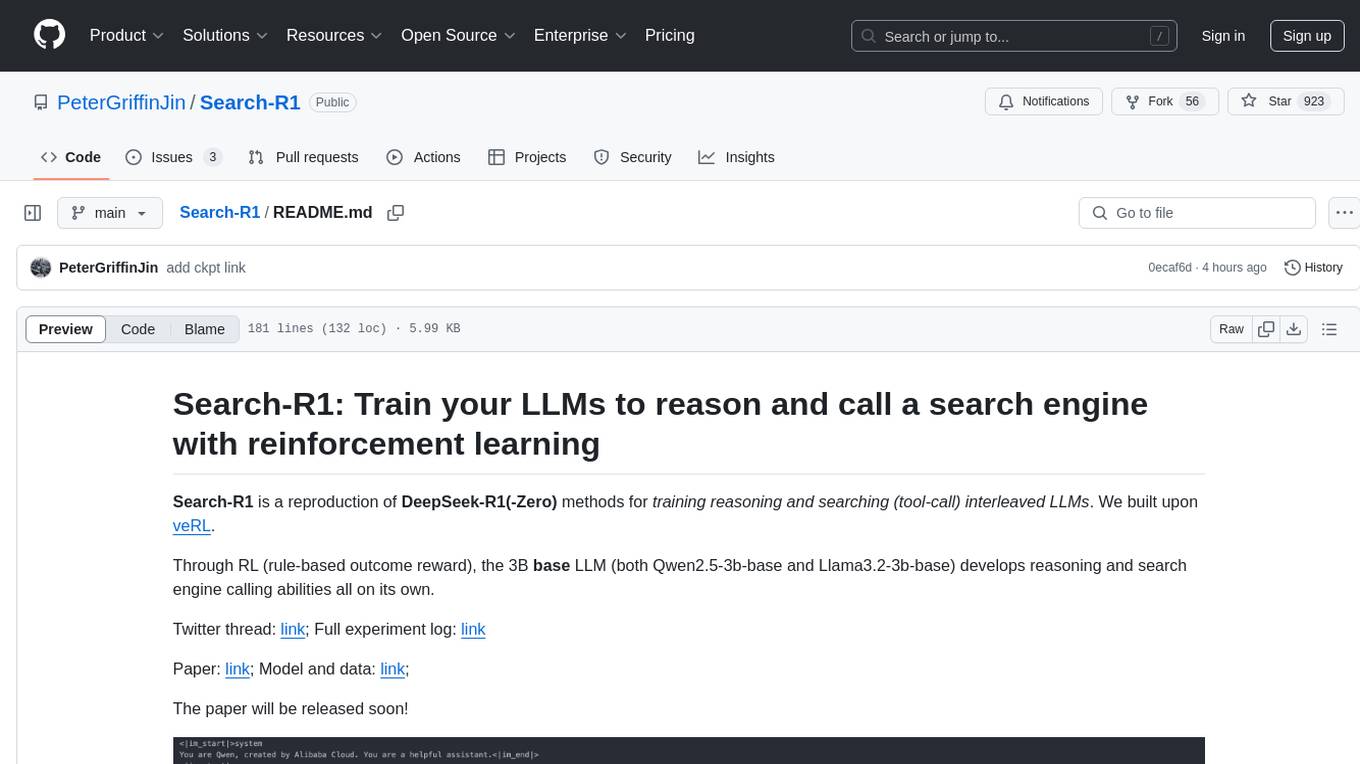
Search-R1
Search-R1 is a tool that trains large language models (LLMs) to reason and call a search engine using reinforcement learning. It is a reproduction of DeepSeek-R1 methods for training reasoning and searching interleaved LLMs, built upon veRL. Through rule-based outcome reward, the base LLM develops reasoning and search engine calling abilities independently. Users can train LLMs on their own datasets and search engines, with preliminary results showing improved performance in search engine calling and reasoning tasks.
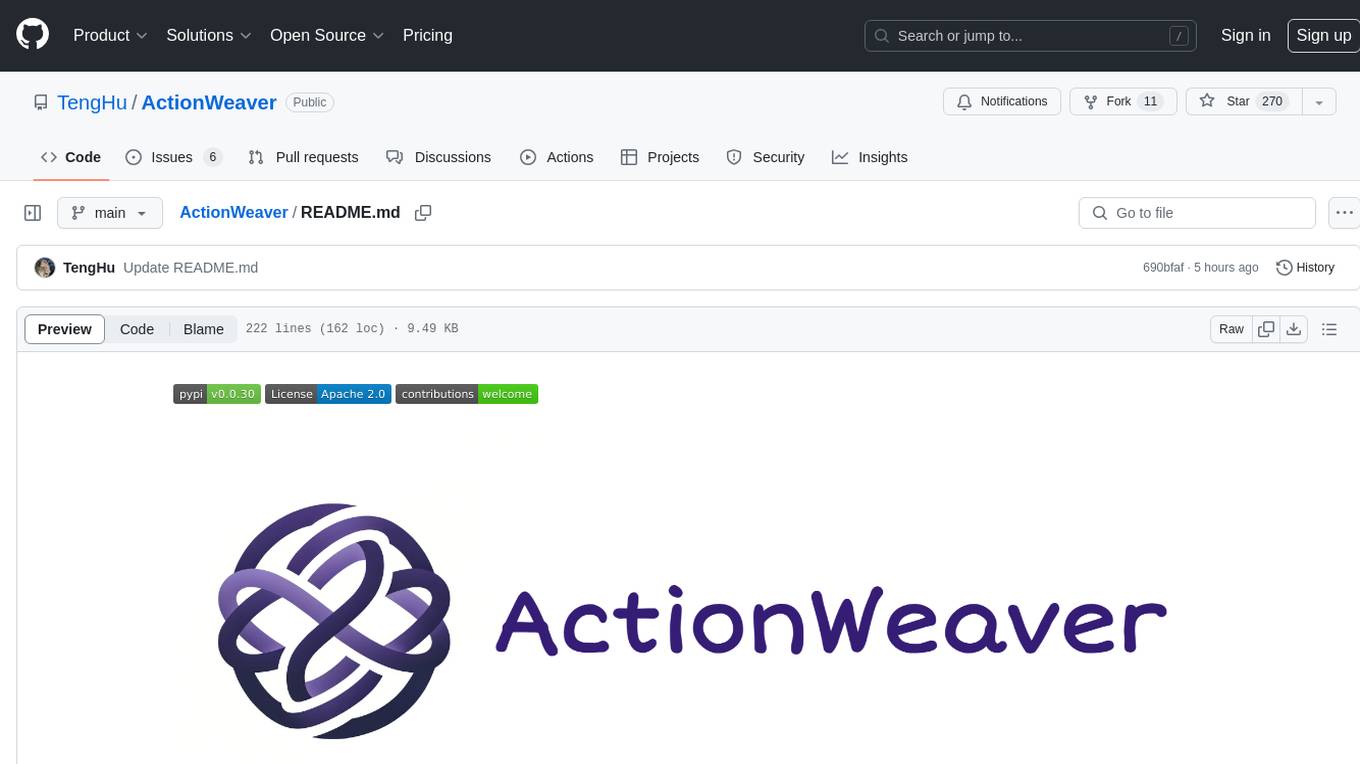
ActionWeaver
ActionWeaver is an AI application framework designed for simplicity, relying on OpenAI and Pydantic. It supports both OpenAI API and Azure OpenAI service. The framework allows for function calling as a core feature, extensibility to integrate any Python code, function orchestration for building complex call hierarchies, and telemetry and observability integration. Users can easily install ActionWeaver using pip and leverage its capabilities to create, invoke, and orchestrate actions with the language model. The framework also provides structured extraction using Pydantic models and allows for exception handling customization. Contributions to the project are welcome, and users are encouraged to cite ActionWeaver if found useful.
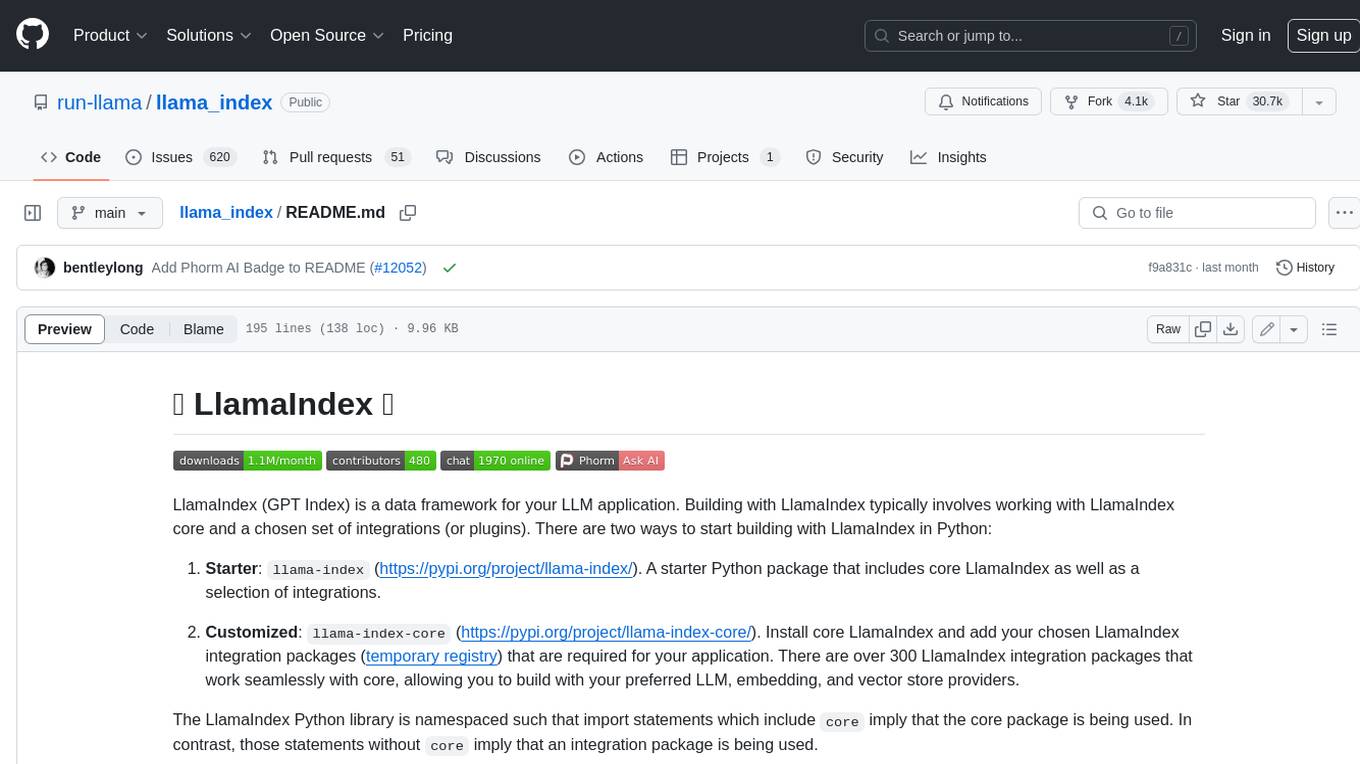
llama_index
LlamaIndex is a data framework for building LLM applications. It provides tools for ingesting, structuring, and querying data, as well as integrating with LLMs and other tools. LlamaIndex is designed to be easy to use for both beginner and advanced users, and it provides a comprehensive set of features for building LLM applications.
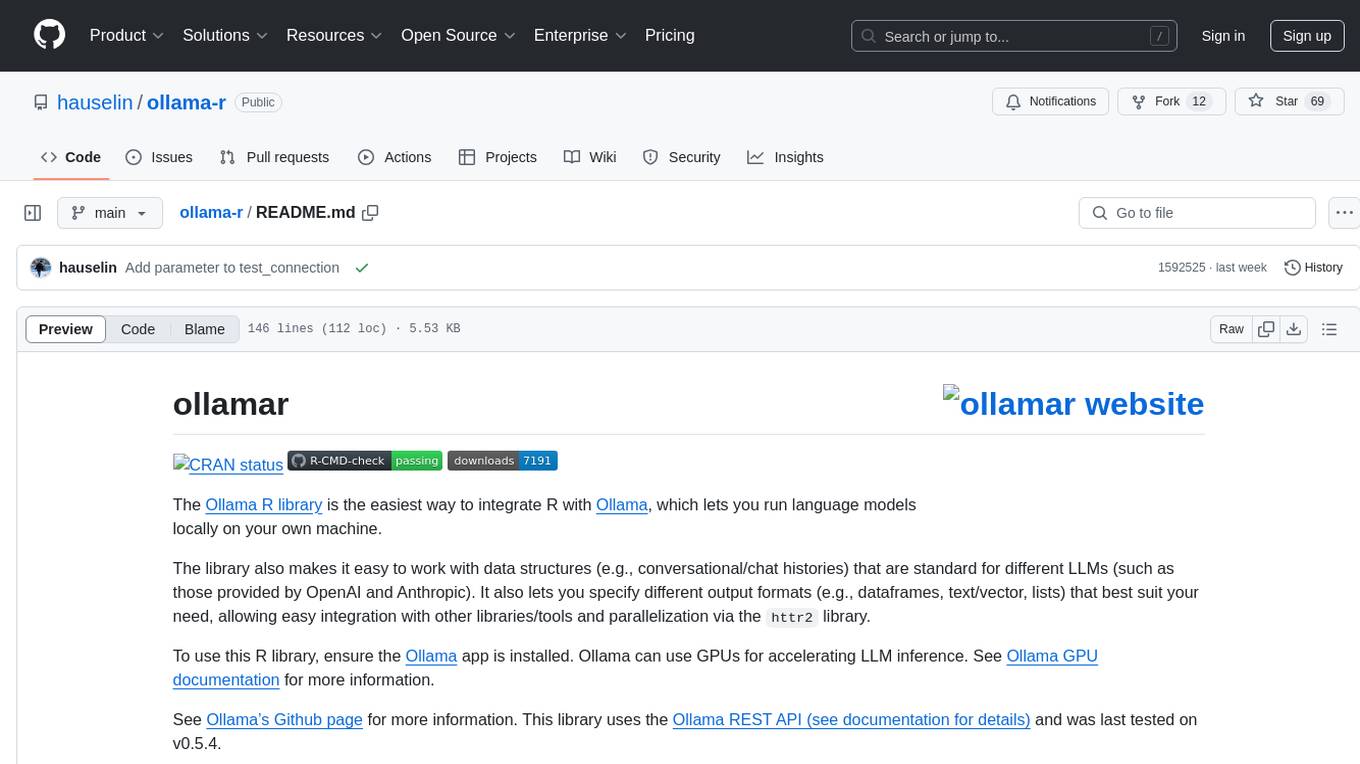
ollama-r
The Ollama R library provides an easy way to integrate R with Ollama for running language models locally on your machine. It supports working with standard data structures for different LLMs, offers various output formats, and enables integration with other libraries/tools. The library uses the Ollama REST API and requires the Ollama app to be installed, with GPU support for accelerating LLM inference. It is inspired by Ollama Python and JavaScript libraries, making it familiar for users of those languages. The installation process involves downloading the Ollama app, installing the 'ollamar' package, and starting the local server. Example usage includes testing connection, downloading models, generating responses, and listing available models.
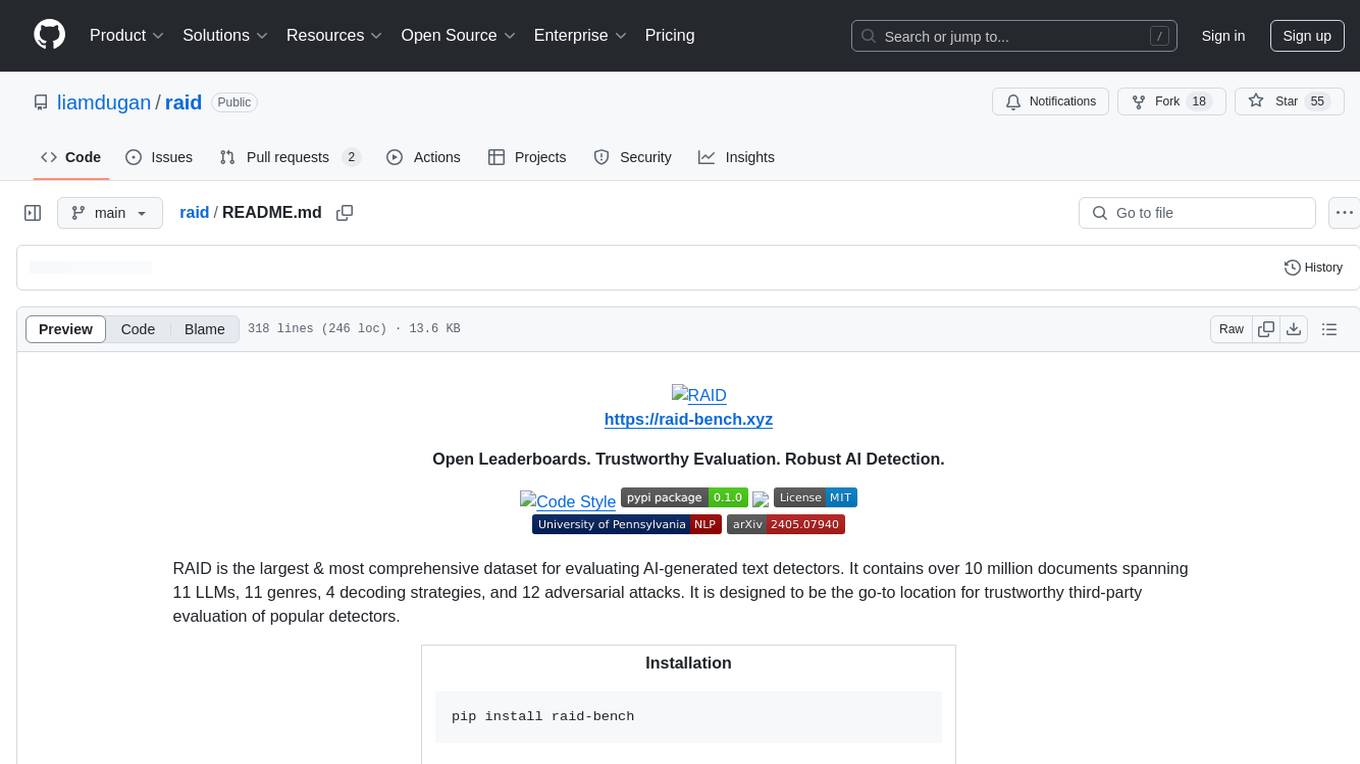
raid
RAID is the largest and most comprehensive dataset for evaluating AI-generated text detectors. It contains over 10 million documents spanning 11 LLMs, 11 genres, 4 decoding strategies, and 12 adversarial attacks. RAID is designed to be the go-to location for trustworthy third-party evaluation of popular detectors. The dataset covers diverse models, domains, sampling strategies, and attacks, making it a valuable resource for training detectors, evaluating generalization, protecting against adversaries, and comparing to state-of-the-art models from academia and industry.
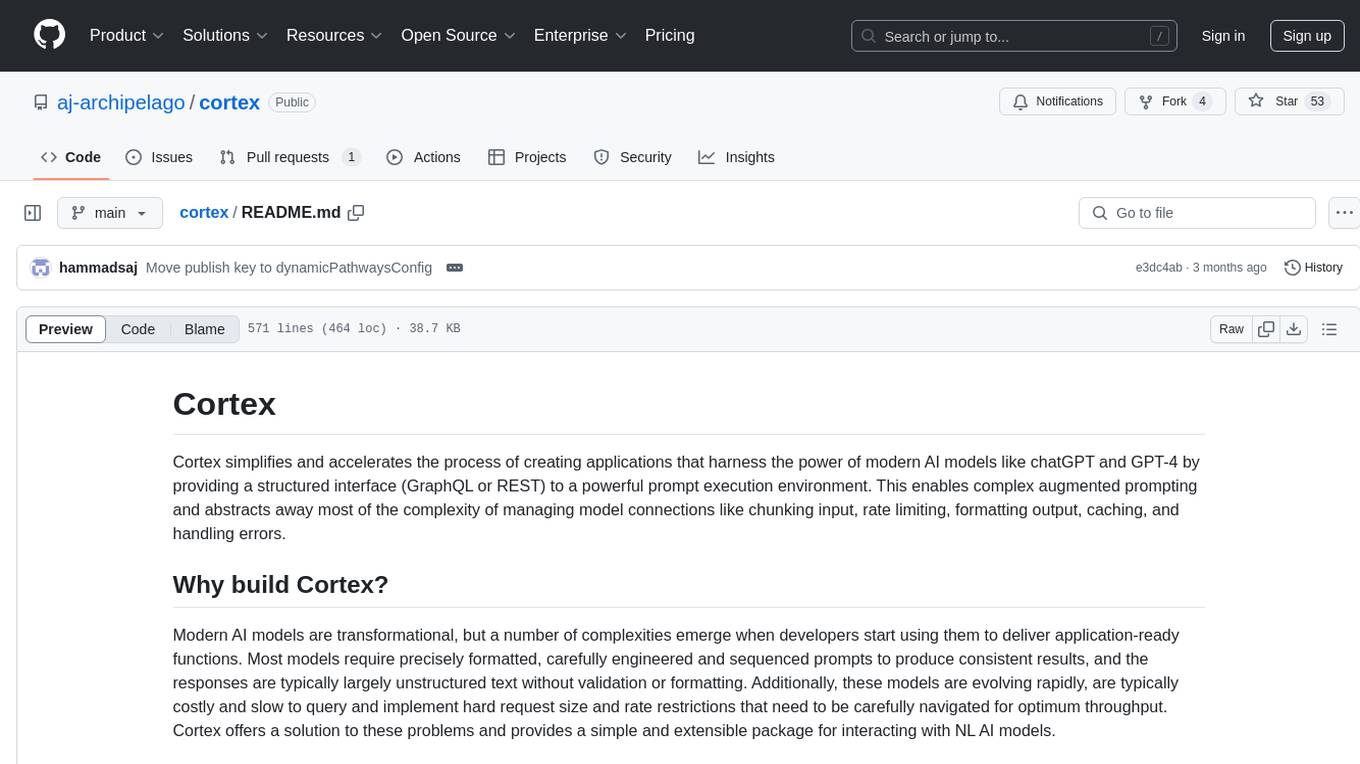
cortex
Cortex is a tool that simplifies and accelerates the process of creating applications utilizing modern AI models like chatGPT and GPT-4. It provides a structured interface (GraphQL or REST) to a prompt execution environment, enabling complex augmented prompting and abstracting away model connection complexities like input chunking, rate limiting, output formatting, caching, and error handling. Cortex offers a solution to challenges faced when using AI models, providing a simple package for interacting with NL AI models.
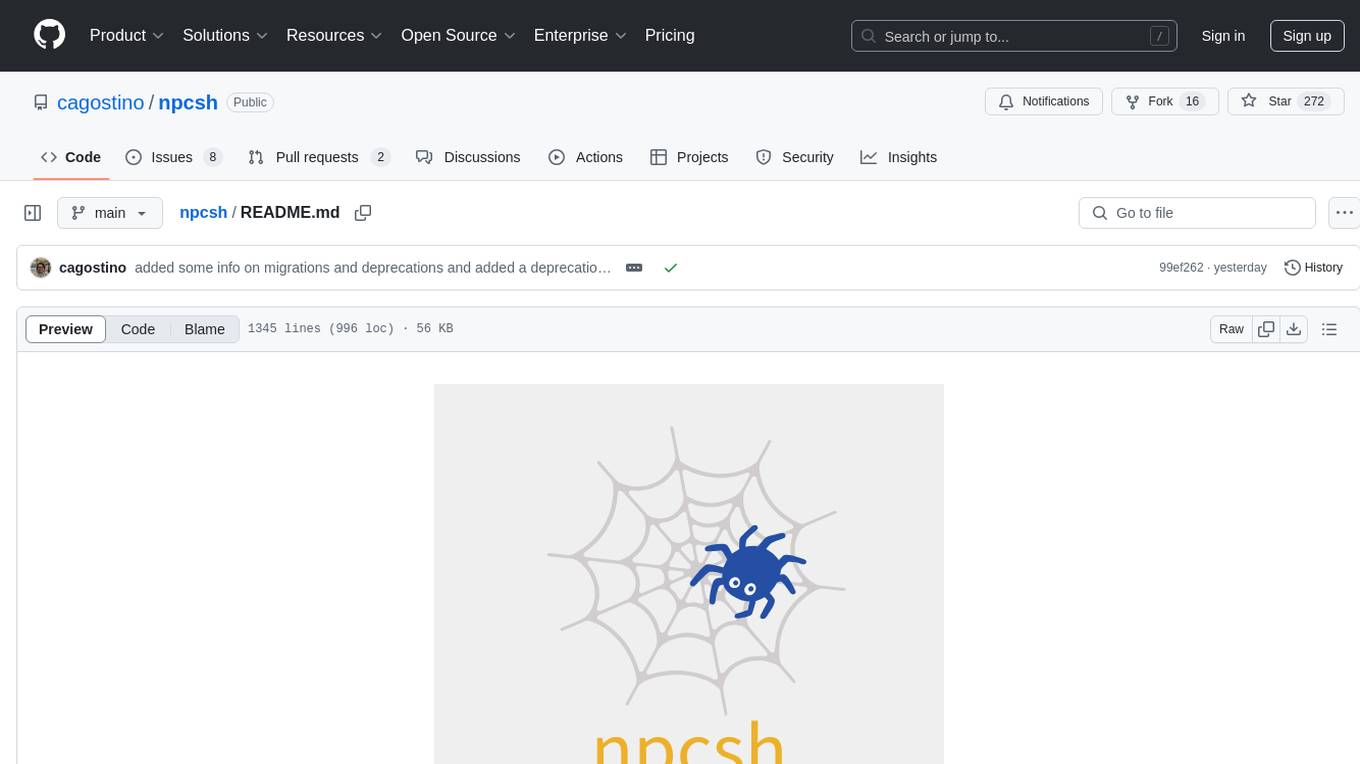
npcsh
`npcsh` is a python-based command-line tool designed to integrate Large Language Models (LLMs) and Agents into one's daily workflow by making them available and easily configurable through the command line shell. It leverages the power of LLMs to understand natural language commands and questions, execute tasks, answer queries, and provide relevant information from local files and the web. Users can also build their own tools and call them like macros from the shell. `npcsh` allows users to take advantage of agents (i.e. NPCs) through a managed system, tailoring NPCs to specific tasks and workflows. The tool is extensible with Python, providing useful functions for interacting with LLMs, including explicit coverage for popular providers like ollama, anthropic, openai, gemini, deepseek, and openai-like providers. Users can set up a flask server to expose their NPC team for use as a backend service, run SQL models defined in their project, execute assembly lines, and verify the integrity of their NPC team's interrelations. Users can execute bash commands directly, use favorite command-line tools like VIM, Emacs, ipython, sqlite3, git, pipe the output of these commands to LLMs, or pass LLM results to bash commands.
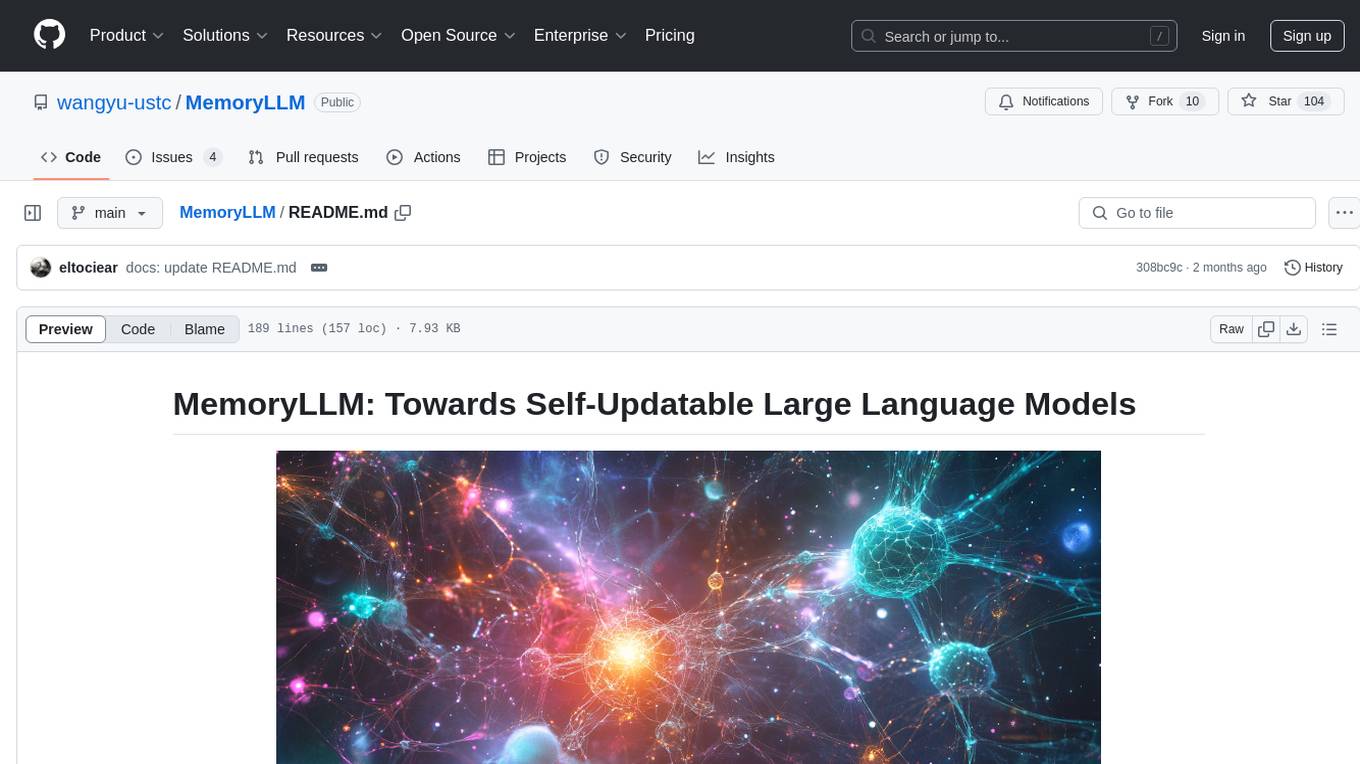
MemoryLLM
MemoryLLM is a large language model designed for self-updating capabilities. It offers pretrained models with different memory capacities and features, such as chat models. The repository provides training code, evaluation scripts, and datasets for custom experiments. MemoryLLM aims to enhance knowledge retention and performance on various natural language processing tasks.
For similar tasks
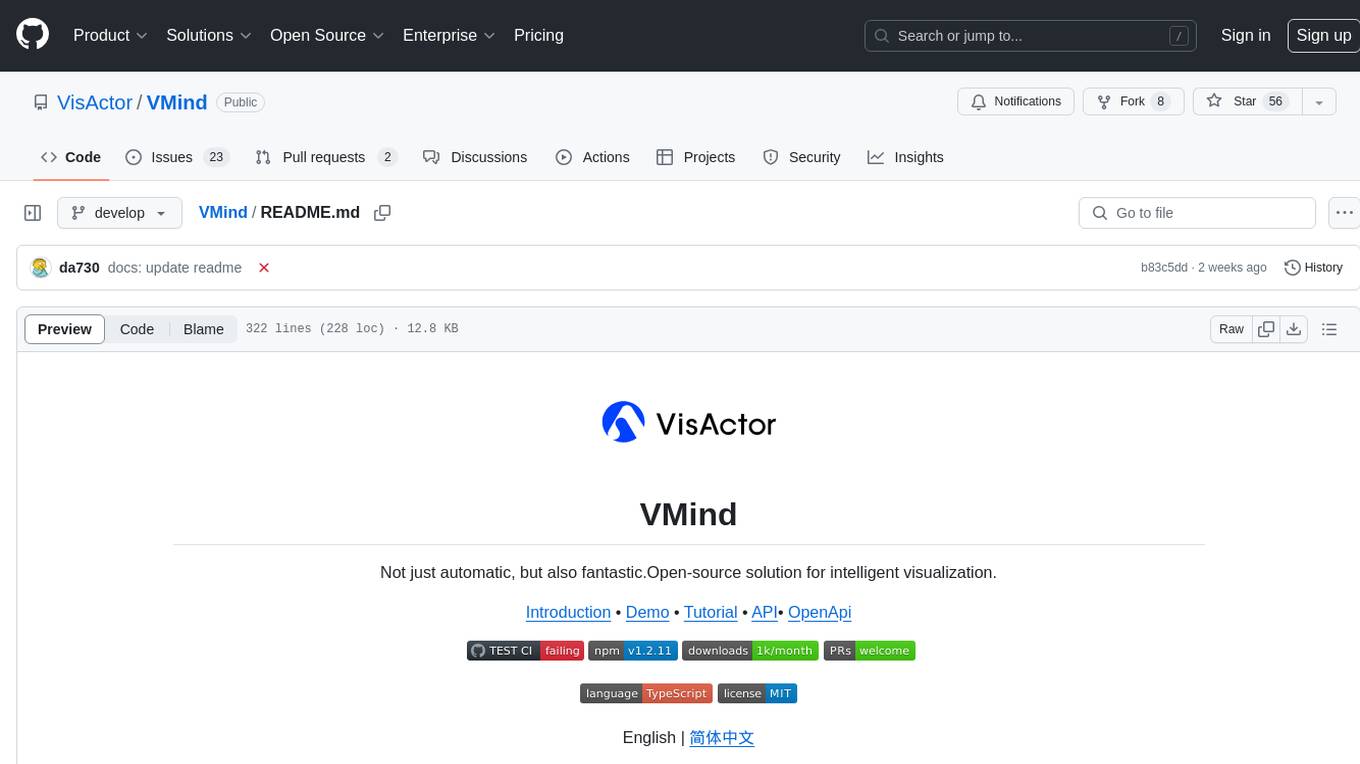
VMind
VMind is an open-source solution for intelligent visualization, providing an intelligent chart component based on LLM by VisActor. It allows users to create chart narrative works with natural language interaction, edit charts through dialogue, and export narratives as videos or GIFs. The tool is easy to use, scalable, supports various chart types, and offers one-click export functionality. Users can customize chart styles, specify themes, and aggregate data using LLM models. VMind aims to enhance efficiency in creating data visualization works through dialogue-based editing and natural language interaction.
For similar jobs
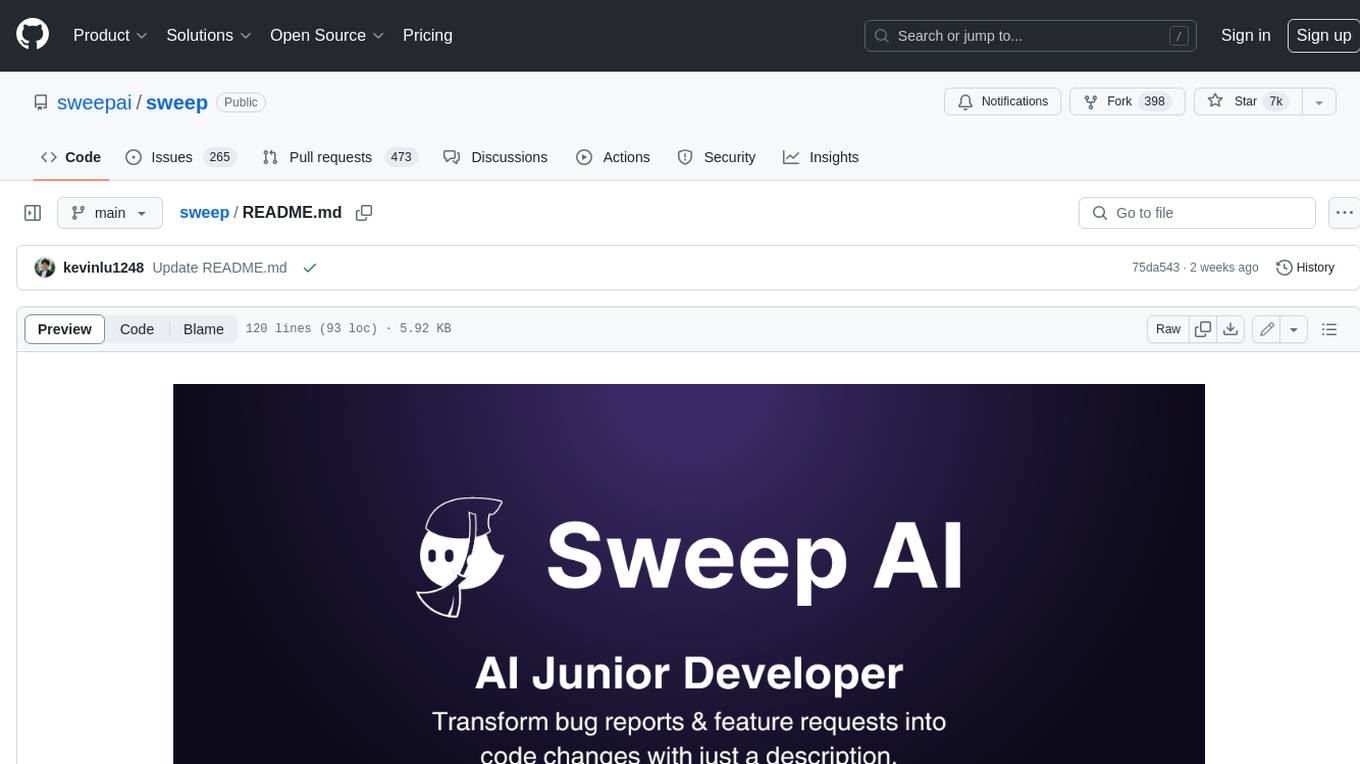
sweep
Sweep is an AI junior developer that turns bugs and feature requests into code changes. It automatically handles developer experience improvements like adding type hints and improving test coverage.
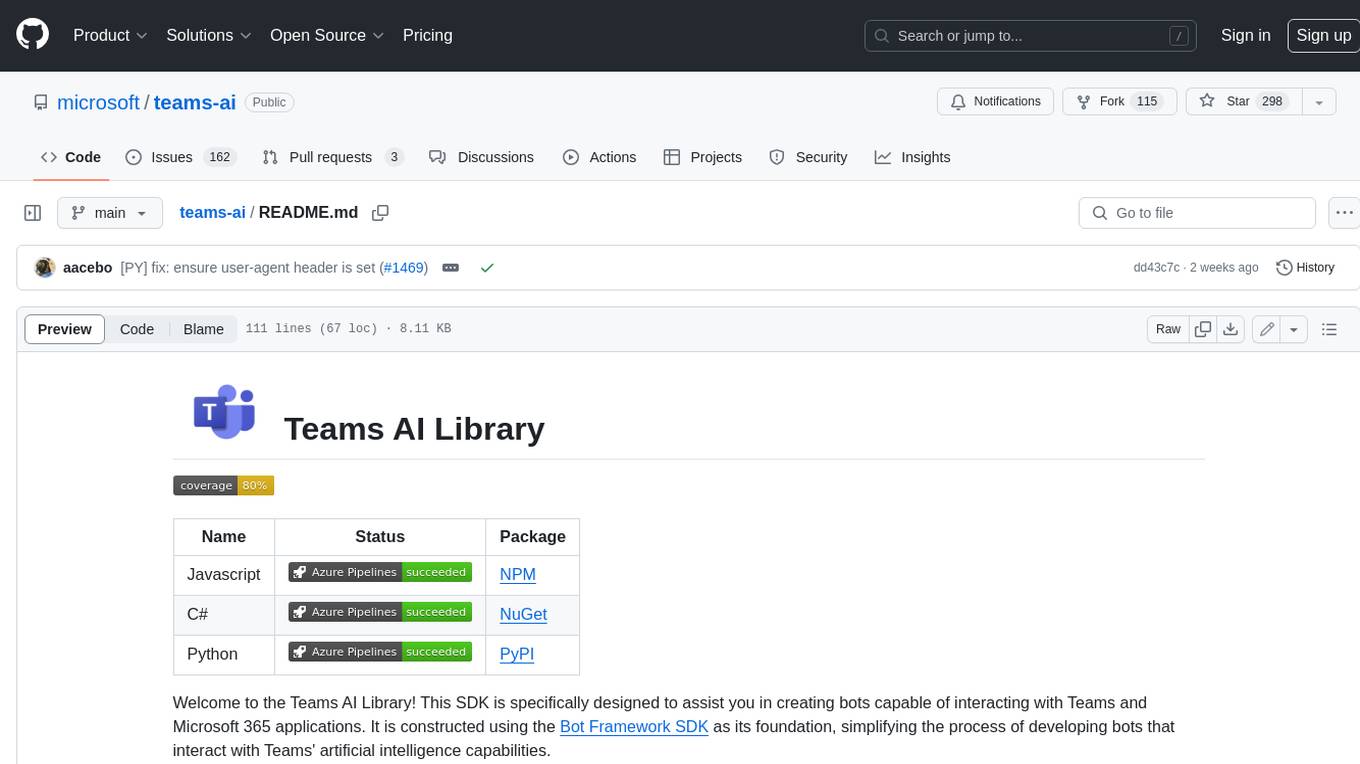
teams-ai
The Teams AI Library is a software development kit (SDK) that helps developers create bots that can interact with Teams and Microsoft 365 applications. It is built on top of the Bot Framework SDK and simplifies the process of developing bots that interact with Teams' artificial intelligence capabilities. The SDK is available for JavaScript/TypeScript, .NET, and Python.
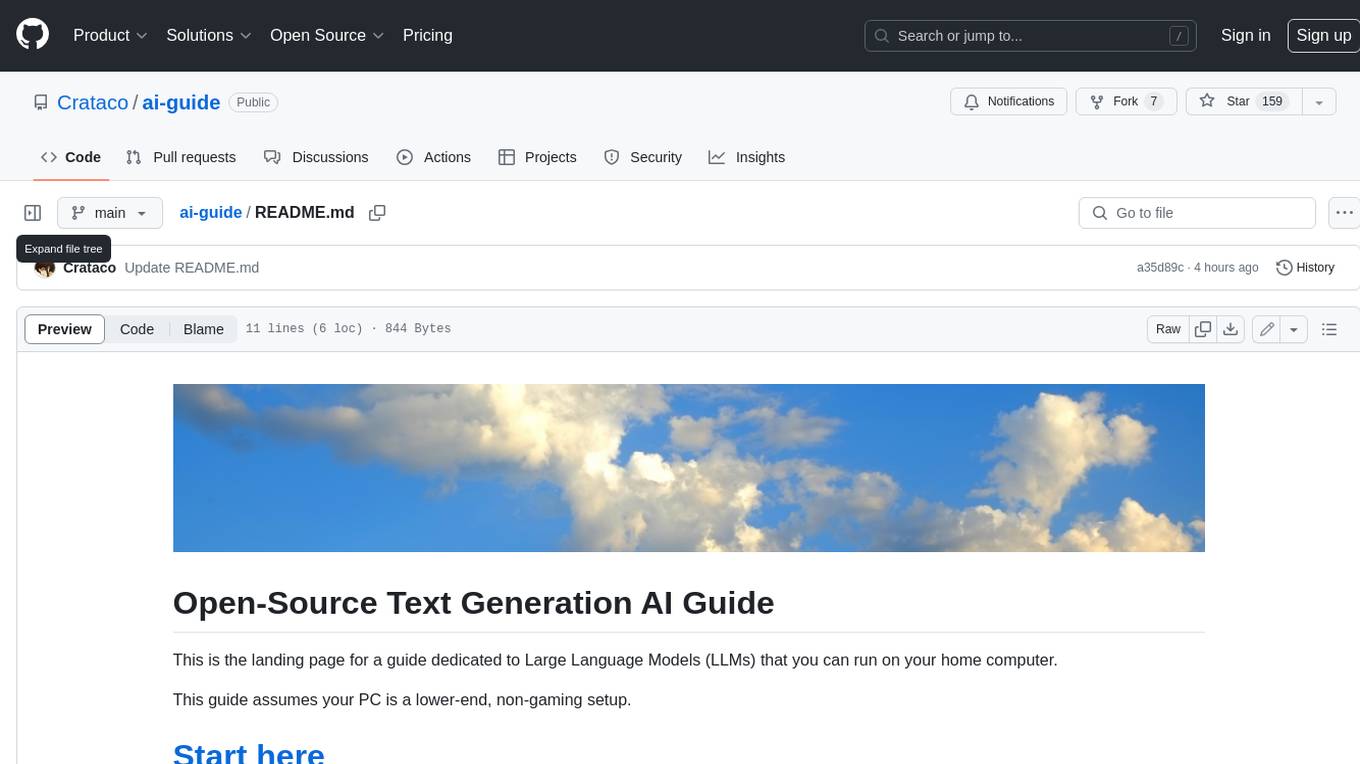
ai-guide
This guide is dedicated to Large Language Models (LLMs) that you can run on your home computer. It assumes your PC is a lower-end, non-gaming setup.
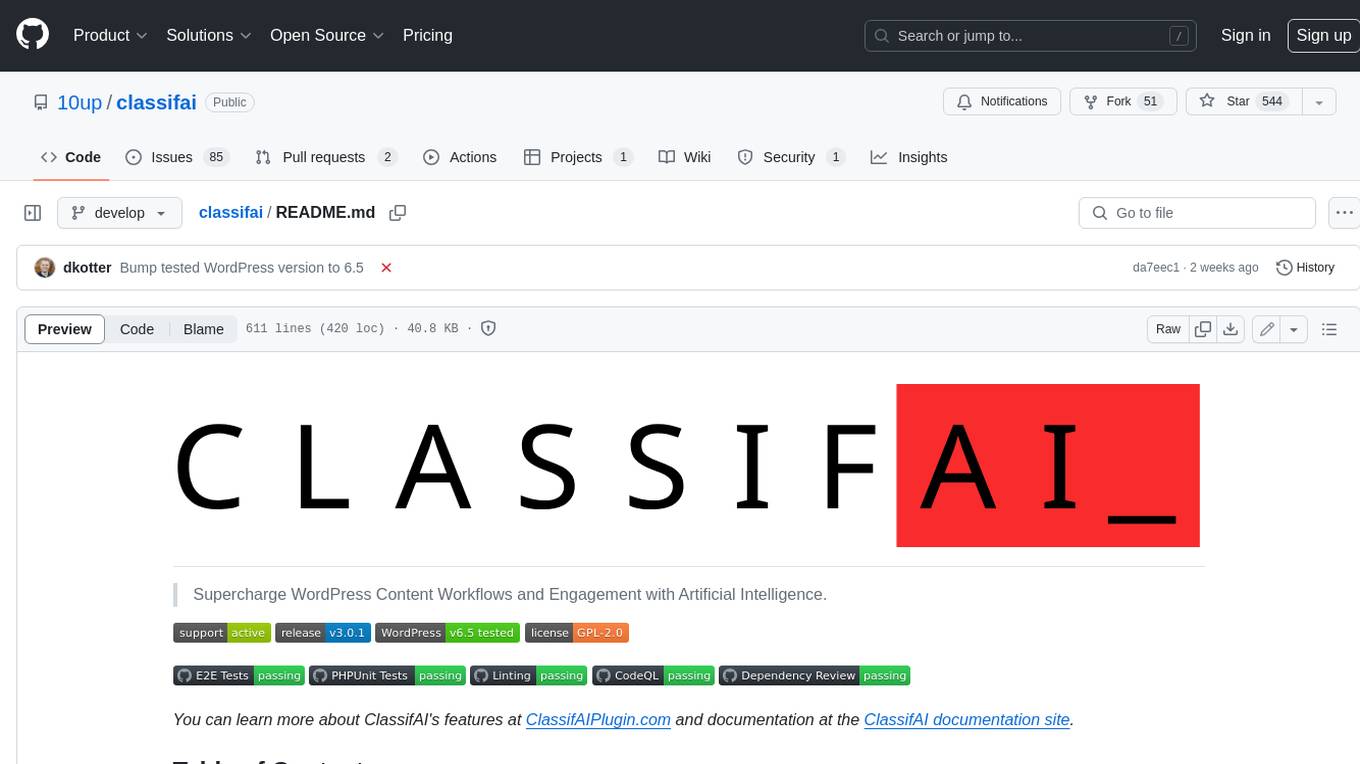
classifai
Supercharge WordPress Content Workflows and Engagement with Artificial Intelligence. Tap into leading cloud-based services like OpenAI, Microsoft Azure AI, Google Gemini and IBM Watson to augment your WordPress-powered websites. Publish content faster while improving SEO performance and increasing audience engagement. ClassifAI integrates Artificial Intelligence and Machine Learning technologies to lighten your workload and eliminate tedious tasks, giving you more time to create original content that matters.
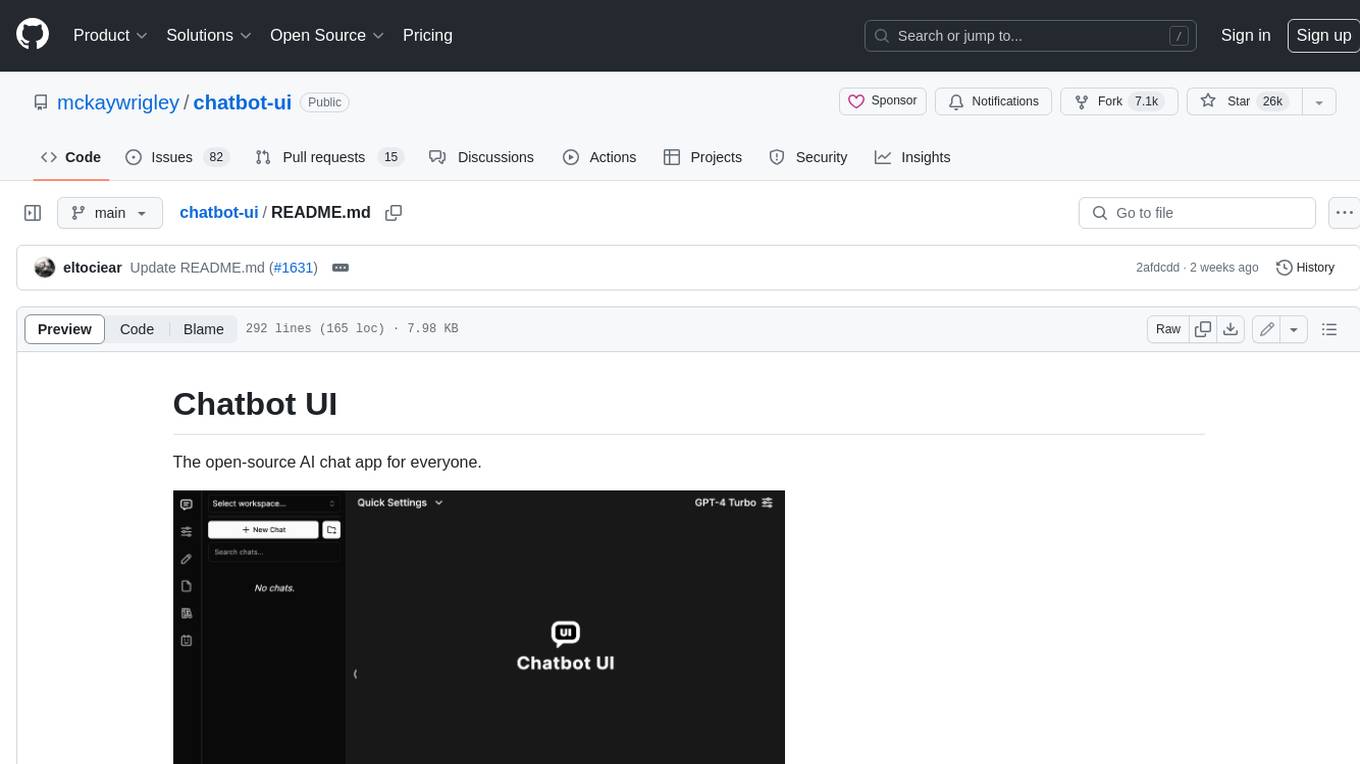
chatbot-ui
Chatbot UI is an open-source AI chat app that allows users to create and deploy their own AI chatbots. It is easy to use and can be customized to fit any need. Chatbot UI is perfect for businesses, developers, and anyone who wants to create a chatbot.
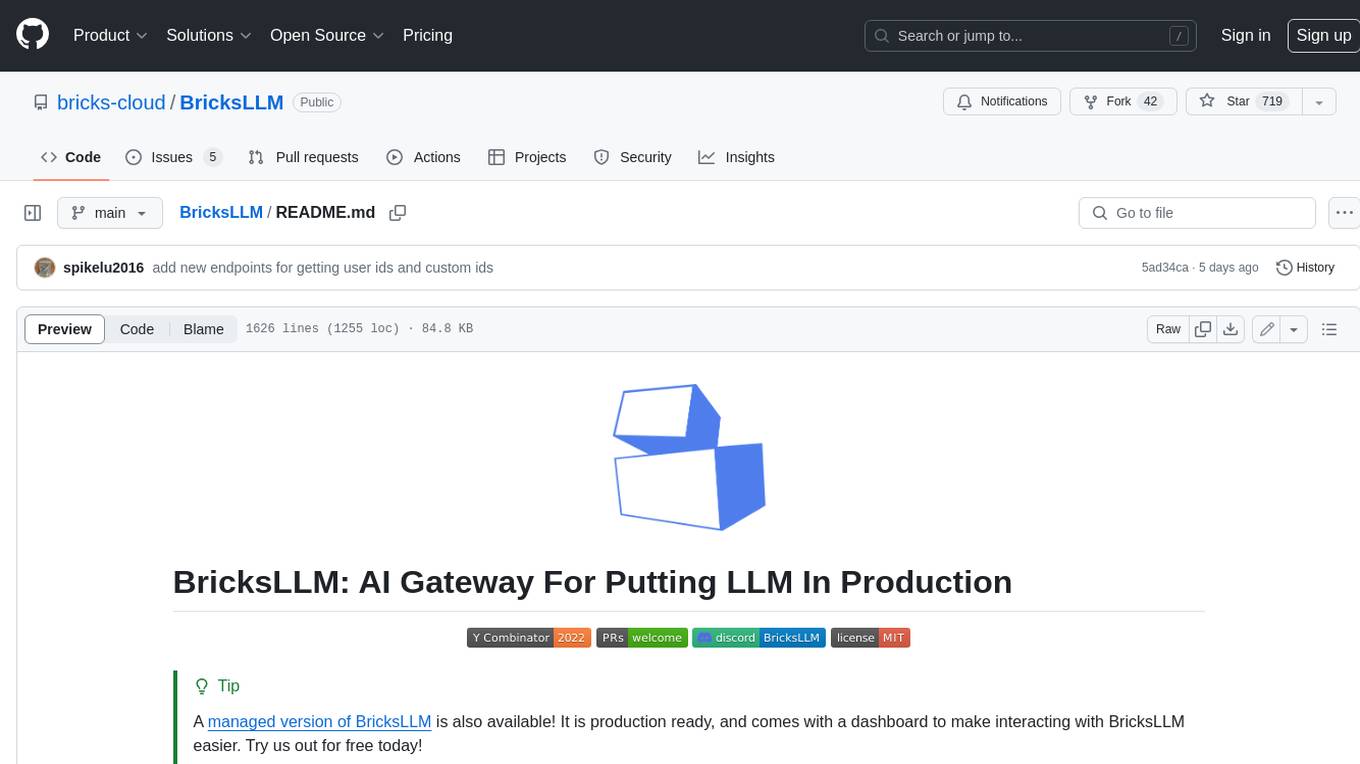
BricksLLM
BricksLLM is a cloud native AI gateway written in Go. Currently, it provides native support for OpenAI, Anthropic, Azure OpenAI and vLLM. BricksLLM aims to provide enterprise level infrastructure that can power any LLM production use cases. Here are some use cases for BricksLLM: * Set LLM usage limits for users on different pricing tiers * Track LLM usage on a per user and per organization basis * Block or redact requests containing PIIs * Improve LLM reliability with failovers, retries and caching * Distribute API keys with rate limits and cost limits for internal development/production use cases * Distribute API keys with rate limits and cost limits for students
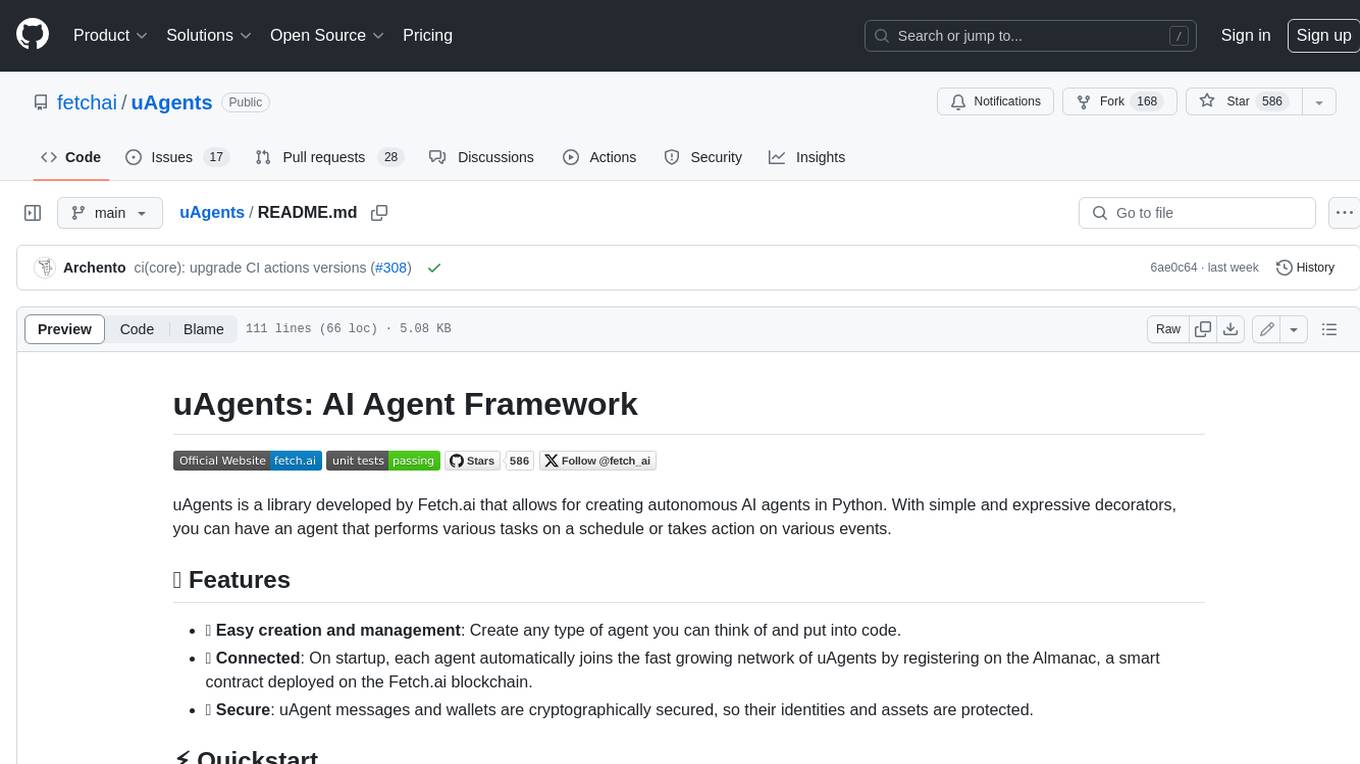
uAgents
uAgents is a Python library developed by Fetch.ai that allows for the creation of autonomous AI agents. These agents can perform various tasks on a schedule or take action on various events. uAgents are easy to create and manage, and they are connected to a fast-growing network of other uAgents. They are also secure, with cryptographically secured messages and wallets.
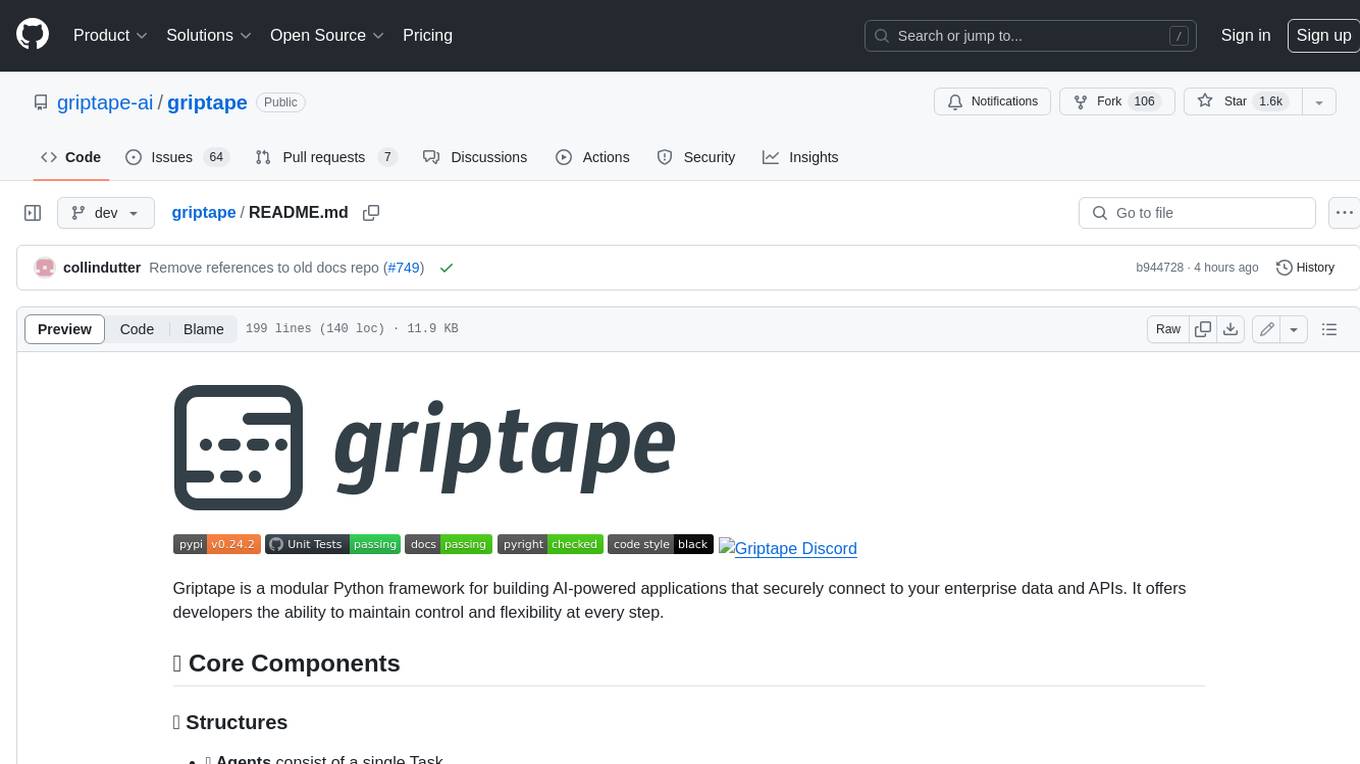
griptape
Griptape is a modular Python framework for building AI-powered applications that securely connect to your enterprise data and APIs. It offers developers the ability to maintain control and flexibility at every step. Griptape's core components include Structures (Agents, Pipelines, and Workflows), Tasks, Tools, Memory (Conversation Memory, Task Memory, and Meta Memory), Drivers (Prompt and Embedding Drivers, Vector Store Drivers, Image Generation Drivers, Image Query Drivers, SQL Drivers, Web Scraper Drivers, and Conversation Memory Drivers), Engines (Query Engines, Extraction Engines, Summary Engines, Image Generation Engines, and Image Query Engines), and additional components (Rulesets, Loaders, Artifacts, Chunkers, and Tokenizers). Griptape enables developers to create AI-powered applications with ease and efficiency.