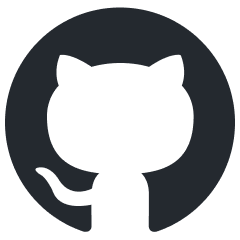
Trace
End-to-end Generative Optimization for AI Agents
Stars: 500
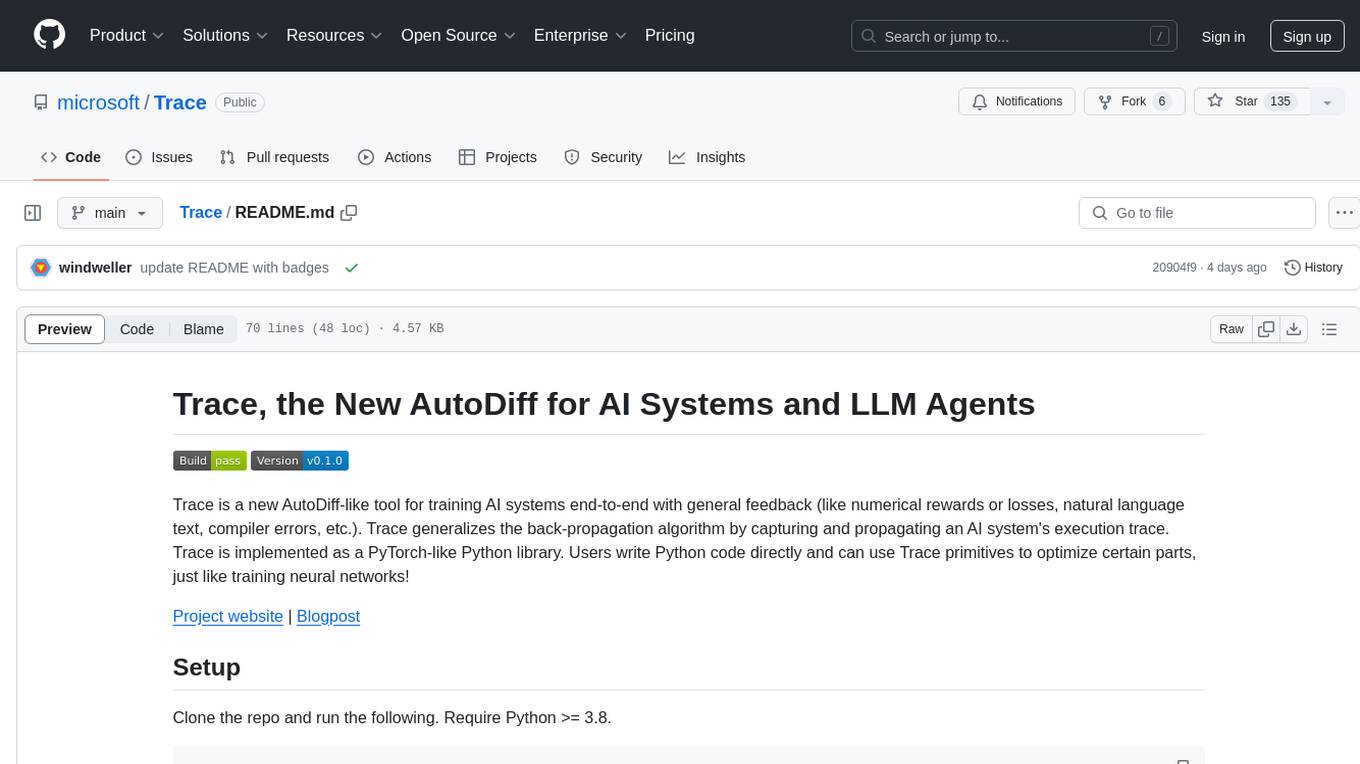
Trace is a new AutoDiff-like tool for training AI systems end-to-end with general feedback. It generalizes the back-propagation algorithm by capturing and propagating an AI system's execution trace. Implemented as a PyTorch-like Python library, users can write Python code directly and use Trace primitives to optimize certain parts, similar to training neural networks.
README:
Trace is a new AutoDiff-like tool for training AI systems end-to-end with general feedback (like numerical rewards or losses, natural language text, compiler errors, etc.). Trace generalizes the back-propagation algorithm by capturing and propagating an AI system's execution trace. Trace is implemented as a PyTorch-like Python library. Users write Python code directly and can use Trace primitives to optimize certain parts, just like training neural networks!
Paper | Project website | Documentation | Blogpost | Discord channel
Simply run
pip install trace-opt
Or for development, clone the repo and run the following.
pip install -e .
The library requires Python >= 3.9. By default (starting with v0.1.3.5), we use LiteLLM as the backend of LLMs. For backward compatibility, we provide backend-support with AutoGen; when installing, users can add [autogen]
tag to install a compatible AutoGen version (e.g., pip install trace-opt[autogen]
). You may require Git Large File Storage if
git is unable to clone the repository.
For questions or reporting bugs, please use Github Issues or post on our Discord channel. We actively check these channels.
- 2025.2.7 Trace was featured in the G-Research NeurIPS highlight by the Science Director Hugh Salimbeni.
- 2024.12.10 Trace was demoed in person at NeurIPS 2024 Expo.
- 2024.11.05 Ching-An Cheng gave a talk at UW Robotics Colloquium on Trace: video.
- 2024.10.21 New paper by Nvidia, Stanford, Visa, & Intel applies Trace to optimize for mapper code of parallel programming (for scientific computing and matrix multiplication). Trace (OptoPrime) learns code achieving 1.3X speed up under 10 minutes, compared to the code optimized by a system engineer expert.
- 2024.9.30 Ching-An Cheng gave a talk to the AutoGen community: link.
- 2024.9.25 Trace Paper is accepted to NeurIPS 2024.
- 2024.9.14 TextGrad is available as an optimizer in Trace.
- 2024.8.18 Allen Nie gave a talk to Pasteur Labs & Institute for Simulation Intelligence.
We have a mailing list for announcements: Signup
Trace has two primitives: node
and bundle
. node
is a primitive to define a node in the computation graph. bundle
is a primitive to define a function that can be optimized.
from opto.trace import node
x = node(1, trainable=True)
y = node(3)
z = x / y
z2 = x / 3 # the int 3 would be converted to a node automatically
list_of_nodes = [x, node(2), node(3)]
node_of_list = node([1, 2, 3])
node_of_list.append(3)
# easy built-in computation graph visualization
z.backward("maximize z", visualize=True, print_limit=25)
Once a node is declared, all the following operations on the node object will be automatically traced.
We built many magic functions to make a node object act like a normal Python object. By marking trainable=True
, we
tell our optimizer that this node's content
can be changed by the optimizer.
For functions, Trace uses decorators like @bundle to wrap over Python functions. A bundled function behaves like any other Python function.
from opto.trace import node, bundle
@bundle(trainable=True)
def strange_sort_list(lst):
'''
Given list of integers, return list in strange order.
Strange sorting, is when you start with the minimum value,
then maximum of the remaining integers, then minimum and so on.
'''
lst = sorted(lst)
return lst
test_input = [1, 2, 3, 4]
test_output = strange_sort_list(test_input)
print(test_output)
Now, after declaring what is trainable and what isn't, and use node
and bundle
to define the computation graph, we
can use the optimizer to optimize the computation graph.
from opto.optimizers import OptoPrime
# we first declare a feedback function
# think of this as the reward function (or loss function)
def get_feedback(predict, target):
if predict == target:
return "test case passed!"
else:
return "test case failed!"
test_ground_truth = [1, 4, 2, 3]
test_input = [1, 2, 3, 4]
epoch = 2
optimizer = OptoPrime(strange_sort_list.parameters())
for i in range(epoch):
print(f"Training Epoch {i}")
test_output = strange_sort_list(test_input)
correctness = test_output.eq(test_ground_truth)
feedback = get_feedback(test_output, test_ground_truth)
if correctness:
break
optimizer.zero_feedback()
optimizer.backward(correctness, feedback)
optimizer.step()
Then, we can use the familiar PyTorch-like syntax to conduct the optimization.
Here is another example of a simple sales agent:
from opto import trace
@trace.model
class Agent:
def __init__(self, system_prompt):
self.system_prompt = system_prompt
self.instruct1 = trace.node("Decide the language", trainable=True)
self.instruct2 = trace.node("Extract name if it's there", trainable=True)
def __call__(self, user_query):
response = trace.operators.call_llm(self.system_prompt,
self.instruct1, user_query)
en_or_es = self.decide_lang(response)
user_name = trace.operators.call_llm(self.system_prompt,
self.instruct2, user_query)
greeting = self.greet(en_or_es, user_name)
return greeting
@trace.bundle(trainable=True)
def decide_lang(self, response):
"""Map the language into a variable"""
return
@trace.bundle(trainable=True)
def greet(self, lang, user_name):
"""Produce a greeting based on the language"""
greeting = "Hola"
return f"{greeting}, {user_name}!"
Imagine we have a feedback function (like a reward function) that tells us how well the agent is doing. We can then optimize this agent online:
from opto.optimizers import OptoPrime
def feedback_fn(generated_response, gold_label='en'):
if gold_label == 'en' and 'Hello' in generated_response:
return "Correct"
elif gold_label == 'es' and 'Hola' in generated_response:
return "Correct"
else:
return "Incorrect"
def train():
epoch = 3
agent = Agent("You are a sales assistant.")
optimizer = OptoPrime(agent.parameters())
for i in range(epoch):
print(f"Training Epoch {i}")
try:
greeting = agent("Hola, soy Juan.")
feedback = feedback_fn(greeting.data, 'es')
except trace.ExecutionError as e:
greeting = e.exception_node
feedback, terminal, reward = greeting.data, False, 0
optimizer.zero_feedback()
optimizer.backward(greeting, feedback)
optimizer.step(verbose=True)
if feedback == 'Correct':
break
return agent
agent = train()
Defining and training an agent through Trace will give you more flexibility and control over what the agent learns.
Level | Tutorial | Run in Colab | Description |
---|---|---|---|
Beginner | Getting Started | Introduces basic primitives like node and bundle . Showcases a code optimization pipeline. |
|
Beginner | Adaptive AI Agent | Introduce primitive model that allows anyone to build self-improving agents that react to environment feedback. Shows how an LLM agent learns to place a shot in a Battleship game. |
|
Intermediate | Multi-Agent Collaboration | N/A | Demonstrates how Trace can be used for multi-agent collaboration environment in Virtualhome. |
Intermediate | NLP Prompt Optimization | Shows how Trace can optimizes prompt and code together jointly for BigBench-Hard 23 tasks. | |
Advanced | Robotic Arm Control | Trace can optimize code to control a robotic arm after observing a full trajectory of interactions. |
Currently, we support three optimizers:
- OPRO: Large Language Models as Optimizers
- TextGrad: TextGrad: Automatic "Differentiation" via Text
- OptoPrime: Our proposed algorithm -- using the entire computational graph to perform parameter update. It is 2-3x faster than TextGrad.
Using our framework, you can seamlessly switch between different optimizers:
optimizer1 = OptoPrime(strange_sort_list.parameters())
optimizer2 = OPRO(strange_sort_list.parameters())
optimizer3 = TextGrad(strange_sort_list.parameters())
Here is a summary of the optimizers:
Computation Graph | Code as Functions | Library Support | Supported Optimizers | Speed | Large Graph | |
---|---|---|---|---|---|---|
OPRO | ❌ | ❌ | ❌ | OPRO | ⚡️ | ✅ |
TextGrad | ✅ | ❌ | ✅ | TextGrad | 🐌 | ✅ |
Trace | ✅ | ✅ | ✅ | OPRO, OptoPrime, TextGrad | ⚡ | ✅ |
The table evaluates the frameworks in the following aspects:
- Computation Graph: Whether the optimizer leverages the computation graph of the workflow.
- Code as Functions: Whether the framework allows users to write actual executable Python functions and not require users to wrap them in strings.
- Library Support: Whether the framework has a library to support the optimizer.
- Speed: TextGrad is about 2-3x slower than OptoPrime (Trace). OPRO has no concept of computational graph, therefore is very fast.
- Large Graph: OptoPrime (Trace) represents the entire computation graph in context, therefore, might have issue with graphs that have more than hundreds of operations. TextGrad does not have the context-length issue, however, might be very slow on large graphs.
We provide a comparison to validate our implementation of TextGrad in Trace:
To produce this table, we ran the TextGrad pip-installed repo on 2024-10-30, and we also include the numbers reported in the TextGrad paper. The LLM APIs are called around the same time to ensure a fair comparison. TextGrad paper's result was reported in 2024-06.
You can also easily implement your own optimizer that works directly with TraceGraph
(more tutorials on how to work
with TraceGraph coming soon).
Currently we rely on LiteLLM or AutoGen v0.2 for LLM caching and API-Key management.
By default, LiteLLM is used. To change the default backend, set the environment variable TRACE_DEFAULT_LLM_BACKEND
on terminal
export TRACE_DEFAULT_LLM_BACKEND="<your LLM backend here>" # 'LiteLLM' or 'AutoGen`
or in python before importing opto
import os
os.environ["TRACE_DEFAULT_LLM_BACKEND"] = "<your LLM backend here>" # 'LiteLLM' or 'AutoGen`
import opto
Set the keys as the environment variables, following the documentation of LiteLLM. For example,
import os
os.environ["OPENAI_API_KEY"] = "<your OpenAI API key here>"
os.environ["ANTHROPIC_API_KEY"] = "<your Anthropic API key here>"
In Trace, we add another environment variable TRACE_LITELLM_MODEL
to set the default model name used by LiteLLM for convenience, e.g.,
export TRACE_LITELLM_MODEL='gpt-4o'
will set all LLM instances in Trace to use gpt-4o
by default.
First install Trace with autogen flag, pip install trace-opt[autogen]
. AutoGen relies on OAI_CONFIG_LIST
, which is a file you put in your working directory. It has the format of:
[
{
"model": "gpt-4",
"api_key": "<your OpenAI API key here>"
},
{
"model": "claude-sonnet-3.5-latest",
"api_key": "<your Anthropic API key here>"
}
]
You can switch between different LLM models by changing the model
field in this configuration file.
Note AutoGen by default will use the first model available in this config file.
You can also set an os.environ
variable OAI_CONFIG_LIST
to point to the location of this file or directly set a JSON string as the value of this variable.
For convenience, we also provide a method that directly grabs the API key from the environment variable OPENAI_API_KEY
or ANTHROPIC_API_KEY
.
However, doing so, we specify the model version you use, which is gpt-4o
for OpenAI and claude-sonnet-3.5-latest
for Anthropic.
If you use this code in your research please cite the following publication:
@article{cheng2024trace,
title={Trace is the Next AutoDiff: Generative Optimization with Rich Feedback, Execution Traces, and LLMs},
author={Cheng, Ching-An and Nie, Allen and Swaminathan, Adith},
journal={arXiv preprint arXiv:2406.16218},
year={2024}
}
Improving Parallel Program Performance Through DSL-Driven Code Generation with LLM Optimizers Work from Stanford, NVIDIA, Intel, Visa Research.
@article{wei2024improving,
title={Improving Parallel Program Performance Through DSL-Driven Code Generation with LLM Optimizers},
author={Wei, Anjiang and Nie, Allen and Teixeira, Thiago SFX and Yadav, Rohan and Lee, Wonchan and Wang, Ke and Aiken, Alex},
journal={arXiv preprint arXiv:2410.15625},
year={2024}
}
The Importance of Directional Feedback for LLM-based Optimizers Explains the role of feedback in LLM-based optimizers. An early work that influenced Trace's clean separation between the platform, optimizer, and feedback.
@article{nie2024importance,
title={The Importance of Directional Feedback for LLM-based Optimizers},
author={Nie, Allen and Cheng, Ching-An and Kolobov, Andrey and Swaminathan, Adith},
journal={arXiv preprint arXiv:2405.16434},
year={2024}
}
A previous version of Trace was tested with gpt-4-0125-preview on numerical optimization, simulated traffic control, big-bench-hard, and llf-metaworld tasks, which demonstrated good optimization performance on multiple random seeds; please see the paper for details.
Note For gpt-4o, please use the version gpt-4o-2024-08-06 (onwards), which fixes the structured output issue of gpt-4o-2024-05-13. While gpt-4 works reliably most of the time, we've found gpt-4o-2024-05-13 often hallucinates even in very basic optimization problems and does not follow instructions. This might be due to the current implementation of optimizers rely on outputing in json format. Issues of gpt-4o with json have been reported in the communities ( see example).
- Trace is an LLM-based optimization framework for research purpose only.
- The current release is a beta version of the library. Features and more documentation will be added, and some functionalities may be changed in the future.
- System performance may vary by workflow, dataset, query, and response, and users are responsible for determining the accuracy of generated content.
- System outputs do not represent the opinions of Microsoft.
- All decisions leveraging outputs of the system should be made with human oversight and not be based solely on system outputs.
- Use of the system must comply with all applicable laws, regulations, and policies, including those pertaining to privacy and security.
- The system should not be used in highly regulated domains where inaccurate outputs could suggest actions that lead to injury or negatively impact an individual's legal, financial, or life opportunities.
This project welcomes contributions and suggestions. Most contributions require you to agree to a Contributor License Agreement (CLA) declaring that you have the right to, and actually do, grant us the rights to use your contribution. For details, visit https://cla.opensource.microsoft.com.
When you submit a pull request, a CLA bot will automatically determine whether you need to provide a CLA and decorate the PR appropriately (e.g., status check, comment). Simply follow the instructions provided by the bot. You will only need to do this once across all repos using our CLA.
This project has adopted the Microsoft Open Source Code of Conduct. For more information see the Code of Conduct FAQ or contact [email protected] with any additional questions or comments.
This project may contain trademarks or logos for projects, products, or services. Authorized use of Microsoft trademarks or logos is subject to and must follow Microsoft's Trademark & Brand Guidelines. Use of Microsoft trademarks or logos in modified versions of this project must not cause confusion or imply Microsoft sponsorship. Any use of third-party trademarks or logos are subject to those third-party's policies.
For Tasks:
Click tags to check more tools for each tasksFor Jobs:
Alternative AI tools for Trace
Similar Open Source Tools
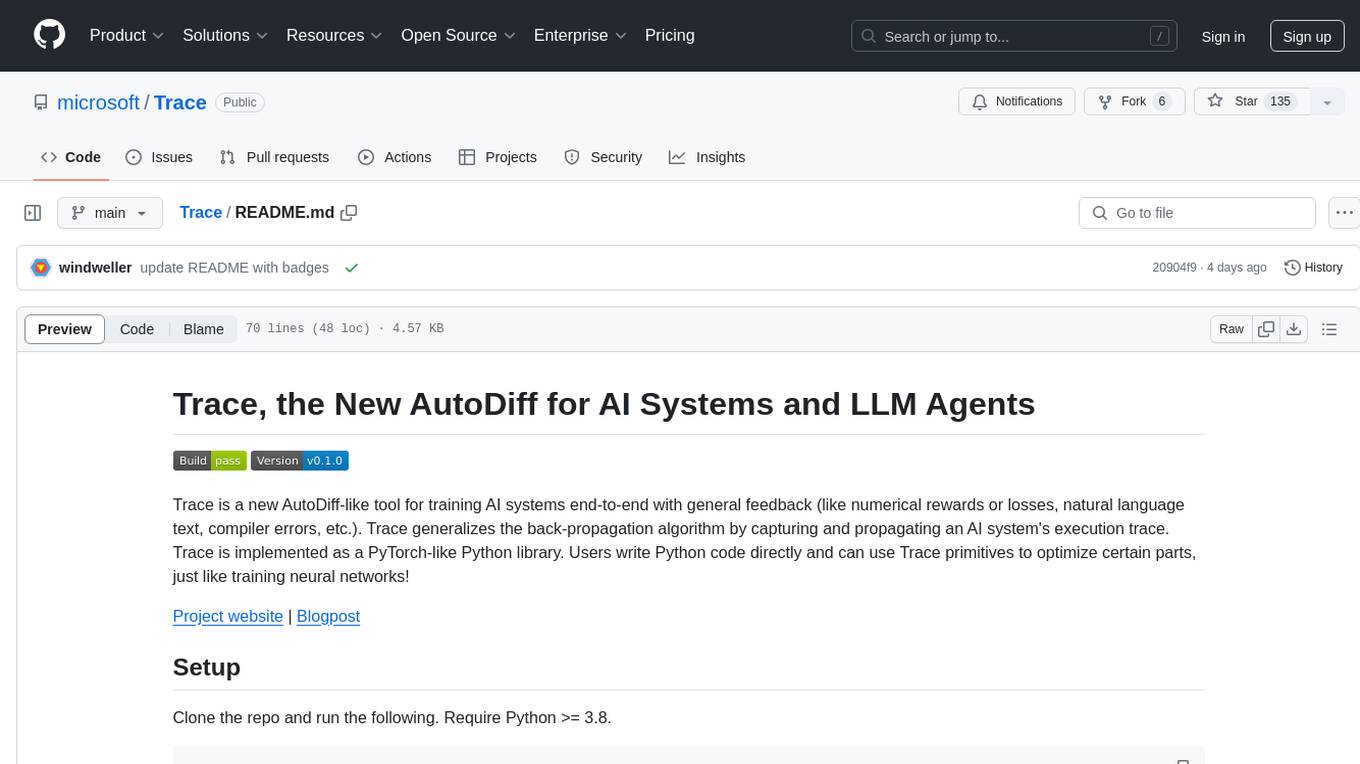
Trace
Trace is a new AutoDiff-like tool for training AI systems end-to-end with general feedback. It generalizes the back-propagation algorithm by capturing and propagating an AI system's execution trace. Implemented as a PyTorch-like Python library, users can write Python code directly and use Trace primitives to optimize certain parts, similar to training neural networks.
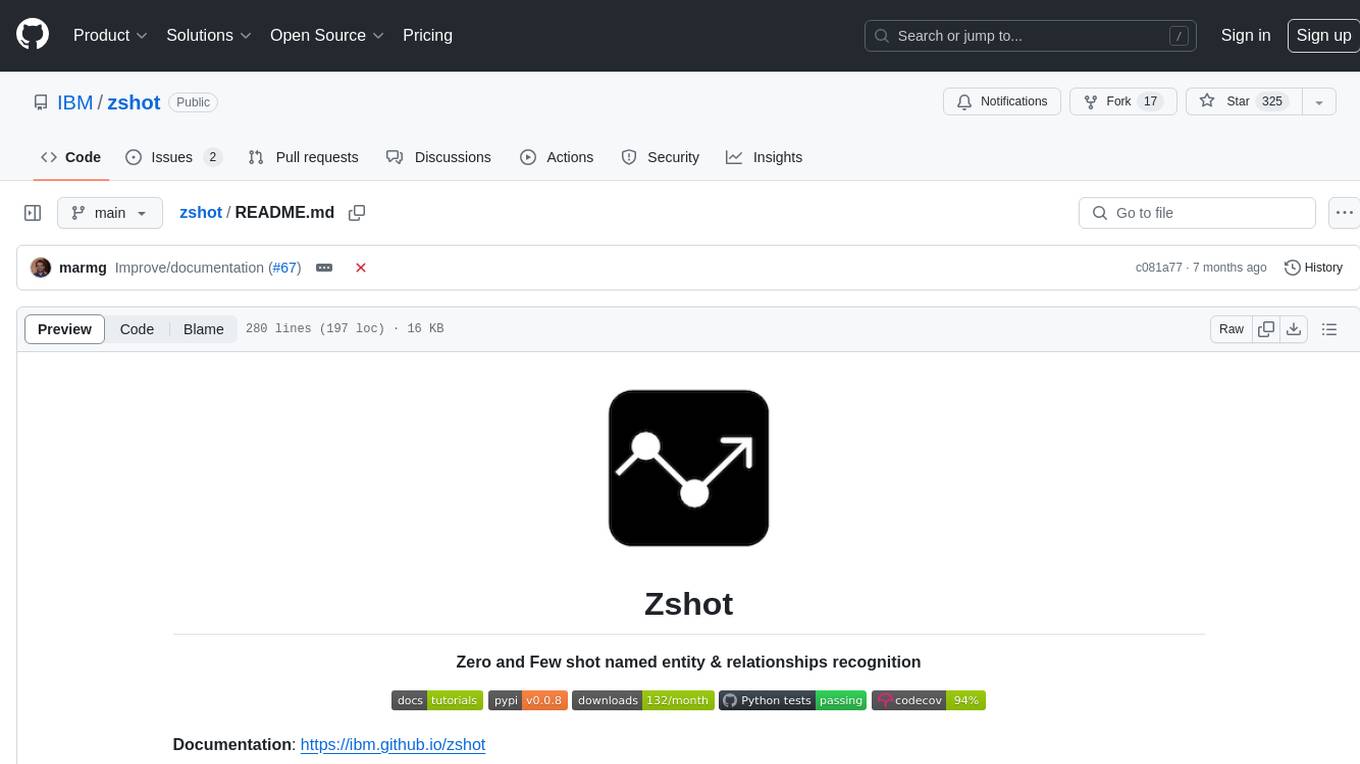
zshot
Zshot is a highly customizable framework for performing Zero and Few shot named entity and relationships recognition. It can be used for mentions extraction, wikification, zero and few shot named entity recognition, zero and few shot named relationship recognition, and visualization of zero-shot NER and RE extraction. The framework consists of two main components: the mentions extractor and the linker. There are multiple mentions extractors and linkers available, each serving a specific purpose. Zshot also includes a relations extractor and a knowledge extractor for extracting relations among entities and performing entity classification. The tool requires Python 3.6+ and dependencies like spacy, torch, transformers, evaluate, and datasets for evaluation over datasets like OntoNotes. Optional dependencies include flair and blink for additional functionalities. Zshot provides examples, tutorials, and evaluation methods to assess the performance of the components.
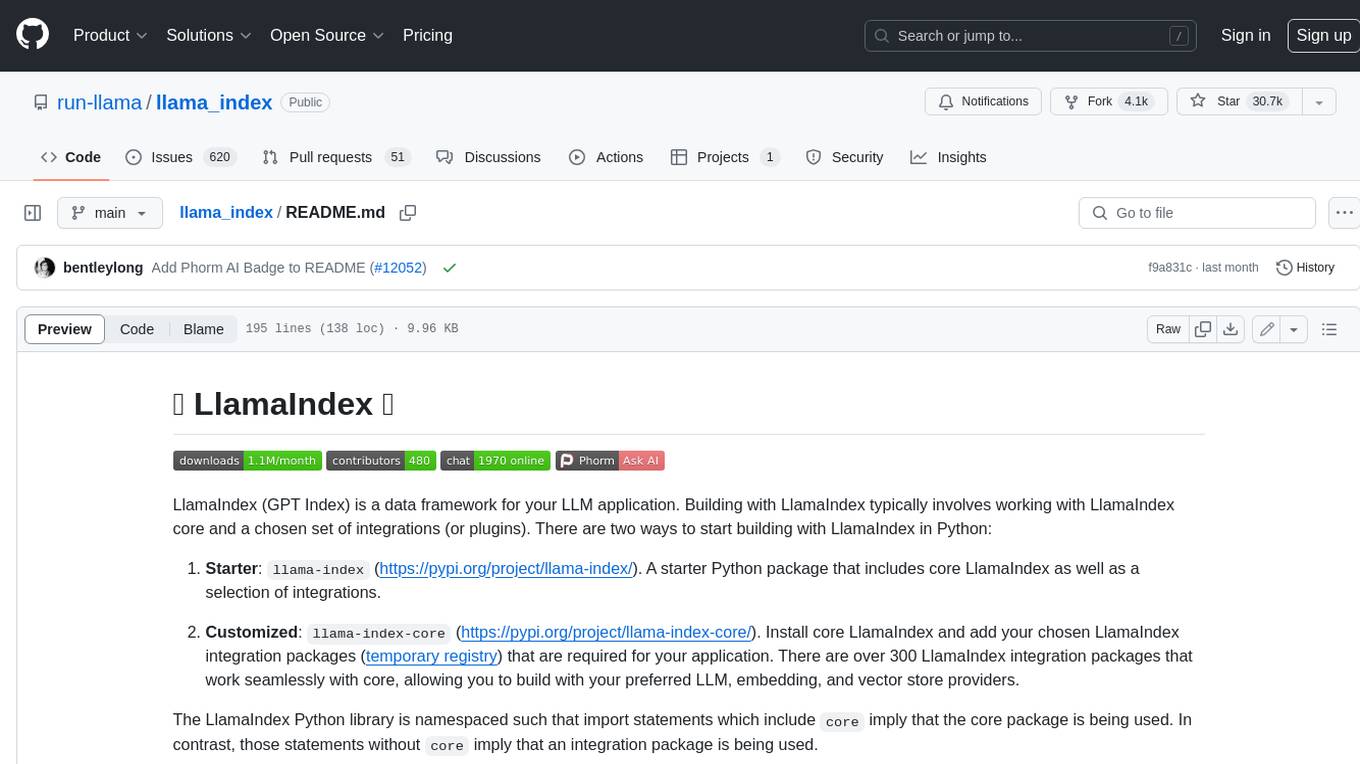
llama_index
LlamaIndex is a data framework for building LLM applications. It provides tools for ingesting, structuring, and querying data, as well as integrating with LLMs and other tools. LlamaIndex is designed to be easy to use for both beginner and advanced users, and it provides a comprehensive set of features for building LLM applications.
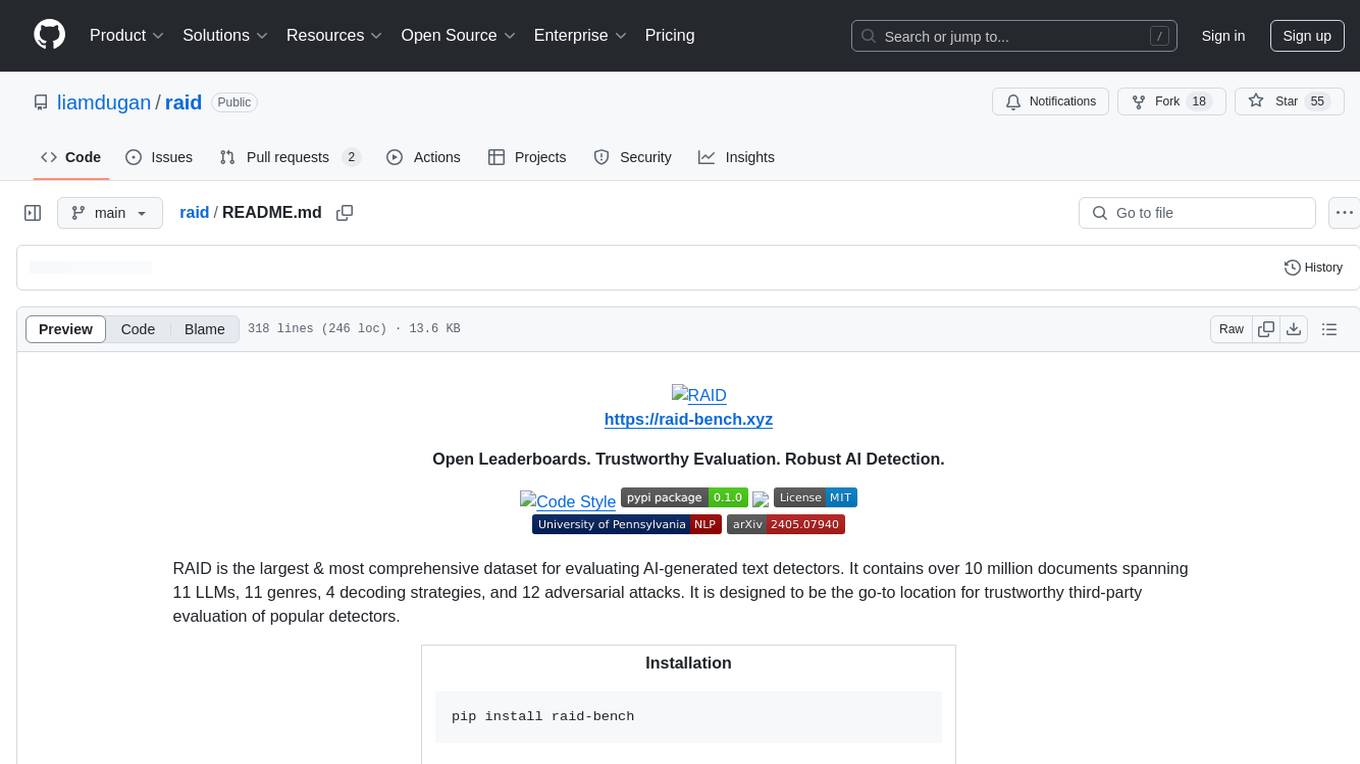
raid
RAID is the largest and most comprehensive dataset for evaluating AI-generated text detectors. It contains over 10 million documents spanning 11 LLMs, 11 genres, 4 decoding strategies, and 12 adversarial attacks. RAID is designed to be the go-to location for trustworthy third-party evaluation of popular detectors. The dataset covers diverse models, domains, sampling strategies, and attacks, making it a valuable resource for training detectors, evaluating generalization, protecting against adversaries, and comparing to state-of-the-art models from academia and industry.
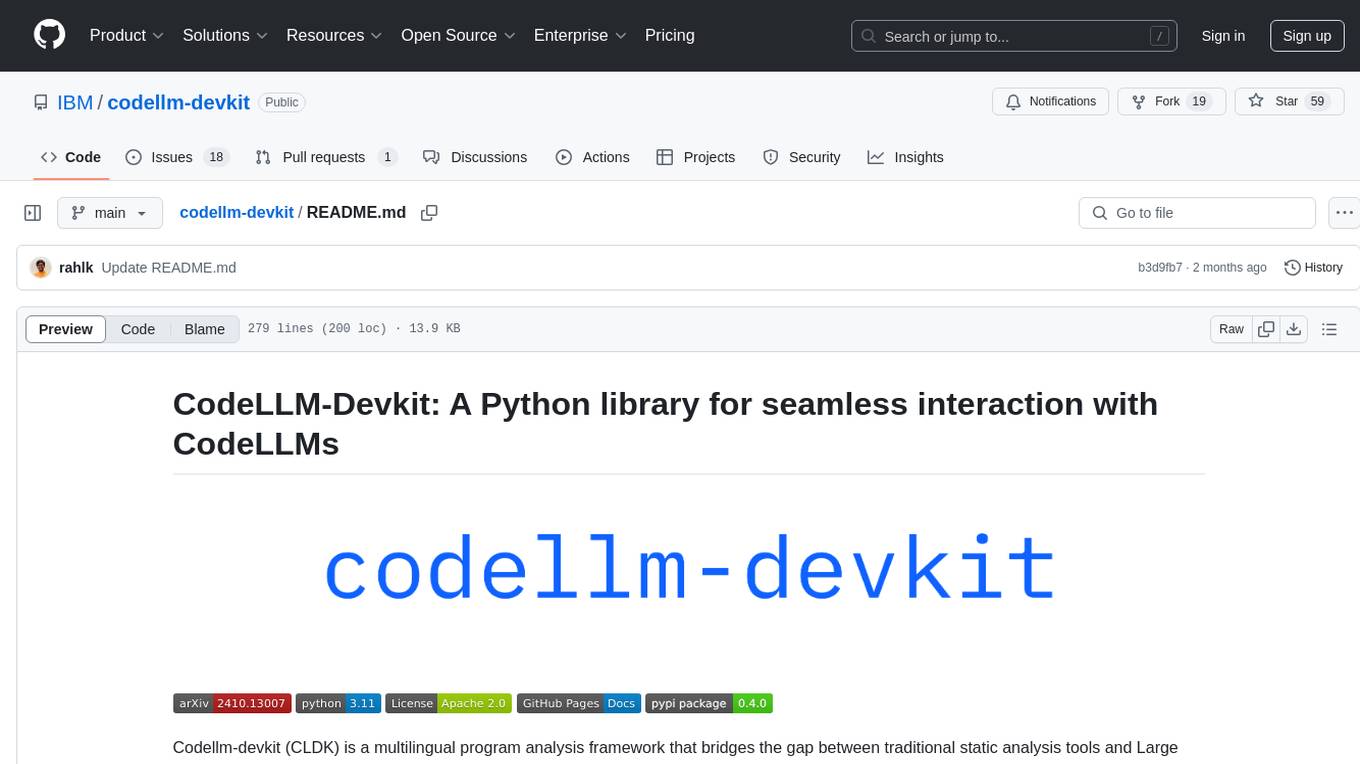
codellm-devkit
Codellm-devkit (CLDK) is a Python library that serves as a multilingual program analysis framework bridging traditional static analysis tools and Large Language Models (LLMs) specialized for code (CodeLLMs). It simplifies the process of analyzing codebases across multiple programming languages, enabling the extraction of meaningful insights and facilitating LLM-based code analysis. The library provides a unified interface for integrating outputs from various analysis tools and preparing them for effective use by CodeLLMs. Codellm-devkit aims to enable the development and experimentation of robust analysis pipelines that combine traditional program analysis tools and CodeLLMs, reducing friction in multi-language code analysis and ensuring compatibility across different tools and LLM platforms. It is designed to seamlessly integrate with popular analysis tools like WALA, Tree-sitter, LLVM, and CodeQL, acting as a crucial intermediary layer for efficient communication between these tools and CodeLLMs. The project is continuously evolving to include new tools and frameworks, maintaining its versatility for code analysis and LLM integration.
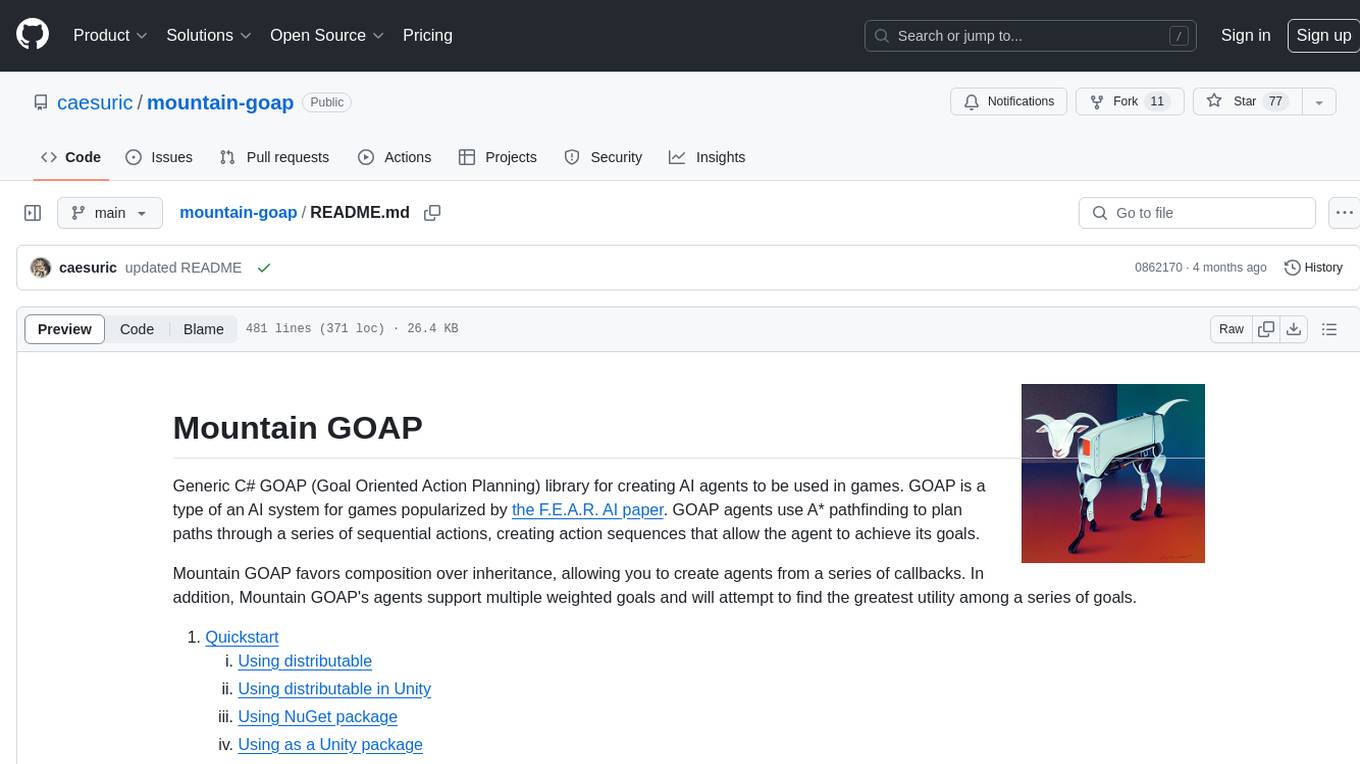
mountain-goap
Mountain GOAP is a generic C# GOAP (Goal Oriented Action Planning) library for creating AI agents in games. It favors composition over inheritance, supports multiple weighted goals, and uses A* pathfinding to plan paths through sequential actions. The library includes concepts like agents, goals, actions, sensors, permutation selectors, cost callbacks, state mutators, state checkers, and a logger. It also features event handling for agent planning and execution. The project structure includes examples, API documentation, and internal classes for planning and execution.
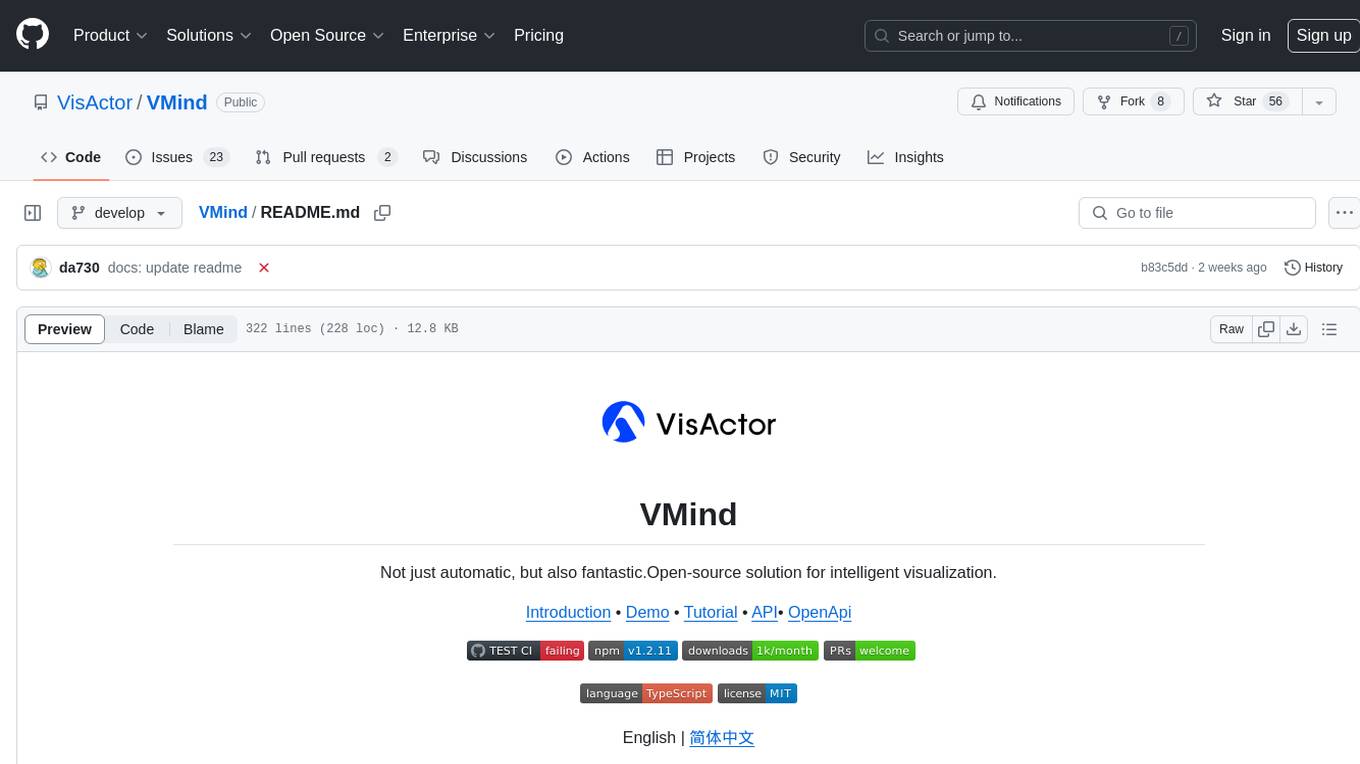
VMind
VMind is an open-source solution for intelligent visualization, providing an intelligent chart component based on LLM by VisActor. It allows users to create chart narrative works with natural language interaction, edit charts through dialogue, and export narratives as videos or GIFs. The tool is easy to use, scalable, supports various chart types, and offers one-click export functionality. Users can customize chart styles, specify themes, and aggregate data using LLM models. VMind aims to enhance efficiency in creating data visualization works through dialogue-based editing and natural language interaction.
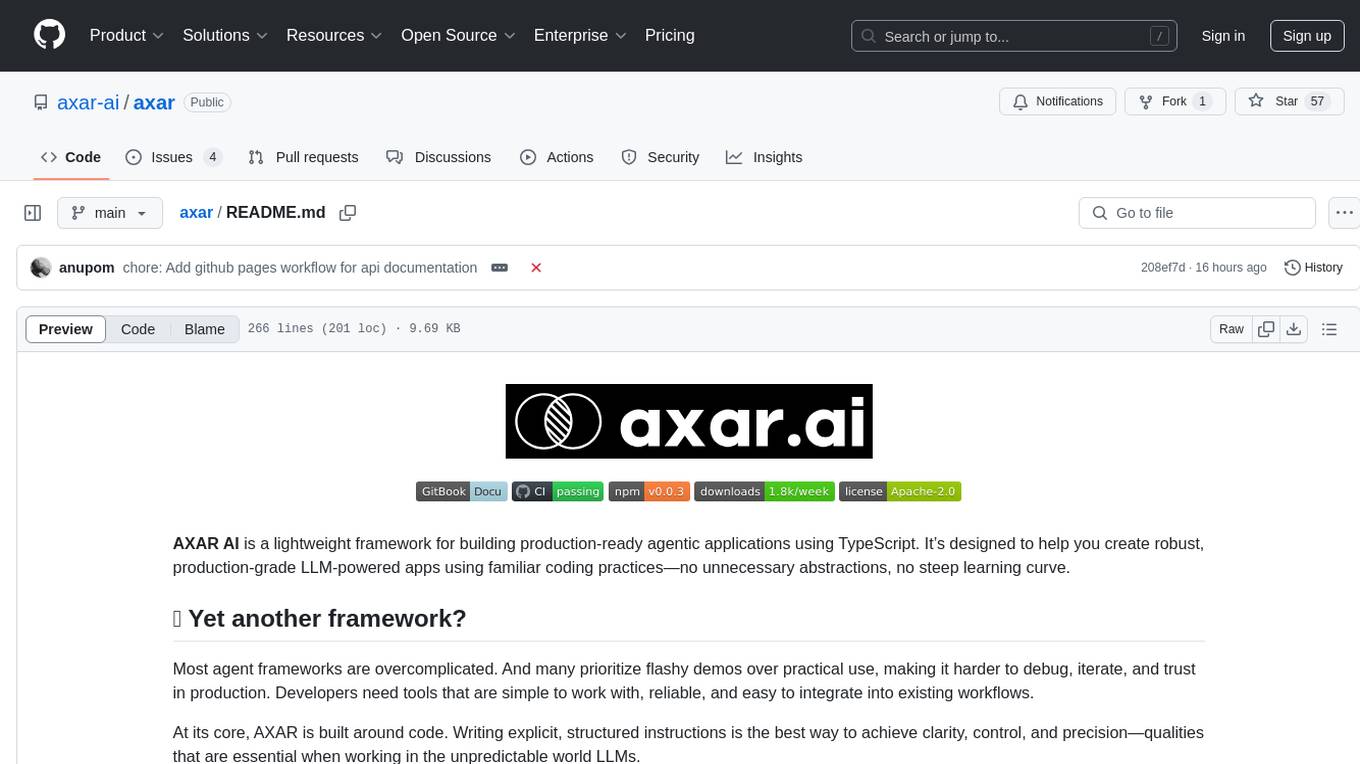
axar
AXAR AI is a lightweight framework designed for building production-ready agentic applications using TypeScript. It aims to simplify the process of creating robust, production-grade LLM-powered apps by focusing on familiar coding practices without unnecessary abstractions or steep learning curves. The framework provides structured, typed inputs and outputs, familiar and intuitive patterns like dependency injection and decorators, explicit control over agent behavior, real-time logging and monitoring tools, minimalistic design with little overhead, model agnostic compatibility with various AI models, and streamed outputs for fast and accurate results. AXAR AI is ideal for developers working on real-world AI applications who want a tool that gets out of the way and allows them to focus on shipping reliable software.
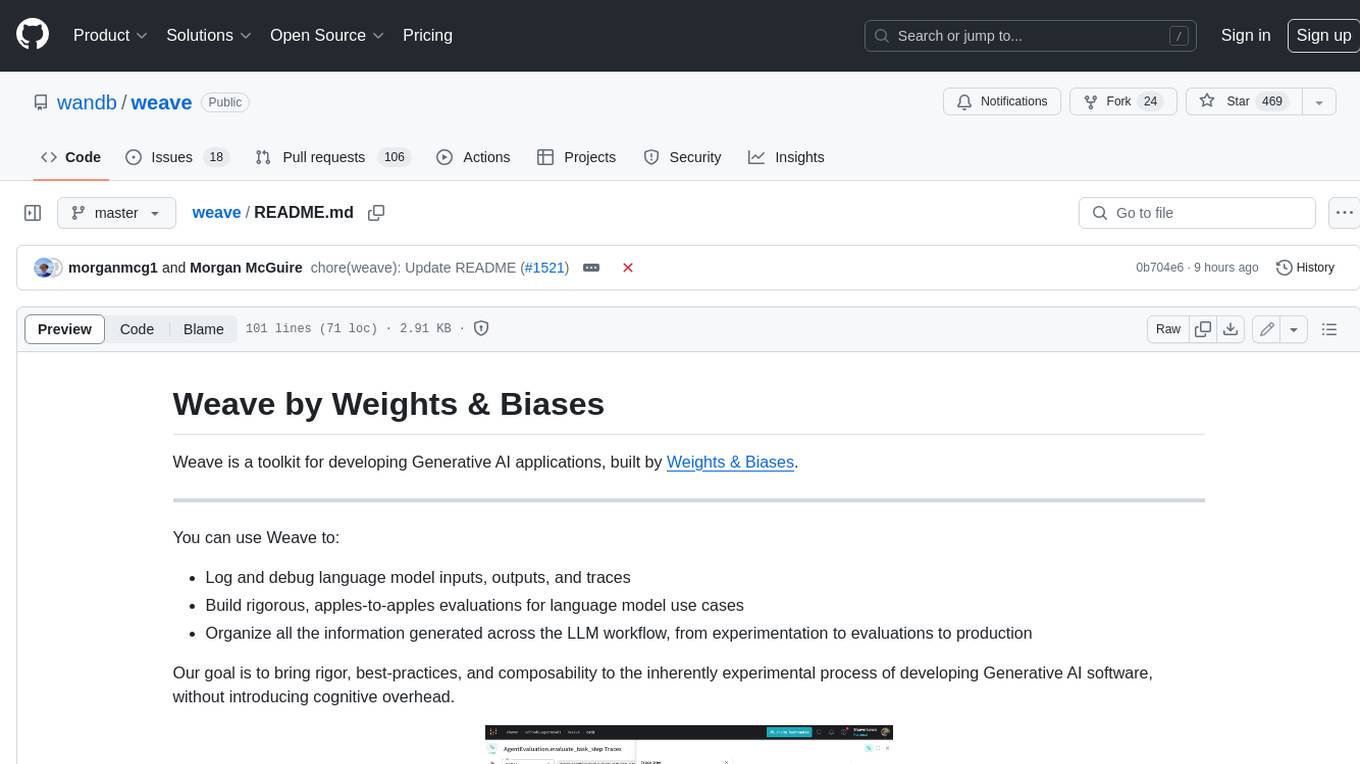
weave
Weave is a toolkit for developing Generative AI applications, built by Weights & Biases. With Weave, you can log and debug language model inputs, outputs, and traces; build rigorous, apples-to-apples evaluations for language model use cases; and organize all the information generated across the LLM workflow, from experimentation to evaluations to production. Weave aims to bring rigor, best-practices, and composability to the inherently experimental process of developing Generative AI software, without introducing cognitive overhead.
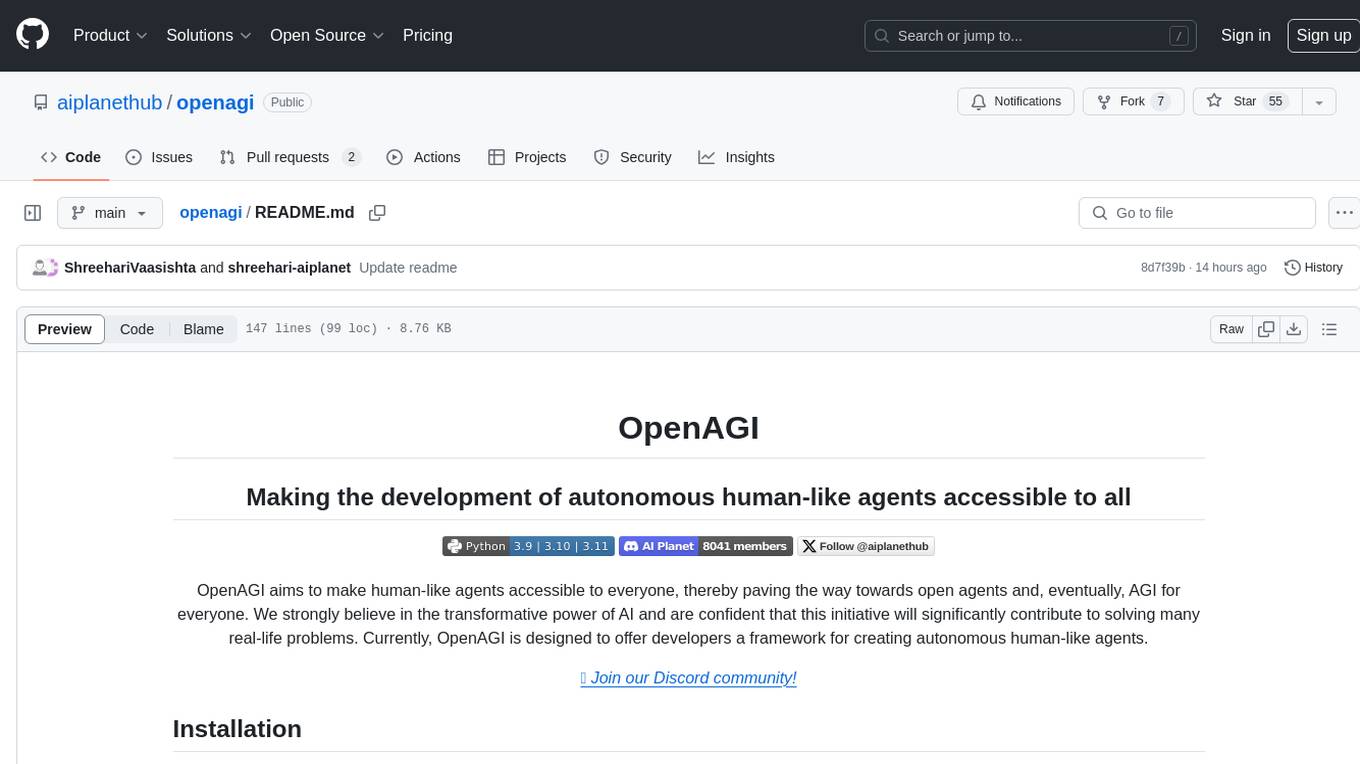
openagi
OpenAGI is a framework designed to make the development of autonomous human-like agents accessible to all. It aims to pave the way towards open agents and eventually AGI for everyone. The initiative strongly believes in the transformative power of AI and offers developers a platform to create autonomous human-like agents. OpenAGI features a flexible agent architecture, streamlined integration and configuration processes, and automated/manual agent configuration generation. It can be used in education for personalized learning experiences, in finance and banking for fraud detection and personalized banking advice, and in healthcare for patient monitoring and disease diagnosis.
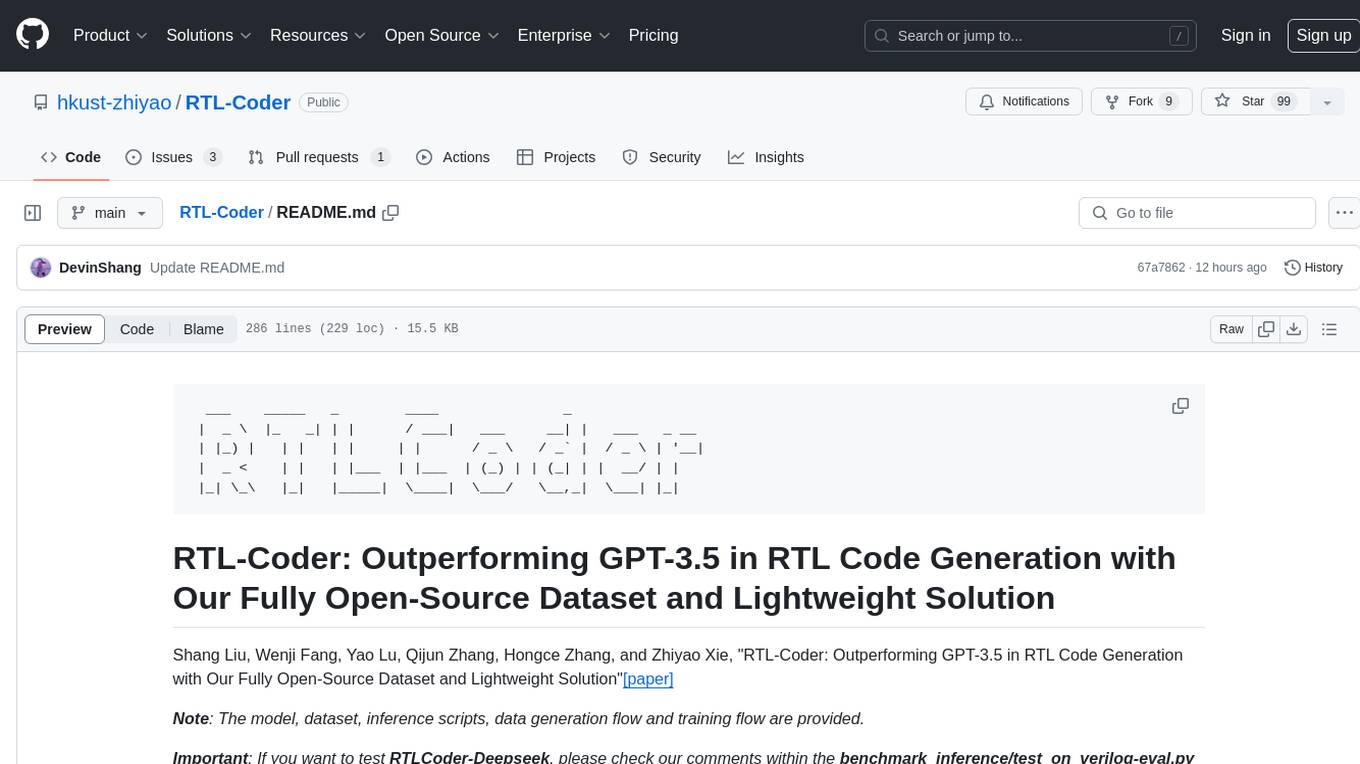
RTL-Coder
RTL-Coder is a tool designed to outperform GPT-3.5 in RTL code generation by providing a fully open-source dataset and a lightweight solution. It targets Verilog code generation and offers an automated flow to generate a large labeled dataset with over 27,000 diverse Verilog design problems and answers. The tool addresses the data availability challenge in IC design-related tasks and can be used for various applications beyond LLMs. The tool includes four RTL code generation models available on the HuggingFace platform, each with specific features and performance characteristics. Additionally, RTL-Coder introduces a new LLM training scheme based on code quality feedback to further enhance model performance and reduce GPU memory consumption.
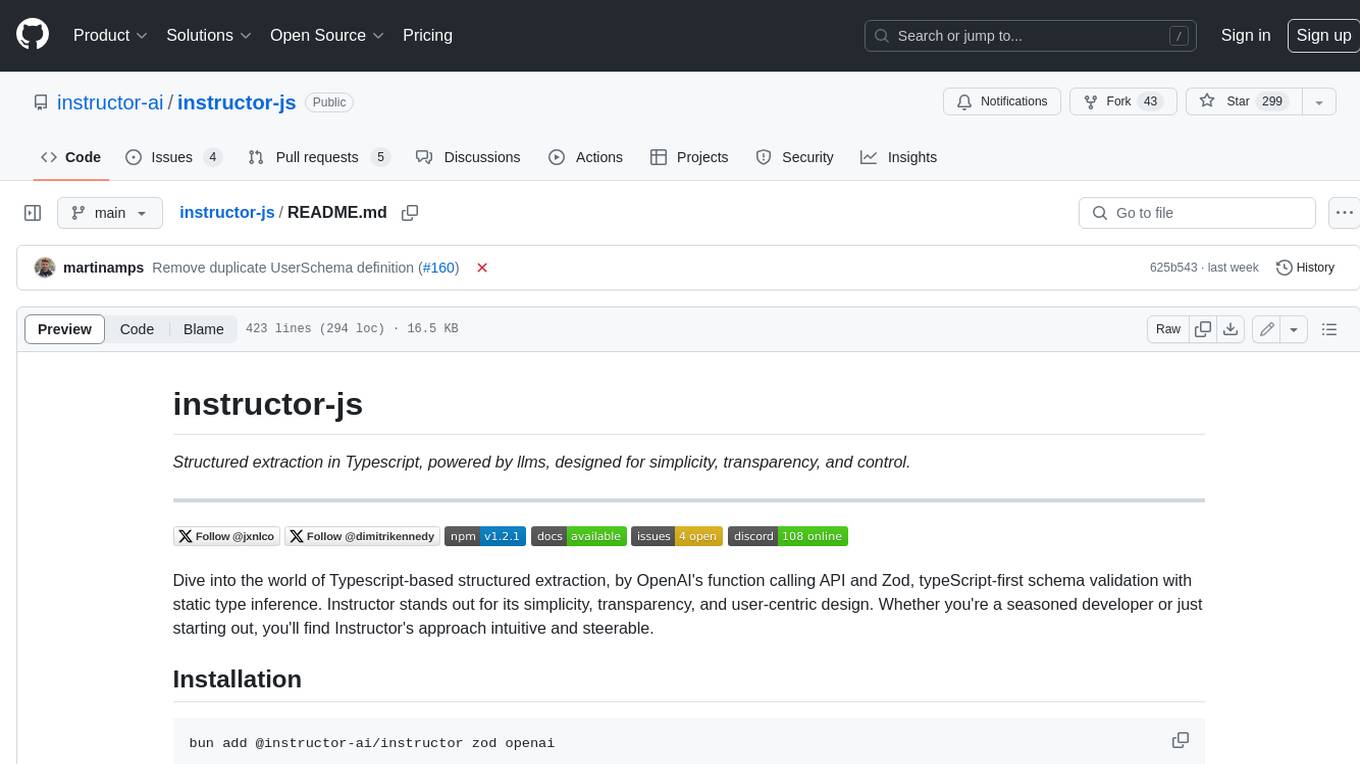
instructor-js
Instructor is a Typescript library for structured extraction in Typescript, powered by llms, designed for simplicity, transparency, and control. It stands out for its simplicity, transparency, and user-centric design. Whether you're a seasoned developer or just starting out, you'll find Instructor's approach intuitive and steerable.
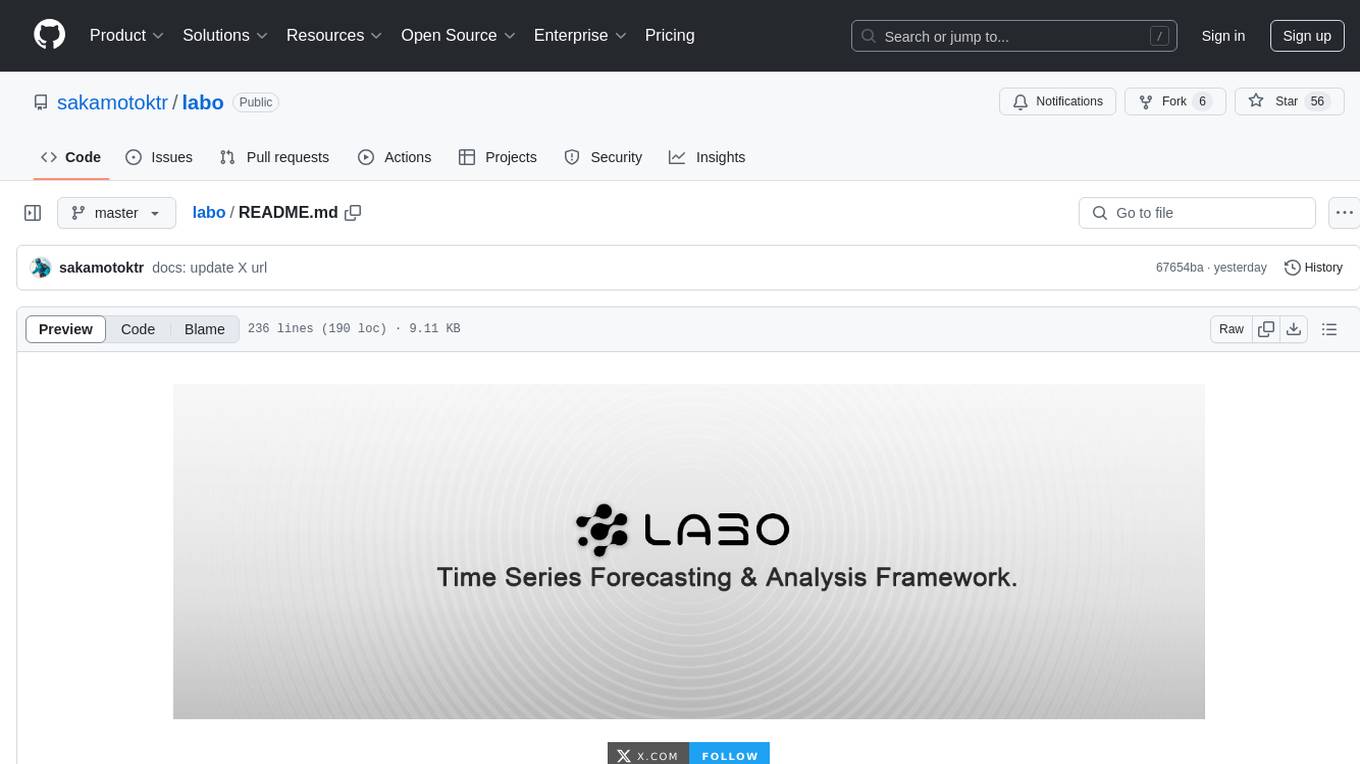
labo
LABO is a time series forecasting and analysis framework that integrates pre-trained and fine-tuned LLMs with multi-domain agent-based systems. It allows users to create and tune agents easily for various scenarios, such as stock market trend prediction and web public opinion analysis. LABO requires a specific runtime environment setup, including system requirements, Python environment, dependency installations, and configurations. Users can fine-tune their own models using LABO's Low-Rank Adaptation (LoRA) for computational efficiency and continuous model updates. Additionally, LABO provides a Python library for building model training pipelines and customizing agents for specific tasks.
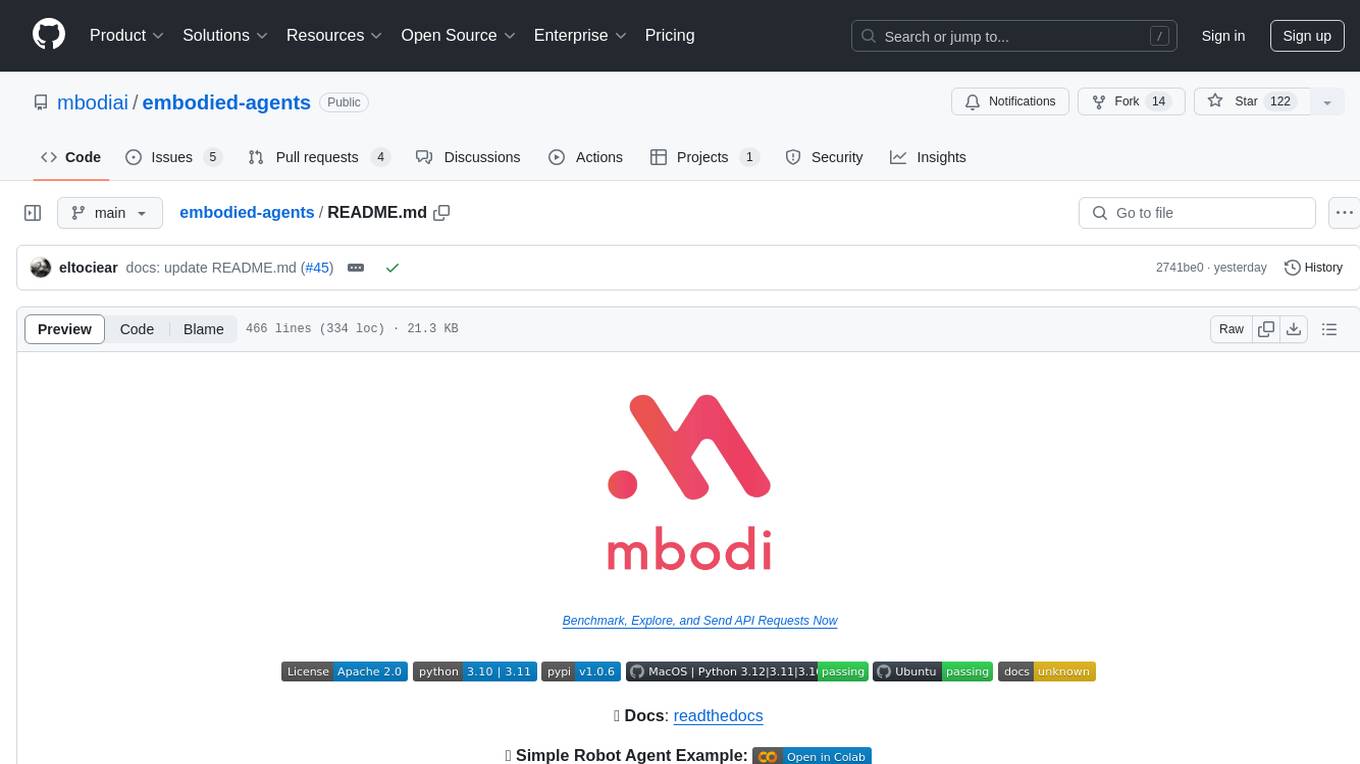
embodied-agents
Embodied Agents is a toolkit for integrating large multi-modal models into existing robot stacks with just a few lines of code. It provides consistency, reliability, scalability, and is configurable to any observation and action space. The toolkit is designed to reduce complexities involved in setting up inference endpoints, converting between different model formats, and collecting/storing datasets. It aims to facilitate data collection and sharing among roboticists by providing Python-first abstractions that are modular, extensible, and applicable to a wide range of tasks. The toolkit supports asynchronous and remote thread-safe agent execution for maximal responsiveness and scalability, and is compatible with various APIs like HuggingFace Spaces, Datasets, Gymnasium Spaces, Ollama, and OpenAI. It also offers automatic dataset recording and optional uploads to the HuggingFace hub.
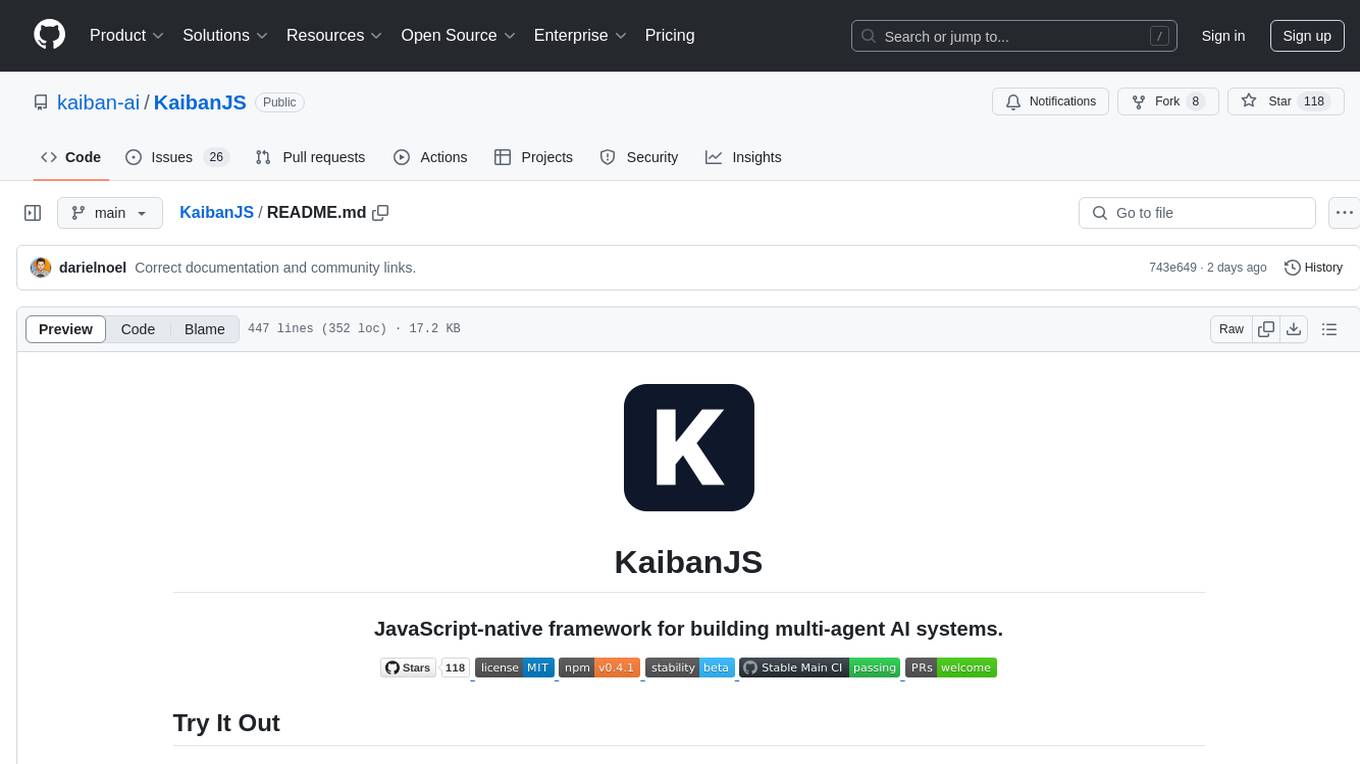
KaibanJS
KaibanJS is a JavaScript-native framework for building multi-agent AI systems. It enables users to create specialized AI agents with distinct roles and goals, manage tasks, and coordinate teams efficiently. The framework supports role-based agent design, tool integration, multiple LLMs support, robust state management, observability and monitoring features, and a real-time agentic Kanban board for visualizing AI workflows. KaibanJS aims to empower JavaScript developers with a user-friendly AI framework tailored for the JavaScript ecosystem, bridging the gap in the AI race for non-Python developers.
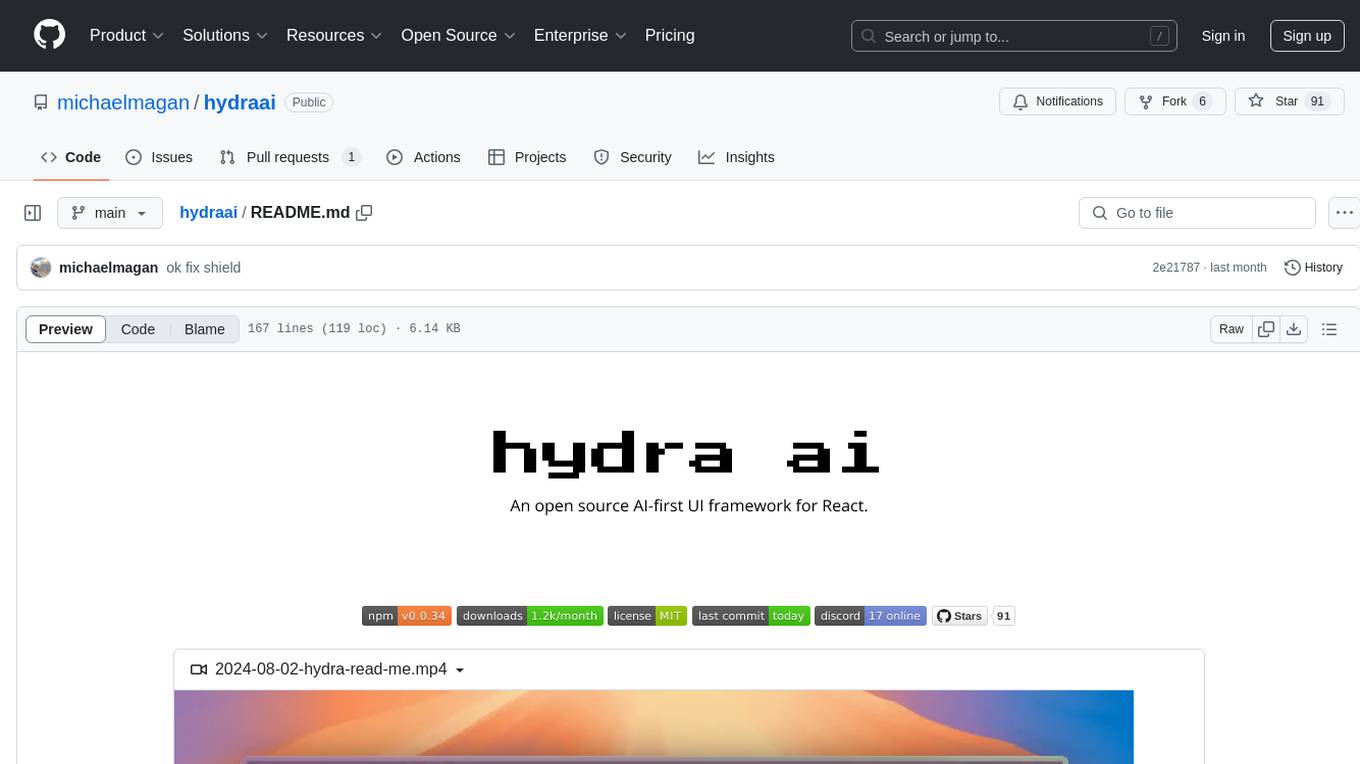
hydraai
Generate React components on-the-fly at runtime using AI. Register your components, and let Hydra choose when to show them in your App. Hydra development is still early, and patterns for different types of components and apps are still being developed. Join the discord to chat with the developers. Expects to be used in a NextJS project. Components that have function props do not work.
For similar tasks
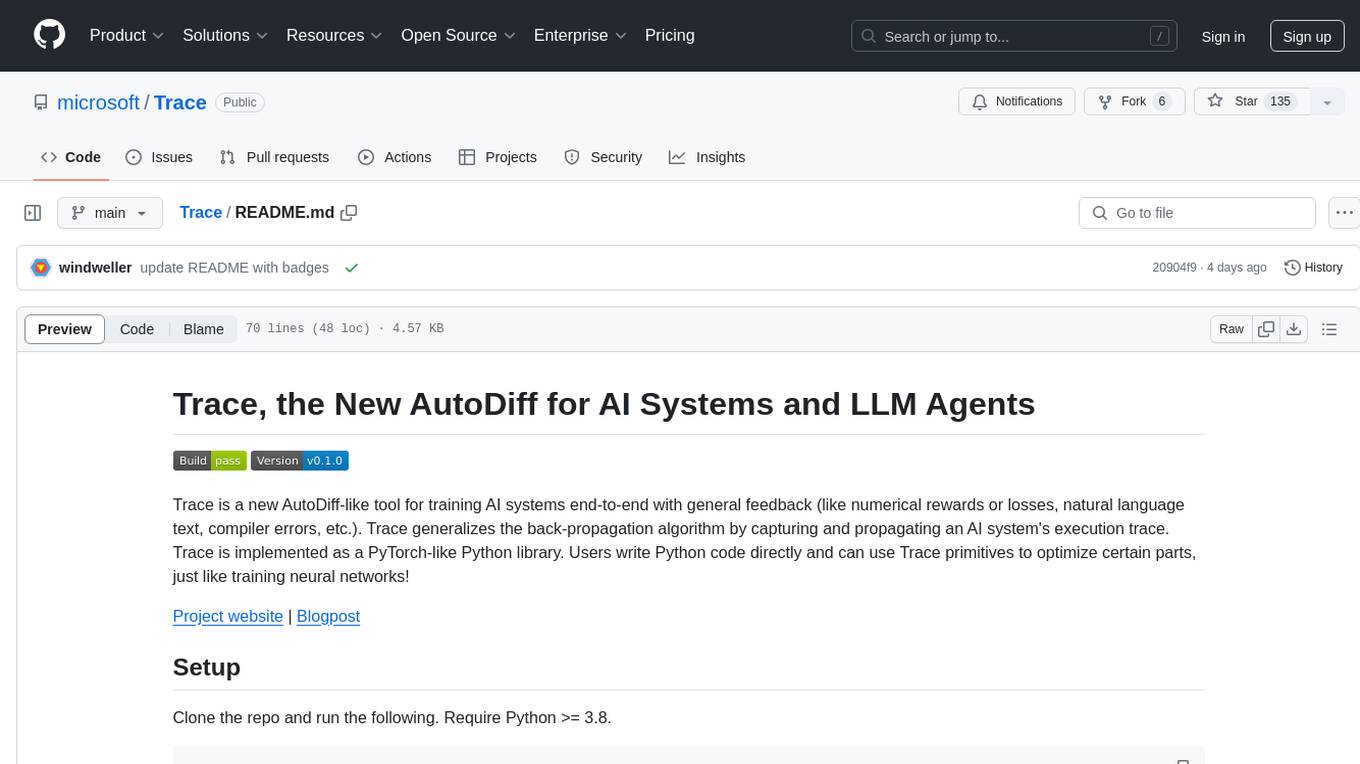
Trace
Trace is a new AutoDiff-like tool for training AI systems end-to-end with general feedback. It generalizes the back-propagation algorithm by capturing and propagating an AI system's execution trace. Implemented as a PyTorch-like Python library, users can write Python code directly and use Trace primitives to optimize certain parts, similar to training neural networks.
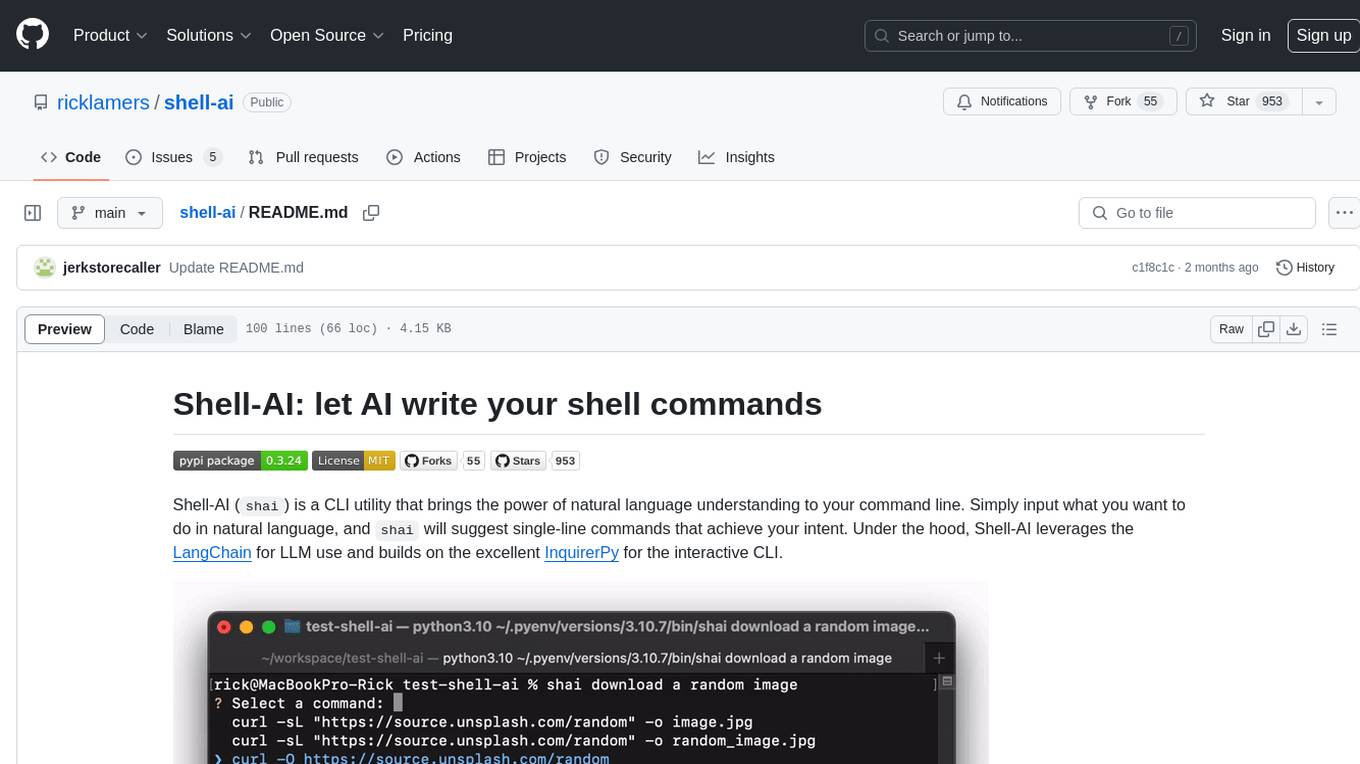
shell-ai
Shell-AI (`shai`) is a CLI utility that enables users to input commands in natural language and receive single-line command suggestions. It leverages natural language understanding and interactive CLI tools to enhance command line interactions. Users can describe tasks in plain English and receive corresponding command suggestions, making it easier to execute commands efficiently. Shell-AI supports cross-platform usage and is compatible with Azure OpenAI deployments, offering a user-friendly and efficient way to interact with the command line.
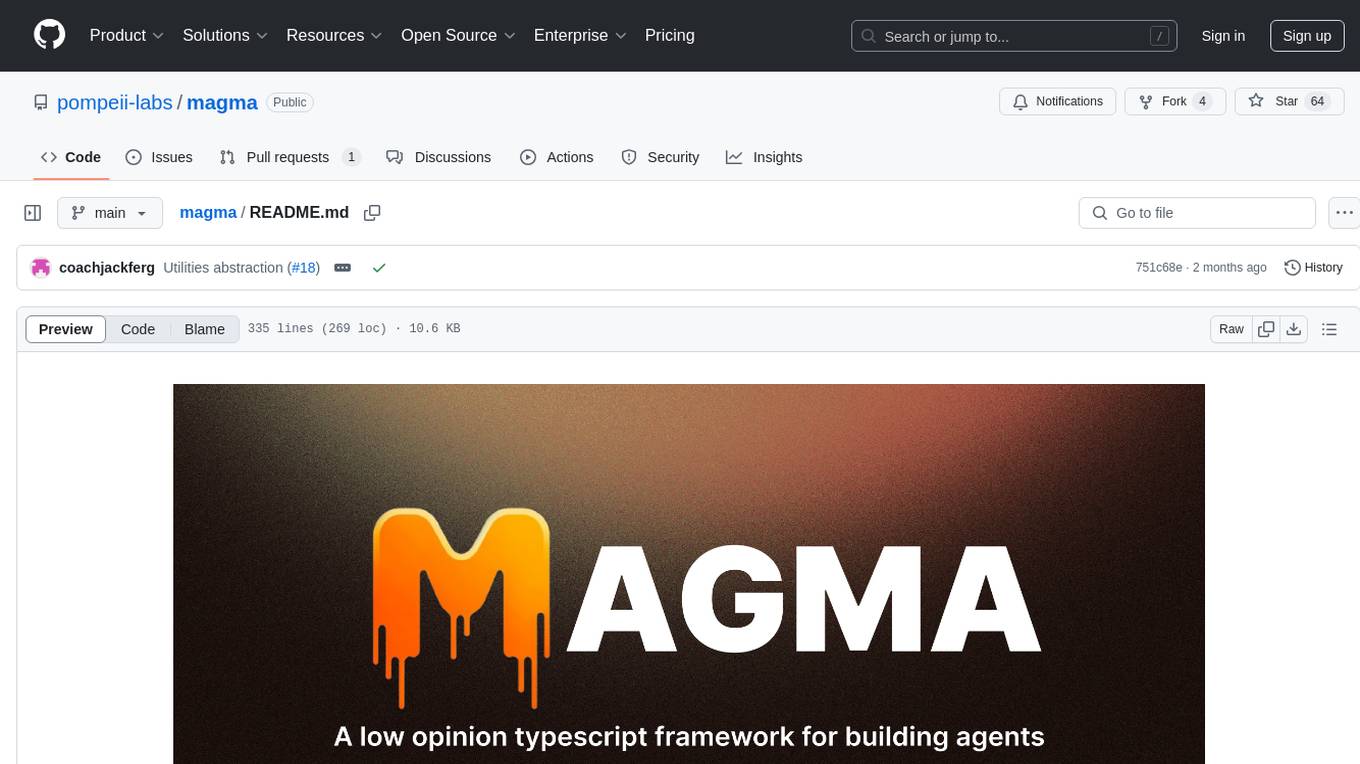
magma
Magma is a powerful and flexible framework for building scalable and efficient machine learning pipelines. It provides a simple interface for creating complex workflows, enabling users to easily experiment with different models and data processing techniques. With Magma, users can streamline the development and deployment of machine learning projects, saving time and resources.
For similar jobs
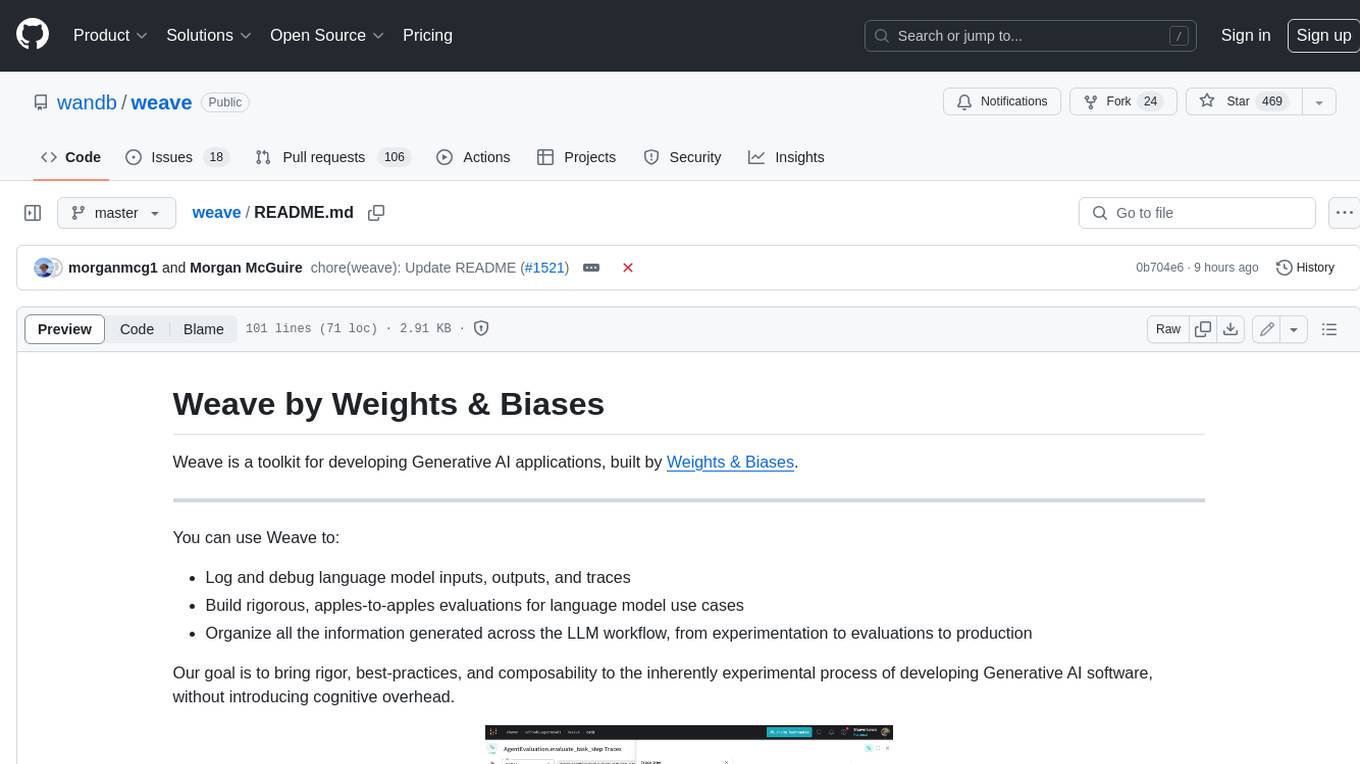
weave
Weave is a toolkit for developing Generative AI applications, built by Weights & Biases. With Weave, you can log and debug language model inputs, outputs, and traces; build rigorous, apples-to-apples evaluations for language model use cases; and organize all the information generated across the LLM workflow, from experimentation to evaluations to production. Weave aims to bring rigor, best-practices, and composability to the inherently experimental process of developing Generative AI software, without introducing cognitive overhead.
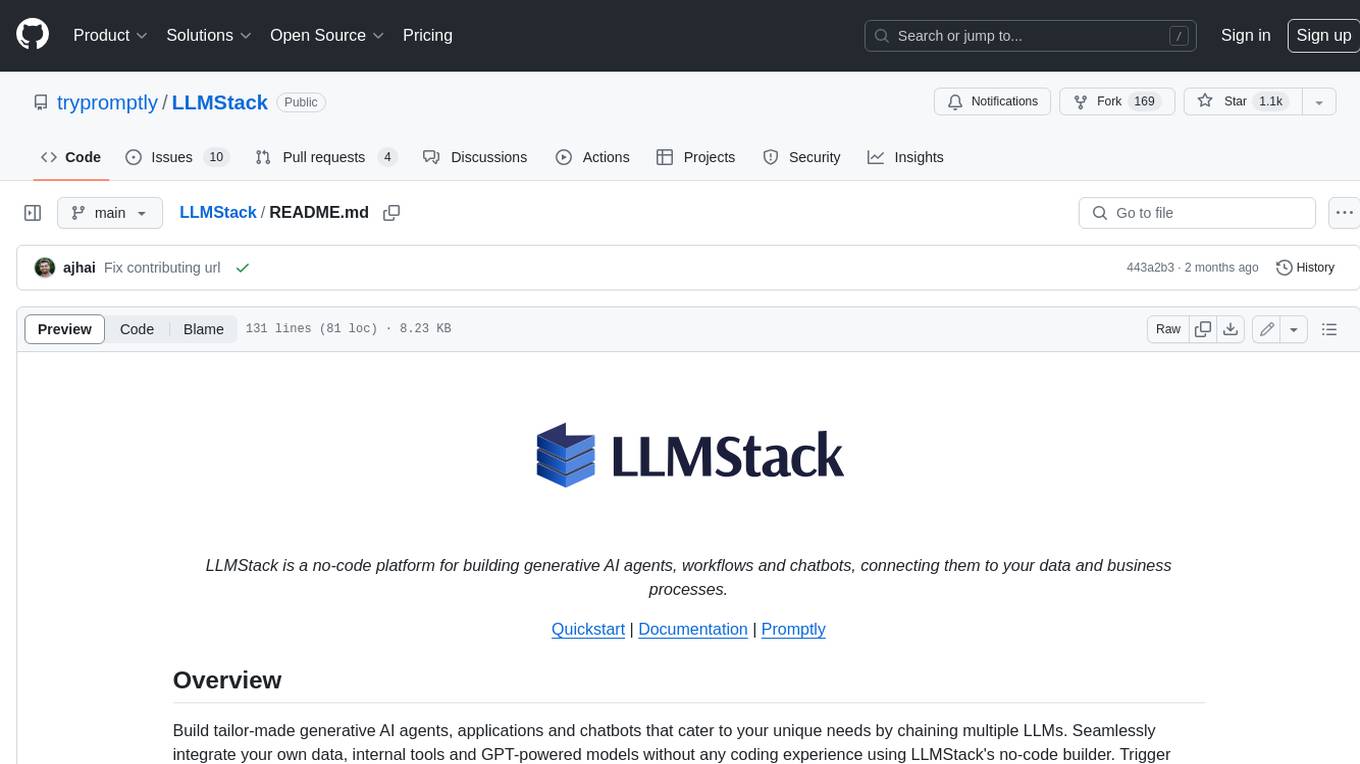
LLMStack
LLMStack is a no-code platform for building generative AI agents, workflows, and chatbots. It allows users to connect their own data, internal tools, and GPT-powered models without any coding experience. LLMStack can be deployed to the cloud or on-premise and can be accessed via HTTP API or triggered from Slack or Discord.
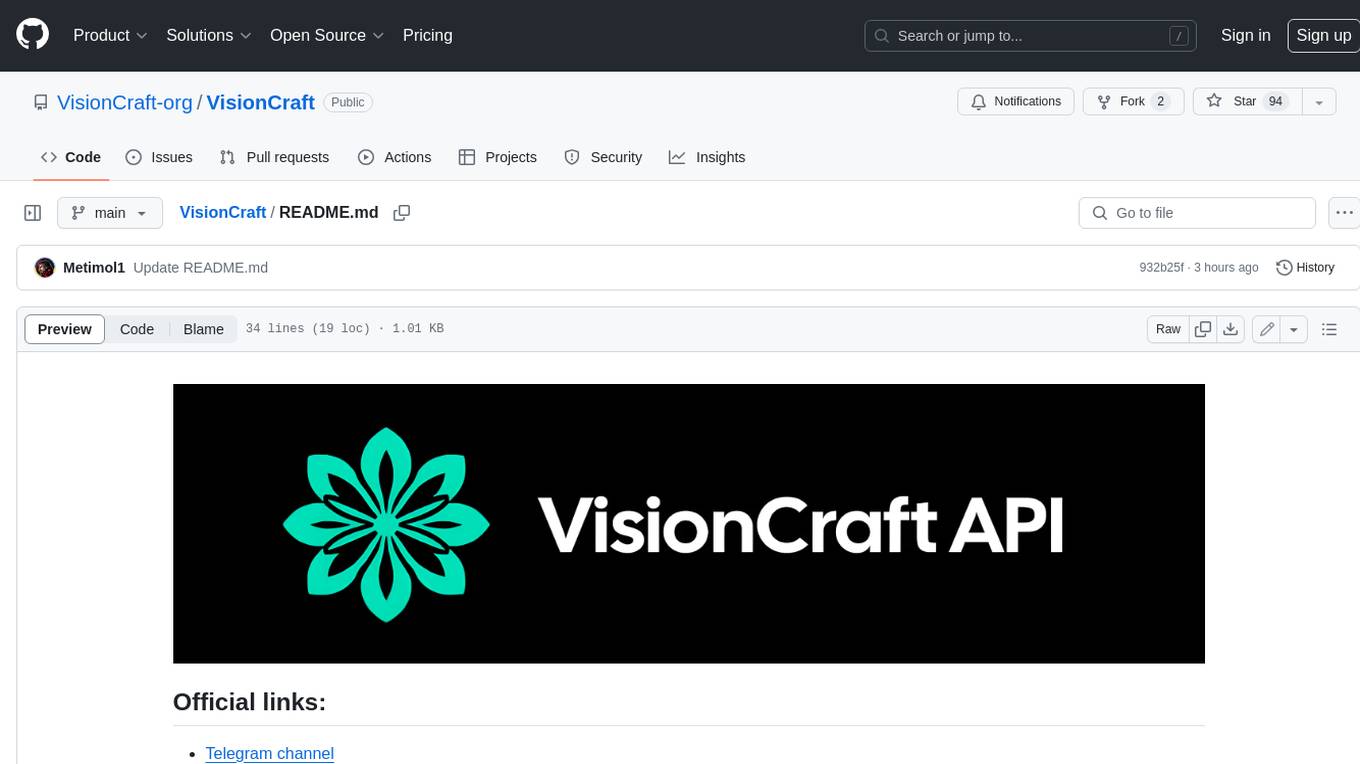
VisionCraft
The VisionCraft API is a free API for using over 100 different AI models. From images to sound.
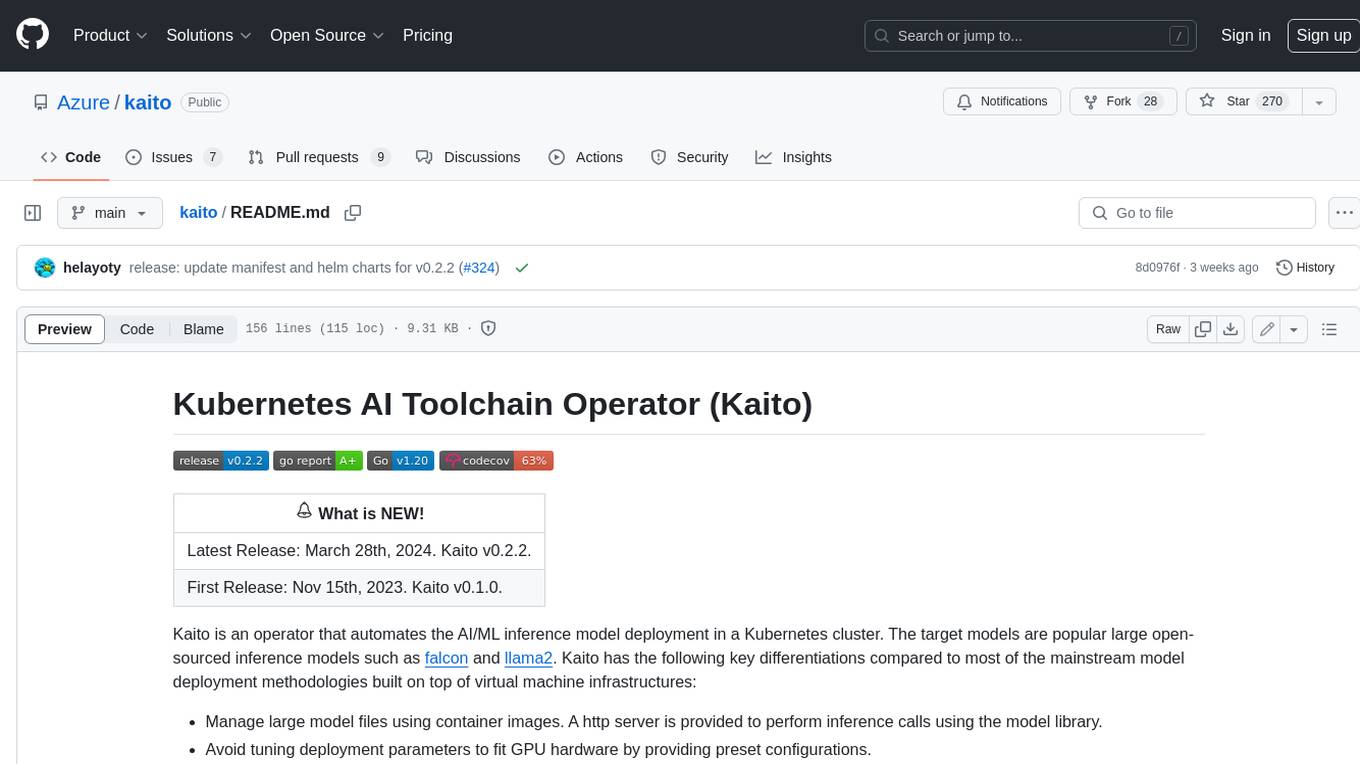
kaito
Kaito is an operator that automates the AI/ML inference model deployment in a Kubernetes cluster. It manages large model files using container images, avoids tuning deployment parameters to fit GPU hardware by providing preset configurations, auto-provisions GPU nodes based on model requirements, and hosts large model images in the public Microsoft Container Registry (MCR) if the license allows. Using Kaito, the workflow of onboarding large AI inference models in Kubernetes is largely simplified.
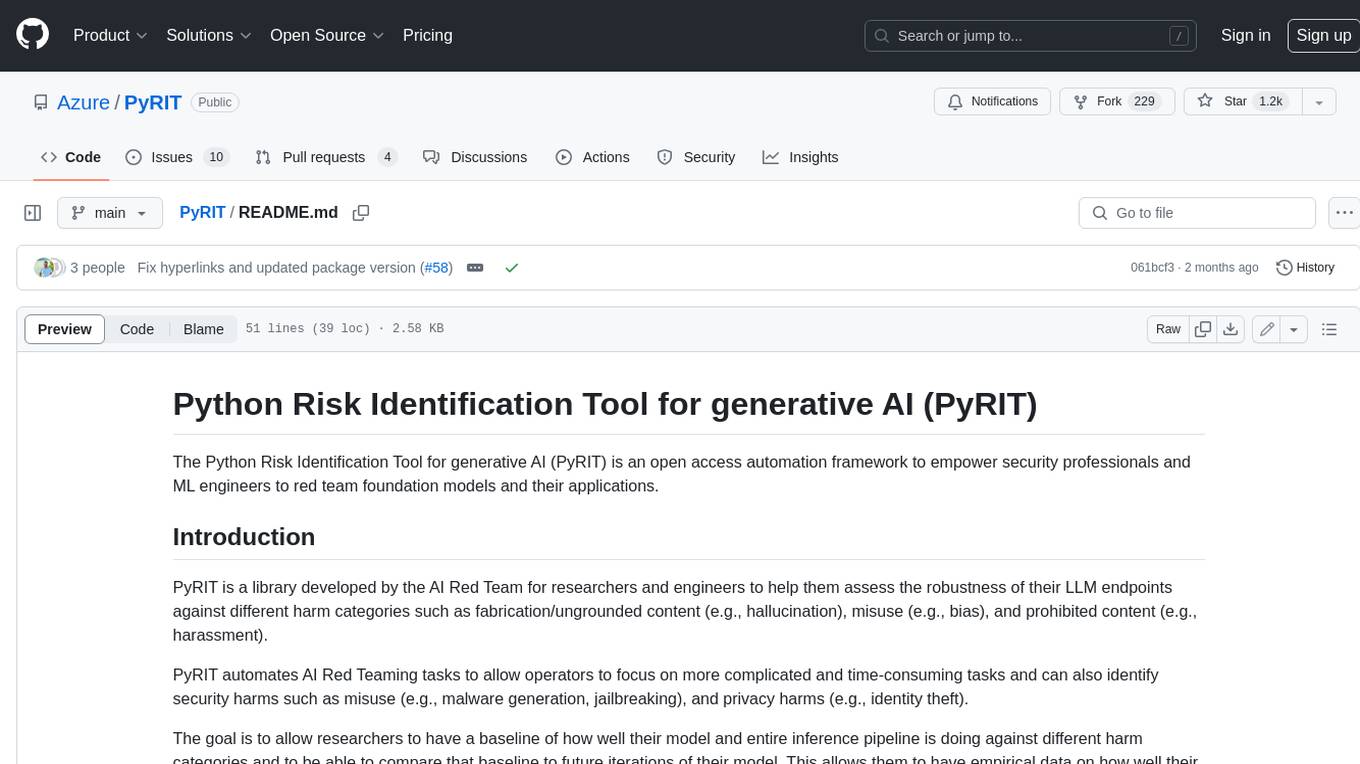
PyRIT
PyRIT is an open access automation framework designed to empower security professionals and ML engineers to red team foundation models and their applications. It automates AI Red Teaming tasks to allow operators to focus on more complicated and time-consuming tasks and can also identify security harms such as misuse (e.g., malware generation, jailbreaking), and privacy harms (e.g., identity theft). The goal is to allow researchers to have a baseline of how well their model and entire inference pipeline is doing against different harm categories and to be able to compare that baseline to future iterations of their model. This allows them to have empirical data on how well their model is doing today, and detect any degradation of performance based on future improvements.
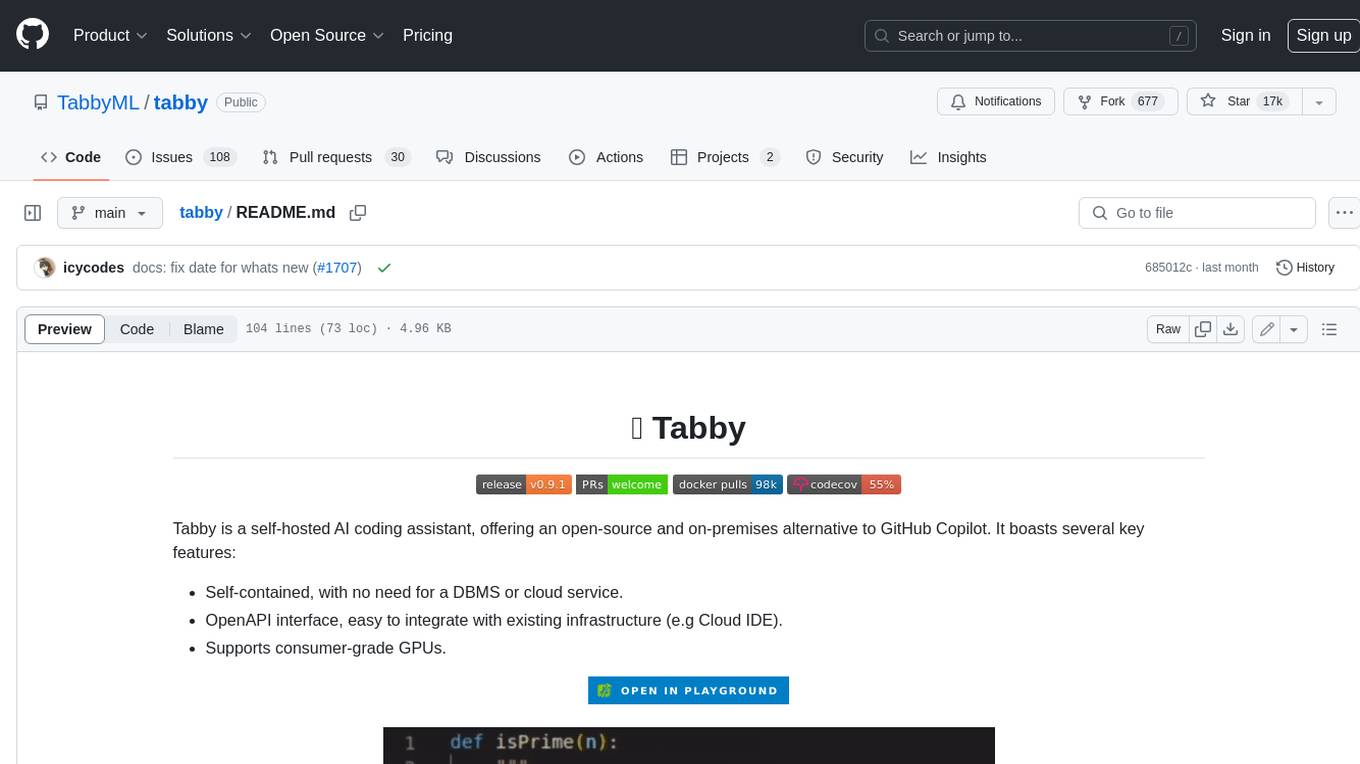
tabby
Tabby is a self-hosted AI coding assistant, offering an open-source and on-premises alternative to GitHub Copilot. It boasts several key features: * Self-contained, with no need for a DBMS or cloud service. * OpenAPI interface, easy to integrate with existing infrastructure (e.g Cloud IDE). * Supports consumer-grade GPUs.
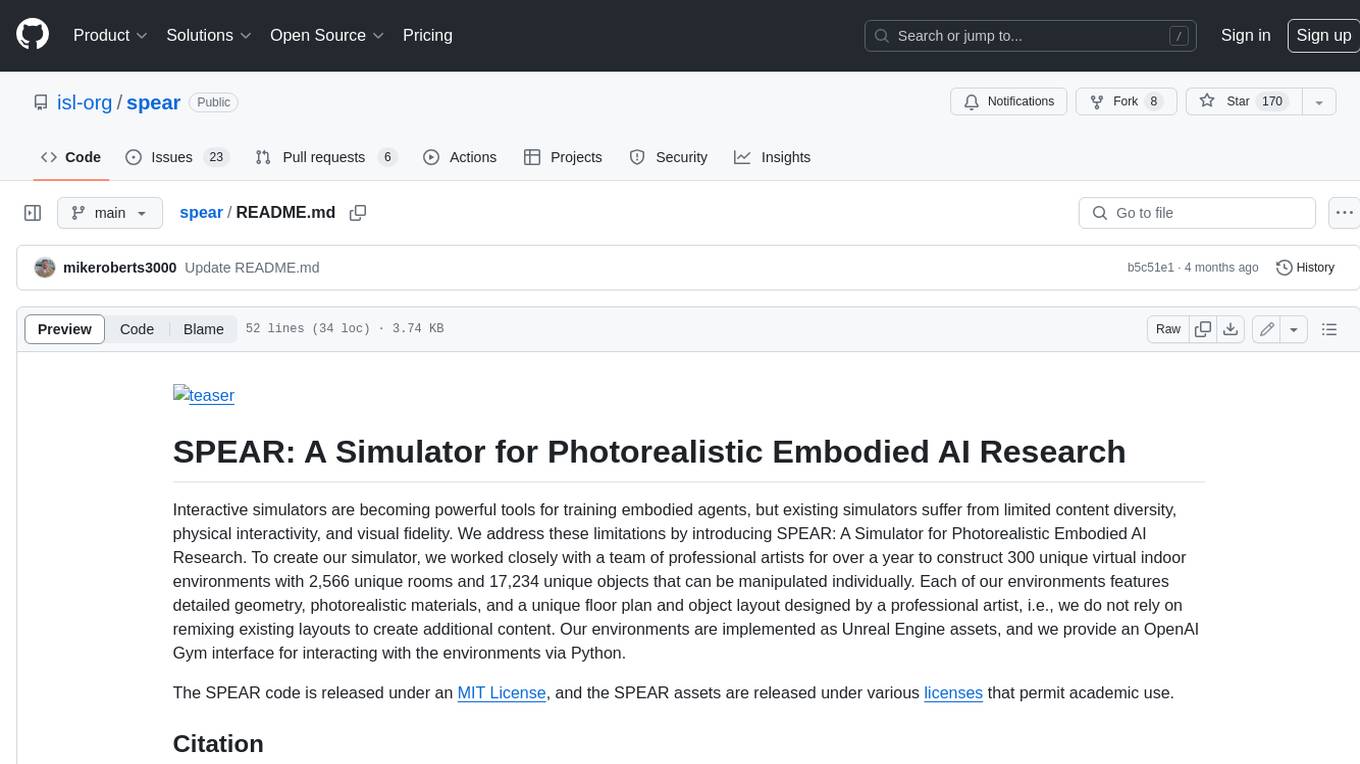
spear
SPEAR (Simulator for Photorealistic Embodied AI Research) is a powerful tool for training embodied agents. It features 300 unique virtual indoor environments with 2,566 unique rooms and 17,234 unique objects that can be manipulated individually. Each environment is designed by a professional artist and features detailed geometry, photorealistic materials, and a unique floor plan and object layout. SPEAR is implemented as Unreal Engine assets and provides an OpenAI Gym interface for interacting with the environments via Python.
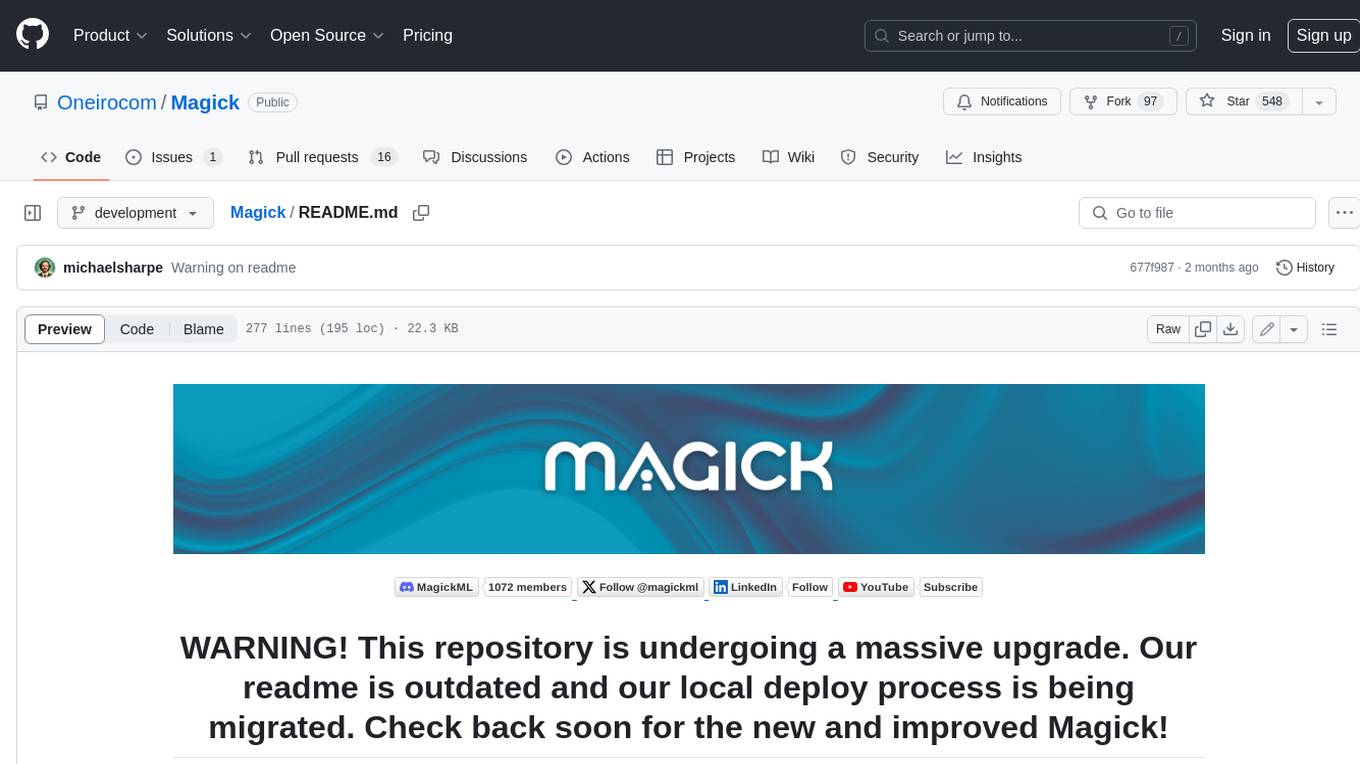
Magick
Magick is a groundbreaking visual AIDE (Artificial Intelligence Development Environment) for no-code data pipelines and multimodal agents. Magick can connect to other services and comes with nodes and templates well-suited for intelligent agents, chatbots, complex reasoning systems and realistic characters.