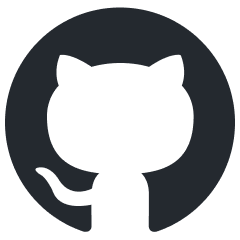
mountain-goap
A GOAP (Goal Oriented Action Planning) AI library, written in C#.
Stars: 77
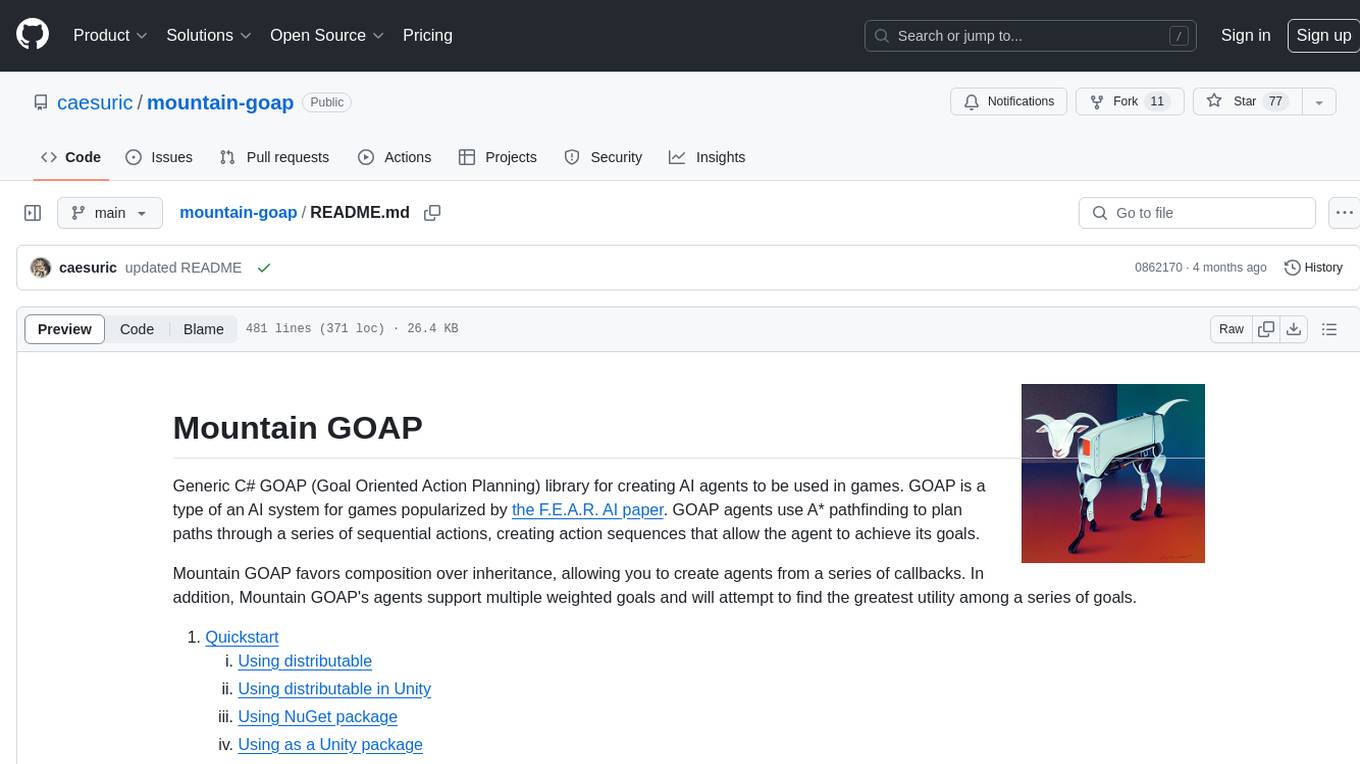
Mountain GOAP is a generic C# GOAP (Goal Oriented Action Planning) library for creating AI agents in games. It favors composition over inheritance, supports multiple weighted goals, and uses A* pathfinding to plan paths through sequential actions. The library includes concepts like agents, goals, actions, sensors, permutation selectors, cost callbacks, state mutators, state checkers, and a logger. It also features event handling for agent planning and execution. The project structure includes examples, API documentation, and internal classes for planning and execution.
README:
Generic C# GOAP (Goal Oriented Action Planning) library for creating AI agents to be used in games. GOAP is a type of an AI system for games popularized by the F.E.A.R. AI paper. GOAP agents use A* pathfinding to plan paths through a series of sequential actions, creating action sequences that allow the agent to achieve its goals.
Mountain GOAP favors composition over inheritance, allowing you to create agents from a series of callbacks. In addition, Mountain GOAP's agents support multiple weighted goals and will attempt to find the greatest utility among a series of goals.
- Quickstart
- Concepts & API
- Events
- Logger
- Examples
- Project structure
- Roadmap
- Other open source GOAP projects
- License Acknowledgements
Download the release, unzip, and include the DLL in your project. In Visual Studio, you can do this by right-clicking on "Dependencies" in the Solution Explorer, then clicking "Add COM Reference," clicking "Browse," and browsing to the DLL.
Download the release, unzip to a folder, and drag the folder into your Unity project.
If you are not using Unity, you can download and use MountainGoap as a NuGet package.
Right click your package and click "Manage NuGet Packages," then search for "MountainGoap" and install the package.
In the works.
Clone the repo and copy the code in the MountainGoap folder to your repo.
No matter which method of installation you use, you can access MountainGoap by using the MountainGoap
namespace as a prefix to the library classes, or by including the following line in your code:
using MountainGoap;
Agents are indivdual entities that act within your game or simulated world. The simplest example of instantiating an agent is this:
Agent agent = new Agent();
In practice, you will want to pass the agent constructor various things it needs to make a functional agent. Read on to understand what kinds of objects you should pass your agents.
When you want your agent to act, just call the following:
agent.Step();
What kind of timeframe is represented by a "step" will vary based on your engine. In a turn based game, a step might be one turn. In a realtime engine like Unity, you might call agent.Step()
on every Update()
cycle. In a turn-based game you will probably want to call agent.Step()
on every turn. In the case of turn-based games, you can force at least one action to be taken per turn by calling agent.Step(mode: StepMode.OneAction)
. If you want the entire action sequence to be completed in one turn, you can call agent.Step(mode: StepMode.AllActions)
.
For additional granularity, you can also call the functions agent.Plan()
, agent.ClearPlan()
, agent.PlanAsync()
, and agent.ExecutePlan()
. See the full API docs for additional details.
The agent stores a dictionary of objects called its state. This state can include anything, but simple values work best with goals and actions. If you need to reference complex game state, however, this is not a problem -- sensors, covered below, can be used to translate complex values like map states into simpler ones, like booleans. More on that below.
State can be passed into the agent constructor, like so:
Agent agent = new Agent(
state: new Dictionary<string, object> {
{ "nearOtherAgent", false },
{ "otherAgents", new List<Agent>() }
}
);
Goals dictate the state values that the agent is trying to achieve. Goals have relatively simple constructors, taking just a dictionary of keys and values the agent wants to see in its state and a weight that indicates how important the goal is. The higher the weight, the more important the goal.
Goals can be passed into the agent constructor, like so:
Goal goal = new Goal(
desiredState: new Dictionary<string, object> {
{ "nearOtherAgent", true }
},
weight: 2f
);
Agent agent = new Agent(
goals: new List<Goal> {
goal
}
);
Extreme goals attempt to maximize or minimize a numeric state value. They take similar parameters to normal goals, but the values in the dictionary must be booleans. If the boolean is true, the goal will attempt to maximize the value. If the boolean is false, the goal will attempt to minimize the value. The state value must be a numeric type for this to work correctly.
Example that will try to maximize the agent's health:
ExtremeGoal goal = new ExtremeGoal(
desiredState: new Dictionary<string, object> {
{ "health", true }
},
weight: 2f
);
Comparative goals attempt to make a numeric state value compare in a certain way to a base value. They take similar parameters to normal goals, but the values in the dictionary must be ComparisonValuePair objects. The ComparisonValuePair object specifies a value to use for comparison and a comparison operator to use. The state value must be a numeric type and the same type as the comparison value for this to work correctly.
Example that will try to make the agent's health greater than 50:
ComparativeGoal goal = new ComparativeGoal(
desiredState: new Dictionary<string, object> {
{ "health", new ComparisonValuePair {
Value = 50,
Operator = ComparisonOperator.GreaterThan
} }
},
weight: 2f
);
Actions dictate arbitrary code the agent can execute to affect the world and achieve its goals. Each action, when it runs, will execute the code passed to it, which is called the action executor. Actions can also have preconditions, state values required before the agent is allowed to execute the action, and postconditions, which are values the state is expected to hold if the action is successful. Finally, each action has a cost, which is used in calculating the best plan for the agent.
Actions return an ExecutionStatus
enum to say if they succeeded or not. If they succeed, the postconditions will automatically be set to the values passed to the action constructor. If the ExecutionStatus
returned is ExecutionStatus.Executing
, the action will be considered in progress, and the executor will be called again on the next Step command.
Actions can be passed into the agent constructor, like so:
Action giveHugAction = new Action(
executor: (Agent agent, Action action) => {
Console.WriteLine("hugged someone");
return ExecutionStatus.Succeeded;
},
preconditions: new Dictionary<string, object> {
{ "nearOtherAgent", true }
},
postconditions: new Dictionary<string, object> {
{ "wasHugged", true }
},
cost: 0.5f
);
Agent agent = new Agent(
actions: new List<Action> {
giveHugAction
}
);
Comparative preconditions are preconditions that are calculated by comparing a state value to a target value. They take similar parameters to normal preconditions, but the values in the dictionary must be ComparisonValuePair objects.
Example where energy must be greater than zero to walk:
Action walk = new Action(
executor: (Agent agent, Action action) => {
Console.WriteLine("I'm walkin' here!");
return ExecutionStatus.Succeeded;
},
comparativePreconditions: new() {
{ "energy", new() { Operator = ComparisonOperator.GreaterThan, Value = 0 } }
},
);
Arithmetic postconditions are postconditions that are calculated by performing arithmetic on other state values. They take similar parameters to normal postconditions, but the values in the dictionary are added to the existing state value instead of replacing it. Note that the state value and the postcondition value must be of the same numeric type for this to work correctly.
Example that will add 10 to the agent's health as a postcondition:
Action heal = new Action(
executor: (Agent agent, Action action) => {
Console.WriteLine("healed for 10 hp");
return ExecutionStatus.Succeeded;
},
arithmeticPostconditions: new Dictionary<string, object> {
{ "health", 10 }
},
cost: 0.5f
);
Note that normal Goals use simple equality checks and cannot tell that a value is closer or further away from the goal value. If you want to use an arithmetic postcondition and have the library detect that you are moving closer to your goal, use Comparative Goals or Extreme Goals. Both of these types of numerically based goals will calculate distance from a numeric goal value properly.
Parameter postconditions are postconditions that copy one of the parameters passed to the action into the agent state. The structure uses a dictionary of string keys and string values, where the keys are the keys to the parameter value being copied and the values are the state key into which you are copying the value.
Example that will copy the "target" parameter into the "target" state value:
var targets = new List<string> { "target1", "target2" }
Action setTarget = new Action(
executor: (Agent agent, Action action) => {
Console.WriteLine("set target");
return ExecutionStatus.Succeeded;
},
permutationSelectors: new() {
{ "target", PermutationSelectorGenerators.SelectFromCollection(targets) }
},
parameterPostconditions: new Dictionary<string, string> {
{ "target", "target" }
},
cost: 0.5f
);
This example will create permutations of the action that either target "target1"
or "target2"
, and will copy the selected target into the "target"
state value. See permutation selectors for more information on permutation selectors.
Sensors allow an agent to distill information into their state, often derived from other state values. Sensors execute on every Step()
call, and use a sensor handler to execute code. Sensors can be passed into the agent constructor, like so:
Sensor agentProximitySensor = new Sensor(
(Agent agent) => {
if (AgentNearOtherAgent(agent)) agent.State["nearOtherAgent"] = true;
else agent.State["nearOtherAgent"] = false;
}
);
Agent agent = new Agent(
sensors: new List<Sensor> {
agentProximitySensor
}
);
Finally, actions can be constructed with permutation selectors, which will instantiate multiple copies of the action with different parameters for purposes such as target selection. The library comes with some default permutation selector generators, or you can write your own as callbacks. For instance, if you want an action to be evaluated separately with each member of a list as a potential parameter, you would construct the action as so:
Action myAction = new Action(
permutationSelectors: new Dictionary<string, PermutationSelectorCallback> {
{ "target1", PermutationSelectorGenerators.SelectFromCollectionInState<Agent>("otherAgents") },
{ "target2", PermutationSelectorGenerators.SelectFromCollectionInState<Agent>("otherAgents") }
},
executor: (Agent agent, Action action) => {
Console.WriteLine(action.GetParameter("target1").ToString());
Console.WriteLine(action.GetParameter("target2").ToString());
}
);
The code above will create an action that when evaluated for execution in an agent plan will be considered once for every pair combination of elements in the "otherAgents" collection of the agent state, one for target1
, and one for target2
. In order to take advantage of this feature, you can also calculate variable costs based on action parameters using the costCallback
argument in the Action
constructor. See cost callbacks for more information.
Cost callbacks allow you to calculate the cost of an action based on its parameters. This is useful for actions that have variable costs based on the parameters passed to them. For instance, if you have an action that moves the agent to a target, you might want to calculate the cost of the action based on the distance to the target. You can do this by passing a cost callback to the action constructor:
Action moveToTarget = new Action(
executor: (Agent agent, Action action) => {
Console.WriteLine("moved to target");
return ExecutionStatus.Succeeded;
},
costCallback: (action, state) => {
if (action.GetParameter("target") is Agent target) {
var distance = GetDistance(this, target);
return distance;
}
else return float.MaxValue;
}
);
State mutators allow you to mutate the agent state during execution or evaluation of an action. This is useful for actions that have more complex side effects on agent state. For instance, if you have an action that moves the agent to a target, you might want to mutate the agent state to reflect the new position of the agent. You can do this by passing a state mutator to the action constructor:
Action moveToTarget = newAction(
executor: (Agent agent, Action action) => {
Console.WriteLine("moved to target");
return ExecutionStatus.Succeeded;
},
stateMutator: (action, state) => {
if (action.GetParameter("target") is Agent target) {
state["position"] = target.State["position"];
}
}
)
State checkers allow you to check agent state programmatically during execution or evaluation of an action. This is useful for actions that have more complex preconditions than simple equality checks or arithmetic comparisons. For instance, if you have an action that moves the agent to a target, you might want to check that the target is in range. You can do this by passing a state checker to the action constructor:
Action moveToTarget = new Action(
executor: (Agent agent, Action action) => {
Console.WriteLine("moved to target");
return ExecutionStatus.Succeeded;
},
stateChecker: (action, state) => {
if (action.GetParameter("target") is Agent target) {
var distance = GetDistance(this, target);
if (distance > 10) return false;
else return true;
}
return false;
}
);
Full API docs are available here.
Mountain GOAP features a simple event system that allows you to subscribe to events that occur during the agent's planning and execution process.
The following events are available on agents:
- OnAgentActionSequenceCompleted: Called when the agent has finished executing its plan.
- Example usage:
Agent.OnAgentActionSequenceCompleted += (Agent agent) => { Console.WriteLine("Agent finished executing its plan."); };
- Example usage:
- OnAgentStep: Called when the agent executes a step of work.
- Example usage:
Agent.OnAgentStep += (Agent agent) => { Console.WriteLine("Agent is working."); };
- Example usage:
- OnPlanningStarted: Called when the agent begins planning.
- Example usage:
Agent.OnPlanningStarted += (Agent agent) => { Console.WriteLine("Agent started planning."); };
- Example usage:
- OnPlanningFinished: Called when the agent finishes planning.
- Example usage:
Agent.OnPlanningFinished += (Agent agent, Goal? goal, float utility) => { Console.WriteLine("Agent finished planning."); };
- Example usage:
- OnPlanningFinishedForSingleGoal: Called when the agent finishes planning for a single goal.
- Example usage:
Agent.OnPlanningFinishedForSingleGoal += (Agent agent, Goal goal, float utility) => { Console.WriteLine("Agent finished planning for a single goal."); };
- Example usage:
- OnPlanUpdated: Called when the agent generates a viable action sequence.
- Example usage:
Agent.OnPlanUpdated += (Agent agent, List<Action> actionList) => { Console.WriteLine("Agent generated a viable action sequence."); };
- Example usage:
- OnEvaluatedActionNode: Extremely low level debugging tool, called whenever the agent evaluates a potentially viable node in the action graph. The second parameter is a dictionary of all nodes that have been evaluated so far, with the keys as the most recent nodes and the values as the nodes that preceded them. You can use this to reconstruct the chain of actions that led to this point.
- Example usage:
Agent.OnEvaluatedActionNode(ActionNode node, Dictionary<ActionNode, ActionNode> nodes) => { Console.WriteLine("Agent evaluated a node.")}
- Example usage:
The following events are available on actions:
- OnBeginExecuteAction: Called when the agent begins executing an action.
- Example usage:
Action.OnBeginExecuteAction += (Agent agent, Action action, Dictionary<string, object> parameters) => { Console.WriteLine("Agent started executing an action."); };
- Example usage:
- OnFinishExecuteAction: Called when the agent finishes executing an action.
- Example usage:
Action.OnFinishExecuteAction += (Agent agent, Action action, ExecutionStatus status, Dictionary<string, object> parameters) => { Console.WriteLine("Agent finished executing an action."); };
- Example usage:
The following events are available on sensors:
- OnSensorRun: Called when the agent runs a sensor.
- Example usage:
Sensor.OnSensorRun += (Agent agent, Sensor sensor) => { Console.WriteLine("Agent ran a sensor."); };
- Example usage:
Mountain GOAP contains a default logger implementation that can be used to examine agent behavior. After including the MountainGoapLogger code, you can enable the logger using the following code:
_ = new MountainGoapLogger.DefaultLogger(
logToConsole: true,
loggingFile: "agents.log"
);
File or folder | Description |
---|---|
/Examples/ |
Examples of how to use the library |
/Examples/RpgExample/ |
RPG grid-based example. |
/Examples/RpgExample/RpgCharacterFactory.cs |
Static methods for creating character agents. |
/Examples/RpgExample/RpgExample.cs |
Main RPG example entrypoint. |
/Examples/RpgExample/RpgMonsterFactory.cs |
Static methods for creating enemy agents derived from the base character agent. |
/Examples/RpgExample/Utils.cs |
RPG example utility functions. |
/Examples/examples.md |
Examples documentation. |
/Examples/HappinessIncrementer.cs |
Happiness incrementer example. |
/Examples/Program.cs |
Examples entrypoint. |
/MountainGoap/ |
The main library folder |
/MountainGoap/CallbackDelegates/ |
Function signatures for callbacks. |
/MountainGoap/CallbackDelegates/ExecutorCallback.cs |
Function signature for a callback that executes an action. |
/MountainGoap/CallbackDelegates/PermutationSelectorCallbacks.cs |
Function signature for a callback that selects a list of options for an action parameter. |
/MountainGoap/CallbackDelegates/SensorRunCallback.cs |
Function signature for a callback that runs a sensor. |
/MountainGoap/Internals/ |
Internal classes that external applications using the library will not need directly. |
/MountainGoap/Internals/ActionAStar.cs |
Class that calculates AStar for an action graph. |
/MountainGoap/Internals/ActionGraph.cs |
Class that represents an action graph. |
/MountainGoap/Internals/ActionNode.cs |
Class that represents a node in an action graph. |
/MountainGoap/Internals/CopyDictionaryExtensionMethod.cs |
Convenience extension method for copying a dictionary. |
/MountainGoap/Internals/Planner.cs |
Planning class used by agents. |
/MountainGoap/Action.cs |
An action that can be made available to agents. |
/MountainGoap/Agent.cs |
An agent that can figure out plans to execute. |
/MountainGoap/BaseGoal.cs |
A base class for all goal types. |
/MountainGoap/ComparativeGoal.cs |
A goal that compares a value to a pre-existing value |
/MountainGoap/ComparisonOperator.cs |
An enum defining the comparison operators that can be used with ComparativeGoal. |
/MountainGoap/ComparisonValuePair.cs |
A class that represents a comparison value pair. |
/MountainGoap/ExecutionStatus.cs |
An enum defining the execution status of an action. |
/MountainGoap/ExtremeGoal.cs |
A goal that attempts to minimize or maximize a state value. |
/MountainGoap/Goal.cs |
A goal that agents can attempt to accomplish. |
/MountainGoap/PermutationSelectorGenerators.cs |
Generators for lambda functions that return a list of options for an action parameter. |
/MountainGoap/Sensor.cs |
A sensor that generates data for use by an agent. |
/MountainGoapLogging/DefaultLogger.cs |
Example logger implementation that can be used to inspect agent behavior. |
- Tests
- Examples - general and Unity
- ReGoap - C# GOAP library with more direct Unity support, providing Unity Components that can be attached to GameObjects.
Project is MIT licensed, and the main license file can be found here.
The MIT License (MIT)
Copyright (c) 2013 Daniel "BlueRaja" Pflughoeft
Permission is hereby granted, free of charge, to any person obtaining a copy of this software and associated documentation files (the "Software"), to deal in the Software without restriction, including without limitation the rights to use, copy, modify, merge, publish, distribute, sublicense, and/or sell copies of the Software, and to permit persons to whom the Software is furnished to do so, subject to the following conditions:
The above copyright notice and this permission notice shall be included in all copies or substantial portions of the Software.
THE SOFTWARE IS PROVIDED "AS IS", WITHOUT WARRANTY OF ANY KIND, EXPRESS OR IMPLIED, INCLUDING BUT NOT LIMITED TO THE WARRANTIES OF MERCHANTABILITY, FITNESS FOR A PARTICULAR PURPOSE AND NONINFRINGEMENT. IN NO EVENT SHALL THE AUTHORS OR COPYRIGHT HOLDERS BE LIABLE FOR ANY CLAIM, DAMAGES OR OTHER LIABILITY, WHETHER IN AN ACTION OF CONTRACT, TORT OR OTHERWISE, ARISING FROM, OUT OF OR IN CONNECTION WITH THE SOFTWARE OR THE USE OR OTHER DEALINGS IN THE SOFTWARE.
For Tasks:
Click tags to check more tools for each tasksFor Jobs:
Alternative AI tools for mountain-goap
Similar Open Source Tools
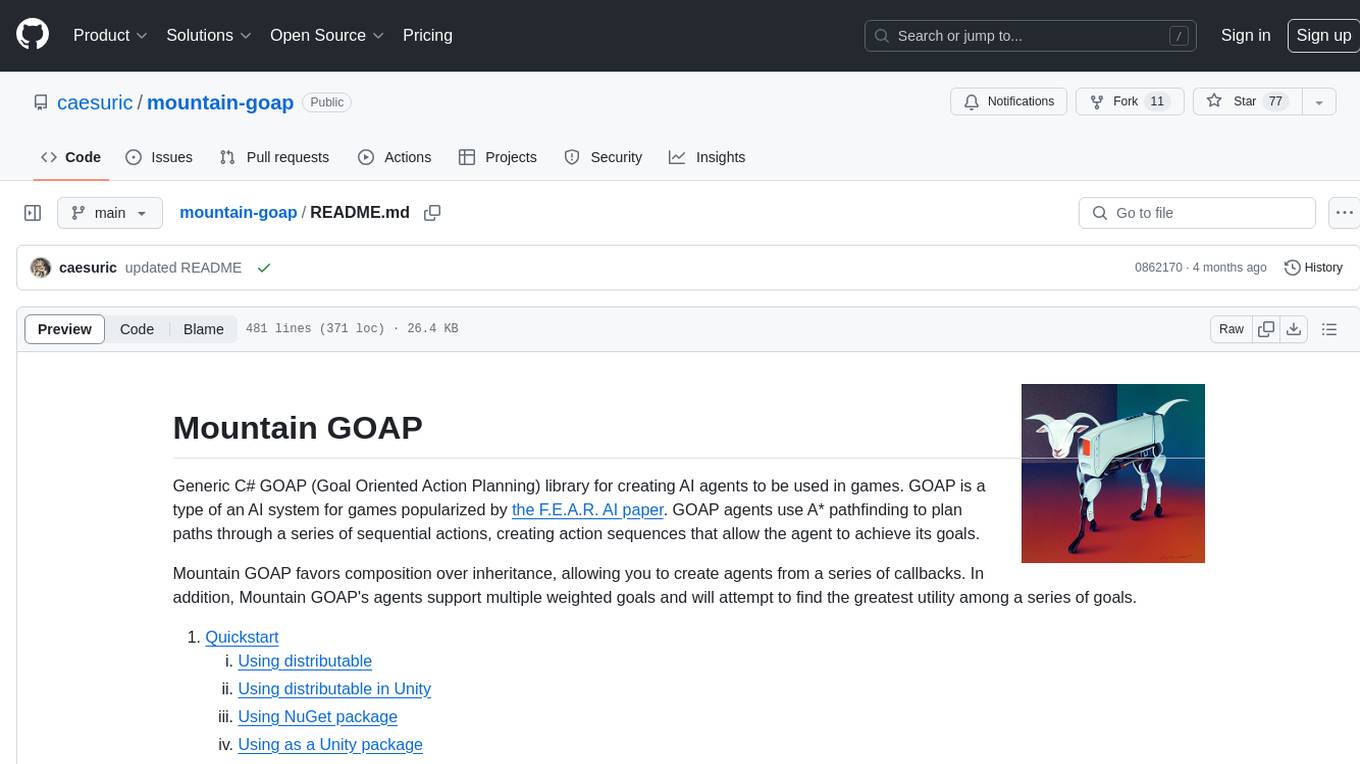
mountain-goap
Mountain GOAP is a generic C# GOAP (Goal Oriented Action Planning) library for creating AI agents in games. It favors composition over inheritance, supports multiple weighted goals, and uses A* pathfinding to plan paths through sequential actions. The library includes concepts like agents, goals, actions, sensors, permutation selectors, cost callbacks, state mutators, state checkers, and a logger. It also features event handling for agent planning and execution. The project structure includes examples, API documentation, and internal classes for planning and execution.
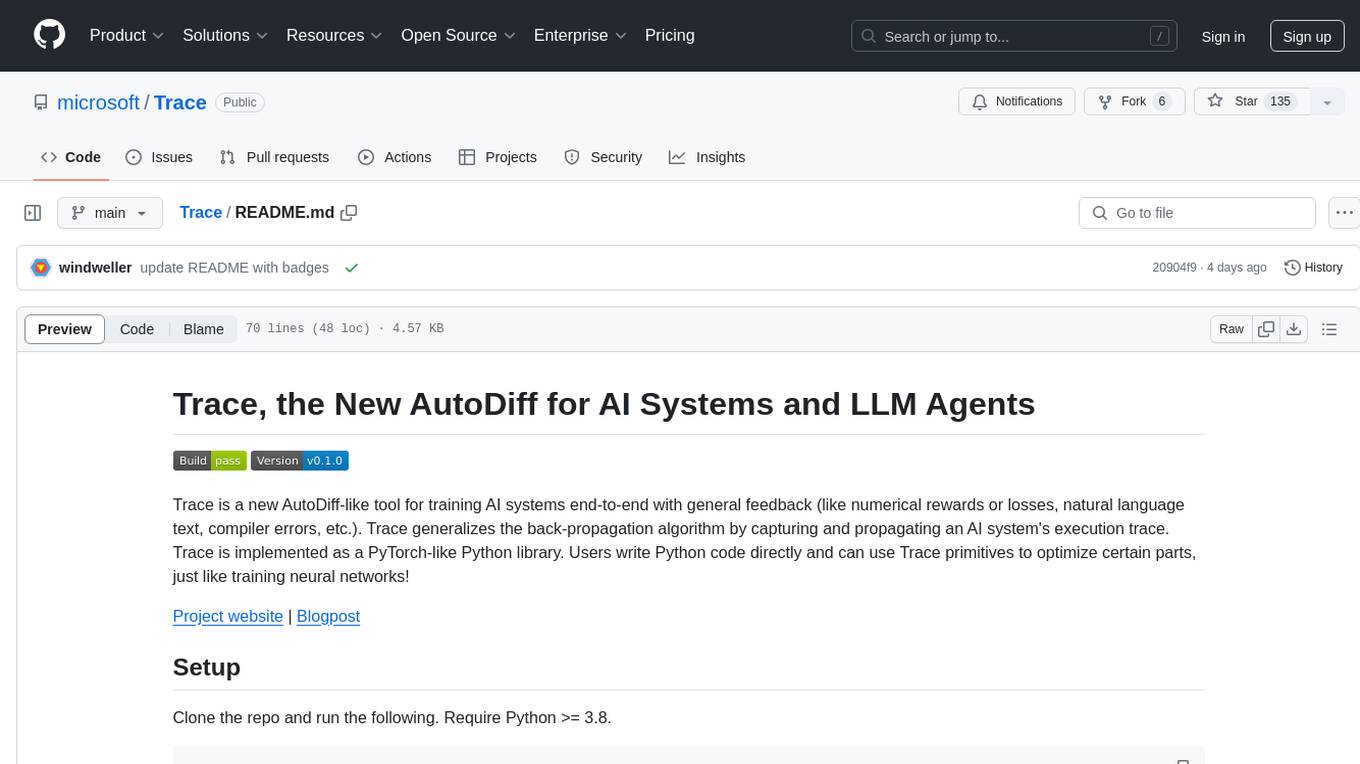
Trace
Trace is a new AutoDiff-like tool for training AI systems end-to-end with general feedback. It generalizes the back-propagation algorithm by capturing and propagating an AI system's execution trace. Implemented as a PyTorch-like Python library, users can write Python code directly and use Trace primitives to optimize certain parts, similar to training neural networks.
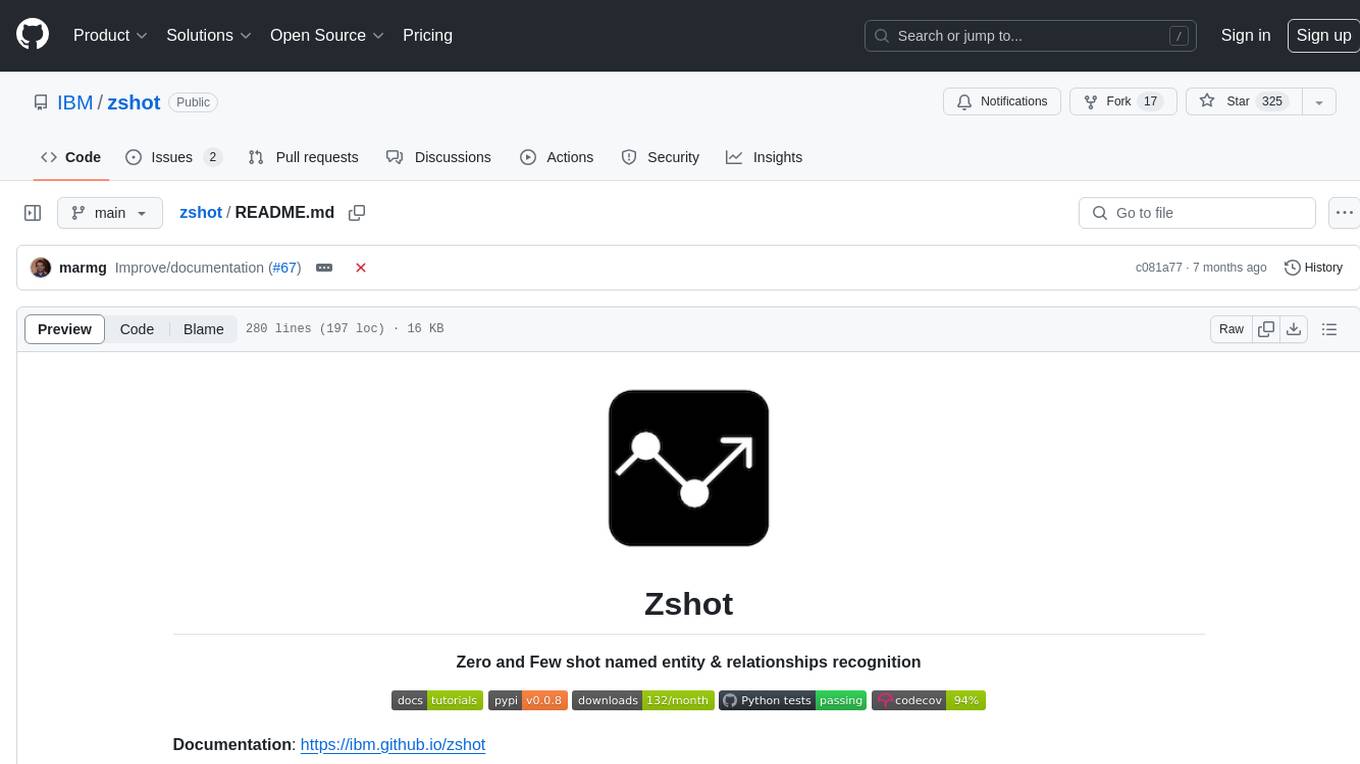
zshot
Zshot is a highly customizable framework for performing Zero and Few shot named entity and relationships recognition. It can be used for mentions extraction, wikification, zero and few shot named entity recognition, zero and few shot named relationship recognition, and visualization of zero-shot NER and RE extraction. The framework consists of two main components: the mentions extractor and the linker. There are multiple mentions extractors and linkers available, each serving a specific purpose. Zshot also includes a relations extractor and a knowledge extractor for extracting relations among entities and performing entity classification. The tool requires Python 3.6+ and dependencies like spacy, torch, transformers, evaluate, and datasets for evaluation over datasets like OntoNotes. Optional dependencies include flair and blink for additional functionalities. Zshot provides examples, tutorials, and evaluation methods to assess the performance of the components.
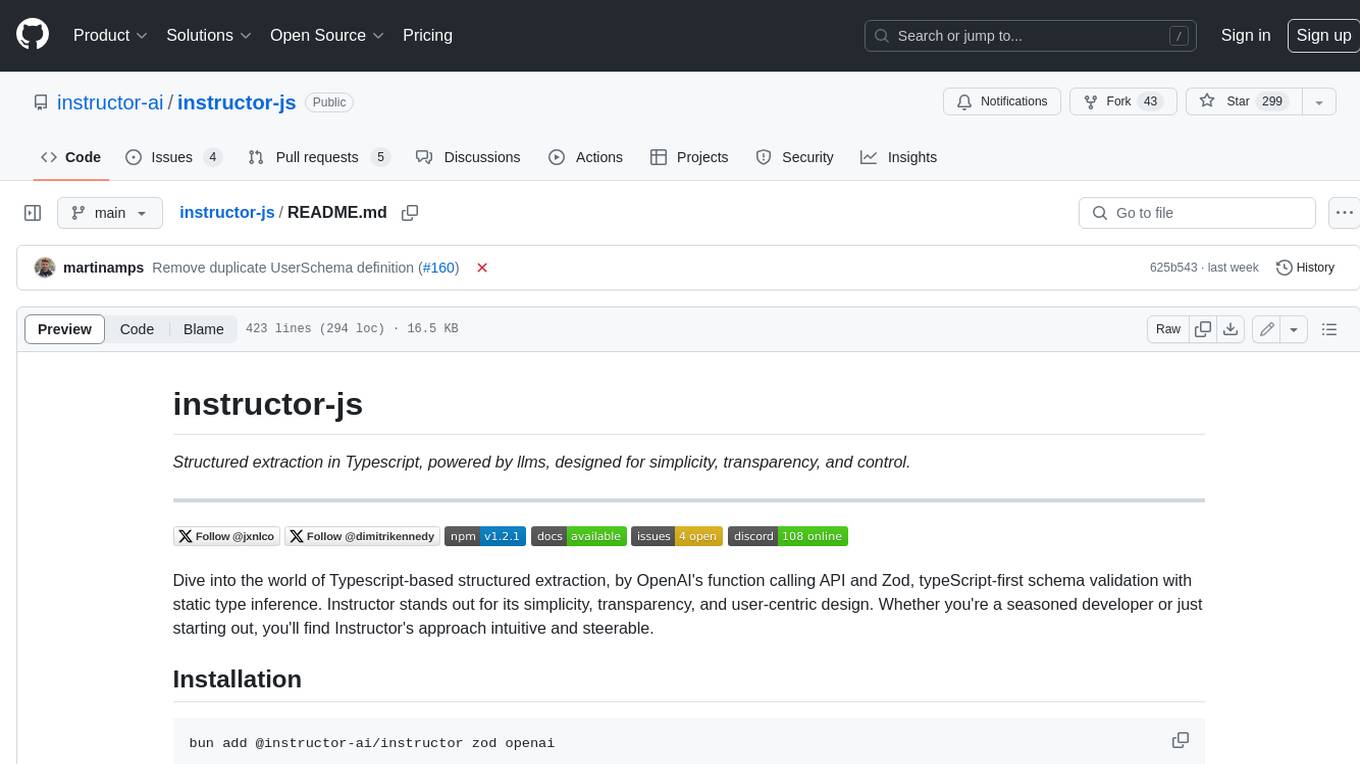
instructor-js
Instructor is a Typescript library for structured extraction in Typescript, powered by llms, designed for simplicity, transparency, and control. It stands out for its simplicity, transparency, and user-centric design. Whether you're a seasoned developer or just starting out, you'll find Instructor's approach intuitive and steerable.
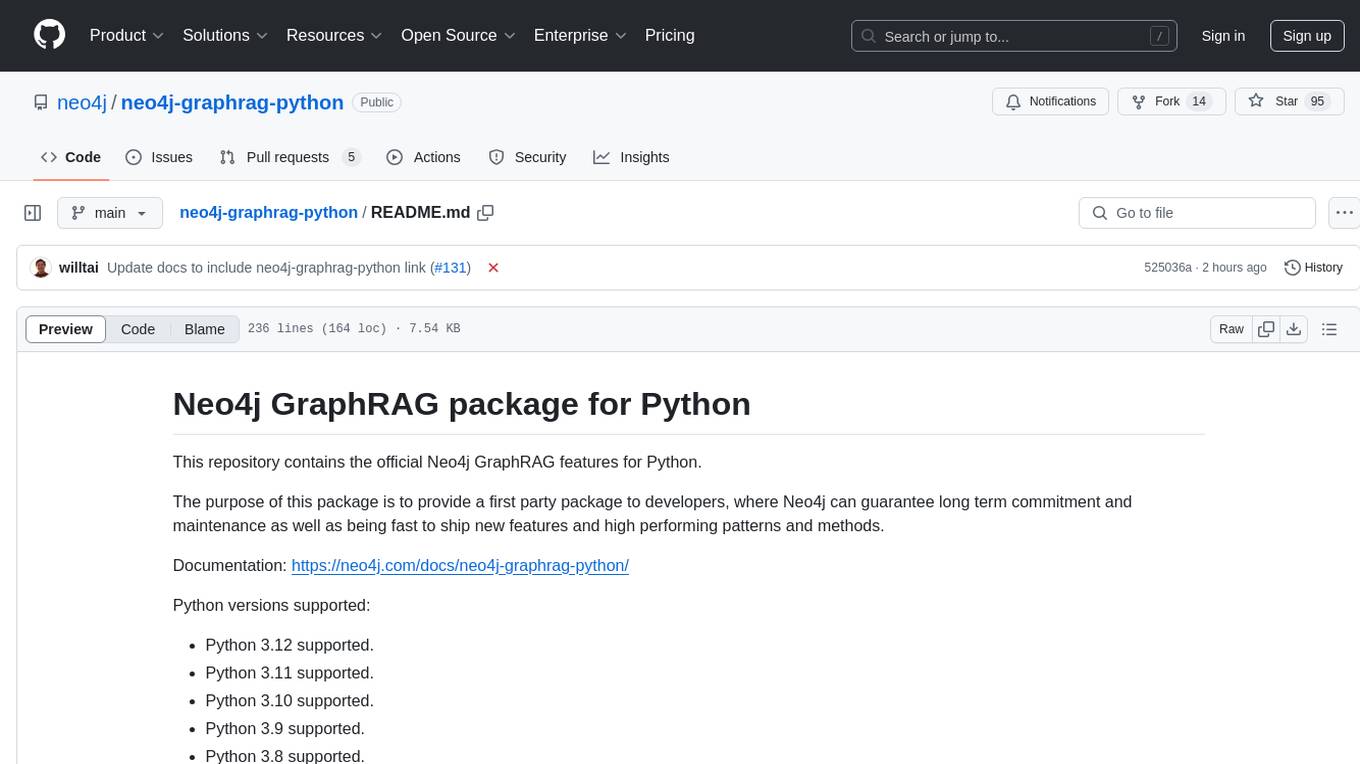
neo4j-graphrag-python
The Neo4j GraphRAG package for Python is an official repository that provides features for creating and managing vector indexes in Neo4j databases. It aims to offer developers a reliable package with long-term commitment, maintenance, and fast feature updates. The package supports various Python versions and includes functionalities for creating vector indexes, populating them, and performing similarity searches. It also provides guidelines for installation, examples, and development processes such as installing dependencies, making changes, and running tests.
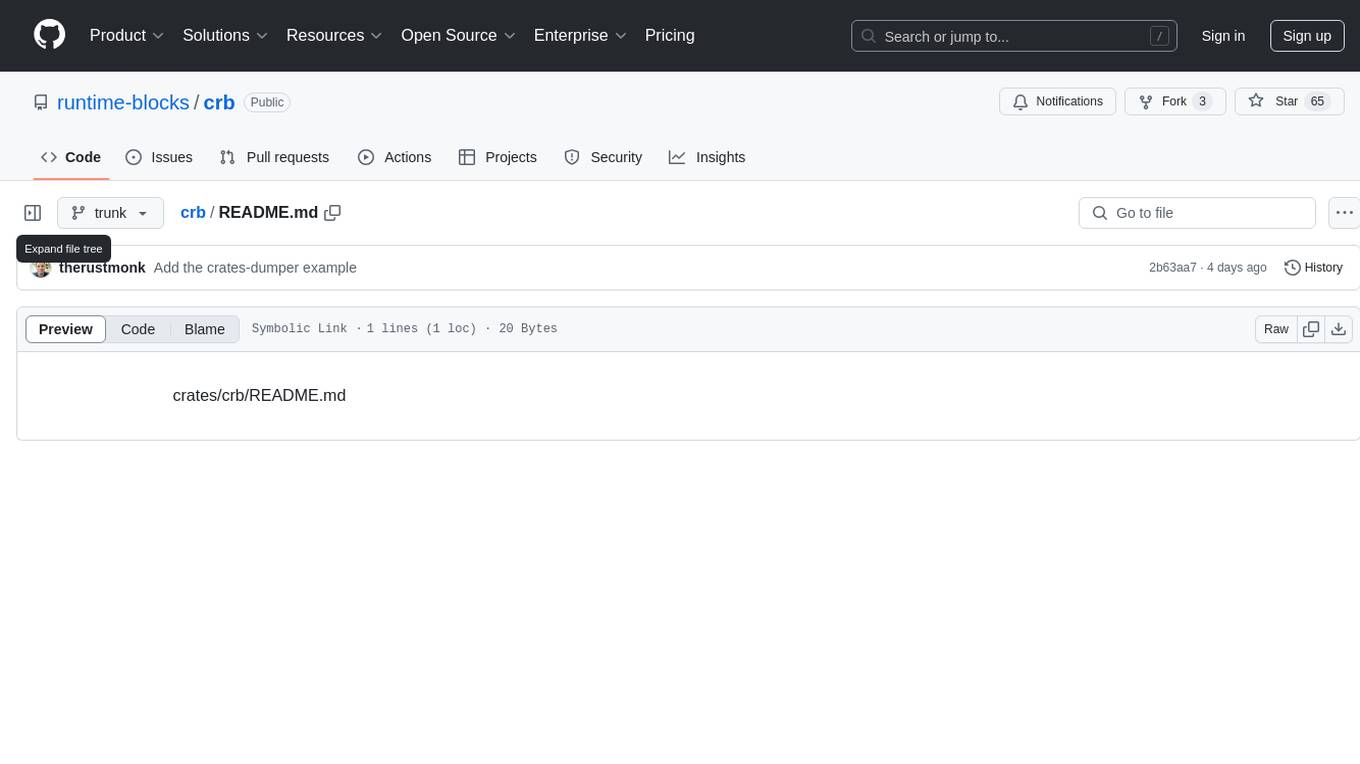
crb
CRB (Composable Runtime Blocks) is a unique framework that implements hybrid workloads by seamlessly combining synchronous and asynchronous activities, state machines, routines, the actor model, and supervisors. It is ideal for building massive applications and serves as a low-level framework for creating custom frameworks, such as AI-agents. The core idea is to ensure high compatibility among all blocks, enabling significant code reuse. The framework allows for the implementation of algorithms with complex branching, making it suitable for building large-scale applications or implementing complex workflows, such as AI pipelines. It provides flexibility in defining structures, implementing traits, and managing execution flow, allowing users to create robust and nonlinear algorithms easily.
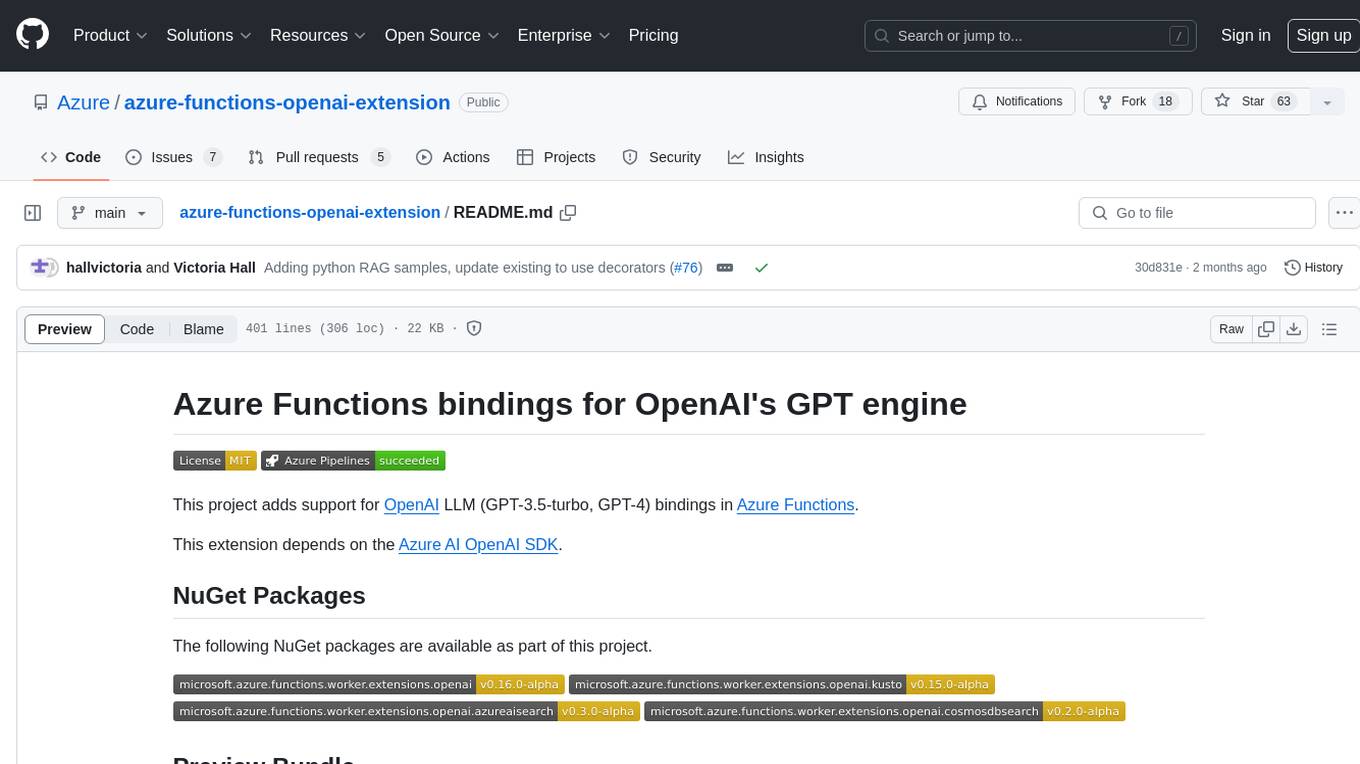
azure-functions-openai-extension
Azure Functions OpenAI Extension is a project that adds support for OpenAI LLM (GPT-3.5-turbo, GPT-4) bindings in Azure Functions. It provides NuGet packages for various functionalities like text completions, chat completions, assistants, embeddings generators, and semantic search. The project requires .NET 6 SDK or greater, Azure Functions Core Tools v4.x, and specific settings in Azure Function or local settings for development. It offers features like text completions, chat completion, assistants with custom skills, embeddings generators for text relatedness, and semantic search using vector databases. The project also includes examples in C# and Python for different functionalities.
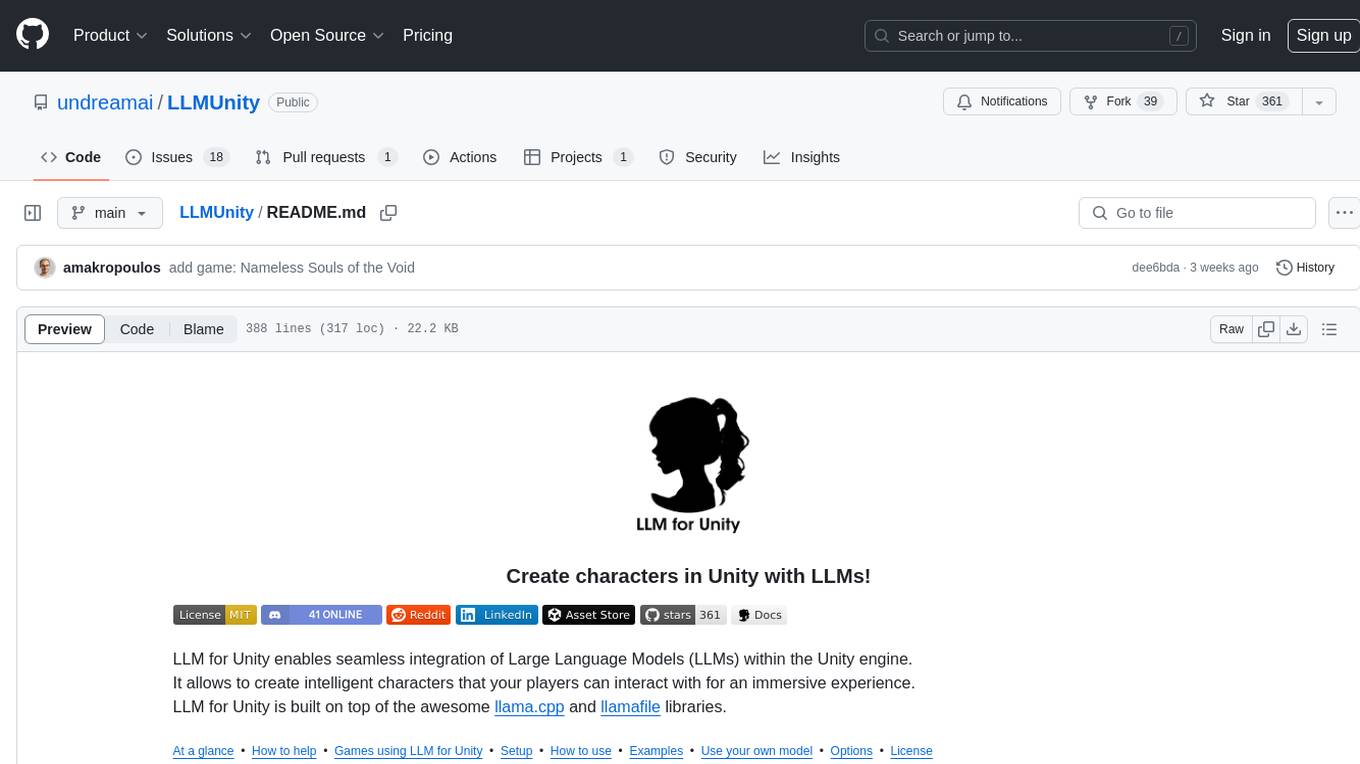
LLMUnity
LLM for Unity enables seamless integration of Large Language Models (LLMs) within the Unity engine, allowing users to create intelligent characters for immersive player interactions. The tool supports major LLM models, runs locally without internet access, offers fast inference on CPU and GPU, and is easy to set up with a single line of code. It is free for both personal and commercial use, tested on Unity 2021 LTS, 2022 LTS, and 2023. Users can build multiple AI characters efficiently, use remote servers for processing, and customize model settings for text generation.
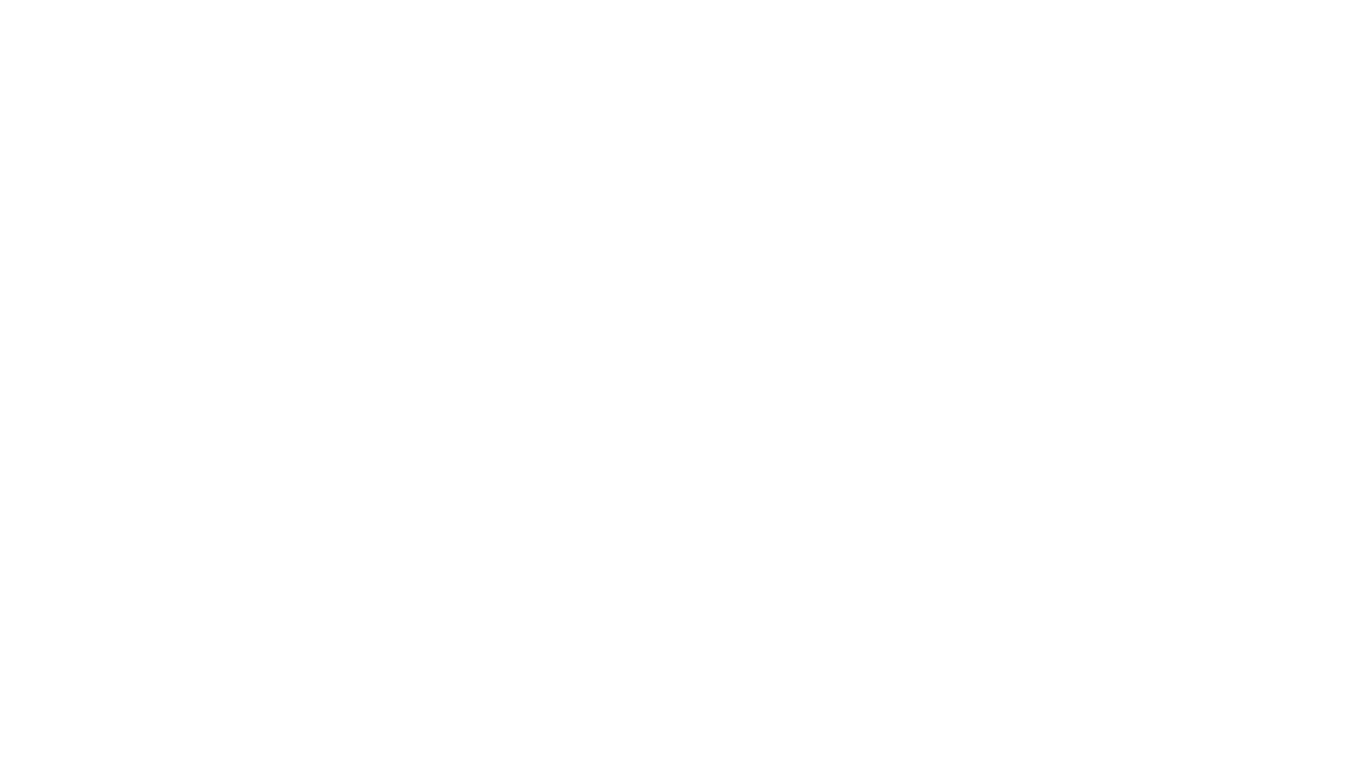
generative-ai
The 'Generative AI' repository provides a C# library for interacting with Google's Generative AI models, specifically the Gemini models. It allows users to access and integrate the Gemini API into .NET applications, supporting functionalities such as listing available models, generating content, creating tuned models, working with large files, starting chat sessions, and more. The repository also includes helper classes and enums for Gemini API aspects. Authentication methods include API key, OAuth, and various authentication modes for Google AI and Vertex AI. The package offers features for both Google AI Studio and Google Cloud Vertex AI, with detailed instructions on installation, usage, and troubleshooting.
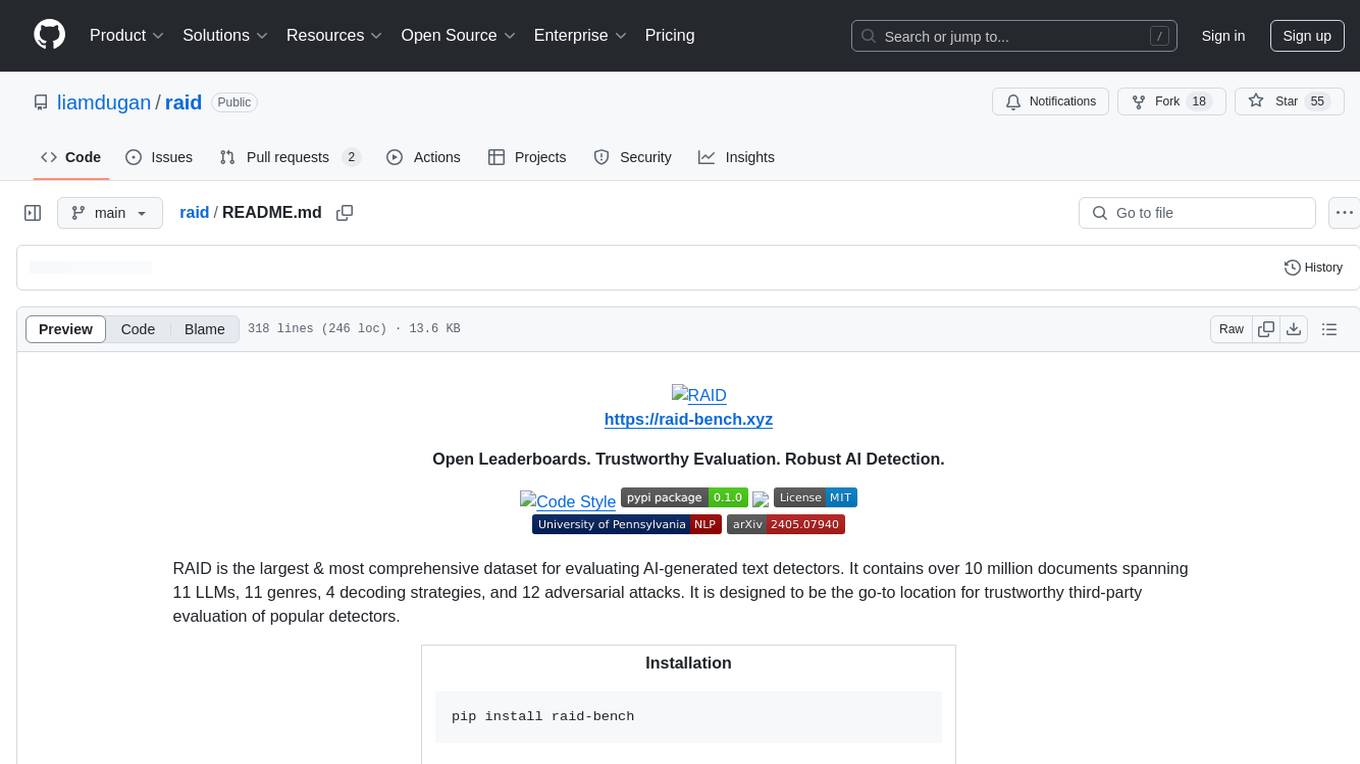
raid
RAID is the largest and most comprehensive dataset for evaluating AI-generated text detectors. It contains over 10 million documents spanning 11 LLMs, 11 genres, 4 decoding strategies, and 12 adversarial attacks. RAID is designed to be the go-to location for trustworthy third-party evaluation of popular detectors. The dataset covers diverse models, domains, sampling strategies, and attacks, making it a valuable resource for training detectors, evaluating generalization, protecting against adversaries, and comparing to state-of-the-art models from academia and industry.
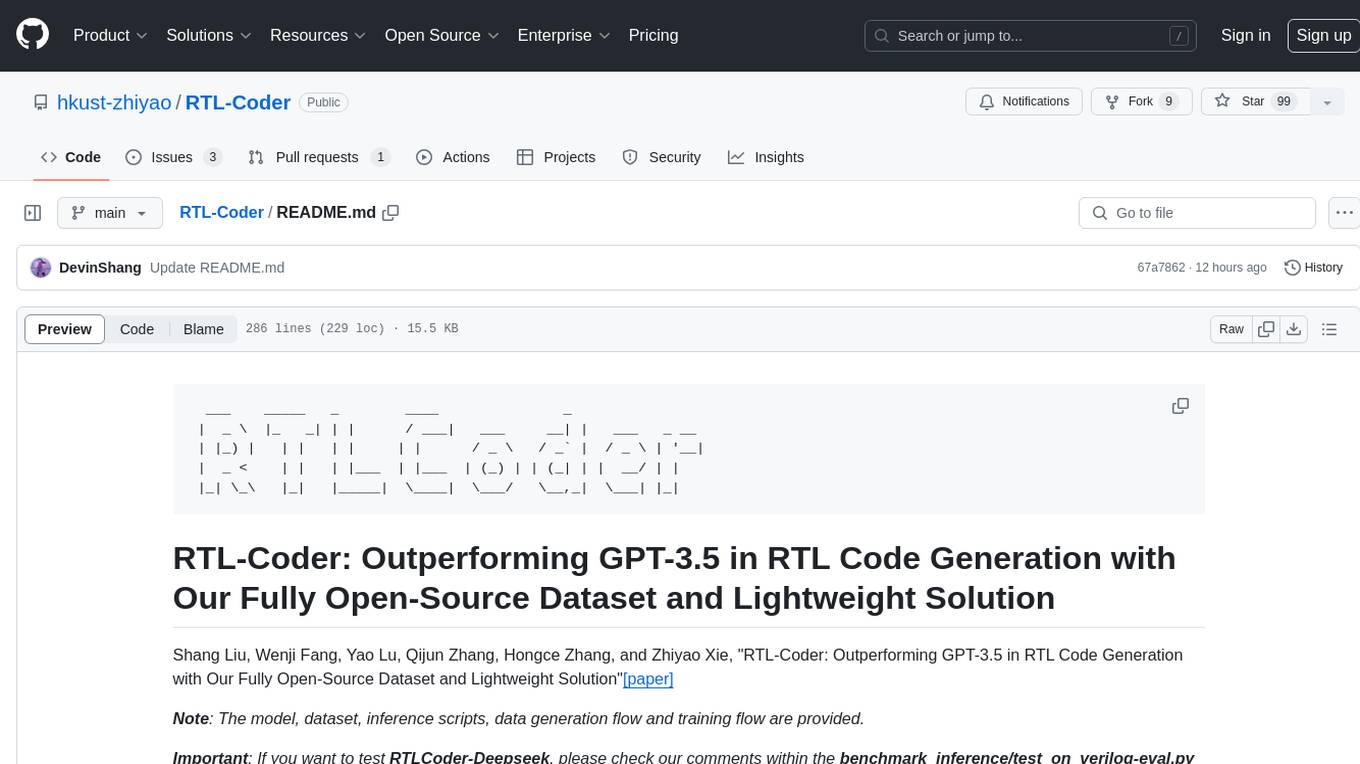
RTL-Coder
RTL-Coder is a tool designed to outperform GPT-3.5 in RTL code generation by providing a fully open-source dataset and a lightweight solution. It targets Verilog code generation and offers an automated flow to generate a large labeled dataset with over 27,000 diverse Verilog design problems and answers. The tool addresses the data availability challenge in IC design-related tasks and can be used for various applications beyond LLMs. The tool includes four RTL code generation models available on the HuggingFace platform, each with specific features and performance characteristics. Additionally, RTL-Coder introduces a new LLM training scheme based on code quality feedback to further enhance model performance and reduce GPU memory consumption.
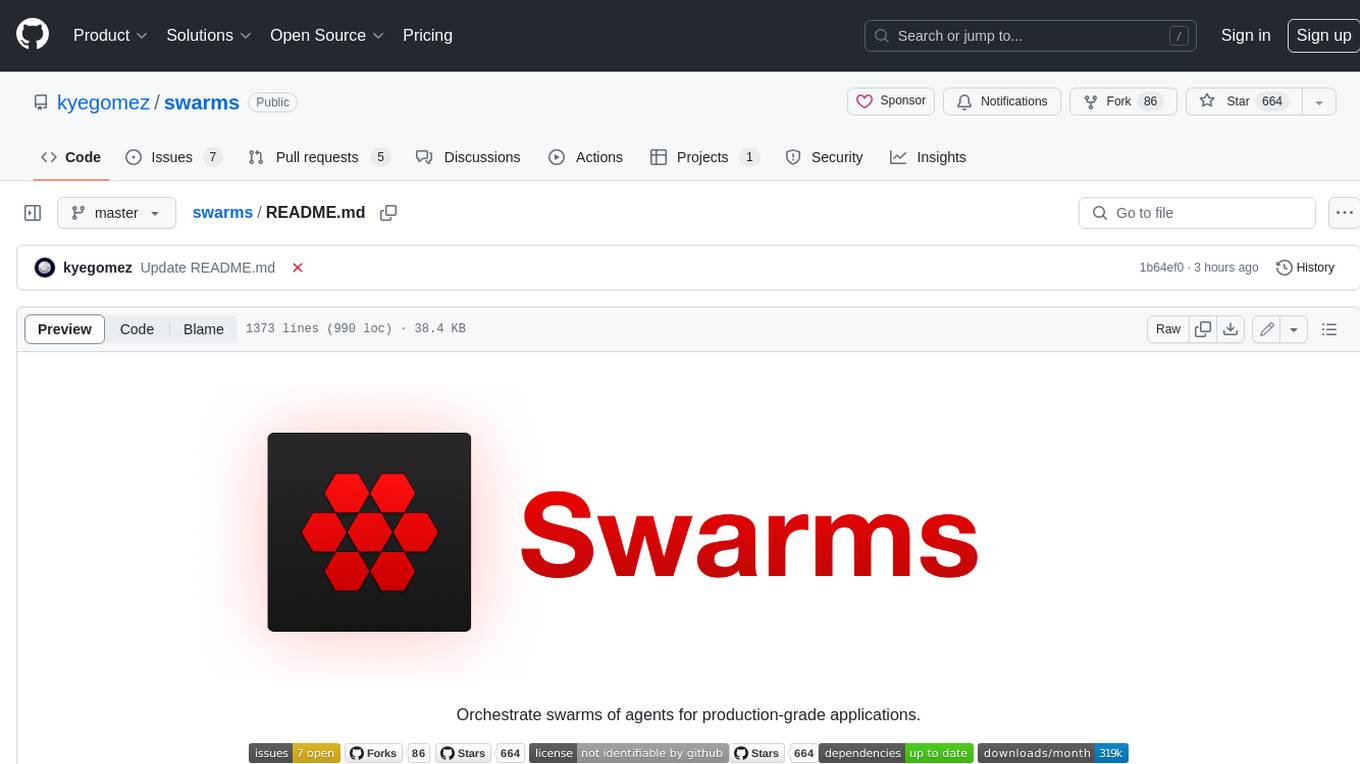
swarms
Swarms provides simple, reliable, and agile tools to create your own Swarm tailored to your specific needs. Currently, Swarms is being used in production by RBC, John Deere, and many AI startups.
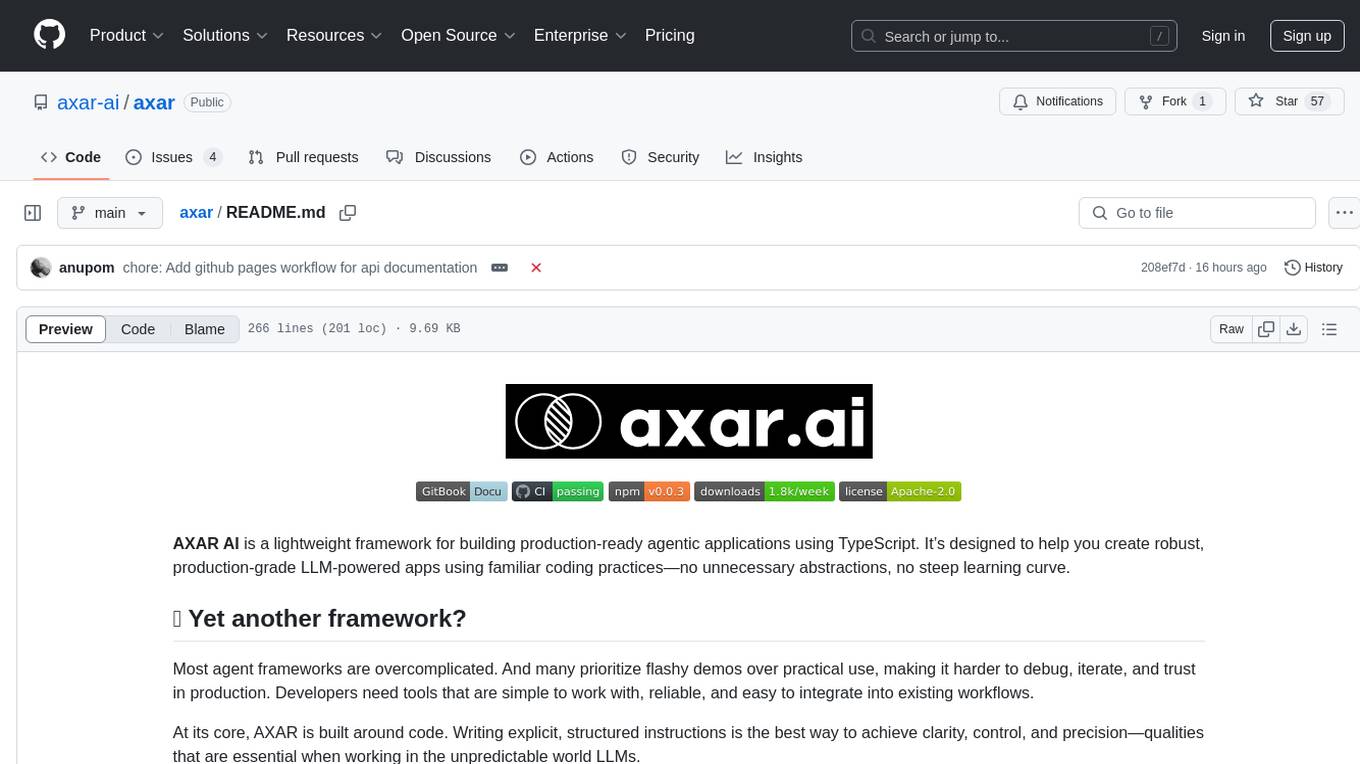
axar
AXAR AI is a lightweight framework designed for building production-ready agentic applications using TypeScript. It aims to simplify the process of creating robust, production-grade LLM-powered apps by focusing on familiar coding practices without unnecessary abstractions or steep learning curves. The framework provides structured, typed inputs and outputs, familiar and intuitive patterns like dependency injection and decorators, explicit control over agent behavior, real-time logging and monitoring tools, minimalistic design with little overhead, model agnostic compatibility with various AI models, and streamed outputs for fast and accurate results. AXAR AI is ideal for developers working on real-world AI applications who want a tool that gets out of the way and allows them to focus on shipping reliable software.
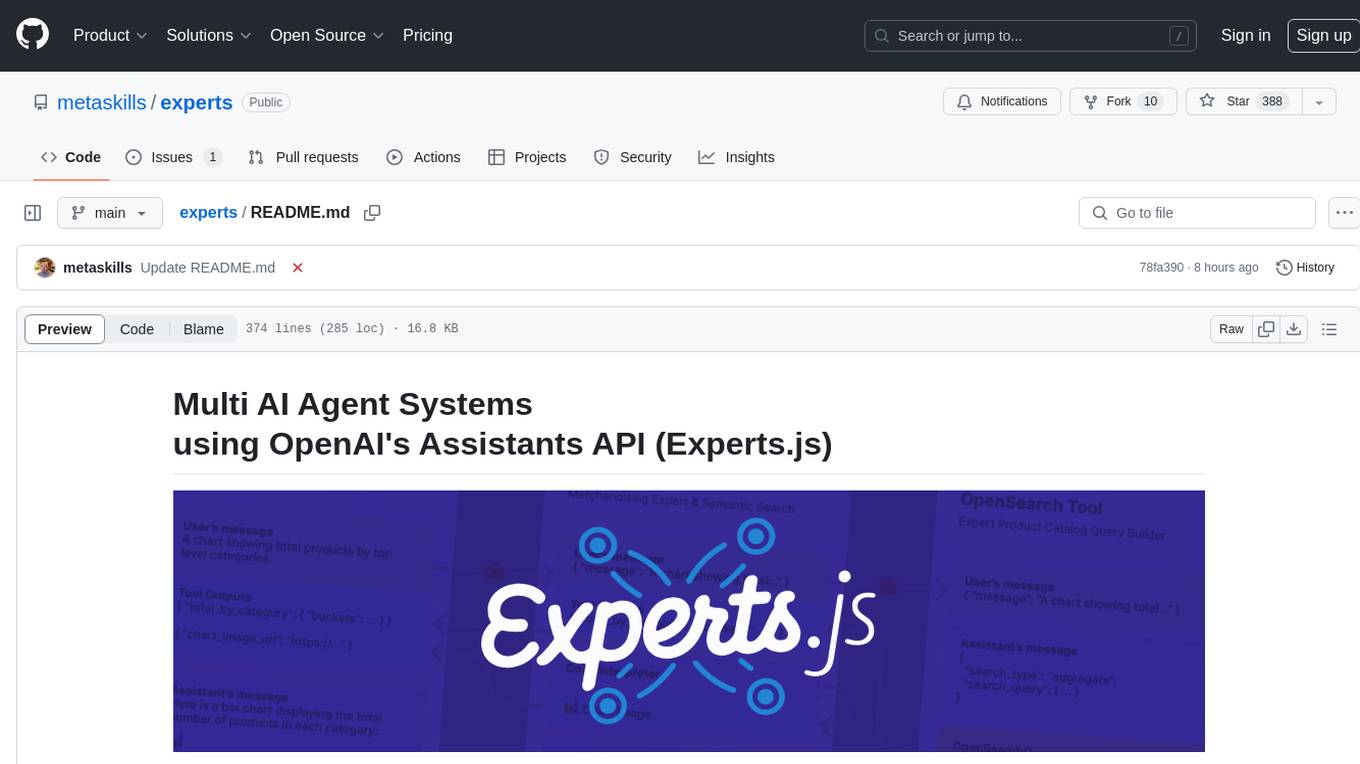
experts
Experts.js is a tool that simplifies the creation and deployment of OpenAI's Assistants, allowing users to link them together as Tools to create a Panel of Experts system with expanded memory and attention to detail. It leverages the new Assistants API from OpenAI, which offers advanced features such as referencing attached files & images as knowledge sources, supporting instructions up to 256,000 characters, integrating with 128 tools, and utilizing the Vector Store API for efficient file search. Experts.js introduces Assistants as Tools, enabling the creation of Multi AI Agent Systems where each Tool is an LLM-backed Assistant that can take on specialized roles or fulfill complex tasks.
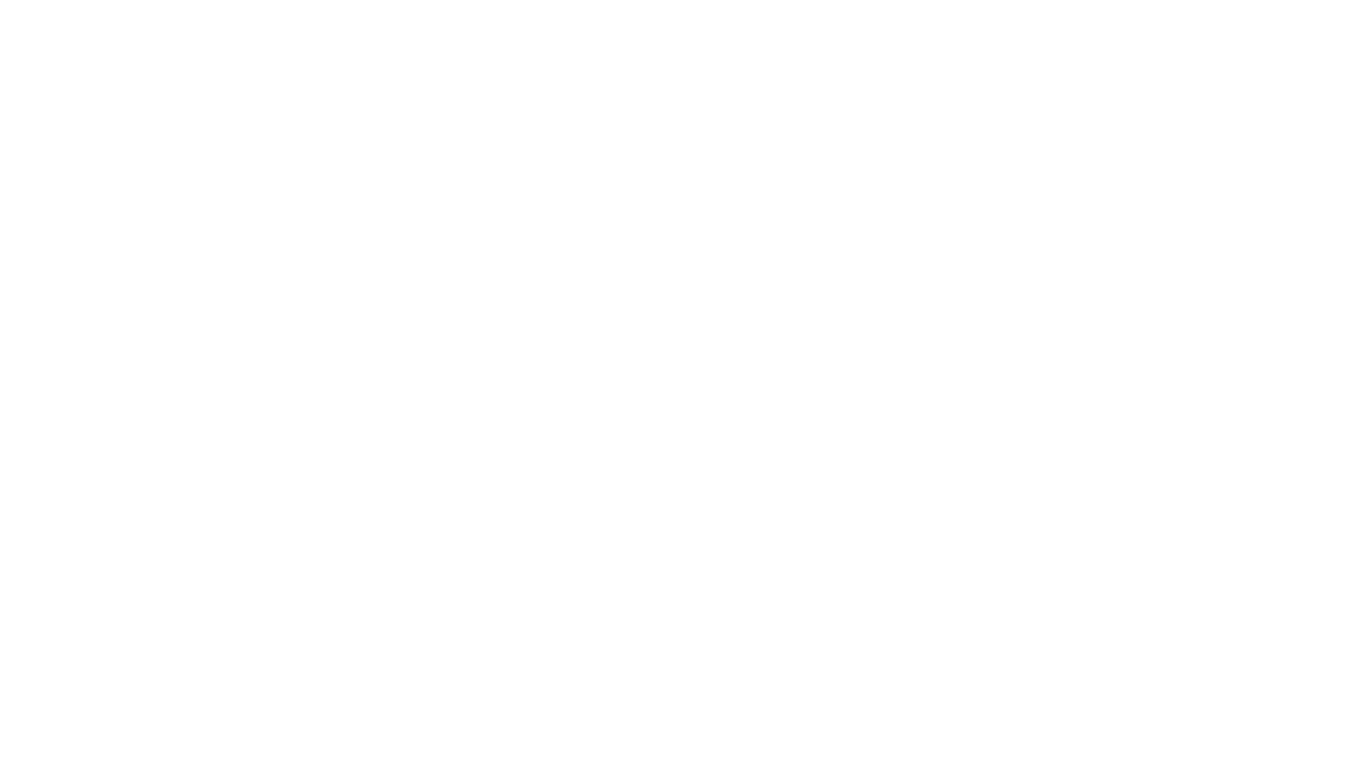
instructor-php
Instructor for PHP is a library designed for structured data extraction in PHP, powered by Large Language Models (LLMs). It simplifies the process of extracting structured, validated data from unstructured text or chat sequences. Instructor enhances workflow by providing a response model, validation capabilities, and max retries for requests. It supports classes as response models and provides features like partial results, string input, extracting scalar and enum values, and specifying data models using PHP type hints or DocBlock comments. The library allows customization of validation and provides detailed event notifications during request processing. Instructor is compatible with PHP 8.2+ and leverages PHP reflection, Symfony components, and SaloonPHP for communication with LLM API providers.
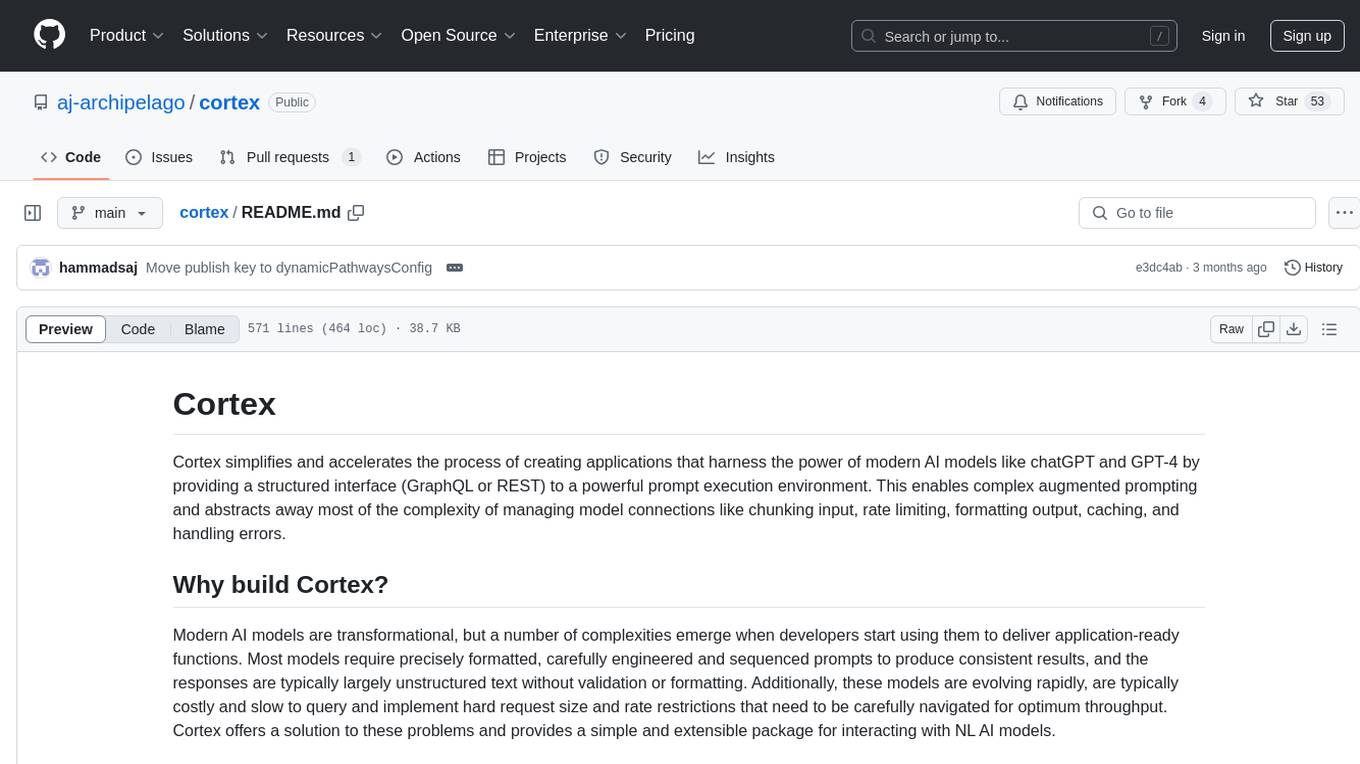
cortex
Cortex is a tool that simplifies and accelerates the process of creating applications utilizing modern AI models like chatGPT and GPT-4. It provides a structured interface (GraphQL or REST) to a prompt execution environment, enabling complex augmented prompting and abstracting away model connection complexities like input chunking, rate limiting, output formatting, caching, and error handling. Cortex offers a solution to challenges faced when using AI models, providing a simple package for interacting with NL AI models.
For similar tasks
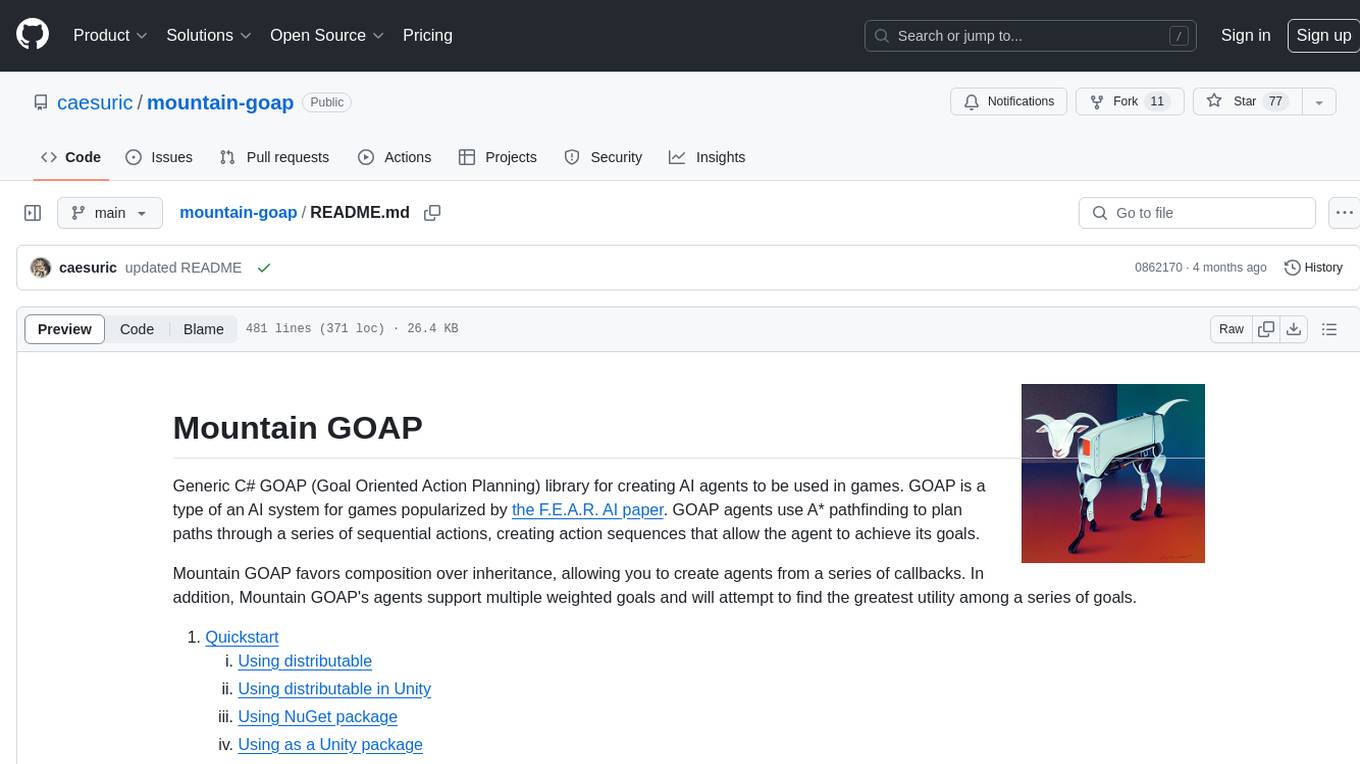
mountain-goap
Mountain GOAP is a generic C# GOAP (Goal Oriented Action Planning) library for creating AI agents in games. It favors composition over inheritance, supports multiple weighted goals, and uses A* pathfinding to plan paths through sequential actions. The library includes concepts like agents, goals, actions, sensors, permutation selectors, cost callbacks, state mutators, state checkers, and a logger. It also features event handling for agent planning and execution. The project structure includes examples, API documentation, and internal classes for planning and execution.
For similar jobs
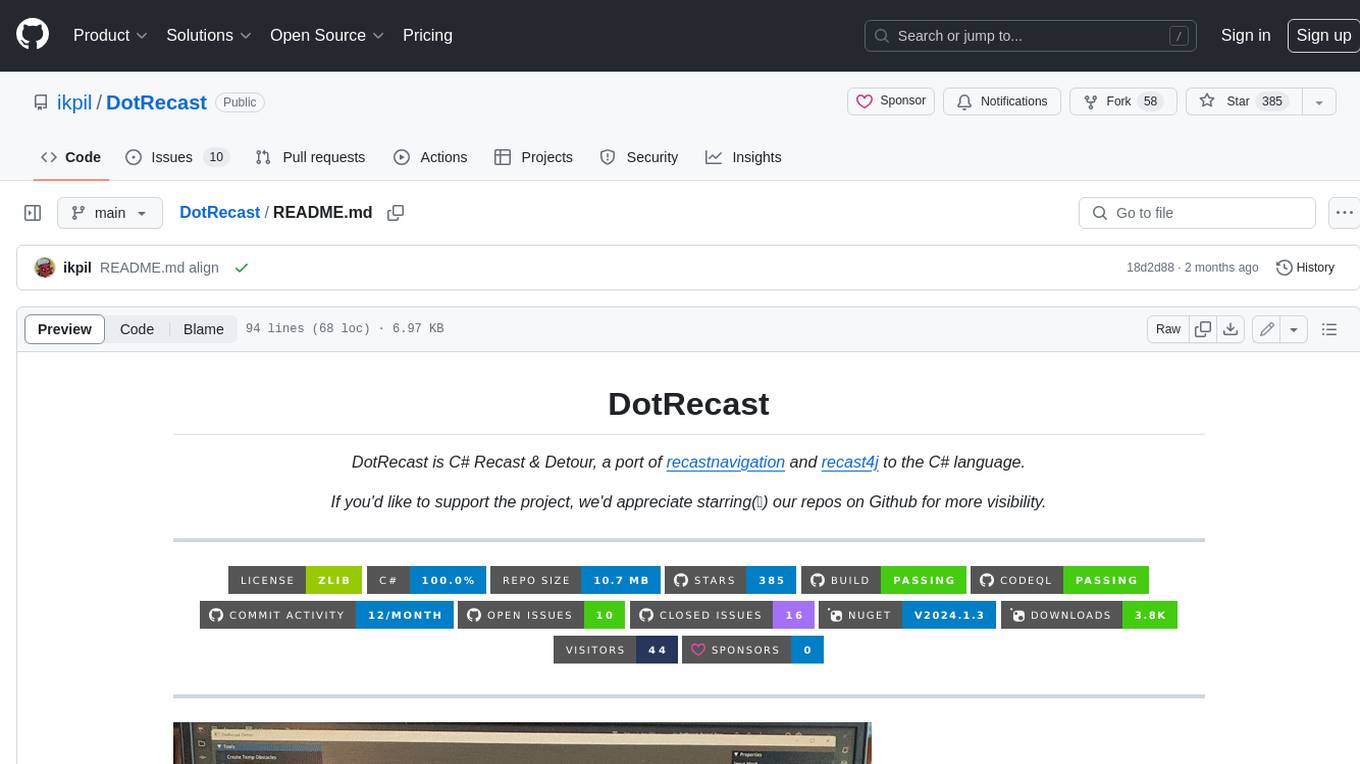
DotRecast
DotRecast is a C# port of Recast & Detour, a navigation library used in many AAA and indie games and engines. It provides automatic navmesh generation, fast turnaround times, detailed customization options, and is dependency-free. Recast Navigation is divided into multiple modules, each contained in its own folder: - DotRecast.Core: Core utils - DotRecast.Recast: Navmesh generation - DotRecast.Detour: Runtime loading of navmesh data, pathfinding, navmesh queries - DotRecast.Detour.TileCache: Navmesh streaming. Useful for large levels and open-world games - DotRecast.Detour.Crowd: Agent movement, collision avoidance, and crowd simulation - DotRecast.Detour.Dynamic: Robust support for dynamic nav meshes combining pre-built voxels with dynamic objects which can be freely added and removed - DotRecast.Detour.Extras: Simple tool to import navmeshes created with A* Pathfinding Project - DotRecast.Recast.Toolset: All modules - DotRecast.Recast.Demo: Standalone, comprehensive demo app showcasing all aspects of Recast & Detour's functionality - Tests: Unit tests Recast constructs a navmesh through a multi-step mesh rasterization process: 1. First Recast rasterizes the input triangle meshes into voxels. 2. Voxels in areas where agents would not be able to move are filtered and removed. 3. The walkable areas described by the voxel grid are then divided into sets of polygonal regions. 4. The navigation polygons are generated by re-triangulating the generated polygonal regions into a navmesh. You can use Recast to build a single navmesh, or a tiled navmesh. Single meshes are suitable for many simple, static cases and are easy to work with. Tiled navmeshes are more complex to work with but better support larger, more dynamic environments. Tiled meshes enable advanced Detour features like re-baking, hierarchical path-planning, and navmesh data-streaming.
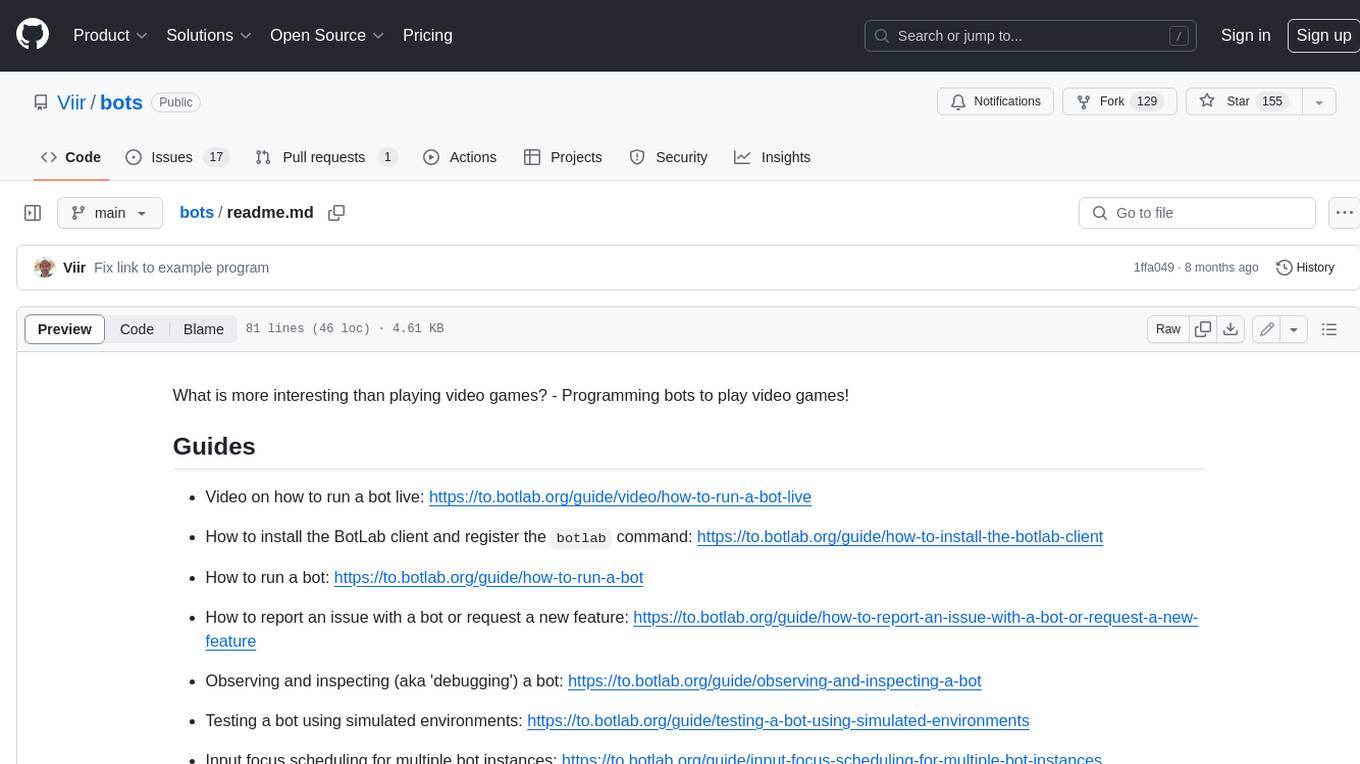
bots
The 'bots' repository is a collection of guides, tools, and example bots for programming bots to play video games. It provides resources on running bots live, installing the BotLab client, debugging bots, testing bots in simulated environments, and more. The repository also includes example bots for games like EVE Online, Tribal Wars 2, and Elvenar. Users can learn about developing bots for specific games, syntax of the Elm programming language, and tools for memory reading development. Additionally, there are guides on bot programming, contributing to BotLab, and exploring Elm syntax and core library.
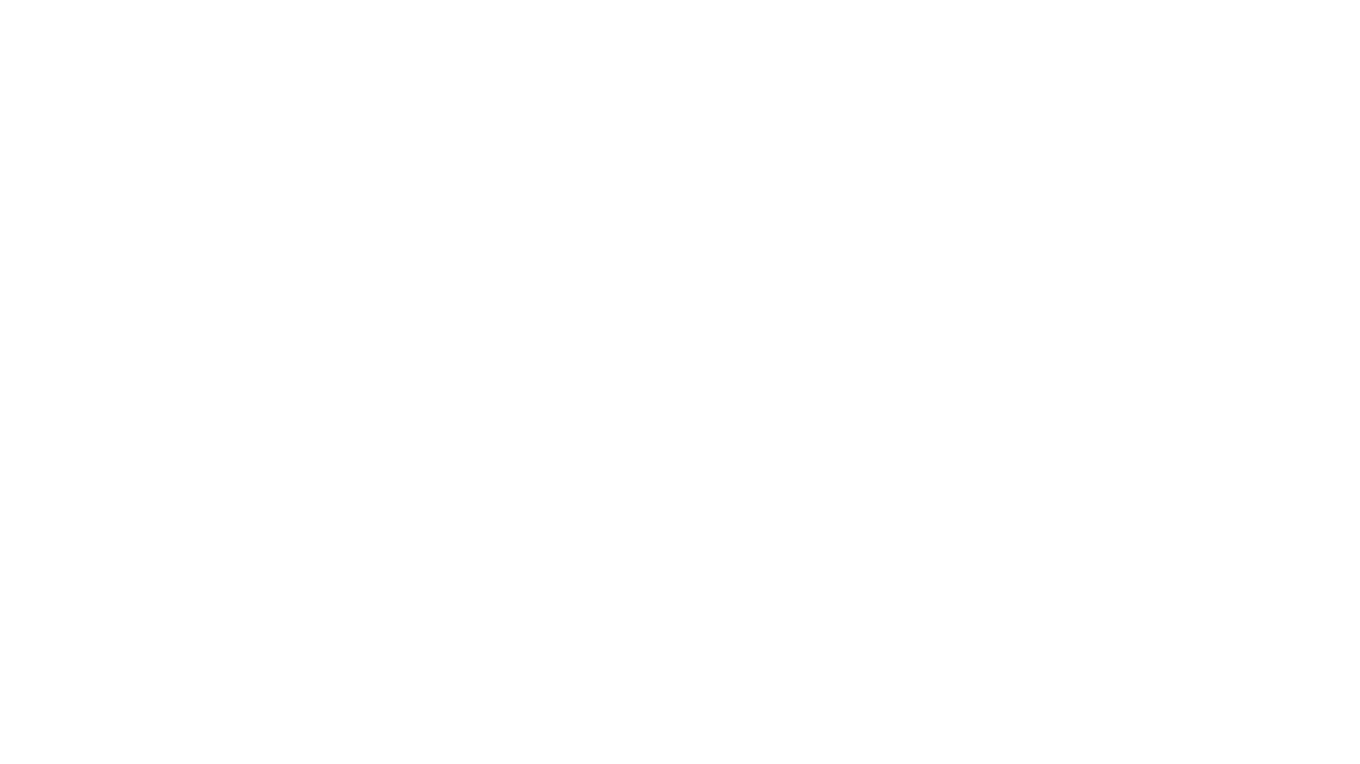
Half-Life-Resurgence
Half-Life-Resurgence is a recreation and expansion project that brings NPCs, entities, and weapons from the Half-Life series into Garry's Mod. The goal is to faithfully recreate original content while also introducing new features and custom content envisioned by the community. Users can expect a wide range of NPCs with new abilities, AI behaviors, and weapons, as well as support for playing as any character and replacing NPCs in Half-Life 1 & 2 campaigns.
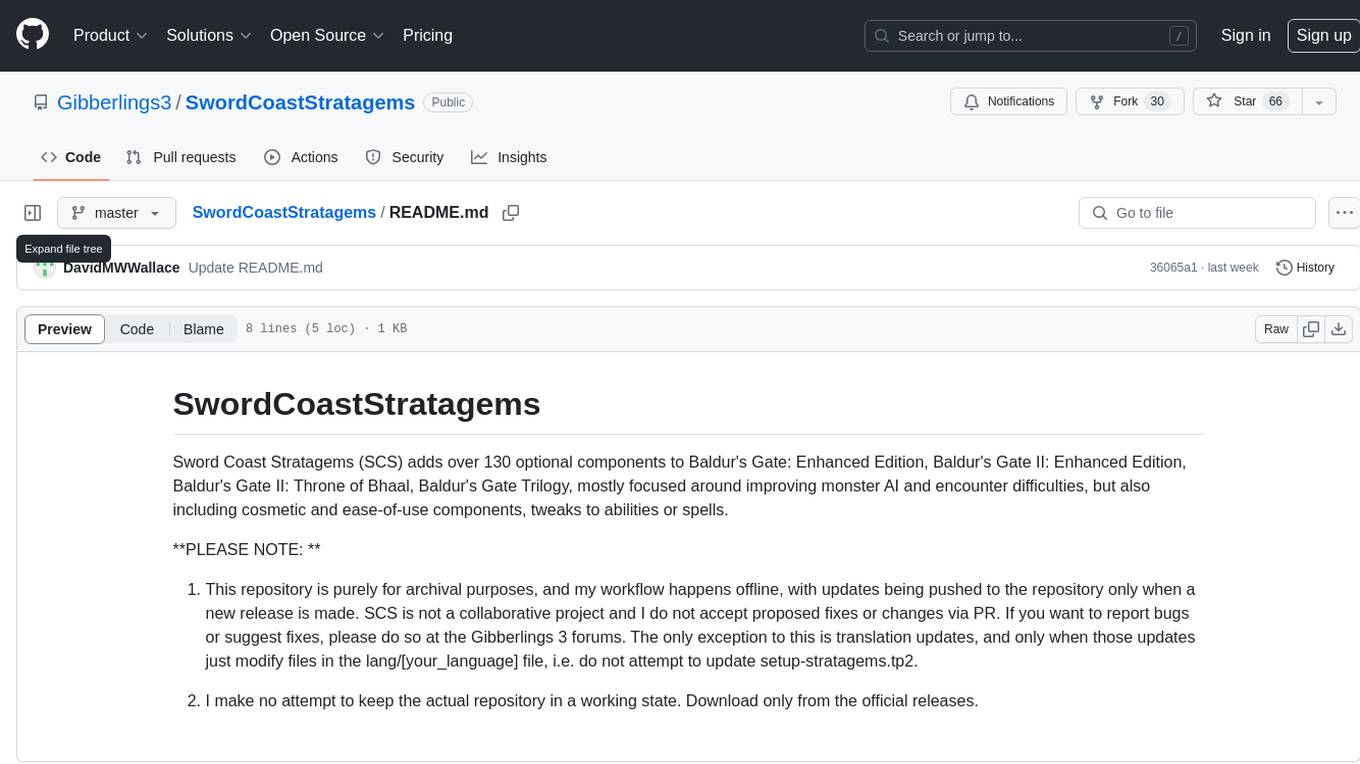
SwordCoastStratagems
Sword Coast Stratagems (SCS) is a mod that enhances Baldur's Gate games by adding over 130 optional components focused on improving monster AI, encounter difficulties, cosmetic enhancements, and ease-of-use tweaks. This repository serves as an archive for the project, with updates pushed only when new releases are made. It is not a collaborative project, and bug reports or suggestions should be made at the Gibberlings 3 forums. The mod is designed for offline workflow and should be downloaded from official releases.
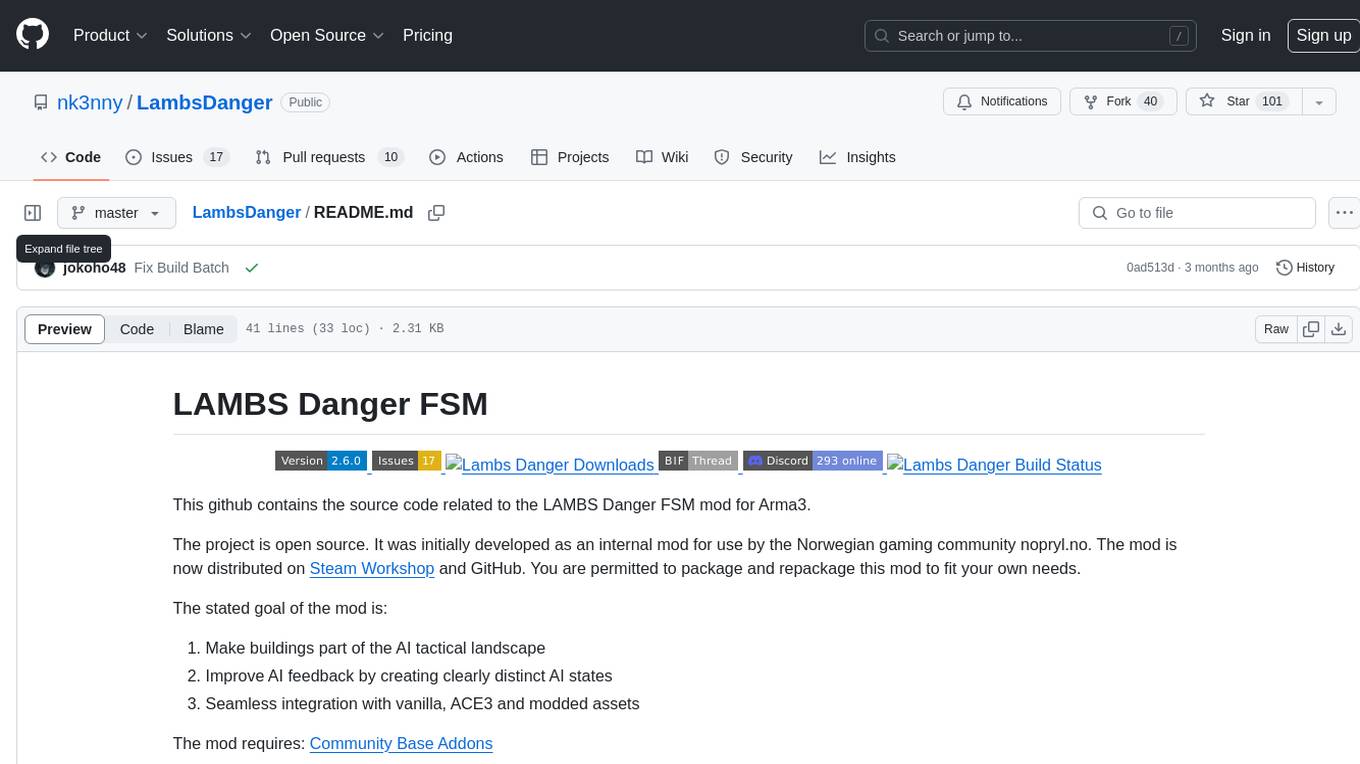
LambsDanger
LAMBS Danger FSM is an open-source mod developed for Arma3, aimed at enhancing the AI behavior by integrating buildings into the tactical landscape, creating distinct AI states, and ensuring seamless compatibility with vanilla, ACE3, and modded assets. Originally created for the Norwegian gaming community, it is now available on Steam Workshop and GitHub for wider use. Users are allowed to customize and redistribute the mod according to their requirements. The project is licensed under the GNU General Public License (GPLv2) with additional amendments.
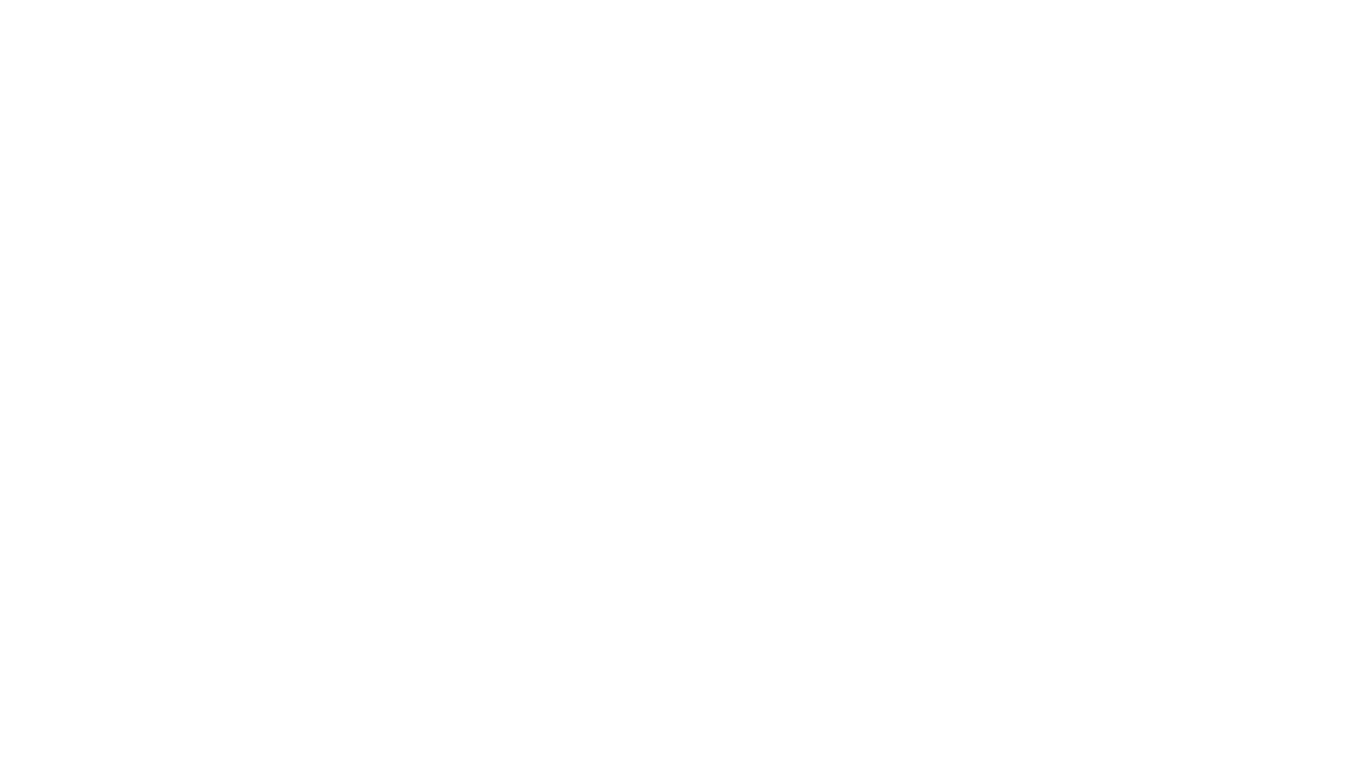
beehave
Beehave is a powerful addon for Godot Engine that enables users to create robust AI systems using behavior trees. It simplifies the design of complex NPC behaviors, challenging boss battles, and other advanced setups. Beehave allows for the creation of highly adaptive AI that responds to changes in the game world and overcomes unexpected obstacles, catering to both beginners and experienced developers. The tool is currently in development for version 3.0.
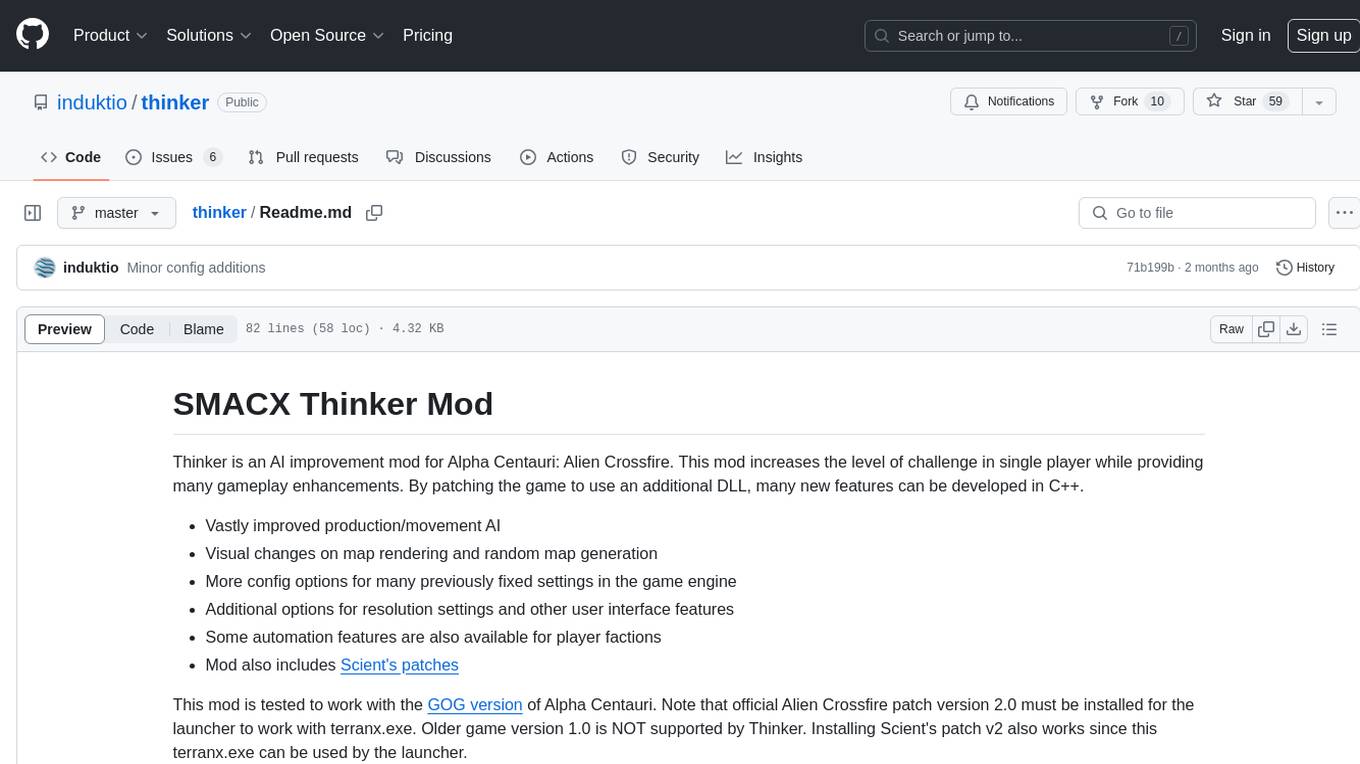
thinker
Thinker is an AI improvement mod for Alpha Centauri: Alien Crossfire that enhances single player challenge and gameplay with features like improved production/movement AI, visual changes on map rendering, more config options, resolution settings, and automation features. It includes Scient's patches and requires the GOG version of Alpha Centauri with the official Alien Crossfire patch version 2.0 installed. The mod provides additional DLL features developed in C++ for a richer gaming experience.
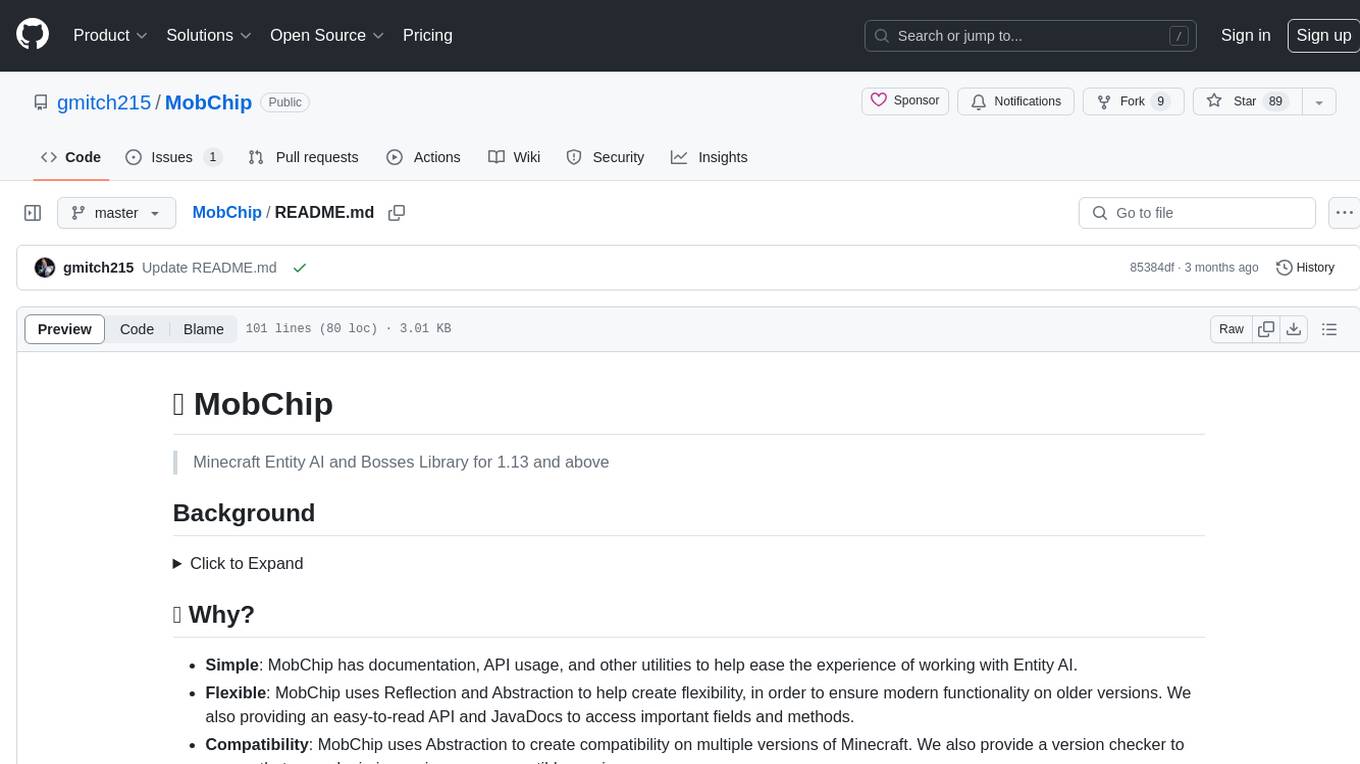
MobChip
MobChip is an all-in-one Entity AI and Bosses Library for Minecraft 1.13 and above. It simplifies the implementation of Minecraft's native entity AI into plugins, offering documentation, API usage, and utilities for ease of use. The library is flexible, using Reflection and Abstraction for modern functionality on older versions, and ensuring compatibility across multiple Minecraft versions. MobChip is open source, providing features like Bosses Library, Pathfinder Goals, Behaviors, Villager Gossip, Ender Dragon Phases, and more.