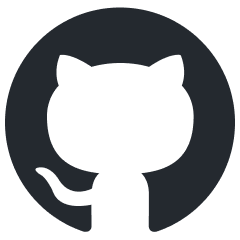
azure-functions-openai-extension
An extension that adds support for Azure OpenAI/ OpenAI bindings in Azure Functions for LLM (GPT-3.5-Turbo, GPT-4, etc)
Stars: 87
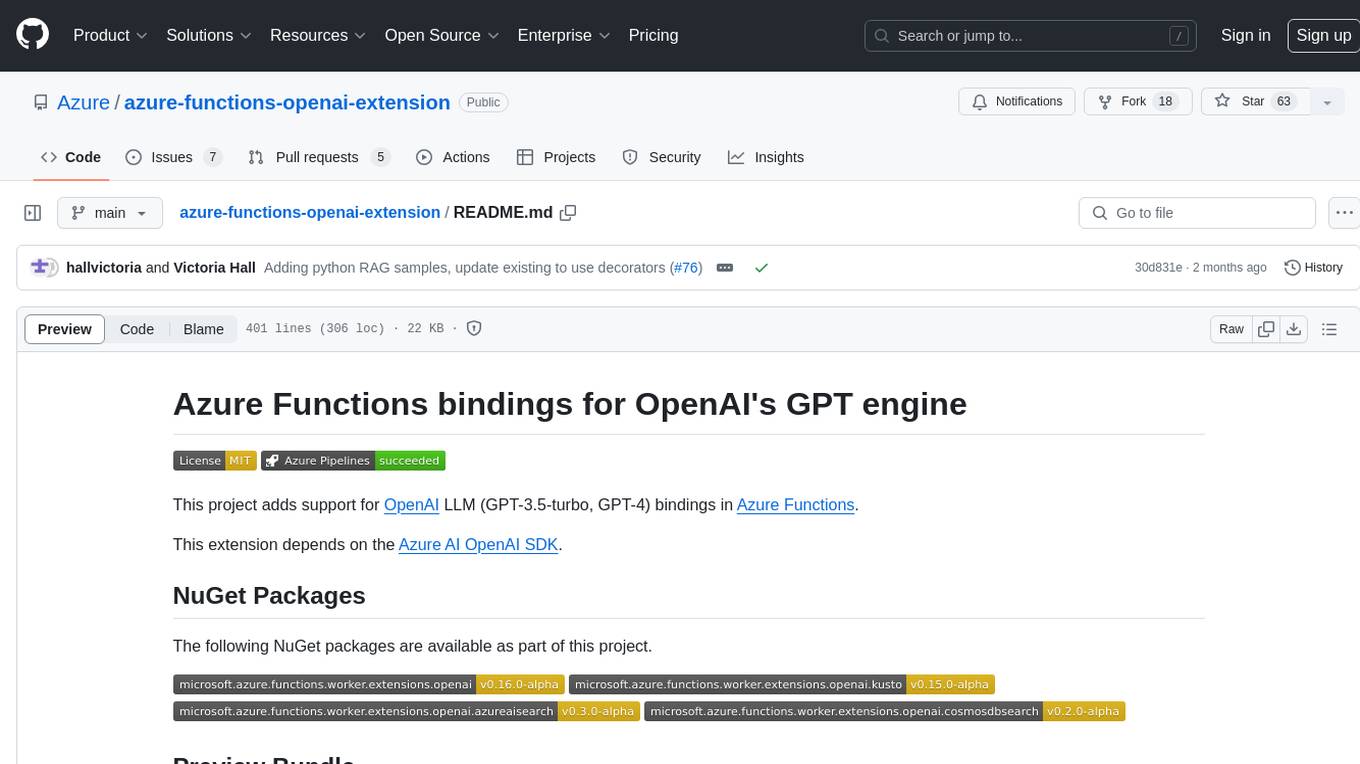
Azure Functions OpenAI Extension is a project that adds support for OpenAI LLM (GPT-3.5-turbo, GPT-4) bindings in Azure Functions. It provides NuGet packages for various functionalities like text completions, chat completions, assistants, embeddings generators, and semantic search. The project requires .NET 6 SDK or greater, Azure Functions Core Tools v4.x, and specific settings in Azure Function or local settings for development. It offers features like text completions, chat completion, assistants with custom skills, embeddings generators for text relatedness, and semantic search using vector databases. The project also includes examples in C# and Python for different functionalities.
README:
This project adds support for OpenAI LLM (GPT-3.5-turbo, GPT-4) bindings in Azure Functions.
This extension depends on the Azure AI OpenAI SDK.
The following NuGet packages are available as part of this project.
Add following section to host.json
of the function app for non dotnet languages to utilise the preview bundle and consume extension packages:
"extensionBundle": {
"id": "Microsoft.Azure.Functions.ExtensionBundle.Preview",
"version": "[4.*, 5.0.0)"
}
- .NET 6 SDK or greater (Visual Studio 2022 recommended)
- Azure Functions Core Tools v4.x
- Update settings in Azure Function or the
local.settings.json
file for local development with the following keys:- For Azure,
AZURE_OPENAI_ENDPOINT
- Azure OpenAI resource (e.g.https://***.openai.azure.com/
) set. - For Azure, assign the user or function app managed identity
Cognitive Services OpenAI User
role on the Azure OpenAI resource. It is strongly recommended to use managed identity to avoid overhead of secrets maintenance, however if there is a need for key based authentication add the settingAZURE_OPENAI_KEY
and its value in the settings. - For non- Azure,
OPENAI_API_KEY
- An OpenAI account and an API key saved into a setting.
If using environment variables, Learn more in .env readme. - Update
CHAT_MODEL_DEPLOYMENT_NAME
andEMBEDDING_MODEL_DEPLOYMENT_NAME
keys to Azure Deployment names or override default OpenAI model names. - If using user assigned managed identity, add
AZURE_CLIENT_ID
to environment variable settings with value as client id of the managed identity. - Visit binding specific samples README for additional settings that might be required for each binding.
- For Azure,
- Azure Storage emulator such as Azurite running in the background
- The target language runtime (e.g. dotnet, nodejs, powershell, python, java) installed on your machine. Refer the official supported versions.
The following features are currently available. More features will be slowly added over time. The language stack specific samples are also available in this repo for dotnet-isolated, java, nodejs, powershell and python. Visit the feature specific folder for utilising those.
The textCompletion
input binding can be used to invoke the OpenAI Chat Completions API and return the results to the function.
The examples below define "who is" HTTP-triggered functions with a hardcoded "who is {name}?"
prompt, where {name}
is the substituted with the value in the HTTP request path. The OpenAI input binding invokes the OpenAI GPT endpoint to surface the answer to the prompt to the function, which then returns the result text as the response content.
Setting a model is optional for non-Azure OpenAI, see here for default model values for OpenAI.
[Function(nameof(WhoIs))]
public static HttpResponseData WhoIs(
[HttpTrigger(AuthorizationLevel.Function, Route = "whois/{name}")] HttpRequestData req,
[TextCompletionInput("Who is {name}?")] TextCompletionResponse response)
{
HttpResponseData responseData = req.CreateResponse(HttpStatusCode.OK);
responseData.WriteString(response.Content);
return responseData;
}
Setting a model is optional for non-Azure OpenAI, see here for default model values for OpenAI.
@app.route(route="whois/{name}", methods=["GET"])
@app.text_completion_input(arg_name="response", prompt="Who is {name}?", max_tokens="100", model = "%CHAT_MODEL_DEPLOYMENT_NAME%")
def whois(req: func.HttpRequest, response: str) -> func.HttpResponse:
response_json = json.loads(response)
return func.HttpResponse(response_json["content"], status_code=200)
Chat completions are useful for building AI-powered assistants.
There are three bindings you can use to interact with the assistant:
- The
assistantCreate
output binding creates a new assistant with a specified system prompt. - The
assistantPost
output binding sends a message to the assistant and saves the response in its internal state. - The
assistantQuery
input binding fetches the assistant history and passes it to the function.
You can find samples in multiple languages with instructions in the chat samples directory.
Assistants build on top of the chat functionality to provide assistants with custom skills defined as functions. This internally uses the function calling feature of OpenAIs GPT models to select which functions to invoke and when.
You can define functions that can be triggered by assistants by using the assistantSkillTrigger
trigger binding.
These functions are invoked by the extension when a assistant signals that it would like to invoke a function in response to a user prompt.
The name of the function, the description provided by the trigger, and the parameter name are all hints that the underlying language model use to determine when and how to invoke an assistant function.
public class AssistantSkills
{
readonly ITodoManager todoManager;
readonly ILogger<AssistantSkills> logger;
// This constructor is called by the Azure Functions runtime's dependency injection container.
public AssistantSkills(ITodoManager todoManager, ILogger<AssistantSkills> logger)
{
this.todoManager = todoManager ?? throw new ArgumentNullException(nameof(todoManager));
this.logger = logger ?? throw new ArgumentNullException(nameof(logger));
}
// Called by the assistant to create new todo tasks.
[Function(nameof(AddTodo))]
public Task AddTodo([AssistantSkillTrigger("Create a new todo task")] string taskDescription)
{
if (string.IsNullOrEmpty(taskDescription))
{
throw new ArgumentException("Task description cannot be empty");
}
this.logger.LogInformation("Adding todo: {task}", taskDescription);
string todoId = Guid.NewGuid().ToString()[..6];
return this.todoManager.AddTodoAsync(new TodoItem(todoId, taskDescription));
}
// Called by the assistant to fetch the list of previously created todo tasks.
[Function(nameof(GetTodos))]
public Task<IReadOnlyList<TodoItem>> GetTodos(
[AssistantSkillTrigger("Fetch the list of previously created todo tasks")] object inputIgnored)
{
this.logger.LogInformation("Fetching list of todos");
return this.todoManager.GetTodosAsync();
}
}
You can find samples in multiple languages with instructions in the assistant samples directory.
OpenAI's text embeddings measure the relatedness of text strings. Embeddings are commonly used for:
- Search (where results are ranked by relevance to a query string)
- Clustering (where text strings are grouped by similarity)
- Recommendations (where items with related text strings are recommended)
- Anomaly detection (where outliers with little relatedness are identified)
- Diversity measurement (where similarity distributions are analyzed)
- Classification (where text strings are classified by their most similar label)
Processing of the source text files typically involves chunking the text into smaller pieces, such as sentences or paragraphs, and then making an OpenAI call to produce embeddings for each chunk independently. Finally, the embeddings need to be stored in a database or other data store for later use.
[Function(nameof(GenerateEmbeddings_Http_RequestAsync))]
public async Task GenerateEmbeddings_Http_RequestAsync(
[HttpTrigger(AuthorizationLevel.Function, "post", Route = "embeddings")] HttpRequestData req,
[EmbeddingsInput("{RawText}", InputType.RawText)] EmbeddingsContext embeddings)
{
using StreamReader reader = new(req.Body);
string request = await reader.ReadToEndAsync();
EmbeddingsRequest? requestBody = JsonSerializer.Deserialize<EmbeddingsRequest>(request);
this.logger.LogInformation(
"Received {count} embedding(s) for input text containing {length} characters.",
embeddings.Count,
requestBody.RawText.Length);
// TODO: Store the embeddings into a database or other storage.
}
@app.function_name("GenerateEmbeddingsHttpRequest")
@app.route(route="embeddings", methods=["POST"])
@app.embeddings_input(arg_name="embeddings", input="{rawText}", input_type="rawText", model="%EMBEDDING_MODEL_DEPLOYMENT_NAME%")
def generate_embeddings_http_request(req: func.HttpRequest, embeddings: str) -> func.HttpResponse:
user_message = req.get_json()
embeddings_json = json.loads(embeddings)
embeddings_request = {
"raw_text": user_message.get("RawText"),
"file_path": user_message.get("FilePath")
}
logging.info(f'Received {embeddings_json.get("count")} embedding(s) for input text '
f'containing {len(embeddings_request.get("raw_text"))} characters.')
# TODO: Store the embeddings into a database or other storage.
return func.HttpResponse(status_code=200)
The semantic search feature allows you to import documents into a vector database using an output binding and query the documents in that database using an input binding. For example, you can have a function that imports documents into a vector database and another function that issues queries to OpenAI using content stored in the vector database as context (also known as the Retrieval Augmented Generation, or RAG technique).
The supported list of vector databases is extensible, and more can be added by authoring a specially crafted NuGet package. Visit the currently supported vector specific folder for specific usage information:
- Azure AI Search - See source code
- Azure Data Explorer - See source code
- Azure Cosmos DB using MongoDB (vCore) - See source code
More may be added over time.
This HTTP trigger function takes a URL of a file as input, generates embeddings for the file, and stores the result into an Azure AI Search Index.
public class EmbeddingsRequest
{
[JsonPropertyName("url")]
public string? Url { get; set; }
}
[Function("IngestFile")]
public static async Task<EmbeddingsStoreOutputResponse> IngestFile(
[HttpTrigger(AuthorizationLevel.Function, "post")] HttpRequestData req)
{
using StreamReader reader = new(req.Body);
string request = await reader.ReadToEndAsync();
EmbeddingsStoreOutputResponse badRequestResponse = new()
{
HttpResponse = new BadRequestResult(),
SearchableDocument = new SearchableDocument(string.Empty)
};
if (string.IsNullOrWhiteSpace(request))
{
return badRequestResponse;
}
EmbeddingsRequest? requestBody = JsonSerializer.Deserialize<EmbeddingsRequest>(request);
if (string.IsNullOrWhiteSpace(requestBody?.Url))
{
throw new ArgumentException("Invalid request body. Make sure that you pass in {\"url\": value } as the request body.");
}
if (!Uri.TryCreate(requestBody.Url, UriKind.Absolute, out Uri? uri))
{
return badRequestResponse;
}
string filename = Path.GetFileName(uri.AbsolutePath);
return new EmbeddingsStoreOutputResponse
{
HttpResponse = new OkObjectResult(new { status = HttpStatusCode.OK }),
SearchableDocument = new SearchableDocument(filename)
};
}
public class EmbeddingsStoreOutputResponse
{
[EmbeddingsStoreOutput("{url}", InputType.Url, "AISearchEndpoint", "openai-index", Model = "%EMBEDDING_MODEL_DEPLOYMENT_NAME%")]
public required SearchableDocument SearchableDocument { get; init; }
public IActionResult? HttpResponse { get; set; }
}
@app.function_name("IngestFile")
@app.route(methods=["POST"])
@app.embeddings_store_output(arg_name="requests", input="{url}", input_type="url", connection_name="AISearchEndpoint", collection="openai-index", model="%EMBEDDING_MODEL_DEPLOYMENT_NAME%")
def ingest_file(req: func.HttpRequest, requests: func.Out[str]) -> func.HttpResponse:
user_message = req.get_json()
if not user_message:
return func.HttpResponse(json.dumps({"message": "No message provided"}), status_code=400, mimetype="application/json")
file_name_with_extension = os.path.basename(user_message["url"])
title = os.path.splitext(file_name_with_extension)[0]
create_request = {
"title": title
}
requests.set(json.dumps(create_request))
response_json = {
"status": "success",
"title": title
}
return func.HttpResponse(json.dumps(response_json), status_code=200, mimetype="application/json")
Tip - To improve context preservation between chunks in case of large documents, specify the max overlap between chunks and also the chunk size. The default values for MaxChunkSize
and MaxOverlap
are 8 * 1024 and 128 characters respectively.
This HTTP trigger function takes a query prompt as input, pulls in semantically similar document chunks into a prompt, and then sends the combined prompt to OpenAI. The results are then made available to the function, which simply returns that chat response to the caller.
Tip - To improve the knowledge for OpenAI model, the number of result sets being sent to the model with system prompt can be increased with binding property - MaxKnowledgeCount
which has default value as 1. Also, the SystemPrompt
in SemanticSearchRequest can be tweaked as per user instructions on how to process the knowledge sets being appended to it.
public class SemanticSearchRequest
{
[JsonPropertyName("prompt")]
public string? Prompt { get; set; }
}
[Function("PromptFile")]
public static IActionResult PromptFile(
[HttpTrigger(AuthorizationLevel.Function, "post")] SemanticSearchRequest unused,
[SemanticSearchInput("AISearchEndpoint", "openai-index", Query = "{prompt}", ChatModel = "%CHAT_MODEL_DEPLOYMENT_NAME%", EmbeddingsModel = "%EMBEDDING_MODEL_DEPLOYMENT_NAME%")] SemanticSearchContext result)
{
return new ContentResult { Content = result.Response, ContentType = "text/plain" };
}
@app.function_name("PromptFile")
@app.route(methods=["POST"])
@app.semantic_search_input(arg_name="result", connection_name="AISearchEndpoint", collection="openai-index", query="{prompt}", embeddings_model="%EMBEDDING_MODEL_DEPLOYMENT_NAME%", chat_model="%CHAT_MODEL_DEPLOYMENT_NAME%")
def prompt_file(req: func.HttpRequest, result: str) -> func.HttpResponse:
result_json = json.loads(result)
response_json = {
"content": result_json.get("Response"),
"content_type": "text/plain"
}
return func.HttpResponse(json.dumps(response_json), status_code=200, mimetype="application/json")
The responses from the above function will be based on relevant document snippets which were previously uploaded to the vector database. For example, assuming you uploaded internal emails discussing a new feature of Azure Functions that supports OpenAI, you could issue a query similar to the following:
POST http://localhost:7127/api/PromptFile
Content-Type: application/json
{
"prompt": "Was a decision made to officially release an OpenAI binding for Azure Functions?"
}
And you might get a response that looks like the following (actual results may vary):
HTTP/1.1 200 OK
Content-Length: 454
Content-Type: text/plain
There is no clear decision made on whether to officially release an OpenAI binding for Azure Functions as per the email "Thoughts on Functions+AI conversation" sent by Bilal. However, he suggests that the team should figure out if they are able to free developers from needing to know the details of AI/LLM APIs by sufficiently/elegantly designing bindings to let them do the "main work" they need to do. Reference: Thoughts on Functions+AI conversation.
IMPORTANT: Azure OpenAI requires you to specify a deployment when making API calls instead of a model. The deployment is a specific instance of a model that you have deployed to your Azure OpenAI resource. In order to make code more portable across OpenAI and Azure OpenAI, the bindings in this extension use the Model
, ChatModel
and EmbeddingsModel
to refer to either the OpenAI model or the Azure OpenAI deployment ID, depending on whether you're using OpenAI or Azure OpenAI.
All samples in this project rely on default model selection, which assumes the models are named after the OpenAI models. If you want to use an Azure OpenAI deployment, you'll want to configure the Model
, ChatModel
and EmbeddingsModel
properties explicitly in your binding configuration. Here are a couple examples:
// "gpt-35-turbo" is the name of an Azure OpenAI deployment
[Function(nameof(WhoIs))]
public static string WhoIs(
[HttpTrigger(AuthorizationLevel.Function, Route = "whois/{name}")] HttpRequest req,
[TextCompletion("Who is {name}?", Model = "gpt-35-turbo")] TextCompletionResponse response)
{
return response.Content;
}
public class SemanticSearchRequest
{
[JsonPropertyName("prompt")]
public string? Prompt { get; set; }
}
// "my-gpt-4" and "my-ada-2" are the names of Azure OpenAI deployments corresponding to gpt-4 and text-embedding-ada-002 models, respectively
[Function("PromptEmail")]
public IActionResult PromptEmail(
[HttpTrigger(AuthorizationLevel.Function, "post")] SemanticSearchRequest unused,
[SemanticSearchInput("KustoConnectionString", "Documents", Query = "{prompt}", ChatModel = "my-gpt-4", EmbeddingsModel = "my-ada-2")] SemanticSearchContext result)
{
return new ContentResult { Content = result.Response, ContentType = "text/plain" };
}
- Chat Completion - gpt-3.5-turbo
- Embeddings - text-embedding-ada-002
- Text Completion - gpt-3.5-turbo
While using non-Azure OpenAI, you can omit the Model specification in attributes to use the default models.
This project welcomes contributions and suggestions. Most contributions require you to agree to a Contributor License Agreement (CLA) declaring that you have the right to, and actually do, grant us the rights to use your contribution. For details, visit https://cla.opensource.microsoft.com.
When you submit a pull request, a CLA bot will automatically determine whether you need to provide a CLA and decorate the PR appropriately (e.g., status check, comment). Simply follow the instructions provided by the bot. You will only need to do this once across all repos using our CLA.
This project has adopted the Microsoft Open Source Code of Conduct. For more information see the Code of Conduct FAQ or contact [email protected] with any additional questions or comments.
This project may contain trademarks or logos for projects, products, or services. Authorized use of Microsoft trademarks or logos is subject to and must follow Microsoft's Trademark & Brand Guidelines. Use of Microsoft trademarks or logos in modified versions of this project must not cause confusion or imply Microsoft sponsorship. Any use of third-party trademarks or logos are subject to those third-party's policies.
For Tasks:
Click tags to check more tools for each tasksFor Jobs:
Alternative AI tools for azure-functions-openai-extension
Similar Open Source Tools
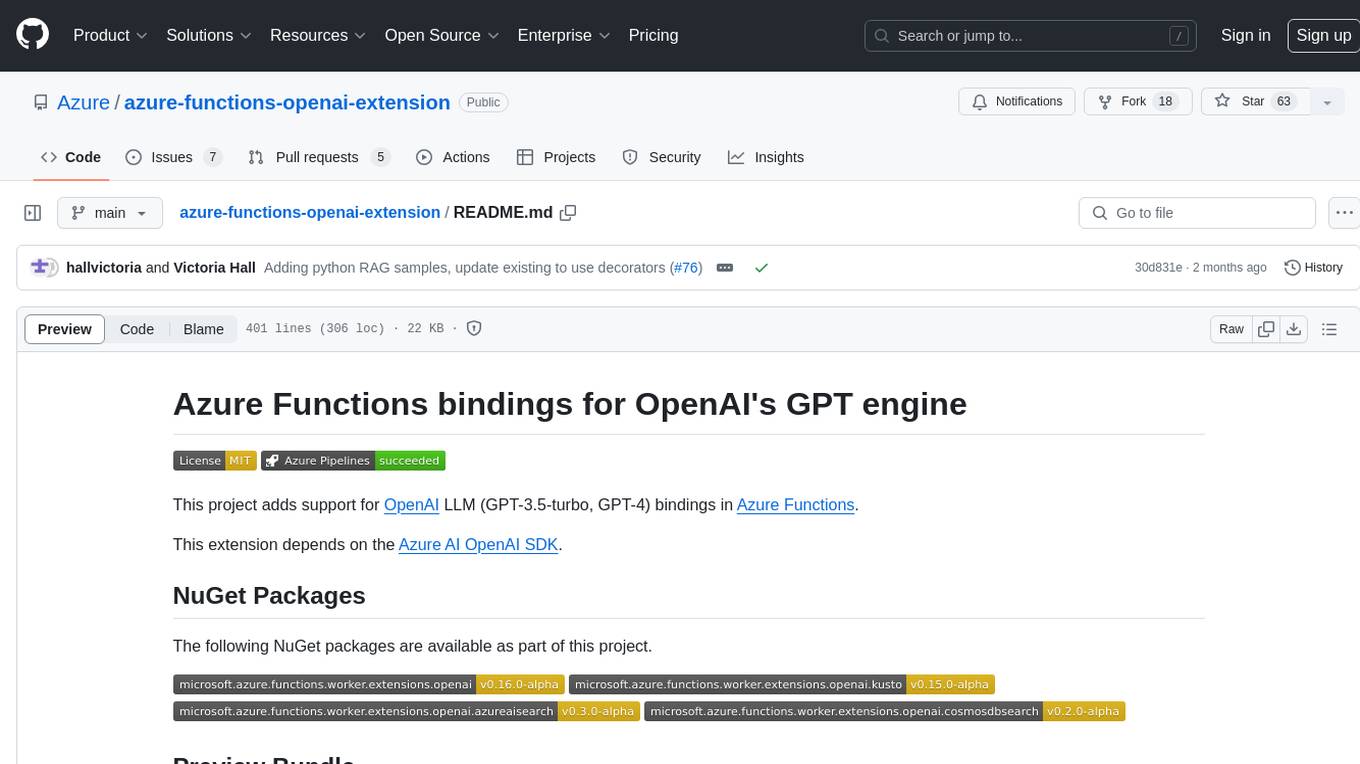
azure-functions-openai-extension
Azure Functions OpenAI Extension is a project that adds support for OpenAI LLM (GPT-3.5-turbo, GPT-4) bindings in Azure Functions. It provides NuGet packages for various functionalities like text completions, chat completions, assistants, embeddings generators, and semantic search. The project requires .NET 6 SDK or greater, Azure Functions Core Tools v4.x, and specific settings in Azure Function or local settings for development. It offers features like text completions, chat completion, assistants with custom skills, embeddings generators for text relatedness, and semantic search using vector databases. The project also includes examples in C# and Python for different functionalities.
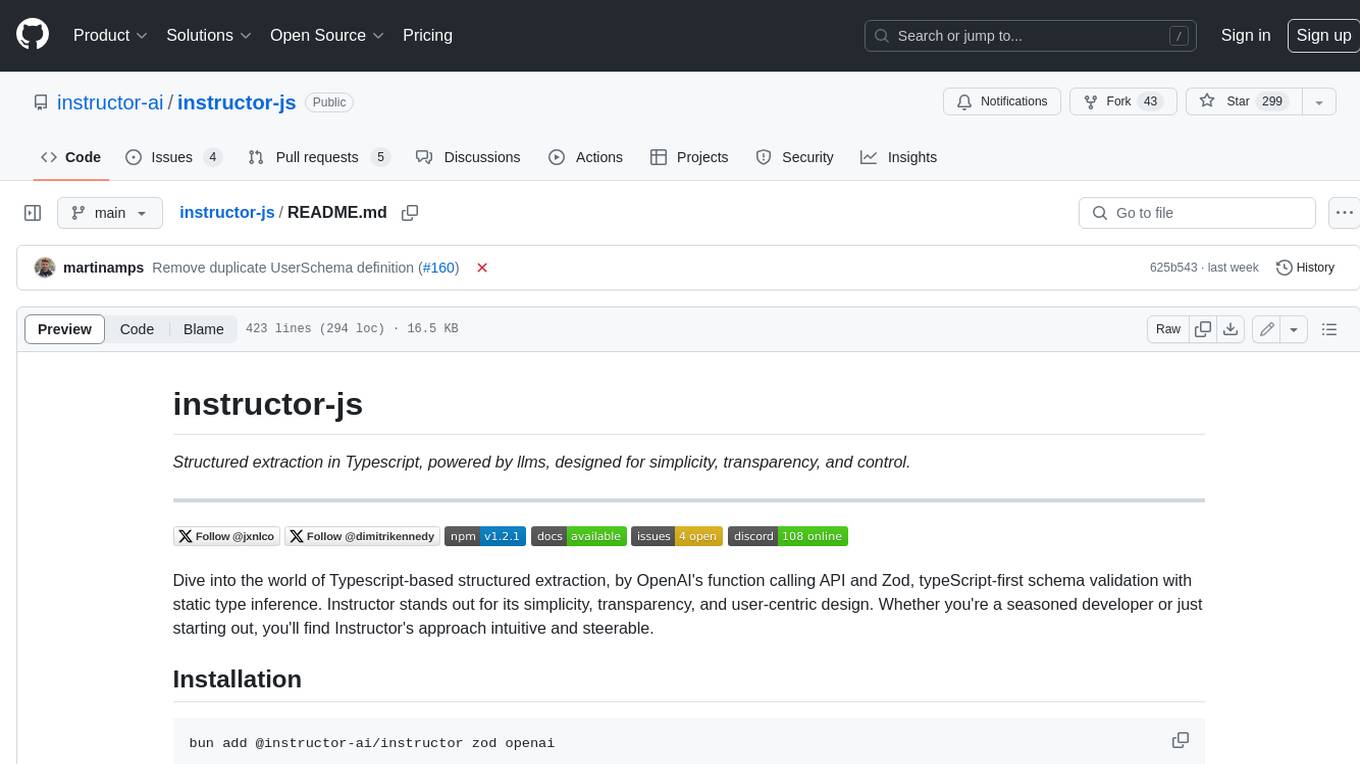
instructor-js
Instructor is a Typescript library for structured extraction in Typescript, powered by llms, designed for simplicity, transparency, and control. It stands out for its simplicity, transparency, and user-centric design. Whether you're a seasoned developer or just starting out, you'll find Instructor's approach intuitive and steerable.
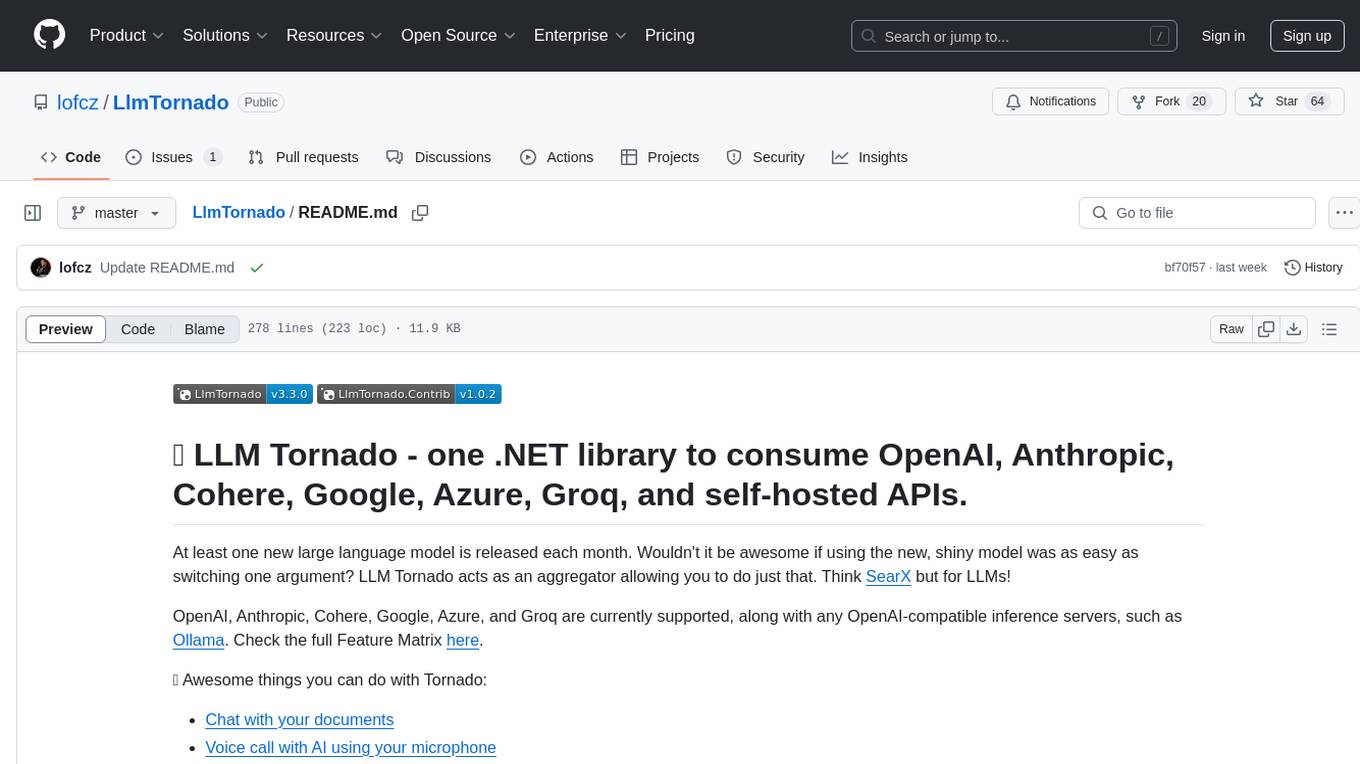
LlmTornado
LLM Tornado is a .NET library designed to simplify the consumption of various large language models (LLMs) from providers like OpenAI, Anthropic, Cohere, Google, Azure, Groq, and self-hosted APIs. It acts as an aggregator, allowing users to easily switch between different LLM providers with just a change in argument. Users can perform tasks such as chatting with documents, voice calling with AI, orchestrating assistants, generating images, and more. The library exposes capabilities through vendor extensions, making it easy to integrate and use multiple LLM providers simultaneously.
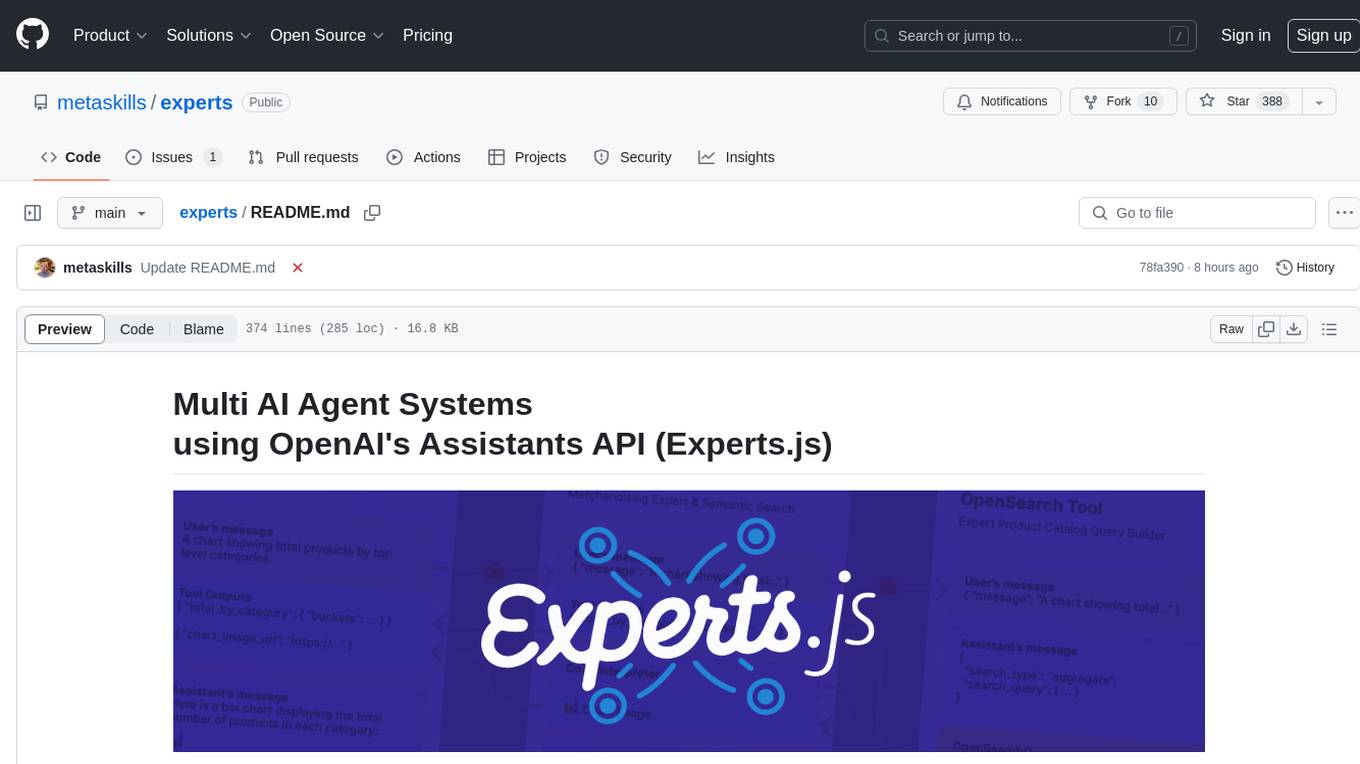
experts
Experts.js is a tool that simplifies the creation and deployment of OpenAI's Assistants, allowing users to link them together as Tools to create a Panel of Experts system with expanded memory and attention to detail. It leverages the new Assistants API from OpenAI, which offers advanced features such as referencing attached files & images as knowledge sources, supporting instructions up to 256,000 characters, integrating with 128 tools, and utilizing the Vector Store API for efficient file search. Experts.js introduces Assistants as Tools, enabling the creation of Multi AI Agent Systems where each Tool is an LLM-backed Assistant that can take on specialized roles or fulfill complex tasks.
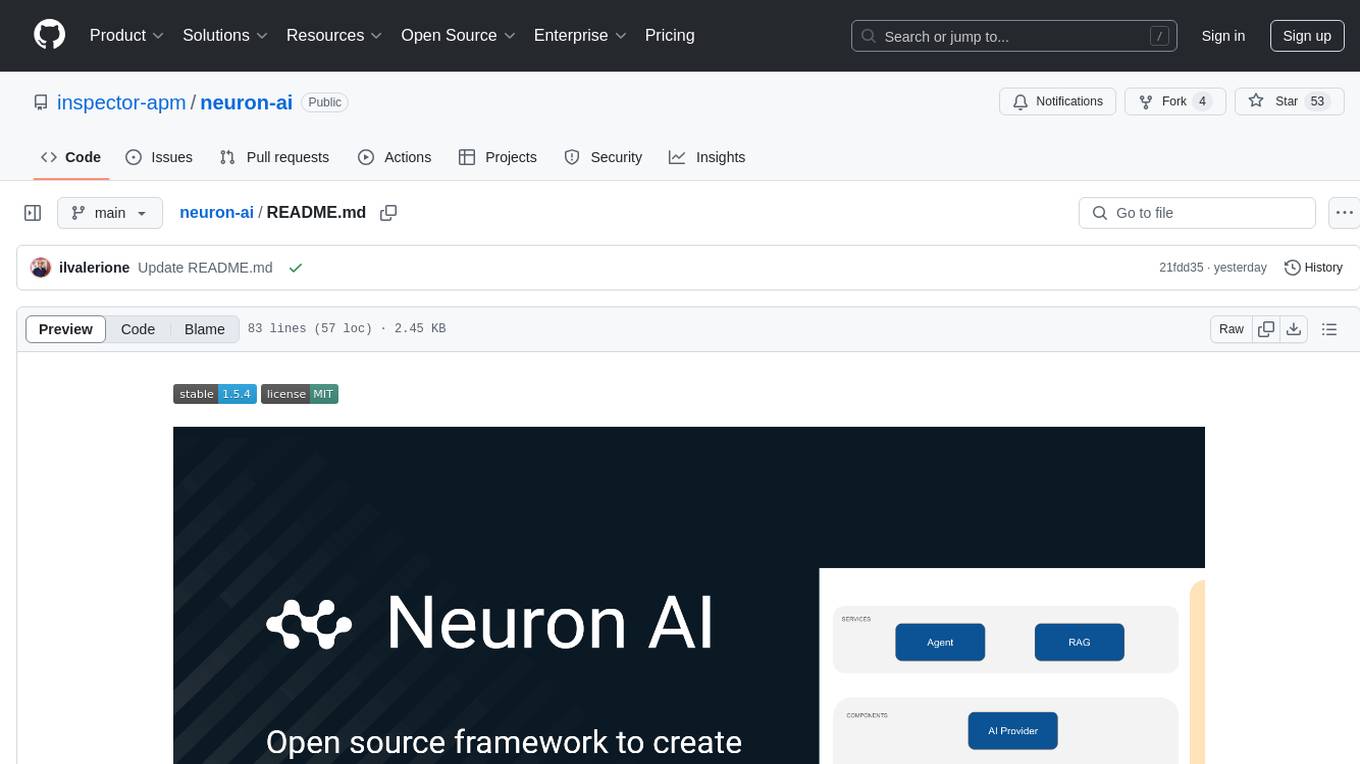
neuron-ai
Neuron AI is a PHP framework that provides an Agent class for creating fully functional agents to perform tasks like analyzing text for SEO optimization. The framework manages advanced mechanisms such as memory, tools, and function calls. Users can extend the Agent class to create custom agents and interact with them to get responses based on the underlying LLM. Neuron AI aims to simplify the development of AI-powered applications by offering a structured framework with documentation and guidelines for contributions under the MIT license.
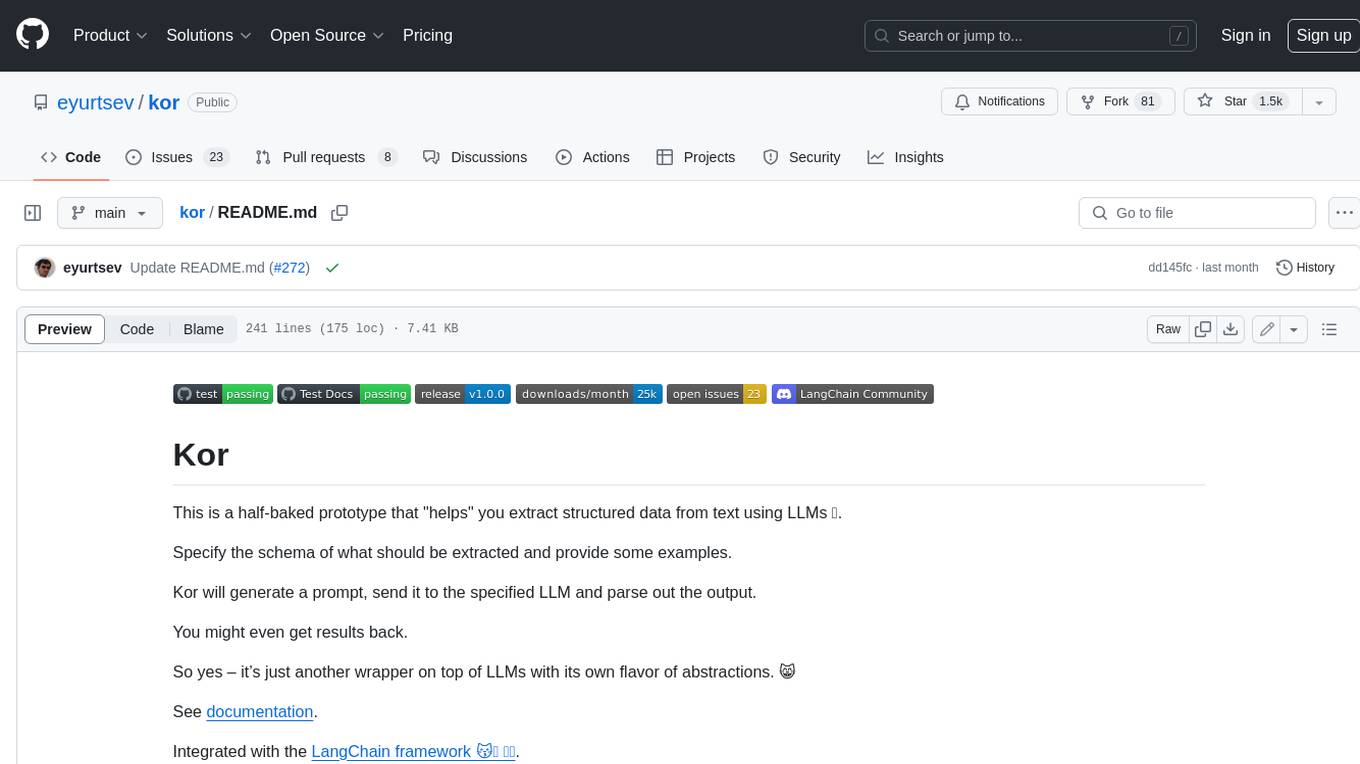
kor
Kor is a prototype tool designed to help users extract structured data from text using Language Models (LLMs). It generates prompts, sends them to specified LLMs, and parses the output. The tool works with the parsing approach and is integrated with the LangChain framework. Kor is compatible with pydantic v2 and v1, and schema is typed checked using pydantic. It is primarily used for extracting information from text based on provided reference examples and schema documentation. Kor is designed to work with all good-enough LLMs regardless of their support for function/tool calling or JSON modes.
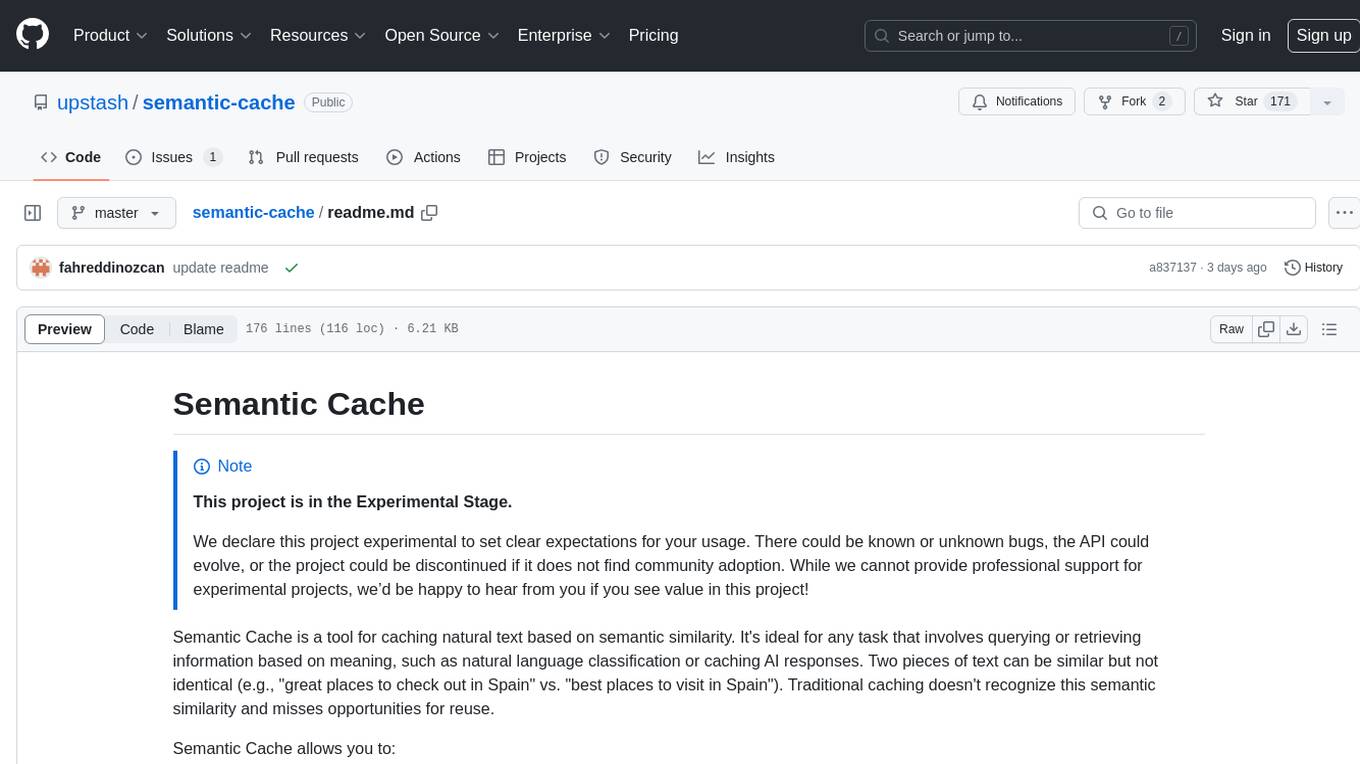
semantic-cache
Semantic Cache is a tool for caching natural text based on semantic similarity. It allows for classifying text into categories, caching AI responses, and reducing API latency by responding to similar queries with cached values. The tool stores cache entries by meaning, handles synonyms, supports multiple languages, understands complex queries, and offers easy integration with Node.js applications. Users can set a custom proximity threshold for filtering results. The tool is ideal for tasks involving querying or retrieving information based on meaning, such as natural language classification or caching AI responses.
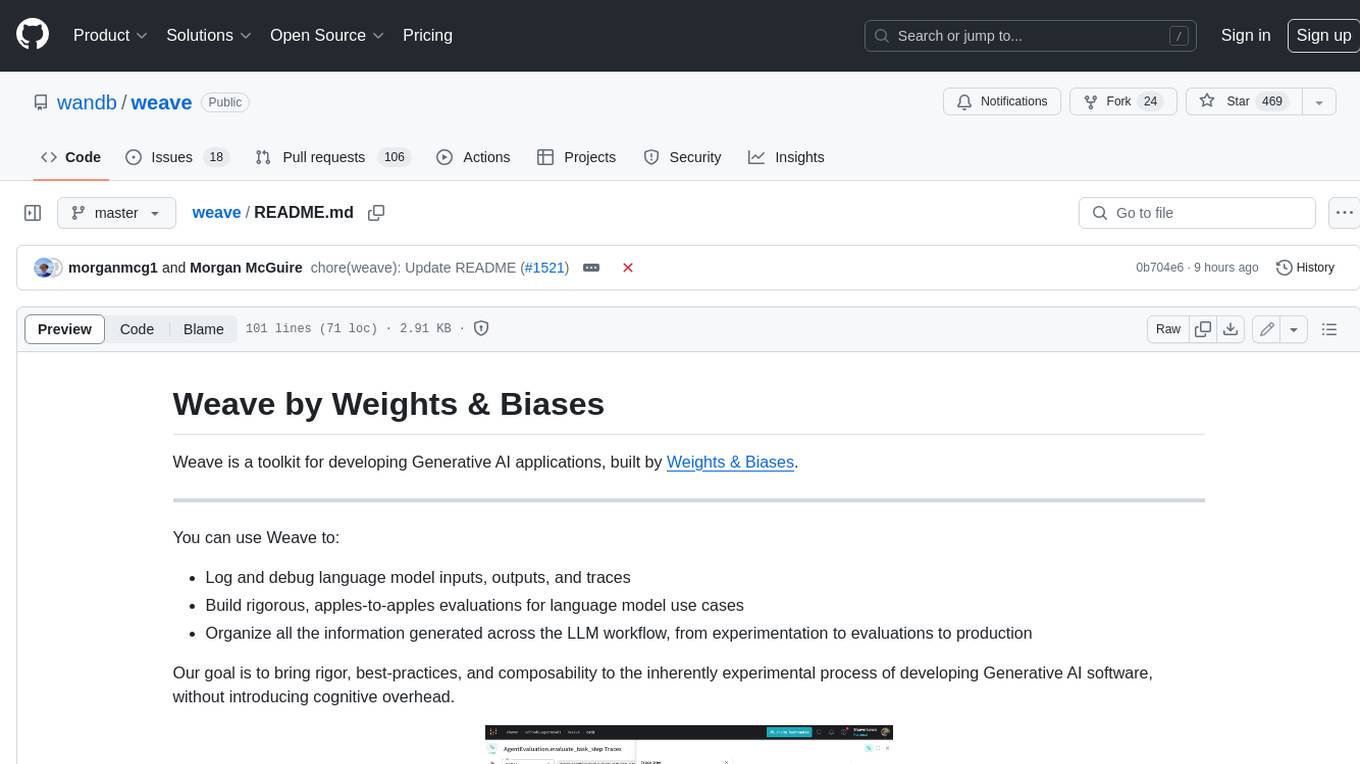
weave
Weave is a toolkit for developing Generative AI applications, built by Weights & Biases. With Weave, you can log and debug language model inputs, outputs, and traces; build rigorous, apples-to-apples evaluations for language model use cases; and organize all the information generated across the LLM workflow, from experimentation to evaluations to production. Weave aims to bring rigor, best-practices, and composability to the inherently experimental process of developing Generative AI software, without introducing cognitive overhead.
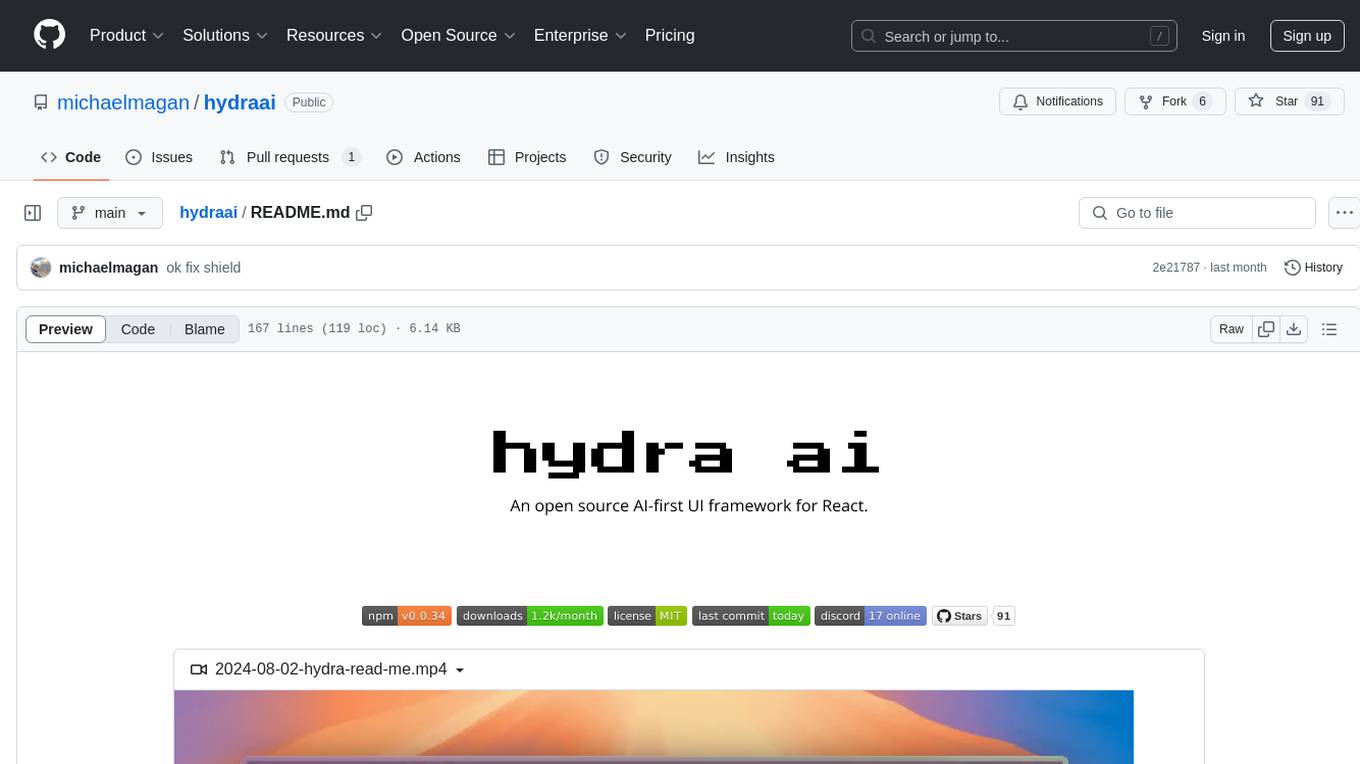
hydraai
Generate React components on-the-fly at runtime using AI. Register your components, and let Hydra choose when to show them in your App. Hydra development is still early, and patterns for different types of components and apps are still being developed. Join the discord to chat with the developers. Expects to be used in a NextJS project. Components that have function props do not work.
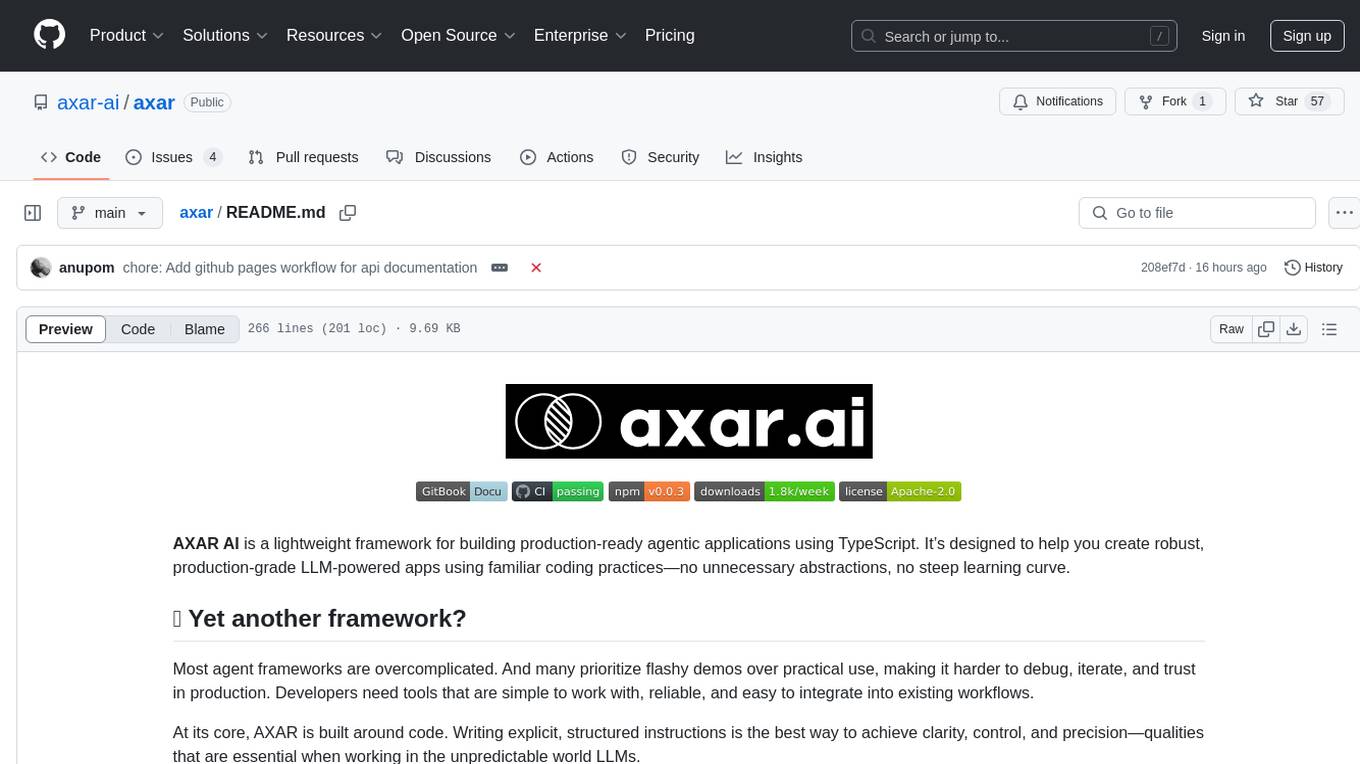
axar
AXAR AI is a lightweight framework designed for building production-ready agentic applications using TypeScript. It aims to simplify the process of creating robust, production-grade LLM-powered apps by focusing on familiar coding practices without unnecessary abstractions or steep learning curves. The framework provides structured, typed inputs and outputs, familiar and intuitive patterns like dependency injection and decorators, explicit control over agent behavior, real-time logging and monitoring tools, minimalistic design with little overhead, model agnostic compatibility with various AI models, and streamed outputs for fast and accurate results. AXAR AI is ideal for developers working on real-world AI applications who want a tool that gets out of the way and allows them to focus on shipping reliable software.
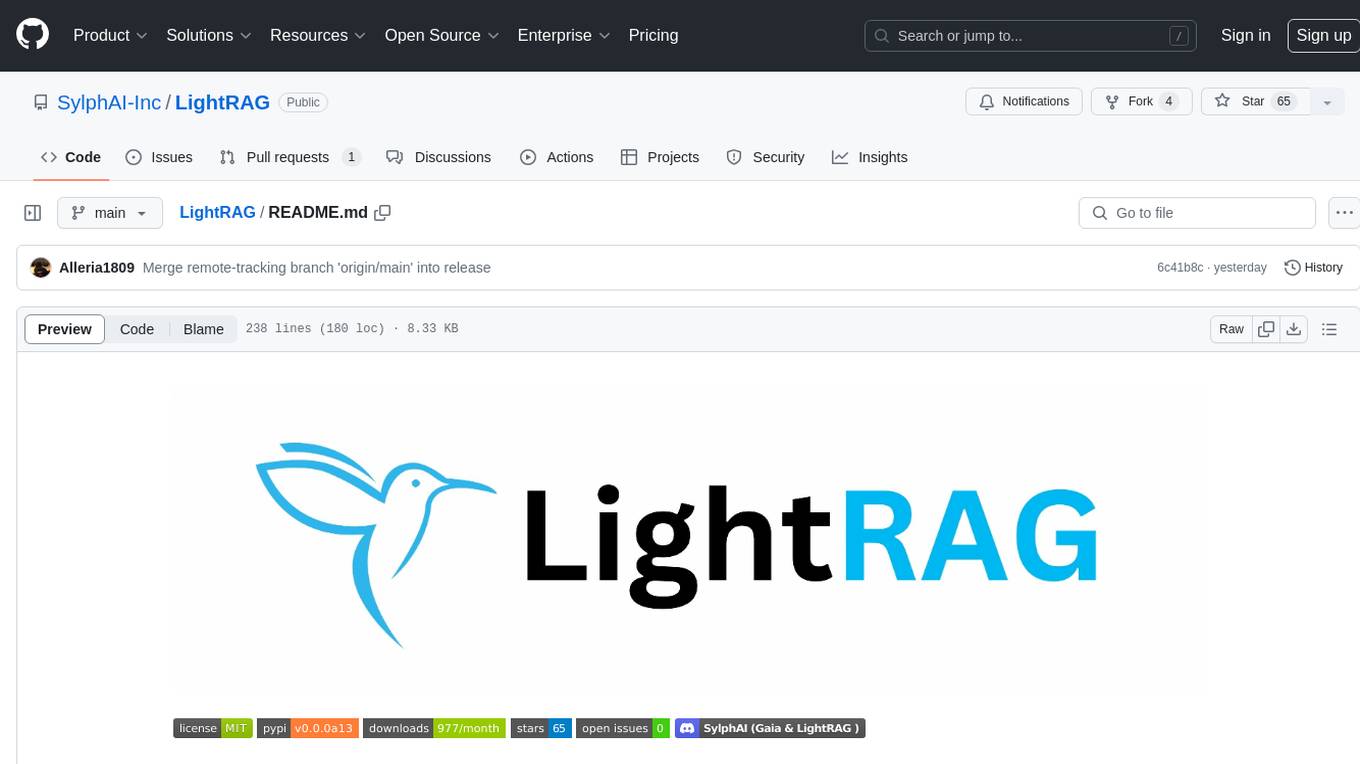
LightRAG
LightRAG is a PyTorch library designed for building and optimizing Retriever-Agent-Generator (RAG) pipelines. It follows principles of simplicity, quality, and optimization, offering developers maximum customizability with minimal abstraction. The library includes components for model interaction, output parsing, and structured data generation. LightRAG facilitates tasks like providing explanations and examples for concepts through a question-answering pipeline.
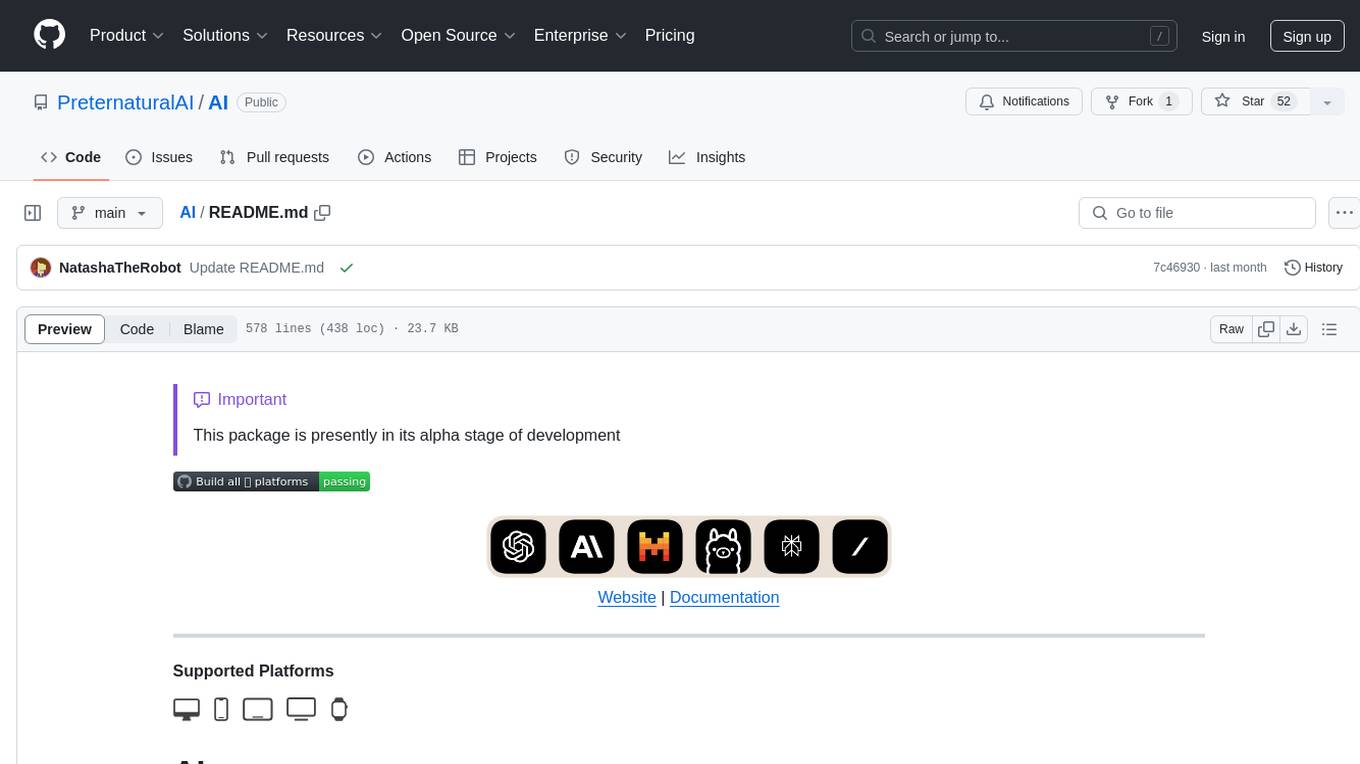
AI
AI is an open-source Swift framework for interfacing with generative AI. It provides functionalities for text completions, image-to-text vision, function calling, DALLE-3 image generation, audio transcription and generation, and text embeddings. The framework supports multiple AI models from providers like OpenAI, Anthropic, Mistral, Groq, and ElevenLabs. Users can easily integrate AI capabilities into their Swift projects using AI framework.
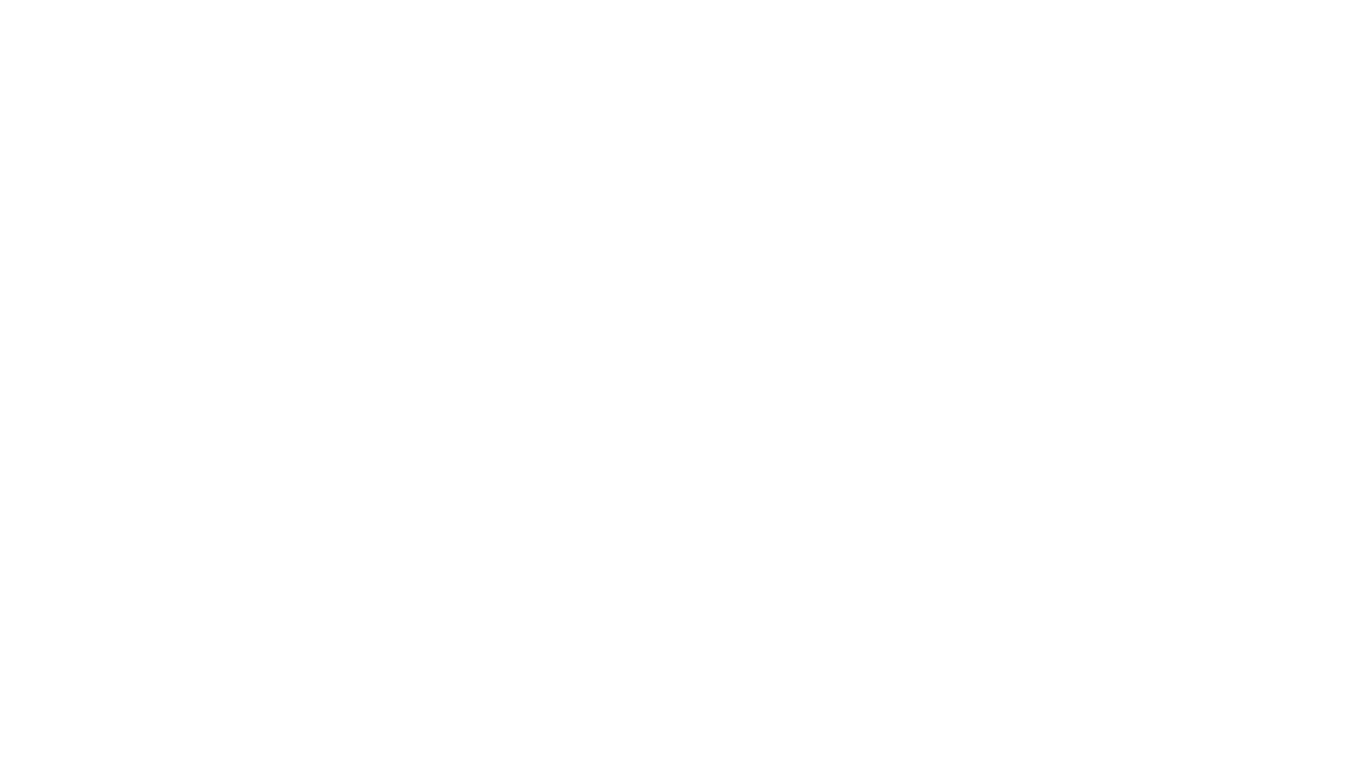
generative-ai
The 'Generative AI' repository provides a C# library for interacting with Google's Generative AI models, specifically the Gemini models. It allows users to access and integrate the Gemini API into .NET applications, supporting functionalities such as listing available models, generating content, creating tuned models, working with large files, starting chat sessions, and more. The repository also includes helper classes and enums for Gemini API aspects. Authentication methods include API key, OAuth, and various authentication modes for Google AI and Vertex AI. The package offers features for both Google AI Studio and Google Cloud Vertex AI, with detailed instructions on installation, usage, and troubleshooting.
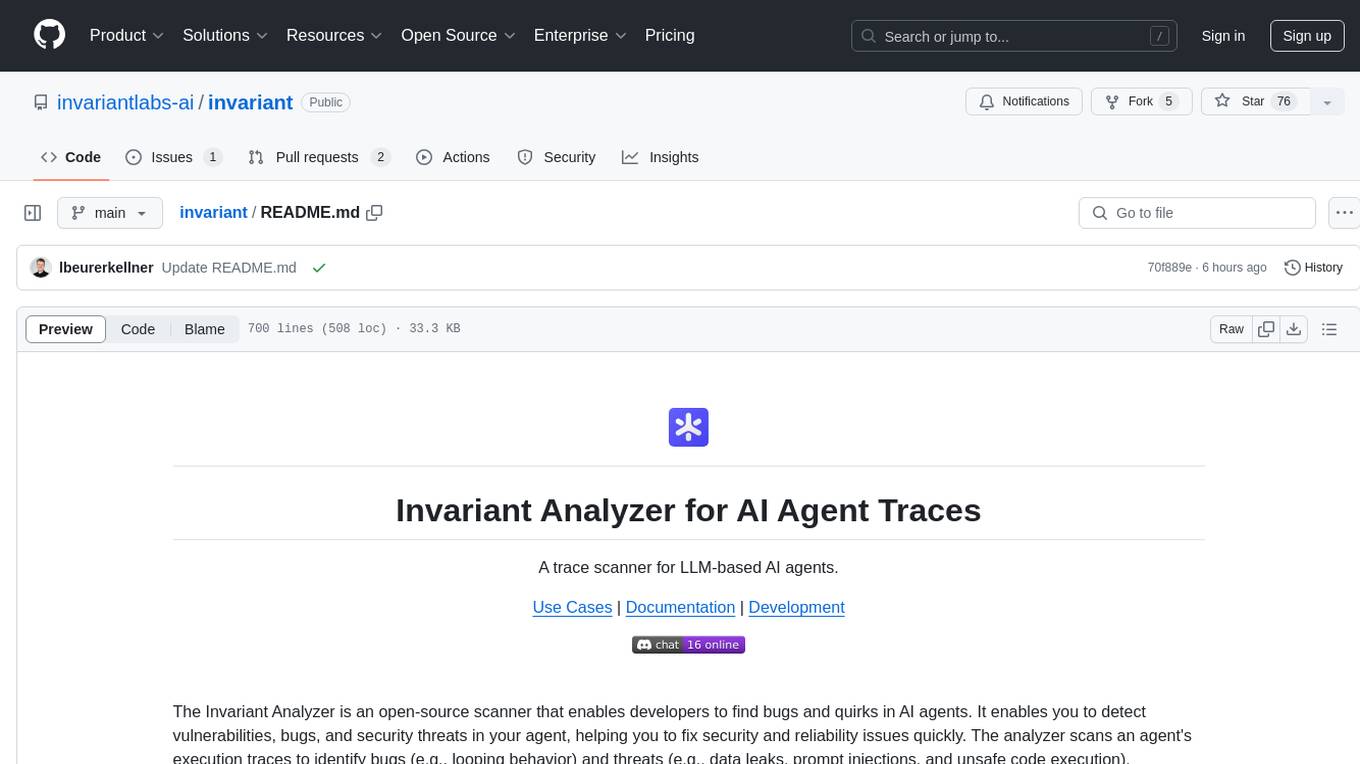
invariant
Invariant Analyzer is an open-source scanner designed for LLM-based AI agents to find bugs, vulnerabilities, and security threats. It scans agent execution traces to identify issues like looping behavior, data leaks, prompt injections, and unsafe code execution. The tool offers a library of built-in checkers, an expressive policy language, data flow analysis, real-time monitoring, and extensible architecture for custom checkers. It helps developers debug AI agents, scan for security violations, and prevent security issues and data breaches during runtime. The analyzer leverages deep contextual understanding and a purpose-built rule matching engine for security policy enforcement.
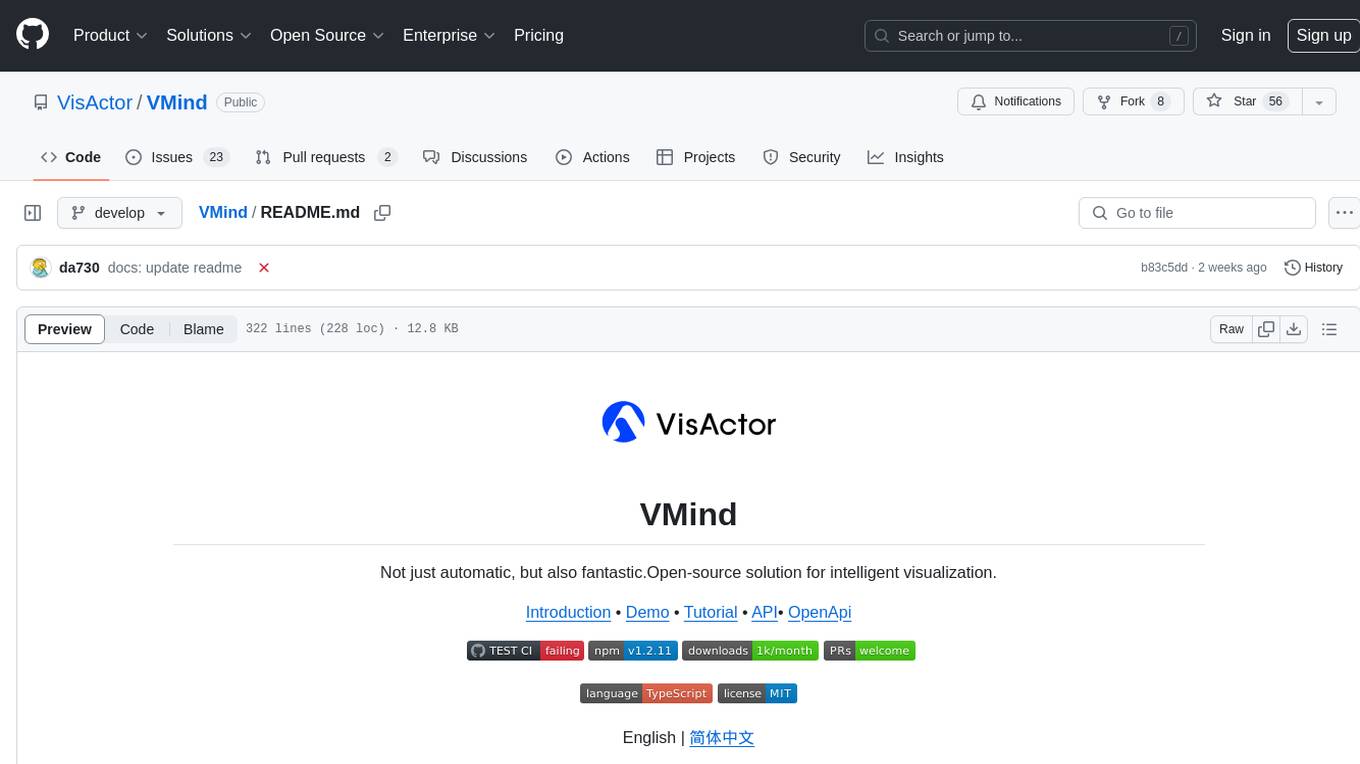
VMind
VMind is an open-source solution for intelligent visualization, providing an intelligent chart component based on LLM by VisActor. It allows users to create chart narrative works with natural language interaction, edit charts through dialogue, and export narratives as videos or GIFs. The tool is easy to use, scalable, supports various chart types, and offers one-click export functionality. Users can customize chart styles, specify themes, and aggregate data using LLM models. VMind aims to enhance efficiency in creating data visualization works through dialogue-based editing and natural language interaction.
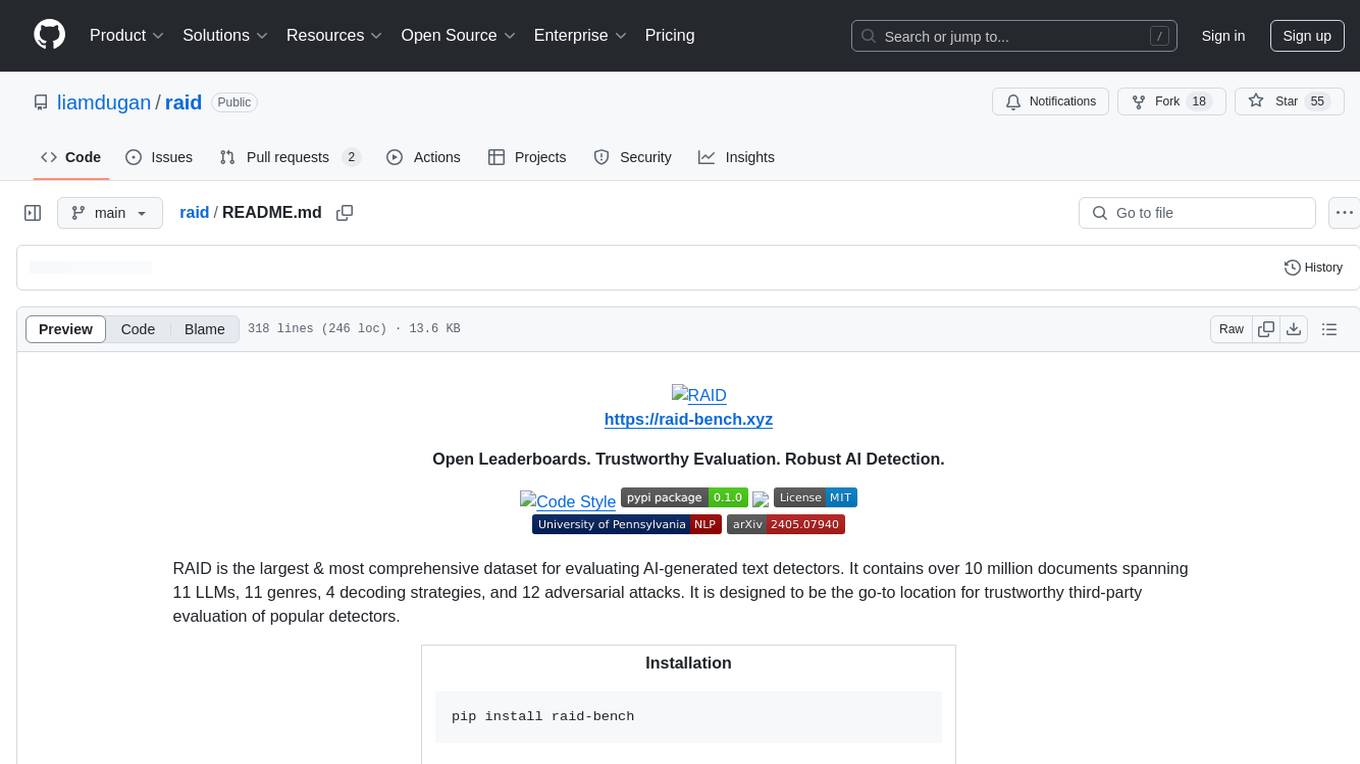
raid
RAID is the largest and most comprehensive dataset for evaluating AI-generated text detectors. It contains over 10 million documents spanning 11 LLMs, 11 genres, 4 decoding strategies, and 12 adversarial attacks. RAID is designed to be the go-to location for trustworthy third-party evaluation of popular detectors. The dataset covers diverse models, domains, sampling strategies, and attacks, making it a valuable resource for training detectors, evaluating generalization, protecting against adversaries, and comparing to state-of-the-art models from academia and industry.
For similar tasks
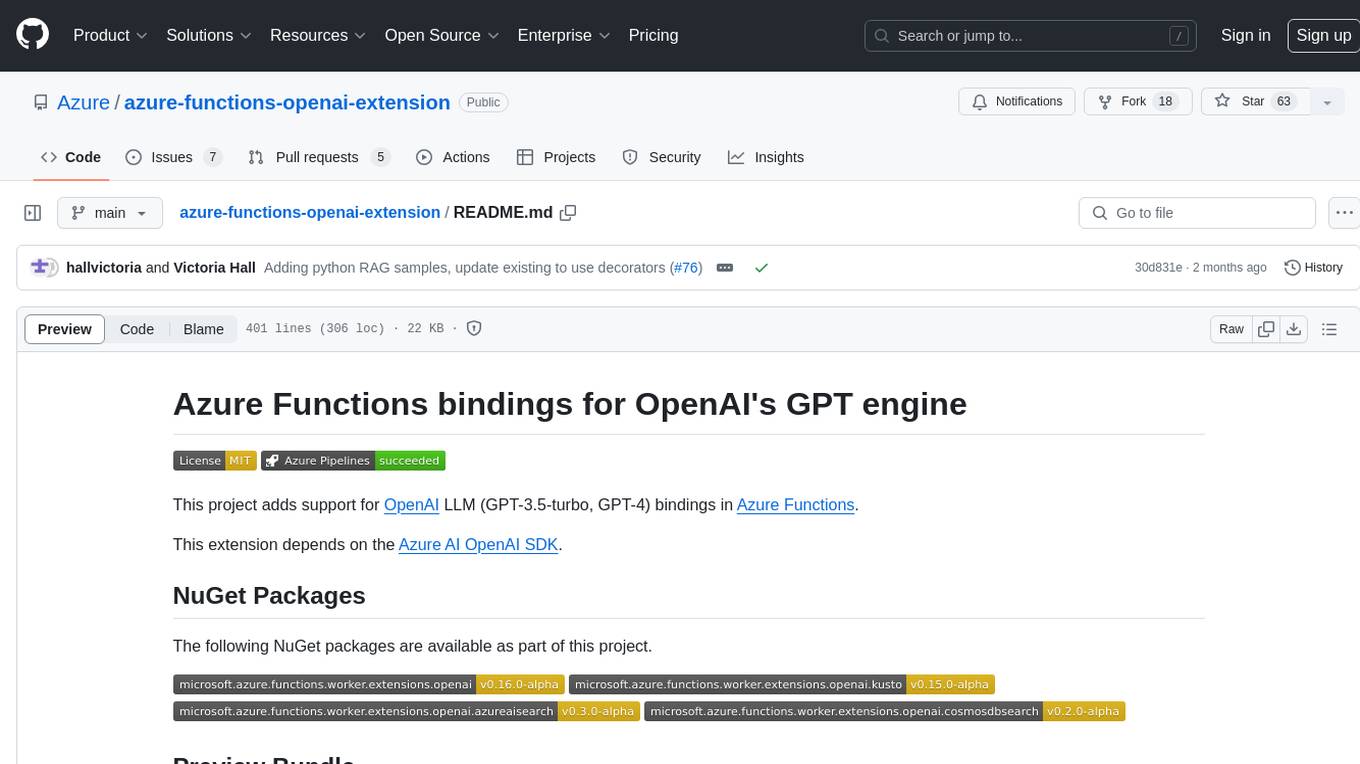
azure-functions-openai-extension
Azure Functions OpenAI Extension is a project that adds support for OpenAI LLM (GPT-3.5-turbo, GPT-4) bindings in Azure Functions. It provides NuGet packages for various functionalities like text completions, chat completions, assistants, embeddings generators, and semantic search. The project requires .NET 6 SDK or greater, Azure Functions Core Tools v4.x, and specific settings in Azure Function or local settings for development. It offers features like text completions, chat completion, assistants with custom skills, embeddings generators for text relatedness, and semantic search using vector databases. The project also includes examples in C# and Python for different functionalities.
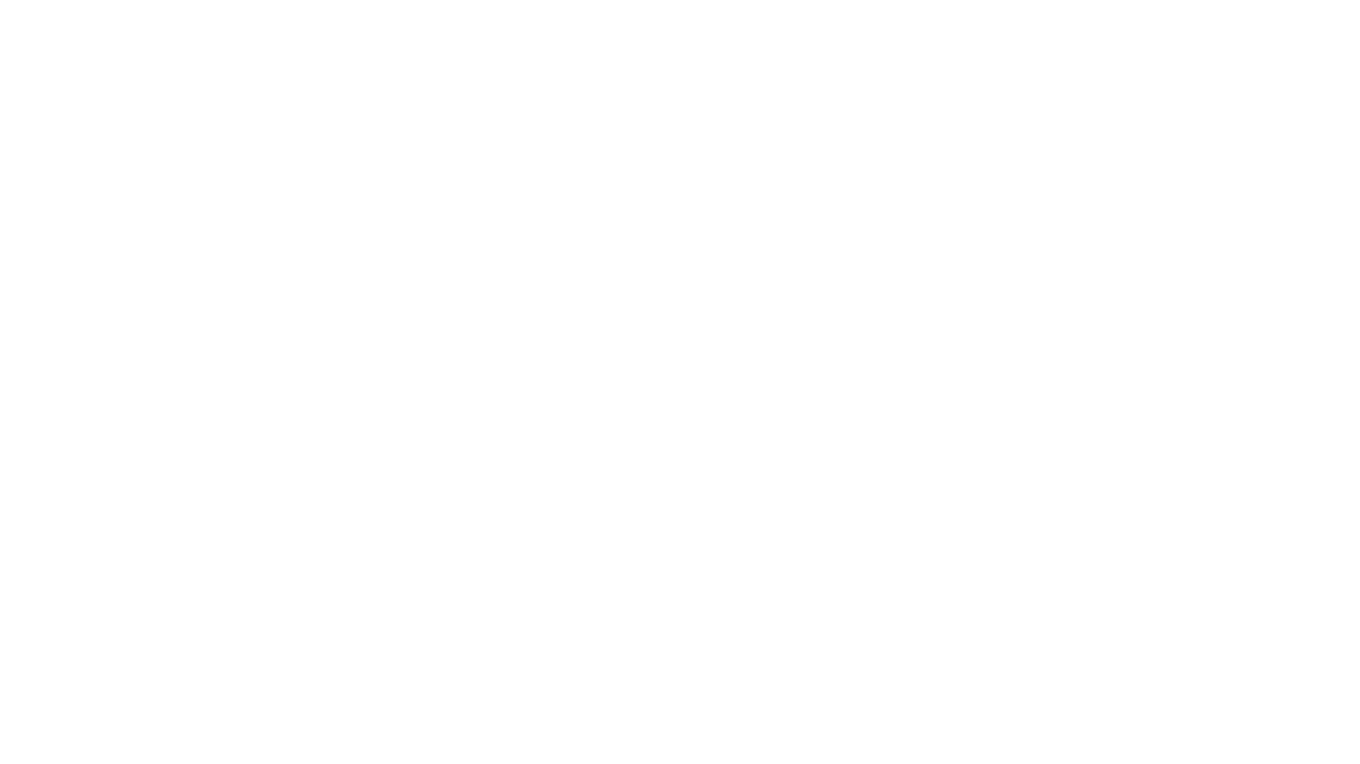
embedJs
EmbedJs is a NodeJS framework that simplifies RAG application development by efficiently processing unstructured data. It segments data, creates relevant embeddings, and stores them in a vector database for quick retrieval.
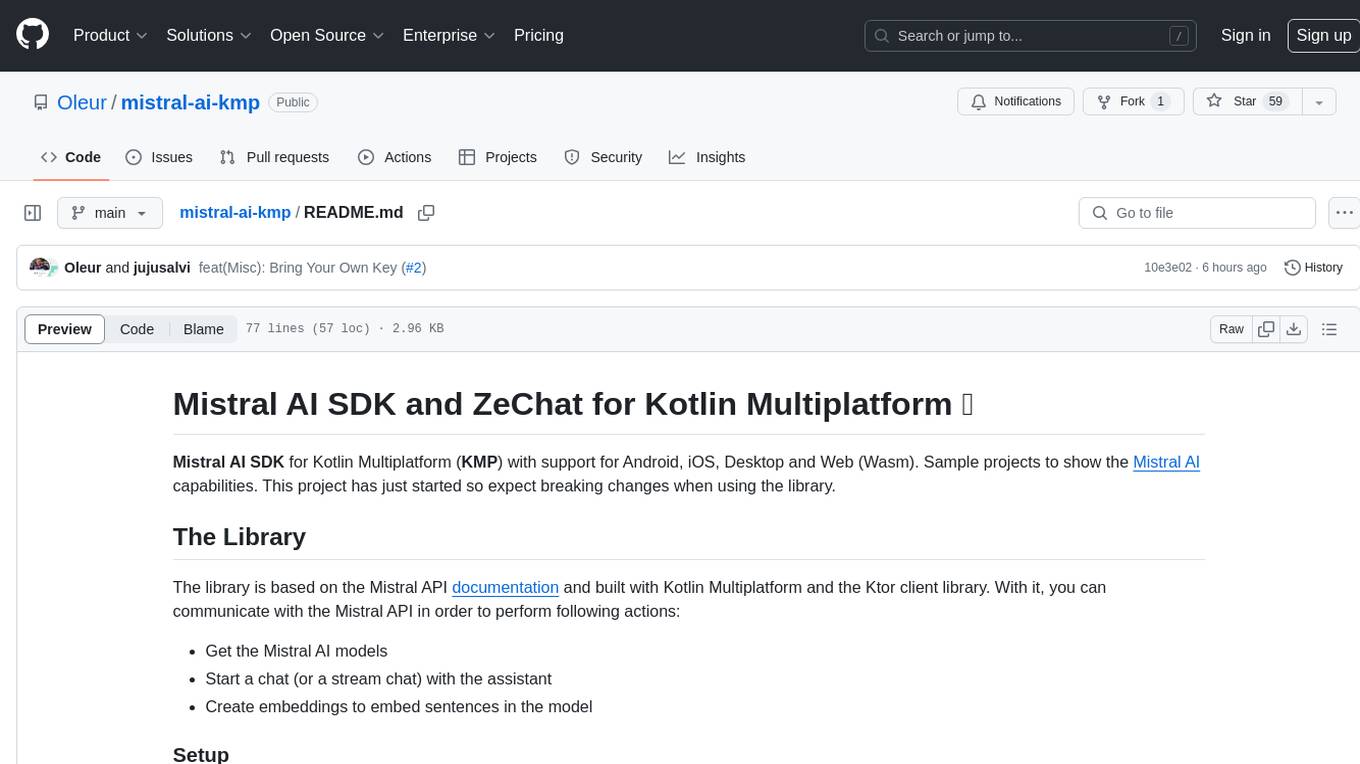
mistral-ai-kmp
Mistral AI SDK for Kotlin Multiplatform (KMP) allows communication with Mistral API to get AI models, start a chat with the assistant, and create embeddings. The library is based on Mistral API documentation and built with Kotlin Multiplatform and Ktor client library. Sample projects like ZeChat showcase the capabilities of Mistral AI SDK. Users can interact with different Mistral AI models through ZeChat apps on Android, Desktop, and Web platforms. The library is not yet published on Maven, but users can fork the project and use it as a module dependency in their apps.
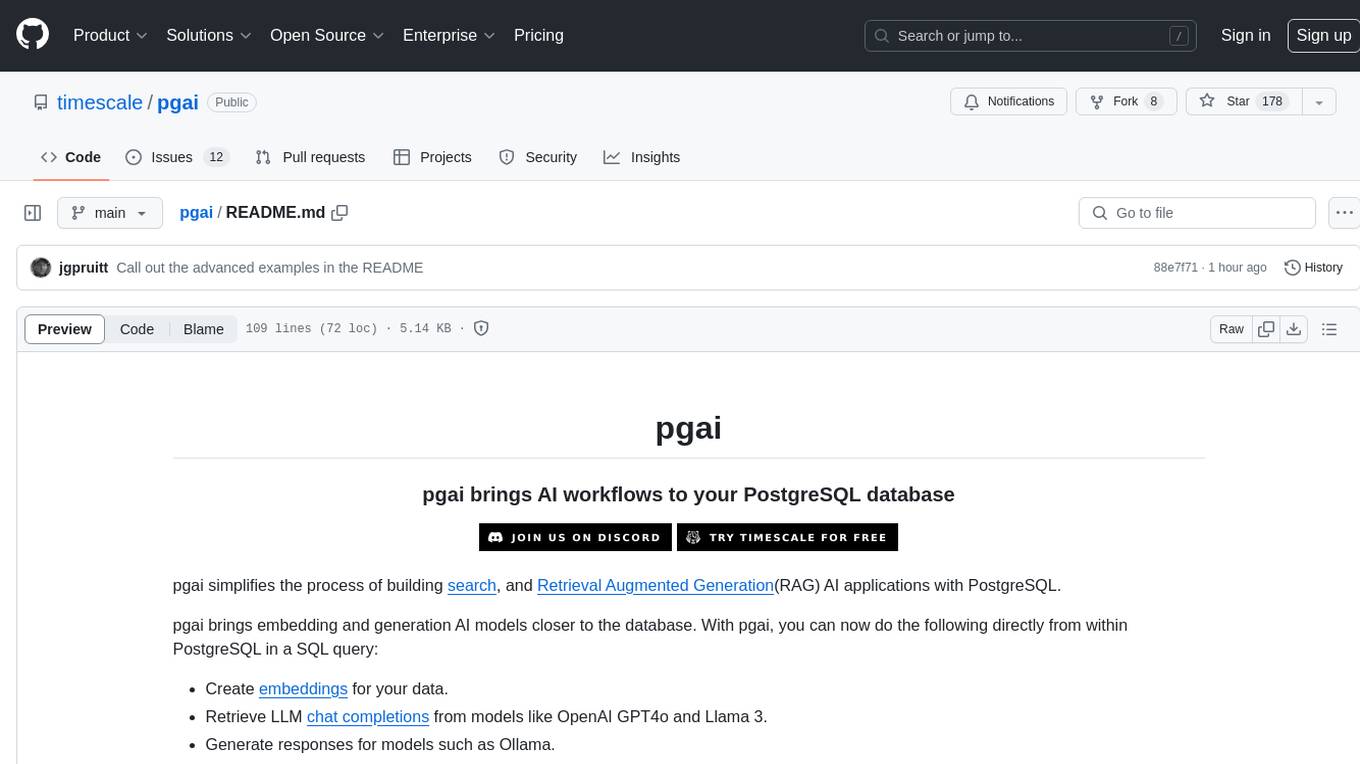
pgai
pgai simplifies the process of building search and Retrieval Augmented Generation (RAG) AI applications with PostgreSQL. It brings embedding and generation AI models closer to the database, allowing users to create embeddings, retrieve LLM chat completions, reason over data for classification, summarization, and data enrichment directly from within PostgreSQL in a SQL query. The tool requires an OpenAI API key and a PostgreSQL client to enable AI functionality in the database. Users can install pgai from source, run it in a pre-built Docker container, or enable it in a Timescale Cloud service. The tool provides functions to handle API keys using psql or Python, and offers various AI functionalities like tokenizing, detokenizing, embedding, chat completion, and content moderation.
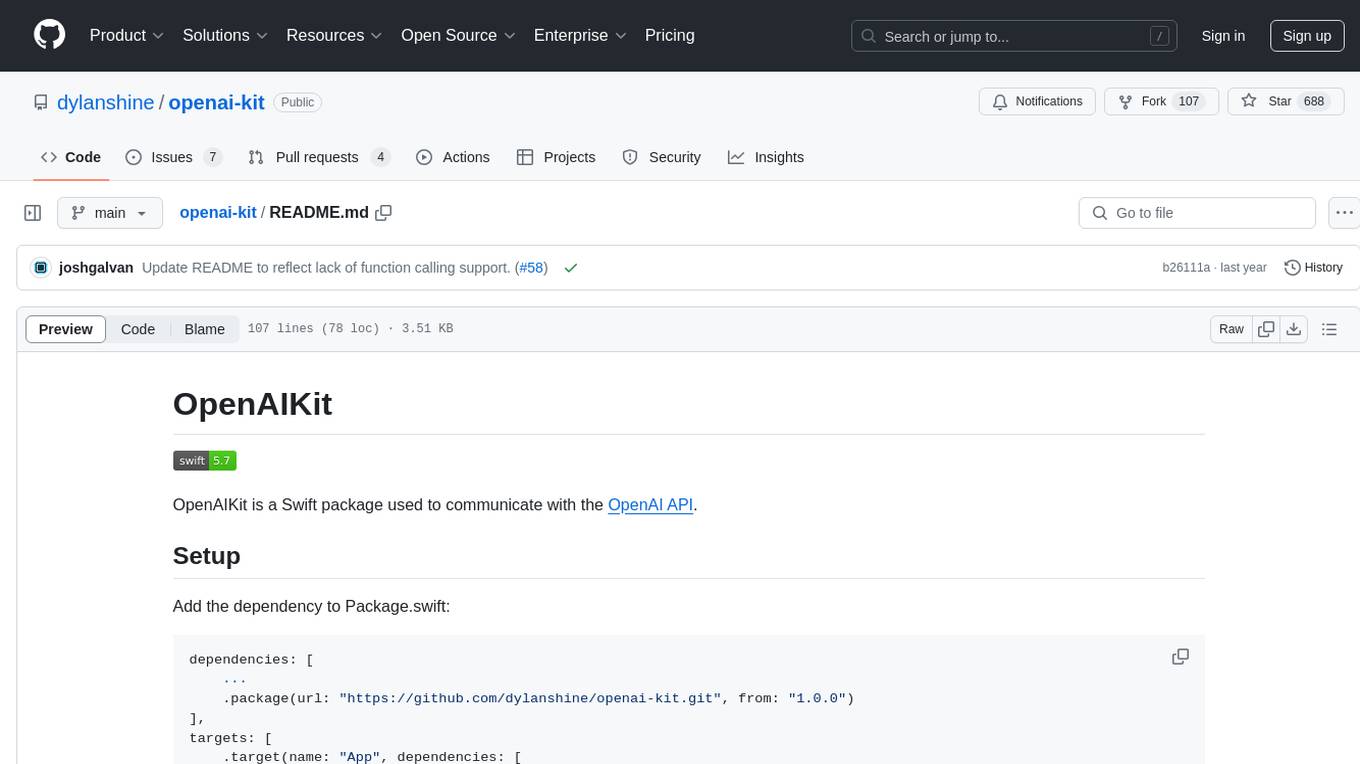
openai-kit
OpenAIKit is a Swift package designed to facilitate communication with the OpenAI API. It provides methods to interact with various OpenAI services such as chat, models, completions, edits, images, embeddings, files, moderations, and speech to text. The package encourages the use of environment variables to securely inject the OpenAI API key and organization details. It also offers error handling for API requests through the `OpenAIKit.APIErrorResponse`.
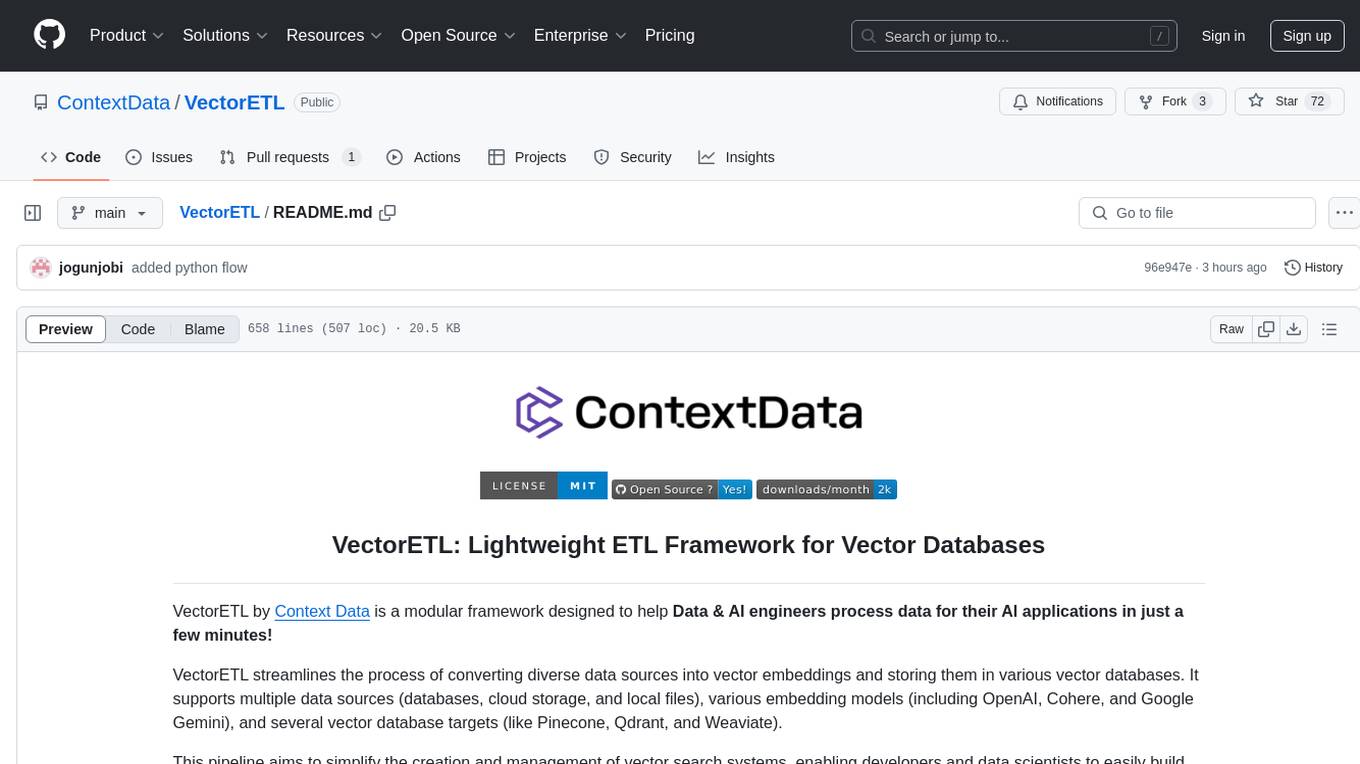
VectorETL
VectorETL is a lightweight ETL framework designed to assist Data & AI engineers in processing data for AI applications quickly. It streamlines the conversion of diverse data sources into vector embeddings and storage in various vector databases. The framework supports multiple data sources, embedding models, and vector database targets, simplifying the creation and management of vector search systems for semantic search, recommendation systems, and other vector-based operations.
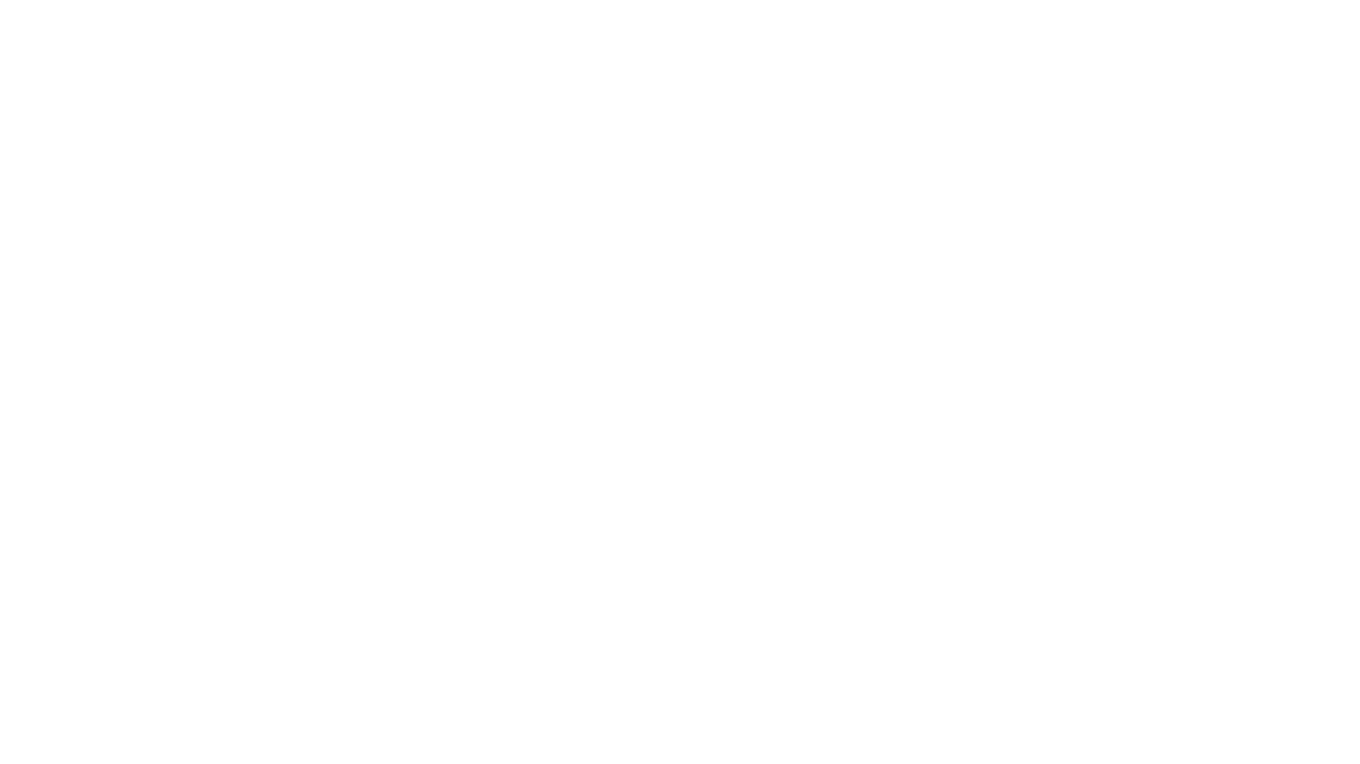
LLamaWorker
LLamaWorker is a HTTP API server developed to provide an OpenAI-compatible API for integrating Large Language Models (LLM) into applications. It supports multi-model configuration, streaming responses, text embedding, chat templates, automatic model release, function calls, API key authentication, and test UI. Users can switch models, complete chats and prompts, manage chat history, and generate tokens through the test UI. Additionally, LLamaWorker offers a Vulkan compiled version for download and provides function call templates for testing. The tool supports various backends and provides API endpoints for chat completion, prompt completion, embeddings, model information, model configuration, and model switching. A Gradio UI demo is also available for testing.
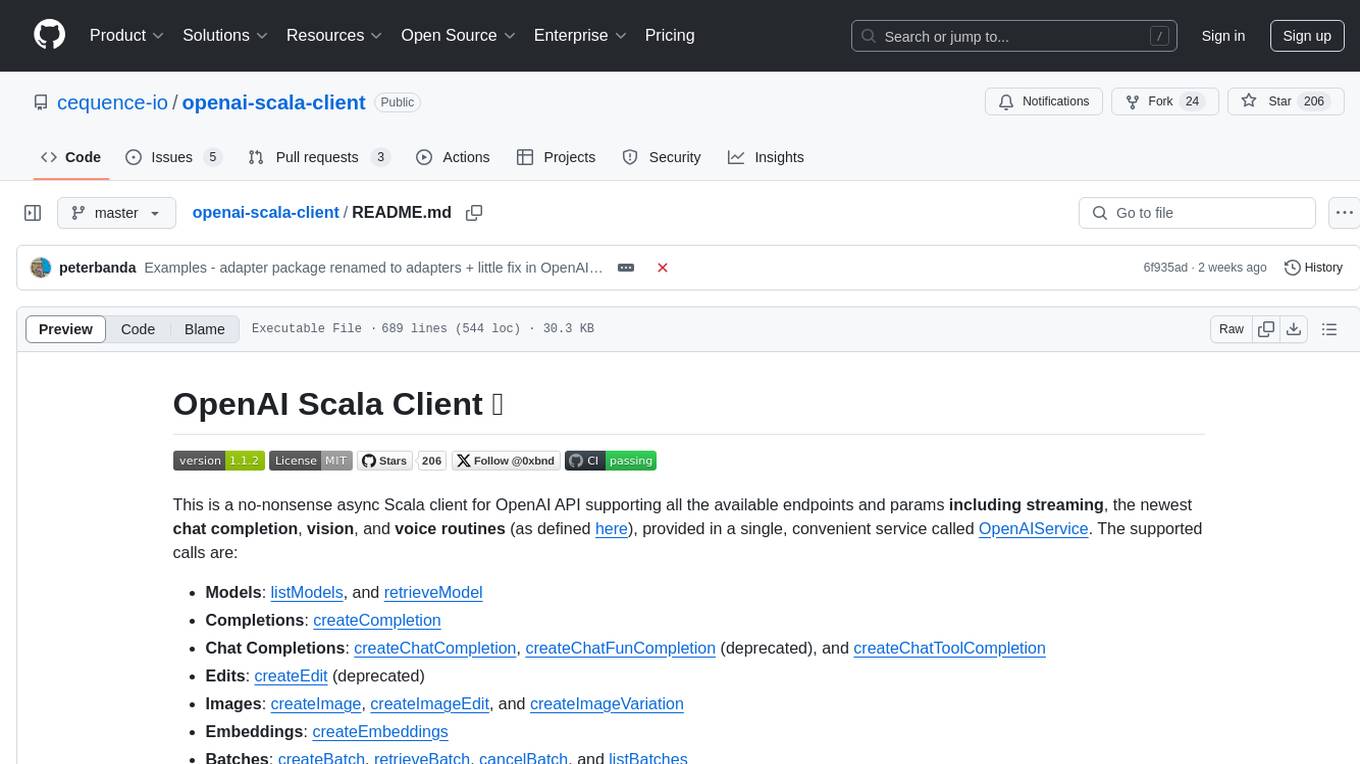
openai-scala-client
This is a no-nonsense async Scala client for OpenAI API supporting all the available endpoints and params including streaming, chat completion, vision, and voice routines. It provides a single service called OpenAIService that supports various calls such as Models, Completions, Chat Completions, Edits, Images, Embeddings, Batches, Audio, Files, Fine-tunes, Moderations, Assistants, Threads, Thread Messages, Runs, Run Steps, Vector Stores, Vector Store Files, and Vector Store File Batches. The library aims to be self-contained with minimal dependencies and supports API-compatible providers like Azure OpenAI, Azure AI, Anthropic, Google Vertex AI, Groq, Grok, Fireworks AI, OctoAI, TogetherAI, Cerebras, Mistral, Deepseek, Ollama, FastChat, and more.
For similar jobs
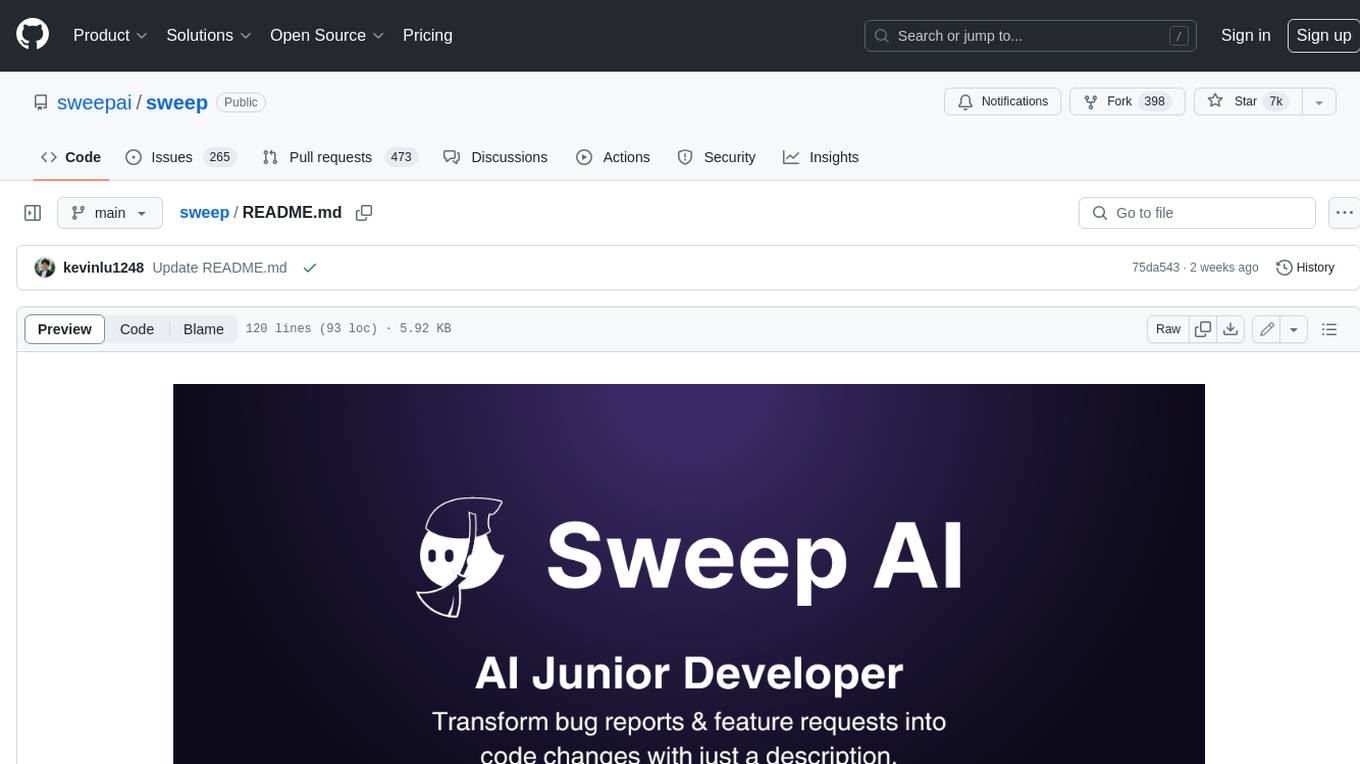
sweep
Sweep is an AI junior developer that turns bugs and feature requests into code changes. It automatically handles developer experience improvements like adding type hints and improving test coverage.
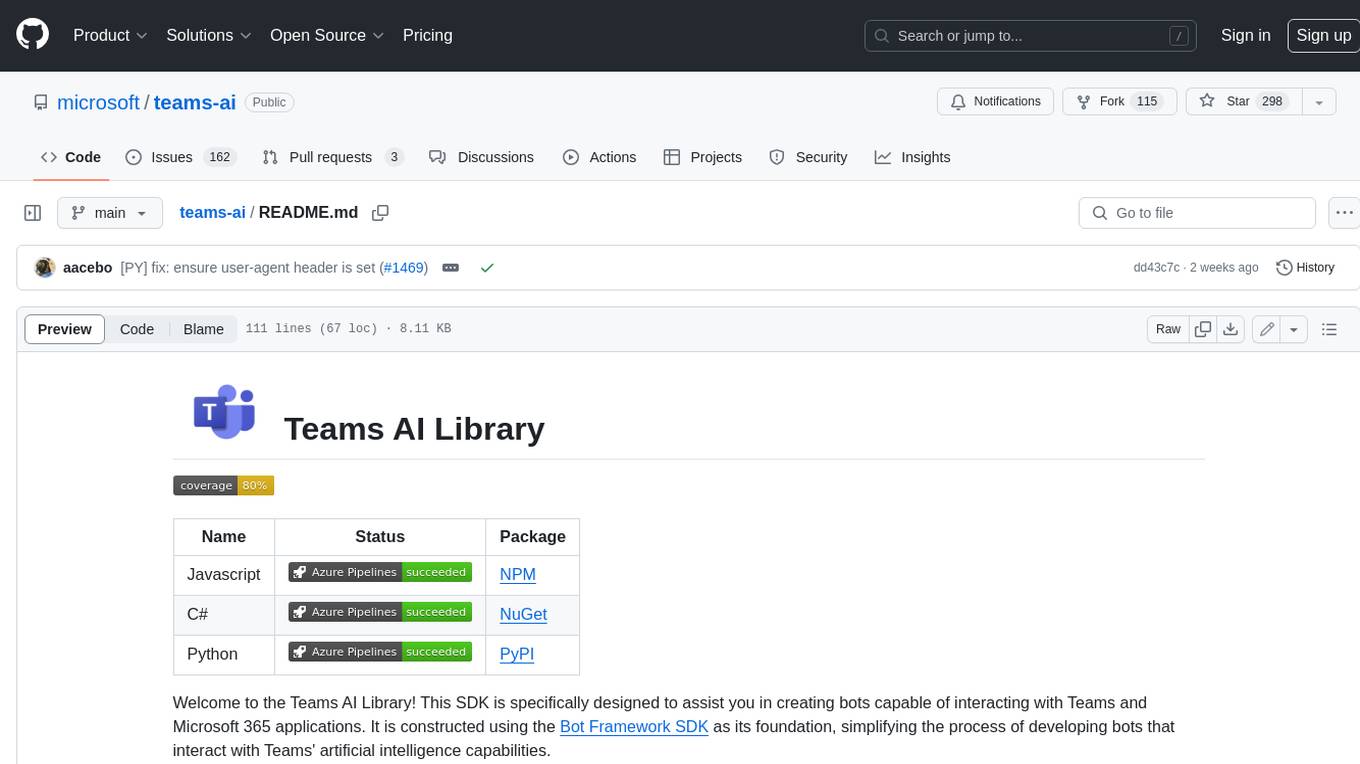
teams-ai
The Teams AI Library is a software development kit (SDK) that helps developers create bots that can interact with Teams and Microsoft 365 applications. It is built on top of the Bot Framework SDK and simplifies the process of developing bots that interact with Teams' artificial intelligence capabilities. The SDK is available for JavaScript/TypeScript, .NET, and Python.
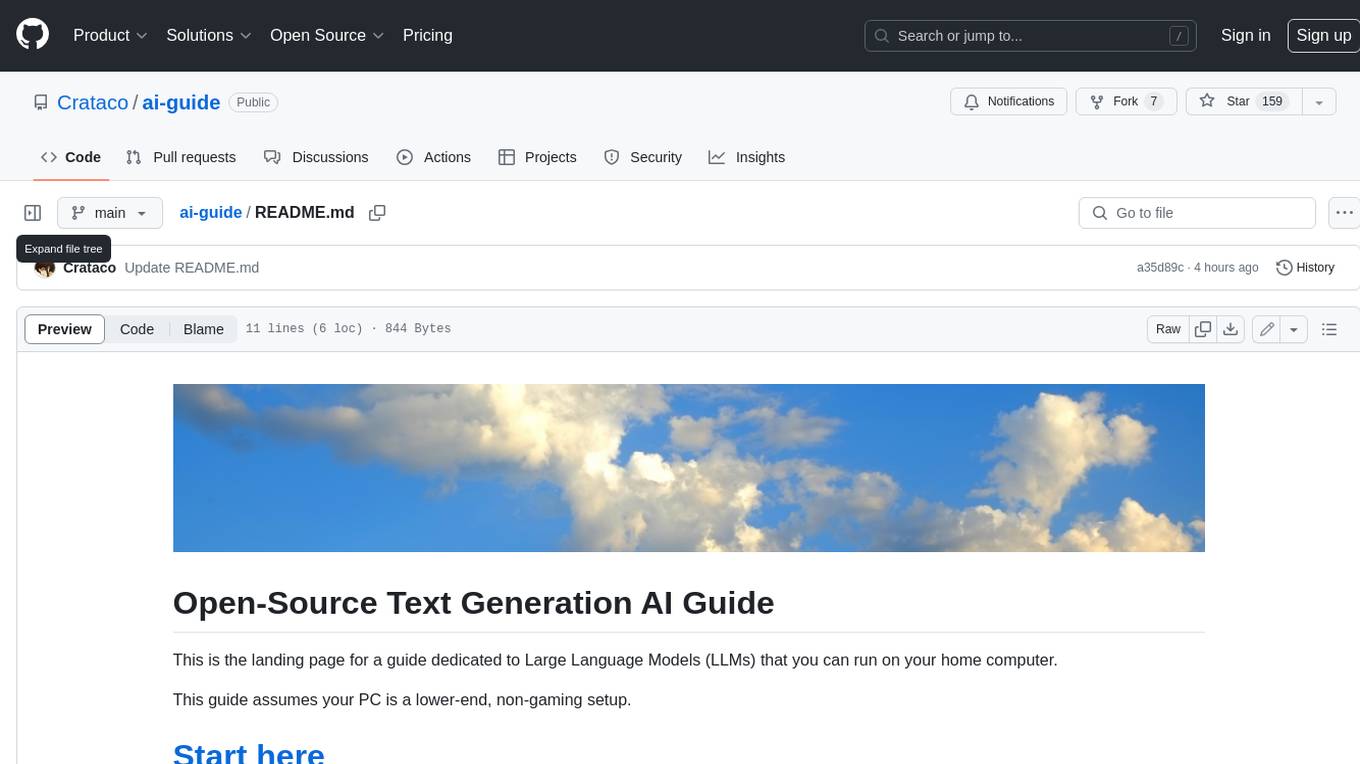
ai-guide
This guide is dedicated to Large Language Models (LLMs) that you can run on your home computer. It assumes your PC is a lower-end, non-gaming setup.
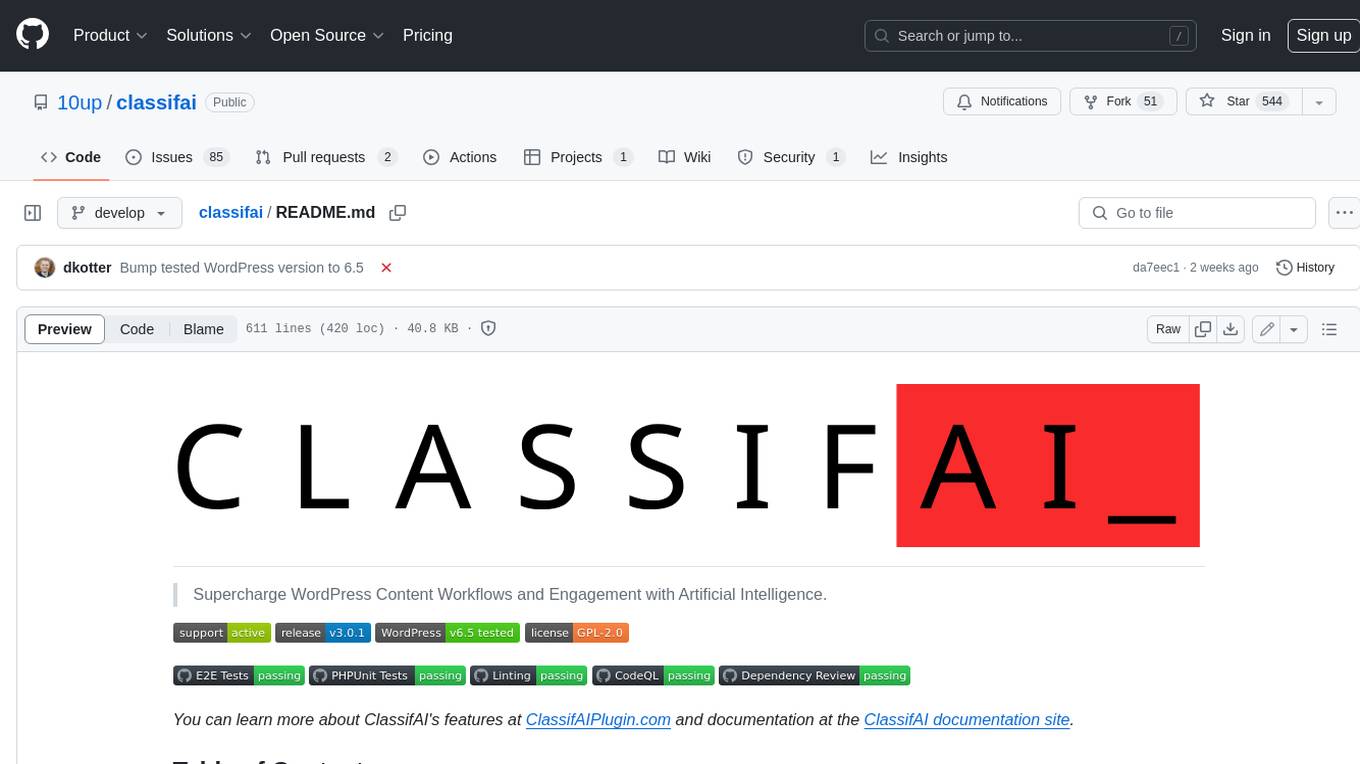
classifai
Supercharge WordPress Content Workflows and Engagement with Artificial Intelligence. Tap into leading cloud-based services like OpenAI, Microsoft Azure AI, Google Gemini and IBM Watson to augment your WordPress-powered websites. Publish content faster while improving SEO performance and increasing audience engagement. ClassifAI integrates Artificial Intelligence and Machine Learning technologies to lighten your workload and eliminate tedious tasks, giving you more time to create original content that matters.
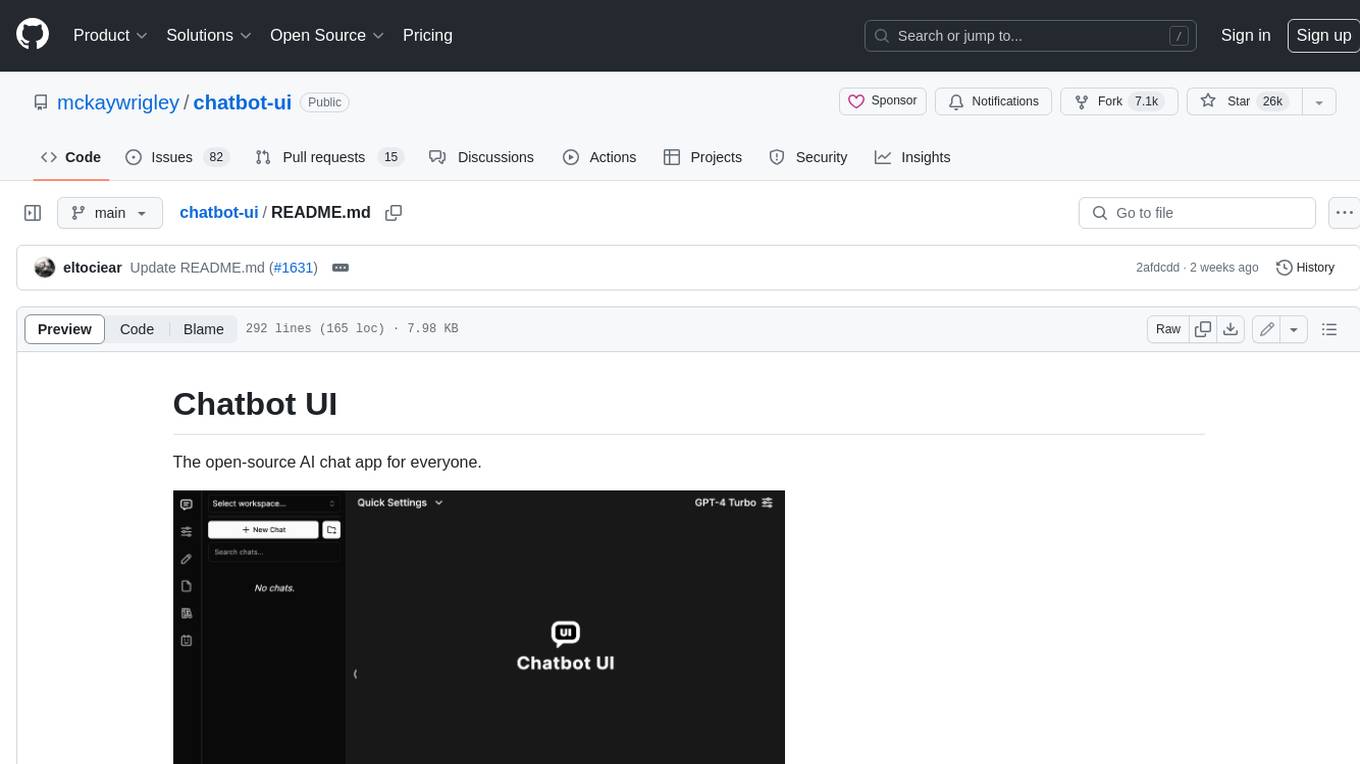
chatbot-ui
Chatbot UI is an open-source AI chat app that allows users to create and deploy their own AI chatbots. It is easy to use and can be customized to fit any need. Chatbot UI is perfect for businesses, developers, and anyone who wants to create a chatbot.
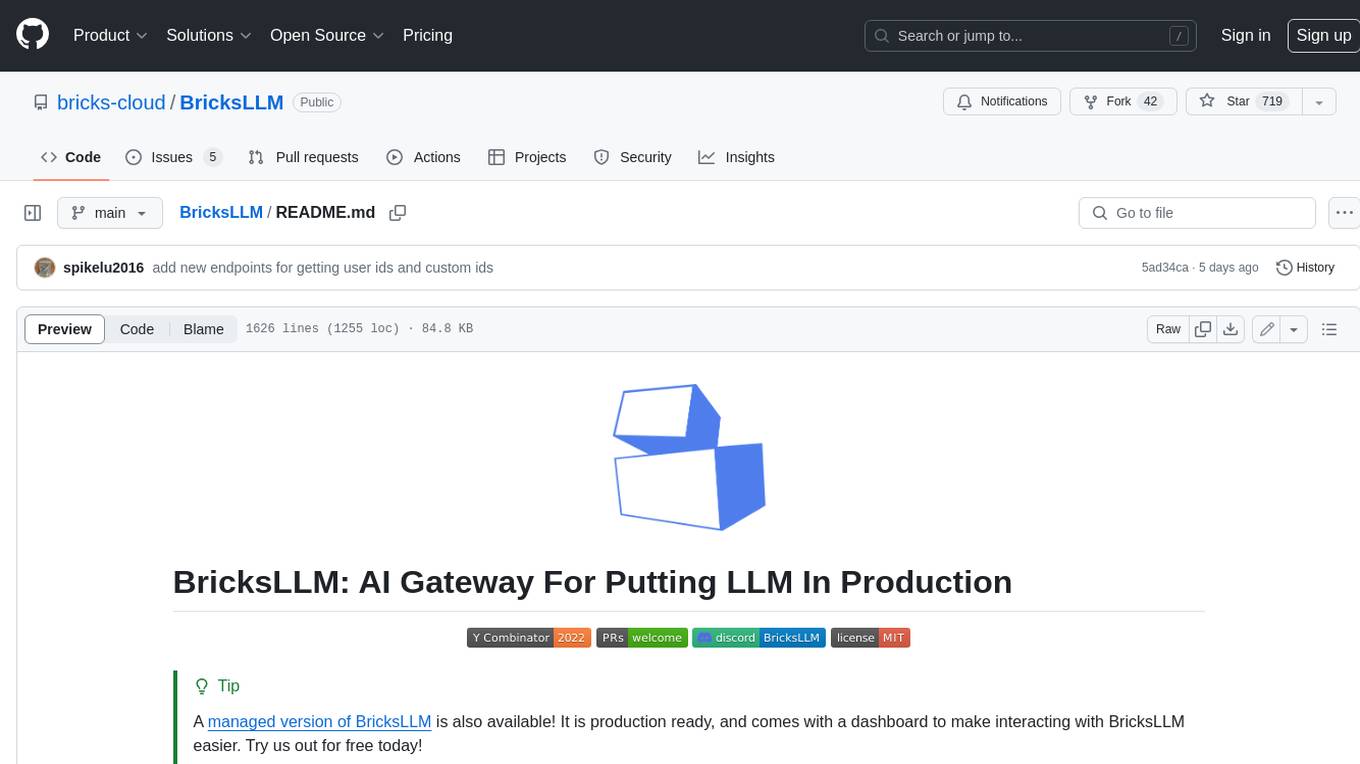
BricksLLM
BricksLLM is a cloud native AI gateway written in Go. Currently, it provides native support for OpenAI, Anthropic, Azure OpenAI and vLLM. BricksLLM aims to provide enterprise level infrastructure that can power any LLM production use cases. Here are some use cases for BricksLLM: * Set LLM usage limits for users on different pricing tiers * Track LLM usage on a per user and per organization basis * Block or redact requests containing PIIs * Improve LLM reliability with failovers, retries and caching * Distribute API keys with rate limits and cost limits for internal development/production use cases * Distribute API keys with rate limits and cost limits for students
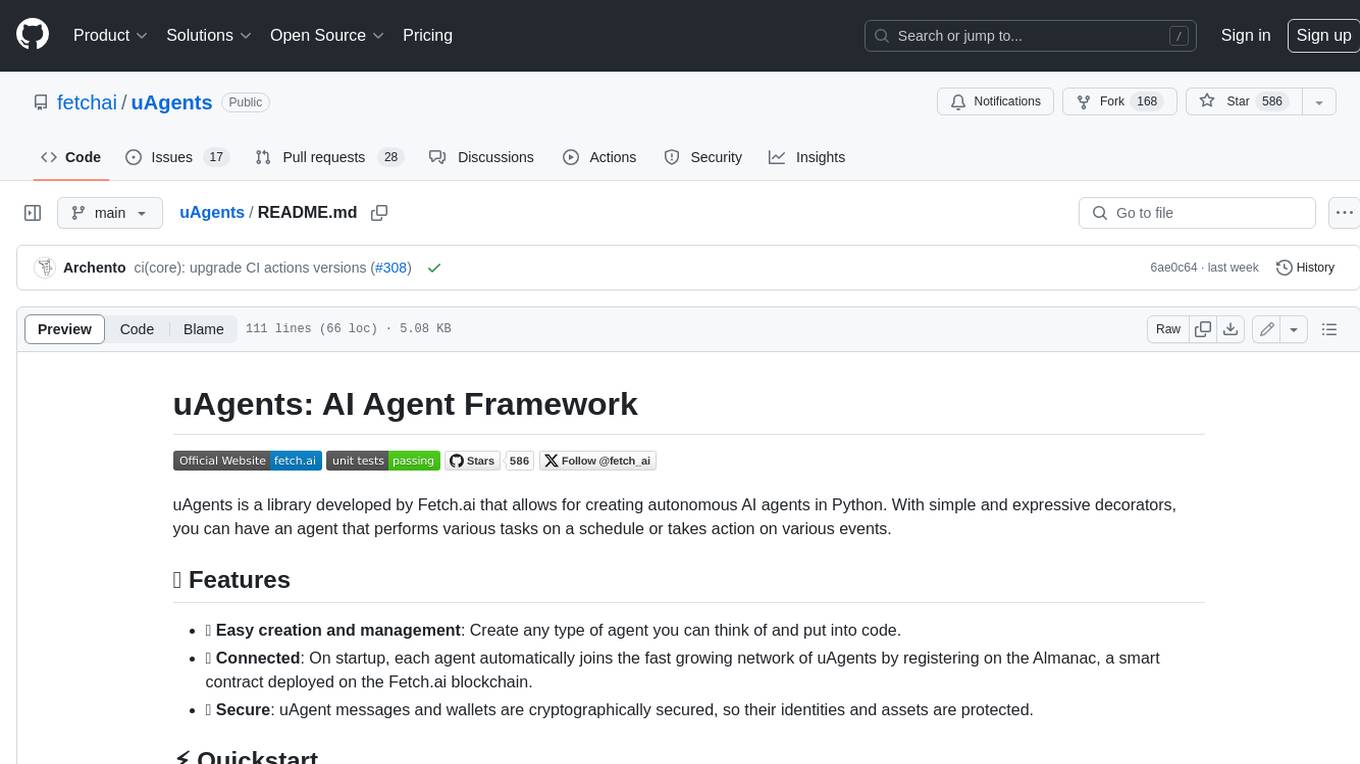
uAgents
uAgents is a Python library developed by Fetch.ai that allows for the creation of autonomous AI agents. These agents can perform various tasks on a schedule or take action on various events. uAgents are easy to create and manage, and they are connected to a fast-growing network of other uAgents. They are also secure, with cryptographically secured messages and wallets.
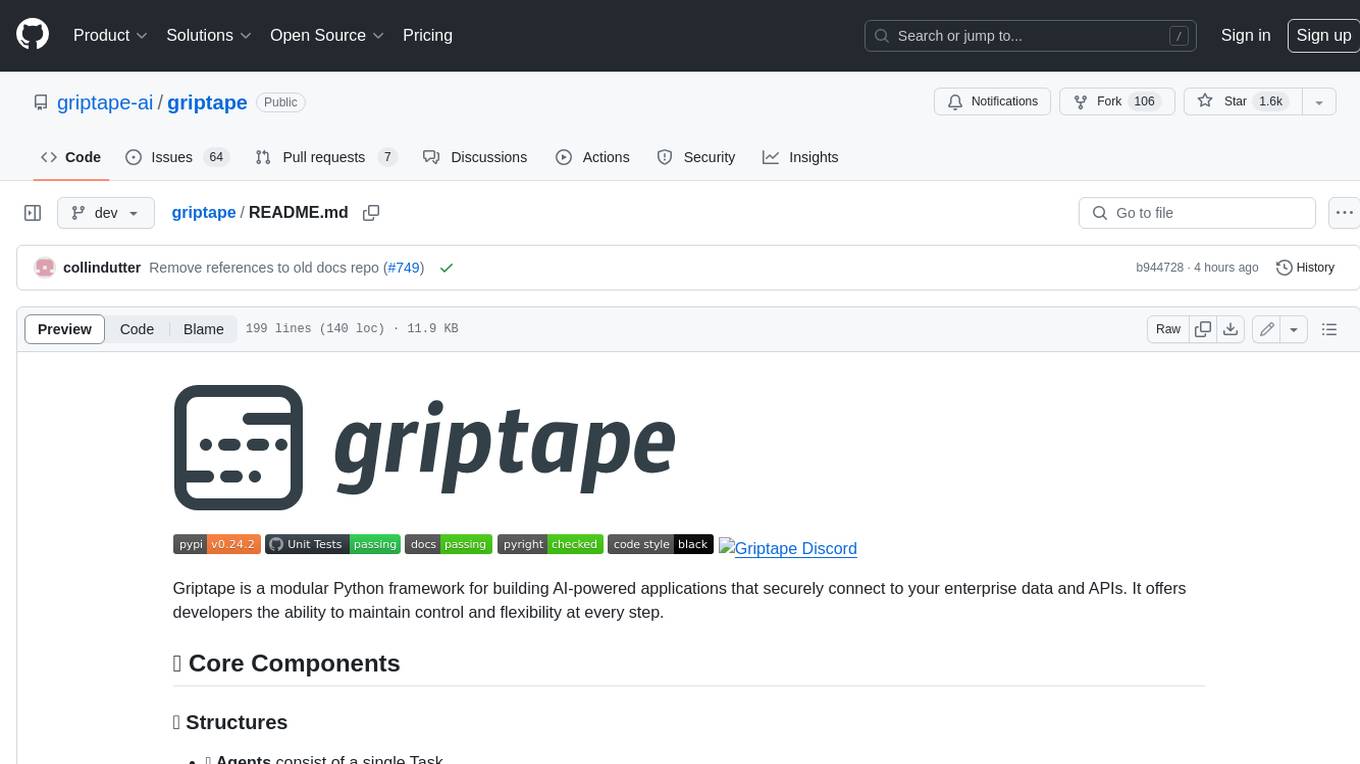
griptape
Griptape is a modular Python framework for building AI-powered applications that securely connect to your enterprise data and APIs. It offers developers the ability to maintain control and flexibility at every step. Griptape's core components include Structures (Agents, Pipelines, and Workflows), Tasks, Tools, Memory (Conversation Memory, Task Memory, and Meta Memory), Drivers (Prompt and Embedding Drivers, Vector Store Drivers, Image Generation Drivers, Image Query Drivers, SQL Drivers, Web Scraper Drivers, and Conversation Memory Drivers), Engines (Query Engines, Extraction Engines, Summary Engines, Image Generation Engines, and Image Query Engines), and additional components (Rulesets, Loaders, Artifacts, Chunkers, and Tokenizers). Griptape enables developers to create AI-powered applications with ease and efficiency.